Relevance of Queueing Theory in a Supermarket: A Case Study
Ieee account.
- Change Username/Password
- Update Address
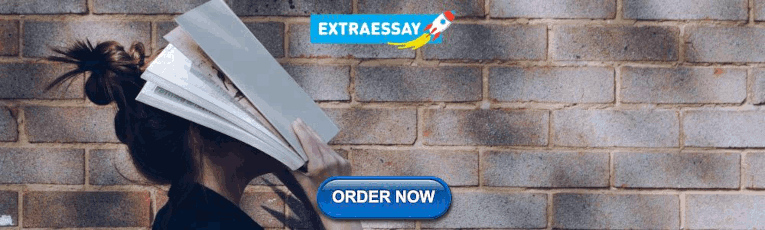
Purchase Details
- Payment Options
- Order History
- View Purchased Documents
Profile Information
- Communications Preferences
- Profession and Education
- Technical Interests
- US & Canada: +1 800 678 4333
- Worldwide: +1 732 981 0060
- Contact & Support
- About IEEE Xplore
- Accessibility
- Terms of Use
- Nondiscrimination Policy
- Privacy & Opting Out of Cookies
A not-for-profit organization, IEEE is the world's largest technical professional organization dedicated to advancing technology for the benefit of humanity. © Copyright 2024 IEEE - All rights reserved. Use of this web site signifies your agreement to the terms and conditions.

An official website of the United States government
The .gov means it’s official. Federal government websites often end in .gov or .mil. Before sharing sensitive information, make sure you’re on a federal government site.
The site is secure. The https:// ensures that you are connecting to the official website and that any information you provide is encrypted and transmitted securely.
- Publications
- Account settings
Preview improvements coming to the PMC website in October 2024. Learn More or Try it out now .
- Advanced Search
- Journal List
- Springer Nature - PMC COVID-19 Collection

Queueing Problems in Emergency Departments: A Review of Practical Approaches and Research Methodologies
Amir elalouf.
1 Department of Management, Bar-Ilan University, Ramat-Gan, Israel
Guy Wachtel
2 Department of Industrial Engineering and Management, Ariel University, Ariel, Israel
Associated Data
The datasets generated during and/or analyzed during the current study are available from the corresponding author on reasonable request.
Problems related to patient scheduling and queueing in emergency departments are gaining increasing attention in theory, in the fields of operations research and emergency and healthcare services, and in practice. This paper aims to provide an extensive review of studies addressing queueing-related problems explicitly related to emergency departments. We have reviewed 229 articles and books spanning seven decades and have sought to organize the information they contain in a manner that is accessible and useful to researchers seeking to gain knowledge on specific aspects of such problems. We begin by presenting a historical overview of applications of queueing theory to healthcare-related problems. We subsequently elaborate on managerial approaches used to enhance efficiency in emergency departments. These approaches include bed management, fast-track, dynamic resource allocation, grouping/prioritization of patients, and triage approaches. Finally, we discuss scientific methodologies used to analyze and optimize these approaches: algorithms, priority models, queueing models, simulation, and statistical approaches.
Introduction
In recent decades, healthcare systems—and emergency departments (EDs) in particular—have been facing a massive increase in demand, which has led to a continuous need to improve and optimize operational processes and quality control methods. Along with this trend, healthcare systems’ managers have begun to encounter logistical and operational problems that previously did not present a most noticeable concern. Many of these problems relate to the deployment of resources and patient flow planning through hospital departments: How should we schedule nurse and physician shifts to optimize their schedules and maximize the quality of care that patients receive? How can we optimize the workflow of staff members faced with large numbers of incoming patients? How should we direct the patient flow in emergency and routine scenarios?
Queueing theory and models have the capacity to address questions such as these in a systematic way, thereby providing managers with efficient solutions and tools for maintaining and improving performance. In 2013, Lakshmi and Sivakumar [ 1 ] produced a comprehensive literature review regarding the application of queueing theory in healthcare. Yet, in recent years, there has been increasing focus on problems that are specific to the ED, along with an urgent need to solve them. In 2018, Hu et al. [ 2 ] reviewed relevant articles published since 1970 to examine the contributions of queueing theory (QT) in modeling EDs and assess the strengths and limitations of this application: the ED is the first station that patients encounter when entering the hospital system; therefore, it is characterized by high demand levels. Every patient must get treatment and be assigned correctly to a specific department (being hospitalized or not), as the ED professional staff also needs to deal with a vast variety of medical conditions and injuries, high levels of uncertainty, and as a result, high-stress levels. More recently, in 2020, Ortíz-Barrios and Alfaro-Saíz [ 3 ] undertook a systematic review of methodological approaches to support process improvement in EDs. They reviewed scholarly articles published between 1993 and 2019 and categorized the selected papers considering the leading ED problems.
In our present work, we aim to provide researchers and healthcare managers with a clear summary of the current managerial approaches used for solving queueing-related problems in EDs and the scientific methodologies used for investigating these problems.
In Fig. 1 , we show a diagram of the ED process flow and the relevant issues that address it. The figure presents the patient flow through the healthcare system and emphasizes the need for coordination between interacting departments. At each phase of the process, we show the relevant corresponding queueing theory aspect.

ED’s process flow diagram and its relevant queueing theory aspects
The present paper conducts a systematic literature review to answer the primary question of which theories and models can provide efficient solutions and tools to maintain and improve ED performance. Answers to this question allow detecting and classifying the scope and topics of the current state-of-the-art, gaps and limitations, managerial implications, and future research directions.
Ultimately, our review is intended to serve as an efficient guide for navigating through the extensive research literature in those diverse aspects that are related to queueing applications in the EDs.
Methodology
The main purpose of the study was to answer the following research questions: What are the applications of queueing theory to healthcare and especially to problems related to the EDs? What managerial approaches help improve the efficiency of these EDs? What are the scientific methodologies used to analyze and optimize the organizational methods? Since we aimed for relevant high-quality literature that fits the above purposes and topics, we used a systematic method for collecting potential literature. The papers were first retrieved by identifying search keywords and exploring various electronic journal databases, such as Google Scholar, PubMed, Science Direct, JSTOR, ProQuest, Emerald, Elsevier, SpringerLink, and Wiley. To ensure that we collect as much data as possible, those databases were explored both using a Python script and manually for applications of queueing problems, healthcare, operations research, simulations, and modeling. The main keywords and their frequency of occurrence are presented in Fig. 2 . The letters’ size and boldness illustrate their dominance and occurrence in published papers. This gives the reader a wide look on the queueing-related frequency of topics in the ED environment.

Illustration of key words frequency in the review
The search process yielded many references, including referred journal articles, conference proceedings, dissertations, unpublished works, and books, which we systematically investigated for inclusion in the review. Relevant works were extracted by carrying out a comprehensive search of various databases. Figure 3 emphasizes the distribution and frequency throughout the years of published articles on ED queueing problems that have been considered for this literature review.

Frequency of published studies related to the field of queueing theory with emphasis to ED environment
As shown in Fig. 3 , the number of studies has grown significantly as the addressed fields are expanding greatly, mainly in the last decade, and there was a need to perform a deep verification of the studies. Therefore, we checked each paper or work manually and studied it to verify its quality and relevance to the literature review and ignored works that did not meet the criteria. Eventually, we reviewed 229 peer-reviewed works, papers, and books from the last seven decades dealing with queueing theory or related healthcare and operations research topics. We identified keywords corresponding to research methodologies such as discrete-event simulation (DES), algorithms, queueing models, and priority models; keywords corresponding to ED operational concerns such as overcrowding, patient flow, length of stay (LOS), and waiting time; and general keywords such as hospital and emergency department. In selecting studies for inclusion in this review, we sought to cover both the origins of research on ED queueing and state-of-the-art research and methods. Hence, we read each study, arranged its data (authors, title, date, and keywords), and summarized its contents into a Microsoft Excel 365 table. We subsequently grouped the studies according to specific topics to organize the information in a straightforward manner that would enable researchers to access the specific information they need. Papers were excluded based on their irrelevance to the study. We only included articles that were written in English and focused on queueing methods. Our study method was as follows: we performed a general literature survey including all the leading publications and conference proceedings. The literature survey was conducted by search using keywords that include words such as queueing, emergency department, scheduling, discrete-event-simulation, length-of-stay, waiting time, patients flow, hospitals, and more. The data were arranged in a final Microsoft Excel 2016 table that includes the title, authors, journal, date of publication, number of citations, keywords (given by the authors of the papers or us), and a summary. A review of this initial survey was concluded to fill possible holes and make it more comprehensive. In the last step, we searched for the relevant DOI (Digital Object Identifier) assigned to each publication that will provide a continuous link to its location on the web and allow its quick availability to the searcher. Since the DOI link is assigned by the publisher only at the time of publication of the article, activation of each of the links has resulted in a final update of the data previously collected in the electronic databases mentioned above.
This review is organized as follows: We first present a historical survey of early research (1950s–1980s) on queueing-related problems in healthcare systems in general and in EDs specifically. We then discuss methods used in practice to manage patient flow and workflow in EDs and related to queueing theory. Next, we present scientific methods applied to the investigation of queueing-related problems in EDs.
Early Applications of Queueing Theory in Research of Healthcare Systems
Research related to queueing in healthcare systems dates back to the early 1950s, beginning with the works by Bailey [ 4 , 5 ] who examined queues and appointment systems in hospital outpatient departments, focusing on patient waiting times as an outcome of interest. Bailey was the first to simplify the patient flow problem into a problem description that could be modeled using queueing theory and to use this approach to evaluate appointment systems and emergency bed allocations. In 1952, Welch and Bailey [ 6 ] carried out a study on appointment systems (an extension was published by Welch in 1964, after his death [ 7 ]). They offered recommendations to reduce patient waiting time while ensuring that medical staff’s time would not be wasted. The researchers’ suggestions, which took into account the punctuality of the patients and medical staff, included dividing appointment intervals into periods equal to the average time spent on each patient and calling two patients to arrive at the clinic’s opening time.
In 1969, Bell and Allen [ 8 ] used queueing theory to investigate emergency ambulance service planning with a constraint to fulfill 95% or 99% of the demand. A 1969 study by Taylor et al. [ 9 ] was the first to adopt a pure queueing theory approach to examine emergency services. Specifically, the authors gathered 8 weeks of data regarding emergency anesthetic services in a hospital in Northampton. They used a queueing theory approach to make predictions regarding the outcomes (in terms of patient waiting times) of various alternative scenarios (e.g., one versus two anesthetists on-call). They highlighted the potential benefits of this prediction approach for decision-making regarding the number of staff members on-call and the allocation of duties among them.
In the 1970s, the foundations for most current research on queueing in healthcare systems began to be established. During this time, studies began to apply queueing theory to diverse healthcare-related problems while considering patient waiting time as the primary index for quality of care. For example, Haussmann [ 10 ] modeled patient care processes in a burn unit and, in line with prior studies on queueing in healthcare systems, used empirical data to define the model’s parameters. He used the model to predict how staffing or inpatient load changes would affect the quality of nursing care (as measured by waiting time). Gupta et al. [ 11 ] considered allocating workforce for messenger services in a hospital. Milliken et al. [ 12 ] developed a queueing theory model to predict hospital delivery rooms’ utilization. Keller and Laughhunn [ 13 ] proposed an application of queueing theory to a congestion problem in an outpatient clinic, and Cooper and Corcoran [ 14 ] and McClain [ 15 ] addressed bed planning models in hospitals. Larson [ 16 ] designed efficient algorithms for facility location and redistricting in urban ambulance services. Moore [ 17 ] used queueing theory to address a problem of dissatisfaction due to waiting time for healthcare services in Dallas, TX, and Scott et al. [ 18 ] addressed reducing the response time of ambulance systems.
Notably, preliminary simulation- and algorithm-based studies of ED queueing were published during this period as well. In 1976, Collings and Stoneman [ 19 ] were the first to present a general queue approach that can be applied in an ED environment. They studied the M/M/ ∞ queue with varying arrival and departure rates in a hospital ICU (intensive care unit) and showed that their model is well-suited to the ED environment, where it is important to meet nearly all demands immediately. The authors showed that it is unlikely that the hourly variation in patient arrival rates to the unit will significantly affect the number of occupied beds. In 1978, Ladany and Turban [ 20 ] developed a pioneering simulation model for operational planning and staffing of EDs, which they used to identify optimal numbers of service stations and their staffing patterns under various conditions. The authors suggested that their simulation framework could serve as a tool for planning, budgeting, decision-making, and managerial control since it provided decision-makers with the capacity to make predictions about costs, patient waiting times, and facility idleness.
By the 1980s, queueing theory had become a well-established approach to various problems encountered in healthcare settings. Researchers and managers, such as Kao and Tung [ 21 ] and Worthington [ 22 , 23 ], began to build on this knowledge to solve straightforward queueing problems (e.g., optimizing the number of staff members allocated to a department, given data on the typical rate of incoming patients), and devising new means of addressing queueing-related challenges in practice. In parallel, researchers began to develop increasingly sophisticated methodologies to understand these challenges and identify means of improving healthcare systems’ capacity to handle them. We address these developments in what follows, with a specific focus on EDs.
Practical Approaches to Managing ED Queueing
As noted above, in recent decades, researchers and practitioners have developed creative new methods to improve ED performance. In Fig. 4 , we present the distribution of such managerial studies over the years; we can observe a drastic increase in the number of studies during the years which shows the interest and importance of such studies. Extensive research has sought to provide guidance on how such practices should be implemented and identify which infrastructure changes ED managers might need to initiate in their departments. In what follows, we review these methods, addressing their history, development, and current potential research opportunities. Mainly, we shed light on the following techniques: triage approaches, bed management, dynamic resource allocation, the grouping of patients and prioritization of specific groups, and fast-track.

Frequency of published queueing-related studies on the managerial approaches applied to the ED environment
Triage Approaches
The word “triage” comes from the French word “trier,” which means “to sort.” The medical usage of the term dates back to the 1800s when medical officers on the battlefield realized that sorting wounded soldiers according to their medical needs (unsalvageable; in need of immediate attention; less urgent) could improve the efficiency of their treatment. The formal concept of triage was first introduced in EDs in the late 1950s and early 1960s when a significant patient volume increase occurred. During this time, triage was carried out by triage nurses, whose competence had to be tested.
Brillman et al. [ 24 ] and Gilboy et al. [ 25 ] were among the first to point out the problematic nature of this type of triage. Brillman et al. examined agreement among observers regarding the need for ED care and the ability to predict at triage the need for admission to the hospital. They used a crossover design in which each subject was subjected to three types of triage: nurse-guided triage first, computer-guided triage second (or vice versa), and physician triage last. The authors found significant variability among physicians, nurses, and the computer program regarding triage decisions. Gilboy et al. emphasized the need for standardization and quality to ensure reliable, reproducible triage nurse decisions. Additional researchers began to question the reliability of triage methods: Wuertz et al. [ 26 ] and Fernandes et al. [ 27 ], for example, measured inter-rater and intra-rater agreement within existing ED triage systems. Wuertz et al., who based their study on five standardized patient scenarios of complaints, concluded that triage assessments (both inter-rater and intra-rater) by experienced personnel are inconsistent. These results challenged the reliability of current ED triage practices. Fernandes et al. conducted an experimental study using standardized patient scenarios with emergency triage nurses and attending emergency physicians. They found fair intra-rater agreement among the nurses rating the severity of patient conditions and a consensus in inter-rater assessment (between nurses and physicians) of triage classification.
In light of these concerns, subsequent studies sought to improve the triage process using a combination of nurse and doctor triage, referred to as team triage. Subash et al. [ 28 ] observed a scenario of 3 h of combined doctor and nurse triage within 8 days. They found that this type of triage led to earlier medical assessment compared with nurse triage and that the benefit would carry on for the rest of the day, even after standard nurse triage had resumed. The authors concluded that the implementation of team triage has the potential to improve ED efficiency substantially. In 2006, Choi et al. [ 29 ] evaluated how stationing a senior ED doctor in triage instead of a separate consultation room influenced the waiting time and processing time of an ED without extra staff. The approach, called Triage Rapid Initial Assessment by a doctor (TRIAD), was implemented in an ED for seven shifts of 9 h each. The authors found that TRIAD use substantially reduced the waiting time and processing time of the ED. Two systematic literature reviews of triage-related interventions were done by Wiler et al. [ 30 ] who dealt with triage strategies that may improve front-end operations in EDs, and Oredsson et al. [ 31 ] who showed that team triage is likely to result in a shorter waiting time and shorter LOS; furthermore, it is most likely to result in fewer patients leaving without being seen, compared with triage processes that do not involve physicians. Burström et al. [ 32 ] compared the performance of different triage models (physician-led team triage, nurse first/emergency physician second, and nurse first/junior physician second) used in three Swedish EDs. They observed that physician-led team triage seemed advantageous in efficiency and quality indicators compared to the other two models. In a subsequent study, Burström et al. [ 33 ] compared efficiency and quality measures before and after the replacement of a nurse triage model in an ED with physician-led team triage (implemented during the daytime and early evenings on weekdays). Physician-led triage was shown to improve the efficiency and quality of EDs.
Additional recent studies concerning team triage methods include the work of Traub et al. [ 34 ], who proposed that the mechanism by which team triage improves ED throughput is that of Rapid Medical Assessment (RMA). Namely, a physician’s presence enables patient medical needs to be assessed and processed more quickly. The authors observed that implementing an RMA system in the ED of their institution (the Mayo Clinic) improved patient LOS but did not reduce the number of patients left without being seen. Lauks et al. [ 35 ] introduced the concept of the Medical Team Evaluation (MTE), encompassing team triage, quick registration, redesign of triage rooms, and electronic medical records. The authors carried out an observational study investigating how the implementation of such a method affects door-to-doctor (waiting) time and ED LOS. Notably, the authors observed an improvement in LOS only among the least urgent patients. Jarvis [ 36 ] performed a literature review to identify evidence-based strategies to reduce the amount of time spent by patients in the ED. The author noted that the use of doctor triage, rapid assessment, streaming, and the co-location of a primary care clinician in the ED have all been shown to improve patient flow.
Several studies based on queueing principles have sought to evaluate and compare various triage approaches. Connelly and Bair [ 37 ], for example, showed that DES could be used to assess the efficacy of triage operations and used the approach to compare two triage methods. Ruohonen et al. [ 38 ] used a simulation model to evaluate a team triage method (in their study, the triage team comprised a nurse and a doctor, in addition to a receptionist). He et al. [ 39 ] also proposed a simulation approach to examine different triage strategies.
Finally, several studies have examined specific characteristics of the triage process to identify potential areas for improvement. Farrohknia et al. [ 40 ] evaluated the reliability of several triage scales designed as decision support systems to guide the triage nurse to a correct decision. Similarly, a study by Claudio et al. [ 41 ] evaluated the ESI’s (Emergency Severity Index) usefulness as a clinical decision support method for prioritizing triage patients, and more recently, Hinson et al. [ 42 ] checked the influence of its accuracy on nurse triage. Olofsson et al. [ 43 ] investigated the influence of staff behavior during triage on ED elderly patients’ feelings and satisfaction. O’Connor et al. [ 44 ] evaluated the effect of ED crowding on triage destination and waiting times. In 2018, Gardner et al. [ 45 ] studied a revised triage approach identifying eligible patients at triage based on complaint and illness severity and reallocating a nurse practitioner (NP) into the triage area of an urban ED. This process was shown to improve the ED throughput and reduce the number of patients left without being seen.
A more recent paper by Hodgson and Traub [ 46 ] deals with diverse variations of patient assignment systems, including provider-in-triage/team triage, fast-tracks/vertical pathways, and rotational patient assignment. The authors discussed the theory behind such system variations and reviewed potential benefits of specific models of patient assignment found in the current literature.
It results from the above review, and is shown in Fig. 5 , that triage approaches in healthcare and more specifically in the EDs have been studied and applicated throughout the years, with a massive increase in the recent decade. This is due to the technological improvements over those years, from both academic and managerial points of view. As noted above, in the early years, the medical triage methods were rather simple and even intuitive, and the breakthrough in that field occurred in the 1990s with studies regarding the usefulness of the triage methods and their reliability. Since the first decade of the twenty-first century, additional methods could be developed thanks to the increasing computation capacity and availability of data in hospital systems. A large amount of information is continuously gathered into those systems and is now available, not only to medical decision-makers but also for academic use. As online data are available, more than ever, to the triage staff, real-time decisions are now made possible that considerably improve the ED triage process. It is also expected that future technological developments will make this process more and more effective and increase its importance in resolving queueing problems in the EDs.

Frequency of published queueing-related studies in the managerial field of triage in the ED environment
Bed Management
ED departments have always dealt with bed management problems, well before the problem was formally defined. Broadly, bed management refers to the allocation of beds to incoming patients in a manner that ensures that enough beds are available for emergency patients while not “wasting” bed space, i.e., leaving too many beds empty for too long. Traditionally, bed management decisions have been the ED staff’s responsibility (sometimes, but not always, formally referred to as bed managers): the shift manager, triage staff, or the nurses/physicians who received patients. Until recently, these decisions were not necessarily made systematically but rather were based on the decision-maker’s experience.
In 1994, Green and Armstrong [ 47 ] formally defined the problem of bed management as “keeping a balance between flexibility for admitting emergency patients and high bed occupancy” and outlined the bed manager’s responsibilities. Boaden et al. [ 48 ] adopted a similar definition of the problem while emphasizing that a bed manager’s decisions should take into account operational (immediate) considerations and strategic factors that affect the flow of patients. They also identified several key criteria that bed managers must fulfill to be effective in their roles: For example, the bed manager should be able to deal with crisis scenarios and resource shortages. To do so, the bed manager should have knowledge about the specific ED and its work methods, as well as familiarity with the patients in it; he or she should be able to exercise authority over the staff and patients and should possess the ability to obtain relevant information and send data to other parts of the hospital if needed (for example, information relating to occupancy in other departments).
Several field studies published in the 2000s have shown that effective bed management can indeed improve ED efficiency. Proudlove et al. [ 49 ] claimed that efficient bed management can play a key role in resolving overcrowding in EDs and accident departments. In their work, the authors provided a clear scheme of the patient’s “journey” through the hospital and bed management’s role in that journey. In 2008, a study by Howell et al. [ 50 ] empirically showed that “active” bed management — in which a designated bed manager assesses bed availability in various departments and “reshuffles” bed assignments according to the needs — can substantially reduce patient waiting times. A study by Ben-Tovim et al. [ 51 ] showed that applying the managerial approach of “lean thinking” to hospital processes such as bed management enabled a hospital in Australia to cope with increasing demand. Notably, the approaches adopted in these studies were not based on queueing theory principles but rather on managerial expertise.
The study of bed management from a queueing theory perspective is still in relatively early stages of development, suggesting that there is a great deal of room for researchers to improve and optimize bed management procedures. The first studies in queueing theory that began to address bed management were published in the 1980s and early 1990s. These included the papers by Kao and Tung [ 21 ] and Worthington [ 23 , 24 ] which referred to waiting lists for healthcare services, in which patients effectively queued for available beds.
In 2001, Mackay [ 52 ] proposed quantitative models for bed occupancy management and planning (the bed occupancy management and planning system and Sorensen’s multi-phased bed modeling) and elaborated on his experiences applying these models to healthcare systems in Australia. Mackay noted that these models could provide healthcare planners and managers with important information about patient flows and bed numbers suitable for different resource planning strategies. However, they cannot yet replace the information obtained through the day-to-day process of bed management. In 2002, Gorunescu et al. [ 53 , 54 ] published two of the best-known papers addressing queueing theory and bed management combined. In the first paper [ 53 ], they used an M/PH/c queueing theory model to describe patient movement through a hospital department and proposed an optimization approach to improve the use of hospital resources. Their model was based on the premise of balancing the cost of empty beds against the cost of turning patients away. In their second paper, Gorunescu et al. [ 54 ] checked how changing admission rates, LOS, and bed allocation affects bed occupancy. They found that 10–15% of the beds should stay empty to ensure high responsiveness and cost-effectiveness.
In the last 10 years, bed management studies using operation research and queueing theory have gained momentum. In 2012, Hall [ 55 ] devoted a chapter of his Handbook of Healthcare System Scheduling to an overview of bed management from an operations research perspective. He defined the need for beds as a function of the population’s health and age, technology (e.g., the capacity to serve patients in an outpatient setting rather than to hospitalize them), efficiency and quality of serving patients, and, finally, efficiency in bed management (e.g., capacity to coordinate and predict patient flow). The purpose of bed management, according to Hall, is to reduce the time when beds are unoccupied and thus unproductive. He noted the importance of acknowledging that not all beds are alike and provided a classification of hospital beds according to their availability and purpose (adult/young care, surgical, etc.). Finally, he proposed a few indices for measuring the performance of bed management processes: e.g., throughput per bed, waiting time for beds, and occupancy level.
Recent operations-focused modeling studies of bed management include the work of Mackay et al. [ 56 ], who built a simulation model aimed at facilitating collaborative decision-making in hospitals with regard to patient flow and bed management. Tsai and Lin [ 57 ] proposed a multi-attribute-value theory application for bed management using preference-based decision rules. Building on previous research in queueing theory, they developed and solved a mathematical programming model that used empirical data from EDs and took into account patient preferences. Their simulations suggested that it is possible to significantly improve bed assignment quality in the ED regarding resource utilization and patient satisfaction. Recently, an interesting simulation study of bed allocation to reduce blocking probability in EDs has been performed by Wu et al. [ 58 ] using a case study in China. They demonstrated that the blocking probabilities can be significantly reduced under different priority assignment cases and total number of beds.
Wargon et al. [ 59 ] proposed a queueing model and a DES approach to optimize bed management in the ED, with the ultimate aim of reducing transfers from the ED to other institutions. Like Mackay et al. [ 56 ], they suggested that simulation is a powerful tool for bed management decision-making. Their model’s key benefit is its simplicity, making it very flexible and easy for ED staff to adapt to their needs. In 2015, Belciug and Gorunescu [ 60 ] proposed a complex “What-if analysis” of resource allocation by creating a framework that integrated (1) an M/PH/c/N queueing system (N is the maximum capacity of patients in the ED); (2) a compartmental model; and (3) an evolution-based optimization approach. The efficiency of the combination of queueing theory and evolutionary optimization was proven on the task of optimizing patient management and healthcare costs. They illustrated their model’s applicability using data from a geriatric department of a hospital in London, UK. Most recently, Folake et al. [ 61 ] analyzed the use of queueing models in healthcare with an emphasis on accident and emergency department (AED) of a city hospital. They determined the optimal bed count and its performance measure for improving the patient flow.
As noted above, and is shown in Fig. 6 , bed management was already an addressed issue, even before its formal definition in the 1990s and has undergone a steady increase in interest since then. Since 1980 and also during the first decade of the current millennium, its importance was shown by researchers to help with the crowding, waiting times, and utilization of the hospital’s resources. At the same time, a new stream of literature began to improve this important task: the queueing models for bed managing. Using theoretical models of increasing complexity, researchers were able to design various real-life case studies and to optimize them analytically, by heuristics and simulations. Those models remain in place, but as already available resources continuously experience wear and tear and new or improved resources are brought into the hospitals, they can still be improved and modified to suit real-life scenarios. This could be done, for example, by dividing the various resources into servers and sub-servers, for various beds and armchairs etc.; that in addition to applying those models to various and specific EDs with different settings.

Frequency of published queueing-related studies on bed management in the ED environment
Dynamic Resource Allocation
About two decades ago, researchers and practitioners began to propose flexibility in resources such as the number of beds (and the number of patients occupying them), nurses, physicians, and even patients as a means of reducing crowding in hospital departments. Baschung et al. [ 62 ], for example, described a concept of “floating bed,” in which individual clinic departments only have the minimum of fixed beds and the other beds are shared among the different departments in a flexible way. The method was implemented successfully in a Swiss hospital; however, the authors did not specify how it influenced ED processes. Notably, no further practical application of the specific method was reported.
In 2005, Bard and Purnomo [ 63 ] designed a branch-and-price algorithmic methodology for dynamic nurse scheduling based on fix shifts and floating nurses according to the needs. The computational results showed that a daily adjustment problem can be solved efficiently for up to 200 nurses. Another example of the “floating nurses” method can be found in the study from 2012 by Wang et al. [ 64 ]. The authors constructed a simulation-based research at a large community hospital and showed, among other findings, that adding a floating nurse can substantially improve the LOS in the ED, and it can lead to a 33% reduction in LOS, which is substantial. Zlotnik et al. [ 65 ] used support vector regression and M5P trees to forecast visits and to create dynamic nursing allocation. Their results showed that ED visits can be potentially managed with dynamic nurse staff allocation, resulting in improvements in both understaffing risk reduction and direct personnel cost savings. The aim of a research by Bornemann-Shepherd et al. [ 66 ] was to improve safety, as well as patient and staff satisfaction. They found a significant improvement of that satisfaction when creating an inpatient unit within the ED in which floating nurses from the hospitalization department work when the ED’s crowding demands that.
Another use of flexible resources can be found in the paper of Tan et al. [ 67 ] who considered the dynamic allocation of physicians at the ED. They proposed an online and historical data-driven approach and showed by simulation that their approach allows the ED to better cope with the demands and service levels needed. In 2017, Bakker and Tsui [ 68 ] proposed a dynamic allocation method of specialists for efficient patient scheduling using a data-driven approach and a DES. The authors were able to show that their method improves patient service quality as well as waiting times without change in resource capacity. In a more classical aspect of resources, Luscombe and Kozan [ 69 ] proposed a dynamic scheduling framework to provide real-time support for managing resources in the ED. The authors used the settings of parallel machine and flexible job shop environments to schedule beds and task resources allocation in a dynamic way. The heuristic solution method was implemented as a dynamic algorithm that receives information about patient arrivals and treatment tasks and incorporates the new data into an efficient resource allocation schedule.
A novel definition of dynamic resource allocation was made in 2015 by Elalouf and Wachtel [ 70 ], who studied the use of dynamic patient allocation for the ED and patient benefits. In this article, the authors proposed an algorithmic approach for optimizing the implementation of the so-called floating patient method and assessed the approach using empiric observations. They showed that they could thus ameliorate the work rate and crowding balance between the ED and the other departments. In subsequent work, Elalouf and Wachtel [ 71 ] incorporated the method into an algorithm aimed at optimizing the scheduling of patient examinations, assuming a constraint on the maximal LOS allowed in the ED. In 2017, they analyzed a holistic approach to the dynamic patient allocation method, considering crowding in the ED and other hospital departments [ 72 ]. They also considered the extent to which information has been made available about the patient’s condition in addition to other factors such as its severity and the effect of crowding on treatment time. They suggested that their tool can enhance decision-makers’ capacity to balance crowding in the ED with crowding in other hospitalization departments.
In contrary to other fields, the dynamic resource allocation field is relatively a new one in the EDs, as research in this area has gained momentum only in the last decade. Although the field has not been studied in depth before and therefore no research literature is available, the term “floating” applied to nurses and beds was familiar and used in practice with respect to ED teams. The resources previously considered were beds and nurses as they were available and flexible for allocation. In the last decade, additional resources have been taken into account as the need for limited budget solutions has arisen and the quantity and availability of online data from hospital information systems are constantly increasing. Thanks to advances in information availability, these resources can now be managed in the same way as in other industries to improve the efficiency of the ED queuing system. As shown in Fig. 7 , the massive growth in the number of relevant publications involving dynamic allocation in EDs began in the second decade of the present century with the mentioned increase in availability of data and the possibility to “float” physicians, specialists, and even patients themselves for the benefit of the system and patients. Future research may address those new areas and look for the best strategies to assign them.

Frequency of published queueing-related studies on dynamic resource allocation in the ED environment
Grouping of Patients and Prioritization of Specific Groups
The concept of grouping and prioritizing patients in the hospital is not a new one. It entails giving shift managers/ED managers the ability to group patients with similar conditions together or prioritize specific treatments when resources are limited. The most widely known patient classification system is the diagnosis-related groups (DRG) system. This classification system was first introduced by McGuire [ 73 ] and has undergone considerable modifications over the years. Sanderson and Mountney [ 74 ] reviewed the concepts on which patient groups are based in order to identify means of better adapting healthcare grouping designs to the needs of the population. They emphasized the potential of appropriate group design to facilitate data analysis and, consequently, contribute to effective planning and management of healthcare resources. King et al. [ 75 ] applied lean thinking concepts to show the impact of streaming on waiting times and total durations of stay in the ED. El-Darzi et al. [ 76 ] proposed a novel grouping methodology based on the premise that identifying groups of patients with common characteristics and detecting the workload associated with each group might help predict resource needs and improve resource utilization. The methodology involved grouping patients according to their LOS, by fitting Gaussian mixture models to empirical LOS observations. Gorunescu et al. [ 77 ] further developed this method and used surgical data to assess the models they proposed. Xu et al. [ 78 ] proposed a patient grouping method based on their required medical procedures and compared several grouping techniques applied to real ED data.
In recent years, a group at Pennsylvania State University has produced extensive research on methodologies for prioritizing ED patients, though not with a queueing-related focus. Claudio and Okudan [ 79 ], for example, used a hypothetical case study to investigate the use of multi-attribute utility theory in the prioritization of ED patients. Their method addressed cases where it is necessary to prioritize multiple patients who present the same acuity level (implying that treatment prioritization is not a straightforward task) but with different vital signs. Fields et al. [ 80 ] subsequently carried out a study that acknowledged the reality in which multiple decision-makers (e.g., nurses and other staff members) disagree on the priority rankings of different patients, a problem called the rank aggregation problem. Ashour and Okudan Kremer [ 81 ] developed an algorithm based on a management philosophy called Group Technology (GT), which is grounded on the premise that knowledge about groups leads to efficient problem-solving. The authors applied the algorithm to triage analysis in an ED system. In a subsequent study [ 82 ], they extended this idea to develop a Dynamic Grouping and Prioritization (DGP) algorithm that identifies the appropriate criteria for grouping patients and prioritizes them according to patient- and system-related information. This approach’s simulation demonstrated its superiority to a baseline grouping and prioritization method (based on the ESI) in terms of patient average LOS in the ED.
Recently, several grouping and prioritization methods have emerged that explicitly address queueing problems in the ED. Tan et al. [ 83 ] proposed using a dynamic-priority queue to dispatch patients to consultations with doctors. They tested the proposed model using a simulation and observed that it effectively reduced patient LOS and improved patient flow. In 2019, Ding et al. [ 84 ] analyzed the patient routing behaviors of ED decision-makers in four EDs of metro Vancouver. They proposed a general discrete choice framework, consistent with queueing literature, as a tool to analyze prioritization behaviors in multi-class queues. They observed that decision-makers in all four EDs applied a delay-dependent (dynamic) prioritization approach across different triage levels. In the same year, Zhang et al. [ 85 ] proposed a new patient queueing model with priority weight to optimize the ED management and analyze the impact of prioritization on the outpatient queueing system in an ED with limited medical resources.
Since it was first formally introduced, the concept of grouping patients changed drastically. Although it is proven, and well known, that efficient implementation of patient grouping can improve patient care and workload management in the ED, the optimal way to apply the concept is still not found. Since the ESI was developed in 1999 by Gilboy et al. [ 25 ] who brought the idea of grouping to all EDs, the grouping and prioritization methods have been addressed in studies whose number has increased significantly in the recent years as shown in Fig. 8 . Grouping criteria evolved, since the initial one defined by the first use of the ESI, i.e., the characteristics of patients such as symptoms and risk situation and now commonly involve the required medical procedures. Other streams attacked the prioritization of patient groups when using the entire hospital’s information systems and the real-life cases that can arise from such use. From the perspective of queueing theory, this field is still very open for improvement as it can be well combined with other managerial methods with various definitions and models.

Frequency of published queueing-related studies in the managerial field of grouping and prioritization in the ED environment
The fast-track method is one of the best-known and most commonly used methods for directing patient flow in EDs. In effect, this method’s premise is similar to that of the grouping and prioritization approach discussed above. It entails treating acutely ill patients separately from minimally ill patients in the ED, such that the latter can be seen and discharged rapidly. The fast-track method originated in the EDs themselves during the 1980s. Researchers in medicine and management began to write about it in the late 1980s and 1990s; they studied its usefulness and explored means of improving its implementation. Meislin et al. [ 86 ], for example, tested a fast-track method for 10 weeks in a teaching hospital and showed that it decreased patient waiting times (as compared with the previous system the hospital had in place) and increased patient satisfaction. In 1992, Wright et al. [ 87 ] reviewed a 1-year implementation of this method and reported high satisfaction levels among patients and medical staff. Cardello [ 88 ] studied the introduction of nurse practitioners to help reduce waiting time for ambulant nonurgent cases in fast-track program. Fernandes et al. [ 89 ] applied a managerial approach called Continuous Quality Improvement (CQI) to improve fast-track care in a hospital ED; the authors observed that the approach reduced patient LOS. They continued [ 90 ] to explore the outcomes of their hospital’s fast-track approach; they observed that (1) reducing patient LOS was associated with a decrease in the number of ED patients who left without seeing a physician; and (2) many patients leaving the ED without being seen had been classified as urgent (opposed to nonurgent) at presentation. Cooke et al. [ 91 ] investigated the influence of the use of a fast-track on waiting times within accident and emergency (A&E) departments in the UK. They assessed that it decreases the number of patients enduring long waits without delaying the care of those with a more severe injury.
Subsequent field studies on the implementation of the fast-track method include the work of Sanchez et al. [ 92 ], who studied the influence of the opening of a fast-track area in an ED in a US hospital. They concluded that the opening of the fast-track area improved ED effectiveness, as measured by a decrease in waiting times and LOS, without leading to deterioration in the quality of care provided, as measured by rates of mortality and revisits. Darrab et al. [ 93 ] examined the use of a fast-track method in a Canadian hospital; they showed that fast-tracking lower-acuity patients reduced overall LOS and the left-without-being-seen rate without affecting higher-acuity patients in the ED. A study by Nash et al. [ 94 ] evaluated the efficacy of a newly developed fast-track area in a university-affiliated ED and concluded that patients did move more quickly through the department after adding the fast-track unit. Combs et al. [ 95 ] evaluated the outcomes of adopting a fast-track approach in an Australian hospital. They determined that the fast-track reduced congestion in the ED waiting area and improved staff morale. Considine et al. [ 96 ], who also studied a fast-track program in Australia, concluded that the fast-track decreased LOS in the ED for non-admitted patients without compromising waiting times and LOS for other ED patients. Oredsson et al. [ 31 ], discussed above, carried out a systematic review of studies on interventions aimed at improving flow processes in EDs. They showed that introducing a fast-track for patients with less severe symptoms consistently results in shorter waiting time, shorter LOS, and fewer patients leaving without being seen.
Recent field studies evaluating adjustments to fast-track processes include a controlled study by White et al. [ 97 ], who showed that a Lean-based reorganization of fast-track process flow improved fast-track ED performance (in terms of LOS, percent of patients discharged within 1 h, and room use), without adding expense. Manno et al. [ 98 ] examined a fast-track system in which lower-acuity patients were streamed to specialized care areas and determined that they were seen quickly by specialists and safely discharged or admitted to the hospital without diverting resources from patients with high-acuity illness or injury.
During the 1990s, owing to advancements in technology and processing power, many researchers began to use simulation models (as will be discussed in detail in the section dealing with scientific modeling) to evaluate and seek methods of improving the implementation of the fast-track method. Kraitsik and Bossmeyer [ 99 ], for example, developed a simulation to evaluate the establishment of a fast-track lane adjacent to the main ED and a large-capacity lab to improve patient flow. McGuire [ 100 ] carried out a simulation study for improving patient flow in the ED of a specific hospital that implemented a fast-track system. Their simulations yielded several recommendations, including adding an additional registration clerk during peak hours, adding a holding area for waiting patients, extending the fast-track lane hours, and using more senior physicians instead of residents in the fast-track area. Garcia et al. [ 101 ] used a simulation approach to determine whether a specific hospital would benefit from implementing a fast-track method; they found that a fast-track system using a minimal number of resources would indeed greatly reduce patient waiting times. Kirtland et al. [ 102 ] examined 11 alternatives to improve patient flow in an ED in Maryland and found that the use of a fast-track method, combined with treating the patients at the same place in which they wait and using point-of-care lab testing procedures, could substantially reduce the average patient’s LOS. In the early 2000s, Sinreich and Marmor [ 103 – 105 ] developed simulation tools to assist ED decision-makers; these tools divided patients into categories—one of them was a “fast-track” category—and used these categories to predict patient LOS (see “Sect. 5.3 ” for additional details). Marmor et al. [ 106 ] also developed a methodology for ED design to help the ED managers and used it to compare triage and fast-track operational models in different hospitals. La and Jewkes [ 107 ] used DES to model an ED’s fast-track system and determined an optimal fast-track strategy to improve performance measures. They used real data to evaluate the effectiveness of several fast-track strategies within a hospital ED and showed practical implications for reducing patient wait times in EDs.
Additional queueing theory-based approaches to the analysis of fast-track applications include the works of Roche and Cochran in 2007 [ 108 ] and 2009 [ 109 ], who developed a queueing network model to describe a scenario in which acute patients are treated in specific areas of the ED and “fast-tracked” patients are flexible in terms of where in the ED they can be treated. Their model can be used to derive recommendations to increase ED utilization and minimize the number of “walk-aways,” i.e., patients who leave without receiving treatment, with the help of operation research methods. Recently, Fitzgerald et al. [ 110 ] used a queue-based Monte Carlo analysis to support decision-making for implementing an ED fast-track. They expanded the simple queueing model with a DES that enabled them to calculate waiting times. Their results indicated that implementing a fast-track can reduce patient waiting times without increasing demand for nursing resources.
In conclusion, the fast-track is one of the most intuitive method for reducing the average LOS in the ED, and therefore, it has been well studied during the years since the late 1980s, as shown in Fig. 9 , and proved to be very effective in terms of both LOS and patient satisfaction. Two major streams of literature are strongly recognizable for this method: one includes the field studies, which are most common as the fast-track method is applied in many places around the world and with many settings. The other stream is the simulation-based research that increased drastically since the early years of the millennium, with the growing availability of data from the hospital information systems. Although case studies with specific implementation are still used commonly, most of the current research in the field is based on simulations whose effectiveness varies with the data availability of the specific hospital or ED.

Frequency of published fast-track studies in the ED environment
Scientific Modeling Methods
This section focuses on the diverse scientific modeling methods and approaches used to investigate ED applications of queueing-related problems in operations research. In 2010, Eitel et al. [ 111 ] described the ED as a service business and then discussed specific methods, such as triage, bedside registration, Lean and Six Sigma management methods, statistical forecasting, queueing systems, discrete-event simulation modeling, and others, to improve the ED quality and flow. In 2011, Wiler et al. [ 112 ] reviewed and compared modeling approaches to describe, and at times predict, ED patient load and crowding and evaluated their limitations. Saghafian et al. [ 113 ] reviewed many papers to demonstrate the contribution of operations research and management science to ED patient flow problems. More recently, Palmer et al. [ 114 ] performed a systematic literature review of operations research methods for modeling patient flow and outcomes within community healthcare. Ahsan et al. [ 115 ] reviewed various analytical models utilized to optimize ED resources for the improvement of patient flow and highlighted the benefits and limitations of these models. A range of modeling techniques including agent-based modeling and simulation, discrete-event simulation, queueing models, simulation optimization, and mathematical modeling have been reviewed in their work. In the present work, we built on Wiler et al. [ 110 ] classification, presenting a similar one and expanding it to accommodate additional approaches and more recent research. The methods included in our work are presented in the following order: queueing, priority and simulation models, statistical methods, and additional algorithms and computational methods.
The total number of relevant articles reviewed in this section as function of the year of publication is shown in Fig. 10 . Its growth in recent years emphasizes the interest and importance of further research and development of such modeling methods. It is expected that the continuously growing technological developments and the increasing availability of data as previously mentioned in “4.1” change the way that the EDs (and healthcare systems) work, and therefore can change the models needed.

Frequency of published queueing-related studies on the scientific modeling methods used in the ED environment
Queueing Models
Computational queueing models arise directly from general queueing theory. Leading textbooks on fundamentals of queueing theory and modeling that can contribute to general knowledge are those of Gross et al. [ 116 ] and Bhat [ 117 ]. According to Green’s chapter (“Queueing Theory and Modeling”) [ 118 ] in the Handbook of Healthcare Delivery Systems : “A queueing model is a mathematical description of a queueing system which makes some specific assumptions about the probabilistic nature of the arrival and service processes, the number and type of servers, and the queue discipline and organization.” A basic queueing system is defined in the same chapter as “a service system where ‘customers’ arrive at a bank of ‘servers’ and require some service from one of them.” As noted in “Sect. 3 ” above, one of the first prominent works to be published in this field was Keller and Laughhunn’s [ 13 ] discussion of a queueing model addressing physician capacity in an outpatient clinic and its influence on clinic efficiency. A good review of queueing models in the field of healthcare may be found in the above chapter by Green [ 118 ]. Fomundam and Herrmann [ 119 ] also reviewed queueing models in healthcare systems, focusing on waiting time and utilization analysis, system design, and appointment systems.
Later on, Mehandiratta [ 120 ] attempted to analyze in his paper the theory and instances of use of queueing theory in healthcare organizations around the world and the benefits acquired from the same. Palvannan and Teow [ 121 ] applied queueing theory to healthcare, using queueing models in two hospitals to estimate and analyze service capacity. Some additional papers, such as that of Bain et al. [ 122 ] and more recent ones by Cho et al. [ 123 ], Bittencourt et al. [ 124 ], and Lin et al. [ 125 ], deal with the application of queueing theory and modeling to healthcare in general.
This area has been continually studied over the years since the 1990s, although it has received much attention during the last 2 decades, as shown in Fig. 11 . Even before that, there were works referring to queueing models specifically in the ED environment. One of the first works in this area was presented by Collings and Stoneman [ 19 ]. They derived an M/M/∞ queue with varying arrival and departure rates to study the influence of patient arrival rates on bed occupancy levels. However, the most significant works involving ED queueing models began to appear around the beginning of the twenty-first century. Gorunescu et al. [ 53 , 54 ], for example, used a queueing approach to model bed occupancy in EDs as well as in other hospital departments. In 2004, McManus et al. [ 126 ] constructed a queueing theory-based model for resource allocation in various hospital activities, including EDs, and validated it in an intensive care unit. Green et al. [ 127 ] evaluated the effectiveness of a queueing model in identifying ED staffing patterns to reduce the fraction of patients who leave the ED without being seen. De Bruin et al. [ 128 ] used a queueing model to analyze congestion in the flow of emergency cardiac patients and to allocate numbers of beds, given a required service level (operationalized as percentage of refused admissions). Creemers et al. [ 129 ] showed how queueing models could quantify the relationship between capacity utilization, waiting time, and patient service, thus enabling decision-makers to improve the performance of healthcare systems, including that of EDs. Mayhew and Smith [ 130 ] used a queueing model to analyze patient flow in accident and emergency departments to evaluate government-imposed targets’ feasibility for treatment completion times. Laskowski et al. [ 131 ] utilized both agent-based and queueing models to investigate patient access and patient flow through EDs towards identifying means of reducing waiting times. Further examples of application of queueing models to ED systems and their improvement in the first decade of the twenty-first century may also be found in the publications of Morton and Bevan [ 132 ], Au et al. [ 133 ], and Tseytlin [ 134 ].

Frequency of published studies on computational queueing models arising directly from general queueing theory, with emphasis to the ED environment
More recently, Tan et al. [ 83 ] presented a hospital ED case where the queueing process can be modeled using a time-varying M/M/s queue with re-entrant patients, and tested this model using simulation. Hagen et al. [ 135 ] dealt more specifically with priority queueing models applied to ICUs and EDs; these specific models deserve special attention and will be referred to in a separate section. Wiler et al. [ 136 ] derived an ED patient flow model based on queueing theory that predicts the effects of various patient crowding scenarios on the number of patients who leave the ED without being seen. Yom-Tov and Mandelbaum [ 137 ] analyzed — in a paper based also on a previous work by Yom-Tov from 2010 [ 138 ] — a queueing model called Erlang-R that helps answer questions such as how many servers (physicians/nurses) are required to achieve predetermined service levels. Batt and Terwiesch [ 139 ] reviewed a number of queueing models and focused on the so-called Erlang-A model when studying queue abandonment from an ED. Vass and Szabo [ 140 ] applied M/M/3 queueing model to ED patient data collected over 1 year to evaluate the ED’s performance. Additional publications of interest by Alavi-Moghaddam et al. [ 141 ], Cantero and Redondo [ 142 ], Mohseni [ 143 ], and Huang et al. [ 144 ] deal to some extent with potential contribution of queueing theory and models to the improvement of that performance and will not be expanded here.
Rotich [ 145 ] used M/M/s queueing model to determine the optimum waiting and service cost in a hospital ICU emergency service. Xu and Chan [ 146 ] used queueing models which accurately predict the number of patient arrivals to the ED for the purpose of reducing waiting times in the ED via diversion and analyzed the quality of those models. Jáuregui et al. [ 147 ] analyzed the emergency service of a public hospital in Mexico by applying the concepts and relations of queueing theory. They evaluated the minimum number of doctors necessary to satisfy the current and future emergency service demands for different scenarios and added recommendations to the managerial staff. Recently, Hu et al. [ 2 ] evaluated the contributions of queueing theory in modeling EDs and assessed the strengths and limitations of this application. They concluded that queueing models tend to oversimplify operations but that combining these models with simulations (as further discussed in the specific subsection on simulation modeling) should be a powerful approach. Joseph [ 148 ] reviewed and analyzed the use and benefits of the application of queueing theory and modeling to ED resources and operations in general.
Additional noteworthy queueing models include priority queueing models such as those of Siddharthan et al. [ 149 ] and more recent ones in the 2000s; they will be discussed separately in the following subsection.
Priority Models
Priority models (sometimes called priority queueing models) are a family of queueing models developed to be goal-oriented and deserve special mention. Here we address a field of models that uses pure queueing theory models and definitions and is different from the grouping and prioritization field that arose in parallel. As such, this field is rather new in the ED environment as shown in Fig. 12 .

Frequency of published priority models studies in the ED environment
Green [ 150 ] referred to the triage system in an ED as a classic example of a priority queue. In their review of methodologies for modeling ED patient flow (discussed above), Wiler et al. [ 110 ] suggested that models based on priority principles are appropriate for ED settings, where acuity, in addition to the time of presentation, determines the order in which patients are serviced (i.e., the sickest patients are prioritized over less-acute patients). Already in 1996, Siddharthan et al. [ 149 ] classified patients into emergency and non-emergency care and provided evidence that non‐emergency patients contribute to lengthy delays in the ED for all classes of patients. They proposed a priority queueing model to reduce average waiting times. Two additional works of Au-Yeung et al. are of interest: In the first one dated 2006 [ 151 ], they used a simulation modeling to provide some insights into the effects of prioritizing different classes of patients in a real accident and emergency unit based in London, UK. In the second one from 2007 [ 152 ], they developed an Approximate Generating Function Analysis (AGFA) technique, experimenting it with different patient-handling priority schemes and comparing the AGFA moments with the results from a discrete-event simulation.
Additional examples of studies using priority queueing models include the work of Laskowski et al. [ 131 ], who developed a framework of multiple-priority queue systems as a means of forecasting patient waiting times. Tan et al. [ 83 ] developed a dynamic-priority queue model in which the priority levels are assigned to queueing patients in the ED shift with staff availability. The authors modeled the queueing process using a time-varying M/M/s queue with re-entrant patients and tested it using simulation. Hagen et al. [ 135 ] used a priority queueing model to analyze the differences between prioritizing admissions by expected LOS or patient severity. An interesting conclusion of this work was that prioritizing patients by severity not only considerably reduced delays for critical cases but also increased the average waiting time for all patients. Lin et al. [ 153 ] developed a priority queueing model to describe the flow and waiting times of patients waiting for ED service and subsequently for hospitalization in an inpatient unit. The model assumed that emergency patients are characterized by different priority levels and incorporated priority-specific waiting time targets.
More recently, a paper by De Boeck et al. [ 154 ] dealt with confronting the physicians with the challenging task of prioritizing between boarding patients and patients currently under treatment in the ED. Their case study showed that system performance is optimized by applying a policy that gives priority to patients currently under treatment in the ED. In 2020, Hou and Zhao [ 155 ] presented a practical approach to estimate the waiting times for multi-class patients and applied it to reduce the waiting time for high priority patients. Using a case study from an ED, they found that the proposed approach can efficiently prioritize patient flows in decreasing waiting times.
Simulation Models
Simulation is a very popular approach for describing complex processes and the ED environment as such is not different. As visible in Fig. 13 , this area has been well studied since the 1990s with massive growth in the 2000s due to the availability of data and computational power. We see on the figure a peak in the occurrence of such studies at the end of the first decade of the present century. It can be explained by the fact that their use has been very effective since then, thus reducing to some extent the demand for further theoretical research. A vast number of ED studies using computer simulations have been published over the years; thus, we will review in detail only the most important ones.

Frequency of published simulation-based studies in the ED environment
Ladany and Turban [ 20 ] were among the first to use a queueing simulation model to provide a framework for the planning and staffing of an ED. They emphasized the benefits of a simulation approach as a means for ED managers to obtain information about costs, waiting times of patients, and idleness of facilities. Saunders et al. [ 156 ] used simulation software to develop a computer simulation model of ED operations. The authors varied selected input data systematically to determine the effects of various (simulated) factors on patient throughput time, queue sizes, and resource utilization rates. Lopez-Valcarcel and Perez [ 157 ] claimed that simulation is an effective tool for the analysis of the complex problem of scheduling in the ED and that the result can be used to improve its quality care and efficiency. In the same year, McGuire [ 100 ] also used simulation software to identify means of reducing patient LOS in EDs. Nearly a decade later, Samaha et al. [ 158 ] published a similar model based on empirical data that proved to be very helpful in the ED of their hospital. Ruohonen et al. [ 38 ] used a simulation approach — that was previously discussed in “Sect. 4.1 ” — to demonstrate the capacity of a team triage method to improve ED operations.
The increasing availability of powerful computers has led to the development and extensive use of DES, a computerized simulation approach in which discontinuous systems are modeled as networks of interdependent discrete events. DES has proven to be effective for representing queueing systems in general and ED systems in particular and has become a preferred simulation method over the years. Indeed, a literature review by Günal and Pidd [ 159 ] noted that the number of studies of DES applications to EDs has increased since the beginning of the twenty-first century.
Jun et al. [ 160 ] reviewed applications of DES modeling to healthcare clinics and systems (including EDs), primarily in areas of patient flow and resource allocation. Connelly and Bair [ 37 ] explored the potential of DES methods as a tool for analyzing ED operations and illustrated its potential by comparing the efficacy of two alternative triage methods (as discussed above in “Sect. 4.1 ”). Komashie and Mousavi [ 161 ] also developed a DES approach to model the operations of an ED. They used their model to provide ED managers of a London hospital with new insights and system improvement recommendations. Duguay and Fatah [ 162 ] used Arena software to carry out a DES study of a Canadian ED in order to derive recommendations to reduce patient waiting times and improve overall service delivery and system throughput. Hoot et al. [ 163 ] drew from theoretical studies to develop a DES of patient flow and used it to forecast overcrowding occurrences in an ED in real-time; they subsequently used observational data to validate the model’s forecasts [ 164 ]. Bair et al. [ 165 ] used a DES model of ED patient flow to investigate the effect of inpatient boarding on ED efficiency in terms of the US National Emergency Department Crowding Scale (NEDOCS) score and the rate of patients who leave without being seen. The results of their analysis helped to quantify the impact of patient boarding on ED crowding. Further papers that use DES modeling for solving queueing issues in EDs have been published in the same years, among them we notice those of Brailsford and Hilton [ 166 ], Davies [ 167 ], Kolker [ 168 ], Khare et al. [ 169 ], and Fletcher and Worthington [ 170 ]. Between 2009 and 2011, we also identify the works of Marmor et al. [ 171 ], Tseytlin [ 133 ], Marmor [ 172 ], and Zeltyn et al. [ 173 ], who belong to a common research group that specializes in the development of DES modeling techniques and their application to decision support for ED issues like staffing.
Lim et al. [ 174 ] used DES analysis to model interactions between physicians and “delegates” they supervise (such as residents, nurse practitioners, and assistants). They compared their model with a simulation model that did not incorporate such interactions and showed that the formerly produced recommendations yielding substantially better outcomes (e.g., in terms of patient LOS) than the latter did. In the same year, La et al. [ 107 ] used DES to model an ED’s “fast-track” system to derive recommendations for improving performance measures. Hurwitz et al. [ 175 ] developed an event-driven flexible simulation platform to quantify and manage ED crowding. In a further work [ 176 ], they demonstrated the ability of such a simulation model to recreate and predict site-specific patient flow in two very different EDs. El-Rifai et al. [ 177 ] focused on human resources organization in an ED and developed a stochastic optimization model for shift scheduling in it. Then they evaluated the resulting personnel schedules using a DES model and performed numerical analyses with data from a French hospital to compare different personnel scheduling strategies. Ahalt et al. [ 178 ] used DES to address ED crowding problems when the identified need for emergency services exceeds available resources for patient care in the ED, hospital, or both. Using data from a large academic hospital, they evaluated and compared three metrics commonly used in practice as future crowding indicators. More recently, Gulhane [ 179 ] presented a study that aimed to manage queues in hospital using DES simulation. He concluded that issues in hospital were well resolved through that simulation and modifications in queueing have been done. Castanheira-Pinto et al. [ 180 ] presented a methodology to assist the design process of an ED using a DES simulation technique. The ED of a hospital in Portugal was considered for testing that technique. The methodology proved to be very useful in determining an optimized operation for complex and non-linear systems.
Although DES has proven to be a beneficial simulation approach for ED contexts, several additional simulation approaches have been used successfully in these settings and warrant a mention here. Some of these are discussed in the work of Mohiuddin et al. [ 181 ], who undertook a systematic review to investigate the different computer simulation methods and their contribution to the analysis of patient flow within EDs in the UK. One of these approaches is the system dynamics approach (SD), which is used to understand complex healthcare systems’ behavior over time by capturing aggregate (instead of individual) patient flows. Brailsford et al. [ 182 ], for example, applied a system dynamics simulation to an extensive complex ED distribution system in the UK city of Nottingham and used it to identify potential bottlenecks and points that might benefit from intervention. Thorwarth et al. [ 183 ] used a simulation model within structured modeling methods, to investigate flexible workload management for an ED environment. They applied the results to derive a generalized analytic expression describing settings that lead to an instable queueing system and affected service quality; they provided thus the decision-makers with a tool which allows identifying and preventing such conditions.
In 2010, Van Sambeek et al. [ 184 ] carried out a literature review of decision-making models according to healthcare problems and showed that 46% of the studies used computer simulation. Paul et al. [ 185 ] performed a systematic review of ED simulation literature from 1970 to 2006 to highlight these simulation studies’ contributions to their understanding of ED overcrowding and to discuss how simulation can be better used as a tool to address this problem. Their review did not include a more recent simulation technique — the so-called agent-based simulation (ABS) approach, commonly used to model complex systems composed of interacting, autonomous “agents.” Laskowski and Mukhi [ 186 ] used this approach to simulate the performance of a stand-alone ED and multiple interacting EDs throughout a Canadian city. Jones and Evans [ 187 ] used an agent-based approach to model the influence of ED physician scheduling on patient waiting times. Liu et al. [ 188 ] identified ABS modeling as an excellent tool to deal with complex system like an ED and introduced such a generalized computational model for simulating ED performance. More recently, Yousefi and Ferreira [ 189 ] presented a pure agent-based simulation combined with a group decision-making technique to improve the performance of an ED.
Additional simulation studies in ED queueing use multiple simulation methods or simulations combined with a simple queueing model. The recent review by Hu et al. [ 2 ] provides a detailed discussion of such combinations and their advantages. To avoid model complexity and requirement of large amount of data collection, Ceglowski et al. [ 190 ] described a unique approach of simulation by combining data mining with discrete-event simulation and could thus provide insight into the complex relationship between patient urgency, treatment and disposal, and the occurrence of queues for treatment in the ED. Saghafian et al. [ 191 ] separated patients (and resources) into different streams and used various analytic and simulation models to determine that this streaming policy will improve ED performance in some situations. Uriarte et al. [ 192 ] used a combination of DES with multi-objective optimization to help decision-makers reduce ED patient LOS and waiting times. Fitzgerald et al. [ 110 ] supplemented DES with a queue-based simulation to demonstrate the impact of implementing a fast-track method in an ED on patient waiting times and service quality.
Finally, a new literature review of simulation modeling applied to EDs by Salmon et al. [ 193 ] covering all English language papers from the year 2000 expanded previous reviews on simulation models in EDs such as the systematic review by Paul et al. [ 185 ]. They showed that most of the works are academic, based essentially on DES, and focused on capacity, process, and workforce issues at an operational level.
Statistical Methods (Regression Analysis and Forecasting)
Statistical methods can provide robust mathematical tools for forecasting crowding in EDs. As such, and in a similar way to other fields that address the ED environment, we can observe a big and increasing interest in this field in the present century, as shown in Fig. 14 . As noted in a review by Wargon et al. [ 194 ], two of the most commonly used mathematical approaches for predicting patient attendance at EDs or walk-in clinics are regression analysis and time series analysis. (The authors noted that these models tend to achieve good performance, with errors ranging from 4.2 to 14.4%.) Regression and time series analyses applied to ED issues are discussed comprehensively in the paper of Wiler et al. [ 112 ]. A thorough description of regression techniques to be used in EDs can be found in that of Jones et al. [ 195 ] and will not be expanded here.

Frequency of published statistical models studies in the ED environment
Notable examples of applications of these methods to ED settings are included in the works of Krochmal and Ryley [ 196 ], who analyzed the increase in LOS and its resultant costs in a given ED using a two-tailed t -test regression method, and of Forster et al. [ 197 ], who used a time series method to investigate the effect of hospital occupancy on ED LOS and patient disposition. Asaro et al. [ 198 ] applied regression methods to data from a large urban academic hospital to analyze the factors influencing ED patients’ likelihood of leaving the ED without being seen. In 2008, McCarthy et al. [ 199 ] evaluated the appropriateness of prediction models by whether the data met key Poisson regression assumptions, for predicting demand for ED services in an academic hospital. They found that the demand at that facility was well approximated by a Poisson regression model. Jones et al. [ 200 ] presented a multivariate time series approach to modeling and forecasting demand in the ED. They concluded that this approach provides an adequate ED census forecast but is insufficient for predicting and managing ED resource availability. Schweigler et al. [ 201 ] investigated whether models using time series methods can generate accurate short-term forecasts of ED bed occupancy. Two of the models the authors examined were found to robustly forecast ED bed occupancy 4 and 12 h in advance at three different EDs. Further applications of those statistical methods to ED issues in the first decade of the twenty-first century may be found in papers by Steindel and Howanitz [ 202 ], Liew et al. [ 203 ], Rogers et al. [ 204 ], and Jones et al. [ 205 ]. Jones and Olsen [ 206 ], Oskaynak and Brennan [ 207 ], and Mandelbaum et al. [ 208 ] also published papers that apply methods of statistical data analysis to queueing and LOS studies in diverse EDs. Cote et al. [ 209 ] created a tutorial for ED medical directors in which they applied regression-based forecasting models to data obtained from a large teaching hospital’s ED; the versatility of the regression analysis was shown to accommodate a variety of forecasting situations readily. Shanmugam [ 210 ] demonstrated by an analysis and interpretation of real data from a hospital ED in Malta that queueing concepts and tools using probabilistic- and statistical-based approach help to efficiently manage the ED in the presence of impatient waiting patients. Afilal et al. [ 211 ] proposed a new approach to classifying ED patients in addition to time series-based models to forecast long- and short-term daily census in a city hospital in France. These models showed outstanding performance in terms of their capacity to predict the annual total flow of ED patients (accuracy of up to 91.24%) and their robustness to epidemic periods. Whitt and Zhang [ 212 ] developed an aggregate stochastic model of an ED based on a careful study of data on individual patient arrival times and length of stay in a specific hospital. In 2019, Das et al. [ 213 ] proposed a functional regression model based on customer arrival and departure time instances from an in-control ED system in a major academic hospital, and validated it using simulation and real data case studies. In 2020, Wachtel and Elalouf [ 214 ] proposed an algorithm based on a statistical analysis that considered combination of data from the hospital’s information systems and field observations to identify known and new influential factors on LOS and crowding at the ED and to treat them.
Additional Algorithms and Computational Methods
According to Wiler et al. [ 112 ], implementation of complex mathematical models aims to forecast or optimize various ED outcomes. Larson [ 13 ] was one of the first to develop a computationally efficient algorithm to analyze queueing problems related to emergency healthcare services; his model was designed for vehicle location and response district design problems. Panayiotopoulos and Vassilacopoulos [ 215 ] approached sophisticated operational problems related to a hospital ED using a general simulation algorithm that could be implemented on a small computer. However, algorithmic methods acquired strong momentum only at the beginning of the present century with the increasing use of large computers in healthcare systems, as shown in Fig. 15 .

Frequency of published algorithmic-based models studies in the ED environment
Topaloglu [ 216 ] presented a multi-objective goal programming model for scheduling work hours for emergency medicine residents. The model was tested in the ED of a prominent local university hospital and allowed good quality scheduling at reasonable times. Yeh and Lin [ 217 ] used a genetic algorithm coupled with simulation techniques to derive a nurse schedule that optimized the quality of service at a hospital ED. El-Darzi et al. [ 76 ] and Gorunescu et al. [ 77 ] applied various clustering algorithms to evaluate LOS-based methods of grouping and prioritizing patients (see discussion in “Sect. 4.4 ,” above). Wang et al. [ 218 ] utilized the expectation–maximization (EM) algorithm for modeling the throughput of EDs via available time series data. Real-world data from an ED in London helped to demonstrate the effectiveness of the introduced algorithm. Lin et al. [ 153 ] used two numerical algorithms to estimate patient waiting time in the ED.
Elalouf and Wachtel [ 70 , 71 ] developed fully polynomial-time approximation scheme (FPTAS) algorithms to assist ED decision-makers in optimally scheduling evaluations for waiting patients. The authors used these algorithms in combination with the “floating patients” method discussed above and evaluated their efficacy in simulations with real-life data [ 71 , 72 ]. Memari et al. [ 219 ] used genetic algorithms to optimize service allocations and patient flow in EDs. Ashour et al. (2016) [ 82 ] developed a dynamic algorithm that optimizes patient classification and prioritization to minimize patient LOS. Luscombe and Kozan [ 69 ] also used a dynamic algorithm to provide real-time support for managing resources in the ED. Their algorithm was tested against several test instances based on data from a real ED and achieved solutions within 5% of the optimal. Allihaibi et al. [ 220 ] developed a novel blocking patient flow (BPF) heuristic algorithm for predicting waiting times in the ED. Kuo et al. [ 221 ] applied machine learning algorithms for real-time and personalized waiting time prediction in EDs. At last, a most recent study by Pak et al. [ 222 ] uses such machine learning (ML) algorithms to optimize waiting times and significantly improve their forecasting. We are aware that this domain is a very promising one and therefore a serious candidate for intensive future research.
Finally, some researchers have experimented with alternative scientific approaches to solving ED queueing problems which have not yet been widely adopted. Examples include a game theory approach (Nash equilibrium) introduced by Hamilton et al. [ 223 ] to characterize ED overcrowding as an equilibrium state, optimal control methods described by Chockalingam et al. [ 224 ] for solving resource allocation problems, and additional studies by Do and Shunko [ 225 ] and Wang et al. [ 226 ]. A review of ED computational methods that are “not classified elsewhere” can be found in the work of Armony et al. [ 227 ]. Chaou et al. [ 228 ] and Li et al. [ 229 ] also used such interesting approaches in EDs systems analysis.
This study constitutes a systematic review of literature related to queueing-related problems in ED (and other healthcare) settings. We have sought to provide an overview of practical approaches used by ED decision-makers to manage patient flow, in addition to an in-depth discussion of the scientific methodologies used to study and improve ED operations. The increasing number of studies addressing the ED queues makes it beneficial and crucial to combine state-of-the-art queueing theory methods with managerial methods that are already applicable in practice.
Our primary goal was to provide researchers with an accessible and well-organized tool for extracting information on specific topics of interest, and our efforts concentrated throughout this work on its achievement. We reviewed 229 articles and books, from 1952 to 2021, all addressing queueing theory models and managerial methods applicable in the ED. As a result, we have been exposed to the fact that the number of studies has increased significantly as the addressed fields are expanding greatly, especially in the last decade. Our paper categorizes ED crowding into two parts: queueing theory and managerial techniques. This approach allows researchers from both aspects to find the most relevant models for their interests easily. As explained above, finding the proper model for a specific scenario is crucial for applying it in real life. This categorization can also help researchers that are new to queueing theory in EDs to get a good insight into the historical and more recent developments of this area.
As for the managerial point of view, we aimed that the methodology and detailed literature review will improve the availability of needed queuing concepts and tools to decision-makers who continuously face queueing issues in EDs. We hope also that the use of the present paper will help them to make their work more efficient and to achieve better performance in their systems.
It is a challenging task to introduce changes in an ongoing system without having a significant, and eventually bad, influence on the system and the patients. Using queueing theory models as a tool helps ED decision-makers to observe solutions and changes before their application becomes mandatory. It is even more important to combine the managerial method with the corresponding queueing model and ensure that this model fits the real-life settings. The present review aims to be an efficient tool for queueing theory scientists and healthcare managers to help them achieve the best fit between the managerial method and the scientific model.
Furthermore, some specified methods are still evolving and advancing, and the amount of data is growing continuously. Hence upcoming works that are still needed to reduce the remaining gaps between the current study and the ED needs can rely on newer approaches. Modern optimization methods such as machine learning ones have shown to be promising and we feel they justify future research. New managerial approaches like “floating” physicians, specialists, and patients are also promising and have the potential for further deliberations.
Although the present literature review examined an abundance of papers and identified multiple queueing methods for improving performance in the EDs, it could not fully address the whole scope of the domain. For example, key modeling methodologies that analyze and predict ED patient load and crowding, such as formula-based equations and other mathematical models, are expected to improve significantly in further studies. Future research should focus on those effective models and techniques that still require more development or are absent from this research.
Also, any future research from the managerial or the mathematical side should address the real life, changing environments of EDs in recent years. Until two decades ago, most studies aimed to evaluate and describe the managerial methods. With the technological developments since the early 2000s and the increasing availability of data and methods to analyze them, researchers are now able to evaluate mathematical and managerial models accurately and efficiently with greater ease. Technological advances in recent years seem to be bringing the next breakthrough in this field with more frequent use of the Internet of Things, high distribution of cellphones, radio-frequency identification (RFID), Bluetooth and Wi-Fi sensors, and strong hardware. These new technologies change the way that EDs (and healthcare systems) work, and therefore can change the models needed. For example, dynamic and online data regarding patients in the ED and even the hospitalization departments can now be considered and influence grouping, prioritization and resource decisions, and staff allocation. Hence, future studies can focus on technological developments that have the potential to improve and solve queueing problems in EDs.
Another aspect, that should be considered in future research, is the dynamic and hybrid structure of healthcare systems in recent years, which emphasizes the importance of combining the mathematical and managerial models with the technological aspects. This may open the door to new field studies and completely innovative methods. The COVID-19 pandemic had stressed the significance of a rapid and dynamic response in EDs. Even in a time of “no crisis,” with population growth, the need arises for a quick response in a time of high uncertainty and a limited budget, emphasizing the importance of effective implementation of queuing methods; even under these conditions, the proposed techniques as well as future ones are expected to increase efficiency and aid medical teams in providing adequate care for patients in the ED.
This study was funded by The Israel National Institute for Health Policy Research.
Data Availability
Declarations.
The authors declare no competing interests.
Publisher's Note
Springer Nature remains neutral with regard to jurisdictional claims in published maps and institutional affiliations.
Contributor Information
Amir Elalouf, Email: [email protected] .
Guy Wachtel, Email: li.ca.leira@WyuG .
Academia.edu no longer supports Internet Explorer.
To browse Academia.edu and the wider internet faster and more securely, please take a few seconds to upgrade your browser .
Enter the email address you signed up with and we'll email you a reset link.
- We're Hiring!
- Help Center
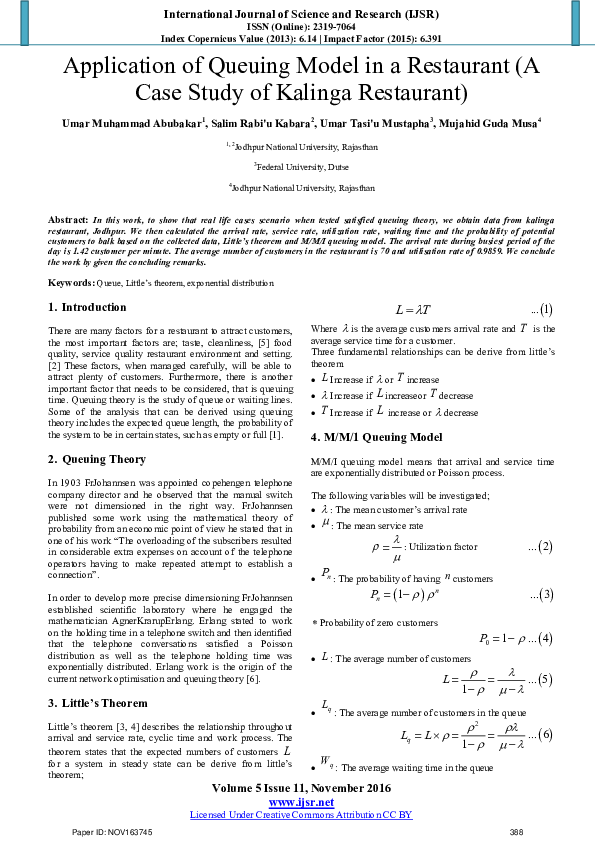
Application of Queuing Model in a Restaurant (A Case Study of Kalinga Restaurant)

International Journal of Science and Research (IJSR)
Related Papers
joel gichengo
The ultimate objective of the analysis of queuing systems is to understand the behaviour of their underlying process so that informed and intelligent decisions can be made by the management. The application of queuing concepts is an attempt to minimize cost through minimization of inefficiency and delays in a system. Various methods of solving queuing problems have been proposed. In this study we have explored single –server Markovian queuing model with both interarrival and service times following exponential distribution with parameters and , respectively, and unlimited queue size with FIFO queuing discipline and unlimited customer population. We apply this model to catering data and estimate parameters for the same. A sensitivity analysis is the carried out to evaluate stability of the system.
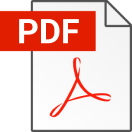
Waiting time and service system is a part of our daily life in various service areas such as: restaurant, ATM, Clinic, Toll tax, Ticket window in cinema, Bus stand and Railway station. Here, our focus is on Restaurant Management System. Every restaurant loses their customers just because of a long queue and maximum waiting time. Restaurant provides more waiting chairs only. We need to be improving the service time which shows improvement in queuing situation. Here, we apply queuing model. This paper aims to shows the multi-channel queuing model M/M/S. We use M/M/S queuing model since this restaurant has 10 service stations. We have obtained one month daily customers data from a restaurant named "Vinayak restaurant" in Laxmangarh, Sikar city. Using little's theorem and the multi-channel waiting line model M/M/S. we have determined the arrival rate λ service rate µ. The utilization rate ρ and the average waiting time in the queue before getting service. At Vinayak restaurant, the arrival rate λ is 1.40 customers per minute (cpm). Customer waiting time is 10 minute and the utilization rate is 0.49. We have discussed the benefits of applying queuing model to a busy restaurant.
IOSR Journals publish within 3 days
Bryan Sandoval
The Bulletin of Society for Mathematical Services and Standards
Nikita Chandra
SSRN Electronic Journal
wellington garikai bonga
Queuing is the common activity of customers or people to avail the desired service, which could be processed or distributed one at a time. Bank ATMs would avoid losing their customers due to a long wait on the line. The bank initially provides one ATM in every branch. But, one ATM would not serve a purpose when customers withdraw to use ATM and try to use other bank ATM. Thus the service time needs to be improved to maintain the customers. This paper shows that the queuing theory used to solve this problem. We obtain the data from a bank ATM in a city. We then derive the arrival rate, service rate, utilization rate, waiting time in the queue and the average number of customers in the queue based on the data using Little's theorem and M/M/I queuing model. The arrival rate at a bank ATM on Sunday during banking time is 1 customer per minute (cpm) while the service rate is 1.50 cpm. The average number of customer in the ATM is 2 and the utilization period is 0.70. We conclude the paper by discussing the benefits of performing queuing analysis to a busy ATM.
Mohani Abdul
E-banking provides users, working with a home computer attached to the network to their bank, with the ability to authorize payments, reconcile accounts, and access a variety of other banking services with the help of ATM, Visa Card, Master Card, Q-cash, E-cash, Ready cash, Mobile, Internet etc. E-banking has a lot of benefits which add value to customers' satisfaction. For collecting the data a highly structured questionnaire was made. The questionnaire was designed into four parts. The statistical techniques used for the analysis were the descriptive analysis, ANOVA analysis and Chi-Square test. There are some associated challenges identified in the study that seems to hinder the success of e-banking services and thus constitute a major concern to both financial institutions and customers. The paper recommends various measures should be put in place to make e-banking system smooth, effective and more secure.
Intan Uswatun Khasanah
The purposes of this study were to 1) map the value chain of hazardous waste management, 2) analyze value chain governance an Indonesianc ompany. This research used the descriptive method that used value chain analysis, value chain governance analysis, gap analysis. Based on value chain analysis, there are nine actors in the whole hazardous waste management value chain in PT XYZ: operational raw material suppliers, 3rd party logistic supplier, recycle facility, transporter, collector, utilizator, processor, microcapsule facilitator and generator of hazardous waste. The relationship between PT XYZ and the operational raw material supplier is modular, between PT XYZ and 3rd party logistic supplier is captive, and between PT XYZ and the recycle facility is more likely modular. The results of gap analysisare some attributes that are still below the average likerecycle facility with the type of waste to be treated, landfill treatment which has competitive prices, the collector who has a competitive price and processor which has speed of processing time, the transporter that ha s the accuracy of the transport time, recycle facility processors, and the transporterhave competitive prices.
John Adanse
RELATED PAPERS
BEST: International Journal of Management, Information Technology and Engineering (BEST: IJMITE)
BEST Journals
Research & Reviews: Journals of Statistics and mathematical Sciences
Anthony Onoja
Tabish S. A.
International Journal of Science and Research [IJSR]
chidi lloyd
Prof. Mona Nasr
Diego Vásquez Silva
Mathematics and Statistics
Horizon Research Publishing(HRPUB) Kevin Nelson
Okoro Joshua
Monira Alam
Gerontechnology
Kardi Teknomo
Mohammad Anisur Rahman
Karthick Salem
Yoga Subramanian
American Journal of Theoretical and Applied Statistics
Etaga Harrison
Solomon Anab
American Journal of Operations Research
Sulaiman Sani
Elliot Mbunge
Sreenu Banoth
Syamsiah Badruddin
Nazım Çatalbaş
Satya Prakash Rout
Independent Journal of Management & Production
Gordon Bubou
- We're Hiring!
- Help Center
- Find new research papers in:
- Health Sciences
- Earth Sciences
- Cognitive Science
- Mathematics
- Computer Science
- Academia ©2024
- Search Search Please fill out this field.
What Is Queuing Theory?
- How It Works
Special Considerations
- Applications
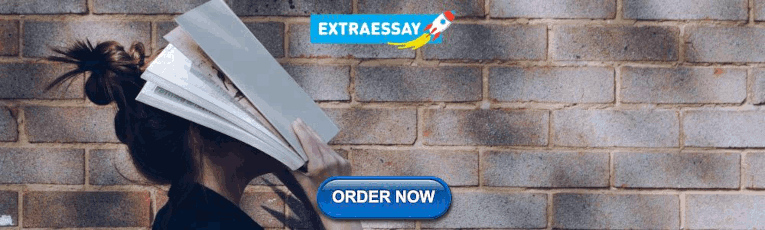
The Bottom Line
- Marketing Essentials
Queuing Theory Definition, Elements, and Example
:max_bytes(150000):strip_icc():format(webp)/Group1805-3b9f749674f0434184ef75020339bd35.jpg)
Investopedia / Ellen Lindner
Queuing theory is a branch of mathematics that studies how lines form, how they function, and why they malfunction. Queuing theory examines every component of waiting in line, including the arrival process and the number of customers among others, which might be people, data packets, cars, or anything else.
Real-life applications of queuing theory cover a wide range of businesses. Its findings may be used to provide faster customer service, increase traffic flow, improve order shipments from a warehouse, or design data networks and call centers .
Key Takeaways
- Queuing theory is the study of the movement of people, objects, or information through a line.
- Studying congestion and its causes in a process is used to help create more efficient and cost-effective services and systems.
- Often used as an operations management tool, queuing theory can address staffing, scheduling, and customer service shortfalls.
- Some queuing is acceptable in business. If there's never a queue, it's a sign of overcapacity.
- Queuing theory aims to achieve a balance that is efficient and affordable.
How Queuing Theory Works
Queuing theory aims to design balanced systems that serve customers quickly and efficiently but do not cost too much to be sustainable. As a branch of operations research, queuing theory can help inform business decisions on how to build more efficient and cost-effective workflow systems.
The origin of queuing theory can be traced to the early 1900s in a study of the Copenhagen telephone exchange by Agner Krarup Erlang, a Danish engineer, statistician, and mathematician. His work led to the Erlang theory of efficient networks and the field of telephone network analysis.
At its most basic level, queuing theory involves the analysis of arrivals at a facility, such as a bank or a fast-food restaurant , and an analysis of the processes currently in place to serve them. The end result is a set of conclusions that aim to identify any flaws in the system and suggest how they can be ameliorated. The fundamental unit of telecommunications traffic in voice systems is called the Erlang.
Queuing theory as an operations management technique is commonly used to determine and streamline staffing needs, scheduling, and inventory in order to improve overall customer service. It is often used by Six Sigma practitioners to improve processes.
Queues are not necessarily a negative aspect of a business, as their absence suggests overcapacity.
In queuing theory, the process being studied is broken down into six distinct parameters. These include the following:
Queues can occur whenever resources are limited. Some queuing is tolerable in any business since a total absence of a queue would suggest a costly overcapacity .
The psychology of queuing is related to queuing theory. This is the component of queuing that deals with the natural irritation felt by many people who are forced to queue for service, whether they're waiting to check out at the supermarket or waiting for a website to load.
A call-back option while waiting to speak to a customer representative by phone is one example of a solution to customer impatience. A more old-fashioned example is the system used by many delis, which issues customer service numbers to allow people to track their progress to the front of the queue.
Supositorio.com offers free online queuing theory calculators with a choice of queuing models.
Applications of Queuing Theory
The queuing theory can be used and applied in a number of different situations. For instance, it can be applied to:
- Business logistics
- Banking and finance
- Telecommunications
- Project management
- Emergency services, such as fire, police, and ambulance
How it works and how involved it may be depends on the complexity of the case involved.
Example of Queuing Theory
A paper by Stanford Graduate School of Business Professor Lawrence Wein et al. used queuing theory to analyze a variety of possible emergency responses to an airborne bioterrorism attack in a public place. The model pointed to specific actions that could be taken to reduce the wait time for emergency care, thus decreasing the potential number of deaths.
Queuing theory is useful, if not quite so urgent, in guiding the logistics of many businesses. The operations department for a delivery company, for example, is likely to use queuing theory to help it smooth out the kinks in its systems for moving packages from a warehouse to a customer. In this case, the line being studied is comprised of boxes of goods waiting to be delivered to customers.
By applying queuing theory, a business can develop more efficient systems, processes, pricing mechanisms, staffing solutions, and arrival management strategies to reduce customer wait times and increase the number of customers that can be served.
How Do You Use Queuing Theory?
Queuing theory is used to identify and correct points of congestion in a process. The queue may consist of people, things, or information. In any case, they are being forced to wait for service. That is inefficient, bad for business, and annoying (when the queue consists of people). Queuing theory is used to analyze the existing process and map out alternatives with a better result.
Who Invented Queuing Theory?
Agner Krarup Erlang, a Danish mathematician, statistician, and engineer, is credited with creating not only queuing theory but the entire field of telephone traffic engineering.
In the early 20th century, Erlang was head of a technical laboratory at the Copenhagen Telephone Co. His extensive studies of wait time in automated telephone services and his proposals for more efficient networks were widely adopted by telephone companies.
What Are the Basic Elements of Queuing Theory?
A study of a line using queuing theory would break it down into six elements: the arrival process, the queue or service capacity, the number of servers available, the size of the client population, the queuing discipline (such as first-in, first-out), and the departure process. Creating a model of the entire process from start to finish allows the cause or causes of congestion to be identified and addressed.
Queuing theory is a mathematic discipline that looks at lines—specifically, how they form, how they work, and why they sometimes don't work. Queuing is an unavoidable facet of doing business, with customers apt to contend with physical or digital lines, depending on what they are trying to purchase. Queuing has an impact on manufacturing, inventory, shipping, and distribution. As a result, queuing impacts profits and revenues. Findings from queuing theory can be used to improve customer service, traffic flow, and order shipments, among other aspects of running a business.
A.K. Erlang via McGill Faculty of Medicine and Sciences. “ The Theory of Probabilities and Telephone Conversations .” Nyt Tidsskrift for Matematik B , vol 20, 1909, p.33.
Wein Lawerence M., David Craft, and Edward Kaplan via Stanford Business School of Graduate " Emergency Response to Anthrax Attack ," National Academy of Sciences of the United States of America, vol. 100, issue 7, April 2003, Pages 4346–4351.
:max_bytes(150000):strip_icc():format(webp)/close-up-focus-on-call-center-headset-device--headset-voip-system-with-telephone-answer-machine-technology-at-operation-office-desk-for-hotline-telemarketing-and-network-operator-concept-1016109338-77f05aface5e4aa6b4e387e17de9d412.jpg)
- Terms of Service
- Editorial Policy
- Privacy Policy
- Your Privacy Choices
Choose a language:
Queuing theory: definition, history & real-life applications & examples.
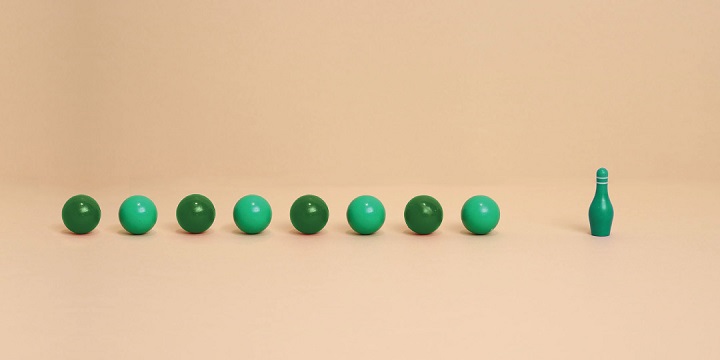
Queuing theory is a powerful tool to analyze the daily phenomenon of waiting in line. Discover how to define queuing theory, how it started, why it’s important, and how it can be applied to real-life situations.
1. What is queuing theory? 2. How did queuing theory start? 3. What are the different types of queuing systems? 4. Why is queuing theory important? 5. The application of queuing theory & queuing theory examples 6. How does queue psychology impact queuing?
1. What is queuing theory?
Queuing theory refers to the mathematical study of the formation, function, and congestion of waiting lines, or queues. It’s also referred to as queueing theory, queue theory, and waiting line theory.
At its core, a queuing situation involves two parts:
- Someone or something that requests a service—usually referred to as the customer, job, or request.
- Someone or something that completes or delivers the services—usually referred to as the server.
To illustrate, let’s take two examples. First, when looking at the queuing situation at a bank, the customers are people seeking to deposit or withdraw money, and the servers are the bank tellers.
Second, when looking at the queuing situation of a printer, the customers are the requests that have been sent to the printer, and the server is the printer.
Queuing theory scrutinizes the entire system of waiting in line, including elements like the customer arrival rate, number of servers, number of customers, capacity of the waiting area, average service completion time, and queuing discipline.
Queuing discipline refers to the rules of the queue, for example whether it behaves based on a principle of first-in-first-out , last-in-first-out, prioritized, or serve-in-random-order.
2. How did queuing theory start?
Queuing theory was first introduced in the early 20 th century by Danish mathematician and engineer Agner Krarup Erlang .
Erlang worked for the Copenhagen Telephone Exchange and wanted to analyze and optimize its operations.
He sought to determine how many circuits were needed to provide an acceptable level of telephone service, for people not to be “on hold” (or in a telephone queue) for too long. He was also curious to find out how many telephone operators were needed to process a given volume of calls.
His mathematical analysis culminated in his 1920 paper “Telephone Waiting Times”, which contained some of the first queuing models and served as the foundation of applied queuing theory.
The international unit of telephone traffic is called the Erlang in his honor.
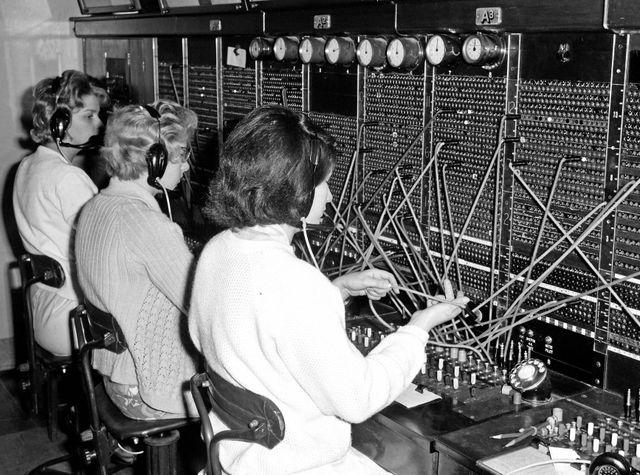
Image via Reddit
3. What are the different types of queuing systems?
Queuing theory uses the Kendall notation to classify the different types of queuing systems, or nodes. Queuing nodes are classified using the notation A/S/c/K/N/D where:
- A is the arrival process
- S is the mathematical distribution of the service time
- c is the number of servers
- K is the capacity of the queue, omitted if unlimited
- N is the number of possible customers, omitted if unlimited
- D is the queuing discipline, assumed first-in-first-out if omitted
For example, think of an ATM. It can serve:
- One customer at a time
- In a first-in-first-out order
- With a randomly-distributed arrival process and service distribution time
- Unlimited queue capacity
- Unlimited number of possible customers.
Queuing theory would describe this system as a M/M/1 queuing model (“M” here stands for Markovian , a statistical process to describe randomness).
Queuing theory calculators out there often require choosing a queuing model from the Kendall notation before calculating inputs.
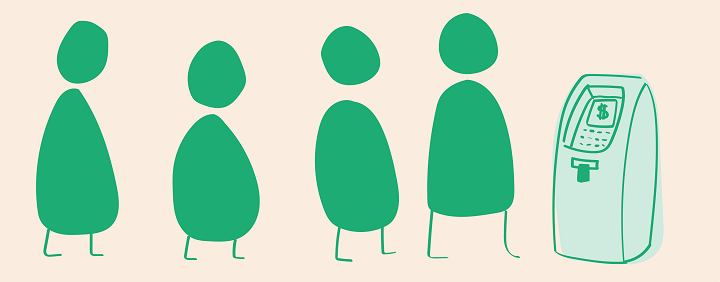
4. Why is queuing theory important?
Waiting in line is a part of everyday life because as a process it has several important functions. Queues are a fair and essential way of dealing with the flow of customers when there are limited resources. Negative outcomes arise if a queue process isn’t established to deal with overcapacity.
For example, when too many visitors navigate to a website, the website will slow and crash if it doesn’t have a way to change the speed at which it processes requests or a way to queue visitors .
Related: What is an Online Queue System?
Or, imagine planes waiting for a runway to land. When there is an excess of planes, the absence of a queue would have real safety implications as planes all tried to land at the same time.
The importance of queuing theory comes from the fact that it helps describe features of queues, like average wait time, and provides the tools for optimizing queues. From a business sense, queuing theory in operation research informs the construction of efficient and cost-effective workflow systems.
5. The application of queuing theory
Queuing theory is powerful because the ubiquity of queue situations means it has countless and diverse applications.
Queuing theory has been applied, just to name a few, to:
- Telecommunications
- Transportation
- Emergency services
- Industrial engineering
- Project management
- Operation research
Before we look at some specific applications, it’s helpful to understand Little’s Law, a formula that helps to operationalize queuing theory in many of these applications.
Do you know the 6 simple rules of queue psychology?
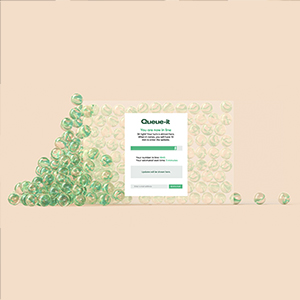
Little’s Law
Little’s Law connects the capacity of a queuing system, the average time spent in the system, and the average arrival rate into the system without knowing any other features of the queue. The formula is quite simple and is written as follows:
or transformed to solve for the other two variables so that:
- L is the average number of customers in the system
- λ (lambda) is the average arrival rate into the system
- W is the average amount of time spent in the system
Project management processes like Lean and Kanban wouldn’t exist without the Little’s Law queuing models. They’re critical for business applications, in which Little’s Law can be written in plain English as:
Queuing theory examples & types of queuing models
Little’s Law gives powerful insights because it lets us solve for important variables like the average wait of in a queue or the number of customers in queue simply based on two other inputs.
A line at a cafe
For example, if you’re waiting in line at a Starbucks, Little’s Law can estimate how long it would take to get your coffee.
Assume there are 15 people in line, one server, and 2 people are served per minute. To estimate this, you’d use Little’s Law in the form:
Showing that you could expect to wait 7.5 minutes for your coffee.
Image by John Yorke
A line in a virtual waiting room
At Queue-it, we show visitors their wait time in the online queue using a queuing model based on Little’s Law, adding in factors to account for no-shows and re-entries:
- L is the number of users ahead in line
- λ (lambda) is rate of redirects to the website or app, in users per minute
- N is the no-show ratio
- R is the re-entry rate to the queue
- W is the estimated wait time, in minutes
Military process optimization
We can look at a process optimization example from the military, courtesy of Process.st .
In this real-life example , the military needed to determine the ideal amount of time B-2 stealth bombers would be in maintenance. There are only 20 B-2 aircraft and they need to be ready at a moment’s notice. But they require frequent maintenance, which can range anywhere from 18 to 45 days.
Using Little’s Law helps find the balance of aircraft in use versus aircraft under maintenance.
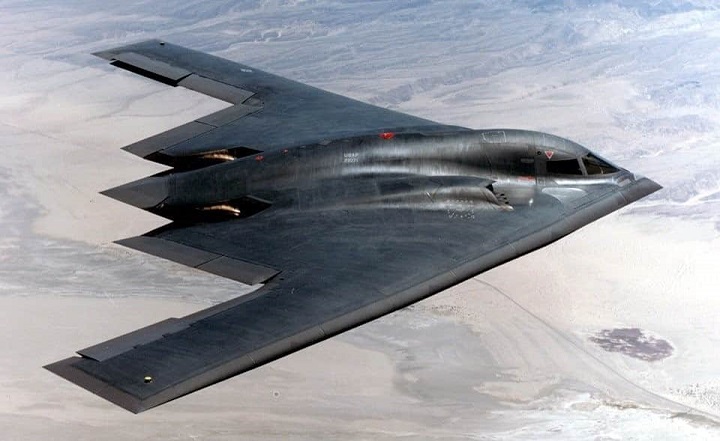
Image by skeeze from Pixabay
Based on flight schedule analysis, it was calculated that three B-2 bombers would be under maintenance at any given time. The rate at which bombers entered maintenance was also calculated to be roughly every 7 days. So:
- L = number of items in WIP (maintenance) = 3
- A = arrival/departure rate = 1 every 7 days = 1/7 days
- W = the average amount of time spent in maintenance = ???
Put into Little’s Law, this leaves us with:
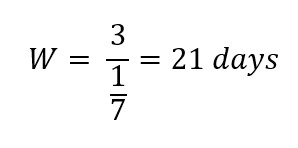
Therefore, the target lead time for B-2 bomber maintenance needed to be 21 days to meet the demands of both available aircraft and the regular flight schedules.
6. How does queue psychology impact queuing?
Queuing theory is helpful in explaining the math behind how queues run. But when queues involve humans, queue psychology is important to understand the queue experience as well.
Queue psychology research shows it’s not the length of the wait that determines how positive or negative the queue experience is, but rather how people feel while waiting.
Related: The Psychology of Queuing Revealed in 6 Simple Rules
For example, unoccupied time feels longer than occupied time. Distractions or the ability to do something else while in line makes time feel like it goes by faster. And uncertain waits feel longer than known, finite waits.
That’s why the callback option on customer service lines is so popular. You can feel the anxiety go down when you get the option to be called back in 10 minutes, freeing you to do something else instead of listening to that terrible muzak for an unknown amount of time.
For queuing situations involving people—like websites that use an online queuing system —the psychological rules governing the queues are just as important as the mathematical ones.
(This blog has been updated since it was written in 2020).

- Free Case Studies
- Business Essays
Write My Case Study
Buy Case Study
Case Study Help
- Case Study For Sale
- Case Study Service
- Hire Writer
Case Study on Queuing Theory
Queuing theory case study:.
Queuing theory is the branch of probability theory, which is aimed to research the rational choice of the structure and system of service on the basis of the streams of demands for service, the type and the length of a queue.
In queuing theory the methods of probability theory and mathematical statistics are applied. The first attempts to research the problems of queues and their management were carried out in Denmark in the beginning of the 20th century.Queuing theory appeared during the research of the telecommunication systems and the manner of their work. The telephone operators connected the people who tried to ring someone up one by one.People had to wait in a queue in order to make a call. Of course, the queues were different and on the basis of the types of the queues various systems of management appeared.
We Will Write a Custom Case Study Specifically For You For Only $13.90/page!
Later on queuing theory was applied in other spheres of the human life: business, office, store, plant, factory, cafe, hospital, etc.Queuing theory is connected not just with probability theory and mathematics but psychology and social studies, because staying in a queue is a serious psychological discomfort and disappointment for everyone. People get angry, irritated and face stress and the further activity of an individual is quite personal and unpredictable. For example, if one sees a queue, he can just leave it and the firm loses a client, so its task is to make the process of staying in the queue favourable and less irritable.Various techniques and methods are used in this case, for example, offices and hospitals have TV sets which catch clients’ attention; stores have the special shelves of products which attract consumer’s attention while he waits in a queue.Queuing theory is an interesting topic for the research, because it touches upon every sphere of the human life, as every day people stay in a queue and even do not imagine that the queue is managed in the certain professional way.
The student is able to research the general information about queuing theory and that focus on the definite case which dwells on the direct application of the methodology of the theory. The student is asked to analyze the cause and effect of the problem which has occurred in the case and try to solve the problem using the knowledge on queuing theory and its major aspects.When a case study becomes a serious challenge for students, they can use the pluses of the web and take advantage of a free example case study on queuing theory. Experienced writers share their knowledge and skills in order to provide students with a good free sample case study on queuing theory and teach them to analyze the topic well and format the paper professionally.
Related posts:
- Case Study on Classical Management Theory
- Case Study on Game Theory
- Case Study on Piaget’s Theory
- Case study Theory Y
- Hence ELM theory case study
- Counseling Theory: Case Study
- Biological Theory Case Study
Quick Links
Privacy Policy
Terms and Conditions
Testimonials
Our Services
Case Study Writing Service
Case Studies For Sale
Our Company
Welcome to the world of case studies that can bring you high grades! Here, at ACaseStudy.com, we deliver professionally written papers, and the best grades for you from your professors are guaranteed!
[email protected] 804-506-0782 350 5th Ave, New York, NY 10118, USA
Acasestudy.com © 2007-2019 All rights reserved.

Hi! I'm Anna
Would you like to get a custom case study? How about receiving a customized one?
Haven't Found The Case Study You Want?
For Only $13.90/page
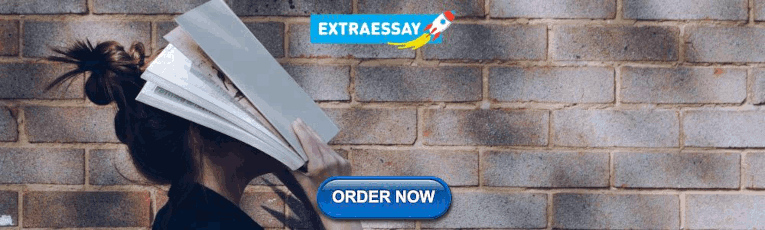
COMMENTS
Studies such as Molla, (2017); Wang (2017) apply queuing theory to analyze the mean number of customers served at one time, the mean waiting time in the queue, the average time in the system, the ...
In order to quantify waiting times and clarify the queue's related problems, queuing theory has been widely used. Queuing theory is defined as being the body of knowledge about waiting lines (Prabhu, 1997) and a queuing system may be defined as a system in which customers' arrival is being placed upon a finite capacity on the resource side ...
This paper aims to show that queuing theory satisfies the model when tested with a real-case scenario. We obtained the data from a restaurant in Jakarta. We then derive the arrival rate, service rate, utilization rate, waiting time in queue and the probability of potential customers to balk based on the data using Little's Theorem and M/M/1 ...
In line with these, the following are the objectives of the study: (a) to obtain the average standard time of queuing time and service time for one (1) banking day during the quarantine implementation in the Philippines; (b) to determine and apply Queueing Theory on gathered data, and (c) to develop and propose methods to improve the current ...
Queueing theory, broadly speaking, concerns the design and analysis of resource-constrained systems in which customers need to potentially wait for access to a resource. This paradigm arises in many applied settings, ranging from service and health operations to communications and computer systems to ride-sharing and job-matching platforms to ...
beginning of the study of queuing theory. In this section, we will discuss two common concepts in queuing theory. A. Little's Theorem Little's theorem [7] describes the relationship between throughput rate (i.e. arrival and service rate), cycle time and work in process (i.e. number of customers/jobs in the system).
This study focuses on the queuing system to improve the service of vaccination in Quezon City that had been observed within a parameter of arrival time, waiting time in queue and time of service of the vaccine. The researchers gathered data through direct time study. Using a watch and timer, the data was collected from each procedure.
queuing theory, queuing models are used to approximate a real queuing situation or system so that the queuing behaviour can be analysed mathematically. It has been used successfully in the studies of queue behaviour problems, optimization problems and the statistical inference of queuing system (Xiao and Zhang, 2009).
Abstract: This paper presents an analysis of a study that uses queueing theory to investigate the rate at which customers arrive and receive service in a supermarket over the course of one week during predetermined time intervals. The data collected over a one-week period includes the number of customers arriving at specific intervals, along with their corresponding service and arrival rates.
The study of bed management from a queueing theory perspective is still in relatively early stages of development, suggesting that there is a great deal of room for researchers to improve and optimize bed management procedures. The first studies in queueing theory that began to address bed management were published in the 1980s and early 1990s.
Queuing theory is the study of queue or waiting lines. Some of the analysis that can be derived using queuing theory includes the expected queue length, the probability of the system to be in certain states, such as empty or full [1]. 2. Queuing Theory Where is the average customers arrival rate and T is the average service time for a customer.
Case study: queuing problem. In this section, we study all districts of Tehran, Iran. Data is collected from Tehran Emergency Medical Services Center. 3.3.1. Current system. First, someone calls the emergency medical center. One operator quickly figures out the nature and urgency of the call and assesses whether the emergency requires an ambulance.
Queuing Theory: A mathematical method of analyzing the congestions and delays of waiting in line. Queuing theory examines every component of waiting in line to be served, including the arrival ...
Queuing theory uses the Kendall notation to classify the different types of queuing systems, or nodes. Queuing nodes are classified using the notation A/S/c/K/N/D where:. A is the arrival process; S is the mathematical distribution of the service time; c is the number of servers; K is the capacity of the queue, omitted if unlimited; N is the number of possible customers, omitted if unlimited
Researchers have previously used queuing theory to model the restaurant operation[1], reduce cycle time in a busy fast food restaurant[2,3], as well as to increase throughput and efficiency[4,1]. The study of waiting line, called queuing theory, is one of the oldest and most widely used quantitative analysis techniques.
This paper discusses the use of Queuing Network Theory to study the effect of batch size and throughput in optimizing resource utilization, particularly machine resources in a manufacturing system. A factory in the manufacturing industry performing assembly operations in its production lines was the focus of this study. ... In the case where ...
by the truck users (this was achieved using a combination of time study and work sampling techniques), (b) the unit queueing cost per truck user (this proved to be the most laborious, time consuming and tricky part of the whole study), and (c) the average demand rate for truck services. Table 1. Evaluation of the actual average daily queueing cost.
1. [PDF] This paper presents an analysis of a study that uses queueing theory to investigate the rate at which customers arrive and receive service in a supermarket over the course of one week during predetermined time intervals. The data collected over a one-week period includes the number of customers arriving at specific intervals, along ...
Conclusions. In the case study described above, queueing theory was found to be a valuable tool for describing and evaluating a global network of information processing sys tems and communications links. A first-order, predesign, "table-top" evaluation was required and queueing theory fitted the bill.
Queuing Theory Case Study: Queuing theory is the branch of probability theory, which is aimed to research the rational choice of the structure and system of service on the basis of the streams of demands for service, the type and the length of a queue. In queuing theory the methods of probability theory and mathematical statistics are applied ...
This case Bamboo House India: A Case of Queuing Theory, Operations Research (OR) is seen as a discipline of applied sciences where analytical methods are used to resolve complex situations for better decision making. There are many OR techniques such as Project Management, Game Theory, Logistics, Social Networking/Transportation Forecasting models, Queuing Theory, etc. Queuing Theory (study of ...