Sampling Methods In Reseach: Types, Techniques, & Examples
Saul Mcleod, PhD
Editor-in-Chief for Simply Psychology
BSc (Hons) Psychology, MRes, PhD, University of Manchester
Saul Mcleod, PhD., is a qualified psychology teacher with over 18 years of experience in further and higher education. He has been published in peer-reviewed journals, including the Journal of Clinical Psychology.
Learn about our Editorial Process
Olivia Guy-Evans, MSc
Associate Editor for Simply Psychology
BSc (Hons) Psychology, MSc Psychology of Education
Olivia Guy-Evans is a writer and associate editor for Simply Psychology. She has previously worked in healthcare and educational sectors.
On This Page:
Sampling methods in psychology refer to strategies used to select a subset of individuals (a sample) from a larger population, to study and draw inferences about the entire population. Common methods include random sampling, stratified sampling, cluster sampling, and convenience sampling. Proper sampling ensures representative, generalizable, and valid research results.
- Sampling : the process of selecting a representative group from the population under study.
- Target population : the total group of individuals from which the sample might be drawn.
- Sample: a subset of individuals selected from a larger population for study or investigation. Those included in the sample are termed “participants.”
- Generalizability : the ability to apply research findings from a sample to the broader target population, contingent on the sample being representative of that population.
For instance, if the advert for volunteers is published in the New York Times, this limits how much the study’s findings can be generalized to the whole population, because NYT readers may not represent the entire population in certain respects (e.g., politically, socio-economically).
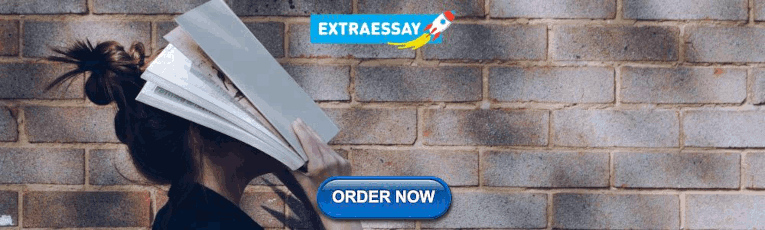
The Purpose of Sampling
We are interested in learning about large groups of people with something in common in psychological research. We call the group interested in studying our “target population.”
In some types of research, the target population might be as broad as all humans. Still, in other types of research, the target population might be a smaller group, such as teenagers, preschool children, or people who misuse drugs.
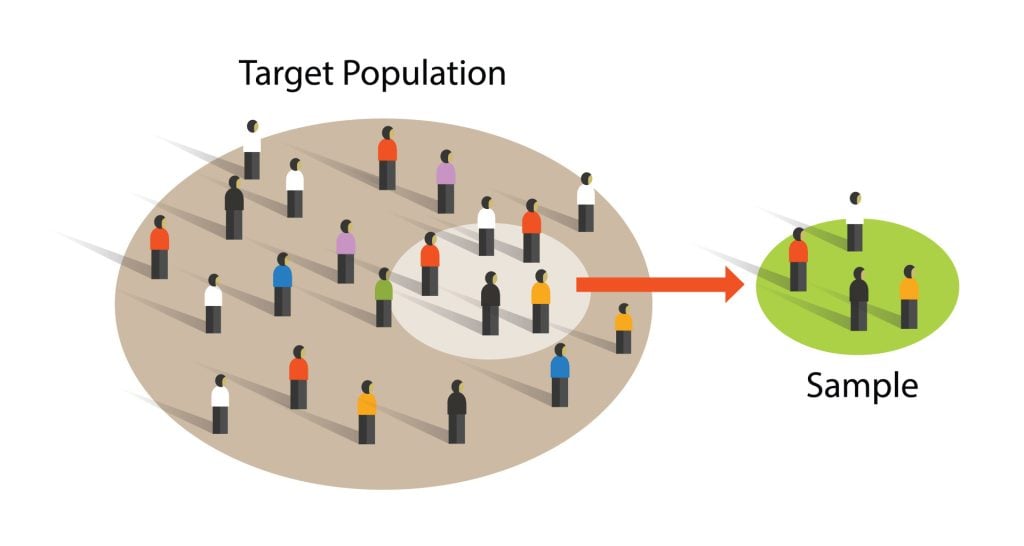
Studying every person in a target population is more or less impossible. Hence, psychologists select a sample or sub-group of the population that is likely to be representative of the target population we are interested in.
This is important because we want to generalize from the sample to the target population. The more representative the sample, the more confident the researcher can be that the results can be generalized to the target population.
One of the problems that can occur when selecting a sample from a target population is sampling bias. Sampling bias refers to situations where the sample does not reflect the characteristics of the target population.
Many psychology studies have a biased sample because they have used an opportunity sample that comprises university students as their participants (e.g., Asch ).
OK, so you’ve thought up this brilliant psychological study and designed it perfectly. But who will you try it out on, and how will you select your participants?
There are various sampling methods. The one chosen will depend on a number of factors (such as time, money, etc.).
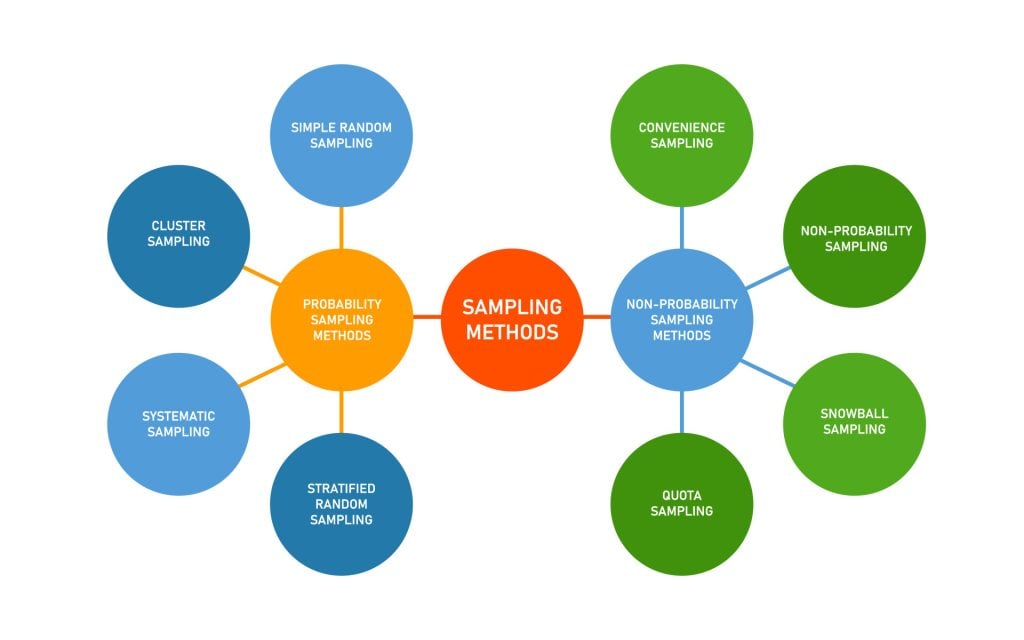
Random Sampling
Random sampling is a type of probability sampling where everyone in the entire target population has an equal chance of being selected.
This is similar to the national lottery. If the “population” is everyone who bought a lottery ticket, then everyone has an equal chance of winning the lottery (assuming they all have one ticket each).
Random samples require naming or numbering the target population and then using some raffle method to choose those to make up the sample. Random samples are the best method of selecting your sample from the population of interest.
- The advantages are that your sample should represent the target population and eliminate sampling bias.
- The disadvantage is that it is very difficult to achieve (i.e., time, effort, and money).
Stratified Sampling
During stratified sampling , the researcher identifies the different types of people that make up the target population and works out the proportions needed for the sample to be representative.
A list is made of each variable (e.g., IQ, gender, etc.) that might have an effect on the research. For example, if we are interested in the money spent on books by undergraduates, then the main subject studied may be an important variable.
For example, students studying English Literature may spend more money on books than engineering students, so if we use a large percentage of English students or engineering students, our results will not be accurate.
We have to determine the relative percentage of each group at a university, e.g., Engineering 10%, Social Sciences 15%, English 20%, Sciences 25%, Languages 10%, Law 5%, and Medicine 15%. The sample must then contain all these groups in the same proportion as the target population (university students).
- The disadvantage of stratified sampling is that gathering such a sample would be extremely time-consuming and difficult to do. This method is rarely used in Psychology.
- However, the advantage is that the sample should be highly representative of the target population, and therefore we can generalize from the results obtained.
Opportunity Sampling
Opportunity sampling is a method in which participants are chosen based on their ease of availability and proximity to the researcher, rather than using random or systematic criteria. It’s a type of convenience sampling .
An opportunity sample is obtained by asking members of the population of interest if they would participate in your research. An example would be selecting a sample of students from those coming out of the library.
- This is a quick and easy way of choosing participants (advantage)
- It may not provide a representative sample and could be biased (disadvantage).
Systematic Sampling
Systematic sampling is a method where every nth individual is selected from a list or sequence to form a sample, ensuring even and regular intervals between chosen subjects.
Participants are systematically selected (i.e., orderly/logical) from the target population, like every nth participant on a list of names.
To take a systematic sample, you list all the population members and then decide upon a sample you would like. By dividing the number of people in the population by the number of people you want in your sample, you get a number we will call n.
If you take every nth name, you will get a systematic sample of the correct size. If, for example, you wanted to sample 150 children from a school of 1,500, you would take every 10th name.
- The advantage of this method is that it should provide a representative sample.
Sample size
The sample size is a critical factor in determining the reliability and validity of a study’s findings. While increasing the sample size can enhance the generalizability of results, it’s also essential to balance practical considerations, such as resource constraints and diminishing returns from ever-larger samples.
Reliability and Validity
Reliability refers to the consistency and reproducibility of research findings across different occasions, researchers, or instruments. A small sample size may lead to inconsistent results due to increased susceptibility to random error or the influence of outliers. In contrast, a larger sample minimizes these errors, promoting more reliable results.
Validity pertains to the accuracy and truthfulness of research findings. For a study to be valid, it should accurately measure what it intends to do. A small, unrepresentative sample can compromise external validity, meaning the results don’t generalize well to the larger population. A larger sample captures more variability, ensuring that specific subgroups or anomalies don’t overly influence results.
Practical Considerations
Resource Constraints : Larger samples demand more time, money, and resources. Data collection becomes more extensive, data analysis more complex, and logistics more challenging.
Diminishing Returns : While increasing the sample size generally leads to improved accuracy and precision, there’s a point where adding more participants yields only marginal benefits. For instance, going from 50 to 500 participants might significantly boost a study’s robustness, but jumping from 10,000 to 10,500 might not offer a comparable advantage, especially considering the added costs.

Related Articles
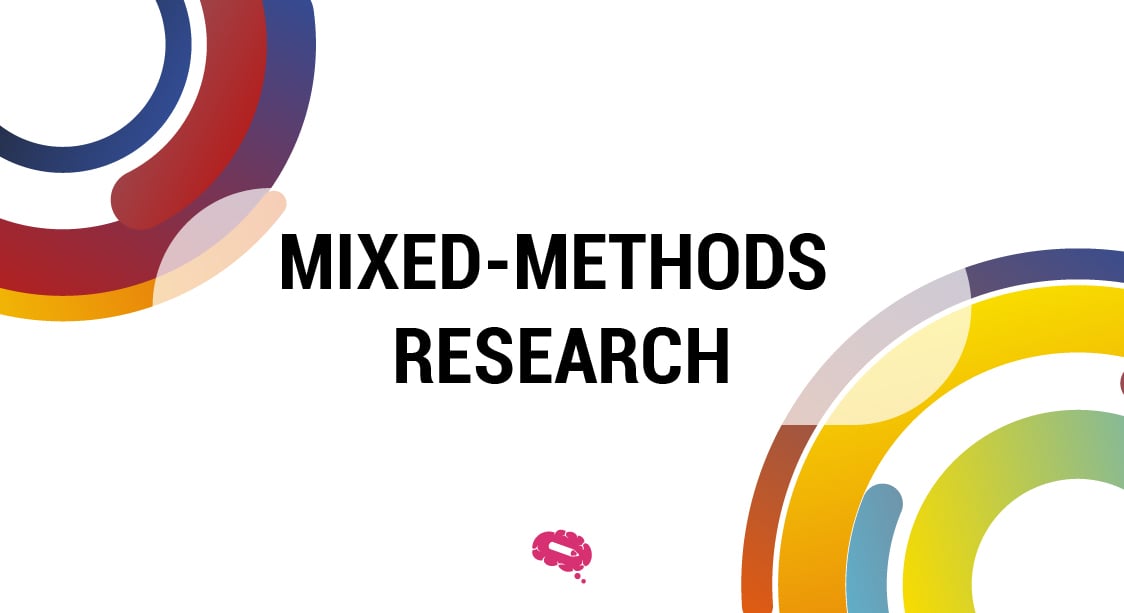
Research Methodology
Mixed Methods Research
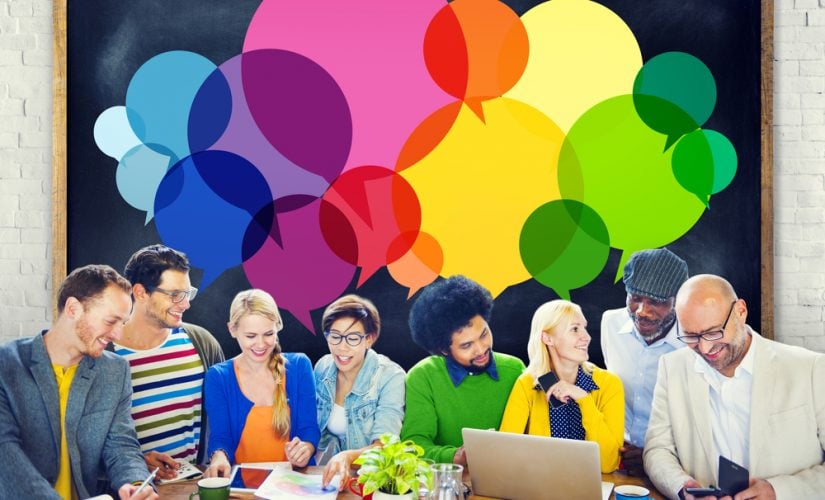
Conversation Analysis
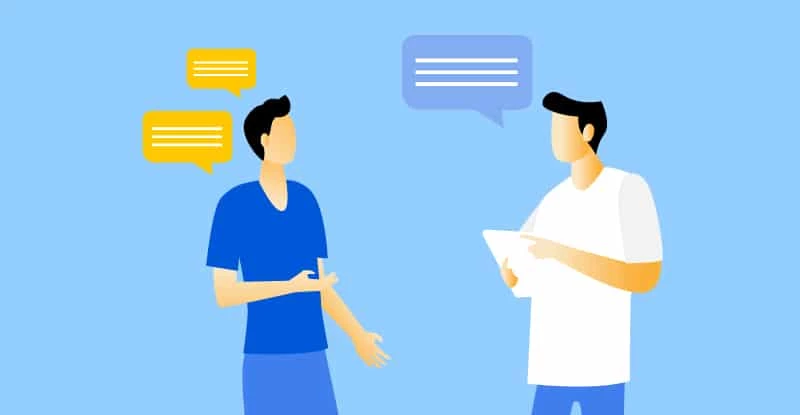
Discourse Analysis
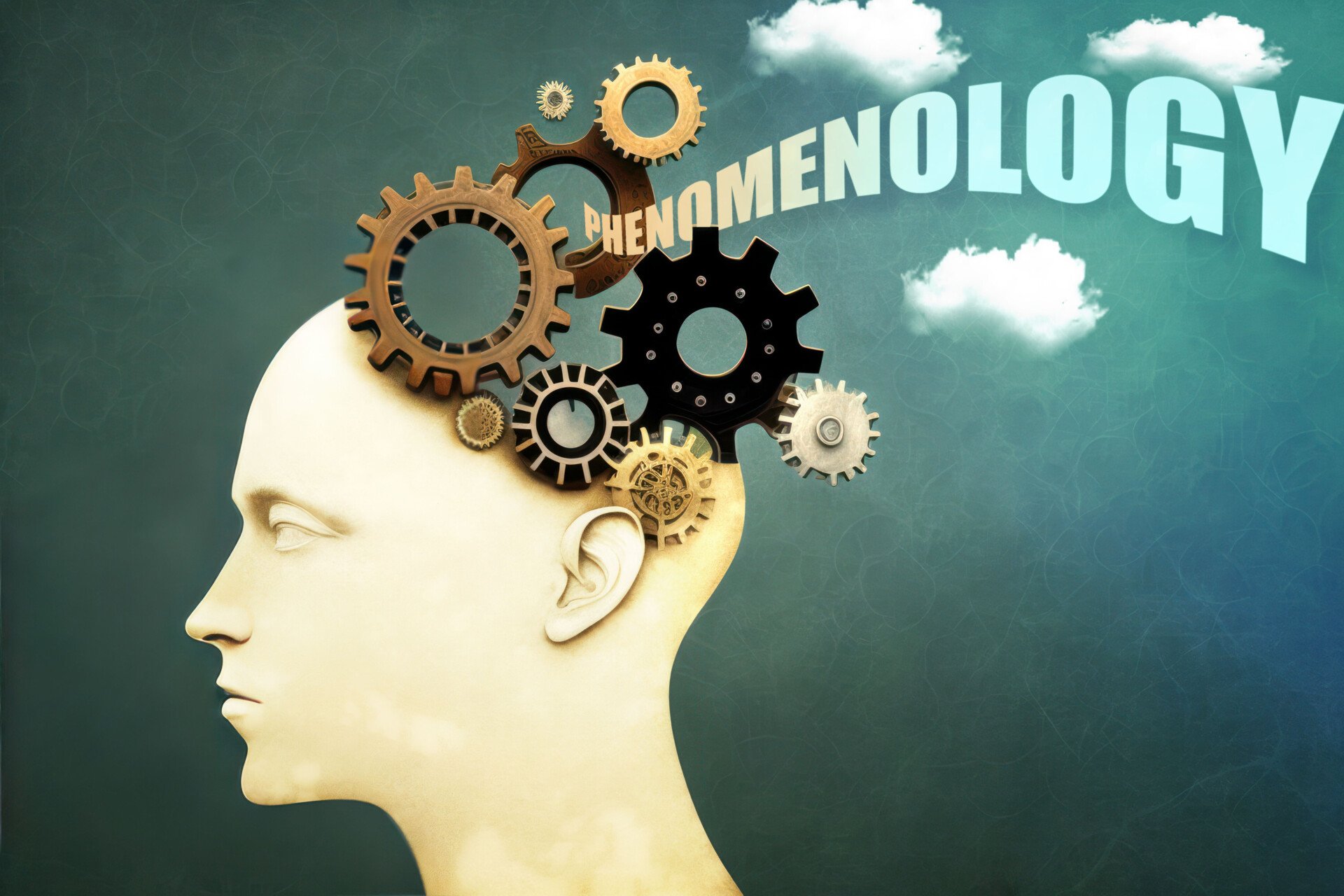
Phenomenology In Qualitative Research
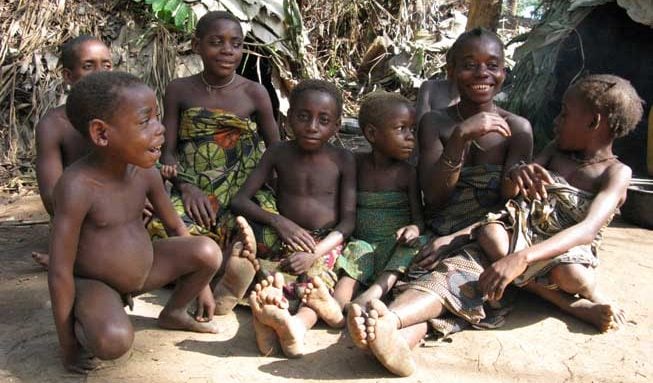
Ethnography In Qualitative Research
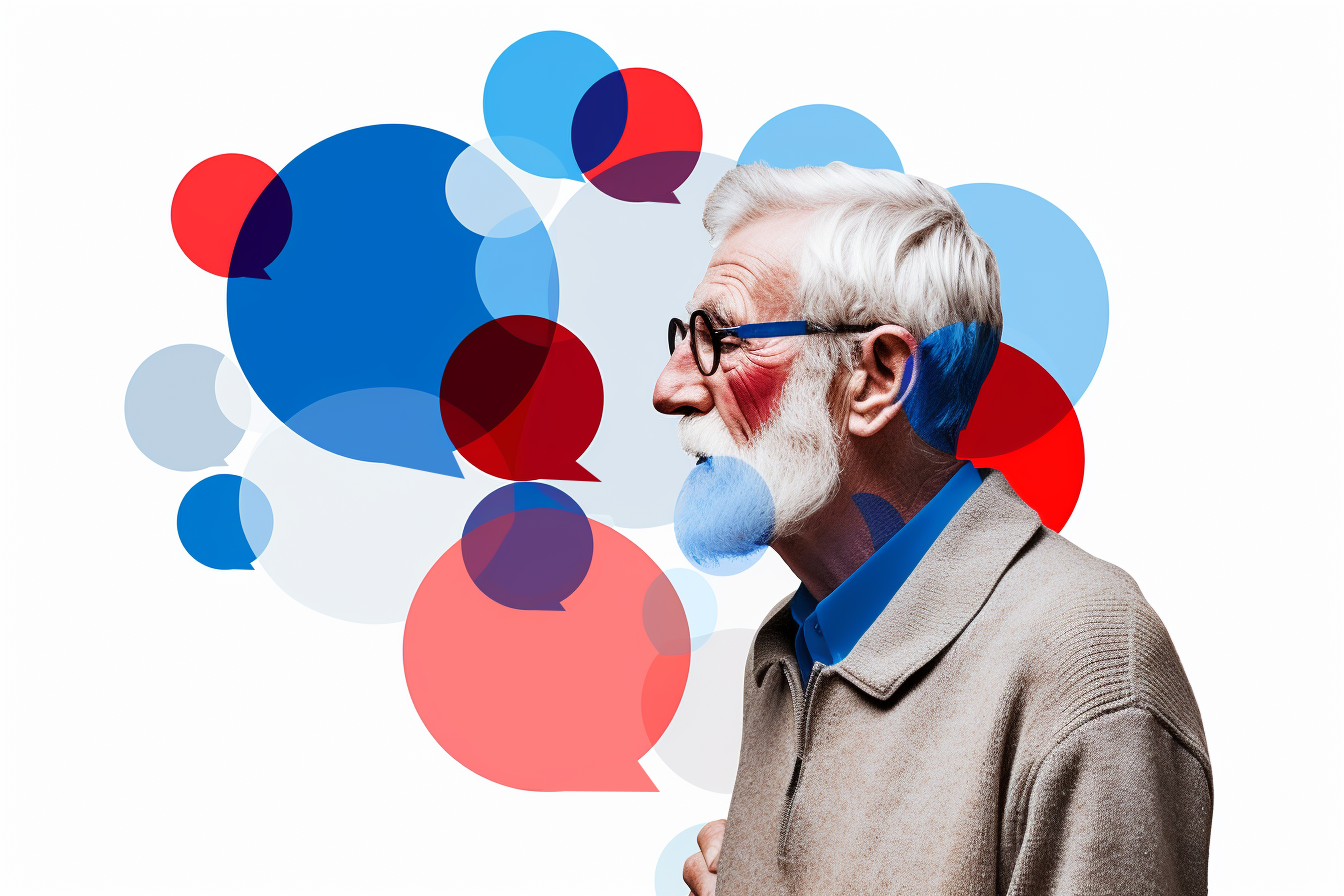
Narrative Analysis In Qualitative Research

Sampling Methods & Strategies 101
Everything you need to know (including examples)
By: Derek Jansen (MBA) | Expert Reviewed By: Kerryn Warren (PhD) | January 2023
If you’re new to research, sooner or later you’re bound to wander into the intimidating world of sampling methods and strategies. If you find yourself on this page, chances are you’re feeling a little overwhelmed or confused. Fear not – in this post we’ll unpack sampling in straightforward language , along with loads of examples .
Overview: Sampling Methods & Strategies
- What is sampling in a research context?
- The two overarching approaches
Simple random sampling
Stratified random sampling, cluster sampling, systematic sampling, purposive sampling, convenience sampling, snowball sampling.
- How to choose the right sampling method
What (exactly) is sampling?
At the simplest level, sampling (within a research context) is the process of selecting a subset of participants from a larger group . For example, if your research involved assessing US consumers’ perceptions about a particular brand of laundry detergent, you wouldn’t be able to collect data from every single person that uses laundry detergent (good luck with that!) – but you could potentially collect data from a smaller subset of this group.
In technical terms, the larger group is referred to as the population , and the subset (the group you’ll actually engage with in your research) is called the sample . Put another way, you can look at the population as a full cake and the sample as a single slice of that cake. In an ideal world, you’d want your sample to be perfectly representative of the population, as that would allow you to generalise your findings to the entire population. In other words, you’d want to cut a perfect cross-sectional slice of cake, such that the slice reflects every layer of the cake in perfect proportion.
Achieving a truly representative sample is, unfortunately, a little trickier than slicing a cake, as there are many practical challenges and obstacles to achieving this in a real-world setting. Thankfully though, you don’t always need to have a perfectly representative sample – it all depends on the specific research aims of each study – so don’t stress yourself out about that just yet!
With the concept of sampling broadly defined, let’s look at the different approaches to sampling to get a better understanding of what it all looks like in practice.
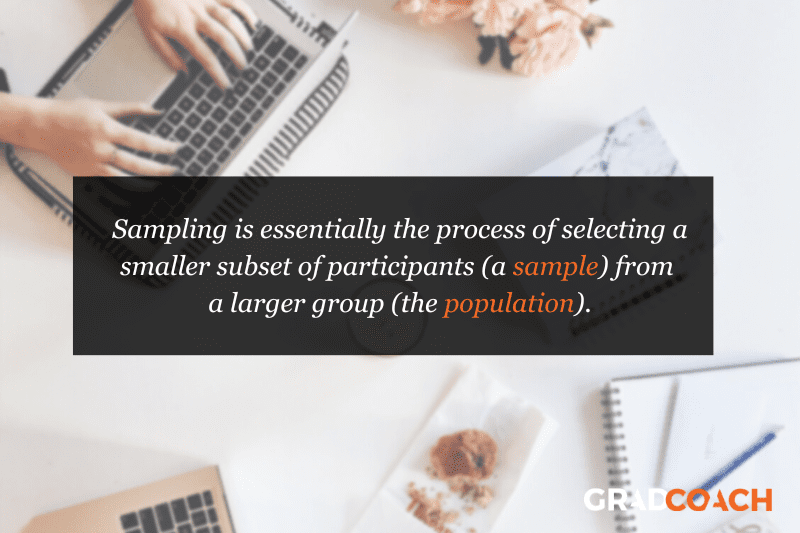
The two overarching sampling approaches
At the highest level, there are two approaches to sampling: probability sampling and non-probability sampling . Within each of these, there are a variety of sampling methods , which we’ll explore a little later.
Probability sampling involves selecting participants (or any unit of interest) on a statistically random basis , which is why it’s also called “random sampling”. In other words, the selection of each individual participant is based on a pre-determined process (not the discretion of the researcher). As a result, this approach achieves a random sample.
Probability-based sampling methods are most commonly used in quantitative research , especially when it’s important to achieve a representative sample that allows the researcher to generalise their findings.
Non-probability sampling , on the other hand, refers to sampling methods in which the selection of participants is not statistically random . In other words, the selection of individual participants is based on the discretion and judgment of the researcher, rather than on a pre-determined process.
Non-probability sampling methods are commonly used in qualitative research , where the richness and depth of the data are more important than the generalisability of the findings.
If that all sounds a little too conceptual and fluffy, don’t worry. Let’s take a look at some actual sampling methods to make it more tangible.
Need a helping hand?
Probability-based sampling methods
First, we’ll look at four common probability-based (random) sampling methods:
Importantly, this is not a comprehensive list of all the probability sampling methods – these are just four of the most common ones. So, if you’re interested in adopting a probability-based sampling approach, be sure to explore all the options.
Simple random sampling involves selecting participants in a completely random fashion , where each participant has an equal chance of being selected. Basically, this sampling method is the equivalent of pulling names out of a hat , except that you can do it digitally. For example, if you had a list of 500 people, you could use a random number generator to draw a list of 50 numbers (each number, reflecting a participant) and then use that dataset as your sample.
Thanks to its simplicity, simple random sampling is easy to implement , and as a consequence, is typically quite cheap and efficient . Given that the selection process is completely random, the results can be generalised fairly reliably. However, this also means it can hide the impact of large subgroups within the data, which can result in minority subgroups having little representation in the results – if any at all. To address this, one needs to take a slightly different approach, which we’ll look at next.
Stratified random sampling is similar to simple random sampling, but it kicks things up a notch. As the name suggests, stratified sampling involves selecting participants randomly , but from within certain pre-defined subgroups (i.e., strata) that share a common trait . For example, you might divide the population into strata based on gender, ethnicity, age range or level of education, and then select randomly from each group.
The benefit of this sampling method is that it gives you more control over the impact of large subgroups (strata) within the population. For example, if a population comprises 80% males and 20% females, you may want to “balance” this skew out by selecting a random sample from an equal number of males and females. This would, of course, reduce the representativeness of the sample, but it would allow you to identify differences between subgroups. So, depending on your research aims, the stratified approach could work well.
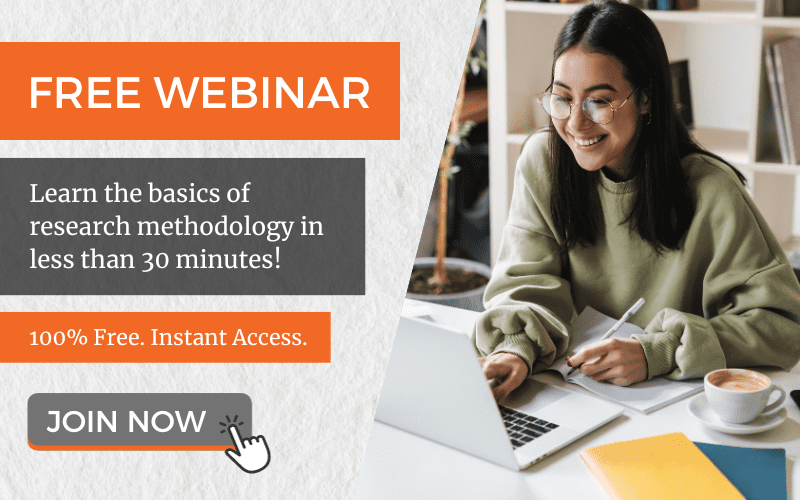
Next on the list is cluster sampling. As the name suggests, this sampling method involves sampling from naturally occurring, mutually exclusive clusters within a population – for example, area codes within a city or cities within a country. Once the clusters are defined, a set of clusters are randomly selected and then a set of participants are randomly selected from each cluster.
Now, you’re probably wondering, “how is cluster sampling different from stratified random sampling?”. Well, let’s look at the previous example where each cluster reflects an area code in a given city.
With cluster sampling, you would collect data from clusters of participants in a handful of area codes (let’s say 5 neighbourhoods). Conversely, with stratified random sampling, you would need to collect data from all over the city (i.e., many more neighbourhoods). You’d still achieve the same sample size either way (let’s say 200 people, for example), but with stratified sampling, you’d need to do a lot more running around, as participants would be scattered across a vast geographic area. As a result, cluster sampling is often the more practical and economical option.
If that all sounds a little mind-bending, you can use the following general rule of thumb. If a population is relatively homogeneous , cluster sampling will often be adequate. Conversely, if a population is quite heterogeneous (i.e., diverse), stratified sampling will generally be more appropriate.
The last probability sampling method we’ll look at is systematic sampling. This method simply involves selecting participants at a set interval , starting from a random point .
For example, if you have a list of students that reflects the population of a university, you could systematically sample that population by selecting participants at an interval of 8 . In other words, you would randomly select a starting point – let’s say student number 40 – followed by student 48, 56, 64, etc.
What’s important with systematic sampling is that the population list you select from needs to be randomly ordered . If there are underlying patterns in the list (for example, if the list is ordered by gender, IQ, age, etc.), this will result in a non-random sample, which would defeat the purpose of adopting this sampling method. Of course, you could safeguard against this by “shuffling” your population list using a random number generator or similar tool.
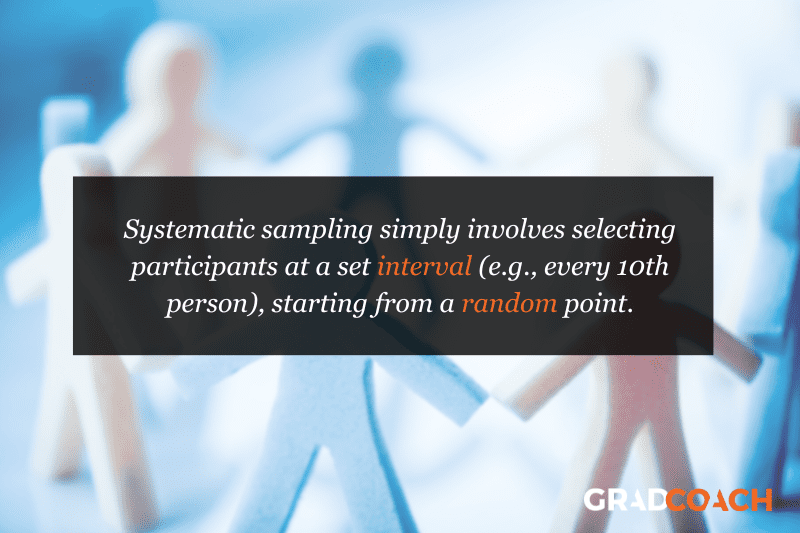
Non-probability-based sampling methods
Right, now that we’ve looked at a few probability-based sampling methods, let’s look at three non-probability methods :
Again, this is not an exhaustive list of all possible sampling methods, so be sure to explore further if you’re interested in adopting a non-probability sampling approach.
First up, we’ve got purposive sampling – also known as judgment , selective or subjective sampling. Again, the name provides some clues, as this method involves the researcher selecting participants using his or her own judgement , based on the purpose of the study (i.e., the research aims).
For example, suppose your research aims were to understand the perceptions of hyper-loyal customers of a particular retail store. In that case, you could use your judgement to engage with frequent shoppers, as well as rare or occasional shoppers, to understand what judgements drive the two behavioural extremes .
Purposive sampling is often used in studies where the aim is to gather information from a small population (especially rare or hard-to-find populations), as it allows the researcher to target specific individuals who have unique knowledge or experience . Naturally, this sampling method is quite prone to researcher bias and judgement error, and it’s unlikely to produce generalisable results, so it’s best suited to studies where the aim is to go deep rather than broad .
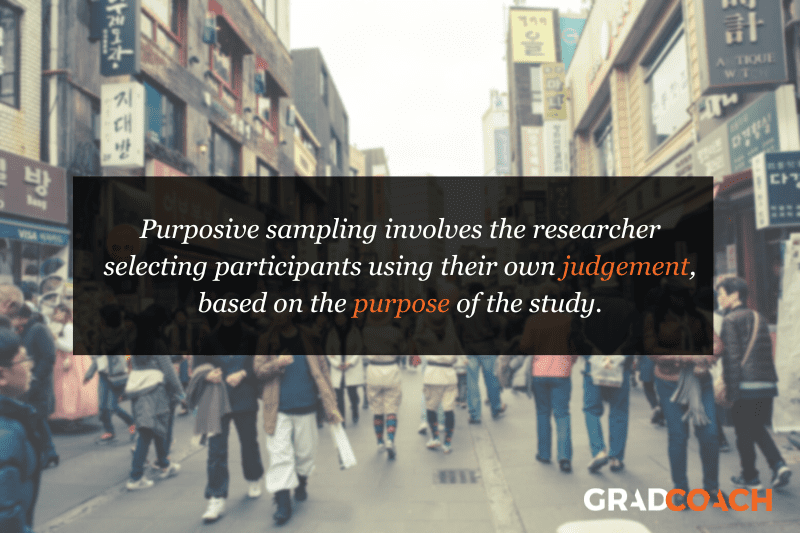
Next up, we have convenience sampling. As the name suggests, with this method, participants are selected based on their availability or accessibility . In other words, the sample is selected based on how convenient it is for the researcher to access it, as opposed to using a defined and objective process.
Naturally, convenience sampling provides a quick and easy way to gather data, as the sample is selected based on the individuals who are readily available or willing to participate. This makes it an attractive option if you’re particularly tight on resources and/or time. However, as you’d expect, this sampling method is unlikely to produce a representative sample and will of course be vulnerable to researcher bias , so it’s important to approach it with caution.
Last but not least, we have the snowball sampling method. This method relies on referrals from initial participants to recruit additional participants. In other words, the initial subjects form the first (small) snowball and each additional subject recruited through referral is added to the snowball, making it larger as it rolls along .
Snowball sampling is often used in research contexts where it’s difficult to identify and access a particular population. For example, people with a rare medical condition or members of an exclusive group. It can also be useful in cases where the research topic is sensitive or taboo and people are unlikely to open up unless they’re referred by someone they trust.
Simply put, snowball sampling is ideal for research that involves reaching hard-to-access populations . But, keep in mind that, once again, it’s a sampling method that’s highly prone to researcher bias and is unlikely to produce a representative sample. So, make sure that it aligns with your research aims and questions before adopting this method.
How to choose a sampling method
Now that we’ve looked at a few popular sampling methods (both probability and non-probability based), the obvious question is, “ how do I choose the right sampling method for my study?”. When selecting a sampling method for your research project, you’ll need to consider two important factors: your research aims and your resources .
As with all research design and methodology choices, your sampling approach needs to be guided by and aligned with your research aims, objectives and research questions – in other words, your golden thread. Specifically, you need to consider whether your research aims are primarily concerned with producing generalisable findings (in which case, you’ll likely opt for a probability-based sampling method) or with achieving rich , deep insights (in which case, a non-probability-based approach could be more practical). Typically, quantitative studies lean toward the former, while qualitative studies aim for the latter, so be sure to consider your broader methodology as well.
The second factor you need to consider is your resources and, more generally, the practical constraints at play. If, for example, you have easy, free access to a large sample at your workplace or university and a healthy budget to help you attract participants, that will open up multiple options in terms of sampling methods. Conversely, if you’re cash-strapped, short on time and don’t have unfettered access to your population of interest, you may be restricted to convenience or referral-based methods.
In short, be ready for trade-offs – you won’t always be able to utilise the “perfect” sampling method for your study, and that’s okay. Much like all the other methodological choices you’ll make as part of your study, you’ll often need to compromise and accept practical trade-offs when it comes to sampling. Don’t let this get you down though – as long as your sampling choice is well explained and justified, and the limitations of your approach are clearly articulated, you’ll be on the right track.
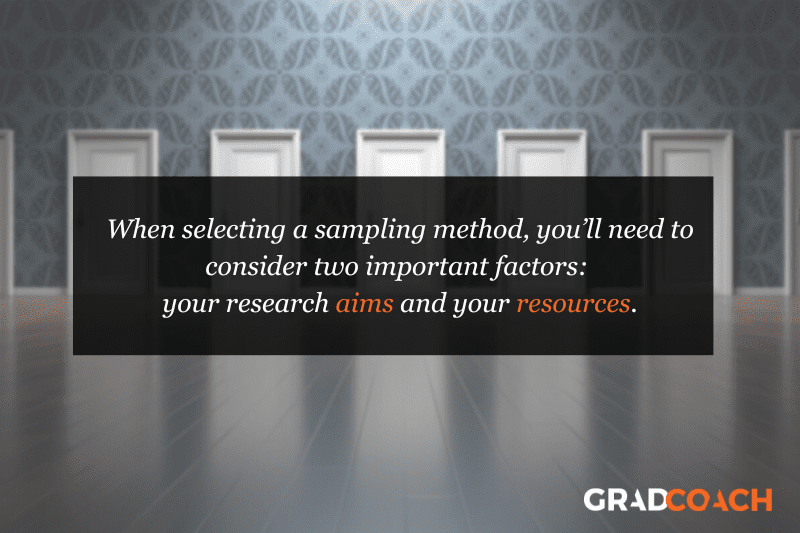
Let’s recap…
In this post, we’ve covered the basics of sampling within the context of a typical research project.
- Sampling refers to the process of defining a subgroup (sample) from the larger group of interest (population).
- The two overarching approaches to sampling are probability sampling (random) and non-probability sampling .
- Common probability-based sampling methods include simple random sampling, stratified random sampling, cluster sampling and systematic sampling.
- Common non-probability-based sampling methods include purposive sampling, convenience sampling and snowball sampling.
- When choosing a sampling method, you need to consider your research aims , objectives and questions, as well as your resources and other practical constraints .
If you’d like to see an example of a sampling strategy in action, be sure to check out our research methodology chapter sample .
Last but not least, if you need hands-on help with your sampling (or any other aspect of your research), take a look at our 1-on-1 coaching service , where we guide you through each step of the research process, at your own pace.
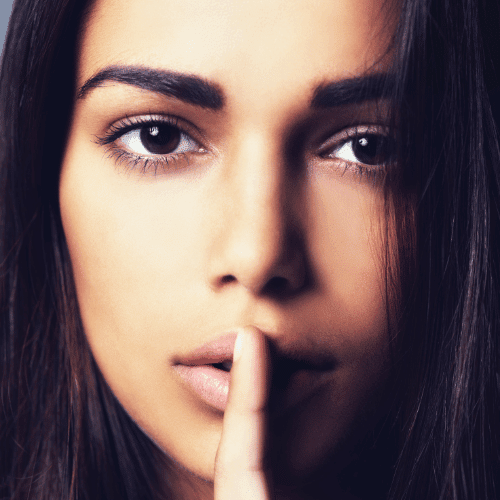
Psst... there’s more!
This post was based on one of our popular Research Bootcamps . If you're working on a research project, you'll definitely want to check this out ...
You Might Also Like:
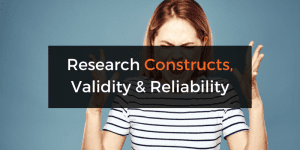
Excellent and helpful. Best site to get a full understanding of Research methodology. I’m nolonger as “clueless “..😉
Excellent and helpful for junior researcher!
Grad Coach tutorials are excellent – I recommend them to everyone doing research. I will be working with a sample of imprisoned women and now have a much clearer idea concerning sampling. Thank you to all at Grad Coach for generously sharing your expertise with students.
Submit a Comment Cancel reply
Your email address will not be published. Required fields are marked *
Save my name, email, and website in this browser for the next time I comment.
- Print Friendly
- Privacy Policy

Home » Sampling Methods – Types, Techniques and Examples
Sampling Methods – Types, Techniques and Examples
Table of Contents

Sampling refers to the process of selecting a subset of data from a larger population or dataset in order to analyze or make inferences about the whole population.
In other words, sampling involves taking a representative sample of data from a larger group or dataset in order to gain insights or draw conclusions about the entire group.
Sampling Methods
Sampling methods refer to the techniques used to select a subset of individuals or units from a larger population for the purpose of conducting statistical analysis or research.
Sampling is an essential part of the Research because it allows researchers to draw conclusions about a population without having to collect data from every member of that population, which can be time-consuming, expensive, or even impossible.
Types of Sampling Methods
Sampling can be broadly categorized into two main categories:
Probability Sampling
This type of sampling is based on the principles of random selection, and it involves selecting samples in a way that every member of the population has an equal chance of being included in the sample.. Probability sampling is commonly used in scientific research and statistical analysis, as it provides a representative sample that can be generalized to the larger population.
Type of Probability Sampling :
- Simple Random Sampling: In this method, every member of the population has an equal chance of being selected for the sample. This can be done using a random number generator or by drawing names out of a hat, for example.
- Systematic Sampling: In this method, the population is first divided into a list or sequence, and then every nth member is selected for the sample. For example, if every 10th person is selected from a list of 100 people, the sample would include 10 people.
- Stratified Sampling: In this method, the population is divided into subgroups or strata based on certain characteristics, and then a random sample is taken from each stratum. This is often used to ensure that the sample is representative of the population as a whole.
- Cluster Sampling: In this method, the population is divided into clusters or groups, and then a random sample of clusters is selected. Then, all members of the selected clusters are included in the sample.
- Multi-Stage Sampling : This method combines two or more sampling techniques. For example, a researcher may use stratified sampling to select clusters, and then use simple random sampling to select members within each cluster.
Non-probability Sampling
This type of sampling does not rely on random selection, and it involves selecting samples in a way that does not give every member of the population an equal chance of being included in the sample. Non-probability sampling is often used in qualitative research, where the aim is not to generalize findings to a larger population, but to gain an in-depth understanding of a particular phenomenon or group. Non-probability sampling methods can be quicker and more cost-effective than probability sampling methods, but they may also be subject to bias and may not be representative of the larger population.
Types of Non-probability Sampling :
- Convenience Sampling: In this method, participants are chosen based on their availability or willingness to participate. This method is easy and convenient but may not be representative of the population.
- Purposive Sampling: In this method, participants are selected based on specific criteria, such as their expertise or knowledge on a particular topic. This method is often used in qualitative research, but may not be representative of the population.
- Snowball Sampling: In this method, participants are recruited through referrals from other participants. This method is often used when the population is hard to reach, but may not be representative of the population.
- Quota Sampling: In this method, a predetermined number of participants are selected based on specific criteria, such as age or gender. This method is often used in market research, but may not be representative of the population.
- Volunteer Sampling: In this method, participants volunteer to participate in the study. This method is often used in research where participants are motivated by personal interest or altruism, but may not be representative of the population.
Applications of Sampling Methods
Applications of Sampling Methods from different fields:
- Psychology : Sampling methods are used in psychology research to study various aspects of human behavior and mental processes. For example, researchers may use stratified sampling to select a sample of participants that is representative of the population based on factors such as age, gender, and ethnicity. Random sampling may also be used to select participants for experimental studies.
- Sociology : Sampling methods are commonly used in sociological research to study social phenomena and relationships between individuals and groups. For example, researchers may use cluster sampling to select a sample of neighborhoods to study the effects of economic inequality on health outcomes. Stratified sampling may also be used to select a sample of participants that is representative of the population based on factors such as income, education, and occupation.
- Social sciences: Sampling methods are commonly used in social sciences to study human behavior and attitudes. For example, researchers may use stratified sampling to select a sample of participants that is representative of the population based on factors such as age, gender, and income.
- Marketing : Sampling methods are used in marketing research to collect data on consumer preferences, behavior, and attitudes. For example, researchers may use random sampling to select a sample of consumers to participate in a survey about a new product.
- Healthcare : Sampling methods are used in healthcare research to study the prevalence of diseases and risk factors, and to evaluate interventions. For example, researchers may use cluster sampling to select a sample of health clinics to participate in a study of the effectiveness of a new treatment.
- Environmental science: Sampling methods are used in environmental science to collect data on environmental variables such as water quality, air pollution, and soil composition. For example, researchers may use systematic sampling to collect soil samples at regular intervals across a field.
- Education : Sampling methods are used in education research to study student learning and achievement. For example, researchers may use stratified sampling to select a sample of schools that is representative of the population based on factors such as demographics and academic performance.
Examples of Sampling Methods
Probability Sampling Methods Examples:
- Simple random sampling Example : A researcher randomly selects participants from the population using a random number generator or drawing names from a hat.
- Stratified random sampling Example : A researcher divides the population into subgroups (strata) based on a characteristic of interest (e.g. age or income) and then randomly selects participants from each subgroup.
- Systematic sampling Example : A researcher selects participants at regular intervals from a list of the population.
Non-probability Sampling Methods Examples:
- Convenience sampling Example: A researcher selects participants who are conveniently available, such as students in a particular class or visitors to a shopping mall.
- Purposive sampling Example : A researcher selects participants who meet specific criteria, such as individuals who have been diagnosed with a particular medical condition.
- Snowball sampling Example : A researcher selects participants who are referred to them by other participants, such as friends or acquaintances.
How to Conduct Sampling Methods
some general steps to conduct sampling methods:
- Define the population: Identify the population of interest and clearly define its boundaries.
- Choose the sampling method: Select an appropriate sampling method based on the research question, characteristics of the population, and available resources.
- Determine the sample size: Determine the desired sample size based on statistical considerations such as margin of error, confidence level, or power analysis.
- Create a sampling frame: Develop a list of all individuals or elements in the population from which the sample will be drawn. The sampling frame should be comprehensive, accurate, and up-to-date.
- Select the sample: Use the chosen sampling method to select the sample from the sampling frame. The sample should be selected randomly, or if using a non-random method, every effort should be made to minimize bias and ensure that the sample is representative of the population.
- Collect data: Once the sample has been selected, collect data from each member of the sample using appropriate research methods (e.g., surveys, interviews, observations).
- Analyze the data: Analyze the data collected from the sample to draw conclusions about the population of interest.
When to use Sampling Methods
Sampling methods are used in research when it is not feasible or practical to study the entire population of interest. Sampling allows researchers to study a smaller group of individuals, known as a sample, and use the findings from the sample to make inferences about the larger population.
Sampling methods are particularly useful when:
- The population of interest is too large to study in its entirety.
- The cost and time required to study the entire population are prohibitive.
- The population is geographically dispersed or difficult to access.
- The research question requires specialized or hard-to-find individuals.
- The data collected is quantitative and statistical analyses are used to draw conclusions.
Purpose of Sampling Methods
The main purpose of sampling methods in research is to obtain a representative sample of individuals or elements from a larger population of interest, in order to make inferences about the population as a whole. By studying a smaller group of individuals, known as a sample, researchers can gather information about the population that would be difficult or impossible to obtain from studying the entire population.
Sampling methods allow researchers to:
- Study a smaller, more manageable group of individuals, which is typically less time-consuming and less expensive than studying the entire population.
- Reduce the potential for data collection errors and improve the accuracy of the results by minimizing sampling bias.
- Make inferences about the larger population with a certain degree of confidence, using statistical analyses of the data collected from the sample.
- Improve the generalizability and external validity of the findings by ensuring that the sample is representative of the population of interest.
Characteristics of Sampling Methods
Here are some characteristics of sampling methods:
- Randomness : Probability sampling methods are based on random selection, meaning that every member of the population has an equal chance of being selected. This helps to minimize bias and ensure that the sample is representative of the population.
- Representativeness : The goal of sampling is to obtain a sample that is representative of the larger population of interest. This means that the sample should reflect the characteristics of the population in terms of key demographic, behavioral, or other relevant variables.
- Size : The size of the sample should be large enough to provide sufficient statistical power for the research question at hand. The sample size should also be appropriate for the chosen sampling method and the level of precision desired.
- Efficiency : Sampling methods should be efficient in terms of time, cost, and resources required. The method chosen should be feasible given the available resources and time constraints.
- Bias : Sampling methods should aim to minimize bias and ensure that the sample is representative of the population of interest. Bias can be introduced through non-random selection or non-response, and can affect the validity and generalizability of the findings.
- Precision : Sampling methods should be precise in terms of providing estimates of the population parameters of interest. Precision is influenced by sample size, sampling method, and level of variability in the population.
- Validity : The validity of the sampling method is important for ensuring that the results obtained from the sample are accurate and can be generalized to the population of interest. Validity can be affected by sampling method, sample size, and the representativeness of the sample.
Advantages of Sampling Methods
Sampling methods have several advantages, including:
- Cost-Effective : Sampling methods are often much cheaper and less time-consuming than studying an entire population. By studying only a small subset of the population, researchers can gather valuable data without incurring the costs associated with studying the entire population.
- Convenience : Sampling methods are often more convenient than studying an entire population. For example, if a researcher wants to study the eating habits of people in a city, it would be very difficult and time-consuming to study every single person in the city. By using sampling methods, the researcher can obtain data from a smaller subset of people, making the study more feasible.
- Accuracy: When done correctly, sampling methods can be very accurate. By using appropriate sampling techniques, researchers can obtain a sample that is representative of the entire population. This allows them to make accurate generalizations about the population as a whole based on the data collected from the sample.
- Time-Saving: Sampling methods can save a lot of time compared to studying the entire population. By studying a smaller sample, researchers can collect data much more quickly than they could if they studied every single person in the population.
- Less Bias : Sampling methods can reduce bias in a study. If a researcher were to study the entire population, it would be very difficult to eliminate all sources of bias. However, by using appropriate sampling techniques, researchers can reduce bias and obtain a sample that is more representative of the entire population.
Limitations of Sampling Methods
- Sampling Error : Sampling error is the difference between the sample statistic and the population parameter. It is the result of selecting a sample rather than the entire population. The larger the sample, the lower the sampling error. However, no matter how large the sample size, there will always be some degree of sampling error.
- Selection Bias: Selection bias occurs when the sample is not representative of the population. This can happen if the sample is not selected randomly or if some groups are underrepresented in the sample. Selection bias can lead to inaccurate conclusions about the population.
- Non-response Bias : Non-response bias occurs when some members of the sample do not respond to the survey or study. This can result in a biased sample if the non-respondents differ from the respondents in important ways.
- Time and Cost : While sampling can be cost-effective, it can still be expensive and time-consuming to select a sample that is representative of the population. Depending on the sampling method used, it may take a long time to obtain a sample that is large enough and representative enough to be useful.
- Limited Information : Sampling can only provide information about the variables that are measured. It may not provide information about other variables that are relevant to the research question but were not measured.
- Generalization : The extent to which the findings from a sample can be generalized to the population depends on the representativeness of the sample. If the sample is not representative of the population, it may not be possible to generalize the findings to the population as a whole.
About the author
Muhammad Hassan
Researcher, Academic Writer, Web developer
You may also like

Purposive Sampling – Methods, Types and Examples

Cluster Sampling – Types, Method and Examples

Volunteer Sampling – Definition, Methods and...

Quota Sampling – Types, Methods and Examples

Convenience Sampling – Method, Types and Examples

Stratified Random Sampling – Definition, Method...

Statistics Made Easy
Types of Sampling Methods (With Examples)
Researchers are often interested in answering questions about populations like:
- What is the average height of a certain species of plant?
- What is the average weight of a certain species of bird?
- What percentage of citizens in a certain city support a certain law?
One way to answer these questions is to go around and collect data on every single individual in the population of interest.
However, this is typically too costly and time-consuming which is why researchers instead take a sample of the population and use the data from the sample to draw conclusions about the population as a whole.

There are many different methods researchers can potentially use to obtain individuals to be in a sample. These are known as sampling methods .
In this post we share the most commonly used sampling methods in statistics, including the benefits and drawbacks of the various methods.
Probability Sampling Methods
The first class of sampling methods is known as probability sampling methods because every member in a population has an equal probability of being selected to be in the sample.
Simple random sample

Definition: Every member of a population has an equal chance of being selected to be in the sample. Randomly select members through the use of a random number generator or some means of random selection.
Example: We put the names of every student in a class into a hat and randomly draw out names to get a sample of students.
Benefit: Simple random samples are usually representative of the population we’re interested in since every member has an equal chance of being included in the sample.
Stratified random sample

Definition: Split a population into groups. Randomly select some members from each group to be in the sample.
Example: Split up all students in a school according to their grade – freshman, sophomores, juniors, and seniors. Ask 50 students from each grade to complete a survey about the school lunches.
Benefit: Stratified random samples ensure that members from each group in the population are included in the survey.
Cluster random sample

Definition: Split a population into clusters. Randomly select some of the clusters and include all members from those clusters in the sample.
Example: A company that gives whale watching tours wants to survey its customers. Out of ten tours they give one day, they randomly select four tours and ask every customer about their experience.
Benefit: Cluster random samples get every member from some of the groups, which is useful when each group is reflective of the population as a whole.
Systematic random sample

Definition: Put every member of a population into some order. Choosing a random starting point and select every n th member to be in the sample.
Example: A teacher puts students in alphabetical order according to their last name, randomly chooses a starting point, and picks every 5th student to be in the sample.
Benefit: Systematic random samples are usually representative of the population we’re interested in since every member has an equal chance of being included in the sample.
Non-probability Sampling Methods
Another class of sampling methods is known as non-probability sampling methods because not every member in a population has an equal probability of being selected to be in the sample.
This type of sampling method is sometimes used because it’s much cheaper and more convenient compared to probability sampling methods. It’s often used during exploratory analysis when researchers simply want to gain an initial understanding of a population.
However, the samples that result from these sampling methods cannot be used to draw inferences about the populations they came from because they typically aren’t representative samples.
Convenience sample
Definition: Choose members of a population that are readily available to be included in the sample.
Example: A researcher stands in front of a library during the day and polls people that happen to walk by.
Drawback: Location and time of day will affect the results. More than likely, the sample will suffer from undercoverage bias since certain people (e.g. those who work during the day) will not be represented as much in the sample.
Voluntary response sample
Definition: A researcher puts out a request for volunteers to be included in a study and members of a population voluntarily decide to be included in the sample or not.
Example: A radio host asks listeners to go online and take a survey on his website.
Drawback: People who voluntarily respond will likely have stronger opinions (positive or negative) than the rest of the population, which makes them an unrepresentative sample. Using this sampling method, the sample is likely to suffer from nonresponse bias – certain groups of people are simply less likely to provide responses.
Snowball sample
Definition: Researchers recruit initial subjects to be in a study and then ask those initial subjects to recruit additional subjects to be in the study. Using this approach, the sample size “snowballs” bigger and bigger as each additional subject recruits more subjects.
Example: Researchers are conducting a study of individuals with rare diseases, but it’s difficult to find individuals who actually have the disease. However, if they can find just a few initial individuals to be in the study then they can ask them to recruit further individuals they may know through a private support group or through some other means.
Drawback: Sampling bias is likely to occur. Because initial subjects recruit additional subjects, it’s likely that many of the subjects will share similar traits or characteristics that might be unrepresentative of the larger population under study. Thus, findings from the sample can’t be extrapolated to the population.
Read more about snowballing sampling here .
Purposive sample
Definition: Researchers recruit individuals based on who they think will be most useful based on the purpose of their study.
Example: Researchers want to know about the opinions that individuals in a city have about a potential new rock climbing gym being placed in the city square so they purposely seek out individuals that hang out at other rock climbing gyms around the city.
Drawback: The individuals in the sample are unlikely to be representative of the overall population. Thus, findings from the sample can’t be extrapolated to the population.
Featured Posts

Hey there. My name is Zach Bobbitt. I have a Masters of Science degree in Applied Statistics and I’ve worked on machine learning algorithms for professional businesses in both healthcare and retail. I’m passionate about statistics, machine learning, and data visualization and I created Statology to be a resource for both students and teachers alike. My goal with this site is to help you learn statistics through using simple terms, plenty of real-world examples, and helpful illustrations.
9 Replies to “Types of Sampling Methods (With Examples)”
Thank You for explaining the sampling types with simple easy to understand examples. According to me this feedback falls under voluntary type as you ask to fill forms online which not compulsory ……. hope I understand the concept.
Insightful. Thanks for sharing these notes.
Wow this is amazing it’s really understandable
I am so glad I found your site
Nice topic before reading this I have no idea about the topic but reading it makes me understand the whole concept of it thanks to you for making this topic simple. The words you use and the examples you gave were like very simple to understand for a person. The topic may be explained in another way in difficult manner but you choosed simple words to make understand the difficult topic. Thank you sir for this information.
I really enjoyed the whole course. A great course about sampling.
Simple rendom sampling Stratified random sample Cluster random sample Systematic random sample Non-probability Sampling Methods Convenience sample Voluntary response sample Snowball sample Purposive sample
All the topic explained in a very simple way really amazing Thanks 👍
Compelling statistical work. Good job and congratulations.
Thank you for your support and feedback Ruth! We greatly appreciate it!
irrevocably perfect !
Leave a Reply Cancel reply
Your email address will not be published. Required fields are marked *
Join the Statology Community
Sign up to receive Statology's exclusive study resource: 100 practice problems with step-by-step solutions. Plus, get our latest insights, tutorials, and data analysis tips straight to your inbox!
By subscribing you accept Statology's Privacy Policy.
- En español – ExME
- Em português – EME
What are sampling methods and how do you choose the best one?
Posted on 18th November 2020 by Mohamed Khalifa
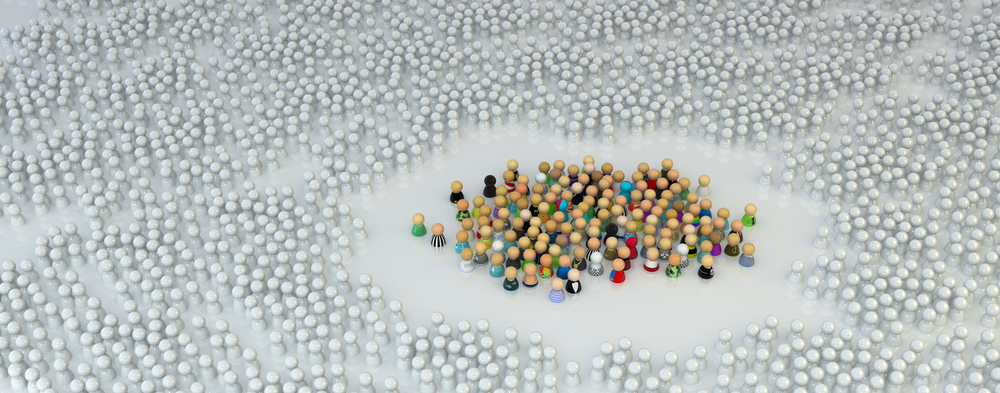
This tutorial will introduce sampling methods and potential sampling errors to avoid when conducting medical research.
Introduction to sampling methods
Examples of different sampling methods, choosing the best sampling method.
It is important to understand why we sample the population; for example, studies are built to investigate the relationships between risk factors and disease. In other words, we want to find out if this is a true association, while still aiming for the minimum risk for errors such as: chance, bias or confounding .
However, it would not be feasible to experiment on the whole population, we would need to take a good sample and aim to reduce the risk of having errors by proper sampling technique.
What is a sampling frame?
A sampling frame is a record of the target population containing all participants of interest. In other words, it is a list from which we can extract a sample.
What makes a good sample?
A good sample should be a representative subset of the population we are interested in studying, therefore, with each participant having equal chance of being randomly selected into the study.
We could choose a sampling method based on whether we want to account for sampling bias; a random sampling method is often preferred over a non-random method for this reason. Random sampling examples include: simple, systematic, stratified, and cluster sampling. Non-random sampling methods are liable to bias, and common examples include: convenience, purposive, snowballing, and quota sampling. For the purposes of this blog we will be focusing on random sampling methods .
Example: We want to conduct an experimental trial in a small population such as: employees in a company, or students in a college. We include everyone in a list and use a random number generator to select the participants
Advantages: Generalisable results possible, random sampling, the sampling frame is the whole population, every participant has an equal probability of being selected
Disadvantages: Less precise than stratified method, less representative than the systematic method
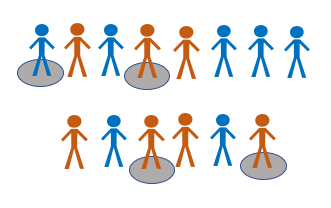
Example: Every nth patient entering the out-patient clinic is selected and included in our sample
Advantages: More feasible than simple or stratified methods, sampling frame is not always required
Disadvantages: Generalisability may decrease if baseline characteristics repeat across every nth participant
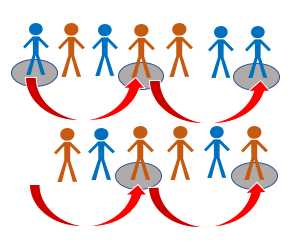
Example: We have a big population (a city) and we want to ensure representativeness of all groups with a pre-determined characteristic such as: age groups, ethnic origin, and gender
Advantages: Inclusive of strata (subgroups), reliable and generalisable results
Disadvantages: Does not work well with multiple variables
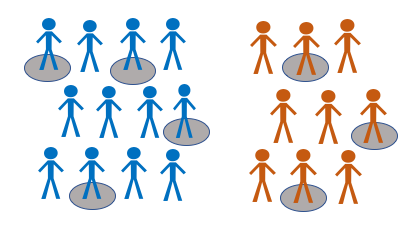
Example: 10 schools have the same number of students across the county. We can randomly select 3 out of 10 schools as our clusters
Advantages: Readily doable with most budgets, does not require a sampling frame
Disadvantages: Results may not be reliable nor generalisable
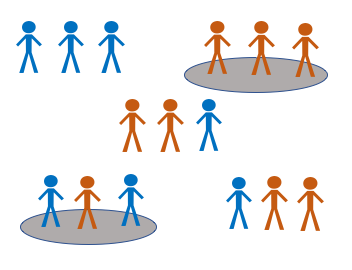
How can you identify sampling errors?
Non-random selection increases the probability of sampling (selection) bias if the sample does not represent the population we want to study. We could avoid this by random sampling and ensuring representativeness of our sample with regards to sample size.
An inadequate sample size decreases the confidence in our results as we may think there is no significant difference when actually there is. This type two error results from having a small sample size, or from participants dropping out of the sample.
In medical research of disease, if we select people with certain diseases while strictly excluding participants with other co-morbidities, we run the risk of diagnostic purity bias where important sub-groups of the population are not represented.
Furthermore, measurement bias may occur during re-collection of risk factors by participants (recall bias) or assessment of outcome where people who live longer are associated with treatment success, when in fact people who died were not included in the sample or data analysis (survivors bias).
By following the steps below we could choose the best sampling method for our study in an orderly fashion.
Research objectiveness
Firstly, a refined research question and goal would help us define our population of interest. If our calculated sample size is small then it would be easier to get a random sample. If, however, the sample size is large, then we should check if our budget and resources can handle a random sampling method.
Sampling frame availability
Secondly, we need to check for availability of a sampling frame (Simple), if not, could we make a list of our own (Stratified). If neither option is possible, we could still use other random sampling methods, for instance, systematic or cluster sampling.
Study design
Moreover, we could consider the prevalence of the topic (exposure or outcome) in the population, and what would be the suitable study design. In addition, checking if our target population is widely varied in its baseline characteristics. For example, a population with large ethnic subgroups could best be studied using a stratified sampling method.
Random sampling
Finally, the best sampling method is always the one that could best answer our research question while also allowing for others to make use of our results (generalisability of results). When we cannot afford a random sampling method, we can always choose from the non-random sampling methods.
To sum up, we now understand that choosing between random or non-random sampling methods is multifactorial. We might often be tempted to choose a convenience sample from the start, but that would not only decrease precision of our results, and would make us miss out on producing research that is more robust and reliable.
References (pdf)
Mohamed Khalifa
Leave a reply cancel reply.
Your email address will not be published. Required fields are marked *
Save my name, email, and website in this browser for the next time I comment.
No Comments on What are sampling methods and how do you choose the best one?
Thank you for this overview. A concise approach for research.
really helps! am an ecology student preparing to write my lab report for sampling.
I learned a lot to the given presentation.. It’s very comprehensive… Thanks for sharing…
Very informative and useful for my study. Thank you
Oversimplified info on sampling methods. Probabilistic of the sampling and sampling of samples by chance does rest solely on the random methods. Factors such as the random visits or presentation of the potential participants at clinics or sites could be sufficiently random in nature and should be used for the sake of efficiency and feasibility. Nevertheless, this approach has to be taken only after careful thoughts. Representativeness of the study samples have to be checked at the end or during reporting by comparing it to the published larger studies or register of some kind in/from the local population.
Thank you so much Mr.mohamed very useful and informative article
Subscribe to our newsletter
You will receive our monthly newsletter and free access to Trip Premium.
Related Articles
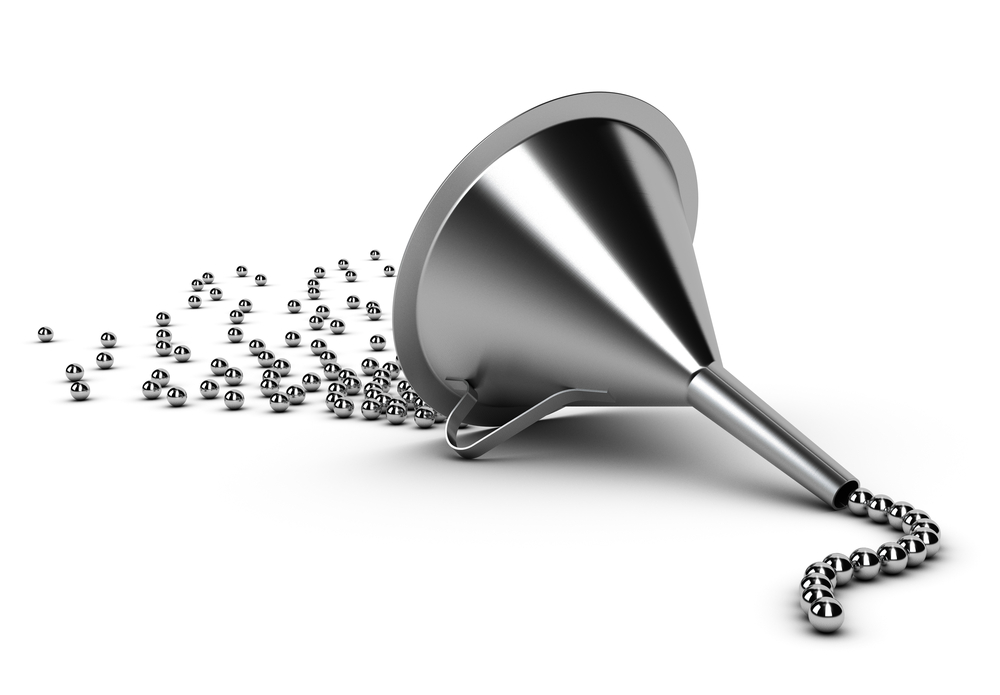
How to read a funnel plot
This blog introduces you to funnel plots, guiding you through how to read them and what may cause them to look asymmetrical.
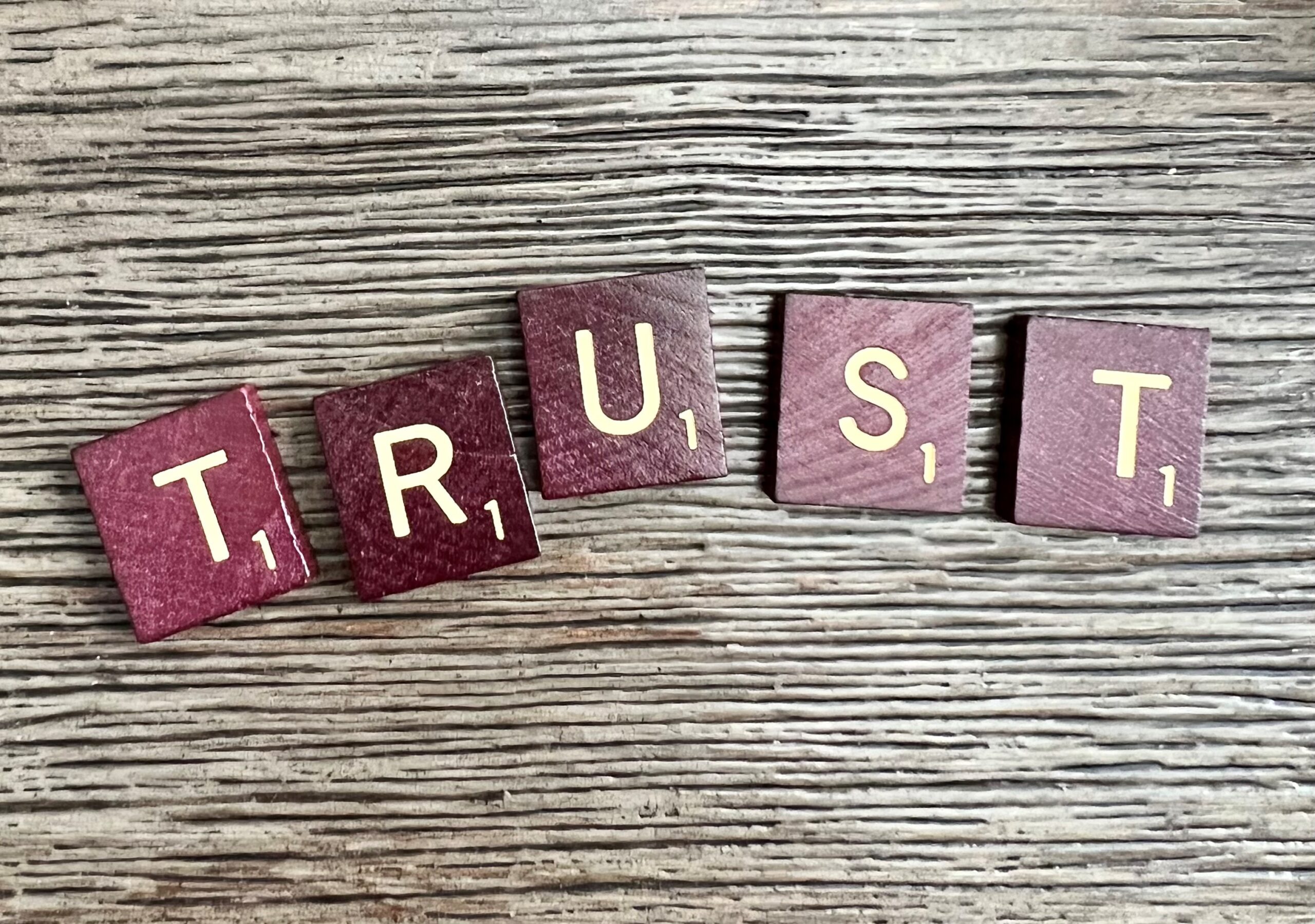
Internal and external validity: what are they and how do they differ?
Is this study valid? Can I trust this study’s methods and design? Can I apply the results of this study to other contexts? Learn more about internal and external validity in research to help you answer these questions when you next look at a paper.
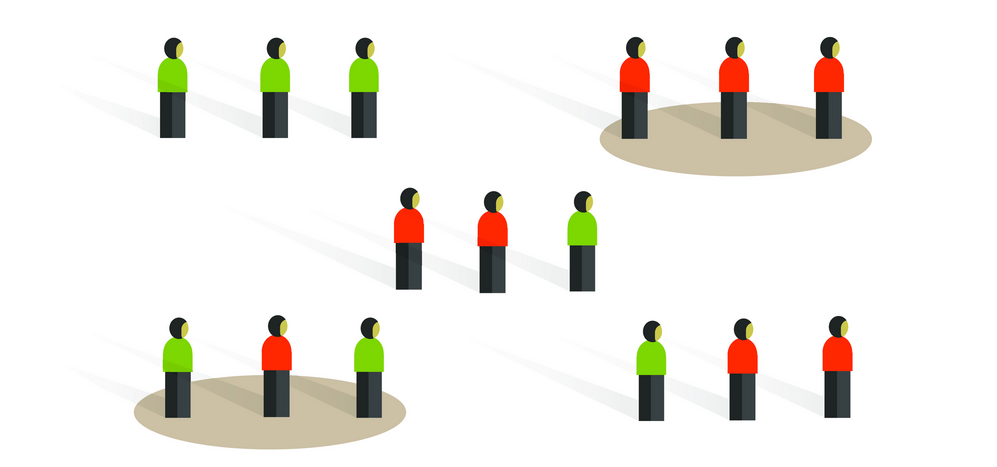
Cluster Randomized Trials: Concepts
This blog summarizes the concepts of cluster randomization, and the logistical and statistical considerations while designing a cluster randomized controlled trial.
Have a language expert improve your writing
Run a free plagiarism check in 10 minutes, automatically generate references for free.
- Knowledge Base
- Methodology
- Sampling Methods | Types, Techniques, & Examples
Sampling Methods | Types, Techniques, & Examples
Published on 3 May 2022 by Shona McCombes . Revised on 10 October 2022.
When you conduct research about a group of people, it’s rarely possible to collect data from every person in that group. Instead, you select a sample. The sample is the group of individuals who will actually participate in the research.
To draw valid conclusions from your results, you have to carefully decide how you will select a sample that is representative of the group as a whole. There are two types of sampling methods:
- Probability sampling involves random selection, allowing you to make strong statistical inferences about the whole group. It minimises the risk of selection bias .
- Non-probability sampling involves non-random selection based on convenience or other criteria, allowing you to easily collect data.
You should clearly explain how you selected your sample in the methodology section of your paper or thesis.
Table of contents
Population vs sample, probability sampling methods, non-probability sampling methods, frequently asked questions about sampling.
First, you need to understand the difference between a population and a sample , and identify the target population of your research.
- The population is the entire group that you want to draw conclusions about.
- The sample is the specific group of individuals that you will collect data from.
The population can be defined in terms of geographical location, age, income, and many other characteristics.
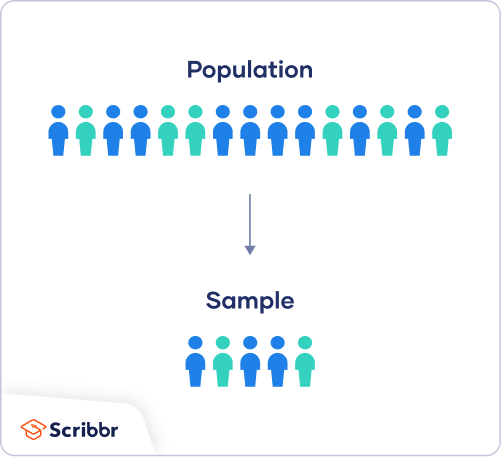
It is important to carefully define your target population according to the purpose and practicalities of your project.
If the population is very large, demographically mixed, and geographically dispersed, it might be difficult to gain access to a representative sample.
Sampling frame
The sampling frame is the actual list of individuals that the sample will be drawn from. Ideally, it should include the entire target population (and nobody who is not part of that population).
You are doing research on working conditions at Company X. Your population is all 1,000 employees of the company. Your sampling frame is the company’s HR database, which lists the names and contact details of every employee.
Sample size
The number of individuals you should include in your sample depends on various factors, including the size and variability of the population and your research design. There are different sample size calculators and formulas depending on what you want to achieve with statistical analysis .
Prevent plagiarism, run a free check.
Probability sampling means that every member of the population has a chance of being selected. It is mainly used in quantitative research . If you want to produce results that are representative of the whole population, probability sampling techniques are the most valid choice.
There are four main types of probability sample.
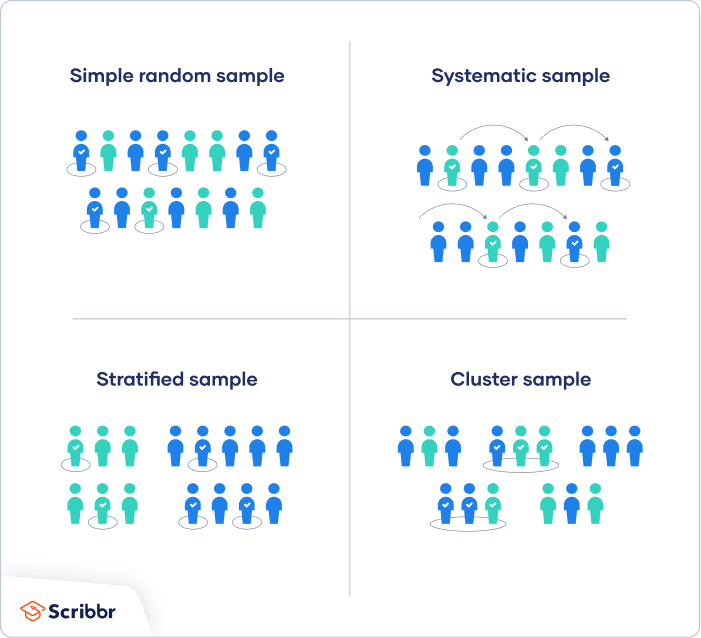
1. Simple random sampling
In a simple random sample , every member of the population has an equal chance of being selected. Your sampling frame should include the whole population.
To conduct this type of sampling, you can use tools like random number generators or other techniques that are based entirely on chance.
You want to select a simple random sample of 100 employees of Company X. You assign a number to every employee in the company database from 1 to 1000, and use a random number generator to select 100 numbers.
2. Systematic sampling
Systematic sampling is similar to simple random sampling, but it is usually slightly easier to conduct. Every member of the population is listed with a number, but instead of randomly generating numbers, individuals are chosen at regular intervals.
All employees of the company are listed in alphabetical order. From the first 10 numbers, you randomly select a starting point: number 6. From number 6 onwards, every 10th person on the list is selected (6, 16, 26, 36, and so on), and you end up with a sample of 100 people.
If you use this technique, it is important to make sure that there is no hidden pattern in the list that might skew the sample. For example, if the HR database groups employees by team, and team members are listed in order of seniority, there is a risk that your interval might skip over people in junior roles, resulting in a sample that is skewed towards senior employees.
3. Stratified sampling
Stratified sampling involves dividing the population into subpopulations that may differ in important ways. It allows you draw more precise conclusions by ensuring that every subgroup is properly represented in the sample.
To use this sampling method, you divide the population into subgroups (called strata) based on the relevant characteristic (e.g., gender, age range, income bracket, job role).
Based on the overall proportions of the population, you calculate how many people should be sampled from each subgroup. Then you use random or systematic sampling to select a sample from each subgroup.
The company has 800 female employees and 200 male employees. You want to ensure that the sample reflects the gender balance of the company, so you sort the population into two strata based on gender. Then you use random sampling on each group, selecting 80 women and 20 men, which gives you a representative sample of 100 people.
4. Cluster sampling
Cluster sampling also involves dividing the population into subgroups, but each subgroup should have similar characteristics to the whole sample. Instead of sampling individuals from each subgroup, you randomly select entire subgroups.
If it is practically possible, you might include every individual from each sampled cluster. If the clusters themselves are large, you can also sample individuals from within each cluster using one of the techniques above. This is called multistage sampling .
This method is good for dealing with large and dispersed populations, but there is more risk of error in the sample, as there could be substantial differences between clusters. It’s difficult to guarantee that the sampled clusters are really representative of the whole population.
The company has offices in 10 cities across the country (all with roughly the same number of employees in similar roles). You don’t have the capacity to travel to every office to collect your data, so you use random sampling to select 3 offices – these are your clusters.
In a non-probability sample , individuals are selected based on non-random criteria, and not every individual has a chance of being included.
This type of sample is easier and cheaper to access, but it has a higher risk of sampling bias . That means the inferences you can make about the population are weaker than with probability samples, and your conclusions may be more limited. If you use a non-probability sample, you should still aim to make it as representative of the population as possible.
Non-probability sampling techniques are often used in exploratory and qualitative research . In these types of research, the aim is not to test a hypothesis about a broad population, but to develop an initial understanding of a small or under-researched population.
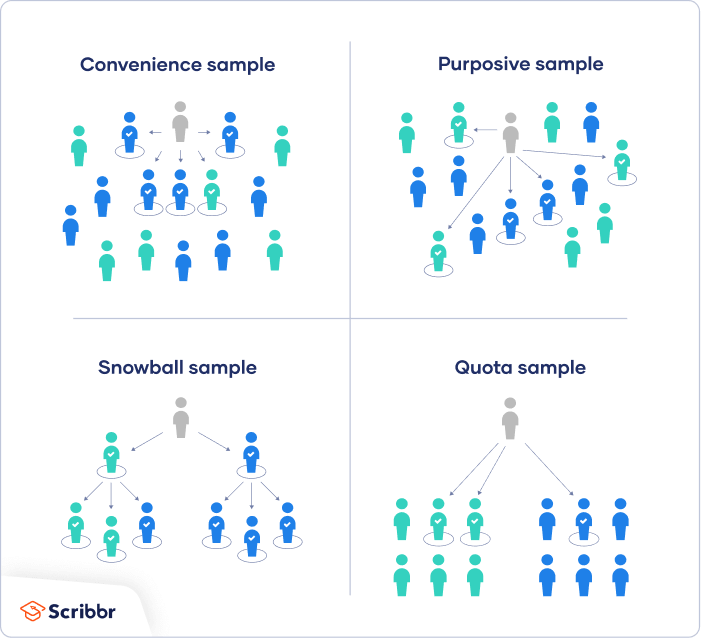
1. Convenience sampling
A convenience sample simply includes the individuals who happen to be most accessible to the researcher.
This is an easy and inexpensive way to gather initial data, but there is no way to tell if the sample is representative of the population, so it can’t produce generalisable results.
You are researching opinions about student support services in your university, so after each of your classes, you ask your fellow students to complete a survey on the topic. This is a convenient way to gather data, but as you only surveyed students taking the same classes as you at the same level, the sample is not representative of all the students at your university.
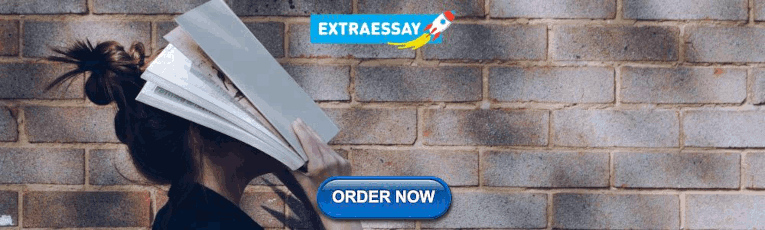
2. Voluntary response sampling
Similar to a convenience sample, a voluntary response sample is mainly based on ease of access. Instead of the researcher choosing participants and directly contacting them, people volunteer themselves (e.g., by responding to a public online survey).
Voluntary response samples are always at least somewhat biased, as some people will inherently be more likely to volunteer than others.
You send out the survey to all students at your university and many students decide to complete it. This can certainly give you some insight into the topic, but the people who responded are more likely to be those who have strong opinions about the student support services, so you can’t be sure that their opinions are representative of all students.
3. Purposive sampling
Purposive sampling , also known as judgement sampling, involves the researcher using their expertise to select a sample that is most useful to the purposes of the research.
It is often used in qualitative research , where the researcher wants to gain detailed knowledge about a specific phenomenon rather than make statistical inferences, or where the population is very small and specific. An effective purposive sample must have clear criteria and rationale for inclusion.
You want to know more about the opinions and experiences of students with a disability at your university, so you purposely select a number of students with different support needs in order to gather a varied range of data on their experiences with student services.
4. Snowball sampling
If the population is hard to access, snowball sampling can be used to recruit participants via other participants. The number of people you have access to ‘snowballs’ as you get in contact with more people.
You are researching experiences of homelessness in your city. Since there is no list of all homeless people in the city, probability sampling isn’t possible. You meet one person who agrees to participate in the research, and she puts you in contact with other homeless people she knows in the area.
A sample is a subset of individuals from a larger population. Sampling means selecting the group that you will actually collect data from in your research.
For example, if you are researching the opinions of students in your university, you could survey a sample of 100 students.
Statistical sampling allows you to test a hypothesis about the characteristics of a population. There are various sampling methods you can use to ensure that your sample is representative of the population as a whole.
Samples are used to make inferences about populations . Samples are easier to collect data from because they are practical, cost-effective, convenient, and manageable.
Probability sampling means that every member of the target population has a known chance of being included in the sample.
Probability sampling methods include simple random sampling , systematic sampling , stratified sampling , and cluster sampling .
In non-probability sampling , the sample is selected based on non-random criteria, and not every member of the population has a chance of being included.
Common non-probability sampling methods include convenience sampling , voluntary response sampling, purposive sampling , snowball sampling , and quota sampling .
Sampling bias occurs when some members of a population are systematically more likely to be selected in a sample than others.
Cite this Scribbr article
If you want to cite this source, you can copy and paste the citation or click the ‘Cite this Scribbr article’ button to automatically add the citation to our free Reference Generator.
McCombes, S. (2022, October 10). Sampling Methods | Types, Techniques, & Examples. Scribbr. Retrieved 24 June 2024, from https://www.scribbr.co.uk/research-methods/sampling/
Is this article helpful?
Shona McCombes
Other students also liked, what is quantitative research | definition & methods, a quick guide to experimental design | 5 steps & examples, controlled experiments | methods & examples of control.
- Skip to main content
- Skip to primary sidebar
- Skip to footer
- QuestionPro

- Solutions Industries Gaming Automotive Sports and events Education Government Travel & Hospitality Financial Services Healthcare Cannabis Technology Use Case NPS+ Communities Audience Contactless surveys Mobile LivePolls Member Experience GDPR Positive People Science 360 Feedback Surveys
- Resources Blog eBooks Survey Templates Case Studies Training Help center

Home Market Research
Sampling Methods: Guide To All Types with Examples
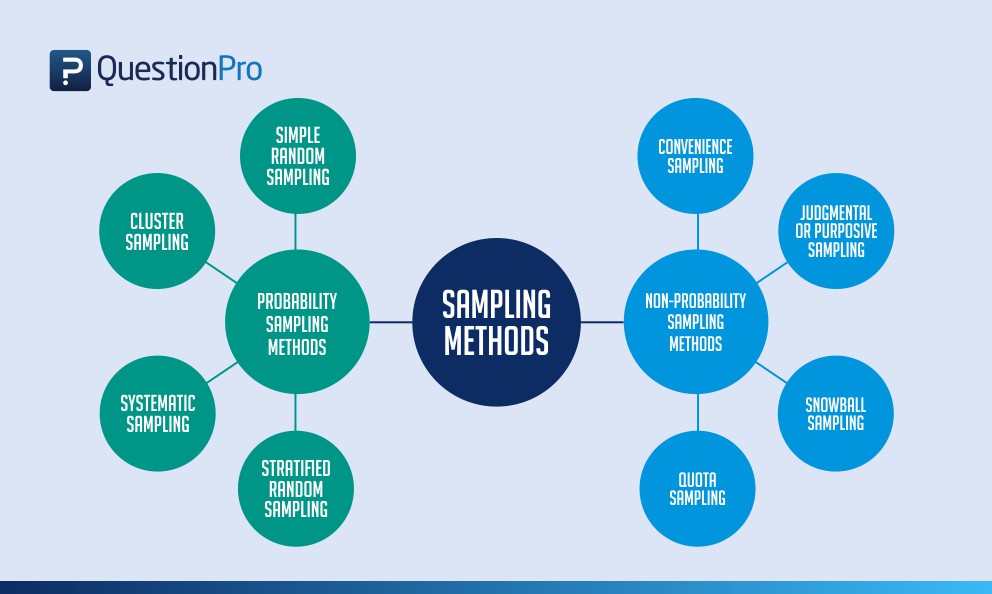
Sampling is an essential part of any research project. The right sampling method can make or break the validity of your research, and it’s essential to choose the right method for your specific question. In this article, we’ll take a closer look at some of the most popular sampling methods and provide real-world examples of how they can be used to gather accurate and reliable data.
LEARN ABOUT: Research Process Steps
From simple random sampling to complex stratified sampling , we’ll explore each method’s pros, cons, and best practices. So, whether you’re a seasoned researcher or just starting your journey, this article is a must-read for anyone looking to master sampling methods. Let’s get started!
Content Index
What is sampling?
Types of sampling: sampling methods, types of probability sampling with examples:, uses of probability sampling, types of non-probability sampling with examples, uses of non-probability sampling, how do you decide on the type of sampling to use, difference between probability sampling and non-probability sampling methods.
Sampling is a technique of selecting individual members or a subset of the population to make statistical inferences from them and estimate the characteristics of the whole population. Different sampling methods are widely used by researchers in market research so that they do not need to research the entire population to collect actionable insights.
It is also a time-convenient and cost-effective method and hence forms the basis of any research design . Sampling techniques can be used in research survey software for optimum derivation.
For example, suppose a drug manufacturer would like to research the adverse side effects of a drug on the country’s population. In that case, it is almost impossible to conduct a research study that involves everyone. In this case, the researcher decides on a sample of people from each demographic and then researches them, giving him/her indicative feedback on the drug’s behavior.
Learn more about Audience by QuestionPro
Sampling in market action research is of two types – probability sampling and non-probability sampling. Let’s take a closer look at these two methods of sampling.
- Probability sampling: Probability sampling is a sampling technique where a researcher selects a few criteria and chooses members of a population randomly. All the members have an equal opportunity to participate in the sample with this selection parameter.
- Non-probability sampling: In non-probability sampling, the researcher randomly chooses members for research. This sampling method is not a fixed or predefined selection process. This makes it difficult for all population elements to have equal opportunities to be included in a sample.
This blog discusses the various probability and non-probability sampling methods you can implement in any market research study.
LEARN ABOUT: Survey Sampling
Probability sampling is a technique in which researchers choose samples from a larger population based on the theory of probability. This sampling method considers every member of the population and forms samples based on a fixed process.
For example, in a population of 1000 members, every member will have a 1/1000 chance of being selected to be a part of a sample. Probability sampling eliminates sampling bias in the population and allows all members to be included in the sample.
There are four types of probability sampling techniques:
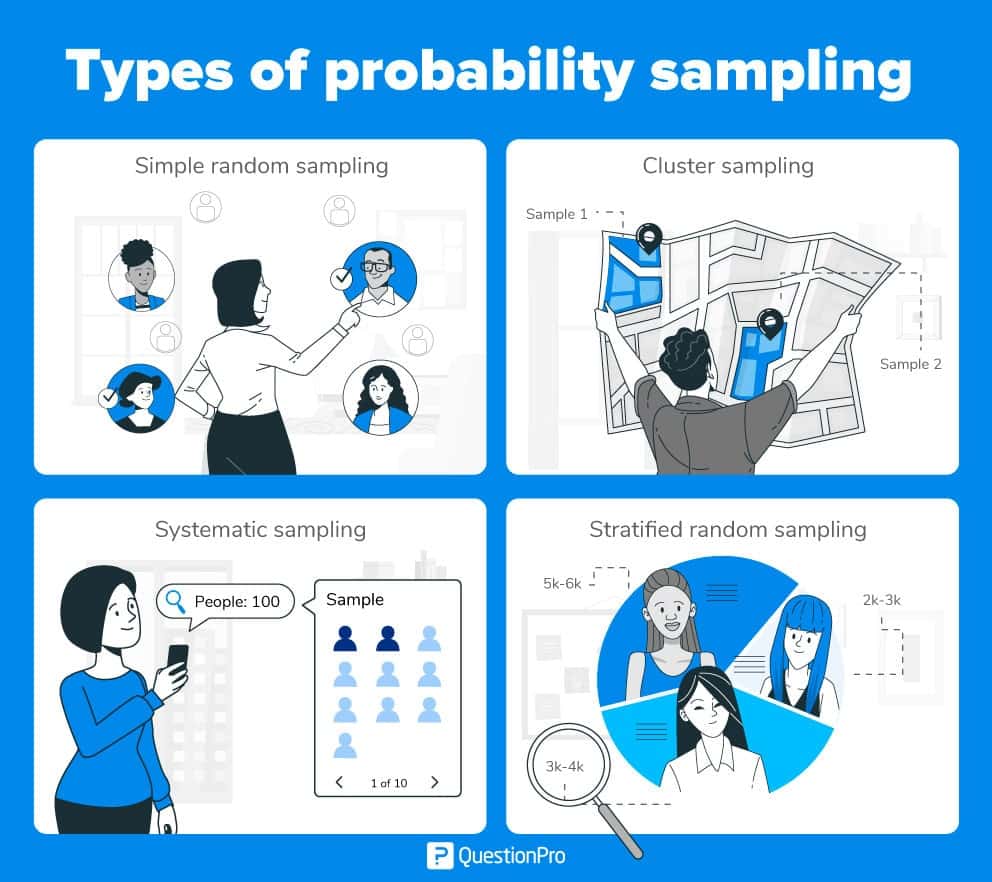
- Simple random sampling: One of the best probability sampling techniques that helps in saving time and resources is the Simple Random Sampling method. It is a reliable method of obtaining information where every single member of a population is chosen randomly, merely by chance. Each individual has the same probability of being chosen to be a part of a sample. For example, in an organization of 500 employees, if the HR team decides on conducting team-building activities, they would likely prefer picking chits out of a bowl. In this case, each of the 500 employees has an equal opportunity of being selected.
- Cluster sampling: Cluster sampling is a method where the researchers divide the entire population into sections or clusters representing a population. Clusters are identified and included in a sample based on demographic parameters like age, sex, location, etc. This makes it very simple for a survey creator to derive effective inferences from the feedback. For example, suppose the United States government wishes to evaluate the number of immigrants living in the Mainland US. In that case, they can divide it into clusters based on states such as California, Texas, Florida, Massachusetts, Colorado, Hawaii, etc. This way of conducting a survey will be more effective as the results will be organized into states and provide insightful immigration data.
- Systematic sampling: Researchers use the systematic sampling method to choose the sample members of a population at regular intervals. It requires selecting a starting point for the sample and sample size determination that can be repeated at regular intervals. This type of sampling method has a predefined range; hence, this sampling technique is the least time-consuming. For example, a researcher intends to collect a systematic sample of 500 people in a population of 5000. He/she numbers each element of the population from 1-5000 and will choose every 10th individual to be a part of the sample (Total population/ Sample Size = 5000/500 = 10).
- Stratified random sampling: Stratified random sampling is a method in which the researcher divides the population into smaller groups that don’t overlap but represent the entire population. While sampling, these groups can be organized, and then draw a sample from each group separately. For example, a researcher looking to analyze the characteristics of people belonging to different annual income divisions will create strata (groups) according to the annual family income. Eg – less than $20,000, $21,000 – $30,000, $31,000 to $40,000, $41,000 to $50,000, etc. By doing this, the researcher concludes the characteristics of people belonging to different income groups. Marketers can analyze which income groups to target and which ones to eliminate to create a roadmap that would bear fruitful results.
LEARN ABOUT: Purposive Sampling
There are multiple uses of probability sampling:
- Reduce Sample Bias: Using the probability sampling method, the research bias in the sample derived from a population is negligible to non-existent. The sample selection mainly depicts the researcher’s understanding and inference. Probability sampling leads to higher-quality data collection as the sample appropriately represents the population.
- Diverse Population: When the population is vast and diverse, it is essential to have adequate representation so that the data is not skewed toward one demographic . For example, suppose Square would like to understand the people that could make their point-of-sale devices. In that case, a survey conducted from a sample of people across the US from different industries and socio-economic backgrounds helps.
- Create an Accurate Sample: Probability sampling helps the researchers plan and create an accurate sample. This helps to obtain well-defined data.
The non-probability method is a sampling method that involves a collection of feedback based on a researcher or statistician’s sample selection capabilities and not on a fixed selection process. In most situations, the output of a survey conducted with a non-probable sample leads to skewed results, which may not represent the desired target population. But, there are situations, such as the preliminary stages of research or cost constraints for conducting research, where non-probability sampling will be much more useful than the other type.
Four types of non-probability sampling explain the purpose of this sampling method in a better manner:
- Convenience sampling: This method depends on the ease of access to subjects such as surveying customers at a mall or passers-by on a busy street. It is usually termed as convenience sampling because of the researcher’s ease of carrying it out and getting in touch with the subjects. Researchers have nearly no authority to select the sample elements, and it’s purely done based on proximity and not representativeness. This non-probability sampling method is used when there are time and cost limitations in collecting feedback. In situations with resource limitations, such as the initial stages of research, convenience sampling is used. For example, startups and NGOs usually conduct convenience sampling at a mall to distribute leaflets of upcoming events or promotion of a cause – they do that by standing at the mall entrance and giving out pamphlets randomly.
- Judgmental or purposive sampling: Judgmental or purposive samples are formed at the researcher’s discretion. Researchers purely consider the purpose of the study, along with the understanding of the target audience. For instance, when researchers want to understand the thought process of people interested in studying for their master’s degree. The selection criteria will be: “Are you interested in doing your masters in …?” and those who respond with a “No” are excluded from the sample.
- Snowball sampling: Snowball sampling is a sampling method that researchers apply when the subjects are difficult to trace. For example, surveying shelterless people or illegal immigrants will be extremely challenging. In such cases, using the snowball theory, researchers can track a few categories to interview and derive results. Researchers also implement this sampling method when the topic is highly sensitive and not openly discussed—for example, surveys to gather information about HIV Aids. Not many victims will readily respond to the questions. Still, researchers can contact people they might know or volunteers associated with the cause to get in touch with the victims and collect information.
- Quota sampling: In Quota sampling , members in this sampling technique selection happens based on a pre-set standard. In this case, as a sample is formed based on specific attributes, the created sample will have the same qualities found in the total population. It is a rapid method of collecting samples.
Non-probability sampling is used for the following:
- Create a hypothesis: Researchers use the non-probability sampling method to create an assumption when limited to no prior information is available. This method helps with the immediate return of data and builds a base for further research.
- Exploratory research: Researchers use this sampling technique widely when conducting qualitative research, pilot studies, or exploratory research .
- Budget and time constraints: The non-probability method when there are budget and time constraints, and some preliminary data must be collected. Since the survey design is not rigid, it is easier to pick respondents randomly and have them take the survey or questionnaire .
For any research, it is essential to choose a sampling method accurately to meet the goals of your study. The effectiveness of your sampling relies on various factors. Here are some steps expert researchers follow to decide the best sampling method.
- Jot down the research goals. Generally, it must be a combination of cost, precision, or accuracy.
- Identify the effective sampling techniques that might potentially achieve the research goals.
- Test each of these methods and examine whether they help achieve your goal.
- Select the method that works best for the research.
Unlock the power of accurate sampling!
We have looked at the different types of sampling methods above and their subtypes. To encapsulate the whole discussion, though, the significant differences between probability sampling methods and non-probability sampling methods are as below:
Probability Sampling is a sampling technique in which samples from a larger population are chosen using a method based on the theory of probability. | Non-probability sampling is a sampling technique in which the researcher selects samples based on the researcher’s subjective judgment rather than random selection. | |
Random sampling method. | Non-random sampling method | |
The population is selected randomly. | The population is selected arbitrarily. | |
The research is conclusive. | The research is exploratory. | |
Since there is a method for deciding the sample, the population demographics are conclusively represented. | Since the sampling method is arbitrary, the population demographics representation is almost always skewed. | |
Takes longer to conduct since the research design defines the selection parameters before the market research study begins. | This type of sampling method is quick since neither the sample nor the selection criteria of the sample are undefined. | |
This type of sampling is entirely unbiased; hence, the results are also conclusive. | This type of sampling is entirely biased, and hence the results are biased, too, rendering the research speculative. | |
In probability sampling, there is an underlying hypothesis before the study begins, and this method aims to prove the hypothesis. | In non-probability sampling, the hypothesis is derived after conducting the research study. |
Now that we have learned how different sampling methods work and are widely used by researchers in market research so that they don’t need to research the entire population to collect actionable insights, let’s go over a tool that can help you manage these insights.
LEARN ABOUT: 12 Best Tools for Researchers
QuestionPro understands the need for an accurate, timely, and cost-effective method to select the proper sample; that’s why we bring QuestionPro Software, a set of tools that allow you to efficiently select your target audience , manage your insights in an organized, customizable repository and community management for post-survey feedback.
Don’t miss the chance to elevate the value of research.
FREE TRIAL RICHIEDI DEMO
MORE LIKE THIS
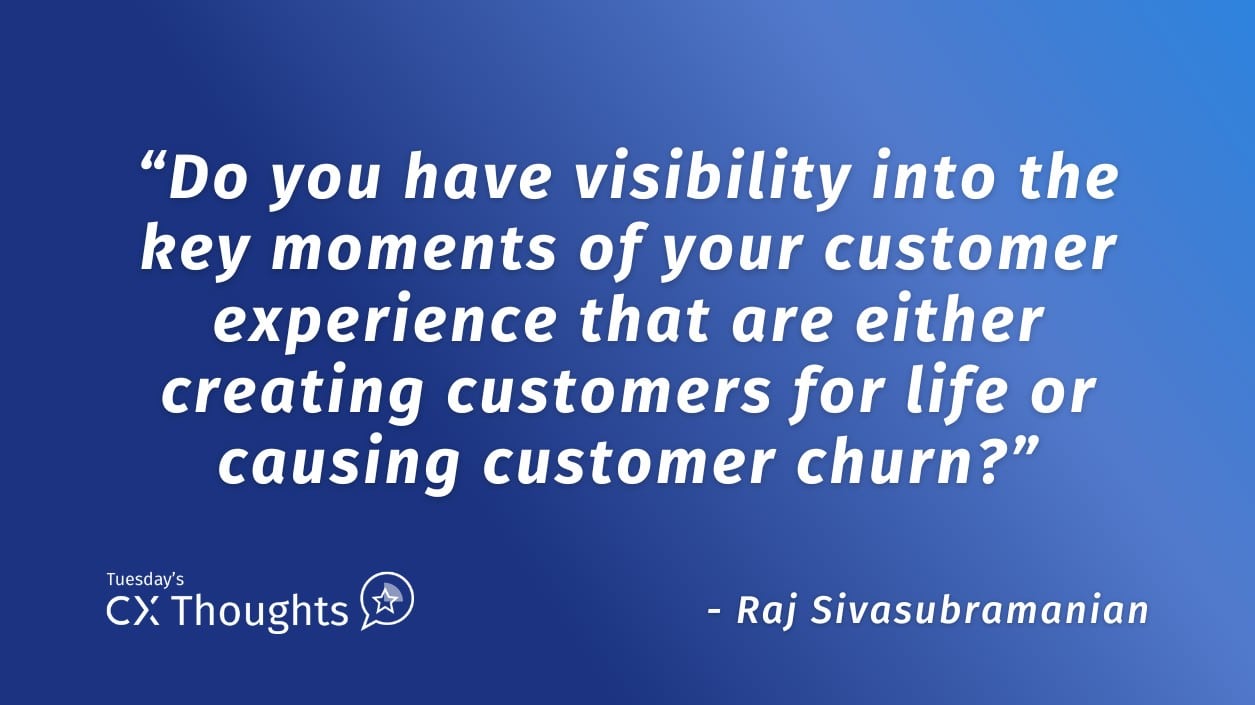
The Item I Failed to Leave Behind — Tuesday CX Thoughts
Jun 25, 2024
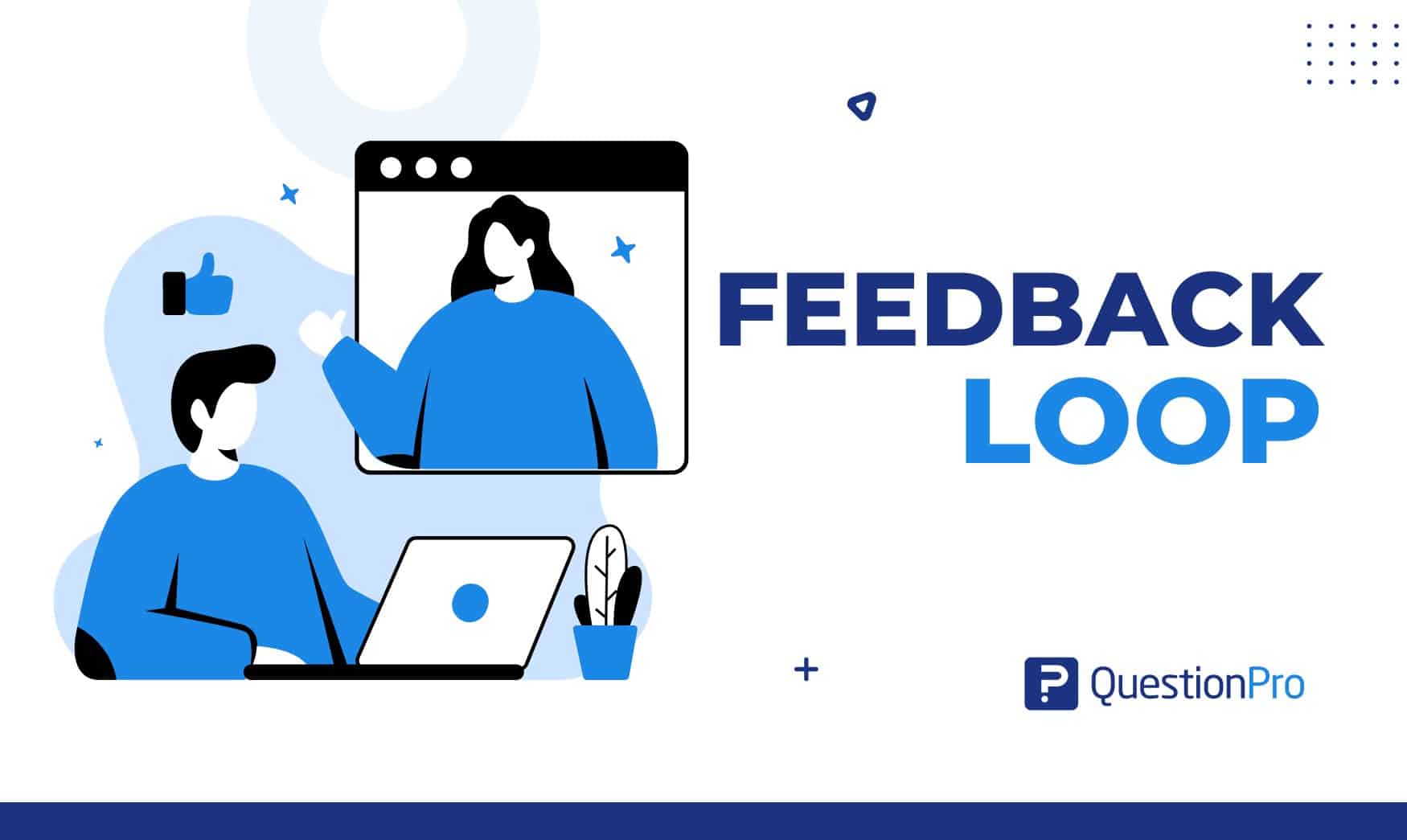
Feedback Loop: What It Is, Types & How It Works?
Jun 21, 2024
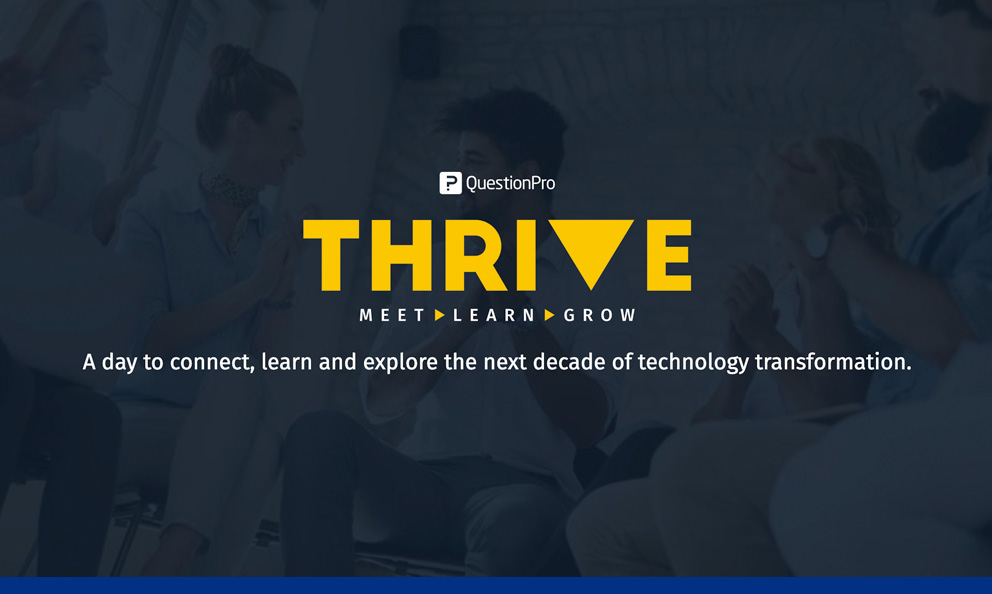
QuestionPro Thrive: A Space to Visualize & Share the Future of Technology
Jun 18, 2024
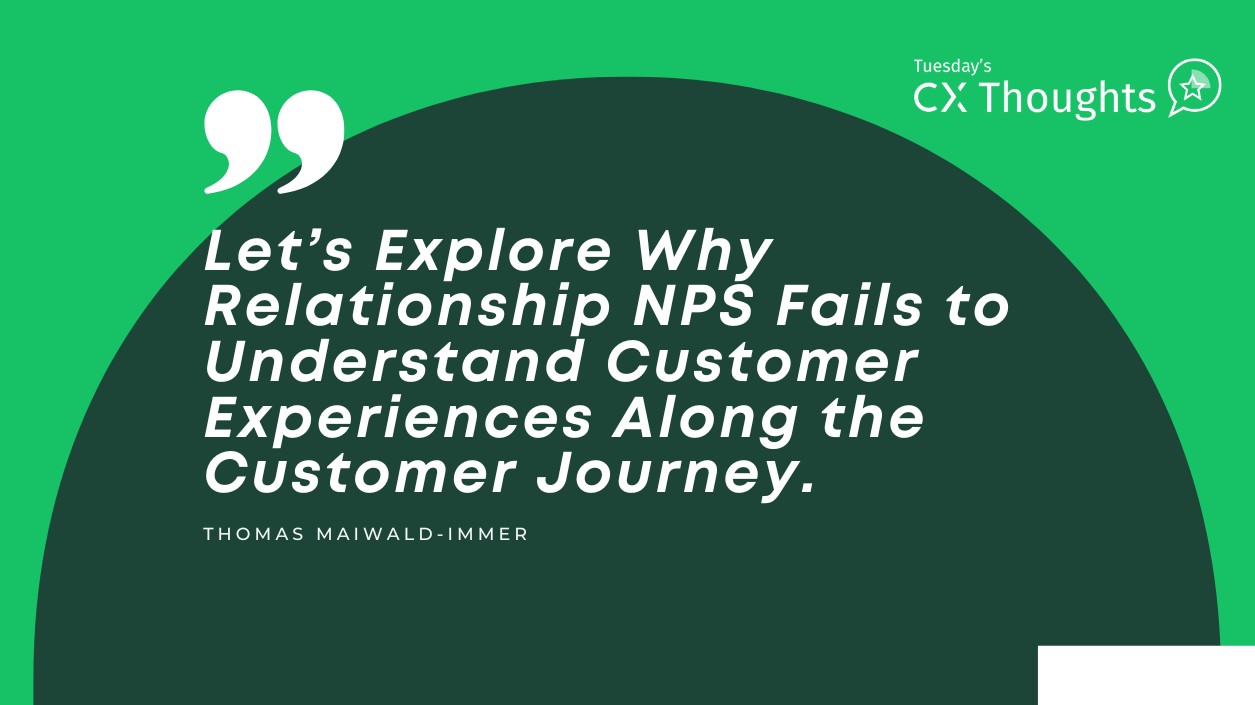
Relationship NPS Fails to Understand Customer Experiences — Tuesday CX
Other categories.
- Academic Research
- Artificial Intelligence
- Assessments
- Brand Awareness
- Case Studies
- Communities
- Consumer Insights
- Customer effort score
- Customer Engagement
- Customer Experience
- Customer Loyalty
- Customer Research
- Customer Satisfaction
- Employee Benefits
- Employee Engagement
- Employee Retention
- Friday Five
- General Data Protection Regulation
- Insights Hub
- Life@QuestionPro
- Market Research
- Mobile diaries
- Mobile Surveys
- New Features
- Online Communities
- Question Types
- Questionnaire
- QuestionPro Products
- Release Notes
- Research Tools and Apps
- Revenue at Risk
- Survey Templates
- Training Tips
- Tuesday CX Thoughts (TCXT)
- Uncategorized
- Video Learning Series
- What’s Coming Up
- Workforce Intelligence
- Scroll to top
- Light Dark Light Dark
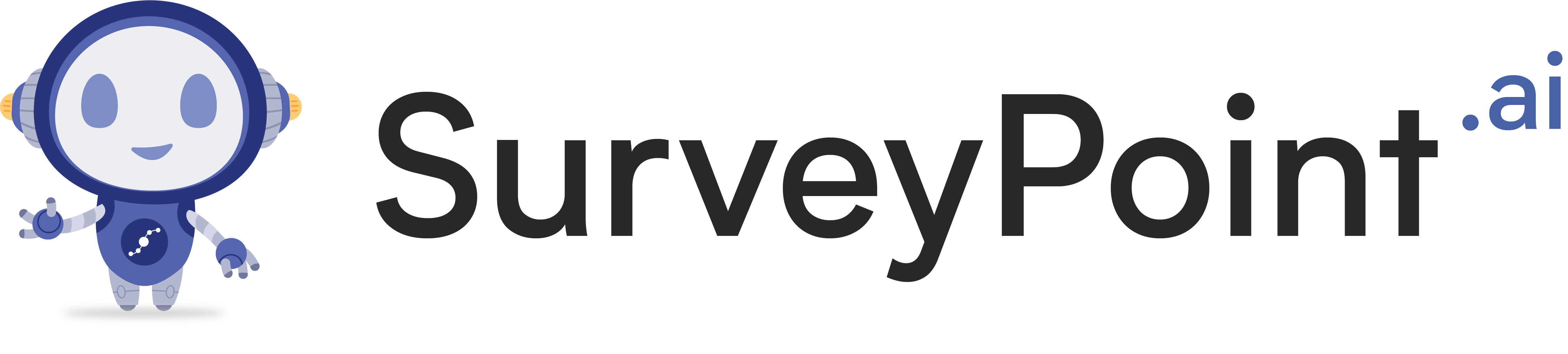
Cart review
No products in the cart.
7 Powerful Steps in Sampling Design for Effective Research
- Author Survey Point Team
- Published January 3, 2024
Unlock the secrets of effective research with these 7 powerful steps in sampling design. Elevate your research game and ensure precision from the outset. Dive into a world of insights and methodology that guarantees meaningful results.
Research forms the foundation of knowledge and understanding in any field. The quality and validity of research depend largely on the sampling design used. An effective sampling design ensures unbiased and reliable results that can be generalized to the entire population. In this article, we will explore seven powerful steps in sampling design that researchers can follow to conduct effective research.
Table of Contents
1. Define the Research Objectives
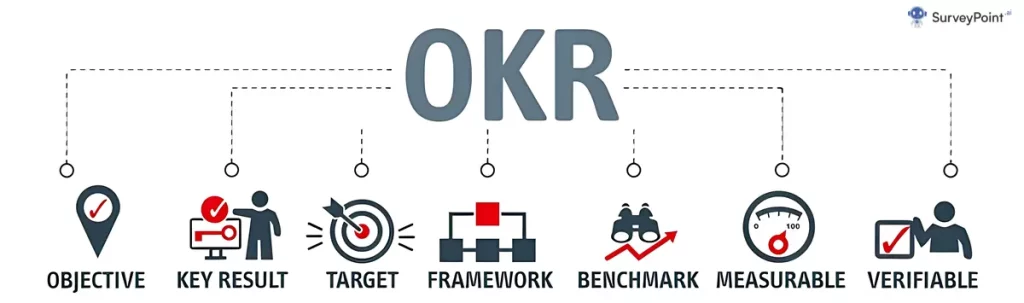
Before diving into the sampling design process, it is vital to define the research objectives. Clearly determining what you aim to achieve through the research will guide the entire sampling design. Whether it is to study consumer behavior, analyze market trends, or explore the impact of a specific intervention, outlining the research objectives provides a clear roadmap for sampling.
Example: Without a clear research objective, sampling becomes directionless, leading to inaccurate results that do not contribute to meaningful insights.
2. Identify the Target Population
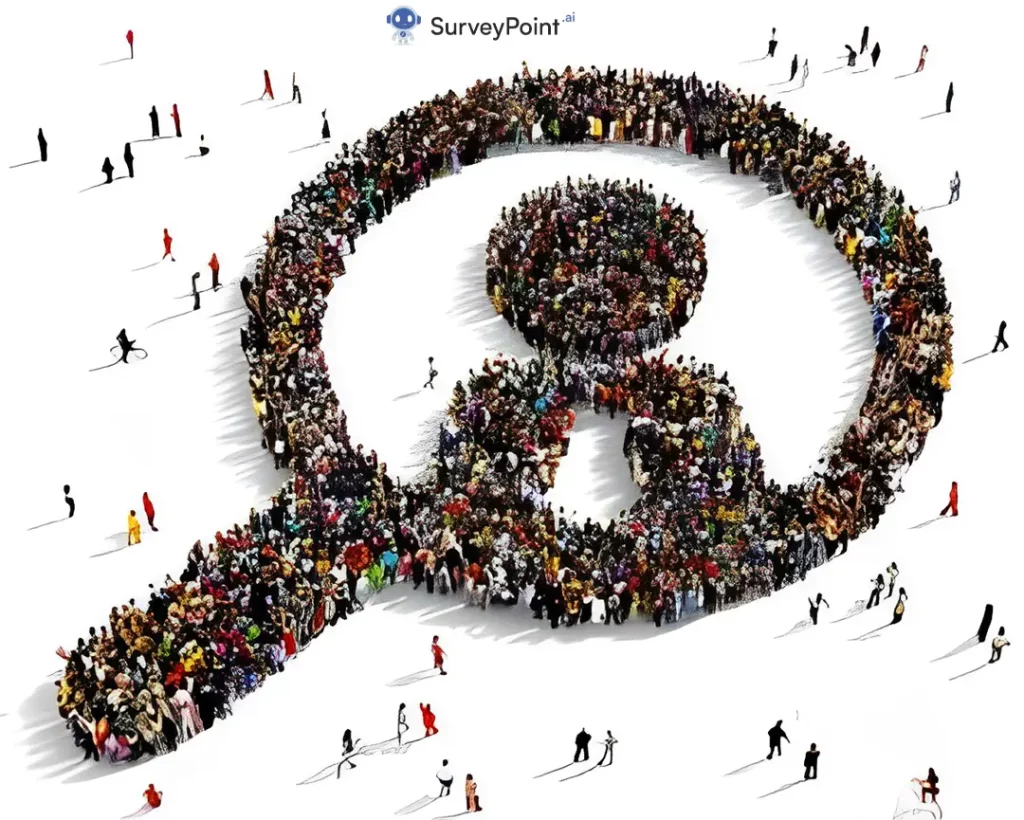
After defining the research objectives, identifying the target population is the next crucial step. The target population represents the group of individuals or elements that the research aims to generalize the findings to. It is essential to clearly define and understand the demographics, characteristics, and parameters of the target population before moving forward with sampling.
Example: Identifying the target population allows researchers to ensure that the sampled individuals represent the broader group accurately, increasing the external validity of the study.
3. Determine the Sample Size
Determining the appropriate sample size is a critical factor in sampling design. A sample size that is too small may not accurately represent the target population, while a sample size that is too large may result in unnecessary costs and resources. Determining the sample size requires considering various factors, such as desired level of precision, variability within the population, and available resources.
Example: The sample size should strike a balance between statistical reliability and practical feasibility. A larger sample size increases the precision of the estimates, while a smaller sample size may result in wider confidence intervals.
4. Select the Sampling Technique
Various sampling techniques exist, each catering to different research scenarios. The choice of sampling technique depends on the nature of the research, available resources, and the level of precision required. Common sampling techniques include simple random sampling, stratified sampling, cluster sampling, and systematic sampling.
Example: Understanding the different sampling techniques allows researchers to choose the most appropriate method for their specific research, ensuring representative and reliable results.
5. Implement the Sampling Strategy
Once the sampling technique is selected, it is time to implement the sampling strategy. This involves identifying the potential sampling units and selecting the actual sample elements from the target population. Researchers must avoid any biases and ensure randomness in the selection process to maintain the integrity of the research findings.
Example: Implementing the sampling strategy meticulously enables researchers to minimize potential biases and increase the chances of obtaining accurate results that can be generalized to the larger population.
6. Collect Data from the Sample: Steps in Sampling Design
With the sample selected, data collection becomes the next crucial step. Researchers can use various methods, such as surveys, interviews, observations, or experiments, to collect the necessary data. It is essential to follow the research design and consider data quality measures to ensure the reliability and validity of the collected information.
Example: Collecting data from the sample involves establishing effective communication channels, designing appropriate data collection instruments, and capturing the information accurately to minimize measurement errors.
7. Analyze and Interpret the Findings
Once the data is collected, it is time to analyze and interpret the findings. This involves applying statistical techniques, conducting hypothesis testing, and drawing meaningful conclusions. Researchers should ensure they have the necessary analytical skills or collaborate with experts in data analysis to derive accurate and insightful results.
Example: Analyzing and interpreting the findings allows researchers to draw meaningful conclusions and make informed decisions based on the evidence obtained through the research process.
Top 10 Sampling Techniques along with their respective Pros and Cons :
Simple Random Sampling | – Easy to implement | – May not represent specific subgroups adequately |
Stratified Sampling | – Ensures representation of subgroups | – Requires accurate classification of units |
Systematic Sampling | – Simple and easy to implement | – Susceptible to periodic patterns in the data |
Cluster Sampling | – Reduces costs and resources | – Potential for increased sampling error |
Convenience Sampling | – Quick and cost-effective | – Lack of representativeness |
Snowball Sampling | – Useful for hard-to-reach populations | – Potential for bias due to network connections |
Purposive Sampling | – Allows for targeted inclusion of specific cases | – Limited generalizability |
Quota Sampling | – Ensures representation of specific characteristics | – Potential for bias if quotas are not accurately defined |
Multi-Stage Sampling | – Allows for more complex and detailed studies | – Increased complexity and potential for errors |
Time-Location Sampling | – Useful for studying behaviors at specific times and locations | – Limited generalizability outside specified times and locations |
This table provides a quick overview of the strengths and weaknesses of each sampling technique, aiding researchers in selecting the most appropriate method for their specific research objectives.
Frequently Asked Questions (FAQs)
Q: Why is defining research objectives the first step in sampling design?
A: Defining research objectives sets a clear direction for the study, ensuring focus and purpose in the subsequent steps.
Q: How does the selection of a sampling frame impact research outcomes?
A: The sampling frame defines the accessible population, influencing the generalizability of results to the broader context.
Q: What factors influence the choice of a sampling technique?
A: Research objectives and the nature of the study guide the choice of a sampling technique, ensuring alignment with the research goals.
Q: Why is determining the sample size crucial in sampling design?
A: The sample size strikes a delicate balance, ensuring accuracy in representation while maintaining manageability.
Q: How do data collection methods align with the chosen sampling design?
A: The sampling design informs the selection of data collection methods, ensuring synergy for a comprehensive research approach.
Q: Why is analysis and interpretation the culmination of the sampling design process?
A: Analysis and interpretation transform raw data into actionable knowledge, realizing the objectives set at the beginning of the research journey.
Sampling design plays a fundamental role in conducting effective research. By following the seven powerful steps outlined in this article – defining research objectives, identifying the target population, determining the sample size, selecting the sampling technique, implementing the sampling strategy, collecting data from the sample, and analyzing and interpreting the findings – researchers can ensure reliable, valid, and generalizable results. Adopting a systematic and rigorous approach to sampling design will ultimately enhance the impact of research across various fields.
Remember, a solid sampling design empowers researchers to capture the essence of a larger population, revealing valuable insights that drive progress and innovation.
Survey Point Team
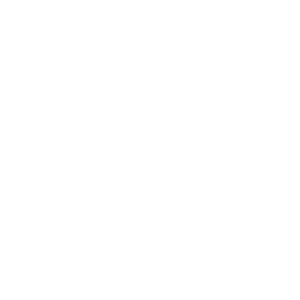

Maximise Research Impact with Diverse Sampling Strategies: How and Why to Increase Diversity in Samples

Dr Yuzana Khine Zaw
What is sampling in research.
Sampling is one of the first stages of a research study. It means deciding the group you will collect data from in your research. Sampling is used to study a smaller portion of the population to make generalisable claims about the larger population. 1 The extent to which the claims are generalisable or “valid” depends on having a good sampling strategy. The specific sampling strategies varies, depending on the aims and type of research (quantitative, qualitative).
Crafting a winning sampling strategy
When designing a sampling strategy, researchers already have a research question in mind and a plan of how the study should be executed. The goal may be to explore a phenomenon (inductive) or to test an existing theory (deductive). This plan may include quantitative or qualitative research methods.
The sampling strategy will be informed by these aims and objectives. Quantitative studies tend to prioritise having a large sample size or use a “random sampling” method to ensure validity and generalisability. 1 Qualitative research tends to have smaller samples and use a more “purposive,” non-probabilistic (not random) approach. This involves selecting specific participants who can provide rich information to address the aims and objectives of the research. 1
Importance and the benefits of encouraging diversity in sampling
Regardless of which sampling method is used, a good sampling strategy encourages diversity to ensure research findings are valid, reliable, and generalisable. For example, a clinical trial to test a drug intended to be used on the general population would need to include a very large and diverse sample to account for all demographics. As certain subgroups of patients may respond differently to interventions, a non-diversified sample could mean that the results of the trial are not valid (drug is not safe/not effective) for the entire population.
Diversity is also important for smaller qualitative studies. “Maximum variation” sampling in qualitative research aims to recruit participants who are widely different from each other to obtain as many diverse perspectives as possible. 1 While diversity in sampling within quantitative studies helps ensure validity and generalisability, diversity in qualitative studies supports researchers to capture a wide range of different perspectives, creating more inclusive and representative research findings. This is particularly important for minimising inequalities in healthcare research, as certain hard-to-reach or underprivileged populations often miss out on opportunities resulting from research outcomes.
Maximising Positive Impact
Beyond conducting “good” research (research that is valid, generalisable, and reliable), a key goal is to produce as much of a positive impact on the world as possible. Ensuring diversity in sampling helps researchers reach and take into consideration the voices of those who are normally marginalised and underprivileged. By actively encouraging a diverse sample that includes minority voices, researchers can produce a better impact on society through equitable, inclusive, and representative research.
Given, L. M. (Ed.). (2008). The Sage encyclopedia of qualitative research methods . Sage publications.
What are your current challenges.
Privacy Policy

Kelsie Brewer
My expertise.
I’m a qualitative researcher with expertise in patient-centred outcomes.
My Experience
With a background in qualitative research, I have been working in program evaluation and health outcomes research since I graduated with a Master of Education from Vanderbilt University in 2015. Before working in clinical outcome assessment (COA) research, my focus was on evaluating pediatric community health programs using mixed methods research. I have experience developing surveys, moderating focus groups, and interviewing pediatric and adult participants.
For the past three years, I have more directly worked in the development, validation, and modification of COAs through qualitative research. My experience includes working across a wide range of therapeutic areas including oncology, neurology, rheumatology, and rare disease.
My guiding principles
I am passionate about ensuring patient voice is included in a meaningful way to impact drug development and overall health outcomes research.

Richard Simpson
I am an experienced Finance Director who has spent more than 30 years in senior financial positions and a specific interest in driving growth in small, owner-managed businesses.
Since qualifying as an accountant in 1990, I have worked senior finance and general management positions in the UK, mainland Europe, North America and South East Asia. My sector experience includes pharmaceuticals, advertising and marketing, professional services, broadcasting, food and technology.
For the past twenty years I have worked with a portfolio of smaller, usually owner-managed businesses helping them to achieve medium-term growth plans and enable their organisations to adapt to changing external environments.
I am passionate about providing owner-managers with the information and understanding they need to make their businesses succeed.

My background includes high-level administrative support and working with cross-functional teams in the healthcare and banking industries.
My experience
With nine years of executive administration and management support experience in the healthcare and banking industries, I am eager to bring my skills and passion to the Sprout team. I enjoy working in a collaborative environment where growth and exploration is actively encouraged.
I look forward to not only growing professionally, but also contributing to Sprout’s positive impact on patients’ lives.
Strive to be helpful and resourceful in any situation. Be open-minded and stay hungry.

Beverly Romero
I’m a qualitative resear cher with expertise in patient – centered outcomes and a focus in pediatrics and rare disease.
With an expertise in qualitative methods, I have been working in the field of patient – centered outcomes in a consulting environment since 2010. During this time, I have had the opportunity to hone my skills in the development and validation of clinical outcome assessments (COAs) and consulting on the selection of COA endpoints for clinical trials. I have conducted hundreds of qualitative interviews with clinicians, caregivers, and patients of all ages.
My experience includes a wide variety of therapeutic areas, including gastroenterology, CNS disorders, rare genetic disorders, immunology, and dermatology. I am particularly passionate about research in the area of rare disease and pediatrics.
My prior work experience includes over 5 years in the communication s field and 15+ years as a university – level writing instructor.

Justina Tol
My background includes maintaining strong relationships with clients, project management and coordination, financial tracking and reporting, and risk management.
My experience is in various industries and working environments where I aimed for clients’ maximum satisfaction, thereby strengthening customer loyalty. While always aiming to create value, I have experience in taking accountability for a portfolio of clients that need regular management to support operationally and strategically. I enjoy having the responsibility of the full-service overseeing while also initiating and driving improvements to strategies and tactical plans resulting in enhanced efficiency and quality.
My focus is representing the stakeholders’ needs and goals to the various cross-functional teams to ensure quality service, resulting in client satisfaction and operational excellence, and encouraging new and repeat business opportunities.

Rachel Cotter
I am an Office Manager with a background in Bookkeeping and ISO Management.
Over the last thirteen years I have worked within the Information Technology industry which has given me great technical knowledge paired with finance experience. I started out as an Office Assistant but having gained exposure quite quickly to all aspects of the business I decided to focus on getting a bookkeeping certification. That soon developed into an Accounts Assistant role and then swiftly onto Office Management. During that time, I played a key part in obtaining ISO accreditation for the company and maintaining the QMS, ISMS and Environmental system, alongside the day to day tasks of Office Management and Accounts function.
I am very much looking forward to furthering my career and knowledge with Sprout.
I am passionate about finding better ways of working, utilising systems to their fullest and improving procedures for everyone; in the interest of saving time and cost. I get great satisfaction from helping others and being a supportive member of any team.

Kara Giannone
Scientist with research expertise in qualitative research methodology, patient-reported outcomes, and digital health interventions.
I graduated with my MPH in Behavioral Science and Health Education from Emory University in 2019. After my graduate degree I worked for an academic institution both as a qualitative researcher and as a project manager in the digital health space. I have experience in translating public health and medical interventions to a digital platform while ensuring usability standards are maintained and user experience is maximized to meet study aims.
As a qualitative researcher I have experience working on projects in various areas including urology, oncology, immunology, psychiatry, hematology, gastroenterology, health policy and management, epidemiology, and health promotion.
I believe that any health-based organization or company that is aiming to positively impact quality of life needs to allow space for the patient voice. I am passionate about facilitating that exchange through qualitative research.

Yuzana Khine Zaw
I’m a medical anthropologist with qualitative research experience in clinical studies.
I have seven years of experience in academia, conducting qualitative social science research on antimicrobial resistance in Southeast Asia. I completed a PhD in Public Health and Policy at the London School of Hygiene and Tropical Medicine where I focused on exploring the social and political contexts of antimicrobial resistance and care in Myanmar through an ethnographic study.
Prior to this, I completed a master’s in International Health and Tropical Medicine at the University of Oxford where I examined the social role of diagnostic biomarker testing in antibiotic prescription. I also studied pre-medicine during my bachelor’s and spent four years doing lab-based research at Georgetown University’s Lombardi Comprehensive Cancer Center. There, I completed an undergraduate honors thesis on chemoprevention mechanisms. I am therefore, well-versed in health and medicine, across different disease and therapeutic areas including febrile illness, cancer, diabetes, malaria, and HIV.
Real-world solutions should be holistic and go beyond the technical. To achieve this, I believe in the importance of listening to and incorporating everyone’s voices, particularly those whose lives we are most impacting – the patients.

Anna de la Motte
I’m a qualitative scientist with research and consulting expertise in patient-centered outcomes and qualitative research methods.
I have over five years’ experience in health outcomes research, four of which were in a pediatric setting. I completed my MSEd degree at the University of Pennsylvania where I focused on the role of health in education. My master’s thesis examined the effect of a free play intervention on feelings of social connectivity and academic performance.
For the last five years, I’ve worked on the development of novel pediatric patient-reported outcome (PRO) measures and modification of existing measures for specific pediatric clinical populations. Most recently, I worked in the patient-centered research division of a large CRO, providing project management, research, and consulting services to multiple international pharmaceutical companies. I have experience conducting qualitative research with both pediatric and adult populations and have experience across a broad range of therapeutic areas, including oncology, nephrology, gastroenterology, and sleep medicine.
My passion as a COA researcher is working creatively to ensure that the voices and priorities of different patient populations are central in clinical decision-making and the development of new treatments.

Emily Whyte
I am a junior project manager providing support to senior project managers and specializing in pharma and wellness projects, behavior change initiatives and patient support programs.
I have worked in marketing and project management in a variety of roles including as a British Army Officer. With my immunology background, I have a deeper understanding of the relevancy of the project work we do at Sprout, and my army experience means I have the ability to work under pressure to meet important deadlines and budgets.
I am excited to play a role in delivering evidence-based programs which provide practical and effective solutions for patients and healthcare teams, ultimately improving the quality of healthcare given and received.

Anna Werchowiecki
I specialize in setting up and delivering pharma-led projects, behavior change initiatives and patient support programs.
I’ve worked in account management, project management and resource management. With over five years’ experience in the pharma industry, I’ve collaborated with many of the top pharmaceutical companies on a variety of projects. These range from multi-channel international patient support programs to discrete local projects to disease awareness initiatives.
Always be prepared – as well as on time and to budget!

Chloe Patel
I have recently completed my PhD at the University of Warwick that has assessed the role of maternal weight, experience and cognition, and media on the role of food-related parenting practices. Prior to undertaking a PhD, I worked for a global CRO on various patient centered outcomes projects.
I have over 10 years’ experience in health research. I have worked in academic and commercial settings with healthcare professionals, patients, and caregivers.
I am passionate about the impact that research has on the lives of patients, families, and wider healthcare setting; and I value turning research findings into meaningful actions.

Laura Meade
My PhD in health psychology had a focus on public health, and I’m also a certified health and wellness coach. I now specialize in improving patient adherence to treatment recommendations, including adherence to non-pharmacological treatments.
I’ve worked with patients in a variety of contexts. As a coach, I support patients on a one-to-one basis to implement behavior changes recommended by HCPs. I’ve worked in primary care, as well as in the academic, clinical and commercial worlds. I’ve developed community health initiatives to enhance understanding of how emotions impact health behavior, and the personal, social and environmental impacts on patient adherence. Using psychological theory and techniques, I developed and delivered interventions for patients with long-term conditions, with a focus on enhancing exercise adherence in patients with chronic pain.
I’m passionate about translating research into practice. I enjoy working with both patients and healthcare providers to explore the best ways to support patients adhere to treatment recommendations.

Christina Jackson
I’m a behavioral psychologist specializing in improving adherence to medication for long-term conditions, and changing healthcare professional (HCP) behavior to improve services.
I’ve been working in the adherence field for more than a decade. I completed my PhD in adherence interventions at UCL and then worked for a behavior change agency before setting up Sprout with Lina in 2017. I focus on global and local initiatives to support people with long-term conditions with adherence and other self-management issues, and on projects focused on changing HCP behavior to improve services. I’ve worked for nine of the top 15 pharmaceutical companies, on more than 20 patient support programs.
I love translating the latest findings from academic research into workable, real-world solutions that can help people with long-term conditions and their healthcare team. I’m particularly interested in digital approaches to behavior change and maintaining participant engagement through a program journey.

Alicia Hughes
I am a Health Psychologist with expertise in symptom experience and adjustment to long-term conditions. I have a special focus on supporting patients with persistent physical symptoms, specifically fatigue.
I have over 10 years’ experience in health research and development of patient behaviour change initiatives. I have worked in academia and industry, with health-care professionals and directly with patients. I completed my PhD at King’s College London assessing psychological correlates of fatigue and have continued to build on this work exploring fatigue in a range of patient populations. The evidence-based models arising from my research have informed the development of novel and targeted interventions to help people manage fatigue and its impact on patient’s quality of life.
I am passionate about the role of psychology in improving patients’ health-related quality of life.

Lina Eliasson
I specialize in understanding, measuring and improving treatment adherence and other behaviors that impact health. I also have experience in identification and validation of clinical outcomes assessments (COAs) and in patient preference studies.
My background spans both academic research and the commercial world. Following completion of my PhD on adherence to oral oncology drugs from UCL School of Pharmacy, I worked as a post-doc researcher at Imperial College London. I then joined a behavior change agency, where I oversaw the development, implementation and evaluation of patient and caregiver support programs delivered by multi-disciplinary healthcare teams, which I trained and supervised. Before founding Sprout with Dr Christina Jackson in 2017, I led the European COA team for one of the world’s largest clinical research organisations.
Throughout my career, I’ve collaborated with numerous leading pharmaceutical and biotech companies globally across a wide range of disease categories.
I value transparency in processes and communication, and having the freedom to recommend the best possible strategies for my clients.

Sarah Clifford
I’m a health psychologist with research and consulting expertise in patient-centered outcomes and treatment adherence.
I have over 20 years’ experience in health research, including over 10 years of consulting with the life sciences industry. For my PhD, I designed and conducted treatment adherence-related studies at the London/UCL School of Pharmacy – my research helped inform the foundation of the New Medicines Service, a community pharmacy-delivered service in England to support patients with their prescribed medication.
For the last 10 years, I’ve worked in clinical research organizations (CROs) in the US, providing research, consulting and strategy to a wide range of national and international pharmaceutical and medical device companies. Most recently I was the US West Coast Divisional Principal for a large CRO, leading a multi-disciplinary patient-centered outcomes team. I have experience across a broad range of therapeutic areas, including gastroenterology, respiratory health, rheumatology and immunology.
I’m driven by a desire to have a meaningful impact on the lives of people with long-term conditions.

Vanessa Cooper
I am a psychologist who specializes in understanding patients’ perceptions and experiences of illness, treatment and healthcare services, and developing interventions to address unmet need.
I have over 20 years’ experience working as a health psychology researcher in universities, the NHS and behavior change agencies. After completing my PhD on adherence to antiretroviral therapy for HIV, I was a senior researcher at UCL School of Pharmacy as part of a multidisciplinary team developing and evaluating theory-based interventions to enhance adherence by addressing perceptual and practical barriers to treatment.
I then worked at Brighton and Sussex University Hospitals NHS Trust where I designed and managed a program of research to develop HIV services to better meet the needs of an ageing population. Since 2015 I’ve worked as a consultant behavioral scientist, conducting primary and secondary research to understand people’s perceptions and experiences of illness, treatment and healthcare services and developing and evaluating healthcare interventions and patient support programs. I’ve worked across a wide range of disease areas and multimorbidity.
I am passionate about co-creation – working with stakeholders including patients, patient organizations, healthcare professionals and pharmaceutical industry – to inspire and develop innovative support programs and services that meet the needs and preferences of end-users.

Emma Lyon Carroll
I’m a senior project manager specializing in pharma, wellness, patient support and behavior change projects.
My background over the last nine years has been client service, account management and project management in medical communications, healthcare advertising and most recently patient behavior change and adherence support programs. I’ve worked on large and small, global and local projects for many of the top pharmaceutical companies in areas including cardiovascular, gastroenterology, dermatology, oncology and rare disease. I enjoy planning, organizing and bringing together a wide range of stakeholders to deliver evidence-based programs that provide valued support to patients.
I am passionate about supporting patient populations whilst providing exceptional client service, delivering projects on time and budget.

Roxana Bahar
I’m a medical sociologist with research and consulting expertise in patient-centered outcomes, shared decision-making and qualitative research methods.
I have over 15 years’ experience in health research, including 8 years consulting within the life sciences industry. For my PhD, I designed and implemented a multi-phase, qualitative research study to understand racial/ethnic disparities in Caesarean section rates, and to evaluate shared decision-making practices between clinicians and patients. This study involved multiple rounds of in-depth interviews with a diverse group of patients and clinicians, along with over 300 hours of participant observation on the labor and delivery floor of a major US hospital, where I observed how clinical decisions are made in real time.
For the last 8 years, I’ve worked in clinical research organizations (CROs) in the US, providing research, consulting and strategy to a wide range of national and international pharmaceutical and medical device companies. Most recently I was a patient-reported outcomes researcher at a large CRO, where I managed several multi-site research studies with the goal of evaluating, adapting or developing patient-centered clinical outcome measures for FDA approval. I have experience across a broad range of therapeutic areas, including gastroenterology, oncology, nephrology and immunology, as well as experience conducting research with adult, adolescent and pediatric patients and their caregivers.
I am passionate about improving patients’ quality of life and health outcomes by bringing their voices, preferences and experiences to the forefront of healthcare research and decision making.
- the inherent variability of the process estimator
- the measurement error
- the number of independent replications (sample size)
- the efficiency of the sampling scheme.
Example 1: We want to compare the effect of two different coolants on the resulting surface finish from a turning operation. It is decided to run one lot, change the coolant and then run another lot. With this sampling scheme, there is no way to distinguish the coolant effect from the lot effect or from tool wear considerations. There is systematic sampling error in this sampling scheme.
Example 2: We wish to examine the effect of two pre-clean procedures on the uniformity of an oxide growth process. We clean one cassette of wafers with one method and another cassette with the other method. We load one cassette in the front of the furnace tube and the other cassette in the middle. To complete the run, we fill the rest of the tube with other lots. With this sampling scheme, there is no way to distinguish between the effect of the different pre-clean methods and the cassette effect or the tube location effect. Again, we have systematic sampling errors.
- Case Study 1 (Sampling Plan)
- Case Study 2 (Sampling Plan)
Have a language expert improve your writing
Run a free plagiarism check in 10 minutes, generate accurate citations for free.
- Knowledge Base
Methodology
Cluster Sampling | A Simple Step-by-Step Guide with Examples
Published on September 7, 2020 by Lauren Thomas . Revised on June 22, 2023.
In cluster sampling , researchers divide a population into smaller groups known as clusters . They then randomly select among these clusters to form a sample .
Cluster sampling is a method of probability sampling that is often used to study large populations, particularly those that are widely geographically dispersed. Researchers usually use pre-existing units such as schools or cities as their clusters.
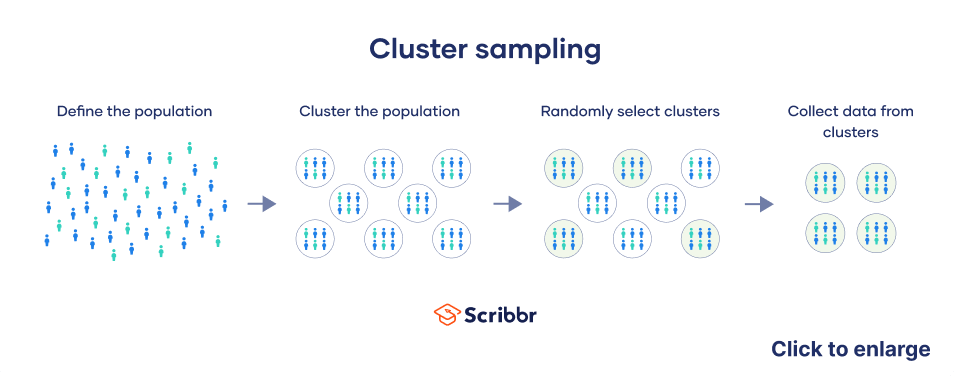
Table of contents
How to cluster sample, multistage cluster sampling, advantages and disadvantages, other interesting articles, frequently asked questions about cluster sampling.
The simplest form of cluster sampling is single-stage cluster sampling . It involves 4 key steps.
Step 1: Define your population
As with other forms of sampling, you must first begin by clearly defining the population you wish to study.
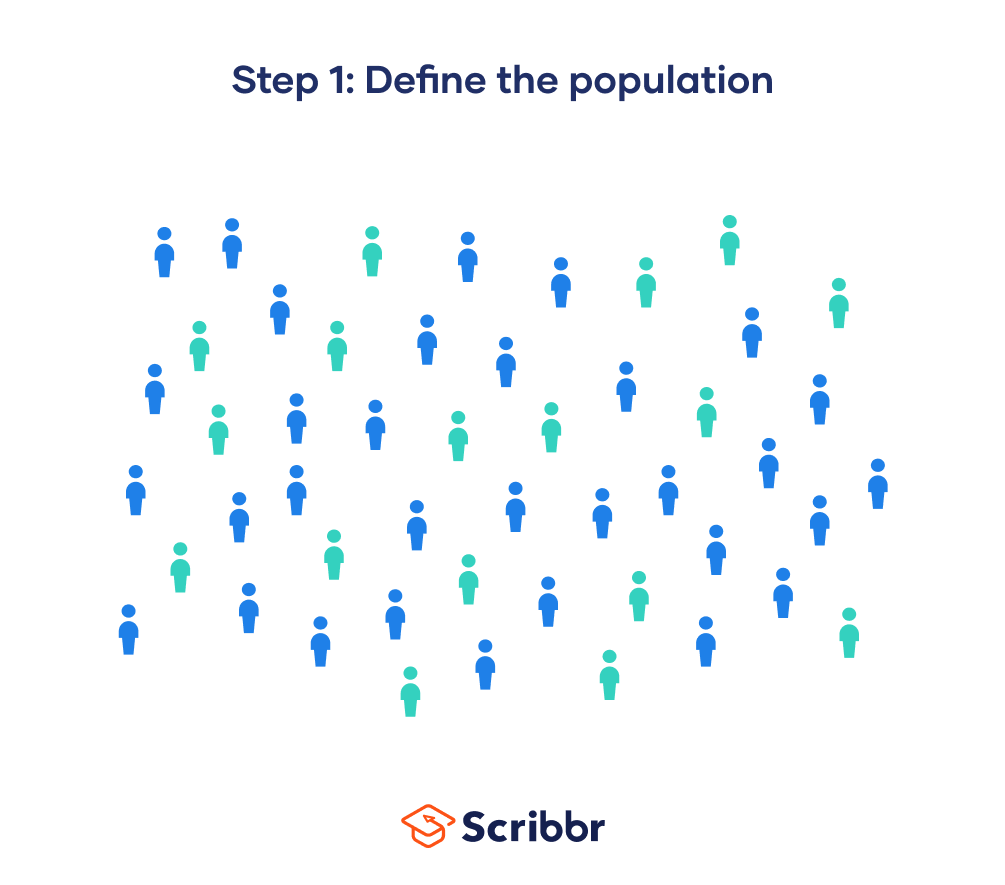
Step 2: Divide your sample into clusters
This is the most important part of the process. The quality of your clusters and how well they represent the larger population determines the validity of your results. Ideally, you would like for your clusters to meet the following criteria:
- Each cluster’s population should be as diverse as possible. You want every potential characteristic of the entire population to be represented in each cluster.
- Each cluster should have a similar distribution of characteristics as the distribution of the population as a whole.
- Taken together, the clusters should cover the entire population.
- There not be any overlap between clusters (i.e. the same people or units do not appear in more than one cluster).
Ideally, each cluster should be a mini-representation of the entire population. However, in practice, clusters often do not perfectly represent the population’s characteristics, which is why this method provides less statistical certainty than simple random sampling , and is more prone to research biases like selection bias .
Because clusters are usually naturally occurring groups, such as schools, cities, or households, they are often more homogenous than the population as a whole. You should be aware of this when performing your study, as it might affect its validity.
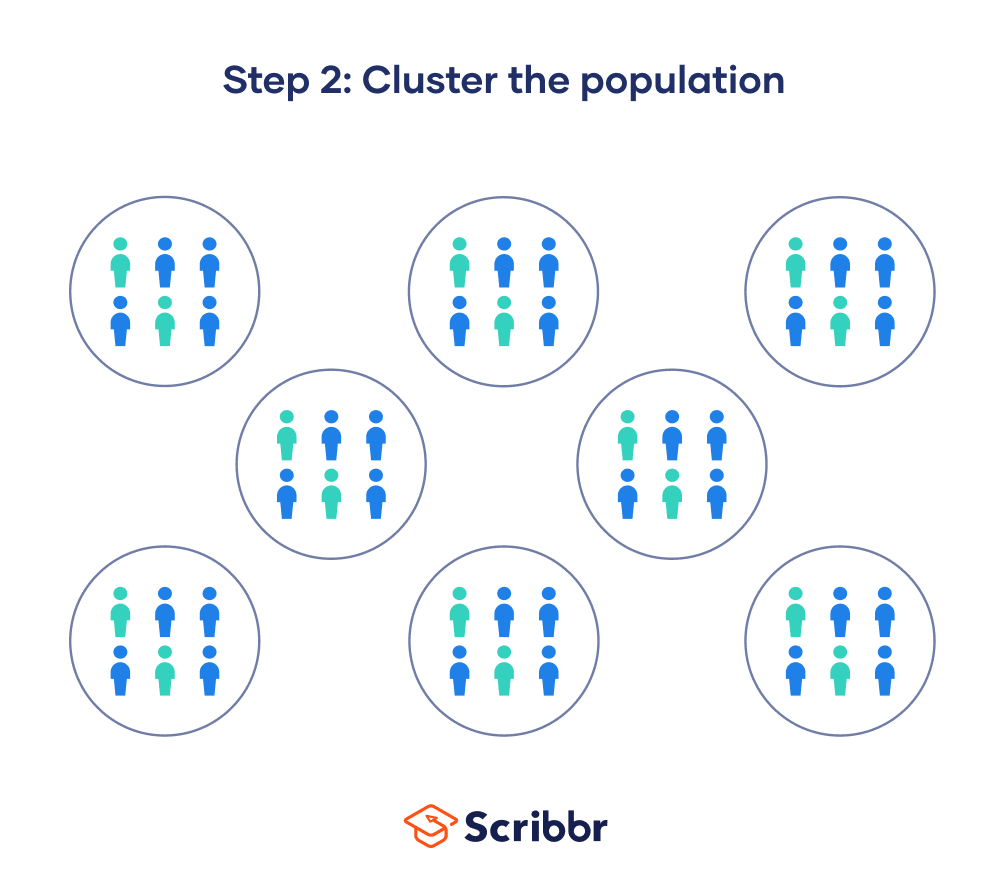
Step 3: Randomly select clusters to use as your sample
If each cluster is itself a mini-representation of the larger population, randomly selecting and sampling from the clusters allows you to imitate simple random sampling, which in turn supports the validity of your results.
Conversely, if the clusters are not representative, then random sampling will allow you to gather data on a diverse array of clusters, which should still provide you with an overview of the population as a whole.
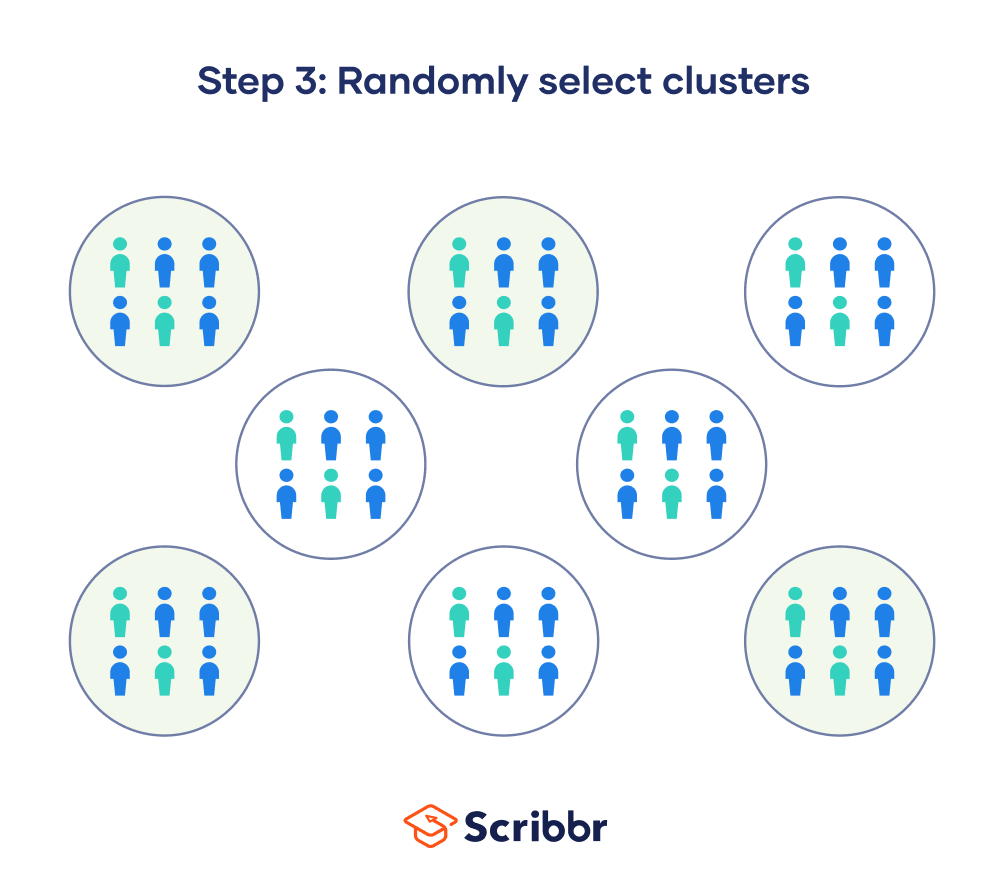
You choose the number of clusters based on how large you want your sample size to be. This in turn is based on the estimated size of the entire seventh-grade population, your desired confidence interval and confidence level , and your best guess of the standard deviation (a measure of how spread apart the values in a population are) of the reading levels of the seventh-graders.
Step 4: Collect data from the sample
You then conduct your study and collect data from every unit in the selected clusters.
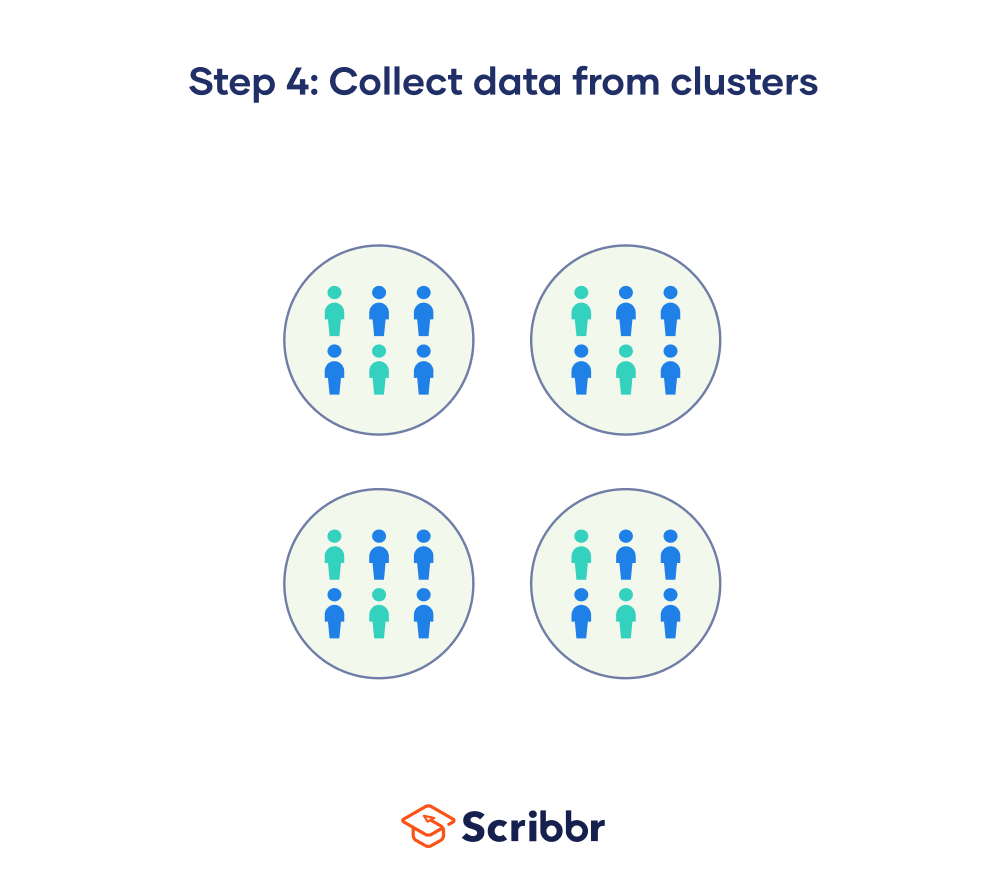
Here's why students love Scribbr's proofreading services
Discover proofreading & editing
In multistage cluster sampling , rather than collect data from every single unit in the selected clusters, you randomly select individual units from within the cluster to use as your sample.
You can then collect data from each of these individual units – this is known as double-stage sampling .
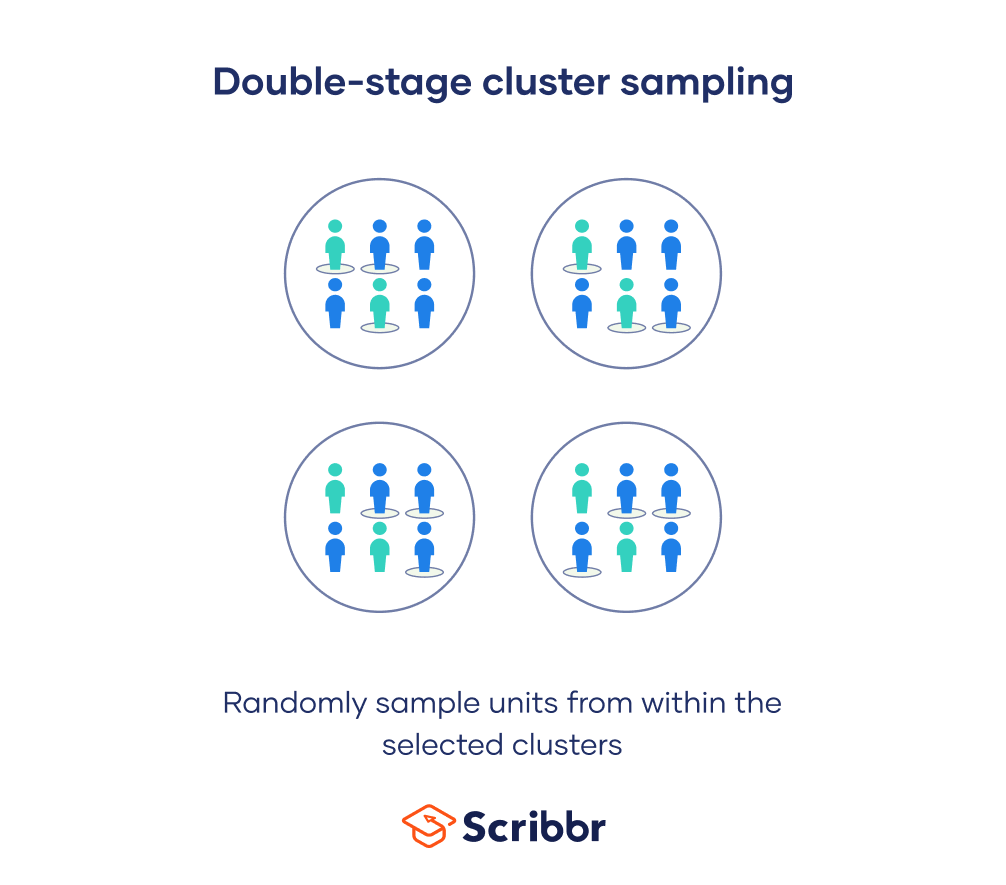
You can also continue this procedure, taking progressively smaller and smaller random samples, which is usually called multistage sampling .
You should use this method when it is infeasible or too expensive to test the entire cluster.
- From each school, you randomly select a sample of seventh-grade classes.
- From within those classes, you randomly select a sample of students.
Cluster sampling is commonly used for its practical advantages, but it has some disadvantages in terms of statistical validity.
- Cluster sampling is time- and cost-efficient, especially for samples that are widely geographically spread and would be difficult to properly sample otherwise.
- Because cluster sampling uses randomization, if the population is clustered properly, your study will have high external validity because your sample will reflect the characteristics of the larger population.
Disadvantages
- Internal validity is less strong than with simple random sampling, particularly as you use more stages of clustering.
- If your clusters are not a good mini-representation of the population as a whole, then it is more difficult to rely upon your sample to provide valid results, and is very likely to be biased .
- Cluster sampling is much more complex to plan than other forms of sampling.
If you want to know more about statistics , methodology , or research bias , make sure to check out some of our other articles with explanations and examples.
- Student’s t -distribution
- Normal distribution
- Null and Alternative Hypotheses
- Chi square tests
- Confidence interval
- Quartiles & Quantiles
- Stratified sampling
- Data cleansing
- Reproducibility vs Replicability
- Peer review
- Prospective cohort study
Research bias
- Implicit bias
- Cognitive bias
- Placebo effect
- Hawthorne effect
- Hindsight bias
- Affect heuristic
- Social desirability bias
Receive feedback on language, structure, and formatting
Professional editors proofread and edit your paper by focusing on:
- Academic style
- Vague sentences
- Style consistency
See an example
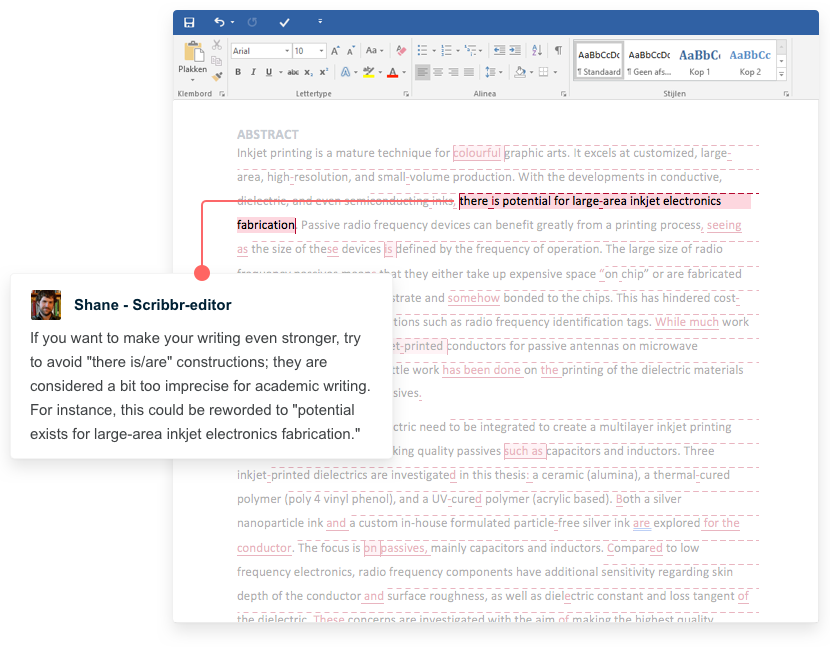
Cluster sampling is a probability sampling method in which you divide a population into clusters, such as districts or schools, and then randomly select some of these clusters as your sample.
The clusters should ideally each be mini-representations of the population as a whole.
There are three types of cluster sampling : single-stage, double-stage and multi-stage clustering. In all three types, you first divide the population into clusters, then randomly select clusters for use in your sample.
- In single-stage sampling , you collect data from every unit within the selected clusters.
- In double-stage sampling , you select a random sample of units from within the clusters.
- In multi-stage sampling , you repeat the procedure of randomly sampling elements from within the clusters until you have reached a manageable sample.
Cluster sampling is more time- and cost-efficient than other probability sampling methods , particularly when it comes to large samples spread across a wide geographical area.
However, it provides less statistical certainty than other methods, such as simple random sampling , because it is difficult to ensure that your clusters properly represent the population as a whole.
Cite this Scribbr article
If you want to cite this source, you can copy and paste the citation or click the “Cite this Scribbr article” button to automatically add the citation to our free Citation Generator.
Thomas, L. (2023, June 22). Cluster Sampling | A Simple Step-by-Step Guide with Examples. Scribbr. Retrieved June 24, 2024, from https://www.scribbr.com/methodology/cluster-sampling/
Is this article helpful?
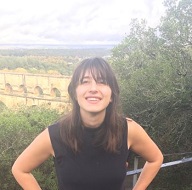
Lauren Thomas
Other students also liked, multistage sampling | introductory guide & examples, stratified sampling | definition, guide & examples, simple random sampling | definition, steps & examples, "i thought ai proofreading was useless but..".
I've been using Scribbr for years now and I know it's a service that won't disappoint. It does a good job spotting mistakes”
Find Study Materials for
- Explanations
- Business Studies
- Combined Science
- Engineering
- English Literature
- Environmental Science
- Human Geography
- Macroeconomics
- Microeconomics
- Social Studies
- Browse all subjects
- Read our Magazine
Create Study Materials
- Flashcards Create and find the best flashcards.
- Notes Create notes faster than ever before.
- Study Sets Everything you need for your studies in one place.
- Study Plans Stop procrastinating with our smart planner features.
- Sampling Plan
Do you like free samples? I do too! Unfortunately, this is not an explanation of free samples, but it's an article about something that sounds quite similar - a sampling plan.
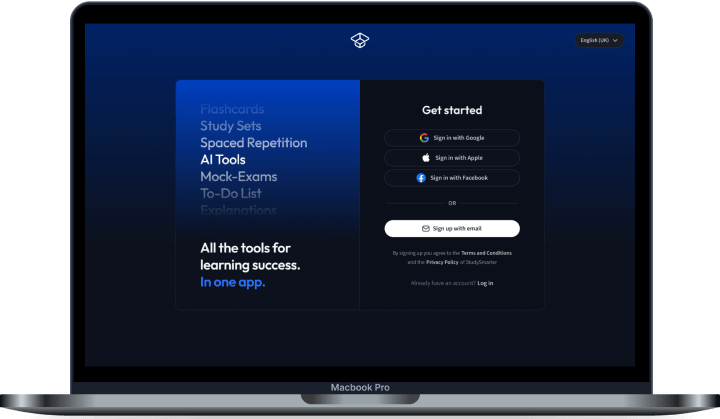
Create learning materials about Sampling Plan with our free learning app!
- Instand access to millions of learning materials
- Flashcards, notes, mock-exams and more
- Everything you need to ace your exams
- Customer Driven Marketing Strategy
- Digital Marketing
- Integrated Marketing Communications
- International Marketing
- Introduction to Marketing
- Marketing Campaign Examples
- Marketing Information Management
- Behavioral Targeting
- Customer Relationship Management
- Ethics in Marketing
- Experimental Research
- Focus Groups
- Interview in Research
- Market Calculations
- Market Mapping
- Market Research
- Marketing Analytics
- Marketing Information System
- Marketing KPIs
- Methods of Market Research
- Multi level Marketing
- Neuromarketing
- Observational Research
- Online Focus Groups
- PED and YED
- Primary Market Research
- Research Instrument
- Secondary Market Research
- Survey Research
- Understanding Markets and Customers
- Marketing Management
- Strategic Marketing Planning
This might not be a term you are very familiar with, but it is a significant part of marketing. We know how important research is for marketing. We need to know the target audience to plan a successful marketing campaign, and a sampling plan is essential to make it successful. Wondering how? Keep reading to find out!
Sampling Plan Definition
Knowing the target audience is vital to understanding their needs and wants. Researchers need to study the population to draw conclusions. These conclusions will serve as a basis for constructing a suitable marketing campaign. But observing every person in the selected location is impractical and, at times, impossible. Therefore, researchers select a group of individuals representative of the population. A sampling plan is an outline based on which research is conducted.
A sampling plan outlines the individuals chosen to represent the target population under consideration for research purposes.
It is crucial to verify that the sampling plan is representative of all kinds of people to draw accurate conclusions.
Sampling Plan Research
The sampling plan is an essential part of the implementation phase in market research - it is the first step of implementing market research.
Check out our explanation of market research to find out more.
Researchers decide the sampling unit, size, and procedure when creating a sampling plan.
Deciding the sampling unit involves defining the target population. The area of interest for the research may contain people that may be out of the scope of the research. Therefore, the researcher must first identify the type of people within the research's parameters.
The sample size will specify how many people from the sampling unit will be surveyed or studied. Usually, in realistic cases, the target population is colossal. Analyzing every single individual is an arduous task. Therefore, the researcher must decide which individuals should be considered and how many people to survey.
The sampling procedure decides how the sample size is chosen. Researchers can do this based on both probability sampling methods and non-probability sampling methods. We will talk about this in more detail in the following sections.
Sampling Plan Types
The sampling plan mainly consists of two different types of methods - one based on probability methods and the other based on non-probability methods .
In the probability sampling method, the researcher lists a few criteria and then chooses people randomly from the population. In this method, all people of the population have an equal chance to be selected. The probability methods are further classified into:
1. Simple Random Sampling - as the name suggests, this type of sampling picks individuals randomly from the selection.
2. Cluster Sampling - the whole population gets divided into groups or clusters. Researchers then survey people from the selected clusters.
3. Systematic Sampling - researchers select individuals at a regular interval; for example, the researcher will select every 15th person on the list for interviews.
4. Stratified Sampling - researchers divide the group into smaller subgroups called strata based on their characteristics. Researchers then pick individuals at random from the strata.
Difference between cluster sampling and stratified sampling
In cluster sampling, all individuals are put into different groups, and all people in the selected groups are studied.
In stratified sampling, all the individuals are put into different groups, and some people from all groups are surveyed.
A non-probability method involves choosing people at random without any defined criteria. This means that not everybody has an equal chance of being selected for the survey. N on-probability techniques can be further classified into:
1. Convenience Sampling - this depends on the ease of accessing a person of interest.
2. Judgemental Sampling - also known as purposive sampling, includes selecting people with a particular characteristic that supports the scope of the research.
3. Snowball Sampling - used when trying to find people with traits that are difficult to trace. In such cases, the researcher would find one or two people with the traits and then ask them to refer to people with similar characteristics.
4. Quota Sampling - this involves collecting information from a homogenous group.
Steps of a Sample Plan
A sampling plan helps researchers collect data and get results quicker, as only a group of individuals is selected to be studied instead of the whole population. But how is a sampling plan conducted? What are the steps of a sample plan?
A sampling plan study consists of 5 main steps:
1. Sample Definition - this step involves identifying the research goals or what the research is trying to achieve. Defining the sample will help the researcher identify what they have to look for in the sample.
2. Sample Selection - after the sample definition, researchers now have to obtain a sample frame. The sample frame will give the researchers a list of the population from which the researcher chooses people to sample.
3. Sample Size Determination - the sample size is the number of individuals that will be considered while determining the sampling plan. This step defines the number of individuals that the researcher will survey.
4. Sample Design - in this step, the samples are picked from the population. Researchers can select individuals based on probability or non-probability methods.
5. Sample Assessment - this step ensures that the samples chosen are representative enough of the population and ensures quality data collection.
After these processes are finalized, researchers carry forward with the rest of the research, such as drawing conclusions that form a basis for the marketing campaign.
Probability sampling methods are more complex, costly, and time-consuming than non-probability methods.
Sampling Plans Example
Different methods of sampling plans help to yield different types of data. The sampling plan will depend on the company's research goals and limitations. Given below are a few examples of companies that use different types of sampling plans:
1. Simple Random Sampling - A district manager wants to evaluate employee satisfaction at a store. Now, he would go to the store, pick a few employees randomly, and ask them about their satisfaction. Every employee has an equal chance of being selected by the district manager for the survey.
2. Cluster Sampling - A reputed private school is planning to launch in a different city. To gain a better insight into the city, they divided the population based on families with school-aged kids and people with high incomes. These insights will help them decide if starting a branch in that particular city would be worth it or not.
3. Systematic Sampling - A supermarket with many branches decides to reallocate its staff to improve efficiency. The manager decides that every third person, chosen per their employee number, would be transferred to a different location.
4. Stratified Sampling - A research startup is trying to understand people's sleep patterns based on different age groups. Therefore, the whole sampling unit gets divided into different age groups (or strata), such as 0-3 months, 4-12 months, 1-2 years, 3-5 years, 6-12 years, and so on. Some people from all the groups are studied.
5. Convenience Sampling - An NGO is trying to get people to sign up for a "street-clean" program as part of the Earth Day campaign. They have stationed themselves on the sidewalks of a busy shopping street, and are approaching people who pass them by to try and pursue them to join the program.
6. Judgemental Sampling - A real estate company is trying to determine how the rental price hike affects people. To find the answer to this question, they would only have to consider people that live in rented houses, meaning that people who own a home would be excluded from this survey.
7. Snowball Sampling - A pharmaceutical company is trying to get a list of patients with leukemia. As the company cannot go to hospitals to ask for patients' information, they would first find a couple of patients with the illness and then ask them to refer patients with the same illness.
8. Quota Sampling - Recruiters that want to hire employees with a degree from a particular school will group them into a separate subgroup. This type of selection is called quota selection.
Sampling plan - Key takeaways
- During a sampling plan in research, the sampling unit, the sampling size, and the sampling procedure are determined.
- The sample size will specify how many people from the sampling unit will be surveyed or studied.
- The sampling procedure decides how researchers will select the sample size.
- The methods of probability sampling include simple random, cluster, systematic, and stratified sampling.
- The non-probability sampling plan methods include convenience, judgemental, snowball, and quota sampling.
- Sample definition, sample selection, sample size determination, sample design, and sample assessment are the steps of a sample plan.
Flashcards in Sampling Plan 18
Define sampling plan.
A sampling plan outlines the individuals chosen to represent the target population under consideration for research purposes.
The sampling plan is a part of the _________ phase.
During a sampling plan in research, _____________, ___________, and the sampling procedure are decided.
During a sampling plan in research, the sampling unit , the sampling size , and the sampling procedure are decided.
The ___________ involves deciding the target population.
sampling unit
The sample size
will specify how many people from the sampling unit will be surveyed or studied.
What are the two types of sampling plans?
Probability and non-probability sampling .
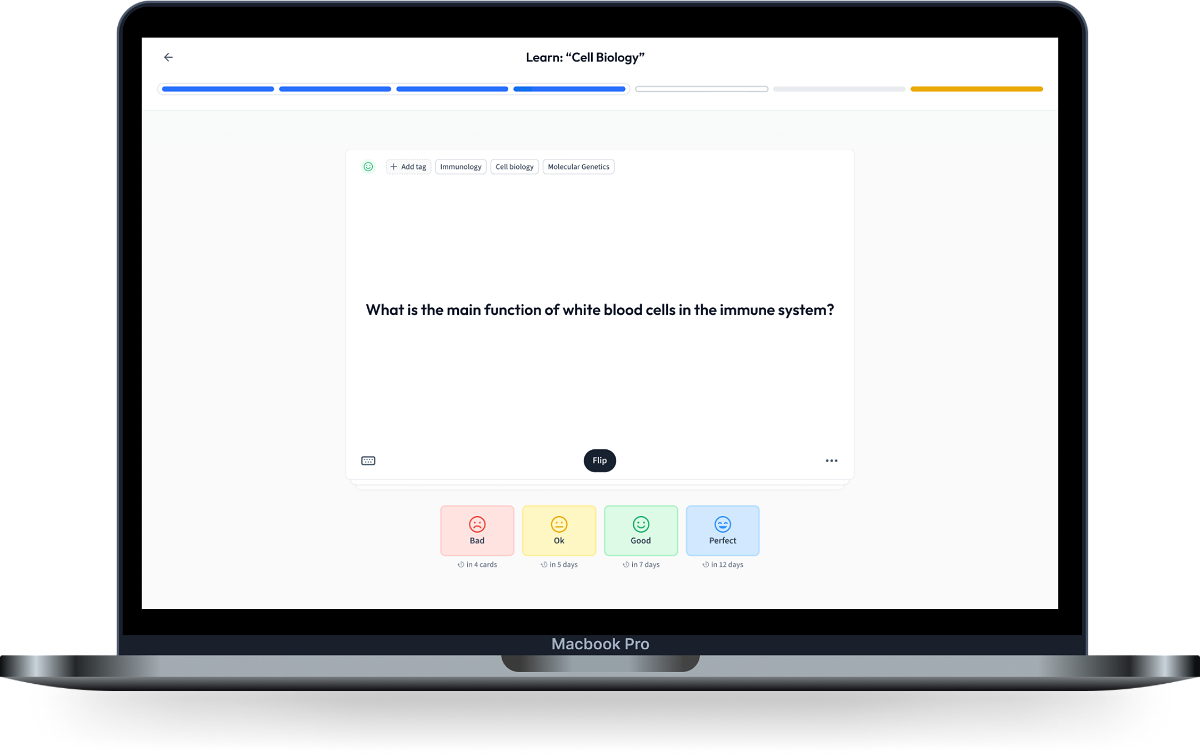
Learn with 18 Sampling Plan flashcards in the free StudySmarter app
We have 14,000 flashcards about Dynamic Landscapes.
Already have an account? Log in
Frequently Asked Questions about Sampling Plan
What is a sample plan in marketing?
Researchers need to study the population to draw conclusions. But observing every person in the selected location is impractical and, at times, impossible. Therefore, researchers select a group of individuals representative of the population. A sampling plan outlines the individuals chosen to represent the target population under consideration for research purposes.
What is a sampling plan and its types?
The sampling plan mainly consists of two different types of methods - one based on probability methods and the other based on non-probability methods. Probability sampling methods include simple random, cluster, systematic, and stratified sampling. The non-probability sampling methods include convenience, judgemental, snowball, and quota sampling.
Why is the sampling plan important?
The sampling plan is an essential part of the implementation phase in market research - it is the first step of implementing market research. Observing every person in the selected location is impractical. Therefore, researchers select a group of individuals representative of the population called the sampling unit. This is outlined in the sampling plan.
What should a marketing plan include?
A good marketing plan should include the target market, the unique selling proposition, SWOT analysis, marketing strategies, the budget, and the duration of the research.
What are the components of a sampling plan?
The sample definition, sample selection, sample size determination, sample design, and sample assessment are the components of a sampling plan.
Test your knowledge with multiple choice flashcards
The ___________ involves deciding the target population.
The sample size
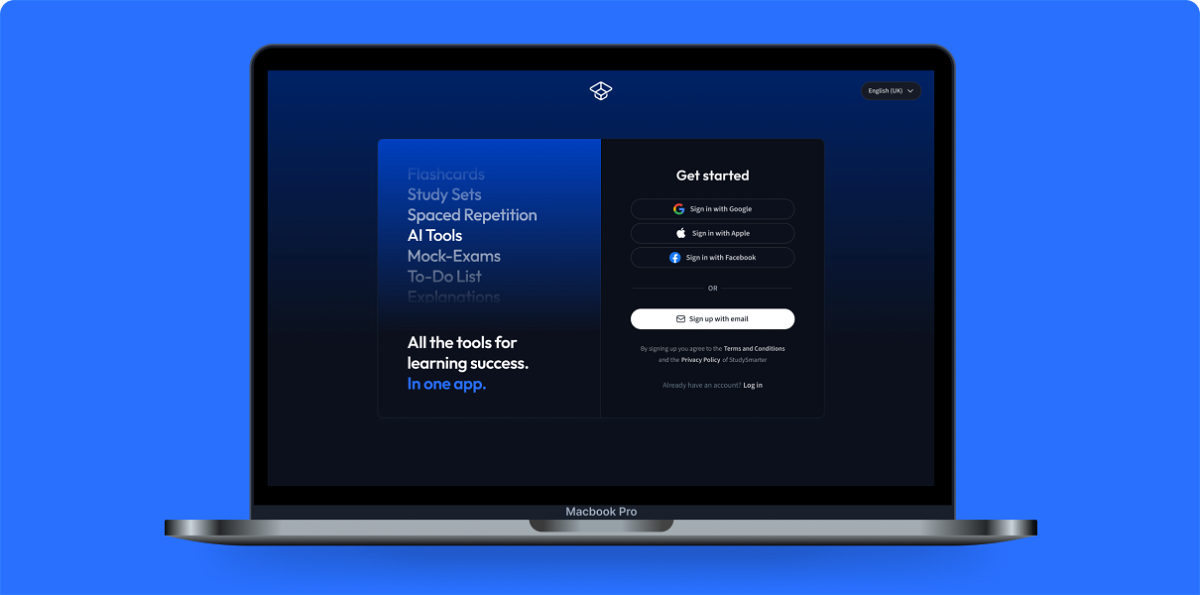
Join the StudySmarter App and learn efficiently with millions of flashcards and more!
Keep learning, you are doing great.
Discover learning materials with the free StudySmarter app
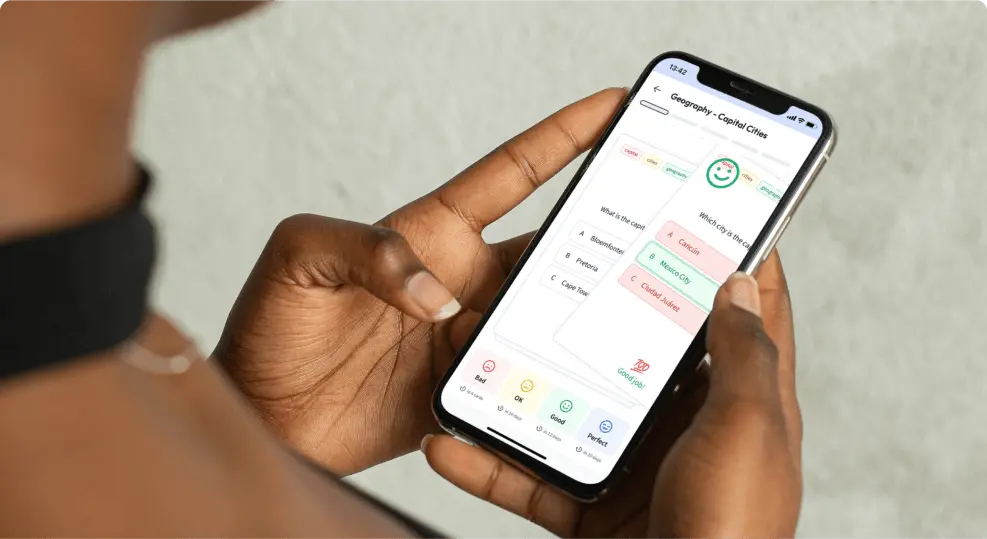
About StudySmarter
StudySmarter is a globally recognized educational technology company, offering a holistic learning platform designed for students of all ages and educational levels. Our platform provides learning support for a wide range of subjects, including STEM, Social Sciences, and Languages and also helps students to successfully master various tests and exams worldwide, such as GCSE, A Level, SAT, ACT, Abitur, and more. We offer an extensive library of learning materials, including interactive flashcards, comprehensive textbook solutions, and detailed explanations. The cutting-edge technology and tools we provide help students create their own learning materials. StudySmarter’s content is not only expert-verified but also regularly updated to ensure accuracy and relevance.
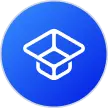
StudySmarter Editorial Team
Team Marketing Teachers
- 9 minutes reading time
- Checked by StudySmarter Editorial Team
Study anywhere. Anytime.Across all devices.
Create a free account to save this explanation..
Save explanations to your personalised space and access them anytime, anywhere!
By signing up, you agree to the Terms and Conditions and the Privacy Policy of StudySmarter.
Sign up to highlight and take notes. It’s 100% free.
Join over 22 million students in learning with our StudySmarter App
The first learning app that truly has everything you need to ace your exams in one place
- Flashcards & Quizzes
- AI Study Assistant
- Study Planner
- Smart Note-Taking
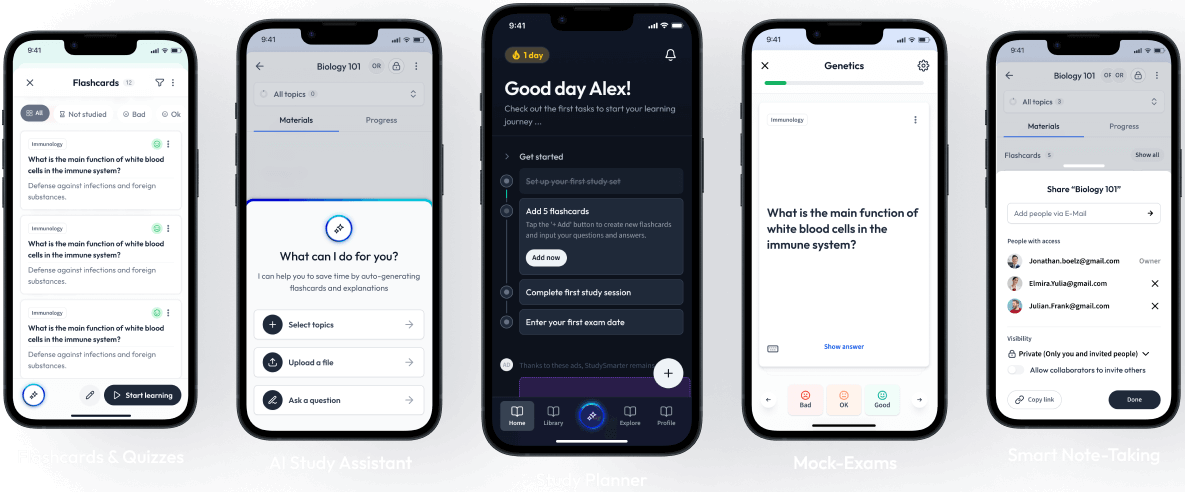
Get unlimited access with a free StudySmarter account.
- Instant access to millions of learning materials.
- Flashcards, notes, mock-exams, AI tools and more.
- Everything you need to ace your exams.
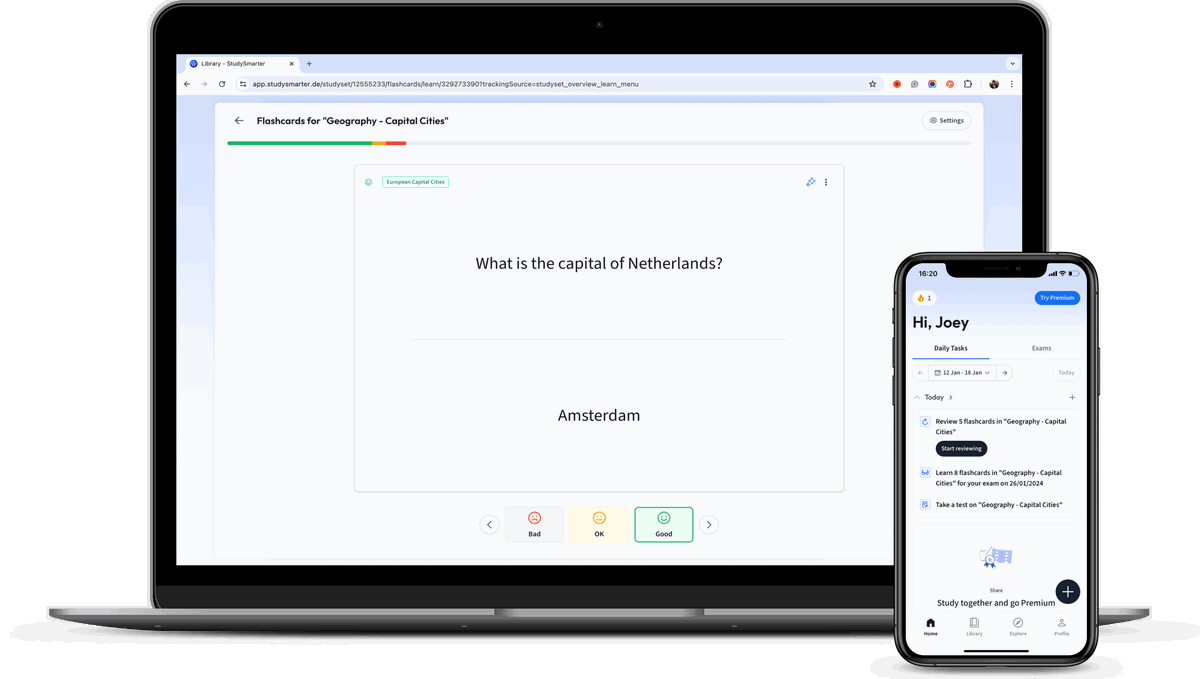
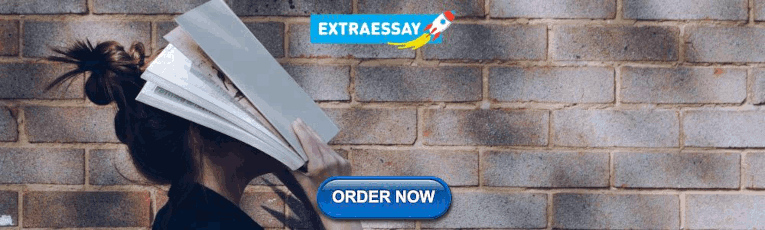
IMAGES
VIDEO
COMMENTS
Learn how to select a representative sample for your research using probability and non-probability methods. See examples of simple random, systematic, stratified and cluster sampling techniques.
Sampling methods in psychology refer to strategies used to select a subset of individuals (a sample) from a larger population, to study and draw inferences about the entire population. Common methods include random sampling, stratified sampling, cluster sampling, and convenience sampling. Proper sampling ensures representative, generalizable, and valid research results.
Stratified random sampling. Stratified random sampling is similar to simple random sampling, but it kicks things up a notch. As the name suggests, stratified sampling involves selecting participants randomly, but from within certain pre-defined subgroups (i.e., strata) that share a common trait.For example, you might divide the population into strata based on gender, ethnicity, age range or ...
Understand sampling methods in research, from simple random sampling to stratified, systematic, and cluster sampling. Learn how these sampling techniques boost data accuracy and representation, ensuring robust, reliable results. Check this article to learn about the different sampling method techniques, types and examples.
This is often used to ensure that the sample is representative of the population as a whole. Cluster Sampling: In this method, the population is divided into clusters or groups, and then a random sample of clusters is selected. Then, all members of the selected clusters are included in the sample. Multi-Stage Sampling: This method combines two ...
Systematic random sample. Definition: Put every member of a population into some order. Choosing a random starting point and select every nth member to be in the sample. Example: A teacher puts students in alphabetical order according to their last name, randomly chooses a starting point, and picks every 5th student to be in the sample. Benefit ...
Learn about different sampling methods, such as simple, systematic, stratified, and cluster sampling, and how to choose the best one for your study. See examples, advantages, disadvantages, and potential errors to avoid.
A sample is a subset of individuals from a larger population. Sampling means selecting the group that you will actually collect data from in your research. For example, if you are researching the opinions of students in your university, you could survey a sample of 100 students.
Sampling Strategies in Qualitative Research In: The SAGE Handbook of Qualitative Data Analysis By: Tim Rapley Edited by: Uwe Flick Pub. Date: 2013 ... factors tied to delay. So, for example, in rheumatoid arthritis in adults, the central issue was family doctors not recognizing the patient's problems as disease related. • Finally, as I had ...
Major Sampling Schemes Probability Schemes: Sampling Scheme Description Simple Every individual in the sampling frame (i.e., desired population) has an equal and independent chance of being chosen for the study. Stratified Sampling frame is divided into sub-sections comprising groups that are relatively homogeneous with respect to one or more characteristics and a
Step 1: Define your population. Like other methods of sampling, you must decide upon the population that you are studying. In systematic sampling, you have two choices for data collection: You can select your sample ahead of time from a list and then approach the selected subjects to collect data, or.
Abstract. Knowledge of sampling methods is essential to design quality research. Critical questions are provided to help researchers choose a sampling method. This article reviews probability and non-probability sampling methods, lists and defines specific sampling techniques, and provides pros and cons for consideration.
Sampling in market action research is of two types - probability sampling and non-probability sampling. Let's take a closer look at these two methods of sampling. Probability sampling:Probability sampling is a sampling technique where a researcher selects a few criteria and chooses members of a population randomly.
Whether it is to study consumer behavior, analyze market trends, or explore the impact of a specific intervention, outlining the research objectives provides a clear roadmap for sampling. Example: Without a clear research objective, sampling becomes directionless, leading to inaccurate results that do not contribute to meaningful insights. 2.
A qualitative sampling plan describes how many observations, interviews, focus-group discussions or cases are needed to ensure that the findings will contribute rich data. In quantitative studies, the sampling plan, including sample size, is determined in detail in beforehand but qualitative research projects start with a broadly defined ...
Probability sampling is a sampling method that involves randomly selecting a sample, or a part of the population that you want to research. It is also sometimes called random sampling. To qualify as being random, each research unit (e.g., person, business, or organization in your population) must have an equal chance of being selected.
Regardless of which sampling method is used, a good sampling strategy encourages diversity to ensure research findings are valid, reliable, and generalisable. For example, a clinical trial to test a drug intended to be used on the general population would need to include a very large and diverse sample to account for all demographics.
Convenience/ Accidental Sampling: Accidental sampling (sometimes known as grab, convenience or. opportunity sampling) is a type of non-probability sam pling which involves the samp le being drawn ...
Data Collection for PPC. 3.3.3. Define Sampling Plan. 3.3.3.2. Choosing a Sampling Scheme. A sampling scheme is a detailed description of what data will be obtained and how this will be done. In PPC we are faced with two different situations for developing sampling schemes. The first is when we are conducting a controlled experiment.
A population frame is the source material or device from which a sample is drawn. It is a list of all those within a population who can be sampled, and may include individuals, households or institutions. A population frame is a basis of the characteristics that are required to be observed by the study. An example of population frame is "People ...
The simplest form of cluster sampling is single-stage cluster sampling. It involves 4 key steps. Research example. You are interested in the average reading level of all the seventh-graders in your city. It would be very difficult to obtain a list of all seventh-graders and collect data from a random sample spread across the city.
1. Sample Definition - this step involves identifying the research goals or what the research is trying to achieve. Defining the sample will help the researcher identify what they have to look for in the sample. 2. Sample Selection - after the sample definition, researchers now have to obtain a sample frame.
Multiple sampling plan (MSP) is a generalization of single and double sampling plans that enhances the efficiency by reducing the number of sample items needed for inspection. ... analyzed, and contrasted with the traditional sampling plan under various settings. In addition, an example is presented using the developed graphical user interface ...
The 5-4 decision granted requests by Ohio, Indiana and West Virginia, as well as U.S. Steel Corp, pipeline operator Kinder Morgan and industry groups, to halt enforcement of the EPA's "Good ...