
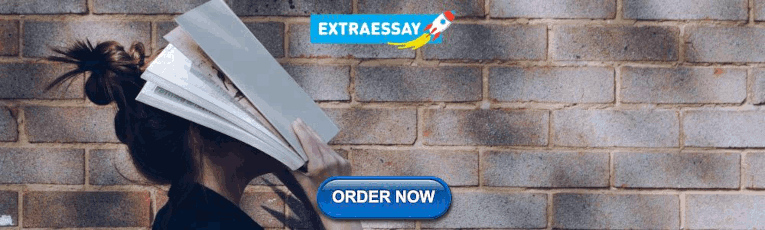
7 QC Tools for Process Improvement | PDF | Case Study

From Where Did the 7 QC Tools Come?

Why we use The 7 QC Tools for Process Improvement?
What is the use of 7 qc tools.

The 7 QC Tools:
- Flow Charts
- Cause and Effect Diagram (Fishbone or Ishikawa)
- Pareto Chart
- Scatter Diagram
- Control Chart
👉 Download 7 QC Tools PDF file
[1] flow charts :.
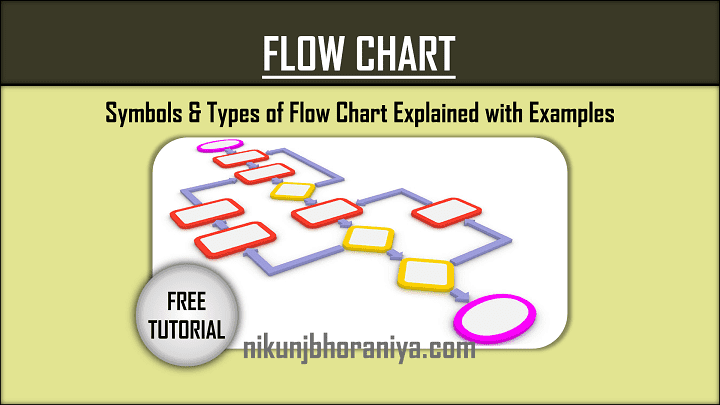
[2] Cause and Effect Diagram :
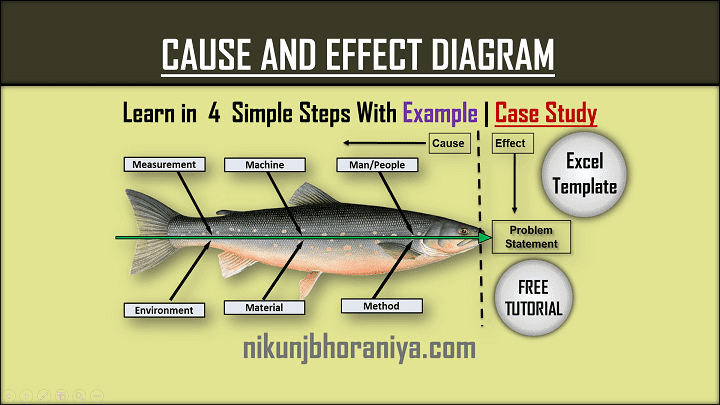
[3] Check Sheet :

[4] Histogram :
➨ types of histogram:.

[5] Pareto Chart :

[6] Scatter Diagram :
➨ different names of the scatter diagram:, ➨ different correlation between two variables in the scatter plot:.

[7] Control Chart :

Related Posts
22 comments.

very good presentation skill and to the point explaination

Thanks for your feedback and kind comment!!!
How to download???
You can check the individual articles!!!
Best in short... Great work Nikunj
Thank you very much for your kind comment!!!
Simply wonderful. Thanks very much!
this is a great initiative , well done
Thank you for your kind words!!!
Thanks and happy learning!!!
Nice teaching
Thank you and Happy Learning!!!
Great good initiative 👍 a How to Download
Thank you for your kind word!!
This is so helpful
Thanks for your feedback
HOW MAKE PARETO
Sir can you please share process audit checklist
You can reach us at: [email protected]
Post a Comment
Contact form.
To read this content please select one of the options below:
Please note you do not have access to teaching notes, an empirical study into the use of 7 quality control tools in higher education institutions (heis).
The TQM Journal
ISSN : 1754-2731
Article publication date: 13 September 2022
Issue publication date: 5 September 2023
The main purpose of this study is to revisit Ishikawa's statement: “95% of problems in processes can be accomplished using the original 7 Quality Control (QC) tools”. The paper critically investigates the validity of this statement in higher education institutions (HEIs). It involves analysis of the usage of the 7 QC tools and identifying the barriers, benefits, challenges and critical success factors (CSFs) for the application of the 7 QC tools in a HEI setting.
Design/methodology/approach
An online survey instrument was developed, and as this is a global study, survey participants were contacted via social networks such as LinkedIn. Target respondents were HEIs educators or professionals who are knowledgeable about the 7 QC tools promulgated by Dr Ishikawa. Professionals who work in administrative sectors, such as libraries, information technology and human resources were included in the study. A number of academics who teach the 7 basic tools of QC were also included in the study. The survey link was sent to over 200 educators and professionals and 76 complete responses were obtained.
The primary finding of this study shows that the diffusion of seven QC tools is not widespread in the context of HEIs. Less than 8% of the respondents believe that more than 90% of process problems can be solved by applying the 7 QC tools. These numbers show that modern-quality problems may need more than the 7 basic QC basic tools and there may be a need to revisit the role and contribution of these tools to solve problems in the higher education sector. Tools such as Pareto chart and cause and effect diagram have been widely used in the context of HEIs. The most important barriers highlighted are related to the lack of knowledge about the benefits and about how and when to apply these tools. Among the challenges are the “lack of knowledge of the tools and their applications” and “lack of training in the use of the tools”. The main benefits mentioned by the respondents were “the identification of areas for improvement, problem definition, measurement, and analysis”. According to this study, the most important factors critical for the success of the initiative were “management support”, “widespread training” and “having a continuous improvement program in place”.
Research limitations/implications
The exploratory study provides an initial understanding about the 7 QC tools application in HEIs, and their benefits, challenges and critical success factors, which can act as guidelines for implementation in HEIs. Surveys alone cannot provide deeper insights into the status of the application of 7 QC tools in HEIs, and therefore qualitative studies in the form of semi-structured interviews should be carried out in the future.
Originality/value
This article contributes with an exploratory empirical study on the extent of the use of 7 QC tools in the university processes. The authors claim that this is the first empirical study looking into the use of the 7 QC tools in the university sector.
- 7 quality control tools
- Higher education institutions (HEIs)
- Quality improvement
Mathur, S. , Antony, J. , Olivia, M. , Fabiane Letícia, L. , Shreeranga, B. , Raja, J. and Ayon, C. (2023), "An empirical study into the use of 7 quality control tools in higher education institutions (HEIs)", The TQM Journal , Vol. 35 No. 7, pp. 1777-1798. https://doi.org/10.1108/TQM-07-2022-0222
Emerald Publishing Limited
Copyright © 2022, Emerald Publishing Limited
Related articles
We’re listening — tell us what you think, something didn’t work….
Report bugs here
All feedback is valuable
Please share your general feedback
Join us on our journey
Platform update page.
Visit emeraldpublishing.com/platformupdate to discover the latest news and updates
Questions & More Information
Answers to the most commonly asked questions here

What are the 7 basic quality tools, and how can they change your business for the better?
Reading time: about 6 min
What are the 7 basic quality tools?
- Stratification
- Check sheet (tally sheet)
- Cause and effect diagram (fishbone or Ishikawa diagram)
- Pareto chart (80-20 rule)
- Scatter diagram
- Control chart (Shewhart chart)
The ability to identify and resolve quality-related issues quickly and efficiently is essential to anyone working in quality assurance or process improvement. But statistical quality control can quickly get complex and unwieldy for the average person, making training and quality assurance more difficult to scale.
Thankfully, engineers have discovered that most quality control problems can be solved by following a few key fundamentals. These fundamentals are called the seven basic tools of quality.
With these basic quality tools in your arsenal, you can easily manage the quality of your product or process, no matter what industry you serve.
Learn about these quality management tools and find templates to start using them quickly.
Where did the quality tools originate?
Kaoru Ishikawa, a Japanese professor of engineering, originally developed the seven quality tools (sometimes called the 7 QC tools) in the 1950s to help workers of various technical backgrounds implement effective quality control measures.
At the time, training programs in statistical quality control were complex and intimidating to workers with non-technical backgrounds. This made it difficult to standardize effective quality control across operations. Companies found that simplifying the training to user-friendly fundamentals—or seven quality tools—ensured better performance at scale
7 quality tools
1. stratification.
Stratification analysis is a quality assurance tool used to sort data, objects, and people into separate and distinct groups. Separating your data using stratification can help you determine its meaning, revealing patterns that might not otherwise be visible when it’s been lumped together.
Whether you’re looking at equipment, products, shifts, materials, or even days of the week, stratification analysis lets you make sense of your data before, during, and after its collection.
To get the most out of the stratification process, consider which information about your data’s sources may affect the end results of your data analysis. Make sure to set up your data collection so that that information is included.
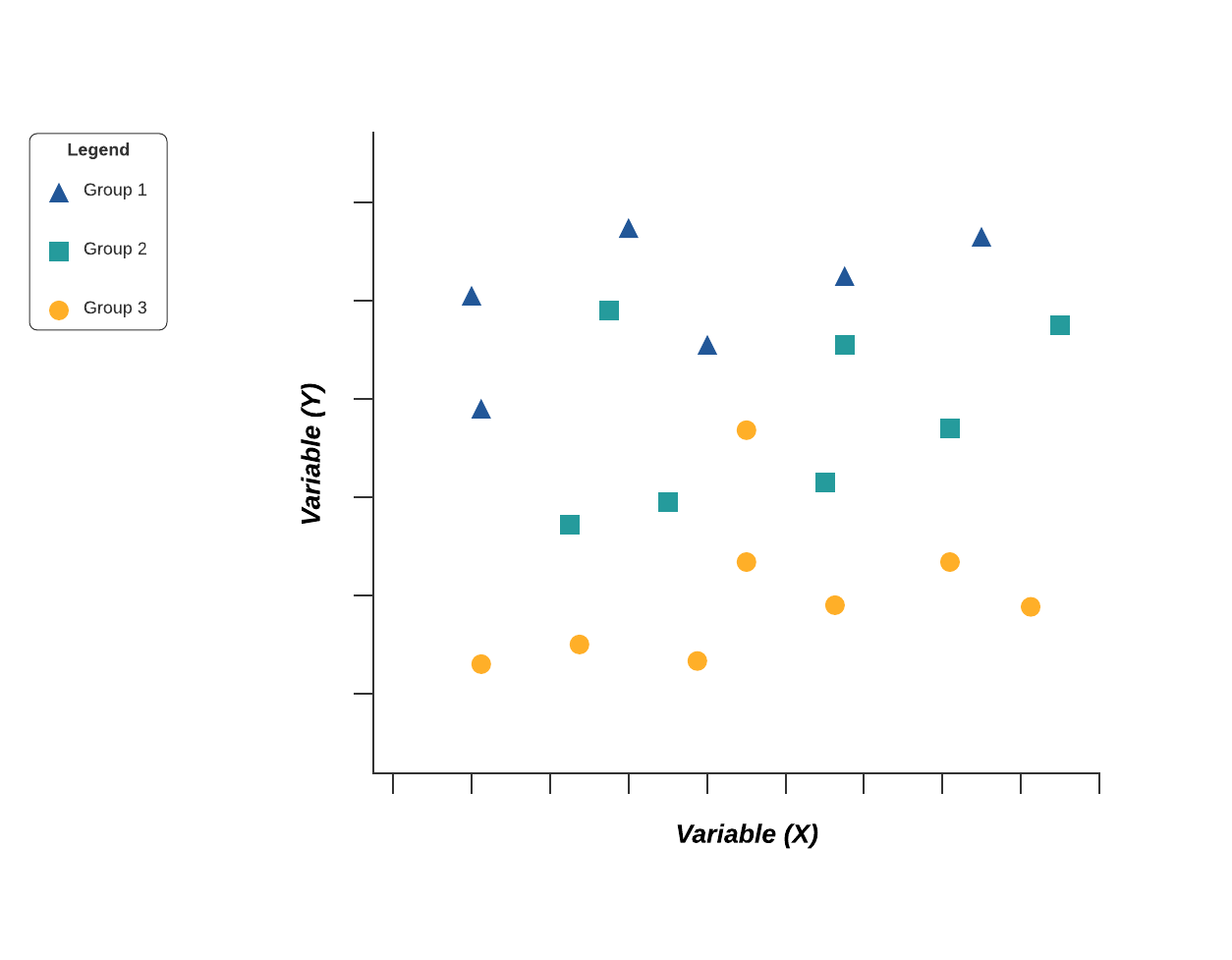
2. Histogram
Quality professionals are often tasked with analyzing and interpreting the behavior of different groups of data in an effort to manage quality. This is where quality control tools like the histogram come into play.
The histogram represents frequency distribution of data clearly and concisely amongst different groups of a sample, allowing you to quickly and easily identify areas of improvement within your processes. With a structure similar to a bar graph, each bar within a histogram represents a group, while the height of the bar represents the frequency of data within that group.
Histograms are particularly helpful when breaking down the frequency of your data into categories such as age, days of the week, physical measurements, or any other category that can be listed in chronological or numerical order.
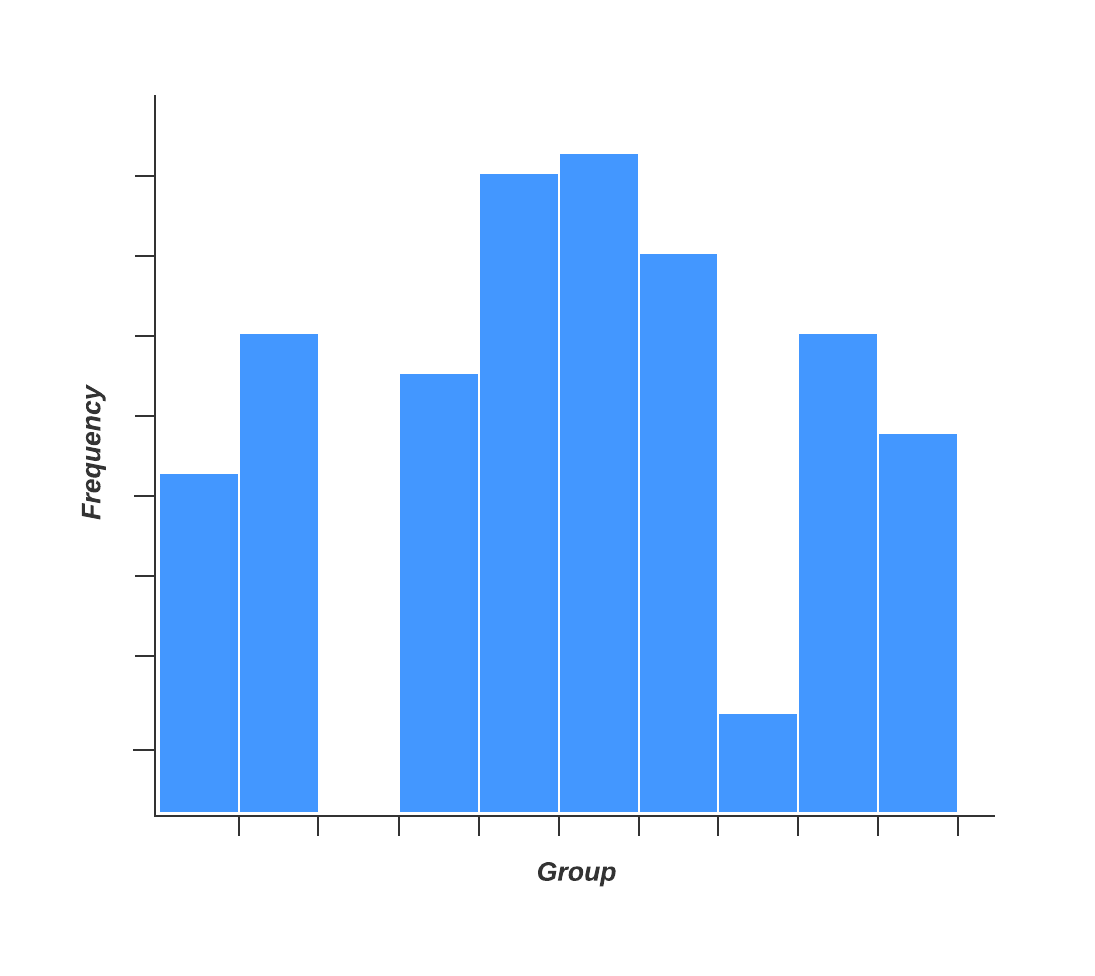
3. Check sheet (or tally sheet)
Check sheets can be used to collect quantitative or qualitative data. When used to collect quantitative data, they can be called a tally sheet. A check sheet collects data in the form of check or tally marks that indicate how many times a particular value has occurred, allowing you to quickly zero in on defects or errors within your process or product, defect patterns, and even causes of specific defects.
With its simple setup and easy-to-read graphics, check sheets make it easy to record preliminary frequency distribution data when measuring out processes. This particular graphic can be used as a preliminary data collection tool when creating histograms, bar graphs, and other quality tools.

4. Cause-and-effect diagram (also known as a fishbone or Ishikawa diagram)
Introduced by Kaoru Ishikawa, the fishbone diagram helps users identify the various factors (or causes) leading to an effect, usually depicted as a problem to be solved. Named for its resemblance to a fishbone, this quality management tool works by defining a quality-related problem on the right-hand side of the diagram, with individual root causes and sub-causes branching off to its left.
A fishbone diagram’s causes and subcauses are usually grouped into six main groups, including measurements, materials, personnel, environment, methods, and machines. These categories can help you identify the probable source of your problem while keeping your diagram structured and orderly.

5. Pareto chart (80-20 rule)
As a quality control tool, the Pareto chart operates according to the 80-20 rule. This rule assumes that in any process, 80% of a process’s or system’s problems are caused by 20% of major factors, often referred to as the “vital few.” The remaining 20% of problems are caused by 80% of minor factors.
A combination of a bar and line graph, the Pareto chart depicts individual values in descending order using bars, while the cumulative total is represented by the line.
The goal of the Pareto chart is to highlight the relative importance of a variety of parameters, allowing you to identify and focus your efforts on the factors with the biggest impact on a specific part of a process or system.
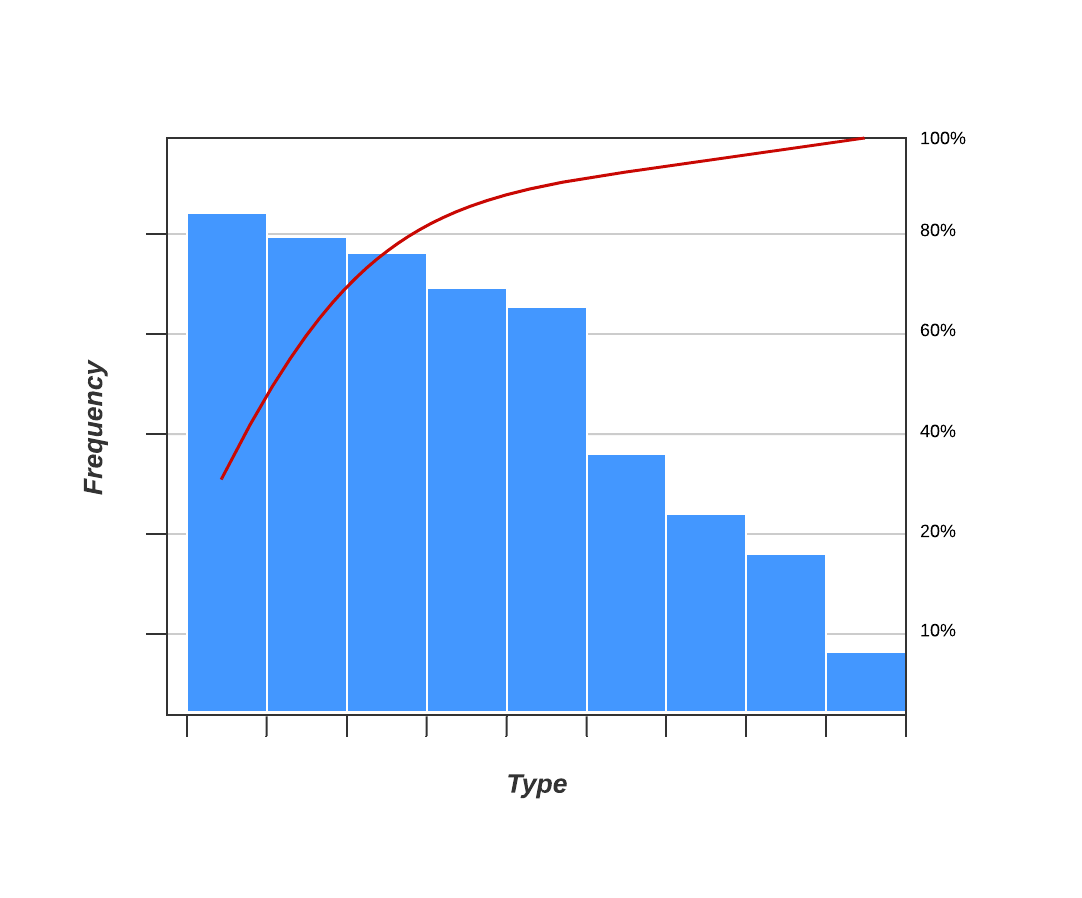
6. Scatter diagram
Out of the seven quality tools, the scatter diagram is most useful in depicting the relationship between two variables, which is ideal for quality assurance professionals trying to identify cause and effect relationships.
With dependent values on the diagram’s Y-axis and independent values on the X-axis, each dot represents a common intersection point. When joined, these dots can highlight the relationship between the two variables. The stronger the correlation in your diagram, the stronger the relationship between variables.
Scatter diagrams can prove useful as a quality control tool when used to define relationships between quality defects and possible causes such as environment, activity, personnel, and other variables. Once the relationship between a particular defect and its cause has been established, you can implement focused solutions with (hopefully) better outcomes.
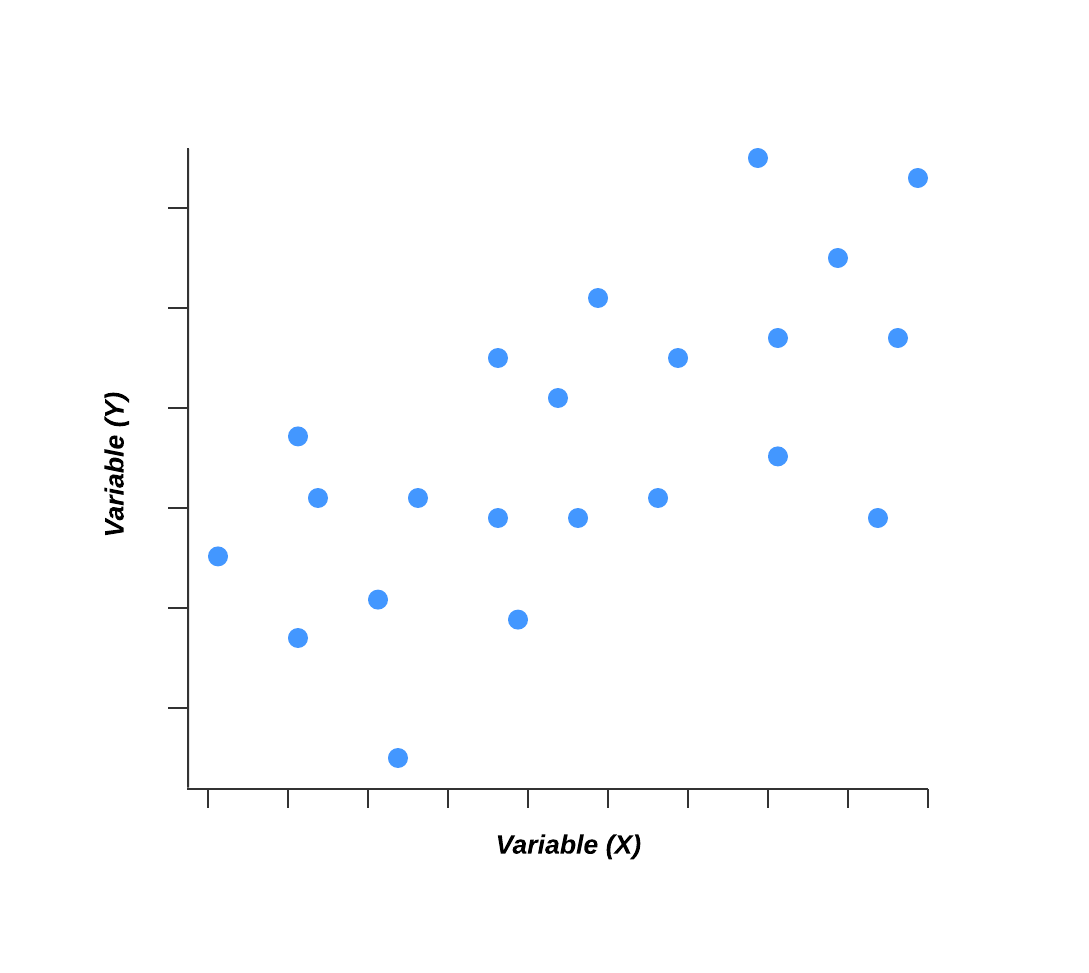
7. Control chart (also called a Shewhart chart)
Named after Walter A. Shewhart, this quality improvement tool can help quality assurance professionals determine whether or not a process is stable and predictable, making it easy for you to identify factors that might lead to variations or defects.
Control charts use a central line to depict an average or mean, as well as an upper and lower line to depict upper and lower control limits based on historical data. By comparing historical data to data collected from your current process, you can determine whether your current process is controlled or affected by specific variations.
Using a control chart can save your organization time and money by predicting process performance, particularly in terms of what your customer or organization expects in your final product.

Bonus: Flowcharts
Some sources will swap out stratification to instead include flowcharts as one of the seven basic QC tools. Flowcharts are most commonly used to document organizational structures and process flows, making them ideal for identifying bottlenecks and unnecessary steps within your process or system.
Mapping out your current process can help you to more effectively pinpoint which activities are completed when and by whom, how processes flow from one department or task to another, and which steps can be eliminated to streamline your process.

Learn how to create a process improvement plan in seven steps.
Lucidchart, a cloud-based intelligent diagramming application, is a core component of Lucid Software's Visual Collaboration Suite. This intuitive, cloud-based solution empowers teams to collaborate in real-time to build flowcharts, mockups, UML diagrams, customer journey maps, and more. Lucidchart propels teams forward to build the future faster. Lucid is proud to serve top businesses around the world, including customers such as Google, GE, and NBC Universal, and 99% of the Fortune 500. Lucid partners with industry leaders, including Google, Atlassian, and Microsoft. Since its founding, Lucid has received numerous awards for its products, business, and workplace culture. For more information, visit lucidchart.com.
Related articles

In this article we’ll talk about how to improve visualization, even if you are not a visual presentation expert.

Struggling to decide which process improvement methodology to use? Learn about the top approaches—Six Sigma, Lean, TQM, Just-in-time, and others—and the diagrams that can help you implement these techniques starting today.
Bring your bright ideas to life.
or continue with
Application of 7 quality control (7 QC) tools for quality management: A case study of a liquid chemical warehousing
Ieee account.
- Change Username/Password
- Update Address
Purchase Details
- Payment Options
- Order History
- View Purchased Documents
Profile Information
- Communications Preferences
- Profession and Education
- Technical Interests
- US & Canada: +1 800 678 4333
- Worldwide: +1 732 981 0060
- Contact & Support
- About IEEE Xplore
- Accessibility
- Terms of Use
- Nondiscrimination Policy
- Privacy & Opting Out of Cookies
A not-for-profit organization, IEEE is the world's largest technical professional organization dedicated to advancing technology for the benefit of humanity. © Copyright 2024 IEEE - All rights reserved. Use of this web site signifies your agreement to the terms and conditions.
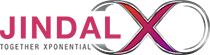
- Digital Customer Experience
- Business Process Outsourcing
- Revenue Management (CPG)
- Revenue Cycle Management (RCM)
- Digital Customer Acquisition & Retention
- Digital Transformation
- Artificial Intelligence (AI)
- Banking & Financial Services
- Manufacturing
- On-demand Travel
- Consumer Packaged Goods (CPG)
- About JindalX
- Brand Story
- Case Studies
- White Papers
- Business Process Outsourcing (BPO)
7 Quality Tools in BPO: Essentials for a Successful Business
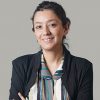
Business Process Outsourcing (BPO) has become an integral part of modern business operations. By delegating specific tasks or processes to third-party service providers, organizations can streamline their operations, reduce costs, and improve efficiency. However, to ensure the success of a BPO initiative, it is crucial to maintain and monitor the quality of outsourced processes. This is where the 7 Quality Tools in BPO come into play. In this comprehensive guide, we will explore what is BPO, its significance, and the 7 quality tools in BPO through a real-world case study and relevant statistics.
Understanding BPO
What is bpo.
Business Process Outsourcing, commonly known as BPO, refers to the practice of contracting specific business tasks or processes to external service providers. These processes can encompass a wide range of functions, including customer support services , finance and accounting, human resources, data entry, and more. BPO allows organizations to focus on their core competencies while benefiting from cost savings, operational efficiency, and access to specialized skills.
The Significance of BPO
As we have understood What is BPO? Let us now go through the significance of BPO in today’s business landscape as it cannot be overstated:
- Cost Efficiency : Outsourcing can significantly reduce labour and operational costs, making it an attractive option for businesses looking to maximize profitability.
- Global Talent Pool : BPO providers often operate in regions with a skilled workforce, providing access to specialized skills that may not be available in-house.
- Scalability : BPO services can be scaled up or down according to business needs, providing flexibility in resource allocation.
- Focus on Core Competencies : Outsourcing non-core functions allows organizations to concentrate on their primary objectives and strategic initiatives.
The 7 Quality Tools in BPO
To ensure the success of BPO partnerships and maintain high-quality standards, organizations should employ the following 7 quality tools in BPO:
1. Flowcharts and Process Maps
Flowcharts and process maps provide a visual representation of the workflow, making it easier to identify bottlenecks, redundancies, and inefficiencies in outsourced processes. They serve as a blueprint for process improvement.
2. Cause-and-Effect Diagrams (Fishbone Diagrams)
Fishbone diagrams help pinpoint the root causes of issues or defects in BPO processes. By identifying these causes, organizations can implement corrective actions and prevent future problems.
3. Pareto Charts
Pareto charts help prioritize issues or problem areas by showing which factors contribute the most to a particular problem. This tool assists in focusing resources on critical improvement areas.
4. Histograms
Histograms provide a graphical representation of data distribution, enabling organizations to understand the variability in their processes. This insight is crucial for maintaining consistency in BPO operations.
5. Control Charts
Control charts monitor process performance over time, helping organizations detect any deviations from established standards. This tool facilitates early intervention and ensures process stability.
6. Scatter Diagrams
Scatter diagrams help identify potential correlations or relationships between different variables. In a BPO context, this can be used to understand how changes in one aspect of the process may affect another.
7 Check Sheets
Check sheets are simple data collection tools that enable organizations to track and record specific data points. They are valuable for ongoing monitoring and data-driven decision-making in BPO operations.
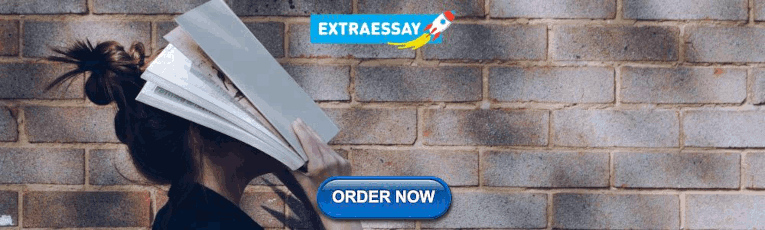
Case Study – Improving Customer Support in an E-commerce BPO
Let’s illustrate the importance of these 7 quality tools in BPO with a real-world case study:
An e-commerce company decided to outsource its customer support operations to a BPO provider to manage the increasing volume of customer inquiries. However, the company faced challenges related to customer satisfaction, response times, and issue resolution rates. Here 7 quality tools in BPO played a crucial role in overcoming the challenges.
Application of 7 Quality Tools in BPO
Flowcharts and process maps:.
Application : These visual representations provide a clear overview of the entire BPO process, including its various steps and decision points.
Use : BPO providers use flowcharts and process maps to identify bottlenecks, redundancies, and opportunities for process optimization. It helps everyone involved understand the workflow, making it easier to discuss and implement improvements.
Cause-and-Effect Diagrams (Fishbone Diagrams):
Application : Fishbone diagrams are used to analyse complex problems and identify their root causes.
Use : BPO teams employ these diagrams to dissect issues within a process, such as increased error rates or delays. By identifying underlying causes, they can develop strategies to address these issues and prevent their recurrence.
Pareto Charts:
Application : Pareto charts help prioritize problems or issues by showing which factors contribute the most to a particular problem.
Use : BPO managers use Pareto charts to focus their resources on the most critical issues affecting the quality of their services. This ensures that efforts are directed toward the areas with the greatest impact.
Histograms:
Application : Histograms visualize the distribution of data, providing insights into data variability.
Use : In BPO, histograms are used to understand how data is spread across a process, helping to identify variations or inconsistencies. This information is crucial for maintaining process consistency and quality.
Control Charts:
Application : Control charts monitor process performance over time by tracking key performance indicators (KPIs).
Use : BPO teams use control charts to ensure that their processes are stable and within acceptable limits. When a process exceeds these limits, it indicates a potential issue that requires investigation and corrective action.
Scatter Diagrams:
Application : Scatter diagrams help identify potential relationships or correlations between two variables.
Use : In BPO, scatter diagrams are used to explore how changes in one variable might affect another. For example, they can assess how changes in response times may impact customer satisfaction scores.
Check Sheets:
Application : Check sheets are simple data collection tools that enable systematic data recording.
Use : BPO providers use check sheets to gather data on specific aspects of their processes. This data is then analysed to make informed decisions, track progress, and identify trends or patterns.
- Improved Response Times : The BPO provider streamlined the response process, reducing average response times by 31%.
- Enhanced Customer Satisfaction : Customer satisfaction scores increased by 20% due to faster responses and accurate information.
- Reduced Complaints : Customer complaints related to response times and incorrect information decreased by 70%.
- Higher Efficiency and Productivity : By eliminating bottlenecks and inefficiencies identified through flowcharts and process maps, the BPO provider achieved higher efficiency levels. This, in turn, translated into increased productivity, allowing the team to handle more inquiries and tasks within the same timeframe.
- Cost Savings : While not directly mentioned in the case study, the improvements in efficiency, reduced complaints, and increased customer satisfaction can be associated with cost savings. A more efficient process requires fewer resources, and satisfied customers are less likely to churn or require costly escalations.
- Data-Driven Decision-Making : The implementation of check sheets and control charts enabled the BPO provider to collect and analyse data systematically. This data-driven approach to decision-making not only facilitated process improvements but also provided valuable insights for ongoing optimization.
- Employee Engagement : As process improvements and increased customer satisfaction became apparent, employee morale and engagement within the BPO team also improved. Employees took pride in their work and were motivated to maintain the higher service quality standards.
Statistics on BPO Quality Improvement
Here are some relevant statistics showcasing the impact of quality improvement efforts in BPO:
- According to a Deloitte survey, 59% of organizations outsource to reduce costs, while 57% do so to focus on their core business functions.
- The International Association of Outsourcing Professionals (IAOP) reports that 78% of organizations believe that outsourcing gives them a competitive advantage.
- A study by Accenture found that 86% of organizations experienced cost savings through outsourcing, with an average cost reduction of 15%.
- Quality improvement efforts in BPO can lead to significant gains. A case study by Six Sigma Daily reported a 28% increase in process efficiency and a 22% reduction in defects after implementing Six Sigma quality tools in a BPO operation.
Business Process Outsourcing offers numerous advantages, but its success relies heavily on maintaining high-quality standards. The 7 Quality Tools in BPO – flowcharts, cause-and-effect diagrams, Pareto charts, histograms, control charts, scatter diagrams, and check sheets – play a vital role in achieving and sustaining this quality. By applying these tools, organizations can optimize their BPO processes, reduce costs, enhance customer satisfaction, and gain a competitive edge in today’s global business landscape.
If you are ready to transform your business and do more with business process outsourcing model, then you must connect with JindalX . You will be able to learn more about BPO, it’s advantages, tools, benefits to your company, and even integrate with us. JindalX has been a leading BPO company for over 2 decades and has been able to keep their customer satisfaction game at top.
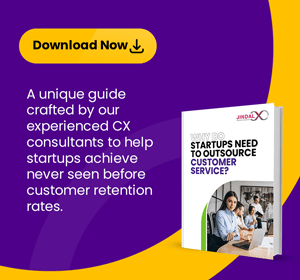
Customer Service Outsourcing Guide for Startups
Unlock Major Pitfalls to Avoid and a Solid Foolproof Personalized CX strategy
Latest Posts
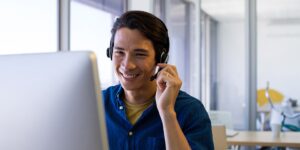
9 Customer-Focused Call Center KPIs You Need to Start Tracking Today
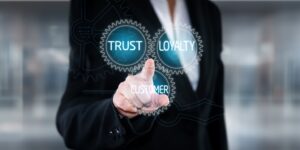
Building Consumer Trust Is the Long-Term Capital for The BFSI Sector
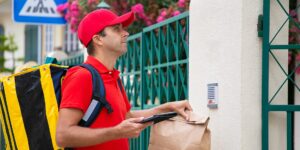
From Cold Food to Late Deliveries: Call Center Services Have Got Your Back
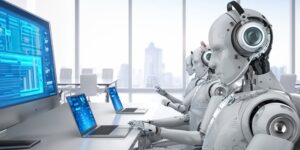
AI In Customer Service: Balancing Automation and Human Touch
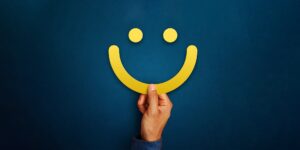
4 Customer Experience Game-Changers: Stay Ahead of the Curve
Leave a comment.
Your email address will not be published.
Save my name, email, and website in this browser for the next time I comment.
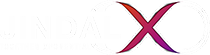
- Customer Experience
- Content Curation
- Revenue Cycle Management
- Banking & Financial Services
- Manufacturing & Consumer
- The JindalX Proposition
- Leadership Team
- Statutory Compliance
- Current Openings
- Optimization Partners
Contact Number

An official website of the United States government
Here’s how you know
Official websites use .gov A .gov website belongs to an official government organization in the United States.
Secure .gov websites use HTTPS A lock ( A locked padlock ) or https:// means you’ve safely connected to the .gov website. Share sensitive information only on official, secure websites.
- Heart-Healthy Living
- High Blood Pressure
- Sickle Cell Disease
- Sleep Apnea
- Information & Resources on COVID-19
- The Heart Truth®
- Learn More Breathe Better®
- Blood Diseases and Disorders Education Program
- Publications and Resources
- Blood Disorders and Blood Safety
- Sleep Science and Sleep Disorders
- Lung Diseases
- Health Disparities and Inequities
- Heart and Vascular Diseases
- Precision Medicine Activities
- Obesity, Nutrition, and Physical Activity
- Population and Epidemiology Studies
- Women’s Health
- Research Topics
- Clinical Trials
- All Science A-Z
- Grants and Training Home
- Policies and Guidelines
- Funding Opportunities and Contacts
- Training and Career Development
- Email Alerts
- NHLBI in the Press
- Research Features
- Past Events
- Upcoming Events
- Mission and Strategic Vision
- Divisions, Offices and Centers
- Advisory Committees
- Budget and Legislative Information
- Jobs and Working at the NHLBI
- Contact and FAQs
- NIH Sleep Research Plan
- < Back To Health Topics
Study Quality Assessment Tools
In 2013, NHLBI developed a set of tailored quality assessment tools to assist reviewers in focusing on concepts that are key to a study’s internal validity. The tools were specific to certain study designs and tested for potential flaws in study methods or implementation. Experts used the tools during the systematic evidence review process to update existing clinical guidelines, such as those on cholesterol, blood pressure, and obesity. Their findings are outlined in the following reports:
- Assessing Cardiovascular Risk: Systematic Evidence Review from the Risk Assessment Work Group
- Management of Blood Cholesterol in Adults: Systematic Evidence Review from the Cholesterol Expert Panel
- Management of Blood Pressure in Adults: Systematic Evidence Review from the Blood Pressure Expert Panel
- Managing Overweight and Obesity in Adults: Systematic Evidence Review from the Obesity Expert Panel
While these tools have not been independently published and would not be considered standardized, they may be useful to the research community. These reports describe how experts used the tools for the project. Researchers may want to use the tools for their own projects; however, they would need to determine their own parameters for making judgements. Details about the design and application of the tools are included in Appendix A of the reports.
Quality Assessment of Controlled Intervention Studies - Study Quality Assessment Tools
*CD, cannot determine; NA, not applicable; NR, not reported
Guidance for Assessing the Quality of Controlled Intervention Studies
The guidance document below is organized by question number from the tool for quality assessment of controlled intervention studies.
Question 1. Described as randomized
Was the study described as randomized? A study does not satisfy quality criteria as randomized simply because the authors call it randomized; however, it is a first step in determining if a study is randomized
Questions 2 and 3. Treatment allocation–two interrelated pieces
Adequate randomization: Randomization is adequate if it occurred according to the play of chance (e.g., computer generated sequence in more recent studies, or random number table in older studies). Inadequate randomization: Randomization is inadequate if there is a preset plan (e.g., alternation where every other subject is assigned to treatment arm or another method of allocation is used, such as time or day of hospital admission or clinic visit, ZIP Code, phone number, etc.). In fact, this is not randomization at all–it is another method of assignment to groups. If assignment is not by the play of chance, then the answer to this question is no. There may be some tricky scenarios that will need to be read carefully and considered for the role of chance in assignment. For example, randomization may occur at the site level, where all individuals at a particular site are assigned to receive treatment or no treatment. This scenario is used for group-randomized trials, which can be truly randomized, but often are "quasi-experimental" studies with comparison groups rather than true control groups. (Few, if any, group-randomized trials are anticipated for this evidence review.)
Allocation concealment: This means that one does not know in advance, or cannot guess accurately, to what group the next person eligible for randomization will be assigned. Methods include sequentially numbered opaque sealed envelopes, numbered or coded containers, central randomization by a coordinating center, computer-generated randomization that is not revealed ahead of time, etc. Questions 4 and 5. Blinding
Blinding means that one does not know to which group–intervention or control–the participant is assigned. It is also sometimes called "masking." The reviewer assessed whether each of the following was blinded to knowledge of treatment assignment: (1) the person assessing the primary outcome(s) for the study (e.g., taking the measurements such as blood pressure, examining health records for events such as myocardial infarction, reviewing and interpreting test results such as x ray or cardiac catheterization findings); (2) the person receiving the intervention (e.g., the patient or other study participant); and (3) the person providing the intervention (e.g., the physician, nurse, pharmacist, dietitian, or behavioral interventionist).
Generally placebo-controlled medication studies are blinded to patient, provider, and outcome assessors; behavioral, lifestyle, and surgical studies are examples of studies that are frequently blinded only to the outcome assessors because blinding of the persons providing and receiving the interventions is difficult in these situations. Sometimes the individual providing the intervention is the same person performing the outcome assessment. This was noted when it occurred.
Question 6. Similarity of groups at baseline
This question relates to whether the intervention and control groups have similar baseline characteristics on average especially those characteristics that may affect the intervention or outcomes. The point of randomized trials is to create groups that are as similar as possible except for the intervention(s) being studied in order to compare the effects of the interventions between groups. When reviewers abstracted baseline characteristics, they noted when there was a significant difference between groups. Baseline characteristics for intervention groups are usually presented in a table in the article (often Table 1).
Groups can differ at baseline without raising red flags if: (1) the differences would not be expected to have any bearing on the interventions and outcomes; or (2) the differences are not statistically significant. When concerned about baseline difference in groups, reviewers recorded them in the comments section and considered them in their overall determination of the study quality.
Questions 7 and 8. Dropout
"Dropouts" in a clinical trial are individuals for whom there are no end point measurements, often because they dropped out of the study and were lost to followup.
Generally, an acceptable overall dropout rate is considered 20 percent or less of participants who were randomized or allocated into each group. An acceptable differential dropout rate is an absolute difference between groups of 15 percentage points at most (calculated by subtracting the dropout rate of one group minus the dropout rate of the other group). However, these are general rates. Lower overall dropout rates are expected in shorter studies, whereas higher overall dropout rates may be acceptable for studies of longer duration. For example, a 6-month study of weight loss interventions should be expected to have nearly 100 percent followup (almost no dropouts–nearly everybody gets their weight measured regardless of whether or not they actually received the intervention), whereas a 10-year study testing the effects of intensive blood pressure lowering on heart attacks may be acceptable if there is a 20-25 percent dropout rate, especially if the dropout rate between groups was similar. The panels for the NHLBI systematic reviews may set different levels of dropout caps.
Conversely, differential dropout rates are not flexible; there should be a 15 percent cap. If there is a differential dropout rate of 15 percent or higher between arms, then there is a serious potential for bias. This constitutes a fatal flaw, resulting in a poor quality rating for the study.
Question 9. Adherence
Did participants in each treatment group adhere to the protocols for assigned interventions? For example, if Group 1 was assigned to 10 mg/day of Drug A, did most of them take 10 mg/day of Drug A? Another example is a study evaluating the difference between a 30-pound weight loss and a 10-pound weight loss on specific clinical outcomes (e.g., heart attacks), but the 30-pound weight loss group did not achieve its intended weight loss target (e.g., the group only lost 14 pounds on average). A third example is whether a large percentage of participants assigned to one group "crossed over" and got the intervention provided to the other group. A final example is when one group that was assigned to receive a particular drug at a particular dose had a large percentage of participants who did not end up taking the drug or the dose as designed in the protocol.
Question 10. Avoid other interventions
Changes that occur in the study outcomes being assessed should be attributable to the interventions being compared in the study. If study participants receive interventions that are not part of the study protocol and could affect the outcomes being assessed, and they receive these interventions differentially, then there is cause for concern because these interventions could bias results. The following scenario is another example of how bias can occur. In a study comparing two different dietary interventions on serum cholesterol, one group had a significantly higher percentage of participants taking statin drugs than the other group. In this situation, it would be impossible to know if a difference in outcome was due to the dietary intervention or the drugs.
Question 11. Outcome measures assessment
What tools or methods were used to measure the outcomes in the study? Were the tools and methods accurate and reliable–for example, have they been validated, or are they objective? This is important as it indicates the confidence you can have in the reported outcomes. Perhaps even more important is ascertaining that outcomes were assessed in the same manner within and between groups. One example of differing methods is self-report of dietary salt intake versus urine testing for sodium content (a more reliable and valid assessment method). Another example is using BP measurements taken by practitioners who use their usual methods versus using BP measurements done by individuals trained in a standard approach. Such an approach may include using the same instrument each time and taking an individual's BP multiple times. In each of these cases, the answer to this assessment question would be "no" for the former scenario and "yes" for the latter. In addition, a study in which an intervention group was seen more frequently than the control group, enabling more opportunities to report clinical events, would not be considered reliable and valid.
Question 12. Power calculation
Generally, a study's methods section will address the sample size needed to detect differences in primary outcomes. The current standard is at least 80 percent power to detect a clinically relevant difference in an outcome using a two-sided alpha of 0.05. Often, however, older studies will not report on power.
Question 13. Prespecified outcomes
Investigators should prespecify outcomes reported in a study for hypothesis testing–which is the reason for conducting an RCT. Without prespecified outcomes, the study may be reporting ad hoc analyses, simply looking for differences supporting desired findings. Investigators also should prespecify subgroups being examined. Most RCTs conduct numerous post hoc analyses as a way of exploring findings and generating additional hypotheses. The intent of this question is to give more weight to reports that are not simply exploratory in nature.
Question 14. Intention-to-treat analysis
Intention-to-treat (ITT) means everybody who was randomized is analyzed according to the original group to which they are assigned. This is an extremely important concept because conducting an ITT analysis preserves the whole reason for doing a randomized trial; that is, to compare groups that differ only in the intervention being tested. When the ITT philosophy is not followed, groups being compared may no longer be the same. In this situation, the study would likely be rated poor. However, if an investigator used another type of analysis that could be viewed as valid, this would be explained in the "other" box on the quality assessment form. Some researchers use a completers analysis (an analysis of only the participants who completed the intervention and the study), which introduces significant potential for bias. Characteristics of participants who do not complete the study are unlikely to be the same as those who do. The likely impact of participants withdrawing from a study treatment must be considered carefully. ITT analysis provides a more conservative (potentially less biased) estimate of effectiveness.
General Guidance for Determining the Overall Quality Rating of Controlled Intervention Studies
The questions on the assessment tool were designed to help reviewers focus on the key concepts for evaluating a study's internal validity. They are not intended to create a list that is simply tallied up to arrive at a summary judgment of quality.
Internal validity is the extent to which the results (effects) reported in a study can truly be attributed to the intervention being evaluated and not to flaws in the design or conduct of the study–in other words, the ability for the study to make causal conclusions about the effects of the intervention being tested. Such flaws can increase the risk of bias. Critical appraisal involves considering the risk of potential for allocation bias, measurement bias, or confounding (the mixture of exposures that one cannot tease out from each other). Examples of confounding include co-interventions, differences at baseline in patient characteristics, and other issues addressed in the questions above. High risk of bias translates to a rating of poor quality. Low risk of bias translates to a rating of good quality.
Fatal flaws: If a study has a "fatal flaw," then risk of bias is significant, and the study is of poor quality. Examples of fatal flaws in RCTs include high dropout rates, high differential dropout rates, no ITT analysis or other unsuitable statistical analysis (e.g., completers-only analysis).
Generally, when evaluating a study, one will not see a "fatal flaw;" however, one will find some risk of bias. During training, reviewers were instructed to look for the potential for bias in studies by focusing on the concepts underlying the questions in the tool. For any box checked "no," reviewers were told to ask: "What is the potential risk of bias that may be introduced by this flaw?" That is, does this factor cause one to doubt the results that were reported in the study?
NHLBI staff provided reviewers with background reading on critical appraisal, while emphasizing that the best approach to use is to think about the questions in the tool in determining the potential for bias in a study. The staff also emphasized that each study has specific nuances; therefore, reviewers should familiarize themselves with the key concepts.
Quality Assessment of Systematic Reviews and Meta-Analyses - Study Quality Assessment Tools
Guidance for Quality Assessment Tool for Systematic Reviews and Meta-Analyses
A systematic review is a study that attempts to answer a question by synthesizing the results of primary studies while using strategies to limit bias and random error.424 These strategies include a comprehensive search of all potentially relevant articles and the use of explicit, reproducible criteria in the selection of articles included in the review. Research designs and study characteristics are appraised, data are synthesized, and results are interpreted using a predefined systematic approach that adheres to evidence-based methodological principles.
Systematic reviews can be qualitative or quantitative. A qualitative systematic review summarizes the results of the primary studies but does not combine the results statistically. A quantitative systematic review, or meta-analysis, is a type of systematic review that employs statistical techniques to combine the results of the different studies into a single pooled estimate of effect, often given as an odds ratio. The guidance document below is organized by question number from the tool for quality assessment of systematic reviews and meta-analyses.
Question 1. Focused question
The review should be based on a question that is clearly stated and well-formulated. An example would be a question that uses the PICO (population, intervention, comparator, outcome) format, with all components clearly described.
Question 2. Eligibility criteria
The eligibility criteria used to determine whether studies were included or excluded should be clearly specified and predefined. It should be clear to the reader why studies were included or excluded.
Question 3. Literature search
The search strategy should employ a comprehensive, systematic approach in order to capture all of the evidence possible that pertains to the question of interest. At a minimum, a comprehensive review has the following attributes:
- Electronic searches were conducted using multiple scientific literature databases, such as MEDLINE, EMBASE, Cochrane Central Register of Controlled Trials, PsychLit, and others as appropriate for the subject matter.
- Manual searches of references found in articles and textbooks should supplement the electronic searches.
Additional search strategies that may be used to improve the yield include the following:
- Studies published in other countries
- Studies published in languages other than English
- Identification by experts in the field of studies and articles that may have been missed
- Search of grey literature, including technical reports and other papers from government agencies or scientific groups or committees; presentations and posters from scientific meetings, conference proceedings, unpublished manuscripts; and others. Searching the grey literature is important (whenever feasible) because sometimes only positive studies with significant findings are published in the peer-reviewed literature, which can bias the results of a review.
In their reviews, researchers described the literature search strategy clearly, and ascertained it could be reproducible by others with similar results.
Question 4. Dual review for determining which studies to include and exclude
Titles, abstracts, and full-text articles (when indicated) should be reviewed by two independent reviewers to determine which studies to include and exclude in the review. Reviewers resolved disagreements through discussion and consensus or with third parties. They clearly stated the review process, including methods for settling disagreements.
Question 5. Quality appraisal for internal validity
Each included study should be appraised for internal validity (study quality assessment) using a standardized approach for rating the quality of the individual studies. Ideally, this should be done by at least two independent reviewers appraised each study for internal validity. However, there is not one commonly accepted, standardized tool for rating the quality of studies. So, in the research papers, reviewers looked for an assessment of the quality of each study and a clear description of the process used.
Question 6. List and describe included studies
All included studies were listed in the review, along with descriptions of their key characteristics. This was presented either in narrative or table format.
Question 7. Publication bias
Publication bias is a term used when studies with positive results have a higher likelihood of being published, being published rapidly, being published in higher impact journals, being published in English, being published more than once, or being cited by others.425,426 Publication bias can be linked to favorable or unfavorable treatment of research findings due to investigators, editors, industry, commercial interests, or peer reviewers. To minimize the potential for publication bias, researchers can conduct a comprehensive literature search that includes the strategies discussed in Question 3.
A funnel plot–a scatter plot of component studies in a meta-analysis–is a commonly used graphical method for detecting publication bias. If there is no significant publication bias, the graph looks like a symmetrical inverted funnel.
Reviewers assessed and clearly described the likelihood of publication bias.
Question 8. Heterogeneity
Heterogeneity is used to describe important differences in studies included in a meta-analysis that may make it inappropriate to combine the studies.427 Heterogeneity can be clinical (e.g., important differences between study participants, baseline disease severity, and interventions); methodological (e.g., important differences in the design and conduct of the study); or statistical (e.g., important differences in the quantitative results or reported effects).
Researchers usually assess clinical or methodological heterogeneity qualitatively by determining whether it makes sense to combine studies. For example:
- Should a study evaluating the effects of an intervention on CVD risk that involves elderly male smokers with hypertension be combined with a study that involves healthy adults ages 18 to 40? (Clinical Heterogeneity)
- Should a study that uses a randomized controlled trial (RCT) design be combined with a study that uses a case-control study design? (Methodological Heterogeneity)
Statistical heterogeneity describes the degree of variation in the effect estimates from a set of studies; it is assessed quantitatively. The two most common methods used to assess statistical heterogeneity are the Q test (also known as the X2 or chi-square test) or I2 test.
Reviewers examined studies to determine if an assessment for heterogeneity was conducted and clearly described. If the studies are found to be heterogeneous, the investigators should explore and explain the causes of the heterogeneity, and determine what influence, if any, the study differences had on overall study results.
Quality Assessment Tool for Observational Cohort and Cross-Sectional Studies - Study Quality Assessment Tools
Guidance for Assessing the Quality of Observational Cohort and Cross-Sectional Studies
The guidance document below is organized by question number from the tool for quality assessment of observational cohort and cross-sectional studies.
Question 1. Research question
Did the authors describe their goal in conducting this research? Is it easy to understand what they were looking to find? This issue is important for any scientific paper of any type. Higher quality scientific research explicitly defines a research question.
Questions 2 and 3. Study population
Did the authors describe the group of people from which the study participants were selected or recruited, using demographics, location, and time period? If you were to conduct this study again, would you know who to recruit, from where, and from what time period? Is the cohort population free of the outcomes of interest at the time they were recruited?
An example would be men over 40 years old with type 2 diabetes who began seeking medical care at Phoenix Good Samaritan Hospital between January 1, 1990 and December 31, 1994. In this example, the population is clearly described as: (1) who (men over 40 years old with type 2 diabetes); (2) where (Phoenix Good Samaritan Hospital); and (3) when (between January 1, 1990 and December 31, 1994). Another example is women ages 34 to 59 years of age in 1980 who were in the nursing profession and had no known coronary disease, stroke, cancer, hypercholesterolemia, or diabetes, and were recruited from the 11 most populous States, with contact information obtained from State nursing boards.
In cohort studies, it is crucial that the population at baseline is free of the outcome of interest. For example, the nurses' population above would be an appropriate group in which to study incident coronary disease. This information is usually found either in descriptions of population recruitment, definitions of variables, or inclusion/exclusion criteria.
You may need to look at prior papers on methods in order to make the assessment for this question. Those papers are usually in the reference list.
If fewer than 50% of eligible persons participated in the study, then there is concern that the study population does not adequately represent the target population. This increases the risk of bias.
Question 4. Groups recruited from the same population and uniform eligibility criteria
Were the inclusion and exclusion criteria developed prior to recruitment or selection of the study population? Were the same underlying criteria used for all of the subjects involved? This issue is related to the description of the study population, above, and you may find the information for both of these questions in the same section of the paper.
Most cohort studies begin with the selection of the cohort; participants in this cohort are then measured or evaluated to determine their exposure status. However, some cohort studies may recruit or select exposed participants in a different time or place than unexposed participants, especially retrospective cohort studies–which is when data are obtained from the past (retrospectively), but the analysis examines exposures prior to outcomes. For example, one research question could be whether diabetic men with clinical depression are at higher risk for cardiovascular disease than those without clinical depression. So, diabetic men with depression might be selected from a mental health clinic, while diabetic men without depression might be selected from an internal medicine or endocrinology clinic. This study recruits groups from different clinic populations, so this example would get a "no."
However, the women nurses described in the question above were selected based on the same inclusion/exclusion criteria, so that example would get a "yes."
Question 5. Sample size justification
Did the authors present their reasons for selecting or recruiting the number of people included or analyzed? Do they note or discuss the statistical power of the study? This question is about whether or not the study had enough participants to detect an association if one truly existed.
A paragraph in the methods section of the article may explain the sample size needed to detect a hypothesized difference in outcomes. You may also find a discussion of power in the discussion section (such as the study had 85 percent power to detect a 20 percent increase in the rate of an outcome of interest, with a 2-sided alpha of 0.05). Sometimes estimates of variance and/or estimates of effect size are given, instead of sample size calculations. In any of these cases, the answer would be "yes."
However, observational cohort studies often do not report anything about power or sample sizes because the analyses are exploratory in nature. In this case, the answer would be "no." This is not a "fatal flaw." It just may indicate that attention was not paid to whether the study was sufficiently sized to answer a prespecified question–i.e., it may have been an exploratory, hypothesis-generating study.
Question 6. Exposure assessed prior to outcome measurement
This question is important because, in order to determine whether an exposure causes an outcome, the exposure must come before the outcome.
For some prospective cohort studies, the investigator enrolls the cohort and then determines the exposure status of various members of the cohort (large epidemiological studies like Framingham used this approach). However, for other cohort studies, the cohort is selected based on its exposure status, as in the example above of depressed diabetic men (the exposure being depression). Other examples include a cohort identified by its exposure to fluoridated drinking water and then compared to a cohort living in an area without fluoridated water, or a cohort of military personnel exposed to combat in the Gulf War compared to a cohort of military personnel not deployed in a combat zone.
With either of these types of cohort studies, the cohort is followed forward in time (i.e., prospectively) to assess the outcomes that occurred in the exposed members compared to nonexposed members of the cohort. Therefore, you begin the study in the present by looking at groups that were exposed (or not) to some biological or behavioral factor, intervention, etc., and then you follow them forward in time to examine outcomes. If a cohort study is conducted properly, the answer to this question should be "yes," since the exposure status of members of the cohort was determined at the beginning of the study before the outcomes occurred.
For retrospective cohort studies, the same principal applies. The difference is that, rather than identifying a cohort in the present and following them forward in time, the investigators go back in time (i.e., retrospectively) and select a cohort based on their exposure status in the past and then follow them forward to assess the outcomes that occurred in the exposed and nonexposed cohort members. Because in retrospective cohort studies the exposure and outcomes may have already occurred (it depends on how long they follow the cohort), it is important to make sure that the exposure preceded the outcome.
Sometimes cross-sectional studies are conducted (or cross-sectional analyses of cohort-study data), where the exposures and outcomes are measured during the same timeframe. As a result, cross-sectional analyses provide weaker evidence than regular cohort studies regarding a potential causal relationship between exposures and outcomes. For cross-sectional analyses, the answer to Question 6 should be "no."
Question 7. Sufficient timeframe to see an effect
Did the study allow enough time for a sufficient number of outcomes to occur or be observed, or enough time for an exposure to have a biological effect on an outcome? In the examples given above, if clinical depression has a biological effect on increasing risk for CVD, such an effect may take years. In the other example, if higher dietary sodium increases BP, a short timeframe may be sufficient to assess its association with BP, but a longer timeframe would be needed to examine its association with heart attacks.
The issue of timeframe is important to enable meaningful analysis of the relationships between exposures and outcomes to be conducted. This often requires at least several years, especially when looking at health outcomes, but it depends on the research question and outcomes being examined.
Cross-sectional analyses allow no time to see an effect, since the exposures and outcomes are assessed at the same time, so those would get a "no" response.
Question 8. Different levels of the exposure of interest
If the exposure can be defined as a range (examples: drug dosage, amount of physical activity, amount of sodium consumed), were multiple categories of that exposure assessed? (for example, for drugs: not on the medication, on a low dose, medium dose, high dose; for dietary sodium, higher than average U.S. consumption, lower than recommended consumption, between the two). Sometimes discrete categories of exposure are not used, but instead exposures are measured as continuous variables (for example, mg/day of dietary sodium or BP values).
In any case, studying different levels of exposure (where possible) enables investigators to assess trends or dose-response relationships between exposures and outcomes–e.g., the higher the exposure, the greater the rate of the health outcome. The presence of trends or dose-response relationships lends credibility to the hypothesis of causality between exposure and outcome.
For some exposures, however, this question may not be applicable (e.g., the exposure may be a dichotomous variable like living in a rural setting versus an urban setting, or vaccinated/not vaccinated with a one-time vaccine). If there are only two possible exposures (yes/no), then this question should be given an "NA," and it should not count negatively towards the quality rating.
Question 9. Exposure measures and assessment
Were the exposure measures defined in detail? Were the tools or methods used to measure exposure accurate and reliable–for example, have they been validated or are they objective? This issue is important as it influences confidence in the reported exposures. When exposures are measured with less accuracy or validity, it is harder to see an association between exposure and outcome even if one exists. Also as important is whether the exposures were assessed in the same manner within groups and between groups; if not, bias may result.
For example, retrospective self-report of dietary salt intake is not as valid and reliable as prospectively using a standardized dietary log plus testing participants' urine for sodium content. Another example is measurement of BP, where there may be quite a difference between usual care, where clinicians measure BP however it is done in their practice setting (which can vary considerably), and use of trained BP assessors using standardized equipment (e.g., the same BP device which has been tested and calibrated) and a standardized protocol (e.g., patient is seated for 5 minutes with feet flat on the floor, BP is taken twice in each arm, and all four measurements are averaged). In each of these cases, the former would get a "no" and the latter a "yes."
Here is a final example that illustrates the point about why it is important to assess exposures consistently across all groups: If people with higher BP (exposed cohort) are seen by their providers more frequently than those without elevated BP (nonexposed group), it also increases the chances of detecting and documenting changes in health outcomes, including CVD-related events. Therefore, it may lead to the conclusion that higher BP leads to more CVD events. This may be true, but it could also be due to the fact that the subjects with higher BP were seen more often; thus, more CVD-related events were detected and documented simply because they had more encounters with the health care system. Thus, it could bias the results and lead to an erroneous conclusion.
Question 10. Repeated exposure assessment
Was the exposure for each person measured more than once during the course of the study period? Multiple measurements with the same result increase our confidence that the exposure status was correctly classified. Also, multiple measurements enable investigators to look at changes in exposure over time, for example, people who ate high dietary sodium throughout the followup period, compared to those who started out high then reduced their intake, compared to those who ate low sodium throughout. Once again, this may not be applicable in all cases. In many older studies, exposure was measured only at baseline. However, multiple exposure measurements do result in a stronger study design.
Question 11. Outcome measures
Were the outcomes defined in detail? Were the tools or methods for measuring outcomes accurate and reliable–for example, have they been validated or are they objective? This issue is important because it influences confidence in the validity of study results. Also important is whether the outcomes were assessed in the same manner within groups and between groups.
An example of an outcome measure that is objective, accurate, and reliable is death–the outcome measured with more accuracy than any other. But even with a measure as objective as death, there can be differences in the accuracy and reliability of how death was assessed by the investigators. Did they base it on an autopsy report, death certificate, death registry, or report from a family member? Another example is a study of whether dietary fat intake is related to blood cholesterol level (cholesterol level being the outcome), and the cholesterol level is measured from fasting blood samples that are all sent to the same laboratory. These examples would get a "yes." An example of a "no" would be self-report by subjects that they had a heart attack, or self-report of how much they weigh (if body weight is the outcome of interest).
Similar to the example in Question 9, results may be biased if one group (e.g., people with high BP) is seen more frequently than another group (people with normal BP) because more frequent encounters with the health care system increases the chances of outcomes being detected and documented.
Question 12. Blinding of outcome assessors
Blinding means that outcome assessors did not know whether the participant was exposed or unexposed. It is also sometimes called "masking." The objective is to look for evidence in the article that the person(s) assessing the outcome(s) for the study (for example, examining medical records to determine the outcomes that occurred in the exposed and comparison groups) is masked to the exposure status of the participant. Sometimes the person measuring the exposure is the same person conducting the outcome assessment. In this case, the outcome assessor would most likely not be blinded to exposure status because they also took measurements of exposures. If so, make a note of that in the comments section.
As you assess this criterion, think about whether it is likely that the person(s) doing the outcome assessment would know (or be able to figure out) the exposure status of the study participants. If the answer is no, then blinding is adequate. An example of adequate blinding of the outcome assessors is to create a separate committee, whose members were not involved in the care of the patient and had no information about the study participants' exposure status. The committee would then be provided with copies of participants' medical records, which had been stripped of any potential exposure information or personally identifiable information. The committee would then review the records for prespecified outcomes according to the study protocol. If blinding was not possible, which is sometimes the case, mark "NA" and explain the potential for bias.
Question 13. Followup rate
Higher overall followup rates are always better than lower followup rates, even though higher rates are expected in shorter studies, whereas lower overall followup rates are often seen in studies of longer duration. Usually, an acceptable overall followup rate is considered 80 percent or more of participants whose exposures were measured at baseline. However, this is just a general guideline. For example, a 6-month cohort study examining the relationship between dietary sodium intake and BP level may have over 90 percent followup, but a 20-year cohort study examining effects of sodium intake on stroke may have only a 65 percent followup rate.
Question 14. Statistical analyses
Were key potential confounding variables measured and adjusted for, such as by statistical adjustment for baseline differences? Logistic regression or other regression methods are often used to account for the influence of variables not of interest.
This is a key issue in cohort studies, because statistical analyses need to control for potential confounders, in contrast to an RCT, where the randomization process controls for potential confounders. All key factors that may be associated both with the exposure of interest and the outcome–that are not of interest to the research question–should be controlled for in the analyses.
For example, in a study of the relationship between cardiorespiratory fitness and CVD events (heart attacks and strokes), the study should control for age, BP, blood cholesterol, and body weight, because all of these factors are associated both with low fitness and with CVD events. Well-done cohort studies control for multiple potential confounders.
Some general guidance for determining the overall quality rating of observational cohort and cross-sectional studies
The questions on the form are designed to help you focus on the key concepts for evaluating the internal validity of a study. They are not intended to create a list that you simply tally up to arrive at a summary judgment of quality.
Internal validity for cohort studies is the extent to which the results reported in the study can truly be attributed to the exposure being evaluated and not to flaws in the design or conduct of the study–in other words, the ability of the study to draw associative conclusions about the effects of the exposures being studied on outcomes. Any such flaws can increase the risk of bias.
Critical appraisal involves considering the risk of potential for selection bias, information bias, measurement bias, or confounding (the mixture of exposures that one cannot tease out from each other). Examples of confounding include co-interventions, differences at baseline in patient characteristics, and other issues throughout the questions above. High risk of bias translates to a rating of poor quality. Low risk of bias translates to a rating of good quality. (Thus, the greater the risk of bias, the lower the quality rating of the study.)
In addition, the more attention in the study design to issues that can help determine whether there is a causal relationship between the exposure and outcome, the higher quality the study. These include exposures occurring prior to outcomes, evaluation of a dose-response gradient, accuracy of measurement of both exposure and outcome, sufficient timeframe to see an effect, and appropriate control for confounding–all concepts reflected in the tool.
Generally, when you evaluate a study, you will not see a "fatal flaw," but you will find some risk of bias. By focusing on the concepts underlying the questions in the quality assessment tool, you should ask yourself about the potential for bias in the study you are critically appraising. For any box where you check "no" you should ask, "What is the potential risk of bias resulting from this flaw in study design or execution?" That is, does this factor cause you to doubt the results that are reported in the study or doubt the ability of the study to accurately assess an association between exposure and outcome?
The best approach is to think about the questions in the tool and how each one tells you something about the potential for bias in a study. The more you familiarize yourself with the key concepts, the more comfortable you will be with critical appraisal. Examples of studies rated good, fair, and poor are useful, but each study must be assessed on its own based on the details that are reported and consideration of the concepts for minimizing bias.
Quality Assessment of Case-Control Studies - Study Quality Assessment Tools
Guidance for Assessing the Quality of Case-Control Studies
The guidance document below is organized by question number from the tool for quality assessment of case-control studies.
Did the authors describe their goal in conducting this research? Is it easy to understand what they were looking to find? This issue is important for any scientific paper of any type. High quality scientific research explicitly defines a research question.
Question 2. Study population
Did the authors describe the group of individuals from which the cases and controls were selected or recruited, while using demographics, location, and time period? If the investigators conducted this study again, would they know exactly who to recruit, from where, and from what time period?
Investigators identify case-control study populations by location, time period, and inclusion criteria for cases (individuals with the disease, condition, or problem) and controls (individuals without the disease, condition, or problem). For example, the population for a study of lung cancer and chemical exposure would be all incident cases of lung cancer diagnosed in patients ages 35 to 79, from January 1, 2003 to December 31, 2008, living in Texas during that entire time period, as well as controls without lung cancer recruited from the same population during the same time period. The population is clearly described as: (1) who (men and women ages 35 to 79 with (cases) and without (controls) incident lung cancer); (2) where (living in Texas); and (3) when (between January 1, 2003 and December 31, 2008).
Other studies may use disease registries or data from cohort studies to identify cases. In these cases, the populations are individuals who live in the area covered by the disease registry or included in a cohort study (i.e., nested case-control or case-cohort). For example, a study of the relationship between vitamin D intake and myocardial infarction might use patients identified via the GRACE registry, a database of heart attack patients.
NHLBI staff encouraged reviewers to examine prior papers on methods (listed in the reference list) to make this assessment, if necessary.
Question 3. Target population and case representation
In order for a study to truly address the research question, the target population–the population from which the study population is drawn and to which study results are believed to apply–should be carefully defined. Some authors may compare characteristics of the study cases to characteristics of cases in the target population, either in text or in a table. When study cases are shown to be representative of cases in the appropriate target population, it increases the likelihood that the study was well-designed per the research question.
However, because these statistics are frequently difficult or impossible to measure, publications should not be penalized if case representation is not shown. For most papers, the response to question 3 will be "NR." Those subquestions are combined because the answer to the second subquestion–case representation–determines the response to this item. However, it cannot be determined without considering the response to the first subquestion. For example, if the answer to the first subquestion is "yes," and the second, "CD," then the response for item 3 is "CD."
Question 4. Sample size justification
Did the authors discuss their reasons for selecting or recruiting the number of individuals included? Did they discuss the statistical power of the study and provide a sample size calculation to ensure that the study is adequately powered to detect an association (if one exists)? This question does not refer to a description of the manner in which different groups were included or excluded using the inclusion/exclusion criteria (e.g., "Final study size was 1,378 participants after exclusion of 461 patients with missing data" is not considered a sample size justification for the purposes of this question).
An article's methods section usually contains information on sample size and the size needed to detect differences in exposures and on statistical power.
Question 5. Groups recruited from the same population
To determine whether cases and controls were recruited from the same population, one can ask hypothetically, "If a control was to develop the outcome of interest (the condition that was used to select cases), would that person have been eligible to become a case?" Case-control studies begin with the selection of the cases (those with the outcome of interest, e.g., lung cancer) and controls (those in whom the outcome is absent). Cases and controls are then evaluated and categorized by their exposure status. For the lung cancer example, cases and controls were recruited from hospitals in a given region. One may reasonably assume that controls in the catchment area for the hospitals, or those already in the hospitals for a different reason, would attend those hospitals if they became a case; therefore, the controls are drawn from the same population as the cases. If the controls were recruited or selected from a different region (e.g., a State other than Texas) or time period (e.g., 1991-2000), then the cases and controls were recruited from different populations, and the answer to this question would be "no."
The following example further explores selection of controls. In a study, eligible cases were men and women, ages 18 to 39, who were diagnosed with atherosclerosis at hospitals in Perth, Australia, between July 1, 2000 and December 31, 2007. Appropriate controls for these cases might be sampled using voter registration information for men and women ages 18 to 39, living in Perth (population-based controls); they also could be sampled from patients without atherosclerosis at the same hospitals (hospital-based controls). As long as the controls are individuals who would have been eligible to be included in the study as cases (if they had been diagnosed with atherosclerosis), then the controls were selected appropriately from the same source population as cases.
In a prospective case-control study, investigators may enroll individuals as cases at the time they are found to have the outcome of interest; the number of cases usually increases as time progresses. At this same time, they may recruit or select controls from the population without the outcome of interest. One way to identify or recruit cases is through a surveillance system. In turn, investigators can select controls from the population covered by that system. This is an example of population-based controls. Investigators also may identify and select cases from a cohort study population and identify controls from outcome-free individuals in the same cohort study. This is known as a nested case-control study.
Question 6. Inclusion and exclusion criteria prespecified and applied uniformly
Were the inclusion and exclusion criteria developed prior to recruitment or selection of the study population? Were the same underlying criteria used for all of the groups involved? To answer this question, reviewers determined if the investigators developed I/E criteria prior to recruitment or selection of the study population and if they used the same underlying criteria for all groups. The investigators should have used the same selection criteria, except for study participants who had the disease or condition, which would be different for cases and controls by definition. Therefore, the investigators use the same age (or age range), gender, race, and other characteristics to select cases and controls. Information on this topic is usually found in a paper's section on the description of the study population.
Question 7. Case and control definitions
For this question, reviewers looked for descriptions of the validity of case and control definitions and processes or tools used to identify study participants as such. Was a specific description of "case" and "control" provided? Is there a discussion of the validity of the case and control definitions and the processes or tools used to identify study participants as such? They determined if the tools or methods were accurate, reliable, and objective. For example, cases might be identified as "adult patients admitted to a VA hospital from January 1, 2000 to December 31, 2009, with an ICD-9 discharge diagnosis code of acute myocardial infarction and at least one of the two confirmatory findings in their medical records: at least 2mm of ST elevation changes in two or more ECG leads and an elevated troponin level. Investigators might also use ICD-9 or CPT codes to identify patients. All cases should be identified using the same methods. Unless the distinction between cases and controls is accurate and reliable, investigators cannot use study results to draw valid conclusions.
Question 8. Random selection of study participants
If a case-control study did not use 100 percent of eligible cases and/or controls (e.g., not all disease-free participants were included as controls), did the authors indicate that random sampling was used to select controls? When it is possible to identify the source population fairly explicitly (e.g., in a nested case-control study, or in a registry-based study), then random sampling of controls is preferred. When investigators used consecutive sampling, which is frequently done for cases in prospective studies, then study participants are not considered randomly selected. In this case, the reviewers would answer "no" to Question 8. However, this would not be considered a fatal flaw.
If investigators included all eligible cases and controls as study participants, then reviewers marked "NA" in the tool. If 100 percent of cases were included (e.g., NA for cases) but only 50 percent of eligible controls, then the response would be "yes" if the controls were randomly selected, and "no" if they were not. If this cannot be determined, the appropriate response is "CD."
Question 9. Concurrent controls
A concurrent control is a control selected at the time another person became a case, usually on the same day. This means that one or more controls are recruited or selected from the population without the outcome of interest at the time a case is diagnosed. Investigators can use this method in both prospective case-control studies and retrospective case-control studies. For example, in a retrospective study of adenocarcinoma of the colon using data from hospital records, if hospital records indicate that Person A was diagnosed with adenocarcinoma of the colon on June 22, 2002, then investigators would select one or more controls from the population of patients without adenocarcinoma of the colon on that same day. This assumes they conducted the study retrospectively, using data from hospital records. The investigators could have also conducted this study using patient records from a cohort study, in which case it would be a nested case-control study.
Investigators can use concurrent controls in the presence or absence of matching and vice versa. A study that uses matching does not necessarily mean that concurrent controls were used.
Question 10. Exposure assessed prior to outcome measurement
Investigators first determine case or control status (based on presence or absence of outcome of interest), and then assess exposure history of the case or control; therefore, reviewers ascertained that the exposure preceded the outcome. For example, if the investigators used tissue samples to determine exposure, did they collect them from patients prior to their diagnosis? If hospital records were used, did investigators verify that the date a patient was exposed (e.g., received medication for atherosclerosis) occurred prior to the date they became a case (e.g., was diagnosed with type 2 diabetes)? For an association between an exposure and an outcome to be considered causal, the exposure must have occurred prior to the outcome.
Question 11. Exposure measures and assessment
Were the exposure measures defined in detail? Were the tools or methods used to measure exposure accurate and reliable–for example, have they been validated or are they objective? This is important, as it influences confidence in the reported exposures. Equally important is whether the exposures were assessed in the same manner within groups and between groups. This question pertains to bias resulting from exposure misclassification (i.e., exposure ascertainment).
For example, a retrospective self-report of dietary salt intake is not as valid and reliable as prospectively using a standardized dietary log plus testing participants' urine for sodium content because participants' retrospective recall of dietary salt intake may be inaccurate and result in misclassification of exposure status. Similarly, BP results from practices that use an established protocol for measuring BP would be considered more valid and reliable than results from practices that did not use standard protocols. A protocol may include using trained BP assessors, standardized equipment (e.g., the same BP device which has been tested and calibrated), and a standardized procedure (e.g., patient is seated for 5 minutes with feet flat on the floor, BP is taken twice in each arm, and all four measurements are averaged).
Question 12. Blinding of exposure assessors
Blinding or masking means that outcome assessors did not know whether participants were exposed or unexposed. To answer this question, reviewers examined articles for evidence that the outcome assessor(s) was masked to the exposure status of the research participants. An outcome assessor, for example, may examine medical records to determine the outcomes that occurred in the exposed and comparison groups. Sometimes the person measuring the exposure is the same person conducting the outcome assessment. In this case, the outcome assessor would most likely not be blinded to exposure status. A reviewer would note such a finding in the comments section of the assessment tool.
One way to ensure good blinding of exposure assessment is to have a separate committee, whose members have no information about the study participants' status as cases or controls, review research participants' records. To help answer the question above, reviewers determined if it was likely that the outcome assessor knew whether the study participant was a case or control. If it was unlikely, then the reviewers marked "no" to Question 12. Outcome assessors who used medical records to assess exposure should not have been directly involved in the study participants' care, since they probably would have known about their patients' conditions. If the medical records contained information on the patient's condition that identified him/her as a case (which is likely), that information would have had to be removed before the exposure assessors reviewed the records.
If blinding was not possible, which sometimes happens, the reviewers marked "NA" in the assessment tool and explained the potential for bias.
Question 13. Statistical analysis
Were key potential confounding variables measured and adjusted for, such as by statistical adjustment for baseline differences? Investigators often use logistic regression or other regression methods to account for the influence of variables not of interest.
This is a key issue in case-controlled studies; statistical analyses need to control for potential confounders, in contrast to RCTs in which the randomization process controls for potential confounders. In the analysis, investigators need to control for all key factors that may be associated with both the exposure of interest and the outcome and are not of interest to the research question.
A study of the relationship between smoking and CVD events illustrates this point. Such a study needs to control for age, gender, and body weight; all are associated with smoking and CVD events. Well-done case-control studies control for multiple potential confounders.
Matching is a technique used to improve study efficiency and control for known confounders. For example, in the study of smoking and CVD events, an investigator might identify cases that have had a heart attack or stroke and then select controls of similar age, gender, and body weight to the cases. For case-control studies, it is important that if matching was performed during the selection or recruitment process, the variables used as matching criteria (e.g., age, gender, race) should be controlled for in the analysis.
General Guidance for Determining the Overall Quality Rating of Case-Controlled Studies
NHLBI designed the questions in the assessment tool to help reviewers focus on the key concepts for evaluating a study's internal validity, not to use as a list from which to add up items to judge a study's quality.
Internal validity for case-control studies is the extent to which the associations between disease and exposure reported in the study can truly be attributed to the exposure being evaluated rather than to flaws in the design or conduct of the study. In other words, what is ability of the study to draw associative conclusions about the effects of the exposures on outcomes? Any such flaws can increase the risk of bias.
In critical appraising a study, the following factors need to be considered: risk of potential for selection bias, information bias, measurement bias, or confounding (the mixture of exposures that one cannot tease out from each other). Examples of confounding include co-interventions, differences at baseline in patient characteristics, and other issues addressed in the questions above. High risk of bias translates to a poor quality rating; low risk of bias translates to a good quality rating. Again, the greater the risk of bias, the lower the quality rating of the study.
In addition, the more attention in the study design to issues that can help determine whether there is a causal relationship between the outcome and the exposure, the higher the quality of the study. These include exposures occurring prior to outcomes, evaluation of a dose-response gradient, accuracy of measurement of both exposure and outcome, sufficient timeframe to see an effect, and appropriate control for confounding–all concepts reflected in the tool.
If a study has a "fatal flaw," then risk of bias is significant; therefore, the study is deemed to be of poor quality. An example of a fatal flaw in case-control studies is a lack of a consistent standard process used to identify cases and controls.
Generally, when reviewers evaluated a study, they did not see a "fatal flaw," but instead found some risk of bias. By focusing on the concepts underlying the questions in the quality assessment tool, reviewers examined the potential for bias in the study. For any box checked "no," reviewers asked, "What is the potential risk of bias resulting from this flaw in study design or execution?" That is, did this factor lead to doubt about the results reported in the study or the ability of the study to accurately assess an association between exposure and outcome?
By examining questions in the assessment tool, reviewers were best able to assess the potential for bias in a study. Specific rules were not useful, as each study had specific nuances. In addition, being familiar with the key concepts helped reviewers assess the studies. Examples of studies rated good, fair, and poor were useful, yet each study had to be assessed on its own.
Quality Assessment Tool for Before-After (Pre-Post) Studies With No Control Group - Study Quality Assessment Tools
Guidance for Assessing the Quality of Before-After (Pre-Post) Studies With No Control Group
Question 1. Study question
Question 2. Eligibility criteria and study population
Did the authors describe the eligibility criteria applied to the individuals from whom the study participants were selected or recruited? In other words, if the investigators were to conduct this study again, would they know whom to recruit, from where, and from what time period?
Here is a sample description of a study population: men over age 40 with type 2 diabetes, who began seeking medical care at Phoenix Good Samaritan Hospital, between January 1, 2005 and December 31, 2007. The population is clearly described as: (1) who (men over age 40 with type 2 diabetes); (2) where (Phoenix Good Samaritan Hospital); and (3) when (between January 1, 2005 and December 31, 2007). Another sample description is women who were in the nursing profession, who were ages 34 to 59 in 1995, had no known CHD, stroke, cancer, hypercholesterolemia, or diabetes, and were recruited from the 11 most populous States, with contact information obtained from State nursing boards.
To assess this question, reviewers examined prior papers on study methods (listed in reference list) when necessary.
Question 3. Study participants representative of clinical populations of interest
The participants in the study should be generally representative of the population in which the intervention will be broadly applied. Studies on small demographic subgroups may raise concerns about how the intervention will affect broader populations of interest. For example, interventions that focus on very young or very old individuals may affect middle-aged adults differently. Similarly, researchers may not be able to extrapolate study results from patients with severe chronic diseases to healthy populations.
Question 4. All eligible participants enrolled
To further explore this question, reviewers may need to ask: Did the investigators develop the I/E criteria prior to recruiting or selecting study participants? Were the same underlying I/E criteria used for all research participants? Were all subjects who met the I/E criteria enrolled in the study?
Question 5. Sample size
Did the authors present their reasons for selecting or recruiting the number of individuals included or analyzed? Did they note or discuss the statistical power of the study? This question addresses whether there was a sufficient sample size to detect an association, if one did exist.
An article's methods section may provide information on the sample size needed to detect a hypothesized difference in outcomes and a discussion on statistical power (such as, the study had 85 percent power to detect a 20 percent increase in the rate of an outcome of interest, with a 2-sided alpha of 0.05). Sometimes estimates of variance and/or estimates of effect size are given, instead of sample size calculations. In any case, if the reviewers determined that the power was sufficient to detect the effects of interest, then they would answer "yes" to Question 5.
Question 6. Intervention clearly described
Another pertinent question regarding interventions is: Was the intervention clearly defined in detail in the study? Did the authors indicate that the intervention was consistently applied to the subjects? Did the research participants have a high level of adherence to the requirements of the intervention? For example, if the investigators assigned a group to 10 mg/day of Drug A, did most participants in this group take the specific dosage of Drug A? Or did a large percentage of participants end up not taking the specific dose of Drug A indicated in the study protocol?
Reviewers ascertained that changes in study outcomes could be attributed to study interventions. If participants received interventions that were not part of the study protocol and could affect the outcomes being assessed, the results could be biased.
Question 7. Outcome measures clearly described, valid, and reliable
Were the outcomes defined in detail? Were the tools or methods for measuring outcomes accurate and reliable–for example, have they been validated or are they objective? This question is important because the answer influences confidence in the validity of study results.
An example of an outcome measure that is objective, accurate, and reliable is death–the outcome measured with more accuracy than any other. But even with a measure as objective as death, differences can exist in the accuracy and reliability of how investigators assessed death. For example, did they base it on an autopsy report, death certificate, death registry, or report from a family member? Another example of a valid study is one whose objective is to determine if dietary fat intake affects blood cholesterol level (cholesterol level being the outcome) and in which the cholesterol level is measured from fasting blood samples that are all sent to the same laboratory. These examples would get a "yes."
An example of a "no" would be self-report by subjects that they had a heart attack, or self-report of how much they weight (if body weight is the outcome of interest).
Question 8. Blinding of outcome assessors
Blinding or masking means that the outcome assessors did not know whether the participants received the intervention or were exposed to the factor under study. To answer the question above, the reviewers examined articles for evidence that the person(s) assessing the outcome(s) was masked to the participants' intervention or exposure status. An outcome assessor, for example, may examine medical records to determine the outcomes that occurred in the exposed and comparison groups. Sometimes the person applying the intervention or measuring the exposure is the same person conducting the outcome assessment. In this case, the outcome assessor would not likely be blinded to the intervention or exposure status. A reviewer would note such a finding in the comments section of the assessment tool.
In assessing this criterion, the reviewers determined whether it was likely that the person(s) conducting the outcome assessment knew the exposure status of the study participants. If not, then blinding was adequate. An example of adequate blinding of the outcome assessors is to create a separate committee whose members were not involved in the care of the patient and had no information about the study participants' exposure status. Using a study protocol, committee members would review copies of participants' medical records, which would be stripped of any potential exposure information or personally identifiable information, for prespecified outcomes.
Question 9. Followup rate
Higher overall followup rates are always desirable to lower followup rates, although higher rates are expected in shorter studies, and lower overall followup rates are often seen in longer studies. Usually an acceptable overall followup rate is considered 80 percent or more of participants whose interventions or exposures were measured at baseline. However, this is a general guideline.
In accounting for those lost to followup, in the analysis, investigators may have imputed values of the outcome for those lost to followup or used other methods. For example, they may carry forward the baseline value or the last observed value of the outcome measure and use these as imputed values for the final outcome measure for research participants lost to followup.
Question 10. Statistical analysis
Were formal statistical tests used to assess the significance of the changes in the outcome measures between the before and after time periods? The reported study results should present values for statistical tests, such as p values, to document the statistical significance (or lack thereof) for the changes in the outcome measures found in the study.
Question 11. Multiple outcome measures
Were the outcome measures for each person measured more than once during the course of the before and after study periods? Multiple measurements with the same result increase confidence that the outcomes were accurately measured.
Question 12. Group-level interventions and individual-level outcome efforts
Group-level interventions are usually not relevant for clinical interventions such as bariatric surgery, in which the interventions are applied at the individual patient level. In those cases, the questions were coded as "NA" in the assessment tool.
General Guidance for Determining the Overall Quality Rating of Before-After Studies
The questions in the quality assessment tool were designed to help reviewers focus on the key concepts for evaluating the internal validity of a study. They are not intended to create a list from which to add up items to judge a study's quality.
Internal validity is the extent to which the outcome results reported in the study can truly be attributed to the intervention or exposure being evaluated, and not to biases, measurement errors, or other confounding factors that may result from flaws in the design or conduct of the study. In other words, what is the ability of the study to draw associative conclusions about the effects of the interventions or exposures on outcomes?
Critical appraisal of a study involves considering the risk of potential for selection bias, information bias, measurement bias, or confounding (the mixture of exposures that one cannot tease out from each other). Examples of confounding include co-interventions, differences at baseline in patient characteristics, and other issues throughout the questions above. High risk of bias translates to a rating of poor quality; low risk of bias translates to a rating of good quality. Again, the greater the risk of bias, the lower the quality rating of the study.
In addition, the more attention in the study design to issues that can help determine if there is a causal relationship between the exposure and outcome, the higher quality the study. These issues include exposures occurring prior to outcomes, evaluation of a dose-response gradient, accuracy of measurement of both exposure and outcome, and sufficient timeframe to see an effect.
Generally, when reviewers evaluate a study, they will not see a "fatal flaw," but instead will find some risk of bias. By focusing on the concepts underlying the questions in the quality assessment tool, reviewers should ask themselves about the potential for bias in the study they are critically appraising. For any box checked "no" reviewers should ask, "What is the potential risk of bias resulting from this flaw in study design or execution?" That is, does this factor lead to doubt about the results reported in the study or doubt about the ability of the study to accurately assess an association between the intervention or exposure and the outcome?
The best approach is to think about the questions in the assessment tool and how each one reveals something about the potential for bias in a study. Specific rules are not useful, as each study has specific nuances. In addition, being familiar with the key concepts will help reviewers be more comfortable with critical appraisal. Examples of studies rated good, fair, and poor are useful, but each study must be assessed on its own.
Quality Assessment Tool for Case Series Studies - Study Quality Assessment Tools
Background: development and use - study quality assessment tools.
Learn more about the development and use of Study Quality Assessment Tools.
Last updated: July, 2021
For enquiries call:
+1-469-442-0620

Top Six Sigma Case Study 2024
Home Blog Quality Top Six Sigma Case Study 2024

Six Sigma is an array of methods and resources for enhancing corporate operations. When Bill Smith was an engineer at Motorola, he introduced it in 1986 to find and eliminate mistakes and defects, reduce variance, and improve quality and efficiency. Six Sigma was first used in manufacturing as a quality control tool. When long-term defect levels are less than 3.4 defects per million opportunities (DPMO), Six Sigma quality is reached.
Six Sigma case study offers a glimpse into how various companies have harnessed the five distinct phases: defining, measuring, analyzing, improving, and controlling, principles of Six Sigma to overcome challenges, streamline processes, and improve across diverse industries.
What Are Six Sigma Case Studies, and Why Are They Important?
Six Sigma case studies examples show how Six Sigma techniques have been used in businesses to solve issues or enhance operations. For practitioners and companies pondering enforcing Six Sigma concepts, these case studies are an invaluable resource to learn the advantages and efficacy of Six Sigma adoption.
Here are the reasons why six sigma case study is important:
Success Illustration: Case studies demonstrate how Six Sigma projects generate tangible advantages like better productivity, fewer defects, and more customer satisfaction while providing unambiguous evidence of their efficacy.
Learning Opportunities: They deliver vital insights to use Six Sigma tools and processes realistically and allow others to learn from successful approaches and avoid common errors.
ROI Demonstration: Case studies provide quantitative data to show the return on investment from Six Sigma projects, which helps justify resources and get support for future initiatives.
Promoting Adoption: They cultivate a continuous improvement culture and show how Six Sigma concepts can be used in different situations and sectors, which encourages other businesses to embrace the methodology.
Become a Six Sigma Certified Professional and lead process improvement teams to success. Learn how to streamline processes and drive organizational growth in any industry. Join our Lean 6 Sigma training courses and transform your career trajectory with valuable skills and industry recognition.
Six Sigma Case Studies
Let us discuss some real-world case study on six sigma examples of successful Six Sigma undertakings through case studies:
1. Six Sigma Success: Catalent Pharma Solutions
Do you know how Six Sigma techniques turned things around for Catalent Pharma Solutions?
Six Sigma methodologies, initially presented by Motorola in 1986 and prominently used by General Electric during CEO Jack Welch's leadership, are essential for enhancing customer contentment via defect minimization. Catalent Pharma Solutions, a top pharmaceutical development business, employed Six Sigma to address high mistake rates in its Zydis product line. By applying statistical analysis and automation, training employees to various belt levels, and implementing Six Sigma procedures, Catalent was able to maintain product batches and boost production. This case study illustrates how Six Sigma approaches are beneficial for businesses across all industries as they can improve processes, prevent losses, and aid in cost reduction.
2. TDLR's Record Management: A Six Sigma Success Story
The Texas Department of Licensing and Regulation (TDLR) faced escalating costs due to the storage of records, prompting a Six Sigma initiative led by Alaric Robertson. By implementing Six Sigma methodologies, process mapping, and systematic review, TDLR successfully reduced storage costs and streamlined record management processes. With a team effort and strategic changes, TDLR has achieved significant cost savings and improved efficiency. The project also led to the establishment of a robust records management department within TDLR.
3. Six Sigma Environmental Success: Baxter Manufacturing
Baxter Manufacturing utilized Six Sigma principles to enhance its environmental performance and aim for greater efficiency. Through the implementation of Lean manufacturing and accurate data collection, Baxter reduced waste generation while doubling revenue and maintaining waste levels. With a cross-functional team trained in Six Sigma, the company achieved significant water and cost savings without major investments in technology. It led to promotions for team leaders and showcased the effectiveness of Six Sigma in improving environmental sustainability.
4. Aerospace Manufacturer Boosts Efficiency With Six Sigma
Have you heard about how Six Sigma principles transformed an aerospace parts manufacturer? Here is the 6 Sigma case study for aerospace parts manufacturer
A small aerospace parts manufacturer used Six Sigma to cut machining cycle time, reducing costs. Key engineers obtained Six Sigma certification and led the project, involving management and operators. Using DMAIC, they analyzed data, identified root causes, and implemented lean solutions. The process yielded a 46% reduction in cycle time and an 80% decrease in variation, enhanced productivity and profitability. The case highlights how Six Sigma principles can benefit businesses of all sizes and emphasizes the importance of training for successful implementation.
Enroll in the Lean Six Sigma Green Belt certification online training to advance your career! Gain expertise in process improvement and organizational transformation with expert-led training and real-world case studies. Start now to become a certified professional in quality management.
5. Ford Motors: Driving Success
This is a case study on Six Sigma i ncorporated by Ford Motors to streamline processes, improve quality, significantly reduce costs, and reduce environmental impact. Initially met with skepticism, Ford's implementation overcame challenges, achieving remarkable results: $2.19 billion in waste reduction, $1 billion in savings, and a five-point increase in customer satisfaction. Ford's Consumer-driven Six Sigma initiative set a benchmark in the automotive industry and proved the efficacy of data-driven problem-solving. Despite obstacles, Ford's Six Sigma exemplifies transformative success in process improvement and customer satisfaction enhancement.
6. 3M's Pollution Prevention Six Sigma Success
Have you checked out how 3M tackled pollution with Six Sigma? It's pretty remarkable. 3M leveraged Six Sigma to pioneer pollution prevention, saving $1 billion and averting 2.6 million pounds of pollutants over 31 years. With 55,000 employees trained and 45,000 Lean Six Sigma projects completed, they focused on waste reduction and energy efficiency. Results included a 61% decrease in volatile air emissions and a 64% reduction in EPA Toxic Release Inventory. Surpassing goals, they doubled Pollution Prevention Pays projects and showcased Six Sigma's prowess in cost-saving measures.
7. Microsoft Sigma Story Lean Six Sigma
By using Lean Six Sigma case studies, Microsoft increased customer interactions and profitability through waste removal and process optimization. They concentrated on improving the quality of the current process and reducing problems by utilizing the DMAIC technique. Eight areas were the focus of waste elimination: motion, inventory, non-value-added procedures, waiting periods, overproduction, defects, and underutilized staff talent. Microsoft streamlined processes and encouraged innovation, which allowed them to maintain productivity and client satisfaction even as technology changed.
8. Xerox's Lean Six Sigma Success Story Six Sigma
It is another important case study of the Six Sigma project. When Xerox implemented Lean Six Sigma in 2003, the organization underwent a significant transformation. They reduced variance and eliminated waste as they painstakingly optimized internal operations. It improved their operational effectiveness and raised the caliber of their goods and services. Through extensive training programs for staff members, Xerox enabled its employees to spearhead projects aimed at improving different departments and functions. The organization saw significant improvements in customer satisfaction and service performance.
9. A Green Belt Project Six Sigma Case Study
It is one of the best examples of a Six Sigma case study. Anne Cesarone's Green Belt project successfully reduced router configuration time by 16 minutes, a remarkable 55% improvement. Anne maintained router inventory, made improvements to documentation and configuration files, and started router requests sooner by resolving last-minute requests and setup mistakes. The initiative resulted in less router programming time from 29 to 13 minutes, an increase in router order lead time of 11 days, and a 60% drop in incorrect configurations. These raised customer happiness and increased operational effectiveness while proving the benefits of process improvement initiatives.
10. Improving Street Maintenance Payments with Lean Six Sigma
Jessica Shirley-Saenz, a Black Belt at the City of San Antonio, used Lean Six Sigma to address delays in street maintenance payments Lean Six Sigma case study examples. Contractors were experiencing extended payment times, risking project delays and city infrastructure integrity. Root causes included payment rejections and delayed invoicing. By implementing quantity tolerance thresholds, centralizing documentation processes, and updating payment workflows, monthly payment requests increased from 97 to 116. Rejected payments decreased from 17 to 12, reducing the rejection percentage from 58% to 42%, saving $6.6 million.
Six Sigma's effectiveness spans industries, from healthcare to technology. Case studies demonstrate its ability to optimize processes and improve outcomes. From healthcare facilities streamlining patient care to tech companies enhancing software development, Six Sigma offers adaptable solutions for diverse challenges. These real-world examples illustrate how its methodologies drive efficiency, quality, and customer satisfaction. Professionals can learn valuable lessons from using Six Sigma in healthcare studies, identify strategies to overcome obstacles and facilitate continuous improvement. Organizations can emulate best practices and implement similar initiatives to achieve measurable results by studying successful implementations.
Ready to enhance your skills and advance your career with Six Sigma certification? Join our comprehensive KnowledgeHut's best lean Six Sigma courses to master Six Sigma principles and methodologies. Become a sought-after professional in IT, Manufacturing, Healthcare, Finance, and more industries. Enroll now to accelerate your career growth!
Frequently Asked Questions (FAQs)
Six Sigma case studies are available in various formats and places, such as books, academic journals, professional publications, and Internet sites. Many companies that have effectively adopted Six Sigma publish their case studies on their websites or at industry exhibitions and conferences.
Six Sigma case studies provide insightful information on how businesses have addressed certain issues, enhanced procedures, and produced noticeable outcomes. Professionals gain knowledge about best practices, prevalent errors to avoid, and creative problem-solving methods in several industries and circumstances.
Professionals can share their Six Sigma case studies through industry forums, professional networking platforms, blogs, and social media. They can submit their case studies to publications or at conferences and workshops to reach a wider audience within the Six Sigma community.

Shivender Sharma
Shivendra Sharma, an accomplished author of the international bestseller 'Being Yogi,' is a multifaceted professional. With an MBA in HR and a Lean Six Sigma Master Black Belt, he boasts 15 years of experience in business and digital transformation, strategy consulting, and process improvement. As a member of the Technical Committee of the International Association of Six Sigma Certification (IASSC), he has led multi-million dollar savings through organization-wide transformation projects. Shivendra's expertise lies in deploying Lean and Six Sigma tools across global stakeholders in EMEA, North America, and APAC, achieving remarkable business results.
Avail your free 1:1 mentorship session.
Something went wrong
Upcoming Quality Management Batches & Dates

- Work & Careers
- Life & Arts
Become an FT subscriber
Limited time offer save up to 40% on standard digital.
- Global news & analysis
- Expert opinion
- Special features
- FirstFT newsletter
- Videos & Podcasts
- Android & iOS app
- FT Edit app
- 10 gift articles per month
Explore more offers.
Standard digital.
- FT Digital Edition
Premium Digital
Print + premium digital.
Then $75 per month. Complete digital access to quality FT journalism on any device. Cancel anytime during your trial.
- 10 additional gift articles per month
- Global news & analysis
- Exclusive FT analysis
- Videos & Podcasts
- FT App on Android & iOS
- Everything in Standard Digital
- Premium newsletters
- Weekday Print Edition
Complete digital access to quality FT journalism with expert analysis from industry leaders. Pay a year upfront and save 20%.
- Everything in Print
- Everything in Premium Digital
The new FT Digital Edition: today’s FT, cover to cover on any device. This subscription does not include access to ft.com or the FT App.
Terms & Conditions apply
Explore our full range of subscriptions.
Why the ft.
See why over a million readers pay to read the Financial Times.
International Edition
Help | Advanced Search
Computer Science > Computer Vision and Pattern Recognition
Title: aniportrait: audio-driven synthesis of photorealistic portrait animation.
Abstract: In this study, we propose AniPortrait, a novel framework for generating high-quality animation driven by audio and a reference portrait image. Our methodology is divided into two stages. Initially, we extract 3D intermediate representations from audio and project them into a sequence of 2D facial landmarks. Subsequently, we employ a robust diffusion model, coupled with a motion module, to convert the landmark sequence into photorealistic and temporally consistent portrait animation. Experimental results demonstrate the superiority of AniPortrait in terms of facial naturalness, pose diversity, and visual quality, thereby offering an enhanced perceptual experience. Moreover, our methodology exhibits considerable potential in terms of flexibility and controllability, which can be effectively applied in areas such as facial motion editing or face reenactment. We release code and model weights at this https URL
Submission history
Access paper:.
- HTML (experimental)
- Other Formats
References & Citations
- Google Scholar
- Semantic Scholar
BibTeX formatted citation

Bibliographic and Citation Tools
Code, data and media associated with this article, recommenders and search tools.
- Institution
arXivLabs: experimental projects with community collaborators
arXivLabs is a framework that allows collaborators to develop and share new arXiv features directly on our website.
Both individuals and organizations that work with arXivLabs have embraced and accepted our values of openness, community, excellence, and user data privacy. arXiv is committed to these values and only works with partners that adhere to them.
Have an idea for a project that will add value for arXiv's community? Learn more about arXivLabs .
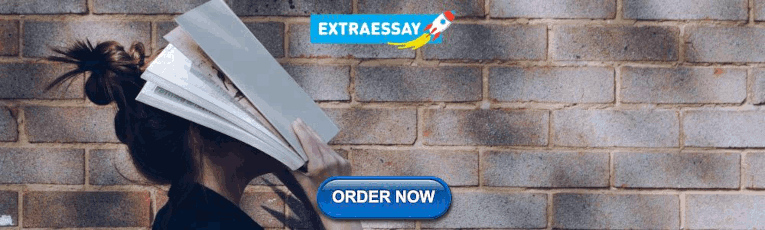
IMAGES
VIDEO
COMMENTS
The 7 QC tools, when combined with the systematic approach of the PDCA cycle, can yield impressive results, as demonstrated in our case study. Addressing quality issues requires a methodical, data ...
Flow Charts. Cause and Effect Diagram (Fishbone or Ishikawa) Checksheet. Histogram. Pareto Chart. Scatter Diagram. Control Chart. Note: We are considering here the Flow chart in this article as a part of 7 Basic QC Tools. Also, you can consider stratification as a part of this tool.
7 Basic Quality Tool Templates. These templates will help you get started using the seven basic quality tools. Just download the spreadsheets and begin entering your own data. Cause-and-effect diagram template (Excel) Check sheet template (Excel) Control chart template (Excel) Histogram template (Excel)
Search more than 1,000 examples of case studies sharing quality solutions to real-world problems. Find more case studies Featured Case Studies Classic Case Studies ... Using DMAIC and other quality tools, team members improved the process' sigma level from 0.7 to 3.3. Read the Case Study.
It involves analysis of the usage of the 7 QC tools and identifying the barriers, benefits, challenges and critical success factors (CSFs) for the application of the 7 QC tools in a HEI setting.,An online survey instrument was developed, and as this is a global study, survey participants were contacted via social networks such as LinkedIn.
Stratification. Histogram. Check sheet (tally sheet) Cause and effect diagram (fishbone or Ishikawa diagram) Pareto chart (80-20 rule) Scatter diagram. Control chart (Shewhart chart) The ability to identify and resolve quality-related issues quickly and efficiently is essential to anyone working in quality assurance or process improvement.
Jun 2014. M Varsha. Magar. Varsha M. Magar, "Application Of 7 Quality Control (7 Qc) Tools For Continuous Improvement Of Man-Ufacturing Processes", " Vol -2, Issue-4, June -2014 (Ijeres)" Increase ...
According to previous studies, 95% of quality problems can be solved by simple tools such as the basic quality tools. In this study, the data were tested by control charts, and were found to be ...
The purpose of the study was to apply some of the seven quality control tools (7 QC tools) for reducing delay the delivery problem. The Cause and Effect Diagram was found out the root causes of this problem and the Pareto Chart was used to help ordering the important of delay the delivery problem, it was found that coordination problem was the major cause. Finally, the Matrix Diagram was used ...
Topics to be covered in this course include: Introduction to methods and tools and the relationships between them based on a case study. Presentation of the following tools: Check sheet. Histogram. Pareto Chart. Scatter plot. Control chart. Ishikawa.
On the Use of Quality Tools: A Case Study. Fábio A. Fernandes, Sérgio D. Sousa, Member, IAENG and Isabel Lopes. . Abstract— Organizations need to improve their processes to continually achieve customer satisfaction and, to do that in an effective and efficient way, should use quality tools. The main objective of this research project is to ...
In this paper a review of systematic use of 7 QC tools is presented. The main aim of this paper is to provide an easy introduction of 7 QC tools and to improve the quality level of manufacturing ...
The 7 Quality Tools are widely applied by many industries for product and process improvements, and to solve critical quality problems. 7QC tools are extensively used in various Problem Solving Techniques which are listed below: 8D Problem Solving Methodology. PDCA Deming Cycle for Continuous improvement in product and processes.
The purpose of the study was to apply some of the seven quality control tools (7 QC tools) for reducing delay the delivery problem. The Cause and Effect Diagram was found out the root causes of this problem and the Pareto Chart was used to help ordering the important of delay the delivery problem, it was found that coordination problem was the major cause. Finally, the Matrix Diagram was used ...
PurposeThe main purpose of this study is to revisit Ishikawa's statement: "95% of problems in processes can be accomplished using the original 7 Quality Control (QC) tools". The paper critically investigates the validity of this statement in higher education institutions (HEIs). It involves analysis of the usage of the 7 QC tools and identifying the barriers, benefits, challenges and ...
In this paper a review of systematic use of 7 QC tools is presented. The main aim of this paper is to provide an easy introduction of 7 QC tools and to improve the quality level of manufacturing processes by applying it.QC tools are the means for Collecting data , analyzing data , identifying root causes and measuring the results. these tools are related to numerical data processing .All of ...
The study results are comparable to a study in health-care organizations by McDermott et al. (Citation 2022) in regard to the cause and effect diagram being one of the most commonly used quality tools, with stratification being one of the least commonly used quality tools. The differences between the studies may potentially be due to ...
Business-related examples and case studies. Downloadable resources for learning 7 basic quality tools and quality management. Your queries will be responded by the Instructor himself. Start using 7 basic quality tools to their full potential to become proficient at tools of quality today! Either you're new to excel and quality tools, or you've ...
The 7 Basic Tools of Quality. E-Learning 45 Minutes Beginner Self-paced / e-Learning. You will learn what the 7 basic tools of quality are and how they are used. You will receive worksheet templates making it easy to apply the tools in your day-to-day work. The 7 basic tools of quality - E-Learning. Watch on.
This e-learning course uses a case study to describe all 7 tools of quality and to demonstrate their application. World-Class Training Learn from TÜV SÜD's industry experts and training specialists. Flexible Learning Style Study in your own time, at your own pace. Continuous Feedback Get prompt feedback from content-embedded assessment
Case Study - Improving Customer Support in an E-commerce BPO. Let's illustrate the importance of these 7 quality tools in BPO with a real-world case study: Background. An e-commerce company decided to outsource its customer support operations to a BPO provider to manage the increasing volume of customer inquiries.
In 2013, NHLBI developed a set of tailored quality assessment tools to assist reviewers in focusing on concepts that are key to a study's internal validity. The tools were specific to certain study designs and tested for potential flaws in study methods or implementation. Experts used the tools during the systematic evidence review process to ...
PurposeThe main objective of this study is to investigate the 7 new quality control or the 7 new management tools and their use in manufacturing organisations. This research investigates the understanding, knowledge of the tools and the level of application of the tools within the manufacturing sector. In addition, this paper aims to identify the benefits, challenges and critical success ...
This case study describes how the AHRQ QI Toolkit helped them in this endeavor. Just a few years ago, clinicians and administrators at Cedars-Sinai Medical Center in Los Angeles were questioning the usefulness of quality measures based on hospital inpatient administrative claims data, such as the AHRQ Quality Indicators (QIs).
This site provides pharmacists with recently released health literacy tools and other resources from the Agency for Healthcare Research and Quality (AHRQ). PSNet AHRQ's Patient Safety Network (PSNet) features a collection of the latest news and resources on patient safety, i nnovations and toolkits, opportunities for free CME, and trainings.
Access the Worksheet and Directions in Word (25 KB) and Worksheet and Directions in PDF (157 KB). Plan-Do-Study-Act Directions and Examples. The Plan-Do-Study-Act (PDSA) method is a way to test a change that is implemented. Going through the prescribed four steps guides the thinking process into breaking down the task into steps and then evaluating the outcome, improving on it, and testing again.
This is a case study on Six Sigma incorporated by Ford Motors to streamline processes, improve quality, significantly reduce costs, and reduce environmental impact. Initially met with skepticism, Ford's implementation overcame challenges, achieving remarkable results: $2.19 billion in waste reduction, $1 billion in savings, and a five-point ...
Saudi Arabia's Israel strategy upended by anger over Gaza war; Netflix adaptation of sci-fi novel 'The Three-Body Problem' sparks anger in China
In this study, we propose AniPortrait, a novel framework for generating high-quality animation driven by audio and a reference portrait image. Our methodology is divided into two stages. Initially, we extract 3D intermediate representations from audio and project them into a sequence of 2D facial landmarks. Subsequently, we employ a robust diffusion model, coupled with a motion module, to ...