- Research article
- Open access
- Published: 20 May 2020
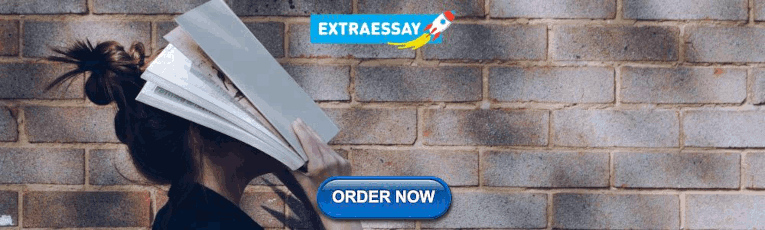
Students’ perceptions on distance education: A multinational study
- Patricia Fidalgo 1 ,
- Joan Thormann 2 ,
- Oleksandr Kulyk 3 &
- José Alberto Lencastre 4
International Journal of Educational Technology in Higher Education volume 17 , Article number: 18 ( 2020 ) Cite this article
363k Accesses
128 Citations
12 Altmetric
Metrics details
Many universities offer Distance Education (DE) courses and programs to address the diverse educational needs of students and to stay current with advancing technology. Some Institutions of Higher Education (IHE) that do not offer DE find it difficult to navigate through the steps that are needed to provide such courses and programs. Investigating learners’ perceptions, attitudes and willingness to try DE can provide guidance and recommendations for IHEs that are considering expanding use of DE formats. A survey was distributed to undergraduate students in Portugal, UAE and Ukraine. The results of this pilot study showed that in all three countries, students’ major concerns about such programs were time management, motivation, and English language skills. Although students were somewhat apprehensive many indicated they were interested in taking DE courses. Six recommendations informed by interpretation of students’ responses and the literature, are offered to assist institutions who want to offer DE as part of their educational strategy.
Introduction
The World Wide Web has made information access and distribution of educational content available to a large fraction of the world’s population and helped to move Distance Education (DE) to the digital era. DE has become increasingly common in many universities worldwide (Allen & Seaman, 2017 ). Nonetheless, there are still many universities that do not provide this opportunity because it is not part of their institutional culture. As DE becomes more prevalent, countries and Institutions of Higher Education (IHE) that do not provide DE courses will need to look at this option to retain and expand their student population. (Keegan, 1994 ; Nakamura, 2017 ).
In order to develop such programs, it is useful to determine if students are receptive to taking such online courses and are prepared to do so. This study addresses students’ perceptions and their interest in DE. In addition, it provides a comparative analysis across three countries whose IHEs do not have extensive offerings in DE. The results of this research provide some strategies to encourage and support students to take DE courses.
Literature review
A seminal article by Keegan ( 1980 ) presents key aspects of DE. Some of the elements are: physical separation of teacher and learner, learning occurs in the context of an educational institution, technical media are used, teacher and learner communicate, face to face meetings are possible, and an industrial model of providing education is used. More recently varying definitions of DE seem to be based on the perspective of various educators and to reflect the educational culture of each country and IHE. However, some common descriptors seem to be accepted by most stakeholders in the field. Distance education is an educational experience where instructors and learners are separated in time and space (Keegan, 2002 ) which means it can happen away from an academic institution and can lead to a degree or credential (Gunawardena, McIsaac, & Jonassen, 2008 ).
Although there are different types of DE, this research focuses on online learning. The following types of online learning will be investigated: synchronous, asynchronous, blended, massive online open courses (MOOC), and open schedule online courses. In synchronous instruction, teachers and learners meet (usually online) for a session at a predetermined time. According to Watts ( 2016 ) live streaming video and/or audio are used for synchronous interaction. Although videoconferencing allows participants to see each other this is not considered a face-to-face interaction because of the physical separation (Keegan, 1980 ).
Asynchronous instruction means that teachers and learners do not have synchronous sessions and that students have access to course content through the Internet at any time they want or need. Communication among the participants occurs mainly through email and online forums and is typically moderated by the instructor (Watts, 2016 ). According to Garrison ( 2000 ) “Asynchronous collaborative learning may well be the defining technology of the postindustrial era of distance education.” (p.12) Yet another type of DE is blended learning (BL). Garrison and Kanuka ( 2004 ) define BL as combining face-to-face classroom time with online learning experiences. Although it is not clear as to how much time is allocated to online in the blended model “the real test of blended learning is the effective integration of the two main components (face-to-face and Internet technology) such that we are not just adding on to the existing dominant approach or method.” (p.97) In the BL format different teaching strategies and instructional technology can be used to help individuals who have different learning styles, needs and interests (Tseng & Walsh Jr., 2016 ).
Another type of DE is MOOCs (Massive Online Open Courses). This format was first introduced in 2006 and offers distributed open online courses that are available without cost to a very large number of participants (Cormier, McAuley, Siemens, & Stewart, 2010 ). MOOCs origins can be traced to the Open Access Initiative in 2002 which advocates sharing knowledge freely through the Internet. By providing educational opportunities MOOCs can address the increasing demand for training and education (Zawacki-Richter & Naidu, 2016 ). Finally, in open schedule online courses students work asynchronously with all the materials being provided digitally. Although there are deadlines for submitting assignments, students working at their own pace have some independence as to when they do their coursework (Campus Explorer, 2019 ).
There are advantages and disadvantages in taking DE courses. Some of the advantages are self-paced study, time and space flexibility, time saving (no commute between home and school) and the fact that a distance learning course often costs less. Disadvantages include a sense of isolation, the struggle with staying motivated, lack of face-to-face interaction, difficulty in getting immediate feedback, the need for constant and reliable access to technology, and occasionally some difficulty with accreditation (De Paepe, Zhu, & Depryck, 2018 ; Lei & Gupta, 2010 ; Venter, 2003 ; Zuhairi, Wahyono, & Suratinah, 2006 ).
Most of the literature concerning student perception of DE courses, both blended and entirely online, involves students who have enrolled in online courses. Some articles address comparisons of perceptions between face-to-face and online students regarding DE (Daniels & Feather, 2002 ; Dobbs, del Carmen, & Waid-Lindberg, 2017 ; Hannay & Newvine, 2006 ; Lanier, 2006 ). Additional studies address adult and undergraduate students and cover many aspects of the online experience (Dobbs et al., 2017 ; Horspool & Lange, 2012 ; Seok, DaCosta, Kinsell, & Tung, 2010b , a ). However, little, if any research has been conducted that only addresses perceptions of students who live in countries in which few IHEs offer online courses.
In a study comparing online and face-to-face learning, Horspool and Lange ( 2012 ) found that students chose to take online courses to avoid travel time to class and scheduling problems. A majority of both face-to-face and online students did not experience technological issues. Both groups also found that communication with the instructor was adequate. Online students indicated that instructor response time to questions was prompt. By contrast online students perceived peer communication as occurring much less often. Course satisfaction was comparable for both formats (Horspool & Lange, 2012 ). Responses to another survey concerning online and traditional course formats found that students’ reasons for taking online courses included flexibility to accommodate work and family schedules, the ability to avoid commuting to the university and more online courses being available to them (Dobbs et al., 2017 ). Both online and traditional students agreed that traditional courses were easier, and they learned more in that format. They also concurred that online courses required more effort. Experienced online students indicated that the quality of their courses was good while traditional students who had never taken an online course felt that the quality of online courses was lower.
There is additional research that focuses on students including those enrolled in community colleges, MOOCs, blended learning as well as adult learners. Community college students’ and instructors’ perceptions of effectiveness of online courses were compared by Seok et al. ( 2010b , a ). The researchers focused on pedagogical characteristics (management, Universal Design for Learning, interaction, teaching design and content) and technical features (interface, navigation and support). In addition, responses were examined based on various aspects of the subjects’ demographics. Two surveys with 99 items were distributed electronically. One survey was for instructors and the other for students. In general, instructors and students indicated that teaching and learning online was effective. Female students responded more positively to most questions concerning effectiveness, and instructors also found it more positive (Seok et al., 2010b , a ).
Students who enrolled in a MOOC were motivated to take other courses in this format based on their perception that it was useful for achieving their goals. In addition, their motivation was high if the course was posted on a platform that was easy to use (Aharony & Bar-Ilan, 2016 ). This study also found that as students proceeded through the course, they gained confidence.
Blended learning was examined by Kurt and Yildirim ( 2018 ) to determine student satisfaction and what they considered to be important features of the blended format. The results indicated that the Turkish students who participated, almost unanimously felt that BL was beneficial and that their own role and the instructors’ role was central to their satisfaction. The authors stated, “the prominent components in the process have been identified as face-to-face lessons, the features of online course materials, LMS used, design-specific activities, process-based measurement and evaluation, student-student interaction and out-of-class sharing respectively.” (p. 439) DE has a growth potential and offers the opportunity to reach many people (Fidalgo, 2012 ), hence it can be used as a technique for mass education (Perraton, 2008 ). According to Perraton ( 2008 ) DE can be adapted to the needs of current and previous generations who did not complete their education. DE can also reach individuals who live in remote locations and do not have the means to attend school.
Methodology
Study goals.
The goal of this pilot study is to examine what undergraduate students’ perceptions are concerning DE and their willingness to enroll in this type of course. This study focuses on three countries that do not offer extensive DE accredited programs. By comparing three countries with similar DE profiles, commonalties and differences that are relevant and useful can be found. When the IHEs from these countries decide or have the conditions to move towards DE, the results of this study may help them adapt this format to their particular context and students’ needs. Results may also help IHEs plan their strategy for offering online courses to current and future students and attract prospective students who otherwise would not be able to enroll in the face-to-face courses that are available.
Research questions
Have undergraduate students taken an online course previously?
What are undergraduate students’ perceptions of distance education?
What are the reasons for undergraduate students to enroll/not enroll is distance education courses?
What preparation do undergraduate students feel they need to have before taking distance education courses?
What is the undergraduate students’ receptivity towards enrolling in distance education courses?
What types of distance education would undergraduate students be interested in taking?
This research was conducted at IHEs in three countries (Portugal, Ukraine and UAE). A description of each country’s sociodemographic and technological use provides a context for this study.
Portugal, a country located at the western end of the European continent, has a resident population of just over 10 million people (Instituto Nacional de Estatistica, 2019 ). Data collected by Instituto Nacional de Estatistica in 2019 indicated that almost 81% of households in Portugal had Internet access at home. According to the Portuguese National Statistical Institute ( 2019 ), the rate of Internet use by the adult population is about 76%. Among this population, people who attend or have completed secondary and higher education have a higher percentage of Internet use (98%) (Instituto Nacional de Estatistica, 2019 ).
The most used devices to access the Internet are smartphones and laptops. Regarding computer tasks, the most frequent ones are copying and moving files and folders and transferring files from the computer to other devices (PORDATA - Base de Dados Portugal Contemporâneo, 2017 ).
Among Internet users, 80% use social networks, which is a higher percentage than the European Union (EU) average. Mobile Internet access (outside the home and workplace and on portable devices) is 84% and maintains a strong growth trend (Instituto Nacional de Estatistica, 2019 ).
Ukraine is one of the post-soviet countries located in Eastern Europe and it strives to be integrated in economic and political structures of the EU. The current population of the country is 42 million. Despite the low incomes of many Ukrainians, modern technological devices are widespread among the population. The State Statistics Service of Ukraine ( 2019 ) reported that there were 26 million Internet subscribers in the country in the beginning of 2019. However, Ukrainians do not have a high level of digital literacy yet. According to the Digital Transformation Ministry of Ukraine (Communications Department of the Secretariat of the CMU, 2019 ), almost 38% of Ukrainian people aged from 18 to 70 have poor skills in computer literacy and 15.1% of the citizens have no computer skills.
According to the survey conducted by the Digital Transformation Ministry of Ukraine (The Cabinet of Ministers of Ukraine, 2019 ) 27.5% Ukrainian families have a tablet, and 30.6% have one smart phone, 26.4% have two smart phones, 16.5% have three smart phones and 10.8% have four and more smart phones. As for laptops, 42.7% Ukrainian families have a laptop and 45.6% have a desktop computer (The Cabinet of Ministers of Ukraine, 2019 ). The data from the ministry did not indicate if families have multiple devices, however the data shows that technological devices are widespread.
The United Arab Emirates (UAE) is a country located in the Persian Gulf that borders with Oman and Saudi Arabia. The UAE has a population of 9.77 million and is one of the richest countries in the world based on gross domestic product (GDP) per capita. The resident population consists of 11,5% Emiratis and the remaining residents are expats from countries such as India, Pakistan, Philippines, Egypt and others (Global Media Insight, 2020 ).
Regarding technology use, 91% of the residents use mobile Internetand over 98% of the households have Internet access (Knoema, 2018 ). Mobile devices such as smartphones are used to access the Internet mainly at home or at work (Federal Competitiveness and Statistics Authority, 2017 ).
In 2017 the most frequent Internet activities were: sending/receiving emails (61%), posting information or instant messaging (55%), getting information about goods or services (45%), reading or downloading online newspapers, magazines or electronic books (41%) and telephoning over the Internet/VOIP (33%). Downloading movies, images, music, watching TV or video, or listening to radio or music is also a frequent activity performed by 27% of the Internet users followed by Internet banking (25%) and purchasing or ordering good and services (22%) (Federal Competitiveness and Statistics Authority, 2017 ).
While these three countries were selected due to the location of the researchers and thus provided convenience samples, the three countries have a similar lack of DE offerings. Online surveys were emailed to students enrolled in a variety of undergraduate face-to-face courses during the fall semester of 2018. The students in Portugal and the UAE were enrolled in a teacher education program and the survey was emailed to two course sections in Portugal (73 students) and four course sections in the UAE (108 students). At the IHE in Ukraine, students were majoring in applied mathematics, philology, diagnostics, social work and philosophy, and surveys were emailed to 102 students who were enrolled in five course sections. In Portugal and Ukraine, the URL for the online survey was emailed by the instructor of all the course sections. In the UAE the instructor who emailed the URL for the survey taught two of the course sections. The students in the other two sections knew this instructor from taking courses with her previously. The students participating in this study were a convenience sample based on the disciplines taught by the researchers.
Data collection
An online survey with 10 closed questions about undergraduate students’ perception and receptivity towards enrolling in DE courses was developed by the researchers. Ary, Jacobs, Sorensen, and Walker ( 2010 ) compared traditional methods (i.e. face-to-face, paper and pencil) with web-based surveys and found the latter to be are more effective for gathering data from many participants. The questions designed by the researchers were informed by their experience/practice as well as in-depth literature review. The survey was created to respond to the research questions that guided this study. Response choices to the multiple-choice questions were based on issues and concerns related to DE. Students’ responses were collected towards the end of the first semester of the 2018/19 academic year.
The survey was developed to address research questions that assess undergraduate students’ perceptions of DE and students’ receptivity towards enrolling in DE courses (c.f. Appendix ). The use of surveys allows researchers to “obtain information about thoughts, feelings, attitudes, beliefs, values, perceptions, personality and behavioral intentions of research participants.” (Johnson & Christensen, 2014 , p. 192) The survey questions included multiple response formats: Likert scale, select more than one response and multiple choice. Surveys for Portugal were presented in Portuguese. In Ukraine the surveys were translated into Ukrainian. Since English is the language of instruction at the UAE institution, their survey was in English. The URL for the survey was emailed to students by their instructors and was available in an online Google Form. The survey took approximately 10 min to complete. The study consisted of a “self-selected” convenience sample.
Data analysis
Survey results were recorded in Google Forms and an Excel spreadsheet was used to collect students’ responses. Descriptive statistics of the responses to the survey are presented in graphs and tables with percentages of responses displayed. The descriptive statistics provide summaries about the sample’s answers to each of the questions as well as measures of variability (or spread) and central tendency.
Research approval and data management
The research proposal was submitted to the Research and Grants Committee and approved by the Institutional Review Board of the college in the UAE. No personal information (name, College ID number or any other type of information that allows the identification of students) was asked from the students in the surveys. The surveys were anonymous. Only the Principal Investigator (PI) had access to all the data collected. The data will be stored in the PI’s password protected computer for 5 years.
Fifty five of the 73 Portuguese students who received the survey responded and 98 of the 108 UAE students responded. In the Ukraine 102 students were sent surveys and 70 responded. Below are participants’ responses to questions concerning age, gender, as well as level of confidence using the computer and the Internet.
Students’ age range was from 17 to 50 years old. Most students’ age ranges were between 17 and 29 years. Survey responses indicated that 7% of the students in the UAE were male and 93% female, in the Ukraine 43% were male and 57% female and in Portugal 9% male, and 91% female.
Participants were asked about their level of confidence using a computer and the Internet. Results are presented in Table 1 .
The use of participants from three countries allows the study of trends and to determine differences and/or similarities of perceptions about DE. Although the students were enrolled in courses in diverse content areas, they were all undergraduates, almost all under 30 years old, and most were confident using the computer and Internet. These demographic similarities provided a relatively cohesive group for this study while allowing a comparison across countries.
A range of questions were asked about students’ attitudes towards and experience with DE. To determine the participants’ experience with DE two questions were asked.
The data indicates that out of 223 students who responded to the survey, a total of 63 students have taken DE courses. Half of the Ukraine students, about one quarter of the UAE students and only 5% of students in the group from Portugal had taken DE courses (Fig. 1 ). As shown in Fig. 2 , of the students who have had previous experience in DE, most Ukraine students have taken one or two online courses, most UAE students have taken one course and a few Portuguese students have taken one course.
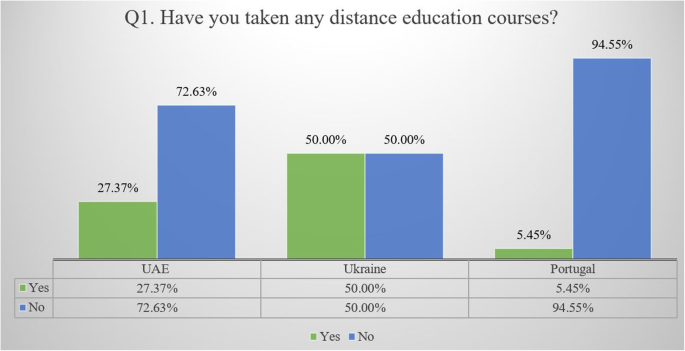
Students that have taken distance education courses
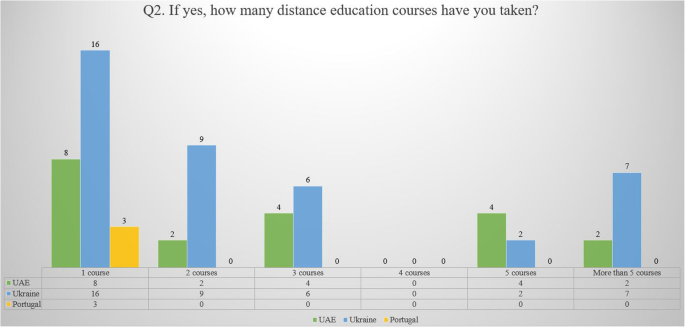
Number of distance education courses taken
More than half of Portuguese students, about two thirds of the Ukraine students and a little over one third of UAE students had a Very favorable or Favorable attitude towards DE. Approximately one third of Portuguese and Ukraine students were Neutral/Unable to judge their attitude. A little less than half of UAE students also indicated this. A small percentage of Portuguese, and one fifth of UAE students indicated their attitude was Very unfavorable or Unfavorable and no Ukraine students reported this (Table 2 ).
More than one third of Portuguese students shared that managing class and study time, saving time by choosing study location and working at their own pace were reasons to enroll in DE. About two thirds of the students from Ukraine reported that working at their own pace and managing their study time were reasons to enroll. A little more than half of these students reported that reasons for enrolling in DE included managing class time, saving time by selecting study location and not having to travel to school as well as having more options for courses or colleges to attend. Almost half of the UAE students had similar reasons for enrolling in a DE courses including managing class and study time, saving time by choosing study location and working at their own pace. In addition, a little more than half of the UAE students also shared that having more options for courses or colleges to attend were reasons to enroll. The reasons that were selected the least by all three groups were that courses were less expensive and enrolling in a preferred program (Tables 3 and 4 ).
Students were given eleven options as to why they would not enroll in DE courses, which are displayed in Tables 5 and 6 . Two reasons that were chosen most often were difficulty staying motivated and preferring face-to-face classes. A small number of Ukraine students reported this as a reason to not enroll in DE courses. Difficulty getting immediate feedback was also a concern for UAE students. Close to one third in the three groups indicated that difficulty contacting the instructor and interacting with peers as well as missing campus life are reasons for not enrolling. About one tenth of Portuguese, one fifth of Ukraine and one fifth of the UAE students reported difficulty getting accreditation as a reason for not enrolling. Not knowing enough about DE was indicated by one tenth of Portuguese, one fifth of Ukraine and one fifth of the UAE students. Only a small number of all the students indicated three categories that are frequently cited in the literature as preventing students from enrolling, these include access to technology, feeling of isolation and too great an expense.
Tables 7 and 8 show student responses to a question regarding the preparation they think they would need before enrolling in a DE course. A little over one tenth of the Portuguese students indicated that they needed better computer equipment, writing skills and a dedicated study space. About one quarter of these students reported they need better skills in the following areas: time management, computer and English language skills, as well as needing to have learning goals and objectives. Having a better Internet connection and the need to develop a study plan was shared by approximately one third of these students. Finally, the highest rated prerequisite for these Portuguese students was to be more motivated.
Few of the Ukraine students felt that they needed better computer equipment or skills, a dedicated study space or a better Internet connection at home. Their concerns focused on their behaviors as students since half or a little more than half felt they needed to be more motivated, have learning objectives and goals, a study plan and better management skills. About one third of these students also reported that they needed better English language skills.
The UAE students were less confident than the Ukraine students about computer skills and needing better equipment and a better Internet connection at home. Almost half of these UAE students reported their need for a study plan and motivation as their most pressing needs. Better management and English language skills were recorded by about one third of the students. One quarter of the UAE students felt they needed better writing skills and a dedicated study space.
Table 9 shows students’ interest in enrolling in DE courses. Almost one quarter of the Ukraine students are Extremely interested in taking DE courses and almost half are Somewhat interested. This contrasts with the students from Portugal who indicated that only 5% are Extremely interested and almost a quarter Somewhat interested. The UAE students’ interest in enrolling fell in between the students from the two other countries. One fifth to almost one third of all three groups were Neutral/Unable to judge. About one tenth of students from Ukraine reported Not being very interested or Not at all interested which contrasts with the Portuguese and UAE students whose numbers were about one half and one quarter respectively.
Tables 10 and 11 show the types of DE that the students were interested in trying. Portuguese students favored Open schedule courses, followed by Blended learning and Synchronous. Few of these students were interested in MOOCs and Asynchronous. More than half of the students from Ukraine were interested in MOOCs and Blended learning followed by Open schedule. About one third of these students were interested in Synchronous and Asynchronous. UAE students most popular formats were Open schedule and Blended learning followed by Synchronous and Asynchronous. There was little interest in MOOCs by the UAE students. Few Portuguese and Ukraine students indicated that they would not take a DE course, however, almost a quarter of the UAE students indicated this.
Data indicates close to a 100% of the UAE residents use the Internet at home or on their mobile devices (Knoema, 2018 ). By contrast a smaller percentage of individuals use the Internet in Portugal and the Ukraine (Infographics, 2019 ). Internet use in each country does not seem to greatly impact UAE students’ opinions regarding DE.
Students’ perceptions of DE vary across the participants from the three countries. Portuguese and Ukrainian students rated DE more favorably than UAE students. Half of the Ukrainian students have experience with DE which might account for their favorable attitude. In contrast, in Portugal only a very small percentage of the students had experience. However, this does not seem to have negatively influenced their attitude towards DE. The interest level and engagement with new technologies by Portuguese students may help explain the favorable perception the participants had toward DE. A study by Costa, Faria, and Neto ( 2018 ) found that 90% of Portuguese students use new technologies and 69% of them use new technologies more than an hour and a half a day. Based on three European studies, Diário de Noticias ( 2011 ) stated that Portuguese students “appear at the forefront of those who best master information and communication technologies (ICT).” (para.1) Another factor influencing respondents might be that currently, and for the first time, the Portuguese government has passed a law that will regulate DE in the country. This new law will open the possibility for other IHEs to provide DE courses that lead to a degree.
Ukrainian students reported a high level of confidence in operating technological devices. The reason for this may be, in part, because of state educational requirements. Since the end of the 1990s, all Ukrainian students in secondary schools have at least one computer course as a mandatory element of their curriculum. This course covers a wide range of issues, which vary from information society theory to applied aspects of computer usage. Among the seven learning goals of this course three address digital literacy (Ministry of Education and Science of Ukraine, 2017 ). Ukrainian students who responded to the survey have taken computer courses for at least 5 years.
In the UAE, most DE courses and programs are not accredited by the Ministry of Education (United Arab Emirates Ministry of Education, 2016 ), which may account for UAE students lack of experience and their inability to judge this type of instruction.
It is worth analyzing the reasons why students enrolled or would enroll in DE courses. The reasons for taking DE courses, such as time management issues, are supported by studies concerning self-regulation and higher retention rates (Bradley, Browne, & Kelley, 2017 ; Peck, Stefaniak, & Shah, 2018 ). Students’ interest in having more control of their study time is also mentioned as one of the primary benefits of DE (Alahmari, 2017 ; Lei & Gupta, 2010 ). Regarding the reasons for not enrolling in DE courses, participants from the three countries mentioned difficulty contacting instructors and peers. Also, more than half of the students in Portugal and the UAE indicated they preferred face-to-face classes. Most students have spent their entire academic lives in traditional classes where interaction and immediate feedback from instructors and peers are more common. These concerns may be why students perceive they would lose a familiar type of interaction and have to engage with classroom participants in a new and different way (Carver & Kosloski Jr., 2015 ; Morris & Clark, 2018 ; Robinson & Hullinger, 2008 ; Summers, Waigandt, & Whittaker, 2005 ). It should be noted that the Portuguese and UAE students were enrolled in teacher education programs and are training to be face-to-face teachers. They may not understand the potential of DE format and are not preparing or expecting to use DE in their professional careers.
Difficulty being motivated was another reason chosen by the participants of the three countries to not enroll in DE courses. The lack of experience in this type of educational format may help explain student lack of confidence with their ability to study and stay on task. This response contrasts with the reasons reported for enrolling in DE courses such as controlling their study time. On one hand, participants like the prospect of having the ability to manage their own time. On the other hand, they are concerned they may lack the discipline they need to be successful.
Although the literature indicates that access to technology, isolation and expense are reasons frequently cited as preventing students from enrolling in DE courses (Lei & Gupta, 2010 ; Venter, 2003 ; Zuhairi et al., 2006 ), these reasons were selected by a very small percentage of the participants of this study. Access and affordability of technology has rapidly increased over the last decade which may help explain this inconsistency. Students may understand that DE courses are now less expensive than traditional university courses (Piletic, 2018 ) and they do not cite this as a reason for not enrolling. Relatively few students indicated they would feel isolated. Since this generation is in constant communication using technology (Diário de Notícias, 2011 ) they may not associate DE learning with isolation. However, it is interesting to note that there was a greater concern for interacting with instructors and peers than isolation.
The Ukrainian students are the most receptive to enrolling in DE courses. This is consistent with their positive perception of this type of learning. In addition, the previous experience of half of the participants may influence their interest as well as encourage their peers’ receptivity. UAE students do not have much experience and fewer than half are open to enrolling in DE courses. This may be due to their lack of experience and other concerns previously mentioned. Only one third of the Portuguese participants indicated their interest in enrolling in DE courses. This is in contrast with almost two thirds saying they had a favorable or very favorable attitude. The reasons for this inconsistency are not evident.
In terms of preparation needed to take DE courses, technical concerns were less of an issue for the participants of all three countries than skills and behaviors. Most participants’ answers focused on student skills including computer, English language and time management. Behaviors such as developing a study plan, having learning goals and objectives and being more motivated were also mentioned. The perceived need for better English language skills was expressed by about one third of the participants, none of whom have English as their native language. English speaking countries have been dominant in DE making English the most commonly used language in online learning (Sadykova & Dautermann, 2009 ). Regarding time management, half of the Ukrainian students expressed their need for improvement in contrast to approximately one third of the participants from the other countries. The difference among responses may be because the Ukrainian students are more self-reflective, or the others are more disciplined. Although both DE and face-to-face courses have deadlines for tasks and assessments, in the face-to-face courses, students meet in person with their instructors who may support and press them to do their work. Lack of in person contact may account for the participants feeling they need to improve these skills when taking DE courses (De Paepe et al., 2018 ). Students expressed concerns about lacking certain skills and having certain behaviors that would lead them to be reluctant to enroll in DE courses. The need for help and preparation are some of the concerns that participants reported. Perceived needs may account for the students’ apprehensions regarding taking DE courses. To promote this type of instruction, IHEs could address students’ concerns (Mahlangu, 2018 ).
Open schedule and blended learning courses were the two preferred formats stated by the participants. The reason that Open schedule is the most popular may be that it provides more freedom than other types of courses. Blended learning offers the familiar face-to-face instruction and some of the conveniences of DE which may be why participants are interested in this model.
Studies regarding the use of MOOCs in all three countries have been conducted indicating that researchers in these locations are aware that this course format is of potential interest to local students (Eppard & Reddy, 2017 ; Gallacher, 2014 ; Gonçalves, Chumbo, Torres, & Gonçalves, 2016 ; Sharov, Liapunova, & Sharova, 2019 ; Strutynska & Umryk, 2016 ). Ukrainian students selected MOOCs much more than students in the other countries. The reason for this may be that these students are more knowledgeable about MOOCs, because this type of course is usually at no cost and/or offered by prestigious IHEs (Cormier et al., 2010 ). However, this study did not ask why students were interested in MOOCs or other types of DE courses.
Limitations and future research
While this study offers useful information regarding undergraduate students’ perception and receptivity in taking DE courses, it has limited generalizability because of the size of the sample and the type of statistical analysis performed. Participants from two of the countries were enrolled in teacher education programs and were primarily female, thus future studies would benefit from including more students in diverse programs and a more equitable gender distribution.
Since many IHEs also offer programs for graduate students it would be useful to survey these students about their opinion and availability to enroll in DE courses. This would provide additional information for IHEs that are interested in developing DE programs.
There were some inconsistencies in the students’ responses such as Portuguese students’ interest in enrolling in DE courses not matching their favorable/ very favorable attitude towards DE. It would be helpful to conduct future research regarding this and other inconsistencies.
A study is currently being planned to collect data that will provide a larger and more diverse sample and include additional IHEs. This future research will potentially increase the available knowledge on how to provide DE for a greater number of students.
Conclusion and recommendations
Further development of DE courses and programs at IHEs in countries such as Portugal, UAE and Ukraine have good prospects. The students’ primary concerns regarding taking DE courses were similar among the three countries. These concerns included time management, motivation, and English language skills. However, this did not totally diminish participants interest in taking online courses especially for the Ukrainian students.
Based on this research, there are some obstacles that can be addressed to support the expansion of DE in the three countries that were studied and in other countries. The following recommendations may assist IHEs in promoting DE.
Recommendations for preparation within IHEs
IHEs can take proactive steps to prepare DE offerings, however, a one-size fit all model may not be appropriate for all countries and IHEs. Each institution needs to develop their own plan that meets the needs of their students and faculty. Data from this pilot study and the literature (Elbaum, McIntyre, & Smith, 2002 ; Hashim & Tasir, 2014 ; Hux et al., 2018 ) suggest that following steps might be taken:
Assess readiness to take DE courses through a survey and have students speak with counselors.
Provide pre-DE courses to build skills and behaviors based on students’ concerns.
Train instructors to develop and deliver DE courses that help to overcome obstacles such as motivation and time management.
Offer courses in a blended learning format to familiarize students with online learning which may provide a transitional model.
Recommendations for IHE outreach
This study shows that there is some student interest in enrolling in online courses. It is not sufficient for IHEs to make changes internally within their own institution. IHEs need to develop external strategies and actions that help advance the development of DE:
Promote DE in social media to target potential students and encourage them to take courses.
Urge government agencies to accredit DE courses and programs.
This pilot study provides some background information that may help IHEs to offer DE courses. Additional research about students’ preferences and needs regarding DE should be conducted. The sample size, IHEs included and participating countries could be expanded in order to gain a greater understanding.
Different cultural characteristics need to be taken into account in the development of online courses and programs. DE is being increasingly included by IHEs all around the world. To stay current, universities will need to find ways to offer DE to their current and prospective students.
Acknowledgements
Not applicable.
This research was not funded.
Author information
Authors and affiliations.
Curriculum and Instruction Division, Emirates College for Advanced Education, Abu Dhabi, United Arab Emirates
Patricia Fidalgo
Educational Technology Division, Lesley University, Cambridge, MA, USA
Joan Thormann
Philosophy Department, Oles Honchar Dnipro National University, Dnipropetrovs’ka oblast, Ukraine
Oleksandr Kulyk
Department of Curricular Studies and Educational Technology, University of Minho, Braga, Portugal
José Alberto Lencastre
You can also search for this author in PubMed Google Scholar
Contributions
Patricia Fidalgo: design of the work, data collection, analysis, interpretation of data, and draft of the work. Joan Thormann: design of the work, analysis, interpretation of data, and draft of the work. Oleksandr Kulyk: data collection, interpretation of data, and draft of the work. José Alberto Lencastre: data collection. The author(s) read and approved the final manuscript.
Corresponding author
Correspondence to Patricia Fidalgo .
Ethics declarations
Competing interests.
The authors declare that they have no competing interests.
Additional information
Publisher’s note.
Springer Nature remains neutral with regard to jurisdictional claims in published maps and institutional affiliations.
Online Survey Questions
1. If the students have taken any distance education courses previously and if yes, how many;
2. What are the students’ perceptions of distance education;
3. What are the reasons students would enroll in distance education courses;
4. What are the reasons students would not enroll in a distance education course;
5. What preparation do students feel they need before taking distance education courses;
6. What is the level of students’ interest towards enrolling in distance education courses;
7. What types of distance education would students be interested in trying;
8. What is the students’ age;
9. What is the students’ gender;
10. How confident do students feel using a computer and the Internet.
Rights and permissions
Open Access This article is licensed under a Creative Commons Attribution 4.0 International License, which permits use, sharing, adaptation, distribution and reproduction in any medium or format, as long as you give appropriate credit to the original author(s) and the source, provide a link to the Creative Commons licence, and indicate if changes were made. The images or other third party material in this article are included in the article's Creative Commons licence, unless indicated otherwise in a credit line to the material. If material is not included in the article's Creative Commons licence and your intended use is not permitted by statutory regulation or exceeds the permitted use, you will need to obtain permission directly from the copyright holder. To view a copy of this licence, visit http://creativecommons.org/licenses/by/4.0/ .
Reprints and permissions
About this article
Cite this article.
Fidalgo, P., Thormann, J., Kulyk, O. et al. Students’ perceptions on distance education: A multinational study. Int J Educ Technol High Educ 17 , 18 (2020). https://doi.org/10.1186/s41239-020-00194-2
Download citation
Received : 11 December 2019
Accepted : 18 March 2020
Published : 20 May 2020
DOI : https://doi.org/10.1186/s41239-020-00194-2
Share this article
Anyone you share the following link with will be able to read this content:
Sorry, a shareable link is not currently available for this article.
Provided by the Springer Nature SharedIt content-sharing initiative
- Distance education
- Multinational study
- Perceptions of distance education
- Undergraduate students
Thank you for visiting nature.com. You are using a browser version with limited support for CSS. To obtain the best experience, we recommend you use a more up to date browser (or turn off compatibility mode in Internet Explorer). In the meantime, to ensure continued support, we are displaying the site without styles and JavaScript.
- View all journals
- My Account Login
- Explore content
- About the journal
- Publish with us
- Sign up for alerts
- Open access
- Published: 09 January 2024
Online vs in-person learning in higher education: effects on student achievement and recommendations for leadership
- Bandar N. Alarifi 1 &
- Steve Song 2
Humanities and Social Sciences Communications volume 11 , Article number: 86 ( 2024 ) Cite this article
6681 Accesses
1 Citations
2 Altmetric
Metrics details
- Science, technology and society
This study is a comparative analysis of online distance learning and traditional in-person education at King Saud University in Saudi Arabia, with a focus on understanding how different educational modalities affect student achievement. The justification for this study lies in the rapid shift towards online learning, especially highlighted by the educational changes during the COVID-19 pandemic. By analyzing the final test scores of freshman students in five core courses over the 2020 (in-person) and 2021 (online) academic years, the research provides empirical insights into the efficacy of online versus traditional education. Initial observations suggested that students in online settings scored lower in most courses. However, after adjusting for variables like gender, class size, and admission scores using multiple linear regression, a more nuanced picture emerged. Three courses showed better performance in the 2021 online cohort, one favored the 2020 in-person group, and one was unaffected by the teaching format. The study emphasizes the crucial need for a nuanced, data-driven strategy in integrating online learning within higher education systems. It brings to light the fact that the success of educational methodologies is highly contingent on specific contextual factors. This finding advocates for educational administrators and policymakers to exercise careful and informed judgment when adopting online learning modalities. It encourages them to thoroughly evaluate how different subjects and instructional approaches might interact with online formats, considering the variable effects these might have on learning outcomes. This approach ensures that decisions about implementing online education are made with a comprehensive understanding of its diverse and context-specific impacts, aiming to optimize educational effectiveness and student success.
Similar content being viewed by others
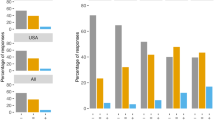
Elementary school teachers’ perspectives about learning during the COVID-19 pandemic
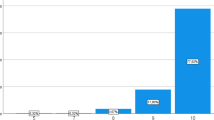
Quality of a master’s degree in education in Ecuador
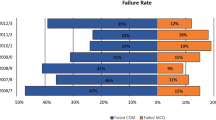
Impact of video-based learning in business statistics: a longitudinal study
Introduction.
The year 2020 marked an extraordinary period, characterized by the global disruption caused by the COVID-19 pandemic. Governments and institutions worldwide had to adapt to unforeseen challenges across various domains, including health, economy, and education. In response, many educational institutions quickly transitioned to distance teaching (also known as e-learning, online learning, or virtual classrooms) to ensure continued access to education for their students. However, despite this rapid and widespread shift to online learning, a comprehensive examination of its effects on student achievement in comparison to traditional in-person instruction remains largely unexplored.
In research examining student outcomes in the context of online learning, the prevailing trend is the consistent observation that online learners often achieve less favorable results when compared to their peers in traditional classroom settings (e.g., Fischer et al., 2020 ; Bettinger et al., 2017 ; Edvardsson and Oskarsson, 2008 ). However, it is important to note that a significant portion of research on online learning has primarily focused on its potential impact (Kuhfeld et al., 2020 ; Azevedo et al., 2020 ; Di Pietro et al., 2020 ) or explored various perspectives (Aucejo et al., 2020 ; Radha et al., 2020 ) concerning distance education. These studies have often omitted a comprehensive and nuanced examination of its concrete academic consequences, particularly in terms of test scores and grades.
Given the dearth of research on the academic impact of online learning, especially in light of Covid-19 in the educational arena, the present study aims to address that gap by assessing the effectiveness of distance learning compared to in-person teaching in five required freshmen-level courses at King Saud University, Saudi Arabia. To accomplish this objective, the current study compared the final exam results of 8297 freshman students who were enrolled in the five courses in person in 2020 to their 8425 first-year counterparts who has taken the same courses at the same institution in 2021 but in an online format.
The final test results of the five courses (i.e., University Skills 101, Entrepreneurship 101, Computer Skills 101, Computer Skills 101, and Fitness and Health Culture 101) were examined, accounting for potential confounding factors such as gender, class size and admission scores, which have been cited in past research to be correlated with student achievement (e.g., Meinck and Brese, 2019 ; Jepsen, 2015 ) Additionally, as the preparatory year at King Saud University is divided into five tracks—health, nursing, science, business, and humanity, the study classified students based on their respective disciplines.
Motivation for the study
The rapid expansion of distance learning in higher education, particularly highlighted during the recent COVID-19 pandemic (Volk et al., 2020 ; Bettinger et al., 2017 ), underscores the need for alternative educational approaches during crises. Such disruptions can catalyze innovation and the adoption of distance learning as a contingency plan (Christensen et al., 2015 ). King Saud University, like many institutions worldwide, faced the challenge of transitioning abruptly to online learning in response to the pandemic.
E-learning has gained prominence in higher education due to technological advancements, offering institutions a competitive edge (Valverde-Berrocoso et al., 2020 ). Especially during conditions like the COVID-19 pandemic, electronic communication was utilized across the globe as a feasible means to overcome barriers and enhance interactions (Bozkurt, 2019 ).
Distance learning, characterized by flexibility, became crucial when traditional in-person classes are hindered by unforeseen circumstance such as the ones posed by COVID-19 (Arkorful and Abaidoo, 2015 ). Scholars argue that it allows students to learn at their own pace, often referred to as self-directed learning (Hiemstra, 1994 ) or self-education (Gadamer, 2001 ). Additional advantages include accessibility, cost-effectiveness, and flexibility (Sadeghi, 2019 ).
However, distance learning is not immune to its own set of challenges. Technical impediments, encompassing network issues, device limitations, and communication hiccups, represent formidable hurdles (Sadeghi, 2019 ). Furthermore, concerns about potential distractions in the online learning environment, fueled by the ubiquity of the internet and social media, have surfaced (Hall et al., 2020 ; Ravizza et al., 2017 ). The absence of traditional face-to-face interactions among students and between students and instructors is also viewed as a potential drawback (Sadeghi, 2019 ).
Given the evolving understanding of the pros and cons of distance learning, this study aims to contribute to the existing literature by assessing the effectiveness of distance learning, specifically in terms of student achievement, as compared to in-person classroom learning at King Saud University, one of Saudi Arabia’s largest higher education institutions.
Academic achievement: in-person vs online learning
The primary driving force behind the rapid integration of technology in education has been its emphasis on student performance (Lai and Bower, 2019 ). Over the past decade, numerous studies have undertaken comparisons of student academic achievement in online and in-person settings (e.g., Bettinger et al., 2017 ; Fischer et al., 2020 ; Iglesias-Pradas et al., 2021 ). This section offers a concise review of the disparities in academic achievement between college students engaged in in-person and online learning, as identified in existing research.
A number of studies point to the superiority of traditional in-person education over online learning in terms of academic outcomes. For example, Fischer et al. ( 2020 ) conducted a comprehensive study involving 72,000 university students across 433 subjects, revealing that online students tend to achieve slightly lower academic results than their in-class counterparts. Similarly, Bettinger et al. ( 2017 ) found that students at for-profit online universities generally underperformed when compared to their in-person peers. Supporting this trend, Figlio et al. ( 2013 ) indicated that in-person instruction consistently produced better results, particularly among specific subgroups like males, lower-performing students, and Hispanic learners. Additionally, Kaupp’s ( 2012 ) research in California community colleges demonstrated that online students faced lower completion and success rates compared to their traditional in-person counterparts (Fig. 1 ).
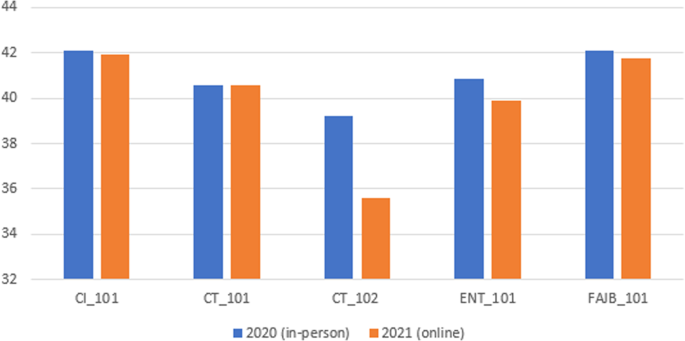
The figure compared student achievement in the final tests in the five courses by year, using independent-samples t-tests; the results show a statistically-significant drop in test scores from 2020 (in person) to 2021 (online) for all courses except CT_101.
In contrast, other studies present evidence of online students outperforming their in-person peers. For example, Iglesias-Pradas et al. ( 2021 ) conducted a comparative analysis of 43 bachelor courses at Telecommunication Engineering College in Malaysia, revealing that online students achieved higher academic outcomes than their in-person counterparts. Similarly, during the COVID-19 pandemic, Gonzalez et al. ( 2020 ) found that students engaged in online learning performed better than those who had previously taken the same subjects in traditional in-class settings.
Expanding on this topic, several studies have reported mixed results when comparing the academic performance of online and in-person students, with various student and instructor factors emerging as influential variables. Chesser et al. ( 2020 ) noted that student traits such as conscientiousness, agreeableness, and extraversion play a substantial role in academic achievement, regardless of the learning environment—be it traditional in-person classrooms or online settings. Furthermore, Cacault et al. ( 2021 ) discovered that online students with higher academic proficiency tend to outperform those with lower academic capabilities, suggesting that differences in students’ academic abilities may impact their performance. In contrast, Bergstrand and Savage ( 2013 ) found that online classes received lower overall ratings and exhibited a less respectful learning environment when compared to in-person instruction. Nevertheless, they also observed that the teaching efficiency of both in-class and online courses varied significantly depending on the instructors’ backgrounds and approaches. These findings underscore the multifaceted nature of the online vs. in-person learning debate, highlighting the need for a nuanced understanding of the factors at play.
Theoretical framework
Constructivism is a well-established learning theory that places learners at the forefront of their educational experience, emphasizing their active role in constructing knowledge through interactions with their environment (Duffy and Jonassen, 2009 ). According to constructivist principles, learners build their understanding by assimilating new information into their existing cognitive frameworks (Vygotsky, 1978 ). This theory highlights the importance of context, active engagement, and the social nature of learning (Dewey, 1938 ). Constructivist approaches often involve hands-on activities, problem-solving tasks, and opportunities for collaborative exploration (Brooks and Brooks, 1999 ).
In the realm of education, subject-specific pedagogy emerges as a vital perspective that acknowledges the distinctive nature of different academic disciplines (Shulman, 1986 ). It suggests that teaching methods should be tailored to the specific characteristics of each subject, recognizing that subjects like mathematics, literature, or science require different approaches to facilitate effective learning (Shulman, 1987 ). Subject-specific pedagogy emphasizes that the methods of instruction should mirror the ways experts in a particular field think, reason, and engage with their subject matter (Cochran-Smith and Zeichner, 2005 ).
When applying these principles to the design of instruction for online and in-person learning environments, the significance of adapting methods becomes even more pronounced. Online learning often requires unique approaches due to its reliance on technology, asynchronous interactions, and potential for reduced social presence (Anderson, 2003 ). In-person learning, on the other hand, benefits from face-to-face interactions and immediate feedback (Allen and Seaman, 2016 ). Here, the interplay of constructivism and subject-specific pedagogy becomes evident.
Online learning. In an online environment, constructivist principles can be upheld by creating interactive online activities that promote exploration, reflection, and collaborative learning (Salmon, 2000 ). Discussion forums, virtual labs, and multimedia presentations can provide opportunities for students to actively engage with the subject matter (Harasim, 2017 ). By integrating subject-specific pedagogy, educators can design online content that mirrors the discipline’s methodologies while leveraging technology for authentic experiences (Koehler and Mishra, 2009 ). For instance, an online history course might incorporate virtual museum tours, primary source analysis, and collaborative timeline projects.
In-person learning. In a traditional brick-and-mortar classroom setting, constructivist methods can be implemented through group activities, problem-solving tasks, and in-depth discussions that encourage active participation (Jonassen et al., 2003 ). Subject-specific pedagogy complements this by shaping instructional methods to align with the inherent characteristics of the subject (Hattie, 2009). For instance, in a physics class, hands-on experiments and real-world applications can bring theoretical concepts to life (Hake, 1998 ).
In sum, the fusion of constructivism and subject-specific pedagogy offers a versatile approach to instructional design that adapts to different learning environments (Garrison, 2011 ). By incorporating the principles of both theories, educators can tailor their methods to suit the unique demands of online and in-person learning, ultimately providing students with engaging and effective learning experiences that align with the nature of the subject matter and the mode of instruction.
Course description
The Self-Development Skills Department at King Saud University (KSU) offers five mandatory freshman-level courses. These courses aim to foster advanced thinking skills and cultivate scientific research abilities in students. They do so by imparting essential skills, identifying higher-level thinking patterns, and facilitating hands-on experience in scientific research. The design of these classes is centered around aiding students’ smooth transition into university life. Brief descriptions of these courses are as follows:
University Skills 101 (CI 101) is a three-hour credit course designed to nurture essential academic, communication, and personal skills among all preparatory year students at King Saud University. The primary goal of this course is to equip students with the practical abilities they need to excel in their academic pursuits and navigate their university lives effectively. CI 101 comprises 12 sessions and is an integral part of the curriculum for all incoming freshmen, ensuring a standardized foundation for skill development.
Fitness and Health 101 (FAJB 101) is a one-hour credit course. FAJB 101 focuses on the aspects of self-development skills in terms of health and physical, and the skills related to personal health, nutrition, sports, preventive, psychological, reproductive, and first aid. This course aims to motivate students’ learning process through entertainment, sports activities, and physical exercises to maintain their health. This course is required for all incoming freshmen students at King Saud University.
Entrepreneurship 101 (ENT 101) is a one-hour- credit course. ENT 101 aims to develop students’ skills related to entrepreneurship. The course provides students with knowledge and skills to generate and transform ideas and innovations into practical commercial projects in business settings. The entrepreneurship course consists of 14 sessions and is taught only to students in the business track.
Computer Skills 101 (CT 101) is a three-hour credit course. This provides students with the basic computer skills, e.g., components, operating systems, applications, and communication backup. The course explores data visualization, introductory level of modern programming with algorithms and information security. CT 101 course is taught for all tracks except those in the human track.
Computer Skills 102 (CT 102) is a three-hour credit course. It provides IT skills to the students to utilize computers with high efficiency, develop students’ research and scientific skills, and increase capability to design basic educational software. CT 102 course focuses on operating systems such as Microsoft Office. This course is only taught for students in the human track.
Structure and activities
These courses ranged from one to three hours. A one-hour credit means that students must take an hour of the class each week during the academic semester. The same arrangement would apply to two and three credit-hour courses. The types of activities in each course are shown in Table 1 .
At King Saud University, each semester spans 15 weeks in duration. The total number of semester hours allocated to each course serves as an indicator of its significance within the broader context of the academic program, including the diverse tracks available to students. Throughout the two years under study (i.e., 2020 and 2021), course placements (fall or spring), course content, and the organizational structure remained consistent and uniform.
Participants
The study’s data comes from test scores of a cohort of 16,722 first-year college students enrolled at King Saud University in Saudi Arabia over the span of two academic years: 2020 and 2021. Among these students, 8297 were engaged in traditional, in-person learning in 2020, while 8425 had transitioned to online instruction for the same courses in 2021 due to the Covid-19 pandemic. In 2020, the student population consisted of 51.5% females and 48.5% males. However, in 2021, there was a reversal in these proportions, with female students accounting for 48.5% and male students comprising 51.5% of the total participants.
Regarding student enrollment in the five courses, Table 2 provides a detailed breakdown by average class size, admission scores, and the number of students enrolled in the courses during the two years covered by this study. While the total number of students in each course remained relatively consistent across the two years, there were noticeable fluctuations in average class sizes. Specifically, four out of the five courses experienced substantial increases in class size, with some nearly doubling in size (e.g., ENT_101 and CT_102), while one course (CT_101) showed a reduction in its average class size.
In this study, it must be noted that while some students enrolled in up to three different courses within the same academic year, none repeated the same exam in both years. Specifically, students who failed to pass their courses in 2020 were required to complete them in summer sessions and were consequently not included in this study’s dataset. To ensure clarity and precision in our analysis, the research focused exclusively on student test scores to evaluate and compare the academic effectiveness of online and traditional in-person learning methods. This approach was chosen to provide a clear, direct comparison of the educational impacts associated with each teaching format.
Descriptive analysis of the final exam scores for the two years (2020 and 2021) were conducted. Additionally, comparison of student outcomes in in-person classes in 2020 to their online platform peers in 2021 were conducted using an independent-samples t -test. Subsequently, in order to address potential disparities between the two groups arising from variables such as gender, class size, and admission scores (which serve as an indicator of students’ academic aptitude and pre-enrollment knowledge), multiple regression analyses were conducted. In these multivariate analyses, outcomes of both in-person and online cohorts were assessed within their respective tracks. By carefully considering essential aforementioned variables linked to student performance, the study aimed to ensure a comprehensive and equitable evaluation.
Study instrument
The study obtained students’ final exam scores for the years 2020 (in-person) and 2021 (online) from the school’s records office through their examination management system. In the preparatory year at King Saud University, final exams for all courses are developed by committees composed of faculty members from each department. To ensure valid comparisons, the final exam questions, crafted by departmental committees of professors, remained consistent and uniform for the two years under examination.
Table 3 provides a comprehensive assessment of the reliability of all five tests included in our analysis. These tests exhibit a strong degree of internal consistency, with Cronbach’s alpha coefficients spanning a range from 0.77 to 0.86. This robust and consistent internal consistency measurement underscores the dependable nature of these tests, affirming their reliability and suitability for the study’s objectives.
In terms of assessing test validity, content validity was ensured through a thorough review by university subject matter experts, resulting in test items that align well with the content domain and learning objectives. Additionally, criterion-related validity was established by correlating students’ admissions test scores with their final required freshman test scores in the five subject areas, showing a moderate and acceptable relationship (0.37 to 0.56) between the test scores and the external admissions test. Finally, construct validity was confirmed through reviews by experienced subject instructors, leading to improvements in test content. With guidance from university subject experts, construct validity was established, affirming the effectiveness of the final tests in assessing students’ subject knowledge at the end of their coursework.
Collectively, these validity and reliability measures affirm the soundness and integrity of the final subject tests, establishing their suitability as effective assessment tools for evaluating students’ knowledge in their five mandatory freshman courses at King Saud University.
After obtaining research approval from the Research Committee at King Saud University, the coordinators of the five courses (CI_101, ENT_101, CT_101, CT_102, and FAJB_101) supplied the researchers with the final exam scores of all first-year preparatory year students at King Saud University for the initial semester of the academic years 2020 and 2021. The sample encompassed all students who had completed these five courses during both years, resulting in a total of 16,722 students forming the final group of participants.
Limitations
Several limitations warrant acknowledgment in this study. First, the research was conducted within a well-resourced major public university. As such, the experiences with online classes at other types of institutions (e.g., community colleges, private institutions) may vary significantly. Additionally, the limited data pertaining to in-class teaching practices and the diversity of learning activities across different courses represents a gap that could have provided valuable insights for a more thorough interpretation and explanation of the study’s findings.
To compare student achievement in the final tests in the five courses by year, independent-samples t -tests were conducted. Table 4 shows a statistically-significant drop in test scores from 2020 (in person) to 2021 (online) for all courses except CT_101. The biggest decline was with CT_102 with 3.58 points, and the smallest decline was with CI_101 with 0.18 points.
However, such simple comparison of means between the two years (via t -tests) by subjects does not account for the differences in gender composition, class size, and admission scores between the two academic years, all of which have been associated with student outcomes (e.g., Ho and Kelman, 2014 ; De Paola et al., 2013 ). To account for such potential confounding variables, multiple regressions were conducted to compare the 2 years’ results while controlling for these three factors associated with student achievement.
Table 5 presents the regression results, illustrating the variation in final exam scores between 2020 and 2021, while controlling for gender, class size, and admission scores. Importantly, these results diverge significantly from the outcomes obtained through independent-sample t -test analyses.
Taking into consideration the variables mentioned earlier, students in the 2021 online cohort demonstrated superior performance compared to their 2020 in-person counterparts in CI_101, FAJB_101, and CT_101, with score advantages of 0.89, 0.56, and 5.28 points, respectively. Conversely, in the case of ENT_101, online students in 2021 scored 0.69 points lower than their 2020 in-person counterparts. With CT_102, there were no statistically significant differences in final exam scores between the two cohorts of students.
The study sought to assess the effectiveness of distance learning compared to in-person learning in the higher education setting in Saudi Arabia. We analyzed the final exam scores of 16,722 first-year college students in King Saud University in five required subjects (i.e., CI_101, ENT_101, CT_101, CT_102, and FAJB_101). The study initially performed a simple comparison of mean scores by tracks by year (via t -tests) and then a number of multiple regression analyses which controlled for class size, gender composition, and admission scores.
Overall, the study’s more in-depth findings using multiple regression painted a wholly different picture than the results obtained using t -tests. After controlling for class size, gender composition, and admissions scores, online students in 2021 performed better than their in-person instruction peers in 2020 in University Skills (CI_101), Fitness and Health (FAJB_101), and Computer Skills (CT_101), whereas in-person students outperformed their online peers in Entrepreneurship (ENT_101). There was no meaningful difference in outcomes for students in the Computer Skills (CT_102) course for the two years.
In light of these findings, it raises the question: why do we observe minimal differences (less than a one-point gain or loss) in student outcomes in courses like University Skills, Fitness and Health, Entrepreneurship, and Advanced Computer Skills based on the mode of instruction? Is it possible that when subjects are primarily at a basic or introductory level, as is the case with these courses, the mode of instruction may have a limited impact as long as the concepts are effectively communicated in a manner familiar and accessible to students?
In today’s digital age, one could argue that students in more developed countries, such as Saudi Arabia, generally possess the skills and capabilities to effectively engage with materials presented in both in-person and online formats. However, there is a notable exception in the Basic Computer Skills course, where the online cohort outperformed their in-person counterparts by more than 5 points. Insights from interviews with the instructors of this course suggest that this result may be attributed to the course’s basic and conceptual nature, coupled with the availability of instructional videos that students could revisit at their own pace.
Given that students enter this course with varying levels of computer skills, self-paced learning may have allowed them to cover course materials at their preferred speed, concentrating on less familiar topics while swiftly progressing through concepts they already understood. The advantages of such self-paced learning have been documented by scholars like Tullis and Benjamin ( 2011 ), who found that self-paced learners often outperform those who spend the same amount of time studying identical materials. This approach allows learners to allocate their time more effectively according to their individual learning pace, providing greater ownership and control over their learning experience. As such, in courses like introductory computer skills, it can be argued that becoming familiar with fundamental and conceptual topics may not require extensive in-class collaboration. Instead, it may be more about exposure to and digestion of materials in a format and at a pace tailored to students with diverse backgrounds, knowledge levels, and skill sets.
Further investigation is needed to more fully understand why some classes benefitted from online instruction while others did not, and vice versa. Perhaps, it could be posited that some content areas are more conducive to in-person (or online) format while others are not. Or it could be that the different results of the two modes of learning were driven by students of varying academic abilities and engagement, with low-achieving students being more vulnerable to the limitations of online learning (e.g., Kofoed et al., 2021 ). Whatever the reasons, the results of the current study can be enlightened by a more in-depth analysis of the various factors associated with such different forms of learning. Moreover, although not clear cut, what the current study does provide is additional evidence against any dire consequences to student learning (at least in the higher ed setting) as a result of sudden increase in online learning with possible benefits of its wider use being showcased.
Based on the findings of this study, we recommend that educational leaders adopt a measured approach to online learning—a stance that neither fully embraces nor outright denounces it. The impact on students’ experiences and engagement appears to vary depending on the subjects and methods of instruction, sometimes hindering, other times promoting effective learning, while some classes remain relatively unaffected.
Rather than taking a one-size-fits-all approach, educational leaders should be open to exploring the nuances behind these outcomes. This involves examining why certain courses thrived with online delivery, while others either experienced a decline in student achievement or remained largely unaffected. By exploring these differentiated outcomes associated with diverse instructional formats, leaders in higher education institutions and beyond can make informed decisions about resource allocation. For instance, resources could be channeled towards in-person learning for courses that benefit from it, while simultaneously expanding online access for courses that have demonstrated improved outcomes through its virtual format. This strategic approach not only optimizes resource allocation but could also open up additional revenue streams for the institution.
Considering the enduring presence of online learning, both before the pandemic and its accelerated adoption due to Covid-19, there is an increasing need for institutions of learning and scholars in higher education, as well as other fields, to prioritize the study of its effects and optimal utilization. This study, which compares student outcomes between two cohorts exposed to in-person and online instruction (before and during Covid-19) at the largest university in Saudi Arabia, represents a meaningful step in this direction.
Data availability
The datasets generated during and/or analyzed during the current study are available from the corresponding author upon reasonable request.
Allen IE, Seaman J (2016) Online report card: Tracking online education in the United States . Babson Survey Group
Anderson T (2003) Getting the mix right again: an updated and theoretical rationale for interaction. Int Rev Res Open Distrib Learn , 4 (2). https://doi.org/10.19173/irrodl.v4i2.149
Arkorful V, Abaidoo N (2015) The role of e-learning, advantages and disadvantages of its adoption in higher education. Int J Instruct Technol Distance Learn 12(1):29–42
Google Scholar
Aucejo EM, French J, Araya MP, Zafar B (2020) The impact of COVID-19 on student experiences and expectations: Evidence from a survey. Journal of Public Economics 191:104271. https://doi.org/10.1016/j.jpubeco.2020.104271
Article PubMed PubMed Central Google Scholar
Azevedo JP, Hasan A, Goldemberg D, Iqbal SA, and Geven K (2020) Simulating the potential impacts of COVID-19 school closures on schooling and learning outcomes: a set of global estimates. World Bank Policy Research Working Paper
Bergstrand K, Savage SV (2013) The chalkboard versus the avatar: Comparing the effectiveness of online and in-class courses. Teach Sociol 41(3):294–306. https://doi.org/10.1177/0092055X13479949
Article Google Scholar
Bettinger EP, Fox L, Loeb S, Taylor ES (2017) Virtual classrooms: How online college courses affect student success. Am Econ Rev 107(9):2855–2875. https://doi.org/10.1257/aer.20151193
Bozkurt A (2019) From distance education to open and distance learning: a holistic evaluation of history, definitions, and theories. Handbook of research on learning in the age of transhumanism , 252–273. https://doi.org/10.4018/978-1-5225-8431-5.ch016
Brooks JG, Brooks MG (1999) In search of understanding: the case for constructivist classrooms . Association for Supervision and Curriculum Development
Cacault MP, Hildebrand C, Laurent-Lucchetti J, Pellizzari M (2021) Distance learning in higher education: evidence from a randomized experiment. J Eur Econ Assoc 19(4):2322–2372. https://doi.org/10.1093/jeea/jvaa060
Chesser S, Murrah W, Forbes SA (2020) Impact of personality on choice of instructional delivery and students’ performance. Am Distance Educ 34(3):211–223. https://doi.org/10.1080/08923647.2019.1705116
Christensen CM, Raynor M, McDonald R (2015) What is disruptive innovation? Harv Bus Rev 93(12):44–53
Cochran-Smith M, Zeichner KM (2005) Studying teacher education: the report of the AERA panel on research and teacher education. Choice Rev Online 43 (4). https://doi.org/10.5860/choice.43-2338
De Paola M, Ponzo M, Scoppa V (2013) Class size effects on student achievement: heterogeneity across abilities and fields. Educ Econ 21(2):135–153. https://doi.org/10.1080/09645292.2010.511811
Dewey, J (1938) Experience and education . Simon & Schuster
Di Pietro G, Biagi F, Costa P, Karpinski Z, Mazza J (2020) The likely impact of COVID-19 on education: reflections based on the existing literature and recent international datasets. Publications Office of the European Union, Luxembourg
Duffy TM, Jonassen DH (2009) Constructivism and the technology of instruction: a conversation . Routledge, Taylor & Francis Group
Edvardsson IR, Oskarsson GK (2008) Distance education and academic achievement in business administration: the case of the University of Akureyri. Int Rev Res Open Distrib Learn, 9 (3). https://doi.org/10.19173/irrodl.v9i3.542
Figlio D, Rush M, Yin L (2013) Is it live or is it internet? Experimental estimates of the effects of online instruction on student learning. J Labor Econ 31(4):763–784. https://doi.org/10.3386/w16089
Fischer C, Xu D, Rodriguez F, Denaro K, Warschauer M (2020) Effects of course modality in summer session: enrollment patterns and student performance in face-to-face and online classes. Internet Higher Educ 45:100710. https://doi.org/10.1016/j.iheduc.2019.100710
Gadamer HG (2001) Education is self‐education. J Philos Educ 35(4):529–538
Garrison DR (2011) E-learning in the 21st century: a framework for research and practice . Routledge. https://doi.org/10.4324/9780203838761
Gonzalez T, de la Rubia MA, Hincz KP, Comas-Lopez M, Subirats L, Fort S, & Sacha GM (2020) Influence of COVID-19 confinement on students’ performance in higher education. PLOS One 15 (10). https://doi.org/10.1371/journal.pone.0239490
Hake RR (1998) Interactive-engagement versus traditional methods: a six-thousand-student survey of mechanics test data for introductory physics courses. Am J Phys 66(1):64–74. https://doi.org/10.1119/1.18809
Article ADS Google Scholar
Hall ACG, Lineweaver TT, Hogan EE, O’Brien SW (2020) On or off task: the negative influence of laptops on neighboring students’ learning depends on how they are used. Comput Educ 153:1–8. https://doi.org/10.1016/j.compedu.2020.103901
Harasim L (2017) Learning theory and online technologies. Routledge. https://doi.org/10.4324/9780203846933
Hiemstra R (1994) Self-directed learning. In WJ Rothwell & KJ Sensenig (Eds), The sourcebook for self-directed learning (pp 9–20). HRD Press
Ho DE, Kelman MG (2014) Does class size affect the gender gap? A natural experiment in law. J Legal Stud 43(2):291–321
Iglesias-Pradas S, Hernández-García Á, Chaparro-Peláez J, Prieto JL (2021) Emergency remote teaching and students’ academic performance in higher education during the COVID-19 pandemic: a case study. Comput Hum Behav 119:106713. https://doi.org/10.1016/j.chb.2021.106713
Jepsen C (2015) Class size: does it matter for student achievement? IZA World of Labor . https://doi.org/10.15185/izawol.190
Jonassen DH, Howland J, Moore J, & Marra RM (2003) Learning to solve problems with technology: a constructivist perspective (2nd ed). Columbus: Prentice Hall
Kaupp R (2012) Online penalty: the impact of online instruction on the Latino-White achievement gap. J Appli Res Community Coll 19(2):3–11. https://doi.org/10.46569/10211.3/99362
Koehler MJ, Mishra P (2009) What is technological pedagogical content knowledge? Contemp Issues Technol Teacher Educ 9(1):60–70
Kofoed M, Gebhart L, Gilmore D, & Moschitto R (2021) Zooming to class?: Experimental evidence on college students’ online learning during COVID-19. SSRN Electron J. https://doi.org/10.2139/ssrn.3846700
Kuhfeld M, Soland J, Tarasawa B, Johnson A, Ruzek E, Liu J (2020) Projecting the potential impact of COVID-19 school closures on academic achievement. Educ Res 49(8):549–565. https://doi.org/10.3102/0013189x20965918
Lai JW, Bower M (2019) How is the use of technology in education evaluated? A systematic review. Comput Educ 133:27–42
Meinck S, Brese F (2019) Trends in gender gaps: using 20 years of evidence from TIMSS. Large-Scale Assess Educ 7 (1). https://doi.org/10.1186/s40536-019-0076-3
Radha R, Mahalakshmi K, Kumar VS, Saravanakumar AR (2020) E-Learning during lockdown of COVID-19 pandemic: a global perspective. Int J Control Autom 13(4):1088–1099
Ravizza SM, Uitvlugt MG, Fenn KM (2017) Logged in and zoned out: How laptop Internet use relates to classroom learning. Psychol Sci 28(2):171–180. https://doi.org/10.1177/095679761667731
Article PubMed Google Scholar
Sadeghi M (2019) A shift from classroom to distance learning: advantages and limitations. Int J Res Engl Educ 4(1):80–88
Salmon G (2000) E-moderating: the key to teaching and learning online . Routledge. https://doi.org/10.4324/9780203816684
Shulman LS (1986) Those who understand: knowledge growth in teaching. Edu Res 15(2):4–14
Shulman LS (1987) Knowledge and teaching: foundations of the new reform. Harv Educ Rev 57(1):1–22
Tullis JG, Benjamin AS (2011) On the effectiveness of self-paced learning. J Mem Lang 64(2):109–118. https://doi.org/10.1016/j.jml.2010.11.002
Valverde-Berrocoso J, Garrido-Arroyo MDC, Burgos-Videla C, Morales-Cevallos MB (2020) Trends in educational research about e-learning: a systematic literature review (2009–2018). Sustainability 12(12):5153
Volk F, Floyd CG, Shaler L, Ferguson L, Gavulic AM (2020) Active duty military learners and distance education: factors of persistence and attrition. Am J Distance Educ 34(3):1–15. https://doi.org/10.1080/08923647.2019.1708842
Vygotsky LS (1978) Mind in society: the development of higher psychological processes. Harvard University Press
Download references
Author information
Authors and affiliations.
Department of Sports and Recreation Management, King Saud University, Riyadh, Saudi Arabia
Bandar N. Alarifi
Division of Research and Doctoral Studies, Concordia University Chicago, 7400 Augusta Street, River Forest, IL, 60305, USA
You can also search for this author in PubMed Google Scholar
Contributions
Dr. Bandar Alarifi collected and organized data for the five courses and wrote the manuscript. Dr. Steve Song analyzed and interpreted the data regarding student achievement and revised the manuscript. These authors jointly supervised this work and approved the final manuscript.
Corresponding author
Correspondence to Bandar N. Alarifi .
Ethics declarations
Competing interests.
The author declares no competing interests.
Ethical approval
This study was approved by the Research Ethics Committee at King Saud University on 25 March 2021 (No. 4/4/255639). This research does not involve the collection or analysis of data that could be used to identify participants (including email addresses or other contact details). All information is anonymized and the submission does not include images that may identify the person. The procedures used in this study adhere to the tenets of the Declaration of Helsinki.
Informed consent
This article does not contain any studies with human participants performed by any of the authors.
Additional information
Publisher’s note Springer Nature remains neutral with regard to jurisdictional claims in published maps and institutional affiliations.
Supplementary information
Rights and permissions.
Open Access This article is licensed under a Creative Commons Attribution 4.0 International License, which permits use, sharing, adaptation, distribution and reproduction in any medium or format, as long as you give appropriate credit to the original author(s) and the source, provide a link to the Creative Commons license, and indicate if changes were made. The images or other third party material in this article are included in the article’s Creative Commons license, unless indicated otherwise in a credit line to the material. If material is not included in the article’s Creative Commons license and your intended use is not permitted by statutory regulation or exceeds the permitted use, you will need to obtain permission directly from the copyright holder. To view a copy of this license, visit http://creativecommons.org/licenses/by/4.0/ .
Reprints and permissions
About this article
Cite this article.
Alarifi, B.N., Song, S. Online vs in-person learning in higher education: effects on student achievement and recommendations for leadership. Humanit Soc Sci Commun 11 , 86 (2024). https://doi.org/10.1057/s41599-023-02590-1
Download citation
Received : 07 June 2023
Accepted : 21 December 2023
Published : 09 January 2024
DOI : https://doi.org/10.1057/s41599-023-02590-1
Share this article
Anyone you share the following link with will be able to read this content:
Sorry, a shareable link is not currently available for this article.
Provided by the Springer Nature SharedIt content-sharing initiative
Quick links
- Explore articles by subject
- Guide to authors
- Editorial policies

REVIEW article
Analyzing trends in online learning in higher education in the brics countries provisionally accepted.
- 1 Kazan Federal University, Russia
- 2 Financial University under the Government of the Russian Federation, Russia
- 3 MGIMO University, Russia
- 4 Peoples' Friendship University of Russia, Russia
The final, formatted version of the article will be published soon.
This paper aims to provide a holistic overview of research trends examining online learning in higher education in BRICS countries, an acronym for an association of five major emerging economies: Brazil, Russia, India, China, and South Africa, after COVID-19 using bibliometric analysis. Researchers utilized VOSviewer's software to analyze the data using a bibliometric approach. 971 publications from the SCOPUS database were found appropriate for this research based on the inclusion and exclusion criteria. The results of the number of documents showed that there has been an increase in the amount of research on online learning in higher education in the BRICS countries. Also, the results showed that researchers from China and South Africa had the highest publications on online learning. However, researchers in Russia had very few publications. In addition, the co-authorship relationships among the BRICS countries revealed specific relationships of scholars with their colleagues from other countries. The results revealed that scholars from Indian and South African researchers had close collaborations, and Russian researchers did not have any collaborations with other countries. Finally, the results revealed two lines of research trends. The first line of research has focused on e-learning, online learning, students, and teaching. The second line of COVID-19 has focused on the pandemic, learning, online education, and students. Based on the results, recommendations are made for further research and higher education.
Keywords: Online Learning, higher education, BRICS countries, Bibliometric, Distance Education
Received: 29 Mar 2024; Accepted: 08 May 2024.
Copyright: © 2024 Masalimova, Orekhovskaya, Pivovarov, Borovikova, Zhirkova and Chauzova. This is an open-access article distributed under the terms of the Creative Commons Attribution License (CC BY) . The use, distribution or reproduction in other forums is permitted, provided the original author(s) or licensor are credited and that the original publication in this journal is cited, in accordance with accepted academic practice. No use, distribution or reproduction is permitted which does not comply with these terms.
* Correspondence: Prof. Alfiya R. Masalimova, Kazan Federal University, Kazan, Russia
People also looked at
COVID-19 : 10 Recommendations to plan distance learning solutions

- Examine the readiness and choose the most relevant tools.
Decide on the use high-technology and low-technology solutions based on the reliability of local power supplies, internet connectivity, and digital skills of teachers and students. This could range through integrated digital learning platforms, video lessons, MOOCs, to broadcasting through radios and TVs.
- Ensure inclusion of the distance learning programmes.
Implement measures to ensure that students including those with disabilities or from low-income backgrounds have access to distance learning programmes, if only a limited number of them have access to digital devices. Consider temporarily decentralizing such devices from computer labs to families and support them with internet connectivity.
- Protect data privacy and data security.
Assess data security when uploading data or educational resources to web spaces, as well as when sharing them with other organizations or individuals. Ensure that the use of applications and platforms does not violate students’ data privacy.
- Prioritize solutions to address psychosocial challenges before teaching.
Mobilize available tools to connect schools, parents, teachers and students with each other. Create communities to ensure regular human interactions, enable social caring measures, and address possible psychosocial challenges that students may face when they are isolated.
- Plan the study schedule of the distance learning programmes.
Organize discussions with stakeholders to examine the possible duration of school closures and decide whether the distance learning programme should focus on teaching new knowledge or enhance students’ knowledge of prior lessons. Plan the schedule depending on the situation of the affected zones, level of studies, needs of students needs, and availability of parents. Choose the appropriate learning methodologies based on the status of school closures and home-based quarantines. Avoid learning methodologies that require face-to-face communication.
- Provide support to teachers and parents on the use of digital tools.
Organize brief training or orientation sessions for teachers and parents as well, if monitoring and facilitation are needed. Help teachers to prepare the basic settings such as solutions to the use of internet data if they are required to provide live streaming of lessons.
- Blend appropriate approaches and limit the number of applications and platforms.
Blend tools or media that are available for most students, both for synchronous communication and lessons, and for asynchronous learning. Avoid overloading students and parents by asking them to download and test too many applications or platforms.
- Develop distance learning rules and monitor students’ learning process.
Define the rules with parents and students on distance learning. Design formative questions, tests, or exercises to monitor closely students’ learning process. Try to use tools to support submission of students’ feedback and avoid overloading parents by requesting them to scan and send students’ feedback.
- Define the duration of distance learning units based on students’ self-regulation skills.
Keep a coherent timing according to the level of the students’ self-regulation and metacognitive abilities especially for livestreaming classes. Preferably, the unit for primary school students should not be more than 20 minutes, and no longer than 40 minutes for secondary school students.
- Create communities and enhance connection.
Create communities of teachers, parents and school managers to address sense of loneliness or helplessness, facilitate sharing of experience and discussion on coping strategies when facing learning difficulties.
- More on UNESCO’s response to Coronavirus’ impact on education

Related items
More on this subject.
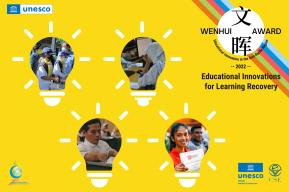
Other recent news
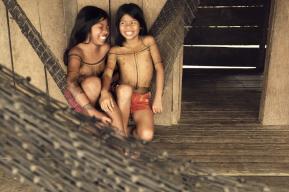

An official website of the United States government
The .gov means it’s official. Federal government websites often end in .gov or .mil. Before sharing sensitive information, make sure you’re on a federal government site.
The site is secure. The https:// ensures that you are connecting to the official website and that any information you provide is encrypted and transmitted securely.
- Publications
- Account settings
Preview improvements coming to the PMC website in October 2024. Learn More or Try it out now .
- Advanced Search
- Journal List
- Pak J Med Sci
- v.36(COVID19-S4); 2020 May
Advantages, Limitations and Recommendations for online learning during COVID-19 pandemic era
Khadijah mukhtar.
1 Khadijah Mukhtar, BDS, MME. Assistant Professor, DME. University College of Medicine and Dentistry, The University of Lahore, Lahore, Pakistan
Kainat Javed
2 Kainat Javed, MBBS, MME. Assistant Professor, DME. University College of Medicine and Dentistry, The University of Lahore, Lahore, Pakistan
Mahwish Arooj
3 Mahwish Arooj, MBBS, M. Phil, MME, PhD Physiology. Associate Professor, Physiology and Director DME, University College of Medicine and Dentistry, The University of Lahore, Lahore, Pakistan
Ahsan Sethi
4 Ahsan Sethi, BDS, MPH, MMEd, FHEA, MAcadMEd, FDTFEd, PhD Medical Education Assistant Professor, Institute of Health Professions Education and Research, Khyber Medical University, Peshawar, Pakistan
During COVID-19 pandemic, the institutions in Pakistan have started online learning. This study explores the perception of teachers and students regarding its advantages, limitations and recommendations.
This qualitative case study was conducted from March to April 2020. Using maximum variation sampling, 12 faculty members and 12 students from University College of Medicine and University College of Dentistry, Lahore were invited to participate. Four focus group interviews, two each with the faculty and students of medicine and dentistry were carried out. Data were transcribed verbatim and thematically analyzed using Atlas Ti.
The advantages included remote learning, comfort, accessibility, while the limitations involved inefficiency and difficulty in maintaining academic integrity. The recommendations were to train faculty on using online modalities and developing lesson plan with reduced cognitive load and increased interactivities.
Conclusion:
The current study supports the use of online learning in medical and dental institutes, considering its various advantages. Online learning modalities encourage student-centered learning and they are easily manageable during this lockdown situation.
INTRODUCTION
The spread of COVID-19 has led to the closure of educational institutions all over the world. This tested the preparedness of universities to deal with a crisis that requires the help of advanced technology including hardware and software to enable effective online learning. Such closure accelerated the development of the online learning environments so that learning would not be disrupted. 1 Many institutions have become interested in how to best deliver course content online, engage learners and conduct assessments. Hence, COVID-19 while being a hazard to humanity, has evolved institutions to invest in online learning.
Online learning systems are web-based software for distributing, tracking, and managing courses over the Internet. 2 It involves the implementation of advancements in technology to direct, design and deliver the learning content, and to facilitate two-way communication between students and faculty. 3 They contain features such as whiteboards, chat rooms, polls, quizzes, discussion forums and surveys that allow instructors and students to communicate online and share course content side by side. These can offer productive and convenient ways to achieve learning goals. In Pakistan, the institutions are using Microsoft Teams, Google meet, Edmodo and Moodle as learning management systems along with their applications for video conferencing. 4 Other commonly used video conferencing solutions include Zoom, Skype for business, WebEx and Adobe connect etc.
According to our literature review, three previous studies were found, 5 - 7 supporting online learning from Pakistan. The two studies at Dow University of Health Sciences, Karachi and Lahore Medical and Dental College, Lahore reported high student satisfaction with online learning modalities. The study from Khyber Pakhtunkhwa assessed the feasibility of online learning among students, trainees and faculty members. They reported good technology access, online skills, and preparedness for online discussions among participants across the medical education continuum.
With the increase in use of online modalities during COVID-19, it is necessary to assess their effectiveness with regards to teaching and learning from various stakeholders. 8 Therefore, the current study explores the perception of faculty members and students regarding the advantages, limitations and recommendations for online learning in Pakistan. The study is timely as Higher Education Commission (HEC) is in the process of implementing online learning across all the universities in Pakistan. The findings will help identify the required changes on priority basis to make it more practical and worthwhile.
This qualitative case study was conducted from March to April 2020 in two medical and dental institutes. Ethical approval for this study was taken from ethical review board of University of Lahore (Ref No. ERC/02/20/02, dated February 25, 2020). Using maximum variation sampling 12 faculty members and 12 students from University College of Medicine and University College of Dentistry, Lahore were invited to participate. In addition to learning management system ‘Moodle’, these colleges have recently adopted ‘Zoom’ for interactive teaching in small and large group formats. The participants were also involved in online Problem-Based Learning sessions, along with regular online assessments during COVID-19 pandemic.
An interview guide was developed to explore faculty and students’ perception about online learning modalities, its advantages, limitations and recommendations. The interview guide was piloted to ensure comprehensiveness and then also validated by two medical education experts. 9 Two interviewers who were not involved in teaching and assessment of students conducted four focus group interviews (n=6 in each group) with faculty members (n=12) and students (n=12) of medicine and dentistry. The faculty and students were from both basic sciences (1 st and 2 nd year) and clinical sciences (3 rd , 4 th and final year). All interviews were recorded through ‘Zoom’ and subsequently transcribed verbatim. The data were thematically analyzed: compiling, disassembling, reassembling and interpretation by all the authors independently and then corroborated to ensure analytical triangulation.
The faculty members were predominantly females from both basic and clinical sciences with age range from 30-64 years. The students were from all professional years of MBBS and BDS program ( Table-I ).
Participant characteristics.
Total six themes, two each for advantages, limitations and recommendations were extracted from the transcribed data after qualitative analysis ( Table-II ).
E-learning advantages, limitations and recommendations by Students and Faculty.
Faculty opined that online learning helped ensure remote learning, it was manageable, and students could conveniently access teachers and teaching materials. It also reduced use of traveling resources and other expenses. It eased administrative tasks such as recording of lectures and marking attendance. Both the students and teachers had an opinion that online learning modalities had encouraged student-centeredness during this lockdown situation. The student had become self-directed learners and they learnt asynchronously at any time in a day.
Limitations
Faculty members and students said that through online learning modalities they were unable to teach and learn practical and clinical work. They could only teach and assess knowledge component. Due to lack of immediate feedback, teachers were unable to assess students’ understanding during online lecturing. The students also reported limited attention span and resource intensive nature of online learning as a limitation. Some teachers also mentioned that during online study, students misbehaved and tried to access online resources during assessments.
Recommendations
Teachers and students suggested continuous faculty development. They recommended a reduction in cognitive load and increased interactivities during online teaching. Those in clinical years suggested ways to start online Case Based Learning. However, some were also of the opinion that there should be revision classes along with psychomotor hands on teaching after the COVID-19 pandemic is under control. To enhance quality, they suggested buying premium software and other proctoring software to detect cheating and plagiarism.
The current study reported advantages, limitations and recommendations to improve online learning during lockdown of institutions due to COVID-19 pandemic. This study interprets perspectives of medical/dental students and faculty members, which showed that online learning modalities are flexible and effective source of teaching and learning along with some pitfalls. According to the teachers and students, online learning is a flexible and effective source of teaching and learning as most of them agreed upon the fact that this helps in distant learning with easy administration and accessibility along with less use resource and time. Regardless of time limit, students can easily access the learning material. This flexibility over face to face teaching has been reported in the literature as well. 2 The students also become self-directed learners, which is an important competency for encouraging lifelong learning among health professionals. 10 , 11
Both the faculty members and students viewed inefficiency to teach psychomotor skills, resource intensiveness and mismanaged decorum during sessions as limitations of online learning. Even though, hands-on sessions such as laboratory and clinical skills teaching have been disrupted during COVID-19 pandemic, we believe that online simulated patients or role plays can be used teach history taking, clinical reasoning and communication skills. Sharing recorded videos of laboratory and clinical skills demonstration is also worthwhile. Faculty members also complained about lack of students’ feedback regarding understanding of subject. Research showed that regular two-way feedback helps enhance self-efficacy and motivation. 12 The interaction between facilitator, learner and study material along with emotional and social support are essential ingredients for effective learning. 13 , 14 Internet connectivity issues also adversely impacted learning through online modalities, however, simply improving internet package/speed would help resolve this. Government should also take immediate measures and telecommunication companies should invest in expanding its 4G services across the country.
Recommendations reflect that decorum can be maintained by thorough supervision of students, setting ground rules for online interaction, counselling and disciplinary actions. 15 According to students, the attention span during online learning was even shorter than face to face sessions as also supported by the literature. 16 This can be managed by using flipped classroom learning modalities, giving shorter lectures and increasing teacher-student interaction. As ‘assessment drives learning’, so online formative assessments can be conducted through Socrative and Kahoot etc. Faculty needs training and students orientation in using online learning tools. 17 Investment in buying premium software packages will also help overcome many limitations and is therefore recommended.
Limitations of the Study
As the study participants belonged to the medical and dental college from a single private-sector university of Punjab, therefore the findings are only applicable to similar contexts. For generalizability, a survey based on our findings should be conducted across the province or country. Despite the limitations, the findings offer an understanding of the advantages, limitations and recommendations for improvement in online learning, which is the need of the day.
The current study supports the use of online learning in medical and dental institutes, considering its various advantages. E-learning modalities encourage student-centered learning and they are easily manageable during this lockdown situation. It is worth considering here that currently online learning is at a nascent stage in Pakistan. It started as ‘emergency remote learning’, and with further investments we can overcome any limitations. There is a need to train faculty on the use of online modalities and developing lesson plan with reduced cognitive load and increased interactivities.
Author’s Contribution
AS and MA conceived the idea , designed the study and are responsible for integrity of research.
KM and KJ collected the data.
All the authors contributed towards data analysis and writing the manuscript and approved the final version.
Acknowledgements
The authors would like to acknowledge the participants for their time and contributions.
Conflict of interest: None.
Education ∪ Math ∪ Technology
- Instructional Routines
- Presentations
- Privacy Policy
March 11, 2020 / 2 Comments
Online Learning Recommendations
Given that many schools (and entire school districts) may be closed down during the coronavirus outbreak, I decided to write this post with recommendations for schools that may attempt to implement online learning during this time. I read through this review of the research on online learning , which contains these high-level recommendations. There are some caveats with this research, especially given that most research on online learning has been done with older students and that the sample sizes with k to 12 students are relatively small. That being said, some evidence for effectiveness is better than no evidence at all. A further caveat: these recommendations are based on effect sizes, which I have not included since they are notoriously unreliable to compare.
- Instruction combining online and face-to-face elements had a larger advantage relative to purely face-to-face instruction than did purely online instruction. If your school is closed completely, then this may be impossible. That being said, online programs such as Zoom or Big Marker may allow for some “face to face” interaction to occur. These programs will also help with the next recommendation.
- Effect sizes were larger for studies in which the online instruction was collaborative or instructor-directed than in those studies where online learners worked independently. This basically means that you should design activities that are either led by a teacher or activities that have students work together in small groups. Resources like Google Docs and Skype will be helpful for students working together but given the high possibility that some students will engage in off-task and/or anti-social behaviour (such as teasing or bullying), having some moderation and oversight of these online spaces will be helpful.
- Elements such as video or online quizzes do not appear to influence the amount that students learn in online classes. Creating a bunch of video lessons of a talking head working through some math problems and then quizzing students on what they have learned afterwards is not supported by the existing evidence on online learning. Given that educator planning time is in short supply, it’s probably best to plan other types of activities.
- Online learning can be enhanced by giving learners control of their interactions with media and prompting learner reflection. A good example of a program that allows for this is Geogebra . See for example this set of constructions puzzles that require students to think and make decisions while they work through the problems. Another example is the DreamBox Learning math program , which also requires students to actively engage with mathematics. Disclaimer: I work for DreamBox Learning as a mathematician and senior curriculum designer.
- Providing guidance for learning for groups of students appears less successful than using such mechanisms with individual learners. This recommendation suggests that feedback and support for students should be individualized for online learning, rather than given to the entire group. This does not necessarily mean that one should avoid providing scaffolds (such as guiding questions) to the entire group or that teachers necessarily need to work with individual students, only that whatever guidance and feedback is provided, it should be directed where possible to individual students.
Based on my experience as a parent to my sons, who have both engaged with online learning and are in elementary school and high school right now, I have some further recommendations.
- Actively engage the learning guardians of students in the process of learning. My sons’ experiences have been far more productive when we have sat down with them while they work through the online course material. This does not mean that we do the work for our children, but rather than we are there to support, encourage, and nurture their development as learners. It will be helpful to offer explicit advice for how learning guardians can support their learners, especially given the range of knowledge and experience those learning guardians will bring to the task. You may even want to include videos of what class looks like and descriptions of instructional routines that learning guardians can use with their learners. Also, offer suggestions of activities to learning guardians that they can do with their children in their care that are not on a computer and do not require the learning guardians to be experts in any particular subject matter.
- Skip watch-the-video-then-fill-in-the-blank-spaces activities. I can say from experience that these types of activities are ubiquitous in online learning and result in nearly no learning. I watched my son listen to a video in one tab while dutifully recording the answers in his worksheet in another. I quizzed him 5 minutes later and he could remember literally nothing at all from the worksheet or the video. Given that completing these particular courses was a requirement at his school, I taught him a much more productive learning strategy. First, attempt the worksheet and fill in every blank, even if one has to guess. Next, watch the entire video without writing or doing anything else. Now go back to the worksheet and change as many of the answers as one can without going back to the video. Rewatch or listen to the video with the worksheet and change answers as necessary. This is still a terrible experience but it at least has the possibility to result in some learning.
- Provide devices for students to work if at all possible or at least ensure that any online learning activities can be completed with a smartphone. While access to computers and the Internet keeps increasing , there are still households that do not have access and so providing equitable access to resources to all families is a key responsibility of schools, particularly when expecting students to engage in online learning.
- Where possible, engage students in synchronous activities rather than asynchronous activities. One of the more successful online classes my son took was with the Art of Problem Solving . Each week my son met with the entire class in an online chat program where the teacher mostly posed questions and occasionally told the students information, while the students responded to the questions in the online platform. He also had a physical textbook, a bank of unlimited practice problems to work on, and challenging problems to complete each week. The chat program was nothing amazing, but it mostly kept my son engaged for the full 90-minute sessions.
- Use simple assignments that do not require students to navigate complex instructions. Even with assignments with simple instructions, there is a lot of potential for student learning. Given that your students will be working remotely and with limited direct support, you don’t want students spending too much of their time figuring out what they are trying to accomplish.
What other recommendations for teachers and schools who may be suddenly engaged in online learning do you have? What question do you have that I have not yet answered?
- Author info
Add yours →
Evan Weinberg says:
We are in week six of closure over in Vietnam, and I can say that I agree with your assertions here. The ones with the most lasting impact are #5 in your first list (individual feedback/learning is better than whole class) and #4 in your second (synchronous is better than asynchronous).
The unifying thread to both is that students seem to quickly miss the social interactions of being in a physical space together. Whether they are introverts or extroverts, all students have expressed how much they value opportunities to connect with each other and with me as their teacher.
It is true that our students interact online much more than we do. A lot has been made out online learning really being successful for this new generation because it is so natural to them. This crisis has made clear that even with the reality of our students’ comfort with online channels, in-person interactions are as important as ever.
March 11, 2020 — 11:47 am
- Links to Resources for Shifting Instruction Online – Illustrative Mathematics
Leave a Reply Cancel reply
Your email address will not be published. Required fields are marked *
Notify me of followup comments via e-mail. You can also subscribe without commenting.
This site uses Akismet to reduce spam. Learn how your comment data is processed .
That’s Mathematics
Previous post
Why Is a Negative Times a Negative Positive?
Administrator
Popular posts
- Why Is a Negative Times a Negative Positive? 110.7k views
- What is Conceptual Understanding? 53.9k views
- Teachers are made, not born 47k views
- Online Learning Recommendations 41.1k views
- The difference between instrumental and relational understanding 29k views
- Philosophy of Educational Technology 20.7k views
- Paper use in schools 20.6k views
- Some problems with ebooks in schools 17.9k views
- 20 things every teacher should do 15.6k views
- Why teach math? 15.4k views
Archived posts
Recent posts.
The Transformative Power of Games in Learning
April 18, 2024
Tracking Whole Group Conversations
March 20, 2024
Instructional Routines for Math
March 15, 2024
Welcome to my AI-Generated Math Ted Talk
March 11, 2024
AI in Education
March 9, 2024
Email address:
I have read the privacy policy.
A Digital Recommendation System for Personalized Learning to Enhance Online Education: A Review
Ieee account.
- Change Username/Password
- Update Address
Purchase Details
- Payment Options
- Order History
- View Purchased Documents
Profile Information
- Communications Preferences
- Profession and Education
- Technical Interests
- US & Canada: +1 800 678 4333
- Worldwide: +1 732 981 0060
- Contact & Support
- About IEEE Xplore
- Accessibility
- Terms of Use
- Nondiscrimination Policy
- Privacy & Opting Out of Cookies
A not-for-profit organization, IEEE is the world's largest technical professional organization dedicated to advancing technology for the benefit of humanity. © Copyright 2024 IEEE - All rights reserved. Use of this web site signifies your agreement to the terms and conditions.
A systematic literature review on educational recommender systems for teaching and learning: research trends, limitations and opportunities
- Published: 14 September 2022
- Volume 28 , pages 3289–3328, ( 2023 )
Cite this article
- Felipe Leite da Silva ORCID: orcid.org/0000-0002-2799-3503 1 ,
- Bruna Kin Slodkowski ORCID: orcid.org/0000-0002-9028-366X 1 ,
- Ketia Kellen Araújo da Silva ORCID: orcid.org/0000-0003-4722-8072 1 &
- Sílvio César Cazella ORCID: orcid.org/0000-0003-2343-893X 1 , 2
9462 Accesses
23 Citations
Explore all metrics
Recommender systems have become one of the main tools for personalized content filtering in the educational domain. Those who support teaching and learning activities, particularly, have gained increasing attention in the past years. This growing interest has motivated the emergence of new approaches and models in the field, in spite of it, there is a gap in literature about the current trends on how recommendations have been produced, how recommenders have been evaluated as well as what are the research limitations and opportunities for advancement in the field. In this regard, this paper reports the main findings of a systematic literature review covering these four dimensions. The study is based on the analysis of a set of primary studies ( N = 16 out of 756, published from 2015 to 2020) included according to defined criteria. Results indicate that the hybrid approach has been the leading strategy for recommendation production. Concerning the purpose of the evaluation, the recommenders were evaluated mainly regarding the quality of accuracy and a reduced number of studies were found that investigated their pedagogical effectiveness. This evidence points to a potential research opportunity for the development of multidimensional evaluation frameworks that effectively support the verification of the impact of recommendations on the teaching and learning process. Also, we identify and discuss main limitations to clarify current difficulties that demand attention for future research.
Similar content being viewed by others
Panorama of Recommender Systems to Support Learning
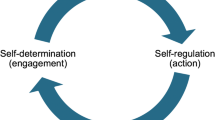
Recommender systems to support learners’ Agency in a Learning Context: a systematic review
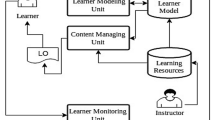
A systematic literature review on adaptive content recommenders in personalized learning environments from 2015 to 2020
Avoid common mistakes on your manuscript.
1 Introduction
Digital technologies are increasingly integrated into different application domains. Particularly in education, there is a vast interest in using them as mediators of the teaching and learning process. In such a task, the computational apparatus serves as an instrument to support human knowledge acquisition from different educational methodologies and pedagogical practices (Becker, 1993 ).
In this sense, Educational Recommender Systems (ERS) play an important role for both educators and students (Maria et al., 2019 ). For instructors, these systems can contribute to their pedagogical practices through recommendations that improve their planning and assist in educational resources filtering. As for the learners, through preferences and educational constraints recognition, recommenders can contribute for their academic performance and motivation by indicating personalized learning content (Garcia-Martinez & Hamou-Lhadj, 2013 ).
Despite the benefits, there are known issues upon the usage of the recommender system in the educational domain. One of the main challenges is to find an appropriate correspondence between the expectations of users and the recommendations (Cazella et al., 2014 ). Difficulties arise from differences in learner’s educational interests and needs (Verbert et al., 2012 ). The variety of student’s individual factors that can influence the learning process (Buder & Schwind, 2012 ) is one of the challenging matters that makes it complex to be overcome. On a recommender standpoint, this reflects an input diversity with potential to tune recommendations for users.
In another perspective, from a technological and artificial intelligence standpoint, the ERS are likely to suffer from already known issues noted on the general-purpose ones, such as the cold start and data sparsity problems (Garcia-Martinez & Hamou-Lhadj, 2013 ). Furthermore, problems are related to the approach used to generate recommendations. For instance, the overspecialization is inherently associated with the way that content-based recommender systems handle data (Iaquinta et al., 2008 ; Khusro et al., 2016 ). These issues pose difficulties to design recommenders that best suit the user’s learning needs and that distance themselves from user’s dissatisfaction in the short and long term.
From an educational point of view, issues emerge on how to evaluate ERS effectiveness. A usual strategy to measure the quality of educational recommenders is to apply the traditional recommender’s evaluation methods (Erdt et al., 2015 ). This approach determines system quality based on performance properties, such as its precision and prediction accuracy. Nevertheless, in the educational domain, system effectiveness needs to take into account the students’ learning performance. This dimension brings new complexities on how to successfully evaluate ERS.
As ERS topic has gradually increased in attraction for scientific community (Zhong et al., 2019 ), extensive research have been carried out in recent years to address these issues (Manouselis et al. 2010 ; Manouselis et al., 2014 ; Tarus et al., 2018 ; George & Lal, 2019 ). ERS has become a field of application and combination of different computational techniques, such as data mining, information filtering and machine learning, among others (Tarus et al., 2018 ). This scenario indicates a diversity in the design and evaluation of recommender systems that support teaching and learning activities. Nonetheless, research is dispersed in literature and there is no recent study that encompasses the current scientific efforts in the field that reveals how such issues are addressed in current research. Reviewing evidence, and synthesizing findings of current approaches in how ERS produce recommendations, how ERS are evaluated and what are research limitations and opportunities can provide a panoramic perspective of the research topic and support practitioners and researchers for implementation and future research directions.
From the aforementioned perspective, this work aims to investigate and summarize the main trends and research opportunities on ERS topic through a Systematic Literature Review (SLR). The study was conducted based on the last six years publications, particularly, regarding to recommenders that support teaching and learning process.
Main trends referrer to recent research direction on the ERS field. They are analyzed in regard to how recommender systems produce recommendations and how they are evaluated. As mentioned above, these are significant dimensions related to current issues of the area. Specifically for the recommendation production, this paper provides a three-axis-based analysis centered on systems underlying techniques, input data and results presentation.
Additionally, research opportunities in the field of ERS as well as their main limitations are highlighted. Because current comprehension of these aspects is fragmented in literature, such an analysis can shed light for future studies.
The SLR was carried out using Kitchenham and Charters ( 2007 ) guidelines. The SLR is the main method for summarizing evidence related to a topic or a research question (Kitchenham et al., 2009 ). Kitchenham and Charters ( 2007 ) guidelines, in turn, are one of the leading orientations for reviews on information technology in education (Dermeval et al., 2020 ).
The remainder of this paper is structured as follows. In Section 2 , the related works are presented. Section 3 details the methodology used in carrying out the SLR. Section 4 covers the SLR results and related discussion. Section 5 presents the conclusion.
2 Related works
In the field of education, there is a growing interest in technologies that support teaching and learning activities. For this purpose, ERS are strategic solutions to provide a personalized educational experience. Research in this sense has attracted the attention of the scientific community and there has been an effort to map and summarize different aspects of the field in the last 6 years.
In Drachsler et al. ( 2015 ) a comprehensive review of technology enhanced learning recommender systems was carried out. The authors analyzed 82 papers published from 2000 to 2014 and provided an overview of the area. Different aspects were analyzed about recommenders’ approach, source of information and evaluation. Additionally, a categorization framework is presented and the study includes the classification of selected papers according to it.
Klašnja-Milićević et al. ( 2015 ) conducted a review on recommendation systems for e-learning environments. The study focuses on requirements, challenges, (dis)advantages of techniques in the design of this type of ERS. An analysis on collaborative tagging systems and their integration in e-learning platform recommenders is also discussed.
Ferreira et al. ( 2017 ) investigated particularities of research on ERS in Brazil. Papers published between 2012 and 2016 in three Brazilian scientific vehicles were analyzed. Rivera et al. ( 2018 ) presented a big picture of the ERS area through a systematic mapping. The study covered a larger set of papers and aimed to detect global characteristics in ERS research. Aiming at the same focus, however, setting different questions and repositories combination, Pinho, Barwaldt, Espíndola, Torres, Pias, Topin, Borba and Oliveira (2019) performed a systematic review on ERS. In these works, it is observed the common concern of providing insights about the systems evaluation methods and the main techniques adopted in the recommendation process.
Nascimento et al. ( 2017 ) carried out a SLR covering learning objects recommender systems based on the user’s learning styles. Learning objects metadata standards, learning style theoretical models, e-learning systems used to provide recommendations and the techniques used by the ERS were investigated.
Tarus et al ( 2018 ) and George and Lal ( 2019 ) concentrated their reviews on ontology-based ERS. Tarus et al. ( 2018 ) examined research distribution in a period from 2005 to 2014 according to their years of publication. Furthermore, the authors summarized the techniques, knowledge representation, ontology types and ontology representations covered in the papers. George and Lal ( 2019 ), in turn, update the contributions of Tarus et al. ( 2018 ), investigating papers published between 2010 and 2019. The authors also discuss how ontology-based ERS can be used to address recommender systems traditional issues, such as cold start problem and rating sparsity.
Ashraf et al. ( 2021 ) directed their attention to investigate course recommendation systems. Through a comprehensive review, the study summarized the techniques and parameters used by this type of ERS. Additionally, a taxonomy of the factors taken into account in the course recommendation process was defined. Salazar et al. ( 2021 ), on the other hand, conducted a review on affectivity-based ERS. Authors presented a macro analysis, identifying the main authors and research trends, and summarized different recommender systems aspects, such as the techniques used in affectivity analysis, the source of affectivity data collection and how to model emotions.
Khanal et al. ( 2019 ) reviewed e-learning recommendation systems based on machine learning algorithms. A total of 10 papers from two scientific vehicles and published between 2016 and 2018 were examined. The study focal point was to investigate four categories of recommenders: those based on collaborative filtering, content-based filtering, knowledge and a hybrid strategy. The dimensions analyzed were the machine learning algorithms used, the recommenders’ evaluation process, inputs and outputs characterization and recommenders’ challenges addressed.
2.1 Related works gaps and contribution of this study
The studies presented in the previous section have a diversity of scope and dimensions of analysis, however, in general, they can be classified into two distinct groups. The first, focus on specific subjects of ERS field, such as similar methods of recommendations (George & Lal, 2019 ; Khanal et al., 2019 ; Salazar et al., 2021 ; Tarus et al., 2018 ) and same kind of recommendable resources (Ashraf et al., 2021 ; Nascimento et al., 2017 ). This type of research scrutinizes the particularities of the recommenders and highlights aspects that are difficult to be identified in reviews with a broader scope. Despite that, most of the reviews concentrate on analyses of recommenders’ operational features and have limited discussion on crosswise issues, such as ERS evaluation and presentation approaches. Khanal et al. ( 2019 ), specifically, makes contributions regarding evaluation, but the analysis is limited to four types of recommender systems.
The second group is composed of wider scope reviews and include recommendation models based on a diversity of methods, inputs and outputs strategies (Drachsler et al., 2015 ; Ferreira et al., 2017 ; Klašnja-Milićević et al., 2015 ; Pinho et al., 2019 ; Rivera et al., 2018 ). Due to the very nature of systematic mappings, the research conducted by Ferreira et al. ( 2017 ) and Rivera et al. ( 2018 ) do not reach in depth some topics, for example, the data synthesized on the evaluations of the ERS are delimited to indicate only the methods used. Ferreira et al. ( 2017 ), in particular, aims to investigate only Brazilian recommendation systems, offering partial contributions to an understanding of the state of the art of the area. In Pinho et al. ( 2019 ) it is noted the same limitation of the systematic mappings. The review was reported with a restricted number of pages, making it difficult to detail the findings. On the other hand, Drachsler et al. ( 2015 ) and, Klašnja-Milićević et al. ( 2015 ) carried out comprehensive reviews that summarizes specific and macro dimensions of the area. However, the papers included in their reviews were published until 2014 and there is a gap on the visto que advances and trends in the field in the last 6 years.
Given the above, as far as the authors are aware, there is no wide scope secondary study that aggregate the research achievements on recommendation systems that support teaching and learning in recent years. Moreover, a review in this sense is necessary since personalization has become an important feature in the teaching and learning context and ERS are one of main tools to deal with different educational needs and preferences that affect individuals’ learning process.
In order to widen the frontiers of knowledge in the field of research, this review aims to contribute to the area by presenting a detailed analysis of the following dimensions: how recommendations are produced and presented, how recommender systems are evaluated and what are the studies limitations and research opportunities. Specifically, to summarize the current knowledge, a SLR was conducted based on four research questions (Section 3.1 ). The review focused on papers published from 2015 to 2020 in scientific journals. A quality assessment was performed to select the most mature systems. The data found on the investigated topics are summarized and discussed in Section 4 .
3 Methodology
This study is based on the SLR methodology for gathering evidences related to the research topic investigated. As stated by Kitchenham and Charters ( 2007 ) and Kitchenham et al. ( 2009 ), this method provides the means for aggregate evidences from current research prioritizing the impartiality and reproducibility of the review. Therefore, a SLR is based on a process that entails the development of a review protocol that guides the selection of relevant studies and the subsequent extraction of data for analysis.
Guidelines for SLR are widely described in literature and the method can be applied for gathering evidences in different domains, such as, medicine and social science (Khan et al., 2003 ; Pai et al., 2004 ; Petticrew & Roberts, 2006 ; Moher et al., 2015 ). Particularly for informatics in education area, Kitchenham and Charters ( 2007 ) guidelines have been reported as one of the main orientations (Dermeval et al, 2020 ). Their approach appears in several studies (Petri & Gresse von Wangenheim, 2017 ; Medeiros et al., 2019 ; Herpich et al, 2019 ) including mappings and reviews on ERS field (Rivera et al., 2018 ; Tarus et al., 2018 ).
As mentioned in Section 1 , Kitchenham and Charters ( 2007 ) guidelines were used in the conducted SLR. They are based on three main stages: the first for planning the review, the second for conducting it and the last for the results report. Following these orientations, the review was structured in three phases with seven main activities distributed among them as depicted in Fig. 1 .
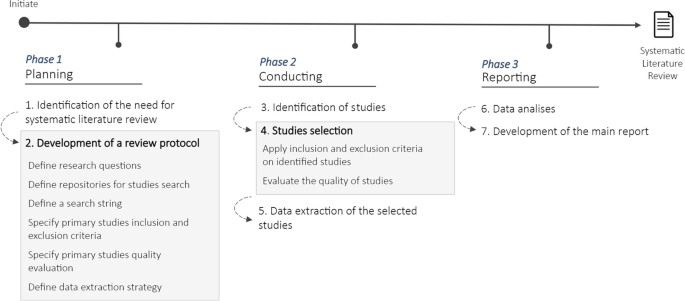
Systematic literature review phases and activities
The first was the planning phase. The identification of the need for a SLR about teaching and learning support recommenders and the development of the review protocol occurred on this stage. In activity 1, the search for SLR with the intended scope of this study was performed. The result did not return compatible papers with this review scope. Papers identified are described in Section 2 . In activity 2, the review process was defined. The protocol was elaborated through rounds of discussion by the authors until consensus was reached. The activity 2 output were the research questions, search strategy, papers selection strategy and the data extraction method.
The next was the conducting phase. At this point, activities for relevant papers identification (activity 3) and selection (activities 4) were executed. In Activity 3, searches were carried out in seven repositories indicated by Dermeval et al. ( 2020 ) as relevant to the area of informatics in education. Authors applied the search string into these repositories search engines, however, due to the large number of returned research, the authors established the limit of 600 to 800 papers that would be analyzed. Thus, three repositories whose sum of search results was within the established limits were chosen. The list of potential repositories considered for this review and the selected ones is listed in Section 3.1 . The search string used is also shown in Section 3.1 .
In activity 4, studies were selected through two steps. In the first, inclusion and exclusion criteria were applied to each identified paper. Accepted papers had they quality assessed in the second step. Parsifal Footnote 1 was used to manage planning and conducting phase data. Parsifal is a web system, adhering to Kitchenham and Charters ( 2007 ) guidelines, that helps in SLR conduction. At the end of this step, relevant data were extracted (activity 5) and registered in a spreadsheet. Finally, in the reporting phase, the extracted data were analyzed in order to answer the SLR research questions (activity 6) and the results were recorded in this paper (activity 7).
3.1 Research question, search string and repositories
Teaching and learning support recommender systems have particularities of configuration, design and evaluation method. Therefore, the following research questions (Table 1 ) were elaborated in an effort to synthesize these knowledge as well as the main limitations and research opportunities in the field from the perspective of the most recent studies:
Regarding the search strategy, papers were selected from three digital repositories (Table 2 ). For the search, “Education” and “Recommender system” were defined as the keywords and synonyms were derived from them as secondary terms (Table 3 ). From these words, the following search string was elaborated:
("Education" OR "Educational" OR "E-learning" OR "Learning" OR "Learn") AND ("Recommender system" OR "Recommender systems" OR "Recommendation system" OR "Recommendation systems" OR "Recommending system" OR "Recommending systems")
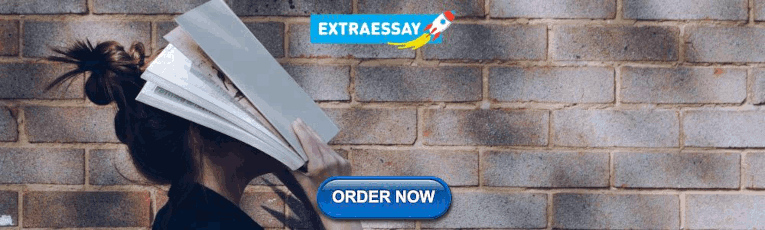
3.2 Inclusion and exclusion criteria
The first step for the selection of papers was performed through the application of objective criteria, thus a set of inclusion and exclusion criteria was defined. The approved papers formed a group that comprises the primary studies with potential relevance for the scope of the SLR. Table 4 lists the defined criteria. In the description column of Table 4 , the criteria are informed and in the id column they are identified with a code. The latter was defined appending an abbreviation of the respective kind of criteria (IC for Inclusion Criteria and EC for Exclusion Criteria) with an index following the sequence of the list. The Id is used for referencing its corresponding criterion in the rest of this document.
Since the focus of this review is on the analysis of recent ERS publications, only studies from the past 6 years (2015–2020) were screened (see IC1). Targeting mature recommender systems, only full papers from scientific journals that present the recommendation system evaluation were considered (see IC2, IC4 and IC7). Also, solely works written in English language were selected, because they are the most expressive in quantity and are within the reading ability of the authors (see IC3). Search string was verified on papers’ title, abstract and keywords to ensure only studies related to the ERS field were screened (see IC5). The IC6, specifically, delimited the subject of selected papers and aligned it to the scope of the review. Additionally, it prevented the selection of secondary studies in process (e.g., others reviews or systematic mappings). Conversely, exclusion criteria were defined to clarify that papers contrasting with the inclusion criteria should be excluded from review (see EC1 to EC8). Finally, duplicate searches were marked and, when all criteria were met, only the latest was selected.
3.3 Quality evaluation
The second step in studies selection activity was the quality evaluation of the papers. A set of questions were defined with answers of different weights to estimate the quality of the studies. The objective of this phase was to filter researches with higher: (i) validity; (ii) details of the context and implications of the research; and (iii) description of the proposed recommenders. Research that detailed the configuration of the experiment and carried out an external validation of the ERS obtained higher weight in the quality assessment. Hence, the questions related to recommender evaluation (QA8 and QA9) ranged from 0 to 3, while the others, from 0 to 2. The questions and their respective answers are presented in Table 7 (see Appendix). Each paper evaluated had a total weight calculated according to Formula 1 :
Papers total weight range from 0 to 10. Only works that reached the minimum weight of 7 were accepted.
3.4 Screening process
Papers screening process occurred as shown in Fig. 2 . Initially, three authors carried out the identification of the studies. In this activity, the search string was applied into search engines of the repositories along with the inclusion and exclusion criteria through filtering settings. Two searches were undertaken on the three repositories at distinct moments, one in November 2020 and another in January 2021. The second one was performed to ensure that all 2020 published papers in the repositories were counted. A number of 756 preliminary primary studies were returned and their metadata were registered in Parsifal.
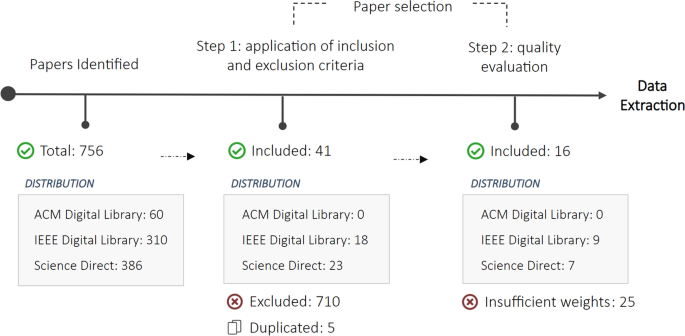
Flow of papers search and selection
Following the protocol, the selection activity was initiated. At the start, the duplicity verification feature of Parsifal was used. A total of 5 duplicate papers were returned and the oldest copies were ignored. Afterwards, papers were divided into groups and distributed among the authors. Inclusion and exclusion criteria were applied through titles and abstracts reading. In cases which were not possible to determine the eligibility of the papers based on these two fields, the body of text was read until it was possible to apply all criteria accurately. Finally, 41 studies remained for the next step. Once more, papers were divided into three groups and each set of works was evaluated by one author. Studies were read in full and weighted according to each quality assessment question. At any stage of this process, when questions arose, the authors defined a solution through consensus. As a final result of the selection activity, 16 papers were approved for data extraction.
3.5 Procedure for data analysis
Data from selected papers were extracted in a data collection form that registered general information and specific information. The general information extracted was: reviewer identification, date of data extraction and title, authors and origin of the paper. General information was used to manage the data extraction activity. The specific information was: recommendation approach, recommendation techniques, input parameters, data collection strategy, method for data collection, evaluation methodology, evaluation settings, evaluation approaches, evaluation metrics. This information was used to answer the research questions. Tabulated records were interpreted and a descriptive summary with the findings was prepared.
4 Results and discussion
In this section, the SLR results are presented. Firstly, an overview of the selected papers is introduced. Next, the finds are analyzed from the perspective of each research question in a respective subsection.
4.1 Selected papers overview
Each selected paper presents a distinct recommendation approach that advances the ERS field. Following, an overview of these studies is provided.
Sergis and Sampson ( 2016 ) present a recommendation system that supports educators’ teaching practices through the selection of learning objects from educational repositories. It generates recommendations based on the level of instructors’ proficiency on ICT Competences. In Tarus et al. ( 2017 ), the recommendations are targeted at students. The study proposes an e-learning resource recommender based on both user and item information mapped through ontologies.
Nafea et al. ( 2019 ) propose three recommendation approaches. They combine item ratings with student’s learning styles for learning objects recommendation. Klašnja-Milićević et al. ( 2018 ) present a recommender of learning materials based on tags defined by the learners. The recommender is incorporated in Protus e-learning system.
In Wan and Niu ( 2016 ), a recommender based on mixed concept mapping and immunological algorithms is proposed. It produces sequences of learning objects for students. In a different approach, the same authors incorporate the self-organization theory into ERS. Wan and Niu ( 2018 ) deals with the notion of self-organizing learning objects. In this research, resources behave as individuals who can move towards learners. This movement results in recommendations and is triggered based on students’ learning attributes and actions. Wan and Niu ( 2020 ), in turn, self-organization refers to the approach of students motivated by their learning needs. The authors propose an ERS that recommends self-organized cliques of learners and, based on these, recommend learning objects.
Zapata et al. ( 2015 ) developed a learning object recommendation strategy for teachers. The study describes a methodology based on collaborative methodology and voting aggregation strategies for the group recommendations. This approach is implemented in the Delphos recommender system. In a similar research line, Rahman and Abdullah ( 2018 ) show an ERS that recommends Google results tailored to students’ academic profile. The proposed system classifies learners into groups and, according to the similarity of their members, indicates web pages related to shared interests.
Wu et al. ( 2015 ) propose a recommendation system for e-learning environments. In this study, complexity and uncertainties related to user profile data and learning activities is modeled through tree structures combined with fuzzy logic. Recommendations are produced from matches of these structures. Ismail et al. ( 2019 ) developed a recommender to support informal learning. It suggests Wikipedia content taking into account unstructured textual platform data and user behavior.
Huang et al. ( 2019 ) present a system for recommending optional courses. The system indications rely on the student’s curriculum time constraints and similarity of academic performance between him and senior students. The time that individuals dedicate for learning is also a relevant factor in Nabizadeh et al. ( 2020 ). In this research, a learning path recommender that includes lessons and learning objects is proposed. Such a system estimates the learner’s good performance score and, based on that, produces a learning path that satisfies their time constraints. The recommendation approach also provides indication of auxiliary resources for those who do not reach the estimated performance.
Fernandez-Garcia et al. ( 2020 ) deals with recommendations of disciplines through a dataset with few instances and sparse. The authors developed a model based on several techniques of data mining and machine learning to support students’ decision in choosing subjects. Wu et al. ( 2020 ) create a recommender that captures students’ mastery of a topic and produces a list of exercises with a level of difficulty adapted to them. Yanes et al. ( 2020 ) developed a recommendation system, based on different machine learning algorithms, that provides appropriate actions to assist teachers to improve the quality of teaching strategies.
4.2 How teaching and learning support recommender systems produce recommendations?
The process of generating recommendations is analyzed based on two axes. Underlying techniques of recommender systems are discussed first then input parameters are covered. Studies details are provided in Table 5 .
4.2.1 Techniques approaches
Through selected papers analysis is observed that hybrid recommendation systems are predominant in selected papers. Such recommenders are characterized by computing predictions through a set of two or more algorithms in order to mitigate or avoid the limitations of pure recommendation systems (Isinkaye et al., 2015 ). From sixteen analyzed papers, thirteen (p = 81,25%) are based on hybridization. This tendency seems to be related with the support that hybrid approach provides for development of recommender systems that must meet multiple educational needs of users. For example, Sergis and Sampson ( 2016 ) proposed a recommender based on two main techniques: fuzzy set to deal with uncertainty about teacher competence level and Collaborative Filtering (CF) to select learning objects based on neighbors who may have competences similarities. In Tarus et al. ( 2017 ) students and learning resources profiles are represented as ontologies. The system calculates predictions based on them and recommends learning items through a mechanism that applies collaborative filtering followed by a sequential pattern mining algorithm.
Moreover, the hybrid approach that combines CF and Content-Based Filtering (CBF), although a traditional technique (Bobadilla, Ortega, Hernando and Gutiérrez, 2013), it seems to be not popular in teaching and learning support recommender systems research. From the selected papers, only Nafea et al. ( 2019 ) has a proposal in this regard. Additionally, the extracted data indicates that a significant number of hybrid recommendation systems (p = 53.85%, n = 7) have been built based on the combination of methods of treatment or representation of data, such as the use of ontologies and fuzzy sets, with methods to generate recommendation. For example, Wu et al. ( 2015 ) structure users profile data and learning activities through fuzzy trees. In such structures the values assigned to their nodes are represented by fuzzy sets. The fuzzy tree data model and users’ ratings feed a tree structured data matching method and a CF algorithm for similarities calculation.
Collaborative filtering recommendation paradigm, in turn, plays an important role in research. Nearly a third of the studies (p = 30.77%, n = 4) that propose hybrid recommenders includes a CF-based strategy. In fact, this is the most frequent pure technique on the research set. A total of 31.25%( n = 5) are based on a CF adapted version or combine it with other approaches. CBF-based recommenders, in contrast, have not shared the same popularity. This technique is an established recommendation approach that produces results based on the similarity between items known to the user and others recommendable items (Bobadilla et al., 2013 ). Only Nafea et al. ( 2019 ) propose a CBF-based recommendation system.
Also, CF user-based variant is widely used in analyzed research. In this version, predictions are calculated by similarity between users, as opposed to the item-based version where predictions are based on item similarities (Isinkaye et al., 2015 ). All CF-based recommendation systems identified, whether pure or combined with other techniques, use this variant.
The above finds seem to be related to the growing perception, in the education domain, of the relevance of a student-centered teaching and learning process (Krahenbuhl, 2016 ; Mccombs, 2013 ). Recommendation approaches that are based on users’ profile, such as interests, needs, and capabilities, naturally fit this notion and are more widely used than those based on other information such as the characteristics of the recommended items.
4.2.2 Input parameters approaches
In regard to the inputs consumed in the recommendation process, collected data shows that the main parameters are attributes related to users’ educational profile. Examples are ICT competences (Sergis & Sampson, 2016 ); learning objectives (Wan & Niu, 2018 ; Wu et al., 2015 ), learning styles (Nafea et al., 2019 ), learning levels (Tarus et al., 2017 ) and different academic data (Yanes et al., 2020 ; Fernández-García et al., 2020). Only 25% ( n = 4) of the systems apply item-related information in the recommendation process. Furthermore, with the exception of the Nafea et al. ( 2019 ) CBF-based recommendation, the others are based on a combination of items and users’ information. A complete list of the identified input parameters is provided in Table 5 .
Academic information and learning styles, compared to others parameters, features highly on research. They appear, respectively, in 37.5% ( n = 6) and 31.25% ( n = 5) papers. Student’s scores (Huang et al., 2019 ), academic background (Yanes et al., 2020 ), learning categories (Wu et al., 2015 ) and subjects taken (Fernández-García et al.,2020) are some of the academic data used. Learning styles, in turn, are predominantly based on Felder ( 1988 ) theory. Wan and Niu ( 2016 ), exceptionally, combine Felder ( 1988 ), Kolb et al. ( 2001 ) and Betoret ( 2007 ) to build a specific notion of learning styles. This is also used in two other researchers, carried out by the same authors, and has a questionnaire also developed by them (Wan & Niu, 2018 , 2020 ).
Regarding the way inputs are captured, it was observed that explicit feedback is prioritized over others data collection strategies. In this approach, users have to directly provide the information that will be used in the process of preparing recommendations (Isinkaye et al., 2015 ). Half of analyzed studies are based only on explicit feedback. The use of graphical interface components (Klašnja-Milićević et al., 2018 ), questionnaires (Wan & Niu, 2016 ) and manual entry of datasets (Wu et al., 2020 ; Yanes et al., 2020 ) are the main methods identified.
Only 18.75%( n = 3) ERS rely solely on gathering information through implicit feedback, that is, when inputs are inferred by the system (Isinkaye et al., 2015 ). This type of data collection appears to be more popular when applied with an explicit feedback method for enhancing the prediction tasks. Recommenders that combine both approaches occur in 31.25%( n = 5) of the studies. Implicit data collection methods identified are user’s data usage tracking, as access, browsing and rating history (Rahman & Abdullah, 2018 ; Sergis & Sampson, 2016 ; Wan & Niu, 2018 ), data extraction from another system (Ismail et al., 2019 ), users data session monitoring (Rahman & Abdullah, 2018 ) and data estimation (Nabizadeh et al., 2020 ).
The aforementioned results indicate that, in the context of the teaching and learning support recommender systems, the implicit collection of data has usually been explored in a complementary way to the explicit one. A possible rationale is that the inference of information is noisy and less accurate (Isinkaye et al., 2015 ) and, therefore, the recommendations produced from it involve greater complexity to be adjusted to the users’ expectations (Nichols, 1998 ). This aspect makes it difficult to apply the strategy in isolation and can be a factor that produces greater user dissatisfaction when compared to the disadvantage of the acquisition load of the explicit strategy inputs.
4.3 How teaching and learning support recommender systems present recommendations?
From the analyzed paper, two approaches for presenting recommendations are identified. The majority of the proposed ERS are based on a listing of ranked items according to a per-user prediction calculation (p = 87.5%, n = 14). This strategy is applied in all cases where the supported task is to find good items that assist users in teaching and learning tasks (Ricci et al., 2015 ; Drachsler et al., 2015 ). The second one, is based on a learning pathway generation. In this case, recommendations are displayed through a series of linked items tied by some prerequisites. Only 2 recommenders use this approach. In them, the sequence is established by learning objects association attributes (Wan & Niu, 2016 ) and by a combination of prior knowledge of the user, the time he has available and a learning score (Nabizadeh et al., 2020 ). These ERS are associated with the item sequence recommendation task and are intended to guide users who wish to achieve a specific knowledge (Drachsler et al., 2015 ).
In a further examination, it is observed that more than a half (62.5%, n = 10) do not present details of how recommendations list is presented to the end user. In Huang et al. ( 2019 ), for example, there is a vague description of a production of predicted scores for students and a list of the top-n optional courses and it is not specified how this list is displayed. This may be related to the fact that most of these recommenders do not report an integration into another system (e.g., learning management systems) or the purpose of making it available as a standalone tool (e.g., web or mobile recommendation system). The absence of such requirements mitigates the need for the development of a refined presentation interface. Only Tarus et al. ( 2017 ), Wan and Niu ( 2018 ) and Nafea et al. ( 2019 ) propose recommenders incorporated in an e-learning system and do not detail the way in which the results are exhibited. In the six papers that provide insights about recommendation presentation, a few of them (33.33%, n = 2), have a graphical interface that explicitly seeks to capture the attention of the user who may be performing another task in the system. This approach highlights recommendations and is common in commercial systems (Beel, Langer and Genzmehr, 2013). In Rahman and Abdullah ( 2018 ), a panel entitled “recommendations for you” is used. In Ismail et al. ( 2019 ), a pop-up box with suggestions is displayed to the user. The other part of the studies exhibits organic recommendations, i.e., naturally arranged items for user interaction (Beel et al., 2013 ).
In Zapata et al. ( 2015 ), after the user defines some parameters, a list of recommended learning objects that are returned similarly to a search engine result. As for the aggregation methods, another item recommended by the system, only the strategy that fits better to the interests of the group is recommended. The result is visualized through a five-star Likert scale that represents the users’ consensus rating. In Klašnja-Milićević et al. ( 2018 ) and Wu et al. ( 2015 ), the recommenders’ results are listed in the main area of the system. In Nabizadeh et al. ( 2020 ) the learning path occupies a panel on the screen and the items associated with it are displayed as the user progresses through the steps. The view of the auxiliary learning objects is not described in the paper. These three last recommenders do not include filtering settings and distance themselves from the archetype of a search engine.
Also, a significant number of researches are centralized on learning objects recommendations (p = 56.25%, n = 9). Other researches recommendable items identified are learning activities (Wu et al., 2015 ), pedagogical actions (Yanes et al., 2020 ), web pages (Ismail et al., 2019 ; Rahman & Abdullah, 2018 ), exercises (Wu et al., 2020 ), aggregation methods (Zapata et al., 2015 ), lessons (Nabizadeh et al., 2020 ) and subjects (Fernández-García et al., 2020). None of the study relates the way of displaying results to the recommended item. This is a topic that needs further investigation to answer whether there are more appropriate ways to present specific types of items to the user.
4.4 How teaching and learning support recommender systems are evaluated?
In ERS, there are three main evaluation methodologies (Manouselis et al., 2013 ). One of them is the offline experiment, which is based on the use of pre-collected or simulated data to test recommenders’ prediction quality (Shani & Gunawardana, 2010 ). User study is the second approach. It takes place in a controlled environment where information related to real interactions of users are collected (Shani & Gunawardana, 2010 ). This type of evaluation can be conducted, for example, through a questionnaire and A/B tests (Shani & Gunawardana, 2010 ). Finally, the online experiment, also called real life testing, is one in which recommenders are used under real conditions by the intended users (Shani & Gunawardana, 2010 ).
In view of these definitions, the analyzed researches comprise only user studies and offline experiments in reported experiments. Each of these methods were identified in 68.75% ( n = 11) papers respectively. Note that they are not exclusive for all cases and therefore the sum of the percentages is greater than 100%. For example, Klašnja-Milićević et al. ( 2018 ) and Nafea et al. ( 2019 ) assessed the quality of ERS predictions from datasets analysis and also asked users to use the systems to investigate their attractiveness. Both evaluation methods are carried out jointly in 37.5%( n = 6) papers. When comparing with methods exclusive usage, each one is conducted at 31.25% ( n = 5). Therefore, the two methods seem to have a balanced popularity. Real-life tests, on the contrary, although they are the ones that best demonstrate the quality of a recommender (Shani & Gunawardana, 2010 ), are the most avoided, probably due to the high cost and complexity of execution.
An interesting finding concerns user study methods used in research. When associated with offline experiments, the user satisfaction assessment is the most common ( p = 80%, n = 5). Of these, only Nabizadeh et al. ( 2020 ) performed an in-depth evaluation combining a satisfaction questionnaire with an experiment to verify the pedagogical effectiveness of their recommender. Wu et al. ( 2015 ), in particular, does not include a satisfaction survey. They conducted a qualitative investigation of user interactions and experiences.
Although questionnaires assist in identification of users’ valuables information, it is sensitive to respondents’ intentions and can be biased with erroneous answers (Shani & Gunawardana, 2010 ). Papers that present only user studies, in contrast, have a higher rate of experiments that results in direct evidence about the recommender’s effectiveness in teaching and learning. All papers in this group have some investigation in this sense. Wan and Niu ( 2018 ), for example, verified whether the recommender influenced the academic score of students and their time to reach a learning objective. Rahman and Abdullah ( 2018 ) investigated whether the recommender impacted the time students took to complete a task.
Regarding the purpose of the evaluations, ten distinct research goals were identified. Through Fig. 3 , it is observed that the occurrence of accuracy investigation excelled the others. Only 1 study did not carry out experiments in this regard. Different traditional metrics were identified for measuring the accuracy of recommenders. The Mean Absolute Error (MAE), in particular, has the higher frequency. Table 6 lists the main metrics identified.
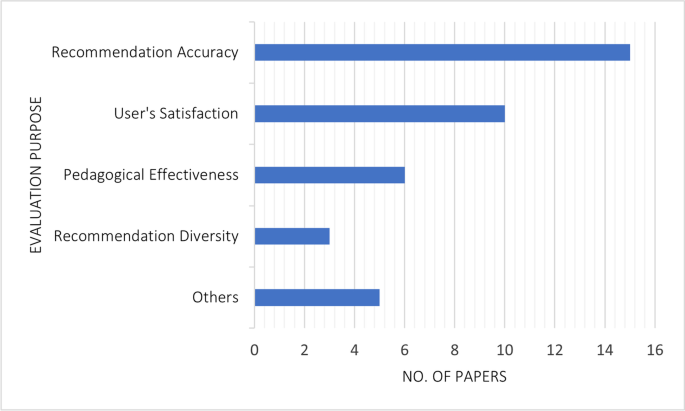
Evaluation purpose of recommender systems in selected papers
The system attractiveness analysis, through the verification of user satisfaction, has the second highest occurrence. It is present in 62.5% ( n = 10) studies. The pedagogical effectiveness evaluation of the ERS has a reduced participation in the studies and occurs in only 37.5% ( n = 6). Experiments to examine recommendations diversity, user’s profile elicitation accuracy, evolution process, user’s experience and interactions, entropy, novelty and perceived usefulness and easiness were also identified, albeit to a lesser extent.
Also, 81.25% ( n = 13) papers presented experiments to achieve multiple purposes. For example, in Wan and Niu ( 2020 ) an evaluation is carried out to investigate recommenders’ pedagogical effectiveness, student satisfaction, accuracy, diversity of recommendations and entropy. Only in Huang et al. ( 2019 ), Fernandez-Garcia et al. ( 2020 ) and Yanes et al. ( 2020 ) evaluated a single recommender system dimension.
The upper evidence suggests an engagement of the scientific community in demonstrating the quality of the recommender systems developed through multidimensional analysis. However, offline experiments and user studies, particularly those based on questionnaires, are mostly adopted and can lead to incomplete or biased interpretations. Thus, such data also signalize the need for a greater effort to conduct real life tests and experiments that lead to an understanding of the real impact of recommenders on the teaching and learning process. Researches that synthesize and discuss the empirical possibilities of evaluating the pedagogical effectiveness of ERS can help to increase the popularity of these experiments.
Through papers analysis is also find that the results of offline experiments are usually based on a greater amount of data compared to user studies. In this group, 63.64% ( n = 7) of evaluation datasets have records of more than 100 users. User studies, on the other hand, predominate sets of up to 100 participants in the experiments (72.72%, n = 8). In general, offline assessments that have smaller datasets are those that occur in association with a user study. This is because the data for both experiments usually come from the same subjects (Nafea et al., 2019 ; Tarus et al., 2017 ). The cost (e.g., time and money) related to surveying participants for the experiment is possibly a determining factor in defining appropriate samples.
Furthermore, it is also verified that the greater parcel of offline experiments has a 70/30% division approach for training and testing data. Nguyen et al. ( 2021 ) give some insights in this sense arguing that this is the most suitable ratio for training and validating machine learning models. Further details on recommendation systems evaluation approaches and metrics are presented in Table 6 .
4.5 What are the limitations and research opportunities related to the teaching and learning support recommender systems field?
The main limitations observed in selected papers are presented below. They are based on articles’ explicit statements and on authors’ formulations. In this section, only those that are transverse to the majority of the studies are listed. Next, a set of research opportunities for future investigations are pointed out.
4.5.1 Research limitations
Research limitations are factors that hinders current progress in the ERS field. Knowing these factors can assist researchers to attempt coping with them on their study and mitigate the possibility of the area stagnation, that is, when new proposed recommenders does not truly generate better outcomes than the baselines (Anelli et al., 2021 ; Dacrema et al., 2021 ). As a result of this SLR, research limitations were identified in three strands that are presented below.
Reproducibility restriction
The majority of the papers report a specifically collected dataset to evaluate the proposed ERS. The main reason for this is the scarcity of public datasets suited to the research’s needs, as highlighted by some authors (Nabizadeh et al., 2020 ; Tarus et al., 2017 ; Wan & Niu, 2018 ; Wu et al., 2015 ; Yanes et al., 2020 ). Such approach restricts the feasibility of experiment reproduction and makes it difficult to compare recommenders. In fact, this is an old issue in the ERS field. Verbert et al. ( 2011 ) observed, in the beginning of the last decade, the necessity to improve reproducibility and comparison on ERS in order to provide stronger conclusions about their validity and generalizability. Although there was an effort in this direction in the following years based on a broad educational dataset sharing, currently, most of the known ones (Çano & Morisio, 2015 ; Drachsler et al., 2015 ) are retired, and the remaining, proved not to be sufficient to meet current research demands. Of the analyzed studies, only Wu et al. ( 2020 ) use public educational datasets.
Due to the fact that datasets sharing play an important role for recommenders’ model reproduction and comparison in the same conditions, this finding highlight the need of a research community effort for the creation of means to supply this need (e.g., development of public repositories) in order to mitigate current reproducibility limitation.
Dataset size / No of subjects
As can be observed on Table 6 , a few experimental results are based on a large amount of data. Only five studies have information from 1000 or more users. In particular, the offline evaluation conducted by Wu et al. ( 2015 ), despite having an extensive dataset, uses MovieLens records and is not based on real information related to teaching and learning. Another limitation concerns where data comes from, it is usually from a single origin (e.g., class of a college).
Although experiments based on small datasets can reveal the relevance of an ERS, an evaluation based on a large-scale dataset should provide stronger conclusions on recommendation effectiveness (Verbert et al., 2011 ). Experiments based on larger and more diverse data (e.g., users from different areas and domains) would contribute to most generalizable results. On another hand, scarcity of public dataset may be impairing the quantity and diversity of data used on scientific experiments in the ERS field. As reported by Nabizadeh et al. ( 2020 ), the increasement of the size of the experiment is costly in different aspects. If more public dataset were available, researchers would be more likely to find the ones that could be aligned to their needs and, naturally, increasing the size of their experiment. In this sense, they could be favored by reducing data acquisition difficulty and cost. Furthermore, the scientific community would access users’ data out of their surrounding context and could base their experiments on diversified data.
Lack of in-depth investigation of the impact of known issues in the recommendation system field
Cold start, overspecialization and sparsity are some known challenges in the field of recommender systems (Khusro et al., 2016 ). They are mainly related to a reduced and unequally distributed number of users’ feedback or item description used for generating recommendations (Kunaver & Požrl, 2017 ). These issues also permeate the ERS Field. For instance, in Cechinel et al. ( 2011 ) is reported that on a sample of more than 6000 learning objects from Merlot repository was observed a reduced number of users ratings over items. Cechinel et al. ( 2013 ), in turn, observed, in a dataset from the same repository, a pattern of few users rating several resources while the vast number of them rating 5 or less. Since such issues directly impact the quality of recommendations, teaching and learning support recommenders should be evaluated considering such issues to clarify in which extent they can be effective in real life situations. Conversely, in this SLR, we detected an expressive number of papers (43.75%, n = 7) that do not analyze or discuss how the recommenders behave or handle, at least partially, these issues. Studies that rely on experiments to examine such aspects would elucidate more details of the quality of the proposed systems.
4.5.2 Research opportunities
From the analyzed papers, a set of research opportunities were identified. They are based on gaps related to the subjects explored through the research questions of this SLR. The identified opportunities provide insights of under-explored topics that need further investigation taking into account their potential to contribute to the advancement of the ERS field. Research opportunities were identified in three strands that are presented below.
Study of the potential of overlooked user’s attributes
The papers examined present ERS based on a variety of inputs. Preferences, prior knowledge, learning style, and learning objectives are some examples (Table 5 has the complete list). Actually, as reported by Chen and Wang ( 2021 ), this is aligned with a current research trend of investigating the relationships between individual differences and personalized learning. Nevertheless, one evidence that rises from this SLR also confirms that “some essential individual differences are neglected in existing works” (Chen & Wang, 2021 ). The papers sample suggests a lack of studies that incorporate, in recommendation model, others notably relevant information, such as emotional state and cultural context of students (Maravanyika & Dlodlo, 2018 ; Salazar et al., 2021 ; Yanes et al., 2020 ). This indicates that further investigation is needed in order to clarify the true contributions and existing complexities of collect, measure and apply these other parameters. In this sense, an open research opportunity refers to the investigation of these other users’ attributes in order to explore the impact of such characteristics on the quality of ERS results.
Increase studies on the application of ERS in informal learning situations
Informal learning refers to a type of learning that, typically, occurs out of an education institution (Pöntinen et al., 2017 ). In it, learners do not follow a structured curriculum or have a domain expert to guide him (Pöntinen et al., 2017 ; Santos & Ali, 2012 ). Such aspects influence how ERS can support users. For instance, in informal settings, content can come from multiple providers, as a consequence, it can be delivered without taking into account a proper pedagogical sequence. ERS targeting this scenario, in turn, should concentrate on organizing and sequencing recommendations guiding users’ learning process (Drachsler et al., 2009 ).
Although literature highlight the existence of significative differences on the design of educational recommenders that involves formal or informal learning circumstance (Drachsler et al., 2009 ;Okoye et al, 2012 ; Manouselis et al., 2013 ; Harrathi & Braham, 2021 ), through this SLR was observed that current studies tend to not be explicit in reporting this characteristic. This scenario makes it difficult to obtain a clear landscape of the current field situation in this dimension. Nonetheless, through the characteristics of the proposed ERS, it was observed that current research seems to be concentrated on the formal learning context. This is because recommenders from analyzed papers usually use data that are maintained by institutional learning systems. Moreover, recommendations, predominantly, do not provide a pedagogical sequencing to support self-directed and self-paced learning (e.g., recommendations that build a learning path to lead to specific knowledge). Conversely, informal learning has increasingly gained attention of the scientific community with the emergence of the coronavirus pandemic (Watkins & Marsick, 2020 ).
In view of this, the lack of studies of ERS targeting informal learning settings open a research opportunity. Specifically, further investigation focused on the design and evaluation of recommenders that take into consideration different contexts (ex. location or used device) and that guide users through a learning sequence to achieve a specific knowledge would figure prominently in this context considering the less structured format informal learning circumstances has in terms of learning objectives and learning support.
Studies on the development of multidimensional evaluation frameworks
Evidence from this study shows that the main purpose of ERS evaluation has been to assess recommender’s accuracy and users’ satisfaction (Section 4.4 ). This result, connected with Erdt et al. ( 2015 ) reveals a two decade of evaluation predominantly based on these two goals. Even though others evaluation purposes had a reduced participation in research, they are also critical for measuring the success of ERS. Moubayed et al. ( 2018 ), for example, highlights two e-learning systems evaluation aspects, one is concerned with how to properly evaluate the student performance, the other refers to measuring learners’ learning gains through systems usage. Tahereh et al. ( 2013 ) identifies that stakeholder and indicators associated with technological quality are relevant to consider in educational system assessment. From the perspective of recommender systems field, there are also important aspects to be analyzed in the context of its application in the educational domain such as novelty and diversity (Pu et al., 2011 ; Cremonesi et al., 2013 ; Erdt et al., 2015 ).
Upon this context, it is noted that, although evaluating recommender's accuracy and users’ satisfaction give insights about the value of the ERS, they are not sufficient to fully indicate the quality of the system in supporting the learning process. Other different factors reported in literature are relevant to take in consideration. However, to the best of our knowledge, there is no framework that identifies and organizes these factors to be considered in an ERS evaluation, leading to difficulties for the scientific community to be aware of them and incorporate them in studies.
Because the evaluation of ERS needs to be a joint effort between computer scientists and experts from other domains (Erdt et al., 2015 ), further investigation should be carried out seeking the development of a multidimensional evaluation framework that encompass evaluation requirements based on a multidisciplinary perspective. Such studies would clarify the different dimensions that have the potential to contribute to better ERS evaluation and could even identify which one should be prioritized to truly assess learning impact with reduced cost.
5 Conclusion
In recent years, there has been an extensive scientific effort to develop recommenders that meet different educational needs; however, research is dispersed in literature and there is no recent study that encompasses the current scientific efforts in the field.
Given this context, this paper presents an SLR that aims to analyze and synthesize the main trends, limitations and research opportunities related to the teaching and learning support recommender systems area. Specifically, this study contributes to the field providing a summary and an analysis of the current available information about the teaching and learning support recommender systems topic in four dimensions: (i) how the recommendations are produced (ii) how the recommendations are presented to the users (iii) how the recommender systems are evaluated and (iv) what are the limitations and opportunities for research in the area.
Evidences are based on primary studies published from 2015 to 2020 from three repositories. Through this review, it is provided an overarching perspective of current evidence-based practice in ERS in order to support practitioners and researchers for implementation and future research directions. Also, research limitations and opportunities are summarized in light of current studies.
The findings, in terms of current trends, shows that hybrid techniques are the most used in teaching and learning support recommender systems field. Furthermore, it is noted that approaches that naturally fit a user centered design (e.g., techniques that allow to represent students’ educational constraints) have been prioritized over that based on other aspects, like item characteristics (e.g., CBF Technique). Results show that these approaches have been recognized as the main means to support users with recommendations in their teaching and learning process and provide directions for practitioners and researchers who seek to base their activities and investigations on evidence from current studies. On the other hand, this study also reveals that highly featured techniques in the major topic of general recommender systems, such as the bandit-based and the deep learning ones (Barraza-Urbina & Glowacka, 2020 ; Zhang et al., 2020 ), have been underexplored, implying a mismatch between the areas. Therefore, the result of this systematic review indicates that a greater scientific effort should be employed to investigate the potential of these uncovered approaches.
With respect to recommendation presentation, the organic display is the most used strategy. However, most of the researches have the tendency to not show details of the used approach making it difficult to understand the state of the art of this dimension. Furthermore, among other results, it is observed that the majority of the ERS evaluation are based on the accuracy of recommenders and user's satisfaction analysis. Such a find open research opportunity scientific community for the development of multidimensional evaluation frameworks that effectively support the verification of the impact of recommendations on the teaching and learning process.
Lastly, the limitations identified indicate that difficulties related to obtaining data to carry out evaluations of ERS is a reality that extends for more than a decade (Verbert et al., 2011 ) and call for scientific community attention for the treatment of this situation. Likewise, the lack of in-depth investigation of the impact of known issues in the recommendation system field, another limitation identified, points to the importance of aspects that must be considered in the design and evaluation of these systems in order to provide a better elucidation of their potential application in a real scenario.
With regard to research limitations and opportunities, some of this study findings indicate the need for a greater effort in the conduction of evaluations that provide direct evidence of the systems pedagogical effectiveness and the development of a multidimensional evaluation frameworks for ERS is suggested as a research opportunity. Also, it was observed a scarcity of public dataset usage on current studies that leads to limitation in terms of reproducibility and comparison of recommenders. This seems to be related to a restricted number of public datasets currently available, and such aspect can also be affecting the size of experiments conducted by researchers.
In terms of limitations of this study, the first refers to the number of datasources used for paper selection. Only the repositories mentioned in Section 3.1 were considered. Thus, the scope of this work is restricted to evidence from publications indexed by these platforms. Furthermore, only publications written in English were examined, thus, results of papers written in other languages are beyond the scope of this work. Also, the research limitations and opportunities presented on Section 4.5 were identified based on the extracted data used to answer this SLR research questions, therefore they are limited to their scope. As a consequence, limitations and opportunities of the ERS field that surpass this context were not identified nor discussed in this study. Finally, the SLR was directed to papers published in scientific journals and, due to this, the results obtained do not reflect the state of the area from the perspective of conference publications. In future research, it is intended to address such limitations.
Data availability statement
The datasets generated during the current study correspond to the papers identified through the systematic literature review and the quality evaluation results (refer to Section 3.4 in paper). They are available from the corresponding author on reasonable request.
http://parsif.al/
Anelli, V. W., Bellogín, A., Di Noia, T., & Pomo, C. (2021). Revisioning the comparison between neural collaborative filtering and matrix factorization. Proceedings of the Fifteenth ACM Conference on Recommender Systems , 521–529. https://doi.org/10.1145/3460231.3475944
Ashraf, E., Manickam, S., & Karuppayah, S. (2021). A comprehensive review of curse recommender systems in e-learning. Journal of Educators Online, 18 , 23–35. https://www.thejeo.com/archive/2021_18_1/ashraf_manickam__karuppayah
Google Scholar
Barraza-Urbina, A., & Glowacka, D. (2020). Introduction to Bandits in Recommender Systems. Proceedings of the Fourteenth ACM Conference on Recommender Systems , 748–750. https://doi.org/10.1145/3383313.3411547
Becker, F. (1993). Teacher epistemology: The daily life of the school (1st ed.). Editora Vozes.
Beel, J., Langer, S., & Genzmehr, M. (2013). Sponsored vs. Organic (Research Paper) Recommendations and the Impact of Labeling. In T. Aalberg, C. Papatheodorou, M. Dobreva, G. Tsakonas, & C. J. Farrugia (Eds.), Research and Advanced Technology for Digital Libraries (Vol. 8092, pp. 391–395). Springer Berlin Heidelberg. https://doi.org/10.1007/978-3-642-40501-3_44
Chapter Google Scholar
Betoret, F. (2007). The influence of students’ and teachers’ thinking styles on student course satisfaction and on their learning process. Educational Psychology, 27 (2), 219–234. https://doi.org/10.1080/01443410601066701
Article Google Scholar
Bobadilla, J., Serradilla, F., & Hernando, A. (2009). Collaborative filtering adapted to recommender systems of e-learning. Knowledge-Based Systems, 22 (4), 261–265. https://doi.org/10.1016/j.knosys.2009.01.008
Bobadilla, J., Ortega, F., Hernando, A., & Gutiérrez, A. (2013). Recommender systems survey. Knowledge-Based Systems, 46 , 109–132. https://doi.org/10.1016/j.knosys.2013.03.012
Buder, J., & Schwind, C. (2012). Learning with personalized recommender systems: A psychological view. Computers in Human Behavior, 28 (1), 207–216. https://doi.org/10.1016/j.chb.2011.09.002
Çano, E., & Morisio, M. (2015). Characterization of public datasets for Recommender Systems. (2015 IEEE 1 st ) International Forum on Research and Technologies for Society and Industry Leveraging a better tomorrow (RTSI) , 249–257. https://doi.org/10.1109/RTSI.2015.7325106
Cazella, S. C., Behar, P. A., Schneider, D., Silva, KKd., & Freitas, R. (2014). Developing a learning objects recommender system based on competences to education: Experience report. New Perspectives in Information Systems and Technologies, 2 , 217–226. https://doi.org/10.1007/978-3-319-05948-8_21
Cechinel, C., Sánchez-Alonso, S., & García-Barriocanal, E. (2011). Statistical profiles of highly-rated learning objects. Computers & Education, 57 (1), 1255–1269. https://doi.org/10.1016/j.compedu.2011.01.012
Cechinel, C., Sicilia, M. -Á., Sánchez-Alonso, S., & García-Barriocanal, E. (2013). Evaluating collaborative filtering recommendations inside large learning object repositories. Information Processing & Management, 49 (1), 34–50. https://doi.org/10.1016/j.ipm.2012.07.004
Chen, S. Y., & Wang, J.-H. (2021). Individual differences and personalized learning: A review and appraisal. Universal Access in the Information Society, 20 (4), 833–849. https://doi.org/10.1007/s10209-020-00753-4
Cremonesi, P., Garzotto, F., & Turrin, R. (2013). User-centric vs. system-centric evaluation of recommender systems. In P. Kotzé, G. Marsden, G. Lindgaard, J. Wesson, & M. Winckler (Eds.), Human-Computer Interaction – INTERACT 2013, 334–351. Springer Berlin Heidelberg. https://doi.org/10.1007/978-3-642-40477-1_21
Dacrema, M. F., Boglio, S., Cremonesi, P., & Jannach, D. (2021). A troubling analysis of reproducibility and progress in recommender systems research. ACM Transactions on Information Systems, 39 (2), 1–49. https://doi.org/10.1145/3434185
Dermeval, D., Coelho, J.A.P.d.M., & Bittencourt, I.I. (2020). Mapeamento Sistemático e Revisão Sistemática da Literatura em Informática na Educação. Metodologia de Pesquisa Científica em Informática na Educação: Abordagem Quantitativa . Porto Alegre. https://jodi-ojs-tdl.tdl.org/jodi/article/view/442
Drachsler, H., Hummel, H. G. K., & Koper, R. (2009). Identifying the goal, user model and conditions of recommender systems for formal and informal learning. Journal of Digital Information, 10 (2), 1–17. https://jodi-ojs-tdl.tdl.org/jodi/article/view/442
Drachsler, H., Verbert, K., Santos, O. C., & Manouselis, N. (2015). Panorama of Recommender Systems to Support Learning. In F. Ricci, L. Rokach, & B. Shapira (Eds.), Recommender Systems Handbook (pp. 421–451). Springer. https://doi.org/10.1007/978-1-4899-7637-6_12
Erdt, M., Fernández, A., & Rensing, C. (2015). Evaluating recommender systems for technology enhanced learning: A quantitative survey. IEEE Transactions on Learning Technologies, 8 (4), 326–344. https://doi.org/10.1109/TLT.2015.2438867
Felder, R. (1988). Learning and teaching styles in engineering education. Journal of Engineering Education, 78 , 674–681. Washington.
Fernandez-Garcia, A. J., Rodriguez-Echeverria, R., Preciado, J. C., Manzano, J. M. C., & Sanchez-Figueroa, F. (2020). Creating a recommender system to support higher education students in the subject enrollment decision. IEEE Access, 8 , 189069–189088. https://doi.org/10.1109/ACCESS.2020.3031572
Ferreira, V., Vasconcelos, G., & França, R. (2017). Mapeamento Sistemático sobre Sistemas de Recomendações Educacionais. Proceedings of the XXVIII Brazilian Symposium on Computers in Education , 253-262. https://doi.org/10.5753/cbie.sbie.2017.253
Garcia-Martinez, S., & Hamou-Lhadj, A. (2013). Educational recommender systems: A pedagogical-focused perspective. Multimedia Services in Intelligent Environments. Smart Innovation, Systems and Technologies, 25 , 113–124. https://doi.org/10.1007/978-3-319-00375-7_8
George, G., & Lal, A. M. (2019). Review of ontology-based recommender systems in e-learning. Computers & Education, 142 , 103642–103659. https://doi.org/10.1016/j.compedu.2019.103642
Harrathi, M., & Braham, R. (2021). Recommenders in improving students’ engagement in large scale open learning. Procedia Computer Science, 192 , 1121–1131. https://doi.org/10.1016/j.procs.2021.08.115
Herpich, F., Nunes, F., Petri, G., & Tarouco, L. (2019). How Mobile augmented reality is applied in education? A systematic literature review. Creative Education, 10 , 1589–1627. https://doi.org/10.4236/ce.2019.107115
Huang, L., Wang, C.-D., Chao, H.-Y., Lai, J.-H., & Yu, P. S. (2019). A score prediction approach for optional course recommendation via cross-user-domain collaborative filtering. IEEE Access, 7 , 19550–19563. https://doi.org/10.1109/ACCESS.2019.2897979
Iaquinta, L., Gemmis, M. de,Lops, P., Semeraro, G., Filannino, M.& Molino, P. (2008). Introducing serendipity in a content-based recommender system. Proceedings of the Eighth International Conference on Hybrid Intelligent Systems , 168-173, https://doi.org/10.1109/HIS.2008.25
Isinkaye, F. O., Folajimi, Y. O., & Ojokoh, B. A. (2015). Recommendation systems: Principles, methods and evaluation. Egyptian Informatics Journal, 16 (3), 261–273. https://doi.org/10.1016/j.eij.2015.06.005
Ismail, H. M., Belkhouche, B., & Harous, S. (2019). Framework for personalized content recommendations to support informal learning in massively diverse information Wikis. IEEE Access, 7 , 172752–172773. https://doi.org/10.1109/ACCESS.2019.2956284
Khan, K. S., Kunz, R., Kleijnen, J., & Antes, G. (2003). Five steps to conducting a systematic review. Journal of the Royal Society of Medicine, 96 (3), 118–121. https://doi.org/10.1258/jrsm.96.3.118
Khanal, S. S., Prasad, P. W. C., Alsadoon, A., & Maag, A. (2019). A systematic review: Machine learning based recommendation systems for e-learning. Education and Information Technologies, 25 (4), 2635–2664. https://doi.org/10.1007/s10639-019-10063-9
Khusro, S., Ali, Z., & Ullah, I. (2016). Recommender Systems: Issues, Challenges, and Research Opportunities. In K. Kim & N. Joukov (Eds.), Lecture Notes in Electrical Engineering (Vol. 376, pp. 1179–1189). Springer. https://doi.org/10.1007/978-981-10-0557-2_112
Kitchenham, B. A., & Charters, S. (2007). Guidelines for performing Systematic Literature Reviews in Software Engineering. Technical Report EBSE 2007–001 . Keele University and Durham University Joint Report. https://www.elsevier.com/data/promis_misc/525444systematicreviewsguide.pdf .
Kitchenham, B., Pearl Brereton, O., Budgen, D., Turner, M., Bailey, J., & Linkman, S. (2009). Systematic literature reviews in software engineering – A systematic literature review. Information and Software Technology, 51 (1), 7–15. https://doi.org/10.1016/j.infsof.2008.09.009
Klašnja-Milićević, A., Ivanović, M., & Nanopoulos, A. (2015). Recommender systems in e-learning environments: A survey of the state-of-the-art and possible extensions. Artificial Intelligence Review, 44 (4), 571–604. https://doi.org/10.1007/s10462-015-9440-z
Klašnja-Milićević, A., Vesin, B., & Ivanović, M. (2018). Social tagging strategy for enhancing e-learning experience. Computers & Education, 118 , 166–181. https://doi.org/10.1016/j.compedu.2017.12.002
Kolb, D., Boyatzis, R., Mainemelis, C., (2001). Experiential Learning Theory: Previous Research and New Directions Perspectives on Thinking, Learning and Cognitive Styles , 227–247.
Krahenbuhl, K. S. (2016). Student-centered Education and Constructivism: Challenges, Concerns, and Clarity for Teachers. The Clearing House: A Journal of Educational Strategies, Issues and Ideas, 89 (3), 97–105. https://doi.org/10.1080/00098655.2016.1191311
Kunaver, M., & Požrl, T. (2017). Diversity in recommender systems – A survey. Knowledge-Based Systems, 123 , 154–162. https://doi.org/10.1016/j.knosys.2017.02.009
Manouselis, N., Drachsler, H., Vuorikari, R., Hummel, H., & Koper, R. (2010). Recommender systems in technology enhanced learning. In F. Ricci, L. Rokach, B. Shapira, & P. Kantor (Eds.), Recommender Systems Handbook (pp. 387–415). Springer. https://doi.org/10.1007/9780-387-85820-3_12
Manouselis, N., Drachsler, H., Verbert, K., & Santos, O. C. (2014). Recommender systems for technology enhanced learning . Springer. https://doi.org/10.1007/978-1-4939-0530-0
Book Google Scholar
Manouselis, N., Drachsler, H., Verbert, K., & Duval, E. (2013). Challenges and Outlook. Recommender Systems for Learning , 63–76. https://doi.org/10.1007/978-1-4614-4361-2
Maravanyika, M., & Dlodlo, N. (2018). An adaptive framework for recommender-based learning management systems. Open Innovations Conference (OI), 2018 , 203–212. https://doi.org/10.1109/OI.2018.8535816
Maria, S. A. A., Cazella, S. C., & Behar, P. A. (2019). Sistemas de Recomendação: conceitos e técnicas de aplicação. Recomendação Pedagógica em Educação a Distância , 19–47, Penso.
McCombs, B. L. (2013). The Learner-Centered Model: Implications for Research Approaches. In Cornelius-White, J., Motschnig-Pitrik, R. & Lux, M. (eds), Interdisciplinary Handbook of the Person-Centered Approach , 335–352. 10.1007/ 978-1-4614-7141-7_23
Medeiros, R. P., Ramalho, G. L., & Falcao, T. P. (2019). A systematic literature review on teaching and learning introductory programming in higher education. IEEE Transactions on Education, 62 (2), 77–90. https://doi.org/10.1109/te.2018.2864133
Moher, D., Shamseer, L., Clarke, M., Ghersi, D., Liberati, A., Petticrew, M., Shekelle, P., Stewart, L. A., PRISMA-P Group. (2015). Preferred reporting items for systematic review and meta-analysis protocols (PRISMA-P) 2015 statement. Systematic Reviews, 4 (1), 1. https://doi.org/10.1186/2046-4053-4-1
Moubayed, A., Injadat, M., Nassif, A. B., Lutfiyya, H., & Shami, A. (2018). E-Learning: Challenges and research opportunities using machine learning & data analytics. IEEE Access, 6 , 39117–39138. https://doi.org/10.1109/access.2018.2851790
Nabizadeh, A. H., Gonçalves, D., Gama, S., Jorge, J., & Rafsanjani, H. N. (2020). Adaptive learning path recommender approach using auxiliary learning objects. Computers & Education, 147 , 103777–103793. https://doi.org/10.1016/j.compedu.2019.103777
Nafea, S. M., Siewe, F., & He, Y. (2019). On Recommendation of learning objects using Felder-Silverman learning style model. IEEE Access, 7 , 163034–163048. https://doi.org/10.1109/ACCESS.2019.2935417
Nascimento, P. D., Barreto, R., Primo, T., Gusmão, T., & Oliveira, E. (2017). Recomendação de Objetos de Aprendizagem baseada em Modelos de Estilos de Aprendizagem: Uma Revisão Sistemática da Literatura. Proceedings of XXVIII Brazilian Symposium on Computers in Education- SBIE, 2017 , 213–222. https://doi.org/10.5753/cbie.sbie.2017.213
Nguyen, Q. H., Ly, H.-B., Ho, L. S., Al-Ansari, N., Le, H. V., Tran, V. Q., Prakash, I., & Pham, B. T. (2021). Influence of data splitting on performance of machine learning models in prediction of shear strength of soil. Mathematical Problems in Engineering, 2021 , 1–15. https://doi.org/10.1155/2021/4832864
Nichols, D. M. (1998). Implicit rating and filtering. Proceedings of the Fifth Delos Workshop: Filtering and Collaborative Filtering , 31–36.
Okoye, I., Maull, K., Foster, J., & Sumner, T. (2012). Educational recommendation in an informal intentional learning system. Educational Recommender Systems and Technologies , 1–23. https://doi.org/10.4018/978-1-61350-489-5.ch001
Pai, M., McCulloch, M., Gorman, J. D., Pai, N., Enanoria, W., Kennedy, G., Tharyan, P., & Colford, J. M., Jr. (2004). Systematic reviews and meta-analyses: An illustrated, step-by-step guide. The National Medical Journal of India, 17 (2), 86–95.
Petri, G., & Gresse von Wangenheim, C. (2017). How games for computing education are evaluated? A systematic literature review. Computers & Education, 107 , 68–90. https://doi.org/10.1016/j.compedu.2017.01.00
Petticrew, M., & Roberts, H. (2006). Systematic reviews in the social sciences a practical guide. Blackwell Publishing . https://doi.org/10.1002/9780470754887
Pinho, P. C. R., Barwaldt, R., Espindola, D., Torres, M., Pias, M., Topin, L., Borba, A., & Oliveira, M. (2019). Developments in educational recommendation systems: a systematic review. Proceedings of 2019 IEEE Frontiers in Education Conference (FIE) . https://doi.org/10.1109/FIE43999.2019.9028466
Pöntinen, S., Dillon, P., & Väisänen, P. (2017). Student teachers’ discourse about digital technologies and transitions between formal and informal learning contexts. Education and Information Technologies, 22 (1), 317–335. https://doi.org/10.1007/s10639-015-9450-0
Pu, P., Chen, L., & Hu, R. (2011). A user-centric evaluation framework for recommender systems. Proceedings of the fifth ACM conference on Recommender systems , 157–164. https://doi.org/10.1145/2043932.2043962
Rahman, M. M., & Abdullah, N. A. (2018). A personalized group-based recommendation approach for web search in E-Learning. IEEE Access, 6 , 34166–34178. https://doi.org/10.1109/ACCESS.2018.2850376
Ricci, F., Rokach, L., & Shapira, B. (2015). Recommender Systems: Introduction and Challenges. I Ricci, F., Rokach, L., Shapira, B. (eds), Recommender Systems Handbook , 1–34. https://doi.org/10.1007/978-1-4899-7637-6_1
Rivera, A. C., Tapia-Leon, M., & Lujan-Mora, S. (2018). Recommendation Systems in Education: A Systematic Mapping Study. Proceedings of the International Conference on Information Technology & Systems (ICITS 2018) , 937–947. https://doi.org/10.1007/978-3-319-73450-7_89
Salazar, C., Aguilar, J., Monsalve-Pulido, J., & Montoya, E. (2021). Affective recommender systems in the educational field. A systematic literature review. Computer Science Review, 40 , 100377. https://doi.org/10.1016/j.cosrev.2021.100377
Santos, I. M., & Ali, N. (2012). Exploring the uses of mobile phones to support informal learning. Education and Information Technologies, 17 (2), 187–203. https://doi.org/10.1007/s10639-011-9151-2
Sergis, S., & Sampson, D. G. (2016). Learning object recommendations for teachers based on elicited ICT competence profiles. IEEE Transactions on Learning Technologies, 9 (1), 67–80. https://doi.org/10.1109/TLT.2015.2434824
Shani, G., & Gunawardana, A. (2010). Evaluating recommendation systems. In F. Ricci, L. Rokach, B. Shapira, & P. Kantor (Eds.), Recommender Systems Handbook (pp. 257–297). Springer. https://doi.org/10.1007/978-0-387-85820-3_8
Tahereh, M., Maryam, T. M., Mahdiyeh, M., & Mahmood, K. (2013). Multi dimensional framework for qualitative evaluation in e-learning. 4th International Conference on e-Learning and e-Teaching (ICELET 2013), 69–75. https://doi.org/10.1109/icelet.2013.6681648
Tarus, J. K., Niu, Z., & Yousif, A. (2017). A hybrid knowledge-based recommender system for e-learning based on ontology and sequential pattern mining. Future Generation Computer Systems, 72 , 37–48. https://doi.org/10.1016/j.future.2017.02.049
Tarus, J. K., Niu, Z., & Mustafa, G. (2018). Knowledge-based recommendation: A review of ontology-based recommender systems for e-learning. Artificial Intelligence Review, 50 (1), 21–48. https://doi.org/10.1007/s10462-017-9539-5
Verbert, K., Manouselis, N., Ochoa, X., Wolpers, M., Drachsler, H., Bosnic, I., & Duval, E. (2012). Context-aware recommender systems for learning: A survey and future challenges. IEEE Transactions on Learning Technologies, 5 (4), 318–335. https://doi.org/10.1109/TLT.2012.11
Verbert, K., Drachsler, H., Manouselis, N., Wolpers, M., Vuorikari, R., & Duval, E. (2011). Dataset-Driven Research for Improving Recommender Systems for Learning. Proceedings of the 1st International Conference on Learning Analytics and Knowledge , 44–53. https://doi.org/10.1145/2090116.2090122
Wan, S., & Niu, Z. (2016). A learner oriented learning recommendation approach based on mixed concept mapping and immune algorithm. Knowledge-Based Systems, 103 , 28–40. https://doi.org/10.1016/j.knosys.2016.03.022
Wan, S., & Niu, Z. (2018). An e-learning recommendation approach based on the self-organization of learning resource. Knowledge-Based Systems, 160 , 71–87. https://doi.org/10.1016/j.knosys.2018.06.014
Wan, S., & Niu, Z. (2020). A hybrid E-Learning recommendation approach based on learners’ influence propagation. IEEE Transactions on Knowledge and Data Engineering, 32 (5), 827–840. https://doi.org/10.1109/TKDE.2019.2895033
Watkins, K. E., & Marsick, V. J. (2020). Informal and incidental learning in the time of COVID-19. Advances in Developing Human Resources, 23 (1), 88–96. https://doi.org/10.1177/1523422320973656
Wu, D., Lu, J., & Zhang, G. (2015). A Fuzzy Tree Matching-based personalized E-Learning recommender system. IEEE Transactions on Fuzzy Systems, 23 (6), 2412–2426. https://doi.org/10.1109/TFUZZ.2015.2426201
Wu, Z., Li, M., Tang, Y., & Liang, Q. (2020). Exercise recommendation based on knowledge concept prediction. Knowledge-Based Systems, 210 , 106481–106492. https://doi.org/10.1016/j.knosys.2020.106481
Yanes, N., Mostafa, A. M., Ezz, M., & Almuayqil, S. N. (2020). A machine learning-based recommender system for improving students learning experiences. IEEE Access, 8 , 201218–201235. https://doi.org/10.1109/ACCESS.2020.3036336
Zapata, A., Menéndez, V. H., Prieto, M. E., & Romero, C. (2015). Evaluation and selection of group recommendation strategies for collaborative searching of learning objects. International Journal of Human-Computer Studies, 76 , 22–39. https://doi.org/10.1016/j.ijhcs.2014.12.002
Zhang, S., Yao, L., Sun, A., & Tay, Y. (2020). Deep learning based recommender system. ACM Computing Surveys, 52 (1), 1–38. https://doi.org/10.1145/3285029
Zhong, J., Xie, H., & Wang, F. L. (2019). The research trends in recommender systems for e-learning: A systematic review of SSCI journal articles from 2014 to 2018. Asian Association of Open Universities Journal, 14 (1), 12–27. https://doi.org/10.1108/AAOUJ-03-2019-0015
Download references
Author information
Authors and affiliations.
Centro de Estudos Interdisciplinares em Novas Tecnologias da Educação, Universidade Federal do Rio Grande do Sul, Porto Alegre, Rio Grande do Sul, Brazil
Felipe Leite da Silva, Bruna Kin Slodkowski, Ketia Kellen Araújo da Silva & Sílvio César Cazella
Departamento de Ciências Exatas e Sociais Aplicadas, Universidade Federal de Ciências da Saúde de Porto Alegre, Porto Alegre, Rio Grande do Sul, Brazil
Sílvio César Cazella
You can also search for this author in PubMed Google Scholar
Contributions
Felipe Leite da Silva: Conceptualization, Methodology approach, Data curation, Writing – original draft. Bruna Kin Slodkowski: Data curation, Writing – original draft. Ketia Kellen Araújo da Silva: Data curation, Writing – original draft. Sílvio César Cazella: Supervision and Monitoring of the research; Writing – review & editing.
Corresponding author
Correspondence to Felipe Leite da Silva .
Ethics declarations
Informed consent.
This research does not involve human participation as research subject, therefore research subject consent does not apply.
Authors consent with the content presented in the submitted manuscript.
Financial and non-financial interests
The authors have no relevant financial or non-financial interests to disclose.
Research involving human participants and/or animals
This research does not involve an experiment with human or animal participation.
Competing interests
The authors have no competing interests to declare that are relevant to the content of this article.
Additional information
Publisher's note.
Springer Nature remains neutral with regard to jurisdictional claims in published maps and institutional affiliations.
Electronic supplementary material
Below is the link to the electronic supplementary material.
Supplementary file1 (PDF 241 KB)
Rights and permissions.
Springer Nature or its licensor holds exclusive rights to this article under a publishing agreement with the author(s) or other rightsholder(s); author self-archiving of the accepted manuscript version of this article is solely governed by the terms of such publishing agreement and applicable law.
Reprints and permissions
About this article
da Silva, F.L., Slodkowski, B.K., da Silva, K.K.A. et al. A systematic literature review on educational recommender systems for teaching and learning: research trends, limitations and opportunities. Educ Inf Technol 28 , 3289–3328 (2023). https://doi.org/10.1007/s10639-022-11341-9
Download citation
Received : 05 November 2021
Accepted : 05 September 2022
Published : 14 September 2022
Issue Date : March 2023
DOI : https://doi.org/10.1007/s10639-022-11341-9
Share this article
Anyone you share the following link with will be able to read this content:
Sorry, a shareable link is not currently available for this article.
Provided by the Springer Nature SharedIt content-sharing initiative
- Educational recommender systems
- E-learning recommendation
- Systematic literature review
- Computer-mediated teaching and learning
- Find a journal
- Publish with us
- Track your research

- Areas of Study
- Courses and Curriculum
- Open Courses
- Register for a Program
- Certificate Program
- Associate in Actuarial Science
- Associate in Addiction Counseling
- Associate in Agriculture Food And Resources
- Associate in Animal Science
- Associate in Anti Terrorism Security
- Associate in Behavior Analysis In Special Education
- Associate in Bioethics
- Associate in Biosystems
- Associate in Blockchain Technology & Digital Currency
- Associate in Business Communication
- Associate in Business Management
- Associate in Chemistry
- Associate in Climatology
- Associate in Cloud Computing
- Associate in Computer Engineering
- Associate in Computer Programming
- Associate in Computer Science
- Associate in Criminal Justice
- Associate in Culinary Arts
- Associate in Cultural Theological Communication
- Associate in Cybersecurity And Hacking
- Associate in Data Communication And Networking
- Associate in Database Administrator
- Associate in Early Childhood Education
- Associate in Ecotechnology
- Associate in Ecotourism
- Associate in Education
- Associate in Educational Technology
- Associate in Electric Vehicle Engineering
- Associate in Electrochemical Engineering
- Associate in Electronic Engineering
- Associate in English Literature
- Associate in Environmental Science
- Associate in eVTOL Engineering
- Associate in Fashion Design
- Associate in Fine Arts
- Associate in Foreign Trade
- Associate in Genetic Engineering
- Associate in Geography
- Associate in Geology
- Associate in Geophysical Sciences
- Associate in Graphic Design
- Associate in Health Sciences
- Associate in History
- Associate in Human Resources
- Associate in Integrated Water Management
- Associate in International Finance
- Associate in International Marketing
- Associate in Islamic Studies
- Associate in Kinesiology And Physiotherapy
- Associate in Library technology
- Associate in Linguistics
- Associate in Management
- Associate in Management Information Systems
- Associate in Maritime Management
- Associate in Metallurgy
- Associate in Micro and Multimode Grid Design
- Associate in Modern Power and Energy Systems
- Associate in Multimedia Design and Digital Art
- Associate in Nutrition
- Associate in Oil Gas And Energy Engineering
- Associate in Operations Management
- Associate in Optoelectronic Systems
- Associate in Organizational Development
- Associate in Organizational Diversity
- Associate in Pedagogical Training For Professionals
- Associate in Physical Culture And Sports
- Associate in Physics
- Associate in Public Health
- Associate in Quantum Computing Technology
- Associate in Radio And Television Production
- Associate in Scenography
- Associate in Social Media Marketing
- Associate in Sociology
- Associate in Sports Marketing
- Associate in Sports Psychology
- Associate in Sustainable Design and Construction
- Associate in Sustainable Materials Science
- Associate in Sustainable Natural Resources Management
- Associate in Sustainable Tourism
- Associate in Synthetic Biology
- Associate in Virtual Archival Science
- Associate of Adult Education
- Associate of Advertising
- Associate of Agriculture
- Associate of American History
- Associate of Biology
- Associate of Chemical Engineering
- Associate of Civil Engineering
- Associate of Communications
- Associate of Construction Management
- Associate of Economics
- Associate of Educational Administration
- Associate of Electrical Engineering
- Associate of Finance
- Associate of Healthcare Administration
- Associate of Human Resources Management
- Associate of Humanities
- Associate of Hydrology
- Associate of Industrial Engineering
- Associate of Information Systems
- Associate of Information Technology
- Associate of Interior Design
- Associate of International Relations
- Associate of Journalism
- Associate of Legal Studies
- Associate of Logistics
- Associate of Marketing
- Associate of Mass Media and Communication
- Associate of Mathematics
- Associate of Mechanical Engineering
- Associate of Mining Engineering
- Associate of Music
- Associate of Nutrition Science
- Associate of Philosophy
- Associate of Physical Education
- Associate of Political Science
- Associate of Project Management
- Associate of Psychology
- Associate of Renewable Energy
- Associate of Software Engineering
- Associate of Sport Science
- Associate of Statistics
- Associate of Strategic Management
- Associate of Technical Writing
- Associate of Telecommunications
- Associate of Theater
- Associate of Theology
- Associate of Tourism Planning and Development
- Associate of Travel and Tourism
- Associate of Unmanned Areal Systems Engineering
- Associates in Artificial Intelligence
- Associates in Engineering Systems
- Associates in Physical Anthropology
- Associates in Social Sciences
- Associates in Sociocultural Anthropology
- Associates in Systems Engineering
- Associates of Accounting
- Associates of Anthropology
- Associates of Archeology
- Associates of Architecture
- Associates of Art History
- Associates of Banking and Finance
- Associates of Business Administration
- Associates of Public Administration
- Associates of Science
- Associates of Urban Planning
- Associates of Visual and Performing Arts
- Micro and Multimode Grid Design
- Bachelor in Data Communication and Networking
- Bachelor in Actuarial Science
- Bachelor in Addiction Counseling
- Bachelor in Agriculture, Food and Resources
- Bachelor in Animal Science
- Bachelor in Anti Terrorism Security
- Bachelor in Artificial Intelligence
- Bachelor in Arts in Cultural Theological Communication
- Bachelor in Autonomous Vehicle Technology
- Bachelor in Bachelor of Bioethics (BA)
- Bachelor in Behavior Analysis in Special Education
- Bachelor in Bibliotechnology
- Bachelor in Biosystems
- Bachelor in Blockchain Technology & Digital Currency
- Bachelor in Business Communication
- Bachelor in Business Management
- Bachelor in Chemistry
- Bachelor in Cloud Computing
- Bachelor in Computer Science
- Bachelor in Criminal Justice
- Bachelor in Culinary Arts
- Bachelor in Cybersecurity and Hacking
- Bachelor in Database Administrator (BS)
- Bachelor in Ecotechnology
- Bachelor in Ecotourism
- Bachelor in Education (B.Ed, BS)
- Bachelor in Educational Technology
- Bachelor in Electric Vehicle Engineering
- Bachelor in Electrochemical Engineering
- Bachelor in English Literature
- Bachelor in eVTOL Engineering
- Bachelor in Fashion Design (BA)
- Bachelor in Fine Arts
- Bachelor in Foreign Trade
- Bachelor in Genetic Engineering
- Bachelor in Geology
- Bachelor in Geophysical Sciences
- Bachelor in Graphic Design
- Bachelor in Health Sciences
- Bachelor in Integrated Water Management
- Bachelor in International Finance
- Bachelor in International Marketing
- Bachelor in Islamic Studies
- Bachelor in Kinesiology and Physiotherapy
- Bachelor in Linguistics
- Bachelor in Management
- Bachelor in Metallurgy
- Bachelor in Micro and Multimode Grid Design
- Bachelor in Modern Power and Energy Systems
- Bachelor in Multimedia Design and Digital Art
- Bachelor in Nutrigenetics
- Bachelor in Operations Management (BA)
- Bachelor in Optoelectronic Systems
- Bachelor in Organizational Development
- Bachelor in Organizational Diversity
- Bachelor in Physical Anthropology
- Bachelor in Physical Culture And Sports
- Bachelor in Public Relations
- Bachelor in Quantum Computing Technology
- Bachelor in Radio And Television Production
- Bachelor in Scenography
- Bachelor in Social Media Marketing
- Bachelor in Social Sciences
- Bachelor in Sociocultural Anthropology
- Bachelor in Sport Management
- Bachelor in Sports Marketing
- Bachelor in Sports Psychology
- Bachelor in Sustainable Design and Construction
- Bachelor in Sustainable Materials Science
- Bachelor in Sustainable Natural Resources Management
- Bachelor in Sustainable Tourism
- Bachelor in Synthetic Biology
- Bachelor in Virtual Archival Science
- Bachelor of Adult Education
- Bachelor of Advertising
- Bachelor of Animation
- Bachelor of Art History
- Bachelor of Biohacking and Nutrigenomics
- Bachelor of Educational Administration
- Bachelor of Healthcare Administration
- Bachelor of Human Resources Management
- Bachelor of Hydrology
- Bachelor of Information Technology
- Bachelor of Mass Media and Communication
- Bachelor of Philosophy
- Bachelor of Physical Education
- Bachelor of Project Management
- Bachelor of Public Administration
- Bachelor of Software Engineering
- Bachelor of Sport Science
- Bachelor of Sports Science
- Bachelor of Statistics
- Bachelor of Strategic Management
- Bachelor of Technical Writing
- Bachelor of Theater
- Bachelor of Theology
- Bachelor of Tourism Planning and Development
- Bachelor of Travel and Tourism
- Bachelor of Unmanned Areal Systems Engineering
- Bachelor of Urban Planning
- Bachelor of Visual and Performing Arts
- Bachelor of Web Design
- Bachelors in Energy Storage and Battery Technology
- Bachelors in Accounting
- Bachelors in Accounting & Finance
- Bachelors in Agronomy Engineering
- Bachelors in Anthropology
- Bachelors in Architecture
- Bachelors in Automotive Engineering
- Bachelors in Banking and Finance
- Bachelors in Biology
- Bachelors in Business Administration
- Bachelors in Chemical Engineering
- Bachelors in Civil Engineering
- Bachelors in Communications
- Bachelors in Computer Engineering
- Bachelors in Criminal Justice
- Bachelors in Criminology
- Bachelors in Early Childhood Education
- Bachelors in Economics
- Bachelors in Electrical Engineering
- Bachelors in Electromechanical Engineering
- Bachelors in Electronic Engineering
- Bachelors in Engineering
- Bachelors in Environmental Science
- Bachelors in Finance
- Bachelors in Finance and Banking
- Bachelors in Health Sciences
- Bachelors in History
- Bachelors in Hospitality Management
- Bachelors in Human Resources
- Bachelors in Humanities
- Bachelors in Industrial Engineering
- Bachelors in Information Systems
- Bachelors in Interior Design
- Bachelors in International Business
- Bachelors in International Relations
- Bachelors in Journalism
- Bachelors in Legal Studies
- Bachelors in Logistics
- Bachelors in Marketing
- Bachelors in Mathematics
- Bachelors in Mechanical Engineering
- Bachelors in Mine Engineering
- Bachelors in Mining Engineering
- Bachelors in Music
- Bachelors in Nutrition
- Bachelors in Oil Gas and Energy Engineering
- Bachelors in Pedagogy
- Bachelors in Physics
- Bachelors in Political Science
- Bachelors in Psychology
- Bachelors in Public Health
- Bachelors in Public Security
- Bachelors in Renewable Energy
- Bachelors in Sociology
- Bachelors in Systems Engineering
- Bachelors in Telecommunications
- Bachelors in Zoology
- DBA – Doctor of Business Administration
- Doctor | in Actuarial Science
- Doctor | in Agriculture Food And Resources
- Doctor | in Animal Science
- Doctor | in Biosystems
- Doctor | in Cultural Theological Communication
- Doctor | in Cybersecurity And Hacking
- Doctor | in Early Childhood Education
- Doctor | in Ecotechnology
- Doctor | In Ecotourism
- Doctor | In Educational Technology
- Doctor | In Electronic Engineering
- Doctor | In Foreign Trade
- Doctor | of Biology (PhD)
- Doctor in Addiction Counseling
- Doctor in Behavior Analysis In Special Education
- Doctor in Bibliotechnology
- Doctor in Business Management
- Doctor in Data Communication And Networking
- Doctor of Adult Education
- Doctor of Agriculture
- Doctor of American History
- Doctor of Animation
- Doctor of Anthropology
- Doctor of Archaeology
- Doctor of Architecture (Ph.D.)
- Doctor of Art History
- Doctor of Artificial Intelligence
- Doctor of Autonomous Vehicle Technology
- Doctor of Biohacking and Nutrigenomics
- Doctor of Business Management (DBM)
- Doctor of Civil Engineering (D.Sc)
- Doctor of Cloud Computing
- Doctor of Economics (PhD)
- Doctor of Educational Administration (PhD)
- Doctor of Electric Vehicle Engineering
- Doctor of Electrical Engineering (D.Sc, PhD)
- Doctor of Electrochemical Engineering
- Doctor of Engineering Systems (D.Sc)
- Doctor of eVTOL Engineering
- Doctor of Finance (PhD)
- Doctor of Humanities
- Doctor of Hydrology
- Doctor of Industrial Engineering (D.Sc)
- Doctor of International Relations (D.Sc)
- Doctor of Legal Studies (PhD)
- Doctor of Logistics
- Doctor of Mass Media and Communication
- Doctor of Mechanical Engineering (D.Sc)
- Doctor of Micro and Multimode Grid Design
- Doctor of Mining Engineering
- Doctor of Music
- Doctor of Nutrition Science
- Doctor of Optoelectronic Systems
- Doctor of Project Management (PhD)
- Doctor of Public Administration
- Doctor of Public Health (PhD, D.Sc)
- Doctor of Quantum Computing
- Doctor of Renewable Energy
- Doctor of Sociology (PhD, D.Sc)
- Doctor of Software Engineering
- Doctor of Sport Management
- Doctor of Sport Science
- Doctor of Statistics
- Doctor of Technical Writing
- Doctor of Telecommunications (D.Sc)
- Doctor of Theater
- Doctor of Tourism Planning and Development
- Doctor of Travel and Tourism
- Doctor of Unmanned Aerial Systems Engineering
- Doctor of Visual and Performing Arts
- Doctor of Web Design
- Doctoral Degree Programs
- Doctoral in International Finance
- Doctorate in Accounting
- Doctorate in Actuarial Science
- Doctorate in Adult Counseling
- Doctorate in Advertising
- Doctorate in Agriculture Food And Resources
- Doctorate in Animal Science
- Doctorate in Anti Terrorism Security
- Doctorate in Behavior Analysis In Special Education
- Doctorate in Bibliotechnology
- Doctorate in Bioethics
- Doctorate in Biosystems
- Doctorate in Business Administration (DBA, PhD)
- Doctorate in Business Communication
- Doctorate in Business Management
- Doctorate in Chemical Engineering (D.Sc)
- Doctorate in Chemistry
- Doctorate in Clinical Nutrition
- Doctorate in Communication Online (D.Sc)
- Doctorate in Computer Engineering
- Doctorate in Computer Science
- Doctorate in Conflict Resolution & Peace Building
- Doctorate in Criminal Justice
- Doctorate in Culinary Arts
- Doctorate in Cultural Theological Communication
- Doctorate in Cyber Security & Hacking
- Doctorate in Data Communication And Networking
- Doctorate in Digital Marketing
- Doctorate in Early Childhood Education
- Doctorate in Ecotechnology
- Doctorate in Ecotourism
- Doctorate in Education
- Doctorate in Educational Technology
- Doctorate in Electronic Engineering
- Doctorate in Environmental Science
- Doctorate in Fashion Design
- Doctorate in Fine Arts
- Doctorate in Foreign Trade
- Doctorate in Genetic Engineering
- Doctorate in Geophysical Sciences
- Doctorate in Global Health
- Doctorate in Health Sciences
- Doctorate in Healthcare Administration Programs (PhD)
- Doctorate in Hospital Administration
- Doctorate in Human Resource Management (PhD)
- Doctorate in Human Resources
- Doctorate in Information Systems (D.Sc)
- Doctorate in Information Technology (D.Sc)
- Doctorate in Integrated Water Management
- Doctorate in Interior Design (PhD)
- Doctorate in International Marketing
- Doctorate in International Relations
- Doctorate in Islamic Studies
- Doctorate in Journalism (PhD)
- Doctorate in Kinesiology & Physiotherapy
- Doctorate in Linguistics
- Doctorate in Management
- Doctorate in Maritime Management
- Doctorate in Marketing (PhD)
- Doctorate in Mathematics
- Doctorate in Metallurgy
- Doctorate in Modern Power and Energy Systems
- Doctorate in Multimedia Design and Digital Art
- Doctorate in Oil Gas And Energy Engineering
- Doctorate in Organizational Development
- Doctorate in Organizational Diversity
- Doctorate in Pedagogical Training For Professionals
- Doctorate in Physical Anthropology
- Doctorate in Physical Culture And Sports
- Doctorate in Physical Education (D.Sc)
- Doctorate in Political Science
- Doctorate in Psychology (PhD, DPsy)
- Doctorate in Radio And Television Production
- Doctorate in Scenography
- Doctorate in Security Management
- Doctorate in Social Media Marketing
- Doctorate in Sociocultural Anthropology
- Doctorate in Sports Management
- Doctorate in Sports Marketing
- Doctorate in Sports Psychology
- Doctorate in Strategic Leadership
- Doctorate in Strategic Management
- Doctorate in Sustainable Design and Construction
- Doctorate in Sustainable Materials Science
- Doctorate in Sustainable Natural Resources Management
- Doctorate in Sustainable Tourism
- Doctorate in Synthetic Biology
- Doctorate in Virtual Archival Science
- Doctorate of Theology
- DS – Doctorate in Science
- Online Doctorate in Health Administration
- Online Doctorate in Hospitality
- Online Doctorate in Philosophy
- Postdoctoral in Bioethics
- School of Social and Human Studies
- Master in Actuarial Science
- Master in Addiction Counseling
- Master in Agriculture Food And Resources
- Master in Animal Science
- Master in Anti Terrorism Security
- Master in Autonomous Vehicle Technology
- Master in Behavior Analysis In Special Education
- Master in Bibliotechnology
- Master in Bioethics
- Master in Biosystems
- Master in Blockchain Technology and Digital Currency
- Master in Business Communication
- Master in Chemistry
- Master in Climatology
- Master in Cloud Computing
- Master in Computer Programming
- Master in Criminal Justice
- Master in Culinary Arts
- Master in Cultural Theological Communication
- Master in Cybersecurity And Hacking
- Master in Data Communication And Networking
- Master in Database Administrator
- Master in Early Childhood Education
- Master in Ecotechnology
- Master in Ecotourism
- Master in Educational Technology
- Master in Electric Vehicle Engineering
- Master in Electronic Engineering
- Master in Energy Storage and Battery Technology
- Master in Engineering Systems (MS)
- Master in eVTOL Engineering
- Master in Fashion Design
- Master in Fine Arts
- Master in Foreign Trade
- Master in Geography
- Master in Geophysical Sciences
- Master in Graphic Design
- Master in Health Sciences
- Master in History
- Master in Industrial Engineering
- Master in Integrated Water Management
- Master in International Finance
- Master in Islamic Studies
- Master in Kinesiology And Physiotherapy
- Master in Linguistics
- Master in Management
- Master in Management Information Systems
- Master in Mass Media and Communication
- Master in Metallurgy
- Master in Micro and Multimode Grid Design
- Master in Microbiology
- Master in Modern Power and Energy Systems
- Master in Multimedia Design and Digital Art
- Master in Nutritional Science
- Master in Oil Gas And Energy Engineering
- Master in Organizational Development
- Master in Organizational Diversity
- Master in Pedagogical Training For Professionals
- Master in Philosophy
- Master in Physical Anthropology
- Master in Physical Culture And Sports
- Master in Public Administration (MA)
- Master in Quantum Computing
- Master in Radio And Television Production
- Master in Scenography
- Master in Social Media Marketing
- Master in Social Sciences
- Master in Sociocultural Anthropology
- Master in Sociology (MA, MS)
- Master in Software Engineering
- Master in Sport Management
- Master in Sport Science
- Master in Sports Marketing
- Master in Sports Psychology
- Master in Statistics
- Master in Strategic Management
- Master in Sustainable Design and Construction
- Master in Sustainable Materials Science
- Master in Sustainable Natural Resources Management
- Master in Sustainable Tourism
- Master in Synthetic Biology
- Master in Technical Writing
- Master in Theater
- Master in Theology
- Master in Unmanned Aerial Systems Engineering (UAV and Drone Technology)
- Master in Virtual Archival Science
- Master of Adult Education
- Master of Advertising (MS, MBA)
- Master of Agriculture (MS)
- Master of American History
- Master of Animation
- Master of Anthropology (MA)
- Master of Archeology (MA)
- Master of Art History (MA)
- Master of Artificial Intelligence
- Master of Banking and Finance (MA)
- Master of Biohacking and Nutrigenomics
- Master of Biology (MS)
- Master of Business Management (MS, MBM)
- Master of Chemical Engineering (MS)
- Master of Computer Engineering
- Master of Construction Management
- Master of Human Resources Management (MHRM)
- Master of Humanities (MA)
- Master of Hydrology (MS)
- Master of Interior Design (MA)
- Master of International Relations (MS)
- Master of Journalism (MA, MJ)
- Master of Logistics (MA)
- Master of Marketing (MS, MBA, MPA)
- Master of Mathematics (MS)
- Master of Mechanical Engineering (MS)
- Master of Mining Engineering (MS)
- Master of Music (MA)
- Master of Nutrition Science
- Master of Physical Education (MS)
- Master of Renewable Energy (MS)
- Master of Science in Educational Administration (MS)
- Master of Science in Healthcare Administration (MS)
- Master of Tourism Planning and Development
- Master of Travel and Tourism
- Master of Urban Planning
- Master of Visual and Performing Arts
- Master of Web Design
- Masters in Accounting
- Masters in Architecture
- Masters in Business Administration
- Masters in Business Management
- Masters in Civil Engineering
- Masters in Communication
- Masters in Computer Science
- Masters in Economics
- Masters in Education
- Masters in Electrical Engineering
- Masters in Environmental Science
- Masters in Finance
- Masters in Human Resources
- Masters in Information Systems
- Masters in Information Technology
- Masters in International Business
- Masters in International Marketing
- Masters in International Relations
- Masters in Legal Studies
- Masters in Political Science
- Masters in Project Management
- Masters in Psychology
- Masters in Public Health
- Masters in Telecommunications
- Postdoctoral in Actuarial Science
- Postdoctoral in Addiction Counseling
- Postdoctoral in Animal Science
- Postdoctoral in Anti Terrorism Security
- Postdoctoral in Autonomous Vehicle Technology
- Postdoctoral in Behavior Analysis In Special Education
- Postdoctoral in Bibliotechnology
- Postdoctoral in Biohacking and Nutrigenomics
- Postdoctoral in Biosystems
- Postdoctoral in Blockchain Technology and Digital Currency
- Postdoctoral in Business Communication
- Postdoctoral in Business Management
- Postdoctoral in Chemistry
- Postdoctoral in Cloud Computing
- Postdoctoral in Computer Engineering
- Postdoctoral in Computer Science
- Postdoctoral in Criminal Justice
- Postdoctoral in Culinary Arts
- Postdoctoral in Cultural Theological Communication
- Postdoctoral in Cybersecurity And Hacking
- Postdoctoral in Data Communication And Networking
- Postdoctoral in Early Childhood Education
- Postdoctoral in Ecotechnology
- Postdoctoral in Ecotourism
- Postdoctoral in Education
- Postdoctoral in Educational Technology
- Postdoctoral in Electrical Vehicle Engineering
- Postdoctoral in Electrochemical Engineering
- Postdoctoral in Electronic Engineering
- Postdoctoral in Energy Storage and Battery Technology
- Postdoctoral in Engineering Systems
- Postdoctoral in English Literature
- Postdoctoral in Environmental Science
- Postdoctoral in eVTOL Engineering
- Postdoctoral in Fashion Design
- Postdoctoral in Fine Arts
- Postdoctoral in Foreign Trade
- Postdoctoral in Genetic Engineering
- Postdoctoral in Geophysical Sciences
- Postdoctoral in Graphic Design
- Postdoctoral in Health Sciences
- Postdoctoral in Human Resources
- Postdoctoral in Humanities
- Postdoctoral in Integrated Water Management
- Postdoctoral in International Finance
- Postdoctoral in International Marketing
- Postdoctoral in International Relations
- Postdoctoral in Islamic Studies
- Postdoctoral in Journalism
- Postdoctoral in Kinesiology And Physiotherapy
- Postdoctoral in Linguistics
- Postdoctoral in Logistics
- Postdoctoral in Management
- Postdoctoral in Marketing
- Postdoctoral in Mass Communication
- Postdoctoral in Mathematics
- Postdoctoral in Metallurgy
- Postdoctoral in Micro and Multimode Grid Design
- Postdoctoral in Mining Engineering
- Postdoctoral in Modern Power and Energy Systems
- Postdoctoral in Multimedia Design and Digital Art
- Postdoctoral in Music
- Postdoctoral in Nutrigenetics
- Postdoctoral in Nutrition
- Postdoctoral in Oil, Gas and Energy Engineering
- Postdoctoral in Organizational Development
- Postdoctoral in Organizational Diversity
- Postdoctoral in Pedagogical Training For Professionals
- Postdoctoral in Philosophy
- Postdoctoral in Physical Anthropology
- Postdoctoral in Physical Culture And Sports
- Postdoctoral in Political Science
- Postdoctoral in Public Administration
- Postdoctoral in Public Health
- Postdoctoral in Quantum Computing
- Postdoctoral in Radio And Television Production
- Postdoctoral in Social Media Marketing
- Postdoctoral in Social Sciences
- Postdoctoral in Sociocultural Anthropology
- Postdoctoral in Sociology
- Postdoctoral in Software Engineering
- Postdoctoral in Sport Management
- Postdoctoral in Sport Science
- Postdoctoral in Sports Marketing
- Postdoctoral in Sports Psychology
- Postdoctoral in Statistics
- Postdoctoral in Strategic Management
- Postdoctoral in Sustainable Design and Construction
- Postdoctoral in Sustainable Materials Science
- Postdoctoral in Sustainable Natural Resources Management
- Postdoctoral in Sustainable Tourism
- Postdoctoral in Synthetic Biology
- Postdoctoral in Technical Writing
- Postdoctoral in Theater
- Postdoctoral in Theology
- Postdoctoral in Virtual Archival Science
- Postdoctoral of Biology
- Postdoctoral of Industrial Engineering
- Postdoctoral of Information Systems
- Postdoctoral of Legal Studies
- Postdoctoral Research in Accounting
- Postdoctoral Research in Adult Education
- Postdoctoral Research in Advertising
- Postdoctoral Research in Agriculture
- Postdoctoral Research in American History
- Postdoctoral Research in Animation
- Postdoctoral Research in Anthropology
- Postdoctoral Research in Archeology
- Postdoctoral Research in Architecture
- Postdoctoral Research in Art History
- Postdoctoral Research in Artificial Intelligence
- Postdoctoral Research in Banking and Finance
- Postdoctoral Research in Business Administration
- Postdoctoral Research in Business Management
- Postdoctoral Research in Chemical Engineering
- Postdoctoral Research in Civil Engineering
- Postdoctoral Research in Communications
- Postdoctoral Research in Economics
- Postdoctoral Research in Educational Administration
- Postdoctoral Research in Electrical Engineering
- Postdoctoral Research in Finance
- Postdoctoral Research in Healthcare Administration
- Postdoctoral Research in Human Resources Management
- Postdoctoral Research in Information Technology
- Postdoctoral Research in Interior Design
- Postdoctoral Research in International Relations
- Postdoctoral Research in Mechanical Engineering
- Postdoctoral Research in Physical Education
- Postdoctoral Research in Project Management
- Postdoctoral Research in Psychology
- Postdoctoral Research in Renewable Energy
- Postdoctoral Research in Scenography
- Postdoctoral Research in Telecommunications
- Postdoctoral Research in Tourism Planning and Development
- Postdoctoral Research in Travel and Tourism
- Postdoctoral Research in Unmanned Aerial Systems Engineering (UAV and drone technology)
- Postdoctoral Research in Urban Planning
- Postdoctoral Research in Visual and Performing Arts
- Postdoctoral Research in Web Design
- Postdoctoral Research Program
Distance Learning at AIU is enhanced by vast academic resources and innovative technologies build into the Virtual Campus: Hundreds of self-paced courses with video lectures and step by step lessons, thousands of optional assignments, 140,000 e-books, the Social Media & Networking platform allowing collaboration/chat/communications between students, and MYAIU develop students holistically in 11 areas beyond just academics.
The world is YOUR campus!”, that is the message of AIU’s month magazine Campus Mundi. Hear the voices and see the faces that make up AIU. Campus Mundi brings the world of AIU to you every months with inspirational stories, news and achievements by AIU members from around the world (students and staff are located in over 200 countries).
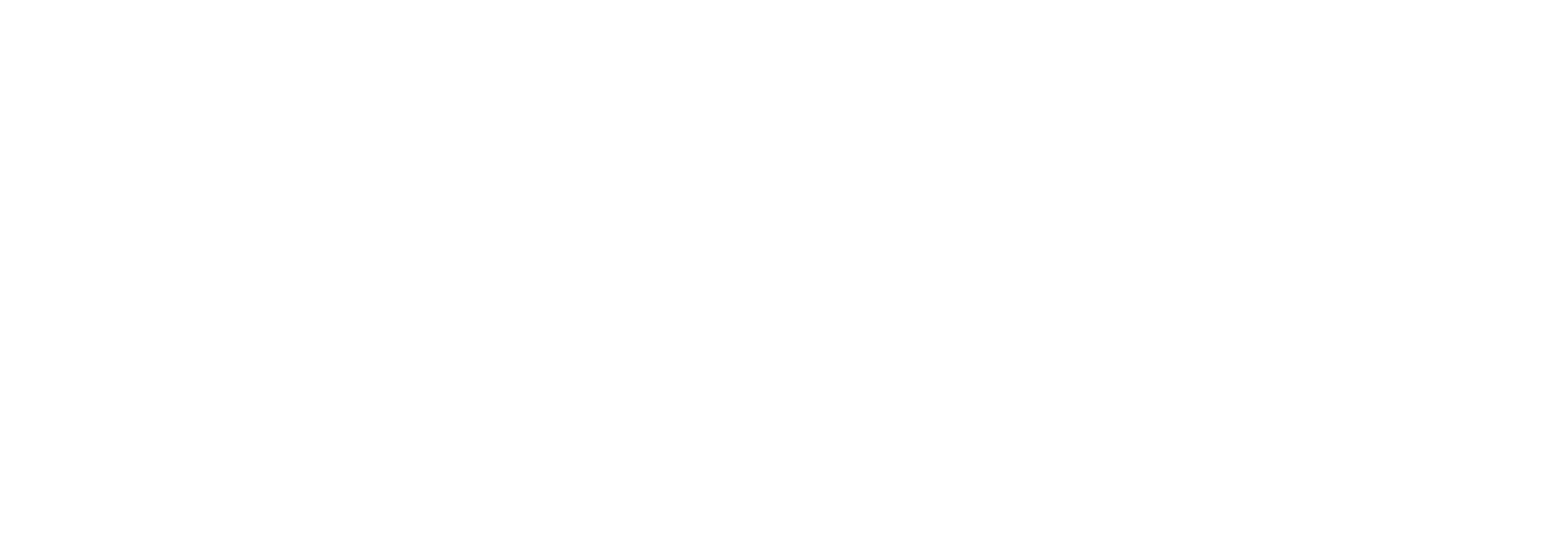
Please enter your credentials
Student Advisor Administrator Admissions Quiz Editor Link Exchange
keep me logged-in
The Complete Guide To Distance Learning Program: What It Is, And How To Get Started

In recent years, distance learning has emerged as a powerful educational tool, offering flexibility and accessibility to learners worldwide. According to the National Center for Education Statistics (NCES), around 7.5 million U.S. students depend upon online classes for higher education. In fact, the Covid-19 pandemic has played a big role in accelerating the popularity of remote learning from 2020. It has reshaped the modern learning system especially in higher education offering students flexibility in learning. It is quite an evolution to step out of a traditional classroom setting and enter the digital world of learning where nothing can stop a curious mind from gaining knowledge.

Source: U.S. Department of Education, National Center for Education Statistics
Definition and Significance of Distance Learning
What is distance learning program.
Distance learning, also known as online learning or e-learning, encompasses various educational formats that allow students to learn remotely, often utilizing digital technologies and the same internet connection. This mode of education transcends geographical boundaries, enabling individuals to access quality education regardless of location or time constraints.
The significance of distance learning lies in its ability to democratize education, making it accessible to a diverse range of learners, including working professionals, individuals with disabilities, and those residing in remote areas with limited access to traditional educational institutions. Moreover, distance learning offers flexibility, allowing students to balance their studies with other commitments, such as work or family responsibilities. For Atlantic international University our model of Academic Freedom and self determination where the student is empowered to chart his/her own unique path have led to a customized Learning Management System (LMS) and Virtual Campus. At the core of it is freedom of choice bringing together daily live classes, weekly optional assignments, AI assisted course creation, Mini-Courses to facilitate microlearning, Symposiums and Webinars for students and staff to exchange ideas and robust library resources such as 260,000 books accessible online. As a community component Gamification of the learning process such as earning badges, unlocking gifts and AIULink which is AIU’s social media platform/networking via AIULink create the opportunity for online students to join together and collaborate.
Overview of the Article’s Focus
This article provides a comprehensive guide to everything educators and learners need to know for successful distance learning programs. We will explore critical components that contribute to the effectiveness of distance learning, including:
- Technological Infrastructure: This section provides an overview of the essential technological tools and platforms required for seamless online learning experiences, such as learning management systems (LMS), video conferencing software, and collaboration tools.
- Pedagogical Strategies: Insights into effective teaching methodologies tailored for distance learning environments, including asynchronous and synchronous learning approaches, interactive multimedia content, and assessment strategies that promote engagement and learning outcomes.
- Student Support Services: This section discusses the importance of robust student support services in distance learning programs , including online tutoring, academic advising, and technical assistance, to enhance student success and retention rates.
- Accessibility and Inclusivity: Consider accessibility standards and practices to ensure that distance learning programs accommodate diverse learners, including those with disabilities, linguistic differences, or varying learning preferences.
- Best Practices for Engagement and Motivation: Strategies for fostering active participation, collaboration, and intrinsic motivation among distance learners, such as gamification, peer interaction, and personalized learning experiences.
- Evaluation and Continuous Improvement: Guidance on monitoring and evaluating the effectiveness of distance learning programs through feedback mechanisms, data analytics, and iterative improvements to enhance overall quality and learner satisfaction.
What Are the Infrastructure Requirements for Successful Distance Learning Programs?
Access to reliable internet connectivity.
- Reliable internet connectivity is paramount for seamless participation in online education, remote learning classes online, and virtual classrooms.
- Internet access enables students to engage in e-learning initiatives, access Internet-based education resources, take online classes and receive remote instruction.
- It facilitates web-based distance learning courses, through digital education platforms, ensuring uninterrupted access to distance education courses and online learning opportunities.
Availability of Suitable Devices (Computers, Tablets, etc.)
- Access to suitable devices such as computers, tablets, or smartphones is essential for accessing online education resources and participating in remote learning activities.
- These devices allow students to connect to their virtual classroom, engage in e-learning initiatives, and access digital education platforms.
- Ensuring the availability of devices enables students to fully leverage distance education courses and take advantage of online learning opportunities from anywhere.
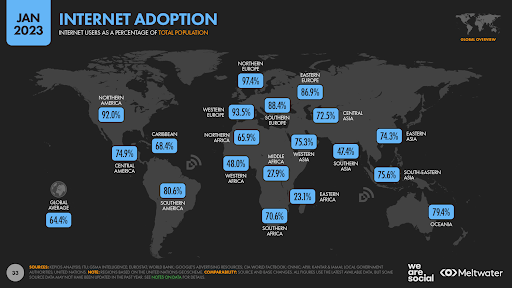
Necessary Software and Applications for Online Learning
- The availability of necessary software and applications is crucial for facilitating compelling online learning experiences.
- These software tools enable students to interact with online courses and materials, participate in virtual classrooms, and engage in collaborative activities.
- Essential software and applications may include learning management systems (LMS), video conferencing tools, interactive multimedia resources, and productivity suites tailored for digital education platforms. Some examples are: Moodle, Blackboard, AtNova or self-development such as .NET used by AIU.

By addressing these infrastructure requirements, educational institutions can create an online environment more conducive to successful distance learning programs, ensuring students can access the tools and resources necessary for engaging and impactful online education experiences.
Academic Preparedness for Successful Distance Learning Programs
- Understanding the Expectations of Distance Learning Courses:
In transitioning to online education, whether through remote learning, virtual classrooms , or e-learning initiatives, students must grasp the unique expectations of distance learning courses. Unlike traditional classroom settings, where face-to-face interactions may dominate, online education relies heavily on web-based and digital learning platforms.
Students must familiarize themselves with the structure of these online courses, including assignment deadlines, communication protocols, and participation expectations. Understanding these expectations is crucial for navigating distance education courses effectively and maximizing online learning opportunities.
- Importance of Self-Discipline and Motivation:
One of the cornerstones of academic preparedness in online education is the cultivation of self-discipline and motivation. Without the physical presence of instructors or classmates to provide immediate accountability, students in online program must take proactive measures to stay engaged and on track.
Remote instruction requires high self-motivation to overcome potential distractions and maintain consistent study habits. Developing time management skills, setting realistic goals, and staying organized are essential for sustaining academic progress in web-based learning environments. Self-discipline and motivation play pivotal roles in ensuring success in distance education courses.
- Assessing One’s Readiness for Online Education:
Before embarking on distance education courses, students must assess their readiness for online learning. This involves candidly evaluating one’s learning style, technological proficiency, and personal circumstances. While online learning offers flexibility and convenience, it also requires a degree of independence and adaptability.
Students should reflect on their ability to thrive in remote online learning platforms and environments, considering their comfort with digital tools, availability of reliable internet connectivity, and capacity to manage competing priorities. Assessing readiness for online education enables individuals to make informed decisions about pursuing distance learning programs. It ensures they are adequately prepared to meet the challenges and opportunities presented by web-based learning platforms.
How Does Time Management and Organization Work in Online Education?
Time management and organization are paramount in navigating the complexities of online education and remote learning. With virtual classrooms open schedule online courses and e-learning initiatives, students must proactively create a study schedule and adhere to it diligently. Prioritizing tasks and assignments ensure that essential deadlines are met in distance education courses.
Leveraging digital education platforms and web-based learning tools facilitates efficient time management, allowing students to streamline their workflow and optimize productivity. Techniques such as breaking tasks into manageable chunks and utilizing time-blocking strategies can further enhance organization and focus. By embracing these practices, online learners can effectively balance their academic responsibilities with other commitments, maximizing online learning opportunities and achieving success in remote instruction.
- Communication and Interaction in Online Education
Communication and interaction play vital roles in online education, facilitating engagement and collaboration among students and instructors. Through digital education platforms and web-based learning environments, participants can leverage various tools and channels to connect with peers and instructors. Utilizing online platforms for communication enables students to engage in remote instruction, participate actively in virtual classrooms, and contribute to discussion forums within distance education courses.
Actively seeking clarification and feedback when needed fosters a supportive online learning environment and community where questions are addressed and ideas are exchanged. By embracing these communication channels, students can enhance their understanding of course material, develop critical thinking skills, and build meaningful connections with peers and instructors, ultimately enriching the online learning experience and maximizing online learning opportunities.
- Access to Support Services
Access to Support Services is integral for success in online education. Firstly, for traditional classroom courses, the availability of academic advising and tutoring services ensures students receive guidance and assistance in their educational journey, enhancing their understanding and performance. Secondly, accessibility to technical support for troubleshooting issues with digital platforms or devices enables smooth navigation of online learning environments, reducing potential barriers to learning.
Lastly, utilizing online libraries and resources for research and study materials expands students’ access to information, facilitating comprehensive learning experiences. These support services collectively create a conducive and supportive environment for students pursuing distance education courses and online programs, empowering them to achieve their educational goals effectively.
- Strategies for Success
Success in online education requires proactive engagement and collaboration within virtual classrooms and digital education platforms. Actively participating in course materials online class, fosters more profound understanding and retention, enhancing learning outcomes in remote instruction. Collaborating with classmates on group projects and assignments promotes teamwork and shared knowledge, enriching the online learning experience.
Additionally, seeking opportunities for networking and professional development within internet-based higher education and communities broadens horizons and cultivates valuable connections. By embracing these strategies, students can navigate distance education courses effectively, leveraging online learning opportunities to their fullest potential. Through active engagement, collaboration, and networking, learners can thrive in the dynamic landscape of remote learning, achieving academic excellence and personal growth in online education.
At AIU we Pledge for a better world
>> How long does it take to complete a bachelor’s program? Click Here
Conclusion- Elevate your online education offerings
Throughout this guide, Distance learning has emerged as a transformative force in education, providing unprecedented flexibility and accessibility to learners worldwide. As defined, distance learning transcends geographical boundaries, empowering individuals to access quality education through internet-based platforms and digital education tools. Its significance lies in democratizing education, making it accessible to diverse learners, including professionals, individuals with disabilities, and those in remote areas.
This article has explored various aspects crucial for success in distance education, including technological infrastructure, learning process, academic preparedness, time management, communication, and support services. By embracing these strategies, learners can navigate the complexities of online education, maximize their learning opportunities, and succeed in the dynamic landscape of remote learning.

Author Bio
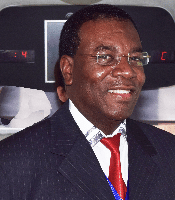
Dr. Franklin Valcin , an esteemed Instructional Designer and Professor at Atlantic International University (AIU), leads the charge in shaping the future of higher education with the significance of andragogy-driven adult education. With a keen focus on integrating advanced technologies and innovative methodologies, Dr. Valcin plays a pivotal role in crafting AIU’s dynamic academic programs. As part of AIU’s academic department, he spearheads initiatives to harness the power of Artificial Intelligence (AI) for personalized learning experiences. With the support and guidance of Dr. Valcin, Atlantic International University ensures that the unique and unrepeatable programs we offer meet the demands of today’s job market and anticipate the needs of tomorrow’s learners.
Exploring University Majors: A Guide to Selecting Your Path
Understanding the human skeletal system: structure, function, and significance, related posts.

Navigating University Majors: A Guide to Popular Fields of Study
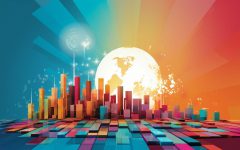
Time to Act: Pioneering Sustainable Development for a Resilient 2030
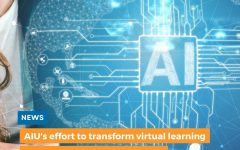
AIU Receives Widespread Media Coverage for Innovative Efforts in Education and AI
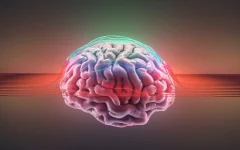
Unveiling the Mind’s Window: The Remarkable Link Between Eye Movements and Cognition
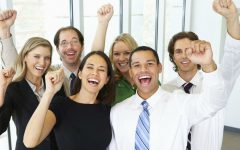
Striking a Balance: Navigating Work-Life Harmony for Enhanced Wellbeing
Popular Tags
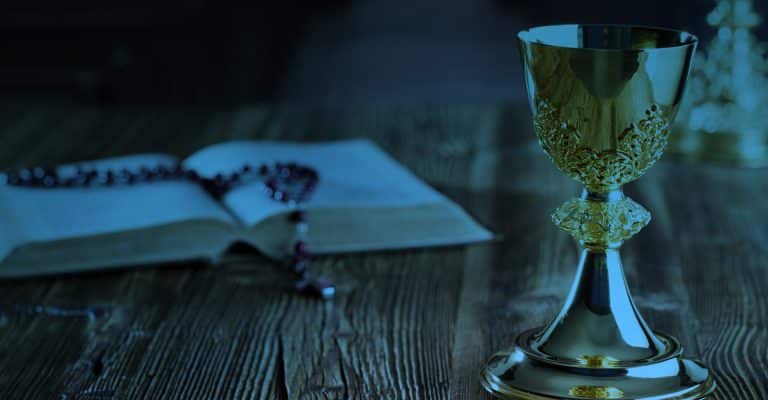
Contact Us Atlantic International University
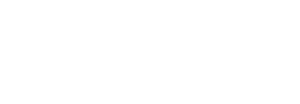
Quick Links
Home | Spanish | Online Courses | Available Courses | Vrtual Campus | Career Center | Available Positions | Ask Career Coach | The Job Interview | Resume Writing | Accreditation | Areas of Study | Bachelor Degree Programs | Masters Degree Programs | Doctoral Degree Programs | Course & Curriculum | Human Rights | Online Library | Representations | Student Publication | Sponsors | General Information | Mission & Vision | School of Business and Economics | School of Science and Engineering | School of Social an Human Studies | Media Center | Admission Requirements | Apply Online | Tuition | Faculty & Staff | Distance Learning Overview | Student Testimonials | Frequently Asked Questions | Register for Program | Privacy Policy | FAQ
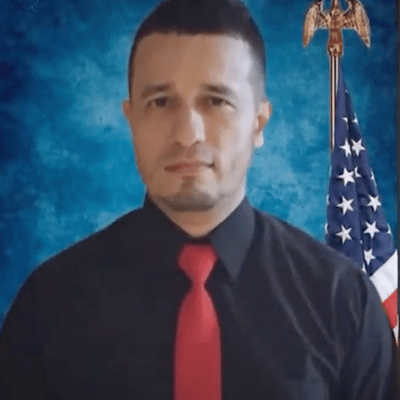
- Open access
- Published: 12 May 2024
Distance plus attention for binding affinity prediction
- Julia Rahman 1 na1 ,
- M. A. Hakim Newton 2 , 3 na1 ,
- Mohammed Eunus Ali 4 &
- Abdul Sattar 2
Journal of Cheminformatics volume 16 , Article number: 52 ( 2024 ) Cite this article
200 Accesses
1 Altmetric
Metrics details
Protein-ligand binding affinity plays a pivotal role in drug development, particularly in identifying potential ligands for target disease-related proteins. Accurate affinity predictions can significantly reduce both the time and cost involved in drug development. However, highly precise affinity prediction remains a research challenge. A key to improve affinity prediction is to capture interactions between proteins and ligands effectively. Existing deep-learning-based computational approaches use 3D grids, 4D tensors, molecular graphs, or proximity-based adjacency matrices, which are either resource-intensive or do not directly represent potential interactions. In this paper, we propose atomic-level distance features and attention mechanisms to capture better specific protein-ligand interactions based on donor-acceptor relations, hydrophobicity, and \(\pi \) -stacking atoms. We argue that distances encompass both short-range direct and long-range indirect interaction effects while attention mechanisms capture levels of interaction effects. On the very well-known CASF-2016 dataset, our proposed method, named Distance plus Attention for Affinity Prediction (DAAP), significantly outperforms existing methods by achieving Correlation Coefficient (R) 0.909, Root Mean Squared Error (RMSE) 0.987, Mean Absolute Error (MAE) 0.745, Standard Deviation (SD) 0.988, and Concordance Index (CI) 0.876. The proposed method also shows substantial improvement, around 2% to 37%, on five other benchmark datasets. The program and data are publicly available on the website https://gitlab.com/mahnewton/daap.
Scientific Contribution Statement
This study innovatively introduces distance-based features to predict protein-ligand binding affinity, capitalizing on unique molecular interactions. Furthermore, the incorporation of protein sequence features of specific residues enhances the model’s proficiency in capturing intricate binding patterns. The predictive capabilities are further strengthened through the use of a deep learning architecture with attention mechanisms, and an ensemble approach, averaging the outputs of five models, is implemented to ensure robust and reliable predictions.
Introduction
Conventional drug discovery, as noted by a recent study [ 1 ], is a resource-intensive and time-consuming process that typically lasts for about 10 to 15 years and costs approximately 2.558 billion USD to bring each new drug successfully to the market. Computational approaches can expedite the drug discovery process by identifying drug molecules or ligands that have high binding affinities towards disease-related proteins and would thus form strong transient bonds to inhibit protein functions [ 2 , 3 , 4 ]. In a typical drug development pipeline, a pool of potential ligands is usually given, and the ligands exhibiting strong binding affinities are identified as the most promising drug candidates against a target protein. In essence, protein-ligand binding affinity values serve as a scoring method to narrow the search space for virtual screening [ 5 ].
Existing computational methods for protein-ligand binding affinity prediction include both traditional machine learning and deep learning-based approaches. Early methods used Kernel Partial Least Squares [ 6 ], Support Vector Regression (SVR) [ 7 ], Random Forest (RF) Regression [ 8 ], and Gradient Boosting [ 9 ]. However, just like various other domains [ 10 , 11 , 12 , 13 , 14 ], drug discovery has also seen significant recent advancements [ 15 , 16 , 17 , 18 ] from the computational power and extensive datasets used in deep learning. Deep learning models for protein-ligand binding affinity prediction take protein-ligand docked complexes as input and give binding affinity values as output. Moreover, these models use various input features to capture the global characteristics of the proteins and the ligands and their local interactions in the pocket areas where the ligands get docked into the proteins.
Recent deep learning models for protein-ligand binding affinity prediction include DeepDTA [ 19 ], Pafnucy [ 20 ], \(K_\text {DEEP}\) [ 21 ], DeepAtom [ 22 ], DeepDTAF [ 23 ], BAPA [ 5 ], SFCNN [ 24 ], DLSSAffinity [ 4 ] EGNA [ 25 ], CAPLA [ 26 ] and ResBiGAAT [ 27 ]. DeepDTA [ 19 ] introduced a Convolutional Neural Network (CNN) model with input features Simplified Molecular Input Line Entry System (SMILES) sequences for ligands and full-length protein sequences. Pafnucy and \(K_{DEEP}\) used a 3D-CNN with 4D tensor representations of the protein-ligand complexes as input features. DeepAtom employed a 3D-CNN to automatically extract binding-related atomic interaction patterns from voxelized complex structures. DeepDTAF combined global contextual features and local binding area-related features with dilated convolution to capture multiscale long-range interactions. BAPA introduced a deep neural network model for affinity prediction, featuring descriptor embeddings and an attention mechanism to capture local structural details. SFCNN employed a 3D-CNN with simplified 4D tensor features having only basic atomic type information. DLSSAffinity employed 1D-CNN with pocket-ligand structural pairs as local features and ligand SMILES and protein sequences as global features. EGNA introduced an empirical graph neural network (GNN) that utilizes graphs to represent proteins, ligands, and their interactions in the pocket areas. CAPLA [ 26 ] utilized a cross-attention mechanism within a CNN along with sequence-level input features for proteins and ligands and structural features for secondary structural elements. ResBiGAAT [ 27 ] integrates a deep Residual Bidirectional Gated Recurrent Unit (Bi-GRU) with two-sided self-attention mechanisms, utilizing both protein and ligand sequence-level features along with their physicochemical properties for efficient prediction of protein-ligand binding affinity.
In this work, we consider the effective capturing of protein-ligand interaction as a key to making further progress in binding affinity prediction. However, as we see from the literature, a sequential feature-based model such as DeepDTA was designed mainly to capture long-range interactions between proteins and ligands, not considering local interactions. CAPLA incorporates cross-attention mechanisms along with sequence-based features to indirectly encompass short-range interactions to some extent. ResBiGAAT employs a residual Bi-GRU architecture and two-sided self-attention mechanisms to capture long-term dependencies between protein and ligand molecules, utilizing SMILES representations, protein sequences, and diverse physicochemical properties for improved binding affinity prediction. On the other hand, structural feature-based models such as Pafnucy, \(K_{DEEP}\) and SFCNN use 3D grids, 4D tensors, or molecular graph representations. These features provide valuable insights into the pocket region of the protein-ligand complexes but incur significant computational costs in terms of memory and processing time. Additionally, these features have limitations in capturing long-range indirect interactions among protein-ligand pairs. DLSSAffinity aims to bridge the gap between short- and long-range interactions by considering both sequential and structural features. Moreover, DLSSAffinity uses 4D tensors for Cartesian coordinates and atom-level features to represent interactions between heavy atoms in the pocket areas of the protein-ligand complexes. These representations of interactions are still indirect, considering the importance of protein-ligand interaction in binding affinity. EGNA tried to use graphs and Boolean-valued adjacency matrices to capture protein-ligand interactions to some extent. However, EGNA’s interaction graph considers only edges between each pair of a \(C_\beta \) atom in the pocket areas of the protein and a heavy atom in the ligand when their distance is below a threshold of \(10\mathring{A}\) .
Inspired by the use of distance measures in protein structure prediction [ 14 , 28 , 29 ], in this work, we employ distance-based input features in protein-ligand binding affinity prediction. To be more specific, we use distances between donor-acceptor [ 30 ], hydrophobic [ 31 , 32 ], and \(\pi \) -stacking [ 31 , 32 ] atoms as interactions between such atoms play crucial roles in protein-ligand binding. These distance measures between various types of atoms could essentially capture more direct and more precise information about protein-ligand interactions than using sequence-based features or various other features representing the pocket areas of the protein-ligand complexes. Moreover, the distance values could more directly capture both short- and long-range interactions than adjacency-based interaction graphs of EGNA or tensor-based pocket area representations of DLSSAffinity. Besides capturing protein-ligand interactions, we also consider only those protein residues with donor, hydrophobic, and \(\pi \) -stacking atoms in this work. Considering only these selective residues is also in contrast with all other methods that use all the protein residues. For ligand representation, we use SMILES strings. After concatenating all input features, we use an attention mechanism to effectively weigh the significance of various input features. Lastly, we enhance the predictive performance of our model by adopting an ensembling approach, averaging the outputs of several trained models.
We name our proposed method as Distance plus Attention for Affinity Prediction (DAAP). On the very well-known CASF-2016 dataset, DAAP significantly outperforms existing methods by achieving the Correlation Coefficient (R) 0.909, Root Mean Squared Error (RMSE) 0.987, Mean Absolute Error (MAE) 0.745, Standard Deviation (SD) 0.988, and Concordance Index (CI) 0.876. DAAP also shows substantial improvement, ranging from 2% to 37%, on five other benchmark datasets. The program and data are publicly available on the website https://gitlab.com/mahnewton/daap .
In our study, we first demonstrate the robustness of our deep architecture through five-fold cross-validation. Subsequently, the learning curve, as depicted in Fig. 1 , illustrates the dynamics of training and validation loss, providing insights into the stability and reliability of the learning process. Furthermore, we provide a comprehensive performance comparison of our proposed model with current state-of-the-art predictors. We also provide an in-depth analysis of the experimental results. The effectiveness of our proposed features is substantiated through an ablation study and a detailed analysis of input features.
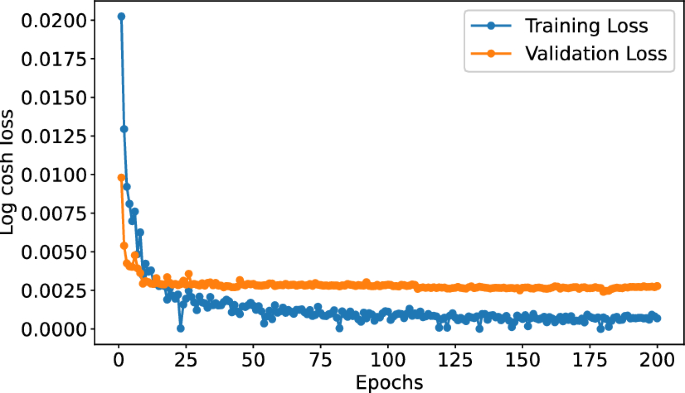
Training and validation loss curve of DAAP
Five-fold cross-validation
This study employs a five-fold cross-validation approach to evaluate the performance of the proposed model thoroughly, demonstrating the robustness of the deep architecture. Table 1 provides the average performance metrics (R, RMSE, MAE, SD, and CI) along with their corresponding standard deviations derived from the 5-fold cross-validation on the CASF \(-\) 2016.290 test set when the model is trained with PDBbind2016 and PDBbind2020 datasets. This presentation highlights the predictor’s predictive accuracy and reliability, emphasising the proposed model’s effectiveness.
Average ensemble
Our proposed approach leverages an attention-based deep learning architecture to predict binding affinity. The input feature set comprises distance matrices, sequence-based features for specific protein residues, and SMILES sequences. To enhance the robustness and mitigate the effects of variability and overfitting, we train five models and employ arithmetic averaging for ensembling. Average ensembling is more suitable than max voting ensembling when dealing with real values.
Table 2 shows the results of five models and their averages when all models have the identical setting of their training parameters and the training datasets. We see that the ensemble results are better than the results of the individual models in both the PDBbind2016 and PDBbind2020 training datasets. To check that the proposed approach is robust over the variability in the training datasets, we also train five models but each with a different training subset. These training subsets were obtained by using sampling with replacement. Table 3 shows the results of these five models and their averages.
Tables 2 and 3 depict that the ensemble results are better than the results of the individual results in both training sets. It might seem counterintuitive to see the average results are better than all the individual results, but note that these are not simple average of averages. When the ensemble results are compared across Tables 2 and 3 , the best results are observed in Table 2 for the PDBbind2020 training set. All evaluation metrics R, RMSE, SD, MAE, and CI display improved performance when using the same training data (Table 2 ) compared to different varying training data (Table 3 ) in PDBbind2020 data set. Accordingly, we choose the ensemble with the same training data for PDBbind2020 (Table 2 ) as our final binding affinity prediction model. Conversely, for PDBbind2016, superior outcomes are obtained from the varied training subsets in Table 3 . Henceforth, the best-performing models using PDBbind2016 and PDBbind2020 will be referred to as DAAP16 and DAAP20, respectively, in subsequent discussions.
Comparison with state-of-the-art methods
In our comparative analysis, we assess the performance of our proposed affinity predictor, DAAP, on the CASF-2016 test set, compared to nine recent state-of-the-art predictors: Pafnucy [ 20 ], DeepDTA [ 19 ], OnionNet [ 3 ], DeepDTAF [ 23 ], SFCNN [ 24 ] DLSSAffinity [ 4 ], EGNA [ 25 ], CAPLA [ 26 ] and ResBiGAAT [ 27 ]. Notably, the most recent predictors have surpassed the performance of the initial four, prompting us to focus our comparison on their reported results. For the latter five predictors, we detail the methodology of obtaining their results as follows:
DLSSAffinity We rely on the results available on DLSSAffinity’s GitHub repository, as direct prediction for specific target proteins is not possible due to the unavailability of its trained model.
SFCNN Utilizing the provided weights and prediction code from SFCNN, we replicate their results, except for CASF-2013. The ambiguity regarding the inclusion of CASF-2013 data in their training set (sourced from the PDBbind database version 2019) leads us to omit these from our comparison.
EGNA We have adopted EGNA’s published results for the CASF-2016 test set with 285 protein-ligand complexes due to differing Uniclust30 database versions for HHM feature construction. We applied EGNA’s code with our HHM features for the other five test sets to ensure a consistent evaluation framework.
CAPLA Predictions are made based on the features given in CAPLA’s GitHub, except for the ADS.74 dataset, where we can’t predict results due to the unavailability of feature sets. Their results are the same as their reported results.
ResBiGAAT We included ResBiGAAT’s published results in our analysis after encountering discrepancies with their online server using the same SMILES sequences and protein sequences from test PDB files as us. Variations in results, particularly for PDB files with multiple chains, led us to rely on their reported data, as it yielded more consistent and higher accuracies than our attempts.
In Table 4 , the first 8 methods, namely Pafnucy, DeepDTA, OnionNet, DeepDTAF, DLSSAffinity, SFCNN, \(EGNA^*\) and CAPLA reported on 290 CASF-2016 protein-ligand complexes. To make a fair comparison with these 8 methods, we compared our proposed method DAAP16 and DAAP20 on those 290 protein-ligand complexes. From the data presented in the Table 4 , it is clear that our DAAP20 approach outperforms all the 8 predictors, achieving the highest R-value of 0.909, the highest CI value of 0.876, the lowest RMSE of 0.987, the lowest MAE of 0.745, and the lowest SD of 0.988. Specifically, compared to the closest state-of-the-art predictor, CAPLA, our approach demonstrated significant improvements, with approximately 5% improvement in R, 12% in RMSE, 14% in MAE, 11% in SD, and 4% in CI metrics, showcasing its superior predictive capabilities. As 3 of the recent predictors, namely SFCNN, EGNA, and ResBiGAAT, reported their result for the 285 protein-ligand complexes on the CASF-2016 dataset, to make a fair comparison with them as well, we assess our predictor, DAAP, on these 285 proteins as well. From the data presented in Table 4 , the results revealed that, across all metrics, DAAP20 outperformed these three predictors on 285 proteins as well. Particularly, compared to the recent predictor ResBiGAAT, our approach demonstrated notable improvements, with around 6% improvement in R, 19% in RMSE, 20% in MAE, and 5% in CI metrics, highlighting its superior predictive capabilities.
Table 5 presents a comprehensive evaluation of the prediction performance of our proposed DAAP approach on five other well-known test sets CASF \(-\) 2013.87, CASF \(-\) 2013.195 ADS.74, CSAR-HiQ.51 and CSAR-HiQ.36. Across these test sets, our DAAP approaches demonstrate superior predictive performance in protein-ligand binding affinity. On the CASF \(-\) 2013.87 dataset, EGNA surpasses CAPLA with higher R-value and CI-value of 0.752 and 0.767, respectively, while CAPLA records lower RMSE, MAE and SD values of 1.512, 1.197, and 1.521. In contrast, our DAAP20 surpasses both, excelling in all metrics with an R of 0.811, RMSE of 1.324, MAE of 1.043, SD of 1.332, and CI of 0.813, with DAAP16 also delivering robust performance. For the CASF \(-\) 2013.195 test set, a similar trend is observed with our DAAP20 approach outperforming the nearest state-of-the-art predictor by a significant margin of 8%-20% across all evaluation metrics. The DAAP16 approach, not DAAP20, stands out on the ADS.74 dataset by surpassing predictors like Pafnucy, SFCNN and EGNA, showcasing substantial improvements of approximately 12%-37% in various metrics. When evaluating the CSAR-HiQ.51 and CSAR-HiQ.36 datasets against six state-of-the-art predictors, DAAP20 consistently outperforms all, indicating enhancements of 2%-20% and 3%-31%, respectively. Although DAAP16 does not surpass ResBiGAAT in CSAR-HiQ.51, it notably excels in the CSAR-HiQ.36 dataset, outperforming ResBiGAAT in all metrics except MAE. These results underscore the exceptional predictive capabilities of our DAAP approach across diverse datasets and evaluation criteria, consistently surpassing existing state-of-the-art predictors.
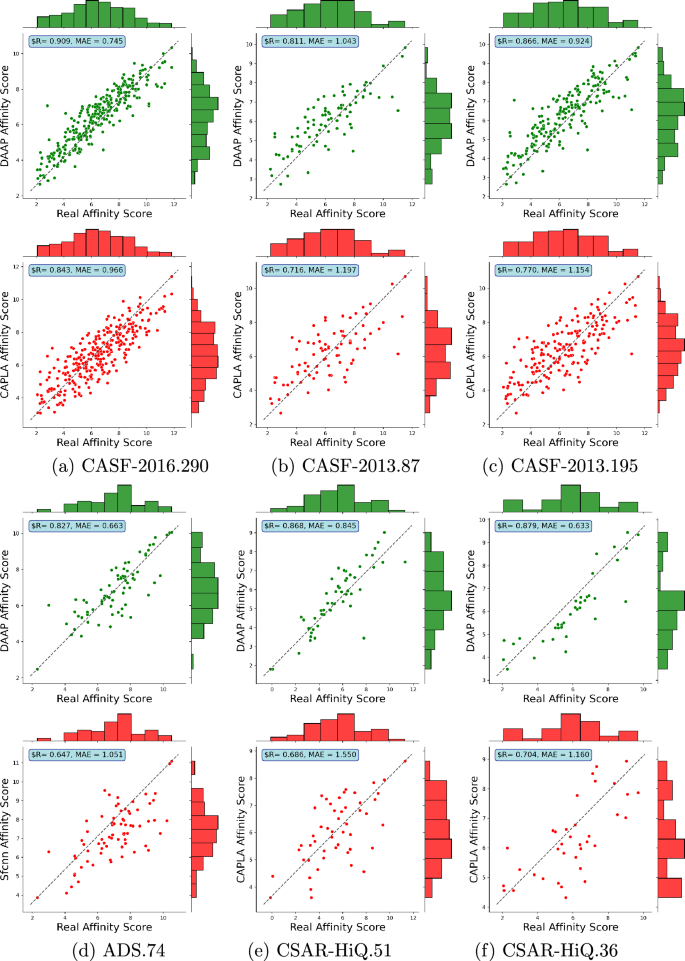
The distributions of real and predicted binding affinity values by our predictor (green) and the closest state-of-the-art predictor (red) across the six test sets
Figure 2 presents the distributions of actual and predicted binding affinities for our best DAAP approach and the closest state-of-the-art predictor. In all six test sets, a clear linear correlation and low mean absolute error (MAE) between predicted and actual binding affinity values can be observed for our DAAP model, demonstrating the strong performance of our model across these test sets. The other predictors show scattering over larger areas. In our analysis, we could not consider ResBiGAAT in the CSAR-HiQ.51 and CSAR-HiQ.36 datasets due to the unavailability of their results.
Ablation study and explainability
A significant contribution of this work is utilising distance matrix input features to capture critical information about the protein-ligand relationship. Specifically, we employ a concatenation of three distance maps, representing donor-acceptor, hydrophobic, and \(\pi \) -stacking interactions, as input features, effectively conveying essential protein-ligand bonding details. Following finalising our prediction architecture by incorporating two additional features derived from protein and SMILES sequences, we conduct an in-depth analysis of the impact of various combinations of these distance matrices as features. In the case of protein features, residues are selected based on which distance maps are considered.
Table 6 illustrates the outcomes obtained from experimenting with different combinations of distance maps and selected protein residue and ligand SMILES features on the CASF \(-\) 2016.290 test set. We devise four unique combinations, employing three distinct distance maps for both the PDBbind2016 and PDBbind2020 training datasets. Additionally, we explore a combination that integrates donor-acceptor, hydrophobic, and \(\pi \) -stacking distance maps with features from all protein residues, denoted as DA + \(\pi \) S + HP + FP, to evaluate the impact of using all residues versus selected ones.
From the information presented in Table 6 , it is evident that utilizing the donor-acceptor (DA) solely distance maps yields the lowest performance across both training sets, particularly when different combinations of distance maps are paired with selective protein residues. However, as expected, the combination of the three distance maps, namely DA, \(\pi \) S ( \(\pi \) -stacking), and HP (Hydrophobicity), demonstrates superior performance compared to other combinations. Notably, the combination of DA and HP outperforms the other two combinations but falls short of our best-performing feature set. The ensemble of DA, \(\pi \) S, HP and all protein residues exhibit the least favourable outcomes among the tested combinations. This result aligns with our expectations, as Hydrophobic interactions are the most prevalent in protein-ligand binding, underscoring their significance in feature analysis.
Integrating an attention mechanism into our model is crucial in achieving improved results. After consolidating the outputs of three 1D-CNN blocks, we employ attention, each receiving inputs from distance maps, protein sequences, and ligand sequences. The dimension of the feature is 384. As depicted in Fig. 3 , the heatmap visualization highlights the differential attention weights assigned to various features, with brighter and darker regions indicating higher weights to certain features, thus improving binding affinity predictions. This process underscores the mechanism’s ability to discern and elevate critical features, showing that not all features are equally important. Further emphasizing the significance of attention, a comparative analysis using the same model architecture without the attention mechanism on the same features-shown in the last row of Table 6 demonstrates its vital role in boosting predictive accuracy. This comparison not only reinforces the value of the attention mechanism in detecting intricate patterns within the feature space but also significantly enhances the model’s predictive capabilities.
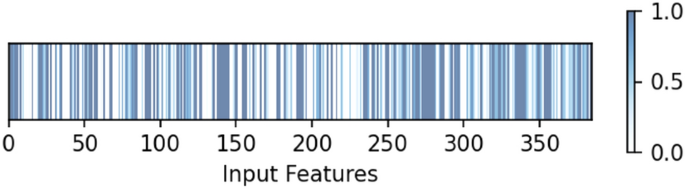
Visualization of attention maps for concatenated features in the 1o0h protein-ligand complex of the CASF \(-\) 2016.290 dataset
Statistical analysis
In assessing the statistical significance of performance differences between DAAP and its closest competitors, Wilcoxon Signed Ranked Tests at a 95% confidence level were conducted. Comparisons included DAAP against CAPLA for CASF \(-\) 2016.290, CASF \(-\) 2013.87, CASF \(-\) 2013.195, CSAR-HiQ.36, and CSAR-HiQ.51 datasets and between DAAP and SFCNN for the ADS.74 test set. Unfortunately, ResBiGAAT’s results were unavailable for inclusion in the analysis. Table 7 depicts that DAAP demonstrated statistical significance compared to the closest state-of-the-art predictor across various test sets, as indicated by p-values ranging from 0.000 to 0.047. The consistently negative mean Z-values, ranging from \(-\) 14.71 to \(-\) 5.086, suggest a systematic improvement in predictive performance. Moreover, higher mean rankings, ranging from 19.5 to 144.5, further emphasize the overall superiority of DAAP. Notably, the superior performance is observed across diverse datasets, including CASF \(-\) 2016.290, CASF \(-\) 2013.87, CASF \(-\) 2013.195, ADS.74, CSAR-HiQ.51, and CSAR-HiQ.36. These findings underscore the robustness and effectiveness of DAAP in predicting protein-ligand binding affinity.
Screening results
In this section, we scrutinize the effectiveness of our predicted affinity scores to accurately differentiate between active binders (actives) and non-binders (decoys) throughout the screening procedure. To this end, we have carefully curated a subset of seven hand-verified targets from the Database of Useful Decoys: Enhanced (DUD-E), accessible via https://dude.docking.org , to serve as our evaluative benchmark. The details about seven targets are given in Table 8 . This table underscores the diversity and challenges inherent in the dataset, reflecting a wide range of D/A ratios that present a comprehensive framework for evaluating the discriminatory power of our predicted affinity scores.
To construct protein-ligand complexes for these targets, we employed AutoDock Vina, configuring the docking grid to a \(20\mathring{A} \times 20\mathring{A} \times 20\mathring{A}\) cube centred on the ligand’s position. This setup and 32 consecutive Monte-Carlo sampling iterations identified the optimal pose for each molecule pair. Our evaluation of the screening performance utilizes two pivotal metrics: the Receiver Operating Characteristic (ROC) curve [ 33 ] and the Enrichment Factor (EF) [ 34 ]. Figure 4 shows the ROC curve and the EF graph for a detailed examination of a predictive model’s efficacy in virtual screening. The ROC curve’s analysis, with AUC values spanning from 0.63 to 0.76 for the seven targets, illustrates our model’s proficient capability in differentiating between actives and decoys. These values, closely approaching the top-left corner of the graph, denote a high true positive rate alongside a low false positive rate, underscoring our model’s efficacy.
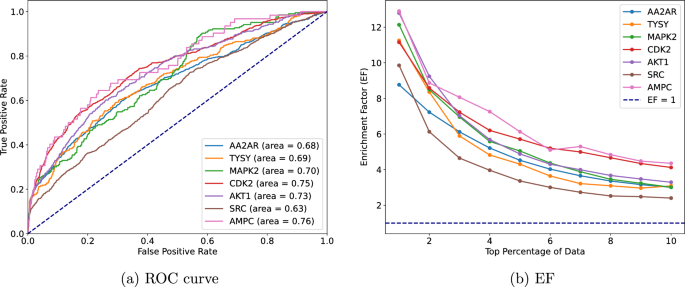
Screening Performance of the Predictive Model: Roc curve (left) and EF (right)
Furthermore, the EF graph of Fig. 4 provides a quantitative assessment of the model’s success in prioritizing active compounds within the top fractions of the dataset, notably the top 1% to 10%. Initial EF values ranging from 12.3 to 9.9 for the top 1% underscore our model’s exceptional ability to enrich active compounds beyond random chance significantly. This pronounced enrichment highlights the model’s utility in the early identification of promising candidates. However, the observed gradual decline in EF values with increasing dataset fractions aligns with expectations, reflecting the challenge of sustaining high enrichment levels across broader selections.
Conclusions
In our protein-ligand binding affinity prediction, we introduce atomic-level distance map features encompassing donor-acceptor, hydrophobic, and \(\pi \) -stacking interactions, providing deeper insights into interactions for precise predictions, both for short and long-range. We enhance our model further with specific protein sequence features of specific residues and ligand SMILES information. These features are integrated into an attention-based 1D-CNN architecture that is used a number of times for ensemble-based performance enhancement, resulting in superior results compared to existing methods across six benchmark datasets. Remarkably, on the CASF-2016 dataset, our model achieves a Correlation Coefficient (R) of 0.909, Root Mean Squared Error (RMSE) of 0.987, Mean Absolute Error (MAE) of 0.745, Standard Deviation (SD) of 0.988, and Concordance Index (CI) of 0.876, signifying its potential to advance drug discovery binding affinity prediction. The program and data are publicly available on the website https://gitlab.com/mahnewton/daap .
We describe the protein-ligand dataset used in our work. We also describe our proposed method in terms of its input features, output representations, and deep learning architectures.
Protein-ligand datasets
In the domain of protein-ligand binding affinity research, one of the primary sources for training, validation, and test sets is the widely recognized PDBbind database [ 35 ]. This database is meticulously curated. It comprises experimentally verified protein-ligand complexes. Each complex encompasses the three-dimensional structures of a protein-ligand pair alongside its corresponding binding affinities expressed as \(pK_d\) values. The PDBbind database ( http://www.pdbbind.org.cn/ ) is subdivided into two primary subsets: the general set and the refinement set . The PDBbind version 2016 dataset (named PDBbind2016) contains 9221 and 3685 unique protein-ligand complexes, while the PDBbind version 2020 dataset (named PDBbind2020) includes 14127 and 5316 protein-ligand complexes in the general and refinement sets, respectively.
Similar to the most recent state-of-the-art affinity predictors such as Pafnucy [ 20 ], DeepDTAF [ 23 ], OnionNet [ 3 ], DLSSAffinity [ 4 ], LuEtAl [ 36 ], EGNA [ 25 ] and CAPLA [ 26 ], our DAAP16 method is trained using the 9221 + 3685 = 12906 protein-ligand complexes in the general and refinement subsets of the PDBbind dataset version 2016 . Following the same training-validation set formation approach of the recent predictors such as Pafnucy, OnionNet, DeepDTAF, DLSSAffinity and CAPLA, we put 1000 randomly selected protein-ligand complexes in the validation set and the remaining 11906 distinct protein-ligand pairs in the training set. Another version of DAAP, named DAAP20, was generated using the PDBbind database version 2020 , which aligns with the training set of ResBiGAAT [ 27 ]. To avoid overlap, we filtered out protein-ligand complexes common between the PDBbind2020 training set and the six independent test sets. After this filtering process, 19027 unique protein-ligand complexes were retained for training from the initial pool of 19443 in PDBbind2020.
To ensure a rigorous and impartial assessment of the effectiveness of our proposed approach, we employ six well-established, independent blind test datasets. There is no overlap of protein-ligand complexes between the training sets and these six independent test sets.
CASF-2016.290 The 290 protein-ligand complexes, commonly referred to as CASF-2016, are selected from the PDBbind version 2016 core set ( http://www.pdbbind.org.cn/casf.php ) and have become the gold standard test set for recent affinity predictors such as DLSSAffinity [ 4 ], LuEtAl [ 36 ], EGNA [ 25 ] and CAPLA [ 26 ].
CASF-2013.87 and CASF-2013.195 Similar to the approach taken by DLSSAffinity [ 4 ], we carefully curated 87 unique protein-ligand complexes from the CASF-2013 dataset, which originally consists of 195 complexes ( http://www.pdbbind.org.cn/casf.php ). These 87 complexes were chosen to ensure no overlap with our training set or the CASF-2016 test set. Additionally, we use the entire set of 195 complexes as another test set, named CASF \(-\) 2013.195.
ADS.74 This test set from SFCNN [ 24 ] comprises 74 protein-ligand complexes sourced from the Astex diverse set [ 37 ].
CSAR-HiQ.51 and CSAR-HiQ.36 These two test datasets contain 51 and 36 protein-ligand complexes from the well-known CSAR [ 38 ] dataset. Recent affinity predictors such as EGNA [ 25 ], CAPLA and ResBiGAAT [ 26 , 27 ] have employed CSAR as a benchmark dataset. To get our two test datasets, we have followed the procedure of CAPLA and filtered out protein-ligand complexes with duplicate PDB IDs from two distinct CSAR subsets containing 176 and 167 protein-ligand complexes, respectively.
Input features
Given protein-ligand complexes in the datasets, we extract three distinctive features from proteins, ligands, and protein-ligand binding pockets. We describe these below.
Protein representation
We employ three distinct features for encoding protein sequences: one-hot encoding of amino acids, a Hidden Markov model based on multiple sequence alignment features (HHM), and seven physicochemical properties.
In the one-hot encoding scheme for the 20 standard amino acids and non-standard amino acids, each amino acid is represented by a 21-dimensional vector. This vector contains twenty “0 s” and one “1”, where the position of the “1” corresponds to the amino acid index in the protein sequence.
To construct the HHM features, we have run an iterative searching tool named HHblits [ 39 ] against the Uniclust30 database ( http://wwwuser.gwdg.de/~compbiol/uniclust/2020_06/ ) as of June 2020. This process allows us to generate HHM sequence profile features for the proteins in our analysis. Each resulting .hhm feature file contains 30 columns corresponding to various parameters such as emission frequencies, transition frequencies, and Multiple Sequence Alignment (MSA) diversities for each residue. Like EGNA, for columns 1 to 27, the numbers are transformed into frequencies using the formula \(f = 2^{-0.001*p}\) , where f represents the frequency, and p is the pseudo-count. This transformation allows the conversion of these parameters into frequency values. Columns 28 to 30 are normalized using the equation: \(f = \frac{0.001*p}{20}\) . This normalization process ensures that these columns are appropriately scaled for further analysis and interpretation.
The seven physicochemical properties [ 14 , 29 ] for each amino acid residue are steric parameter (graph shape index), hydrophobicity, volume, polarisability, isoelectric point, helix probability, and sheet probability. When extracting these three features for protein residues, we focused exclusively on the 20 standard amino acid residues. If a residue is non-standard, we assigned a feature value of 0.0.
In our approach, we initially concatenate all three features sequentially for the entire protein sequence. Subsequently, to enhance the specificity of our model, we employ a filtering strategy where residues lacking donor [ 40 ], hydrophobic [ 31 ], and \(\pi \) -stacking [ 32 ] atoms within their amino acid side chains are excluded from the analysis. Additionally, to prevent overlap, we select unique residues after identification based on donor, hydrophobic, or \(\pi \) -stacking atoms for each protein sequence. The rationale behind this filtering is to focus on residues that are actively involved in critical interactions relevant to protein-ligand binding. The resulting feature dimension for each retained protein residue is 58. This feature set includes one-hot encoding of amino acids, a Hidden Markov model based on multiple sequence alignment features (HHM), and seven physicochemical properties. These features are comprehensively summarised in Table 9 for clarity.
Considering the variable numbers of residues that proteins can possess, we have considered a standardized protein sequence length to align with the fixed-size requirements of deep learning algorithms. In our initial experiments exploring various sequence lengths in the datasets, we found that a maximum length of 500 yields better performance in terms of pearson correlation coefficient (R) and mean absolute error (MAE). If the number of selected residues falls below 500, we pad the sequence with zeros; conversely, if it exceeds 500, we truncate it to 500 from the initial position of the sequence. The final dimension of each protein is \(500\times 58\) .
Ligand representation
We use SMILES to represent ligands. SMILES is a widely adopted one-dimensional representation of chemical structures of ligands [ 41 ]. To convert ligand properties such as atoms, bonds, and rings from ligand SDF files into SMILES strings, we use the Open Babel chemical tool [ 42 ]. The SMILES strings comprise 64 unique characters, each corresponding to a specific numeric digit ranging from 1 to 64. For example, the SMILES string “HC(O=)N” is represented as [12, 42, 1, 48, 40, 31, 14]. In line with our protein representation approach, we set a fixed length of 150 characters for each SMILES string.
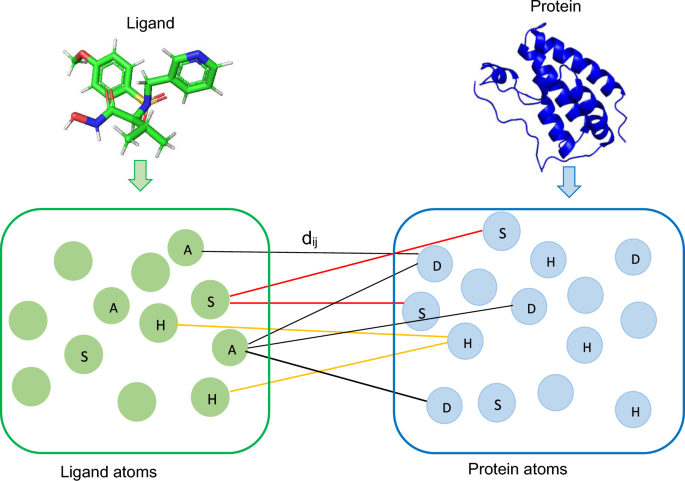
Various distance measures that potentially capture protein-ligand interactions. In the figure, \(d_{ij}\) represents the distance between a donor (D), hydrophobic (H), or \(\pi \) -stacking (S) atom i in the protein and the corresponding acceptor (A), hydrophobic (H), or \(\pi \) -stacking (S) atom j in the ligand. Empty circles represent other atom types. Different colour lines represent different types of interactions
Binding pocket representation
A binding pocket refers to a cavity located either on the surface or within the interior of a protein. A binding pocket possesses specific characteristics that make it suitable for binding a ligand [ 43 ]. Protein residues within the binding pocket region exert a direct influence, while residues outside this binding site can also have a far-reaching impact on affinity prediction. Among various protein-ligand interactions within the binding pocket regions, donor-acceptor atoms [ 30 ], hydrophobic contacts [ 31 , 32 ], and \(\pi \) -stacking [ 31 , 32 ] interactions are the most prevalent, and these interactions could significantly contribute to the enhancement of affinity score prediction. The formation of the protein-ligand complexes involves donor atoms from the proteins and acceptor atoms from the ligands. This process is subject to stringent chemical and geometric constraints associated with protein donor groups and ligand acceptors [ 30 ]. Hydrophobic interactions stand out as the primary driving force in protein-ligand interactions, while \(\pi \) -stacking interactions, particularly involving aromatic rings, play a substantial role in protein-ligand interactions [ 32 ]. However, there are instances where donor-acceptor interactions alone may not suffice, potentially failing to capture other interactions that do not conform to traditional donor-acceptor patterns. In such scenarios, hydrophobic contacts and \(\pi \) -stacking interactions become essential as they could provide valuable insights for accurate affinity prediction.
We employ three types of distance matrices in our work shown in Fig. 5 to capture protein-ligand interactions. The first one is the donor-acceptor distance matrix , which considers distances between protein donor atoms and acceptor ligand atoms, with data sourced from mol2/SDF files. We ensure that all ligand atoms contribute to the distance matrix construction, even in cases where ligands lack explicit acceptor atoms. Furthermore, we calculate the hydrophobic distance matrix by measuring the distance between hydrophobic protein atoms and hydrophobic ligand atoms, ensuring the distance is less than \(4.5\mathring{A}\) [ 31 ]. Similarly, we compute the \(\pi \) - stacking distance matrix by considering protein and ligand \(\pi \) -stacking atoms and applying a distance threshold of \(4.0\mathring{A}\) [ 32 ]. These three types of atoms are selected from the heavy atoms, referring to any atom that is not hydrogen.
We discretize the initially calculated real-valued distance matrices representing the three types of interactions into binned distance matrices. These matrices are constrained within a maximum distance threshold of \(20\mathring{A}\) . The decision to set a maximum distance threshold of \(20\mathring{A}\) for capturing the binding pocket’s spatial context is informed by practices in both affinity prediction and protein structure prediction fields. Notably, methodologies like Pafnucy [ 20 ], DLSSAffinity [ 4 ], and EGNA [ 25 ], as well as advanced protein structure prediction models such as AlphaFold [ 28 ] and trRosetta [ 44 ], utilize a 20Å range to define interaction spaces or predict structures. This consensus on the 20Å threshold reflects its sufficiency in providing valuable spatial information necessary for accurate modeling. The distance values ranging from \(0\mathring{A} - 20\mathring{A}\) are discretized into 40 bins, each with a \(0.5\mathring{A}\) interval. Any distance exceeding \(20\mathring{A}\) is assigned to the \(41^{st}\) bin. In our experimentation, we explored different distance ranges ( \(20\mathring{A}\) , \(25\mathring{A}\) , \(30\mathring{A}\) , \(35\mathring{A}\) , and \(40\mathring{A}\) ) while maintaining a uniform bin interval of \(0.5\mathring{A}\) . Among these ranges, \(20\mathring{A}\) yielded optimal results, and as such, we adopted it for our final analysis. Following this binning process, the original real-valued distances in the matrices are substituted with their corresponding bin numbers. Subsequently, we convert the 2D distance matrix into a 1D feature vector. We concatenate the three 1D vectors representing the three distinct interactions into a single vector to construct the final feature vector. To ensure consistency, the maximum length of the feature vector is set to 1000 for each pocket.
Output representations
This binding affinity is measured in the dissociation constant ( \(K_d\) ). For simplicity in calculations, the actual affinity score \(K_d\) is commonly converted into \(pK_d\) by taking the negative logarithm of \(K_d\) .
Deep learning architectures
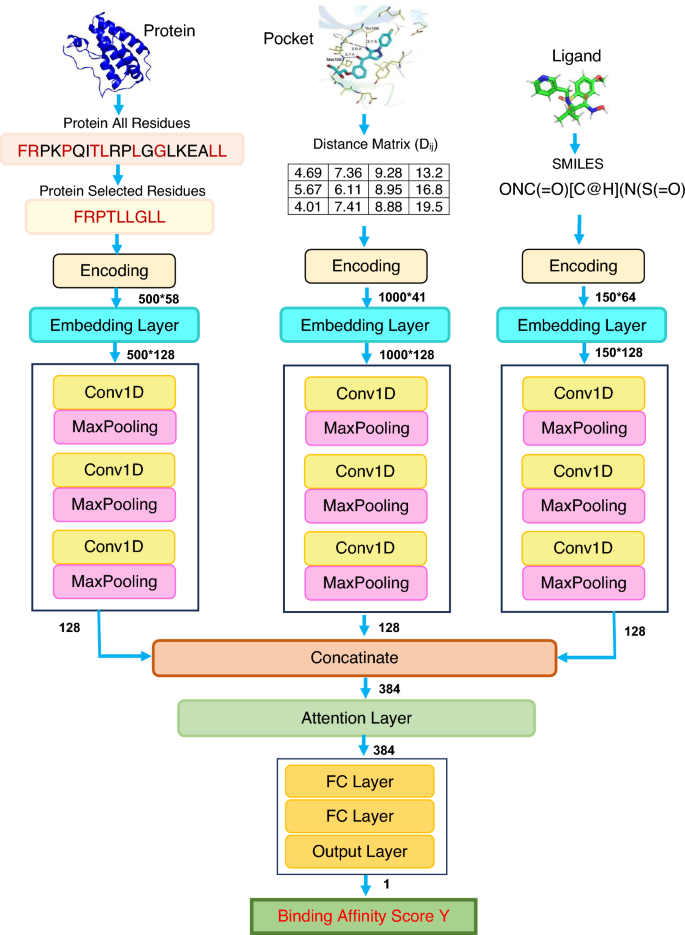
The proposed model architecture
We propose a deep-learning regression model to predict protein-ligand binding affinities, shown in Fig. 6 . Our model comprises three integral components: convolutional neural network (CNN), attention mechanism, and fully connected neural network (FCNN). Before feeding to the CNN block, information from three distinct feature sources (proteins, ligands, and interactions) is encoded and subsequently processed through the embedding layer. The embedding layer transforms the inputs into fixed-length vectors of a predefined size (in this case, 128 dimensions), enabling more effective feature representation with reduced dimensionality. During training, our model operates with a batch size of 16 and is optimized using the Adam optimizer and a learning rate set at 0.001. We adopt the log cosh loss function for this work to optimise the model’s performance. The training regimen consists of 200 epochs, with the best model selected based on the validation loss, and a dropout rate of 0.2 is applied. The explored hyperparameter settings are summarised in Table 10 . We have explored these settings, and after preliminary experiments, we have selected these values which are emboldened.
Convolutional neural network
Much like DLSSAffinity [ 4 ], our model employs three 1D-CNN blocks, each dedicated to processing distinct feature sources: proteins, ligands, and interactions in pockets. Each of these 1D-CNN blocks comprises three convolutional layers paired with three Maxpooling layers. The configuration of the first two 1D-CNN blocks includes 32, 64, and 128 filters, each with corresponding filter lengths of 4, 8, and 12. In contrast, the 1D-CNN block responsible for handling SMILES sequence inputs features filters with 4, 6, and 8 adjusted lengths. Each of the three 1D-CNN blocks in our model generates a 128-dimensional output. Subsequently, before progressing to the next stage, the outputs of these three 1D-CNN blocks are concatenated and condensed into a unified 384-dimensional output.
Attention mechanism
In affinity prediction, attention mechanisms serve as crucial components in neural networks, enabling models to allocate varying levels of focus to distinct facets of input data [ 5 ]. These mechanisms play a critical role in weighing the significance of different features or entities when assessing their interaction strength. The attention mechanism uses the formula below.
We use the Scaled Dot-Product Attention [ 45 ] mechanism to calculate and apply attention scores to the input data. The attention mechanism calculates query ( Q ), key ( K ), and value ( V ) matrices from the input data. In this context, Q is a vector capturing a specific aspect of the input, K represents the context or memory of the model with each key associated with a value, and V signifies the values linked to the keys. It computes attention scores using the dot product of Q and K matrices, scaled by the square root of the dimensionality ( \(d_k\) ). Subsequently, a softmax function normalises the attention scores. Finally, the output is generated as a weighted summation of the value (V) matrix, guided by the computed attention scores.
Notably, the output of the concatenation layer passes through the attention layer. The input to the attention layer originates from the output of the concatenation layer, preserving the same dimensionality as the input data. This design ensures the retention of crucial structural information throughout the attention mechanism.
Fully connected neural network
The output of the attention layer transitions into the subsequent stage within our model architecture, known as the Fully Connected Neural Network (FCNN) block. The FCNN block consists of two fully connected (FC) layers, where the two layers have 256 and 128 nodes respectively. The final stage in our proposed prediction model is the output layer, which follows the last FC layer.
Evaluation metrics
We comprehensively evaluate our affinity prediction model using five well-established performance metrics. The Pearson Correlation Coefficient (R) [ 4 , 24 , 26 , 36 ] measures the linear relationship between predicted and actual values. The Root Mean Square Error (RMSE) [ 4 , 24 , 26 ] and the Mean Absolute Error (MAE) [ 24 , 26 ] assess prediction accuracy and error dispersion. The Standard Deviation (SD) [ 4 , 24 , 26 , 36 ] evaluates prediction consistency, and the Concordance Index (CI) [ 26 , 36 ] determines the model’s ability to rank protein-ligand complexes accurately. Higher R and CI values and lower RMSE, MAE, and SD values indicate better prediction accuracy. These metrics are collectively very robust measures for comparison of our model’s performance against that of the state-of-the-art techniques in the field of affinity prediction.
N : the number of protein-ligand complexes
\(Y_{\text {act}}\) : experimentally measured actual binding affinity values for the protein-ligand complexes
\(Y_{\text {pred}}\) : the predicted binding affinity values for the given protein-ligand complexes
\(y_{\text {act}_i}\) and \(y_{\text {pred}_i}\) : respectively the actual and predicted binding affinity value of the \(i^{th}\) protein-ligand complex
a : is slope
b : interpretation of the linear regression line of the predicted and actual values. Z : the normalization constant, i.e. the number of data pairs with different label values.
h ( u ): the step function that returns 1.0, 0.5, and 0.0for \(u>0\) , \(u = 0\) , and \(u<0\) respectively.
Availability of data and materials
The program and corresponding data are publicly available on the website https://gitlab.com/mahnewton/daap .
DiMasi JA, Grabowski HG, Hansen RW (2016) Innovation in the pharmaceutical industry: new estimates of r &d costs. J Health Econ 47:20–33
Article PubMed Google Scholar
Gilson MK, Zhou H-X (2007) Calculation of protein-ligand binding affinities. Ann Rev Biophys Biomol Str 36(1):21–42
Article CAS Google Scholar
Zheng L, Fan J, Mu Y (2019) Onionnet: a multiple-layer intermolecular-contact-based convolutional neural network for protein-ligand binding affinity prediction. ACS Omega 4(14):15956–15965
Article CAS PubMed PubMed Central Google Scholar
Wang H, Liu H, Ning S, Zeng C, Zhao Y (2022) Dlssaffinity: protein-ligand binding affinity prediction via a deep learning model. Phys Chem Chem Phys 24(17):10124–10133
Article CAS PubMed Google Scholar
Seo S, Choi J, Park S, Ahn J (2021) Binding affinity prediction for protein-ligand complex using deep attention mechanism based on intermolecular interactions. BMC Bioinform 22(1):1–15
Article Google Scholar
Deng W, Breneman C, Embrechts MJ (2004) Predicting protein- ligand binding affinities using novel geometrical descriptors and machine-learning methods. J Chem Inf Comput Sci 44(2):699–703
Li L, Wang B, Meroueh SO (2011) Support vector regression scoring of receptor-ligand complexes for rank-ordering and virtual screening of chemical libraries. J Chem Inf Modeling 51(9):2132–2138
Ballester PJ, Mitchell JB (2010) A machine learning approach to predicting protein-ligand binding affinity with applications to molecular docking. Bioinformatics 26(9):1169–1175
Li H, Peng J, Sidorov P, Leung Y, Leung K-S, Wong M-H, Lu G, Ballester PJ (2019) Classical scoring functions for docking are unable to exploit large volumes of structural and interaction data. Bioinformatics 35(20):3989–3995
Deng L, Platt J. Ensemble deep learning for speech recognition. In: Proc. Interspeech. 2014
Chen C, Seff A, Kornhauser A, Xiao J. Deepdriving: learning affordance for direct perception in autonomous driving. In: Proceedings of the IEEE International Conference on Computer Vision, 2015; pp. 2722–2730
Lin T-Y, RoyChowdhury A, Maji S (2017) Bilinear convolutional neural networks for fine-grained visual recognition. IEEE Trans Pattern Anal Mach Intell 40(6):1309–1322
Newton MH, Rahman J, Zaman R, Sattar A. Enhancing protein contact map prediction accuracy via ensembles of inter-residue distance predictors. Computational Biology and Chemistry, 2022; 107700.
Rahman J, Newton MH, Hasan MAM, Sattar A (2022) A stacked meta-ensemble for protein inter-residue distance prediction. Comput Biol Med 148:105824
Isert C, Atz K, Schneider G (2023) Structure-based drug design with geometric deep learning. Curr Opin Struct Biol 79:102548
Krentzel D, Shorte SL, Zimmer C (2023) Deep learning in image-based phenotypic drug discovery. Trend Cell Biol 33(7):538–554
Yang L, Jin C, Yang G, Bing Z, Huang L, Niu Y, Yang L (2023) Transformer-based deep learning method for optimizing admet properties of lead compounds. Phys Chem Chem Phys 25(3):2377–2385
Masters MR, Mahmoud AH, Wei Y, Lill MA (2023) Deep learning model for efficient protein-ligand docking with implicit side-chain flexibility. J Chem Inf Modeling 63(6):1695–1707
Öztürk H, Özgür A, Ozkirimli E (2018) Deepdta: deep drug-target binding affinity prediction. Bioinformatics 34(17):821–829
Stepniewska-Dziubinska MM, Zielenkiewicz P, Siedlecki P (2018) Development and evaluation of a deep learning model for protein-ligand binding affinity prediction. Bioinformatics 34(21):3666–3674
Jiménez J, Skalic M, Martinez-Rosell G, De Fabritiis G (2018) \(k_{deep}\) : protein-ligand absolute binding affinity prediction via 3d-convolutional neural networks. J Chem Inf Modeling 58(2):287–296
Li Y, Rezaei MA, Li C, Li X (2019) Deepatom: a framework for protein-ligand binding affinity prediction. In: 2019 IEEE International Conference on Bioinformatics and Biomedicine (BIBM), pp. 303–310, IEEE
Wang K, Zhou R, Li Y, Li M (2021) Deepdtaf: a deep learning method to predict protein-ligand binding affinity. Brief Bioinf 22(5):072
Wang Y, Wei Z, Xi L (2022) Sfcnn: a novel scoring function based on 3d convolutional neural network for accurate and stable protein-ligand affinity prediction. BMC Bioinform 23(1):1–18
Xia C, Feng S-H, Xia Y, Pan X, Shen H-B (2023) Leveraging scaffold information to predict protein-ligand binding affinity with an empirical graph neural network. Brief Bioinf. https://doi.org/10.1093/bib/bbac603
Jin Z, Wu T, Chen T, Pan D, Wang X, Xie J, Quan L, Lyu Q (2023) Capla: improved prediction of protein-ligand binding affinity by a deep learning approach based on a cross-attention mechanism. Bioinformatics 39(2):049
Abdelkader GA, Njimbouom SN, Oh T-J, Kim J-D (2023) Resbigaat: Residual bi-gru with attention for protein-ligand binding affinity prediction. Computational Biology and Chemistry, 107969
Senior AW, Evans R, Jumper J, Kirkpatrick J, Sifre L, Green T, Qin C, Žídek A, Nelson AW, Bridgland A et al (2020) Improved protein structure prediction using potentials from deep learning. Nature 577(7792):706–710
Rahman J, Newton MH, Islam MKB, Sattar A (2022) Enhancing protein inter-residue real distance prediction by scrutinising deep learning models. Sci Rep 12(1):787
Raschka S, Wolf AJ, Bemister-Buffington J, Kuhn LA (2018) Protein-ligand interfaces are polarized: discovery of a strong trend for intermolecular hydrogen bonds to favor donors on the protein side with implications for predicting and designing ligand complexes. J Computer-aided Mol Design 32:511–528
Jubb HC, Higueruelo AP, Ochoa-Montaño B, Pitt WR, Ascher DB, Blundell TL (2017) Arpeggio: a web server for calculating and visualising interatomic interactions in protein structures. J Mol Biol 429(3):365–371
Freitas RF, Schapira M (2017) A systematic analysis of atomic protein-ligand interactions in the pdb. Medchemcomm 8(10):1970–1981
Empereur-Mot C, Guillemain H, Latouche A, Zagury J-F, Viallon V, Montes M (2015) Predictiveness curves in virtual screening. J Cheminf 7(1):1–17
Li H, Zhang H, Zheng M, Luo J, Kang L, Liu X, Wang X, Jiang H (2009) An effective docking strategy for virtual screening based on multi-objective optimization algorithm. BMC Bioinf 10:1–12
Liu T, Lin Y, Wen X, Jorissen RN, Gilson MK (2007) Bindingdb: a web-accessible database of experimentally determined protein-ligand binding affinities. Nucleic Acids Res 35(suppl–1):198–201
Lu Y, Liu J, Jiang T, Guan S, Wu H. Protein-ligand binding affinity prediction based on deep learning. In: International Conference on Intelligent Computing, 2022; pp. 310–316. Springer.
Hartshorn MJ, Verdonk ML, Chessari G, Brewerton SC, Mooij WT, Mortenson PN, Murray CW (2007) Diverse, high-quality test set for the validation of protein- ligand docking performance. J Med Chem 50(4):726–741
Dunbar JB Jr, Smith RD, Yang C-Y, Ung PM-U, Lexa KW, Khazanov NA, Stuckey JA, Wang S, Carlson HA (2011) Csar benchmark exercise of 2010: selection of the protein-ligand complexes. J Chem Inf Modeling 51(9):2036–2046
Remmert M, Biegert A, Hauser A, Söding J (2012) Hhblits: lightning-fast iterative protein sequence searching by hmm-hmm alignment. Nat Methods 9(2):173–175
Hydrogen donor and acceptor atoms of the amino acid. https://www.imgt.org/IMGTeducation/Aide-memoire/_UK/aminoacids/charge/ . Accessed: 13-08-2023
Weininger D (1988) Smiles, a chemical language and information system. 1. introduction to methodology and encoding rules. J Chem Inf Comput Sci 28(1):31–36
O’Boyle NM, Banck M, James CA, Morley C, Vandermeersch T, Hutchison GR (2011) Open babel: An open chemical toolbox. J Cheminf 3(1):1–14
Google Scholar
Stank A, Kokh DB, Fuller JC, Wade RC (2016) Protein binding pocket dynamics. Accounts Chem Res 49(5):809–815
Yang J, Anishchenko I, Park H, Peng Z, Ovchinnikov S, Baker D (2020) Improved protein structure prediction using predicted interresidue orientations. Proc Natl Acad Sci 117(3):1496–1503
Vaswani A, Shazeer N, Parmar N, Uszkoreit J, Jones L, Gomez AN, Kaiser Ł, Polosukhin I (2017) Attention is all you need. Advances in neural information processing systems 30
Download references
Acknowledgements
This research is partially supported by the research seed grant awarded to M.A.H.N. at the University of Newcastle. The research team acknowledges the valuable assistance of the Griffith University eResearch Service & Specialised Platforms team for granting access to their High-Performance Computing Cluster, which played a crucial role in completing this research endeavour.
This research is partially supported by the research seed Grant awarded to M.A.H.N. at the University of Newcastle.
Author information
Julia Rahman and M. A. Hakim Newton are co-first-authors and contributed equally.
Authors and Affiliations
School of Information and Communication Technology, Griffith University, 170 Kessels Rd, Nathan, 4111, QLD, Australia
Julia Rahman
Institute for Integrated and Intelligent Systems (IIIS), Griffith University, 170 Kessels Rd, Nathan, 4111, QLD, Australia
M. A. Hakim Newton & Abdul Sattar
School of Information and Physical Sciences, University of Newcastle, University Dr, Callaghan, 2308, NSW, Australia
M. A. Hakim Newton
Department of Computer Science & Engineering, Bangladesh University of Engineering and Technology, Palashi, 1205, Dhaka, Bangladesh
Mohammed Eunus Ali
You can also search for this author in PubMed Google Scholar
Contributions
The contributions of the authors to this work were as follows: J.R. and M.A.H.N. played equal roles in all aspects of the project, including conceptualization, data curation, formal analysis, methodology, software development, and writing of the initial draft. M.E.A. helped in the concept development, review and editing of the manuscript. A.S. actively engaged in discussions, facilitated funding acquisition, provided supervision, and thoroughly reviewed the manuscript.
Corresponding author
Correspondence to Julia Rahman .
Ethics declarations
Competing interests.
No Conflict of interest is declared.
Additional information
Publisher's note.
Springer Nature remains neutral with regard to jurisdictional claims in published maps and institutional affiliations.
Rights and permissions
Open Access This article is licensed under a Creative Commons Attribution 4.0 International License, which permits use, sharing, adaptation, distribution and reproduction in any medium or format, as long as you give appropriate credit to the original author(s) and the source, provide a link to the Creative Commons licence, and indicate if changes were made. The images or other third party material in this article are included in the article’s Creative Commons licence, unless indicated otherwise in a credit line to the material. If material is not included in the article’s Creative Commons licence and your intended use is not permitted by statutory regulation or exceeds the permitted use, you will need to obtain permission directly from the copyright holder. To view a copy of this licence, visit http://creativecommons.org/licenses/by/4.0/ . The Creative Commons Public Domain Dedication waiver ( http://creativecommons.org/publicdomain/zero/1.0/ ) applies to the data made available in this article, unless otherwise stated in a credit line to the data.
Reprints and permissions
About this article
Cite this article.
Rahman, J., Newton, M.A.H., Ali, M.E. et al. Distance plus attention for binding affinity prediction. J Cheminform 16 , 52 (2024). https://doi.org/10.1186/s13321-024-00844-x
Download citation
Received : 01 December 2023
Accepted : 24 April 2024
Published : 12 May 2024
DOI : https://doi.org/10.1186/s13321-024-00844-x
Share this article
Anyone you share the following link with will be able to read this content:
Sorry, a shareable link is not currently available for this article.
Provided by the Springer Nature SharedIt content-sharing initiative
- Binding affinity
- Distance matrix
- Donor-acceptor
- Hydrophobicity
- \(\pi \) -Stacking
- Deep learning
Journal of Cheminformatics
ISSN: 1758-2946
- Submission enquiries: [email protected]
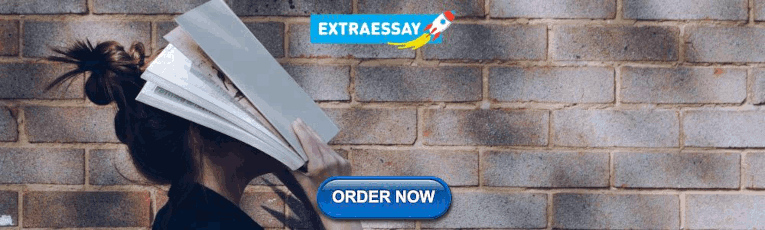
IMAGES
VIDEO
COMMENTS
This review examines the transformation of educational practices to online and distance learning during the COVID-19 pandemic. It specifically focuses on the challenges, innovative approaches, and successes of this transition, emphasizing the integration of educational technology, student well-being, and teacher development. The COVID-19 pandemic has significantly transformed the educational ...
Watts, L. (2016). Synchronous and asynchronous communication in distance learning: A review of the literature. Quarterly Review of Distance Education, 17(1), 23-32. Google Scholar Zawacki-Richter, O., & Naidu, S. (2016). Mapping research trends from 35 years of publications in distance education. Distance Education, 37(3), 245-269.
This study aims to reveal the main trends and findings of the studies examining the use of learning analytics in distance education. For this purpose, journal articles indexed in the SSCI index in the Web of Science database were reviewed, and a total of 400 journal articles were analysed within the scope of this study. The systematic review method was used as the research methodology. The ...
Recommendation Systems on E-Learning and Social Learning: A Systematic Review. The Electronic Journal of e-Learning, 19(5), ... disciplines is an imperative tool in online learning environments as it promotes distance learning and grants the ... The first part defines the research questions and outlines a general vision
Understanding components that influence students' enjoyment of distance higher education is increasingly important to enhance academic performance and retention. Although there is a growing body of research about students' engagement with online learning, a research gap exists concerning whether fun affect students' enjoyment. A contributing factor to this situation is that the meaning ...
We wanted to broaden the conversation about online and distance learning research, practice, and even policy about how to make learning with technologies accessible, relevant, and supportive of multiple intersectional identities. ... The authors make recommendations to educators and researchers while considering how technologies work for and ...
Part-Time Distance Learning Students. Higher education distance learning has been steadily on the rise worldwide, with the added impetus of the Covid-19 pandemic leading to the rapid growth of remote learning (Melgaard et al., Citation 2022).Part-time distance learning students grapple with a distinct set of challenges, including role conflicts, financial constraints, time limitations, and the ...
In their research, Kahu et al. (Citation 2014) argued that the notion that distance education removes time and space barriers to education is misleading and instead distance education just changes the nature of these barriers. Their research with first-time distance education students in New Zealand found that new students bought into the ...
recommendations for leadership ... This study is a comparative analysis of online distance learning and traditional in-person ... learning, as identified in existing research.
The outcomes of this research contribute to the field of e-learning technology, guiding the design and implementation of course RSs, to facilitate personalized and relevant recommendations for ...
Bozkurt A (2019) From distance education to open and distance learning: a holistic evaluation of history, definitions, and theories. Handbook of research on learning in the age of transhumanism ...
1.1. Related literature. Online learning is a form of distance education which mainly involves internet‐based education where courses are offered synchronously (i.e. live sessions online) and/or asynchronously (i.e. students access course materials online in their own time, which is associated with the more traditional distance education).
Sveu čilište Jurja Dobrile u Puli. Preradovićeva 1/1, 52000 Pula. Tel +385 52 377 032. Hrvatska. [email protected]. Abstract: The present paper aims to review distance learning in the context of ...
Last March, the vast majority of them took part in an impromptu experiment when most schools locked their doors to protect against the novel coronavirus. Overnight, teachers were forced to figure out how to translate face-to-face lessons into remote-learning lesson plans. As schools kick off the 2020-21 school year, there are many unknowns.
This paper aims to provide a holistic overview of research trends examining online learning in higher education in BRICS countries, an acronym for an association of five major emerging economies: Brazil, Russia, India, China, and South Africa, after COVID-19 using bibliometric analysis. Researchers utilized VOSviewer's software to analyze the data using a bibliometric approach. 971 ...
UNESCO is sharing 10 recommendations to ensure that learning remains uninterrupted during this period. Examine the readiness and choose the most relevant tools. Decide on the use high-technology and low-technology solutions based on the reliability of local power supplies, internet connectivity, and digital skills of teachers and students.
The mental health of students learning online is a critical task for many countries around the globe. The research purpose was to analyse the factors affecting the quality of mental health of young individuals who learnt under conditions of not total lockdowns but adaptive quarantine restrictions. The research involved 186 volunteers from ...
Modular distance learning has been found to enhance family ties, promote independent learning, and be cost-effective for ensuring continuous learning continuity (Dargo et al., 2021). ...
ST14 Six problems (31.25%) were rated as 'moderate,' which mainly covered concerns about the appropriateness of activities to students' mode of learning, their comprehension of learning content ...
Results: The advantages included remote learning, comfort, accessibility, while the limitations involved inefficiency and difficulty in maintaining academic integrity. The recommendations were to train faculty on using online modalities and developing lesson plan with reduced cognitive load and increased interactivities.
This recommendation suggests that feedback and support for students should be individualized for online learning, rather than given to the entire group. This does not necessarily mean that one should avoid providing scaffolds (such as guiding questions) to the entire group or that teachers necessarily need to work with individual students, only ...
The evolution of distance education (DE) in the Philippines is generally divided into 5 major. generations. As reviewed by dela P ena-Bandalaria in 200 7, the earliest distance ed ucation ...
In the literature, there have been a lot of recommendation systems proposed in the field of distance learning. In this section, we present the recent works in this domain. Bousbahi and Chorfi [ 19 ] have proposed a recommender system, in order to suggest the most appropriate MOOCs (from different providers) in response to a specific request of ...
Personalized Recommendation Method of Online Distance Teaching Resources Based on User Profiles. Conference paper; First Online: 17 January 2024; pp 303-317; ... it is very necessary to apply recommendation technology to the field of education. The research of recommendation technology in this paper is based on the network teaching platform ...
One review also synthesized evidence from studies conducted before and during COVID to provide recommendations around instructional changes that may apply post-COVID ... Tabata, L. N., & Johnsrud, L. K. (2008). The impact of faculty attitudes toward technology, distance education, and innovation. Research in Higher Education, 49, 625-646 ...
This review delves into using e-learning technology and personalized recommendation systems in education. It examines 60 articles from prominent databases and identifies the different methods used in recommendation systems, such as collaborative and content-based approaches with a recent shift towards machine learning. However, the current personalized recommendation system faces challenges ...
In this research, a learning path recommender that includes lessons and learning objects is proposed. ... These three last recommenders do not include filtering settings and distance themselves from the archetype of a search engine. ... S., & Niu, Z. (2018). An e-learning recommendation approach based on the self-organization of learning ...
In recent years, distance learning has emerged as a powerful educational tool, offering flexibility and accessibility to learners worldwide. According to the National Center for Education Statistics (NCES), around 7.5 million U.S. students depend upon online classes for higher education. In fact, the Covid-19 pandemic has played a big role in accelerating the popularity of remote learning from ...
RECOMMENDATION #1- IMPLEMENTATION STRATEGIES: Establish a community college task force to design, implement, and evaluate pilot projects in distance learning over a four-year period. Modify current regulations to allow policies and practices governed by the recommendations which follow. Include representatives from appropriate consultation ...
In our study, we first demonstrate the robustness of our deep architecture through five-fold cross-validation. Subsequently, the learning curve, as depicted in Fig. 1, illustrates the dynamics of training and validation loss, providing insights into the stability and reliability of the learning process.Furthermore, we provide a comprehensive performance comparison of our proposed model with ...