Cyber risk and cybersecurity: a systematic review of data availability
- Open access
- Published: 17 February 2022
- Volume 47 , pages 698–736, ( 2022 )
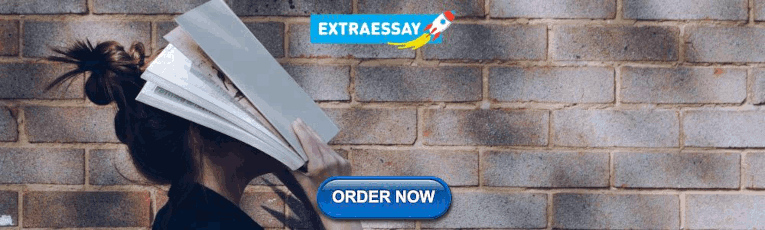
Cite this article
You have full access to this open access article
- Frank Cremer 1 ,
- Barry Sheehan ORCID: orcid.org/0000-0003-4592-7558 1 ,
- Michael Fortmann 2 ,
- Arash N. Kia 1 ,
- Martin Mullins 1 ,
- Finbarr Murphy 1 &
- Stefan Materne 2
61k Accesses
59 Citations
42 Altmetric
Explore all metrics
Cybercrime is estimated to have cost the global economy just under USD 1 trillion in 2020, indicating an increase of more than 50% since 2018. With the average cyber insurance claim rising from USD 145,000 in 2019 to USD 359,000 in 2020, there is a growing necessity for better cyber information sources, standardised databases, mandatory reporting and public awareness. This research analyses the extant academic and industry literature on cybersecurity and cyber risk management with a particular focus on data availability. From a preliminary search resulting in 5219 cyber peer-reviewed studies, the application of the systematic methodology resulted in 79 unique datasets. We posit that the lack of available data on cyber risk poses a serious problem for stakeholders seeking to tackle this issue. In particular, we identify a lacuna in open databases that undermine collective endeavours to better manage this set of risks. The resulting data evaluation and categorisation will support cybersecurity researchers and the insurance industry in their efforts to comprehend, metricise and manage cyber risks.
Similar content being viewed by others
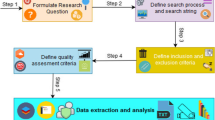
Cyber Security Threats and Vulnerabilities: A Systematic Mapping Study
Mamoona Humayun, Mahmood Niazi, … Sajjad Mahmood
Artificial Intelligence and Fraud Detection
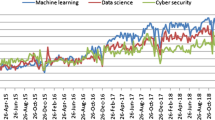
Cybersecurity data science: an overview from machine learning perspective
Iqbal H. Sarker, A. S. M. Kayes, … Alex Ng
Avoid common mistakes on your manuscript.
Introduction
Globalisation, digitalisation and smart technologies have escalated the propensity and severity of cybercrime. Whilst it is an emerging field of research and industry, the importance of robust cybersecurity defence systems has been highlighted at the corporate, national and supranational levels. The impacts of inadequate cybersecurity are estimated to have cost the global economy USD 945 billion in 2020 (Maleks Smith et al. 2020 ). Cyber vulnerabilities pose significant corporate risks, including business interruption, breach of privacy and financial losses (Sheehan et al. 2019 ). Despite the increasing relevance for the international economy, the availability of data on cyber risks remains limited. The reasons for this are many. Firstly, it is an emerging and evolving risk; therefore, historical data sources are limited (Biener et al. 2015 ). It could also be due to the fact that, in general, institutions that have been hacked do not publish the incidents (Eling and Schnell 2016 ). The lack of data poses challenges for many areas, such as research, risk management and cybersecurity (Falco et al. 2019 ). The importance of this topic is demonstrated by the announcement of the European Council in April 2021 that a centre of excellence for cybersecurity will be established to pool investments in research, technology and industrial development. The goal of this centre is to increase the security of the internet and other critical network and information systems (European Council 2021 ).
This research takes a risk management perspective, focusing on cyber risk and considering the role of cybersecurity and cyber insurance in risk mitigation and risk transfer. The study reviews the existing literature and open data sources related to cybersecurity and cyber risk. This is the first systematic review of data availability in the general context of cyber risk and cybersecurity. By identifying and critically analysing the available datasets, this paper supports the research community by aggregating, summarising and categorising all available open datasets. In addition, further information on datasets is attached to provide deeper insights and support stakeholders engaged in cyber risk control and cybersecurity. Finally, this research paper highlights the need for open access to cyber-specific data, without price or permission barriers.
The identified open data can support cyber insurers in their efforts on sustainable product development. To date, traditional risk assessment methods have been untenable for insurance companies due to the absence of historical claims data (Sheehan et al. 2021 ). These high levels of uncertainty mean that cyber insurers are more inclined to overprice cyber risk cover (Kshetri 2018 ). Combining external data with insurance portfolio data therefore seems to be essential to improve the evaluation of the risk and thus lead to risk-adjusted pricing (Bessy-Roland et al. 2021 ). This argument is also supported by the fact that some re/insurers reported that they are working to improve their cyber pricing models (e.g. by creating or purchasing databases from external providers) (EIOPA 2018 ). Figure 1 provides an overview of pricing tools and factors considered in the estimation of cyber insurance based on the findings of EIOPA ( 2018 ) and the research of Romanosky et al. ( 2019 ). The term cyber risk refers to all cyber risks and their potential impact.
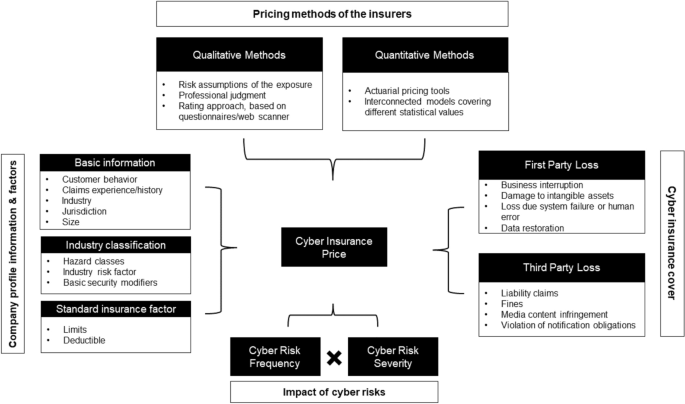
An overview of the current cyber insurance informational and methodological landscape, adapted from EIOPA ( 2018 ) and Romanosky et al. ( 2019 )
Besides the advantage of risk-adjusted pricing, the availability of open datasets helps companies benchmark their internal cyber posture and cybersecurity measures. The research can also help to improve risk awareness and corporate behaviour. Many companies still underestimate their cyber risk (Leong and Chen 2020 ). For policymakers, this research offers starting points for a comprehensive recording of cyber risks. Although in many countries, companies are obliged to report data breaches to the respective supervisory authority, this information is usually not accessible to the research community. Furthermore, the economic impact of these breaches is usually unclear.
As well as the cyber risk management community, this research also supports cybersecurity stakeholders. Researchers are provided with an up-to-date, peer-reviewed literature of available datasets showing where these datasets have been used. For example, this includes datasets that have been used to evaluate the effectiveness of countermeasures in simulated cyberattacks or to test intrusion detection systems. This reduces a time-consuming search for suitable datasets and ensures a comprehensive review of those available. Through the dataset descriptions, researchers and industry stakeholders can compare and select the most suitable datasets for their purposes. In addition, it is possible to combine the datasets from one source in the context of cybersecurity or cyber risk. This supports efficient and timely progress in cyber risk research and is beneficial given the dynamic nature of cyber risks.
Cyber risks are defined as “operational risks to information and technology assets that have consequences affecting the confidentiality, availability, and/or integrity of information or information systems” (Cebula et al. 2014 ). Prominent cyber risk events include data breaches and cyberattacks (Agrafiotis et al. 2018 ). The increasing exposure and potential impact of cyber risk have been highlighted in recent industry reports (e.g. Allianz 2021 ; World Economic Forum 2020 ). Cyberattacks on critical infrastructures are ranked 5th in the World Economic Forum's Global Risk Report. Ransomware, malware and distributed denial-of-service (DDoS) are examples of the evolving modes of a cyberattack. One example is the ransomware attack on the Colonial Pipeline, which shut down the 5500 mile pipeline system that delivers 2.5 million barrels of fuel per day and critical liquid fuel infrastructure from oil refineries to states along the U.S. East Coast (Brower and McCormick 2021 ). These and other cyber incidents have led the U.S. to strengthen its cybersecurity and introduce, among other things, a public body to analyse major cyber incidents and make recommendations to prevent a recurrence (Murphey 2021a ). Another example of the scope of cyberattacks is the ransomware NotPetya in 2017. The damage amounted to USD 10 billion, as the ransomware exploited a vulnerability in the windows system, allowing it to spread independently worldwide in the network (GAO 2021 ). In the same year, the ransomware WannaCry was launched by cybercriminals. The cyberattack on Windows software took user data hostage in exchange for Bitcoin cryptocurrency (Smart 2018 ). The victims included the National Health Service in Great Britain. As a result, ambulances were redirected to other hospitals because of information technology (IT) systems failing, leaving people in need of urgent assistance waiting. It has been estimated that 19,000 cancelled treatment appointments resulted from losses of GBP 92 million (Field 2018 ). Throughout the COVID-19 pandemic, ransomware attacks increased significantly, as working from home arrangements increased vulnerability (Murphey 2021b ).
Besides cyberattacks, data breaches can also cause high costs. Under the General Data Protection Regulation (GDPR), companies are obliged to protect personal data and safeguard the data protection rights of all individuals in the EU area. The GDPR allows data protection authorities in each country to impose sanctions and fines on organisations they find in breach. “For data breaches, the maximum fine can be €20 million or 4% of global turnover, whichever is higher” (GDPR.EU 2021 ). Data breaches often involve a large amount of sensitive data that has been accessed, unauthorised, by external parties, and are therefore considered important for information security due to their far-reaching impact (Goode et al. 2017 ). A data breach is defined as a “security incident in which sensitive, protected, or confidential data are copied, transmitted, viewed, stolen, or used by an unauthorized individual” (Freeha et al. 2021 ). Depending on the amount of data, the extent of the damage caused by a data breach can be significant, with the average cost being USD 392 million Footnote 1 (IBM Security 2020 ).
This research paper reviews the existing literature and open data sources related to cybersecurity and cyber risk, focusing on the datasets used to improve academic understanding and advance the current state-of-the-art in cybersecurity. Furthermore, important information about the available datasets is presented (e.g. use cases), and a plea is made for open data and the standardisation of cyber risk data for academic comparability and replication. The remainder of the paper is structured as follows. The next section describes the related work regarding cybersecurity and cyber risks. The third section outlines the review method used in this work and the process. The fourth section details the results of the identified literature. Further discussion is presented in the penultimate section and the final section concludes.
Related work
Due to the significance of cyber risks, several literature reviews have been conducted in this field. Eling ( 2020 ) reviewed the existing academic literature on the topic of cyber risk and cyber insurance from an economic perspective. A total of 217 papers with the term ‘cyber risk’ were identified and classified in different categories. As a result, open research questions are identified, showing that research on cyber risks is still in its infancy because of their dynamic and emerging nature. Furthermore, the author highlights that particular focus should be placed on the exchange of information between public and private actors. An improved information flow could help to measure the risk more accurately and thus make cyber risks more insurable and help risk managers to determine the right level of cyber risk for their company. In the context of cyber insurance data, Romanosky et al. ( 2019 ) analysed the underwriting process for cyber insurance and revealed how cyber insurers understand and assess cyber risks. For this research, they examined 235 American cyber insurance policies that were publicly available and looked at three components (coverage, application questionnaires and pricing). The authors state in their findings that many of the insurers used very simple, flat-rate pricing (based on a single calculation of expected loss), while others used more parameters such as the asset value of the company (or company revenue) or standard insurance metrics (e.g. deductible, limits), and the industry in the calculation. This is in keeping with Eling ( 2020 ), who states that an increased amount of data could help to make cyber risk more accurately measured and thus more insurable. Similar research on cyber insurance and data was conducted by Nurse et al. ( 2020 ). The authors examined cyber insurance practitioners' perceptions and the challenges they face in collecting and using data. In addition, gaps were identified during the research where further data is needed. The authors concluded that cyber insurance is still in its infancy, and there are still several unanswered questions (for example, cyber valuation, risk calculation and recovery). They also pointed out that a better understanding of data collection and use in cyber insurance would be invaluable for future research and practice. Bessy-Roland et al. ( 2021 ) come to a similar conclusion. They proposed a multivariate Hawkes framework to model and predict the frequency of cyberattacks. They used a public dataset with characteristics of data breaches affecting the U.S. industry. In the conclusion, the authors make the argument that an insurer has a better knowledge of cyber losses, but that it is based on a small dataset and therefore combination with external data sources seems essential to improve the assessment of cyber risks.
Several systematic reviews have been published in the area of cybersecurity (Kruse et al. 2017 ; Lee et al. 2020 ; Loukas et al. 2013 ; Ulven and Wangen 2021 ). In these papers, the authors concentrated on a specific area or sector in the context of cybersecurity. This paper adds to this extant literature by focusing on data availability and its importance to risk management and insurance stakeholders. With a priority on healthcare and cybersecurity, Kruse et al. ( 2017 ) conducted a systematic literature review. The authors identified 472 articles with the keywords ‘cybersecurity and healthcare’ or ‘ransomware’ in the databases Cumulative Index of Nursing and Allied Health Literature, PubMed and Proquest. Articles were eligible for this review if they satisfied three criteria: (1) they were published between 2006 and 2016, (2) the full-text version of the article was available, and (3) the publication is a peer-reviewed or scholarly journal. The authors found that technological development and federal policies (in the U.S.) are the main factors exposing the health sector to cyber risks. Loukas et al. ( 2013 ) conducted a review with a focus on cyber risks and cybersecurity in emergency management. The authors provided an overview of cyber risks in communication, sensor, information management and vehicle technologies used in emergency management and showed areas for which there is still no solution in the literature. Similarly, Ulven and Wangen ( 2021 ) reviewed the literature on cybersecurity risks in higher education institutions. For the literature review, the authors used the keywords ‘cyber’, ‘information threats’ or ‘vulnerability’ in connection with the terms ‘higher education, ‘university’ or ‘academia’. A similar literature review with a focus on Internet of Things (IoT) cybersecurity was conducted by Lee et al. ( 2020 ). The review revealed that qualitative approaches focus on high-level frameworks, and quantitative approaches to cybersecurity risk management focus on risk assessment and quantification of cyberattacks and impacts. In addition, the findings presented a four-step IoT cyber risk management framework that identifies, quantifies and prioritises cyber risks.
Datasets are an essential part of cybersecurity research, underlined by the following works. Ilhan Firat et al. ( 2021 ) examined various cybersecurity datasets in detail. The study was motivated by the fact that with the proliferation of the internet and smart technologies, the mode of cyberattacks is also evolving. However, in order to prevent such attacks, they must first be detected; the dissemination and further development of cybersecurity datasets is therefore critical. In their work, the authors observed studies of datasets used in intrusion detection systems. Khraisat et al. ( 2019 ) also identified a need for new datasets in the context of cybersecurity. The researchers presented a taxonomy of current intrusion detection systems, a comprehensive review of notable recent work, and an overview of the datasets commonly used for assessment purposes. In their conclusion, the authors noted that new datasets are needed because most machine-learning techniques are trained and evaluated on the knowledge of old datasets. These datasets do not contain new and comprehensive information and are partly derived from datasets from 1999. The authors noted that the core of this issue is the availability of new public datasets as well as their quality. The availability of data, how it is used, created and shared was also investigated by Zheng et al. ( 2018 ). The researchers analysed 965 cybersecurity research papers published between 2012 and 2016. They created a taxonomy of the types of data that are created and shared and then analysed the data collected via datasets. The researchers concluded that while datasets are recognised as valuable for cybersecurity research, the proportion of publicly available datasets is limited.
The main contributions of this review and what differentiates it from previous studies can be summarised as follows. First, as far as we can tell, it is the first work to summarise all available datasets on cyber risk and cybersecurity in the context of a systematic review and present them to the scientific community and cyber insurance and cybersecurity stakeholders. Second, we investigated, analysed, and made available the datasets to support efficient and timely progress in cyber risk research. And third, we enable comparability of datasets so that the appropriate dataset can be selected depending on the research area.
Methodology
Process and eligibility criteria.
The structure of this systematic review is inspired by the Preferred Reporting Items for Systematic Reviews and Meta-Analyses (PRISMA) framework (Page et al. 2021 ), and the search was conducted from 3 to 10 May 2021. Due to the continuous development of cyber risks and their countermeasures, only articles published in the last 10 years were considered. In addition, only articles published in peer-reviewed journals written in English were included. As a final criterion, only articles that make use of one or more cybersecurity or cyber risk datasets met the inclusion criteria. Specifically, these studies presented new or existing datasets, used them for methods, or used them to verify new results, as well as analysed them in an economic context and pointed out their effects. The criterion was fulfilled if it was clearly stated in the abstract that one or more datasets were used. A detailed explanation of this selection criterion can be found in the ‘Study selection’ section.
Information sources
In order to cover a complete spectrum of literature, various databases were queried to collect relevant literature on the topic of cybersecurity and cyber risks. Due to the spread of related articles across multiple databases, the literature search was limited to the following four databases for simplicity: IEEE Xplore, Scopus, SpringerLink and Web of Science. This is similar to other literature reviews addressing cyber risks or cybersecurity, including Sardi et al. ( 2021 ), Franke and Brynielsson ( 2014 ), Lagerström (2019), Eling and Schnell ( 2016 ) and Eling ( 2020 ). In this paper, all databases used in the aforementioned works were considered. However, only two studies also used all the databases listed. The IEEE Xplore database contains electrical engineering, computer science, and electronics work from over 200 journals and three million conference papers (IEEE 2021 ). Scopus includes 23,400 peer-reviewed journals from more than 5000 international publishers in the areas of science, engineering, medicine, social sciences and humanities (Scopus 2021 ). SpringerLink contains 3742 journals and indexes over 10 million scientific documents (SpringerLink 2021 ). Finally, Web of Science indexes over 9200 journals in different scientific disciplines (Science 2021 ).
A search string was created and applied to all databases. To make the search efficient and reproducible, the following search string with Boolean operator was used in all databases: cybersecurity OR cyber risk AND dataset OR database. To ensure uniformity of the search across all databases, some adjustments had to be made for the respective search engines. In Scopus, for example, the Advanced Search was used, and the field code ‘Title-ABS-KEY’ was integrated into the search string. For IEEE Xplore, the search was carried out with the Search String in the Command Search and ‘All Metadata’. In the Web of Science database, the Advanced Search was used. The special feature of this search was that it had to be carried out in individual steps. The first search was carried out with the terms cybersecurity OR cyber risk with the field tag Topic (T.S. =) and the second search with dataset OR database. Subsequently, these searches were combined, which then delivered the searched articles for review. For SpringerLink, the search string was used in the Advanced Search under the category ‘Find the resources with all of the words’. After conducting this search string, 5219 studies could be found. According to the eligibility criteria (period, language and only scientific journals), 1581 studies were identified in the databases:
Scopus: 135
Springer Link: 548
Web of Science: 534
An overview of the process is given in Fig. 2 . Combined with the results from the four databases, 854 articles without duplicates were identified.
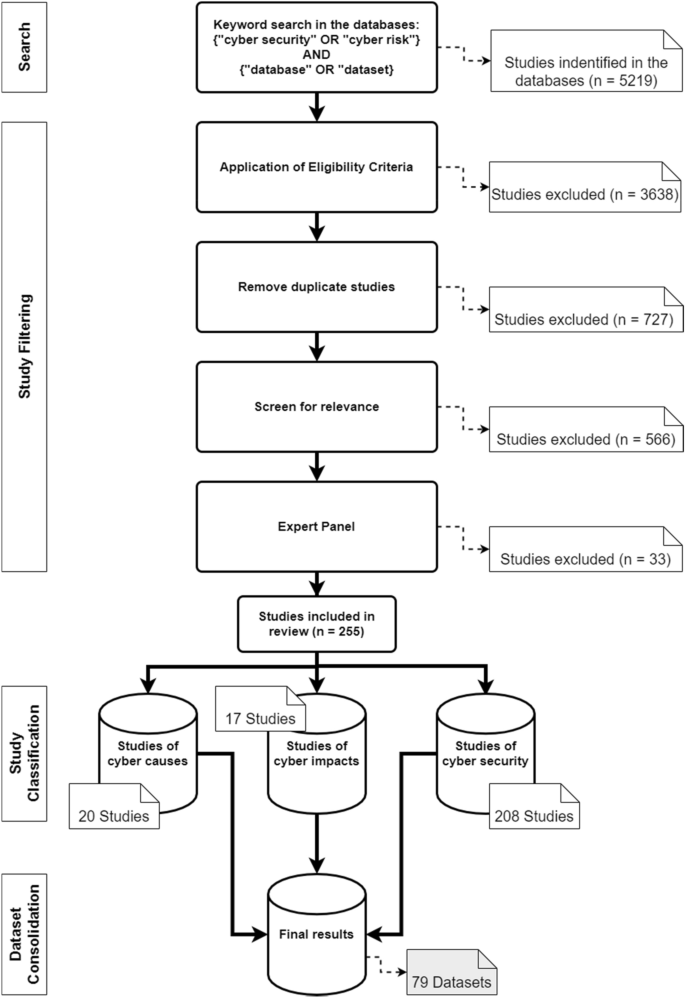
Literature search process and categorisation of the studies
Study selection
In the final step of the selection process, the articles were screened for relevance. Due to a large number of results, the abstracts were analysed in the first step of the process. The aim was to determine whether the article was relevant for the systematic review. An article fulfilled the criterion if it was recognisable in the abstract that it had made a contribution to datasets or databases with regard to cyber risks or cybersecurity. Specifically, the criterion was considered to be met if the abstract used datasets that address the causes or impacts of cyber risks, and measures in the area of cybersecurity. In this process, the number of articles was reduced to 288. The articles were then read in their entirety, and an expert panel of six people decided whether they should be used. This led to a final number of 255 articles. The years in which the articles were published and the exact number can be seen in Fig. 3 .
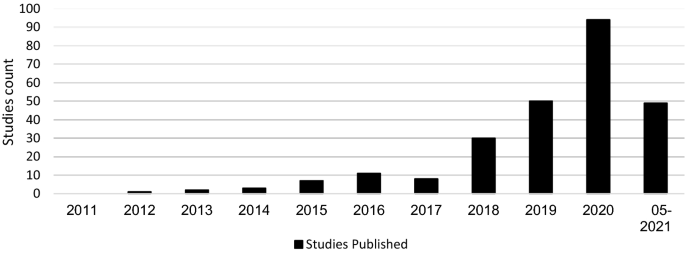
Distribution of studies
Data collection process and synthesis of the results
For the data collection process, various data were extracted from the studies, including the names of the respective creators, the name of the dataset or database and the corresponding reference. It was also determined where the data came from. In the context of accessibility, it was determined whether access is free, controlled, available for purchase or not available. It was also determined when the datasets were created and the time period referenced. The application type and domain characteristics of the datasets were identified.
This section analyses the results of the systematic literature review. The previously identified studies are divided into three categories: datasets on the causes of cyber risks, datasets on the effects of cyber risks and datasets on cybersecurity. The classification is based on the intended use of the studies. This system of classification makes it easier for stakeholders to find the appropriate datasets. The categories are evaluated individually. Although complete information is available for a large proportion of datasets, this is not true for all of them. Accordingly, the abbreviation N/A has been inserted in the respective characters to indicate that this information could not be determined by the time of submission. The term ‘use cases in the literature’ in the following and supplementary tables refers to the application areas in which the corresponding datasets were used in the literature. The areas listed there refer to the topic area on which the researchers conducted their research. Since some datasets were used interdisciplinarily, the listed use cases in the literature are correspondingly longer. Before discussing each category in the next sections, Fig. 4 provides an overview of the number of datasets found and their year of creation. Figure 5 then shows the relationship between studies and datasets in the period under consideration. Figure 6 shows the distribution of studies, their use of datasets and their creation date. The number of datasets used is higher than the number of studies because the studies often used several datasets (Table 1 ).
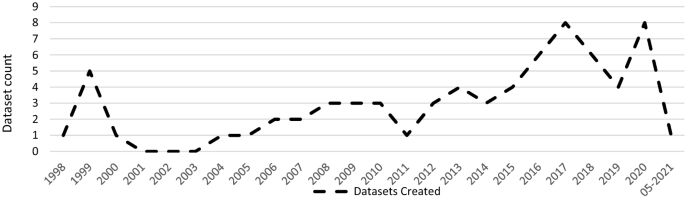
Distribution of dataset results
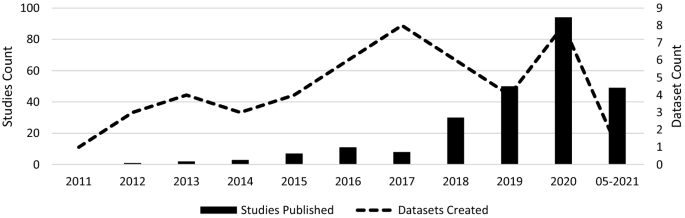
Correlation between the studies and the datasets
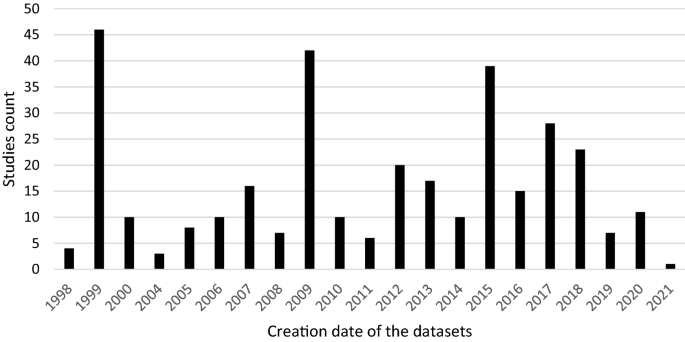
Distribution of studies and their use of datasets
Most of the datasets are generated in the U.S. (up to 58.2%). Canada and Australia rank next, with 11.3% and 5% of all the reviewed datasets, respectively.
Additionally, to create value for the datasets for the cyber insurance industry, an assessment of the applicability of each dataset has been provided for cyber insurers. This ‘Use Case Assessment’ includes the use of the data in the context of different analyses, calculation of cyber insurance premiums, and use of the information for the design of cyber insurance contracts or for additional customer services. To reasonably account for the transition of direct hyperlinks in the future, references were directed to the main websites for longevity (nearest resource point). In addition, the links to the main pages contain further information on the datasets and different versions related to the operating systems. The references were chosen in such a way that practitioners get the best overview of the respective datasets.
Case datasets
This section presents selected articles that use the datasets to analyse the causes of cyber risks. The datasets help identify emerging trends and allow pattern discovery in cyber risks. This information gives cybersecurity experts and cyber insurers the data to make better predictions and take appropriate action. For example, if certain vulnerabilities are not adequately protected, cyber insurers will demand a risk surcharge leading to an improvement in the risk-adjusted premium. Due to the capricious nature of cyber risks, existing data must be supplemented with new data sources (for example, new events, new methods or security vulnerabilities) to determine prevailing cyber exposure. The datasets of cyber risk causes could be combined with existing portfolio data from cyber insurers and integrated into existing pricing tools and factors to improve the valuation of cyber risks.
A portion of these datasets consists of several taxonomies and classifications of cyber risks. Aassal et al. ( 2020 ) propose a new taxonomy of phishing characteristics based on the interpretation and purpose of each characteristic. In comparison, Hindy et al. ( 2020 ) presented a taxonomy of network threats and the impact of current datasets on intrusion detection systems. A similar taxonomy was suggested by Kiwia et al. ( 2018 ). The authors presented a cyber kill chain-based taxonomy of banking Trojans features. The taxonomy built on a real-world dataset of 127 banking Trojans collected from December 2014 to January 2016 by a major U.K.-based financial organisation.
In the context of classification, Aamir et al. ( 2021 ) showed the benefits of machine learning for classifying port scans and DDoS attacks in a mixture of normal and attack traffic. Guo et al. ( 2020 ) presented a new method to improve malware classification based on entropy sequence features. The evaluation of this new method was conducted on different malware datasets.
To reconstruct attack scenarios and draw conclusions based on the evidence in the alert stream, Barzegar and Shajari ( 2018 ) use the DARPA2000 and MACCDC 2012 dataset for their research. Giudici and Raffinetti ( 2020 ) proposed a rank-based statistical model aimed at predicting the severity levels of cyber risk. The model used cyber risk data from the University of Milan. In contrast to the previous datasets, Skrjanc et al. ( 2018 ) used the older dataset KDD99 to monitor large-scale cyberattacks using a cauchy clustering method.
Amin et al. ( 2021 ) used a cyberattack dataset from the Canadian Institute for Cybersecurity to identify spatial clusters of countries with high rates of cyberattacks. In the context of cybercrime, Junger et al. ( 2020 ) examined crime scripts, key characteristics of the target company and the relationship between criminal effort and financial benefit. For their study, the authors analysed 300 cases of fraudulent activities against Dutch companies. With a similar focus on cybercrime, Mireles et al. ( 2019 ) proposed a metric framework to measure the effectiveness of the dynamic evolution of cyberattacks and defensive measures. To validate its usefulness, they used the DEFCON dataset.
Due to the rapidly changing nature of cyber risks, it is often impossible to obtain all information on them. Kim and Kim ( 2019 ) proposed an automated dataset generation system called CTIMiner that collects threat data from publicly available security reports and malware repositories. They released a dataset to the public containing about 640,000 records from 612 security reports published between January 2008 and 2019. A similar approach is proposed by Kim et al. ( 2020 ), using a named entity recognition system to extract core information from cyber threat reports automatically. They created a 498,000-tag dataset during their research (Ulven and Wangen 2021 ).
Within the framework of vulnerabilities and cybersecurity issues, Ulven and Wangen ( 2021 ) proposed an overview of mission-critical assets and everyday threat events, suggested a generic threat model, and summarised common cybersecurity vulnerabilities. With a focus on hospitality, Chen and Fiscus ( 2018 ) proposed several issues related to cybersecurity in this sector. They analysed 76 security incidents from the Privacy Rights Clearinghouse database. Supplementary Table 1 lists all findings that belong to the cyber causes dataset.
Impact datasets
This section outlines selected findings of the cyber impact dataset. For cyber insurers, these datasets can form an important basis for information, as they can be used to calculate cyber insurance premiums, evaluate specific cyber risks, formulate inclusions and exclusions in cyber wordings, and re-evaluate as well as supplement the data collected so far on cyber risks. For example, information on financial losses can help to better assess the loss potential of cyber risks. Furthermore, the datasets can provide insight into the frequency of occurrence of these cyber risks. The new datasets can be used to close any data gaps that were previously based on very approximate estimates or to find new results.
Eight studies addressed the costs of data breaches. For instance, Eling and Jung ( 2018 ) reviewed 3327 data breach events from 2005 to 2016 and identified an asymmetric dependence of monthly losses by breach type and industry. The authors used datasets from the Privacy Rights Clearinghouse for analysis. The Privacy Rights Clearinghouse datasets and the Breach level index database were also used by De Giovanni et al. ( 2020 ) to describe relationships between data breaches and bitcoin-related variables using the cointegration methodology. The data were obtained from the Department of Health and Human Services of healthcare facilities reporting data breaches and a national database of technical and organisational infrastructure information. Also in the context of data breaches, Algarni et al. ( 2021 ) developed a comprehensive, formal model that estimates the two components of security risks: breach cost and the likelihood of a data breach within 12 months. For their survey, the authors used two industrial reports from the Ponemon institute and VERIZON. To illustrate the scope of data breaches, Neto et al. ( 2021 ) identified 430 major data breach incidents among more than 10,000 incidents. The database created is available and covers the period 2018 to 2019.
With a direct focus on insurance, Biener et al. ( 2015 ) analysed 994 cyber loss cases from an operational risk database and investigated the insurability of cyber risks based on predefined criteria. For their study, they used data from the company SAS OpRisk Global Data. Similarly, Eling and Wirfs ( 2019 ) looked at a wide range of cyber risk events and actual cost data using the same database. They identified cyber losses and analysed them using methods from statistics and actuarial science. Using a similar reference, Farkas et al. ( 2021 ) proposed a method for analysing cyber claims based on regression trees to identify criteria for classifying and evaluating claims. Similar to Chen and Fiscus ( 2018 ), the dataset used was the Privacy Rights Clearinghouse database. Within the framework of reinsurance, Moro ( 2020 ) analysed cyber index-based information technology activity to see if index-parametric reinsurance coverage could suggest its cedant using data from a Symantec dataset.
Paté-Cornell et al. ( 2018 ) presented a general probabilistic risk analysis framework for cybersecurity in an organisation to be specified. The results are distributions of losses to cyberattacks, with and without considered countermeasures in support of risk management decisions based both on past data and anticipated incidents. The data used were from The Common Vulnerability and Exposures database and via confidential access to a database of cyberattacks on a large, U.S.-based organisation. A different conceptual framework for cyber risk classification and assessment was proposed by Sheehan et al. ( 2021 ). This framework showed the importance of proactive and reactive barriers in reducing companies’ exposure to cyber risk and quantifying the risk. Another approach to cyber risk assessment and mitigation was proposed by Mukhopadhyay et al. ( 2019 ). They estimated the probability of an attack using generalised linear models, predicted the security technology required to reduce the probability of cyberattacks, and used gamma and exponential distributions to best approximate the average loss data for each malicious attack. They also calculated the expected loss due to cyberattacks, calculated the net premium that would need to be charged by a cyber insurer, and suggested cyber insurance as a strategy to minimise losses. They used the CSI-FBI survey (1997–2010) to conduct their research.
In order to highlight the lack of data on cyber risks, Eling ( 2020 ) conducted a literature review in the areas of cyber risk and cyber insurance. Available information on the frequency, severity, and dependency structure of cyber risks was filtered out. In addition, open questions for future cyber risk research were set up. Another example of data collection on the impact of cyberattacks is provided by Sornette et al. ( 2013 ), who use a database of newspaper articles, press reports and other media to provide a predictive method to identify triggering events and potential accident scenarios and estimate their severity and frequency. A similar approach to data collection was used by Arcuri et al. ( 2020 ) to gather an original sample of global cyberattacks from newspaper reports sourced from the LexisNexis database. This collection is also used and applied to the fields of dynamic communication and cyber risk perception by Fang et al. ( 2021 ). To create a dataset of cyber incidents and disputes, Valeriano and Maness ( 2014 ) collected information on cyber interactions between rival states.
To assess trends and the scale of economic cybercrime, Levi ( 2017 ) examined datasets from different countries and their impact on crime policy. Pooser et al. ( 2018 ) investigated the trend in cyber risk identification from 2006 to 2015 and company characteristics related to cyber risk perception. The authors used a dataset of various reports from cyber insurers for their study. Walker-Roberts et al. ( 2020 ) investigated the spectrum of risk of a cybersecurity incident taking place in the cyber-physical-enabled world using the VERIS Community Database. The datasets of impacts identified are presented below. Due to overlap, some may also appear in the causes dataset (Supplementary Table 2).
Cybersecurity datasets
General intrusion detection.
General intrusion detection systems account for the largest share of countermeasure datasets. For companies or researchers focused on cybersecurity, the datasets can be used to test their own countermeasures or obtain information about potential vulnerabilities. For example, Al-Omari et al. ( 2021 ) proposed an intelligent intrusion detection model for predicting and detecting attacks in cyberspace, which was applied to dataset UNSW-NB 15. A similar approach was taken by Choras and Kozik ( 2015 ), who used machine learning to detect cyberattacks on web applications. To evaluate their method, they used the HTTP dataset CSIC 2010. For the identification of unknown attacks on web servers, Kamarudin et al. ( 2017 ) proposed an anomaly-based intrusion detection system using an ensemble classification approach. Ganeshan and Rodrigues ( 2020 ) showed an intrusion detection system approach, which clusters the database into several groups and detects the presence of intrusion in the clusters. In comparison, AlKadi et al. ( 2019 ) used a localisation-based model to discover abnormal patterns in network traffic. Hybrid models have been recommended by Bhattacharya et al. ( 2020 ) and Agrawal et al. ( 2019 ); the former is a machine-learning model based on principal component analysis for the classification of intrusion detection system datasets, while the latter is a hybrid ensemble intrusion detection system for anomaly detection using different datasets to detect patterns in network traffic that deviate from normal behaviour.
Agarwal et al. ( 2021 ) used three different machine learning algorithms in their research to find the most suitable for efficiently identifying patterns of suspicious network activity. The UNSW-NB15 dataset was used for this purpose. Kasongo and Sun ( 2020 ), Feed-Forward Deep Neural Network (FFDNN), Keshk et al. ( 2021 ), the privacy-preserving anomaly detection framework, and others also use the UNSW-NB 15 dataset as part of intrusion detection systems. The same dataset and others were used by Binbusayyis and Vaiyapuri ( 2019 ) to identify and compare key features for cyber intrusion detection. Atefinia and Ahmadi ( 2021 ) proposed a deep neural network model to reduce the false positive rate of an anomaly-based intrusion detection system. Fossaceca et al. ( 2015 ) focused in their research on the development of a framework that combined the outputs of multiple learners in order to improve the efficacy of network intrusion, and Gauthama Raman et al. ( 2020 ) presented a search algorithm based on Support Vector machine to improve the performance of the detection and false alarm rate to improve intrusion detection techniques. Ahmad and Alsemmeari ( 2020 ) targeted extreme learning machine techniques due to their good capabilities in classification problems and handling huge data. They used the NSL-KDD dataset as a benchmark.
With reference to prediction, Bakdash et al. ( 2018 ) used datasets from the U.S. Department of Defence to predict cyberattacks by malware. This dataset consists of weekly counts of cyber events over approximately seven years. Another prediction method was presented by Fan et al. ( 2018 ), which showed an improved integrated cybersecurity prediction method based on spatial-time analysis. Also, with reference to prediction, Ashtiani and Azgomi ( 2014 ) proposed a framework for the distributed simulation of cyberattacks based on high-level architecture. Kirubavathi and Anitha ( 2016 ) recommended an approach to detect botnets, irrespective of their structures, based on network traffic flow behaviour analysis and machine-learning techniques. Dwivedi et al. ( 2021 ) introduced a multi-parallel adaptive technique to utilise an adaption mechanism in the group of swarms for network intrusion detection. AlEroud and Karabatis ( 2018 ) presented an approach that used contextual information to automatically identify and query possible semantic links between different types of suspicious activities extracted from network flows.
Intrusion detection systems with a focus on IoT
In addition to general intrusion detection systems, a proportion of studies focused on IoT. Habib et al. ( 2020 ) presented an approach for converting traditional intrusion detection systems into smart intrusion detection systems for IoT networks. To enhance the process of diagnostic detection of possible vulnerabilities with an IoT system, Georgescu et al. ( 2019 ) introduced a method that uses a named entity recognition-based solution. With regard to IoT in the smart home sector, Heartfield et al. ( 2021 ) presented a detection system that is able to autonomously adjust the decision function of its underlying anomaly classification models to a smart home’s changing condition. Another intrusion detection system was suggested by Keserwani et al. ( 2021 ), which combined Grey Wolf Optimization and Particle Swam Optimization to identify various attacks for IoT networks. They used the KDD Cup 99, NSL-KDD and CICIDS-2017 to evaluate their model. Abu Al-Haija and Zein-Sabatto ( 2020 ) provide a comprehensive development of a new intelligent and autonomous deep-learning-based detection and classification system for cyberattacks in IoT communication networks that leverage the power of convolutional neural networks, abbreviated as IoT-IDCS-CNN (IoT-based Intrusion Detection and Classification System using Convolutional Neural Network). To evaluate the development, the authors used the NSL-KDD dataset. Biswas and Roy ( 2021 ) recommended a model that identifies malicious botnet traffic using novel deep-learning approaches like artificial neural networks gutted recurrent units and long- or short-term memory models. They tested their model with the Bot-IoT dataset.
With a more forensic background, Koroniotis et al. ( 2020 ) submitted a network forensic framework, which described the digital investigation phases for identifying and tracing attack behaviours in IoT networks. The suggested work was evaluated with the Bot-IoT and UINSW-NB15 datasets. With a focus on big data and IoT, Chhabra et al. ( 2020 ) presented a cyber forensic framework for big data analytics in an IoT environment using machine learning. Furthermore, the authors mentioned different publicly available datasets for machine-learning models.
A stronger focus on a mobile phones was exhibited by Alazab et al. ( 2020 ), which presented a classification model that combined permission requests and application programme interface calls. The model was tested with a malware dataset containing 27,891 Android apps. A similar approach was taken by Li et al. ( 2019a , b ), who proposed a reliable classifier for Android malware detection based on factorisation machine architecture and extraction of Android app features from manifest files and source code.
Literature reviews
In addition to the different methods and models for intrusion detection systems, various literature reviews on the methods and datasets were also found. Liu and Lang ( 2019 ) proposed a taxonomy of intrusion detection systems that uses data objects as the main dimension to classify and summarise machine learning and deep learning-based intrusion detection literature. They also presented four different benchmark datasets for machine-learning detection systems. Ahmed et al. ( 2016 ) presented an in-depth analysis of four major categories of anomaly detection techniques, which include classification, statistical, information theory and clustering. Hajj et al. ( 2021 ) gave a comprehensive overview of anomaly-based intrusion detection systems. Their article gives an overview of the requirements, methods, measurements and datasets that are used in an intrusion detection system.
Within the framework of machine learning, Chattopadhyay et al. ( 2018 ) conducted a comprehensive review and meta-analysis on the application of machine-learning techniques in intrusion detection systems. They also compared different machine learning techniques in different datasets and summarised the performance. Vidros et al. ( 2017 ) presented an overview of characteristics and methods in automatic detection of online recruitment fraud. They also published an available dataset of 17,880 annotated job ads, retrieved from the use of a real-life system. An empirical study of different unsupervised learning algorithms used in the detection of unknown attacks was presented by Meira et al. ( 2020 ).
New datasets
Kilincer et al. ( 2021 ) reviewed different intrusion detection system datasets in detail. They had a closer look at the UNS-NB15, ISCX-2012, NSL-KDD and CIDDS-001 datasets. Stojanovic et al. ( 2020 ) also provided a review on datasets and their creation for use in advanced persistent threat detection in the literature. Another review of datasets was provided by Sarker et al. ( 2020 ), who focused on cybersecurity data science as part of their research and provided an overview from a machine-learning perspective. Avila et al. ( 2021 ) conducted a systematic literature review on the use of security logs for data leak detection. They recommended a new classification of information leak, which uses the GDPR principles, identified the most widely publicly available dataset for threat detection, described the attack types in the datasets and the algorithms used for data leak detection. Tuncer et al. ( 2020 ) presented a bytecode-based detection method consisting of feature extraction using local neighbourhood binary patterns. They chose a byte-based malware dataset to investigate the performance of the proposed local neighbourhood binary pattern-based detection method. With a different focus, Mauro et al. ( 2020 ) gave an experimental overview of neural-based techniques relevant to intrusion detection. They assessed the value of neural networks using the Bot-IoT and UNSW-DB15 datasets.
Another category of results in the context of countermeasure datasets is those that were presented as new. Moreno et al. ( 2018 ) developed a database of 300 security-related accidents from European and American sources. The database contained cybersecurity-related events in the chemical and process industry. Damasevicius et al. ( 2020 ) proposed a new dataset (LITNET-2020) for network intrusion detection. The dataset is a new annotated network benchmark dataset obtained from the real-world academic network. It presents real-world examples of normal and under-attack network traffic. With a focus on IoT intrusion detection systems, Alsaedi et al. ( 2020 ) proposed a new benchmark IoT/IIot datasets for assessing intrusion detection system-enabled IoT systems. Also in the context of IoT, Vaccari et al. ( 2020 ) proposed a dataset focusing on message queue telemetry transport protocols, which can be used to train machine-learning models. To evaluate the performance of machine-learning classifiers, Mahfouz et al. ( 2020 ) created a dataset called Game Theory and Cybersecurity (GTCS). A dataset containing 22,000 malware and benign samples was constructed by Martin et al. ( 2019 ). The dataset can be used as a benchmark to test the algorithm for Android malware classification and clustering techniques. In addition, Laso et al. ( 2017 ) presented a dataset created to investigate how data and information quality estimates enable the detection of anomalies and malicious acts in cyber-physical systems. The dataset contained various cyberattacks and is publicly available.
In addition to the results described above, several other studies were found that fit into the category of countermeasures. Johnson et al. ( 2016 ) examined the time between vulnerability disclosures. Using another vulnerabilities database, Common Vulnerabilities and Exposures (CVE), Subroto and Apriyana ( 2019 ) presented an algorithm model that uses big data analysis of social media and statistical machine learning to predict cyber risks. A similar databank but with a different focus, Common Vulnerability Scoring System, was used by Chatterjee and Thekdi ( 2020 ) to present an iterative data-driven learning approach to vulnerability assessment and management for complex systems. Using the CICIDS2017 dataset to evaluate the performance, Malik et al. ( 2020 ) proposed a control plane-based orchestration for varied, sophisticated threats and attacks. The same dataset was used in another study by Lee et al. ( 2019 ), who developed an artificial security information event management system based on a combination of event profiling for data processing and different artificial network methods. To exploit the interdependence between multiple series, Fang et al. ( 2021 ) proposed a statistical framework. In order to validate the framework, the authors applied it to a dataset of enterprise-level security breaches from the Privacy Rights Clearinghouse and Identity Theft Center database. Another framework with a defensive aspect was recommended by Li et al. ( 2021 ) to increase the robustness of deep neural networks against adversarial malware evasion attacks. Sarabi et al. ( 2016 ) investigated whether and to what extent business details can help assess an organisation's risk of data breaches and the distribution of risk across different types of incidents to create policies for protection, detection and recovery from different forms of security incidents. They used data from the VERIS Community Database.
Datasets that have been classified into the cybersecurity category are detailed in Supplementary Table 3. Due to overlap, records from the previous tables may also be included.
This paper presented a systematic literature review of studies on cyber risk and cybersecurity that used datasets. Within this framework, 255 studies were fully reviewed and then classified into three different categories. Then, 79 datasets were consolidated from these studies. These datasets were subsequently analysed, and important information was selected through a process of filtering out. This information was recorded in a table and enhanced with further information as part of the literature analysis. This made it possible to create a comprehensive overview of the datasets. For example, each dataset contains a description of where the data came from and how the data has been used to date. This allows different datasets to be compared and the appropriate dataset for the use case to be selected. This research certainly has limitations, so our selection of datasets cannot necessarily be taken as a representation of all available datasets related to cyber risks and cybersecurity. For example, literature searches were conducted in four academic databases and only found datasets that were used in the literature. Many research projects also used old datasets that may no longer consider current developments. In addition, the data are often focused on only one observation and are limited in scope. For example, the datasets can only be applied to specific contexts and are also subject to further limitations (e.g. region, industry, operating system). In the context of the applicability of the datasets, it is unfortunately not possible to make a clear statement on the extent to which they can be integrated into academic or practical areas of application or how great this effort is. Finally, it remains to be pointed out that this is an overview of currently available datasets, which are subject to constant change.
Due to the lack of datasets on cyber risks in the academic literature, additional datasets on cyber risks were integrated as part of a further search. The search was conducted on the Google Dataset search portal. The search term used was ‘cyber risk datasets’. Over 100 results were found. However, due to the low significance and verifiability, only 20 selected datasets were included. These can be found in Table 2 in the “ Appendix ”.
The results of the literature review and datasets also showed that there continues to be a lack of available, open cyber datasets. This lack of data is reflected in cyber insurance, for example, as it is difficult to find a risk-based premium without a sufficient database (Nurse et al. 2020 ). The global cyber insurance market was estimated at USD 5.5 billion in 2020 (Dyson 2020 ). When compared to the USD 1 trillion global losses from cybercrime (Maleks Smith et al. 2020 ), it is clear that there exists a significant cyber risk awareness challenge for both the insurance industry and international commerce. Without comprehensive and qualitative data on cyber losses, it can be difficult to estimate potential losses from cyberattacks and price cyber insurance accordingly (GAO 2021 ). For instance, the average cyber insurance loss increased from USD 145,000 in 2019 to USD 359,000 in 2020 (FitchRatings 2021 ). Cyber insurance is an important risk management tool to mitigate the financial impact of cybercrime. This is particularly evident in the impact of different industries. In the Energy & Commodities financial markets, a ransomware attack on the Colonial Pipeline led to a substantial impact on the U.S. economy. As a result of the attack, about 45% of the U.S. East Coast was temporarily unable to obtain supplies of diesel, petrol and jet fuel. This caused the average price in the U.S. to rise 7 cents to USD 3.04 per gallon, the highest in seven years (Garber 2021 ). In addition, Colonial Pipeline confirmed that it paid a USD 4.4 million ransom to a hacker gang after the attack. Another ransomware attack occurred in the healthcare and government sector. The victim of this attack was the Irish Health Service Executive (HSE). A ransom payment of USD 20 million was demanded from the Irish government to restore services after the hack (Tidy 2021 ). In the car manufacturing sector, Miller and Valasek ( 2015 ) initiated a cyberattack that resulted in the recall of 1.4 million vehicles and cost manufacturers EUR 761 million. The risk that arises in the context of these events is the potential for the accumulation of cyber losses, which is why cyber insurers are not expanding their capacity. An example of this accumulation of cyber risks is the NotPetya malware attack, which originated in Russia, struck in Ukraine, and rapidly spread around the world, causing at least USD 10 billion in damage (GAO 2021 ). These events highlight the importance of proper cyber risk management.
This research provides cyber insurance stakeholders with an overview of cyber datasets. Cyber insurers can use the open datasets to improve their understanding and assessment of cyber risks. For example, the impact datasets can be used to better measure financial impacts and their frequencies. These data could be combined with existing portfolio data from cyber insurers and integrated with existing pricing tools and factors to better assess cyber risk valuation. Although most cyber insurers have sparse historical cyber policy and claims data, they remain too small at present for accurate prediction (Bessy-Roland et al. 2021 ). A combination of portfolio data and external datasets would support risk-adjusted pricing for cyber insurance, which would also benefit policyholders. In addition, cyber insurance stakeholders can use the datasets to identify patterns and make better predictions, which would benefit sustainable cyber insurance coverage. In terms of cyber risk cause datasets, cyber insurers can use the data to review their insurance products. For example, the data could provide information on which cyber risks have not been sufficiently considered in product design or where improvements are needed. A combination of cyber cause and cybersecurity datasets can help establish uniform definitions to provide greater transparency and clarity. Consistent terminology could lead to a more sustainable cyber market, where cyber insurers make informed decisions about the level of coverage and policyholders understand their coverage (The Geneva Association 2020).
In addition to the cyber insurance community, this research also supports cybersecurity stakeholders. The reviewed literature can be used to provide a contemporary, contextual and categorised summary of available datasets. This supports efficient and timely progress in cyber risk research and is beneficial given the dynamic nature of cyber risks. With the help of the described cybersecurity datasets and the identified information, a comparison of different datasets is possible. The datasets can be used to evaluate the effectiveness of countermeasures in simulated cyberattacks or to test intrusion detection systems.
In this paper, we conducted a systematic review of studies on cyber risk and cybersecurity databases. We found that most of the datasets are in the field of intrusion detection and machine learning and are used for technical cybersecurity aspects. The available datasets on cyber risks were relatively less represented. Due to the dynamic nature and lack of historical data, assessing and understanding cyber risk is a major challenge for cyber insurance stakeholders. To address this challenge, a greater density of cyber data is needed to support cyber insurers in risk management and researchers with cyber risk-related topics. With reference to ‘Open Science’ FAIR data (Jacobsen et al. 2020 ), mandatory reporting of cyber incidents could help improve cyber understanding, awareness and loss prevention among companies and insurers. Through greater availability of data, cyber risks can be better understood, enabling researchers to conduct more in-depth research into these risks. Companies could incorporate this new knowledge into their corporate culture to reduce cyber risks. For insurance companies, this would have the advantage that all insurers would have the same understanding of cyber risks, which would support sustainable risk-based pricing. In addition, common definitions of cyber risks could be derived from new data.
The cybersecurity databases summarised and categorised in this research could provide a different perspective on cyber risks that would enable the formulation of common definitions in cyber policies. The datasets can help companies addressing cybersecurity and cyber risk as part of risk management assess their internal cyber posture and cybersecurity measures. The paper can also help improve risk awareness and corporate behaviour, and provides the research community with a comprehensive overview of peer-reviewed datasets and other available datasets in the area of cyber risk and cybersecurity. This approach is intended to support the free availability of data for research. The complete tabulated review of the literature is included in the Supplementary Material.
This work provides directions for several paths of future work. First, there are currently few publicly available datasets for cyber risk and cybersecurity. The older datasets that are still widely used no longer reflect today's technical environment. Moreover, they can often only be used in one context, and the scope of the samples is very limited. It would be of great value if more datasets were publicly available that reflect current environmental conditions. This could help intrusion detection systems to consider current events and thus lead to a higher success rate. It could also compensate for the disadvantages of older datasets by collecting larger quantities of samples and making this contextualisation more widespread. Another area of research may be the integratability and adaptability of cybersecurity and cyber risk datasets. For example, it is often unclear to what extent datasets can be integrated or adapted to existing data. For cyber risks and cybersecurity, it would be helpful to know what requirements need to be met or what is needed to use the datasets appropriately. In addition, it would certainly be helpful to know whether datasets can be modified to be used for cyber risks or cybersecurity. Finally, the ability for stakeholders to identify machine-readable cybersecurity datasets would be useful because it would allow for even clearer delineations or comparisons between datasets. Due to the lack of publicly available datasets, concrete benchmarks often cannot be applied.
Average cost of a breach of more than 50 million records.
Aamir, M., S.S.H. Rizvi, M.A. Hashmani, M. Zubair, and J. Ahmad. 2021. Machine learning classification of port scanning and DDoS attacks: A comparative analysis. Mehran University Research Journal of Engineering and Technology 40 (1): 215–229. https://doi.org/10.22581/muet1982.2101.19 .
Article Google Scholar
Aamir, M., and S.M.A. Zaidi. 2019. DDoS attack detection with feature engineering and machine learning: The framework and performance evaluation. International Journal of Information Security 18 (6): 761–785. https://doi.org/10.1007/s10207-019-00434-1 .
Aassal, A. El, S. Baki, A. Das, and R.M. Verma. 2020. 2020. An in-depth benchmarking and evaluation of phishing detection research for security needs. IEEE Access 8: 22170–22192. https://doi.org/10.1109/ACCESS.2020.2969780 .
Abu Al-Haija, Q., and S. Zein-Sabatto. 2020. An efficient deep-learning-based detection and classification system for cyber-attacks in IoT communication networks. Electronics 9 (12): 26. https://doi.org/10.3390/electronics9122152 .
Adhikari, U., T.H. Morris, and S.Y. Pan. 2018. Applying Hoeffding adaptive trees for real-time cyber-power event and intrusion classification. IEEE Transactions on Smart Grid 9 (5): 4049–4060. https://doi.org/10.1109/tsg.2017.2647778 .
Agarwal, A., P. Sharma, M. Alshehri, A.A. Mohamed, and O. Alfarraj. 2021. Classification model for accuracy and intrusion detection using machine learning approach. PeerJ Computer Science . https://doi.org/10.7717/peerj-cs.437 .
Agrafiotis, I., J.R.C.. Nurse, M. Goldsmith, S. Creese, and D. Upton. 2018. A taxonomy of cyber-harms: Defining the impacts of cyber-attacks and understanding how they propagate. Journal of Cybersecurity 4: tyy006.
Agrawal, A., S. Mohammed, and J. Fiaidhi. 2019. Ensemble technique for intruder detection in network traffic. International Journal of Security and Its Applications 13 (3): 1–8. https://doi.org/10.33832/ijsia.2019.13.3.01 .
Ahmad, I., and R.A. Alsemmeari. 2020. Towards improving the intrusion detection through ELM (extreme learning machine). CMC Computers Materials & Continua 65 (2): 1097–1111. https://doi.org/10.32604/cmc.2020.011732 .
Ahmed, M., A.N. Mahmood, and J.K. Hu. 2016. A survey of network anomaly detection techniques. Journal of Network and Computer Applications 60: 19–31. https://doi.org/10.1016/j.jnca.2015.11.016 .
Al-Jarrah, O.Y., O. Alhussein, P.D. Yoo, S. Muhaidat, K. Taha, and K. Kim. 2016. Data randomization and cluster-based partitioning for Botnet intrusion detection. IEEE Transactions on Cybernetics 46 (8): 1796–1806. https://doi.org/10.1109/TCYB.2015.2490802 .
Al-Mhiqani, M.N., R. Ahmad, Z.Z. Abidin, W. Yassin, A. Hassan, K.H. Abdulkareem, N.S. Ali, and Z. Yunos. 2020. A review of insider threat detection: Classification, machine learning techniques, datasets, open challenges, and recommendations. Applied Sciences—Basel 10 (15): 41. https://doi.org/10.3390/app10155208 .
Al-Omari, M., M. Rawashdeh, F. Qutaishat, M. Alshira’H, and N. Ababneh. 2021. An intelligent tree-based intrusion detection model for cyber security. Journal of Network and Systems Management 29 (2): 18. https://doi.org/10.1007/s10922-021-09591-y .
Alabdallah, A., and M. Awad. 2018. Using weighted Support Vector Machine to address the imbalanced classes problem of Intrusion Detection System. KSII Transactions on Internet and Information Systems 12 (10): 5143–5158. https://doi.org/10.3837/tiis.2018.10.027 .
Alazab, M., M. Alazab, A. Shalaginov, A. Mesleh, and A. Awajan. 2020. Intelligent mobile malware detection using permission requests and API calls. Future Generation Computer Systems—the International Journal of eScience 107: 509–521. https://doi.org/10.1016/j.future.2020.02.002 .
Albahar, M.A., R.A. Al-Falluji, and M. Binsawad. 2020. An empirical comparison on malicious activity detection using different neural network-based models. IEEE Access 8: 61549–61564. https://doi.org/10.1109/ACCESS.2020.2984157 .
AlEroud, A.F., and G. Karabatis. 2018. Queryable semantics to detect cyber-attacks: A flow-based detection approach. IEEE Transactions on Systems, Man, and Cybernetics: Systems 48 (2): 207–223. https://doi.org/10.1109/TSMC.2016.2600405 .
Algarni, A.M., V. Thayananthan, and Y.K. Malaiya. 2021. Quantitative assessment of cybersecurity risks for mitigating data breaches in business systems. Applied Sciences (switzerland) . https://doi.org/10.3390/app11083678 .
Alhowaide, A., I. Alsmadi, and J. Tang. 2021. Towards the design of real-time autonomous IoT NIDS. Cluster Computing—the Journal of Networks Software Tools and Applications . https://doi.org/10.1007/s10586-021-03231-5 .
Ali, S., and Y. Li. 2019. Learning multilevel auto-encoders for DDoS attack detection in smart grid network. IEEE Access 7: 108647–108659. https://doi.org/10.1109/ACCESS.2019.2933304 .
AlKadi, O., N. Moustafa, B. Turnbull, and K.K.R. Choo. 2019. Mixture localization-based outliers models for securing data migration in cloud centers. IEEE Access 7: 114607–114618. https://doi.org/10.1109/ACCESS.2019.2935142 .
Allianz. 2021. Allianz Risk Barometer. https://www.agcs.allianz.com/content/dam/onemarketing/agcs/agcs/reports/Allianz-Risk-Barometer-2021.pdf . Accessed 15 May 2021.
Almiani, M., A. AbuGhazleh, A. Al-Rahayfeh, S. Atiewi, and Razaque, A. 2020. Deep recurrent neural network for IoT intrusion detection system. Simulation Modelling Practice and Theory 101: 102031. https://doi.org/10.1016/j.simpat.2019.102031
Alsaedi, A., N. Moustafa, Z. Tari, A. Mahmood, and A. Anwar. 2020. TON_IoT telemetry dataset: A new generation dataset of IoT and IIoT for data-driven intrusion detection systems. IEEE Access 8: 165130–165150. https://doi.org/10.1109/access.2020.3022862 .
Alsamiri, J., and K. Alsubhi. 2019. Internet of Things cyber attacks detection using machine learning. International Journal of Advanced Computer Science and Applications 10 (12): 627–634.
Alsharafat, W. 2013. Applying artificial neural network and eXtended classifier system for network intrusion detection. International Arab Journal of Information Technology 10 (3): 230–238.
Google Scholar
Amin, R.W., H.E. Sevil, S. Kocak, G. Francia III., and P. Hoover. 2021. The spatial analysis of the malicious uniform resource locators (URLs): 2016 dataset case study. Information (switzerland) 12 (1): 1–18. https://doi.org/10.3390/info12010002 .
Arcuri, M.C., L.Z. Gai, F. Ielasi, and E. Ventisette. 2020. Cyber attacks on hospitality sector: Stock market reaction. Journal of Hospitality and Tourism Technology 11 (2): 277–290. https://doi.org/10.1108/jhtt-05-2019-0080 .
Arp, D., M. Spreitzenbarth, M. Hubner, H. Gascon, K. Rieck, and C.E.R.T. Siemens. 2014. Drebin: Effective and explainable detection of android malware in your pocket. In Ndss 14: 23–26.
Ashtiani, M., and M.A. Azgomi. 2014. A distributed simulation framework for modeling cyber attacks and the evaluation of security measures. Simulation 90 (9): 1071–1102. https://doi.org/10.1177/0037549714540221 .
Atefinia, R., and M. Ahmadi. 2021. Network intrusion detection using multi-architectural modular deep neural network. Journal of Supercomputing 77 (4): 3571–3593. https://doi.org/10.1007/s11227-020-03410-y .
Avila, R., R. Khoury, R. Khoury, and F. Petrillo. 2021. Use of security logs for data leak detection: A systematic literature review. Security and Communication Networks 2021: 29. https://doi.org/10.1155/2021/6615899 .
Azeez, N.A., T.J. Ayemobola, S. Misra, R. Maskeliunas, and R. Damasevicius. 2019. Network Intrusion Detection with a Hashing Based Apriori Algorithm Using Hadoop MapReduce. Computers 8 (4): 15. https://doi.org/10.3390/computers8040086 .
Bakdash, J.Z., S. Hutchinson, E.G. Zaroukian, L.R. Marusich, S. Thirumuruganathan, C. Sample, B. Hoffman, and G. Das. 2018. Malware in the future forecasting of analyst detection of cyber events. Journal of Cybersecurity . https://doi.org/10.1093/cybsec/tyy007 .
Barletta, V.S., D. Caivano, A. Nannavecchia, and M. Scalera. 2020. Intrusion detection for in-vehicle communication networks: An unsupervised Kohonen SOM approach. Future Internet . https://doi.org/10.3390/FI12070119 .
Barzegar, M., and M. Shajari. 2018. Attack scenario reconstruction using intrusion semantics. Expert Systems with Applications 108: 119–133. https://doi.org/10.1016/j.eswa.2018.04.030 .
Bessy-Roland, Y., A. Boumezoued, and C. Hillairet. 2021. Multivariate Hawkes process for cyber insurance. Annals of Actuarial Science 15 (1): 14–39.
Bhardwaj, A., V. Mangat, and R. Vig. 2020. Hyperband tuned deep neural network with well posed stacked sparse AutoEncoder for detection of DDoS attacks in cloud. IEEE Access 8: 181916–181929. https://doi.org/10.1109/ACCESS.2020.3028690 .
Bhati, B.S., C.S. Rai, B. Balamurugan, and F. Al-Turjman. 2020. An intrusion detection scheme based on the ensemble of discriminant classifiers. Computers & Electrical Engineering 86: 9. https://doi.org/10.1016/j.compeleceng.2020.106742 .
Bhattacharya, S., S.S.R. Krishnan, P.K.R. Maddikunta, R. Kaluri, S. Singh, T.R. Gadekallu, M. Alazab, and U. Tariq. 2020. A novel PCA-firefly based XGBoost classification model for intrusion detection in networks using GPU. Electronics 9 (2): 16. https://doi.org/10.3390/electronics9020219 .
Bibi, I., A. Akhunzada, J. Malik, J. Iqbal, A. Musaddiq, and S. Kim. 2020. A dynamic DL-driven architecture to combat sophisticated android malware. IEEE Access 8: 129600–129612. https://doi.org/10.1109/ACCESS.2020.3009819 .
Biener, C., M. Eling, and J.H. Wirfs. 2015. Insurability of cyber risk: An empirical analysis. The Geneva Papers on Risk and Insurance—Issues and Practice 40 (1): 131–158. https://doi.org/10.1057/gpp.2014.19 .
Binbusayyis, A., and T. Vaiyapuri. 2019. Identifying and benchmarking key features for cyber intrusion detection: An ensemble approach. IEEE Access 7: 106495–106513. https://doi.org/10.1109/ACCESS.2019.2929487 .
Biswas, R., and S. Roy. 2021. Botnet traffic identification using neural networks. Multimedia Tools and Applications . https://doi.org/10.1007/s11042-021-10765-8 .
Bouyeddou, B., F. Harrou, B. Kadri, and Y. Sun. 2021. Detecting network cyber-attacks using an integrated statistical approach. Cluster Computing—the Journal of Networks Software Tools and Applications 24 (2): 1435–1453. https://doi.org/10.1007/s10586-020-03203-1 .
Bozkir, A.S., and M. Aydos. 2020. LogoSENSE: A companion HOG based logo detection scheme for phishing web page and E-mail brand recognition. Computers & Security 95: 18. https://doi.org/10.1016/j.cose.2020.101855 .
Brower, D., and M. McCormick. 2021. Colonial pipeline resumes operations following ransomware attack. Financial Times .
Cai, H., F. Zhang, and A. Levi. 2019. An unsupervised method for detecting shilling attacks in recommender systems by mining item relationship and identifying target items. The Computer Journal 62 (4): 579–597. https://doi.org/10.1093/comjnl/bxy124 .
Cebula, J.J., M.E. Popeck, and L.R. Young. 2014. A Taxonomy of Operational Cyber Security Risks Version 2 .
Chadza, T., K.G. Kyriakopoulos, and S. Lambotharan. 2020. Learning to learn sequential network attacks using hidden Markov models. IEEE Access 8: 134480–134497. https://doi.org/10.1109/ACCESS.2020.3011293 .
Chatterjee, S., and S. Thekdi. 2020. An iterative learning and inference approach to managing dynamic cyber vulnerabilities of complex systems. Reliability Engineering and System Safety . https://doi.org/10.1016/j.ress.2019.106664 .
Chattopadhyay, M., R. Sen, and S. Gupta. 2018. A comprehensive review and meta-analysis on applications of machine learning techniques in intrusion detection. Australasian Journal of Information Systems 22: 27.
Chen, H.S., and J. Fiscus. 2018. The inhospitable vulnerability: A need for cybersecurity risk assessment in the hospitality industry. Journal of Hospitality and Tourism Technology 9 (2): 223–234. https://doi.org/10.1108/JHTT-07-2017-0044 .
Chhabra, G.S., V.P. Singh, and M. Singh. 2020. Cyber forensics framework for big data analytics in IoT environment using machine learning. Multimedia Tools and Applications 79 (23–24): 15881–15900. https://doi.org/10.1007/s11042-018-6338-1 .
Chiba, Z., N. Abghour, K. Moussaid, A. Elomri, and M. Rida. 2019. Intelligent approach to build a Deep Neural Network based IDS for cloud environment using combination of machine learning algorithms. Computers and Security 86: 291–317. https://doi.org/10.1016/j.cose.2019.06.013 .
Choras, M., and R. Kozik. 2015. Machine learning techniques applied to detect cyber attacks on web applications. Logic Journal of the IGPL 23 (1): 45–56. https://doi.org/10.1093/jigpal/jzu038 .
Chowdhury, S., M. Khanzadeh, R. Akula, F. Zhang, S. Zhang, H. Medal, M. Marufuzzaman, and L. Bian. 2017. Botnet detection using graph-based feature clustering. Journal of Big Data 4 (1): 14. https://doi.org/10.1186/s40537-017-0074-7 .
Cost Of A Cyber Incident: Systematic Review And Cross-Validation, Cybersecurity & Infrastructure Agency , 1, https://www.cisa.gov/sites/default/files/publications/CISA-OCE_Cost_of_Cyber_Incidents_Study-FINAL_508.pdf (2020).
D’Hooge, L., T. Wauters, B. Volckaert, and F. De Turck. 2019. Classification hardness for supervised learners on 20 years of intrusion detection data. IEEE Access 7: 167455–167469. https://doi.org/10.1109/access.2019.2953451 .
Damasevicius, R., A. Venckauskas, S. Grigaliunas, J. Toldinas, N. Morkevicius, T. Aleliunas, and P. Smuikys. 2020. LITNET-2020: An annotated real-world network flow dataset for network intrusion detection. Electronics 9 (5): 23. https://doi.org/10.3390/electronics9050800 .
De Giovanni, A.L.D., and M. Pirra. 2020. On the determinants of data breaches: A cointegration analysis. Decisions in Economics and Finance . https://doi.org/10.1007/s10203-020-00301-y .
Deng, L., D. Li, X. Yao, and H. Wang. 2019. Retracted Article: Mobile network intrusion detection for IoT system based on transfer learning algorithm. Cluster Computing 22 (4): 9889–9904. https://doi.org/10.1007/s10586-018-1847-2 .
Donkal, G., and G.K. Verma. 2018. A multimodal fusion based framework to reinforce IDS for securing Big Data environment using Spark. Journal of Information Security and Applications 43: 1–11. https://doi.org/10.1016/j.jisa.2018.10.001 .
Dunn, C., N. Moustafa, and B. Turnbull. 2020. Robustness evaluations of sustainable machine learning models against data Poisoning attacks in the Internet of Things. Sustainability 12 (16): 17. https://doi.org/10.3390/su12166434 .
Dwivedi, S., M. Vardhan, and S. Tripathi. 2021. Multi-parallel adaptive grasshopper optimization technique for detecting anonymous attacks in wireless networks. Wireless Personal Communications . https://doi.org/10.1007/s11277-021-08368-5 .
Dyson, B. 2020. COVID-19 crisis could be ‘watershed’ for cyber insurance, says Swiss Re exec. https://www.spglobal.com/marketintelligence/en/news-insights/latest-news-headlines/covid-19-crisis-could-be-watershed-for-cyber-insurance-says-swiss-re-exec-59197154 . Accessed 7 May 2020.
EIOPA. 2018. Understanding cyber insurance—a structured dialogue with insurance companies. https://www.eiopa.europa.eu/sites/default/files/publications/reports/eiopa_understanding_cyber_insurance.pdf . Accessed 28 May 2018
Elijah, A.V., A. Abdullah, N.Z. JhanJhi, M. Supramaniam, and O.B. Abdullateef. 2019. Ensemble and deep-learning methods for two-class and multi-attack anomaly intrusion detection: An empirical study. International Journal of Advanced Computer Science and Applications 10 (9): 520–528.
Eling, M., and K. Jung. 2018. Copula approaches for modeling cross-sectional dependence of data breach losses. Insurance Mathematics & Economics 82: 167–180. https://doi.org/10.1016/j.insmatheco.2018.07.003 .
Eling, M., and W. Schnell. 2016. What do we know about cyber risk and cyber risk insurance? Journal of Risk Finance 17 (5): 474–491. https://doi.org/10.1108/jrf-09-2016-0122 .
Eling, M., and J. Wirfs. 2019. What are the actual costs of cyber risk events? European Journal of Operational Research 272 (3): 1109–1119. https://doi.org/10.1016/j.ejor.2018.07.021 .
Eling, M. 2020. Cyber risk research in business and actuarial science. European Actuarial Journal 10 (2): 303–333.
Elmasry, W., A. Akbulut, and A.H. Zaim. 2019. Empirical study on multiclass classification-based network intrusion detection. Computational Intelligence 35 (4): 919–954. https://doi.org/10.1111/coin.12220 .
Elsaid, S.A., and N.S. Albatati. 2020. An optimized collaborative intrusion detection system for wireless sensor networks. Soft Computing 24 (16): 12553–12567. https://doi.org/10.1007/s00500-020-04695-0 .
Estepa, R., J.E. Díaz-Verdejo, A. Estepa, and G. Madinabeitia. 2020. How much training data is enough? A case study for HTTP anomaly-based intrusion detection. IEEE Access 8: 44410–44425. https://doi.org/10.1109/ACCESS.2020.2977591 .
European Council. 2021. Cybersecurity: how the EU tackles cyber threats. https://www.consilium.europa.eu/en/policies/cybersecurity/ . Accessed 10 May 2021
Falco, G. et al. 2019. Cyber risk research impeded by disciplinary barriers. Science (American Association for the Advancement of Science) 366 (6469): 1066–1069.
Fan, Z.J., Z.P. Tan, C.X. Tan, and X. Li. 2018. An improved integrated prediction method of cyber security situation based on spatial-time analysis. Journal of Internet Technology 19 (6): 1789–1800. https://doi.org/10.3966/160792642018111906015 .
Fang, Z.J., M.C. Xu, S.H. Xu, and T.Z. Hu. 2021. A framework for predicting data breach risk: Leveraging dependence to cope with sparsity. IEEE Transactions on Information Forensics and Security 16: 2186–2201. https://doi.org/10.1109/tifs.2021.3051804 .
Farkas, S., O. Lopez, and M. Thomas. 2021. Cyber claim analysis using Generalized Pareto regression trees with applications to insurance. Insurance: Mathematics and Economics 98: 92–105. https://doi.org/10.1016/j.insmatheco.2021.02.009 .
Farsi, H., A. Fanian, and Z. Taghiyarrenani. 2019. A novel online state-based anomaly detection system for process control networks. International Journal of Critical Infrastructure Protection 27: 11. https://doi.org/10.1016/j.ijcip.2019.100323 .
Ferrag, M.A., L. Maglaras, S. Moschoyiannis, and H. Janicke. 2020. Deep learning for cyber security intrusion detection: Approaches, datasets, and comparative study. Journal of Information Security and Applications 50: 19. https://doi.org/10.1016/j.jisa.2019.102419 .
Field, M. 2018. WannaCry cyber attack cost the NHS £92m as 19,000 appointments cancelled. https://www.telegraph.co.uk/technology/2018/10/11/wannacry-cyber-attack-cost-nhs-92m-19000-appointments-cancelled/ . Accessed 9 May 2018.
FitchRatings. 2021. U.S. Cyber Insurance Market Update (Spike in Claims Leads to Decline in 2020 Underwriting Performance). https://www.fitchratings.com/research/insurance/us-cyber-insurance-market-update-spike-in-claims-leads-to-decline-in-2020-underwriting-performance-26-05-2021 .
Fossaceca, J.M., T.A. Mazzuchi, and S. Sarkani. 2015. MARK-ELM: Application of a novel Multiple Kernel Learning framework for improving the robustness of network intrusion detection. Expert Systems with Applications 42 (8): 4062–4080. https://doi.org/10.1016/j.eswa.2014.12.040 .
Franke, U., and J. Brynielsson. 2014. Cyber situational awareness–a systematic review of the literature. Computers & security 46: 18–31.
Freeha, K., K.J. Hwan, M. Lars, and M. Robin. 2021. Data breach management: An integrated risk model. Information & Management 58 (1): 103392. https://doi.org/10.1016/j.im.2020.103392 .
Ganeshan, R., and P. Rodrigues. 2020. Crow-AFL: Crow based adaptive fractional lion optimization approach for the intrusion detection. Wireless Personal Communications 111 (4): 2065–2089. https://doi.org/10.1007/s11277-019-06972-0 .
GAO. 2021. CYBER INSURANCE—Insurers and policyholders face challenges in an evolving market. https://www.gao.gov/assets/gao-21-477.pdf . Accessed 16 May 2021.
Garber, J. 2021. Colonial Pipeline fiasco foreshadows impact of Biden energy policy. https://www.foxbusiness.com/markets/colonial-pipeline-fiasco-foreshadows-impact-of-biden-energy-policy . Accessed 4 May 2021.
Gauthama Raman, M.R., N. Somu, S. Jagarapu, T. Manghnani, T. Selvam, K. Krithivasan, and V.S. Shankar Sriram. 2020. An efficient intrusion detection technique based on support vector machine and improved binary gravitational search algorithm. Artificial Intelligence Review 53 (5): 3255–3286. https://doi.org/10.1007/s10462-019-09762-z .
Gavel, S., A.S. Raghuvanshi, and S. Tiwari. 2021. Distributed intrusion detection scheme using dual-axis dimensionality reduction for Internet of things (IoT). Journal of Supercomputing . https://doi.org/10.1007/s11227-021-03697-5 .
GDPR.EU. 2021. FAQ. https://gdpr.eu/faq/ . Accessed 10 May 2021.
Georgescu, T.M., B. Iancu, and M. Zurini. 2019. Named-entity-recognition-based automated system for diagnosing cybersecurity situations in IoT networks. Sensors (switzerland) . https://doi.org/10.3390/s19153380 .
Giudici, P., and E. Raffinetti. 2020. Cyber risk ordering with rank-based statistical models. AStA Advances in Statistical Analysis . https://doi.org/10.1007/s10182-020-00387-0 .
Goh, J., S. Adepu, K.N. Junejo, and A. Mathur. 2016. A dataset to support research in the design of secure water treatment systems. In CRITIS.
Gong, X.Y., J.L. Lu, Y.F. Zhou, H. Qiu, and R. He. 2021. Model uncertainty based annotation error fixing for web attack detection. Journal of Signal Processing Systems for Signal Image and Video Technology 93 (2–3): 187–199. https://doi.org/10.1007/s11265-019-01494-1 .
Goode, S., H. Hoehle, V. Venkatesh, and S.A. Brown. 2017. USER compensation as a data breach recovery action: An investigation of the sony playstation network breach. MIS Quarterly 41 (3): 703–727.
Guo, H., S. Huang, C. Huang, Z. Pan, M. Zhang, and F. Shi. 2020. File entropy signal analysis combined with wavelet decomposition for malware classification. IEEE Access 8: 158961–158971. https://doi.org/10.1109/ACCESS.2020.3020330 .
Habib, M., I. Aljarah, and H. Faris. 2020. A Modified multi-objective particle swarm optimizer-based Lévy flight: An approach toward intrusion detection in Internet of Things. Arabian Journal for Science and Engineering 45 (8): 6081–6108. https://doi.org/10.1007/s13369-020-04476-9 .
Hajj, S., R. El Sibai, J.B. Abdo, J. Demerjian, A. Makhoul, and C. Guyeux. 2021. Anomaly-based intrusion detection systems: The requirements, methods, measurements, and datasets. Transactions on Emerging Telecommunications Technologies 32 (4): 36. https://doi.org/10.1002/ett.4240 .
Heartfield, R., G. Loukas, A. Bezemskij, and E. Panaousis. 2021. Self-configurable cyber-physical intrusion detection for smart homes using reinforcement learning. IEEE Transactions on Information Forensics and Security 16: 1720–1735. https://doi.org/10.1109/tifs.2020.3042049 .
Hemo, B., T. Gafni, K. Cohen, and Q. Zhao. 2020. Searching for anomalies over composite hypotheses. IEEE Transactions on Signal Processing 68: 1181–1196. https://doi.org/10.1109/TSP.2020.2971438
Hindy, H., D. Brosset, E. Bayne, A.K. Seeam, C. Tachtatzis, R. Atkinson, and X. Bellekens. 2020. A taxonomy of network threats and the effect of current datasets on intrusion detection systems. IEEE Access 8: 104650–104675. https://doi.org/10.1109/ACCESS.2020.3000179 .
Hong, W., D. Huang, C. Chen, and J. Lee. 2020. Towards accurate and efficient classification of power system contingencies and cyber-attacks using recurrent neural networks. IEEE Access 8: 123297–123309. https://doi.org/10.1109/ACCESS.2020.3007609 .
Husák, M., M. Zádník, V. Bartos, and P. Sokol. 2020. Dataset of intrusion detection alerts from a sharing platform. Data in Brief 33: 106530.
IBM Security. 2020. Cost of a Data breach Report. https://www.capita.com/sites/g/files/nginej291/files/2020-08/Ponemon-Global-Cost-of-Data-Breach-Study-2020.pdf . Accessed 19 May 2021.
IEEE. 2021. IEEE Quick Facts. https://www.ieee.org/about/at-a-glance.html . Accessed 11 May 2021.
Kilincer, I.F., F. Ertam, and S. Abdulkadir. 2021. Machine learning methods for cyber security intrusion detection: Datasets and comparative study. Computer Networks 188: 107840. https://doi.org/10.1016/j.comnet.2021.107840 .
Jaber, A.N., and S. Ul Rehman. 2020. FCM-SVM based intrusion detection system for cloud computing environment. Cluster Computing—the Journal of Networks Software Tools and Applications 23 (4): 3221–3231. https://doi.org/10.1007/s10586-020-03082-6 .
Jacobs, J., S. Romanosky, B. Edwards, M. Roytman, and I. Adjerid. 2019. Exploit prediction scoring system (epss). arXiv:1908.04856
Jacobsen, A. et al. 2020. FAIR principles: Interpretations and implementation considerations. Data Intelligence 2 (1–2): 10–29. https://doi.org/10.1162/dint_r_00024 .
Jahromi, A.N., S. Hashemi, A. Dehghantanha, R.M. Parizi, and K.K.R. Choo. 2020. An enhanced stacked LSTM method with no random initialization for malware threat hunting in safety and time-critical systems. IEEE Transactions on Emerging Topics in Computational Intelligence 4 (5): 630–640. https://doi.org/10.1109/TETCI.2019.2910243 .
Jang, S., S. Li, and Y. Sung. 2020. FastText-based local feature visualization algorithm for merged image-based malware classification framework for cyber security and cyber defense. Mathematics 8 (3): 13. https://doi.org/10.3390/math8030460 .
Javeed, D., T.H. Gao, and M.T. Khan. 2021. SDN-enabled hybrid DL-driven framework for the detection of emerging cyber threats in IoT. Electronics 10 (8): 16. https://doi.org/10.3390/electronics10080918 .
Johnson, P., D. Gorton, R. Lagerstrom, and M. Ekstedt. 2016. Time between vulnerability disclosures: A measure of software product vulnerability. Computers & Security 62: 278–295. https://doi.org/10.1016/j.cose.2016.08.004 .
Johnson, P., R. Lagerström, M. Ekstedt, and U. Franke. 2018. Can the common vulnerability scoring system be trusted? A Bayesian analysis. IEEE Transactions on Dependable and Secure Computing 15 (6): 1002–1015. https://doi.org/10.1109/TDSC.2016.2644614 .
Junger, M., V. Wang, and M. Schlömer. 2020. Fraud against businesses both online and offline: Crime scripts, business characteristics, efforts, and benefits. Crime Science 9 (1): 13. https://doi.org/10.1186/s40163-020-00119-4 .
Kalutarage, H.K., H.N. Nguyen, and S.A. Shaikh. 2017. Towards a threat assessment framework for apps collusion. Telecommunication Systems 66 (3): 417–430. https://doi.org/10.1007/s11235-017-0296-1 .
Kamarudin, M.H., C. Maple, T. Watson, and N.S. Safa. 2017. A LogitBoost-based algorithm for detecting known and unknown web attacks. IEEE Access 5: 26190–26200. https://doi.org/10.1109/ACCESS.2017.2766844 .
Kasongo, S.M., and Y.X. Sun. 2020. A deep learning method with wrapper based feature extraction for wireless intrusion detection system. Computers & Security 92: 15. https://doi.org/10.1016/j.cose.2020.101752 .
Keserwani, P.K., M.C. Govil, E.S. Pilli, and P. Govil. 2021. A smart anomaly-based intrusion detection system for the Internet of Things (IoT) network using GWO–PSO–RF model. Journal of Reliable Intelligent Environments 7 (1): 3–21. https://doi.org/10.1007/s40860-020-00126-x .
Keshk, M., E. Sitnikova, N. Moustafa, J. Hu, and I. Khalil. 2021. An integrated framework for privacy-preserving based anomaly detection for cyber-physical systems. IEEE Transactions on Sustainable Computing 6 (1): 66–79. https://doi.org/10.1109/TSUSC.2019.2906657 .
Khan, I.A., D.C. Pi, A.K. Bhatia, N. Khan, W. Haider, and A. Wahab. 2020. Generating realistic IoT-based IDS dataset centred on fuzzy qualitative modelling for cyber-physical systems. Electronics Letters 56 (9): 441–443. https://doi.org/10.1049/el.2019.4158 .
Khraisat, A., I. Gondal, P. Vamplew, J. Kamruzzaman, and A. Alazab. 2020. Hybrid intrusion detection system based on the stacking ensemble of C5 decision tree classifier and one class support vector machine. Electronics 9 (1): 18. https://doi.org/10.3390/electronics9010173 .
Khraisat, A., I. Gondal, P. Vamplew, and J. Kamruzzaman. 2019. Survey of intrusion detection systems: Techniques, datasets and challenges. Cybersecurity 2 (1): 20. https://doi.org/10.1186/s42400-019-0038-7 .
Kilincer, I.F., F. Ertam, and A. Sengur. 2021. Machine learning methods for cyber security intrusion detection: Datasets and comparative study. Computer Networks 188: 16. https://doi.org/10.1016/j.comnet.2021.107840 .
Kim, D., and H.K. Kim. 2019. Automated dataset generation system for collaborative research of cyber threat analysis. Security and Communication Networks 2019: 10. https://doi.org/10.1155/2019/6268476 .
Kim, G., C. Lee, J. Jo, and H. Lim. 2020. Automatic extraction of named entities of cyber threats using a deep Bi-LSTM-CRF network. International Journal of Machine Learning and Cybernetics 11 (10): 2341–2355. https://doi.org/10.1007/s13042-020-01122-6 .
Kirubavathi, G., and R. Anitha. 2016. Botnet detection via mining of traffic flow characteristics. Computers & Electrical Engineering 50: 91–101. https://doi.org/10.1016/j.compeleceng.2016.01.012 .
Kiwia, D., A. Dehghantanha, K.K.R. Choo, and J. Slaughter. 2018. A cyber kill chain based taxonomy of banking Trojans for evolutionary computational intelligence. Journal of Computational Science 27: 394–409. https://doi.org/10.1016/j.jocs.2017.10.020 .
Koroniotis, N., N. Moustafa, and E. Sitnikova. 2020. A new network forensic framework based on deep learning for Internet of Things networks: A particle deep framework. Future Generation Computer Systems 110: 91–106. https://doi.org/10.1016/j.future.2020.03.042 .
Kruse, C.S., B. Frederick, T. Jacobson, and D. Kyle Monticone. 2017. Cybersecurity in healthcare: A systematic review of modern threats and trends. Technology and Health Care 25 (1): 1–10.
Kshetri, N. 2018. The economics of cyber-insurance. IT Professional 20 (6): 9–14. https://doi.org/10.1109/MITP.2018.2874210 .
Kumar, R., P. Kumar, R. Tripathi, G.P. Gupta, T.R. Gadekallu, and G. Srivastava. 2021. SP2F: A secured privacy-preserving framework for smart agricultural Unmanned Aerial Vehicles. Computer Networks . https://doi.org/10.1016/j.comnet.2021.107819 .
Kumar, R., and R. Tripathi. 2021. DBTP2SF: A deep blockchain-based trustworthy privacy-preserving secured framework in industrial internet of things systems. Transactions on Emerging Telecommunications Technologies 32 (4): 27. https://doi.org/10.1002/ett.4222 .
Laso, P.M., D. Brosset, and J. Puentes. 2017. Dataset of anomalies and malicious acts in a cyber-physical subsystem. Data in Brief 14: 186–191. https://doi.org/10.1016/j.dib.2017.07.038 .
Lee, J., J. Kim, I. Kim, and K. Han. 2019. Cyber threat detection based on artificial neural networks using event profiles. IEEE Access 7: 165607–165626. https://doi.org/10.1109/ACCESS.2019.2953095 .
Lee, S.J., P.D. Yoo, A.T. Asyhari, Y. Jhi, L. Chermak, C.Y. Yeun, and K. Taha. 2020. IMPACT: Impersonation attack detection via edge computing using deep Autoencoder and feature abstraction. IEEE Access 8: 65520–65529. https://doi.org/10.1109/ACCESS.2020.2985089 .
Leong, Y.-Y., and Y.-C. Chen. 2020. Cyber risk cost and management in IoT devices-linked health insurance. The Geneva Papers on Risk and Insurance—Issues and Practice 45 (4): 737–759. https://doi.org/10.1057/s41288-020-00169-4 .
Levi, M. 2017. Assessing the trends, scale and nature of economic cybercrimes: overview and Issues: In Cybercrimes, cybercriminals and their policing, in crime, law and social change. Crime, Law and Social Change 67 (1): 3–20. https://doi.org/10.1007/s10611-016-9645-3 .
Li, C., K. Mills, D. Niu, R. Zhu, H. Zhang, and H. Kinawi. 2019a. Android malware detection based on factorization machine. IEEE Access 7: 184008–184019. https://doi.org/10.1109/ACCESS.2019.2958927 .
Li, D.Q., and Q.M. Li. 2020. Adversarial deep ensemble: evasion attacks and defenses for malware detection. IEEE Transactions on Information Forensics and Security 15: 3886–3900. https://doi.org/10.1109/tifs.2020.3003571 .
Li, D.Q., Q.M. Li, Y.F. Ye, and S.H. Xu. 2021. A framework for enhancing deep neural networks against adversarial malware. IEEE Transactions on Network Science and Engineering 8 (1): 736–750. https://doi.org/10.1109/tnse.2021.3051354 .
Li, R.H., C. Zhang, C. Feng, X. Zhang, and C.J. Tang. 2019b. Locating vulnerability in binaries using deep neural networks. IEEE Access 7: 134660–134676. https://doi.org/10.1109/access.2019.2942043 .
Li, X., M. Xu, P. Vijayakumar, N. Kumar, and X. Liu. 2020. Detection of low-frequency and multi-stage attacks in industrial Internet of Things. IEEE Transactions on Vehicular Technology 69 (8): 8820–8831. https://doi.org/10.1109/TVT.2020.2995133 .
Liu, H.Y., and B. Lang. 2019. Machine learning and deep learning methods for intrusion detection systems: A survey. Applied Sciences—Basel 9 (20): 28. https://doi.org/10.3390/app9204396 .
Lopez-Martin, M., B. Carro, and A. Sanchez-Esguevillas. 2020. Application of deep reinforcement learning to intrusion detection for supervised problems. Expert Systems with Applications . https://doi.org/10.1016/j.eswa.2019.112963 .
Loukas, G., D. Gan, and Tuan Vuong. 2013. A review of cyber threats and defence approaches in emergency management. Future Internet 5: 205–236.
Luo, C.C., S. Su, Y.B. Sun, Q.J. Tan, M. Han, and Z.H. Tian. 2020. A convolution-based system for malicious URLs detection. CMC—Computers Materials Continua 62 (1): 399–411.
Mahbooba, B., M. Timilsina, R. Sahal, and M. Serrano. 2021. Explainable artificial intelligence (XAI) to enhance trust management in intrusion detection systems using decision tree model. Complexity 2021: 11. https://doi.org/10.1155/2021/6634811 .
Mahdavifar, S., and A.A. Ghorbani. 2020. DeNNeS: Deep embedded neural network expert system for detecting cyber attacks. Neural Computing & Applications 32 (18): 14753–14780. https://doi.org/10.1007/s00521-020-04830-w .
Mahfouz, A., A. Abuhussein, D. Venugopal, and S. Shiva. 2020. Ensemble classifiers for network intrusion detection using a novel network attack dataset. Future Internet 12 (11): 1–19. https://doi.org/10.3390/fi12110180 .
Maleks Smith, Z., E. Lostri, and J.A. Lewis. 2020. The hidden costs of cybercrime. https://www.mcafee.com/enterprise/en-us/assets/reports/rp-hidden-costs-of-cybercrime.pdf . Accessed 16 May 2021.
Malik, J., A. Akhunzada, I. Bibi, M. Imran, A. Musaddiq, and S.W. Kim. 2020. Hybrid deep learning: An efficient reconnaissance and surveillance detection mechanism in SDN. IEEE Access 8: 134695–134706. https://doi.org/10.1109/ACCESS.2020.3009849 .
Manimurugan, S. 2020. IoT-Fog-Cloud model for anomaly detection using improved Naive Bayes and principal component analysis. Journal of Ambient Intelligence and Humanized Computing . https://doi.org/10.1007/s12652-020-02723-3 .
Martin, A., R. Lara-Cabrera, and D. Camacho. 2019. Android malware detection through hybrid features fusion and ensemble classifiers: The AndroPyTool framework and the OmniDroid dataset. Information Fusion 52: 128–142. https://doi.org/10.1016/j.inffus.2018.12.006 .
Mauro, M.D., G. Galatro, and A. Liotta. 2020. Experimental review of neural-based approaches for network intrusion management. IEEE Transactions on Network and Service Management 17 (4): 2480–2495. https://doi.org/10.1109/TNSM.2020.3024225 .
McLeod, A., and D. Dolezel. 2018. Cyber-analytics: Modeling factors associated with healthcare data breaches. Decision Support Systems 108: 57–68. https://doi.org/10.1016/j.dss.2018.02.007 .
Meira, J., R. Andrade, I. Praca, J. Carneiro, V. Bolon-Canedo, A. Alonso-Betanzos, and G. Marreiros. 2020. Performance evaluation of unsupervised techniques in cyber-attack anomaly detection. Journal of Ambient Intelligence and Humanized Computing 11 (11): 4477–4489. https://doi.org/10.1007/s12652-019-01417-9 .
Miao, Y., J. Ma, X. Liu, J. Weng, H. Li, and H. Li. 2019. Lightweight fine-grained search over encrypted data in Fog computing. IEEE Transactions on Services Computing 12 (5): 772–785. https://doi.org/10.1109/TSC.2018.2823309 .
Miller, C., and C. Valasek. 2015. Remote exploitation of an unaltered passenger vehicle. Black Hat USA 2015 (S 91).
Mireles, J.D., E. Ficke, J.H. Cho, P. Hurley, and S.H. Xu. 2019. Metrics towards measuring cyber agility. IEEE Transactions on Information Forensics and Security 14 (12): 3217–3232. https://doi.org/10.1109/tifs.2019.2912551 .
Mishra, N., and S. Pandya. 2021. Internet of Things applications, security challenges, attacks, intrusion detection, and future visions: A systematic review. IEEE Access . https://doi.org/10.1109/ACCESS.2021.3073408 .
Monshizadeh, M., V. Khatri, B.G. Atli, R. Kantola, and Z. Yan. 2019. Performance evaluation of a combined anomaly detection platform. IEEE Access 7: 100964–100978. https://doi.org/10.1109/ACCESS.2019.2930832 .
Moreno, V.C., G. Reniers, E. Salzano, and V. Cozzani. 2018. Analysis of physical and cyber security-related events in the chemical and process industry. Process Safety and Environmental Protection 116: 621–631. https://doi.org/10.1016/j.psep.2018.03.026 .
Moro, E.D. 2020. Towards an economic cyber loss index for parametric cover based on IT security indicator: A preliminary analysis. Risks . https://doi.org/10.3390/risks8020045 .
Moustafa, N., E. Adi, B. Turnbull, and J. Hu. 2018. A new threat intelligence scheme for safeguarding industry 4.0 systems. IEEE Access 6: 32910–32924. https://doi.org/10.1109/ACCESS.2018.2844794 .
Moustakidis, S., and P. Karlsson. 2020. A novel feature extraction methodology using Siamese convolutional neural networks for intrusion detection. Cybersecurity . https://doi.org/10.1186/s42400-020-00056-4 .
Mukhopadhyay, A., S. Chatterjee, K.K. Bagchi, P.J. Kirs, and G.K. Shukla. 2019. Cyber Risk Assessment and Mitigation (CRAM) framework using Logit and Probit models for cyber insurance. Information Systems Frontiers 21 (5): 997–1018. https://doi.org/10.1007/s10796-017-9808-5 .
Murphey, H. 2021a. Biden signs executive order to strengthen US cyber security. https://www.ft.com/content/4d808359-b504-4014-85f6-68e7a2851bf1?accessToken=zwAAAXl0_ifgkc9NgINZtQRAFNOF9mjnooUb8Q.MEYCIQDw46SFWsMn1iyuz3kvgAmn6mxc0rIVfw10Lg1ovJSfJwIhAK2X2URzfSqHwIS7ddRCvSt2nGC2DcdoiDTG49-4TeEt&sharetype=gift?token=fbcd6323-1ecf-4fc3-b136-b5b0dd6a8756 . Accessed 7 May 2021.
Murphey, H. 2021b. Millions of connected devices have security flaws, study shows. https://www.ft.com/content/0bf92003-926d-4dee-87d7-b01f7c3e9621?accessToken=zwAAAXnA7f2Ikc8L-SADkm1N7tOH17AffD6WIQ.MEQCIDjBuROvhmYV0Mx3iB0cEV7m5oND1uaCICxJu0mzxM0PAiBam98q9zfHiTB6hKGr1gGl0Azt85yazdpX9K5sI8se3Q&sharetype=gift?token=2538218d-77d9-4dd3-9649-3cb556a34e51 . Accessed 6 May 2021.
Murugesan, V., M. Shalinie, and M.H. Yang. 2018. Design and analysis of hybrid single packet IP traceback scheme. IET Networks 7 (3): 141–151. https://doi.org/10.1049/iet-net.2017.0115 .
Mwitondi, K.S., and S.A. Zargari. 2018. An iterative multiple sampling method for intrusion detection. Information Security Journal 27 (4): 230–239. https://doi.org/10.1080/19393555.2018.1539790 .
Neto, N.N., S. Madnick, A.M.G. De Paula, and N.M. Borges. 2021. Developing a global data breach database and the challenges encountered. ACM Journal of Data and Information Quality 13 (1): 33. https://doi.org/10.1145/3439873 .
Nurse, J.R.C., L. Axon, A. Erola, I. Agrafiotis, M. Goldsmith, and S. Creese. 2020. The data that drives cyber insurance: A study into the underwriting and claims processes. In 2020 International conference on cyber situational awareness, data analytics and assessment (CyberSA), 15–19 June 2020.
Oliveira, N., I. Praca, E. Maia, and O. Sousa. 2021. Intelligent cyber attack detection and classification for network-based intrusion detection systems. Applied Sciences—Basel 11 (4): 21. https://doi.org/10.3390/app11041674 .
Page, M.J. et al. 2021. The PRISMA 2020 statement: An updated guideline for reporting systematic reviews. Systematic Reviews 10 (1): 89. https://doi.org/10.1186/s13643-021-01626-4 .
Pajouh, H.H., R. Javidan, R. Khayami, A. Dehghantanha, and K.R. Choo. 2019. A two-layer dimension reduction and two-tier classification model for anomaly-based intrusion detection in IoT backbone networks. IEEE Transactions on Emerging Topics in Computing 7 (2): 314–323. https://doi.org/10.1109/TETC.2016.2633228 .
Parra, G.D., P. Rad, K.K.R. Choo, and N. Beebe. 2020. Detecting Internet of Things attacks using distributed deep learning. Journal of Network and Computer Applications 163: 13. https://doi.org/10.1016/j.jnca.2020.102662 .
Paté-Cornell, M.E., M. Kuypers, M. Smith, and P. Keller. 2018. Cyber risk management for critical infrastructure: A risk analysis model and three case studies. Risk Analysis 38 (2): 226–241. https://doi.org/10.1111/risa.12844 .
Pooser, D.M., M.J. Browne, and O. Arkhangelska. 2018. Growth in the perception of cyber risk: evidence from U.S. P&C Insurers. The Geneva Papers on Risk and Insurance—Issues and Practice 43 (2): 208–223. https://doi.org/10.1057/s41288-017-0077-9 .
Pu, G., L. Wang, J. Shen, and F. Dong. 2021. A hybrid unsupervised clustering-based anomaly detection method. Tsinghua Science and Technology 26 (2): 146–153. https://doi.org/10.26599/TST.2019.9010051 .
Qiu, J., W. Luo, L. Pan, Y. Tai, J. Zhang, and Y. Xiang. 2019. Predicting the impact of android malicious samples via machine learning. IEEE Access 7: 66304–66316. https://doi.org/10.1109/ACCESS.2019.2914311 .
Qu, X., L. Yang, K. Guo, M. Sun, L. Ma, T. Feng, S. Ren, K. Li, and X. Ma. 2020. Direct batch growth hierarchical self-organizing mapping based on statistics for efficient network intrusion detection. IEEE Access 8: 42251–42260. https://doi.org/10.1109/ACCESS.2020.2976810 .
Rahman, Md.S., S. Halder, Md. Ashraf Uddin, and U.K. Acharjee. 2021. An efficient hybrid system for anomaly detection in social networks. Cybersecurity 4 (1): 10. https://doi.org/10.1186/s42400-021-00074-w .
Ramaiah, M., V. Chandrasekaran, V. Ravi, and N. Kumar. 2021. An intrusion detection system using optimized deep neural network architecture. Transactions on Emerging Telecommunications Technologies 32 (4): 17. https://doi.org/10.1002/ett.4221 .
Raman, M.R.G., K. Kannan, S.K. Pal, and V.S.S. Sriram. 2016. Rough set-hypergraph-based feature selection approach for intrusion detection systems. Defence Science Journal 66 (6): 612–617. https://doi.org/10.14429/dsj.66.10802 .
Rathore, S., J.H. Park. 2018. Semi-supervised learning based distributed attack detection framework for IoT. Applied Soft Computing 72: 79–89. https://doi.org/10.1016/j.asoc.2018.05.049 .
Romanosky, S., L. Ablon, A. Kuehn, and T. Jones. 2019. Content analysis of cyber insurance policies: How do carriers price cyber risk? Journal of Cybersecurity (oxford) 5 (1): tyz002.
Sarabi, A., P. Naghizadeh, Y. Liu, and M. Liu. 2016. Risky business: Fine-grained data breach prediction using business profiles. Journal of Cybersecurity 2 (1): 15–28. https://doi.org/10.1093/cybsec/tyw004 .
Sardi, Alberto, Alessandro Rizzi, Enrico Sorano, and Anna Guerrieri. 2021. Cyber risk in health facilities: A systematic literature review. Sustainability 12 (17): 7002.
Sarker, Iqbal H., A.S.M. Kayes, Shahriar Badsha, Hamed Alqahtani, Paul Watters, and Alex Ng. 2020. Cybersecurity data science: An overview from machine learning perspective. Journal of Big Data 7 (1): 41. https://doi.org/10.1186/s40537-020-00318-5 .
Scopus. 2021. Factsheet. https://www.elsevier.com/__data/assets/pdf_file/0017/114533/Scopus_GlobalResearch_Factsheet2019_FINAL_WEB.pdf . Accessed 11 May 2021.
Sentuna, A., A. Alsadoon, P.W.C. Prasad, M. Saadeh, and O.H. Alsadoon. 2021. A novel Enhanced Naïve Bayes Posterior Probability (ENBPP) using machine learning: Cyber threat analysis. Neural Processing Letters 53 (1): 177–209. https://doi.org/10.1007/s11063-020-10381-x .
Shaukat, K., S.H. Luo, V. Varadharajan, I.A. Hameed, S. Chen, D.X. Liu, and J.M. Li. 2020. Performance comparison and current challenges of using machine learning techniques in cybersecurity. Energies 13 (10): 27. https://doi.org/10.3390/en13102509 .
Sheehan, B., F. Murphy, M. Mullins, and C. Ryan. 2019. Connected and autonomous vehicles: A cyber-risk classification framework. Transportation Research Part a: Policy and Practice 124: 523–536. https://doi.org/10.1016/j.tra.2018.06.033 .
Sheehan, B., F. Murphy, A.N. Kia, and R. Kiely. 2021. A quantitative bow-tie cyber risk classification and assessment framework. Journal of Risk Research 24 (12): 1619–1638.
Shlomo, A., M. Kalech, and R. Moskovitch. 2021. Temporal pattern-based malicious activity detection in SCADA systems. Computers & Security 102: 17. https://doi.org/10.1016/j.cose.2020.102153 .
Singh, K.J., and T. De. 2020. Efficient classification of DDoS attacks using an ensemble feature selection algorithm. Journal of Intelligent Systems 29 (1): 71–83. https://doi.org/10.1515/jisys-2017-0472 .
Skrjanc, I., S. Ozawa, T. Ban, and D. Dovzan. 2018. Large-scale cyber attacks monitoring using Evolving Cauchy Possibilistic Clustering. Applied Soft Computing 62: 592–601. https://doi.org/10.1016/j.asoc.2017.11.008 .
Smart, W. 2018. Lessons learned review of the WannaCry Ransomware Cyber Attack. https://www.england.nhs.uk/wp-content/uploads/2018/02/lessons-learned-review-wannacry-ransomware-cyber-attack-cio-review.pdf . Accessed 7 May 2021.
Sornette, D., T. Maillart, and W. Kröger. 2013. Exploring the limits of safety analysis in complex technological systems. International Journal of Disaster Risk Reduction 6: 59–66. https://doi.org/10.1016/j.ijdrr.2013.04.002 .
Sovacool, B.K. 2008. The costs of failure: A preliminary assessment of major energy accidents, 1907–2007. Energy Policy 36 (5): 1802–1820. https://doi.org/10.1016/j.enpol.2008.01.040 .
SpringerLink. 2021. Journal Search. https://rd.springer.com/search?facet-content-type=%22Journal%22 . Accessed 11 May 2021.
Stojanovic, B., K. Hofer-Schmitz, and U. Kleb. 2020. APT datasets and attack modeling for automated detection methods: A review. Computers & Security 92: 19. https://doi.org/10.1016/j.cose.2020.101734 .
Subroto, A., and A. Apriyana. 2019. Cyber risk prediction through social media big data analytics and statistical machine learning. Journal of Big Data . https://doi.org/10.1186/s40537-019-0216-1 .
Tan, Z., A. Jamdagni, X. He, P. Nanda, R.P. Liu, and J. Hu. 2015. Detection of denial-of-service attacks based on computer vision techniques. IEEE Transactions on Computers 64 (9): 2519–2533. https://doi.org/10.1109/TC.2014.2375218 .
Tidy, J. 2021. Irish cyber-attack: Hackers bail out Irish health service for free. https://www.bbc.com/news/world-europe-57197688 . Accessed 6 May 2021.
Tuncer, T., F. Ertam, and S. Dogan. 2020. Automated malware recognition method based on local neighborhood binary pattern. Multimedia Tools and Applications 79 (37–38): 27815–27832. https://doi.org/10.1007/s11042-020-09376-6 .
Uhm, Y., and W. Pak. 2021. Service-aware two-level partitioning for machine learning-based network intrusion detection with high performance and high scalability. IEEE Access 9: 6608–6622. https://doi.org/10.1109/ACCESS.2020.3048900 .
Ulven, J.B., and G. Wangen. 2021. A systematic review of cybersecurity risks in higher education. Future Internet 13 (2): 1–40. https://doi.org/10.3390/fi13020039 .
Vaccari, I., G. Chiola, M. Aiello, M. Mongelli, and E. Cambiaso. 2020. MQTTset, a new dataset for machine learning techniques on MQTT. Sensors 20 (22): 17. https://doi.org/10.3390/s20226578 .
Valeriano, B., and R.C. Maness. 2014. The dynamics of cyber conflict between rival antagonists, 2001–11. Journal of Peace Research 51 (3): 347–360. https://doi.org/10.1177/0022343313518940 .
Varghese, J.E., and B. Muniyal. 2021. An Efficient IDS framework for DDoS attacks in SDN environment. IEEE Access 9: 69680–69699. https://doi.org/10.1109/ACCESS.2021.3078065 .
Varsha, M. V., P. Vinod, K.A. Dhanya. 2017 Identification of malicious android app using manifest and opcode features. Journal of Computer Virology and Hacking Techniques 13 (2): 125–138. https://doi.org/10.1007/s11416-016-0277-z
Velliangiri, S., and H.M. Pandey. 2020. Fuzzy-Taylor-elephant herd optimization inspired Deep Belief Network for DDoS attack detection and comparison with state-of-the-arts algorithms. Future Generation Computer Systems—the International Journal of Escience 110: 80–90. https://doi.org/10.1016/j.future.2020.03.049 .
Verma, A., and V. Ranga. 2020. Machine learning based intrusion detection systems for IoT applications. Wireless Personal Communications 111 (4): 2287–2310. https://doi.org/10.1007/s11277-019-06986-8 .
Vidros, S., C. Kolias, G. Kambourakis, and L. Akoglu. 2017. Automatic detection of online recruitment frauds: Characteristics, methods, and a public dataset. Future Internet 9 (1): 19. https://doi.org/10.3390/fi9010006 .
Vinayakumar, R., M. Alazab, K.P. Soman, P. Poornachandran, A. Al-Nemrat, and S. Venkatraman. 2019. Deep learning approach for intelligent intrusion detection system. IEEE Access 7: 41525–41550. https://doi.org/10.1109/access.2019.2895334 .
Walker-Roberts, S., M. Hammoudeh, O. Aldabbas, M. Aydin, and A. Dehghantanha. 2020. Threats on the horizon: Understanding security threats in the era of cyber-physical systems. Journal of Supercomputing 76 (4): 2643–2664. https://doi.org/10.1007/s11227-019-03028-9 .
Web of Science. 2021. Web of Science: Science Citation Index Expanded. https://clarivate.com/webofsciencegroup/solutions/webofscience-scie/ . Accessed 11 May 2021.
World Economic Forum. 2020. WEF Global Risk Report. http://www3.weforum.org/docs/WEF_Global_Risk_Report_2020.pdf . Accessed 13 May 2020.
Xin, Y., L. Kong, Z. Liu, Y. Chen, Y. Li, H. Zhu, M. Gao, H. Hou, and C. Wang. 2018. Machine learning and deep learning methods for cybersecurity. IEEE Access 6: 35365–35381. https://doi.org/10.1109/ACCESS.2018.2836950 .
Xu, C., J. Zhang, K. Chang, and C. Long. 2013. Uncovering collusive spammers in Chinese review websites. In Proceedings of the 22nd ACM international conference on Information & Knowledge Management.
Yang, J., T. Li, G. Liang, W. He, and Y. Zhao. 2019. A Simple recurrent unit model based intrusion detection system with DCGAN. IEEE Access 7: 83286–83296. https://doi.org/10.1109/ACCESS.2019.2922692 .
Yuan, B.G., J.F. Wang, D. Liu, W. Guo, P. Wu, and X.H. Bao. 2020. Byte-level malware classification based on Markov images and deep learning. Computers & Security 92: 12. https://doi.org/10.1016/j.cose.2020.101740 .
Zhang, S., X.M. Ou, and D. Caragea. 2015. Predicting cyber risks through national vulnerability database. Information Security Journal 24 (4–6): 194–206. https://doi.org/10.1080/19393555.2015.1111961 .
Zhang, Y., P. Li, and X. Wang. 2019. Intrusion detection for IoT based on improved genetic algorithm and deep belief network. IEEE Access 7: 31711–31722.
Zheng, Muwei, Hannah Robbins, Zimo Chai, Prakash Thapa, and Tyler Moore. 2018. Cybersecurity research datasets: taxonomy and empirical analysis. In 11th {USENIX} workshop on cyber security experimentation and test ({CSET} 18).
Zhou, X., W. Liang, S. Shimizu, J. Ma, and Q. Jin. 2021. Siamese neural network based few-shot learning for anomaly detection in industrial cyber-physical systems. IEEE Transactions on Industrial Informatics 17 (8): 5790–5798. https://doi.org/10.1109/TII.2020.3047675 .
Zhou, Y.Y., G. Cheng, S.Q. Jiang, and M. Dai. 2020. Building an efficient intrusion detection system based on feature selection and ensemble classifier. Computer Networks 174: 17. https://doi.org/10.1016/j.comnet.2020.107247 .
Download references
Open Access funding provided by the IReL Consortium.
Author information
Authors and affiliations.
University of Limerick, Limerick, Ireland
Frank Cremer, Barry Sheehan, Arash N. Kia, Martin Mullins & Finbarr Murphy
TH Köln University of Applied Sciences, Cologne, Germany
Michael Fortmann & Stefan Materne
You can also search for this author in PubMed Google Scholar
Corresponding author
Correspondence to Barry Sheehan .
Ethics declarations
Conflict of interest.
On behalf of all authors, the corresponding author states that there is no conflict of interest.
Additional information
Publisher's note.
Springer Nature remains neutral with regard to jurisdictional claims in published maps and institutional affiliations.
Supplementary Information
Below is the link to the electronic supplementary material.
Supplementary file1 (PDF 334 kb)
Supplementary file1 (docx 418 kb), rights and permissions.
Open Access This article is licensed under a Creative Commons Attribution 4.0 International License, which permits use, sharing, adaptation, distribution and reproduction in any medium or format, as long as you give appropriate credit to the original author(s) and the source, provide a link to the Creative Commons licence, and indicate if changes were made. The images or other third party material in this article are included in the article's Creative Commons licence, unless indicated otherwise in a credit line to the material. If material is not included in the article's Creative Commons licence and your intended use is not permitted by statutory regulation or exceeds the permitted use, you will need to obtain permission directly from the copyright holder. To view a copy of this licence, visit http://creativecommons.org/licenses/by/4.0/ .
Reprints and permissions
About this article
Cremer, F., Sheehan, B., Fortmann, M. et al. Cyber risk and cybersecurity: a systematic review of data availability. Geneva Pap Risk Insur Issues Pract 47 , 698–736 (2022). https://doi.org/10.1057/s41288-022-00266-6
Download citation
Received : 15 June 2021
Accepted : 20 January 2022
Published : 17 February 2022
Issue Date : July 2022
DOI : https://doi.org/10.1057/s41288-022-00266-6
Share this article
Anyone you share the following link with will be able to read this content:
Sorry, a shareable link is not currently available for this article.
Provided by the Springer Nature SharedIt content-sharing initiative
- Cyber insurance
- Systematic review
- Cybersecurity
- Find a journal
- Publish with us
- Track your research
- Reference Manager
- Simple TEXT file
People also looked at
Original research article, psychological profiling of hackers via machine learning toward sustainable cybersecurity.

- 1 College of Computing and Information Sciences, Karachi Institute of Economics and Technology, Karachi, Pakistan
- 2 School of Business, American University of Ras Al Khaimah, Ras Al Khaimah, United Arab Emirates
- 3 Department of Computer Science, University of Technology Sydney, Sydney, NSW, Australia
- 4 College of Humanities and Social Sciences, Libraries and Information Department, Princess Nourah Bint Abdulrahman University, Riyadh, Saudi Arabia
This research addresses a challenge of the hacker classification framework based on the “big five personality traits” model (OCEAN) and explores associations between personality traits and hacker types. The method's application prediction performance was evaluated in two groups: Students with hacking experience who intend to pursue information security and ethical hacking and industry professionals who work as White Hat hackers. These professionals were further categorized based on their behavioral tendencies, incorporating Gray Hat traits. The k-means algorithm analyzed intra-cluster dependencies, elucidating variations within different clusters and their correlation with Hat types. The study achieved an 88% accuracy in mapping clusters with Hat types, effectively identifying cyber-criminal behaviors. Ethical considerations regarding privacy and bias in personality profiling methodologies within cybersecurity are discussed, emphasizing the importance of informed consent, transparency, and accountability in data management practices. Furthermore, the research underscores the need for sustainable cybersecurity practices, integrating environmental and societal impacts into security frameworks. This study aims to advance responsible cybersecurity practices by promoting awareness and ethical considerations and prioritizing privacy, equity, and sustainability principles.
1 Introduction
The rise of the Internet has led to a corresponding surge in cybercrime instances, as computers have become integral to various facets of life, including commerce, entertainment, and government operations ( Siddiqi et al., 2022 ). Additionally, the emergence of novel networking models such as mobile, wireless, cognitive, mesh, Internet of Things (IoT), and cloud technologies has further complicated the landscape of cybersecurity ( Islam and Shaikh, 2016 ; Tandera et al., 2017 ; Wong et al., 2020 ). This evolving scenario poses significant challenges in combating cyber threats. Cybercrime utilizes computers as tools and mediums for criminal activities, targeting security objectives such as privacy, confidentiality, availability, and integrity of information. Common cyber crimes encompass phishing, honeypots, social engineering, spoofing, and disseminating viruses or worms.
The discussion on cybercrimes highlights previous research findings indicating that these activities are primarily carried out by individuals with low technical sophistication and are driven by motivations such as fame, financial gain, revenge, and self-satisfaction ( John et al., 1999 ; Gulati et al., 2016 ; Buch et al., 2017 ; Matulessy and Humaira, 2017 ; Suryapranata et al., 2017 ). Hacking, a specialized form of cybercrime, involves illegally accessing personal or sensitive data using technology and knowledge, with various countermeasures such as firewalls and intrusion detection systems in place to mitigate such threats ( Gulati et al., 2016 ; Akdag, 2020 ). Building on this understanding, the concept of “sustainable cybersecurity” is introduced in this study, emphasizing the need for enduring and efficient strategies to adapt to the evolving challenges posed by hackers ( Shackelford et al., 2016 ; Medoh and Telukdarie, 2022 ). This approach aligns with corporate social responsibility (CSR) practices, with an increasing number of managers recognizing cybersecurity as integral to safeguarding customers and the public, thereby expanding risk management practices to encompass the prevention of social-engineering-linked attacks ( Shackelford et al., 2016 ; Medoh and Telukdarie, 2022 ).
In this study, the term “sustainable” indicates the formulation of enduring and efficient cybersecurity strategies. Within this framework, sustainability encompasses the creation of practices, methodologies, and tools capable of persisting and adjusting over time to adeptly confront the continuously evolving challenges presented by hackers. The concept of “Sustainable Cybersecurity” suggests implementing robust and resilient defense mechanisms designed not only to react to existing threats but also to foresee and alleviate potential risks. For instance, social engineering, which focuses on exploiting human psychology to both perpetrate and prevent cyberattacks, diverges from relying solely on technical hacking methods. It is associated with attacks such as phishing emails, deepfakes, and spear phishing ( Siddiqi et al., 2022 ). This field also underscores the application of social psychology to reinforce cybersecurity policies within organizations. Tools such as the Cyber Risk Index (CRI) and the Cybercrime Rapid Identification Tool (CRIT) ( Buch et al., 2017 ) can be implemented and utilized to bolster this approach. The gap between “social engineering” linked attacks and their avoidance measures creates an ongoing challenge for security experts. Therefore, security through technology is not the sole solution; it is the much-needed side to sustain the cyber security world. Even the World Economic Forum declares social engineering cyber-attacks as the reason for organizations' alarming security situation.
This study is grounded in research utilizing data collected from personality trait rating scales ( Buch et al., 2017 ; Novikova and Alexandra, 2019 ; Wong et al., 2020 ), with Matulessy and Humaira (2017) providing insight into hacker personality profiles based on the Big Five Personality Traits model. The aim is to construct a machine learning model capable of predicting and analyzing the personality profiles of hackers, utilizing the Big Five personality model and validating its reliability. Understanding the psychology or personality of hackers is essential for implementing effective preventive measures ( Javaid, 2013 ; Ali et al., 2020 ). The research inquiry addresses the dominant personality traits (openness to experience, conscientiousness, extraversion, agreeableness, and neuroticism) abbreviated as OCEAN that are exhibited by various hacker types (White, Black, and Gray Hats) and how these traits can be accurately identified and categorized through a machine learning-based approach. This identification mechanism holds promise for informing targeted cybercrime prevention strategies. Figure 1 illustrates the research flow and target, detailing a secure model for predicting personality traits. The authors devised a questionnaire based on the OCEAN model and applied machine learning models to classify hacker types.
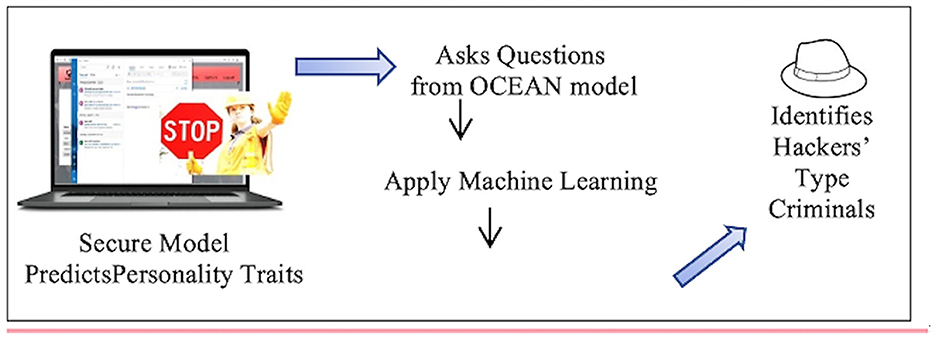
Figure 1 . Research flow and target.
Different sections in this article are as follows. The section covers relevant literature, Section 3 covers the research method, Section 4 covers experimentation and results of the secure model, Section 5 covers discussions on results and threats to validity, and Section 6 concludes the study and highlights potential future work.
2 Literature review
In today's technologically advancing world, cybercrimes are on the rise. This section discusses targeted studies published previously to investigate contemporary cybercriminal acts.
In the realm of cybersecurity research, many studies have leveraged machine learning methodologies to delve into the intricacies of cybercrime data analysis. Concurrently, Geluvaraj et al. (2019) have tackled prevalent cybersecurity challenges, proposing innovative machine-learning solutions for their mitigation. Drawing from diverse machine learning techniques, Zheng et al. (2003) have unraveled concealed patterns within crime data, underscoring the indispensability of data-driven approaches in cybercrime investigations. Meanwhile, Islam et al. (2021) have explored the transformative potential of artificial intelligence and deep learning in bolstering cybersecurity frameworks. Adewumi and Akinyelu (2017) have harnessed machine learning algorithms to discern distinctive authorship patterns and shed light on attributing illicit messages in cyberspace. Pastrana et al. (2018) proposed a comprehensive approach to counter the spread of fake news online, leveraging machine learning technologies. This effort aligns with the rise of blockchain technology, which has become a cornerstone in fortifying applications across mobile and cloud networks, exemplified by the study by Mohammed et al. (2023) and Tamboli et al. (2023) . Furthermore, pioneering approaches, such as the Low-Latency and High-Throughput Multipath routing technique, as elucidated by Ramachandran et al. (2022) , have been devised to counter novel threats such as black hole attacks. Meanwhile, Imran et al. (2019) have employed machine learning and nature-inspired algorithms to scrutinize credit card data, fortifying fraud prevention measures. Against this backdrop, integrating artificial intelligence, machine learning, and IoT technologies has heralded a new era in cybercrime analysis and cybersecurity enhancement, a paradigm eloquently underscored by Sood and Enbody (2013) and epitomized in the broader research landscape.
Bridging the gap between psychology and information security, investigations by Del Pozo et al. (2018) and Chayal and Patel (2021) have illuminated the psychological underpinnings crucial to fortifying cyber defenses. Suryapranata et al. (2017) studied the activities of a user forum to identify the variables that can be used to predict the likelihood of a user being involved in cybercrime. An intervention can benefit in avoiding a crime. The study reports users in an underground forum as providers, advertisers, and buyers ( Fox and Holt, 2021 ). Alashti et al. (2022) employed logistic regression and latent class analysis to identify risk factors associated with juvenile hacking. Odemis et al. (2022) observed the behaviors of Iranian hackers via interviews. It was found that young hackers enjoyed the pleasure of cybercrime. Back et al. (2019) addresses whether we can analyze the psychology and behavior of a hacker by investigating their computer logs. A honeypot system was created for this purpose. Suryapranata et al. (2017) built profiles of cybercriminals by analyzing court records and media documents for incidents in South Korea. It was found that there is a difference in motivation between young and adult hackers.
The hidden Markov Model has been used in various studies to identify the personality traits of cybercriminals over social media networks ( Xie and Wei, 2022 ). The method comprises a training and identification phase. The average likelihood of the observation sequence is performed in the identification phase. The text information posted by users over social media, blogs, and language characteristics can be analyzed using neural networks, logistic regression, and support vector machines for personality analysis ( Golbeck et al., 2011 ; Adali and Golbeck, 2012 ; Lima and De Castro, 2014 ).
Novikova and Alexandra (2019) discuss the Five Factor Model in detail. The Five Factor Model suggests that all people, regardless of their age, gender, or culture, share some essential traits, but every person differs in their degree of manifestation. John et al. (1999) discuss the result of an eight-item Cybercrime Rapid Identification Tool (CRIT). It evaluates the psychometric properties of the proposed scale on samples of secondary school and university students. A study on Personality Prediction Systems from Facebook Users attempts to build a system to predict a person's personality based on user information ( Buch et al., 2017 ). The research mentioned in the above studies discusses cybercrimes in general. This includes the essential five personality traits all humans are divided into, the tool for identifying Cybercrimes, and especially the personality profiles of the hackers.
In the realm of hacker classification, researchers often employ a framework akin to the concept of White, Black, and Gray Hats ( Buch et al., 2017 ). White Hat Hackers, the first category, embody ethical hacking practices. Despite engaging in illegal activities, they channel their skills toward constructive and positive ends, often for the betterment of security systems. Contrastingly, Black Hat Hackers, the second category, operate with nefarious intent, breaching security measures for personal gain. Their activities typically involve theft, exploitation, and the illicit sale of data driven by self-interest. Gray Hat Hackers constitute the third category, occupying a space between the ethical and the malicious. While they may identify and exploit vulnerabilities, their actions are not motivated by financial gain. However, their endeavors still fall within the realm of illegality, as they typically lack consent from the system's owner. Gray Hats often have associations with Black Hat hackers, blurring the lines between ethical and unethical practices. In another perspective Javaid (2013) offered, Gray Hats are portrayed as reformed Black Hats. These individuals, often independent security experts, consultants, or corporate researchers, transition from illicit activities to a more legitimate stance. Notable figures such as Kevin Mitnick exemplify this transformation.
In summary, the delineation between White, Black, and Gray Hats provides a nuanced understanding of hacker motivations and behaviors, shedding light on the spectrum between ethical and malicious hacking practices. Each of the three types of hackers utilizes their skills for different purposes. The previous research defines that each possesses other personality profiles regarding Big Five Personality Traits (OCEAN Model). The research study conducted by Matulessy and Humaira (2017) described the personality profiles of the hackers concerning the Big Five Personality Traits model using 30 hacker subjects and utilized descriptive qualitative research.
The research claims that hackers are positioned in the middle of the personality trait of extraversion regardless of the categories of hackers. White Hats have more dominant personality traits of agreeableness, and Black Hats have more dominant personality traits of openness to experience. In contrast, Gray Hats have more dominant personality traits in terms of neuroticism (see Table 1 ). Table 2 presents the summary of the previous research.
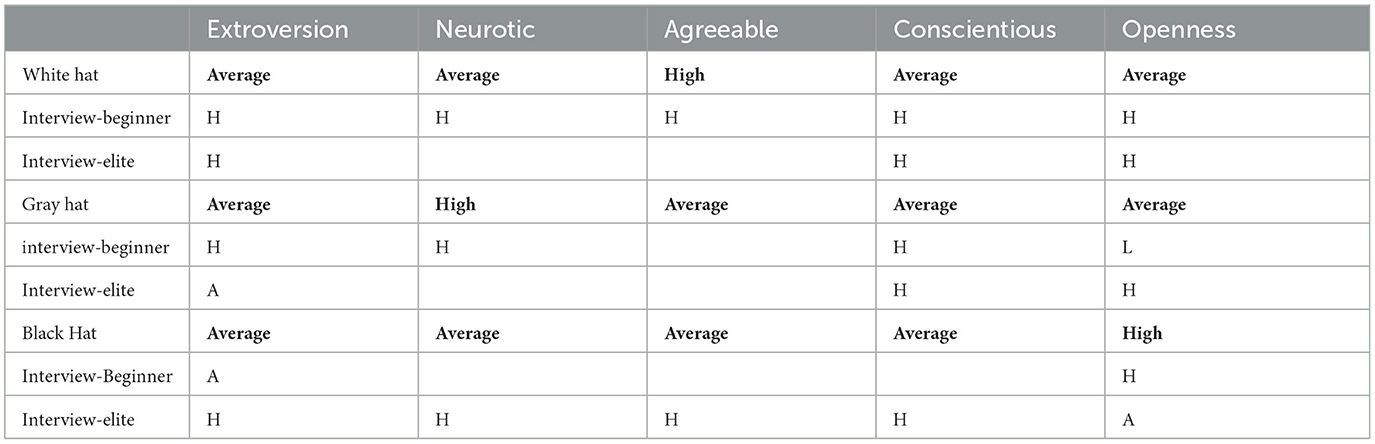
Table 1 . OCEAN traits claimed in earlier research ( Matulessy and Humaira, 2017 ).
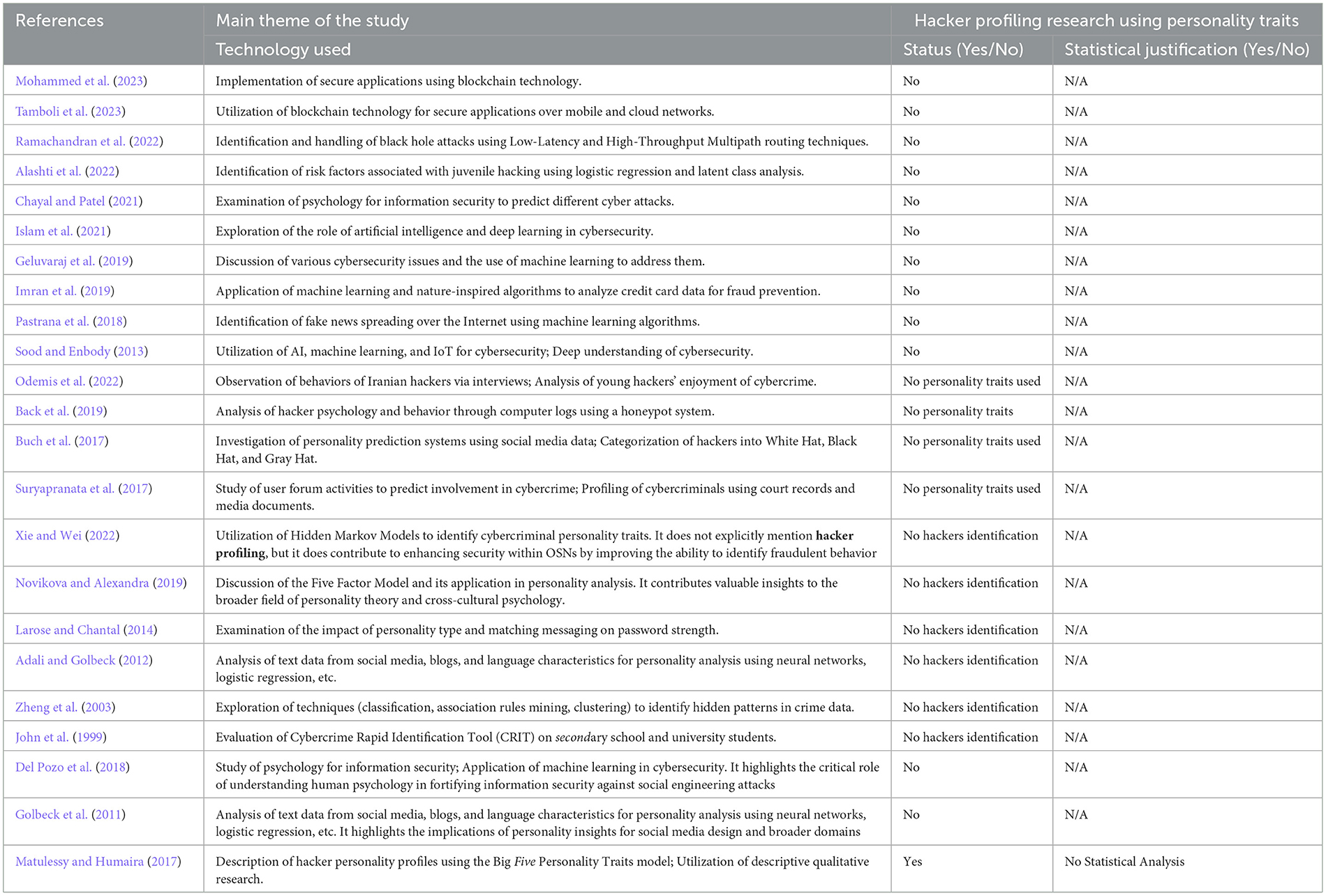
Table 2 . Summary of previous research and hacker profiling gap analysis.
Previous researchers have primarily focused on broad aspects of cybercrime identification and personality prediction. While some studies have explored personality prediction systems utilizing social media platforms ( Buch et al., 2017 ), others have theorized based on research findings obtained from personality trait rating scales, interviews, surveys, and questionnaires ( Matulessy and Humaira, 2017 ; Novikova and Alexandra, 2019 ). However, the scope of investigation in these studies remains somewhat limited, predominantly addressing general trends rather than delving into nuanced aspects of cybercriminal behavior and personality profiling.
This research implements a machine learning-based model that predicts and analyzes the personality profiles of hackers using the Big Five personality model, and it also validates the model on real-life datasets. This study mainly targets White and Gray hackers who either use hacking as their profession or have career motivation to adopt it professionally. For detailed classification, refer to the study by Martineau et al. (2023) and Chng et al. (2022) w.r.t hacker type, their possible motivations, and personality type.
3 Research methodology
Figure 2 shows the research method adopted in this study ( McAlaney et al., 2020 ; Bakas et al., 2021 ). This study uses a machine learning-based approach to validate the classification of different hacker types (White, Black, and Gray Hats) based on their dominant personality traits (openness to experience, conscientiousness, extraversion, agreeableness, and neuroticism).
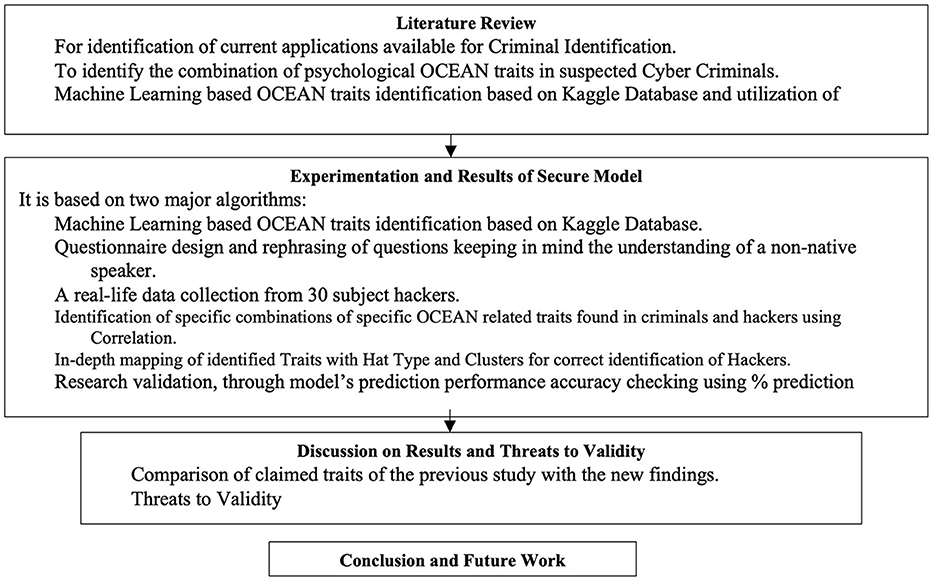
Figure 2 . Research method.
The K-means algorithm was chosen for its simplicity, ease of implementation, and speed. It is widely used for clustering tasks, including in high-volume datasets such as those associated with criminal data, as described by Aldhyani and Alkahtani (2022) . K-means can generate clusters based on similarities in the data, aiming to group data points close to each other while being far from points in other clusters. This study applied K-means to cluster individuals based on their responses to personality trait questions. By clustering individuals with similar personality trait profiles together, the algorithm aids in identifying distinct groups or “clusters” that may correspond to different types of hackers based on their dominant personality traits. The study also seeks to develop an effective hacker identification mechanism that can accurately categorize these traits and contribute to developing targeted cybercrime prevention strategies related to social policies.
The Big Five Inventory is a 44-item inventory that measures an individual on the Big Five Factors (dimensions or traits) of personality. Each of the five factors is then further divided into personality characteristics. The inventory shares some questions for generic OCEAN personality traits. These are given in the dataset of Kaggle ( Akdag, 2020 ). A reduced set of questions was used from the Five Personality questionnaire comprising 40 questions ( Akdag, 2020 ). The users were asked to indicate their favorable responses to the questionnaire items by selecting an appropriate score. After collecting the questions' responses, a machine learning code runs and predicts results against all five personality traits. Based on the results, different hacker types are identified.
3.1 Research questionnaire
There are several instruments to measure the Big Five Trait Factors, such as the Big Five Inventory (BFI), the NEO Personality Inventory-Revised (NEO-PI-R), and the International Personality Item Tool (IPIP). This study used the dataset constructed from the IPIP for our research. This dataset was collected (2016–2018) through an interactive online personality test and comprises 10,12,050 records ( Akdag, 2020 ). The training dataset trains the clustering model for OCEAN trait prediction.
Following the points concluded in the research mentioned in Table 1 , 21 questions were selected. Redundant reverse questions were not included to reduce user frustration. In the questionnaire development process, the reverse questions are normally designed to verify the authenticity of answers recorded by random users.
Suárez Álvarez et al. (2018) demonstrated the conventional way of handling reverse coding. Here, the reduction was made for all reverse-scored questions included in the 40 questions. These were negatively phrased to ensure the user knew his point of view. Including such questions requires reserve scoring. The negative consequences of using the reverse scoring include a) the flawed measurement precision of the instrument, b) the variance of the combined form is reduced, c) examinees' scores differ significantly from those obtained in tests where all of the items are of a similar form, and d) verbal skills influence examinees' responses ( Suárez Álvarez et al., 2018 ). Minor changes in wording can also have a significant effect on responses. One should, therefore, be careful when looking at alternative wordings. Negative words such as “not” should be avoided in questions as respondents easily miss them. In addition, using “not” in a scale such as “Satisfied,” “Neither,” and “Not satisfied” does not provide a true opposite as defined by the Australian Statistic Bureau ( Corallo et al., 2022 ). The questions were then rephrased from native English speakers' style into a more understandable one for non-native speakers. See Table 3 for a detailed set of questions used in this study.
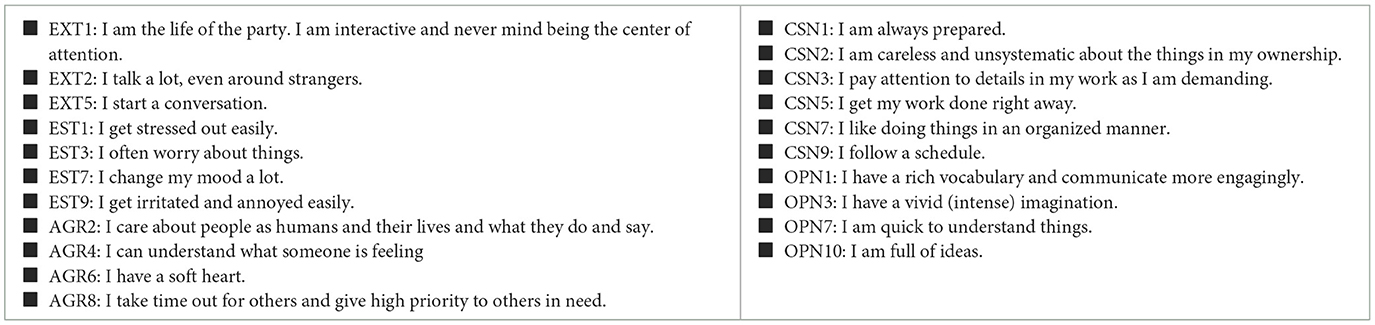
Table 3 . Research questions with no reverse questions.
3.2 Questionnaire reliability
The questionnaire's accuracy and precision, i.e., its internal validity (consistency) or reliability, have been checked using Cronbach's alpha score. Its value was reported as 0.874 in previous research ( Matulessy and Humaira, 2017 ) on 40 questions. In this research's reduced set of 21 questions, its value is 0.82, which shows acceptable reliability, i.e., >0.7. It always gives the same results when applied to the same group at different times or circumstances ( Matulessy and Humaira, 2017 ).
4 Experimentation of results of secure model
4.1 algorithms used and experimentation.
The research experimentation is based on two algorithms:
1. The machine learning-based OCEAN traits identification dataset is from Kaggle, developed by Akdag (2020) .
2. Identification of specific OCEAN traits-related combinations found in criminals and hackers.
The experiment starts with creating an optimal number of clusters on the training dataset downloaded from Kaggle ( Akdag, 2020 ). The k-means algorithm generates clusters (groups of similar data) because of its ease of implementation, simplicity, and speed, which is very appealing in practice. This has been described in detail by Aldhyani and Alkahtani (2022) , who targeted the classification of criminal data. According to the study, K-means is suitable for high-volume crime datasets and can help to extract useful information.
K-means applied in this research is a complete, partitioned clustering technique that attempts to find user-specified clusters (K) represented by their centroids. The distance between any two points in different groups is larger than the distance between any two points within a group. Well-separated clusters do not need to be spherical but can have any shape ( Tan et al., 2016 ).
Figure 3 shows methods Python uses to calculate an optimum cluster value. The KElbowVisualizer or elbow method selects the optimal number of clusters by fitting the model with a range of values for K, which shows that the calculated value of K is 6. The Silhouette coefficient method is used to know the truth about the dataset by computing the density of clusters. This produces a score between 1 and −1, where 1 is a highly dense cluster and −1 is a completely incorrect cluster. Here, the value is approximately 0.06, which shows that the number of clusters in this research is dense and thus correct.
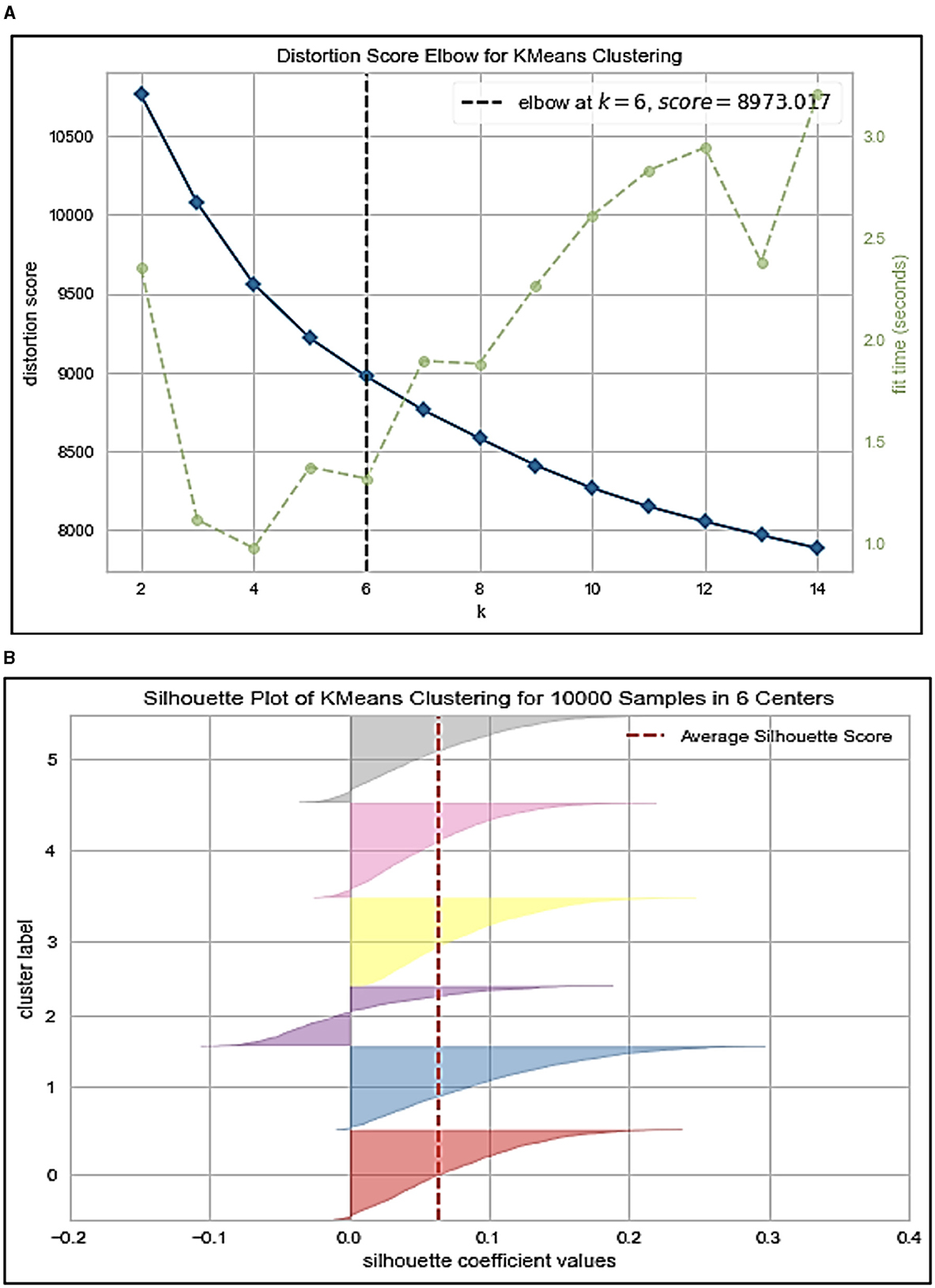
Figure 3 . Optimal cluster number. (A) KElbowVisualizar; (B) Silhouette coefficient.
After clustering the training dataset on 6 clusters (0–5), the score of the five personality traits is calculated individually based on the responses to the questions. Then, the system is trained to predict the cluster for each dataset and calculate each trait's score respectively (see Figure 4 ). Figure 4 shows how many datasets were assigned to identify each cluster; even the worst count shows 6,200 records.
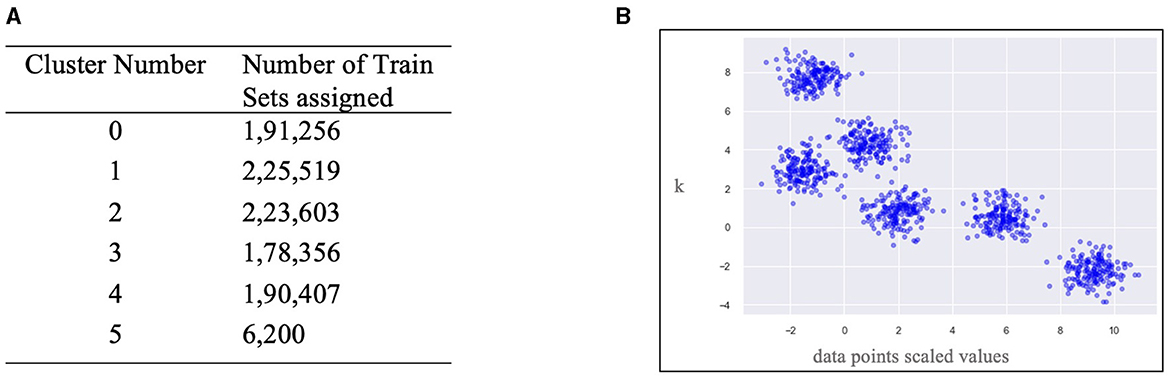
Figure 4 . Cluster's centers picked by K-means Python estimator. (A) Training data distribution across calculated clusters. (B) Train data points spread across calculated clusters.
4.2 Scoring values to identify hackers
The same technique is applied to the responses taken from the test datasets, which were collected on 21 questions and converted into responses. According to the user's responses, the system calculates the score of each personality trait. It determines the cluster where the user belongs to three types of hackers who have one most dominant personality trait among all. In previous research ( Matulessy and Humaira, 2017 ), as shown in Table 1 , for OCEAN traits, the generalized most dominant traits are agreeableness for the White hacker, openness to experience for the Black hacker, and neuroticism for the Gray Hackers. If any of these traits have the maximum score among all four traits, there is a strong possibility that the person can be a hacker or have any illegal intentions.
4.3 Organizational preventive measures
If the user is found to be suspicious, the system temporarily holds that user on a “watch list” before granting further access to the site or organizational sensitive resources. The organization can add its name to the social policy list to use resources under organizational or web access monitoring software. As mentioned earlier, proper social security and communication policies should be designed based on identified “social psychology” and Crime Risk Index, as suggested by Siddiqi et al. (2022) , or Cybercrime Rapid Identification Tool (CRIT), as suggested by Buch et al. (2017) , must be maintained to differentiate naïve users from the one who can harm other colleagues or employer organization.
5 Validation of secure model
Rather than blindly implementing clusters on previous research claims ( Matulessy and Humaira, 2017 ), its proper validation is performed on (a) average scores as well as on (b) clusters using a prediction performance accuracy measurement of machine learning ( Matulessy and Humaira, 2017 ). In validating the secure model, several techniques are applied to ensure the reliability and accuracy of the model's predictions. These include comparing average scores of the test dataset with established category claims for hacker types, validating clustering outcomes through cluster predictions on the test dataset, analyzing correlations between personality traits using Spearman's rho, quantitatively measuring model performance, examining demographic information, and mapping clusters to hacker types based on observed traits. Collectively, these validation techniques ensure the effectiveness and robustness of the model in identifying hacker types based on personality traits.
5.1 Data collection for test set
Test data were collected reliably for a major research project to gather personality traits data across various professional domains in computer science. These data were used to develop a career counseling system for final-year students in higher education institutions. It included responses from final-year students and professionals in domains such as information security, such as hackers, auditors, trainers, and security administrators. The response rate was highly encouraging. Out of 300 records, around 32 were related to hackers, with 30 ultimately included after data cleaning. This aligns with validation criteria from previous psychology research. Despite the lack of progressive research in hacker personality detection, the study aimed to contribute positively to career counseling. The data collection process was based on high trust, as participants and the research team belonged to the same information security professionals community. Data collected from final-year students were deemed reliable due to their field of interest and relevant academic projects noted during their tenure.
5.2 Demographics on test data
Demographics and frequency scores of the collected dataset for Gray and White hackers are given in Table 4 . The total respondents for this study were 30 professionals and final-year students who intend to adopt hacking as their profession. The majority of them were male respondents, whereas only two were female respondents. Table 5 shows all possible details of the collected test dataset.

Table 4 . Hacker type, motivations, and common strategies.
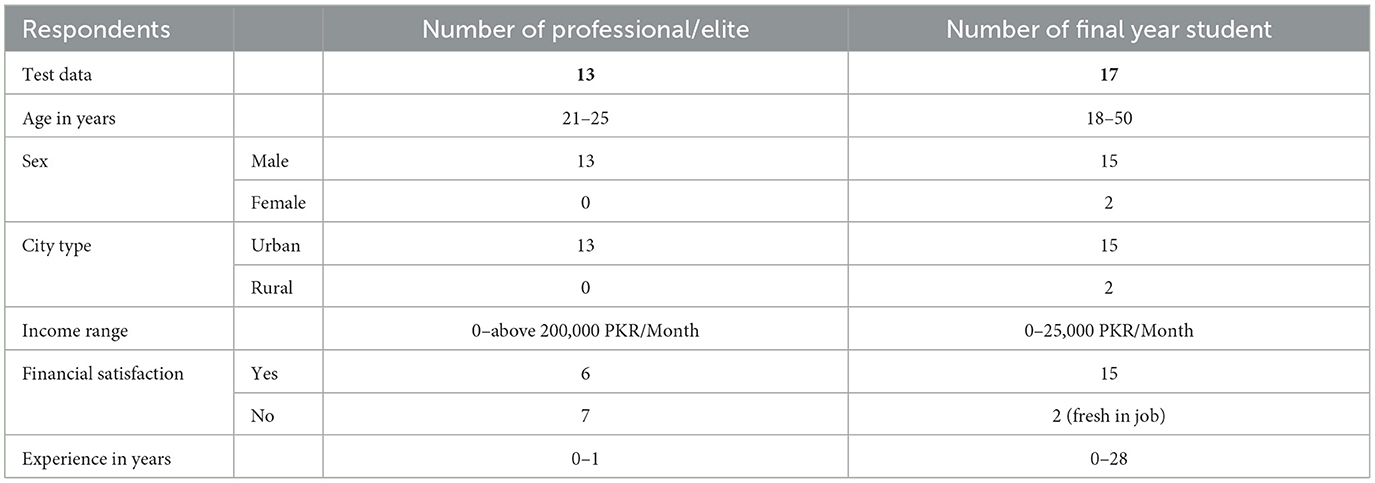
Table 5 . Number of test datasets and their demographics.
5.3 Cluster trends on test dataset with hat type mapping for validation
First, the test dataset was given as an input in the clustering algorithm generated, as discussed in Section 4, and clusters were predicted on all datasets. The cluster distribution will be discussed in detail in later sections.
To better understand the cluster trends and establish their mapping with Hat types, there was a need to consider the correlation between the traits on test data. Correlation is a statistical measure that measures the extent to which two variables are in a linear relationship without calculating cause and effect. This means they constantly change; when one changes, the other also changes. It is measured on a scale of −1/0/+1, which means an indirect, no, or a direct relationship. For the stated reason, Spearman's correlation was applied to the test dataset (see Table 6 ), showing a few other significant but moderate level inter-dependencies between traits in the correlation coefficient range +/– 0.4.
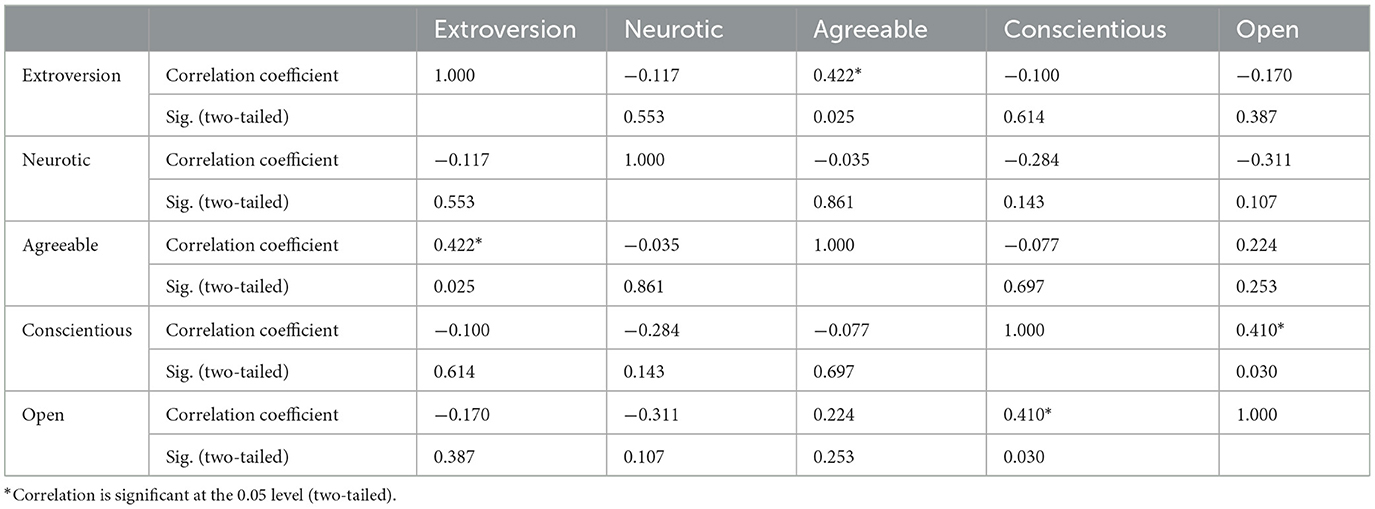
Table 6 . Spearman's rho correlation checking to make multiple trait-based Hat-type selections.
These visible correlations can be generalized as given by Matulessy and Humaira (2017) :
a. The openness to experience keeps conscientiousness closer. High openness was claimed to be the major trait of Black Hats.
b. Neuroticism depends directly on no other traits. High neuroticism was claimed to be the major trait of Gray Hats.
c. Agreeableness keeps extroversion closer. High agreeable was claimed to be the major trait of White Hats.
Figure 5 graphically shows all 6 clusters with average score values and reflects apparent behavior across each cluster on both training and test datasets. It is visible from the two graphs that the K-means clustering algorithm does not just check the average score values while designating the cluster numbers but also reflects intra-cluster trends within specific clusters. Following are the conclusions to map the cluster numbers with the Hat types purely over hacker's data:
1. The first cluster, “Cluster-0,” shows the same trend among both datasets. Still, the training dataset has shown less sense of taking creative challenges by the White Hats because their job demands carefully defined method's adaptation. In this combination, the highest level starts with an extra high level of neuroticism, then comes a high level of agreeableness, conscientiousness, openness, and the last somewhat above-average level of extroversion.
• Conclusion: “Cluster-0” represents Gray Hats with the highest neuroticism; therefore, it does not depend on other traits.
2. The second cluster, which is “Cluster-1,” is closer to the first cluster but has high neuroticism and agreeableness.
• Conclusion: “Cluster-0” represents a switching behavior of White Hats with a Gray Hat tendency. White has the highest level of agreeableness but also has a high level of neuroticism; therefore, it does not depend on any other traits.
3. The third cluster, “Cluster-2,” is closer to the fourth cluster but has an average level of neuroticism.
• Conclusion: “Cluster-2” represents White Hats with average neurotic tendencies and with high agreeableness and average values of extroversion.
4. The fifth cluster, “Cluster-4,” shows the same trend captured on the training and test datasets. The highest trait is agreeableness, followed by openness, an average level of conscientiousness, and an average value of extroversion.
•Conclusion: “Cluster-4” represents White Hats with low neuroticism, high agreeableness, and average values of extroversion.
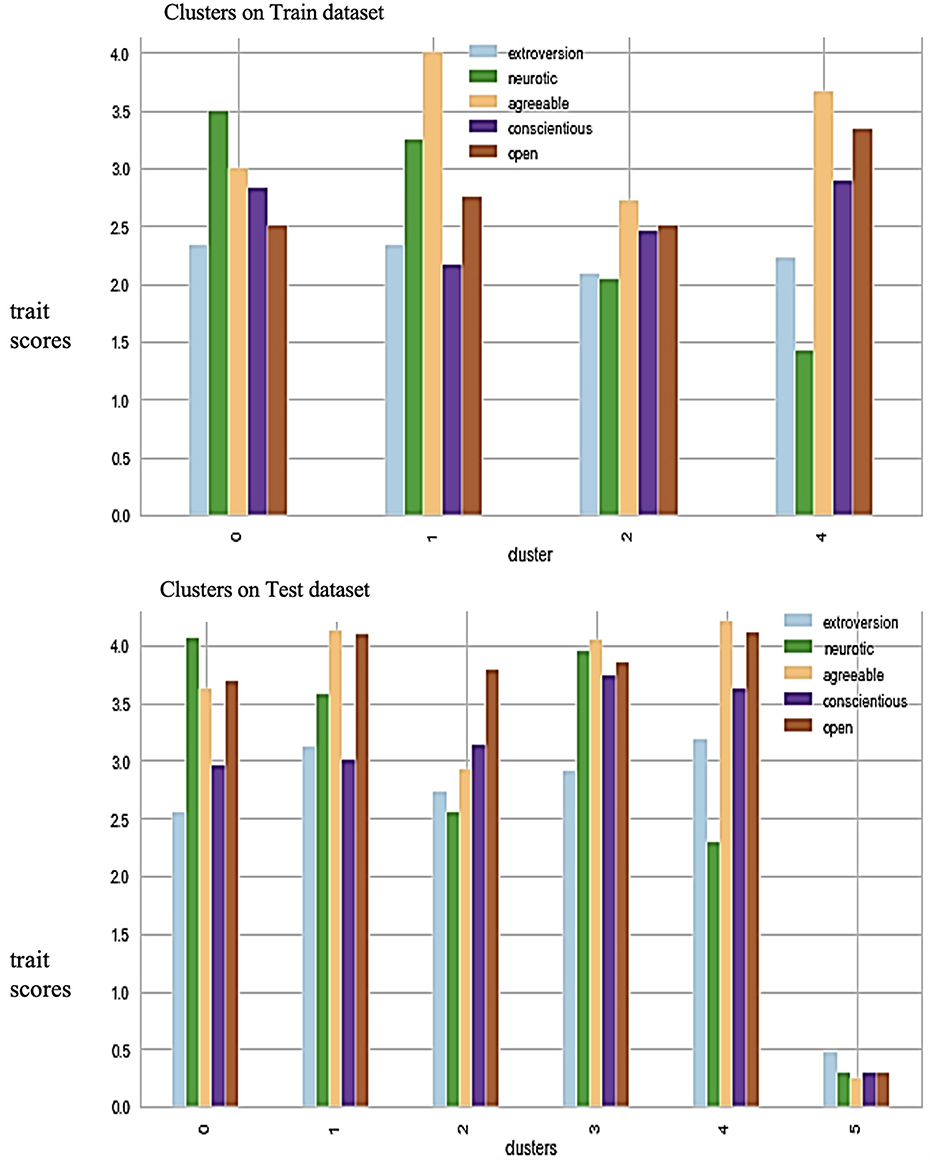
Figure 5 . Average score value against each cluster for OCEAN traits prediction on training data.
See Table 7 for clusters to Hat-type mapping with quantitative values of average scores across each trait for all clusters predicted on the test dataset.
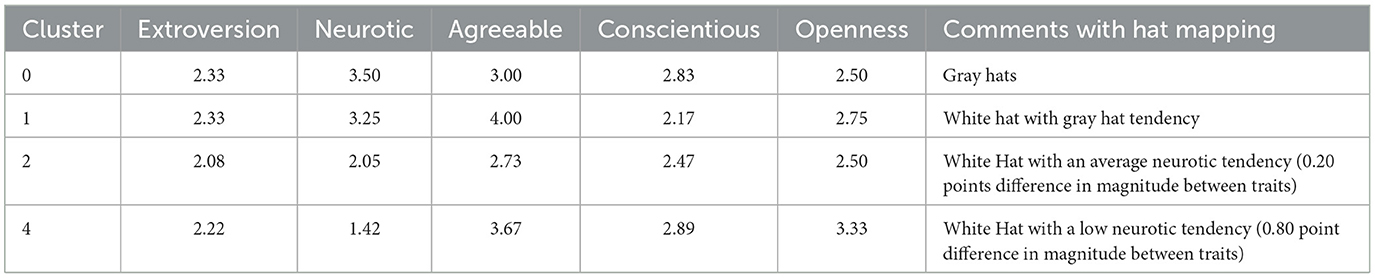
Table 7 . Cluster to Hat-types mapping.
5.4 Validation of average scores
A test dataset of 30 records is collected to check the claims of previous research ( Matulessy and Humaira, 2017 ) without applying the clustering algorithm. In Table 7 , the test dataset matches the previous research's ( Matulessy and Humaira, 2017 ) generalized category claim of White Hats, as shown in Table 1 .
After the detailed experimentation performed in Section 4, a better interpretation of validation results on test data can be made based on Table 7 cluster to Hat-type mappings (see Table 8 ).
1. The average scores of the test dataset for professionals match the generalized score claim for White Hats in previous research ( Matulessy and Humaira, 2017 ) but show an average extroversion value (see Table 6 ); therefore, after the following cluster-level validation, it could be placed under Cluster-4.
2. The average scores of the test dataset for student hackers match the generalized score claim for White hackers ( Matulessy and Humaira, 2017 ) but show an average neuroticism value (see Table 6 ). Therefore, the student's class belongs to the White Hats but tilts toward Gray Hat traits, and after performing the cluster-level validation, it will be placed under Cluster-2.
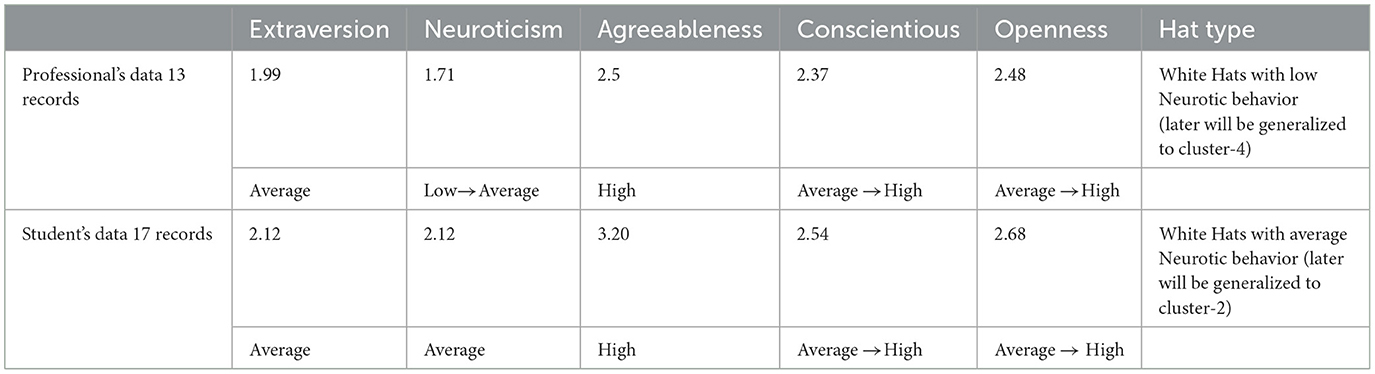
Table 8 . Validation of average trait scores on test datasets about previous research ( Matulessy and Humaira, 2017 ).
5.5 Secure model's clustering model validation
The secure model uses the clusters to predict the criminal's or hacker's personality type.
In this section, the clustering outcomes are validated to visualize the cluster's outcome spread when run over the test dataset (see Figure 6 ). Validation was performed by making cluster predictions over the test dataset using a 6-clusters-based model trained on 21 factors-based train datasets. As shown in Table 9 , the overall prediction performance accuracy is 100% of the time. This can be seen when using the correlation information in Table 6 to better understand the varying trait-wise mapping for hackers in the test dataset.
1. The professional dataset has shown 100% accuracy as they are all White Hats since Cluster-2 and Cluster-4 represent White Hats.
2. The student's dataset predicts 88% of White Hats and 11.7% of Gray Hats or White Hats with Gray Hat tendency. Both Cluster-0 and Cluster-1 show Gray Hat tendencies.
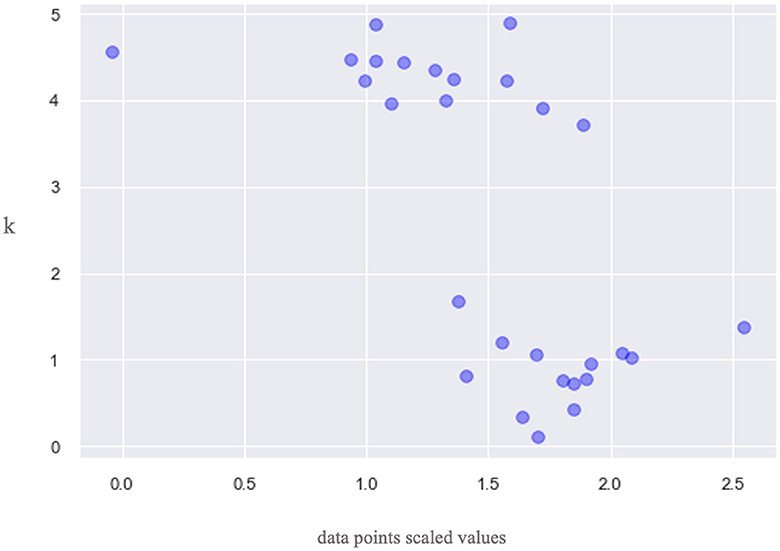
Figure 6 . Test data points spread across the predicted clusters.
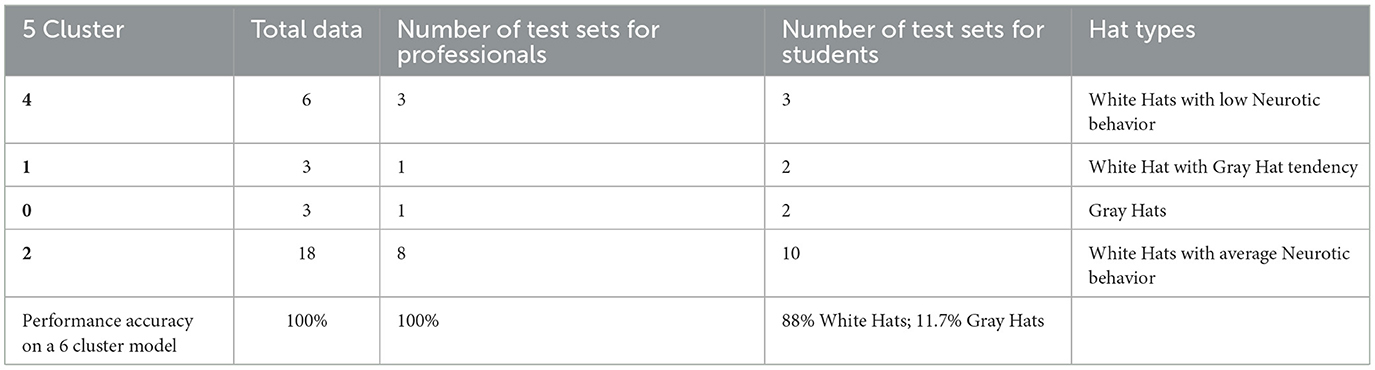
Table 9 . Distribution of test dataset on 5 and 6 cluster-based hacker identification models.
6 Discussion
This research uses machine learning to validate the proposed approach on approximately 30 real-life datasets. The application prediction performance was evaluated on (a) the final-year students who have some experience in hacking and intend to choose information security and ethical hacking as their profession and (b) professionals from the industry who are working as White Hackers. The study aimed to understand cluster trends and their association with different Hat types, requiring consideration of trait correlations in the test data. Spearman's correlation analysis was conducted, revealing moderate inter-dependencies between traits. These correlations were generalized, associating certain traits with specific Hat types.
The clustering analysis highlighted distinct trends across datasets, with clusters exhibiting varying trait compositions. The validation of these clusters using a 6-cluster model showed a high prediction accuracy of 100%, with professionals predominantly classified as White Hats and students displaying a mix of White Hat and Gray Hat tendencies. It has successfully mapped the different clusters with the different Hat types in the test dataset (see Table 9 ) with 88% accuracy. This can predict 11.7% of our false understanding of test data to consider two correctly predicted students as we conceived Gray Hats as White Hats. Previous research ( Matulessy and Humaira, 2017 ) being conducted under the psychology domain only discusses results at generalized higher levels, covers no scientific experimentation, and has no detail of cluster assignments; therefore, it was neither possible to correctly understand individual tests nor the implementation done for cyber-criminal identification as hackers.
6.1 Implications
Incorporating personality profiling methodologies within the realm of cybersecurity elicits profound ethical inquiries necessitating meticulous examination. Chief among these concerns is the pivotal issue of privacy, wherein the acquisition and scrutiny of individuals' personality traits may encroach upon their privacy entitlements, and absent explicit consent and robust protective measures, with a palpable risk of unauthorized access to sensitive personal data, potentially precipitating privacy breaches and data misuse. Additionally, the deployment of personality profiling algorithms introduces the specter of bias, engendering the prospect of unjust treatment or discriminatory practices targeting specific individuals or demographic groups.
Securing informed consent stands as a crucial element in navigating these ethical challenges. Organizations are responsible for ensuring that individuals are comprehensively informed about the intentions and potential consequences of gathering and scrutinizing their personality data for cybersecurity aims. This empowers individuals to make informed decisions regarding their participation, allowing them to grant consent or abstain. Transparency and accountability take center stage in this process, compelling organizations to openly disclose their data management procedures and to shoulder accountability for any ethical implications arising from the application of personality profiling.
Moreover, the cultivation of sustainable cybersecurity practices assumes critical importance in ensuring that security measures are deployed to minimize adverse environmental and societal impacts. This necessitates concerted efforts to curtail the environmental footprint associated with cybersecurity operations, promote social responsibility, and fortify resilience against cyber threats over the long term. Organizations can bolster security postures by integrating sustainability imperatives into cybersecurity frameworks while advancing equitable and environmentally conscious digital ecosystems.
The implications of our research underscore the imperative of comprehending hacker behavior, advocating for ethical considerations in cybersecurity practices, and promoting sustainable security paradigms. Through the dissemination of awareness on these issues, our endeavor is to facilitate informed decision-making and foster responsible cybersecurity practices that accord primacy to principles of privacy, equity, and sustainability.
6.2 Conclusion and future work
Following the research, it can be concluded that at a higher level, the hackers possess personality traits of agreeableness, neuroticism, and openness to experience. K-means algorithm of machine learning can be used to detect the personality traits of hackers. This research is an in-depth study to establish a quantitative and statistically significant mapping between predicted clusters and their respective Hat types using machine learning and correlation techniques. The mapping established in this research justifies the test dataset prediction performance accuracy of ~94%. Cross-validation was not utilized due to the ample size of the training set. Additionally, the training and test sets were distinct. For future work, it is suggested that if reliable access to hackers becomes available, the training set could primarily consist of hacker data, which would then be validated using cross-validation techniques.
The model must also validate the test dataset for Black Hat types from reliable resources. Further work can be done to make this approach more advanced by replacing the questionnaire with some other graphical or pictorial techniques to judge the personalities of employees before the contract signup stage or at the time of the signup process on office systems.
Despite the strength of our approach and findings, it is important to recognize some limitations. One key issue is the size and diversity of our sample. Our study's sample might not be big or varied enough to apply our findings to all hackers. Most of our participants were final-year students and cybersecurity professionals, so our conclusions might not fully represent all hacker personality traits. In addition, since our sample was mostly male, we might not have captured the full range of hacker demographics. Additionally, relying on self-reported data and personality tests could introduce biases. Participants might try to give answers they think are socially desirable, affecting the accuracy of our data.
Finally, our study focused on specific personality traits linked to hackers, but there could be other factors at play in cybersecurity behavior. Future research should aim to overcome these limitations by using more diverse samples, which would help make our findings more reliable and widely applicable.
Data availability statement
The raw data supporting the conclusions of this article will be made available by the authors, without undue reservation.
Ethics statement
Ethical review and approval was not required for the study on human participants in accordance with the local legislation and institutional requirements. Written informed consent from the [patients/ participants OR patients/participants legal guardian/next of kin] was not required to participate in this study in accordance with the national legislation and the institutional requirements.
Author contributions
UH: Conceptualization, Formal analysis, Investigation, Methodology, Writing – original draft, Writing – review and editing. OS: Methodology, Supervision, Validation, Writing – review and editing. KK: Methodology, Supervision, Writing – review and editing. AA: Methodology, Resources, Writing – review and editing. NI: Methodology, Supervision, Writing – review and editing.
The author(s) declare that no financial support was received for the research, authorship, and/or publication of this article.
Conflict of interest
The authors declare that the research was conducted in the absence of any commercial or financial relationships that could be construed as a potential conflict of interest.
Publisher's note
All claims expressed in this article are solely those of the authors and do not necessarily represent those of their affiliated organizations, or those of the publisher, the editors and the reviewers. Any product that may be evaluated in this article, or claim that may be made by its manufacturer, is not guaranteed or endorsed by the publisher.
Adali, S., and Golbeck, J. (2012). “Predicting personality with social behavior,” in 2012 IEEE/ACM International Conference on Advances in Social Networks Analysis and Mining (Istanbul: IEEE), 302–309.
Google Scholar
Adewumi, A. O., and Akinyelu, A. A. (2017). A survey of machine-learning and nature-inspired based credit card fraud detection techniques. Int. J. Syst. Assurance Eng. Manage. 8, 937–953. doi: 10.1007/s13198-016-0551-y
Crossref Full Text | Google Scholar
Akdag, M. (2020). Open Psychometrics, Big Five Personality Test, International Personality Item Pool IPIP-BFFM . Available online at: https://www.kaggle.com/akdagmelih/five-personality-clusters-k-means (accessed November 27, 2023).
Alashti, Z. F., Bojnordi, A. J. J., and Sani, S. M. S. (2022). Toward a carnivalesque analysis of hacking: a qualitative study of Iranian hackers. Asian J. Soc. Sci . 50, 147–155. doi: 10.1016/j.ajss.2022.01.001
Aldhyani, T. H., and Alkahtani, H. (2022). Attacks to autonomous vehicles: a deep learning algorithm for cybersecurity. Sensors 22, 360. doi: 10.3390/s22010360
PubMed Abstract | Crossref Full Text | Google Scholar
Ali, A., Wasim, A., Husam, A., and Manasa, K. N. (2020). Crime analysis and prediction using K-means clustering technique. EPRA Int. J. Econ. Business Rev. 3, 2925–2929. doi: 10.36713/epra2016
Back, S., LaPrade, J., Shehadeh, L., and Kim, M. (2019). “Youth hackers and adult hackers in South Korea: An application of cybercriminal profiling,” in 2019 IEEE European Symposium on Security and Privacy Workshops (EuroS&PW) (Stockholm: IEEE), 410–413.
Bakas, A., Wagner, A., Johnston, S., Kennison, S., and Chan-Tin, E. (2021). Impact of personality types and matching messaging on password strength. EAI Endors. Trans. Secur. Safety . 8, e1-e1. doi: 10.4108/eai.1-6-2021.170012
Buch, R., Dhatri, G., Pooja, K., and Nirali, B. (2017). World of cyber security and cybercrime. RTPL 4, 18–23.
Chayal, N. M., and Patel, N. P. (2021). Review of machine learning and data mining methods to predict different cyberattacks. Data Sci. Intellig. Applicat. 43–51. doi: 10.1007/978-981-15-4474-3_5
Chng, S., Lu, H. Y., Kumar, A., and Yau, D. (2022). Hacker types, motivations and strategies: A comprehensive framework. Comp. Human Behav. Rep. 5, 100167. doi: 10.1016/j.chbr.2022.100167
Corallo, A., Lazoi, M., Lezzi, M., and Luperto, A. (2022). Cybersecurity awareness in the context of the Industrial Internet of Things: a systematic literature review. Comp. Indust. 137, 103614. doi: 10.1016/j.compind.2022.103614
Del Pozo, I., Iturralde, M., and Restrepo, F. (2018). “Social engineering: Application of psychology to information security,” in 2018 6th International Conference on Future Internet of Things and Cloud Workshops (FiCloudW) (Barcelona: IEEE), 108–114.
Fox, B., and Holt, T. J. (2021). Use of a multitheoretic model to understand and classify juvenile computer hacking behavior. Crim. Justice Behav. 48, 943–963. doi: 10.1177/0093854820969754
Geluvaraj, B., Satwik, P. M., and Ashok Kumar, T. A. (2019). “The future of cybersecurity: Major role of artificial intelligence, machine learning, and deep learning in cyberspace,” in International Conference on Computer Networks and Communication Technologies (Cham: Springer), 739–747.
Golbeck, J., Robles, C., Edmondson, M., and Turner, K. (2011). “Predicting personality from twitter,” in 2011 IEEE Third International Conference on Privacy, Security, Risk and Trust and 2011 IEEE Third International Conference on Social Computing (Boston: IEEE), 149–156.
Gulati, J., Priya, B., Bharti, S., and Anu, S. L. (2016). A study of the relationship between performance, temperament, and personality of a software programmer. ACM SIGSOFT Softw. Eng. Notes 41, 1–5. doi: 10.1145/2853073.2853089
Imran, M., Faisal, M., and Islam, N. (2019). “Problems and vulnerabilities of ethical hacking in Pakistan,” in 2019 Second International Conference on Latest Trends in Electrical Engineering and Computing Technologies (INTELLECT) (Karachi: IEEE), 1–6.
Islam, N., Shaikh, A., Qaiser, A., Asiri, Y., Almakdi, S., Sulaiman, A., et al. (2021). Ternion: an autonomous model for fake news detection. Appl. Sci. 11, 9292. doi: 10.3390/app11199292
Islam, N., and Shaikh, Z. A. (2016). “A study of research trends and issues in wireless ad hoc networks,” in Mobile Computing and Wireless Networks: Concepts, Methodologies, Tools, and Applications , ed I. Management Association (IGI Global), 1819–1859. doi: 10.4018/978-1-4666-8751-6.ch081
Javaid, M. A. (2013). Psychology of hackers. SSRN Electr. J . 15, 26. doi: 10.2139/ssrn.2342620
John, P., and Oliver, Sanjay, S. (1999). The Big-Five Trait Taxonomy: History, Measurement, and Theoretical Perspectives . Berkeley: University of California. Available online at: https://personality-project.org/revelle/syllabi/classreadings/john.pdf (accessed March 10, 2024).
Larose, D. T., and Chantal, D. L. (2014). “Discovering knowledge in data: an introduction to data mining,” in IEEE Computer Society, 2nd ed . Hoboken: John Wiley and Sons.
Lima, A. C. E., and De Castro, L. N. (2014). A multi-label, semi-supervised classification approach applied to personality prediction in social media. Neural Netw. 58, 122–130. doi: 10.1016/j.neunet.2014.05.020
Martineau, M., Spiridon, E., and Aiken, M. (2023). A comprehensive framework for cyber behavioral analysis based on a systematic review of cyber profiling literature. Forens. Sci. 3, 452–477 doi: 10.3390/forensicsci3030032
Matulessy, A., and Humaira, N. H. (2017). Hacker personality profiles reviewed in terms of the big five personality traits. Psychol. Behav. Sci. 5, 137–142. doi: 10.11648/j.pbs.20160506.12
McAlaney, J., Hambidge, S., Kimpton, E., and Thackray, H. (2020). “Knowledge is power: an analysis of discussions on hacking forums,” in 2020 IEEE European Symposium on Security and Privacy Workshops (EuroS&PW) (Genoa: IEEE), 477–483.
Medoh, C., and Telukdarie, A. (2022). The future of cybersecurity: a system dynamics approach. Procedia Comp. Sci. 200, 318–326. doi: 10.1016/j.procs.2022.01.230
Mohammed, Z. H., Chankaew, K., Vallabhuni, R. R., Sonawane, V. R., Ambala, S., and Markkandan, S. (2023). Blockchain-enabled bioacoustics signal authentication for cloud-based electronic medical records. Measurem. Sens . 26, 100706. doi: 10.1016/j.measen.2023.100706
Novikova, I. A., and Alexandra, A. V. (2019). “The five-factor model: contemporary personality theory,” in Cross-Cultural Psychology: Contemporary Themes and Perspectives (Hoboken: John Wiley and Sons Press), 685–706.
Odemis, M., Yucel, C., and Koltuksuz, A. (2022). Detecting user behavior in cyber threat intelligence: development of honeypsy system. Secur Commun. Netw. 2022, 7620125. doi: 10.1155/2022/7620125
Pastrana, S., Hutchings, A., Caines, A., and Buttery, P. (2018). “Characterizing eve: Analysing cybercrime actors in a large underground forum,” in International Symposium on Research in Attacks, Intrusions, and Defenses (Cham: Springer), 207–227.
Ramachandran, D., Rajeev Ratna, V., PT, V. R., and Garip, I. (2022). A low-latency and high-throughput multipath technique to overcome black hole attack in mobile ad hoc network (MTBD). Secur. Commun. Netw . 2022, 8067447. doi: 10.1155/2022/8067447
Shackelford, S. J., Raymond, A., Fort, T. L., and Charoen, D. A. (2016). Sustainable Cybersecurity: Applying Lessons from the Green Movement to Managing Cyber Attacks . Chicago: University of Illinios Law Review. Available online at: https://illinoislawrev.web.illinois.edu/wp-content/uploads/2016/10/Shackelford.pdf (accessed March 10, 2024).
Siddiqi, M. A., Pak, W., and Siddiqi, M. A. (2022). A study on the psychology of social engineering-based cyberattacks and existing countermeasures. Appl. Sci . 12, 6042. doi: 10.3390/app12126042
Sood, A. K., and Enbody, R. J. (2013). Crimeware-as-a-service: a survey of commoditized crimeware in the underground market. Int. J. Criti. Infrastruct. Protect. 6, 28–38. doi: 10.1016/j.ijcip.2013.01.002
Suárez Álvarez, J., Pedrosa, I., Lozano, L. M., García Cueto, E., Cuesta Izquierdo, M., and Muñiz Fernández, J. (2018). “Using reversed items in Likert scales: A questionable practice,” in Psicothema , 30. Available online at: https://digibuo.uniovi.es/dspace/bitstream/handle/10651/48979/Using%20.pdf?sequence=1 (accessed March 10, 2024).
PubMed Abstract | Google Scholar
Suryapranata, K. P., Louis, P. K., Gede, H., Yaya, H., Bahtiar, S. A., et al. (2017). “Personality trait prediction based on game character design using a machine learning approach,” in Proc. ICITech (Salatiga: IEEE), 1–5.
Tamboli, M. S., Vallabhuni, R. R., Shinde, A., Kataraki, K., and Makineedi, R. B. (2023). Block chain based integrated data aggregation and segmentation framework by reputation metrics for mobile adhoc networks. Measurem.: Sens. 27, 100803. doi: 10.1016/j.measen.2023.100803
Tan, P. N., Steinbach, M., and Kumar, V. (2016). Introduction to Data Mining . Washington DC: Pearson Education India. Available online at: https://www-users.cse.umn.edu/~kumar001/dmbook/ch7_clustering.pdf (accessed March 10, 2024).
Tandera, T., Derwin, S., Rini, W., and Yen, L. P. (2017). Personality prediction system from Facebook users. Procedia Comp. Sci. 116, 604–611. doi: 10.1016/j.procs.2017.10.016
Wong, S., and Dennis Sai-fu, F. (2020). Development of the cybercrime rapid identification tool for adolescents. Int. J. Environ. Res. Public Health 17, 4691. doi: 10.3390/ijerph17134691
Xie, B., and Wei, N. (2022). “Personality trait identification based on hidden semi-Markov model in online social networks,” in Proceedings of the 2022 7th International Conference on Intelligent Information Technology (ICIIT '22) (New York, NY: Association for Computing Machinery), 52–58. doi: 10.1145/3524889.3524898
Zheng, R., Qin, Y., Huang, Z., and Chen, H. (2003). “Authorship analysis in cybercrime investigation,” in International Conference on Intelligence and Security Informatics (Berlin: Springer), 59–73.
Keywords: hacker identification, personality traits, K-means clustering, cyber security, social engineering
Citation: Hani U, Sohaib O, Khan K, Aleidi A and Islam N (2024) Psychological profiling of hackers via machine learning toward sustainable cybersecurity. Front. Comput. Sci. 6:1381351. doi: 10.3389/fcomp.2024.1381351
Received: 03 February 2024; Accepted: 22 March 2024; Published: 08 April 2024.
Reviewed by:
Copyright © 2024 Hani, Sohaib, Khan, Aleidi and Islam. This is an open-access article distributed under the terms of the Creative Commons Attribution License (CC BY) . The use, distribution or reproduction in other forums is permitted, provided the original author(s) and the copyright owner(s) are credited and that the original publication in this journal is cited, in accordance with accepted academic practice. No use, distribution or reproduction is permitted which does not comply with these terms.
*Correspondence: Osama Sohaib, osama.sohaib@uts.edu.au
This article is part of the Research Topic
Human-Centered Approaches in Modern Software Engineering
Academia.edu no longer supports Internet Explorer.
To browse Academia.edu and the wider internet faster and more securely, please take a few seconds to upgrade your browser .
- We're Hiring!
- Help Center
Computer Hacking
- Most Cited Papers
- Most Downloaded Papers
- Newest Papers
- Save to Library
- Last »
- Ethical Hacking Follow Following
- Hacking Follow Following
- Web Designing Follow Following
- Photoshop Follow Following
- WebGL Follow Following
- Javascript Follow Following
- PENELITIAN PENDIDIKAN MATEMATIKA Follow Following
- Filsafat Ilmu Follow Following
- Hacking and Computer Security Follow Following
- Computer Science Follow Following
Enter the email address you signed up with and we'll email you a reset link.
- Academia.edu Publishing
- We're Hiring!
- Help Center
- Find new research papers in:
- Health Sciences
- Earth Sciences
- Cognitive Science
- Mathematics
- Computer Science
- Academia ©2024

An official website of the United States government
The .gov means it’s official. Federal government websites often end in .gov or .mil. Before sharing sensitive information, make sure you’re on a federal government site.
The site is secure. The https:// ensures that you are connecting to the official website and that any information you provide is encrypted and transmitted securely.
- Publications
- Account settings
Preview improvements coming to the PMC website in October 2024. Learn More or Try it out now .
- Advanced Search
- Journal List
- J Med Internet Res
- PMC10170356

Artificial Intelligence–Based Ethical Hacking for Health Information Systems: Simulation Study
1 School of Computer Science, University of Nottingham, Nottingham, United Kingdom
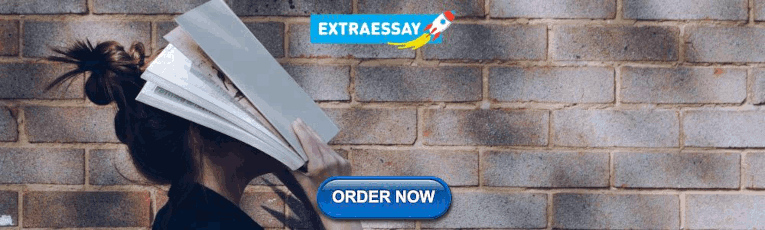
Efpraxia Zamani
2 Information School, University of Sheffield, Sheffield, United Kingdom
Iryna Yevseyeva
3 School of Computer Science and Informatics, De Montfort University, Leicester, United Kingdom
4 School of Computer Science and Electronic Engineering, University of Essex, Colchester, United Kingdom
5 Key Laboratory of Medical Electrophysiology, Ministry of Education & Medical Electrophysiological Key Laboratory of Sichuan Province, Collaborative Innovation Center for Prevention of Cardiovascular Diseases, Institute of Cardiovascular Research, Southwest Medical University, Luzhou, China
Associated Data
The data sets generated and analyzed during this study are available from the corresponding author upon reasonable request.
Health information systems (HISs) are continuously targeted by hackers, who aim to bring down critical health infrastructure. This study was motivated by recent attacks on health care organizations that have resulted in the compromise of sensitive data held in HISs. Existing research on cybersecurity in the health care domain places an imbalanced focus on protecting medical devices and data. There is a lack of a systematic way to investigate how attackers may breach an HIS and access health care records.
This study aimed to provide new insights into HIS cybersecurity protection. We propose a systematic, novel, and optimized (artificial intelligence–based) ethical hacking method tailored specifically for HISs, and we compared it with the traditional unoptimized ethical hacking method. This allows researchers and practitioners to identify the points and attack pathways of possible penetration attacks on the HIS more efficiently.
In this study, we propose a novel methodological approach to ethical hacking in HISs. We implemented ethical hacking using both optimized and unoptimized methods in an experimental setting. Specifically, we set up an HIS simulation environment by implementing the open-source electronic medical record (OpenEMR) system and followed the National Institute of Standards and Technology’s ethical hacking framework to launch the attacks. In the experiment, we launched 50 rounds of attacks using both unoptimized and optimized ethical hacking methods.
Ethical hacking was successfully conducted using both optimized and unoptimized methods. The results show that the optimized ethical hacking method outperforms the unoptimized method in terms of average time used, the average success rate of exploit, the number of exploits launched, and the number of successful exploits. We were able to identify the successful attack paths and exploits that are related to remote code execution, cross-site request forgery, improper authentication, vulnerability in the Oracle Business Intelligence Publisher, an elevation of privilege vulnerability (in MediaTek), and remote access backdoor (in the web graphical user interface for the Linux Virtual Server).
Conclusions
This research demonstrates systematic ethical hacking against an HIS using optimized and unoptimized methods, together with a set of penetration testing tools to identify exploits and combining them to perform ethical hacking. The findings contribute to the HIS literature, ethical hacking methodology, and mainstream artificial intelligence–based ethical hacking methods because they address some key weaknesses of these research fields. These findings also have great significance for the health care sector, as OpenEMR is widely adopted by health care organizations. Our findings offer novel insights for the protection of HISs and allow researchers to conduct further research in the HIS cybersecurity domain.
Introduction
The health care sector is continuously targeted by cyberattackers, who seek to exploit undetected vulnerabilities in critical health infrastructure. Such attacks can cause service disruptions, financial losses, and harm to patients. In the 2017 WannaCry attack on the United Kingdom’s National Health Service (NHS), there was a substantial decrease in patients’ attendances and admissions numbers, which caused a £5.9 million (US $7.1 million) lost in terms of hospital activity [ 1 ]. This study is motivated by recent security incidents that have increased during the COVID-19 pandemic, affecting health care organizations, such as the US Department of Health and Human Services, the World Health Organization (WHO), and pharmaceutical companies [ 2 ]. Specifically, the United States Public Health Service reported that approximately 100 million pieces of patient information were stolen monthly by 2020 [ 3 ]. Fortified Health Security, a leading organization in health care cybersecurity, reported that more than 400 health information system (HIS) providers had been breached, affecting approximately 13.5 million patients [ 4 ]. In such cases, cyberattackers not only destroy the HIS but also gain access to and can modify sensitive health records that may mislead medical diagnosis [ 5 ].
The research community and health care industry have long realized the urgency to protect HISs [ 6 - 12 ]. However, existing cybersecurity research in the health care domain places an imbalanced focus on protecting medical devices [ 13 - 17 ] and medical data [ 18 ], whereas previous studies do not offer a systematic approach for the investigation of HIS breaches or for improving cybersecurity more broadly. In this study, we propose a systematic approach to address this shortcoming based on ethical hacking. Typically, ethical hacking entails analyzing a system to identify potential weak points and then executing attacks to test the robustness of the system. Such approaches often entail using artificial intelligence (AI) and, most typically, reinforcement learning, for example [ 19 ]. However, reinforcement learning has important shortcomings when it comes to the ethical hacking of HISs, namely, reinforcement learning requires large data sets for training purposes, which most often are unavailable. Therefore, as an approach, it can be unreliable [ 20 ]; can cause severe issues for the HIS network [ 21 ]; and requires skills and expertise, neither of which are widely available [ 22 ].
In our study, we address the above limitations by proposing a new optimization module for ethical hacking that uses the ant colony optimization (ACO) algorithm. The algorithm is characterized by positive feedback, distributed computation, and constructive greedy heuristics [ 23 ]. ACO has been previously implemented in the cybersecurity domain, focusing on network intrusion detection, and has recently been proposed for vulnerability analysis and detection [ 24 ].
In this study, we built an HIS simulation platform by implementing an open-source electronic medical record (OpenEMR) system and drew from the ethical hacking framework from the National Institute of Standards and Technology (NIST), which we enriched by integrating ACO within its optimization module as part of our ethical hacking method to examine the exploitation of potential vulnerabilities of HISs. We then demonstrated ethical hacking for the HIS simulation environment using both optimized and unoptimized hacking methods and compared the results.
Our study makes important contributions to the health care industry from a cybersecurity perspective. First, our methodological approach to ethical hacking provides important insights into the protection of HISs. It allows practitioners to identify potential vulnerabilities in their systems and offers researchers several avenues for future research. Second, our optimized ethical hacking approach addresses the weaknesses of preexisting frameworks by proposing an intelligent and maintainable ethical hacking solution. To the best of our knowledge, there is no systematic AI-based ethical hacking method that is tailored for health care organizations. Our research makes a major theoretical and practical contribution to the field of digital health by addressing the security aspects of digital medicine infrastructure, which will ultimately improve the quality of security practices of large health care organizations. In doing so, our findings indirectly inform cognate disciplines, namely information systems literature and cybersecurity, by being centered on a core information system element [ 25 ].
HIS Security
New technologies have been advancing the field of HISs and improving the quality of services in the health care sector [ 26 - 28 ]. Some advanced HISs support medical diagnoses based on existing health records and data gathered from intelligent medical devices. Such systems significantly reduce the workload of health care professionals and enable early detection, diagnosis, and intervention, thereby increasing the success rate of treatment [ 29 , 30 ]. However, new technologies introduce new security risks for HISs, and the lack of sufficient security control is a concern [ 31 ]. According to recent studies, HISs have major security vulnerabilities [ 32 - 34 ] and privacy concerns [ 35 ]. For example, access to insecure web pages and default coded passwords are common vulnerabilities introduced by medical devices [ 36 ]. Similarly, insecure communications on unauthorized and unencrypted web services are also common vulnerabilities because they allow cyberattackers to gain remote access to HISs [ 37 ].
As a result, to date, most studies in the health care cybersecurity domain have focused primarily on increasing the security of medical devices [ 13 - 17 ] and the protection of medical data [ 18 ]. For example, a common approach is to implement data encryption mechanisms [ 13 ], often in combination with scrambling techniques [ 18 ], to protect wavelet-based electrocardiogram (ECG) data both in transit and storage. Other popular solutions involve the design and use of access control schemes to further increase the protection of shared health data [ 14 ], implementation of authentication protocols for wearable devices [ 15 ], and adoption of privacy-aware profile management approaches that help manage the privacy of patient electronic profiles [ 14 ]. In other cases, the proposed solutions involve mechanisms that enhance heartbeat-based security [ 17 ]. However, existing research has not yet offered a systematic approach or methods to investigate and understand how attackers can breach HISs and access health care records. To address this, we discuss the ethical hacking methods that have been proposed by cybersecurity research, which can provide a systematic approach.
Ethical Hacking Methods
Some of the most widely adopted ethical hacking methods are the NIST framework [ 38 ], Penetration Testing Execution Standard (PTES), and framework proposed by the Open Web Application Security Project (OWASP). In addition, different organizations often develop their own organization-specific methods that correspond to their particular organizational needs [ 22 ].
Both ethical hacking and penetration testing are authorized attempts to gain unauthorized access to computer systems or data. Penetration testing is a subset of the ethical hacking methods. Penetration testing assesses a specific aspect of a system that is usually restricted by an outlined scope, whereas ethical hacking has more flexibility without being restricted [ 39 ]. However, systematic ethical hacking or penetration testing typically includes 4 main modules: information gathering, discovery, attacking, and reporting. The tester performs a reconnaissance at the information-gathering stage and collects information about the target HIS. At the discovery stage, the tester attempts to understand the system’s structure of the system and analyze its paths and directories. Next, the tester identifies the vector to attack at the attack stage, which is typically based on the vulnerability scanner results. Finally, at the reporting stage, the tester uses all evidence gathered during the previous stages to prepare a report documenting major findings.
The extent to which such ethical hacking methods will be successful largely depends on the skills and expertise of professional testers involved in penetration testing. However, the number of skilled programmers in cybersecurity, particularly in the health care domain, is limited [ 22 ]. This means that on the one hand, it is difficult to identify the necessary talent for ethical hacking within such complex environments, whereas on the other hand, there is a risk of poorer performance when the required skills are not available.
Ethical Hacking Tools and Solutions
Nettacker, a solution developed by OWASP, contains an optimization module, but it is not as mature, not fully published, and lacks an exploit module. This means that a given user will have to select the exploit tools and payload on their own, which can be challenging for nonexperts in cybersecurity. APT2, the solution offered by the Massachusetts Institute of Technology, uses Network Mapper (Nmap) to scan information. An exploit can be launched from its library, depending on the scanning information, and it has a knowledge base that can record the information of the targeted host. Nevertheless, it lacks an optimization module. This finding suggests that the accuracy and efficiency of ethical hacking risks are inferior. Similar to APT2, Autosploit [ 40 ], a solution that combines Shodan, Censys, Zoomeye, and Metasploit, does not have an optimization module. It is easy to conduct ethical hacking using this solution because it requires only logging into a Shodan account and provides details regarding the targeted host. After performing a search, Shodan will provide the open port, the vulnerabilities that exist, and tools for the exploit, which will then be able to input this information to Metasploit, specifying the local host and the local port [ 41 ]. Metasploit can then run the exploit automatically. However, similar to APT2, Autosploit risks have less accuracy and efficacy because it cannot be optimized. Currently, it is unfeasible to test all possible system configurations. An earlier study attempted to address this problem and proposed the use of generalized binary splitting and the Barinel method to optimize the efficiency of Autosploit [ 40 ]. Although this approach positively influenced Autosploit’s performance, the tool library and database of vulnerabilities stopped being updated in 2019 and are now outdated.
AI-Based Ethical Hacking in HISs
Ethical hacking methods often use AI techniques. Among those most often used is reinforcement learning, which helps identify and analyze vulnerabilities in information systems. To date, reinforcement learning has been successfully applied in simulated environments to analyze vulnerabilities using the Partially Observed Markov Decision Process [ 42 ] and within the context of applied Q-learning with a deep neural network architecture [ 19 ]. However, these approaches tend to offer mostly theoretical insights and are being implemented in MATLAB; to date, they have not been systematically integrated into any ethical hacking method. Another major shortcoming is that reinforcement learning requires a vast amount of data and ample time to train the model. In reality, it is unlikely that a single targeted host will exhibit sufficient vulnerabilities to train the algorithm. Additionally, reinforced learning can be unreliable for ethical hacking. For example, it has been used in the past for learning control policies in Atari games, whereby an agent triggers several bugs to achieve a high score; however, such behavior does not form part of the ethical hacking plan [ 21 ] and causes severe problems for the whole network, which is undesirable. Finally, most importantly, reinforcement learning is characterized by low reproducibility because of its data requirements and because its results can be negatively affected by even small environmental changes such as machine upgrades [ 20 ].
ACO Approach
In this paper, we propose the use of the ACO approach as an optimization algorithm to enhance the optimization module for ethical hacking. This algorithm is characterized by positive feedback, distributed computation, and constructive greedy heuristics [ 23 ] and can be particularly beneficial during attack path analysis, which is the core part of ethical hacking optimization.
ACO is an evolutionary algorithm often used to solve various optimization problems, for example, the traveling salesman problem (TSP). Optimization problems such as the TSP are particularly relevant to identifying and analyzing attack paths as part of ethical hacking, as in both cases, the objective is to construct the shortest path between the point of origin and the target point. In more detail, the goal of the TSP is to identify the shortest or quickest path for a salesman to arrive at their destination while covering all nodes between the point of origin and the target point and visiting them only once. Similarly, in ethical hacking, the goal is to attack the targeted machine by investigating some already known vulnerabilities and their exploitation (exploits) that can be combined to complete the attack successfully.
To date, the ACO approach has been implemented in the cybersecurity domain, focusing on network intrusion detection, which is a passive form of defense. More recently, it was proposed to be efficient for vulnerability analysis and detection, informed by bioinspired cybersecurity research [ 24 ]. On the basis of these earlier findings, our study integrated ACO within the optimization module of ethical hacking to examine its performance regarding the exploitation of potential vulnerabilities of HISs.
Simulation Platform
For the purposes of our study, we set up a virtual environment to avoid acting directly in a real-world setting, thus causing potential damage to the HIS. Specifically, we designed an experiment to simulate an HIS.
Targeted Host and Attack Host
In ethical hacking, the targeted host machine is attacked by the host machine. We installed the Kali Linus System 2021.1 on a virtual machine workstation in our simulation environment, which acts as the attack host. In addition, we installed Ubuntu 20.04.2.0 on another virtual machine workstation, which acted as the targeted host. Table 1 summarizes the hardware details of the target and attack hosts. Information on the software and services of the targeted host that simulates a medical worker is presented in Table 2 .
Hardware details for the targeted machine and attack machine.
a VM: virtual machine.
b Mbps: megabits per second.
c CPU: central processing unit.
Software and services used on the targeted machine.
a OpenEMR: open-source electronic medical record.
As part of our experiment, we adapted the NIST ethical hacking framework [ 38 ] and follow the core planning, discovery, attack, and reporting modules. We first set up a simulation environment by implementing an OpenEMR system and then launched ethical hacking to exploit the vulnerabilities of the simulated HIS.
OpenEMR Implementation
In our HIS simulation platform, we implemented OpenEMR. Overall, OpenEMR is a complex system with key functionalities, including practice management, EMR management, scheduling, electronic billing, prescribing, a patient portal, and a clinical decision support system, and has a complex database of more than 100 tables. We purposefully chose to implement this HIS because it supports a comprehensive security risk-management scheme based on the Health Insurance Portability and Accountability Act and NIST standards [ 43 ]. In addition, it is certified by the Office of the National Coordinator for Health Information Technology, which can run on different platforms such as Windows, Linux, and Mac OS X, and it is the most widely adopted HIS [ 44 ].
AI-Based Ethical Hacking Method
Our adaptation of the NIST ethical hacking framework [ 38 ] consisted of following 6 modules: scanning, discovery, exploitation, optimization, reporting, and control. In other words, we used the original NIST modules, but further enhanced them with 2 additional modules: optimization and control. Table 3 summarizes the key activities of each stage.
Key activities and the National Institute of Standards and Technology (NIST) method coverage of the (artificial intelligence [AI]–based) ethical hacking method.
a Nmap: Network Mapper.
b ACO: ant colony optimization.
We conducted a comparative experiment between AI-based and non–AI-based ethical hacking methods. Although the AI-based experiment followed the 6 stages of the ethical hacking method as indicated above, the non–AI-based experiment followed the same method without executing the optimization module. Optimized and unoptimized penetration tests were performed 50 times to reduce the uncertainty caused by the simulation environment. In each run, information on the time, the number of exploits, and the number of successful exploits were recorded and compared.
Generally, the results from each module were first recorded and then used in each subsequent module. Figure 1 shows the interactions between different modules and the results from each module.

Interactions between different modules.
Scanning Module
As part of the scanning module, we scanned the host information of the targeted machine, including the port, operating system, and installed service of the targeted machine. Nmap was used as a scanning tool to collect this information. Other similar tools included ZMap and Masscan. ZMap has an accuracy rate similar to that of NMap, but its computational time is higher [ 40 ]. Masscan is faster, but its accuracy rate is lower, particularly when the scanning area increases [ 40 ]. Therefore, we selected Nmap because of its accuracy and efficiency (computational time) and because it has more than 200 extension scripts for scanning.
We developed the following 2 versions of Nmap scanning scripts: the first was used for a single IP address and the other was used for an IP address segment. For a single IP address, Nmap scanning imports the IP from the control modules, checks whether the host is alive, and then scans and reports the results. For an IP address segment, the tool adopts multithreading to support multiple IP addresses in an IP address segment, and, as in the previous case, it then scans and reports the results.
Discovery Module
This module focuses on obtaining vulnerability-related information of the target host. Existing vulnerability scanning tools include Nessus, NexSpose, and Xray. Although Nessus and Nexpose have a Metasploit application programming interface, and their vulnerability data set is one of the largest for vulnerability scanning, they are costly, and the education version has a limited number of vulnerabilities and ports.
In this study, Xray was selected for vulnerability scanning using the basic crawler method. Xray is a free vulnerability scanning tool, and their performance is comparable to that of Nessus and Nexpose. Xray supports diverse operating systems such as Windows, Linux, and Mac. As a passive scanning tool, it is much faster than active scanning because the latter requires sending requests to the targeted host and waiting for a response. Passive scanning is also challenging to detect using a targeted host. Xray also supports the use of web scanning. The Xray output is a JSON file that contains the type, payload, and target of the vulnerability. Because the targeted machine is an HIS using OpenEMR, the web scanning module can help detect vulnerabilities in OpenEMR.
Exploiting Module
This module launches attacks on the targeted host by leveraging the information gathered in the previous modules. This module applies ethical hacking tools, namely, SQLMap and Metasploit. Many exploiting tools provide similar performance and functionalities; however, we selected Metasploit as the primary attack tool because it is the most powerful and widely used tool in the field. This tool integrates several application programming interfaces that can be used for manual and automated exploitation using predefined settings. When conducting a manual penetration test, the tester must set up the targeted information and tools used for exploitation. The exploitation procedure is replaced by a resource scripts file that configures the Metasploit when using automated scripts. In our study, we imported output files from Nmap and Xray, ran automated exploits, and extracted the exploitation results.
In addition, as the database is an essential component of the HIS, attacks should be launched as part of ethical hacking, and the vulnerabilities of the database should be exploited. For this purpose, we used SQLMap to conduct attacks on a database that launches attacks by executing malicious SQL commands in the web input. It supports 5 types of SQL injections and can launch other types of exploits, such as XSS (cross-site scripting) injection [ 45 ]. By exploiting database vulnerabilities using SQLMap, the attacker can tamper with or steal digital data and information, remotely control the database, crash the hard disk, and control the system using Trojan viruses [ 46 ]. However, this behavior does not damage the targeted host, which is essential because the penetration test aims to enhance security rather than destroy the system. In our experiment, SQLMap imported the JSON output file from Xray and retrieved the URL for SQL injection. It then launched the attack automatically and exported a file using exploitation results.
Optimizing Module
For the optimizing module, we used ACO as the optimization algorithm for the optimization module. ACO simulates the behavior of ants to identify the shortest path(s) and pheromone-based communication within the colony. Attack path analysis is a core aspect of ethical hacking optimization. In ethical hacking, the goal is to attack the targeted machine using known paths, and the objective is to identify the shortest or fastest path to achieve this. The most common example of using ACO is to solve the TSP, where the shortest or fastest path is searched for by a salesman to deliver goods in all cities by exploring various paths and visiting each city exactly once. Ethical hacking has a similar goal, whereby the objective is to attack the targeted machine by exploiting as few known vulnerabilities as possible to successfully and swiftly complete the attack. Various paths between the origin and target machines can be built by combining exploits and finding the shortest or fastest way to do so. Textbox 1 demonstrates the optimization procedure for ACO.
Algorithm 1 (the ant colony optimization [ACO] algorithm: ACO(Num_Iters, Num_Ants, VulnerList).
NumIters (NumIters >0) # the maximum number of iterations,
NumAnts (NumAnts >0) # the maximum number of ants,
VulnerList # the vulnerability exploits list.
The best path (BestPath) is exported.
1: BestPath ← 0; BestPathDist ← 99999999;
2: For k← 1: NumIters do
3: LocalBestPath ← 0; LocalBestPathDist ← 99999999; # local best path for a single iteration
4: PheromCons ← zeros([][]); # matrix of pheromone concentrations for all pairs of ants.
5: For i← 1: NumAnts do
6: Vulner_i = VulnerList[i];
7: For j← 1: NumAnts do
8: Vulner_j = VulnerList[j];
9: p_ij=compute_Pij(Vulner_i, Vulner_j); # transition probability for pair (i,j).
10: CurrentPath_ij=computeProbablePath(p_ij, Vulner_i, Vulner_j); # path for pair (i,j)
11: CurrentPathDist=computerPathDist(CurrentPath_ij); # distance for path (i,j).
12: PheromCons(i,j) = updatePheromCons(CurrentPath_ij); # update of pheromon matrix
13: If (CurrentPathDist<LocalBestPathDist)
14: LocalBestPath=CurrentPath; # update of the shortest local path
15: LocalPathDist=CurrentPathDist; # update of the shortest local path distance
17: End for #NumAnts with j index
18: End for #NumAnts with i index
19: If (LocalBestPathDist<BestPathDist)
20: BestPath=LocalBestPath; # update of the shortest global path
21: BestPathDist=LocalBestPathDist; # update of the shortest global path distance
23: End for #NumIters
24: return BestPath

where Q is a constant and L k is the length of the k th ant tour.

The transition probability for each pair of nodes i and j for the k th ant can then be computed as follows:

where allowed is the set of not yet visited nodes, α is the weight of the pheromone, and β is the weight of the heuristic value [ 23 ]; here, they are set to α=.7 and β=.7, as suggested in Liu et al [ 47 ].
Each successful use of an exploit increases the concentration of pheromones for a pair of exploits that are connected successfully.
An ant explores a set of nodes vulnerabilities presented in a matrix in an attempt to construct a successful exploitation path. Whenever a successful exploitation is recorded, the current successful path is compared with the global best path found in all runs thus far and updated every time a shorter path is found.
The end condition for each literation was whether all the ants visited all the nodes in the vulnerability matrix. When all iterations have finished, ACO ends and provides results on the global best path.
After the final iteration, the optimization module reports the list of attack paths and prioritizes the paths with the highest pheromone concentration. The output was then stored as a *.csv file, titled “ant_output.csv,” which contains information on the common vulnerabilities and exposures, exploit, and used payload.
Controlling Module
The controlling module imports the results produced from the previous modules, and it is necessary to conduct ethical hacking and launch attacks. Users can control the penetration test via an interactive user interface and set the targeted machine’s IP address or IP address segment of the targeted machine. The module then transmits this information to the information and vulnerability scanning modules. Once the scanning module is completed, users have to decide whether optimization is needed, and based on their decision, the optimization module will be triggered. This, in turn, calls the exploiting module to launch the attack on the targeted host. At the end of the procedure, this module sends its results to the reporting module, recording the time required to carry out ethical hacking for each module.
Reporting Module
The reporting module collected the results of ethical hacking. Two sets of results (.csv files) were generated. The first set reports the time used for each module and the number and success rate of the launched exploits. This information can also be used to evaluate the performance of the algorithm in the optimization module. The second set of results contains information regarding the vulnerabilities themselves and can help users understand the targeted host’s security status and, therefore, act accordingly. Figure 2 summarizes the execution of the ethical hacking framework.

Flowchart of the ethical hacking framework. ACO: ant colony optimization.
Ethical Considerations
As our research does not involve human participants directly or indirectly (eg, observations of public behaviors or secondary analyses of research data), ethics approval, informed consent, and compensation for human participants research were not required. In addition, the design of our study was based on simulations conducted within an experimental setting; as such, it did not raise any privacy or confidentiality concerns.
We performed AI-based (optimized) and non–AI-based (unoptimized) ethical hacking on the target machine (host IP 192.168.1.44). The AI-based experiment followed the novel ethical hacking framework (see the Methods section). The non–AI-based experiment followed the same method but omitted the optimization module. Table 4 shows the key activities across the different modules according to the proposed 6-stage ethical hacking method.
Key activities for the experiment setting for each of the 2 ethical hacking the methods section.
Both the optimized and the unoptimized ethical hacking were run 50 times (50 runs) each to account for the stochastic nature of ACO and to reduce the uncertainty owing to the simulation environment. The information regarding execution time, the number of exploits investigated, and the number of successful exploits used to construct the attack path was recorded for each run.
Table 5 presents the results of 50 runs of comparison of unoptimized and optimized ethical hacking methods, where the average time used to perform the penetration test, the success rate of all penetration tests, and the highest and average rates of exploits with regard to all exploits were used as comparison metrics. The highest numbers of exploits were 11 and 20, the average numbers of launched exploits were 8 and 14, the numbers of successful penetration tests were 32 and 42, the numbers the highest number of successful exploits were 9 and 18, and the average numbers of successful exploits were 5 and 11 for the unoptimized and optimized ethical hacking methods, respectively.
Comparison of the results of optimized and unoptimized ethical hacking after 50 runs.
Figure 3 depicts in a box plot (each box composed by quartiles 1-3) the total number of launched exploits ( Figure 3 A) and successful exploits ( Figure 3 B) for both optimized and unoptimized ethical hacking methods with the average (indicated by X), median (indicated by straight line across the box), and SD (indicated by whiskers, which might go outside of the box plot or overlap with it). Figure 3 C depicts the box plots of the rate of successful exploits with respect to the total number of exploits, and Figure 3 D shows the average execution time for both optimized and unoptimized ethical hacking methods.

Results of the computational experiments for both unoptimized and optimized ethical hacking methods. (A) Total number of exploits; (B) Number of successful exploits; (C) Success rate results; (D) Average execution time.
To show an example of the results in a single run, the last run out of 50 runs for the unoptimized and optimized ethical hacking methods were compared for the penetration test for 192.168.1.44. The results of the unoptimized method show that the method ran for 177 seconds; out of 9 exploits, 7 were successful; and these exploits were related to improper input validation, cross-site request forgery, remote code execution (in Windows Remote Desktop Gateway), denial of service attacks, improper authentication, remote access backdoors, and the deserialization of untrusted data. In the case of the optimized method, the method ran for 153 seconds, and only 6 exploits were investigated, all of which were used to build a successful attack path.
The details of the exploits used for building a successful path are presented in Table 6 , which are related to remote code execution, cross-site request forgery, improper authentication, vulnerability in the Oracle Business Intelligence Publisher, an elevation of privilege vulnerability (in MediaTek), and remote access backdoor (in the web graphical user interface for the Linux Virtual Server).
Exploits used in the successful attack path found by optimized ethical hacking.
a CVE: common vulnerabilities and exposures
Brief Summary of Findings
In this study, we propose a novel methodological approach to ethical hacking in HISs. We conducted a comparable experiment by launching ethical hacking using both the optimized and unoptimized methods. In particular, we set up an HIS simulation environment by implementing the OpenEMR system and followed the NIST ethical hacking framework to perform ethical hacking. We launched 50 rounds of attacks using both the unoptimized and optimized methods. The results show that the optimized ethical hacking method outperforms the unoptimized method in terms of average time used, the average success rate of exploitation, the number of exploits launched, and the number of successful exploits. We were able to identify the successful attack paths and exploits that are related to remote code execution, cross-site request forgery, improper authentication, vulnerability in the Oracle Business Intelligence Publisher, an elevation of privilege vulnerability (in MediaTek), and remote access backdoor (in the web graphical user interface for the Linux Virtual Server). Theoretically, these findings contribute to HISs, ethical hacking methodology, and mainstream AI-based ethical hacking methods. Practically, the findings have great significance for the health care sector, specifically because OpenEMR is widely adopted by health care organizations.
Implications
Our work contributes to the HIS security domain by proposing an AI-based method for ethical hacking that helps identify vulnerabilities in HISs. In particular, we set up a simulation environment by implementing OpenEMR and performed systematic ethical hacking on this virtual platform. Existing cybersecurity research in health care places emphasis on the protection of medical devices [ 13 - 17 ] and medical data [ 18 ], such as data encryption mechanisms [ 13 ], combined or not with scrambling techniques [ 18 ], managing shared health data [ 14 ], securing digital patient profiles [ 14 ], and authentication protocols for wearable devices [ 15 ]. However, this focus disregards the HIS as a holistic system, which can potentially exhibit vulnerabilities in other functions. In addition, such studies typically do not examine how potential attackers can breach the security of HISs and access, for example, ECG records, that is, other records besides those that are strictly patient focused. In this study, we address this shortcoming by providing an approach that considers and approaches an HIS as a holistic system, whereby the novelty of the AI-driven ethical hacking approach is combined with the familiar NIST framework [ 38 ], which we adapted to perform ethical hacking systematically.
Our study further contributes to the ethical hacking methods section by proposing and validating a novel AI-based ethical hacking method that incorporates optimizing and controlling modules. Several ethical hacking methods exist today, including the NIST ethical hacking framework [ 38 ], PTES, and OWASP. However, they all have limitations. For example, Nettacker, a solution developed by OWASP, contains an optimizing module, but it is not as mature, not fully published, and lacks the exploiting and controlling module. The NIST ethical hacking framework and PTES do not have optimized and controlled modules.
Our study also addressed some of the shortcomings of mainstream AI-based ethical hacking methods. Mainstream methods typically adopt reinforcement learning. Reinforcement learning is an area of machine learning concerned with how intelligent agents ought to take action in an environment to maximize the notion of cumulative rewards. This approach differs from supervised and unsupervised learning because reinforcement learning aims to learn the algorithm to obtain the best results in highly complex and uncertain situations [ 48 ]. However, as previously explained, these methods have not yet been integrated into any ethical hacking methods, and reinforcement learning itself has considerable disadvantages when applied to ethical hacking, owing to its requirement for large data sets, the lack of reliability and predictability (which could cause severe problems for the whole system), low reproducibility, and sensitivity to environmental changes [ 20 ]. The use of ACO in our optimizing module addresses these shortcomings. Our implementation of the ACO algorithm as part of the optimization module shows that it can support the conduct of an efficient vulnerability analysis and detection and offers superior results.
Our proposed AI-based ethical hacking method has practical implications, as it addresses the weaknesses of ethical hacking tools such as Nettacker, APT2, and Autosploit [ 40 ], which are used by cybersecurity practitioners. For example, Nettacker lacks an exploit module. This means that a given user will have to select the exploit tools and payload on their own, which can be challenging for nonexperts in cybersecurity. APT2, the solution offered by the Massachusetts Institute of Technology, uses Nmap to scan information; however, it lacks an optimization module. This finding suggests that the accuracy and efficiency of ethical hacking risks are inferior. Similar to APT2, Autosploit [ 40 ] is a solution that combines Shodan, Censys, Zoomeye, and Metasploit, but it does not have an optimization module. The Metasploit can then run the exploit automatically. However, similar to APT2, Autosploit risks having less accuracy and efficacy because it cannot be optimized. Currently, it is unfeasible to test all possible system configurations.
Our proposed approach addresses these limitations. The combined effect of the 2 new modules is that our approach proposes an intelligent and maintainable ethical hacking solution. First, the incorporation of the optimization module supports the identification of the shortest path for the attack, which improves the efficiency of ethical hacking. Second, incorporating the control module provides a user interface and coordinates the other modules so that ethical hacking can be carried out by nonexperts, addressing the challenge of the shortage of security experts in the health care domain.
Limitations and Future Work
One limitation is that the simulation environment is set up in a virtual environment; although it is portable, it can potentially affect the performance of ethical hacking. As we are running the optimized and unoptimized ethical hacking methods in the same simulation environment, we would assume that this will have a limited impact on the comparable experimental results. Another limitation is that ethical hacking is set up in a network with one system or machine in the simulation environment. In real-world practice, it would be ideal to set up a network with multiple connected machines, so that ethical hacking can target multiple systems or machines.
From a cybersecurity defense perspective, future work should consider applying advanced AI techniques in HISs and explore security defense strategies to counteract cyberattacks. For example, future work could consider exploring other AI algorithms that have been used to resolve the TSP problem (eg, genetic algorithms) in the context of optimizing attack paths in ethical hacking. Future studies could also consider integrating advanced security defense strategies, such as Security Information and Event Management, Orchestration Automation and Response [ 49 ], and security operations centers. From an HIS perspective, future research could focus on building a more mature HIS that integrates diagnostic components such as arrhythmia detection and classification in ambulatory ECGs [ 50 ]. Finally, future research could expand the data set to include data from different medical devices, such as magnetocardiogram and magnetic resonance imaging.
In this study, we proposed a novel AI-based ethical hacking method, which we validated using an HIS simulation platform using OpenEMR as the focal HIS. We incorporated 2 new modules into the NIST ethical hacking framework, namely the optimization and control modules, and demonstrated the ethical hacking of the HIS simulation environment using optimized (AI-based) and unoptimized methods. The results show that the optimized ethical hacking method outperforms the unoptimized method in terms of average time used, the average success rate of exploitation, the number of exploits launched, and the number of successful exploits. We were able to identify the successful attack paths and exploits. Theoretically, the findings contribute to HIS literature, ethical hacking methodology and mainstream AI-based ethical hacking method as they address some key weaknesses of these research fields. Practically, these findings have great significance for the health care sector, as OpenEMR is widely adopted by health care organizations. It also addresses some of the key weaknesses of ethical testing tools used by practitioners.
Acknowledgments
CL was supported by the National Natural Science Foundation of China (grant 61803318) and the Scientific-Technological Collaboration Project (grant 2018LZXNYD-FP02).
We would like to thank Kun Ni for his efforts and participation in the creation of the software system.
Abbreviations
Data availability.
Authors' Contributions: YH is the first author. YH and CL contributed to the conception and design of the study. YH and CL contributed to data acquisition. YH, EZ, IY, and CL contributed to data modeling and analysis. YH contributed to the creation of the software system used in the study. YH drafted the manuscript. YH, CL, EZ, and IY have substantively revised it. All the authors contributed to the final work and approved the final version of the manuscript.
Conflicts of Interest: None declared.
Thank you for visiting nature.com. You are using a browser version with limited support for CSS. To obtain the best experience, we recommend you use a more up to date browser (or turn off compatibility mode in Internet Explorer). In the meantime, to ensure continued support, we are displaying the site without styles and JavaScript.
- View all journals
- Explore content
- About the journal
- Publish with us
- Sign up for alerts
- 10 April 2024
Randomness in computation wins computer-science ‘Nobel’
- Davide Castelvecchi
You can also search for this author in PubMed Google Scholar
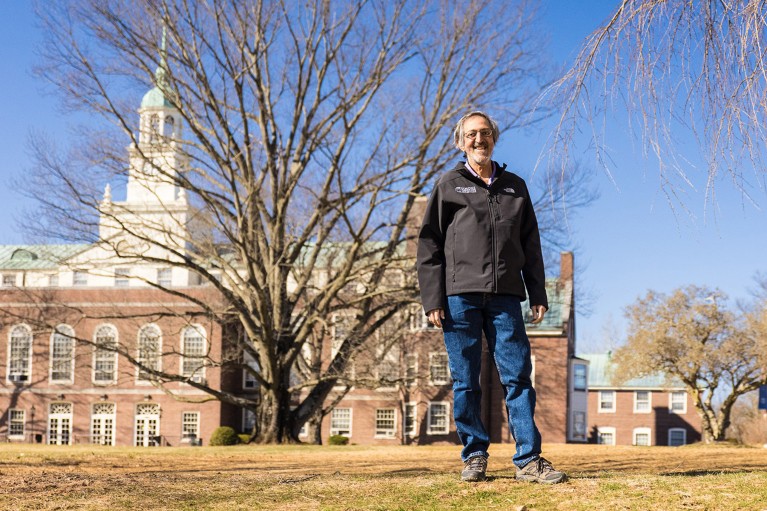
Avi Wigderson received the Turing Award for his foundational contributions to the theory of computation. Credit: Dan Komoda
A leader in the field of computational theory is the latest winner of the A. M. Turing Award, sometimes described as the ‘Nobel Prize’ of computer science.
Avi Wigderson at the Institute for Advanced Study (IAS) in Princeton, New Jersey, is known for work straddling several disciplines, and had already won a share of the Abel Prize , a top mathematics award, three years ago.
He receives the Turing Award “for foundational contributions to the theory of computation, including reshaping our understanding of the role of randomness in computation, and for his decades of intellectual leadership in theoretical computer science”, the Association for Computing Machinery (ACM) in New York City announced on 10 April.
“I was extremely happy, and I didn’t expect this at all,” Wigderson tells Nature . “I’m getting so much love and appreciation from my community that I don’t need prizes.”
‘A towering intellectual force’
Wigderson was born in Haifa, Israel, in 1956. He studied at Technion — Israel Institute of Technology in Haifa and later at Princeton University; he has been at the IAS since 1999. He is known for his work on computational complexity — which studies how certain problems are inherently slow to solve, even in principle — and on randomness in computation. Many practical algorithms make random choices to achieve their objectives more efficiently; in a series of groundbreaking studies in the 1990s, Wigderson and his collaborators showed that conventional, deterministic algorithms can, in principle, be roughly as efficient as ‘randomized’ ones 1 . The results helped to confirm that random algorithms can be as accurate as deterministic ones are.
“Wigderson is a towering intellectual force in theoretical computer science,” said ACM president Yannis Ioannidis in a statement. In addition to Wigderson’s academic achievements, the ACS cited his “friendliness, enthusiasm, and generosity”, which have led him to be a mentor to or collaborate with hundreds of researchers worldwide. Wigderson admits that he is a “big proselytizer” of the intellectual pleasures of his discipline — he wrote a popular book about it and made it freely available on his website . “I think this field is great, and I am happy to explain it to anybody.”
The Turing Award is named after the celebrated British mathematician and code-breaker Alan Turing (1912–54), who in the 1930s laid the conceptual foundations of modern computing. “I feel completely at home with mathematics,” says Wigderson, adding that as an intellectual endeavour, theoretical computer science is indistinguishable from maths. “We prove theorems, like mathematicians.”
doi: https://doi.org/10.1038/d41586-024-01055-y
Impagliazzo, R. & Wigderson, A. in Proc. 29th ACM Symposium on Theory of Computing 220–229 (ACM, 1997).
Download references
Reprints and permissions
Related Articles
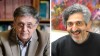
- Mathematics and computing
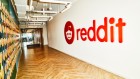
How scientists are making the most of Reddit
Career Feature 01 APR 24
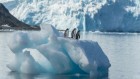
Climate change has slowed Earth’s rotation — and could affect how we keep time
News 27 MAR 24
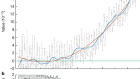
A global timekeeping problem postponed by global warming
Article 27 MAR 24
Group Leader at Católica Biomedical Research Centre and Assistant or Associate Professor at Católica
Group Leader + Assistant/Associate Professor, tenure-track position in Biological and Biomedical Sciences, Data Science, Engineering, related fields.
Portugal (PT)
Católica Biomedical Research Centre
Faculty Positions at SUSTech Department of Biomedical Engineering
We seek outstanding applicants for full-time tenure-track/tenured faculty positions. Positions are available for both junior and senior-level.
Shenzhen, Guangdong, China
Southern University of Science and Technology (Biomedical Engineering)
Locum Associate or Senior Editor, Nature Cancer
To help us to build on the success of Nature Cancer we are seeking a motivated scientist with a strong background in any area of cancer research.
Berlin, Heidelberg or London - Hybrid working model
Springer Nature Ltd
Postdoctoral Research Fellows at Suzhou Institute of Systems Medicine (ISM)
ISM, based on this program, is implementing the reserve talent strategy with postdoctoral researchers.
Suzhou, Jiangsu, China
Suzhou Institute of Systems Medicine (ISM)
The Associate or Senior Editor will contribute to shaping the future of Nature Cancer journal.
Sign up for the Nature Briefing newsletter — what matters in science, free to your inbox daily.
Quick links
- Explore articles by subject
- Guide to authors
- Editorial policies
Princeton University
Princeton engineering, grad alum avi wigderson wins turing award for groundbreaking insights in computer science.
By Scott Lyon
April 10, 2024
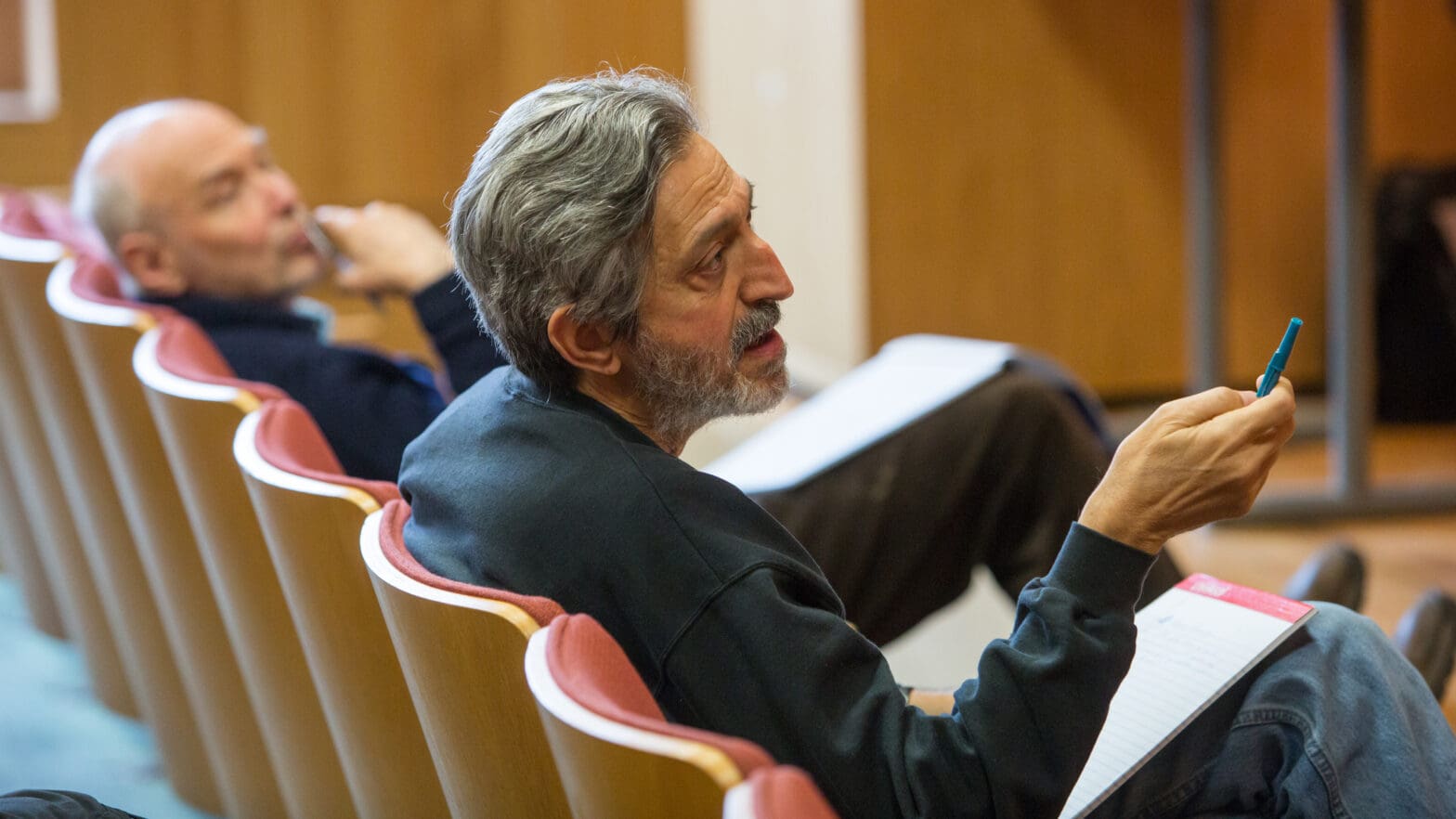
Avi Wigderson has won the 2023 Turing Award from the Association for Computing Machinery. Photos by Andrea Kane, courtesy the Institute for Advanced Study
Princeton graduate alumnus Avi Wigderson has won the 2023 A.M. Turing Award from the Association for Computing Machinery (ACM), recognizing his profound contributions to the mathematical underpinnings of computation.
The Turing Award is considered the highest honor in computer science, often called the “Nobel Prize of Computing.”
Wigderson, the Herbert H. Maass Professor in the Institute for Advanced Study ’s School of Mathematics, earned his Ph.D. from Princeton in 1983 in what was then the Department of Electrical Engineering and Computer Science.
In addition to the Turing Award, he is also the recipient of the 2021 Abel Prize , considered the highest honor in mathematics, from the Norwegian Academy of Science and Letters. He is the only person ever to have won both the Abel Prize and the Turing Award.
“Mathematics is foundational to computer science and Wigderson’s work has connected a wide range of mathematical sub-areas to theoretical computer science,” ACM President Yannis Ioannidis said in a statement released by the organization.
“Avi Wigderson is a giant in the field of theoretical computer science, bringing fundamental insights to deep questions about what can — or cannot — be computed efficiently,” said Jennifer Rexford , Princeton’s provost and Gordon Y.S. Wu Professor of Engineering . “He is also a wonderful colleague and a long-time friend of the University.”
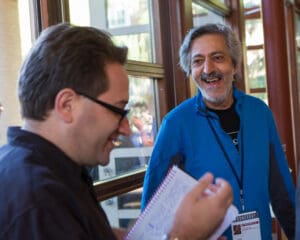
Wigderson is best known for his work on computational complexity theory, especially the role of randomness in computation. Namely, in a series of highly influential works from the 1990s, Wigderson and colleagues proved that computation can be efficient without randomness, shaping algorithm design ever since. He has also established important ideas in several other areas, including protocol design and cryptography, which enables much of today’s digital infrastructure.
While his work is primarily mathematical, the notions he is trying to understand through that work are computational, Wigderson said in a video released by the Institute for Advanced Study (IAS). That approach has earned him a reputation as one of the most versatile minds in either discipline.
“He is one of the most central people in theoretical computer science, generally,” said Ran Raz , a professor of computer science at Princeton, who was Wigderson’s graduate student at the Hebrew University in Jerusalem.
Wigderson has influenced countless students and thinkers, having mentored more than 100 postdocs and collaborated with an unusually broad range of scholars. “He is always able to make connections between things,” Raz said.
“He’s an inspiration,” said Pravesh Kothari , an assistant professor of computer science at Princeton and a former postdoctoral advisee of Wigderson’s at IAS. “He’s a role model. If I could become 10 percent of the researcher he is, it would be a fantastic success for my career.” Kothari also said Wigderson implores young researchers to view the entire endeavor as one field. And that approach shows up in all of his work, connecting disparate problems from sub-disciplines that are normally seen as unrelated.
His research has “set the agenda in theoretical computer science” for decades, Google Senior Vice President Jeff Dean said in the ACM press release. His work has also found its way directly into everyday life.
In a series of findings at the intersection of mathematics and computer science, Wigderson cemented what is known as the zero-knowledge proof, critical in cryptography and digital security. The technique has found purchase in modern applications of privacy, compliance, identity verification and blockchain technology.
Raz said he was amazed at how far Wigderson’s ideas had traveled, from the depths of mathematics to the technologies that enable global enterprise to the everyday lives of billions of people. “It’s quite amazing that these things can be made practical,” Raz said.
Szymon Rusinkiewicz , the David M. Siegel ’83 Professor of Computer Science and department chair, added that Wigderson has been a great friend to Princeton’s computer science community, including to students and young scholars. “He has had a great influence throughout the world of computer science, and we especially feel that at Princeton, where he has been a great mentor and collaborator.”
Wigderson is the recipient of numerous other awards, including the 1994 IMU Abacus Medal, the 2009 Gödel Prize and the 2019 Donald E. Knuth Prize. He is currently a Fellow of the ACM, a member of the American Academy of Arts and Sciences and a member of the National Academy of Sciences.
At Princeton, in addition to his Ph.D., he earned an M.S.E. in 1981, an M.A. in 1982, and he later served on Princeton’s computer science faculty from 1990 to 1992. He joined IAS in 1999, where he established the program in Computer Science and Discrete Mathematics.
Related News
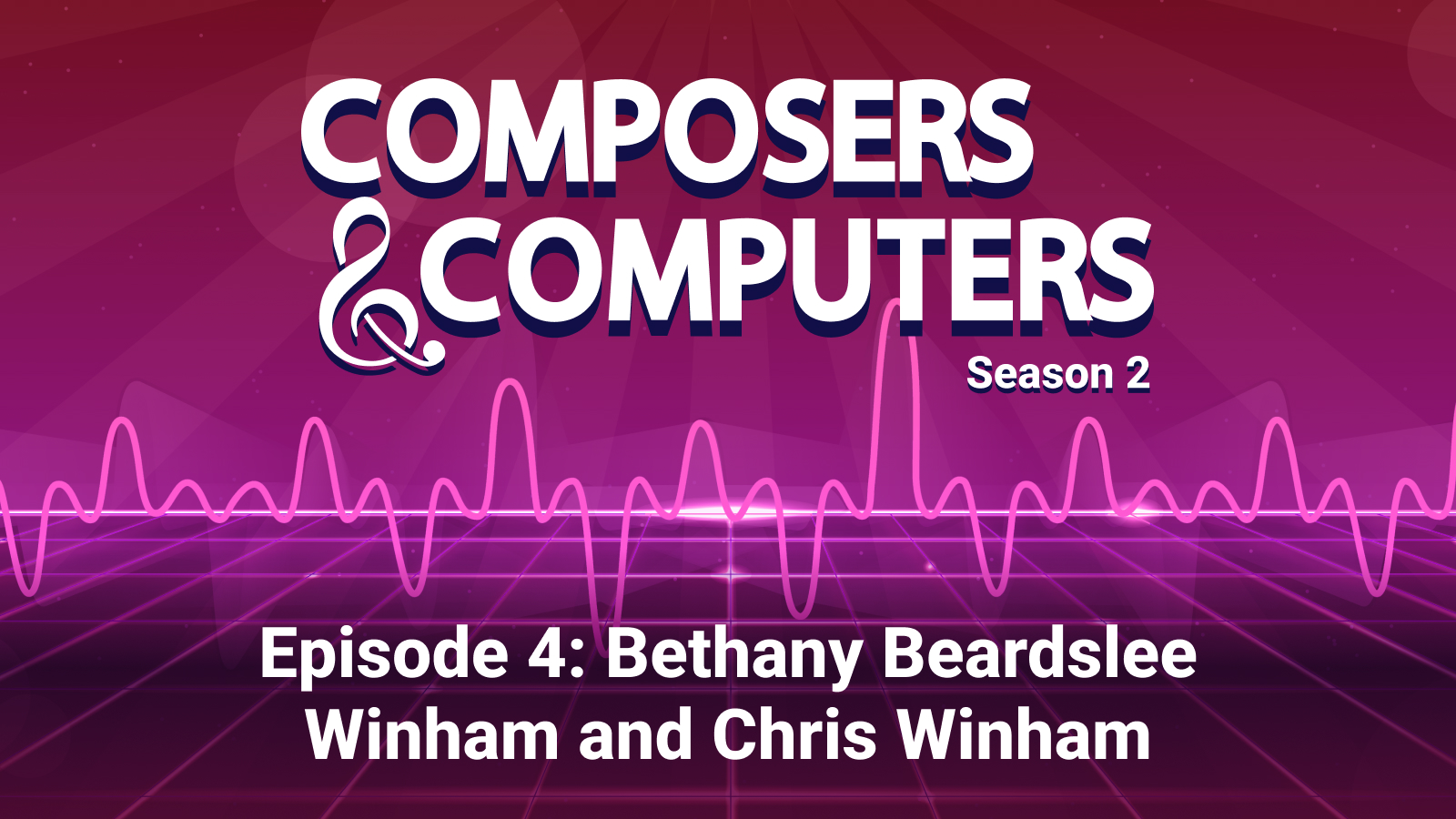
Episode 4: Bethany Beardslee Winham and Chris Winham
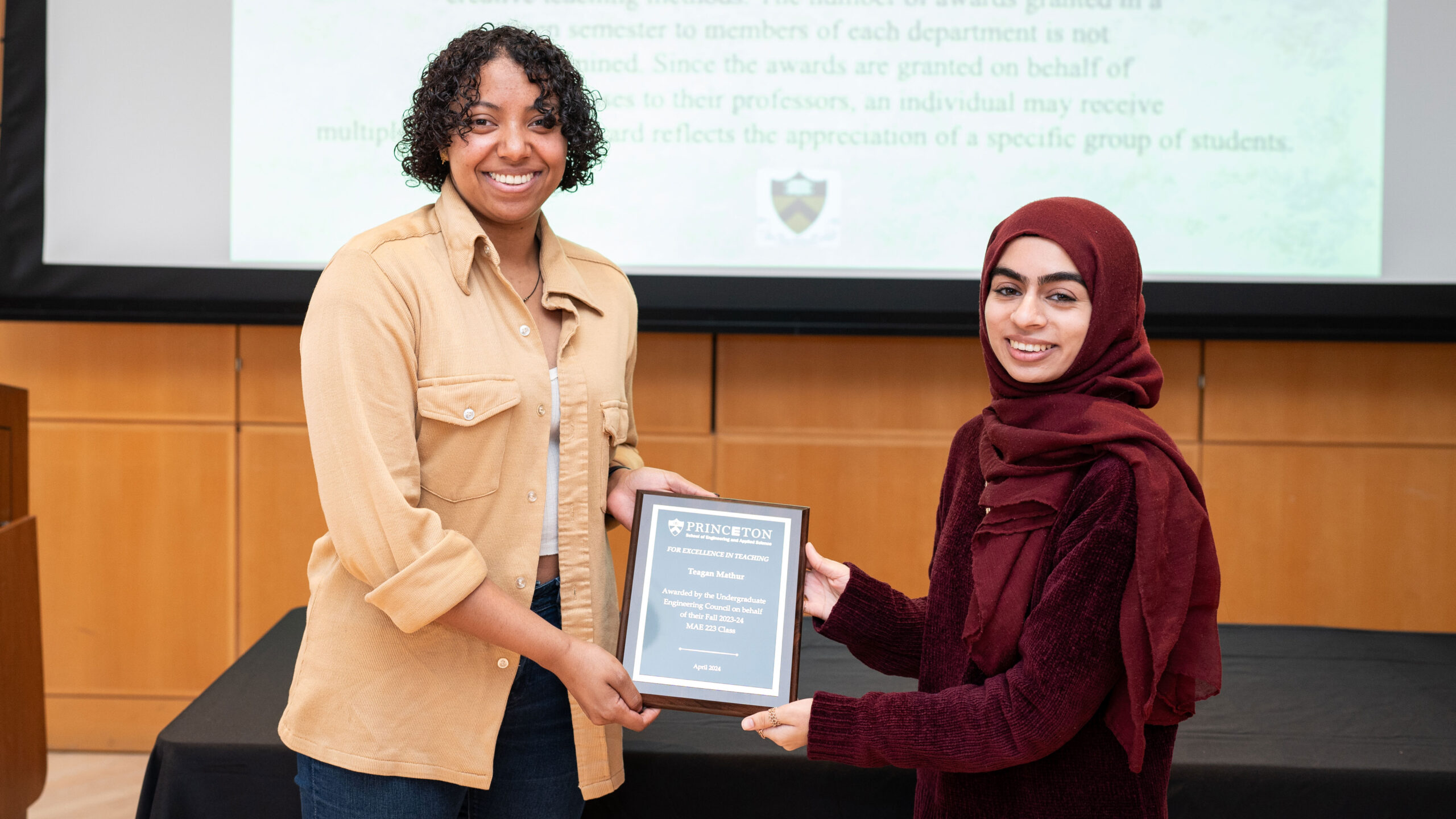
Engineering students recognize exceptional teachers and mentors
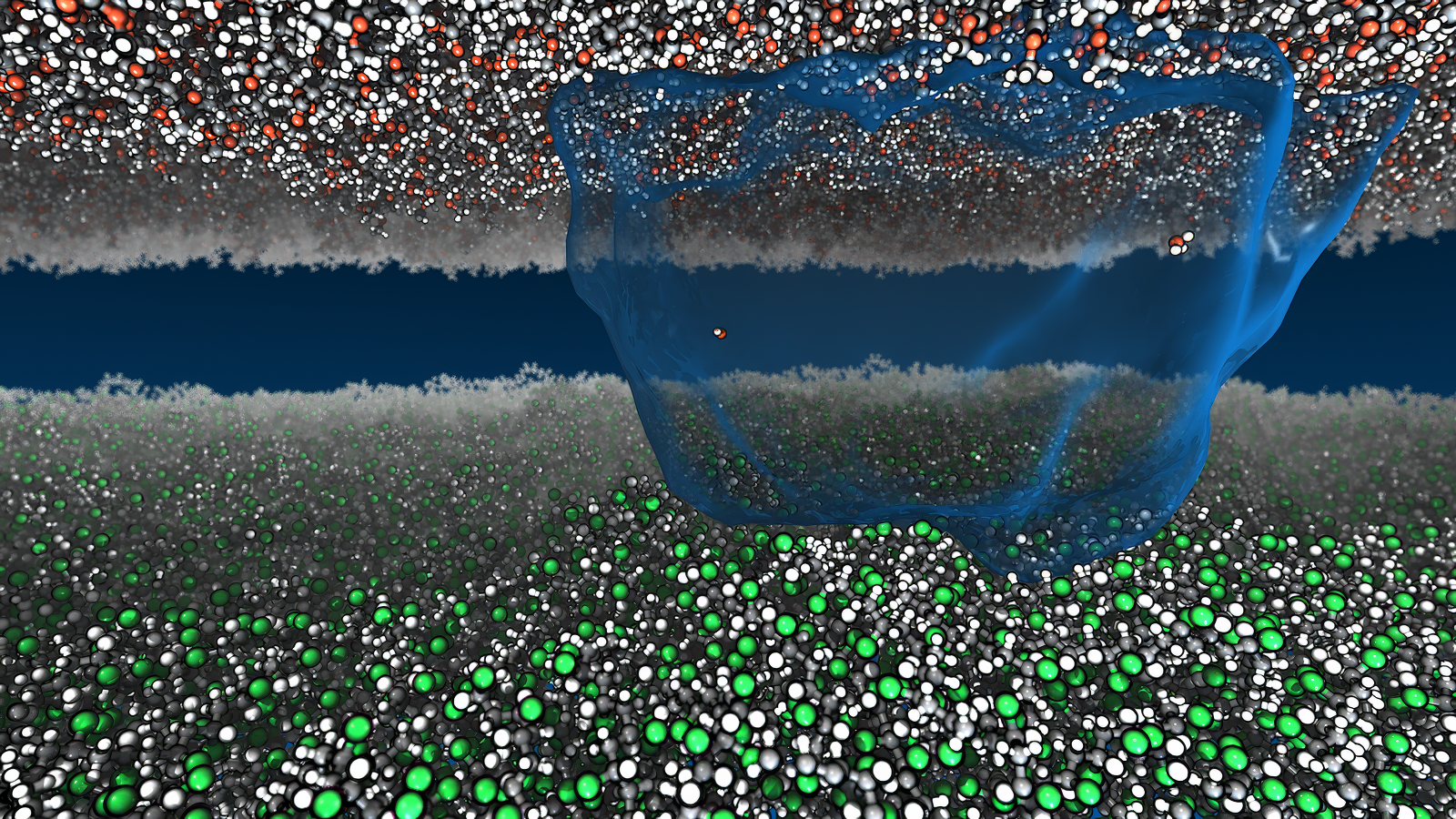
The science of static shock jolted into the 21st century
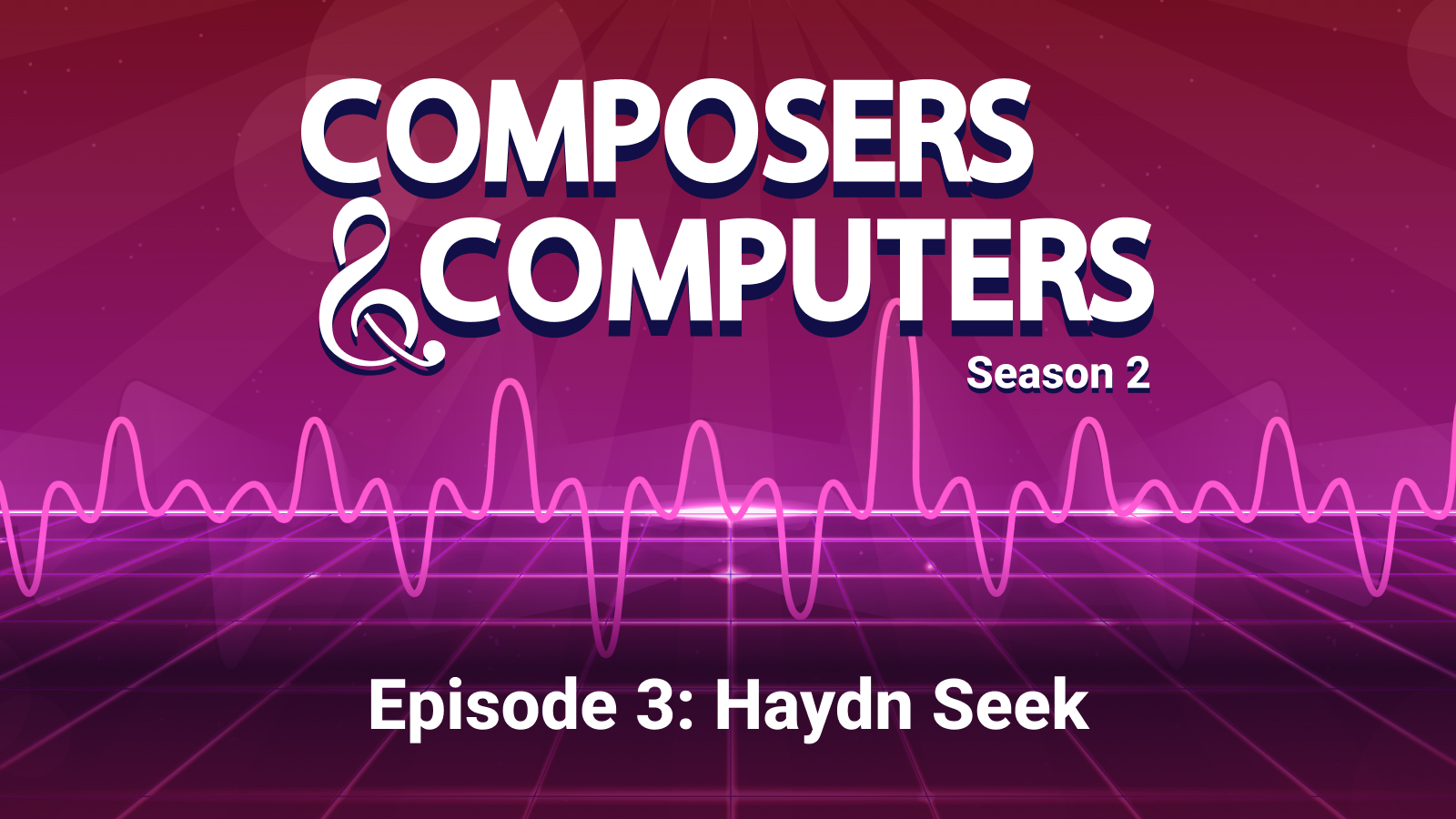
Episode 3: Haydn Seek
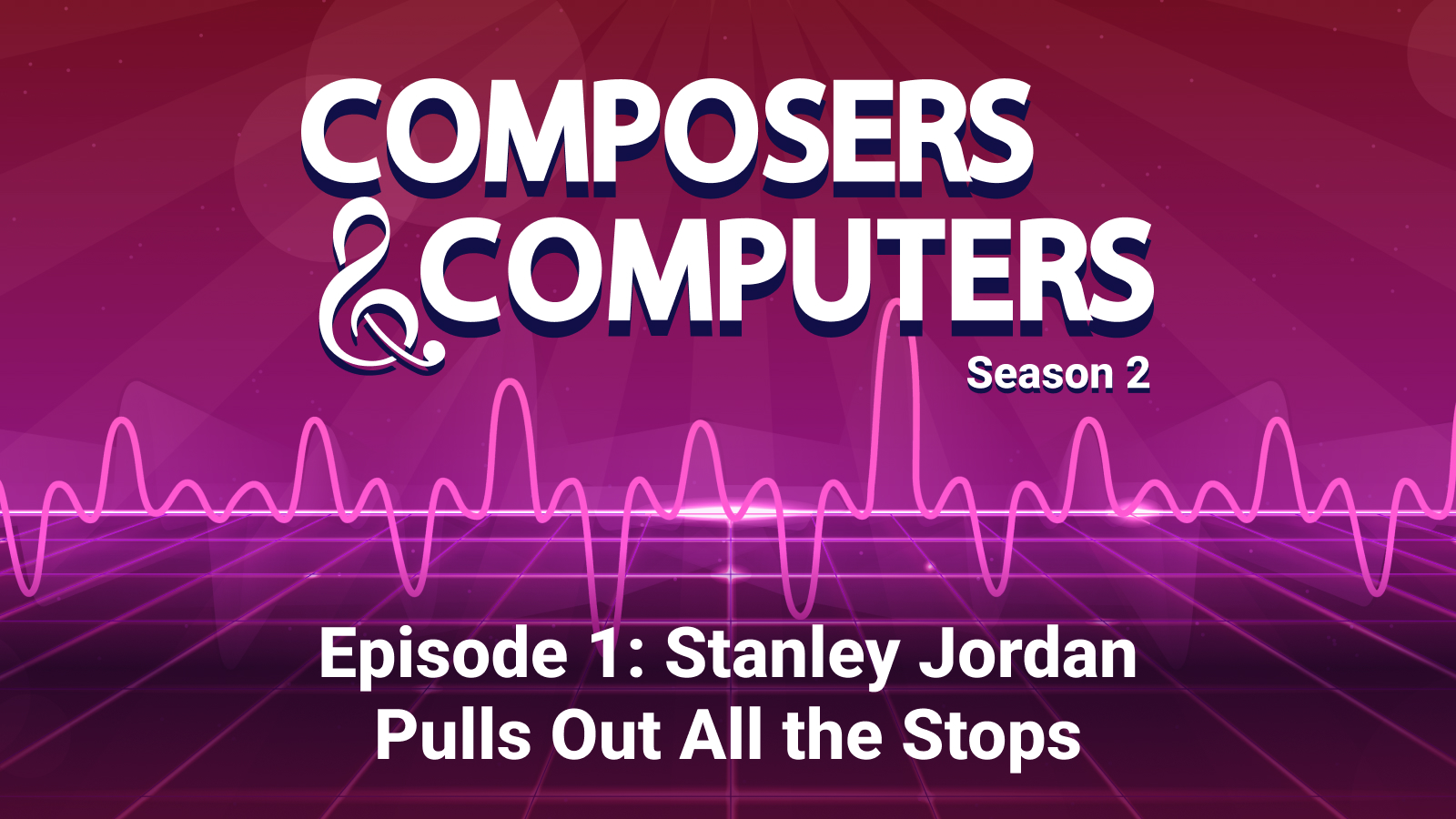
Episode 1: Stanley Jordan Pulls Out All the Stops
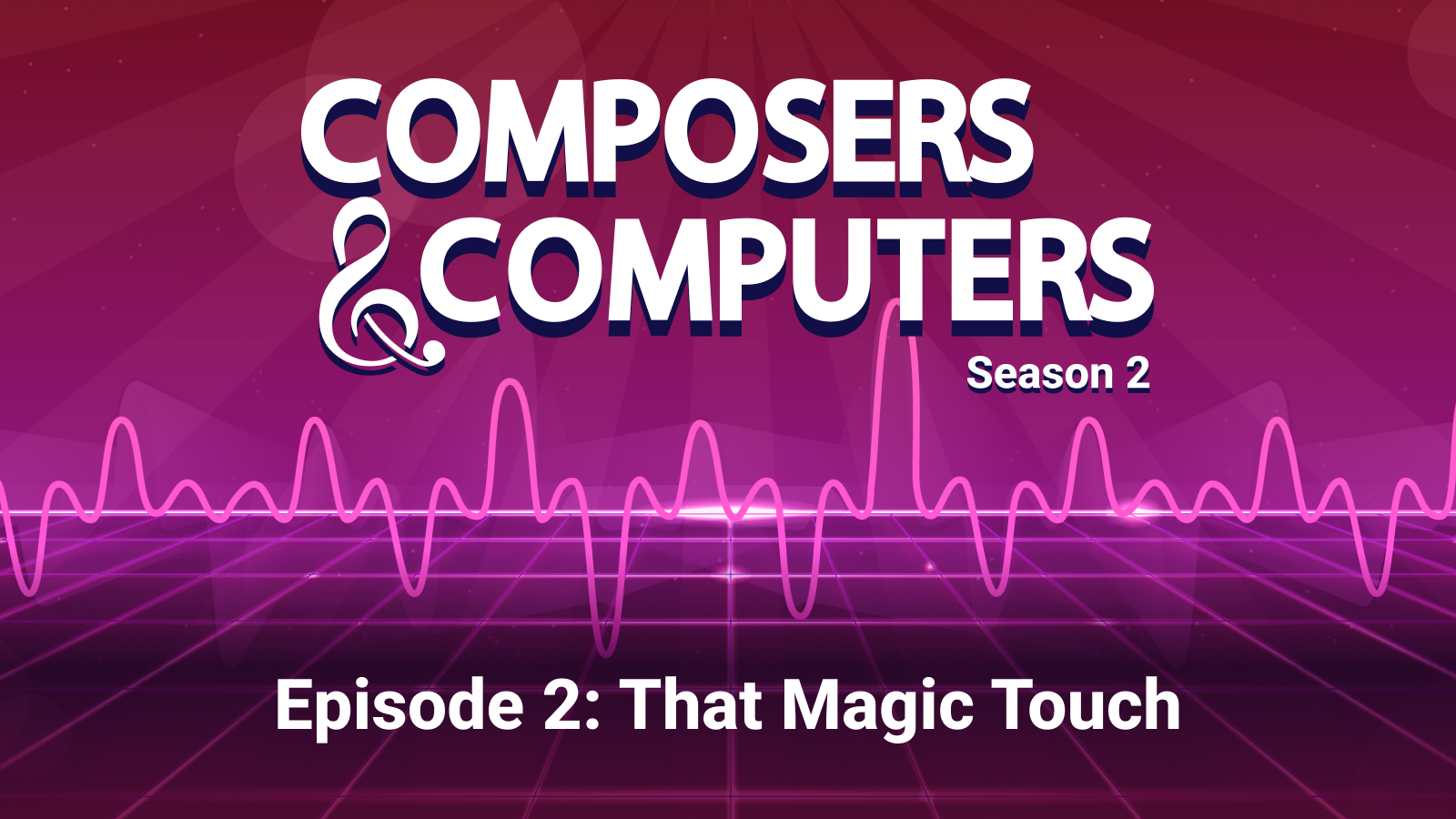
Episode 2: That Magic Touch
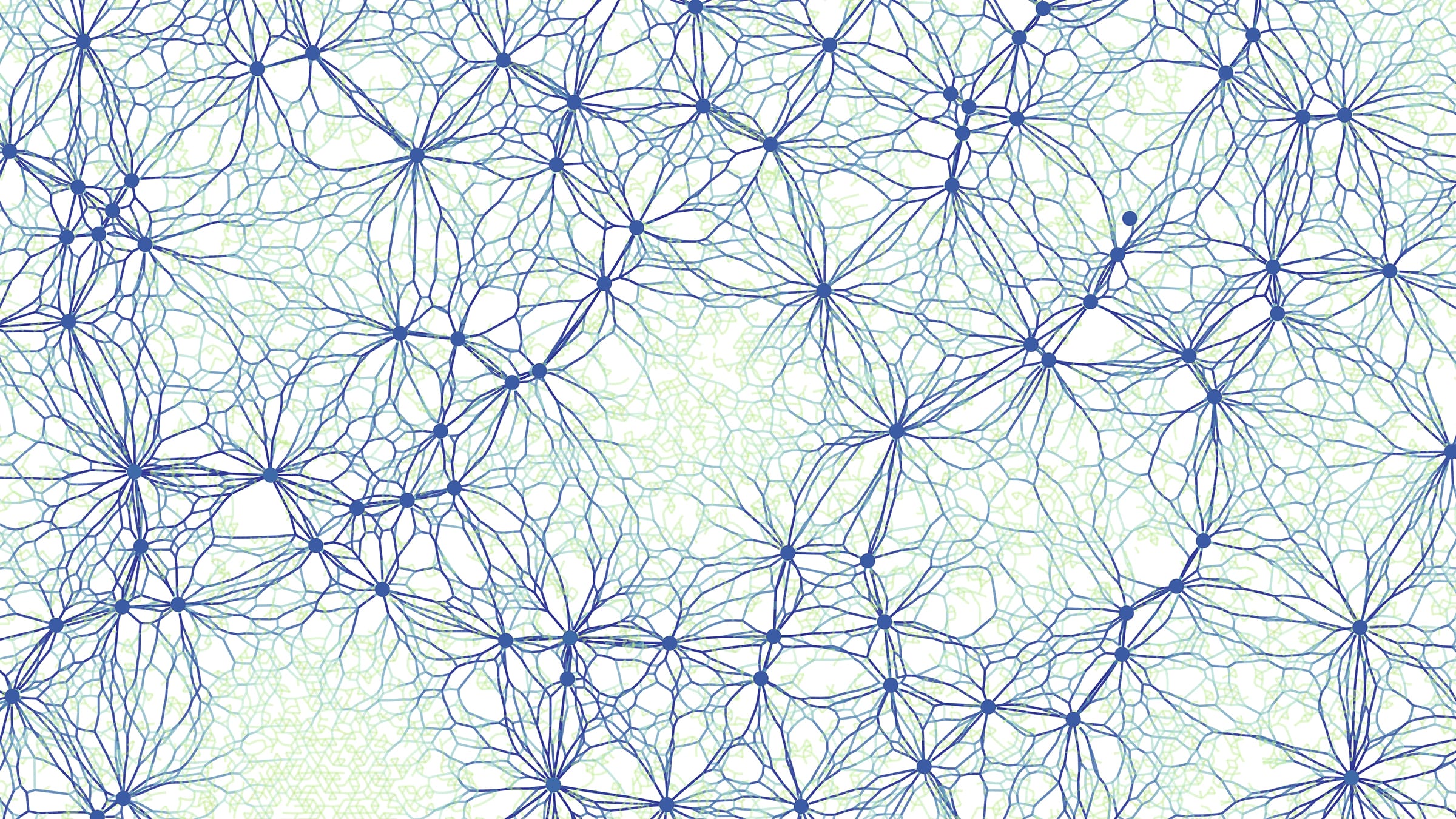
Applied Math
Related department.
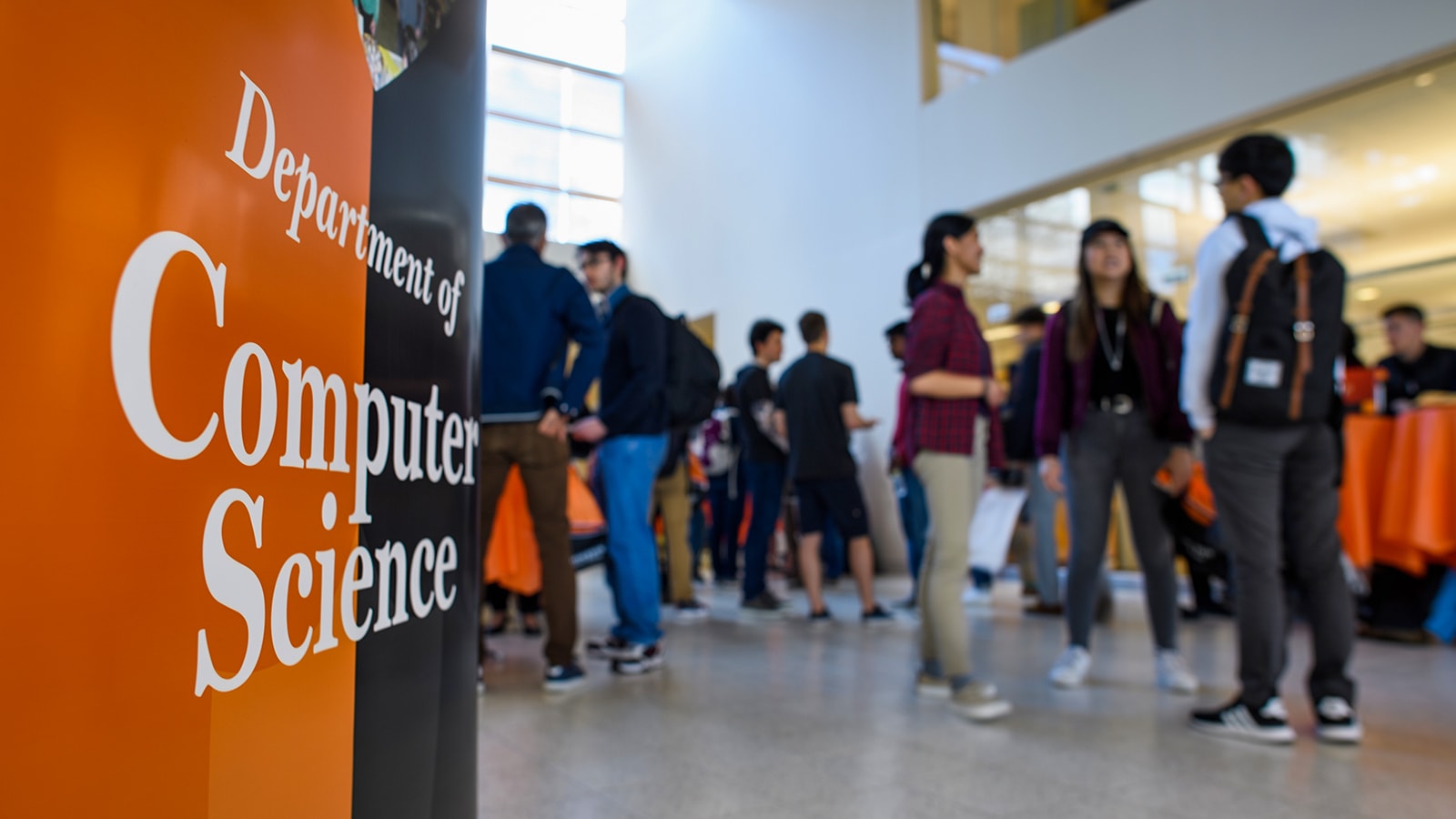
Computer Science
Help | Advanced Search
Computer Science > Human-Computer Interaction
Title: apprentices to research assistants: advancing research with large language models.
Abstract: Large Language Models (LLMs) have emerged as powerful tools in various research domains. This article examines their potential through a literature review and firsthand experimentation. While LLMs offer benefits like cost-effectiveness and efficiency, challenges such as prompt tuning, biases, and subjectivity must be addressed. The study presents insights from experiments utilizing LLMs for qualitative analysis, highlighting successes and limitations. Additionally, it discusses strategies for mitigating challenges, such as prompt optimization techniques and leveraging human expertise. This study aligns with the 'LLMs as Research Tools' workshop's focus on integrating LLMs into HCI data work critically and ethically. By addressing both opportunities and challenges, our work contributes to the ongoing dialogue on their responsible application in research.
Submission history
Access paper:.
- HTML (experimental)
- Other Formats

References & Citations
- Google Scholar
- Semantic Scholar
BibTeX formatted citation

Bibliographic and Citation Tools
Code, data and media associated with this article, recommenders and search tools.
- Institution
arXivLabs: experimental projects with community collaborators
arXivLabs is a framework that allows collaborators to develop and share new arXiv features directly on our website.
Both individuals and organizations that work with arXivLabs have embraced and accepted our values of openness, community, excellence, and user data privacy. arXiv is committed to these values and only works with partners that adhere to them.
Have an idea for a project that will add value for arXiv's community? Learn more about arXivLabs .
- Anniston/Gadsden
As Birmingham computer outage continues, city using paper time sheets
- Updated: Apr. 02, 2024, 6:41 a.m. |
- Published: Apr. 02, 2024, 6:30 a.m.

Birmingham City Hall (Al.com file)
- Joseph D. Bryant | [email protected]
For weeks, Birmingham city workers have been conducting business the old fashioned way — on paper — as many computer systems experienced what Mayor Randall Woodfin’s office called a “network disruption.”
Multiple government sources have told AL.com that the city is the victim of a ransomware attack, with hackers gaining access to the city’s computer systems and demanding payment for the city to get its data back.
“It’s incredibly serious,” said one source at City Hall, speaking on the condition of anonymity because of a longstanding practice that the city’s communication office presents official information to the public.
Rick Journey, the mayor’s director of communications, declined to answer whether the city was the victim of a hacker. He said the city will provide more details later.
“As we have shared with city employees, pay will continue uninterrupted. If they have any questions, we encourage them to contact their payroll coordinator within their departments,” Journey told AL.com. “Finance and HR stand ready to assist those payroll coordinators and did so last week after the pay period to address any concerns.”
Stephen R. Cook, president of the Birmingham Firefighters Association, said that city employees are filling out paper time sheets because of the computer outage.
“Nobody knows if they’re being paid the correct rates and the correct amounts of time because we aren’t getting pay stubs,” he said. “There are a lot of manual processes in place with the network out.”
“There are still some problems to figure out in that manual process that they are working through that need to be clarified, but the finance department is 100 percent willing to make sure that everyone is taken care of and is compensated correctly,” Cook said.
Councilwoman Valerie Abbott said the city council has not received an official briefing. She and two other council members deferred to the mayor’s office to speak as authorities on the computer outage.
“To our knowledge it’s not having a big effect on residents. It is having an impact on businesses and people who are coming to get permits,” said Abbott. “I haven’t had anyone call and say, ‘my garbage isn’t being picked up.’”
City officials have stressed that the 911 system has not been affected. Emergency operations remain functional, Journey has said.
Earlier in the outage, the city’s 3-1-1 telephone information system was knocked offline, and digital payments were restricted. But some operations have since resumed. For instance, patrons are again able to make digital payments for city permits.
A ransomware attack happens when a hacker installs malware to lock computer systems and take vital information, then demands money from individuals, companies or governments to get it back.
It has happened to governments across the country. In Alabama, an attack affected services at the Cullman County Revenue Commissioner’s office in 2023. Montgomery County leaders in 2017 paid $37,000 in a ransomware attack to retrieve its data from criminal hackers.
Steve Morgan, founder of Cybersecurity Ventures and editor-in-chief at Cybercrime Magazine, told AL.com it’s not uncommon for governments to try keeping hacks secret.
“Unfortunately, all too often these start out as so-called ‘outages’ or ‘glitches’ or other descriptions so as to avoid reputational harm, embarrassment, or for other reasons,” said Morgan. “Oftentimes the victim organization will finally come forward announcing that it was in fact a breach or malicious intrusion.”
His national publication tracks new cyberattacks and data breaches in its daily “Who’s Hacked” feed .
“There are incidents when a city or municipality on the advice of law enforcement and/or an outside cybersecurity expert or company may deem it in the best interest of the organization to hold back information until such time that an incident has been fully investigated,” Morgan said.
If you purchase a product or register for an account through a link on our site, we may receive compensation. By using this site, you consent to our User Agreement and agree that your clicks, interactions, and personal information may be collected, recorded, and/or stored by us and social media and other third-party partners in accordance with our Privacy Policy.
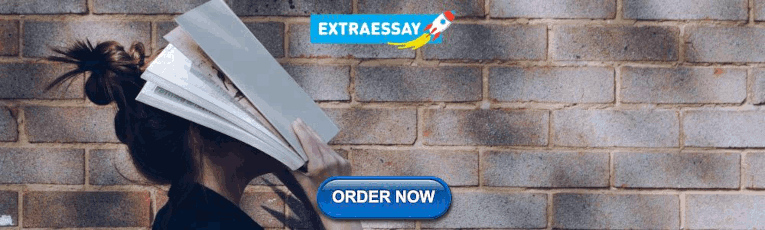
IMAGES
VIDEO
COMMENTS
Understanding and predicting cyber malfeasance is an emerging area of research with the increase in cybercrimes and heightened awareness about cybersecurity in recent years. ... A Hacker's Guide to Computer Security, Microsoft Press, Bellevue, Washington (1985) Google Scholar. ... Amsterdam Law School Research Paper (2018), pp. 2018-2021 ...
Accordingly, the motivations to hack were divided into four themes: 1) compulsion to hack, 2) curiosity, 3) control and attraction to power, and. 4) peer recognition and belonging to a group ...
In 2018, 978 million people globally fell victim to online crime, or cybercrime (Symantec Corporation, 2019).Cybercrime refers to a broad range of criminal activity committed using computers or the internet and encompasses a wide range of offenses such as cyber-stalking, harassment, online fraud, phishing and hacking (Morgan et al., 2016).With the rapid digitization of society, trends indicate ...
Hacking is often used as a catchall to cover all forms of 'unauthorised access to or use of a computer system', but can encompass a very large range of different actors, intensions and activities (Conway 2003: 10; Barber 2001).From criminal hackers, or 'crackers', who maliciously attack or defraud systems for personal gain (Sheoran and Singh 2014: 112); to 'Skript Kiddies', often ...
Like most criminological research, much of the research on hacking has predominantly focused upon the Northern Metropolis. As a result, there is a lack of focus on cybercrime within the Global South, particularly on illegal intrusions into computer systems, more colloquially known as hacking. This article provides a critical overview of hacking in the Global South, highlighting the role of ...
These cyberattacks originate from hard-to-trace sources and often consist of software known as viruses, worms, bots or Trojan horses, depending on how they infect, proliferate and inflict damage ...
One of the reasons that research on hackers has been so limited is that there is no clear definition of what a hacker is, or who may or may not be considered a hacker. Researchers have attempted to define the term hacker, yet overall attempts to craft a definition have been inconsistent and partially complete.
Offensive security-tests are a common way to pro-actively discover potential vulnerabilities. They are performed by specialists, often called penetration-testers or white-hat hackers. The chronic lack of available white-hat hackers prevents sufficient security test coverage of software. Research into automation tries to alleviate this problem by improving the efficiency of security testing. To ...
1. Introduction. Under a term, the hacking one can include any unconventional. way of interacting with systems, i.e. interaction in the way that. was not foreseen as a standard by the designer, [1 ...
Cybercrime is estimated to have cost the global economy just under USD 1 trillion in 2020, indicating an increase of more than 50% since 2018. With the average cyber insurance claim rising from USD 145,000 in 2019 to USD 359,000 in 2020, there is a growing necessity for better cyber information sources, standardised databases, mandatory reporting and public awareness. This research analyses ...
With the significant growth of internet usage, people increasingly share their personal information online. As a result, an enormous amount of personal information and financial transactions become vulnerable to cybercriminals. Phishing is an example of a highly effective form of cybercrime that enables criminals to deceive users and steal important data. Since the first reported phishing ...
Today, social engineering techniques are the most common way of committing cybercrimes through the intrusion and infection of computer systems and information technology (IT) infrastructures (Abraham and Chengalur-Smith 2010, 183).Cybersecurity experts use the term "social engineering" to highlight the "human factor" in digitized systems.
Most ethical hackers, also known as black hat hackers, test systems using different approaches, methodologies, and tools. Because today's life is lived in a digital world, we need to protect our ...
This research addresses a challenge of the hacker classification framework based on the "big five personality traits" model (OCEAN) and explores associations between personality traits and hacker types. The method's application prediction performance was evaluated in two groups: Students with hacking experience who intend to pursue information security and ethical hacking and industry ...
Andrew Ilyas, a computer scientist at the Massachusetts Institute of Technology (MIT) in Cambridge, and one of the paper's authors, says engineers could change the way they train AI. Current methods of securing an algorithm against attacks are slow and difficult. But if you modify the training data to have only human-obvious features, any ...
This paper debates identity and community issues by using computer hackers as an example of shaping identities in virtual communities. The aim is to show that individuals use strategies within these communities to craft identity and use information to assert dominance over less informed hackers.
Ethical hacking is a technique which is used to identify the weaknesses and vulnerabilities in the system or computer network in order to strengthen the system further to prevent the data. The main reason behind studying ethical hacking is to evaluate target system security. This paper helps to generate a brief idea of ethical hacking and all ...
Research concerning computer hackers generally focuses on how to stop them; far less attention is given to the texts they create. Phrack, an online hacker journal that has run almost continuously since 1985, is an important touchstone in hacker literature, widely read by both hackers and telephone and network security professionals.
Therefore, those that conduct computer hacking are commonly known as the hackers (Kumar & Agarwal, 2018 ... This research paper describes what ethical hacking is, what it can do, an ethical ...
Health information systems (HISs) are continuously targeted by hackers, who aim to bring down critical health infrastructure. This study was motivated by recent attacks on health care organizations that have resulted in the compromise of sensitive data held in HISs. Existing research on cybersecurity in the health care domain places an ...
Randomness in computation wins computer-science 'Nobel'. Computer scientist Avi Wigderson is known for clarifying the role of randomness in algorithms, and for studying their complexity. By ...
Computer Science > Computation and Language. arXiv:2403.20329 (cs) ... This paper demonstrates how LLMs can be used to create an extremely effective system to resolve references of various types, by showing how reference resolution can be converted into a language modeling problem, despite involving forms of entities like those on screen that ...
We address prevailing challenges of the brain-powered research, departing from the observation that the literature hardly recover accurate spatial information and require subject-specific models. To address these challenges, we propose UMBRAE, a unified multimodal decoding of brain signals. First, to extract instance-level conceptual and spatial details from neural signals, we introduce an ...
Hackers are computer gurus who are knowledgeable about both hardware and software. [12] TYPES OF HACKERS-1. White Hat Hackers A white hat hacker hacks into a company's or organization's secured ...
Szymon Rusinkiewicz, the David M. Siegel '83 Professor of Computer Science and department chair, added that Wigderson has been a great friend to Princeton's computer science community, including to students and young scholars. "He has had a great influence throughout the world of computer science, and we especially feel that at Princeton ...
Cyber security is the field of technolog ies, processes and activities designed to. protect you f rom h ackers, viruses and malwares. It deals with both security and computer security. Hardware ...
Large Language Models (LLMs) have emerged as powerful tools in various research domains. This article examines their potential through a literature review and firsthand experimentation. While LLMs offer benefits like cost-effectiveness and efficiency, challenges such as prompt tuning, biases, and subjectivity must be addressed. The study presents insights from experiments utilizing LLMs for ...
Stephen R. Cook, president of the Birmingham Firefighters Association, said that city employees are filling out paper time sheets because of the computer outage. "Nobody knows if they're being ...