- Open access
- Published: 27 October 2021
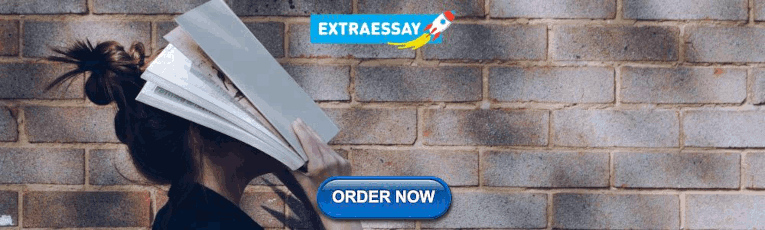
A narrative review on the validity of electronic health record-based research in epidemiology
- Milena A. Gianfrancesco 1 &
- Neal D. Goldstein ORCID: orcid.org/0000-0002-9597-5251 2
BMC Medical Research Methodology volume 21 , Article number: 234 ( 2021 ) Cite this article
12k Accesses
55 Citations
5 Altmetric
Metrics details
Electronic health records (EHRs) are widely used in epidemiological research, but the validity of the results is dependent upon the assumptions made about the healthcare system, the patient, and the provider. In this review, we identify four overarching challenges in using EHR-based data for epidemiological analysis, with a particular emphasis on threats to validity. These challenges include representativeness of the EHR to a target population, the availability and interpretability of clinical and non-clinical data, and missing data at both the variable and observation levels. Each challenge reveals layers of assumptions that the epidemiologist is required to make, from the point of patient entry into the healthcare system, to the provider documenting the results of the clinical exam and follow-up of the patient longitudinally; all with the potential to bias the results of analysis of these data. Understanding the extent of as well as remediating potential biases requires a variety of methodological approaches, from traditional sensitivity analyses and validation studies, to newer techniques such as natural language processing. Beyond methods to address these challenges, it will remain crucial for epidemiologists to engage with clinicians and informaticians at their institutions to ensure data quality and accessibility by forming multidisciplinary teams around specific research projects.
Peer Review reports
The proliferation of electronic health records (EHRs) spurred on by federal government incentives over the past few decades has resulted in greater than an 80% adoption-rate at hospitals [ 1 ] and close to 90% in office-based practices [ 2 ] in the United States. A natural consequence of the availability of electronic health data is the conduct of research with these data, both observational and experimental [ 3 ], due to lower overhead costs and lower burden of study recruitment [ 4 ]. Indeed, a search on PubMed for publications indexed by the MeSH term “electronic health records” reveals an exponential growth in biomedical literature, especially over the last 10 years with an excess of 50,000 publications.
An emerging literature is beginning to recognize the many challenges that still lay ahead in using EHR data for epidemiological investigations. Researchers in Europe identified 13 potential sources of “bias” (bias was defined as a contamination of the data) in EHR-based data covering almost every aspect of care delivery, from selective entrance into the healthcare system, to variation in care and documentation practices, to identification and extraction of the right data for analysis [ 5 ]. Many of the identified contaminants are directly relevant to traditional epidemiological threats to validity [ 4 ]. Data quality has consistently been invoked as a central challenge in EHRs. From a qualitative perspective, healthcare workers have described challenges in the healthcare environment (e.g., heavy workload), imperfect clinical documentation practices, and concerns over data extraction and reporting tools, all of which would impact the quality of data in the EHR [ 6 ]. From a quantitative perspective, researchers have noted limited sensitivity of diagnostic codes in the EHR when relying on discrete codings, noting that upon a manual chart review free text fields often capture the missed information, motivating such techniques as natural language processing (NLP) [ 7 ]. A systematic review of EHR-based studies also identified data quality as an overarching barrier to the use of EHRs in managing the health of the community, i.e. “population health” [ 8 ]. Encouragingly this same review also identified more facilitators than barriers to the use of EHRs in public health, suggesting that opportunities outweigh the challenges. Shortreed et al. further explored these opportunities discussing how EHRs can enhance pragmatic trials, bring additional sophistication to observational studies, aid in predictive modeling, and be linked together to create more comprehensive views of patients’ health [ 9 ]. Yet, as Shortreed and others have noted, significant challenges still remain.
It is our intention with this narrative review to discuss some of these challenges in further detail. In particular, we focus on specific epidemiological threats to validity -- internal and external -- and how EHR-based epidemiological research in particular can exacerbate some of these threats. We note that while there is some overlap in the challenges we discuss with traditional paper-based medical record research that has occurred for decades, the scale and scope of an EHR-based study is often well beyond what was traditionally possible in the manual chart review era and our applied examples attempt to reflect this. We also describe existing and emerging approaches for remediating these potential biases as they arise. A summary of these challenges may be found in Table 1 . Our review is grounded in the healthcare system in the United States, although we expect many of the issues we describe to be applicable regardless of locale; where necessary, we have flagged our comments as specific to the U.S.
Challenge #1: Representativeness
The selection process for how patients are captured in the EHR is complex and a function of geographic, social, demographic, and economic determinants [ 10 ]. This can be termed the catchment of the EHR. For a patient record to appear in the EHR the patient must have been registered in the system, typically to capture their demographic and billing information, and upon a clinical visit, their health details. While this process is not new to clinical epidemiology, what tends to separate EHR-based records from traditional paper-based records is the scale and scope of the data. Patient data may be available for longer periods of time longitudinally, as well as have data corresponding to interactions with multiple, potentially disparate, healthcare systems [ 11 ]. Given the consolidation of healthcare [ 12 ] and aggregated views of multiple EHRs through health information networks or exchanges [ 11 ] the ability to have a complete view of the patients’ total health is increasing. Importantly, the epidemiologist must ascertain whether the population captured within the EHR or EHR-derived data is representative of the population targeted for inference. This is particularly true under the paradigm of population health and inferring the health status of a community from EHR-based records [ 13 ]. For example, a study of Clostridium difficile infection at an urban safety net hospital in Philadelphia, Pennsylvania demonstrated notable differences in risk factors in the hospital’s EHR compared to national surveillance data, suggesting how catchment can influence epidemiologic measures [ 14 ]. Even health-related data captured through health information exchanges may be incomplete [ 15 ].
Several hypothetical study settings can further help the epidemiologist appreciate the relationship between representativeness and validity in EHR research. In the first hypothetical, an EHR-based study is conducted from a single-location federally qualified health center, and in the second hypothetical, an EHR-based study is conducted from a large academic health system. Suppose both studies occur in the same geographic area. It is reasonable to believe the patient populations captured in both EHRs will be quite different and the catchment process could lead to divergent estimates of disease or risk factor prevalence. The large academic health system may be less likely to capture primary care visits, as specialty care may drive the preponderance of patient encounters. However, this is not a bias per se : if the target of inference from these two hypothetical EHR-based studies is the local community, then selection bias becomes a distinct possibility. The epidemiologist must also consider the potential for generalizability and transportability -- two facets of external validity that respectively relate to the extrapolation of study findings to the source population or a different population altogether -- if there are unmeasured effect modifiers, treatment interference, or compound treatments in the community targeted for inference [ 16 ].
There are several approaches for ascertaining representativeness of EHR-based data. Comparing the EHR-derived sample to Census estimates of demography is straightforward but has several important limitations. First, as previously described, the catchment process may be driven by discordant geographical areas, especially for specialty care settings. Second and third, the EHR may have limited or inaccurate information on socioeconomic status, race, and ethnicity that one may wish to compare [ 17 , 18 ], and conversely the Census has limited estimates of health, chiefly disability, fertility, and insurance and payments [ 19 ]. If selection bias is suspected as a result of missing visits in a longitudinal study [ 20 ] or the catchment process in a cross-sectional study [ 21 ], using inverse probability weighting may remediate its influence. Comparing the weighted estimates to the original, non-weighted estimates provides insight into differences in the study participants. In the population health paradigm whereby the EHR is used as a surveillance tool to identify community health disparities [ 13 ], one also needs to be concerned about representativeness. There are emerging approaches for producing such small area community estimates from large observational datasets [ 22 , 23 ]. Conceivably, these approaches may also be useful for identifying issues of representativeness, for example by comparing stratified estimates across sociodemographic or other factors that may relate to catchment. Approaches for issues concerning representativeness specifically as it applies to external validity may be found in these references [ 24 , 25 ].
Challenge #2: Data availability and interpretation
Sub-challenge #2.1: billing versus clinical versus epidemiological needs.
There is an inherent tension in the use of EHR-based data for research purposes: the EHR was never originally designed for research. In the U.S., the Health Information Technology for Economic and Clinical Health Act, which promoted EHRs as a platform for comparative effectiveness research, was an attempt to address this deficiency [ 26 ]. A brief history of the evolution of the modern EHR reveals a technology that was optimized for capturing health details relevant for billing, scheduling, and clinical record keeping [ 27 ]. As such, the availability of data for fundamental markers of upstream health that are important for identifying inequities, such as socioeconomic status, race, ethnicity, and other social determinants of health (SDOH), may be insufficiently captured in the EHR [ 17 , 18 ]. Similarly, behavioral risk factors, such as being a sexual minority person, have historically been insufficiently recorded as discrete variables. It is only recently that such data are beginning to be captured in the EHR [ 28 , 29 ], or techniques such as NLP have made it possible to extract these details when stored in free text notes (described further in “ Unstructured data: clinical notes and reports ” section).
As an example, assessing clinical morbidities in the EHR may be done on the basis of extracting appropriate International Classification of Diseases (ICD) codes, used for billing and reimbursement in the U.S. These codes are known to have low sensitivity despite high specificity for accurate diagnostic status [ 30 , 31 ]. Expressed as predictive values, which depend upon prevalence, presence of a diagnostic code is a likely indicator of a disease state, whereas absence of a diagnostic code is a less reliable indicator of the absence of that morbidity. There may further be variation by clinical domain in that ICD codes may exist but not be used in some specialties [ 32 ], variation by coding vocabulary such as the use of SNOMED for clinical documentation versus ICD for billing necessitating an ontology mapper [ 33 ], and variation by the use of “rule-out” diagnostic codes resulting in false-positive diagnoses [ 34 , 35 , 36 ]. Relatedly is the notion of upcoding, or the billing of tests, procedures, or diagnoses to receive inflated reimbursement, which, although posited to be problematic in EHRs [ 37 ] in at least one study, has not been shown to have occurred [ 38 ]. In the U.S., the billing and reimbursement model, such as fee-for-service versus managed care, may result in varying diagnostic code sensitivities and specificities, especially if upcoding is occurring [ 39 ]. In short, there is potential for misclassification of key health data in the EHR.
Misclassification can potentially be addressed through a validation study (resources permitting) or application of quantitative bias analysis, and there is a rich literature regarding the treatment of misclassified data in statistics and epidemiology. Readers are referred to these texts as a starting point [ 40 , 41 ]. Duda et al. and Shepherd et al. have described an innovative data audit approach applicable to secondary analysis of observational data, such as EHR-derived data, that incorporates the audit error rate directly in the regression analysis to reduce information bias [ 42 , 43 ]. Outside of methodological tricks in the face of imperfect data, researchers must proactively engage with clinical and informatics colleagues to ensure that the right data for the research interests are available and accessible.
Sub-challenge #2.2: Consistency in data and interpretation
For the epidemiologist, abstracting data from the EHR into a research-ready analytic dataset presents a host of complications surrounding data availability, consistency and interpretation. It is easy to conflate the total volume of data in the EHR with data that are usable for research, however expectations should be tempered. Weiskopf et al. have noted such challenges for the researcher: in their study, less than 50% of patient records had “complete” data for research purposes per their four definitions of completeness [ 44 ]. Decisions made about the treatment of incomplete data can induce selection bias or impact precision of estimates (see Challenges #1 , #3 , and #4 ). The COVID-19 pandemic has further demonstrated the challenge of obtaining research data from EHRs across multiple health systems [ 45 ]. On the other hand, EHRs have a key advantage of providing near real-time data as opposed to many epidemiological studies that have a specific endpoint or are retrospective in nature. Such real-time data availability was leveraged during COVID-19 to help healthcare systems manage their pandemic response [ 46 , 47 ]. Logistical and technical issues aside, healthcare and documentation practices are nuanced to their local environments. In fact, researchers have demonstrated how the same research question analyzed in distinct clinical databases can yield different results [ 48 ].
Once the data are obtained, choices regarding operationalization of variables have the potential to induce information bias. Several hypothetical examples can help demonstrate this point. As a first example, differences in laboratory reporting may result in measurement error or misclassification. While the order for a particular laboratory assay is likely consistent within the healthcare system, patients frequently have a choice where to have that order fulfilled. Given the breadth of assays and reporting differences that may differ lab to lab [ 49 ], it is possible that the researcher working with the raw data may not consider all possible permutations. In other words, there may be lack of consistency in the reporting of the assay results. As a second example, raw clinical data requires interpretation to become actionable. A researcher interested in capturing a patient’s Charlson comorbidity index, which is based on 16 potential diagnoses plus the patient’s age [ 50 ], may never find such a variable in the EHR. Rather, this would require operationalization based on the raw data, each of which may be misclassified. Use of such composite measures introduces the notion of “differential item functioning”, whereby a summary indicator of a complexly measured health phenomenon may differ from group to group [ 51 ]. In this case, as opposed to a measurement error bias, this is one of residual confounding in that a key (unmeasured) variable is driving the differences. Remediation of these threats to validity may involve validation studies to determine the accuracy of a particular classifier, sensitivity analysis employing alternative interpretations when the raw data are available, and omitting or imputing biased or latent variables [ 40 , 41 , 52 ]. Importantly, in all cases, the epidemiologists should work with the various health care providers and personnel who have measured and recorded the data present in the EHR, as they likely understand it best.
Furthermore and related to “Billing versus Clinical versus Epidemiological Needs” section, the healthcare system in the U.S. is fragmented with multiple payers, both public and private, potentially exacerbating the data quality issues we describe, especially when linking data across healthcare systems. Single payer systems have enabled large and near-complete population-based studies due to data availability and consistency [ 53 , 54 , 55 ]. Data may also be inconsistent for retrospective longitudinal studies spanning many years if there have been changes to coding standards or practices over time, for example due to the transition from ICD-9 to ICD-10 largely occurring in the mid 2010s or the adoption of the Patient Protection and Affordable Care Act in the U.S. in 2010 with its accompanying changes in billing. Exploratory data analysis may reveal unexpected differences in key variables, by place or time, and recoding, when possible, can enforce consistency.
Sub-challenge #2.3: Unstructured data: clinical notes and reports
There may also be scenarios where structured data fields, while available, are not traditionally or consistently used within a given medical center or by a given provider. For example, reporting of adverse events of medications, disease symptoms, and vaccinations or hospitalizations occurring at different facility/health networks may not always be entered by providers in structured EHR fields. Instead, these types of patient experiences may be more likely to be documented in an unstructured clinical note, report (e.g. pathology or radiology report), or scanned document. Therefore, reliance on structured data to identify and study such issues may result in underestimation and potentially biased results.
Advances in NLP currently allow for information to be extracted from unstructured clinical notes and text fields in a reliable and accurate manner using computational methods. NLP utilizes a range of different statistical, machine learning, and linguistic techniques, and when applied to EHR data, has the potential to facilitate more accurate detection of events not traditionally located or consistently used in structured fields. Various NLP methods can be implemented in medical text analysis, ranging from simplistic and fast term recognition systems to more advanced, commercial NLP systems [ 56 ]. Several studies have successfully utilized text mining to extract information on a variety of health-related issues within clinical notes, such as opioid use [ 57 ], adverse events [ 58 , 59 ], symptoms (e.g., shortness of breath, depression, pain) [ 60 ], and disease phenotype information documented in pathology or radiology reports, including cancer stage, histology, and tumor grade [ 61 ], and lupus nephritis [ 32 ]. It is worth noting that scanned documents involve an additional layer of computation, relying on techniques such as optical character recognition, before NLP can be applied.
Hybrid approaches that combine both narrative and structured data, such as ICD codes, to improve accuracy of detecting phenotypes have also demonstrated high performance. Banerji et al. found that using ICD-9 codes to identify allergic drug reactions in the EHR had a positive predictive value of 46%, while an NLP algorithm in conjunction with ICD-9 codes resulted in a positive predictive value of 86%; negative predictive value also increased in the combined algorithm (76%) compared to ICD-9 codes alone (39%) [ 62 ]. In another example, researchers found that the combination of unstructured clinical notes with structured data for prediction tasks involving in-hospital mortality and 30-day hospital readmission outperformed models using either clinical notes or structured data alone [ 63 ]. As we move forward in analyzing EHR data, it will be important to take advantage of the wealth of information buried in unstructured data to assist in phenotyping patient characteristics and outcomes, capture missing confounders used in multivariate analyses, and develop prediction models.
Challenge #3: Missing measurements
While clinical notes may be useful to recover incomplete information from structured data fields, it may be the case that certain variables are not collected within the EHR at all. As mentioned above, it is important to remember that EHRs were not developed as a research tool (see “ Billing versus clinical versus epidemiological needs ” section), and important variables often used in epidemiologic research may not be typically included in EHRs including socioeconomic status (education, income, occupation) and SDOH [ 17 , 18 ]. Depending upon the interest of the provider or clinical importance placed upon a given variable, this information may be included in clinical notes. While NLP could be used to capture these variables, because they may not be consistently captured, there may be bias in identifying those with a positive mention as a positive case and those with no mention as a negative case. For example, if a given provider inquires about homelessness of a patient based on knowledge of the patient’s situation or other external factors and documents this in the clinical note, we have greater assurance that this is a true positive case. However, lack of mention of homelessness in a clinical note should not be assumed as a true negative case for several reasons: not all providers may feel comfortable asking about and/or documenting homelessness, they may not deem this variable worth noting, or implicit bias among clinicians may affect what is captured. As a result, such cases (i.e. no mention of homelessness) may be incorrectly identified as “not homeless,” leading to selection bias should a researcher form a cohort exclusively of patients who are identified as homeless in the EHR.
Not adjusting for certain measurements missing from EHR data can also lead to biased results if the measurement is an important confounder. Consider the example of distinguishing between prevalent and incident cases of disease when examining associations between disease treatments and patient outcomes [ 64 ]. The first date of an ICD code entered for a given patient may not necessarily be the true date of diagnosis, but rather documentation of an existing diagnosis. This limits the ability to adjust for disease duration, which may be an important confounder in studies comparing various treatments with patient outcomes over time, and may also lead to reverse causality if disease sequalae are assumed to be risk factors.
Methods to supplement EHR data with external data have been used to capture missing information. These methods may include imputation if information (e.g. race, lab values) is collected on a subset of patients within the EHR. It is important to examine whether missingness occurs completely at random or at random (“ignorable”), or not at random (“non-ignorable”), using the data available to determine factors associated with missingness, which will also inform the best imputation strategy to pursue, if any [ 65 , 66 ]. As an example, suppose we are interested in ascertaining a patient's BMI from the EHR. If men were less likely to have BMI measured than women, the probability of missing data (BMI) depends on the observed data (gender) and may therefore be predictable and imputable. On the other hand, suppose underweight individuals were less likely to have BMI measured; the probability of missing data depends on its own value, and as such is non-predictable and may require a validation study to confirm. Alternatively to imputing missing data, surrogate measures may be used, such as inferring area-based SES indicators, including median household income, percent poverty, or area deprivation index, by zip code [ 67 , 68 ]. Lastly, validation studies utilizing external datasets may prove helpful, such as supplementing EHR data with claims data that may be available for a subset of patients (see Challenge #4 ).
As EHRs are increasingly being used for research, there are active pushes to include more structured data fields that are important to population health research, such as SDOH [ 69 ]. Inclusion of such factors are likely to result in improved patient care and outcomes, through increased precision in disease diagnosis, more effective shared decision making, identification of risk factors, and tailoring services to a given population’s needs [ 70 ]. In fact, a recent review found that when individual level SDOH were included in predictive modeling, they overwhelmingly improved performance in medication adherence, risk of hospitalization, 30-day rehospitalizations, suicide attempts, and other healthcare services [ 71 ]. Whether or not these fields will be utilized after their inclusion in the EHR may ultimately depend upon federal and state incentives, as well as support from local stakeholders, and this does not address historic, retrospective analyses of these data.
Challenge #4: Missing visits
Beyond missing variable data that may not be captured during a clinical encounter, either through structured data or clinical notes, there also may be missing information for a patient as a whole. This can occur in a variety of ways; for example, a patient may have one or two documented visits in the EHR and then is never seen again (i.e. right censoring due to lost to follow-up), or a patient is referred from elsewhere to seek specialty care, with no information captured regarding other external issues (i.e. left censoring). This may be especially common in circumstances where a given EHR is more likely to capture specialty clinics versus primary care (see Challenge #1 ). A third scenario may include patients who appear, then are not observed for a long period of time, and then reappear: this case is particularly problematic as it may appear the patient was never lost to follow up but simply had fewer visits. In any of these scenarios, a researcher will lack a holistic view of the patient’s experiences, diagnoses, results, and more. As discussed above, assuming absence of a diagnostic code as absence of disease may lead to information and/or selection bias. Further, it has been demonstrated that one key source of bias in EHRs is “informed presence” bias, where those with more medical encounters are more likely to be diagnosed with various conditions (similar to Berkson’s bias) [ 72 ].
Several solutions to these issues have been proposed. For example, it is common for EHR studies to condition on observation time (i.e. ≥n visits required to be eligible into cohort); however, this may exclude a substantial amount of patients with certain characteristics, incurring a selection bias or limiting the generalizability of study findings (see Challenge #1 ). Other strategies attempt to account for missing visit biases through longitudinal imputation approaches; for example, if a patient missed a visit, a disease activity score can be imputed for that point in time, given other data points [ 73 , 74 ]. Surrogate measures may also be used to infer patient outcomes, such as controlling for “informative” missingness as an indicator variable or using actual number of missed visits that were scheduled as a proxy for external circumstances influencing care [ 20 ]. To address “informed presence” bias described above, conditioning on the number of health-care encounters may be appropriate [ 72 ]. Understanding the reason for the missing visit may help identify the best course of action and before imputing, one should be able to identify the type of missingness, whether “informative” or not [ 65 , 66 ]. For example, if distance to a healthcare location is related to appointment attendance, being able to account for this in analysis would be important: researchers have shown how the catchment of a healthcare facility can induce selection bias [ 21 ]. Relatedly, as telehealth becomes more common fueled by the COVID-19 pandemic [ 75 , 76 ], virtual visits may generate missingness of data recorded in the presence of a provider (e.g., blood pressure if the patient does not have access to a sphygmomanometer; see Challenge #3 ), or necessitate a stratified analysis by visit type to assess for effect modification.
Another common approach is to supplement EHR information with external data sources, such as insurance claims data, when available. Unlike a given EHR, claims data are able to capture a patient’s interaction with the health care system across organizations, and additionally includes pharmacy data such as if a prescription was filled or refilled. Often researchers examine a subset of patients eligible for Medicaid/Medicare and compare what is documented in claims with information available in the EHR [ 77 ]. That is, are there additional medications, diagnoses, hospitalizations found in the claims dataset that were not present in the EHR. In a study by Franklin et al., researchers utilized a linked database of Medicare Advantage claims and comprehensive EHR data from a multi-specialty outpatient practice to determine which dataset would be more accurate in predicting medication adherence [ 77 ]. They found that both datasets were comparable in identifying those with poor adherence, though each dataset incorporated different variables.
While validation studies such as those using claims data allow researchers to gain an understanding as to how accurate and complete a given EHR is, this may only be limited to the specific subpopulation examined (i.e. those eligible for Medicaid, or those over 65 years for Medicare). One study examined congruence between EHR of a community health center and Medicaid claims with respect to diabetes [ 78 ]. They found that patients who were older, male, Spanish-speaking, above the federal poverty level, or who had discontinuous insurance were more likely to have services documented in the EHR as compared to Medicaid claims data. Therefore, while claims data may help supplement and validate information in the EHR, on their own they may underestimate care in certain populations.
Research utilizing EHR data has undoubtedly positively impacted the field of public health through its ability to provide large-scale, longitudinal data on a diverse set of patients, and will continue to do so in the future as more epidemiologists take advantage of this data source. EHR data’s ability to capture individuals that traditionally aren’t included in clinical trials, cohort studies, and even claims datasets allows researchers to measure longitudinal outcomes in patients and perhaps change the understanding of potential risk factors.
However, as outlined in this review, there are important caveats to EHR analysis that need to be taken into account; failure to do so may threaten study validity. The representativeness of EHR data depends on the catchment area of the center and corresponding target population. Tools are available to evaluate and remedy these issues, which are critical to study validity as well as extrapolation of study findings. Data availability and interpretation, missing measurements, and missing visits are also key challenges, as EHRs were not specifically developed for research purposes, despite their common use for such. Taking advantage of all available EHR data, whether it be structured or unstructured fields through NLP, will be important in understanding the patient experience and identifying key phenotypes. Beyond methods to address these concerns, it will remain crucial for epidemiologists and data analysts to engage with clinicians and informaticians at their institutions to ensure data quality and accessibility by forming multidisciplinary teams around specific research projects. Lastly, integration across multiple EHRs, or datasets that encompass multi-institutional EHR records, add an additional layer of data quality and validity issues, with the potential to exacerbate the above-stated challenges found within a single EHR. At minimum, such studies should account for correlated errors [ 79 , 80 ], and investigate whether modularization, or submechanisms that determine whether data are observed or missing in each EHR, exist [ 65 ].
The identified challenges may also apply to secondary analysis of other large healthcare databases, such as claims data, although it is important not to conflate the two types of data. EHR data are driven by clinical care and claims data are driven by the reimbursement process where there is a financial incentive to capture diagnoses, procedures, and medications [ 48 ]. The source of data likely influences the availability, accuracy, and completeness of data. The fundamental representation of data may also differ as a record in a claims database corresponds to a “claim” as opposed to an “encounter” in the EHR. As such, the representativeness of the database populations, the sensitivity and specificity of variables, as well as the mechanisms of missingness in claims data may differ from EHR data. One study that evaluated pediatric quality care measures, such as BMI, noted inferior sensitivity based on claims data alone [ 81 ]. Linking claims data to EHR data has been proposed to enhance study validity, but many of the caveats raised in herein still apply [ 82 ].
Although we focused on epidemiological challenges related to study validity, there are other important considerations for researchers working with EHR data. Privacy and security of data as well as institutional review board (IRB) or ethics board oversight of EHR-based studies should not be taken for granted. For researchers in the U.S., Goldstein and Sarwate described Health Insurance Portability and Accountability Act (HIPAA)-compliant approaches to ensure the privacy and security of EHR data used in epidemiological research, and presented emerging approaches to analyses that separate the data from analysis [ 83 ]. The IRB oversees the data collection process for EHR-based research and through the HIPAA Privacy Rule these data typically do not require informed consent provided they are retrospective and reside at the EHR’s institution [ 84 ]. Such research will also likely receive an exempt IRB review provided subjects are non-identifiable.
Conclusions
As EHRs are increasingly being used for research, epidemiologists can take advantage of the many tools and methods that already exist and apply them to the key challenges described above. By being aware of the limitations that the data present and proactively addressing them, EHR studies will be more robust, informative, and important to the understanding of health and disease in the population.
Availability of data and materials
All data and materials used in this review are described herein.
Abbreviations
Body Mass Index
Electronic Health Record
International Classification of Diseases
Institutional review board/ethics board
Health Insurance Portability and Accountability Act
Natural Language Processing
Social Determinants of Health
Socioeconomic Status
Adler-Milstein J, Holmgren AJ, Kralovec P, et al. Electronic health record adoption in US hospitals: the emergence of a digital “advanced use” divide. J Am Med Inform Assoc. 2017;24(6):1142–8.
Article PubMed PubMed Central Google Scholar
Office of the National Coordinator for Health Information Technology. ‘Office-based physician electronic health record adoption’, Health IT quick-stat #50. dashboard.healthit.gov/quickstats/pages/physician-ehr-adoption-trends.php . Accessed 15 Jan 2019.
Cowie MR, Blomster JI, Curtis LH, et al. Electronic health records to facilitate clinical research. Clin Res Cardiol. 2017;106(1):1–9.
Article PubMed Google Scholar
Casey JA, Schwartz BS, Stewart WF, et al. Using electronic health records for population health research: a review of methods and applications. Annu Rev Public Health. 2016;37:61–81.
Verheij RA, Curcin V, Delaney BC, et al. Possible sources of bias in primary care electronic health record data use and reuse. J Med Internet Res. 2018;20(5):e185.
Ni K, Chu H, Zeng L, et al. Barriers and facilitators to data quality of electronic health records used for clinical research in China: a qualitative study. BMJ Open. 2019;9(7):e029314.
Coleman N, Halas G, Peeler W, et al. From patient care to research: a validation study examining the factors contributing to data quality in a primary care electronic medical record database. BMC Fam Pract. 2015;16:11.
Kruse CS, Stein A, Thomas H, et al. The use of electronic health records to support population health: a systematic review of the literature. J Med Syst. 2018;42(11):214.
Shortreed SM, Cook AJ, Coley RY, et al. Challenges and opportunities for using big health care data to advance medical science and public health. Am J Epidemiol. 2019;188(5):851–61.
In: Smedley BD, Stith AY, Nelson AR, editors. Unequal treatment: confronting racial and ethnic disparities in health care. Washington (DC) 2003.
Chaudhry B, Wang J, Wu S, et al. Systematic review: impact of health information technology on quality, efficiency, and costs of medical care. Ann Intern Med. 2006;144(10):742–52.
Cutler DM, Scott Morton F. Hospitals, market share, and consolidation. JAMA. 2013;310(18):1964–70.
Article CAS PubMed Google Scholar
Cocoros NM, Kirby C, Zambarano B, et al. RiskScape: a data visualization and aggregation platform for public health surveillance using routine electronic health record data. Am J Public Health. 2021;111(2):269–76.
Vader DT, Weldie C, Welles SL, et al. Hospital-acquired Clostridioides difficile infection among patients at an urban safety-net hospital in Philadelphia: demographics, neighborhood deprivation, and the transferability of national statistics. Infect Control Hosp Epidemiol. 2020;42:1–7.
Google Scholar
Dixon BE, Gibson PJ, Frederickson Comer K, et al. Measuring population health using electronic health records: exploring biases and representativeness in a community health information exchange. Stud Health Technol Inform. 2015;216:1009.
PubMed Google Scholar
Hernán MA, VanderWeele TJ. Compound treatments and transportability of causal inference. Epidemiology. 2011;22(3):368–77.
Casey JA, Pollak J, Glymour MM, et al. Measures of SES for electronic health record-based research. Am J Prev Med. 2018;54(3):430–9.
Polubriaginof FCG, Ryan P, Salmasian H, et al. Challenges with quality of race and ethnicity data in observational databases. J Am Med Inform Assoc. 2019;26(8-9):730–6.
U.S. Census Bureau. Health. Available at: https://www.census.gov/topics/health.html . Accessed 19 Jan 2021.
Gianfrancesco MA, McCulloch CE, Trupin L, et al. Reweighting to address nonparticipation and missing data bias in a longitudinal electronic health record study. Ann Epidemiol. 2020;50:48–51 e2.
Goldstein ND, Kahal D, Testa K, Burstyn I. Inverse probability weighting for selection bias in a Delaware community health center electronic medical record study of community deprivation and hepatitis C prevalence. Ann Epidemiol. 2021;60:1–7.
Gelman A, Lax J, Phillips J, et al. Using multilevel regression and poststratification to estimate dynamic public opinion. Unpublished manuscript, Columbia University. 2016 Sep 11. Available at: http://www.stat.columbia.edu/~gelman/research/unpublished/MRT(1).pdf . Accessed 22 Jan 2021.
Quick H, Terloyeva D, Wu Y, et al. Trends in tract-level prevalence of obesity in philadelphia by race-ethnicity, space, and time. Epidemiology. 2020;31(1):15–21.
Lesko CR, Buchanan AL, Westreich D, Edwards JK, Hudgens MG, Cole SR. Generalizing study results: a potential outcomes perspective. Epidemiology. 2017;28(4):553–61.
Westreich D, Edwards JK, Lesko CR, Stuart E, Cole SR. Transportability of trial results using inverse odds of sampling weights. Am J Epidemiol. 2017;186(8):1010–4.
Congressional Research Services (CRS). The Health Information Technology for Economic and Clinical Health (HITECH) Act. 2009. Available at: https://crsreports.congress.gov/product/pdf/R/R40161/9 . Accessed Jan 22 2021.
Hersh WR. The electronic medical record: Promises and problems. Journal of the American Society for Information Science. 1995;46(10):772–6.
Article Google Scholar
Collecting sexual orientation and gender identity data in electronic health records: workshop summary. Washington (DC) 2013.
Committee on the Recommended Social and Behavioral Domains and Measures for Electronic Health Records; Board on Population Health and Public Health Practice; Institute of Medicine. Capturing social and behavioral domains and measures in electronic health records: phase 2. Washington (DC): National Academies Press (US); 2015.
Goff SL, Pekow PS, Markenson G, et al. Validity of using ICD-9-CM codes to identify selected categories of obstetric complications, procedures and co-morbidities. Paediatr Perinat Epidemiol. 2012;26(5):421–9.
Schneeweiss S, Avorn J. A review of uses of health care utilization databases for epidemiologic research on therapeutics. J Clin Epidemiol. 2005;58(4):323–37.
Gianfrancesco MA. Application of text mining methods to identify lupus nephritis from electronic health records. Lupus Science & Medicine. 2019;6:A142.
National Library of Medicine. SNOMED CT to ICD-10-CM Map. Available at: https://www.nlm.nih.gov/research/umls/mapping_projects/snomedct_to_icd10cm.html . Accessed 2 Jul 2021.
Klabunde CN, Harlan LC, Warren JL. Data sources for measuring comorbidity: a comparison of hospital records and medicare claims for cancer patients. Med Care. 2006;44(10):921–8.
Burles K, Innes G, Senior K, Lang E, McRae A. Limitations of pulmonary embolism ICD-10 codes in emergency department administrative data: let the buyer beware. BMC Med Res Methodol. 2017;17(1):89.
Asgari MM, Wu JJ, Gelfand JM, Salman C, Curtis JR, Harrold LR, et al. Validity of diagnostic codes and prevalence of psoriasis and psoriatic arthritis in a managed care population, 1996-2009. Pharmacoepidemiol Drug Saf. 2013;22(8):842–9.
Hoffman S, Podgurski A. Big bad data: law, public health, and biomedical databases. J Law Med Ethics. 2013;41(Suppl 1):56–60.
Adler-Milstein J, Jha AK. Electronic health records: the authors reply. Health Aff. 2014;33(10):1877.
Geruso M, Layton T. Upcoding: evidence from medicare on squishy risk adjustment. J Polit Econ. 2020;12(3):984–1026.
Lash TL, Fox MP, Fink AK. Applying quantitative bias analysis to epidemiologic data. New York: Springer-Verlag New York; 2009.
Book Google Scholar
Gustafson P. Measurement error and misclassification in statistics and epidemiology: impacts and Bayesian adjustments. Boca Raton: Chapman and Hall/CRC; 2004.
Duda SN, Shepherd BE, Gadd CS, et al. Measuring the quality of observational study data in an international HIV research network. PLoS One. 2012;7(4):e33908.
Article CAS PubMed PubMed Central Google Scholar
Shepherd BE, Yu C. Accounting for data errors discovered from an audit in multiple linear regression. Biometrics. 2011;67(3):1083–91.
Weiskopf NG, Hripcsak G, Swaminathan S, et al. Defining and measuring completeness of electronic health records for secondary use. J Biomed Inform. 2013;46(5):830–6.
Kaiser Health News. As coronavirus strikes, crucial data in electronic health records hard to harvest. Available at: https://khn.org/news/as-coronavirus-strikes-crucial-data-in-electronic-health-records-hard-to-harvest/ . Accessed 15 Jan 2021.
Reeves JJ, Hollandsworth HM, Torriani FJ, Taplitz R, Abeles S, Tai-Seale M, et al. Rapid response to COVID-19: health informatics support for outbreak management in an academic health system. J Am Med Inform Assoc. 2020;27(6):853–9.
Grange ES, Neil EJ, Stoffel M, Singh AP, Tseng E, Resco-Summers K, et al. Responding to COVID-19: The UW medicine information technology services experience. Appl Clin Inform. 2020;11(2):265–75.
Madigan D, Ryan PB, Schuemie M, et al. Evaluating the impact of database heterogeneity on observational study results. Am J Epidemiol. 2013;178(4):645–51.
Lippi G, Mattiuzzi C. Critical laboratory values communication: summary recommendations from available guidelines. Ann Transl Med. 2016;4(20):400.
Charlson ME, Pompei P, Ales KL, MacKenzie CR. A new method of classifying prognostic comorbidity in longitudinal studies: development and validation. J Chronic Dis. 1987;40(5):373–83.
Jones RN. Differential item functioning and its relevance to epidemiology. Curr Epidemiol Rep. 2019;6:174–83.
Edwards JK, Cole SR, Troester MA, Richardson DB. Accounting for misclassified outcomes in binary regression models using multiple imputation with internal validation data. Am J Epidemiol. 2013;177(9):904–12.
Satkunasivam R, Klaassen Z, Ravi B, Fok KH, Menser T, Kash B, et al. Relation between surgeon age and postoperative outcomes: a population-based cohort study. CMAJ. 2020;192(15):E385–92.
Melamed N, Asztalos E, Murphy K, Zaltz A, Redelmeier D, Shah BR, et al. Neurodevelopmental disorders among term infants exposed to antenatal corticosteroids during pregnancy: a population-based study. BMJ Open. 2019;9(9):e031197.
Kao LT, Lee HC, Lin HC, Tsai MC, Chung SD. Healthcare service utilization by patients with obstructive sleep apnea: a population-based study. PLoS One. 2015;10(9):e0137459.
Article PubMed PubMed Central CAS Google Scholar
Jung K, LePendu P, Iyer S, Bauer-Mehren A, Percha B, Shah NH. Functional evaluation of out-of-the-box text-mining tools for data-mining tasks. J Am Med Inform Assoc. 2015;22(1):121–31.
Canan C, Polinski JM, Alexander GC, et al. Automatable algorithms to identify nonmedical opioid use using electronic data: a systematic review. J Am Med Inform Assoc. 2017;24(6):1204–10.
Iqbal E, Mallah R, Jackson RG, et al. Identification of adverse drug events from free text electronic patient records and information in a large mental health case register. PLoS One. 2015;10(8):e0134208.
Rochefort CM, Verma AD, Eguale T, et al. A novel method of adverse event detection can accurately identify venous thromboembolisms (VTEs) from narrative electronic health record data. J Am Med Inform Assoc. 2015;22(1):155–65.
Koleck TA, Dreisbach C, Bourne PE, et al. Natural language processing of symptoms documented in free-text narratives of electronic health records: a systematic review. J Am Med Inform Assoc. 2019;26(4):364–79.
Wang L, Luo L, Wang Y, et al. Natural language processing for populating lung cancer clinical research data. BMC Med Inform Decis Mak. 2019;19(Suppl 5):239.
Banerji A, Lai KH, Li Y, et al. Natural language processing combined with ICD-9-CM codes as a novel method to study the epidemiology of allergic drug reactions. J Allergy Clin Immunol Pract. 2020;8(3):1032–1038.e1.
Zhang D, Yin C, Zeng J, et al. Combining structured and unstructured data for predictive models: a deep learning approach. BMC Med Inform Decis Mak. 2020;20(1):280.
Farmer R, Mathur R, Bhaskaran K, Eastwood SV, Chaturvedi N, Smeeth L. Promises and pitfalls of electronic health record analysis. Diabetologia. 2018;61:1241–8.
Haneuse S, Arterburn D, Daniels MJ. Assessing missing data assumptions in EHR-based studies: a complex and underappreciated task. JAMA Netw Open. 2021;4(2):e210184.
Groenwold RHH. Informative missingness in electronic health record systems: the curse of knowing. Diagn Progn Res. 2020;4:8.
Berkowitz SA, Traore CY, Singer DE, et al. Evaluating area-based socioeconomic status indicators for monitoring disparities within health care systems: results from a primary care network. Health Serv Res. 2015;50(2):398–417.
Kind AJH, Buckingham WR. Making neighborhood-disadvantage metrics accessible - the neighborhood atlas. N Engl J Med. 2018;378(26):2456–8.
Cantor MN, Thorpe L. Integrating data on social determinants of health into electronic health records. Health Aff. 2018;37(4):585–90.
Adler NE, Stead WW. Patients in context--EHR capture of social and behavioral determinants of health. N Engl J Med. 2015;372(8):698–701.
Chen M, Tan X, Padman R. Social determinants of health in electronic health records and their impact on analysis and risk prediction: a systematic review. J Am Med Inform Assoc. 2020;27(11):1764–73.
Goldstein BA, Bhavsar NA, Phelan M, et al. Controlling for informed presence bias due to the number of health encounters in an electronic health record. Am J Epidemiol. 2016;184(11):847–55.
Petersen I, Welch CA, Nazareth I, et al. Health indicator recording in UK primary care electronic health records: key implications for handling missing data. Clin Epidemiol. 2019;11:157–67.
Li R, Chen Y, Moore JH. Integration of genetic and clinical information to improve imputation of data missing from electronic health records. J Am Med Inform Assoc. 2019;26(10):1056–63.
Koonin LM, Hoots B, Tsang CA, Leroy Z, Farris K, Jolly T, et al. Trends in the use of telehealth during the emergence of the COVID-19 pandemic - United States, January-March 2020. MMWR Morb Mortal Wkly Rep. 2020;69(43):1595–9.
Barnett ML, Ray KN, Souza J, Mehrotra A. Trends in telemedicine use in a large commercially insured population, 2005-2017. JAMA. 2018;320(20):2147–9.
Franklin JM, Gopalakrishnan C, Krumme AA, et al. The relative benefits of claims and electronic health record data for predicting medication adherence trajectory. Am Heart J. 2018;197:153–62.
Devoe JE, Gold R, McIntire P, et al. Electronic health records vs Medicaid claims: completeness of diabetes preventive care data in community health centers. Ann Fam Med. 2011;9(4):351–8.
Schmajuk G, Li J, Evans M, Anastasiou C, Izadi Z, Kay JL, et al. RISE registry reveals potential gaps in medication safety for new users of biologics and targeted synthetic DMARDs. Semin Arthritis Rheum. 2020 Dec;50(6):1542–8.
Izadi Z, Schmajuk G, Gianfrancesco M, Subash M, Evans M, Trupin L, et al. Rheumatology Informatics System for Effectiveness (RISE) practices see significant gains in rheumatoid arthritis quality measures. Arthritis Care Res. 2020. https://doi.org/10.1002/acr.24444 .
Angier H, Gold R, Gallia C, Casciato A, Tillotson CJ, Marino M, et al. Variation in outcomes of quality measurement by data source. Pediatrics. 2014;133(6):e1676–82.
Lin KJ, Schneeweiss S. Considerations for the analysis of longitudinal electronic health records linked to claims data to study the effectiveness and safety of drugs. Clin Pharmacol Ther. 2016;100(2):147–59.
Goldstein ND, Sarwate AD. Privacy, security, and the public health researcher in the era of electronic health record research. Online J Public Health Inform. 2016;8(3):e207.
U.S. Department of Health and Human Services (HHS). 45 CFR 46. http://www.hhs.gov/ohrp/regulations-and-policy/regulations/45-cfr-46/index.html .
Download references
Acknowledgements
The authors thank Dr. Annemarie Hirsch, Department of Population Health Sciences, Geisinger, for assistance in conceptualizing an earlier version of this work.
Research reported in this publication was supported in part by the National Institute of Arthritis and Musculoskeletal and Skin Diseases of the National Institutes of Health under Award Number K01AR075085 (to MAG) and the National Institute Of Allergy And Infectious Diseases of the National Institutes of Health under Award Number K01AI143356 (to NDG). The content is solely the responsibility of the authors and does not necessarily represent the official views of the National Institutes of Health.
Author information
Authors and affiliations.
Division of Rheumatology, University of California School of Medicine, San Francisco, CA, USA
Milena A. Gianfrancesco
Department of Epidemiology and Biostatistics, Drexel University Dornsife School of Public Health, 3215 Market St., Philadelphia, PA, 19104, USA
Neal D. Goldstein
You can also search for this author in PubMed Google Scholar
Contributions
Both authors conceptualized, wrote, and approved the final submitted version.
Corresponding author
Correspondence to Neal D. Goldstein .
Ethics declarations
Ethics approval and consent to participate.
Not applicable
Consent for publication
Competing interests.
The authors have no competing interests to declare
Additional information
Publisher’s note.
Springer Nature remains neutral with regard to jurisdictional claims in published maps and institutional affiliations.
Rights and permissions
Open Access This article is licensed under a Creative Commons Attribution 4.0 International License, which permits use, sharing, adaptation, distribution and reproduction in any medium or format, as long as you give appropriate credit to the original author(s) and the source, provide a link to the Creative Commons licence, and indicate if changes were made. The images or other third party material in this article are included in the article's Creative Commons licence, unless indicated otherwise in a credit line to the material. If material is not included in the article's Creative Commons licence and your intended use is not permitted by statutory regulation or exceeds the permitted use, you will need to obtain permission directly from the copyright holder. To view a copy of this licence, visit http://creativecommons.org/licenses/by/4.0/ . The Creative Commons Public Domain Dedication waiver ( http://creativecommons.org/publicdomain/zero/1.0/ ) applies to the data made available in this article, unless otherwise stated in a credit line to the data.
Reprints and permissions
About this article
Cite this article.
Gianfrancesco, M.A., Goldstein, N.D. A narrative review on the validity of electronic health record-based research in epidemiology. BMC Med Res Methodol 21 , 234 (2021). https://doi.org/10.1186/s12874-021-01416-5
Download citation
Received : 02 July 2021
Accepted : 28 September 2021
Published : 27 October 2021
DOI : https://doi.org/10.1186/s12874-021-01416-5
Share this article
Anyone you share the following link with will be able to read this content:
Sorry, a shareable link is not currently available for this article.
Provided by the Springer Nature SharedIt content-sharing initiative
- Electronic health records
- Data quality
- Secondary analysis
BMC Medical Research Methodology
ISSN: 1471-2288
- General enquiries: [email protected]
Thank you for visiting nature.com. You are using a browser version with limited support for CSS. To obtain the best experience, we recommend you use a more up to date browser (or turn off compatibility mode in Internet Explorer). In the meantime, to ensure continued support, we are displaying the site without styles and JavaScript.
- View all journals
- Explore content
- About the journal
- Publish with us
- Sign up for alerts
- 25 September 2019
The future of electronic health records
- Jeff Hecht 0
Jeff Hecht is a science writer based in Newton, Massachusetts.
You can also search for this author in PubMed Google Scholar
Credit: Totto Renna
Advances in medical imaging and the proliferation of diagnostic and screening tests have generated mountains of data on patient health. Digital information technology has seemed poised to revolutionize health care in the United States since 2009, when the Obama administration made the technology part of plans to revive a sinking economy. The US government has now spent tens of billions of dollars on putting patient information at doctors’ fingertips.
Access options
Access Nature and 54 other Nature Portfolio journals
Get Nature+, our best-value online-access subscription
24,99 € / 30 days
cancel any time
Subscribe to this journal
Receive 51 print issues and online access
185,98 € per year
only 3,65 € per issue
Rent or buy this article
Prices vary by article type
Prices may be subject to local taxes which are calculated during checkout
Nature 573 , S114-S116 (2019)
doi: https://doi.org/10.1038/d41586-019-02876-y
This article is part of Nature Outlook: Digital health , an editorially independent supplement produced with the financial support of third parties. About this content .
Dinov, I, D. J. Med. Stat. Inform. https://dx.doi.org/10.7243/2053-7662-4-3 (2016).
PubMed Google Scholar
Liberatore, K. Pa. Patient Saf. Advis. 15 (Suppl.), 16–24 (2018).
Google Scholar
Arndt, B. G. et al. Ann. Fam. Med. 15 , 419–426 (2017).
Article PubMed Google Scholar
Shanafelt, T. D. et al. Mayo Clin. Proc. 90 , 1600–1613 (2015).
Download references
Related Articles
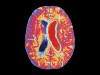
- Information technology
- Health care
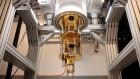
Quantum hacking looms — but ultra-secure encryption is ready to deploy
News 13 AUG 24
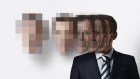
AI ‘deepfake’ faces detected using astronomy methods
News 22 JUL 24
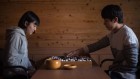
Can AI be superhuman? Flaws in top gaming bot cast doubt
News 08 JUL 24
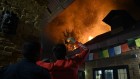
Wildfires are raging in Nepal — climate change isn’t the only culprit
News 14 JUN 24
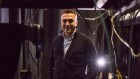
AI’s keen diagnostic eye
Outlook 18 APR 24
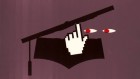
So … you’ve been hacked
Technology Feature 19 MAR 24
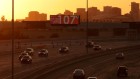
Extreme heat is a huge killer — these local approaches can keep people safe
News 22 AUG 24
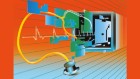
The testing of AI in medicine is a mess. Here’s how it should be done
News Feature 21 AUG 24
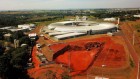
First biolab in South America for studying world’s deadliest viruses is set to open
News 21 AUG 24
Postdoctoral Fellow – Cancer Immunotherapy
Tampa, Florida
H. Lee Moffitt Cancer Center & Research Institute
Postdoctoral Associate - Specialist
Houston, Texas (US)
Baylor College of Medicine (BCM)
Postdoctoral Associate- CAR T Cells, Synthetic Biology
Postdoctoral scholar.
Dr. Glen N. Barber in the OSUCCC-PIIO is looking to hire enthusiastic, self-motivated Postdoctoral Scholars to contribute to cutting-edge research.
Columbus, Ohio
Polaris Recruitment
High-Level Talents at the First Affiliated Hospital of Nanchang University
For clinical medicine and basic medicine; basic research of emerging inter-disciplines and medical big data.
Nanchang, Jiangxi, China
The First Affiliated Hospital of Nanchang University
Sign up for the Nature Briefing newsletter — what matters in science, free to your inbox daily.
Quick links
- Explore articles by subject
- Guide to authors
- Editorial policies
- Research article
- Open access
- Published: 04 September 2014
Implementing electronic health records in hospitals: a systematic literature review
- Albert Boonstra 1 ,
- Arie Versluis 2 &
- Janita F J Vos 1
BMC Health Services Research volume 14 , Article number: 370 ( 2014 ) Cite this article
97k Accesses
166 Citations
57 Altmetric
Metrics details
The literature on implementing Electronic Health Records (EHR) in hospitals is very diverse. The objective of this study is to create an overview of the existing literature on EHR implementation in hospitals and to identify generally applicable findings and lessons for implementers.
A systematic literature review of empirical research on EHR implementation was conducted. Databases used included Web of Knowledge, EBSCO, and Cochrane Library. Relevant references in the selected articles were also analyzed. Search terms included Electronic Health Record (and synonyms), implementation, and hospital (and synonyms). Articles had to meet the following requirements: (1) written in English, (2) full text available online, (3) based on primary empirical data, (4) focused on hospital-wide EHR implementation, and (5) satisfying established quality criteria.
Of the 364 initially identified articles, this study analyzes the 21 articles that met the requirements. From these articles, 19 interventions were identified that are generally applicable and these were placed in a framework consisting of the following three interacting dimensions: (1) EHR context, (2) EHR content, and (3) EHR implementation process.
Conclusions
Although EHR systems are anticipated as having positive effects on the performance of hospitals, their implementation is a complex undertaking. This systematic review reveals reasons for this complexity and presents a framework of 19 interventions that can help overcome typical problems in EHR implementation. This framework can function as a reference for implementers in developing effective EHR implementation strategies for hospitals.
Peer Review reports
In recent years, Electronic Health Records (EHRs) have been implemented by an ever increasing number of hospitals around the world. There have, for example, been initiatives, often driven by government regulations or financial stimulations, in the USA [ 1 ], the United Kingdom [ 2 ] and Denmark [ 3 ]. EHR implementation initiatives tend to be driven by the promise of enhanced integration and availability of patient data [ 4 ], by the need to improve efficiency and cost-effectiveness [ 5 ], by a changing doctor-patient relationship toward one where care is shared by a team of health care professionals [ 5 ], and/or by the need to deal with a more complex and rapidly changing environment [ 6 ].
EHR systems have various forms, and the term can relate to a broad range of electronic information systems used in health care. EHR systems can be used in individual organizations, as interoperating systems in affiliated health care units, on a regional level, or nationwide [ 1 , 2 ]. Health care units that use EHRs include hospitals, pharmacies, general practitioner surgeries, and other health care providers [ 7 ].
The implementation of hospital-wide EHR systems is a complex matter involving a range of organizational and technical factors including human skills, organizational structure, culture, technical infrastructure, financial resources, and coordination [ 8 , 9 ]. As Grimson et al. [ 5 ] argue, implementing information systems (IS) in hospitals is more challenging than elsewhere because of the complexity of medical data, data entry problems, security and confidentiality concerns, and a general lack of awareness of the benefits of Information Technology (IT). Boonstra and Govers [ 10 ] provide three reasons why hospitals differ from many other industries, and these differences might also affect EHR implementations. The first reason is that hospitals have multiple objectives, such as curing and caring for patients, and educating new physicians and nurses. Second, hospitals have complicated and highly varied structures and processes. Third, hospitals have a varied workforce including medical professionals who possess high levels of expertise, power, and autonomy. These distinct characteristics justify a study that focuses on the identification and analysis of the findings of previous studies on EHR implementation in hospitals.
Study aim, theoretical framework, and terminology
In dealing with the complexity of EHR implementation in hospitals, it is helpful to know which factors are seen as important in the literature and to capture the existing knowledge on EHR implementation in hospitals. As such, the objective of this research is to identify, categorize, and analyze the existing findings in the literature on EHR implementation processes in hospitals. This could contribute to greater insight into the underlying patterns and complex relationships involved in EHR implementation and could identify ways to tackle EHR implementation problems. In other words, this study focusses on the identification of factors that determine the progress of EHR implementation in hospitals. The motives behind implementing EHRs in hospitals and the effects on performance of implemented EHR systems are beyond the scope of this paper.
To our knowledge, there have been no systematic reviews of the literature concerning EHR implementation in hospitals and this article therefore fills that gap. Two interesting related review studies on EHR implementation are Keshavjee et al. [ 11 ] and McGinn et al. [ 12 ]. The study of Keshavjee et al. [ 11 ] develops a literature based integrative framework for EHR implementation. McGinn et al. [ 12 ] adopt an exclusive user perspective on EHR and their study is limited to Canada and countries with comparable socio-economic levels. Both studies are not explicitly focused on hospitals and include other contexts such as small clinics and national or regional EHR initiatives.
This systematic review is explicitly focused on hospital-wide, single hospital EHR implementations and identifies empirical studies (that include collected primary data) that reflect this situation. The categorization of the findings from the selected articles draws on Pettigrew’s framework for understanding strategic change [ 13 ]. This model has been widely applied in case study research into organizational contexts [ 14 ], as well as in studies on the implementation of health care innovations [ 15 ]. It generates insights by analyzing three interactive dimensions – context , content , and process – that together shape organizational change. Pettigrew’s framework [ 13 ] is seen as applicable because implementing an EHR artefact is an organization-wide effort. This framework was specifically selected for its focus on organizational change, its ease of understanding, and its relatively general dimensions allowing a broad range of findings to be included. The framework structures and focusses the analysis of the findings from the selected articles.
An organization’s context can be divided into internal and external components. External context refers to the social, economic, political, and competitive environments in which an organization operates. The internal context refers to the structure, culture, resources, capabilities, and politics of an organization. The content covers the specific areas of the transformation under examination. In an EHR implementation, these are the EHR system itself (both hardware and software), the work processes, and everything related to these (e.g. social conditions). The process dimension concerns the processes of change, made up of the plans, actions, reactions, and interactions of the stakeholders, rather than work processes in general. It is important to note that Pettigrew [ 13 ] does not see strategic change as a rational analytical process but rather as an iterative, continuous, multilevel process. This highlights that the outcome of an organizational change will be determined by the context, content, and process of that change. The framework with its three categories, shown in Figure 1 , illustrates the conceptual model used to categorize the findings of this systematic literature review.
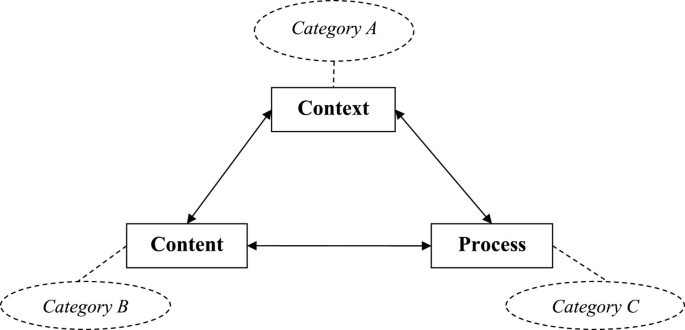
Pettigrew ’ s framework [ 13 ] ] and the corresponding categories.
In the literature, several terms are used to refer to electronic medical information systems. In this article, the term Electronic Health Record (EHR) is used throughout. Commonly used terms identified by ISO (the International Organization for Standardization) [ 16 ] plus another not identified by ISO are outlined below and used in our search. ISO considers Electronic Health Record (EHR) to be an overall term for “ a repository of information regarding the health status of a subject of care , in computer processable form ” [ 16 ], p. 13. ISO uses different terms to describe various types of EHRs. These include Electronic Medical Record (EMR), which is similar to an EHR but restricted to the medical domain. The terms Electronic Patient Record (EPR) and Computerized Patient Record (CPR) are also identified. Häyrinen et al. [ 17 ] view both terms as having the same meaning and referring to a system that contains clinical information from a particular hospital. Another term seen is Electronic Healthcare Record (EHCR) which refers to a system that contains all the available health information on a patient [ 17 ] and can thus be seen as synonymous with EHR [ 16 ]. A term often found in the literature is Computerized Physician Order Entry (CPOE). Although this term is not mentioned by ISO [ 16 ] or by Häyrinen et al. [ 17 ], we included CPOE for three reasons. First, it is considered by many to be a key hospital-wide function of an EHR system e.g. [ 8 , 18 ]. Second, from a preliminary analysis of our initial results, we found that, from the perspective of the implementation process, comparable issues and factors emerged from both CPOEs and EHRs. Third, the implementation of a comprehensive electronic medical record requires physicians to make direct order entries [ 19 ]. Kaushal et al. define a CPOE as “ a variety of computer - based systems that share the common features of automating the medication ordering process and that ensure standardized , legible , and complete orders ” [ 18 ], p. 1410. Other terms found in the literature were not included in this review as they were considered either irrelevant or too broadly defined. Examples of such terms are Electronic Client Record (ECR), Personal Health Record (PHR), Digital Medical Record (DMR), Health Information Technology (HIT), and Clinical Information System (CIS).
Search strategies
In order for a systematic literature review to be comprehensive, it is essential that all terms relevant to the aim of the research are covered in the search. Further, we need to include relevant synonyms and related terms, both for electronic medical information systems and for hospitals. By adding an * to the end of a term, the search engines pick out other forms, and by adding “ “ around words one ensures that only the complete term is searched for. Further, by including a ? as a wildcard character, every possible combination is included in the search.
The search used three categories of keywords. The first category included the following terms as approximate synonyms for hospital: “hospital*”, “healthcare”, and “clinic*”. The second category concerned implementation and included the term “implement*”. For the third category, electronic medical information systems, the following search terms were used: “Electronic Health Record*”, “Electronic Patient Record*”, “Electronic Medical Record*”, “Computeri?ed Patient Record*”, “Electronic Healthcare Record*”, “Computeri?ed Physician Order Entry”.
This relatively large set of keywords was necessary to ensure that articles were not missed in the search, and required a large number of search strategies to cover all those keywords. As we were seeking papers about the implementation of electronic medical information systems in hospitals , the search strategies included the terms shown in Table 1 .
The following three search engines were chosen based on their relevance to the field and their accessibility by the researcher: Web of knowledge, EBSCO, and The Cochrane Library. Most search engines use several databases but not all of them were relevant for this research as they serve a wide range of fields. Appendix A provides an overview of the databases used. The reference lists included in articles that met the selection criteria were checked for other possibly relevant studies that had not been identified in the database search.
The articles identified from the various search strategies had to be academic peer-reviewed articles if they were to be included in our review. Further, they were assessed and had to satisfy the following criteria to be included: (1) written in English, (2) full text available online, (3) based on primary empirical data, (4) focused on hospital-wide EHR implementation, and (5) meeting established quality criteria. A long list of abstracts was generated, and all of them were independently reviewed by two of the authors. They independently reviewed the abstracts, eliminated duplicates and shortlisted abstracts for detailed review. When opinions differed, a final decision over inclusion was made following a discussion between the researchers.
Data analysis
The quality of the articles that survived this filtering was assessed by the first two authors using the Standard Quality Assessment Criteria for Evaluating Primary Research Papers [ 18 ]. In other words, the quality of the articles was jointly assessed by evaluating whether specific criteria had been addressed, resulting in a rating of 2 (fully addressed), 1 (partly addressed), or 0 (not addressed) for each criteria. Different questions are posed for qualitative and quantitative research and, in the event of a mixed-method study, both questionnaires were used. Papers were included if they received at least half of the total possible points, admittedly a relatively liberal cut-off point given comments in the Standard Quality Assessment Criteria for Evaluating Primary Research Papers [ 20 ].
The next step was to extract the findings of the reviewed articles and to analyze these with the aim of reaching general findings on the implementation of EHR systems in hospitals. Categorizing these general findings can increase clarity. The earlier introduced conceptual model, based on Pettigrew’s framework for understanding strategic change, includes three categories: context (A), content (B), and process (C). As our review is specifically aimed at identifying findings related to the implementation process, possible motives for introducing such a system, as well as its effects and outcomes, are outside its scope. The authors held frequent discussions between themselves to discuss the meaning and the categorization of the general findings.
Paper selection
Applying the 18 search strategies listed in Table 1 with the various search engines resulted in 364 articles being identified. The searches were carried out on 12 March 2013 for search strategies 1–15 and on 18 April 2013 for search strategies 16–18. The latter three strategies were added following a preliminary analysis of the first set of results which highlighted several other terms and descriptions for information technology in health care. Not surprisingly, many duplicates were included in the 364 articles, both within and between search engines. Using the Refworks functions for identifying exact and close duplicates, 160 duplicates were found. However, this procedure did not identify all the duplicates present and the second author carried out a manual check that identified an additional 23 duplicates. When removing duplicates, we retained the link to the first search engine that identified the article and, as the Web of Knowledge was the first search engine used, most articles appear to have stemmed from this search engine. This left 181 different articles which were screened on title and abstract to check whether they met the selection criteria. When this was uncertain, the contents of the paper were further investigated. This screening resulted in just 13 articles that met all the selection criteria. We then performed two additional checks for completeness. First, checking the references of these articles identified another nine articles. Second, as suggested by the referees of this paper, we also used the term “introduc*” instead of “implement*”, together with the other two original categories of terms, and the term “provider” instead of “physician”, as part of CPOE. Each of these two searches identified one additional article (see Table 1 ). Of these resulting 24 articles, two proved to be almost identical so one was excluded, resulting in 23 articles for a final quality assessment.The results of the quality assessment can be found in Appendix B. The results show that two articles failed to meet the quality threshold and so 21 articles remained for in-depth analysis. Figure 2 displays the steps taken in this selection procedure.
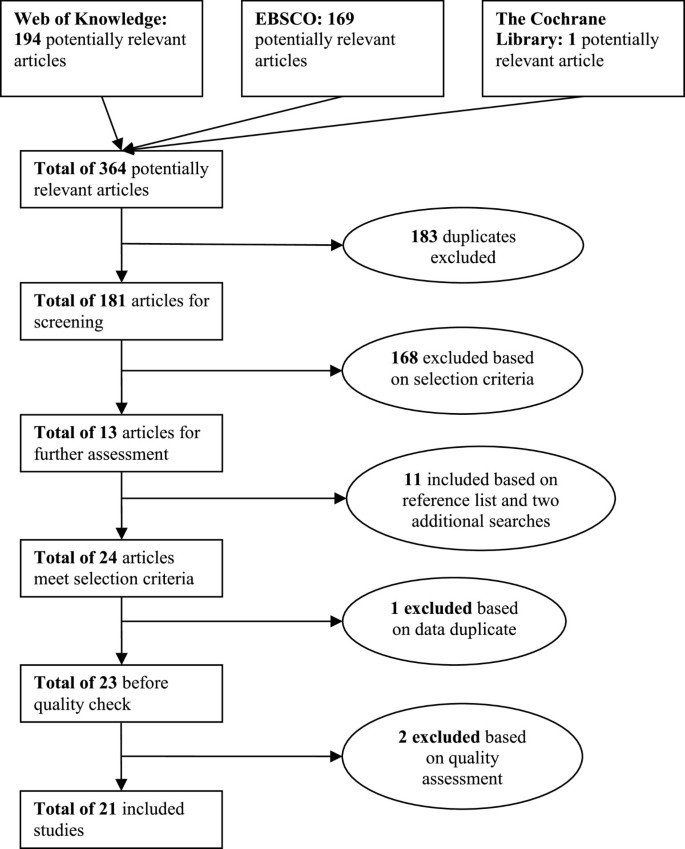
Selection procedure.
To provide greater insight into the context and nature of the 21 remaining articles, an overview is provided in Table 2 . All the studies except one were published after 2000. This reflects the recent increase in effort to implement organization-wide information systems, such as EHR systems, and also increasing incentives from governments to make use of EHR systems in hospitals. Of the 21 studies, 14 can be classified as qualitative, 6 as quantitative, and 1 as a mixed-method study. Most studies were conducted in the USA, with eight in various European countries. Teaching and non-teaching hospitals are almost equally the subject of inquiry, and some researchers have focused on specific types of hospitals such as rural, critical access, or psychiatric hospitals. Ten of the articles were in journals with a five-year impact factor in the Journal Citation Reports 2011 database. There is a huge difference in the number of citations but one should never forget that newer studies have had fewer opportunities to be cited.
Theoretical perspectives of reviewed articles
In research, it is common to use theoretical frameworks when designing an academic study [ 41 ]. Theoretical frameworks provide a way of thinking about and looking at the subject matter and describe the underlying assumptions about the nature of the subject matter [ 42 ]. By building on existing theories, research becomes focused in aiming to enrich and extend the existing knowledge in that particular field [ 42 ]. To provide a more thorough understanding of the selected articles, their theoretical frameworks, if present, are outlined in Table 3 .
It is striking that no specific theoretical frameworks have been used in the research leading to 13 of the 21 selected articles. Most articles simply state their objective as gaining insight into certain aspects of EHR implementation (as shown in Table 1 ) and do not use a particular theoretical approach to identify and categorize findings. As such, these articles add knowledge to the field of EHR implementation but do not attempt to extend existing theories.
Aarts et al. [ 21 ] introduce the notion of the sociotechnical approach: emphasizing the importance of focusing both on the social aspects of an EHR implementation and on the technical aspects of the system. Using the concept of emergent change, they argue that an implementation process is far from linear and predictable due to the contingencies and the organizational complexity that influences the process. A sociotechnical approach and the concept of emergent change are also included in the theoretical framework of Takian et al. [ 37 ]. Aarts et al. [ 21 ] elaborate on the sociotechnical approach when stating that the fit between work processes and the information technology determines the success of the implementation. Aarts and Berg [ 22 ] introduce a model of success or failure in information system implementation. They see creating synergy among the medical work practices, the information system, and the hospital organization as necessary for implementation, and argue that this will only happen if sufficient people accept a change in work practices. Cresswell et al.’s study [ 26 ] is also influenced by sociotechnical principles and draws on Actor-Network Theory. Gastaldi et al. [ 28 ] perceive Electronic Health Records as knowledge management systems and question how such systems can be used to develop knowledge assets. Katsma et al. [ 31 ] focus on implementation success and elaborate on the notion that implementation success is determined by system quality and acceptance through participation. As such, they adopt more of a social view on implementation success rather than a sociotechnical approach. Rivard et al. [ 34 ] examine the difficulties in EHR implementation from a cultural perspective. They not only view culture as a set of assumptions shared by an entire collective (an integration perspective) but also expect subcultures to exist (a differentiation perspective), as well as individual assumptions not shared by a specific (sub-) group (fragmentation perspective). Ford et al. [ 27 ] focus on an entirely different topic and investigate the IT adoption strategies of hospitals using a framework that identifies three strategies. These are the single-vendor strategy (in which all IT is purchased from a single vendor), the best-of-breed strategy (integrating IT from multiple vendors), and the best-of-suit strategy (a hybrid approach using a focal system from one vendor as the basis plus other applications from other vendors).
To summarize, the articles by Aarts et al. [ 21 ], Aarts and Berg [ 22 ], Cresswell et al. [ 26 ], and Takian et al. [ 37 ] apply a sociotechnical framework to focus their research. Gastaldi et al. [ 28 ] see EHRs as a means to renew organizational capabilities. Katsma et al. [ 31 ] use a social framework by focusing on the relevance of an IT system as perceived by the user and the participation of users in the implementation process. Rivard et al. [ 34 ] analyze how organizational cultures can be receptive to EHR implementation. Ford et al. [ 27 ] look at adoption strategies, leading them to focus on the selection procedure for Electronic Health Records. The 13 other studies did not use an explicit theoretical lens in their research.
Implementation-related findings
The process of categorization started by assessing whether a specific finding from a study should be placed in Category A, B, or C. Thirty findings were placed in Category A (context), 31 in Category B (content), and 66 in Category C (process). Comparing and combining the specific findings resulted in several general findings within each category. The general findings are each given a code (category character plus number) and the related code is indicated alongside each specific finding in Appendix C. Findings that were only seen in one article, and thus were lacking support, were discarded.
Category A - context
The context category of an EHR implementation process includes both internal variables (such as resources, capabilities, culture, and politics) and external variables (such as economic, political, and social variables). Six general findings were identified, all but one related to internal variables. An overview of the findings and corresponding articles can be found in Table 4 . The lack of general findings related to external variables reflects our decision to exclude the underlying reasons (e.g. political or social pressures) for implementing an EHR system from this review. Similarly, internal findings related to aspects such as perceived financial benefits or improved quality of care, are outside our scope.
A1: Large (or system-affiliated), urban, not-for-profit, and teaching hospitals are more likely to have implemented an EHR system due to having greater financial capabilities, a greater change readiness, and less focus on profit
The research reviewed shows that larger or system-affiliated hospitals are more likely to have implemented an EHR system, and that this can be explained by their easier access to the large financial resources required. Larger hospitals have more financial resources than smaller hospitals [ 30 ] and system-affiliated hospitals can share costs [ 27 ]. Hospitals situated in urban areas more often have an EHR system than rural hospitals, which is attributed to less knowledge of EHR systems and less support from medical staff in rural hospitals [ 29 ]. The fact that not-for-profit hospitals more often have an EHR system fully implemented and teaching hospitals slightly more often than private hospitals is attributed to the latter’s more wait-and-see approach and the more progressive change-ready nature of public and teaching hospitals [ 27 , 32 ].
A2: EHR implementation requires the selection of a mature vendor who is committed to providing a system that fits the hospital’s specific needs
Although this finding is not a great surprise, it is relevant to discuss it further. A hospital selecting its own vendor can ensure that the system will match the specific needs of that hospital [ 32 ]. Further, it is important to deal with a vendor that has proven itself on the EHR market with mature and successful products. The vendor must also be able to identify hospital workflows and adapt its product accordingly, and be committed to a long-term trusting relationship with the hospital [ 33 ]. With this in mind, the initial price of the system should not be the overriding consideration: the organization should be willing to avoid purely cost-oriented vendors [ 28 ], as costs soon mount if problems arise.
A3: The presence of hospital staff with previous experience of health information technology increases the likelihood of EHR implementation as less uncertainty is experienced by the end-users
In order to be able to work with an EHR system, users must be capable of using information technology such as computers and have adequate typing skills [ 19 , 32 ]. Knowledge of, and previous experience with, EHR systems or other medical information systems reduces uncertainty and disturbance for users, and this results in a more positive attitude towards the system [ 29 , 32 , 37 , 38 ].
A4: An organizational culture that supports collaboration and teamwork fosters EHR implementation success because trust between employees is higher
The influence of organizational culture on the success of organizational change is addressed in almost all the popular approaches to change management, as well as in several of the articles in this literature review. Ash et al. [ 23 , 24 ] and Scott et al. [ 35 ] highlight that a strong culture with a history of collaboration, teamwork, and trust between different stakeholder groups minimizes resistance to change. Boyer et al. [ 25 ] suggest creating a favorable culture that is more adaptive to EHR implementation. However, creating a favorable culture is not necessarily easy: a comprehensive approach including incentives, resource allocation, and a responsible team was used in the example of Boyer et al. [ 25 ].
A5: EHR implementation is most likely in an organization with little bureaucracy and considerable flexibility as changes can be rapidly made
A highly bureaucratic organizational structure hampers change: it slows the process and often leads to inter-departmental conflict [ 19 ]. Specifically, appointing a multidisciplinary team to deal with EHR-related issues can prevent conflict and stimulate collaboration [ 25 ].
A6: EHR system implementation is difficult because cure and care activities must be ensured at all times
During the process of implementing an EHR system, it is of the utmost importance that all relevant information is always available [ 28 , 34 , 39 ]. Ensuring the continuity of quality care while implementing an EHR system is difficult and is an important distinction from many other IT implementations.
Category B - content
The content of the EHR implementation process consists of the EHR system and the corresponding objectives, assumptions, and complementary services. Table 5 lists the five extracted general findings. These focus on both the hardware and software of the EHR system, and its relation to work practices and privacy.
B1: Creating a fit by adapting both the technology and work practices is a key factor in the implementation of EHR
This finding elaborates on the sociotechnical approach identified in the earlier section on the theories adopted in the articles. Several authors [ 21 , 26 , 31 , 37 ] make clear that creating a fit between the EHR system and the existing work practices requires an initial acknowledgement that an EHR implementation is not just a technical project and that existing work practices will change due to the new system. By customizing and adapting the system to meet specific needs, users will become more open to using it [ 19 , 26 , 28 ].
B2: Hardware availability and system reliability, in terms of speed, availability, and a lack of failures, are necessary to ensure EHR use
In several articles, authors highlight the importance of having sufficient hardware. A system can only be used if it is available to the users, and a system will only be used if it works without problems. Ash et al. [ 24 ], Scott et al. [ 35 ], and Weir et al. [ 19 ] refer to the speed of the system as well as to the availability of a sufficient number of adequate terminals see also [ 40 ] in various locations. Systems must be logically structured [ 29 ], reliable [ 32 ], and provide safe information access [ 37 ]. Boyer et al. [ 25 ] also mention the importance of technical aspects but add that these are not sufficient for EHR implementation.
B3: To ensure EHR implementation, the software needs to be user-friendly with regard to ease of use, efficiency in use, and functionality
Some authors distinguish between technical availability and reliability, and the user-friendliness of the software [ 19 , 24 , 32 ]. They argue that it is not sufficient for a system to be available and reliable, it should also be easy and efficient in use, and provide the functionality required for medical staff to give good care. If a system fails to do this, staff will not use the system and will stick to their old ways of working.
B4: An EHR implementation should contain adequate safeguards for patient privacy and confidentiality
Concerns over privacy and confidentiality are recognized by Boyer et al. [ 25 ] and Houser and Johnson [ 29 ] and are considered as a barrier to EHR implementation. Yoon-Flannery et al. [ 40 ] and Takian et al. [ 37 ] also recognize the importance of patient privacy and the need to address this issue by providing training and creating adequate safeguards.
B5: EHR implementation requires a vendor who is willing to adapt its product to hospital work processes
A vendor must be responsive and enable the hospital to develop its product to ensure a good and usable EHR system [ 32 , 33 ]. By so doing, dependence on the vendor decreases and concerns that arise within the hospital can be addressed [ 32 ]. This finding is related to A2 in the sense that an experienced, cooperative, and flexible vendor is needed to deal with the range of interest groups found in hospitals.
Category C - process
This category refers to the actual process of implementing the EHR system. Variables considered are time, change approach, and change management. In our review, this category produced the largest number of general findings (see Table 6 ), as might be expected given our focus on the implementation process. EHR implementation often leads to anxiety, uncertainty, and concerns about a possible negative impact of the EHR on work processes and quality. The process findings, including leadership, resource availability, communication and participation are explicitly aimed at overcoming resistance to EHR implementation. These interventions help to create a positive atmosphere of goal directedness, co-creation and partnership.
C1: Due to their influential position, management’s active involvement and support is positively associated with EHR implementation, and also counterbalances the physicians’ medical dominance
Several authors note the important role that managers play in EHR implementation. Whereas some authors refer to supportive leadership [ 19 , 24 ], others emphasize that strong and active management involvement is needed [ 25 , 32 – 35 ]. Strong leadership is relevant as it effectively counterbalances the physicians’ medical dominance. For instance, Rivard et al. [ 34 ] observe that physicians’ medical dominance and the status and autonomy of other health professionals hinder collaboration and teamwork, and that this complicates EHR implementation. Poon et al. [ 33 ] acknowledge this aspect and argue for strong leadership in order to deal with the otherwise dominant physicians. They also claim that leaders have to set an example and use the system themselves. At the same time, it is motivating that the implementation is managed by leaders who are recognized by the medical staff, for instance by head nurses and physicians or by former physicians and nurses [ 25 , 33 ]. Ovretveit et al. [ 32 ] argue that it helps the implementation if senior management repeatedly declares the EHR implementation to be of the highest priority and supports this with sufficient financial and human resources. Poon et al. [ 33 ] add to this by highlighting that, especially during uncertainties and setbacks, the common vision that guides the EHR implementation has to be communicated to hospital staff. Sufficient human resources include the selection of competent and experienced project leaders who are familiar with EHR implementation. Scott et al. [ 35 ] identify leadership styles for different phases: participatory leadership is valued in selection decisions, whereas a more hierarchical leadership style is preferable in the actual implementation.
C2: Participation of clinical staff in the implementation process increases support for and acceptance of the EHR implementation
Participation of end-users (the clinical staff) generates commitment and enables problems to be quickly solved [ 25 , 26 , 36 ]. Especially because it is very unlikely that the system will be perfect for all, it is important that the clinical staff become the owner, rather than customers, of the system. Clinical staff should participate at all levels and in all steps [ 19 , 28 , 32 , 36 ] from initial system selection onwards [ 35 ]. Ovretveit et al. [ 32 ] propose that this involvement should have an extensive timeframe, starting in the early stages of implementation, when initial vendor requirements are formulated (‘consultation before implementation’), through to the beginning of the use phase. Creating multidisciplinary work groups which determine the content of the EHR and the rules regarding the sharing of information contributes to EHR acceptance [ 25 ] and ensures realistic approaches acceptable to the clinical staff [ 36 ].
C3: Training end-users and providing real-time support is important for EHR implementation success
Frequently, the end-users of a new EHR system lack experience with the specific EHR system or with EHR systems in general. Although it is increasingly hard to imagine society or workplaces without IT, a large specific system, such as an EHR, still requires considerable training on how to use it properly. The importance of training is often underestimated, and inadequate training will create a barrier to EHR use [ 19 , 29 ]. Consequently, adequate training, of appropriate quantity and quality, must be provided at the right times and locations [ 19 , 32 , 36 ]. Simon et al. [ 36 ] add to this the importance of real-time support, preferably provided by peers and super-users.
C4: A comprehensive implementation strategy, offering both clear guidance and room for emergent change, is needed for implementing an EHR system
Several articles highlight aspects of an EHR implementation strategy. A good strategy facilitates EHR implementation [ 19 , 25 ] and consists of careful planning and preparation [ 36 ], a sustainable business plan, effective communication [ 28 , 40 ] and mandatory implementation [ 19 ]. Emergent change is perceived as a key characteristic of EHR implementation in complex organizations such as hospitals [ 21 ], and this suggests an implementation approach based on a development paradigm [ 31 ], which may initially even involve parallel use of paper [ 26 ]. The notion of emergent change has been variously applied, including in the theoretical frameworks of Aarts et al. [ 21 ] and Katsma et al. [ 31 ]. These studies recognize that EHR implementation is relatively unpredictable due to unforeseen contingencies for which one cannot plan. With their emphasis on emergent change with unpredictable outcomes, Aarts et al. [ 21 ] make a case for acknowledging that unexpected and unplanned contingencies will influence the implementation process. They argue that the changes resulting from these contingencies often manifest themselves unexpectedly and must then be dealt with. Additionally, Takian et al. [ 37 ] state that it is crucial to contextualize an EHR implementation so as to be better prepared for unexpected changes.
C5: Establishing an interdisciplinary implementation group consisting of developers, members of the IT department, and end-users fosters EHR implementation success
In line with the arguments for management support and for the participation of clinical staff, Ovretveit et al. [ 32 ], Simon et al. [ 36 ] and Weir et al. [ 19 ] build a case for using an interdisciplinary implementation group. By having all the direct stakeholders working together, a better EHR system can be delivered faster and with fewer problems.
C6: Resistance of clinical staff, in particular of physicians, is a major barrier to EHR implementation, but can be reduced by addressing their concerns
Clinical staff’s attitude is a crucial factor in EHR implementation [ 36 ]. Particularly, the physicians constitute an important group in hospitals. As such, their possible resistance to EHR implementation will form a major barrier [ 29 , 33 ] and may lead to workarounds [ 26 ]. Whether physicians accept or reject an EHR implementation depends on their acceptance of their work practices being transformed [ 22 ]. The likelihood of acceptance will be increased if implementers address the concerns of physicians [ 24 , 28 , 32 , 33 ], but also of other members of clinical staff [ 36 ].
C7: Identifying champions among clinical staff reduces resistance
The previous finding already elaborated on clinical staff resistance and suggested reducing this by addressing their concerns. Another way to reduce their resistance is related to the process of implementation and involves identifying physician champions, typically physicians that are well respected due to their knowledge and contacts [ 32 , 33 ]. Simon et al. [ 36 ] emphasize the importance of identifying champions among each stakeholder group. These champions can provide reassurance to their peers.
C8: Assigning a sufficient number of staff and other resources to the EHR implementation process is important in adequately implementing the system
Implementing a large EHR system requires considerable resources, including human ones. Assigning appropriate people, such as super-users [ 36 ] and a sufficient number of them to that process will increase the likelihood of success [ 19 , 32 , 33 , 36 ]. Further, it is important to have sufficient time and financial resources [ 26 , 32 ]. This finding is also relevant in relation to finding A6 (ensuring good care during organizational change).
These 19 general findings have been identified from the individual findings within the 20 analyzed articles. These findings are all related to one of the three main and interacting dimensions of the framework: six to context, five to content, and eight to process. This identification and explanation of the general findings concludes the results section of this systematic literature review and forms the basis for the discussion below.
This review of the existing academic literature sheds light on the current knowledge regarding EHR implementation. The 21 selected articles all originate from North America or Europe, perhaps reflecting a greater governmental attention to EHR implementation in these regions and, of course, our inclusion of only articles written in English. Two articles were rejected for quality reasons [ 43 , 44 ], see Appendix B. All but one of the selected articles have been published since 2000, reflecting the growing interest in implementing EHR systems in hospitals. Eight articles built their research on a theoretical framework, four of which use the same general lens of the sociotechnical approach [ 21 , 22 , 26 , 37 ]. Katsma et al. [ 31 ] and Rivard et al. [ 34 ] focus more on the social and cultural aspects of EHR implementation, the former on the relevance for, and participation of, users, the latter on three different cultural perspectives. Ford et al. [ 27 ] researched adoption strategies for EHR systems and Gastaldi et al. [ 26 ] consider them as a means to renew organizational capabilities. It is notable that the other reviewed articles did not use a theoretical framework to analyze EHR implementation and made no attempt to elaborate on existing theories.
A total of 127 findings were extracted from the articles, and these findings were categorized using Pettigrew’s framework for strategic change [ 13 ] as a conceptual model including the three dimensions of context, content, and process. To ensure a tight focus, the scope of the review was explicitly limited to findings related to the EHR implementation process, thus excluding the reasons for, barriers to, and outcomes of an EHR implementation.
Some of the findings require further interpretation. Contextual finding A1 relates to the demographics of a hospital. One of the assertions is that privately owned hospitals are less likely than public hospitals to invest in an EHR. The former apparently perceive the costs of EHR implementation to outweigh the benefits. This seems remarkable given that there is a general belief that information technology increases efficiency and reduces process costs, so more than compensating for the high initial investments. It is however important to note that the literature on EHR is ambivalent when it comes to efficiency; several authors record a decrease in the efficiency of work practices [ 25 , 33 , 35 , 38 ], whereas others mention an increase [ 29 , 31 ]. Finding A2 is a reminder of the importance of carefully selecting an appropriate vendor, taking into account experience with the EHR market and the maturity of their products rather than, for example, focussing on the cost price of the system. Given the huge investment costs, the price of an EHR system tends to have a major influence on vendor selection, an aspect that is also promoted by the current European tendering regulations that oblige (semi-) public institutions, like many hospitals, to select the lowest bidder, or the bidder that is economically the most preferable [ 45 ]. The finding that EHR system implementation is difficult because good medical care needs to be ensured at all times (A6) also deserves mention. Essentially, many system implementations in hospitals are different from IT implementations in other contexts because human lives are at stake in hospitals. This not only complicates the implementation process because medical work practices have to continue, it also requires a system to be reliable from the moment it is launched.
The findings regarding the content of the EHR system (Category B) highlight the importance of a suitable software product. A well-defined selection process of the software package and its associated vendor (discussed in A2) is seen as critical (B5). Selection should be based on a careful requirements analysis and an analysis of the experience and quality of the vendor. An important requirement is a sufficient degree of flexibility to customize and adapt the software to meet the needs of users and the work practices of the hospital (finding B1). At the same time the software product should challenge the hospital to rethink and improve its processes. A crucial condition for the acceptance by the diverse user groups of hospitals is the robustness of the EHR system in terms of availability, speed, reliability and flexibility (B2). This also requires adequate hardware in terms of access to computers, and mobile equipment to enable availability at all the locations of the hospital. Perceived ease of use of the system (B4) and the protection of patients’ privacy (B4) are other content factors that can make or break EHR implementation in hospitals.
The findings on the implementation process, our Category C, highlight four aspects that are commonly mentioned in change management approaches as important success factors in organizational change. The active involvement and support of management (C1), the participation of clinical staff (C2), a comprehensive implementation strategy (C4), and using an interdisciplinary implementation group (C5) correspond with three of the ten guidelines offered by Kanter et al. [ 46 ]. These three guidelines are: (1) support a strong leader role; (2) communicate, involve people, and be honest; and (3) craft an implementation plan. As the implementation of an EHR system is an organizational change process it is no surprise that these commonalities are identified in several of the analyzed articles. Three Category C findings (C2, C6, and C7) concern dealing with clinical staff given their powerful positions and potential resistance. Physicians are the most influential medical care providers, and their resistance can delay an EHR implementation [ 23 ], lead to at least some of it being dropped [ 21 , 22 , 34 ], or to it not being implemented at all [ 33 ]. Thus, there is ample evidence of the crucial importance of physicians’ acceptance of an EHR for it to be implemented. This means that clinicians and other key personnel should be highly engaged and motivated to contribute to EHR. Prompt feedback on requests, and high quality support during the implementation, and an EHR that clearly supports clinical work are key issues that contribute to a motivated clinical staff.
Analyzing and comparing the findings enables us to categorize them in terms of subject matter (see Table 7 ). By categorizing the findings in terms of subject, and by totaling the number of articles related to the individual findings on that subject, one can deduce how much attention has been given in the literature to the different topics. This analysis highlights that the involvement of physicians in the implementation process, the quality of the system, and a comprehensive implementation strategy are considered the crucial elements in EHR implementation.
Notwithstanding the useful results, this review and analysis has some limitations. Although we carefully developed and executed the search strategy, we cannot be sure that we found all the relevant articles. Since we focused narrowly on keywords, and these had to be part of an article’s title, we could have excluded relevant articles that used different terminology in their titles. Although searching the reference lists of identified articles did result in several additional articles, some relevant articles might still have been missed. Another limitation is the exclusion of publications in languages other than English. Further, the selection and categorization of specific findings, and the subsequent extraction of general findings, is subjective and depends on the interpretations of the authors, and other researchers might have made different choices. A final limitation is inherent to literature reviews in that the authors of the studies included may have had different motives and aims, and used different methods and interpretative means, in drawing their conclusions.
The existing literature fails to provide evidence of there being a comprehensive approach to implementing EHR systems in hospitals that integrates relevant aspects into an ‘EHR change approach’. The literature is diffuse, and articles seldom build on earlier ones to increase the theoretical knowledge on EHR implementation, notable exceptions being Aarts et al. [ 21 ], Aarts and Berg [ 22 ], Cresswell et al. [ 26 ], and Takian et al. [ 37 ]. The earlier discussion on the various results summarizes the existing knowledge and reveals gaps in the knowledge associated with EHR implementation. The number of EHR implementations in hospitals is growing, as well as the body of literature on this subject. This systematic review of the literature has produced 19 general findings on EHR implementation, which were each placed in one of three categories. A number of these general findings are in line with the wider literature on change management, and others relate to the specific nature of EHR implementation in hospitals.
The findings presented in this article can be viewed as an overview of important subjects that should be addressed in implementing an EHR system. It is clear that EHR systems have particular complexities and should be implemented with great care, and with attention given to context, content, and process issues and to interactions between these issues. As such, we have achieved our research goal by creating a systematic review of the literature on EHR implementation. This paper’s academic contribution is in providing an overview of the existing literature with regard to important factors in EHR implementation in hospitals. Academics interested in this specific field can now more easily access knowledge on EHR implementation in hospitals and can use this article as a starting point and build on the existing knowledge. The managerial contribution lies in the general findings that can be applied as guidelines when implementing EHR in hospitals. We have not set out to provide a single blueprint for implementing an EHR system, but rather to provide guidelines and to highlight points that deserve attention. Recognizing and addressing these aspects can increase the likelihood of getting an EHR system successfully implemented.
Appendix A - List of databases
This appendix provides an overview of all databases included in the used search engines. The databases in italic were excluded for the research as these databases focus on fields not relevant for the subject of EHR implementations.
Web of Knowledge
Web of Science
Biological Abstracts
Journal Citation Reports
Academic Search Premier
AMED - The Allied and Complementary Medicine Database
America : History & Life
American Bibliography of Slavic and East European Studies
Arctic & Antarctic Regions
Art Full Text ( H.W. Wilson )
Art Index Retrospective ( H.W. Wilson )
ATLA Religion Database with ATLASerials
Business Source Premier
Communication & Mass Media Complete
eBook Collection ( EBSCOhost )
Funk & Wagnalls New World Encyclopedia
Historical Abstracts
L ’ Annéephilologique
Library, Information Science & Technology Abstracts
MAS Ultra - School Edition
Military & Government Collection
MLA Directory of Periodicals
MLA International Bibliography
New Testament Abstracts
Old Testament Abstracts
Philosopher ’ s Index
Primary Search
PsycARTICLES
PsycCRITIQUES
Psychology and Behavioral Sciences Collection
Regional Business News
Research Starters - Business
RILM Abstracts of Music Literature
The Cochrane Library
Cochrane Database of Systematic Reviews
Cochrane Central Register of Controlled Trials
Cochrane Methodology Register
Database of Abstracts of Reviews of Effects
Health Technology Assessment Database
NHS Economic Evaluation Database
About The Cochrane Collaboration
Appendix B - Quality assessment
The quality of the articles was assessed with the Standard Quality Assessment Criteria for Evaluating Primary Research Papers [ 18 ]. Assessment was done by questioning whether particular criteria had been addressed, resulting in a rating of 2 (completely addressed), 1 (partly addressed), or 0 (not addressed) points. Table 8 provides the overview of the scores of the articles, (per question) for qualitative studies; Table 9 for quantitative studies; and Table 10 for mixed methods studies. Articles were included if they scored 50% or higher of the total amount of points possible. Based on this assessment, two articles were excluded from the search.
Appendix C - All findings
Table 11 displays all findings from the selected articles. The category number is related to the general finding as discussed in the Results section.
Abramson EL, McGinnis S, Edwards A, Maniccia DM, Moore J, Kaushal R: Electronic health record adoption and health information exchange among hospitals in New York State. J Eval Clin Pract. 2011, 18: 1156-1162.
Article PubMed Google Scholar
Robertson A, Cresswell K, Takian A, Petrakaki D, Crowe S, Cornford T, Sheikh A: Implementation and adoption of nationwide electronic health records in secondary care in England: qualitative analysis of interim results from a prospective national evaluation. Br Med J. 2010, 341: c4564-10.1136/bmj.c4564.
Article Google Scholar
Rigsrevisionen: Extract from the report to the Public Accounts Committee on the implementation of electronic patient records at Danish hospitals. 2011, http://uk.rigsrevisionen.dk/media/1886186/4-2010.pdf , 2011
Google Scholar
Hartswood M, Procter R, Rouncefield M, Slack R: Making a Case in Medical Work: Implications for the Electronic Medical Record. Comput Supported Coop Work. 2003, 12: 241-266. 10.1023/A:1025055829026.
Grimson J, Grimson W, Hasselbring W: The SI Challenge in Health Care. Commun ACM. 2000, 43 (6): 49-55.
Mantzana V, Themistocleous M, Irani Z, Morabito V: Identifying healthcare actors involved in theadoption of information systems. Eur J Inf Syst. 2007, 16: 91-102. 10.1057/palgrave.ejis.3000660.
Boonstra A, Boddy D, Bell S: Stakeholder management in IOS projects: analysis of an attempt to implement an electronic patient file. Eur J Inf Syst. 2008, 17 (2): 100-111. 10.1057/ejis.2008.2.
Jha A, DesRoches CM, Campbell EG, Donelan K, Rao SR, Ferris TF, Shields A, Rosenbaum S, Blumenthal D: Use of Electronic Health Records in US hospitals. N Engl J Med. 2009, 360: 1628-1638. 10.1056/NEJMsa0900592.
Article CAS PubMed Google Scholar
Heeks R: Health information systems: Failure, success and improvisation. Int J Med Inform. 2006, 75: 125-137. 10.1016/j.ijmedinf.2005.07.024.
Boonstra A, Govers MJ: Understanding ERP system implementation in a hospital by analysing stakeholders. N Technol Work Employ. 2009, 24 (2): 177-193. 10.1111/j.1468-005X.2009.00227.x.
Keshavjee K, Bosomworth J, Copen J, Lai J, Kucukyazici B, Liani R, Holbrook AM: Best practices in EMR implementation: a systematic review. Proceed of the 11th International Symposium on Health Information Mangement Research – iSHIMR. 2006, 1-15.
McGinn CA, Grenier S, Duplantie J, Shaw N, Sicotte C, Mathieu L, Leduc Y, Legare F, Gagnon MP: Comparison of use groups perspectives of barriers and facilitator to implementing EHR – a systematic review. BMC Med. 2011, 9: 46-10.1186/1741-7015-9-46.
Article PubMed PubMed Central Google Scholar
Pettigrew AM: Context and action in the transformation of the firm. J Manag Stud. 1987, 24 (6): 649-670. 10.1111/j.1467-6486.1987.tb00467.x.
Hartley J: Case Study Research. Chapter 26. Essential Guide to Qualitative Methods in Organizational Research. Edited by: Cassel C, Symon G. 2004, London: Sage
Hage E, Roo JP, Offenbeek MAG, Boonstra A: Implementation factors and their effect on e-health service adoption in rural communities: a systematic literature review. BMC Health Serv Res. 2013, 13 (19): 1-16.
ISO: Health informatics: Electronic health record - Definition, scope and context. Draft Tech Report. 2004, 03-16. ISO/DTR 20514. available at https://www.iso.org/obp/ui/#iso:std:iso:tr:20514:ed-1:v1:en
Häyrinen K, Saranto K, Nykänen P: Definition, structure, content, use and impacts of electronic health records: A review of the research literature. Int J Med Inform. 2008, 77: 291-304. 10.1016/j.ijmedinf.2007.09.001.
Kaushal R, Shojania KG, Bates DW: Effects of computerized physician order entry and clinical decision support systems on medication safety: a systematic review. Arch Intern Med. 2003, 163 (12): 1409-1416. 10.1001/archinte.163.12.1409.
Weir C, Lincoln M, Roscoe D, Turner C, Moreshead G: Dimensions associated with successful implementation of a hospital based integrated order entry system. Proc Annu Symp Comput Appl [Sic] in Med Care Symp Comput Appl Med Care. 1994, 653: 7.
Kmet LM, Lee RC, Cook LS: Standard quality assessment criteria for evaluating primary research papers from a variety of fields. 2004, Alberta Heritage Foundation for Medical Research, http://www.ihe.ca/documents/HTA-FR13.pdf .
Aarts J, Doorewaard H, Berg M: Understanding implementation: The case of a computerized physician order entry system in a large dutch university medical center. J Am Med Inform Assoc. 2004, 11 (3): 207-216. 10.1197/jamia.M1372.
Aarts J, Berg M: Same systems, different outcomes - Comparing the implementation of computerized physician order entry in two Dutch hospitals. Methods Inf Med. 2006, 45 (1): 53-61.
CAS PubMed Google Scholar
Ash J, Gorman P, Lavelle M, Lyman J, Fournier L: Investigating physician order entry in the field: lessons learned in a multi-center study. Stud Health Technol Inform. 2001, 84 (2): 1107-1111.
Ash JS, Gorman PN, Lavelle M, Payne TH, Massaro TA, Frantz GL, Lyman JA: A cross-site qualitative study of physician order entry. J Am Med Inform Assoc. 2003, 10 (2): 188-200. 10.1197/jamia.M770.
Boyer L, Samuelian J, Fieschi M, Lancon C: Implementing electronic medical records in a psychiatric hospital: A qualitative study. Int J Psychiatry Clin Pract. 2010, 14 (3): 223-227. 10.3109/13651501003717243.
Cresswell KM, Worth A, Sheikh A: Integration of a nationally procured electronic health record system into user work practices. BMC Med Inform Decis Mak. 2012, 12: 15-10.1186/1472-6947-12-15.
Ford EW, Menachemi N, Huerta TR, Yu F: Hospital IT Adoption Strategies Associated with Implementation Success: Implications for Achieving Meaningful Use. J Healthc Manag. 2010, 55 (3): 175-188.
PubMed Google Scholar
Gastaldi L, Lettieri E, Corso M, Masella C: Performance improvement in hospitals: leveraging on knowledge assets dynamics through the introduction of an electronic medical record. Meas Bus Excell. 2012, 16 (4): 14-30. 10.1108/13683041211276410.
Houser SH, Johnson LA: Perceptions regarding electronic health record implementation among health information management professionals in Alabama: a statewide survey and analysis. Perspect Health Inf Manage/AHIMA, Am Health Inf Manage Assoc. 2008, 5: 6-6.
Jaana M, Ward MM, Bahensky JA: EMRs and Clinical IS Implementation in Hospitals: A Statewide Survey. J Rural Health. 2012, 28: 34-43. 10.1111/j.1748-0361.2011.00386.x.
Katsma CP, Spil TAM, Ligt E, Wassenaar A: Implementation and use of an electronic health record: measuring relevance and participation in four hospitals. Int J Healthc Technol Manag. 2007, 8 (6): 625-643. 10.1504/IJHTM.2007.014194.
Ovretveit J, Scott T, Rundall TG, Shortell SM, Brommels M: Improving quality through effective implementation of information technology in healthcare. Int J Qual Health Care. 2007, 19 (5): 259-266. 10.1093/intqhc/mzm031.
Poon EG, Blumenthal D, Jaggi T, Honour MM, Bates DW, Kaushal R: Overcoming barriers to adopting and implementing computerized physician order entry systems in US hospitals. Health Aff. 2004, 23 (4): 184-190. 10.1377/hlthaff.23.4.184.
Rivard S, Lapointe L, Kappos A: An Organizational Culture-Based Theory of Clinical Information Systems Implementation in Hospitals. J Assoc Inf Syst. 2011, 12 (2): 123-162.
Scott JT, Rundall TG, Vogt TM, Hsu J: Kaiser Permanente’s experience of implementing an electronic medical record: a qualitative study. Br Med J. 2005, 331 (7528): 1313-1316. 10.1136/bmj.38638.497477.68.
Simon SR, Keohane CA, Amato M, Coffey M, Cadet M, Zimlichman E: Lessons learned from implementation of computerized provider order entry in 5 community hospitals: a qualitative study. BMC Med Inform Decis Mak. 2013, 13: 67-10.1186/1472-6947-13-67.
Takian A, Sheikh A, Barber N: We are bitter, but we are better off: case study of the implementation of an electronic health record system into a mental health hospital in England. BMC Health Serv Res. 2012, 12: 484-10.1186/1472-6963-12-484.
Ward MM, Vartak S, Schwichtenberg T, Wakefield DS: Nurses’ Perceptions of How Clinical Information System Implementation Affects Workflow and Patient Care. Cin-Comput Inform Nurs. 2011, 29 (9): 502-511. 10.1097/NCN.0b013e31822b8798.
Ward MM, Vartak S, Loes JL, O’Brien J, Mills TR, Halbesleben JRB, Wakefield DS: CAH Staff Perceptions of a Clinical Information System Implementation. Am J Manage Care. 2012, 18 (5): 244-252.
Yoon-Flannery K, Zandieh SO, Kuperman GJ, Langsam DJ, Hyman D, Kaushal R: A qualitative analysis of an electronic health record (EHR) implementation in an academic ambulatory setting. Inform Prim Care. 2008, 16 (4): 277-284.
Van Aken J, Berends H, Van der Bij H: Problem solving in organizations. 2012, New York, USA: Cambridge University Press
Book Google Scholar
Botha ME: Theory development in perspective: the role of conceptual frameworks and models in theory development. J Adv Nurs. 1989, 14 (1): 49-55. 10.1111/j.1365-2648.1989.tb03404.x.
Spetz J, Keane D: Information Technology Implementation in a Rural Hospital: A Cautionary Tale. J Healthc Manag. 2009, 54 (5): 337-347.
Massaro TA: Introducing Physician Order Entry at a Major Academic Medical-Center. Impact Organ Culture Behav Acad Med. 1993, 68 (1): 20-25.
CAS Google Scholar
Lundberg S, Bergman M: Tender evaluation and supplier selection methods in public procurement. J Purch Supply Manage. 2013, 19 (2): 73-83. 10.1016/j.pursup.2013.02.003.
Kanter RM, Stein BA, Jick TD: The Challenge of Organizational Change. 1992, New York, USA: Free Press
Pre-publication history
The pre-publication history for this paper can be accessed here: http://www.biomedcentral.com/1472-6963/14/370/prepub
Download references
Acknowledgement
We acknowledge the Master degree program Change Management at the University of Groningen for supporting this study. We also thank the referees for their valuable comments.
Author information
Authors and affiliations.
Faculty of Economics and Business, University of Groningen, Groningen, The Netherlands
Albert Boonstra & Janita F J Vos
Deloitte Consulting, Amsterdam, The Netherlands
Arie Versluis
You can also search for this author in PubMed Google Scholar
Corresponding author
Correspondence to Albert Boonstra .
Additional information
Competing interests.
The authors declare that they have no competing interests.
Authors’ contributions
AB and JV established the research design and made significant contributions to the interpretation of the results. They supervised AV throughout the study, and participated in writing the final version of this paper. AV contributed substantially to the selection and analysis of included papers, and wrote a preliminary draft of this article. All authors have read and approved the final manuscript.
Authors’ original submitted files for images
Below are the links to the authors’ original submitted files for images.
Authors’ original file for figure 1
Authors’ original file for figure 2, rights and permissions.
This article is published under license to BioMed Central Ltd. This is an Open Access article distributed under the terms of the Creative Commons Attribution License ( http://creativecommons.org/licenses/by/2.0 ), which permits unrestricted use, distribution, and reproduction in any medium, provided the original work is properly credited. The Creative Commons Public Domain Dedication waiver ( http://creativecommons.org/publicdomain/zero/1.0/ ) applies to the data made available in this article, unless otherwise stated.
Reprints and permissions
About this article
Cite this article.
Boonstra, A., Versluis, A. & Vos, J.F.J. Implementing electronic health records in hospitals: a systematic literature review. BMC Health Serv Res 14 , 370 (2014). https://doi.org/10.1186/1472-6963-14-370
Download citation
Received : 23 September 2013
Accepted : 11 August 2014
Published : 04 September 2014
DOI : https://doi.org/10.1186/1472-6963-14-370
Share this article
Anyone you share the following link with will be able to read this content:
Sorry, a shareable link is not currently available for this article.
Provided by the Springer Nature SharedIt content-sharing initiative
- Clinical Staff
- Electronic Health Record
- Electronic Patient Record
- Health Information Technology
- Computerize Physician Order Entry
BMC Health Services Research
ISSN: 1472-6963
- General enquiries: [email protected]
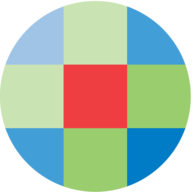
- Get new issue alerts Get alerts
Secondary Logo
Journal logo.
Colleague's E-mail is Invalid
Your message has been successfully sent to your colleague.
Save my selection
Electronic Health Records and Quality of Care
An observational study modeling impact on mortality, readmissions, and complications.
Editor(s): Chu., Danny
From the Stanford University School of Medicine (SY); Stanford University School of Medicine (DM, CC, TH-B), Department of Surgery; Stanford University School of Medicine (KM), Center for Primary Care and Outcomes Research; and Stanford University School of Medicine (TH-B), Biomedical Informatics, Stanford, CA.
Correspondence: Tina M. Hernandez-Boussard, Stanford University School of Medicine, Biomedical Informatics, 1070 Arastradero #373, Stanford, CA 94305-5559, USA (e-mail: [email protected] ).
Abbreviations: AHA = american hospital association, CI = confidence interval, DiD = difference-in-differences, DRG = diagnosis-related group, EHR = electronic health record, HCUP = health care utilization project, LOS = length of stay, OR = odds ratio, PSI = patient safety indicator, SID = state inpatient database.
THB conceived and designed the study, supervised and contributed to the data analysis, interpreted results, and made substantial revisions to the paper; DM contributed to the study design, conducted the data analysis, and contributed to revisions of the paper; SY drafted the paper and contributed to the interpretation of the data analysis; KM contributed to the interpretation of the data analysis and revisions of the paper; and CC contributed to the interpretation of the data analysis and revisions of the paper.
Preliminary findings from this paper were presented at the Academy Health Research Meeting, San Diego, June 8–10, 2014 and at AMIA Annual Symposium, San Francisco, November 14–18, 2015.
Funding Research reported in this publication was supported by the National Cancer Institute of the National Institutes of Health under Award Number R01CA183962. The content is solely the responsibility of the authors and does not necessarily represent the official views of the National Institutes of Health. This project was supported by grant number R01HS024096 from the Agency for Healthcare Research and Quality. The content is solely the responsibility of the authors and does not necessarily represent the official views of the Agency for Healthcare Research and Quality.
The authors have no conflicts of interest to disclose.
This is an open access article distributed under the Creative Commons Attribution License 4.0, which permits unrestricted use, distribution, and reproduction in any medium, provided the original work is properly cited. http://creativecommons.org/licenses/by/4.0
Received February 5, 2016
Received in revised form March 9, 2016
Accepted March 18, 2016
Electronic health records (EHRs) were implemented to improve quality of care and patient outcomes. This study assessed the relationship between EHR-adoption and patient outcomes.
We performed an observational study using State Inpatient Databases linked to American Hospital Association survey, 2011. Surgical and medical patients from 6 large, diverse states were included. We performed univariate analyses and developed hierarchical regression models relating level of EHR utilization and mortality, readmission rates, and complications. We evaluated the effect of EHR adoption on outcomes in a difference-in-differences analysis, 2008 to 2011.
Medical and surgical patients sought care at hospitals reporting no EHR (3.5%), partial EHR (55.2%), and full EHR systems (41.3%). In univariate analyses, patients at hospitals with full EHR had the lowest rates of inpatient mortality, readmissions, and Patient Safety Indicators followed by patients at hospitals with partial EHR and then patients at hospitals with no EHR ( P < 0.05). However, these associations were not robust when accounting for other patient and hospital factors, and adoption of an EHR system was not associated with improved patient outcomes ( P > 0.05).
These results indicate that patients receiving medical and surgical care at hospitals with no EHR system have similar outcomes compared to patients seeking care at hospitals with a full EHR system, after controlling for important confounders.
To date, we have not yet seen the promised benefits of EHR systems on patient outcomes in the inpatient setting. EHRs may play a smaller role than expected in patient outcomes and overall quality of care.
INTRODUCTION
It is thought that health information technology, particularly electronic health records (EHR), will improve quality and efficiency of healthcare organizations, from small practices to large groups. 1 Given these potential benefits, the federal government encouraged EHR adoption under the Health Information Technology for Economic and Clinical Health (HITECH) Act. In response, many hospitals are striving to adopt these systems and demonstrate meaningful use. In 2013, 59% of US hospitals had some type of EHR system. 2 The federal incentive program defined 3 stages for timely adoption of EHR use: stage 1 is EHR adoption, stage 2 is EHR data exchange, and stage 3 is using EHRs to improve patient outcomes. 3 However, despite the widespread adoption of EHR systems, only about 6% of hospitals met all criteria of stage 2 meaningful use. 2–4 Thus, implementing and developing meaningful use for EHRs is still an ongoing process in the American healthcare system.
Electronic health records were originally built for billing purposes, not for research and quality improvement efforts. 5 Accordingly, the impact of EHRs on quality healthcare delivery has focused on physician performance and billing precision. 6 EHR studies often concentrate on process quality metrics, analyzing physician-level variability, and guideline compliance, rather than overall quality improvement or patient outcomes. 7–11 Some have suggested that EHRs have the potential to decrease medical errors by providing improved access to necessary information, better communication and integration of care between different providers and visits, and more efficient documentation and monitoring. 12 Many have used EHRs to decrease prescribing errors by providing real time clinical decision support. 13–16 Other recent studies have begun to use EHRs to track and monitor adverse patient outcomes such as catheter-associated urinary tract infections, deep vein thrombosis, or pulmonary embolism, providing critical data to improve patient safety outcomes. 17,18
Therefore, while several studies have looked at changes in quality attributed to electronic healthcare systems, overall improvements in patient outcomes associated with EHR implementation are still not yet well documented. In particular, the effect of the implementation of an EHR system on inpatient adverse events, inpatient mortality and 30-day all cause readmission for specific surgical, and medical conditions has yet to be explored. We thus sought to determine the association of hospital level-EHR systems with important patient outcomes.
The objective of this study was to determine whether hospitals with fully implemented EHR systems had better patient outcomes compared to hospitals with partial or no implemented EHR system after controlling for other important patient and hospital characteristics. Our study provides new information about the relationship between the implementation of an EHR system and the quality of healthcare delivered in the inpatient setting.
Data Source
We utilized discharge data from the 2011 State Inpatient Databases (SID), Healthcare Cost and Utilization Project (HCUP), Agency for Healthcare Research and Quality from Arkansas, California, Florida, Massachusetts, Mississippi, and New York. 19 SID is an all-capture state database that allows linkage of patients overtime and contains information on patient characteristics, primary and secondary diagnoses, and procedures received. The SID database was linked to the 2011 American Hospital Association (AHA) annual survey database, which contains information on EHR utilization in different hospitals along with other important hospital characteristics. 20
Patient safety indicators (PSI) are based on ICD-9-CM codes and Medicare severity diagnosis-related groups (DRGs), with specific inclusion and exclusion criteria determined by the Agency for Healthcare Research and Quality (AHRQ). 21 Using the PSI software (version 4.5), 22 we identified these adverse events in our dataset. Each PSI includes a unique denominator, numerator, and set of risk adjustors. 23,24
Study Population
Both surgical and medical patients from several diagnostic categories were included in the study. Specifically, surgical patients undergoing pulmonary lobectomy, open abdominal aortic aneurysm repair, endovascular abdominal aortic aneurysm repair, or colectomy were included. Medical patients receiving care for acute myocardial infarction, congestive heart failure, or pneumonia were included. These categories were chosen based on their frequency, contribution to patient comorbidities, and prevalence in the medical literature.
Outcome of Interest
Our main outcomes of interest were inpatient mortality, 30-day all cause readmission rates, PSIs, and length of stay.
Statistical Analysis
We utilized univariate regression analysis to develop descriptive statistics. A hierarchical regression model relating level of EHR utilization and quality of care was developed. The independent variables were level of EHR utilization (no EHR, partial EHR, or full EHR), patient demographics, comorbidities, and medical or surgical group. The dependent variables were mortality, readmissions, and complications (measured by PSIs). Relative-risk difference in differences analyses (DiD) were used to determine the effect of implementing an EHR system on quality of care. 25 The difference-in-differences analyses combined pre–post and treatment–control comparisons to eliminate some types of potential confounding. To do so, the hospitals lacking EHR systems in 2008 were selected and were then split into 3 groups: those that gained full EHRs by 2011 (treatment 1), those that gained only partial EHRs by 2011 (treatment 2), and those that still had no EHRs in 2011 (control). Direct comparison of these groups might be biased by confounders: there must be reasons why some of the hospitals adopted EHRs while others did not, and those reasons might also impact the outcomes of interest, both before and after adoption. Therefore, each hospital's event rates in 2008 are compared with the same hospital's rates in 2011, and the changes in those rates are then used to compare each treatment (i.e., EHR adoption level) with control (no EHR adoption). This analysis assumes that, in the absence of the intervention, the groups would have parallel trends and common external shocks. The use of a modified-Poisson model in these analyses enables estimation of risk ratios rather than odds ratios. 26 All statistical analyses were performed using STATA version 13.0, except for the difference-in-differences analyses, which were performed using both STATA and SAS version 9.3. 27
Patient characteristics are described in Tables 1 and 2 . A total of 137,162 surgical patients and 311,605 medical patients were included. Medical and surgical patients sought care at hospitals reporting no EHR (3.5%), partial EHR (55.2%), and full EHR (41.3%). Of the surgical patients, 2.7% were treated in a hospital with no EHR, 55.9% were treated in a hospital with partial EHR, and 41.4% were treated in a hospital with full EHR. Of the medical patients, 3.9% were treated in a hospital with no EHR, 55.0% of patients were treated in a hospital with partial EHR, and 41.2% were treated in hospitals with full EHR.

In the cross-sectional analyses, surgical patients treated at hospitals with full EHR had higher mortality rates (1.6%) than patients treated at hospitals with partial EHR (1.4%) or at hospitals with no EHR (1.6%) ( P = 0.0086). Surgical patients treated at hospitals with full EHR had higher readmission rates (11.9%) than patients treated at hospitals with partial EHR (11.2%) but lower readmission rates than patients treated at hospitals with no EHR (12.6%) ( P = 0.0005). Surgical patients treated at hospitals with full EHR had higher rates of complications measured by PSIs (3.7%) than patients treated at hospitals with partial EHR (3.0%) or no EHR (3.2%) ( P < 0.0001). Surgical patients treated at hospitals with full EHR had a shorter length of stay (LOS), measured in days (6.38) than patients treated at hospitals with partial EHR (6.85) or no EHR (7.89) ( P < 0.0001) ( Table 3 ).

Medical patients treated at hospitals with full EHR had a lower mortality rate (3.7%) than patients treated at hospitals with partial EHR (4.0%) or no EHR (4.4%) ( P < 0.0001). Medical patients treated at hospitals with full EHR did not have a statistically significant different readmission rate (19.4%) compared to patients treated at hospitals with partial EHR (19.6%) or no EHR (20.3%) ( P = 0.0548). Medical patients treated at hospitals with full EHR did not have a statistically significant difference in complications measured by PSIs (0.9%) compared to patients treated at hospitals with partial EHR (0.9%) or no EHR (0.8%) ( P = 0.196). Patients treated at hospitals with full EHR had a shorter length of stay (5.02) than patients treated at hospitals with partial EHR (5.28) or no EHR (5.76) ( P < 0.0001) ( Table 4 ).

In the multiple regression analysis, there was no statistically significant difference in mortality rate among surgical patients treated at hospitals with full versus no EHR (odds ratio [OR] 1.24, P = 0.1442) or partial versus no EHR (OR 1.24, P = 0.1788) after controlling for important patient and hospital characteristics. There was no statistically significant difference in readmission rates among surgical patients treated at hospitals with full versus no EHR (OR 1.04, P = 0.5605) or partial versus no EHR (OR 1.04, P = 0.5158). There was a significant difference between rates of any complication measured by PSIs among surgical patients treated at hospitals with full versus no EHR (OR 1.22, P = 0.0452) but not between patients treated at hospitals with partial versus no EHR (OR 1.12, P = 0.2679).
There was no statistically significant difference between mortality rates among medical patients treated at hospitals with full EHR versus no EHR (OR 0.97, P = 0.4609) or partial versus no EHR (OR 1.01, P = 0.9375). There was no statistically significant difference between readmission rates among medical patients treated at hospitals with full versus no EHR (OR 0.97, P = 0.2834) or partial versus no EHR (OR 1.00, P = 0.8697). There was no statistically significant difference in complications measured by PSIs among medical patients treated at hospitals with full versus no EHR (OR 1.06, P = 0.6157) or partial versus no EHR (OR 1.13, P = 0.2516) ( Table 5 ).

The difference-in-differences analysis allowed us to estimate the effect of implementing an EHR system on patient outcomes, assuming that the parallel trends and common shocks assumptions are correct. These analyses found statistically significant evidence of an effect in only three cases ( Table 6 ).

There was evidence of reduced risk of PSIs for medical patients in hospitals that had partially implemented EHRs by 2011 compared to those that still lacked EHRs in 2011 (risk ratio 0.673, 95% confidence intervals [CI] [0.45, 1.00], P -value 0.0492). There was also evidence of reduced risk of PSIs for medical patients in hospitals with fully implemented EHRs by 2011 compared with hospitals with no EHRs in 2011 (risk ratio 0.591, CI [0.38, 0.92], P -value 0.0198. Finally there was evidence of reduce risk of inpatient mortality for surgical patients in hospitals with partially implemented EHRs compared with no EHRs (risk ratio 2.031, CI [1.09, 3.79], P -value 0.0259) ( Table 6 ).
This study tested the association between level of EHR implementation in inpatient settings and patient outcomes across 6 large, diverse states for both medical and surgical care. These results provided a preliminary glimpse at EHR meaningful use. Cross-sectional analysis found significant differences in rates of mortality, readmission, and complications between patients at hospitals with full EHR or partial EHR compared to hospitals with no EHR. However, these differences did not hold when adjusted for patient and hospital factors. Furthermore, the effect of EHR adoption was not associated with improved patient outcomes (specifically inpatient mortality, readmissions, and complications). Although EHR systems are thought to improve quality of care, this study suggests that in their current form, EHRs have not begun to reach meaningful use targets and may have a smaller impact than expected on patient outcomes.
This study builds on multiple studies highlighting the limitations of EHR systems on improving quality of care. Although EHRs have been extremely helpful for billing and physician compliance measurements, direct improvement of important patient outcomes have yet to be seen. A possible reason for this is that EHRs thus far have largely served as a recording mechanism after a patient care intervention rather than as an effective checking mechanism during the actual execution phase of patient care interventions. It has also been shown that while basic EHRs are associated with gains in quality measures, less benefit is associated with implementing advanced EHRs, suggesting that initial adoption of EHRs may actually be counterproductive by adding additional complexity into clinical settings. 28 Additionally, such improvements have yet to be translated to improvements in mortality. 29 Lack of improvement in other patient outcome measures has also been demonstrated. For example, 1 study demonstrated that although EHRs were associated with better rates of cholesterol testing, this did not translate to improvements in patients’ actual cholesterol levels. 9 In another study of ambulatory diabetes care in clinics with and without EHRs, patients at EHR enabled clinics actually did worse in rates of meeting 2 year hemoglobin A1c, cholesterol, and blood pressure goals. 30 Furthermore, data suggest that EHR implementation may actually increase the amount of time spent by patients during clinic visits. 31 These studies suggest that EHRs, with their increased documentation requirements, can have unintended consequences, including clinic inefficacy. All of these studies highlight the complexity of quality of care. Accurate documentation and billing is easily obtainable with EHRs but improving recognition of clinical problems and changing provider practice is much more challenging.
Our study does have some limitations. This study relied on administrative data derived from billing claims data, which has limited information on patients’ treatment courses that can affect outcomes. Additionally, this study uses hospital survey data to identify the level or EHR adoption, which is prone to reporting errors. Furthermore, changes in quality of care after the implementation of EHRs may be attributable in part to non-EHR factors, which cannot be fully accounted for in our analysis.
This population-based study builds on existing literature to demonstrate that EHRs are not yet associated with gains in measures of inpatient mortality, readmissions, and PSIs. Results here suggest that differences in outcomes at hospitals with different levels of EHR utilization may be attributable to other patient and hospital factors rather than EHR utilization itself. As federal incentives encourage EHR adoption and hospitals strive for meaningful use, it will be important to further characterize the benefits received from EHRs.
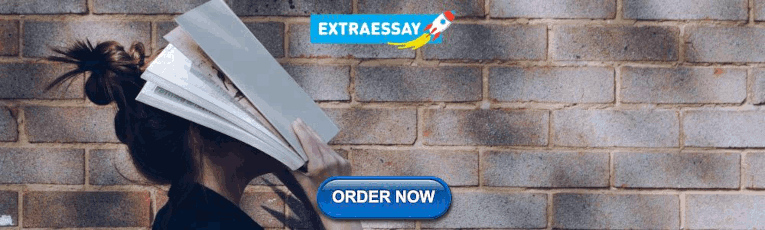
Acknowledgements
The authors thank National Cancer Institute of the National Institutes of Health under Award Number R01CA183962 and Agency for Healthcare Research and Quality (grant number R01HS024096) for the support. The content is solely the responsibility of the authors and does not necessarily represent the official views of the National Institutes of Health and Agency for Healthcare Research and Quality.
- Cited Here |
- PubMed | CrossRef
- View Full Text | PubMed | CrossRef
- + Favorites
- View in Gallery
Readers Of this Article Also Read
Anger and aggression research: a bibliometric analysis from 2012 to 2022, clinical significance of hepatic function in graves disease with type 2..., knowledge of and willingness to perform hands-only cardiopulmonary..., novel electronic health record (ehr) education intervention in large healthcare ..., acute thrombosis of a transplanted renal artery after gastric ulcer bleeding in ....

An official website of the United States government
The .gov means it’s official. Federal government websites often end in .gov or .mil. Before sharing sensitive information, make sure you’re on a federal government site.
The site is secure. The https:// ensures that you are connecting to the official website and that any information you provide is encrypted and transmitted securely.
- Publications
- Account settings
- My Bibliography
- Collections
- Citation manager
Save citation to file
Email citation, add to collections.
- Create a new collection
- Add to an existing collection
Add to My Bibliography
Your saved search, create a file for external citation management software, your rss feed.
- Search in PubMed
- Search in NLM Catalog
- Add to Search
Quality of nursing documentation: Paper-based health records versus electronic-based health records
Affiliation.
- 1 Jordan University of Science and Technology, Irbid, Jordan.
- PMID: 28981172
- DOI: 10.1111/jocn.14097
Aims and objectives: To assess and compare the quality of paper-based and electronic-based health records. The comparison examined three criteria: content, documentation process and structure.
Background: Nursing documentation is a significant indicator of the quality of patient care delivery. It can be either paper-based or organised within the system known as the electronic health records. Nursing documentation must be completed at the highest standards, to ensure the safety and quality of healthcare services. However, the evidence is not clear on which one of the two forms of documentation (paper-based versus electronic health records is more qualified.
Methods: A retrospective, descriptive, comparative design was used to address the study's purposes. A convenient number of patients' records, from two public hospitals, were audited using the Cat-ch-Ing audit instrument. The sample size consisted of 434 records for both paper-based health records and electronic health records from medical and surgical wards.
Results: Electronic health records were better than paper-based health records in terms of process and structure. In terms of quantity and quality content, paper-based records were better than electronic health records. The study affirmed the poor quality of nursing documentation and lack of nurses' knowledge and skills in the nursing process and its application in both paper-based and electronic-based systems.
Conclusion: Both forms of documentation revealed drawbacks in terms of content, process and structure. This study provided important information, which can guide policymakers and administrators in identifying effective strategies aimed at enhancing the quality of nursing documentation.
Relevance to clinical practice: Policies and actions to ensure quality nursing documentation at the national level should focus on improving nursing knowledge, competencies, practice in nursing process, enhancing the work environment and nursing workload, as well as strengthening the capacity building of nursing practice to improve the quality of nursing care and patients' outcomes.
Keywords: content; electronic health records and audit instruments; paper-based; process; quality of nursing documentation; structure.
© 2017 John Wiley & Sons Ltd.
PubMed Disclaimer
Similar articles
- The quality of paper-based versus electronic nursing care plan in Australian aged care homes: A documentation audit study. Wang N, Yu P, Hailey D. Wang N, et al. Int J Med Inform. 2015 Aug;84(8):561-9. doi: 10.1016/j.ijmedinf.2015.04.004. Epub 2015 May 8. Int J Med Inform. 2015. PMID: 26004340
- Description and comparison of documentation of nursing assessment between paper-based and electronic systems in Australian aged care homes. Wang N, Yu P, Hailey D. Wang N, et al. Int J Med Inform. 2013 Sep;82(9):789-97. doi: 10.1016/j.ijmedinf.2013.05.002. Epub 2013 Jun 17. Int J Med Inform. 2013. PMID: 23786709
- Nursing documentation audit--the effect of a VIPS implementation programme in Denmark. Darmer MR, Ankersen L, Nielsen BG, Landberger G, Lippert E, Egerod I. Darmer MR, et al. J Clin Nurs. 2006 May;15(5):525-34. doi: 10.1111/j.1365-2702.2006.01475.x. J Clin Nurs. 2006. PMID: 16629961
- Quality of nursing documentation and approaches to its evaluation: a mixed-method systematic review. Wang N, Hailey D, Yu P. Wang N, et al. J Adv Nurs. 2011 Sep;67(9):1858-75. doi: 10.1111/j.1365-2648.2011.05634.x. Epub 2011 Apr 6. J Adv Nurs. 2011. PMID: 21466578 Review.
- Electronic nursing documentation as a strategy to improve quality of patient care. Kelley TF, Brandon DH, Docherty SL. Kelley TF, et al. J Nurs Scholarsh. 2011 Jun;43(2):154-62. doi: 10.1111/j.1547-5069.2011.01397.x. Epub 2011 Apr 11. J Nurs Scholarsh. 2011. PMID: 21605319 Review.
- Does the health information system in Jordan support equity to improve health outcomes? Assessment and recommendations. Alnawafleh AH, Rashad H. Alnawafleh AH, et al. Arch Public Health. 2024 Apr 12;82(1):48. doi: 10.1186/s13690-024-01269-6. Arch Public Health. 2024. PMID: 38610051 Free PMC article.
- Surgical safety checklist compliance process as a moral hazard: An institutional ethnography. Facey M, Baxter N, Hammond Mobilio M, Peter E, Moulton CA, Paradis E. Facey M, et al. PLoS One. 2024 Feb 26;19(2):e0298224. doi: 10.1371/journal.pone.0298224. eCollection 2024. PLoS One. 2024. PMID: 38408085 Free PMC article.
- Integrating Software Into Hospital Quality Structures: What Practices Do Pharmaceutical Inspector Co-Operation Scheme (PIC/S) Guidelines Recommend? Scione F, Abdelmaula KY, Balzan D, Falzon S, Sultana J. Scione F, et al. J Pharm Technol. 2024 Feb;40(1):50-54. doi: 10.1177/87551225231210723. Epub 2023 Nov 14. J Pharm Technol. 2024. PMID: 38318253 No abstract available.
- Nurses' Documentation Practice and Associated Factors in Eight Public Hospitals, Amhara Region, Ethiopia: A Cross-Sectional Study. Molla F, Temesgen WA, Kerie S, Endeshaw D. Molla F, et al. SAGE Open Nurs. 2024 Jan 23;10:23779608241227403. doi: 10.1177/23779608241227403. eCollection 2024 Jan-Dec. SAGE Open Nurs. 2024. PMID: 38268952 Free PMC article.
- Transforming cancer clinical trials: The integral role of artificial intelligence in electronic health records for efficient patient recruitment. Nashwan AJ, Hani SB. Nashwan AJ, et al. Contemp Clin Trials Commun. 2023 Nov 7;36:101223. doi: 10.1016/j.conctc.2023.101223. eCollection 2023 Dec. Contemp Clin Trials Commun. 2023. PMID: 38034843 Free PMC article.
Publication types
- Search in MeSH
LinkOut - more resources
Full text sources, other literature sources.
- scite Smart Citations
Miscellaneous
- NCI CPTAC Assay Portal
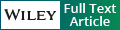
- Citation Manager
NCBI Literature Resources
MeSH PMC Bookshelf Disclaimer
The PubMed wordmark and PubMed logo are registered trademarks of the U.S. Department of Health and Human Services (HHS). Unauthorized use of these marks is strictly prohibited.
Click through the PLOS taxonomy to find articles in your field.
For more information about PLOS Subject Areas, click here .
Loading metrics
Open Access
Peer-reviewed
Research Article
Mapping evidence on the factors contributing to long waiting times and interventions to reduce waiting times within primary health care facilities in South Africa: A scoping review
Roles Conceptualization, Data curation, Formal analysis, Investigation, Methodology, Writing – original draft, Writing – review & editing
* E-mail: [email protected] , [email protected]
Affiliations Discipline of Public Health Medicine, School of Nursing and Public Health, University of KwaZulu-Natal, Durban, South Africa, Programme of Bio & Research Ethics and Medical Law, Nelson R Mandela School of Medicine & School of Nursing and Public Health, College of Health Sciences, University of KwaZulu-Natal, Durban, South Africa

Roles Conceptualization, Data curation, Investigation, Methodology, Supervision, Validation, Writing – original draft, Writing – review & editing
Affiliations Discipline of Public Health Medicine, School of Nursing and Public Health, University of KwaZulu-Natal, Durban, South Africa, Cancer & Infectious Diseases Epidemiology Research Unit (CIDERU), College of Health Sciences, University of KwaZulu-Natal, Durban, South Africa
Roles Conceptualization, Data curation, Formal analysis, Investigation, Methodology, Supervision, Validation, Writing – original draft, Writing – review & editing
Affiliation Programme of Bio & Research Ethics and Medical Law, Nelson R Mandela School of Medicine & School of Nursing and Public Health, College of Health Sciences, University of KwaZulu-Natal, Durban, South Africa
- Ugochinyere I. Nwagbara,
- Khumbulani W. Hlongwana,
- Sylvester C. Chima
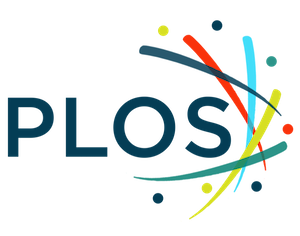
- Published: August 21, 2024
- https://doi.org/10.1371/journal.pone.0299253
- Peer Review
- Reader Comments
Globally, reduction of patient waiting time has been identified as one of the major characteristics of a functional health system. In South Africa, 83% of the general population visiting primary healthcare (PHC) facilities must contend with long waiting times, overcrowding, staff shortages, poor quality of care, an ineffective appointment booking system, and a lack of medication. These experiences may, in turn, affect how patients view service quality.
This scoping review was guided by Arksey and O’Malley methodological framework. The primary literature search of peer-reviewed and review articles was achieved through PubMed/MEDLINE, Google Scholar, Science Direct, and World Health Organization (WHO) library databases, using waiting times, outpatient departments, factors, interventions, and primary healthcare facilities as keywords. Two independent reviewers screened abstracts and full articles, using the set inclusion and exclusion criteria. We used NVIVO ® version 10 software to facilitate thematic analysis of the results from included studies.
From the initial 250 records screened, nine studies were eligible for inclusion in this scoping review. Seven papers identified the factors contributing to waiting time, and five papers mentioned effective interventions implemented to reduce waiting times within PHC facilities. Our analysis produced three (patient factors, staff factors, and administrative systems) and two (manual-based waiting time reduction systems and electronic-based waiting time reduction systems) main themes pertaining to factors contributing to long waiting times and interventions to reduce waiting times, respectively.
Our results revealed that the patients, staff, and administrative systems all contribute to long waiting times within the PHC facilities. Patient waiting times recorded a wider and more evenly spread patient arrival pattern after the identified interventions in our study were implemented. There is a need to constantly strategize on measures such as implementing the use of an electronic appointment scheduling system and database, improving staff training on efficient patient flow management, and regularly assessing and optimizing administrative processes. By continuously monitoring and adapting these strategies, PHC facility managers can create a more efficient and patient-centered healthcare experience.
Citation: Nwagbara UI, Hlongwana KW, Chima SC (2024) Mapping evidence on the factors contributing to long waiting times and interventions to reduce waiting times within primary health care facilities in South Africa: A scoping review. PLoS ONE 19(8): e0299253. https://doi.org/10.1371/journal.pone.0299253
Editor: Arun Kumar Sharma, University College of Medical Sciences, INDIA
Received: January 23, 2023; Accepted: February 7, 2024; Published: August 21, 2024
Copyright: © 2024 Nwagbara et al. This is an open access article distributed under the terms of the Creative Commons Attribution License , which permits unrestricted use, distribution, and reproduction in any medium, provided the original author and source are credited.
Data Availability: All relevant data are within the paper and its Supporting information files.
Funding: The first author (UIN) received a Tuition Remission for her Ph.D. studies from UKZN and partial scholarship funding from the College of Health Sciences (CHS), UKZN, to support her PhD studies. The funders had no role in study design, data collection and analysis, decision to publish, or preparation of the manuscript.
Competing interests: The authors have declared that no competing interests exist.
Abbreviations: CHC, Community Health Centre; CTS, Cape Triage Score; LMICs, Low-and-Middle-Income Countries; MESH, Medical Subject Headings; MMAT, Mixed Method Appraisal Tool; PCC, Population Content Context; PHC, Primary Health Care; PRISMA-ScR, Preferred Reporting Items for Systematic Reviews and Meta-Analyses Extension for Scoping Reviews; SCREEN, Sick Children Require Emergency Evaluation Now; WHO, World Health Organization
Introduction
Globally, successful reduction of patient waiting times has been identified as one of the major characteristics of a functional health system [ 1 ]. South Africa’s public health sector is grappling with long waiting times in primary health care (PHC) facilities, which undermine the functionality of health systems. Consequently, these long waiting times culminate into a range of issues, such as patients’ dissatisfaction with care, interruption of hospital work patterns, impaired access to care and increased medical errors, and patients having to skip appointments, resulting in them moving from one clinic to the other in search of shorter waiting times [ 2 – 6 ]. Patient waiting time is defined as the amount of time spent by patients seeking care at healthcare units before being attended to for consultation and treatment [ 7 – 9 ].
In South Africa, 83% of the general population visiting the PHC facilities have to contend with long waiting times, attributable to overcrowding, staff shortages, poor care quality, an ineffective appointment booking system, a lack of medication, inadequate infrastructure, a lack of appropriate medical equipment, and poor data management [ 10 , 11 ]. Reducing long waiting times and overcrowding is crucial for preventing the spread of infections [ 12 ]. It has been reported that staff shortages caused by attending training or meetings during working hours also contribute to patients’ extended waiting times in PHC facilities [ 13 ].
Given the universal nature of long waiting times, the United States Institute of Medicine (IOM) recommends that at least 90% of patients should be seen within 30 minutes of their scheduled appointment time [ 9 , 14 ]. However, this is not the case in most low-and-middle-income countries (LMICs), and South Africa is no exception as several studies have shown that patients spend between 2–4 hours in outpatient departments before seeing a doctor [ 15 , 16 ]. Long waiting times negatively affect the overall quality of care, patient safety and satisfaction, and clinic staff morale, and compromise the doctor-patient relationship [ 8 ]. In some instances, children brought to PHC centers by carers wait nearly the whole day, only to be turned away at closing time without being seen by any healthcare worker (HCW) [ 17 ]. The South African National Department of Health (NDoH) has identified waiting time as one of the six priority areas for improving quality of care [ 18 ].
Globally, studies have demonstrated a strong inverse relationship between patient satisfaction and waiting times [ 19 – 23 ]. Patients often spend more time waiting than consulting with healthcare providers [ 3 ].
A multidisciplinary team approach remains among the key approaches to rekindle hope that reduced wait times, improved health outcomes, and enhanced patient satisfaction, can be achieved [ 24 ]. It is, however, evident that long waiting time compromises the functionality of health system, yet there is dearth of research on the factors contributing to long waiting times and interventions implemented to reduce waiting times within PHC facilities in South African context. The results of this study will not only be an important contribution to the body of evidence on waiting times and related interventions but may be crucial for designing healthcare delivery and patient satisfaction interventions in South Africa and similar settings.
Scoping review
We conducted a scoping review study. Due to its ability to facilitate the mapping of new concepts, and types of evidence and identifies related gaps [ 25 ]. This review was guided by the Arksey and O’Malley methodological framework [ 26 ]. The framework involves (i) identifying the research question, (ii) identifying relevant studies, (iii) study selection, (iv) charting the data, and (v) collating, summarising, and reporting results. The review protocol has been registered with the Open Science Framework database (registration number: osf.io/fpzwu ). We used the Preferred Reporting Items for Systematic Reviews and Meta-Analyses extension for Scoping Reviews (PRISMA-ScR) checklist to ensure each step in the scoping review process was standardized [ 27 ] ( S1 Appendix ).
Ethical approvals
This study was approved by the UKZN Biomedical Research Ethics Committee (BREC) approval number BREC/00003637/2021.
Identifying the research question
The general research question was: “What is known from the existing literature on the factors contributing to long waiting times and interventions to reduce waiting times within PHC facilities in South Africa?”
Sub-questions were:
- Which factors contribute to long waiting times within PHC facilities in South Africa?
- Which interventions have been implemented to reduce waiting times within PHC facilities in South Africa?
Eligibility criteria
- The Population-Concept-Context (PCC) framework [ 28 ] was used to determine the eligibility of the research question as illustrated below ( Table 1 ).
- PPT PowerPoint slide
- PNG larger image
- TIFF original image
https://doi.org/10.1371/journal.pone.0299253.t001
Inclusion and exclusion criteria
We included original research articles reporting information regarding factors contributing to long waiting times and interventions to reduce waiting times within PHC facilities in South Africa. Articles addressing the research question and utilising any study design published in peer-reviewed journals and in grey literature (research or information material that is not widely published or available and usually does not go through the peer-review process including a wide range of documents and sources, such as reports, theses, conference proceedings, working papers, government documents, non-profit organization publications, patents, and unpublished research studies), were sourced. Articles written in English and published from January 2010 until October 2022 were considered. We excluded case studies, commentaries, editorials, and correspondences. Non-English studies were excluded as previous studies showed that it had a minimal effect on the overall conclusions [ 29 , 30 ].
Literature search
Search terms used were waiting times, outpatient department, factors, interventions, primary healthcare facilities, and South Africa, through PubMed/MEDLINE, Google Scholar, Science Direct, World Health Organization (WHO) library databases, and grey literature. The search strategy was piloted to check the appropriateness of selected electronic databases and keywords. Consistency across databases was maintained where possible. Boolean terms (AND, OR) were used to separate the keywords during the search. Medical Subject Headings (MeSH) terms were also included in the search ( S2 Appendix ).
Study selection
We conducted a comprehensive title screening; all studies that did not address the study’s research question were excluded, along with all the duplicates. All included studies for abstract screening were uploaded to Endnote X7 software. UKZN library services were sought for articles that were difficult to find, failing which we contacted authors directly to request full copies of the articles. The final Endnote database was shared among the review team for abstract screening, i.e., two independent reviewers screened the abstracts and full articles guided by the inclusion criteria. Any discrepancies in the results of the abstract screening were resolved through discussion until a consensus was reached. A third screener was consulted to resolve discrepancies in full-article screening results.
Charting the data
A standardized data extraction tool (Google Form) was used to extract data from all the variables that focused on answering the research questions, namely: author and date, study population, study design, study setting, factors, and interventions. The data extraction tool was piloted by all the reviewers to confirm consistency. The data was extracted by the first author (UIN) and underwent assessment by another reviewer (KWH) independently.
Collating, summarising and reporting the results
This study mapped the existing evidence and summarised the findings as presented across articles. A narrative account of the data extracted from the included studies was analysed using thematic analysis, which is a method that is often used to analyse data in primary qualitative research [ 31 ]. We used NVIVO ® software version 10 to code the data from the included studies. Emerging themes were identified, and data was coded according to these themes. Data was extracted around the following outcomes: factors contributing to long waiting times and interventions to reduce waiting times. Where the presented data was ambiguous or missing, the corresponding authors were contacted for clarification on the process of data extraction.
Our search yielded 1021 articles, but after title screening and elimination of duplicates, two hundred and fifty (250) eligible studies were retained. Two hundred (200) articles were excluded after abstract screening, thus reducing the number of articles eligible for full article screening to fifty (50) articles. After the full article screening, forty-one (41) articles were excluded, and the remaining nine (9) articles were included for data extraction. Results of the articles screened are presented in ( Fig 1 ).
https://doi.org/10.1371/journal.pone.0299253.g001
Characteristics of the included studies
All the included studies were conducted in PHC facilities in South Africa (comprising primary healthcare centres and community healthcare centres (CHCs). Seven papers identified the factors contributing to waiting time [ 33 – 39 ], and five papers mentioned effective interventions implemented in the reduction of waiting times within PHC facilities [ 33 , 34 , 39 – 41 ]. The characteristics of the included studies are illustrated in ( Table 2 ). The study findings have been classified into three main themes: patient factors, staff factors, and administrative systems.
https://doi.org/10.1371/journal.pone.0299253.t002
Factors contributing to long waiting time in PHC facilities
Patient factors.
Access to the facility, through public transport, was a major factor that affected the proper implementation of the appointment scheduling system, as some of the surveyed facilities were in locations with poor access to public transport. This resulted in patients always arriving very early in the morning before the clinic opening time, regardless of their appointment time slot, thereby causing a long queue [ 34 – 36 , 38 ]. The fluctuating patient numbers was another important factor that influenced waiting time as some days witnessed higher patient volume than others and the pattern was less predictable [ 37 ]. Patients’ lack of adherence to the booking system at the CHC resulted in long waiting times and patient overload [ 38 ]. A study showed that the possible reason for patients’ waiting times was because some patients had to see a physician and a professional nurse on the same day and so had to book physicians’ appointments for specific days thereby causing delays [ 33 ].
Staff factors
A before and after study reported that factors associated with long waiting times were due to high work volume [ 36 , 39 ], there was also a lack of efficiency whereby staff members at the service points were busy with something else other than attending to patients while they were waiting, a mismatch where patients are available to be attended to but staff members were not at the service point, queuing problems where patients do not queue in the correct order and staff are not attending to patients in the order that they arrive at the service point, and inappropriate high service time [ 36 ]. A study showed that a shortage of staff contributed to long waiting times and the staff on duty arrived late and did not start working as soon as they arrived [ 37 , 39 ]. Staff meetings during clinic operating hours also influenced waiting times [ 37 ]. Poor time management was another factor, whereby the staff arrived at work late, had unauthorized extensions of meal breaks, preoccupied with non-work related matters and departed before the work closing hour [ 38 ]. A study reported that communication was another factor that influenced waiting time; hence, few of the patients were foreign nationals and did not understand the local language(s). The lack of understanding and poor communication resulted in the women feeling lost and confused, thereby causing a delay in their antenatal care activities [ 37 , 39 ].
Staff attitude and behaviours were seen to contribute to extended clinic visits as the staff worked at a slow pace and had conversations with their colleagues during consultations [ 37 , 39 ]. Patients reported that unfavourable staff attitudes discouraged them from visiting the clinic, and poor impressions of staff attitudes impacted assessments of services [ 39 ].
Administrative systems
The use of an appointment scheduling system, such as diaries and notebooks, to schedule clinic appointments was ineffective, as patients were often overbooked because of the limited pages on the notebooks and diaries. This results in overbooking as the facilities are not able to determine upfront how many patients they have already scheduled for a particular day [ 34 ]. Health service challenges included difficulty in securing appointments, administrative mistakes (especially lost clinic folders and test results), difficulty navigating the clinic system (e.g., failure to collect a queue card or waiting for incorrect services), and negative clinic-patient interactions [ 35 ]. A logistical problem due to the lack of equipment or available rooms to attend to patients, flow problems where staff are available to see patients, and the patients get delayed at some other service point [ 36 , 37 ]. The biggest contributor to waiting time was that follow-up visits were seen before the women who arrived for their initial visits [ 37 ]. Baron and Kaura [ 37 ], stated that patient flow increased long waiting times, and this is a result of a poor queuing system, a lack of signage, and the ineffective orientation of patients within healthcare facilities. The unavailability of equipment negatively impacted on waiting time [ 37 ]. The inefficiencies in the records and patient administration systems contributed to long waiting times in the PHC facilities [ 38 ].
Interventions to reduce waiting times in PHC facilities
Five studies included in this scoping review showed the interventions implemented in PHC facilities to reduce waiting time [ 33 , 34 , 39 – 41 ]. The interventions have been classified into two themes: Manual-based waiting time reduction systems and electronic-based waiting time reduction systems.
Manual-based waiting time reduction system
A study showed that the implementation of the Cape Triage Score (CTS) system in emergency departments in the Northwest province of South Africa reduced waiting times, and referral was much quicker to the correct health professionals and the hospitals [ 33 ]. The patients were triaged by professional nurses with different codes based on the outcomes of their vital signs or the condition of the patient. Stickers were placed on each patient’s hand and file. Patients with a red colour code were sent to professional nurses and physicians immediately, and those with an orange colour code were seen by professional nurses within 10 minutes. Patients with yellow colour codes were seen within 60 min, and patients with green colour codes had to be seen within 240 minutes. The blue colour code pertained to patients who had passed away and needed certification [ 33 ].
A qualitative study in the eThekwini district showed that the Fast Queue strategy was also effective in addressing the overcrowding problem in a health facility among healthcare users [ 39 ]. The users reported that they were attended to quickly as the fast queue enabled them to be separated into different queues, and mothers of young babies liked the fact that they were seated away from the sick people, thus protecting their children from possible infections [ 39 ].
Electronic-based waiting time reduction system
A positive change in waiting time reduction was observed when a patient appointment scheduling system was successfully implemented [ 34 ]. Facilities that improved their patient waiting time recorded a wider and more evenly spread patient arrival pattern after the intervention compared with before [ 34 ].
The implementation of the Sick Children Require Emergency Evaluation Now (SCREEN) tool on the waiting times of critically ill children reduced waiting times for all critically ill children and had a positive impact on the left without being seen (LWBS) rates in all clinics [ 40 ]. A quick response (QR) code was placed on the child’s clothing before they entered the clinic and joined the queue. A QR code is a machine-readable code consisting of an array of black and white squares. The nurses were asked to scan the QR codes to record the Integrated Management of Childhood Illnesses (IMCI) category as (red/yellow/green) to delineate each child’s severity of illness [ 40 ].
A study showed that a simple screening tool implemented for use by laypersons significantly reduced waiting time for critically ill children in primary healthcare clinics [ 41 ].
Our study identified and classified three major factors contributing to waiting time as patient factors, staff factors, and administrative systems, and the interventions that were implemented successfully in reducing waiting times within PHCs were grouped into two main themes: manual-based waiting time reduction systems and electronic-based waiting time reduction systems. The included studies showed that most patients usually arrive and queue outside the clinics very early before the clinic doors or gates are opened [ 34 – 36 , 38 ]. In South Africa, it’s typical to arrive early and wait in queue outside of clinics. This problem is attributable to the lack of an electronic appointment scheduling system [ 42 ], or poor access to the facility using public transport, given that the majority of the users of public healthcare facilities rely on public transport, which often operates at rigid times, such as the early mornings and afternoons in most semi-urban (townships) and rural areas of South Africa [ 34 ]. This also poses security risks, in addition to discomforts that may be imposed by inclement weather. A study by Egbujie et al. [ 34 ] showed that some facilities recorded an evenly spread patient arrival pattern through proper appointment scheduling, and this reduced the daily overbooking of patients and ensured an even spread of booked patients throughout the day [ 34 ]. Patients not honouring their appointments as scheduled impacted adversely on patients’ waiting time [ 38 ]. A before and after study by Daniels et al. [ 36 ], stated that scheduling appointments for quieter times and days in the week and encouraging patients to come at less busy times in the day also reduced waiting times. There is, therefore, a need for urgent scale-up of electronic appointment scheduling systems in all PHCs in South Africa, as it will significantly reduce patient waiting times.
Lack of signage and a poor queuing system reduced patient flow and increased waiting time, as previously reported [ 37 ]. Staff attitudes and behaviours were seen to contribute to extended clinic visits, as some patients stated that negative staff attitudes deterred them from visiting the clinic [ 37 , 39 ]. Continued in-service training for healthcare workers will help improve effective communication and patient satisfaction. Staff reporting late for work, having unauthorized meal breaks, preoccupied with non-work-related matters, and leaving work ahead of time [ 36 , 38 ]. Healthcare workers need to prioritise attending to patients as soon as they arrive, report to work timeously, and close at the appropriate time.
The language barrier was reported to be a factor causing a delay, as few patients were foreign nationals and did not understand the health workers. The lack of understanding and poor communication resulted in the women feeling lost and confused [ 37 ], hence, healthcare workers should be accommodating and use the English language for foreign nationals.
Our study identified some interventions that were successfully implemented in reducing waiting times within primary healthcare facilities, and they were grouped into two main themes: manual-based waiting time reduction systems and electronic-based waiting time reduction systems. The manual-based intervention system showed a significant reduction in waiting time, as triage was applied and professional nurses were able to prioritise attending to more seriously ill patients, resulting in quicker referral to the correct health professionals [ 33 ]. Furthermore, the use of fast queues and queue marshals helped direct users to their respective queues and reduced waiting time [ 39 ]. The use of multiple, managed queues was generally well-received by attendees, according to a qualitative study on the use of a "Fast Queue" in KZN clinics. This was especially true if there was smooth (i.e., unidirectional) flow and effective communication with health workers [ 39 ].
The electronic-based waiting time reduction system showed a positive change in waiting time reduction when the patient appointment scheduling system was properly implemented [ 34 ]. Facilities that improved their patient waiting times recorded a wider and more evenly spread patient arrival pattern after the intervention [ 34 ]. The SCREEN program enabled the quick identification of critically ill children and expedited their care at the point of entry into a clinic, and this significantly reduced waiting times for critically ill children in PHC clinics [ 40 , 41 ].
PHC and community health center (CHC) facility managers may need to constantly strategize on measures to reduce patient waiting times, educate the staff on the best way to approach patients, to increase patient satisfaction and quality of care.
Strengths and limitations
To our knowledge, this is the first scoping review to map evidence on the factors contributing to long waiting times and interventions that have been implemented successfully in reducing waiting times within primary healthcare facilities in South Africa. A few studies were included after a thorough systematic search strategy was conducted. There is still, however, the possibility that relevant articles were omitted. Nevertheless, the absence of some relevant articles does not significantly impact the findings of this study. Future studies may be directed at other jurisdictions and developing countries in Africa and elsewhere, as well as studies designed to evaluate the impact of measures designed to reduce patient waiting at public healthcare facilities.
Conclusions
There was a paucity of studies focusing on factors contributing to long waiting times and interventions implemented to reduce waiting time in primary healthcare facilities in South Africa. Our study findings show the need for future primary research focusing on implementing the use of an electronic appointment scheduling system and database within the PHC facilities for effective patient administrative records, as patients faced difficulties in securing appointments and administrative mistakes occurred due to lost clinic folders and test results. There is a need for continuous training to improve staff attitudes and behaviours as it will positively reduce waiting time, and improve quality of care, and patient satisfaction.
Supporting information
S1 appendix. preferred reporting items for systematic reviews and meta-analyses extension for scoping reviews (prisma-scr) checklist..
https://doi.org/10.1371/journal.pone.0299253.s001
S2 Appendix. PubMed search strategy.
https://doi.org/10.1371/journal.pone.0299253.s002
- 1. National Department of Health South Africa. National Development Plan 2030: Draft National Guideline for Management of Patient waiting Times at Health Facilities. Jan 2019.
- View Article
- Google Scholar
- PubMed/NCBI
- 4. Chima SC. A primer on medical law, bioethics and human rights for African scholars: Chimason Educational Books; 2011.
- 12. World Health Organization. WHO Policy on TB Infection Control in Health-Care Facilities, Congregate Settings and Households. Reducing transmission of TB in health-care facilities. https://www.ncbi.nlm.nih.gov/books/NBK179241/ . 2009.
- 14. Institute of Medicine. Transforming Health Care Scheduling and Access: Getting to now. Washington, DC: The National Academies Press. 2015.
- 16. Ofili A, Ofovwe C. Patients’ assessment of efficiency of services at a teaching hospital in a developing country. 2005.
- 18. National Department of Health South Africa. National Core Standards for Health Establishments in South Africa (Abridged version), NDoH 2011.
- 28. Peters M, Godfrey C, McInerney P, Soares C, Khalil H, Parker D. The Joanna Briggs Institute reviewers’ manual 2015: methodology for JBI scoping reviews. 2015.

An official website of the United States government
The .gov means it’s official. Federal government websites often end in .gov or .mil. Before sharing sensitive information, make sure you’re on a federal government site.
The site is secure. The https:// ensures that you are connecting to the official website and that any information you provide is encrypted and transmitted securely.
- Publications
- Account settings
Preview improvements coming to the PMC website in October 2024. Learn More or Try it out now .
- Advanced Search
- Journal List
- Int J Environ Res Public Health
- PMC10970652

Trends and Patterns in Electronic Health Record Research (1991–2022): A Bibliometric Analysis of Australian Literature
Hongmei xie.
1 College of Business Government and Law, Flinders University, Adelaide, SA 5042, Australia; [email protected] (P.B.); [email protected] (M.B.)
Andreas Cebulla
2 Australian Industrial Transformation Institute, College of Business Government and Law, Flinders University, Adelaide, SA 5042, Australia; [email protected]
Peivand Bastani
Madhan balasubramanian.
3 Menzies Centre for Health Policy and Economics, School of Public Health, Faculty of Medicine and Health, The University of Sydney, Camperdown, NSW 2050, Australia
Associated Data
The data presented in this study are available upon request from the corresponding author.
Electronic Heath Records (EHRs) play vital roles in facilitating streamlined service provision and governance across the Australian health system. Given the recent challenges due to the COVID-19 pandemic, an ageing population, health workforce silos, and growing inefficiencies in traditional systems, a detailed historical analysis of the use of EHR research in Australia is necessary. The aim of this study is to examine the trends and patterns in EHR research in Australia over the past three decades by employing bibliometric methods. A total of 951 articles published in 443 sources were included in the bibliometric analysis. The annual growth rate of EHR research in Australia was about 17.1%. Since 2022, the main trending topics in EHR research were COVID-19, opioid usage, and natural language processing. A thematic analysis indicated aged care, clinical decision support systems, cardiovascular disease, drug allergy, and adverse drug reaction as the “hot” themes in EHR research in Australia. This study reveals a significant uptrend in EHR research in Australia, highlighting the evolving intellectual and collaborative landscape of this interdisciplinary field. The data also provide guidance for policymakers and funding institutions in terms of the most significant contributions and key fields of research while also holding public interest.
1. Introduction
The COVID-19 pandemic has substantially accelerated the adoption of healthcare technology, including the use of electronic health records (EHRs) in health and aged care systems in Australia [ 1 , 2 , 3 , 4 , 5 , 6 ]. In general, an EHR system is an integrated aggregate of patients’ health records developed and maintained by a variety of different healthcare organizations and kept in an electronic format [ 7 ]. EHRs contribute towards improving patient safety, raising the quality of care, increasing patient monitoring, and lowering healthcare costs. Despite the fact that EHR usage in many parts of the world has encountered several challenges in terms of interoperability, privacy, and ethical issues, EHRs play a significant role in meeting the ever-changing and demanding healthcare needs in the growing digital health ecosystem [ 8 , 9 ].
Over the past three decades, EHRs have evolved from transaction-focused systems to more intelligence-powered patient-centric systems [ 10 ]. Particularly in the past ten years, we have been seeing a rapid shift in the dominant design in EHR systems globally, which is mainly due to maturity and the successful implementation of Artificial Intelligence (AI) in the medical arena, as well as the availability of Big Data [ 1 , 11 , 12 , 13 , 14 ]. Patient engagement, interoperability, and predictive analytics have become key aspects in EHR system design. Through EHRs, patients can not only receive information such as reminders, alerts, and test results, but they can also share information and interact with health professionals through health monitoring devices or virtual technologies [ 2 , 15 , 16 , 17 , 18 ]. Interoperability is another area that has been important in patient-centred EHR design [ 19 , 20 ]. Previously, EHRs were usually created and maintained by a single provider. As a consequence of this, medical records have traditionally been kept in compartmentalized locations and computer databases; this presented challenges to the process of information sharing within a patient-centred integrated healthcare system [ 7 , 21 ]. Today, with the advent of Fast Healthcare Interoperability Resources (FHIR) and other consortia like Argonaut and the CARIN Alliance, the door is open to a better coordinated system through standardized Application Programming Interfaces (APIs) [ 22 , 23 , 24 ]. Predictive analytics and clinical decision support systems have also been able to draw actionable insights from the EHR system to inform our health services and individual health outcomes [ 25 , 26 , 27 ].
While EHR research has been undergoing an uptrend globally, there are few studies (mainly reviews or commentaries) that have systematically examined the growth and development of EHR research in Australia. EHR research in Australia has been reported in the literature for a variety of age groups, including children, adolescents, and older people; for a variety of health conditions, including cancer and heart diseases; and in a variety of care settings, including intensive care units and aged care facilities. The growth in EHR research in Australia requires considerable study to both understand the trends in key areas of research as well as to note hot topics and niche research areas. Consequently, the aim of this paper is to illustrate the trends in EHR research in Australia through a bibliometric analysis of the scientific literature. This paper will examine the trends and patterns in scientific outputs of EHR research and investigate frontiers in the context of Australia using publications from 1991 to 2022. This study provides insights into the EHR research landscape in Australia and will also help policymakers identify areas of EHR focus that are necessary for informed policy decisions in healthcare.
2. Materials and Methods
This study employs bibliometric methods to investigate the trends and patterns in Australian literature on electronic health records (EHRs) by conducting both a descriptive analysis and “performance analysis” [ 28 , 29 ] on the publications and using “science mapping” [ 30 , 31 ] techniques with knowledge structures. The analysis also provides a visualization of the data to assist in the identification of developing trends and hot topics. The purpose of a bibliometric analysis is to examine the multiple facets of a body of published literature using both quantitative and visual methods [ 32 , 33 , 34 ]. Bibliometric methods enable researchers to draw their conclusions on accumulated bibliographic data generated by other professionals in the field who express their ideas through citation, collaboration, and writing [ 35 ]. This method is also distinguished by its potential to play an essential part in the formulation of recommendations for public health, notably establishing guidelines or policies [ 36 ].
2.1. Search Strategy
A comprehensive systematic search was conducted utilising the Web of Science Core Collection (WoSCC) database, which was accessed through the Flinders University library. Web of Science is the world’s largest and most comprehensive academic information repository, encompassing the widest range of topics and fields possible, including the majority of the essential academic journals published in the field of EHRs [ 35 , 37 ]. The search was conducted and refined between November and December 2022. The data for this analysis were limited to the end of December 2022 using the WoSCC category with topic search (TS) shown in Box 1 .
The bibliometric search strategy.
- Concept 1: (electronic NEAR/2 Record*)
- Concept 2: (“australia*” OR “new south wales” OR “queensland*” OR “victoria” OR “south australia*” OR “tasmania*” OR “northern territory” OR “australian capital territory” OR “western australia” OR “brisbane” OR “sydney” OR “melbourne” OR “hobart” OR “adelaide” OR “darwin” OR “perth”)
- Concept #1 AND Concept #2
In Concept 1, the keywords were combined using “near/2” proximity operators to cover the commonly seen concepts “electronic health record*” OR “electronic medical record*” for the broadest and most relevant results. The search strategy also included using the Boolean operator “AND” to filter the results to the Australian context.
2.2. Data Processing
The initial search yielded 973 documents. To enhance the relevance of the analysed papers, specific selection criteria were implemented. Papers classified as letter, meeting abstract, editorial material, and news item were excluded, except when they met the classification criteria for article, early access, proceedings paper, or review article. In addition, papers published in languages other than English were excluded from the analysis. Therefore, 19 records were excluded from further analysis based on the above selection criteria. The remaining 954 documents were saved to the WoS Marked List. These documents were then exported to an Excel (version Excel for Microsoft 365) file for duplicate screening. Three duplicates were identified manually and removed from the collection. Sequentially, the WoS Marked List was updated with the final 951 documents as a source of truth for this EHR bibliometric study. To ensure transparency and accuracy, a PRISMA-style flowchart [ 38 ] was employed to outline the steps taken during the data gathering process (see Figure S1 , Supplementary Materials ), and two team members conducted the process independently.
2.3. Data Analysis and Visualisation Tools
These 951 documents from the Marked List of the data source were exported as a plain text file (available upon request) with the selection of the full records and cited references for analysis in R (version 4.2.2) and R Studio (version 2022.12.0). In this study, the analysis conducted through bibliometrix library in R and biblioshiny web interface provided a comprehensive view of the scientific landscape, revealing the key trends, themes, and influential individuals and organizations in terms of EHR research over the past three decades in Australia. Our selection of a 31-year time period was based on the beginning of EHR research in Australia as documented in the WoSCC database in the early 1990s.
Bibliometrix is an R-tool used for comprehensive science mapping analysis, while biblioshiny is a shiny-based web app for user-friendly bibliometric analysis [ 39 ]. The analysis conducted in biblioshiny involved four levels of domain analysis on top of the overview to the dataset, namely sources, authors, affiliations, and documents [ 39 ]. In this study, we particularly focus on a high-level overview for identifying trends and areas of prolific research. In addition to the four levels of domain analysis, the research team also conducted three structures of knowledge analysis through science mapping, including conceptual, intellectual, and social analyses. These analyses helped to identify the underlying themes, concepts, intellectual structures, and social networks that shape EHR research. By applying a clustering algorithm on the keyword network, it is possible to highlight the different themes of a given domain. Each cluster/theme can be represented on a particular plot known as a strategic or thematic map [ 40 ]. In a thematic map, Callon Centrality can be read as the importance of a theme in the entire research field, while Callon Density can be read as a measure of the theme’s development [ 41 , 42 ].
Throughout the bibliometric analysis, and after careful consideration, the team selected Author’s Keywords over Keywords Plus (the citation indexes’ coding used by Web of Science). The decision to use Author’s Keywords was based on the belief that they provided a more comprehensive and up-to-date representation of the themes than Keywords Plus [ 43 ], which can be influenced by unknown factors. A synonyms file was developed to capture repeated words/word meanings. The default parameters provided by the biblioshiny tool were employed for all bibliometric analysis, unless otherwise stated in the results.
3.1. Descriptive Analysis of the Publications
Table 1 presents a detailed summary of the EHR research trends in Australia based on an analysis of 951 documents published between 1991 and 2022 in WoSCC. The data reveal that the annual growth rate of EHR research in Australia is 17.14%, indicating a steady increase in research activity in this field over time. The average age of the documents in the collection is 5.98 years, suggesting that the research represented in the collection is relatively current. The average number of citations per document, which is 12.17, demonstrates the significant impact of the research and the widespread influence of the findings in the field. The trends of EHR publication and their citations can also be visualized in Figure 1 .

Trends in publications and citations from 1991 to 2022.
Summary characteristics of included studies.
Description | Results |
---|---|
Main information about data | |
Timespan | 1991:2022 |
Sources (journals, books, etc.) | 443 |
Documents | 951 |
Annual growth rate % | 17.14 |
Document’s average age | 5.98 |
Average citations per doc | 12.17 |
References | 25,752 |
Document contents | |
Keywords Plus (ID) | 1898 |
Author’s Keywords (DE) | 2434 |
Authors | |
Authors | 4289 |
Authors of single-authored docs | 28 |
Authors collaboration | |
Single-authored docs | 30 |
Co-authors per doc | 5.85 |
International co-authorships % | 19.77 |
Document types | |
article | 791 |
article; early access | 36 |
article; proceedings paper | 10 |
editorial material; early access | 1 |
proceedings paper | 76 |
review | 36 |
review; early access | 1 |
According to Figure S2 ( Supplementary Materials ) of the most relevant authors, affiliations, sources, and locally cited sources, Georgiou A stands out as the most prolific author with 27 published articles, and Westbrook JI and Yu P follow closely behind with 23 and 18 published articles, respectively. The figure also indicates that the University of Melbourne, the University of Sydney, and Monash University are the most prolific contributors to EHR research in Australia, with 218, 201, and 196 articles, respectively. These universities have emerged as the leading centres of EHR research and development in Australia, demonstrating their strength and depth of expertise in this area. Furthermore, this figure illustrates that the International Journal of Medical Informatics has the highest number of publications with 42 articles, followed by the Internal Medicine Journal with 35 articles, while Emergency Medicine Australasia and the Health Information Management Journal are also notable sources, having published 27 and 26 articles, respectively. The Medical Journal of Australia tops the list as the most locally cited source with 532 citations, followed closely by the Journal of the American Medical Informatics Association with 528 citations. The International Journal of Medical Informatics also received significant attention from local researchers with 412 citations. Other highly cited sources include the New England Journal of Medicine , JAMA-Journal of the American Medical Association , and The Lancet with 296, 263, and 257 citations, respectively.
Additionally, the collection is associated with 1898 Keywords Plus (ID) and 2434 Author’s Keywords (DE), indicating the topics and themes addressed by the research. On average, each document in the collection has 5.85 co-authors. The data also show the importance of international collaboration in EHR research, as 19.77% of the co-authorships are international. The data indicate that journal articles are the most prevalent document type in the collection. Specifically, out of the total 951 documents included, 791 were categorized as articles, while 36 were classified as articles and early access papers, and 10 were identified as articles and proceedings papers.
3.2. Trend Topics in EHR Research in Australia Based on Author’s Keywords
Table 2 provides information on the frequency and temporal distribution of Author’s Keywords (DE) in the exported data related to electronic health record research in Australia. The most frequently occurring Author’s Keywords in the data are “electronic health records” (168), “electronic medical records” (69), “e-health” (37), “general practice” (36), and “primary care” (30). Other notable Author’s Keywords include “epidemiology” (28), “emergency department” (24), “hospitals” (22), “medical records” (21), and “residential aged care” (20). This table also includes keywords related to specific health conditions, such as cardiovascular disease (5) and mental health (13), as well as keywords related to implementation (13), evaluation (9), and education (12). Interestingly, there are five instances of the keyword “natural language processing,” indicating that this is a relevant new area of EHR research in Australia in 2022. Furthermore, “digital health” (8), “COVID-19” (14), and “opioid” (5) are also among the frequently used keywords in recent years. These Author’s Keywords can be visualized in the treemap format (see Figure S3 , Supplementary Materials ) to provide a comprehensive understanding of their relative frequencies within the dataset as well as the duration of these topics.
Trend topics in EHR research in Australia.
Item | Freq | Year_q1 | Year_med | Year_q3 |
---|---|---|---|---|
electronic health records | 168 | 2014 | 2017 | 2020 |
electronic medical records | 69 | 2015 | 2019 | 2021 |
e-health | 37 | 2014 | 2016 | 2019 |
general practice | 36 | 2019 | 2020 | 2021 |
primary care | 30 | 2017 | 2019 | 2020 |
epidemiology | 28 | 2017 | 2019 | 2021 |
emergency department | 24 | 2017 | 2020 | 2021 |
hospitals | 22 | 2018 | 2020 | 2021 |
medical records | 21 | 2013 | 2017 | 2018 |
residential aged care | 20 | 2015 | 2018 | 2020 |
privacy | 14 | 2011 | 2014 | 2017 |
COVID-19 | 14 | 2021 | 2022 | 2022 |
implementation | 13 | 2011 | 2018 | 2021 |
mental health | 13 | 2017 | 2018 | 2020 |
education | 12 | 2015 | 2017 | 2018 |
public health | 12 | 2018 | 2021 | 2021 |
information management | 11 | 2008 | 2015 | 2019 |
health information systems | 10 | 2013 | 2016 | 2017 |
inpatients | 10 | 2017 | 2021 | 2021 |
evaluation | 9 | 2013 | 2015 | 2020 |
personal health records | 9 | 2015 | 2016 | 2017 |
digital health | 8 | 2020 | 2021 | 2022 |
audit | 7 | 2011 | 2013 | 2018 |
data quality | 6 | 2014 | 2014 | 2016 |
confidentiality | 6 | 2012 | 2015 | 2017 |
information systems | 5 | 2010 | 2011 | 2017 |
quality | 5 | 2013 | 2013 | 2019 |
cardiovascular disease | 5 | 2013 | 2014 | 2018 |
natural language processing | 5 | 2022 | 2022 | 2022 |
opioid | 5 | 2021 | 2022 | 2022 |
3.3. Conceptual Structure
Figure 2 displays the clustering analysis results based on the values of the Callon Centrality and Callon Density measures for various EHR research themes in Australia. Callon Centrality is a measure of the strength of the connection between a given theme and the other themes in the dataset, while Callon Density measures the degree to which the themes in the cluster are interconnected. The cut-off values for Callon Centrality and Callon Density are determined by the median values of these measures across all clusters. In these EHR research data, the median Callon Centrality is 0.0625, while the median Callon Density is 22.

Conceptual structure—thematic map.
The identified clusters are subsequently categorized into one of the four quadrants based on their Callon Density and Callon Centrality scores. The upper right quadrant is indicative of highly dense and central clusters, which are labelled as “Motor Themes” in this study. The upper left quadrant comprises highly dense but less central clusters, referred to as “Niche Themes”. Similarly, the lower right quadrant represents highly central but less dense clusters, labelled as “Basic Themes”. Lastly, the lower left quadrant represents less central and less dense clusters, referred to as “Emerging or Declining Themes”. As shown in Figure 3 , the “Motor Themes” quadrant includes clusters such as “residential aged care”, “adverse drug reaction”, “obesity”, “cardiovascular disease”, and “children”. The “Niche Themes” quadrant includes clusters such as “monitoring”, “HIV”, “ambulance”, “malnutrition”, “critical care”, and “rehabilitation”. The “Basic Themes” quadrant encompasses clusters such as “electronic health records”, “epidemiology”, “pregnancy”, “general practice”, “medical informatics”, and “mental health”. Finally, the “Emerging or Declining Themes” quadrant includes clusters such as “outcomes”, “pediatric”, “advance care planning”, “trauma”, and “health services”.

Thematic evolution of EHR research in Australia across four time periods.
Figure 3 of thematic evolution provides further information in helping us understand the evolution of Australian EHR research across four time periods. One notable trend is the proliferation of terms and concepts within the field, indicative of a growing complexity and depth of investigation. Moreover, research efforts have expanded beyond the initial stage of exploration between the 1990s and 2010, with new terms and concepts emerging, including “big data”.
3.4. Intellectual Structure
In this study, we explored intellectual structure using the Historiographic Mapping technique. This approach helps to identify historical paths for each research topic, as well as the core authors and documents associated with the topic [ 44 ]. Figure 4 displays the historical evolution of the 30 most cited works in the field of EHRs from 1991 to 2022 in Australia. The nodes in the graph represent individual works labelled with the first author and the year of publication. Each edge represents a direct citation, with the arrows indicating the direction of the citation. The size of each node corresponds to the number of local citations (LCS) that the work has received, while the colour of the node reflects the cluster to which the document belongs. The graph is segmented into four distinct clusters, which represent different research topics and subfields within the EHR domain.

Intellectual structure. Bibliometric historiography showing historical evolution of the most cited works.
One of the most prominent clusters in red in the graph centres around the work of Keith McInnes, whose 2006 article, “General Practitioners’ Use of Computers for Prescribing and Electronic Health Records: Results from A National Survey”, is one of the most cited works in the EHR field. This cluster includes eight documents, which is the second biggest cluster of this graph.
Another significant cluster in blue is the biggest cluster including 15 documents. In this cluster, the most cited works are those by Deutsch (2010), titled “Critical Areas of National Electronic Health Record Programs-Is Our Focus Correct?”; Zhang (2012), titled “The Benefits of Introducing Electronic Health Records in Residential Aged Care Facilities: A Multiple Case Study”; and Andrews (2014), titled “The Australian General Public’s Perceptions of Having a Personally Controlled Electronic Health Record (Pcehr)”. They each have eight local citations.
A third cluster in green contains two documents. In this cluster, Hawley’s work (2014), titled “Sharing of Clinical Data in a Maternity Setting: How Do Paper Hand-Held Records and Electronic Health Records Compare for Completeness?” cited O’sullivan (2011), titled “Just What the Doctor Ordered: Moving Forward with Electronic Health Records”.
A fourth cluster in purple contains two documents. In this cluster, Bernardo’s work (2019), titled “Influenza Immunization Coverage from 2015 to 2017: A National Study of Adult Patients from Australian General Practice”, cited Gonzalez-Chica DA’s 2018 article.
Overall, the graph serves as a visual representation of the EHR field’s historical development, highlighting the key works and research topics that have shaped the field over the past three decades.
3.5. Social Structure
In this study, we use “institutional collaboration” with Betweenness Centrality [ 45 ] and PageRank [ 46 ] to explore social structure. Figure 5 provides information on the collaboration network in electronic health record (EHR) research in Australia. The nodes represent institutions involved in EHR research, while the colours indicate their clustering. There are two major clusters in this network, namely one in red and another in brown.

Social structure—collaboration network based on affiliations.
The red cluster in the network reveals a high level of collaboration and interconnectedness among the institutions. Within this cluster, the University of Sydney had the highest Betweenness Centrality score (181.367522356292) and a high PageRank score (0.0707735905254743), indicating its importance in the network. Other institutions within this cluster, including the University of New South Wales, the University of Wollongong, Macquarie University, and Deakin University, also had relatively high Betweenness and PageRank scores. Meanwhile, the brown cluster, represented by the University of Melbourne, had the highest PageRank score (0.080113721), suggesting its significant influence in the network.
4. Discussion
This study explored the landscape of electronic health record (EHR) research in Australia from 1991 to 2022 through the integration of two bibliometric analysis techniques. The first approach involved a “performance analysis”, which primarily entailed a descriptive examination of the publications. The second approach, “science mapping”, employed knowledge structures to uncover latent patterns in the data. Through the combination of these methods, a comprehensive understanding of the publication trends, the intellectual and social structures, and key themes of EHR research in Australia was achieved.
EHR-related research in Australia has shown a growing trend over the past 31 years, with a significant increase in the past decade. The top five prolific institutions have been concentrated in economically developed provincial states. This is consistent with global trends in EHR research [ 37 ]. The increasing volume of studies and the evolving nature of EHR technologies can inform policy decisions aimed at integrating cutting-edge EHR solutions into clinical practice, improving patient care, and enhancing healthcare efficiency. Moreover, the significant incremental trend in research activity underscores the readiness of the Australian healthcare sector to embrace digital transformations, positioning it to leverage EHR advancements in addressing contemporary healthcare challenges, such as data interoperability, patient privacy concerns, and the need for real-time data analytics in clinical decision making. Therefore, it becomes imperative for policymakers to engage with these research findings actively, translating academic insights into practical strategies that capitalize on the potential of EHR systems to revolutionize healthcare delivery.
The thematic map of EHR research studies at a global level identified themes, such as “clinical decision support”, “primary care”, “epidemiology”, “diabetes”, “palliative care”, “cancer”, “COVID”, and “telehealth” [ 37 ], which, by and large, align with the present study in the Australian context. Similar themes have been observed in other studies, such as in research conducted in Brazil [ 47 ] and in Europe [ 48 , 49 , 50 ], which underscores the universal drive towards interoperability, advancements in cloud computing and security, and the integration of AI and analytics for better decision making and patient care. These commonalities suggest a global consensus on the prioritized areas within EHR research.
However, our study also highlights the growing importance of aged care as a prominent EHR-related theme. The “Motor Themes” quadrant, which includes clusters such as “residential aged care”, “clinical decision support”, “obesity” and “cardiovascular disease”, represents highly dense and central research areas in the Australian EHR landscape, indicating continued research interest and innovation in these areas. The observed trends may be attributed to two factors. Firstly, the Australian government’s response to the findings of the Royal Commission into Aged Care Quality and Safety has prioritized the reform of the elderly care sector, resulting in increased funding towards this area [ 51 , 52 ]. Secondly, the COVID-19 pandemic has accelerated the adoption of technology, including the use of EHRs, in healthcare [ 1 , 2 , 3 , 4 , 6 ]. These factors highlight the need for continued research and innovation in EHRs to address the growing healthcare needs of Australia’s aging population.
This study not only maps the landscape of EHR research, but also pinpoints areas that need practical application and further investigation. For instance, the surge in EHR studies related to COVID-19 underscores the system’s role in pandemic response, facilitating real-time data sharing which is crucial for public health decisions. This finding suggests that future research could delve into optimizing EHR systems for better pandemic preparedness and response, potentially through developing advanced predictive analytics tools within EHR platforms.
Additionally, the emphasis on aged care within our analysis highlights an opportunity to leverage EHR technologies for improving medication management among the elderly, specifically through EHR-based alerts for drug interactions and the potential integration of telehealth service. This application directly addresses the challenges of managing complex medication regimes, illustrating a clear pathway from bibliometric insights to healthcare practice improvements. In consideration of these outcomes, it becomes crucial for policymakers and healthcare providers to strategize the advancement of EHR systems within the Australian setting by fostering user acceptance and integrating EHRs with the aged care system’s monitoring and surveillance frameworks, as well as with disease registries. The implication of a disease registry is also evident in a Brazilian study [ 53 ]. These efforts will not only elevate the standard of elderly care, but also tackle the issues posed by an aging population and the ramifications of the COVID-19 pandemic.
However, the findings of this study should be interpreted in the context of a few limitations. First, it has been argued that Scopus provides a more comprehensive coverage of sources than the Web of Science (WoS) database in fields beyond medicine and physical sciences [ 54 ], while WoS is generally regarded as the source with the highest quality of information. Consequently, there is a possibility that some important papers may have been missed. To overcome this limitation, one potential solution is to utilize additional sources, such as Scopus; compare the differences in records; and incorporate any missing papers in future studies. Second, despite the fact that a bibliometric methodology leverages the precision of quantitative methods, it also encourages reviewers to apply field knowledge toward the synthesis and interpretation of results emerging from the thematic analysis. The validation of identified themes/subdomains is crucial in using bibliometric mapping as a policy-supporting tool, and it requires the input of field experts to confirm the identified themes [ 30 ]. To facilitate the confirmation of themes by field experts, it would be beneficial to further explore the use of an alluvial graph in conjunction with Publication Year Spectroscopy (RPYS). RPYS can trace the historical origins of research fields/topics [ 55 ], while an alluvial graph in a thematic map, which divides the time span into time slices, can track temporal changes over time. Thus, these tools can be useful to reveal the evolution of research themes in a specific field of EHRs. Third, a critical limitation of our study is the constrained exploration of ethical and safety considerations associated with EHRs. Given the substantial importance of ethical issues—ranging from patient privacy and data security to the ethical use of EHR data for research and clinical decision making—our analysis acknowledges the need for a deeper investigation in this area. The ethical landscape surrounding EHRs is complex and evolving, requiring thorough consideration beyond the scope of our current bibliometric methodology. Future research should aim to specifically address these ethical and safety concerns, incorporating qualitative analyses and expert consultations to comprehensively explore the implications of EHR use on patient care and privacy. The scope of the bibliometric search is limited to Australian works. Based on this, although health policymakers in settings similar to Australia may find these results appropriate, it is suggested to interpret the findings conservatively and tailor the results according to local settings.
5. Conclusions
This study identified a steady increase in EHR-related research through the years, indicating the growing interest in the field. A strong level of collaboration was observed among authors and institutions, which highlights the importance of inter-disciplinary research in this area. This comprehensive descriptive analysis, together with the use of the Science Mapping technique, presents a synthesized overview of the topic in this specialized area of EHR in general and for the Australian context in particular. Similar to the global trends and related themes on the priority areas within EHR research, the Australian context shows the same priorities in the areas of clinical decision support systems, cardiovascular diseases, drug allergies, and adverse drug reactions. Overall, this study offers insights into the knowledge structures of EHR-related research and could serve as a valuable reference point for scholars and policymakers alike. The identification of experts and prominent institutions in this field could facilitate informed policy discussions and funding allocations, ultimately benefiting healthcare practices and patient outcomes.
Acknowledgments
We acknowledge the support provided by the Flinders University College of Business Government and Law Writers Retreat Program, which contributed to the development and writing of this paper.
Supplementary Materials
The following supporting information can be downloaded at: https://www.mdpi.com/article/10.3390/ijerph21030361/s1 , Figure S1: Identification and selection of articles for bibliometric analysis; Figure S2: Most relevant authors, affiliations, sources and locally cited sources; Figure S3: Tree map on most used words on EHR research in Australia.
Funding Statement
This research received no external funding.
Author Contributions
H.X. conducted the search and analysis and wrote the first draft of the paper. M.B. supervised the project. H.X. conducted research and collaborated in the development of the search strategy, analysis, and paper drafts. H.X., M.B., P.B. and A.C. were involved in the conceptualization and development of the paper. All authors have read and agreed to the published version of the manuscript.
Institutional Review Board Statement
Not applicable.
Informed Consent Statement
Data availability statement, conflicts of interest.
The authors declare no conflicts of interest.
Disclaimer/Publisher’s Note: The statements, opinions and data contained in all publications are solely those of the individual author(s) and contributor(s) and not of MDPI and/or the editor(s). MDPI and/or the editor(s) disclaim responsibility for any injury to people or property resulting from any ideas, methods, instructions or products referred to in the content.
- Open access
- Published: 19 August 2024
The impact of study habits and personal factors on the academic achievement performances of medical students
- Mohammed A. Aljaffer 1 ,
- Ahmad H. Almadani 1 ,
- Abdullah S. AlDughaither 2 ,
- Ali A. Basfar 2 ,
- Saad M. AlGhadir 2 ,
- Yahya A. AlGhamdi 2 ,
- Bassam N. AlHubaysh 2 ,
- Osamah A. AlMayouf 2 ,
- Saleh A. AlGhamdi 3 ,
- Tauseef Ahmad 4 &
- Hamza M. Abdulghani 5
BMC Medical Education volume 24 , Article number: 888 ( 2024 ) Cite this article
205 Accesses
2 Altmetric
Metrics details
Academic achievement is essential for all students seeking a successful career. Studying habits and routines is crucial in achieving such an ultimate goal.
This study investigates the association between study habits, personal factors, and academic achievement, aiming to identify factors that distinguish academically successful medical students.
A cross-sectional study was conducted at the College of Medicine, King Saud University, Riyadh, Saudi Arabia. The participants consisted of 1st through 5th-year medical students, with a sample size of 336. The research team collected study data using an electronic questionnaire containing three sections: socio-demographic data, personal characteristics, and study habits.
The study results indicated a statistically significant association between self-fulfillment as a motivation toward studying and academic achievement ( p = 0.04). The results also showed a statistically significant correlation between recalling recently memorized information and academic achievement ( p = 0.05). Furthermore, a statistically significant association between preferring the information to be presented in a graphical form rather than a written one and academic achievement was also found ( p = 0.03). Students who were satisfied with their academic performance had 1.6 times greater chances of having a high-grade point average (OR = 1.6, p = 0.08).
The results of this study support the available literature, indicating a correlation between study habits and high academic performance. Further multicenter studies are warranted to differentiate between high-achieving students and their peers using qualitative, semi-structured interviews. Educating the students about healthy study habits and enhancing their learning skills would also be of value.
Peer Review reports
Introduction
Academic performance is a common indicator used to measure student achievement [ 1 , 2 ]. It is a compound process influenced by many factors, among which is study habits [ 2 , 3 ]. Study habit is defined as different individual behavior in relation to studying, and is a combination of study methods and skills [ 2 , 3 , 4 ]. Put differently, study habits involve various techniques that would increase motivation and transform the study process into an effective one, thus enhancing learning [ 5 ]. Students’ perspectives and approaches toward studying were found to be the key factors in predicting their academic success [ 6 , 7 ]. However, these learning processes vary from one student to another due to variations in the students’ cognitive processing [ 8 ].
The study habits of students are the regular practices and habits they exhibit during the learning process [ 9 , 10 ]. Over time, several study habits have been developed, such as time management, setting appropriate goals, choosing a comfortable study environment, taking notes effectively, choosing main ideas, and being organized [ 11 ]. Global research shows that study habits impact academic performance and are the most important predictor of it [ 12 ]. It is difficult for medical students to organize and learn a lot of information, and they need to employ study skills to succeed [ 1 , 2 , 5 , 13 ].
Different lifestyle and social factors could affect students’ academic performance. For instance, Jafari et al. found that native students had better study habits compared to dormitory students [ 1 ]. This discrepancy between native and dormitory students was also indicated by Jouhari et al. who illustrated that dormitory students scored lower in attitude, test strategies, choosing main ideas, and concentration [ 10 ]. Regarding sleeping habits, Curcio G et al. found that students with a regular and adequate sleeping pattern had higher Grade Point Average (GPA) scores [ 14 ]. Lifestyle factors, such as watching television and listening to music, were shown to be unremarkable in affecting students’ grades [ 15 , 16 ]. Social media applications, including WhatsApp, Facebook, and Twitter, distract students during learning [ 16 , 17 ].
Motivation was found to be a major factor in students’ academic success. Bonsaksen et al. found that students who chose “to seek meaning” when studying were associated with high GPA scores [ 18 ]. In addition, low scores on “fear of failure” and high scores on “achieving” correlated with a higher GPA [ 8 , 18 ].
Resource-wise, Alzahrani et al. found that 82.7% of students relied on textbooks assigned by the department, while 46.6% mainly relied on the department’s lecture slides [ 19 ]. The study also indicated that 78.8% perceived that the scientific contents of the lectures were adequate [ 19 ]. Another study found that most students relied on the lecture slides (> 83%) along with their notes, followed by educational videos (76.1%), and reference textbooks (46.1%) [ 20 ]. Striking evidence in that study, as well as in another study, indicated that most students tended to avoid textbooks and opted for lecture slides, especially when preparing for exams [ 20 , 21 ].
Several researchers studied the association between different factors and academic performance; however, more is needed to know about this association in the process of education among medical students [ 15 , 20 , 22 ], with some limitations to the conducted studies. Such limitations include the study sample and using self-reported questionnaires, which may generate inaccurate results. Moreover, in Saudi Arabia in particular, the literature concerning the topic remains limited. Since many students are unsatisfied with their performance and seek improvement [ 10 ], the present study was designed and conducted.
Unlike other studies in the region, this study aims to investigate the relationship between study habits and personal factors and measure their influence on academic achievement. The results of this study could raise awareness regarding the effect of study habits and personal factors on students’ performance and would also guide them toward achieving academic success. The study also seeks to identify the factors that distinguish academically successful students from their peers.
Study design, setting, and participants
This observational cross-sectional study, which took place between June and December 2022, was conducted among students attending the College of Medicine at King Saud University (KSU), Riyadh, Saudi Arabia. Its targeted population included all male and female medical students (first to fifth years) attending KSU during the academic year 2021/2022. Whereas, students at other colleges and universities, those who failed to complete the questionnaire, interns (the students who already graduated), and those who were enrolled in the university’s preparatory year, were all excluded from the current study. The sample size was calculated based on a study conducted in 2015 by Lana Al Shawwa [ 15 ]. Using the sample size formula for a single proportion (0.79), the required sample size was 255 using a confidence interval of 95% and a margin of error of 5%. After adding a 20% margin to accommodate non-responses and incomplete responses, the calculated sample size required for this study was 306. However, our research team collected a total of 336 participants for this study to ensure complete representation.
Study instrument
The research team developed and used an electronic questionnaire. The rationale is that no standardized questionnaire measuring the study objectives was found in the literature. However, the questionnaire was tested on a pilot of 15 students to test its clarity and address any possible misconceptions and ambiguity. The study questionnaire was distributed randomly to this cohort, who were asked to fill out the questionnaire. The students reported a complete understanding of the questionnaire’s contents, so the same questionnaire was used without any modifications. The questionnaire, written in English, consisted of three parts. The first part included eleven questions about the socio-demographic status of the participants. The second part contained twenty-one questions examining personal factors such as sleep and caffeine consumption. The last part included twenty-one questions regarding students’ study habits. The questionnaire was constructed based on an ordinal Likert scale which had: strongly agree, agree, neutral, disagree, and strongly disagree as possible answers. The questionnaire was sent to participants through email and social media applications like Twitter and WhatsApp to increase the study response. An informed consent that clearly states the study’s purpose was taken from all participants at the beginning of the questionnaire. In addition, all participants were assured that the collected data would be anonymous and confidential. Each participant was represented by a code for the sole purpose of analyzing the data. Furthermore, no incentives or rewards were given to the participants for their participation.
Study variables
Socio-demographic information (such as age, gender, and academic year), and personal factors (such as motivation, sleeping status, caffeine consumption, and self-management) were the independent variables. Study habits such as attendance, individual versus group study, memorization techniques, revision, learning style, and strategies were also independent variables.
Academic achievement refers to a student’s success in gaining knowledge and understanding in various subjects, as well as the ability to apply that knowledge effectively [ 23 ]. It is a measure of the student’s progress throughout the educational journey, encompassing both academic achievements and personal growth [ 3 , 24 ]. Academic achievement is judged based on the student’s GPA or performance score. In this study, students’ GPA scores, awareness, and satisfaction regarding their academic performance were the dependent variables.
We divided the study sample into two groups based on the GPA. We considered students with high GPAs to be exposed (i.e. exposed to the study habits we are investigating), and students with low GPAs to be the control group. The purpose of this study was to determine why an exposed group of students gets high grades and what study factors they adopt. Based on this exposure (high achieving students), we concluded what methods they used to achieve higher grades. Those in the first group had a GPA greater or equal to 4.5 (out of 5), while those in the second group had a GPA less than 4.5. The students’ data were kept confidential and never used for any other purpose.
Data analysis
The data collected were analyzed by using IBM SPSS Statistical software for Windows version 24.0. Descriptive statistics such as frequency and percentage were used to describe the socio-demographic data in a tabular form. Furthermore, data for categorical variables, including different study habits, motivation factors, memorizing and revising factors, and lifestyle factors, were tabulated and analyzed using the odds ratio test. Finally, we calculated the odds ratio statistic and a p-value of 0.05 to report the statistical significance of our results.
Ethical approval and consent to Participate
Before conducting the study, the research team obtained the Ethics Committee Approval from the Institutional Review Board of the College of Medicine, KSU, Riyadh, Saudi Arabia (project No. E-22-7044). Participants’ agreement/consent to participate was guaranteed by choosing “agree” after reading the consent form at the beginning of the questionnaire. Participation was voluntary, and consent was obtained from all participants. The research team carried out all methods following relevant guidelines and regulations.
The total 336 medical students participated in the study. All participants completed the study questionnaire, and there were no missing or incomplete data, with all of them being able to participate. As shown in Table 1 9.3% of participants were between 18 and 20, 44.9% were between the ages of 21 and 22, and 35.8% were 23–28 years old. In the current study, 62.5% of the participants were males and 37.5% were females. The proportion of first-year students was 21.4%, 20.8% of second-year students, 20.8% of third-year students, 18.2% of fourth-year students, and 18.8% of fifth-year students, according to academic year levels. Regarding GPA scores, 36.9% scored 4.75-5 and 32.4% scored 4.5–4.74. 23.8% achieved 4-4.49, 6.5% achieved 3-3.99, and only 0.4% achieved 2.99 or less. Participants lived with their families in 94.6% of cases, with friends in 1.2% of cases, and alone in 4.2% of cases. For smoking habits, 86.3% did not smoke, 11% reported using vapes, 2.1% used cigarettes, and 0.6% used Shisha. 91.4% of the participants did not report any chronic illnesses; however, 8.6% did. In addition, 83% had no mental illness, 8.9% had anxiety, 6% had depression, and 2.1% reported other mental illnesses.
Table 2 shows motivational factors associated with academic performance. There was a clear difference in motivation factors between students with high and low achievement in the current study. Students with high GPAs were 1.67 times more motivated toward their careers (OR = 1.67, p = 0.09) than those with low GPAs. Furthermore, significant differences were found between those students who had self-fulfillment or ambitions in life they had ~ 2 times higher (OR = 1.93, p = 0.04) GPA scores than low GPA students. Exam results did not motivate exposed or high GPA students (46%) or control students with low GPA students (41%), but the current study showed test results had little impact on low achiever students (OR = 1.03, p = 0.88). Furthermore, 72.6% of high achievers were satisfied with their academic performance, while only 41% of low achiever students were satisfied. Therefore, students who were satisfied with their academic performance had 1.6 times greater chances of a higher GPA (OR = 1.6, p = 0.08). Students who get support and help from those around them are more likely to get high GPAs (OR = 1.1, p = 0.73) than those who do not receive any support. When students reported feeling a sense of family responsibility, the odds (odds ratio) of their receiving higher grades were 1.15 times higher (OR = 1.15, p = 0.6) compared to those who did not feel a sense of family responsibility. The p-value, which indicates the level of statistical significance, was 0.6.
Table 3 shows the study habits of higher achiever students and low achiever students. Most of the high-achieving students (79.0%) attended most of the lectures and had 1.6 times higher chances of getting higher grades (OR = 1.6, p = 0.2) than those who did not attend regular lectures. The current study found that studying alone had no significant impact on academic achievement in either group. However, those students who had studied alone had lower GPAs (OR = 1.07, p = 0.81). The current study findings reported 29.8% of students walk or stand while studying rather than sit, and they had 1.57 times higher GPA chances compared to students with lower GPAs (OR = 0.73, p = 0.27). High achievers (54.0%) preferred studying early in the morning, and these students had higher chances of achieving good GPAs (OR = 1.3, p = 0.28) than low achiever groups of students. The number of students with high achievement (39.5%) went through the lecture before the lesson was taught. These students had 1.08 times higher chances of achieving than low achiever groups of students. Furthermore, students who made a weekly study schedule had 1.3 times higher chances of being good academic achievers than those who did not (OR = 1.3, p = 0.37). Additionally, high-achieving students paid closer attention to the lecturer (1.2 times higher). In addition, students with high GPAs spent more time studying when exam dates approached (OR = 1.3, p = 0.58).
Table 4 demonstrates the relationship between memorizing and revising with high and low GPA students. It was found that high achiever students (58.9%) studied lectures daily and had 1.4 times higher chances of achieving high grades (OR = 1.4, p = 0.16) than the other group. It was found that most of the high achievers (62.1%) skim the lecture beforehand before memorizing it, which led to 1.8 times higher chances of getting good grades in this exam (OR = 1.8, p = 0.06). One regular activity reported by high GPA students (82.3%) was recalling what had just been memorized. For this recalling technique, we found a significant difference between low-achieving students (OR = 0.8, p = 0.63) and high-achieving students (OR = 1.83, p = 0.05). A high achiever student writes notes before speaking out for the memorizing method, which gives 1.2 times greater chances of getting high grades (OR = 1.2, p = 0.55) than a student who does not write notes. A major difference in the current study was that high GPA achievers (70.2%) revise lectures more frequently than low GPA achievers (57.1%). They had 1.5 times more chances of getting high grades if they practiced and revised this method (OR = 1.5, p = 0.13).
Table 5 illustrates the relationship between negative lifestyle factors and students’ academic performance. The current study found that students are less likely to get high exam grades when they smoke. Students who smoke cigarettes and those who vape are 1.14 and 1.07 times respectively more likely to have a decrease in GPA than those who do not smoke. Those students with chronic illnesses had 1.22 times higher chances of a downgrade in the exam (OR = 1.22, p = 0.49). Additionally, students with high GPAs had higher mental pressures (Anxiety = 1.2, Depression = 1.18, and other mental pressures = 1.57) than those with low GPAs.
Learning is a multifaceted process that evolves throughout our lifetimes. The leading indicator that sets students apart is their academic achievement. Hence, it is crucial to investigate the factors that influence it. The present study examined the relationship between different study habits, personal characteristics, and academic achievement among medical students. In medical education, and more so in Saudi Arabia, there needs to be more understanding regarding such vital aspects.
Regarding motivational factors, the present study found some differences between high and low achievers. Students with high GPA scores were more motivated toward their future careers (OR = 1.67, p = 0.09). The study also indicated that students who had ambitions and sought self-fulfillment were more likely to have high GPA scores, which were statistically significant (OR = 1.93, p = 0.04). This was consistent with Bin Abdulrahman et al. [ 20 ], who indicated that the highest motivation was self-fulfillment and satisfying family dreams, followed by a high educational level, aspirations to join a high-quality residency program, and high income. Their study also found that few students were motivated by the desire to be regarded as unique students. We hypothesize that this probably goes back to human nature, where a highly rewarding incentive becomes the driving force of our work. Hence, schools should utilize this finding in exploring ways to enhance students’ motivation toward learning.
The present study did not find a significant effect of previous exam results on academic performance (OR = 1.03, p = 0.88). However, some studies reported that more than half of the high-achieving students admitted that high scores acquired on previous assessments are an important motivational factor [ 15 , 25 , 26 ]. We hypothesize that as students score higher marks, they become pleased and feel confident with their study approach. This finding shows how positive measurable results influence the students’ mentality.
The present study also explored the social environment surrounding medical students. The results indicated that those who were supported by their friends or family were slightly more likely to score higher GPAs (OR = 1.1, p = 0.73); however, the results did not reach a statistical significance. We hypothesize that a supportive and understanding environment would push the students to be patient and look for a brighter future. Our study results were consistent with previous published studies, which showed an association [ 3 , 27 , 28 , 29 , 30 ]. We hypothesize that students who spend most of their time with their families had less time to study, which made their study time more valuable. The findings of this study will hopefully raise awareness concerning the precious time that students have each day.
The association of different study habits among medical students with high and low GPAs was also studied in our study. It was noted that the high-achieving students try to attend their lectures compared to the lower achievers. This was in line with the previous published studies, which showed that significant differences were observed between the two groups regarding the attendance of lectures, tutorials, practical sessions, and clinical teachings [ 31 , 32 ]. The present study found that most students prefer to study alone, regardless of their level of academic achievement (82.1%). This finding is consistent with the study by Khalid A Bin Abdulrahman et al., which also showed that most students, regardless of their GPA, favored studying alone [ 20 ].
The present study findings suggest that a small number of students (29.8%) prefer to walk or stand while studying rather than sit, with most being high achievers (OR = 1.57, P = 0.15). A study reported that 40.3% of students with high GPAs seemed to favor a certain posture or body position, such as sitting or lying on the floor [ 15 ]. These contradictory findings might indicate that which position to adopt while studying should come down to personal preference and what feels most comfortable to each student. The present study also found that high achievers are more likely to prefer studying early in the morning (OR = 1.3, P = 0.28). The authors did not find similar studies investigating this same association in the literature. However, mornings might allow for more focused studying with fewer distractions, which has been shown to be associated with higher achievement in medical students [ 3 , 15 , 33 ].
Our study also found that 39.5% of the academically successful students reviewed pre-work or went through the material before they were taught it (OR = 1.08, p = 0.75), and 25% were neutral. Similar findings were reported in other studies, showing that academically successful students prepared themselves by doing their pre-work, watching videos, and revising slides [ 3 , 9 , 34 ]. Our study showed that 75% of high-achieving students tend to listen attentively to the lecturer (OR = 1.2, p = 0.48). Al Shawa et al. found no significant differences between the high achievers and low achievers when talking about attending lectures [ 15 ]. This could be due to the quality of teachers and the environment of the college or university.
Regarding the relationship between memorizing and revising with high and low GPA students, the present study found that students who study lectures daily are more likely to score higher than those who do not (OR = 1.4, p = 0.16). This finding is consistent with other studies [ 3 , 19 , 35 ]. For skimming lectures beforehand, an appreciable agreement was noted by high GPA students (62.1%), while only (42%) of low GPA students agreed to it. Similarly, previous published studies also found that highlighting and reading the content before memorization were both common among high-achieving students [ 15 , 36 ]. Furthermore, the present study has found recalling what has just been memorized to be statistically significantly associated with high GPA students (OR = 1.83, p = 0.05). Interestingly, we could not find any study that investigated this as an important factor, which could be justified by the high specificity of this question. Besides, when it comes to writing down/speaking out what has just been memorized, our study has found no recognizable differences between high-achieving students (75%) and low-achieving students (69%), as both categories had remarkably high percentages of reading and writing while studying.
The present study has found no statistical significance between regularly revising the lectures and high GPA ( p > 0.05), unlike the study conducted by Deborah A. Sleight et al. [ 37 ]. The difference in findings between our study and Deborah A. Sleight et al. might be due to a limitation of our study, namely the similar backgrounds of our participants. Another explanation could be related to curricular differences between the institutions where the two studies were conducted. Moreover, a statistically significant correlation between not preferring the data being presented in a written form instead of a graphical form and high GPA scores have been found in their study ( p < 0.05). However, a study conducted by Deborah A. Sleight et al. indicated that 66% of high achievers used notes prepared by other classmates compared to 84% of low achievers. Moreover, their study showed that only 59% of high achievers used tables and graphs prepared by others compared to 92% of low achievers. About 63% and 61% of the students in their study reported using self-made study aids for revision and memory aids, respectively [ 37 ].
The present study also examined the effects of smoking and chronic and mental illness, but found no statistical significance; the majority of both groups responded by denying these factors’ presence in their life. A similar finding by Al Shawwa et al. showed no statistical significance of smoking and caffeine consumption between low GPA and high GPA students [ 15 ]. We hypothesize that our findings occurred due to the study’s broad approach to examining such factors rather than delving deeper into them.
High-achieving students’ habits and factors contributing to their academic achievement were explored in the present study. High-achieving students were found to be more motivated and socially supported than their peers. Moreover, students who attended lectures, concentrated during lectures, studied early in the morning, prepared their weekly schedule, and studied more when exams approached were more likely to have high GPA scores. Studying techniques, including skimming before memorizing, writing what was memorized, active recall, and consistent revision, were adopted by high-achievers. To gain deeper insight into students’ strategies, it is recommended that qualitative semi-structured interviews be conducted to understand what distinguishes high-achieving students from their peers. Future studies should also explore differences between public and private university students. Additionally, further research is needed to confirm this study’s findings and provide guidance to all students. Future studies should collect a larger sample size from a variety of universities in order to increase generalizability.
Limitations and recommendations
The present study has some limitations. All the study’s findings indicated possible associations rather than causation; hence, the reader should approach the results of this study with caution. We recommend in-depth longitudinal studies to provide more insight into the different study habits and their impact on academic performance. Another limitation is that the research team created a self-reported questionnaire to address the study objectives, which carries a potential risk of bias. Hence, we recommend conducting interviews and having personal encounters with the study’s participants to reduce the risk of bias and better understand how different factors affect their academic achievement. A third limitation is that the research team only used the GPA scores as indicators of academic achievement. We recommend conducting other studies and investigating factors that cannot be solely reflected by the GPA, such as the student’s clinical performance and skills. Lastly, all participants included in the study share one background and live in the same environment. Therefore, the study’s findings do not necessarily apply to students who do not belong to such a geographic area and point in time. We recommend that future studies consider the sociodemographic and socioeconomic variations that exist among the universities in Saudi Arabia.
Availability of data materials
The datasets used and/or analyzed during the current study are available from the corresponding author on reasonable request.
Abbreviations
Grade Point Average
King Saud University
Institutional review board
Statistical package for the social sciences
Jafari H, Aghaei A, Khatony A. Relationship between study habits and academic achievement in students of medical sciences in Kermanshah-Iran. Adv Med Educ Pract. 2019;10:637–43.
Article Google Scholar
Abid N, Aslam S, Alghamdi AA, Kumar T. Relationships among students’ reading habits, study skills, and academic achievement in English at the secondary level. Front Psychol. 2023;14:1020269.
Abdulghani HM, Al-Drees AA, Khalil MS, Ahmad F, Ponnamperuma GG, Amin Z. What factors determine academic achievement in high achieving undergraduate medical students? A qualitative study. Med Teach. 2014;36(Suppl 1):S43–48.
Muntean LM, Nireștean A, Sima-Comaniciu A, Mărușteri M, Zăgan CA, Lukacs E. The relationship between personality, motivation and academic performance at Medical students from Romania. Int J Environ Res Public Health 2022, 19(15).
Reza HM, Alireza HJIJME. Investigating study Habits of Library and Information Sciences Students of Isfahan University and Isfahan University of Medical Sciences. 2014, 14:751–757.
Kurtz SM, Silverman JD. The Calgary-Cambridge Referenced Observation guides: an aid to defining the curriculum and organizing the teaching in communication training programmes. Med Educ. 1996;30(2):83–9.
Pun J, Kong B. An exploratory study of communication training for Chinese medicine practitioners in Hong Kong to integrate patients’ conventional medical history. BMC Complement Med Ther. 2023;23(1):10.
İlçin N, Tomruk M, Yeşilyaprak SS, Karadibak D, Savcı S. The relationship between learning styles and academic performance in TURKISH physiotherapy students. BMC Med Educ. 2018;18(1):291.
McKeirnan KC, Colorafi K, Kim AP, Stewart AS, Remsberg CM, Vu M, Bray BS. Study behaviors Associated with Student pharmacists’ academic success in an active Classroom Pharmacy Curriculum. Am J Pharm Educ. 2020;84(7):ajpe7695.
Jouhari Z, Haghani F, Changiz T. Assessment of medical students’ learning and study strategies in self-regulated learning. J Adv Med Educ Professionalism. 2016;4(2):72–9.
Google Scholar
Proctor BE, Prevatt FF, Adams KSS, Reaser A, Petscher Y. Study skills profiles of normal-achieving and academically-struggling College students. J Coll Student Dev. 2006;47(1):37–51.
Kyauta AMASY, Garba HS. The role of guidance and counseling service on academic performance among students of umar suleiman college of education, Gashua, Yobe State, Nigeria. KIU J Humanit. 2017;2(2):59–66.
Eva KW, Bordage G, Campbell C, Galbraith R, Ginsburg S, Holmboe E, Regehr G. Towards a program of assessment for health professionals: from training into practice. Adv Health Sci Education: Theory Pract. 2016;21(4):897–913.
Curcio G, Ferrara M, De Gennaro L. Sleep loss, learning capacity and academic performance. Sleep Med Rev. 2006;10(5):323–37.
Al Shawwa L, Abulaban AA, Abulaban AA, Merdad A, Baghlaf S, Algethami A, Abu-Shanab J, Balkhoyor A. Factors potentially influencing academic performance among medical students. Adv Med Educ Pract. 2015;6:65–75.
Ibrahim NK, Baharoon BS, Banjar WF, Jar AA, Ashor RM, Aman AA, Al-Ahmadi JR. Mobile Phone Addiction and its relationship to Sleep Quality and Academic Achievement of Medical students at King Abdulaziz University, Jeddah, Saudi Arabia. J Res Health Sci. 2018;18(3):e00420.
Alkhalaf AM, Tekian A, Park YS. The impact of WhatsApp use on academic achievement among Saudi medical students. Med Teach. 2018;40(sup1):S10–4.
Bonsaksen T, Brown T, Lim HB, Fong K. Approaches to studying predict academic performance in undergraduate occupational therapy students: a cross-cultural study. BMC Med Educ. 2017;17(1):76.
Alzahrani HA, Alzahrani OH. Learning strategies of medical students in the surgery department, Jeddah, Saudi Arabia. Adv Med Educ Pract. 2012;3:79–87.
Bin Abdulrahman KA, Khalaf AM, Bin Abbas FB, Alanazi OT. Study habits of highly effective medical students. Adv Med Educ Pract. 2021;12:627–33.
Jameel T, Gazzaz ZJ, Baig M, Tashkandi JM, Alharenth NS, Butt NS, Shafique A, Iftikhar R. Medical students’ preferences towards learning resources and their study habits at King Abdulaziz University, Jeddah, Saudi Arabia. BMC Res Notes. 2019;12(1):30.
Abdulghani HM, Alrowais NA, Bin-Saad NS, Al-Subaie NM, Haji AM, Alhaqwi AI. Sleep disorder among medical students: relationship to their academic performance. Med Teach. 2012;34(Suppl 1):S37–41.
Hwang G-J, Wang S-Y, Lai C-L. Effects of a social regulation-based online learning framework on students’ learning achievements and behaviors in mathematics. Comput Educ. 2021;160:104031.
Gamage KAA, Dehideniya D, Ekanayake SY. The role of personal values in learning approaches and student achievements. Behav Sci (Basel Switzerland) 2021, 11(7).
Linn Z, Tashiro Y, Morio K, Hori H. Peer evaluations of group work in different years of medical school and academic achievement: how are they related? BMC Med Educ. 2022;22(1):102.
Avonts M, Michels NR, Bombeke K, Hens N, Coenen S, Vanderveken OM, De Winter BY. Does peer teaching improve academic results and competencies during medical school? A mixed methods study. BMC Med Educ. 2022;22(1):431.
Topor DR, Keane SP, Shelton TL, Calkins SD. Parent involvement and student academic performance: a multiple mediational analysis. J Prev Interv Community. 2010;38(3):183–97.
Veas A, Castejón JL, Miñano P, Gilar-Corbí R. Relationship between parent involvement and academic achievement through metacognitive strategies: a multiple multilevel mediation analysis. Br J Educ Psychol. 2019;89(2):393–411.
Núñez JC, Regueiro B, Suárez N, Piñeiro I, Rodicio ML, Valle A. Student Perception of teacher and parent involvement in Homework and Student Engagement: the mediating role of motivation. Front Psychol. 2019;10:1384.
Abdulghani AH, Ahmad T, Abdulghani HM. The impact of COVID-19 pandemic on anxiety and depression among physical therapists in Saudi Arabia: a cross-sectional study. BMC Med Educ. 2022;22(1):751.
Park KH, Park JH, Kim S, Rhee JA, Kim JH, Ahn YJ, Han JJ, Suh DJ. Students’ perception of the educational environment of medical schools in Korea: findings from a nationwide survey. Korean J Med Educ. 2015;27(2):117–30.
Ahrberg K, Dresler M, Niedermaier S, Steiger A, Genzel L. The interaction between sleep quality and academic performance. J Psychiatr Res. 2012;46(12):1618–22.
Dikker S, Haegens S, Bevilacqua D, Davidesco I, Wan L, Kaggen L, McClintock J, Chaloner K, Ding M, West T, et al. Morning brain: real-world neural evidence that high school class times matter. Soc Cognit Affect Neurosci. 2020;15(11):1193–202.
Pittenger AL, Dimitropoulos E, Foag J, Bishop D, Panizza S, Bishop JR. Closing the Classroom Theory to practice gap by simulating a Psychiatric Pharmacy Practice Experience. Am J Pharm Educ. 2019;83(10):7276.
Walck-Shannon EM, Rowell SF, Frey RF. To what extent do Study habits relate to performance? CBE Life Sci Educ. 2021;20(1):ar6.
Abdulghani HM, Alanazi K, Alotaibi R, Alsubeeh NA, Ahmad T, Haque S. Prevalence of potential dropout thoughts and their influential factors among Saudi Medical Students. 2023, 13(1):21582440221146966.
Sleight DA, Mavis BE. Study skills and academic performance among second-Year Medical students in Problem-based learning. Med Educ Online. 2006;11(1):4599.
Download references
Acknowledgements
The authors are grateful to the Deanship of Scientific Research, King Saud University, for.
support through the Vice Deanship of Scientific Research Chairs.
Author information
Authors and affiliations.
Department of Psychiatry, College of Medicine, King Saud University, Riyadh, Saudi Arabia
Mohammed A. Aljaffer & Ahmad H. Almadani
College of Medicine, King Saud University, Riyadh, Saudi Arabia
Abdullah S. AlDughaither, Ali A. Basfar, Saad M. AlGhadir, Yahya A. AlGhamdi, Bassam N. AlHubaysh & Osamah A. AlMayouf
Department of Psychiatry, College of Medicine, Imam Mohammad Ibn Saud Islamic University, Riyadh, Saudi Arabia
Saleh A. AlGhamdi
Department of Medical Education, College of Medicine, King Saud University, P.O. Box: 230155, Riyadh, 11321, Saudi Arabia
Tauseef Ahmad
Department of Medical Education and Family Medicine, College of Medicine, King Saud University, Riyadh, Saudi Arabia
Hamza M. Abdulghani
You can also search for this author in PubMed Google Scholar
Contributions
Conception or design: AHA, MAA, and HMA. Acquisition, analysis, or interpretation of data: AAB, SMA, ASA, YAA, BNA, OAA and SAA. Drafting the work or revising: TA, AHA, ASA AAB. Final approval of the manuscript: MAA, HMA., AHA, and TA. The author(s) read and approved the final manuscript.
Corresponding author
Correspondence to Tauseef Ahmad .
Ethics declarations
Conflict of interest.
The Authors declare that they have no conflict of interest.
Consent for publication
Not applicable.
Competing interests
The authors declare no competing interests.
Human ethics and consent to participate declarations
Additional information, publisher’s note.
Springer Nature remains neutral with regard to jurisdictional claims in published maps and institutional affiliations.
Rights and permissions
Open Access This article is licensed under a Creative Commons Attribution-NonCommercial-NoDerivatives 4.0 International License, which permits any non-commercial use, sharing, distribution and reproduction in any medium or format, as long as you give appropriate credit to the original author(s) and the source, provide a link to the Creative Commons licence, and indicate if you modified the licensed material. You do not have permission under this licence to share adapted material derived from this article or parts of it. The images or other third party material in this article are included in the article’s Creative Commons licence, unless indicated otherwise in a credit line to the material. If material is not included in the article’s Creative Commons licence and your intended use is not permitted by statutory regulation or exceeds the permitted use, you will need to obtain permission directly from the copyright holder. To view a copy of this licence, visit http://creativecommons.org/licenses/by-nc-nd/4.0/ .
Reprints and permissions
About this article
Cite this article.
Aljaffer, M.A., Almadani, A.H., AlDughaither, A.S. et al. The impact of study habits and personal factors on the academic achievement performances of medical students. BMC Med Educ 24 , 888 (2024). https://doi.org/10.1186/s12909-024-05889-y
Download citation
Received : 26 September 2023
Accepted : 12 August 2024
Published : 19 August 2024
DOI : https://doi.org/10.1186/s12909-024-05889-y
Share this article
Anyone you share the following link with will be able to read this content:
Sorry, a shareable link is not currently available for this article.
Provided by the Springer Nature SharedIt content-sharing initiative
- Medical students
- Study habits
- Academic achievement
- Saudi Arabia
BMC Medical Education
ISSN: 1472-6920
- General enquiries: [email protected]
arXiv's Accessibility Forum starts next month!
Help | Advanced Search
Condensed Matter > Superconductivity
Title: new-record-tc and three-gap 2d superconductors with electronic and phononic topology: kb2c2.
Abstract: Pursuing higher-temperature superconductors under ambient pressure continues to be a prominent topic in materials discovery. Isomorphic structures like MgB2 exhibit potential for conventional BCS-type superconductivity, but their transition temperatures (Tc) have remained below 100 K based on both experimental findings and theoretical predictions. In this study, two new two-dimensional (2D) superconductors with sandwich structures,KB2C2,featuring BC layers in AA and AB stacking configurations,are designed, whose Tc can exceed 112 K,setting a new record in 2D superconductors. The analyses suggest that electrons in {\sigma}-states covalent bonds and high-frequency E phonon modes dominated by the in-plane vibrations of B/C atoms are predominately responsible for electron-phonon coupling (EPC). An exciting robust three-gap superconducting nature stems from the strong and evident three-region distribution characteristic of electronic EPC parameters {\lambda}. When biaxial tensile strain (BTS) is applied, their Tc are boosted above 153 K. The increase in Tc originates from the softening of optical E phonon modes around the {\Gamma} point and acoustic modes around the Q point, rather than an increase of electrons at the Fermi level (EF ) as observed in other similar systems. Thus, phonon plays a more beneficial role in the EPC of BTS cases, highlighting its significance as a medium in BCS superconductors. Moreover, we find KB2C2 exhibits interesting topological properties, spin antivortex, and Ising-type spin splitting. This is the first report of the coexistence of nontrivial topology and superconductivity with such a high Tc. Therefore, KB2C2 may offer promising sandwich structures to explore higher-Tc 2D superconductors, alongside present potential avenues for investigating fundamental quantum physics.
Comments: | 8 pages, 3 figures |
Subjects: | Superconductivity (cond-mat.supr-con) |
Cite as: | [cond-mat.supr-con] |
(or [cond-mat.supr-con] for this version) | |
Focus to learn more arXiv-issued DOI via DataCite (pending registration) |
Submission history
Access paper:.
- HTML (experimental)
- Other Formats

References & Citations
- Google Scholar
- Semantic Scholar
BibTeX formatted citation

Bibliographic and Citation Tools
Code, data and media associated with this article, recommenders and search tools.
- Institution
arXivLabs: experimental projects with community collaborators
arXivLabs is a framework that allows collaborators to develop and share new arXiv features directly on our website.
Both individuals and organizations that work with arXivLabs have embraced and accepted our values of openness, community, excellence, and user data privacy. arXiv is committed to these values and only works with partners that adhere to them.
Have an idea for a project that will add value for arXiv's community? Learn more about arXivLabs .
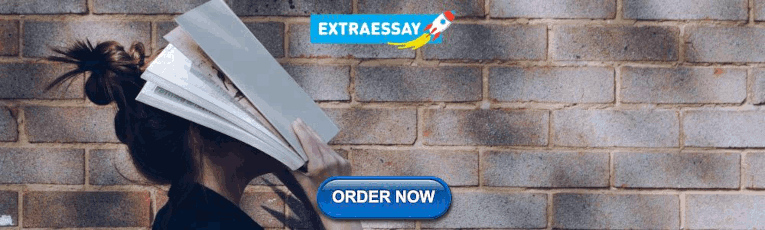
COMMENTS
1. Introduction. In the early 1990s, a trend in the shift from paper-based health records to electronic records started; this was in response to advances in technology as well as the advocacy of the Institute of Medicine in the United States [1,2].As a result of the inadequacies of paper-based health records gradually becoming evident to the healthcare industry [], electronic records have ...
The implementation of Electronic Health Record (EHR) systems is meant to assist providers' evidence-based decision-making 1 and streamline providers' workflow via efficient coordination for patient care. 2 Extant literature has highlighted the benefits of implementing EHR, including improved patient outcomes, enhanced patient safety ...
Electronic Health Record Modernization: Advancing a VA Partnered Research Agenda to Increase Res earch Impacts. Journal of General Internal Medicine, 38(Suppl 4), 965- 973.
The content published in Cureus is the result of clinical experience and/or research by independent individuals or organizations. Cureus is not responsible for the scientific accuracy or reliability of data or conclusions published herein. ... Electronic health record EHR implementation: easy transition from paper to electronic health records ...
As inadequacies of the paper record became increasingly more apparent in 1992 , the Institute of Medicine advocated a shift from a paper-based to an electronic medical record . However, the widespread use of EHRs was delayed by high costs, data entry errors, poor initial physicians' acceptance, and lack of any real incentive.
The proliferation of electronic health records (EHRs) spurred on by federal government incentives over the past few decades has resulted in greater than an 80% adoption-rate at hospitals [] and close to 90% in office-based practices [] in the United States.A natural consequence of the availability of electronic health data is the conduct of research with these data, both observational and ...
Many patients recognize the impact that electronic health records have made. A 2019 poll by the Henry J. Kaiser Family Foundation, a non-profit health-care advocacy organization in San Francisco ...
In the last decade, open-source Electronic Health Record systems (OS-EHRs) have gained notable recognition due to several factors, like, availability without financial barriers, wider compatibility, user-friendliness, and unrestricted modification and restructuring . 11,12 Open-source software in healthcare has also been quite successful as it provides development flexibility, involving users ...
The majority of doctors who use electronic medical records prefer paper records more than electronic medical records because they believe that paper records are much more secure and confidential. This is an indication that the issue of privacy and security on EMR is taken very seriously. ... Robert H. Smith School Research Paper No. RHS-06-011 ...
Background The literature on implementing Electronic Health Records (EHR) in hospitals is very diverse. The objective of this study is to create an overview of the existing literature on EHR implementation in hospitals and to identify generally applicable findings and lessons for implementers. Methods A systematic literature review of empirical research on EHR implementation was conducted ...
Electronic Medical Records (EMRs) are a tool that could potentially improve the outcomes of patient care by providing physicians with access to up-to-date and accurate vital patient information. ... 74 studies were conducted in developed economies, and 58 non-empirical research papers were excluded. The authors then reviewed the remaining ...
An electronic health record (EHR) is a technological inno-vation that consists in digitization of an individual patient's health information. EHRs have already changed the land-scape of biomedical research (Häyrinen et al. 2008; Foley and Fairmichael 2015; Evans 2016). The digitization of a paper-based health record alters its accessibility ...
Table 5 appears in the top ten productivity analyses of authors who contributed to the electronic health records research, their publications, affiliations, totally cited papers, citations, citations impact, and the total cited paper impact. Bates DW, from the USA and affiliated with the Brigham and Women's Hospital, contributed the highest ...
For example, research shows that practice managers migrating from paper-based records have different priorities in EHR implementations than those who previously used legacy EHRs. 10. Previous studies have investigated transitions from paper-based records to locally developed applications 11 and transitioning from a legacy EHR to a more advanced ...
An EHR is an electronic version of a patient's health. record that was historically crea ted, used, and stored in a paper chart. A patient E HR is cr eated, managed, and held. by a healthcare ...
An Electronic Health Record (EHR) is a digital collection and retrieval of a patient's medical records. Widespread implementation of EHR systems decreases health care expenditures.
Abstract. Background: The increased use of electronic health records (EHRs) has resulted in new opportunities for research, but also raises concerns regarding privacy, confidentiality, and patient awareness.Because public trust is essential to the success of the research enterprise, patient perspectives are essential to the development and implementation of ethical approaches to the research ...
Abstract. Electronic health records (EHRs) were implemented to improve quality of care and patient outcomes. This study assessed the relationship between EHR-adoption and patient outcomes. We performed an observational study using State Inpatient Databases linked to American Hospital Association survey, 2011. Surgical and medical patients from ...
The paper-based record was updated manually, resulting in delays for record completion that lasted anywhere from 1 to 6 months or more. ... She was the director of health information management for a long-term care facility, where she helped to implement an electronic health record. Her research interests include childhood obesity. Call for ...
Aims and objectives: To assess and compare the quality of paper-based and electronic-based health records. The comparison examined three criteria: content, documentation process and structure. Background: Nursing documentation is a significant indicator of the quality of patient care delivery. It can be either paper-based or organised within the system known as the electronic health records.
Section 1: Background and Scope. 1.1 BACKGROUND. Electronic Health Records, or EHRs, are a major worldwide initiative to eliminate paper recordings - including Attending Physician Statements (APSs) - of an individual's medical history and current treatment.
1 Terminology Note: Some papers use the terms electronic medical records, electronic health records, and electronic patient records interchangeably, while others draw distinctions between their meanings. For the purpose of consistency and clarification, we use the term electronic health records, shortened to "EHR", in this paper to refer to ...
Background Globally, reduction of patient waiting time has been identified as one of the major characteristics of a functional health system. In South Africa, 83% of the general population visiting primary healthcare (PHC) facilities must contend with long waiting times, overcrowding, staff shortages, poor quality of care, an ineffective appointment booking system, and a lack of medication ...
1. Introduction. The COVID-19 pandemic has substantially accelerated the adoption of healthcare technology, including the use of electronic health records (EHRs) in health and aged care systems in Australia [1,2,3,4,5,6].In general, an EHR system is an integrated aggregate of patients' health records developed and maintained by a variety of different healthcare organizations and kept in an ...
The research team collected study data using an electronic questionnaire containing three sections: socio-demographic data, personal characteristics, and study habits. The study results indicated a statistically significant association between self-fulfillment as a motivation toward studying and academic achievement (p = 0.04).
With the digital transformation, Electronic Medical Records (EMR) have become crucial for operational efficiency and better healthcare. By the end of 2023, all Indonesian healthcare facilities are ...
View a PDF of the paper titled New-record-Tc and three-gap 2D superconductors with electronic and phononic topology: KB2C2, by Hao-Dong Liu and 4 other authors View PDF HTML (experimental) Abstract: Pursuing higher-temperature superconductors under ambient pressure continues to be a prominent topic in materials discovery.