- Skip to main content
- Skip to primary sidebar
- Skip to footer
- QuestionPro

- Solutions Industries Gaming Automotive Sports and events Education Government Travel & Hospitality Financial Services Healthcare Cannabis Technology Use Case NPS+ Communities Audience Contactless surveys Mobile LivePolls Member Experience GDPR Positive People Science 360 Feedback Surveys
- Resources Blog eBooks Survey Templates Case Studies Training Help center

Home Market Research Research Tools and Apps
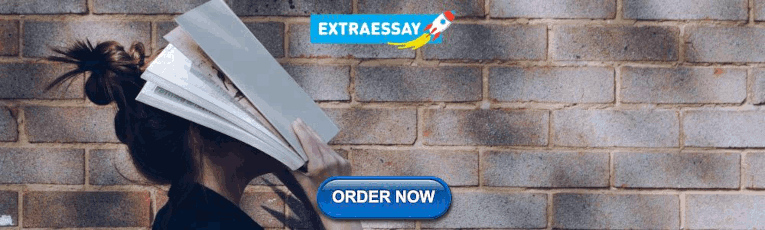
Unit of Analysis: Definition, Types & Examples
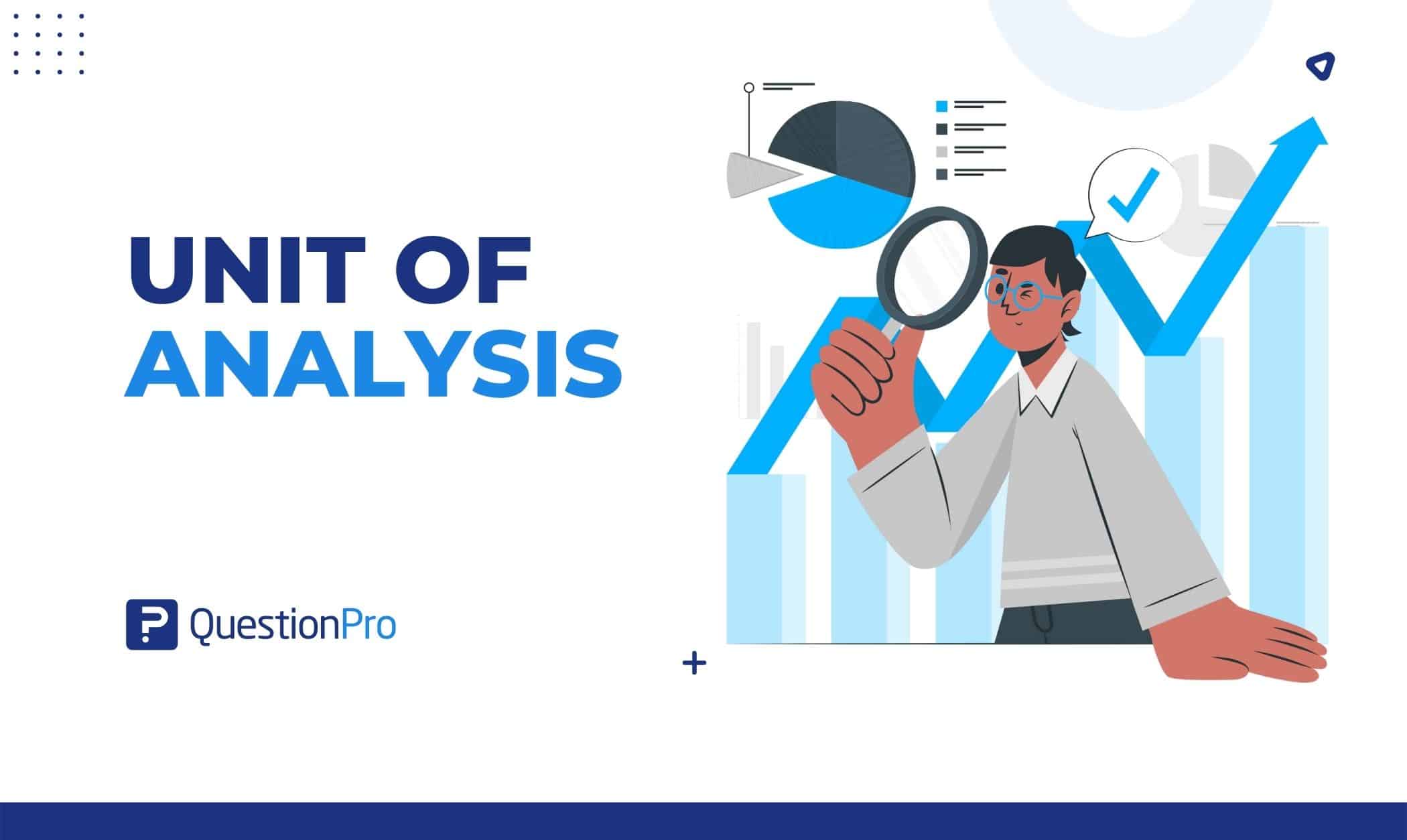
The unit of analysis is the people or things whose qualities will be measured. The unit of analysis is an essential part of a research project. It’s the main thing that a researcher looks at in his research.
A unit of analysis is the object about which you hope to have something to say at the end of your analysis, perhaps the major subject of your research.
In this blog post, we will explore and clarify the concept of the “unit of analysis,” including its definition, various types, and a concluding perspective on its significance.
What is a unit of analysis?
A unit of analysis is the thing you want to discuss after your research, probably what you would regard to be the primary emphasis of your research.
The researcher plans to comment on the primary topic or object in the research as a unit of analysis. The research question plays a significant role in determining it. The “who” or “what” that the researcher is interested in investigating is, to put it simply, the unit of analysis.
In his 2001 book Man, the State, and War, Waltz divides the world into three distinct spheres of study: the individual, the state, and war.
Understanding the reasoning behind the unit of analysis is vital. The likelihood of fruitful research increases if the rationale is understood. An individual, group, organization, nation, social phenomenon, etc., are a few examples.
LEARN ABOUT: Data Analytics Projects
Types of “unit of analysis”
In business research, there are almost unlimited types of possible analytical units. Data analytics and data analysis are closely related processes that involve extracting insights from data to make informed decisions. Even though the most typical unit of analysis is the individual, many research questions can be more precisely answered by looking at other types of units. Let’s find out,
1. Individual Level
The most prevalent unit of analysis in business research is the individual. These are the primary analytical units. The researcher may be interested in looking into:
- Employee actions
- Perceptions
- Attitudes or opinions.
Employees may come from wealthy or low-income families, as well as from rural or metropolitan areas.
A researcher might investigate if personnel from rural areas are more likely to arrive on time than those from urban areas. Additionally, he can check whether workers from rural areas who come from poorer families arrive on time compared to those from rural areas who come from wealthy families.
Each time, the individual (employee) serving as the analytical unit is discussed and explained. Employee analysis as a unit of analysis can shed light on issues in business, including customer and human resource behavior.
For example, employee work satisfaction and consumer purchasing patterns impact business, making research into these topics vital.
Psychologists typically concentrate on research on individuals. This research may significantly aid a firm’s success, as individuals’ knowledge and experiences reveal vital information. Thus, individuals are heavily utilized in business research.
2. Aggregates Level
Social science research does not usually focus on people. However, by combining individuals’ reactions, social scientists frequently describe and explain social interactions, communities, and groupings. Additionally, they research the collective of individuals, including communities, groups, and countries.
Aggregate levels can be divided into Groups (groups with an ad hoc structure) and Organizations (groups with a formal organization).
The following levels of the unit of analysis are made up of groups of people. A group is defined as two or more individuals who interact, share common traits, and feel connected to one another.
Many definitions also emphasize interdependence or objective resemblance (Turner, 1982; Platow, Grace, & Smithson, 2011) and those who identify as group members (Reicher, 1982) .
As a result, society and gangs serve as examples of groups. According to Webster’s Online Dictionary (2012), they can resemble some clubs but be far less formal.
Siblings, identical twins, family, and small group functioning are examples of studies with many units of analysis.
In such circumstances, a whole group might be compared to another. Families, gender-specific groups, pals, Facebook groups, and work departments can all be groups.
By analyzing groups, researchers can learn how they form and how age, experience, class, and gender affect them. When aggregated, an individual’s data describes the group they belong to.
Sociologists study groups like economists and businesspeople to form teams to complete projects. They continually research groups and group behavior.
Organizations
The next level of the unit of analysis is organizations, which are groups of people set up formally. Organizations could include businesses, religious groups, parts of the military, colleges, academic departments, supermarkets, business groups, and so on.
The social organization includes things like sexual composition, styles of leadership, organizational structure, systems of communication, and so on. (Susan & Wheelan, 2005; Chapais & Berman, 2004) . (Lim, Putnam, and Robert, 2010) say that well-known social organizations and religious institutions are among them.
Moody, White, and Douglas (2003) say social organizations are hierarchical. Hasmath, Hildebrandt, and Hsu (2016) say social organizations can take different forms. For example, they can be made by institutions like schools or governments.
Sociology, economics, political science, psychology, management, and organizational communication are some social science fields that study organizations (Douma & Schreuder, 2013) .
Organizations are different from groups in that they are more formal and have better organization. A researcher might want to study a company to generalize its results to the whole population of companies.
One way to look at an organization is by the number of employees, the net annual revenue, the net assets, the number of projects, and so on. He might want to know if big companies hire more or fewer women than small companies.
Organization researchers might be interested in how companies like Reliance, Amazon, and HCL affect our social and economic lives. People who work in business often study business organizations.
LEARN ABOUT: Data Management Framework
3. Social Level
The social level has 2 types,
Social Artifacts Level
Things are studied alongside humans. Social artifacts are human-made objects from diverse communities. Social artifacts are items, representations, assemblages, institutions, knowledge, and conceptual frameworks used to convey, interpret, or achieve a goal (IGI Global, 2017).
Cultural artifacts are anything humans generate that reveals their culture (Watts, 1981).
Social artifacts include books, newspapers, advertising, websites, technical devices, films, photographs, paintings, clothes, poems, jokes, students’ late excuses, scientific breakthroughs, furniture, machines, structures, etc. Infinite.
Humans build social objects for social behavior. As people or groups suggest a population in business research, each social object implies a class of items.
Same-class goods include business books, magazines, articles, and case studies. A business magazine’s quantity of articles, frequency, price, content, and editor in a research study may be characterized.
Then, a linked magazine’s population might be evaluated for description and explanation. Marx W. Wartofsky (1979) defined artifacts as primary artifacts utilized in production (like a camera), secondary artifacts connected to primary artifacts (like a camera user manual), and tertiary objects related to representations of secondary artifacts (like a camera user-manual sculpture).
The scientific study of an artifact reveals its creators and users. The artifact researcher may be interested in advertising, marketing, distribution, buying, etc.
Social Interaction Level
Social artifacts include social interaction. Such as:
- Eye contact with a coworker
- Buying something in a store
- Friendship decisions
- Road accidents
- Airline hijackings
- Professional counseling
- Whatsapp messaging
A researcher might study youthful employees’ smartphone addictions. Some addictions may involve social media, while others involve online games and movies that inhibit connection.
Smartphone addictions are examined as a societal phenomenon. Observation units are probably individuals (employees).
Anthropologists typically study social artifacts. They may be interested in the social order. A researcher who examines social interactions may be interested in how broader societal structures and factors impact daily behavior, festivals, and weddings.
LEARN ABOUT: Level of Analysis
Even though there is no perfect way to do research, it is generally agreed that researchers should try to find a unit of analysis that keeps the context needed to make sense of the data.
Researchers should consider the details of their research when deciding on the unit of analysis.
They should remember that consistent use of these units throughout the analysis process (from coding to developing categories and themes to interpreting the data) is essential to gaining insight from qualitative data and protecting the reliability of the results.
QuestionPro does much more than merely serve as survey software. We have a solution for every sector of the economy and every kind of issue. We also have systems for managing data, such as our research repository, Insights Hub.
LEARN MORE FREE TRIAL
MORE LIKE THIS
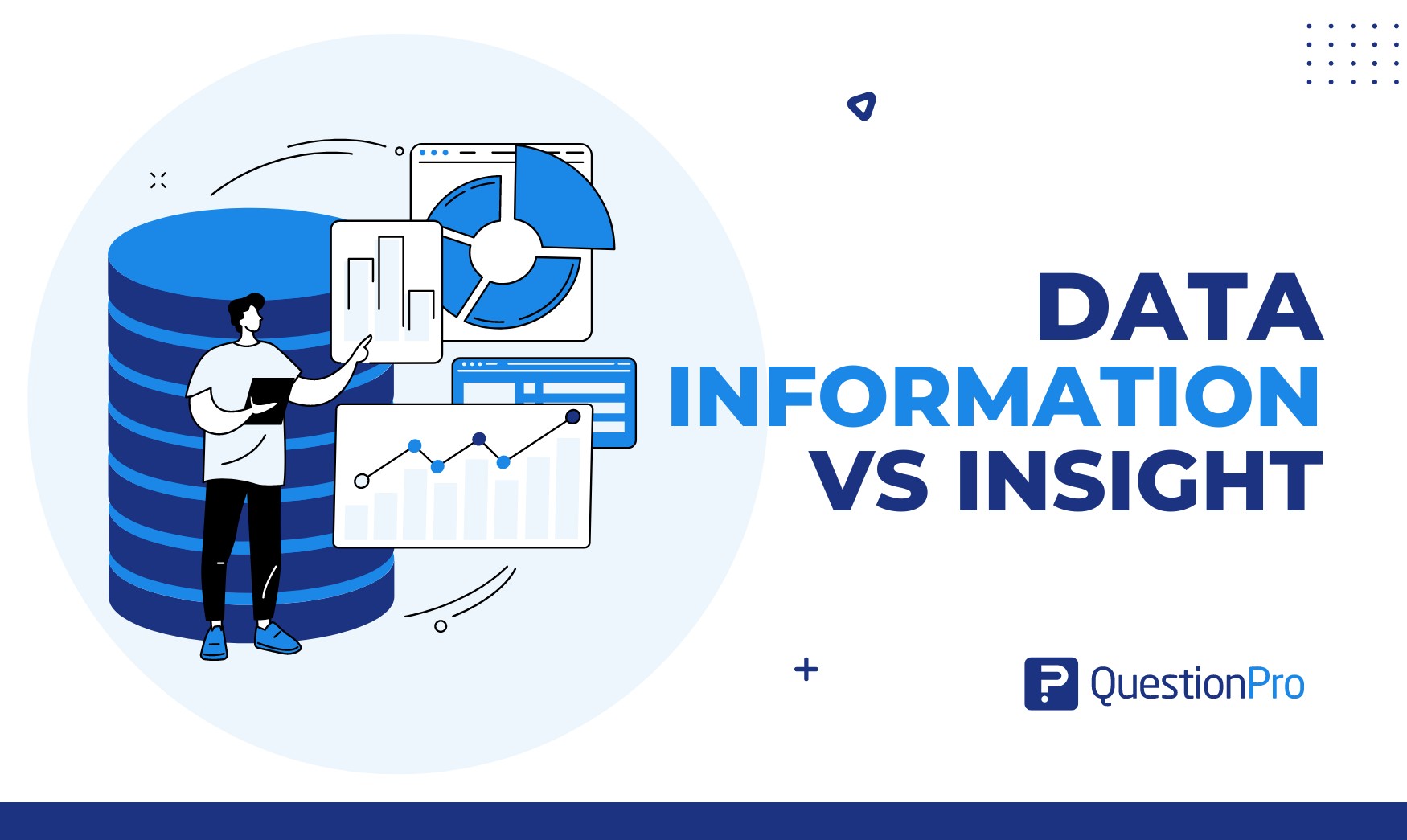
Data Information vs Insight: Essential differences
May 14, 2024
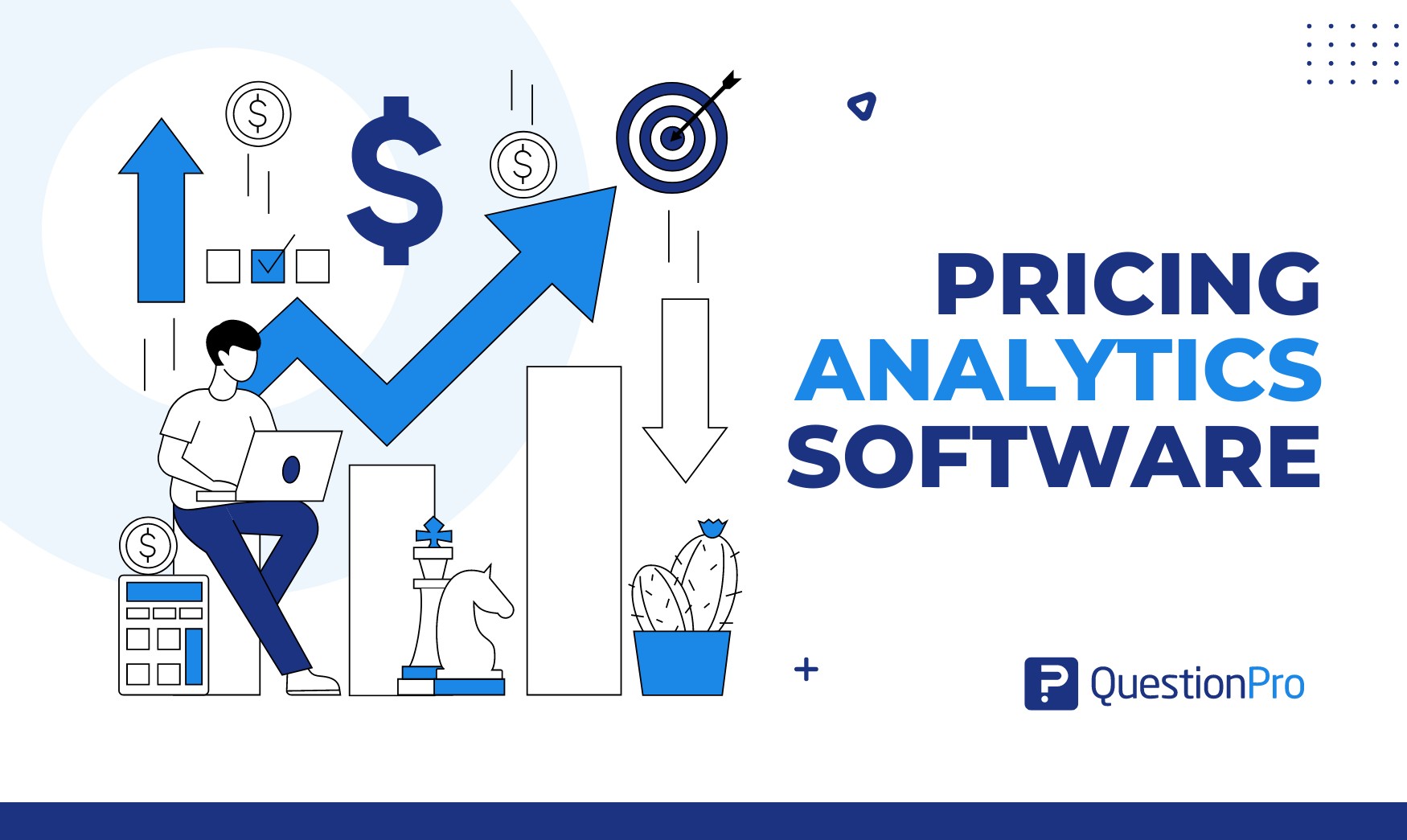
Pricing Analytics Software: Optimize Your Pricing Strategy
May 13, 2024
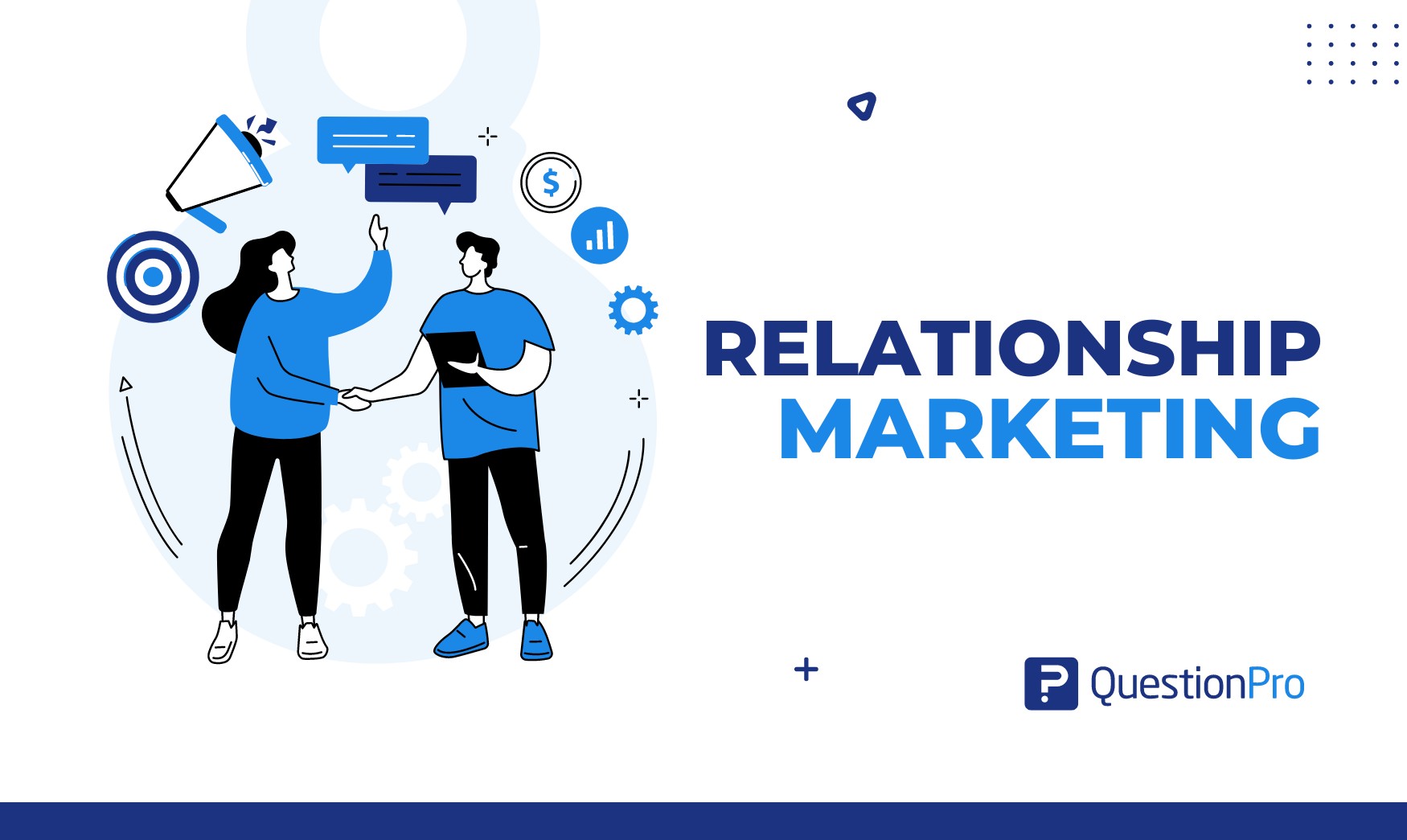
Relationship Marketing: What It Is, Examples & Top 7 Benefits
May 8, 2024
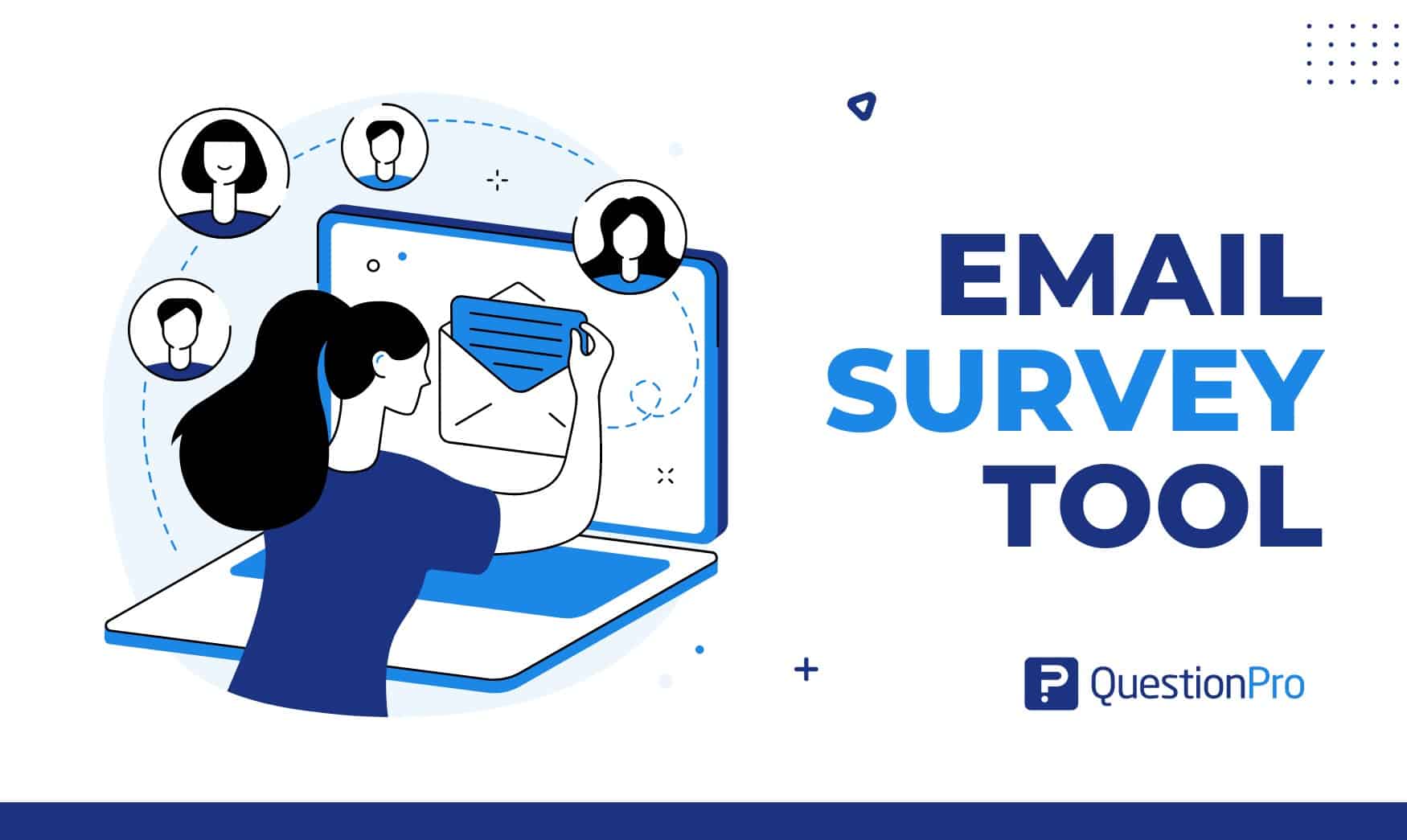
The Best Email Survey Tool to Boost Your Feedback Game
May 7, 2024
Other categories
- Academic Research
- Artificial Intelligence
- Assessments
- Brand Awareness
- Case Studies
- Communities
- Consumer Insights
- Customer effort score
- Customer Engagement
- Customer Experience
- Customer Loyalty
- Customer Research
- Customer Satisfaction
- Employee Benefits
- Employee Engagement
- Employee Retention
- Friday Five
- General Data Protection Regulation
- Insights Hub
- Life@QuestionPro
- Market Research
- Mobile diaries
- Mobile Surveys
- New Features
- Online Communities
- Question Types
- Questionnaire
- QuestionPro Products
- Release Notes
- Research Tools and Apps
- Revenue at Risk
- Survey Templates
- Training Tips
- Uncategorized
- Video Learning Series
- What’s Coming Up
- Workforce Intelligence
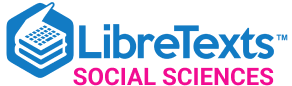
- school Campus Bookshelves
- menu_book Bookshelves
- perm_media Learning Objects
- login Login
- how_to_reg Request Instructor Account
- hub Instructor Commons
Margin Size
- Download Page (PDF)
- Download Full Book (PDF)
- Periodic Table
- Physics Constants
- Scientific Calculator
- Reference & Cite
- Tools expand_more
- Readability
selected template will load here
This action is not available.
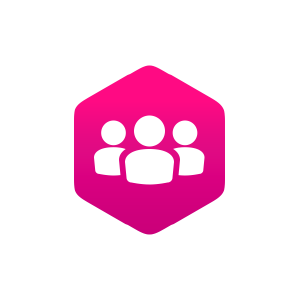
5.7: Communication Research Summary
- Last updated
- Save as PDF
- Page ID 184634
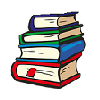
- Scott T. Paynton & Laura K. Hahn with Humboldt State University Students
- Humboldt State University
\( \newcommand{\vecs}[1]{\overset { \scriptstyle \rightharpoonup} {\mathbf{#1}} } \)
\( \newcommand{\vecd}[1]{\overset{-\!-\!\rightharpoonup}{\vphantom{a}\smash {#1}}} \)
\( \newcommand{\id}{\mathrm{id}}\) \( \newcommand{\Span}{\mathrm{span}}\)
( \newcommand{\kernel}{\mathrm{null}\,}\) \( \newcommand{\range}{\mathrm{range}\,}\)
\( \newcommand{\RealPart}{\mathrm{Re}}\) \( \newcommand{\ImaginaryPart}{\mathrm{Im}}\)
\( \newcommand{\Argument}{\mathrm{Arg}}\) \( \newcommand{\norm}[1]{\| #1 \|}\)
\( \newcommand{\inner}[2]{\langle #1, #2 \rangle}\)
\( \newcommand{\Span}{\mathrm{span}}\)
\( \newcommand{\id}{\mathrm{id}}\)
\( \newcommand{\kernel}{\mathrm{null}\,}\)
\( \newcommand{\range}{\mathrm{range}\,}\)
\( \newcommand{\RealPart}{\mathrm{Re}}\)
\( \newcommand{\ImaginaryPart}{\mathrm{Im}}\)
\( \newcommand{\Argument}{\mathrm{Arg}}\)
\( \newcommand{\norm}[1]{\| #1 \|}\)
\( \newcommand{\Span}{\mathrm{span}}\) \( \newcommand{\AA}{\unicode[.8,0]{x212B}}\)
\( \newcommand{\vectorA}[1]{\vec{#1}} % arrow\)
\( \newcommand{\vectorAt}[1]{\vec{\text{#1}}} % arrow\)
\( \newcommand{\vectorB}[1]{\overset { \scriptstyle \rightharpoonup} {\mathbf{#1}} } \)
\( \newcommand{\vectorC}[1]{\textbf{#1}} \)
\( \newcommand{\vectorD}[1]{\overrightarrow{#1}} \)
\( \newcommand{\vectorDt}[1]{\overrightarrow{\text{#1}}} \)
\( \newcommand{\vectE}[1]{\overset{-\!-\!\rightharpoonup}{\vphantom{a}\smash{\mathbf {#1}}}} \)
Communication research is important because it focuses on a common goal—to enhance our interactions with others. In this chapter we highlighted how research is done and the basic steps that guide most research projects—identify the topic, write a research question, define key terms, select a methodology, establish a sample, gather and analyze the data, and finally, interpret and share the results. When conducting research, three factors motivate the choices we make: our intended outcomes, theoretical preferences, and methodological preferences. Depending on these factors, research may lead us to greater understanding, allow us to predict or control a communication situation, or create cultural change.
Conceptualizing Communication research can be done more easily by understanding the three broad methodological approaches/paradigms for conducting Communication research: Rhetorical Methodologies, Quantitative Methodologies, and Qualitative Methodologies. The rhetorical approach evaluates messages in various contexts such as political discourse, art, and popular culture. A variety of methods are available such as neo-aristotelian, fantasy theme, narrative, pentadic, feminist, and ideological criticism. Quantitative methods are characterized by counting phenomena and are useful for predicting communication outcomes or comparing cultures and populations. They include experimental research, surveys, content analysis, and meta-analysis. Qualitative methods offer the opportunity to understand human communication as it occurs in a “natural” context rather than a laboratory setting. This is accomplished through ethnography, focus groups, action research, unobtrusive research, historiography, and case studies. While these approaches share similarities, their focus and specific methods are quite different and produce different outcomes. No research methodology or method is better than another. Instead, approaches to Communication research simply reveal different aspects of human communication in action.
DISCUSSION QUESTIONS
- If you were going to conduct communication research, what topic(s) would be most interesting to you? What specific questions would you want to ask and answer? How would you go about doing this?
- Of the three broad research methodologies, do you find yourself having a preference for one of them? If so, what specific type of research method would you want to use within the area you have a preference for?
- If you were going to conduct research, what outcome would you want to gain from your research? Are you more interested in understanding, prediction/control, or creating social change? What is the value of each of these approaches?
- action research
- case studies
- content analysis
- continuum of intended outcomes
- control group
- critical/cultural change
- ethnography
- experimental group
- experimental research
- fantasy theme
- focus group interviewing
- historiography
- ideological
- meta-analysis
- methodological preferences
- methodology
- neo-Aristotelian
- prediction/control
- qualitative methodologies
- quantitative methodologies
- research focus
- research questions
- rhetorical methodologies
- survey research
- theoretical preferences
- understanding
- unobtrusive research
Contributions and Affiliations
- Survey of Communication Study. Authored by : Scott T Paynton and Linda K Hahn. Provided by : Humboldt State University. Located at : en.wikibooks.org/wiki/Survey_of_Communication_Study/Preface. License : CC BY-SA: Attribution-ShareAlike
Unit of analysis: definition, types, examples, and more
Last updated
16 April 2023
Reviewed by
Cathy Heath
Make research less tedious
Dovetail streamlines research to help you uncover and share actionable insights
- What is a unit of analysis?
A unit of analysis is an object of study within a research project. It is the smallest unit a researcher can use to identify and describe a phenomenon—the 'what' or 'who' the researcher wants to study.
For example, suppose a consultancy firm is hired to train the sales team in a solar company that is struggling to meet its targets. To evaluate their performance after the training, the unit of analysis would be the sales team—it's the main focus of the study.
Different methods, such as surveys , interviews, or sales data analysis, can be used to evaluate the sales team's performance and determine the effectiveness of the training.
- Units of observation vs. units of analysis
A unit of observation refers to the actual items or units being measured or collected during the research. In contrast, a unit of analysis is the entity that a researcher can comment on or make conclusions about at the end of the study.
In the example of the solar company sales team, the unit of observation would be the individual sales transactions or deals made by the sales team members. In contrast, the unit of analysis would be the sales team as a whole.
The firm may observe and collect data on individual sales transactions, but the ultimate conclusion would be based on the sales team's overall performance, as this is the entity that the firm is hired to improve.
In some studies, the unit of observation may be the same as the unit of analysis, but researchers need to define both clearly to themselves and their audiences.
- Unit of analysis types
Below are the main types of units of analysis:
Individuals – These are the smallest levels of analysis.
Groups – These are people who interact with each other.
Artifacts –These are material objects created by humans that a researcher can study using empirical methods.
Geographical units – These are smaller than a nation and range from a province to a neighborhood.
Social interactions – These are formal or informal interactions between society members.
- Importance of selecting the correct unit of analysis in research
Selecting the correct unit of analysis helps reveal more about the subject you are studying and how to continue with the research. It also helps determine the information you should use in the study. For instance, if a researcher has a large sample, the unit of analysis will help decide whether to focus on the whole population or a subset of it.
- Examples of a unit of analysis
Here are examples of a unit of analysis:
Individuals – A person, an animal, etc.
Groups – Gangs, roommates, etc.
Artifacts – Phones, photos, books, etc.
Geographical units – Provinces, counties, states, or specific areas such as neighborhoods, city blocks, or townships
Social interaction – Friendships, romantic relationships, etc.
- Factors to consider when selecting a unit of analysis
The main things to consider when choosing a unit of analysis are:
Research questions and hypotheses
Research questions can be descriptive if the study seeks to describe what exists or what is going on.
It can be relational if the study seeks to look at the relationship between variables. Or, it can be causal if the research aims at determining whether one or more variables affect or cause one or more outcome variables.
Your study's research question and hypothesis should guide you in choosing the correct unit of analysis.
Data availability and quality
Consider the nature of the data collected and the time spent observing each participant or studying their behavior. You should also consider the scale used to measure variables.
Some studies involve measuring every variable on a one-to-one scale, while others use variables with discrete values. All these influence the selection of a unit of analysis.
Feasibility and practicality
Look at your study and think about the unit of analysis that would be feasible and practical.
Theoretical framework and research design
The theoretical framework is crucial in research as it introduces and describes the theory explaining why the problem under research exists. As a structure that supports the theory of a study, it is a critical consideration when choosing the unit of analysis. Moreover, consider the overall strategy for collecting responses to your research questions.
- Common mistakes when choosing a unit of analysis
Below are common errors that occur when selecting a unit of analysis:
Reductionism
This error occurs when a researcher uses data from a lower-level unit of analysis to make claims about a higher-level unit of analysis. This includes using individual-level data to make claims about groups.
However, claiming that Rosa Parks started the movement would be reductionist. There are other factors behind the rise and success of the US civil rights movement. These include the Supreme Court’s historic decision to desegregate schools, protests over legalized racial segregation, and the formation of groups such as the Student Nonviolent Coordinating Committee (SNCC). In short, the movement is attributable to various political, social, and economic factors.
Ecological fallacy
This mistake occurs when researchers use data from a higher-level unit of analysis to make claims about one lower-level unit of analysis. It usually occurs when only group-level data is collected, but the researcher makes claims about individuals.
For instance, let's say a study seeks to understand whether addictions to electronic gadgets are more common in certain universities than others.
The researcher moves on and obtains data on the percentage of gadget-addicted students from different universities around the country. But looking at the data, the researcher notes that universities with engineering programs have more cases of gadget additions than campuses without the programs.
Concluding that engineering students are more likely to become addicted to their electronic gadgets would be inappropriate. The data available is only about gadget addiction rates by universities; thus, one can only make conclusions about institutions, not individual students at those universities.
Making claims about students while the data available is about the university puts the researcher at risk of committing an ecological fallacy.
- The lowdown
A unit of analysis is what you would consider the primary emphasis of your study. It is what you want to discuss after your study. Researchers should determine a unit of analysis that keeps the context required to make sense of the data. They should also keep the unit of analysis in mind throughout the analysis process to protect the reliability of the results.
What is the most common unit of analysis?
The individual is the most prevalent unit of analysis.
Can the unit of analysis and the unit of observation be one?
Some situations have the same unit of analysis and observation. For instance, let's say a tutor is hired to improve the oral French proficiency of a student who finds it difficult. A few months later, the tutor wants to evaluate the student's proficiency based on what they have taught them for the time period. In this case, the student is both the unit of analysis and the unit of observation.
Should you be using a customer insights hub?
Do you want to discover previous research faster?
Do you share your research findings with others?
Do you analyze research data?
Start for free today, add your research, and get to key insights faster
Editor’s picks
Last updated: 11 January 2024
Last updated: 15 January 2024
Last updated: 17 January 2024
Last updated: 12 May 2023
Last updated: 30 April 2024
Last updated: 18 May 2023
Last updated: 25 November 2023
Last updated: 13 May 2024
Latest articles
Related topics, .css-je19u9{-webkit-align-items:flex-end;-webkit-box-align:flex-end;-ms-flex-align:flex-end;align-items:flex-end;display:-webkit-box;display:-webkit-flex;display:-ms-flexbox;display:flex;-webkit-flex-direction:row;-ms-flex-direction:row;flex-direction:row;-webkit-box-flex-wrap:wrap;-webkit-flex-wrap:wrap;-ms-flex-wrap:wrap;flex-wrap:wrap;-webkit-box-pack:center;-ms-flex-pack:center;-webkit-justify-content:center;justify-content:center;row-gap:0;text-align:center;max-width:671px;}@media (max-width: 1079px){.css-je19u9{max-width:400px;}.css-je19u9>span{white-space:pre;}}@media (max-width: 799px){.css-je19u9{max-width:400px;}.css-je19u9>span{white-space:pre;}} decide what to .css-1kiodld{max-height:56px;display:-webkit-box;display:-webkit-flex;display:-ms-flexbox;display:flex;-webkit-align-items:center;-webkit-box-align:center;-ms-flex-align:center;align-items:center;}@media (max-width: 1079px){.css-1kiodld{display:none;}} build next, decide what to build next.

Users report unexpectedly high data usage, especially during streaming sessions.

Users find it hard to navigate from the home page to relevant playlists in the app.

It would be great to have a sleep timer feature, especially for bedtime listening.

I need better filters to find the songs or artists I’m looking for.
Log in or sign up
Get started for free
Choosing the Right Unit of Analysis for Your Research Project
Table of content.
- Understanding the Unit of Analysis in Research
- Factors to Consider When Selecting the Right Unit of Analysis
- Common Mistakes to Avoid
A research project is like setting out on a voyage through uncharted territory; the unit of analysis is your compass, guiding every decision from methodology to interpretation.
It’s the beating heart of your data collection and the lens through which you view your findings. With deep-seated experience in research methodologies , our expertise recognizes that choosing an appropriate unit of analysis not only anchors your study but illuminates paths towards meaningful conclusions.
The right choice empowers researchers to extract patterns, answer pivotal questions, and offer insights into complex phenomena. But tread carefully—selecting an ill-suited unit can distort results or obscure significant relationships within data.
Remember this: A well-chosen unit of analysis acts as a beacon for accuracy and relevance throughout your scholarly inquiry. Continue reading to unlock the strategies for selecting this cornerstone of research design with precision—your project’s success depends on it.
Engage with us as we delve deeper into this critical aspect of research mastery.
Key Takeaways
- Your research questions and hypotheses drive the choice of your unit of analysis, shaping how you collect and interpret data.
- Avoid common mistakes like reductionism , which oversimplifies complex issues, and the ecological fallacy , where group-level findings are wrongly applied to individuals.
- Consider the availability and quality of data when selecting your unit of analysis to ensure your research is feasible and conclusions are valid.
- Differentiate between units of analysis (what you’re analyzing) and units of observation (what or who you’re observing) for clarity in your study.
- Ensure that your chosen unit aligns with both the theoretical framework and practical considerations such as time and resources.
The unit of analysis in research refers to the level at which data is collected and analyzed. It is essential for researchers to understand the different types of units of analysis, as well as their significance in shaping the research process and outcomes.
Definition and Importance
With resonio, the unit of analysis you choose lays the groundwork for your market research focus. Whether it’s individuals, organizations, or specific events, resonio’s platform facilitates targeted data collection and analysis to address your unique research questions. Our tool simplifies this selection process, ensuring that you can efficiently zero in on the most relevant unit for insightful and actionable results.
This crucial component serves as a navigational aid for your market research. The market research tool not only guides you in data collection but also in selecting the most effective sampling methods and approaches to hypothesis testing. Getting robust and reliable data, ensuring your research is both effective and straightforward.
Choosing the right unit of analysis is crucial, as it defines your research’s direction. resonio makes this easier, ensuring your choice aligns with your theoretical approach and data collection methods, thereby enhancing the validity and reliability of your results.
Additionally, resonio aids in steering clear of errors like reductionism and ecological fallacy, ensuring your conclusions match the data’s level of analysis
Difference between Unit of Analysis and Unit of Observation
Understanding the difference between the unit of analysis and observation is key. Let us clarify this distinction: the unit of analysis is what you’ll ultimately analyze, while the unit of observation is what you observe or measure during the study.
For example, in using resonio for educational research, individual test scores are the units of analysis, while the students providing these scores are the units of observation.
This distinction is essential as it clarifies the specific aspect under scrutiny and what will yield measurable data. It also emphasizes that researchers must carefully consider both elements to ensure their alignment with research questions and objectives .
Types of Units of Analysis: Individual, Aggregates, and Social
Choosing the right unit of analysis for a research project is critical. The types of units of analysis include individual, aggregates, and social.
- Individual: This type focuses on analyzing the attributes and characteristics of individual units, such as people or specific objects.
- Aggregates: Aggregates involve analyzing groups or collections of individual units, such as neighborhoods, organizations, or communities.
- Social: Social units of analysis emphasize analyzing broader social entities, such as cultures, societies, or institutions.
When selecting the right unit of analysis for a research project, researchers must consider various factors such as their research questions and hypotheses , data availability and quality, feasibility and practicality, as well as the theoretical framework and research design .
Each of these factors plays a crucial role in determining the most appropriate unit of analysis for the study.
Research Questions and Hypotheses
The research questions and hypotheses play a crucial role in determining the appropriate unit of analysis for a research project. They guide the researcher in identifying what exactly needs to be studied and analyzed, thereby influencing the selection of the most relevant unit of analysis.
The alignment between the research questions/hypotheses and the unit of analysis is essential to ensure that the study’s focus meets its intended objectives. Furthermore, clear research questions and hypotheses help define specific parameters for data collection and analysis, directly impacting which unit of analysis will best serve the study’s purpose.
It’s important to carefully consider how each research question or hypothesis relates to different potential units of analysis , as this connection will shape not only what you are studying but also how you will study it .
Data Availability and Quality
When considering the unit of analysis for a research project, researchers must take into account the availability and quality of data. The chosen unit of analysis should align with the available data sources to ensure that meaningful and accurate conclusions can be drawn.
Researchers need to evaluate whether the necessary data at the chosen level of analysis is accessible and reliable. Ensuring high-quality data will contribute to the validity and reliability of the study , enabling researchers to make sound interpretations and draw robust conclusions from their findings.
Choosing a unit of analysis without considering data availability and quality may lead to limitations in conducting thorough analysis or drawing valid conclusions. It is crucial for researchers to assess both factors before finalizing their selection, as it directly impacts the feasibility, accuracy, and rigor of their research project.
Feasibility and Practicality
When considering the feasibility and practicality of a unit of analysis for a research project, it is essential to assess the availability and quality of data related to the chosen unit.
Researchers should also evaluate whether the selected unit aligns with their theoretical framework and research design. The practical aspects such as time, resources, and potential challenges associated with analyzing the chosen unit must be thoroughly considered before finalizing the decision.
Moreover, it is crucial to ensure that the selected unit of analysis is feasible within the scope of the research questions and hypotheses. Additionally, researchers need to determine if the chosen unit can be effectively studied based on existing literature and sampling techniques utilized in similar studies.
By carefully evaluating these factors, researchers can make informed decisions regarding which unit of analysis will best suit their research goals.
Theoretical Framework and Research Design
The theoretical framework and research design establish the structure for a study based on existing theories and concepts. It guides the selection of the unit of analysis by providing a foundation for understanding how variables interact and influence one another.
Theoretical frameworks help to shape research questions , hypotheses, and data collection methods, ensuring that the chosen unit of analysis aligns with the study’s objectives. Research design serves as a blueprint outlining the procedures and techniques used to gather and analyze data, allowing researchers to make informed decisions regarding their unit of analysis while considering feasibility, practicality, and data availability .
Researchers often make the mistake of reductionism, where they oversimplify complex phenomena by focusing on one aspect. Another common mistake is the ecological fallacy, where conclusions about individual behavior are made based on group-level data.
Reductionism
Reductionism occurs when a researcher oversimplifies a complex phenomenon by analyzing it at too basic a level. This can lead to the loss of important nuances and details critical for understanding the broader context.
For instance, studying individual test scores without considering external factors like teaching quality or student motivation is reductionist. By focusing solely on one aspect, researchers miss out on comprehensive insights that may impact their findings.
In research projects, reductionism limits the depth of analysis and may result in skewed conclusions that don’t accurately reflect the real-world complexities. It’s essential for researchers to avoid reductionism by carefully selecting an appropriate unit of analysis that allows for a holistic understanding of the phenomenon under study.
Ecological Fallacy
The ecological fallacy involves making conclusions about individuals based on group-level data . This occurs when researchers mistakenly assume that relationships observed at the aggregate level also apply to individuals within that group.
For example, if a study finds a correlation between high levels of education and income at the city level, it doesn’t mean the same relationship applies to every individual within that city.
This fallacy can lead to erroneous generalizations and inaccurate assumptions about individuals based on broader trends. It is crucial for researchers to be mindful of this potential pitfall when selecting their unit of analysis, ensuring that their findings accurately represent the specific characteristics and behaviors of the individuals or entities under investigation.
Selecting the appropriate unit of analysis is critical for a research project’s success, shaping its focus and scope. Researchers must carefully align the chosen unit with their study objectives to ensure relevance.
The impact on findings and conclusions from this choice cannot be understated. Correctly choosing the unit of analysis can considerably influence the direction and outcomes of a research undertaking.
Robert Koch
I write about AI, SEO, Tech, and Innovation. Led by curiosity, I stay ahead of AI advancements. I aim for clarity and understand the necessity of change, taking guidance from Shaw: 'Progress is impossible without change,' and living by Welch's words: 'Change before you have to'.
- Privacy Overview
- Strictly Necessary Cookies
- Additional Cookies
This website uses cookies to provide you with the best user experience possible. Cookies are small text files that are cached when you visit a website to make the user experience more efficient. We are allowed to store cookies on your device if they are absolutely necessary for the operation of the site. For all other cookies we need your consent.
You can at any time change or withdraw your consent from the Cookie Declaration on our website. Find the link to your settings in our footer.
Find out more in our privacy policy about our use of cookies and how we process personal data.
Necessary cookies help make a website usable by enabling basic functions like page navigation and access to secure areas of the website. The website cannot properly without these cookies.
If you disable this cookie, we will not be able to save your preferences. This means that every time you visit this website you will need to enable or disable cookies again.
Any cookies that may not be particularly necessary for the website to function and is used specifically to collect user personal data via analytics, ads, other embedded contents are termed as additional cookies.
Please enable Strictly Necessary Cookies first so that we can save your preferences!
- Search Menu
- Author Guidelines
- Submission Site
- Self-Archiving Policy
- Why Submit?
- About Journal of Computer-Mediated Communication
- About International Communication Association
- Editorial Board
- Advertising & Corporate Services
- Journals Career Network
- Journals on Oxford Academic
- Books on Oxford Academic
Article Contents
Defining internet-based, computer-mediated communication, defining units of analysis for internet-based communication, integrating diverse landscapes.
- < Previous
Units of Analysis for Internet Communication
- Article contents
- Figures & tables
- Supplementary Data
John December, Units of Analysis for Internet Communication, Journal of Computer-Mediated Communication , Volume 1, Issue 4, 1 March 1996, JCMC143, https://doi.org/10.1111/j.1083-6101.1996.tb00173.x
- Permissions Icon Permissions
When communication researchers make claims about the relationship of media to individuals or society, they use the term media to mean a variety of things. For example, a researcher might try to prove the claim “television causes X ,” where X might be anything from aggressive behavior to bad vision or a crisis in a sense of self. In this case, is the researcher talking about the television signals in the air, people who work at television stations, people who produce or act in television programs, the television programs themselves, television receiving sets, or some or all of these things?
Of course, the research context, setting, and constraints usually define what is meant by the term television as a medium, but how can researchers talk about new media that involve communication on computer networks? The Internet, a cooperatively run, globally distributed collection of computer networks, provides a communication forum in which an estimated 20-40 million people in 90 countries ( Society , 1995 ) participate. The Internet provides an array of tools for people to use for information retrieval and communication in individual, group, and mass contexts, but can current notions of media be used to define communication on the Internet?
Researchers in past decades have taken many approaches to analyzing human communication on computer and networked communication systems. Using a variety of frameworks for defining units of analysis, these researchers have examined an array of communication settings. For example, some research has explored the relationships between the characteristics of media systems and the characteristics of individuals using them ( Hiltz & Turoff, 1978 ; Johansen, Valle, & Spangler, 1979 ). Other researchers have examined the human component of computer-mediated communication processes in detail, examining social-psychological factors ( Kiesler, Siegel, & McGuire, 1984 ; Kling & Gerson, 1977 ; Lea & Spears, 1991a, 1991b ; McGuire, 1983 ; Spears & Lea, 1992 ; Spears, Lea, & Lee, 1990 ), as well as social context factors ( Feenberg, 1989, 1992 ; Fulk, Schmitz, & Steinfield, 1990 ; Fulk, Steinfield, Schmitz, & Power, 1987 ; Georgoudi & Rosnow, 1985 ; Lea, 1992 ; Martin, O'Shea, Fung, & Spears, 1992 ; Schmitz & Fulk, 1991 ), and social cues ( DeSanctis & Gallupe, 1987 ; Kiesler, 1986 ; McGuire, Kiesler, & Siegel, 1987 ; Rutter, 1987 ; Siegel, Dubrovsky, Kiesler, & McGuire, 1986 ; Sproull & Kiesler, 1986 ). This body of work presents a mixture of results that are very dependent on the context of the research setting. Integration of results, particularly at the theoretical level, is difficult.
Research focusing on media has likewise lead to insights, but little theoretical integration or comparison of results from study to study. Researchers have examined the diffusion and adoption of interactive media and found factors contributing to media technology adoption as well as patterns of how technology use develops in a community ( Markus, 1987, 1990 ; Miles, 1992 ; Rogers, 1983, 1986 ; Valente, 1991 ). Other researchers and writers have looked at media evolution to identify societal and individual changes as a result of the use of communication technology ( Harnad, 1991 ; Havelock, 1986 ; Innis, 1972 ; Levinson, 1990 ; McLuhan, 1964, 1965 ; McLuhan & Fiore, 1967 ; McLuhan & Powers, 1989 ; Vallee, 1982 ). More recently, media researchers have examined the notion of “media richness” to examine media selection in individual and organizational communication ( Daft & Lengel, 1984, 1986 ; Daft, Lengel, & Trevino, 1987 ; Lengel & Daft, 1988 ; Rice & Shook, 1990 ; Trevino, Daft, & Lengel, 1990 ; Trevino, Lengel, Bodensteiner, Gerloff, & Muir, 1990 ; Trevino, Lengel, & Daft, 1987 ). This focus on media uses a variety of frameworks for defining units of analysis, or fails to define any units of analysis. As a result, it is difficult to piece together an integrative model to explain and predict media use, adoption, and evolution patterns, or even classify study results or theoretical statements.
Another approach to researching on-line communication is a focus on language and rhetoric. Researchers in these areas have likewise discovered many insights into the structure and content of computer-mediated communication and how literacy and orality are affected by communication technology ( Baron, 1984 ; Black, Levin, Mehan & Quinn, 1983 ; Ferrara, Brunner & Whittemore, 1991 ; Finnegan, 1988 ; Gurak, 1994 ; Lakoff, 1982 ; Murray, 1991 ; Ochs, 1989 ; Ong, 1977, 1982 ; Shank, 1993 ; Spitzer, 1986 ). These studies have examined a variety of on-line content and used many schemes for defining or discussing units of analysis.
Murray (1991) , for example, looked at electronic documents and mail used for interpersonal and small group communication on a proprietary computer network, and identified cognitive and contextual strategies for writing documents on personal computers and using electronic mail in a study of an IBM project manager and his colleagues. Shank (1993) examined electronic mailing lists involving a large number of people, many of whom are unknown to each other, on the Internet or other networks. Shank argued that communication in these on-line discussion lists is neither oral nor written, but semiotic. Can the Murray and Shank results be compared? Each researcher looked at different uses of computer-mediated communication, and thus used different units of analysis for the communication. Without careful attention to the definition of the units of analysis researchers used, it is difficult to integrate the results of these studies.
Over the decades, research in computer-mediated communication has also explored myriad on-line experiences, focusing on human and social characteristics, media, and language and rhetorical content. However, the research has not led to much successful theoretical integration or cross-study comparisons. Indeed, some research has directly contradicted previous work ( Lea, 1992 ). Researchers have articulated the units of analysis in studies on a case-by case basis. This diversity in terminology has been necessary because of the variety of network settings and communication networks under study. These settings have included stand-alone computer-to-computer communication, electronic mail discussion lists, commercial and proprietary on-line services, commercial communication and group-ware packages, and many other communication systems ( Rapaport, 1991 ). Similarly, the many theoretical approaches have employed diverse terminology and definitions for units of analysis.
Added to this lack of theoretical integration have been the changes and advances in Internet communication technology over the period 1969-1995. While electronic mail and Usenet news-group discussions were the early forms of communication used on the Internet, today the Internet offers a far wider range of tools for information retrieval, communication, and interaction than just text-based discussion and information dissemination ( December, 1995a ). The use of the Internet also has rapidly increased, with some Internet applications, such as the World Wide Web (the system for linking documents associatively using hypertext), experiencing very rapid increases in use and range of expression ( December & Randall, 1995 ).
Those who study Internet communication may utilize this research site to identify commonalties in units of analysis. These common units can assist in research result comparisons and theoretical convergence. Without a common framework for units of analysis, definitions of what is being studied on the Internet can be clouded by a poor definition of the research setting, making cross-study (or even intrastudy) comparisons difficult.
This article proposes an approach to defining units of analysis for Internet communication research. To define these units of analysis, I first define the term Internet computer-mediated communication . This definition identifies the characteristics of Internet communication. I then use this definition as the basis for developing a set of definitions: media space , media class, media object , and media instance as units of analysis for Internet communication studies. Finally, I illustrate these units of analysis with some examples.
Whereas electronic mail has been a frequent subject area for previous communication research, the global Internet today offers a far more diverse set of tools and contexts for communication than it has in the past. Communication on the Internet also exists within developed social and cultural traditions. Therefore, what we mean when we talk about specific forms of Internet communication must be carefully defined, so that consistency in research approaches can be made and areas of inquiry for possible research can be identified.
Approaches to Defining Communication
I define Internet communication here by identifying characteristics of technology to define units of study. I want to note that these units of study are just one part of a total research project. The other concerns for researchers include the characteristics of the people involved in the communication, the social context of the communication, and a host of other possible factors in communication research. Therefore, my definition here captures the qualities of the technology so that researchers then can use this framework as a basis for further identifying the other units and factors important to a particular research study.
This approach makes careful distinctions among terms and units of analysis. This careful approach may seem like overkill, because such an exhaustive definition of a medium has not seemed necessary for studying other forms of media. Television and radio are familiar enough so that a researcher does not usually need to carefully define the object of study. A researcher looking at a particular genre of radio communication, such as AM talk radio, rarely would have to explain further what is meant by this term. The diversity of Internet communication systems and applications, however, motivates a more careful definition of terms and units of analysis. As I will describe below, the Internet cannot be considered to encompass a single medium, but consists of a range of media. Without careful definition of analysis units, researchers might easily obscure whatever part of the Internet research setting they are examining.
To generate a definition of Internet communication, I first analyze the constituent parts of the term Internet-based, computer-mediated communication. I examine each term in successive subsections of this article, each with a heading corresponding to the term. Following this definition, I illustrate by describing some categories of activity the term encompasses.
Internet-based
To say that communication is Internet-based means that, at the data level, it conforms to a particular set of data communications protocols. A protocol is a set of rules for exchanging information. Computer networks use protocols to enable computers connected to a network to send and receive messages. The set of protocols called the TCP/IP protocol suite defines the rules for data exchange on the Internet. This set of protocols, originally developed for a United States Department of Defense research project, integrates a set of services (including electronic mail, file transfer, and remote log-in) that can occur among many computers on local or wide-area networks.
The resulting networks connected with the TCP/IP protocol suite are highly robust. If one section of the network (or a computer host in the network) becomes inoperable, data can be rerouted around the damage in the network. Figure 1 illustrates how these protocols send data over the networks. First, the TCP (Transmission Control Protocol) breaks the data into packets of information. Next, these packets are sent over the network, possibly over different routes, according to IP (Internet Protocol). Finally, these packets are reassembled (or re-sent, in the case of data corruption or loss) in their proper order upon arrival at the destination.
Basic operation of the Internet's TCP/IP packet switching protocols
What this protocol scheme does is create a system for reducing all communication to data exchange, and this commonality of protocol is the essence of Internet communication at the data level. Through cooperation and connections, TCP/IP networks can be connected in larger and larger communication systems. Individual organizations can run their own TCP/IP network (an internet) and connect it with other local, regional, national, and global internet networks. The resulting patchwork of networks, called the Internet (with a capital I), shares the TCP/IP protocol suite. However, the Internet is not a single network, but a cooperatively organized, globally distributed system for exchanging information. The data that traverses this network of networks is Internet communication.
However, the Internet is not the only global network. Other global networks employ different protocols, but can exchange data with the Internet through exchange points called gateways. Non-Internet communication flowing into a gateway point is translated to Internet communications protocols and sent on its way, indistinguishable from the packets that TCP creates when sending a message directly on the Internet. Likewise, communication can flow off the Internet at the gateway points in the same manner: The Internet packets are translated to the non-Internet protocols necessary for communication on another network.
Electronic mail is a popular form of communication exchanged across gateways. Through electronic mail gateways, users on the Internet can exchange electronic mail with other users on (non-Internet) networks, such as BITNET (Because Its Time Network), UUCP (Unix-Unix Copy Protocol), and FidoNet (a network based on personal computer communication over telephone lines). Users of the Internet can also exchange electronic mail with many commercial services such as Prodigy, Delphi, CompuServe, America on-line, and many others ( Chew & Yanoff, 1995 ). The result is that electronic mail disseminates freely throughout the Internet, as well as many other networks. The resulting collection of worldwide networks that exchange electronic mail is called the Matrix ( Quarterman, 1990 ).
Although the free flow of electronic mail blurs the distinction between Internet communication and non-Internet communication in the Matrix, the distinction between the Internet and the Matrix for many other communication applications is crucial. For example, communication using the Internet Gopher protocol cannot easily be shared outside the Internet. Similarly, Telnet, FTP (File Transfer Protocol), and World Wide Web communication are restricted, in most cases to users of the Internet. Commercial on-line services, recognizing the value of access to the Internet for their customers, have been creating more kinds of gateways to the Internet. Commercial on-line services now have many gateways allowing their users to access Telnet, FTP, and World Wide Web applications on the Internet.
The resulting mix of global networks makes the Internet protocol suite the lingua franca of cyberspace, creating a common ground to which many other on-line networks connect through gateways. By having many gateways to the Internet, commercial services can offer users access to the content of the Internet while offering them proprietary, value-added services and content. A researcher studying communication on an on-line service (for example, Prodigy) must be careful to realize that they are not studying Internet communication. While the users of Prodigy may enjoy the free flow of electronic mail from the Internet to Prodigy and back, there are many proprietary services that Prodigy offers that are not available on the Internet.
The emphasis in this discussion on the Internet's boundaries and gateways underscores the first work of a researcher studying Internet communication: to identify what is and what is not Internet communication, and to investigate possible relationships between Internet communication (through gateways) and non-Internet communication. Based on this discussion, I can summarize a definition for Internet-based communication: Internet-based communication takes place on the global collection of networks that use the TCP/IP protocol suite for data exchange.
There are TCP/IP networks (internets, with a small i ) that are not connected to the larger global Internet. These internet networks, therefore, although technically using the TCP/IP protocol suite, are not part of the global Internet based on this definition. People using these local internets cannot access the myriad resources or take part in the cultural and social traditions and artifacts that exist on the global Internet.
The term computer in the context of defining Internet computer-mediated communication means much more than just a device for calculation. In fact, the salient function of a computer as used for communication is not to provide computational capability, but to provide a platform for the operating system and software applications to support network data transmission and user applications.
On the Internet, the relationships among computers commonly follow the client-server model. Like the TCP/IP protocols, the client-server model is a unifying characteristic of Internet communication. A server is a computer and its associated hardware and software applications that act as a repository for information files or software programs. The server sends this information by request across the network to users of client software.
Figure 2 summarizes the client-server relationships. First, a request for information flows from a client to a server. Based on this request, the server sends information back to the client.
The client-server data communications model
Client-server communication also follows a set of protocols. These protocols define the particular application the client and server are using. For example, the Internet Gopher protocol defines an application for structuring information into a system of menus, submenus, and entries. A user of a Gopher client makes a request from a Gopher server for a list of menu items. The Gopher server sends back this list, and the Gopher client displays the list for the user.
The distributed form of the client-server request and serve scheme allows for many efficiencies. Since the client software interacts with the server according to a standard data exchange protocol, the client software can be customized for the user's particular computer host. This means that the server doesn't have to “worry” about the hardware or software particularities of the computer on which client software resides. The client software doesn't have to “worry” about which server of a particular type it requests information from, as all servers of a particular protocol behave the same way.
For example, a Web client that can access any Web server can be developed for Mcintosh computers. This same Web server might be accessed by a Web client written for a Unix workstation running the X-Window System. This sets up a clear demarcation of duties between the client and the server. This makes it easier to develop information because separate versions of information distributed from a server need not be developed for any particular hardware platform. All the customizations necessary for the user's computer are written into client software for that platform.
An analogy to the client-server model is the television broadcast system: A customer can buy any kind of television set (client) to view broadcasts from any over-the-air broadcast tower (server). Whether the user has a wristband TV or a projection screen TV, the set receives information from the broadcast station in a standard format and displays it in a manner appropriate to the user's TV set. Separate TV programming need not be created for each kind of set, regardless of such characteristics as screen size. Further, newly created television stations will be able to send signals to all television sets currently in use.
The client-server model is the key characteristic of the Internet's scheme for communication among applications.
Mediation is the process of intervening or coming between. For Internet communication, mediation also involves literally putting a message into media, or encoding a message into electronic, magnetic, or optical patterns for storage and transmittal. A message on the Internet is encoded, stored, and transmitted according to the rules of the client-server application and the TCP/IP protocol suite.
The transmittal of this mediated message on the Internet can also have a variety of mediation characteristics, including time, distribution, and media type.
The time delay between sending and receiving messages can vary. This time delay can be nearly instantaneous in applications where users take part in (nearly) “real time” text interchange. Internet applications where users engage in this communication include mass and group communication systems, such Internet Relay Chat (IRC), and Multiple User Dialogue/Dimension/Dungeon (MUDs) or variants (known as MU* systems). In other network information retrieval systems, communication can be persistent, that is, exist on a server for on-demand retrieval, such as file of hypertext on a Web server, information on a Gopher server, or files available from an FTP server.
A message on the Internet can be distributed from a sender to receivers according to a variety of schemes. These variations include
• Point to point: A single user sends a message to a single receiver (e.g., electronic mail).
• Point to multipoint: A single user sends a message to a number of specific receivers (e.g., electronic mailing lists) or an application that sends the message to many receivers (e.g., a “mail exploder,” Listserv or Majordomo program).
• Point to server broadcast: A single user sends a message to a server. This server then makes this message available to any user with appropriate client software. With nearly real-time response, this distribution scheme is used for disseminating IRC communication. Alternately, the server may broadcast the incoming message to one or more other servers in a message propagation scheme. This server-to-server distribution scheme is used for propagating Usenet news.
• Point to server narrowcast: A single user sends a message to a server. This server then makes this message available to only a specific group of users employing clients connected directly to that server. Additionally, these users may have to identify themselves with their log-in and password. For example, MU* systems require passwords and IDs for access to the communications system. Usually, only MU* participants can observe the activity on a particular MU* server.
• Server broadcast: A server contains stored information that is available to any user with an appropriate client. This information is broadcast in the sense that the server provides this information to any requesting client. Users can observe this information anonymously. This information is often created by the organization or individuals who own the server. A Web site is an example of this form of distribution.
• Server narrowcast: A server provides information to only a specific set of authorized users. Users typically provide authentication information through their client for access to information on the server.
Internet applications display information in a variety of media types, including text, sound, graphics, images, video, or binary (executable) files. The Multipurpose Internet Mail Extensions (MIME) specifications define these multimedia types commonly used in Internet communication. Text that uses a system of associative linking is called hypertext . Hypertext that employs multimedia is called hypermedia .
The range of characteristics outlined above highlight the range of possibilities for communication on the Internet involving variations in time, distribution scheme, and media type. A researcher should be able to identify each of these characteristics for a particular form of Internet communication under study.
Note that other media may exhibit these same characteristics. For example, radio broadcasts are available in real time to anyone with an appropriate client (radio receiver) within range of the broadcast station. A person calling into a radio program with their voice broadcast as part of the program is using a point to server broadcast form of distribution. The characteristics cited here for time, distribution, and media, typify the mediation process in Internet communication content.
Communication
Although data communication serves as the basis for transmitting messages on computer networks, a communication researcher, unless involved in the detailed technical examination of data transmission, is concerned with the human communication issues involved when people communicate on the Internet. Indeed, Internet-based communication is essentially human communication via the Internet computer network, so any definition of Internet communication necessary involves defining human communication itself. Human communication can be characterized as a process in which people exchange symbols ( Littlejohn, 1989 ). The process of symbol exchange occurs in the context of Internet communication with mediation characteristics as described above, following the client-server model for information exchange and the TCP/IP protocol suite for data exchange.
The content of Internet communication, however, is more complex to describe, but it can often be captured for study. Internet content can be encoded and decoded using a variety of media types (text, graphics, sound, video, executable file). Because of its mediated form, Internet communication often leaves a visible trail of artifacts that can be collected for study. For example, Usenet discussion groups produce a set of articles that can be examined and analyzed. Real-time discourse among a group of participants, such as in MU* systems, can be recorded in the form of a transcript. Other Internet communication artifacts include Web pages, files on Gopher sites, and transcripts of Listserv discussion lists.
The interpretation of these artifacts depends on the goals of the study. Likewise, the symbols important to the researcher will vary. For example, Internet communication using real-time audio-video conferencing can occur with an application called CU-See Me. This application allows participants to transmit pictures and sound, thus displaying many nonverbal and paralinguistic cues not present in much text-based communication on the Internet. Thus, communication on the Internet represents a range of possibilities for symbol creation, and some of these symbols can be similar to those examined in unmediated human communication.
Integration
The above discussion outlines the boundaries of the definition of Internet-based, computer-mediated communication. Using this definition, a researcher can identify what is not Internet communication (e.g., electronic mail exchanged among users of a commercial on-line service such as America Online) as well as what is Internet communication (e.g., users communicating via text exchange in Diversity University's MU*. Clearly, non-Internet communication may include a range of activities, including commercial voice mail, telephone conversations, Local Area Network (LAN) communication, or Computer-Supported Cooperative Work on LANs, that may exhibit many of the qualities of Internet communication. However, users of the Internet experience a much different communication context than non-Internet users. Internet users can access multiple applications for communication (for example, using a Windows environment on a PC or a workstation to use electronic mail, a Web client, and a MU* client all at once). Users of the Internet have access to a large set of possible communication partners and information sources. Internet users also communicate within a particular cultural context on the Internet, with its own shared cultural traditions and symbols, such as typographic symbols representing emotions called smilies .
Based on the above discussion, we arrive at the following definition: Internet-based, computer-mediated communication involves information exchange that takes place on the global, cooperative collection of networks using the TCP/IP protocol suite and the client-server model for data communication. Messages may undergo a range of time and distribution manipulations and encode a variety of media types. The resulting information content exchanged can involve a wide range of symbols people use for communication.
Examples of Internet communication
People use Internet communication for many purposes. I identify some of these major purposes with the broad categories of communication, interaction, and information. These categories are not mutually exclusive: Someone can participate in Internet communication for a combination of communication, information, and interaction at the same time.
Communication: People use the Internet for communication in a one-to-one, one-to-many, or many-to-many setting. This communication can be used for scholarly activity and research or for personal and group communication and discussion. Examples: Usenet, electronic mail, and Listserv.
Interaction: People can use the Internet for the purpose of play or learning, not just for information transfer or discussion. Interaction spaces are often used for social activity and for group interaction and education. Examples: MU*s and IRC.
Information : People use the Internet for dissemination and retrieval of information. This information deals with subject matter covering a wide range of human activities and knowledge. Examples are the World Wide Web, Gopher, and FTP.
Defining what Internet communication is and showing how this definition encompasses a broad range of human communication, interaction, and information purposes are the first steps in characterizing the units of analysis for Internet communication research. In the next section, I use and expand on the terms from this definition of Internet-based, computer-mediated communication to describe more specific units of analysis.
The discussion to this point should help an Internet researcher identify a research study's parameters for data exchange, client-server communication, message mediation characteristics, and communication symbols. The next step is to more precisely define what specific area of communication on the Internet is being examined.
If one researcher states that he or she will study MU* interaction, this could involve observations on a variety of MU* systems, including, potentially, discourse in systems for real-time text interchange, including MUDs (Multiple User Dialogues), MOOs (an object-oriented MUD), or MUSEs (Multiple User Simulation Environment). Even further, the particular MU* studied is extremely important, as MU*s vary in their layout, inhabitants, and activities. Jay's House MOO is very different from Diversity University MOO, for example. Yet, activities among MU*s do share some commonalities that may allow for some cross-comparison of research results. The purpose of this section is to define the units of analysis that help researchers identify what types of communication they are studying on the Internet.
I would like to emphasize again that defining the units of analysis with this framework should not imply that only these units can be profitably used to define a research setting in isolation of other factors. Indeed, as I noted above, a communication research study will involve a wide range of other considerations, such as social setting and context, user characteristics, or the purpose of the communication. But by defining the units of analysis described here, a researcher can help identify what kind of communication system and setting is under study.
The Server-Client-Content Triad
I establish this framework for units of analysis by developing definitions for a set of terms. I use the terms server , client , and content as an organizing triad for these definitions. Defined briefly above, I expand on the definitions of these terms:
Server-A computer and associated software that provide access to information through the Internet in response to requests from client software based on a particular protocol for data exchange. An example is a World Wide Web Server using the NCSA (National Center for Supercomputing Applications) software.
Client-Software that operates on a user's computer for accessing information distributed from servers according to one (or more) protocol(s) for data exchange. An example is a Netscape Communications Corporation World Wide Web client. The Netscape client can access Web servers, and also FTP, Gopher, Telnet, and other protocol servers.
Content-Information that is exchanged, distributed, or available for retrieval or transmittal on networks. Examples are the content of the Usenet newsgroup alt.hypertext, or the text exchanged among users in the Communications Center (a particular room) in the Diversity University MOO.
Internet communication is not a single medium sharing common time, distribution, and sensory characteristics, but a collection of media that differ in these variables. I define a unit of analysis called a media space , which uses the client-server-content triad as the basis for its definition. This concept of media space is one way to describe how the Internet consists of a range of media.
Media space-A media space consists of the set of all servers of a particular type that may provide information in one or more protocols, the corresponding clients that are capable of accessing these servers, and the associated content available for access on these servers.
Note that this definition allows for a space to include servers that may provide information in a variety of protocols, but with the unifying idea that these servers all share the ability to provide information to clients with at least one common protocol. This common protocol usually identifies the type of the servers. This allows for the possibility that a space may include servers with the ability to deliver information in multiple protocols to clients capable of interpreting all of these protocols.
For example, we can consider one Internet media space to be Gopher space: the set of all information (content) provided by Gopher servers, accessible by people using Gopher clients. Note that Web clients can also access Gopher servers, so that Web clients are components of Gopher space.
Another example of an Internet media space is defined by Internet Relay Chat (IRC): IRC space consists of IRC clients accessing the text exchanged among participants from any one of many IRC servers. A user wishing to enter IRC space would need a client, such as Telnet, or a specialized IRC client, to access information on a server. The user potentially has many servers to access worldwide. The discussion on servers, generated and observed by users employing clients, constitutes IRC space. Note that IRC space is disjointed from Gopher space: A Gopher client cannot be used to observe IRC content, nor can IRC clients be used to observe IRC content.
Web space, or the set of all Hypertext Transfer Protocol (HTTP) servers, Web clients, and content on HTTP servers, is itself composed of several spaces. Using the Java programming language, developers can create specialized protocols that can be used to deliver information from Web servers to Java-enabled clients ( December, 1995b ). This delivery mechanism for these specialized Java-defined protocols is still the Web server. However, only Java-enabled Web clients can be used to observe Java-defined content. Therefore, the space defined by “Web hypertext, Web servers, and Web clients” is not the same as “Web hypertext plus Java-defined content, Web servers, and Java-enabled Web clients.” These two spaces share common components (the non-Java content of the Web) and could then be considered to overlap.
This definition of a space corresponds to informal descriptions of other media. Colloquially, we might say that television is a medium, and mean that television is not just a collection of TV sets (clients), nor all of the broadcast TV stations (the servers). Instead, our concept of television as a medium encompasses all TV sets, all broadcast (and cable and satellite) systems, and all programming and production in combination with viewers' observation of this content through television sets (clients). Similarly, on the Internet, a space is not just one client type (e.g., Mosaic as an example of a Web client), nor the collection of all servers of one type, but the entire set of clients and servers along with the content on those servers potentially observable by those clients.
This definition of an Internet media space is useful to capture the idea that there are many different (sometimes overlapping, sometimes disjointed) spheres of activity on the Internet. A space, defined this way, is a seamless forum, in which users can observe any of the content from the servers in that space using their clients.
However, each Internet media space encompasses a vast amount of activity, probably too vast for a single research study. So just as communication researchers may want to focus on a subset of a television for study, such as television news programs or late-night talk shows, so, too, might the researcher in Internet communication need to focus on a subset of an Internet media space. The definitions below subdivide two analysis units to describe more precise units: I use the term media class to define a particular set of content, servers, and clients; the term media object defines a specific unit in a media class with which the user can observe and interact.
Media Class-A media class consists of content, servers, and clients that share a defined set of characteristics.
According to this definition, a media class can be equivalent to a media space. For example, all the Gopher content, servers, and clients comprise a media class, which is also the definition for Gopher space. A researcher, however, can define a subclass of a single space or several spaces in terms of a media class to focus on a particular set of Internet communication for study.
Here are some examples of media classes defined by shared characteristics of servers, clients, or content: (a) The hypertext (content) available from the Web server http://www.w3.org , observable through any Web client. This media class is a subset of Web space. (b) All the available information on the Internet accessible using the NCSA Mosaic Web client. This media class consists of several Internet media spaces, including Gopher space, Web (HTTP) space, FTP space, and others. (c) The set of all hypertext listed in the Clearinghouse for Subject-Oriented Internet Guides ( http://www.lib.umich.edu/chhome.html ). This media class is a subset of Web space. (d) All the available information on the Internet accessible using the HotJava Web client. This media class consists of several Internet spaces, including Gopher space and Web space, plus the specialized protocols and content created with the Java programming language (Java space).
Note how media class differs from media space. An Internet space includes all servers, corresponding clients, and content available on these servers that can be delivered to users. In contrast, a media class could include subsets of one or more media spaces, or even several media spaces. For example, as examples 2 and 4 above, a media class defined by all content accessible through a Web client includes not only Web space, but also the spaces defined by FTP, Gopher, Telnet, and Usenet.
This definition of a media class gives the researcher a way to define areas of study that may include subsets of several media spaces. However, the definitions of media space and media class are still at too high a level of abstraction to actually define something a user can interact with or observe. People cannot watch abstractions such as television (a medium) or even a media class defined by a genre of television (late night talk shows). Instead, television users observe specific programs, such as Late Night with Conan O'Brien, or The Late Show with David Letterman , or The Tonight Show starring Jay Leno , which are broadcast from a specific station and viewed with a particular television set. Similarly, analyzing Internet communication can benefit from having a term for a unit of analysis that is specific enough so that it defines something someone can observe. I use the term media object for this concept.
Media Object-A media object is a member of a media class for which the server, client, and content are completely and unambiguously specified. A media object is concrete, whereas a media class is simply a template for defining media objects. For example, a media object can be defined in this way: The World Wide Web (WWW) Frequently Asked Questions (FAQ) List on the SunSITE Web server sunsite.unc.edu accessed through the Netscape Navigator client for X , version 1.1.
Note that a particular media object might be a member of several different media classes. For example, let the media object, W , be defined by the above example (“the WWW FAQ as viewed through Netscape Navigator for X version 1.1 Web client”). Media object W is a member of the media class that includes all the information at sunsite.unc.edu. W is also part of the media class that includes all content accessible by the Netscape Navigator for X version 1.1 Web client. Still further, W , is a member of the media class whose content is defined by all the FAQ lists available on the Internet.
While the definition of a media object is specific enough to define something a user can observe, a medium may change over time. Therefore, I use the term media instance to capture this idea:
Media instance-A media instance is a media object at a particular time.
Under this definitional scheme, people perceive media instances, not media objects, classes, or spaces. However, colloquially, people often talk in terms of media objects and classes: “I watch TV” (space), “I watch TV news” (class), or “I watch the CBS news on my TV” (object). We, however, can actually experience only media instances, for example, “the CBS news on my TV last night for the first twenty minutes” (instance).
To specify a media instance, a researcher needs to specify a particular point in time for observing a media object. For example, the media object W , defined above, can be used to define the instance: W as observed on August 21, 1995, at 10:25 p.m.
However, a media instance is not yet tied to a particular user's experience and may be still too abstract for some studies. I define media experience to link a media instance to a particular user's perception:
Media experience-A media experience is a particular user's perception of a set of media instances.
Note that a user's media experience might include observation of many media objects, and these media objects might be from different media classes, which may consist of several media spaces. Here are some examples of media experience:
• Susan watched the CBS news broadcast on Wednesday, August 30, 1995, for the first 10 minutes. (Implied in this description is the specification of the server as Susan's local television broadcast station or cable service and her client as her particular model of TV set).
• Tom watched the CBS television program, Late Show with David Letterman, on August 30, 1995, while using his Netscape Web client for Windows ‘95 connected to the Web support site for that program, http://www.cbs.com/lateshow/ .
• Chris participated in the Internet Relay Chat channel #current-events using a Telnet client from 10 p.m., August 30, 1995, to 2 a.m., August 31, 1995, and used his FTP client to retrieve the file ftp://ftp.rpi.edu/pub/communications/internet-cmc.txt at 2 a.m.
Contextualizing Media Units of Analysis
The importance of the above careful delineation of media space, class, object, instance, and experience becomes more apparent when placed in the context of a particular research study. As an example of how this definition system can be applied, I will describe how these terms can help in studying Web-based mass communication using the uses-and-gratifications approach from mass communication theory.
The uses-and-gratifications approach considers consumers of media to be purposive in their choice of media and to actively seek media to fulfill their needs for a variety of uses ( Infante, Rancer, & Womack, 1993 , p. 408). Typologies of uses for mass media consumption, as well as other research and studies, have identified a broad range of gratifications people have in broadcast (one-way, one-to-many messaging) in several mediated communication contexts ( McGuire, 1974 ). Other typologies of uses ( Blumler, 1979 ; Palmgreen, Wenner, & Rayburn, 1980 ; Wenner, 1985, 1986 ) organize human needs met by media in many categories, including, for example, Wenner (1986) :
• Surveillance (e.g., to obtain information about daily life)
• Entertainment/Diversion (e.g., to get away from usual cares and problems)
• Interpersonal utility (e.g., to get interesting things to talk about)
• Parasocial interaction (e.g., to encounter human qualities)
Researchers can use this typology as a basis to test attitudes people have toward media consumption with regard to variables. Two such variables are as gratification obtained ( GO ), defined as “the perceived outcome of engaging in a particular behavior,” and gratification sought ( GS ), “the seeking of a value outcome mediated by the expectancy of obtaining that outcome” ( Palmgreen, Wenner, & Rayburn, 1981 , p. 473). Using these variables, models of user attitudes, such as attitudes of satisfaction, can be tested ( Palmgreen & Rayburn, 1985 ).
In applying uses-and-gratifications to communication, an important consideration is to make sure that the variables such as GS and GO are measured at the level of abstraction for use in a model. In uses-and-gratifications studies, “measurement of GS and GO at the same level of abstraction is crucial to direct comparison of communication outcomes with what is sought” ( Palmgreen, 1984 , p. 34). A GO measured from a subject's “favorite TV news program” (media object) can substantially exceed GS as measured at the level of program type (TV news, which is a media class; Palmgreen, Wenner, & Rayburn, 1981 ). The result is that a model of attitudes that uses these measures of GS and GO at different levels, particularly a discrepancy model that uses the difference between GS and GO in its equation, is faulty in its construction ( Palmgreen, 1984 , p. 34).
In order to illustrate how the definitions of media class, object, and instance can assist a researcher using uses-and-gratifications measurements in a communication context, I define the following media class, object, and instance:
• Media class WebMa g-the set of all Web-based magazines delivered through Web servers and observed using a Web client.
• Media object - the International Teletimes , Web-based magazine at http://www.wimsey.com/teletimes.root/teletimes_home_page.html as observed through the Netscape 1.1 for X client.
• Media instance t -media object T during its June 1995 circulation period.
We can then measure a subject's attitude in terms of the variables GS and GO based on their experience of media instance t , and also their attitudes about media object T as well as media class WebMag . Typically, these measurements are made on a five-point Likert scale asking users to indicate how much they agree with a given statement on a scale ranging from strongly agree to strongly disagree. A model that questions users at the media class level would ask the user questions such as:
• GS question-I read Web-based magazines to relax.
• GO question-Web-based magazines help me relax.
At the media object level, the questions would be specific to particular content:
• GS question-I read International Teletimes to relax.
• GO question- International Teletimes helps me relax.
Note that at this object level, the client is implied to be the user's own Web client.
At the instance level, the particular media object occurring at a fixed time would be the focus:
• GS question-I want to read the June 1995 International Teletimes in order to relax.
• GO question-The June 1995 International Teletimes helped me relax.
The flexibility of these units can permit many media classes, objects, instances, and experiences to be defined for analysis. For example:
• Media class UsenetAlt -the set of all Usenet alt.* newsgroups.
• Media object H -alt.hypertext viewed through Netscape 1.1 for X as served on the usenet.foo.edu server.
• Media instance h -alt.hypertext viewed through Netscape from the usenet.foo.edu server, articles 2498 to 2523 (article numbering may vary across Usenet servers, but, of course, viewers accessing articles on the same server will see the same articles with the same numbers).
The overall purpose of a study may be to make cross-comparisons at the media class level:
• Media class DuComm -the content (discourse plus static MOO structures) of the Diversity University MOO's Communications center.
• Media class LmEntry -the content (discourse plus static MOO structures) of the Lambda MOO's new user entry room.
The purpose of a study might be to compare the discourse in these two different contexts of MOO use (e.g., comparing discourse in these two “rooms” on different MOOs). In order to collect more specific data on user experiences in these classes, the researcher must define objects and instances. The server and content were specified in the class definition. The object definition requires the researcher to specify the client and then instances based on time. For example:
• Media object D -a member of DuComm as viewed through the XYZ MOO client
• Media instance d - D from 10:00 a.m. EST to 11:00 a.m. EST on June 24. 1995.
• Media object L -elements of LmEntry as viewed through the XZY MOO client
• Media instance l - D from 10:00 a.m. EST to 11:00 a.m. EST on June 24, 1995.
The researcher could either analyze the transcript of the discourse (a language approach) or question users' experiences of d and l , or attitudes with regard to D , L , DuComm, or LmEntry. The key is that by carefully defining the levels at which these measurements take place, the research can have a consistent framework for comparing different media experiences.
Media classes can also be described according to their mediated characteristics, as listed above, for time delay, distribution, and media type. For example, we can define media class JavaSun as “all the content available on the server java.sun.com that is observable through a Java-enabled Web client.”
The characteristics of individual objects of type JavaSun share these characteristics: time (persistent), distribution (server broadcast), and media type (hypertext with applet, which is an executable content written using the Java language).
All objects in media class J then have these class characteristics. For example, we can define an object as follows: Object S is the spreadsheet at http://java.sun.com/applets/applets/spreadsheet/index.html viewed through HotJava alpha release 3 for Windows ‘95.
This object has its content and server specified by a URL and its client specified as a particular version of a Java-enabled browser. Object S shares the time, distribution, and media type characteristics as all other objects of type JavaSun. A subclass of JavaSun might be defined in terms of a specific browser: Media class JavaSunW95 includes all the content available on the server java.sun.com that is observable through the HotJava browser for Windows ‘95.
Clearly, S is an object of type JavaSunW95, and all objects of type JavaSunW95 share the same time, distribution, and media type characteristics as objects of type JavaSun. Using class hierarchies consisting of classes and subclasses, related classes can inherit characteristics.
Media classes and objects can be represented graphically. Figure 3 shows symbols that can be used for content, server, and a client.
Symbols for graphic representation of media classes and objects
Figure 4 shows these symbols used to illustrate several media classes. The left figure shows a media class called Web, which is defined by any Web content on any Web server through any Web browser. The dashed lines indicate a variety of possible values for the content, server, or client in the class.
Graphic representation of a media class
The diagram in the middle is for the class JavaSun, a subclass of Web that consists of all the applets on the server java.sun.com that are observable through a Java-enabled client.
Finally, the right diagram in Figure 4 shows the class JavaSunW95, which is a subclass of JavaSun. This class consists of all applets on the java.sun.com server as viewed through the alpha 3 release of the HotJava browser for Windows ‘95.
Media objects can be shown using the same notation as for classes, but with solid lines for the symbols, as a media object has all of its content, server, and client attributes specified. Figure 5 shows three media objects of type JavaSun. The content of each object is the spreadsheet http://java.sun.com/applets/applets/spreadsheet/index.html . The server is java.sun.com, and the three Java-enabled Web clients shown in the diagram complete the definition for three media different objects.
Graphic representation of three media objects
Articulating the vast possibilities for communication on the Internet can be approached using a system of definitions. A definition for Internet communication relies on the precise meaning and technical implication of the words, Internet, computer, mediated, and communication. In approaching Internet communication as a range of media, we can define units of analysis: media space, media class, media object, media instance, and media experience, based on an articulation of levels of abstraction. Using the multiple levels of abstraction inherent in these definitions, communication researchers can define a variety of research settings with a consistent treatment of units of analysis. The overall benefit of this definition of Internet communication is that the diverse landscape of Internet communication can be defined, opening up possibilities for cross-study comparisons as well as theoretical integration.
In addition to using the above definition of Internet communication and the definition framework for media classes, objects, instances, and experiences, communication researchers can define their research setting using consistent terminology and units of analysis. Specifically, the benefits of this use are (a) to provide ways to define units of analysis for measuring variables in many communication contexts, (b) to allow consistent articulation of units and of analysis for study and for cross-study comparisons, and (c) to allow media class and object definitions to articulate the differences in levels of abstraction for measuring experiences based on different media objects.
More work remains in exploring how this system of definitions can be applied to more general on-line communication contexts. The client-server component of this definition is based on a communications system employing client-server communication on the Internet. The growing interconnections among Internet communication and non-Internet services will open up more possibilities for communication and an even more diverse on-line communication environment.
Baron , Naomi, S. ( 1984 ). Computer-mediated communication as a force in language change . Visible Language , 18 ( 2 ), 118 – 141 .
Google Scholar
Black , S. , Levin , J. , Mehan , H. , & Quinn , C. N. ( 1983 ). Real and non-real time interaction: Unraveling multiple threads of discourse . Discourse Processes , 6 ( 1 ), 59 – 75 .
Blumler , J. G. ( 1979 ). The role of theory in uses and gratifications studies . Communication Research , 6 ( 1 ), 9 – 36 .
Chew , J. , & Yanoff , S. ( 1995 ). Inter-network mail guide . [On-line] Available: http://ftp.csd.uwm.edu/pub/internetwork-mail-guide .
Daft , R. L. , & Lengel , R. H. ( 1984 ). Information richness: A new approach to managerial behavior and organizational design . Research in Organizational Behavior , 6 , 191 – 233 .
Daft , R. L. , & Lengel , R. H. ( 1986 ). Organizational information requirements, media richness and structural design . Management Science , 32 , 554 – 571 .
Daft , R. L. , Lengel , R. H. , & Trevino , L. K. ( 1987 ). Message equivocality, media selection, and manager performance: Implications for manager performance . MIS Quarterly , 11 , 355 – 66 .
December , J. ( 1995a ). Internet tools summary . [On-line] Available: http://www.rpi.edu/Internet/Guides/decemj/internet-tools.html .
December , J. ( 1995b ). Presenting Java . Indianapolis, IN: Sams.Net .
Google Preview
December , J. , & Randall , N. ( 1995 ). The World-Wide Web unleashed (2nd ed.). Indianapolis, IN: Sams.Net .
DeSanctis , G. , & Gallupe , R. B. ( 1987 ). A foundation for the study of group decision support systems . Management Science , 33 , 589 – 609 .
Feenberg , A. ( 1989 ). The written world: On the theory and practice of computer conferencing . In R. Mason & A. Kaye (Eds.), Mindweave: Communication, computers and distance education (pp. 22 – 39 ). Oxford: Pergamon Press .
Feenberg , A. ( 1992 ). From information to communication: The French experience with videotex . In M. Lea (ed.), Contexts of computer-mediated communication (pp. 168 – 187 ). New York: Harvester Wheatsheaf .
Ferrara , K. , Brunner , H. , & Whittemore , G. ( 1991 ). Interactive written discourse as an emergent register . Written Communication , 8 ( 1 ), 8 – 34 .
Finnegan , R. H. ( 1988 ). Literacy and orality: Studies in the technology of communication . Oxford, England: Blackwell .
Fulk , J. , Schmitz , J. , & Steinfield , C. ( 1990 ). A social influence model of technology use . In J. Fulk & C. Steinfield (Eds.), Organizations and communication technology (pp. 117 – 140 ). Newbury Park, CA: Sage .
Fulk , J. , Steinfield , C. W. , Schmitz , J. , & Power , J. G. ( 1987 ). A social information processing model of media use in organizations . Communication Research , 14 ( 5 ), 529 – 552 .
Georgoudi , M. , & Rosnow , R. L. ( 1985 ). The emergence of contextualism . Journal of Communication , 35 ( 1 ), 76 – 88 .
Gurak , L. ( 1994 ). The rhetorical dynamics of a community protest in cyberspace: The case of Lotus marketplace . Unpublished doctoral thesis, Rensselaer Polytechnic Institute , Troy, NY.
Harnad , S. ( 1991 ). Post-Gutenberg galaxy: The fourth revolution in the means of production of knowledge . The Public-Access Computer Systems Review , 2 ( 1 ), 39 – 53 .
Havelock , E. A. ( 1986 ). The muse learns to write: Reflections on orality and literacy from antiquity to the present . New Haven, CT: Yale University Press .
Hiltz , S. R. , & Turoff , M. ( 1978 ). The network nation: Human communication via computer . Reading, MA: Addison-Wesley .
Infante , D. A. , Rancer , A. S. , & Womack , D. F. ( 1993 ). Building communication theory (2nd ed.). Prospect Heights, IL: Waveland Press .
Innis , H. A. ( 1972 ). Empire and communications (Rev. ed.). Toronto: University of Toronto Press .
Internet Society . ( 1995 ). Frequently asked questions: What is the internet ? [On-line] Available: http://gopher.isoc.org:70/00/isoc/faq/what-is-internet.txt .
Johansen , R. , Vallee , J. , & Spangler , K. ( 1979 ). Electronic meetings: Technical alternatives and social choices . Reading, MA: Addison-Wesley .
Kiesler , S. ( 1986 ). The hidden message in computer networks . Harvard Business Review , 64 ( 1 ), 46 – 58 .
Kiesler , S. , Siegel , J. , & McGuire , T. W. ( 1984 ). Social psychological aspects of computer-mediated communication . American Psychologist , 39 , 1123 – 1134 .
Kling , R. , & Gerson , E. M. ( 1977 ). The social dynamics of technical innovation in the computing world . Symbolic Interaction , 1 ( 11 ), 132 – 46 .
Lakoff , R. T. ( 1982 ). Some of my favorite writers are literate: The mingling of oral and literate strategies in written communication . In D. Tannen (ed.), Spoken and written language: Exploring orality and literacy, Advances in discourse process (pp. 239 – 260 ). Norwood, NJ: Ablex .
Lea , M. ( 1992 ). Introduction . In M. Lea (ed.), Contexts of computer-mediated communication (pp. 1 – 6 ). New York: Harvester Wheatsheaf .
Lea , M. , & Spears , R. ( 1991a ). Computer-mediated communication, de-individuation, and group decisionmaking . In S. Greenberg (ed.), Computer-supported cooperative work and groupware, people and computers (pp. 155 – 173 ). London: Academic Press .
Lea , M. , & Spears , R. ( 1991b ). Computer-mediated communication, de-individuation, and group decisionmaking . International Journal of Man-machine Studies , 34 , 283 – 301 .
Lengel , R. H. , & Daft , R. L. ( 1988 ). The selection of communication media as an executive skill . Academy of Management Executive , 2 ( 3 ), 225 – 232 .
Levinson , P. ( 1990 ). Computer conferencing in the context of the evolution of media . In L. M. Harasim (ed.), On-line education: Perspectives on a new environment (pp. 3 – 14 ). New York: Praeger .
Littlejohn , S. W. ( 1989 ). Theories of human communication (3rd ed.). Belmont, CA: Wadsworth .
Markus , M. L. ( 1987 ). Toward a “critical mass” theory of interactive media: Universal access . Communication Research , 14 ( 5 ), 491 – 511 .
Markus , M. L. ( 1990 ). Toward a critical mass theory of interactive media: Universal access, interdependence and diffusion . In J. Fulk & C. Steinfield (Eds.), Organizations and communication technology (pp. 194 – 218 ). Newbury Park, CA: Sage .
Martin , L. , O'Shea , T. , Fung , P. , & Spears , R. ( 1992 ). ‘Flaming’ in computer-mediated communication: Observations, explanations, implications . In M. Lea (ed.), Contexts of computer-mediated communication (pp. 89 – 112 ). New York: Harvester Wheatsheaf .
McGuire , T. W. , Kiesler , S. , & Siegel , J. ( 1987 ). Group and computer-mediated discussion effects in risk decision making . Journal of Personality and Social Psychology , 52 ( 5 ), 917 – 930 .
McGuire , W. J. ( 1974 ). Psychological motives and communication gratification . In J. G. Blumler & E. Katz (Eds.), The uses of mass communications: Current perspectives on gratifications research (pp. 167 – 196 ). Beverly Hills, CA: Sage .
McGuire , W. J. ( 1983 ). A contextualist theory of knowledge: Its implications for innovation and reform in psychological research . In L. Berkowitz (ed.), Advances in experimental social psychology , Vol. 16 (pp. 1 – 47 ). New York: Academic Press .
McLuhan , M. ( 1964 ). Understanding media: The extensions of man . New York: McGraw-Hill .
McLuhan , M. ( 1965 ). The Gutenberg galaxy: The making of typographic man . Toronto: University of Toronto Press .
McLuhan , M. , & Fiore , Q. ( 1967 ). The medium is the massage . New York: Bantam Books .
McLuhan , M. , & Powers , B. R. ( 1989 ). The global village: Transformations in world life and media in the 21st century . New York: Oxford University Press .
Miles , I. ( 1992 ). When mediation is the message: How suppliers envisage new markets . In M. Lea (ed.), Contexts of computer-mediated communication (pp. 145 – 167 ). New York: Harvester Wheatsheaf .
Murray , D. E. ( 1991 ). The composing process for computer conversation . Written Communication , 8 ( 1 ), 35 – 55 .
Ochs , Elinor ( 1989 ). Language, affect, and culture . Cambridge, England: Cambridge University Press .
Ong , W. J. ( 1977 ). Interfaces of the word: Studies in the evolution of consciousness and culture . Ithaca, NY: Cornell University Press .
Ong , W. J. ( 1982 ). Orality and literacy: The technologizing of the word New Accents Series . London: Methuen .
Palmgreen , P. ( 1984 ). Uses and gratifications: A theoretical perspective . Communication Yearbook , 8 , 20 – 55 .
Palmgreen , P. , & Rayburn , J. D. ( 1985 ). A comparison of gratification models of media satisfaction . Communication Monographs , 52 , 334 – 346 .
Palmgreen , P. , Wenner , L. A. , & Rayburn , J. D. ( 1980 ). Relations between gratifications sought and obtained: A study of television news . Communication Research , 7 , 161 – 192 .
Palmgreen , P. , Wenner , L. A. , & Rayburn , J. D. ( 1981 ). Gratification discrepancies and news program choice . Communication Research , 8 , 451 – 478 .
Quarterman , J. S. ( 1990 ). The matrix: Computer networks and conferencing systems worldwide . Bedford, MA: Digital Press .
Rapaport , M. ( 1991 ). Computer mediated communications: Bulletin boards, computer conferencing, electronic mail, and information retrieval . New York: Wiley .
Rice , R. E. , & Shook , D. E. ( 1990 ). Voice messaging, coordination, and communication . In J. Galegher , R. E. Kraut , & C. Egido (Eds.), Intellectual teamwork: Social and technological foundations of cooperative work (pp. 327 – 350 ). Hillsdale, NJ: L. Erlbaum Associates .
Rogers , E. M. ( 1983 ). Diffusion of innovations (3rd ed.). New York: Free Press .
Rogers , E. M. ( 1986 ). Communication technology: The new media in society . New York: Free Press .
Rutter , D. R. ( 1987 ). Communicating by telephone . Oxford, England: Pergamon Press .
Schmitz , J. , & Fulk , J. ( 1991 ). Organizational colleagues, media richness, and electronic mail: A test of the social influence model of technology use . Communication Research , 18 ( 4 ), 487 – 523 .
Shank , G. ( 1993 ), Abductive multiloguing the semiotic dynamics of navigating the net , The Arachnet Electronic Journal on Virtual Culture , 1 ( 1 ). [Online]. Available: ftp://ftp.lib.ncsu.edu/pub/stacks/aejvc/aejvc-v1n01-shank-abductive .
Siegel , J. , Dubrovsky , V. , Kiesler , S. , & McGuire , T. ( 1986 ). Group processes in computer- mediated communication . Organizational Behavior and Human Decision Processes , 37 , 157 – 187 .
Spears , R. , & Lea , M. ( 1992 ). Social influence and the influence of the ‘social’ in computer- mediated communication . In M. Lea (ed.), Contexts of computer-mediated communication (pp. 30 – 65 ). New York: Harvester Wheatsheaf .
Spears , R. , Lea , M. , & Lee , S. ( 1990 ). De-individuation and group polarization in computer- mediated communication . British Journal of Social Psychology , 29 , 121 – 134 .
Spitzer , M. ( 1986 ). Writing style in computer conferences . IEEE Transactions on Professional Communications , 29 ( 1 ), 19 – 22 .
Sproull , L. , & Kiesler , S. ( 1986 ). Reducing social context cues: Electronic mail in organizational communication . Management Science , 32 ( 11 ), 1492 – 1513 .
Trevino , L. , Lengel , R. H. , Bodensteiner , W. , Gerloff , E. , & Muir , N. K. ( 1990 ). The richness imperative and cognitive style: The role of individual differences in media choice behavior . Management Communication Quarterly , 4 , 176 – 197 .
Trevino , L. K. , Daft , R. L. , & Lengel , R. H. ( 1990 ). Understanding managers' media choices: A symbolic interactionist perspective . In J. Fulk & C. Steinfield (Eds.), Organizations and communication technology (pp. 71 – 94 ). Newbury Park, CA: Sage .
Trevino , L. K. , Lengel , R. H. , & Daft , R. L. ( 1987 ). Media symbolism, media richness, and media choice in organizations: A symbolic interactionist perspective . Communication Research , 14 ( 5 ), 553 – 74 .
Valente , T. ( 1991 ). Thresholds and critical mass: Mathematical models of diffusion of innovation . Unpublished doctoral dissertation, University of Southern California , Annenberg School for Communication.
Vallee , J. ( 1982 ). The network revolution: Confessions of a computer scientist . Berkeley, CA: And/Or Press .
Wenner , L. A. ( 1985 ). The nature of news gratifications . In K. E. Rosengren , L. A. Wenner , & P. Palmgreen (Eds.), Media gratifications research: Current perspectives (pp. 171 – 193 ). Beverly Hills, CA: Sage .
Wenner , L. A. ( 1986 ). Model specification and theoretical development in gratifications sought and obtained research: A comparison of discrepancy and transactional approaches . Communication Monographs , 53 , 160 – 179 .
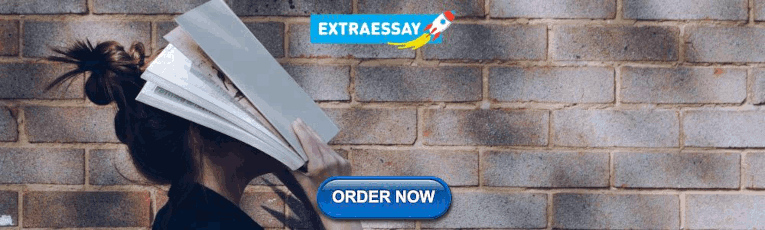
Email alerts
Citing articles via.
- Recommend to Your Librarian
- Advertising and Corporate Services
Affiliations
- Online ISSN 1083-6101
- Copyright © 2024 International Communication Association
- About Oxford Academic
- Publish journals with us
- University press partners
- What we publish
- New features
- Open access
- Institutional account management
- Rights and permissions
- Get help with access
- Accessibility
- Advertising
- Media enquiries
- Oxford University Press
- Oxford Languages
- University of Oxford
Oxford University Press is a department of the University of Oxford. It furthers the University's objective of excellence in research, scholarship, and education by publishing worldwide
- Copyright © 2024 Oxford University Press
- Cookie settings
- Cookie policy
- Privacy policy
- Legal notice
This Feature Is Available To Subscribers Only
Sign In or Create an Account
This PDF is available to Subscribers Only
For full access to this pdf, sign in to an existing account, or purchase an annual subscription.
Popular searches
- How to Get Participants For Your Study
- How to Do Segmentation?
- Conjoint Preference Share Simulator
- MaxDiff Analysis
- Likert Scales
- Reliability & Validity
Request consultation
Do you need support in running a pricing or product study? We can help you with agile consumer research and conjoint analysis.
Looking for an online survey platform?
Conjointly offers a great survey tool with multiple question types, randomisation blocks, and multilingual support. The Basic tier is always free.
Research Methods Knowledge Base
- Navigating the Knowledge Base
- Five Big Words
- Types of Research Questions
- Time in Research
- Types of Relationships
- Types of Data
Unit of Analysis
- Two Research Fallacies
- Philosophy of Research
- Ethics in Research
- Conceptualizing
- Evaluation Research
- Measurement
- Research Design
- Table of Contents
Fully-functional online survey tool with various question types, logic, randomisation, and reporting for unlimited number of surveys.
Completely free for academics and students .
One of the most important ideas in a research project is the unit of analysis . The unit of analysis is the major entity that you are analyzing in your study. For instance, any of the following could be a unit of analysis in a study:
- individuals
- artifacts (books, photos, newspapers)
- geographical units (town, census tract, state)
- social interactions (dyadic relations, divorces, arrests)
Why is it called the ‘unit of analysis’ and not something else (like, the unit of sampling)? Because it is the analysis you do in your study that determines what the unit is . For instance, if you are comparing the children in two classrooms on achievement test scores, the unit is the individual child because you have a score for each child. On the other hand, if you are comparing the two classes on classroom climate, your unit of analysis is the group, in this case the classroom, because you only have a classroom climate score for the class as a whole and not for each individual student. For different analyses in the same study you may have different units of analysis. If you decide to base an analysis on student scores, the individual is the unit. But you might decide to compare average classroom performance. In this case, since the data that goes into the analysis is the average itself (and not the individuals’ scores) the unit of analysis is actually the group. Even though you had data at the student level, you use aggregates in the analysis. In many areas of social research these hierarchies of analysis units have become particularly important and have spawned a whole area of statistical analysis sometimes referred to as hierarchical modeling . This is true in education, for instance, where we often compare classroom performance but collected achievement data at the individual student level.
Cookie Consent
Conjointly uses essential cookies to make our site work. We also use additional cookies in order to understand the usage of the site, gather audience analytics, and for remarketing purposes.
For more information on Conjointly's use of cookies, please read our Cookie Policy .
Which one are you?
I am new to conjointly, i am already using conjointly.

Community Blog
Keep up-to-date on postgraduate related issues with our quick reads written by students, postdocs, professors and industry leaders.
The Unit of Analysis Explained

- By DiscoverPhDs
- October 3, 2020
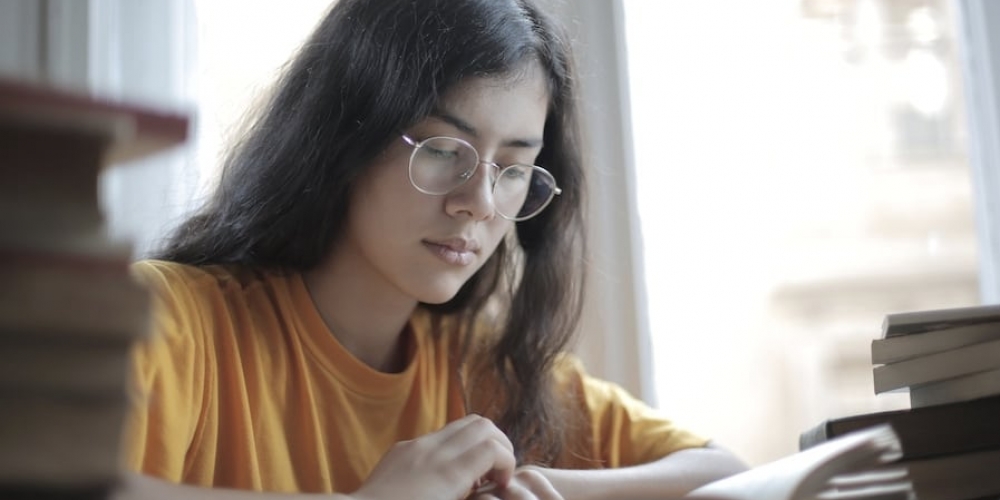
The unit of analysis refers to the main parameter that you’re investigating in your research project or study. Example of the different types of unit analysis that may be used in a project include:
- Individual people
- Groups of people
- Objects such as photographs, newspapers and books
- Geographical unit based on parameters such as cities or counties
- Social parameters such as births, deaths, divorces
The unit of analysis is named as such because the unit type is determined based on the actual data analysis that you perform in your project or study.
For example, if your research is based around data on exam grades for students at two different universities, then the unit of analysis is the data for the individual student due to each student having an exam score associated with them.
Conversely if your study is based on comparing noise level data between two different lecture halls full of students, then your unit of analysis here is the collective group of students in each hall rather than any data associated with an individual student.
In the same research study involving the same students, you may perform different types of analysis and this will be reflected by having different units of analysis. In the example of student exam scores, if you’re comparing individual exam grades then the unit of analysis is the individual student.
On the other hand, if you’re comparing the average exam grade between two universities, then the unit of analysis is now the group of students as you’re comparing the average of the group rather than individual exam grades.
These different levels of hierarchies of units of analysis can become complex with multiple levels. In fact, its complexity has led to a new field of statistical analysis that’s commonly known as hierarchical modelling.
As a researcher, you need to be clear on what your specific research questio n is. Based on this, you can define each data, observation or other variable and how they make up your dataset.
A clarity of your research question will help you identify your analysis units and the appropriate sample size needed to obtain a meaningful result (and is this a random sample/sampling unit or something else).
In developing your research method, you need to consider whether you’ll need any repeated observation of each measurement. You also need to consider whether you’re working with qualitative data/qualitative research or if this is quantitative content analysis.
The unit of analysis of your study is the specifically ‘who’ or what’ it is that your analysing – for example are you analysing the individual student, the group of students or even the whole university. You may have to consider a different unit of analysis based on the concept you’re considering, even if working with the same observation data set.
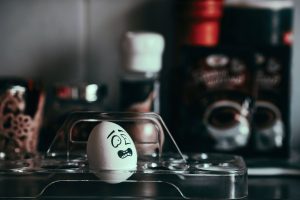
Impostor Syndrome is a common phenomenon amongst PhD students, leading to self-doubt and fear of being exposed as a “fraud”. How can we overcome these feelings?
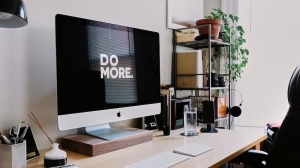
Learn more about using cloud storage effectively, video conferencing calling, good note-taking solutions and online calendar and task management options.
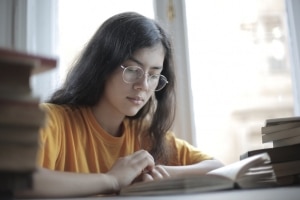
The unit of analysis refers to the main parameter that you’re investigating in your research project or study.
Join thousands of other students and stay up to date with the latest PhD programmes, funding opportunities and advice.
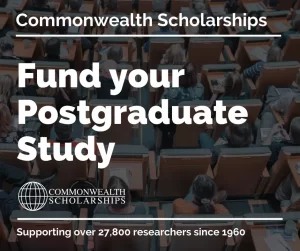
Browse PhDs Now

If you’re about to sit your PhD viva, make sure you don’t miss out on these 5 great tips to help you prepare.
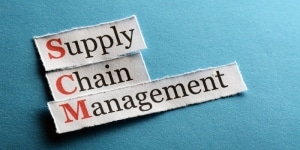
Need to write a list of abbreviations for a thesis or dissertation? Read our post to find out where they go, what to include and how to format them.

Kamal is a second year PhD student University of Toronto in the department of Chemistry. His research is focused on making hydrogen gas more affordable and easier to generate from water to use as a clean energy source.
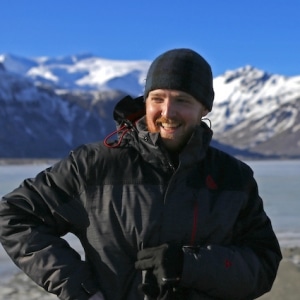
Adam’s about to start his fifth year of PhD research at the University of Massachusetts Amherst, where he’s earning a doctorate in anthropology as an affiliated researcher with the University of Iceland’s medical school.
Join Thousands of Students
- Unit of Analysis: Definition, Types & Examples

Introduction
A unit of analysis is the smallest level of analysis for a research project. It’s important to choose the right unit of analysis because it helps you make more accurate conclusions about your data.
What Is a Unit of Analysis?
A unit of analysis is the smallest element in a data set that can be used to identify and describe a phenomenon or the smallest unit that can be used to gather data about a subject. The unit of analysis will determine how you will define your variables, which are the things that you measure in your data.
If you want to understand why people buy a particular product, you should choose a unit of analysis that focuses on buying behavior. This means choosing a unit of analysis that is relevant to your research topic and question .
For example, if you want to study the needs of soldiers in a war zone, you will need to choose an appropriate unit of analysis for this study: soldiers or the war zone. In this case, choosing the right unit of analysis would be important because it could help you decide if your research design is appropriate for this particular subject and situation.
Why is Choosing the Right Unit of Analysis Important?
The unit of analysis is important because it helps you understand what you are trying to find out about your subject, and it also helps you to make decisions about how to proceed with your research.
Choosing the right unit of analysis is also important because it determines what information you’re going to use in your research. If you have a small sample, then you’ll have to choose whether or not to focus on the entire population or just a subset of it.
If you have a large sample, then you’ll be able to find out more about specific groups within your population. For example, if you want to understand why people buy certain types of products, then you should choose a unit of analysis that focuses on buying behavior.
This means choosing a unit of analysis that is relevant to your research topic and question.
Unit of Analysis vs Unit of Observation
Unit of analysis is a term used to refer to a particular part of a data set that can be analyzed. For example, in the case of a survey, the unit of analysis is an individual: the person who was selected to take part in the survey.
Unit of analysis is used in the social sciences to refer to the individuals or groups that have been studied. It can also be referred to as the unit of observation.
Unit of observation refers to a specific person or group in the study being observed by the researcher. An example would be a particular town, census tract, state, or other geographical location being studied by researchers conducting research on crime rates in that area.
Unit of analysis refers to the individual or group being studied by the researcher. An example would be an entire town being analyzed for crime rates over time.
Types of “Unit of Analysis”
The unit of analysis is a way to understand and study a phenomenon. There are four main types of unit of analysis: individuals, groups, artifacts (books, photos, newspapers), and geographical units (towns, census tracts, states).
- Individuals are the smallest level of analysis. For example, an individual may be a person or an animal. A group can be composed of individuals or a collection of people who interact with each other. For example, an individual might go to college with other individuals or a family might live together as roommates.
- An artifact is anything that can be studied using empirical methods—including books and photos but also any physical object like knives or phones.
- A geographical unit is smaller than an entire country but larger than just one city block or neighborhood; it may be smaller than just two houses but larger than just two houses in the same street.
- Social interactions include dyadic relations (such as friendships or romantic relationships) and divorces among many other things such as arrests.
Examples of Each Type of Unit of Analysis
- Individuals are the smallest unit of analysis. An individual is a person, animal, or thing. For example, an individual can be a person or a building.
- Artifacts are the next largest units of analysis. An artifact is something produced by human beings and is not alive. For example, a child’s toy is an artifact. Artifacts can include any material object that was produced by human activity and which has meaning to someone. Artifacts can be tangible or intangible and may be produced intentionally or accidentally.
- Geographical units are large geographic areas such as states, counties, provinces, etc. Geographical units may also refer to specific locations within these areas such as cities or townships.
- Social interaction refers to interactions between members of society (e.g., family members interacting with each other). Social interaction includes both formal interactions (such as attending school) and informal interactions (such as talking on the phone).
How Does a Social Scientist Choose a Unit of Analysis?
Social scientists choose a unit of analysis based on the purpose of their research, their research question, and the type of data they have. For example, if they are trying to understand the relationship between a person’s personality and their behavior, they would choose to study personality traits.
For example, if a researcher wanted to study the effects of legalizing marijuana on crime rates, they may choose to use administrative data from police departments. However, if they wanted to study how culture influences crime rates, they might use survey data from smaller groups of people who are further removed from the influence of culture (e.g., individuals living in different areas or countries).
Factors to Consider When Choosing a Unit of Analysis
The unit of analysis is the object or person that you are studying, and it determines what kind of data you are collecting and how you will analyze it.
Factors to consider when choosing a unit of analysis include:
- What is your purpose for studying this topic? Is it for a research paper or an article? If so, which type of paper do you want to write?
- What is the most appropriate unit for your study? If you are studying a specific event or period of time, this may be obvious. But if your focus is broader, such as all social sciences or all human development, then you need to determine how broad your scope should be before beginning any research process (see question one above) so that you know where to start in order for it to be effective (see question three below).
- How do other people define their units? This can be helpful when trying to understand what other people mean when they use certain terms like “social science” or “human development” because they may define those terms differently than what you would expect them to.
- The nature of the data collected. Is it quantitative or qualitative? If it’s qualitative, what kind of data is collected? How much time was spent observing each participant/examining their behavior?
- The scale used to measure variables. Is every variable measured on a one-to-one scale (like measurements between people)? Or do some variables only take on discrete values (like yes/no questions)?
The unit of analysis is the smallest part of a data set that you analyze. It’s important to remember that your data is made up of more than just one unit—you have lots of different units in your dataset, and each of those units has its own characteristics that you need to think about when you’re trying to analyze it.
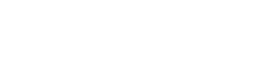
Connect to Formplus, Get Started Now - It's Free!
- Data Collection
- research questions
- unit of analysis
- Olayemi Jemimah Aransiola
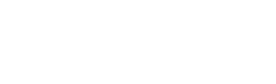
You may also like:
Research Summary: What Is It & How To Write One
Introduction A research summary is a requirement during academic research and sometimes you might need to prepare a research summary...

The McNamara Fallacy: How Researchers Can Detect and to Avoid it.
Introduction The McNamara Fallacy is a common problem in research. It happens when researchers take a single piece of data as evidence...
Projective Techniques In Surveys: Definition, Types & Pros & Cons
Introduction When you’re conducting a survey, you need to find out what people think about things. But how do you get an accurate and...
What is Field Research: Meaning, Examples, Pros & Cons
Introduction Field research is a method of research that deals with understanding and interpreting the social interactions of groups of...
Formplus - For Seamless Data Collection
Collect data the right way with a versatile data collection tool. try formplus and transform your work productivity today..

An official website of the United States government
The .gov means it’s official. Federal government websites often end in .gov or .mil. Before sharing sensitive information, make sure you’re on a federal government site.
The site is secure. The https:// ensures that you are connecting to the official website and that any information you provide is encrypted and transmitted securely.
- Publications
- Account settings
Preview improvements coming to the PMC website in October 2024. Learn More or Try it out now .
- Advanced Search
- Journal List
- v.23(1); 2018 Feb

Directed qualitative content analysis: the description and elaboration of its underpinning methods and data analysis process
Qualitative content analysis consists of conventional, directed and summative approaches for data analysis. They are used for provision of descriptive knowledge and understandings of the phenomenon under study. However, the method underpinning directed qualitative content analysis is insufficiently delineated in international literature. This paper aims to describe and integrate the process of data analysis in directed qualitative content analysis. Various international databases were used to retrieve articles related to directed qualitative content analysis. A review of literature led to the integration and elaboration of a stepwise method of data analysis for directed qualitative content analysis. The proposed 16-step method of data analysis in this paper is a detailed description of analytical steps to be taken in directed qualitative content analysis that covers the current gap of knowledge in international literature regarding the practical process of qualitative data analysis. An example of “the resuscitation team members' motivation for cardiopulmonary resuscitation” based on Victor Vroom's expectancy theory is also presented. The directed qualitative content analysis method proposed in this paper is a reliable, transparent, and comprehensive method for qualitative researchers. It can increase the rigour of qualitative data analysis, make the comparison of the findings of different studies possible and yield practical results.
Introduction
Qualitative content analysis (QCA) is a research approach for the description and interpretation of textual data using the systematic process of coding. The final product of data analysis is the identification of categories, themes and patterns ( Elo and Kyngäs, 2008 ; Hsieh and Shannon, 2005 ; Zhang and Wildemuth, 2009 ). Researchers in the field of healthcare commonly use QCA for data analysis ( Berelson, 1952 ). QCA has been described and used in the first half of the 20th century ( Schreier, 2014 ). The focus of QCA is the development of knowledge and understanding of the study phenomenon. QCA, as the application of language and contextual clues for making meanings in the communication process, requires a close review of the content gleaned from conducting interviews or observations ( Downe-Wamboldt, 1992 ; Hsieh and Shannon, 2005 ).
QCA is classified into conventional (inductive), directed (deductive) and summative methods ( Hsieh and Shannon, 2005 ; Mayring, 2000 , 2014 ). Inductive QCA, as the most popular approach in data analysis, helps with the development of theories, schematic models or conceptual frameworks ( Elo and Kyngäs, 2008 ; Graneheim and Lundman, 2004 ; Vaismoradi et al., 2013 , 2016 ), which should be refined, tested or further developed by using directed QCA ( Elo and Kyngäs, 2008 ). Directed QCA is a common method of data analysis in healthcare research ( Elo and Kyngäs, 2008 ), but insufficient knowledege is available about how this method is applied ( Elo and Kyngäs, 2008 ; Hsieh and Shannon, 2005 ). This may hamper the use of directed QCA by novice qualitative researchers and account for a low application of this method compared with the inductive method ( Elo and Kyngäs, 2008 ; Mayring, 2000 ). Therefore, this paper aims to describe and integrate methods applied in directed QCA.
International databases such as PubMed (including Medline), Scopus, Web of Science and ScienceDirect were searched for retrieval of papers related to QCA and directed QCA. Use of keywords such as ‘directed content analysis’, ‘deductive content analysis’ and ‘qualitative content analysis’ led to 13,738 potentially eligible papers. Applying inclusion criteria such as ‘focused on directed qualitative content analysis’ and ‘published in peer-reviewed journals’; and removal of duplicates resulted in 30 papers. However, only two of these papers dealt with the description of directed QCA in terms of the methodological process. Ancestry and manual searches within these 30 papers revealed the pioneers of the description of this method in international literature. A further search for papers published by the method's pioneers led to four more papers and one monograph dealing with directed QCA ( Figure 1 ).

The search strategy for the identification of papers.
Finally, the authors of this paper integrated and elaborated a comprehensive and stepwise method of directed QCA based on the commonalities of methods discussed in the included papers. Also, the experiences of the current authors in the field of qualitative research were incorporated into the suggested stepwise method of data analysis for directed QCA ( Table 1 ).
The suggested steps for directed content analysis.
While the included papers about directed QCA were the most cited ones in international literature, none of them provided sufficient detail with regard to how to conduct the data analysis process. This might hamper the use of this method by novice qualitative researchers and hinder its application by nurse researchers compared with inductive QCA. As it can be seen in Figure 1 , the search resulted in 5 articles that explain DCA method. The following is description of the articles, along with their strengths and weaknesses. Authors used the strengths in their suggested method as mentioned in Table 1 .
The methods suggested for directed QCA in the international literature
The method suggested by hsieh and shannon (2005).
Hsieh and Shannon (2005) developed two strategies for conducting directed QCA. The first strategy consists of reading textual data and highlighting those parts of the text that, on first impression, appeared to be related to the predetermined codes dictated by a theory or prior research findings. Next, the highlighted texts would be coded using the predetermined codes.
As for the second strategy, the only difference lay in starting the coding process without primarily highlighting the text. In both analysis strategies, the qualitative researcher should return to the text and perform reanalysis after the initial coding process ( Hsieh and Shannon, 2005 ). However, the current authors believe that this second strategy provides an opportunity for recognising missing texts related to the predetermined codes and also newly emerged ones. It also enhances the trustworthiness of findings.
As an important part of the method suggested by Hsieh and Shannon (2005) , the term ‘code’ was used for the different levels of abstraction, but a more precise definition of this term seems to be crucial. For instance, they stated that ‘data that cannot be coded are identified and analyzed later to determine if they represent a new category or a subcategory of an existing code’ (2005: 1282).
It seems that the first ‘code’ in the above sentence indicates the lowest level of abstraction that could be achieved instantly from raw data. However, the ‘code’ at the end of the sentence refers to a higher level of abstraction, because it denotes to a category or subcategory.
Furthermore, the interchangeable and inconsistent use of the words ‘predetermined code’ and ‘category’ could be confusing to novice qualitative researchers. Moreover, Hsieh and Shannon (2005) did not specify exactly which parts of the text, whether highlighted, coded or the whole text, should be considered during the reanalysis of the text after initial coding process. Such a lack of specification runs the risk of missing the content during the initial coding process, especially if the second review of the text is restricted to highlighted sections. One final important omission in this method is the lack of an explicit description of the process through which new codes emerge during the reanalysis of the text. Such a clarification is crucial, because the detection of subtle links between newly emerging codes and the predetermined ones is not straightforward.
The method suggested by Elo and Kyngäs (2008)
Elo and Kyngäs (2008) suggested ‘structured’ and ‘unconstrained’ methods or paths for directed QCA. Accordingly, after determining the ‘categorisation matrix’ as the framework for data collection and analysis during the study process, the whole content would be reviewed and coded. The use of the unconstrained matrix allows the development of some categories inductively by using the steps of ‘grouping’, ‘categorisation’ and ‘abstraction’. The use of a structured method requires a structured matrix upon which data are strictly coded. Hypotheses suggested by previous studies often are tested using this method ( Elo and Kyngäs, 2008 ).
The current authors believe that the label of ‘data gathering by the content’ (p. 110) in the unconstrained matrix path can be misleading. It refers to the data coding step rather than data collection. Also, in the description of the structured path there is an obvious discrepancy with regard to the selection of the portions of the content that fit or do not fit the matrix: ‘… if the matrix is structured, only aspects that fit the matrix of analysis are chosen from the data …’; ‘… when using a structured matrix of analysis, it is possible to choose either only the aspects from the data that fit the categorization frame or, alternatively, to choose those that do not’ ( Elo and Kyngäs, 2008 : 111–112).
Figure 1 in Elo and Kyngäs's paper ( 2008 : 110) clearly distinguished between the structured and unconstrained paths. On the other hand, the first sentence in the above quotation clearly explained the use of the structured matrix, but it was not clear whether the second sentence referred to the use of the structured or unconstrained matrix.
The method suggested by Zhang and Wildemuth (2009)
Considering the method suggested by Hsieh and Shannon (2005) , Zhang and Wildemuth (2009) suggested an eight-step method as follows: (1) preparation of data, (2) definition of the unit of analysis, (3) development of categories and the coding scheme, (4) testing the coding scheme in a text sample, (5) coding the whole text, (6) assessment of the coding's consistency, (7) drawing conclusions from the coded data, and (8) reporting the methods and findings ( Zhang and Wildemuth, 2009 ). Only in the third step of this method, the description of the process of category development, did Zhang and Wildemuth (2009) briefly make a distinction between the inductive versus deductive content analysis methods. On first impression, the only difference between the two approaches seems to be the origin from which categories are developed. In addition, the process of connecting the preliminary codes extracted from raw data with predetermined categories is described. Furthermore, it is not clear whether this linking should be established from categories to primary codes, or vice versa.
The method suggested by Mayring ( 2000 , 2014 )
Mayring ( 2000 , 2014 ) suggested a seven-step method for directed QCA that distinctively differentiated between inductive and deductive methods as follows: (1) determination of the research question and theoretical background, (2) definition of the category system such as main categories and subcategories based on the previous theory and research, (3) establishing a guideline for coding, considering definitions, anchor examples and coding rules, (5) reading the whole text, determining preliminary codes, adding anchor examples and coding rules, (5) revision of the category and coding guideline after working through 10–50% of the data, (6) reworking data if needed, or listing the final category, and (7) analysing and interpreting based on the category frequencies and contingencies.
Mayring suggested that coding rules should be defined to distinctly assign the parts of the text to a particular category. Furthermore, indicating which concrete part of the text serves as typical examples, also known as ‘anchor samples’, and belongs to a particular category was recommended for describing each category ( Mayring, 2000 , 2014 ). The current authors believe that these suggestions help clarify directed QCA and enhance its trustworthiness.
But when the term ‘preliminary coding’ was used, Mayring ( 2000 , 2014 ) did not clearly clarify whether these codes are inductively or deductively created. In addition, Mayring was inclined to apply the quantitative approach implicitly in steps 5 and 7, which is incongruent with the qualitative paradigm. Furthermore, nothing was stated about the possibility of the development of new categories from the textual material: ‘… theoretical considerations can lead to a further categories or rephrasing of categories from previous studies, but the categories are not developed out of the text material like in inductive category formation …’ ( Mayring, 2014 : 97).
Integration and clarification of methods for directed QCA
Directed QCA took different paths when the categorisation matrix contained concepts with higher-level versus lower-level abstractions. In matrices with low abstraction levels, linking raw data to predetermined categories was not difficult, and suggested methods in international nursing literature seem appropriate and helpful. For instance, Elo and Kyngäs (2008) introduced ‘mental well-being threats’ based on the categories of ‘dependence’, ‘worries’, ‘sadness’ and ‘guilt’. Hsieh and Shannon (2005) developed the categories of ‘denial’, ‘anger’, ‘bargaining’, ‘depression’ and ‘acceptance’ when elucidating the stages of grief. Therefore, the low-level abstractions easily could link raw data to categories. The predicament of directed QCA began when the categorisation matrix contained the concepts with high levels of abstraction. The gap regarding how to connect the highly abstracted categories to the raw data should be bridged by using a transparent and comprehensive analysis strategy. Therefore, the authors of this paper integrated the methods of directed QCA outlined in the international literature and elaborated them using the phases of ‘preparation’, ‘organization’ and ‘reporting’ proposed by Elo and Kyngäs (2008) . Also, the experiences of the current authors in the field of qualitative research were incorporated into their suggested stepwise method of data analysis. The method was presented using the example of the “team members’ motivation for cardiopulmonary resuscitation (CPR)” based on Victor Vroom's expectancy theory ( Assarroudi et al., 2017 ). In this example, interview transcriptions were considered as the unit of analysis, because interviews are the most common method of data collection in qualitative studies ( Gill et al., 2008 ).
Suggested method of directed QCA by the authors of this paper
This method consists of 16 steps and three phases, described below: preparation phase (steps 1–7), organisation phase (steps 8–15), and reporting phase (step 16).
The preparation phase:
- The acquisition of general skills . In the first step, qualitative researchers should develop skills including self-critical thinking, analytical abilities, continuous self-reflection, sensitive interpretive skills, creative thinking, scientific writing, data gathering and self-scrutiny ( Elo et al., 2014 ). Furthermore, they should attain sufficient scientific and content-based mastery of the method chosen for directed QCA. In the proposed example, qualitative researchers can achieve this mastery through conducting investigations in original sources related to Victor Vroom's expectancy theory. Main categories pertaining to Victor Vroom's expectancy theory were ‘expectancy’, ‘instrumentality’ and ‘valence’. This theory defined ‘expectancy’ as the perceived probability that efforts could lead to good performance. ‘Instrumentality’ was the perceived probability that good performance led to desired outcomes. ‘Valence’ was the value that the individual personally placed on outcomes ( Vroom, 1964 , 2005 ).
- Selection of the appropriate sampling strategy . Qualitative researchers need to select the proper sampling strategies that facilitate an access to key informants on the study phenomenon ( Elo et al., 2014 ). Sampling methods such as purposive, snowball and convenience methods ( Coyne, 1997 ) can be used with the consideration of maximum variations in terms of socio-demographic and phenomenal characteristics ( Sandelowski, 1995 ). The sampling process ends when information ‘redundancy’ or ‘saturation’ is reached. In other words, it ends when all aspects of the phenomenon under study are explored in detail and no additional data are revealed in subsequent interviews ( Cleary et al., 2014 ). In line with this example, nurses and physicians who are the members of the CPR team should be selected, given diversity in variables including age, gender, the duration of work, number of CPR procedures, CPR in different patient groups and motivation levels for CPR.
- Deciding on the analysis of manifest and/or latent content . Qualitative researchers decide whether the manifest and/or latent contents should be considered for analysis based on the study's aim. The manifest content is limited to the transcribed interview text, but latent content includes both the researchers' interpretations of available text, and participants' silences, pauses, sighs, laughter, posture, etc. ( Elo and Kyngäs, 2008 ). Both types of content are recommended to be considered for data analysis, because a deep understanding of data is preferred for directed QCA ( Thomas and Magilvy, 2011 ).
- Developing an interview guide . The interview guide contains open-ended questions based on the study's aims, followed by directed questions about main categories extracted from the existing theory or previous research ( Hsieh and Shannon, 2005 ). Directed questions guide how to conduct interviews when using directed or conventional methods. The following open-ended and directed questions were used in this example: An open-ended question was ‘What is in your mind when you are called for performing CPR?’ The directed question for the main category of ‘expectancy’ could be ‘How does the expectancy of the successful CPR procedure motivate you to resuscitate patients?’
- Conducting and transcribing interviews . An interview guide is used to conduct interviews for directed QCA. After each interview session, the entire interview is transcribed verbatim immediately ( Poland, 1995 ) and with utmost care ( Seidman, 2013 ). Two recorders should be used to ensure data backup ( DiCicco-Bloom and Crabtree, 2006 ). (For more details concerning skills required for conducting successful qualitative interviews, see Edenborough, 2002 ; Kramer, 2011 ; Schostak, 2005 ; Seidman, 2013 ).
- Specifying the unit of analysis . The unit of analysis may include the person, a program, an organisation, a class, community, a state, a country, an interview, or a diary written by the researchers ( Graneheim and Lundman, 2004 ). The transcriptions of interviews are usually considered units of analysis when data are collected using interviews. In this example, interview transcriptions and filed notes are considered as the units of analysis.
- Immersion in data . The transcribed interviews are read and reviewed several times with the consideration of the following questions: ‘Who is telling?’, ‘Where is this happening?’, ‘When did it happen?’, ‘What is happening?’, and ‘Why?’ ( Elo and Kyngäs, 2008 ). These questions help researchers get immersed in data and become able to extract related meanings ( Elo and Kyngäs, 2008 ; Elo et al., 2014 ).
The organisation phase:
The categorisation matrix of the team members' motivation for CPR.
CPR: cardiopulmonary resuscitation.
- Theoretical definition of the main categories and subcategories . Derived from the existing theory or previous research, the theoretical definitions of categories should be accurate and objective ( Mayring, 2000 , 2014 ). As for this example, ‘expectancy’ as a main category could be defined as the “subjective probability that the efforts by an individual led to an acceptable level of performance (effort–performance association) or to the desired outcome (effort–outcome association)” ( Van Eerde and Thierry, 1996 ; Vroom, 1964 ).
- – Expectancy in the CPR was a subjective probability formed in the rescuer's mind.
- – This subjective probability should be related to the association between the effort–performance or effort–outcome relationship perceived by the rescuer.
- The pre-testing of the categorisation matrix . The categorisation matrix should be tested using a pilot study. This is an essential step, particularly if more than one researcher is involved in the coding process. In this step, qualitative researchers should independently and tentatively encode the text, and discuss the difficulties in the use of the categorisation matrix and differences in the interpretations of the unit of analysis. The categorisation matrix may be further modified as a result of such discussions ( Elo et al., 2014 ). This also can increase inter-coder reliability ( Vaismoradi et al., 2013 ) and the trustworthiness of the study.
- Choosing and specifying the anchor samples for each main category . An anchor sample is an explicit and concise exemplification, or the identifier of a main category, selected from meaning units ( Mayring, 2014 ). An anchor sample for ‘expectancy’ as the main category of this example could be as follows: ‘… the patient with advanced metastatic cancer who requires CPR … I do not envision a successful resuscitation for him.’
An example of steps taken for the abstraction of the phenomenon of expectancy (main category).
CPR: cardiopulmonary resuscitation
- The inductive abstraction of main categories from preliminary codes . Preliminary codes are grouped and categorised according to their meanings, similarities and differences. The products of this categorisation process are known as ‘generic categories’ ( Elo and Kyngäs, 2008 ) ( Table 3 ).
- The establishment of links between generic categories and main categories . The constant comparison of generic categories and main categories results in the development of a conceptual and logical link between generic and main categories, nesting generic categories into the pre-existing main categories and creating new main categories. The constant comparison technique is applied to data analysis throughout the study ( Zhang and Wildemuth, 2009 ) ( Table 3 ).
The reporting phase:
- Reporting all steps of directed QCA and findings . This includes a detailed description of the data analysis process and the enumeration of findings ( Elo and Kyngäs, 2008 ). Findings should be systematically presented in such a way that the association between the raw data and the categorisation matrix is clearly shown and easily followed. Detailed descriptions of the sampling process, data collection, analysis methods and participants' characteristics should be presented. The trustworthiness criteria adopted along with the steps taken to fulfil them should also be outlined. Elo et al. (2014) developed a comprehensive and specific checklist for reporting QCA studies.
Trustworthiness
Multiple terms are used in the international literature regarding the validation of qualitative studies ( Creswell, 2013 ). The terms ‘validity’, ‘reliability’, and ‘generalizability’ in quantitative studies are equivalent to ‘credibility’, ‘dependability’, and ‘transferability’ in qualitative studies, respectively ( Polit and Beck, 2013 ). These terms, along with the additional concept of confirmability, were introduced by Lincoln and Guba (1985) . Polit and Beck added the term ‘authenticity’ to the list. Collectively, they are the different aspects of trustworthiness in all types of qualitative studies ( Polit and Beck, 2013 ).
To ehnance the trustworthiness of the directed QCA study, researchers should thoroughly delineate the three phases of ‘preparation’, ‘organization’, and ‘reporting’ ( Elo et al., 2014 ). Such phases are needed to show in detail how categories are developed from data ( Elo and Kyngäs, 2008 ; Graneheim and Lundman, 2004 ; Vaismoradi et al., 2016 ). To accomplish this, appendices, tables and figures may be used to depict the reduction process ( Elo and Kyngäs, 2008 ; Elo et al., 2014 ). Furthermore, an honest account of different realities during data analysis should be provided ( Polit and Beck, 2013 ). The authors of this paper believe that adopting this 16-step method can enhance the trustworthiness of directed QCA.
Directed QCA is used to validate, refine and/or extend a theory or theoretical framework in a new context ( Elo and Kyngäs, 2008 ; Hsieh and Shannon, 2005 ). The purpose of this paper is to provide a comprehensive, systematic, yet simple and applicable method for directed QCA to facilitate its use by novice qualitative researchers.
Despite the current misconceptions regarding the simplicity of QCA and directed QCA, knowledge development is required for conducting them ( Elo and Kyngäs, 2008 ). Directed QCA is often performed on a considerable amount of textual data ( Pope et al., 2000 ). Nevertheless, few studies have discussed the multiple steps need to be taken to conduct it. In this paper, we have integrated and elaborated the essential steps pointed to by international qualitative researchers on directed QCA such as ‘preliminary coding’, ‘theoretical definition’ ( Mayring, 2000 , 2014 ), ‘coding rule’, ‘anchor sample’ ( Mayring, 2014 ), ‘inductive analysis in directed qualitative content analysis’ ( Elo and Kyngäs, 2008 ), and ‘pretesting the categorization matrix’ ( Elo et al., 2014 ). Moreover, the authors have added a detailed discussion regarding ‘the use of inductive abstraction’ and ‘linking between generic categories and main categories’.
The importance of directed QCA is increased due to the development of knowledge and theories derived from QCA using the inductive approach, and the growing need to test the theories. Directed QCA proposed in this paper, is a reliable, transparent and comprehensive method that may increase the rigour of data analysis, allow the comparison of the findings of different studies, and yield practical results.
Abdolghader Assarroudi (PhD, MScN, BScN) is Assistant Professor in Nursing, Department of Medical‐Surgical Nursing, School of Nursing and Midwifery, Sabzevar University of Medical Sciences, Sabzevar, Iran. His main areas of research interest are qualitative research, instrument development study and cardiopulmonary resuscitation.
Fatemeh Heshmati Nabavi (PhD, MScN, BScN) is Assistant Professor in nursing, Department of Nursing Management, School of Nursing and Midwifery, Mashhad University of Medical Sciences, Mashhad, Iran. Her main areas of research interest are medical education, nursing management and qualitative study.
Mohammad Reza Armat (MScN, BScN) graduated from the Mashhad University of Medical Sciences in 1991 with a Bachelor of Science degree in nursing. He completed his Master of Science degree in nursing at Tarbiat Modarres University in 1995. He is an instructor in North Khorasan University of Medical Sciences, Bojnourd, Iran. Currently, he is a PhD candidate in nursing at the Mashhad School of Nursing and Midwifery, Mashhad University of Medical Sciences, Iran.
Abbas Ebadi (PhD, MScN, BScN) is professor in nursing, Behavioral Sciences Research Centre, School of Nursing, Baqiyatallah University of Medical Sciences, Tehran, Iran. His main areas of research interest are instrument development and qualitative study.
Mojtaba Vaismoradi (PhD, MScN, BScN) is a doctoral nurse researcher at the Faculty of Nursing and Health Sciences, Nord University, Bodø, Norway. He works in Nord’s research group ‘Healthcare Leadership’ under the supervision of Prof. Terese Bondas. For now, this team has focused on conducting meta‐synthesis studies with the collaboration of international qualitative research experts. His main areas of research interests are patient safety, elderly care and methodological issues in qualitative descriptive approaches. Mojtaba is the associate editor of BMC Nursing and journal SAGE Open in the UK.
Key points for policy, practice and/or research
- In this paper, essential steps pointed to by international qualitative researchers in the field of directed qualitative content analysis were described and integrated.
- A detailed discussion regarding the use of inductive abstraction, and linking between generic categories and main categories, was presented.
- A 16-step method of directed qualitative content analysis proposed in this paper is a reliable, transparent, comprehensive, systematic, yet simple and applicable method. It can increase the rigour of data analysis and facilitate its use by novice qualitative researchers.
Declaration of conflicting interests
The author(s) declared no potential conflicts of interest with respect to the research, authorship, and/or publication of this article.
The author(s) received no financial support for the research, authorship, and/or publication of this article.
- Assarroudi A, Heshmati Nabavi F, Ebadi A, et al.(2017) Professional rescuers' experiences of motivation for cardiopulmonary resuscitation: A qualitative study . Nursing & Health Sciences . 19(2): 237–243. [ PubMed ] [ Google Scholar ]
- Berelson B. (1952) Content Analysis in Communication Research , Glenoce, IL: Free Press. [ Google Scholar ]
- Cleary M, Horsfall J, Hayter M. (2014) Data collection and sampling in qualitative research: Does size matter? Journal of Advanced Nursing 70 ( 3 ): 473–475. [ PubMed ] [ Google Scholar ]
- Coyne IT. (1997) Sampling in qualitative research.. Purposeful and theoretical sampling; merging or clear boundaries? Journal of Advanced Nursing 26 ( 3 ): 623–630. [ PubMed ] [ Google Scholar ]
- Creswell JW. (2013) Research Design: Qualitative, Quantitative, and Mixed Methods Approaches , 4th edn. Thousand Oaks, CA: SAGE Publications. [ Google Scholar ]
- DiCicco-Bloom B, Crabtree BF. (2006) The qualitative research interview . Medical Education 40 ( 4 ): 314–321. [ PubMed ] [ Google Scholar ]
- Downe-Wamboldt B. (1992) Content analysis: Method, applications, and issues . Health Care for Women International 13 ( 3 ): 313–321. [ PubMed ] [ Google Scholar ]
- Edenborough R. (2002) Effective Interviewing: A Handbook of Skills and Techniques , 2nd edn. London: Kogan Page. [ Google Scholar ]
- Elo S, Kyngäs H. (2008) The qualitative content analysis process . Journal of Advanced Nursing 62 ( 1 ): 107–115. [ PubMed ] [ Google Scholar ]
- Elo S, Kääriäinen M, Kanste O, et al.(2014) Qualitative content analysis: A focus on trustworthiness . SAGE Open 4 ( 1 ): 1–10. [ Google Scholar ]
- Gill P, Stewart K, Treasure E, et al.(2008) Methods of data collection in qualitative research: Interviews and focus groups . British Dental Journal 204 ( 6 ): 291–295. [ PubMed ] [ Google Scholar ]
- Graneheim UH, Lundman B. (2004) Qualitative content analysis in nursing research: Concepts, procedures and measures to achieve trustworthiness . Nurse Education Today 24 ( 2 ): 105–112. [ PubMed ] [ Google Scholar ]
- Hsieh H-F, Shannon SE. (2005) Three approaches to qualitative content analysis . Qualitative Health Research 15 ( 9 ): 1277–1288. [ PubMed ] [ Google Scholar ]
- Kramer EP. (2011) 101 Successful Interviewing Strategies , Boston, MA: Course Technology, Cengage Learning. [ Google Scholar ]
- Lincoln YS, Guba EG. (1985) Naturalistic Inquiry , Beverly Hills, CA: SAGE Publications. [ Google Scholar ]
- Mayring P. (2000) Qualitative Content Analysis . Forum: Qualitative Social Research 1 ( 2 ): Available at: http://www.qualitative-research.net/fqs-texte/2-00/02-00mayring-e.htm (accessed 10 March 2005). [ Google Scholar ]
- Mayring P. (2014) Qualitative content analysis: Theoretical foundation, basic procedures and software solution , Klagenfurt: Monograph. Available at: http://nbn-resolving.de/urn:nbn:de:0168-ssoar-395173 (accessed 10 May 2015). [ Google Scholar ]
- Poland BD. (1995) Transcription quality as an aspect of rigor in qualitative research . Qualitative Inquiry 1 ( 3 ): 290–310. [ Google Scholar ]
- Polit DF, Beck CT. (2013) Essentials of Nursing Research: Appraising Evidence for Nursing Practice , 7th edn. China: Lippincott Williams & Wilkins. [ Google Scholar ]
- Pope C, Ziebland S, Mays N. (2000) Analysing qualitative data . BMJ 320 ( 7227 ): 114–116. [ PMC free article ] [ PubMed ] [ Google Scholar ]
- Sandelowski M. (1995) Sample size in qualitative research . Research in Nursing & Health 18 ( 2 ): 179–183. [ PubMed ] [ Google Scholar ]
- Schostak J. (2005) Interviewing and Representation in Qualitative Research , London: McGraw-Hill/Open University Press. [ Google Scholar ]
- Schreier M. (2014) Qualitative content analysis . In: Flick U. (ed.) The SAGE Handbook of Qualitative Data Analysis , Thousand Oaks, CA: SAGE Publications Ltd, pp. 170–183. [ Google Scholar ]
- Seidman I. (2013) Interviewing as Qualitative Research: A Guide for Researchers in Education and the Social Sciences , 3rd edn. New York: Teachers College Press. [ Google Scholar ]
- Thomas E, Magilvy JK. (2011) Qualitative rigor or research validity in qualitative research . Journal for Specialists in Pediatric Nursing 16 ( 2 ): 151–155. [ PubMed ] [ Google Scholar ]
- Vaismoradi M, Jones J, Turunen H, et al.(2016) Theme development in qualitative content analysis and thematic analysis . Journal of Nursing Education and Practice 6 ( 5 ): 100–110. [ Google Scholar ]
- Vaismoradi M, Turunen H, Bondas T. (2013) Content analysis and thematic analysis: Implications for conducting a qualitative descriptive study . Nursing & Health Sciences 15 ( 3 ): 398–405. [ PubMed ] [ Google Scholar ]
- Van Eerde W, Thierry H. (1996) Vroom's expectancy models and work-related criteria: A meta-analysis . Journal of Applied Psychology 81 ( 5 ): 575. [ Google Scholar ]
- Vroom VH. (1964) Work and Motivation , New York: Wiley. [ Google Scholar ]
- Vroom VH. (2005) On the origins of expectancy theory . In: Smith KG, Hitt MA. (eds) Great Minds in Management: The Process of Theory Development , Oxford: Oxford University Press, pp. 239–258. [ Google Scholar ]
- Zhang Y, Wildemuth BM. (2009) Qualitative analysis of content . In: Wildemuth B. (ed.) Applications of Social Research Methods to Questions in Information and Library Science , Westport, CT: Libraries Unlimited, pp. 308–319. [ Google Scholar ]
Columbia University Libraries
Data & statistics for journalists: unit of analysis.
- Data vs Statistics
- Geography of Data
Unit of Analysis
- Spatial Data
- Demographics
- Economics & Business
- Government (U.S.)
- International
- Maps & Geospatial Data
- Property / Housing
- Managing Data
- Statistical Software: R
The unit of analysis is the entity that you're analyzing. It's called this because it's your analysis (what you want to examine) that determines what this unit is, rather than the data itself.
For instance, let's say that you have a dataset with 40 students, divided between two classrooms of 20 students each, and a test score for each student. You can analyze this data in several ways:
- Individual unit of analysis: Compare the test scores of each student to the other students. (You're analyzing students, individuals.)
- Group unit of analysis: Compare the average test score of the two classrooms. (You're analyzing the classrooms, comparing two groups of individuals.)
Knowing your unit of analysis is helpful, because it helps you determine what kind of data you need. The other piece of this puzzle is whether you need macrodata (aggregated data) or microdata.
Microdata & Macrodata
So what is the difference between macrodata (aggregated data) and microdata ?
- MICRODATA Contains a record for every individual (e.g., person, company, etc.) in the survey/study. Source for US Census microdata: IPUMS
- MACRODATA (Aggregated Data) Higher-level data compiled from smaller (individual) units of data. For example, Census data in Social Explorer has been aggregated to preserve the confidentiality of individual respondents. Source for US Census macrodata: Social Explorer
- << Previous: Time
- Next: Spatial Data >>
- Last Updated: Nov 20, 2023 6:16 PM
- URL: https://guides.library.columbia.edu/journalism-data
- Donate Books or Items
- Suggestions & Feedback
- Report an E-Resource Problem
- The Bancroft Prizes
- Student Library Advisory Committee
- Jobs & Internships
- Behind the Scenes at Columbia's Libraries
Skip to content
CHOP Evaluates Strategies in the Pediatric Intensive Care Unit (PICU) to Enhance Communication and Ensure Equitable Participation in Research
Published on May 17, 2024
Pediatric Intensive Care Unit (PICU)
In a new retrospective cohort study, researchers at Children’s Hospital of Philadelphia (CHOP) discuss disparities in race and ethnicity when enrolling families to participate in research conducted in its Pediatric Intensive Care Unit (PICU). The findings were published May 15 in an article in the Journal of the American Medical Association (JAMA) Network Open entitled “Racial and Ethnic Disparity in Approach for Pediatric Intensive Care Unit Research Participation.”
Data on consent rate of families participating in research in the PICU remains limited. Prior studies have provided conflicting results on whether there are disparities in rates of enrollment into pediatric research, partly because rates of enrolled subjects are compared with their prevalence in the general US pediatric population.
“We need the right kind of study design to answer this question accurately,” said Nadir Yehya, MD, MSCE , a co-author of the study and an attending physician in the Pediatric Sepsis Program and the Division of Critical Care Medicine at Children's Hospital of Philadelphia.
Researchers reviewed detailed eligibility records of more than 3,100 children from CHOP’s PICU between 2011 and 2021 to see if there were disparities in rates of enrollment among kids who were eligible for these studies. They found that children in the PICU were less likely to be enrolled in studies based on factors such as race and ethnicity, language, religion, and social deprivation. Importantly, a large portion of this was due to eligible subjects not being approached for various reasons. However, even among those approached, Black children remained less likely to be enrolled.
As an example, authors noted that non-English speakers’ lower likelihood of consenting to participate in research in the PICU was in part since researchers were also less likely to approach them due to perceived language barriers. The authors suggest that improving how families are approached and providing research materials in multiple languages could help enhance equitable participation in PICU research.
CHOP now offers a daily interpreter in the PICU to bolster communication with families. The hospital system also plans to add additional resources to help translate lengthy research consent forms into other languages. The authors also suggest that other forms of consent, such as via telephone, could be more broadly adopted to allow families to choose whether to participate in research on their terms, rather than on the schedule of the research team.
“At CHOP we focus on issues that we hope will impact clinical outcomes, and the opportunity to benefit from and contribute to research is one of them,” said Yehya . “Taking a deep dive into our research approach in the PICU empowers us to enhance communication and trust between research teams and families, which simultaneously elevates potential new strategies for research engagement. Our goal is always to change the trajectory of children’s lives and maximize their ability to flourish.”
The CHOP Institutional review board reviewed the study, which was reported in accordance with the Strengthening the Reporting of Observational Studies in Epidemiology (STROBE) guidelines.
Artificial intelligence in strategy
Can machines automate strategy development? The short answer is no. However, there are numerous aspects of strategists’ work where AI and advanced analytics tools can already bring enormous value. Yuval Atsmon is a senior partner who leads the new McKinsey Center for Strategy Innovation, which studies ways new technologies can augment the timeless principles of strategy. In this episode of the Inside the Strategy Room podcast, he explains how artificial intelligence is already transforming strategy and what’s on the horizon. This is an edited transcript of the discussion. For more conversations on the strategy issues that matter, follow the series on your preferred podcast platform .
Joanna Pachner: What does artificial intelligence mean in the context of strategy?
Yuval Atsmon: When people talk about artificial intelligence, they include everything to do with analytics, automation, and data analysis. Marvin Minsky, the pioneer of artificial intelligence research in the 1960s, talked about AI as a “suitcase word”—a term into which you can stuff whatever you want—and that still seems to be the case. We are comfortable with that because we think companies should use all the capabilities of more traditional analysis while increasing automation in strategy that can free up management or analyst time and, gradually, introducing tools that can augment human thinking.
Joanna Pachner: AI has been embraced by many business functions, but strategy seems to be largely immune to its charms. Why do you think that is?
Subscribe to the Inside the Strategy Room podcast
Yuval Atsmon: You’re right about the limited adoption. Only 7 percent of respondents to our survey about the use of AI say they use it in strategy or even financial planning, whereas in areas like marketing, supply chain, and service operations, it’s 25 or 30 percent. One reason adoption is lagging is that strategy is one of the most integrative conceptual practices. When executives think about strategy automation, many are looking too far ahead—at AI capabilities that would decide, in place of the business leader, what the right strategy is. They are missing opportunities to use AI in the building blocks of strategy that could significantly improve outcomes.
I like to use the analogy to virtual assistants. Many of us use Alexa or Siri but very few people use these tools to do more than dictate a text message or shut off the lights. We don’t feel comfortable with the technology’s ability to understand the context in more sophisticated applications. AI in strategy is similar: it’s hard for AI to know everything an executive knows, but it can help executives with certain tasks.
When executives think about strategy automation, many are looking too far ahead—at AI deciding the right strategy. They are missing opportunities to use AI in the building blocks of strategy.
Joanna Pachner: What kind of tasks can AI help strategists execute today?
Yuval Atsmon: We talk about six stages of AI development. The earliest is simple analytics, which we refer to as descriptive intelligence. Companies use dashboards for competitive analysis or to study performance in different parts of the business that are automatically updated. Some have interactive capabilities for refinement and testing.
The second level is diagnostic intelligence, which is the ability to look backward at the business and understand root causes and drivers of performance. The level after that is predictive intelligence: being able to anticipate certain scenarios or options and the value of things in the future based on momentum from the past as well as signals picked in the market. Both diagnostics and prediction are areas that AI can greatly improve today. The tools can augment executives’ analysis and become areas where you develop capabilities. For example, on diagnostic intelligence, you can organize your portfolio into segments to understand granularly where performance is coming from and do it in a much more continuous way than analysts could. You can try 20 different ways in an hour versus deploying one hundred analysts to tackle the problem.
Predictive AI is both more difficult and more risky. Executives shouldn’t fully rely on predictive AI, but it provides another systematic viewpoint in the room. Because strategic decisions have significant consequences, a key consideration is to use AI transparently in the sense of understanding why it is making a certain prediction and what extrapolations it is making from which information. You can then assess if you trust the prediction or not. You can even use AI to track the evolution of the assumptions for that prediction.
Those are the levels available today. The next three levels will take time to develop. There are some early examples of AI advising actions for executives’ consideration that would be value-creating based on the analysis. From there, you go to delegating certain decision authority to AI, with constraints and supervision. Eventually, there is the point where fully autonomous AI analyzes and decides with no human interaction.
Because strategic decisions have significant consequences, you need to understand why AI is making a certain prediction and what extrapolations it’s making from which information.
Joanna Pachner: What kind of businesses or industries could gain the greatest benefits from embracing AI at its current level of sophistication?
Yuval Atsmon: Every business probably has some opportunity to use AI more than it does today. The first thing to look at is the availability of data. Do you have performance data that can be organized in a systematic way? Companies that have deep data on their portfolios down to business line, SKU, inventory, and raw ingredients have the biggest opportunities to use machines to gain granular insights that humans could not.
Companies whose strategies rely on a few big decisions with limited data would get less from AI. Likewise, those facing a lot of volatility and vulnerability to external events would benefit less than companies with controlled and systematic portfolios, although they could deploy AI to better predict those external events and identify what they can and cannot control.
Third, the velocity of decisions matters. Most companies develop strategies every three to five years, which then become annual budgets. If you think about strategy in that way, the role of AI is relatively limited other than potentially accelerating analyses that are inputs into the strategy. However, some companies regularly revisit big decisions they made based on assumptions about the world that may have since changed, affecting the projected ROI of initiatives. Such shifts would affect how you deploy talent and executive time, how you spend money and focus sales efforts, and AI can be valuable in guiding that. The value of AI is even bigger when you can make decisions close to the time of deploying resources, because AI can signal that your previous assumptions have changed from when you made your plan.
Joanna Pachner: Can you provide any examples of companies employing AI to address specific strategic challenges?
Yuval Atsmon: Some of the most innovative users of AI, not coincidentally, are AI- and digital-native companies. Some of these companies have seen massive benefits from AI and have increased its usage in other areas of the business. One mobility player adjusts its financial planning based on pricing patterns it observes in the market. Its business has relatively high flexibility to demand but less so to supply, so the company uses AI to continuously signal back when pricing dynamics are trending in a way that would affect profitability or where demand is rising. This allows the company to quickly react to create more capacity because its profitability is highly sensitive to keeping demand and supply in equilibrium.
Joanna Pachner: Given how quickly things change today, doesn’t AI seem to be more a tactical than a strategic tool, providing time-sensitive input on isolated elements of strategy?
Yuval Atsmon: It’s interesting that you make the distinction between strategic and tactical. Of course, every decision can be broken down into smaller ones, and where AI can be affordably used in strategy today is for building blocks of the strategy. It might feel tactical, but it can make a massive difference. One of the world’s leading investment firms, for example, has started to use AI to scan for certain patterns rather than scanning individual companies directly. AI looks for consumer mobile usage that suggests a company’s technology is catching on quickly, giving the firm an opportunity to invest in that company before others do. That created a significant strategic edge for them, even though the tool itself may be relatively tactical.
Joanna Pachner: McKinsey has written a lot about cognitive biases and social dynamics that can skew decision making. Can AI help with these challenges?
Yuval Atsmon: When we talk to executives about using AI in strategy development, the first reaction we get is, “Those are really big decisions; what if AI gets them wrong?” The first answer is that humans also get them wrong—a lot. [Amos] Tversky, [Daniel] Kahneman, and others have proven that some of those errors are systemic, observable, and predictable. The first thing AI can do is spot situations likely to give rise to biases. For example, imagine that AI is listening in on a strategy session where the CEO proposes something and everyone says “Aye” without debate and discussion. AI could inform the room, “We might have a sunflower bias here,” which could trigger more conversation and remind the CEO that it’s in their own interest to encourage some devil’s advocacy.
We also often see confirmation bias, where people focus their analysis on proving the wisdom of what they already want to do, as opposed to looking for a fact-based reality. Just having AI perform a default analysis that doesn’t aim to satisfy the boss is useful, and the team can then try to understand why that is different than the management hypothesis, triggering a much richer debate.
In terms of social dynamics, agency problems can create conflicts of interest. Every business unit [BU] leader thinks that their BU should get the most resources and will deliver the most value, or at least they feel they should advocate for their business. AI provides a neutral way based on systematic data to manage those debates. It’s also useful for executives with decision authority, since we all know that short-term pressures and the need to make the quarterly and annual numbers lead people to make different decisions on the 31st of December than they do on January 1st or October 1st. Like the story of Ulysses and the sirens, you can use AI to remind you that you wanted something different three months earlier. The CEO still decides; AI can just provide that extra nudge.
Joanna Pachner: It’s like you have Spock next to you, who is dispassionate and purely analytical.
Yuval Atsmon: That is not a bad analogy—for Star Trek fans anyway.
Joanna Pachner: Do you have a favorite application of AI in strategy?
Yuval Atsmon: I have worked a lot on resource allocation, and one of the challenges, which we call the hockey stick phenomenon, is that executives are always overly optimistic about what will happen. They know that resource allocation will inevitably be defined by what you believe about the future, not necessarily by past performance. AI can provide an objective prediction of performance starting from a default momentum case: based on everything that happened in the past and some indicators about the future, what is the forecast of performance if we do nothing? This is before we say, “But I will hire these people and develop this new product and improve my marketing”— things that every executive thinks will help them overdeliver relative to the past. The neutral momentum case, which AI can calculate in a cold, Spock-like manner, can change the dynamics of the resource allocation discussion. It’s a form of predictive intelligence accessible today and while it’s not meant to be definitive, it provides a basis for better decisions.
Joanna Pachner: Do you see access to technology talent as one of the obstacles to the adoption of AI in strategy, especially at large companies?
Yuval Atsmon: I would make a distinction. If you mean machine-learning and data science talent or software engineers who build the digital tools, they are definitely not easy to get. However, companies can increasingly use platforms that provide access to AI tools and require less from individual companies. Also, this domain of strategy is exciting—it’s cutting-edge, so it’s probably easier to get technology talent for that than it might be for manufacturing work.
The bigger challenge, ironically, is finding strategists or people with business expertise to contribute to the effort. You will not solve strategy problems with AI without the involvement of people who understand the customer experience and what you are trying to achieve. Those who know best, like senior executives, don’t have time to be product managers for the AI team. An even bigger constraint is that, in some cases, you are asking people to get involved in an initiative that may make their jobs less important. There could be plenty of opportunities for incorporating AI into existing jobs, but it’s something companies need to reflect on. The best approach may be to create a digital factory where a different team tests and builds AI applications, with oversight from senior stakeholders.
The big challenge is finding strategists to contribute to the AI effort. You are asking people to get involved in an initiative that may make their jobs less important.
Joanna Pachner: Do you think this worry about job security and the potential that AI will automate strategy is realistic?
Yuval Atsmon: The question of whether AI will replace human judgment and put humanity out of its job is a big one that I would leave for other experts.
The pertinent question is shorter-term automation. Because of its complexity, strategy would be one of the later domains to be affected by automation, but we are seeing it in many other domains. However, the trend for more than two hundred years has been that automation creates new jobs, although ones requiring different skills. That doesn’t take away the fear some people have of a machine exposing their mistakes or doing their job better than they do it.
Joanna Pachner: We recently published an article about strategic courage in an age of volatility that talked about three types of edge business leaders need to develop. One of them is an edge in insights. Do you think AI has a role to play in furnishing a proprietary insight edge?
Yuval Atsmon: One of the challenges most strategists face is the overwhelming complexity of the world we operate in—the number of unknowns, the information overload. At one level, it may seem that AI will provide another layer of complexity. In reality, it can be a sharp knife that cuts through some of the clutter. The question to ask is, Can AI simplify my life by giving me sharper, more timely insights more easily?
Joanna Pachner: You have been working in strategy for a long time. What sparked your interest in exploring this intersection of strategy and new technology?
Yuval Atsmon: I have always been intrigued by things at the boundaries of what seems possible. Science fiction writer Arthur C. Clarke’s second law is that to discover the limits of the possible, you have to venture a little past them into the impossible, and I find that particularly alluring in this arena.
AI in strategy is in very nascent stages but could be very consequential for companies and for the profession. For a top executive, strategic decisions are the biggest way to influence the business, other than maybe building the top team, and it is amazing how little technology is leveraged in that process today. It’s conceivable that competitive advantage will increasingly rest in having executives who know how to apply AI well. In some domains, like investment, that is already happening, and the difference in returns can be staggering. I find helping companies be part of that evolution very exciting.
Explore a career with us
Related articles.

Strategic courage in an age of volatility
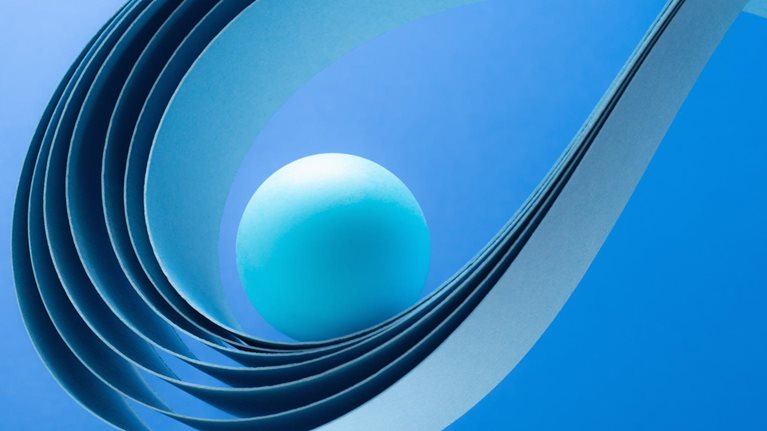
Bias Busters Collection
We've detected unusual activity from your computer network
To continue, please click the box below to let us know you're not a robot.
Why did this happen?
Please make sure your browser supports JavaScript and cookies and that you are not blocking them from loading. For more information you can review our Terms of Service and Cookie Policy .
For inquiries related to this message please contact our support team and provide the reference ID below.
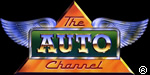
Asia-Pacific Electric Vehicle (EV) Charging Communication Unit Markets 2023-2024 & 2032 with Hyundai Mobis, LG Innotek, and Mitsubishi Electric Dominating - ResearchAndMarkets.com
DUBLIN--( BUSINESS WIRE )--The "Asia-Pacific Electric Vehicle (EV) Charging Communication Unit Market: Focus on Vehicle Type, Propulsion Type, Charging Type, Current Type, Component Type, System Type, and Country - Analysis and Forecast, 2023-2032" report has been added to ResearchAndMarkets.com's offering.
The Asia-Pacific EV charging communication unit market (excluding China) was valued at $8.0 million in 2023, which is expected to grow at a CAGR of 38.70% and reach $151.6 million by 2032.
The EV charging communication unit market has seen swift growth, spurred by rising electric vehicle adoption, demand for rapid charging solutions, and standardization efforts. The primary catalyst is the increasing uptake of electric vehicles, with governments and regulators promoting electric mobility through incentives, emissions targets, and infrastructure investments, driving robust demand for charging infrastructure and communication units.
Market Introduction
The electric vehicle (EV) charging communication unit market in Asia-Pacific (APAC) is rapidly expanding due to a number of important drivers. With the increased acceptance of electric vehicles throughout the region, there is a greater need for efficient and dependable charging infrastructure, including communication units. EV charging communication modules facilitate communication between electric vehicles and charging stations, resulting in seamless and secure charging transactions. These modules provide services like authentication, billing, and data transmission, which improve the overall charging experience for EV owners.
The APAC area, recognized for its strong automotive sector, technical innovation, and favorable regulatory climate for electric mobility, is making considerable investments in charging infrastructure development. Governments and regulatory organizations in APAC countries are enacting laws that promote electric vehicle adoption, including incentives, subsidies, and regulations mandating emissions reductions. Furthermore, the increased emphasis on environmental sustainability and the need to minimize greenhouse gas emissions is driving the shift to electric transportation in APAC. As a result, the number of electric vehicles on the road is increasing exponentially, creating a rise in demand for charging infrastructure, such as communication units.
Overall, the APAC electric vehicle charging communication unit market offers lucrative potential for industry participants to capitalize on the region's growing need for efficient and dependable charging solutions, hence facilitating the shift to a more sustainable transportation ecosystem.
How can this report add value to end users?
Product/Innovation Strategy: The product segment helps the readers understand the different types of EV charging communication units. Also, the study provides the readers with a detailed understanding of the APAC EV charging communication unit market based on application and product.
Growth/Marketing Strategy: To improve the capabilities of their product offerings, players in the APAC EV charging communication unit market are developing unique products. The readers will be able to comprehend the revenue-generating tactics used by players in the APAC EV charging communication unit market by looking at the growth/marketing strategies. Other market participants' tactics, such as go-to-market plans, will also assist readers in making strategic judgments.
Competitive Strategy: Players in the APAC EV charging communication unit market analyzed and profiled in the study include vehicle manufacturers that capture the maximum share of the market. Moreover, a detailed competitive benchmarking of the players operating in the APAC EV charging communication unit market has been done to help the readers understand how players compete against each other, presenting a clear market landscape.
Additionally, comprehensive competitive strategies such as partnerships, agreements, collaborations, and mergers and acquisitions are expected to aid the readers in understanding the untapped revenue pockets in the market.
Key Attributes:
Business Dynamics
Trends: Current and Future
- Increasing Government Influence on the e-Mobility Industry
- Electrification of Fleets
- Increasing Investment in Charging Infrastructure
Business Drivers
- Increasing Adoption of Electric Vehicles (EVs)
- Need for Fast and Reliable Charging Solutions
- Standardization and Interoperability
Business Challenges
- Limited Infrastructure Availability and Geographic Distribution
- Up-Front Vehicle and Charging Infrastructure Costs
Business Strategies
- Product Development
- Market Development
- Corporate Strategies
- Mergers and Acquisitions
- Partnerships, Collaborations, and Joint Ventures
Business Opportunities
- Adequate Rollout of Public EV Charging Stations
- Scaling EV Charging Infrastructure with a Focus on Interoperability
- Future Potential of 5G and Artificial Intelligence
Ecosystem/Ongoing Programs
- Consortiums, Associations, and Regulatory Bodies for Electric Vehicles
- Government Programs and Initiatives
- Programs by Research Institutions and Universities
Supply Chain Network
Technology Roadmap
EV Charging Ecosystem
Compatibility of Hardware with Different Software
- Protocols and Standards
Business Model Analysis
Competitive Benchmarking & Company Profiles
- Company Overview
- Role in the Electric Vehicle Charging Communication Unit
- Product Portfolio
- R&D Analysis
- Analyst View
Companies Featured
- Hyundai Mobis
- Mitsubishi Electric Corporation
- Neusoft Corporation
Market Segmentation:
Vehicle Type
- Passenger Vehicle
- Commercial Vehicle
Propulsion Type
- Battery Electric Vehicle (BEV)
- Plug-In Hybrid Electric Vehicle (PHEV)
Charging Type
- Wired (Plug-In)
- Wireless (Inductive Charging)
Current Type
- Alternating Current (AC)
- Direct Current (DC)
Component Type
System Type
- Electric Vehicle Communication Controller (EVCC)
- Supply Equipment Communication Controller (SECC)
For more information about this report visit https://www.researchandmarkets.com/r/ttesck
About ResearchAndMarkets.com
ResearchAndMarkets.com is the world's leading source for international market research reports and market data. We provide you with the latest data on international and regional markets, key industries, the top companies, new products and the latest trends.
ResearchAndMarkets.com Laura Wood, Senior Press Manager [email protected] For E.S.T Office Hours Call 1-917-300-0470 For U.S./ CAN Toll Free Call 1-800-526-8630 For GMT Office Hours Call +353-1-416-8900
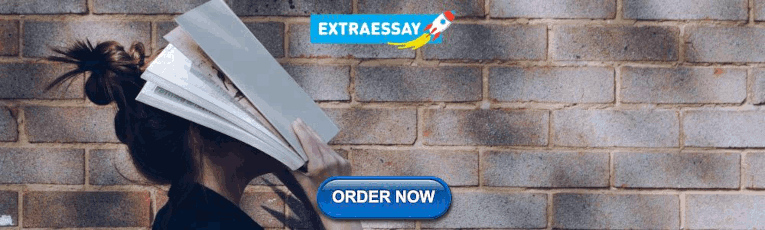
IMAGES
VIDEO
COMMENTS
Topics could include any aspect of the lived experience. For studies where the units of analysis are small-groups or individuals, the researcher might want a methodology that allows for studying interactions, and data collection methods that include direct contact with individuals through observations and/or interviews, diaries or creative methods.
A unit of analysis is the thing you want to discuss after your research, probably what you would regard to be the primary emphasis of your research. The researcher plans to comment on the primary topic or object in the research as a unit of analysis. The research question plays a significant role in determining it.
conducting communication research is an enormous. field of study that requires very skilled and knowl-. edgeable researchers who are aware of its many. areas, approaches, designs, methods, tools ...
Summary. Communication research is important because it focuses on a common goal—to enhance our interactions with others. In this chapter we highlighted how research is done and the basic steps that guide most research projects—identify the topic, write a research question, define key terms, select a methodology, establish a sample, gather and analyze the data, and finally, interpret and ...
A unit of analysis is an object of study within a research project. It is the smallest unit a researcher can use to identify and describe a phenomenon—the 'what' or 'who' the researcher wants to study. For example, suppose a consultancy firm is hired to train the sales team in a solar company that is struggling to meet its targets.
In his 1952 text on the subject of content analysis, Bernard Berelson traced the origins of the method to communication research and then listed what he called six distinguishing features of the approach. As one might expect, the six defining features reflect the concerns of social science as taught in the 1950s, an age in which the calls for an "objective," "systematic," and ...
If a weak unit of analysis is chosen, one of two outcomes may result: 1) If the unit chosen is too precise (i.e., at too much of a micro-level than what is actually needed), the researcher will set in motion an analysis that may miss important contextual information and may require more time and cost than if a broader unit of analysis had been ...
The unit of analysis in research refers to the level at which data is collected and analyzed. It is essential for researchers to understand the different types of units of analysis, as well as their significance in shaping the research process and outcomes. Definition and Importance. With resonio, the unit of analysis you choose lays the ...
Content analysis is a research method used to identify patterns in recorded communication. To conduct content analysis, you systematically collect data from a set of texts, which can be written, oral, or visual: Books, newspapers and magazines. Speeches and interviews. Web content and social media posts. Photographs and films.
Without a common framework for units of analysis, definitions of what is being studied on the Internet can be clouded by a poor definition of the research setting, making cross-study (or even intrastudy) comparisons difficult. This article proposes an approach to defining units of analysis for Internet communication research.
This Guide is presented by the IPR Measurement Commission. The Communicator's Guide to Research, Analysis, and Evaluation was created to help public relations leaders understand how they can apply data, research, and analytics to uncover insights that inform strategic decision making, improve communication performance, and deliver meaningful ...
Unit of Analysis. One of the most important ideas in a research project is the unit of analysis. The unit of analysis is the major entity that you are analyzing in your study. For instance, any of the following could be a unit of analysis in a study: individuals; groups; artifacts (books, photos, newspapers) geographical units (town, census ...
The unit of analysis is named as such because the unit type is determined based on the actual data analysis that you perform in your project or study. For example, if your research is based around data on exam grades for students at two different universities, then the unit of analysis is the data for the individual student due to each student ...
The unit of analysis is a way to understand and study a phenomenon. There are four main types of unit of analysis: individuals, groups, artifacts (books, photos, newspapers), and geographical units (towns, census tracts, states). Individuals are the smallest level of analysis. For example, an individual may be a person or an animal.
Specifying the unit of analysis. The unit of analysis may include the person, a program, an organisation, a class, community, a state, a country, an interview, or a diary written by the researchers (Graneheim and Lundman, 2004). The transcriptions of interviews are usually considered units of analysis when data are collected using interviews.
The importance of communication research, analysis, and evaluation continues to grow due to the pace of change in the marketplace and the corresponding need to make smarter and better decisions at the speed of business. The ability for an organization to successfully transform during this time of dynamic change
Unit of Analysis. The unit of analysis is the entity that you're analyzing. It's called this because it's your analysis (what you want to examine) that determines what this unit is, rather than the data itself. For instance, let's say that you have a dataset with 40 students, divided between two classrooms of 20 students each, and a test score ...
research methods. what are the major purposes of communication research? Click the card to flip 👆. 1. study the processes of message production, transmission, and making making. 2. systematically examine the content or form of communicative messages. 3. communication researchers are interested in studying functions and effects of messages.
the method communication researchers use to describe and interpret the characteristics of a recorded or visual message. ... Unit of analysis is the appropriate message unit to code. There are five types of units including: a. Physical units: space and time devoted to content b. Meaning units, which the remaining four types reside within ...
Study with Quizlet and memorize flashcards containing terms like Which Maslow's hierarchy of needs relates to keeping a roof over one's head? a. Self-actualization b. Self-esteem c. Love/belonging d. Safety e. Physiological, Which purpose for communicating is reflected in the fact that communication can have positive effects on one's health? a. Physical b. Instrumental c. Relational d ...
Without a common framework for units of analysis, definitions of what is being studied on the Internet can be clouded by a poor definition of the research setting, making cross-study (or even intrastudy) comparisons difficult. This article proposes an approach to defining units of analysis for Internet communication research.
Our critical thematic meta-analysis is driven by a quantifying tool - we turn our research lens to the body of literature written by the collective of scholars in the discipline who refer to or rely on decolonization in their research to reveal the way in which the term is connoted over the last decade.
"Taking a deep dive into our research approach in the PICU empowers us to enhance communication and trust between research teams and families, which simultaneously elevates potential new strategies for research engagement. Our goal is always to change the trajectory of children's lives and maximize their ability to flourish."
Yuval Atsmon: When people talk about artificial intelligence, they include everything to do with analytics, automation, and data analysis. Marvin Minsky, the pioneer of artificial intelligence research in the 1960s, talked about AI as a "suitcase word"—a term into which you can stuff whatever you want—and that still seems to be the case.
Study with Quizlet and memorize flashcards containing terms like What is a communication researcher's unit of analysis? (Quiz 1), Which of the following is not a guidance for using communication and respect differences between people? (Quiz 1), Which Malows's hierarchy of needs relates tp keeping a roof over one's head? (Quiz 1) and more.
OpenAI has effectively dissolved a team focused on ensuring the safety of possible future ultra-capable artificial intelligence systems, following the departure of the group's two leaders ...
The Asia-Pacific EV charging communication unit market (excluding China) was valued at $8.0 million in 2023, which is expected to grow at a CAGR of 38.70% and reach $151.6 million by 2032.