Home Blog Design Understanding Data Presentations (Guide + Examples)
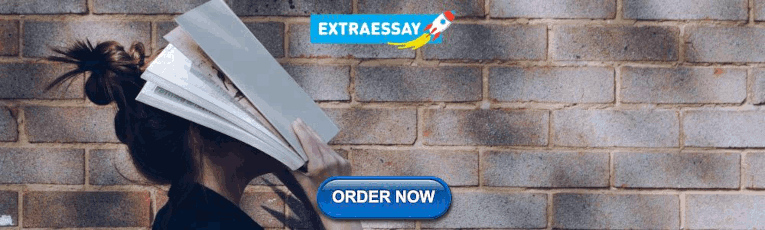
Understanding Data Presentations (Guide + Examples)
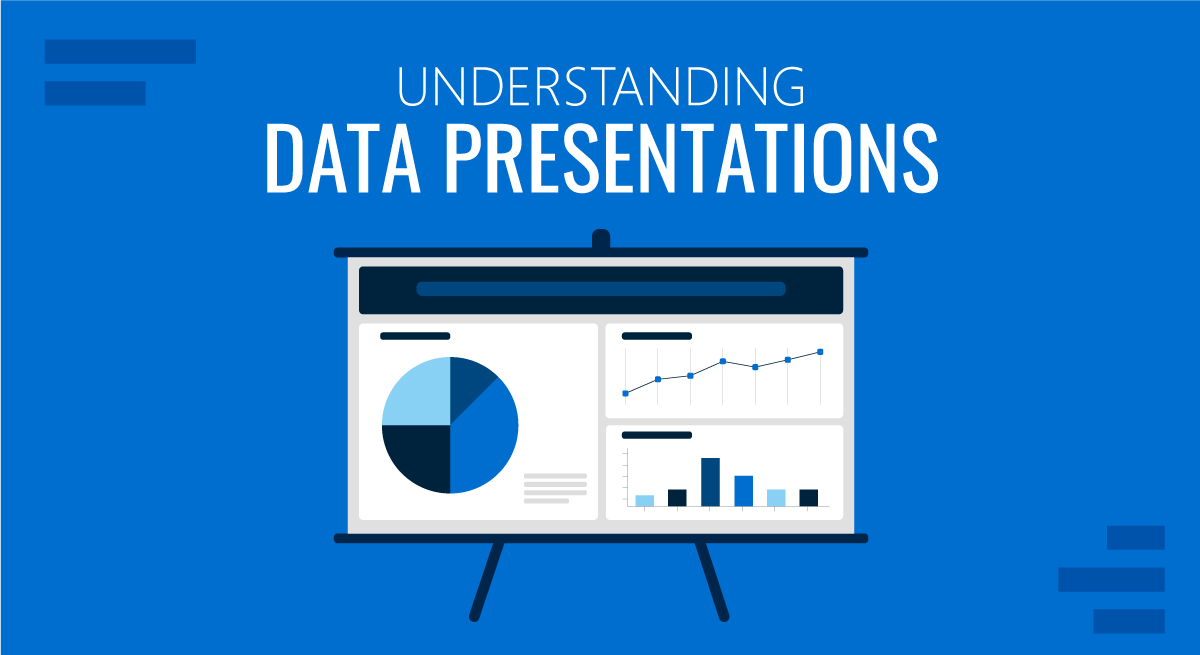
In this age of overwhelming information, the skill to effectively convey data has become extremely valuable. Initiating a discussion on data presentation types involves thoughtful consideration of the nature of your data and the message you aim to convey. Different types of visualizations serve distinct purposes. Whether you’re dealing with how to develop a report or simply trying to communicate complex information, how you present data influences how well your audience understands and engages with it. This extensive guide leads you through the different ways of data presentation.
Table of Contents
What is a Data Presentation?
What should a data presentation include, line graphs, treemap chart, scatter plot, how to choose a data presentation type, recommended data presentation templates, common mistakes done in data presentation.
A data presentation is a slide deck that aims to disclose quantitative information to an audience through the use of visual formats and narrative techniques derived from data analysis, making complex data understandable and actionable. This process requires a series of tools, such as charts, graphs, tables, infographics, dashboards, and so on, supported by concise textual explanations to improve understanding and boost retention rate.
Data presentations require us to cull data in a format that allows the presenter to highlight trends, patterns, and insights so that the audience can act upon the shared information. In a few words, the goal of data presentations is to enable viewers to grasp complicated concepts or trends quickly, facilitating informed decision-making or deeper analysis.
Data presentations go beyond the mere usage of graphical elements. Seasoned presenters encompass visuals with the art of data storytelling , so the speech skillfully connects the points through a narrative that resonates with the audience. Depending on the purpose – inspire, persuade, inform, support decision-making processes, etc. – is the data presentation format that is better suited to help us in this journey.
To nail your upcoming data presentation, ensure to count with the following elements:
- Clear Objectives: Understand the intent of your presentation before selecting the graphical layout and metaphors to make content easier to grasp.
- Engaging introduction: Use a powerful hook from the get-go. For instance, you can ask a big question or present a problem that your data will answer. Take a look at our guide on how to start a presentation for tips & insights.
- Structured Narrative: Your data presentation must tell a coherent story. This means a beginning where you present the context, a middle section in which you present the data, and an ending that uses a call-to-action. Check our guide on presentation structure for further information.
- Visual Elements: These are the charts, graphs, and other elements of visual communication we ought to use to present data. This article will cover one by one the different types of data representation methods we can use, and provide further guidance on choosing between them.
- Insights and Analysis: This is not just showcasing a graph and letting people get an idea about it. A proper data presentation includes the interpretation of that data, the reason why it’s included, and why it matters to your research.
- Conclusion & CTA: Ending your presentation with a call to action is necessary. Whether you intend to wow your audience into acquiring your services, inspire them to change the world, or whatever the purpose of your presentation, there must be a stage in which you convey all that you shared and show the path to staying in touch. Plan ahead whether you want to use a thank-you slide, a video presentation, or which method is apt and tailored to the kind of presentation you deliver.
- Q&A Session: After your speech is concluded, allocate 3-5 minutes for the audience to raise any questions about the information you disclosed. This is an extra chance to establish your authority on the topic. Check our guide on questions and answer sessions in presentations here.
Bar charts are a graphical representation of data using rectangular bars to show quantities or frequencies in an established category. They make it easy for readers to spot patterns or trends. Bar charts can be horizontal or vertical, although the vertical format is commonly known as a column chart. They display categorical, discrete, or continuous variables grouped in class intervals [1] . They include an axis and a set of labeled bars horizontally or vertically. These bars represent the frequencies of variable values or the values themselves. Numbers on the y-axis of a vertical bar chart or the x-axis of a horizontal bar chart are called the scale.
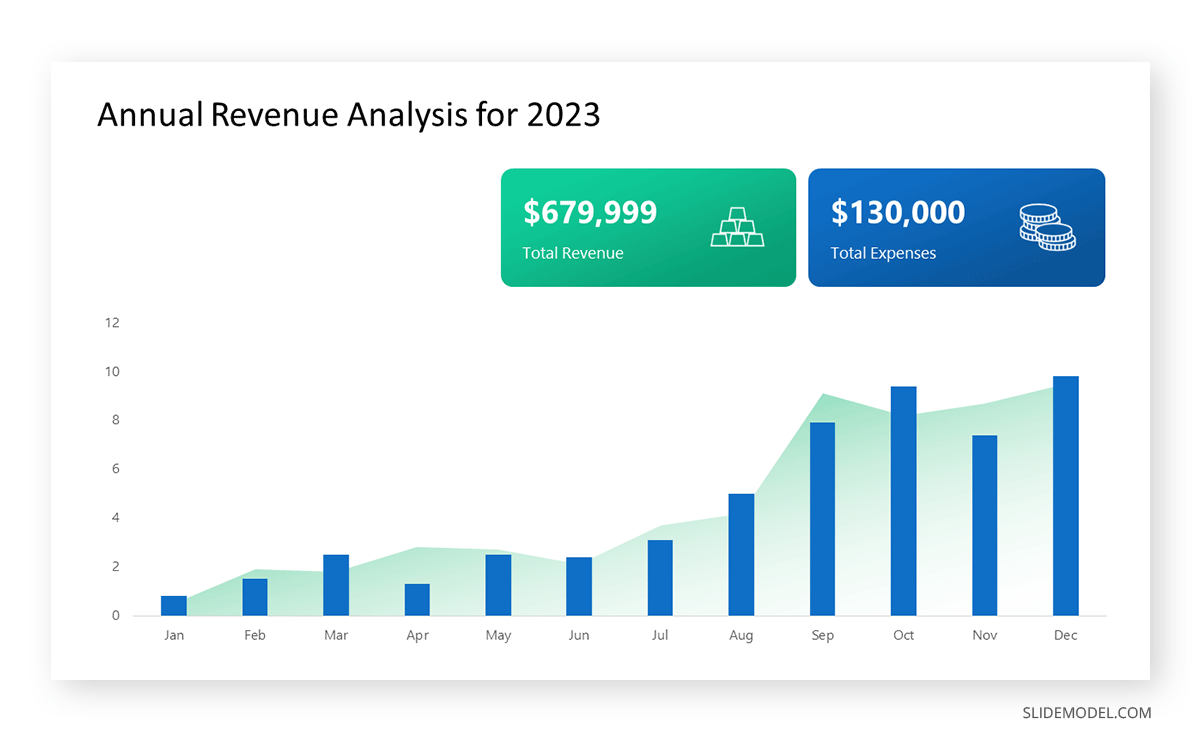
Real-Life Application of Bar Charts
Let’s say a sales manager is presenting sales to their audience. Using a bar chart, he follows these steps.
Step 1: Selecting Data
The first step is to identify the specific data you will present to your audience.
The sales manager has highlighted these products for the presentation.
- Product A: Men’s Shoes
- Product B: Women’s Apparel
- Product C: Electronics
- Product D: Home Decor
Step 2: Choosing Orientation
Opt for a vertical layout for simplicity. Vertical bar charts help compare different categories in case there are not too many categories [1] . They can also help show different trends. A vertical bar chart is used where each bar represents one of the four chosen products. After plotting the data, it is seen that the height of each bar directly represents the sales performance of the respective product.
It is visible that the tallest bar (Electronics – Product C) is showing the highest sales. However, the shorter bars (Women’s Apparel – Product B and Home Decor – Product D) need attention. It indicates areas that require further analysis or strategies for improvement.
Step 3: Colorful Insights
Different colors are used to differentiate each product. It is essential to show a color-coded chart where the audience can distinguish between products.
- Men’s Shoes (Product A): Yellow
- Women’s Apparel (Product B): Orange
- Electronics (Product C): Violet
- Home Decor (Product D): Blue
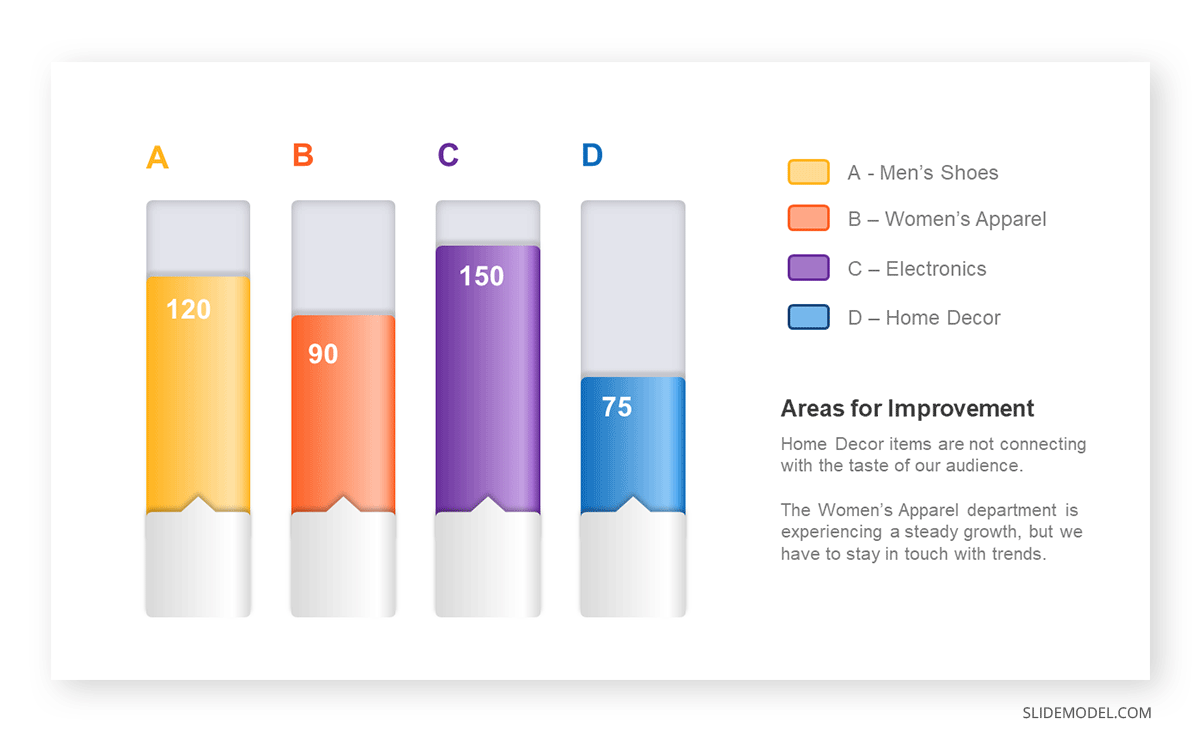
Bar charts are straightforward and easily understandable for presenting data. They are versatile when comparing products or any categorical data [2] . Bar charts adapt seamlessly to retail scenarios. Despite that, bar charts have a few shortcomings. They cannot illustrate data trends over time. Besides, overloading the chart with numerous products can lead to visual clutter, diminishing its effectiveness.
For more information, check our collection of bar chart templates for PowerPoint .
Line graphs help illustrate data trends, progressions, or fluctuations by connecting a series of data points called ‘markers’ with straight line segments. This provides a straightforward representation of how values change [5] . Their versatility makes them invaluable for scenarios requiring a visual understanding of continuous data. In addition, line graphs are also useful for comparing multiple datasets over the same timeline. Using multiple line graphs allows us to compare more than one data set. They simplify complex information so the audience can quickly grasp the ups and downs of values. From tracking stock prices to analyzing experimental results, you can use line graphs to show how data changes over a continuous timeline. They show trends with simplicity and clarity.
Real-life Application of Line Graphs
To understand line graphs thoroughly, we will use a real case. Imagine you’re a financial analyst presenting a tech company’s monthly sales for a licensed product over the past year. Investors want insights into sales behavior by month, how market trends may have influenced sales performance and reception to the new pricing strategy. To present data via a line graph, you will complete these steps.
First, you need to gather the data. In this case, your data will be the sales numbers. For example:
- January: $45,000
- February: $55,000
- March: $45,000
- April: $60,000
- May: $ 70,000
- June: $65,000
- July: $62,000
- August: $68,000
- September: $81,000
- October: $76,000
- November: $87,000
- December: $91,000
After choosing the data, the next step is to select the orientation. Like bar charts, you can use vertical or horizontal line graphs. However, we want to keep this simple, so we will keep the timeline (x-axis) horizontal while the sales numbers (y-axis) vertical.
Step 3: Connecting Trends
After adding the data to your preferred software, you will plot a line graph. In the graph, each month’s sales are represented by data points connected by a line.
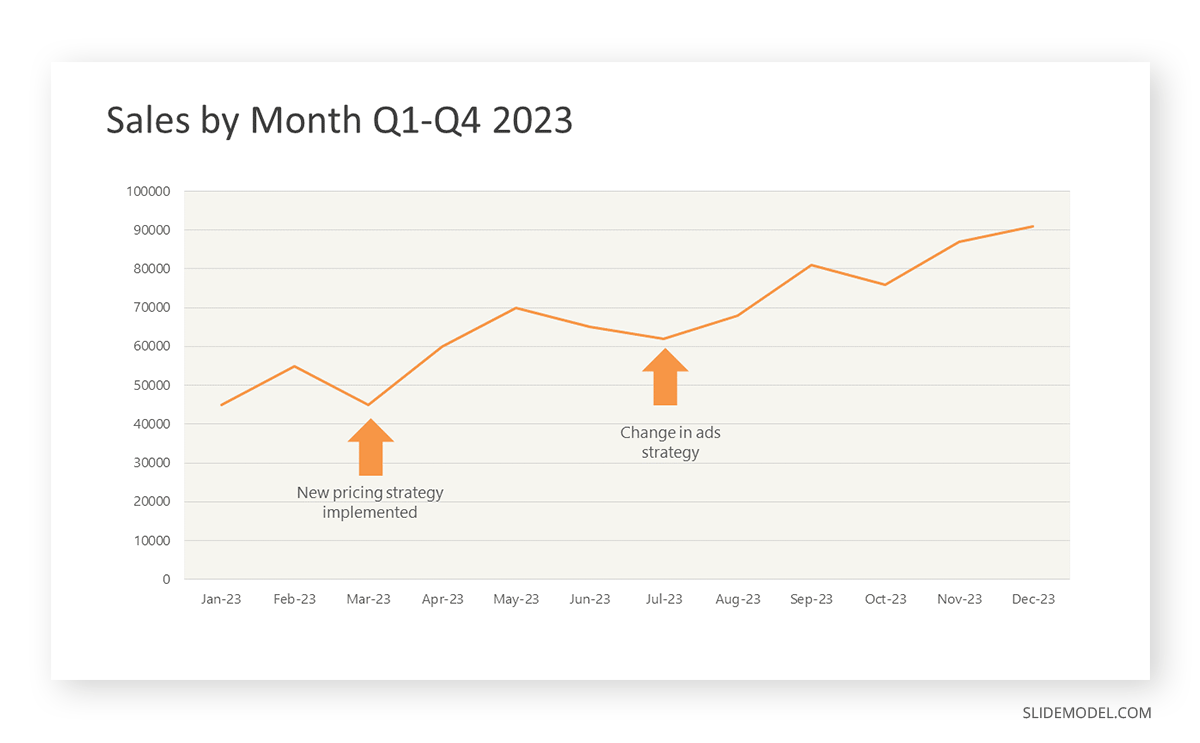
Step 4: Adding Clarity with Color
If there are multiple lines, you can also add colors to highlight each one, making it easier to follow.
Line graphs excel at visually presenting trends over time. These presentation aids identify patterns, like upward or downward trends. However, too many data points can clutter the graph, making it harder to interpret. Line graphs work best with continuous data but are not suitable for categories.
For more information, check our collection of line chart templates for PowerPoint and our article about how to make a presentation graph .
A data dashboard is a visual tool for analyzing information. Different graphs, charts, and tables are consolidated in a layout to showcase the information required to achieve one or more objectives. Dashboards help quickly see Key Performance Indicators (KPIs). You don’t make new visuals in the dashboard; instead, you use it to display visuals you’ve already made in worksheets [3] .
Keeping the number of visuals on a dashboard to three or four is recommended. Adding too many can make it hard to see the main points [4]. Dashboards can be used for business analytics to analyze sales, revenue, and marketing metrics at a time. They are also used in the manufacturing industry, as they allow users to grasp the entire production scenario at the moment while tracking the core KPIs for each line.
Real-Life Application of a Dashboard
Consider a project manager presenting a software development project’s progress to a tech company’s leadership team. He follows the following steps.
Step 1: Defining Key Metrics
To effectively communicate the project’s status, identify key metrics such as completion status, budget, and bug resolution rates. Then, choose measurable metrics aligned with project objectives.
Step 2: Choosing Visualization Widgets
After finalizing the data, presentation aids that align with each metric are selected. For this project, the project manager chooses a progress bar for the completion status and uses bar charts for budget allocation. Likewise, he implements line charts for bug resolution rates.
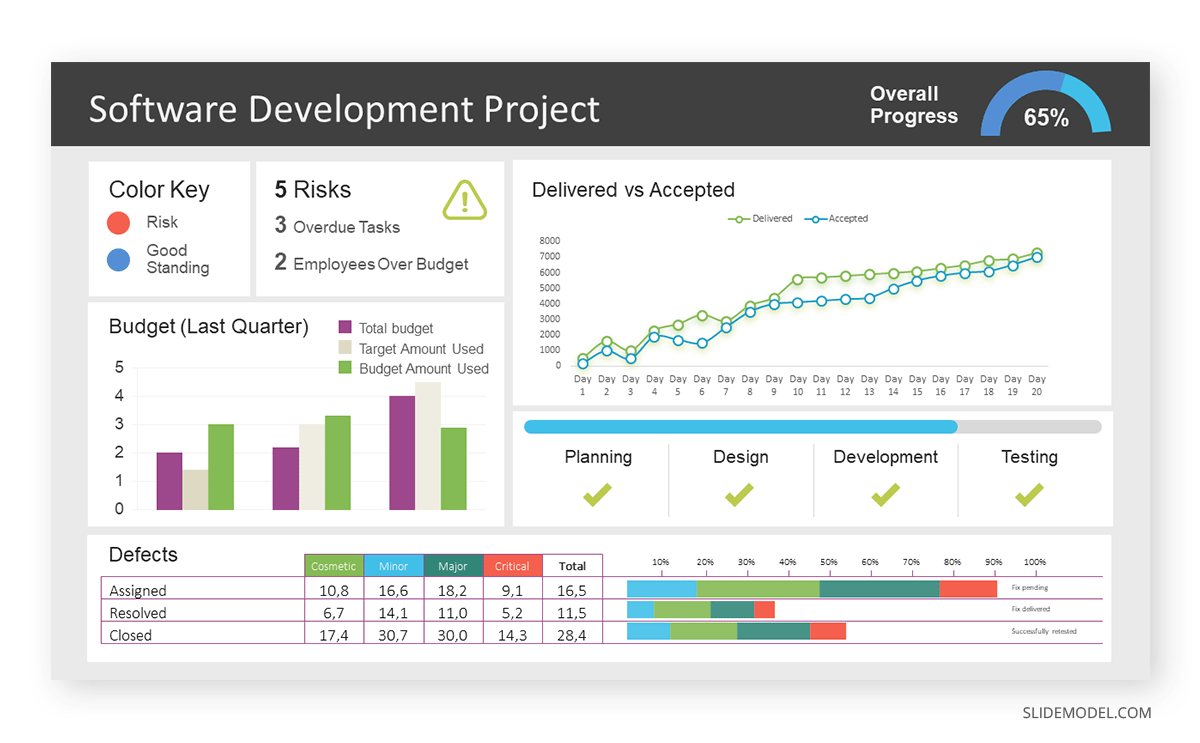
Step 3: Dashboard Layout
Key metrics are prominently placed in the dashboard for easy visibility, and the manager ensures that it appears clean and organized.
Dashboards provide a comprehensive view of key project metrics. Users can interact with data, customize views, and drill down for detailed analysis. However, creating an effective dashboard requires careful planning to avoid clutter. Besides, dashboards rely on the availability and accuracy of underlying data sources.
For more information, check our article on how to design a dashboard presentation , and discover our collection of dashboard PowerPoint templates .
Treemap charts represent hierarchical data structured in a series of nested rectangles [6] . As each branch of the ‘tree’ is given a rectangle, smaller tiles can be seen representing sub-branches, meaning elements on a lower hierarchical level than the parent rectangle. Each one of those rectangular nodes is built by representing an area proportional to the specified data dimension.
Treemaps are useful for visualizing large datasets in compact space. It is easy to identify patterns, such as which categories are dominant. Common applications of the treemap chart are seen in the IT industry, such as resource allocation, disk space management, website analytics, etc. Also, they can be used in multiple industries like healthcare data analysis, market share across different product categories, or even in finance to visualize portfolios.
Real-Life Application of a Treemap Chart
Let’s consider a financial scenario where a financial team wants to represent the budget allocation of a company. There is a hierarchy in the process, so it is helpful to use a treemap chart. In the chart, the top-level rectangle could represent the total budget, and it would be subdivided into smaller rectangles, each denoting a specific department. Further subdivisions within these smaller rectangles might represent individual projects or cost categories.
Step 1: Define Your Data Hierarchy
While presenting data on the budget allocation, start by outlining the hierarchical structure. The sequence will be like the overall budget at the top, followed by departments, projects within each department, and finally, individual cost categories for each project.
- Top-level rectangle: Total Budget
- Second-level rectangles: Departments (Engineering, Marketing, Sales)
- Third-level rectangles: Projects within each department
- Fourth-level rectangles: Cost categories for each project (Personnel, Marketing Expenses, Equipment)
Step 2: Choose a Suitable Tool
It’s time to select a data visualization tool supporting Treemaps. Popular choices include Tableau, Microsoft Power BI, PowerPoint, or even coding with libraries like D3.js. It is vital to ensure that the chosen tool provides customization options for colors, labels, and hierarchical structures.
Here, the team uses PowerPoint for this guide because of its user-friendly interface and robust Treemap capabilities.
Step 3: Make a Treemap Chart with PowerPoint
After opening the PowerPoint presentation, they chose “SmartArt” to form the chart. The SmartArt Graphic window has a “Hierarchy” category on the left. Here, you will see multiple options. You can choose any layout that resembles a Treemap. The “Table Hierarchy” or “Organization Chart” options can be adapted. The team selects the Table Hierarchy as it looks close to a Treemap.
Step 5: Input Your Data
After that, a new window will open with a basic structure. They add the data one by one by clicking on the text boxes. They start with the top-level rectangle, representing the total budget.
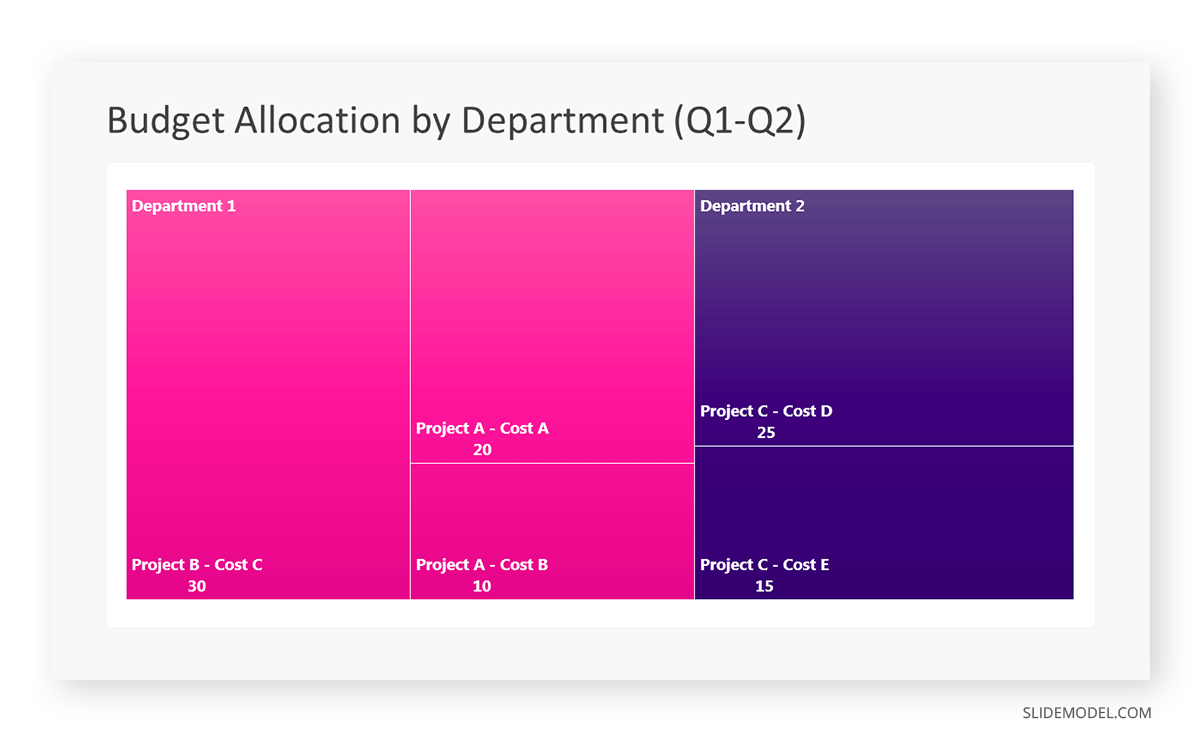
Step 6: Customize the Treemap
By clicking on each shape, they customize its color, size, and label. At the same time, they can adjust the font size, style, and color of labels by using the options in the “Format” tab in PowerPoint. Using different colors for each level enhances the visual difference.
Treemaps excel at illustrating hierarchical structures. These charts make it easy to understand relationships and dependencies. They efficiently use space, compactly displaying a large amount of data, reducing the need for excessive scrolling or navigation. Additionally, using colors enhances the understanding of data by representing different variables or categories.
In some cases, treemaps might become complex, especially with deep hierarchies. It becomes challenging for some users to interpret the chart. At the same time, displaying detailed information within each rectangle might be constrained by space. It potentially limits the amount of data that can be shown clearly. Without proper labeling and color coding, there’s a risk of misinterpretation.
A heatmap is a data visualization tool that uses color coding to represent values across a two-dimensional surface. In these, colors replace numbers to indicate the magnitude of each cell. This color-shaded matrix display is valuable for summarizing and understanding data sets with a glance [7] . The intensity of the color corresponds to the value it represents, making it easy to identify patterns, trends, and variations in the data.
As a tool, heatmaps help businesses analyze website interactions, revealing user behavior patterns and preferences to enhance overall user experience. In addition, companies use heatmaps to assess content engagement, identifying popular sections and areas of improvement for more effective communication. They excel at highlighting patterns and trends in large datasets, making it easy to identify areas of interest.
We can implement heatmaps to express multiple data types, such as numerical values, percentages, or even categorical data. Heatmaps help us easily spot areas with lots of activity, making them helpful in figuring out clusters [8] . When making these maps, it is important to pick colors carefully. The colors need to show the differences between groups or levels of something. And it is good to use colors that people with colorblindness can easily see.
Check our detailed guide on how to create a heatmap here. Also discover our collection of heatmap PowerPoint templates .
Pie charts are circular statistical graphics divided into slices to illustrate numerical proportions. Each slice represents a proportionate part of the whole, making it easy to visualize the contribution of each component to the total.
The size of the pie charts is influenced by the value of data points within each pie. The total of all data points in a pie determines its size. The pie with the highest data points appears as the largest, whereas the others are proportionally smaller. However, you can present all pies of the same size if proportional representation is not required [9] . Sometimes, pie charts are difficult to read, or additional information is required. A variation of this tool can be used instead, known as the donut chart , which has the same structure but a blank center, creating a ring shape. Presenters can add extra information, and the ring shape helps to declutter the graph.
Pie charts are used in business to show percentage distribution, compare relative sizes of categories, or present straightforward data sets where visualizing ratios is essential.
Real-Life Application of Pie Charts
Consider a scenario where you want to represent the distribution of the data. Each slice of the pie chart would represent a different category, and the size of each slice would indicate the percentage of the total portion allocated to that category.
Step 1: Define Your Data Structure
Imagine you are presenting the distribution of a project budget among different expense categories.
- Column A: Expense Categories (Personnel, Equipment, Marketing, Miscellaneous)
- Column B: Budget Amounts ($40,000, $30,000, $20,000, $10,000) Column B represents the values of your categories in Column A.
Step 2: Insert a Pie Chart
Using any of the accessible tools, you can create a pie chart. The most convenient tools for forming a pie chart in a presentation are presentation tools such as PowerPoint or Google Slides. You will notice that the pie chart assigns each expense category a percentage of the total budget by dividing it by the total budget.
For instance:
- Personnel: $40,000 / ($40,000 + $30,000 + $20,000 + $10,000) = 40%
- Equipment: $30,000 / ($40,000 + $30,000 + $20,000 + $10,000) = 30%
- Marketing: $20,000 / ($40,000 + $30,000 + $20,000 + $10,000) = 20%
- Miscellaneous: $10,000 / ($40,000 + $30,000 + $20,000 + $10,000) = 10%
You can make a chart out of this or just pull out the pie chart from the data.
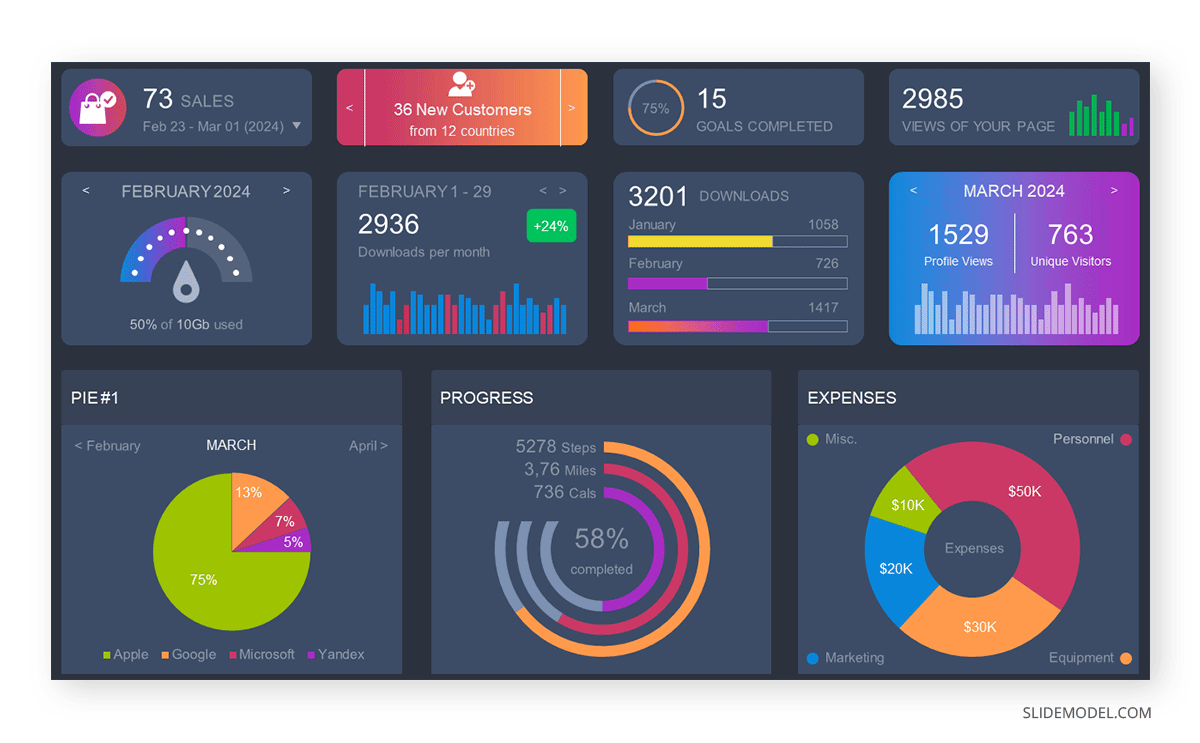
3D pie charts and 3D donut charts are quite popular among the audience. They stand out as visual elements in any presentation slide, so let’s take a look at how our pie chart example would look in 3D pie chart format.
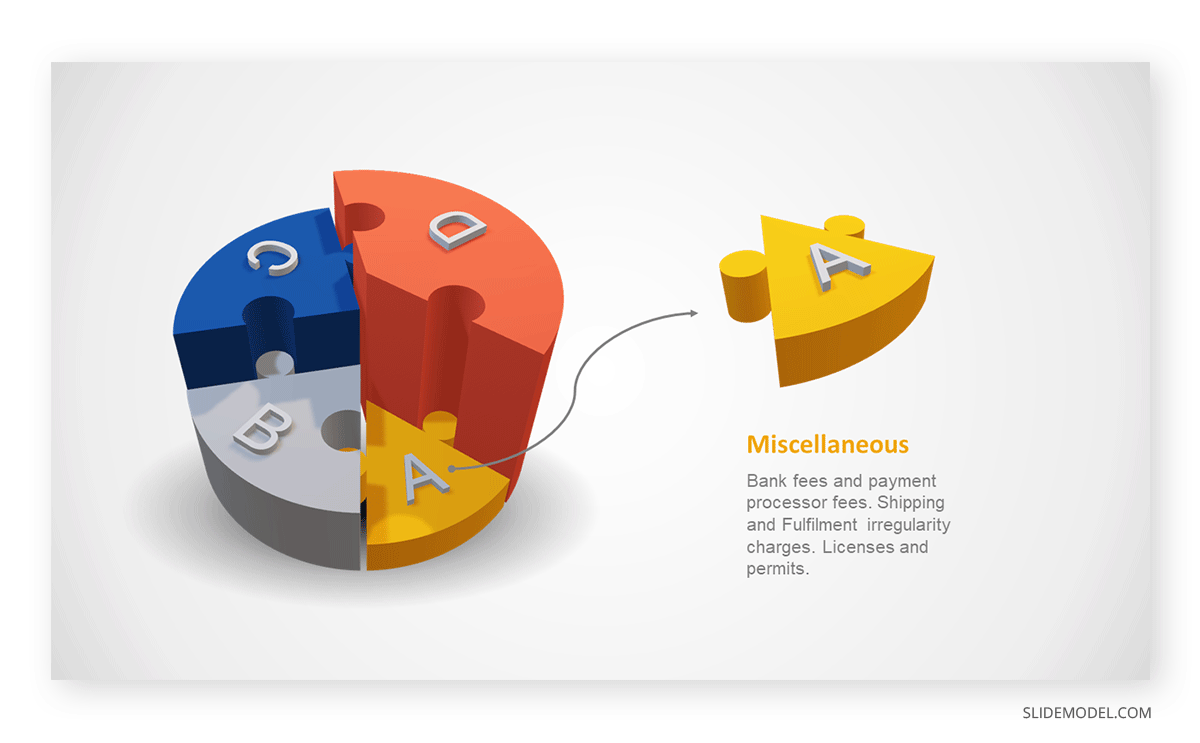
Step 03: Results Interpretation
The pie chart visually illustrates the distribution of the project budget among different expense categories. Personnel constitutes the largest portion at 40%, followed by equipment at 30%, marketing at 20%, and miscellaneous at 10%. This breakdown provides a clear overview of where the project funds are allocated, which helps in informed decision-making and resource management. It is evident that personnel are a significant investment, emphasizing their importance in the overall project budget.
Pie charts provide a straightforward way to represent proportions and percentages. They are easy to understand, even for individuals with limited data analysis experience. These charts work well for small datasets with a limited number of categories.
However, a pie chart can become cluttered and less effective in situations with many categories. Accurate interpretation may be challenging, especially when dealing with slight differences in slice sizes. In addition, these charts are static and do not effectively convey trends over time.
For more information, check our collection of pie chart templates for PowerPoint .
Histograms present the distribution of numerical variables. Unlike a bar chart that records each unique response separately, histograms organize numeric responses into bins and show the frequency of reactions within each bin [10] . The x-axis of a histogram shows the range of values for a numeric variable. At the same time, the y-axis indicates the relative frequencies (percentage of the total counts) for that range of values.
Whenever you want to understand the distribution of your data, check which values are more common, or identify outliers, histograms are your go-to. Think of them as a spotlight on the story your data is telling. A histogram can provide a quick and insightful overview if you’re curious about exam scores, sales figures, or any numerical data distribution.
Real-Life Application of a Histogram
In the histogram data analysis presentation example, imagine an instructor analyzing a class’s grades to identify the most common score range. A histogram could effectively display the distribution. It will show whether most students scored in the average range or if there are significant outliers.
Step 1: Gather Data
He begins by gathering the data. The scores of each student in class are gathered to analyze exam scores.
Names | Score |
---|---|
Alice | 78 |
Bob | 85 |
Clara | 92 |
David | 65 |
Emma | 72 |
Frank | 88 |
Grace | 76 |
Henry | 95 |
Isabel | 81 |
Jack | 70 |
Kate | 60 |
Liam | 89 |
Mia | 75 |
Noah | 84 |
Olivia | 92 |
After arranging the scores in ascending order, bin ranges are set.
Step 2: Define Bins
Bins are like categories that group similar values. Think of them as buckets that organize your data. The presenter decides how wide each bin should be based on the range of the values. For instance, the instructor sets the bin ranges based on score intervals: 60-69, 70-79, 80-89, and 90-100.
Step 3: Count Frequency
Now, he counts how many data points fall into each bin. This step is crucial because it tells you how often specific ranges of values occur. The result is the frequency distribution, showing the occurrences of each group.
Here, the instructor counts the number of students in each category.
- 60-69: 1 student (Kate)
- 70-79: 4 students (David, Emma, Grace, Jack)
- 80-89: 7 students (Alice, Bob, Frank, Isabel, Liam, Mia, Noah)
- 90-100: 3 students (Clara, Henry, Olivia)
Step 4: Create the Histogram
It’s time to turn the data into a visual representation. Draw a bar for each bin on a graph. The width of the bar should correspond to the range of the bin, and the height should correspond to the frequency. To make your histogram understandable, label the X and Y axes.
In this case, the X-axis should represent the bins (e.g., test score ranges), and the Y-axis represents the frequency.
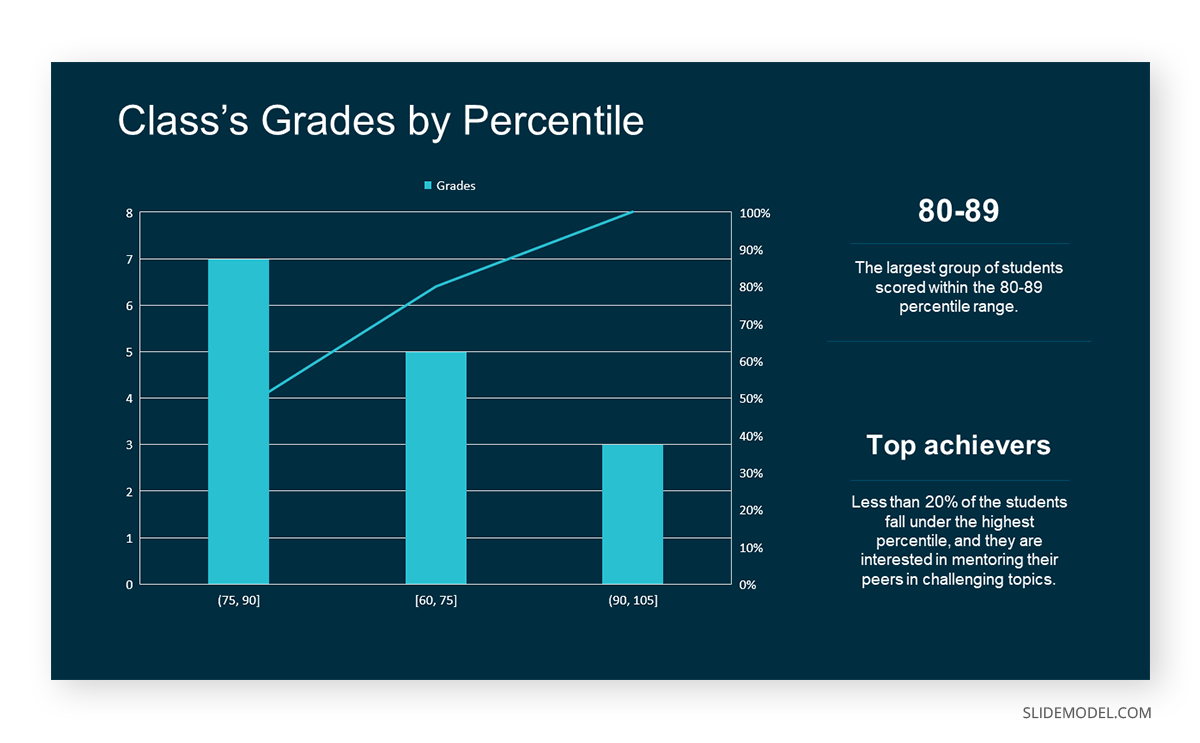
The histogram of the class grades reveals insightful patterns in the distribution. Most students, with seven students, fall within the 80-89 score range. The histogram provides a clear visualization of the class’s performance. It showcases a concentration of grades in the upper-middle range with few outliers at both ends. This analysis helps in understanding the overall academic standing of the class. It also identifies the areas for potential improvement or recognition.
Thus, histograms provide a clear visual representation of data distribution. They are easy to interpret, even for those without a statistical background. They apply to various types of data, including continuous and discrete variables. One weak point is that histograms do not capture detailed patterns in students’ data, with seven compared to other visualization methods.
A scatter plot is a graphical representation of the relationship between two variables. It consists of individual data points on a two-dimensional plane. This plane plots one variable on the x-axis and the other on the y-axis. Each point represents a unique observation. It visualizes patterns, trends, or correlations between the two variables.
Scatter plots are also effective in revealing the strength and direction of relationships. They identify outliers and assess the overall distribution of data points. The points’ dispersion and clustering reflect the relationship’s nature, whether it is positive, negative, or lacks a discernible pattern. In business, scatter plots assess relationships between variables such as marketing cost and sales revenue. They help present data correlations and decision-making.
Real-Life Application of Scatter Plot
A group of scientists is conducting a study on the relationship between daily hours of screen time and sleep quality. After reviewing the data, they managed to create this table to help them build a scatter plot graph:
Participant ID | Daily Hours of Screen Time | Sleep Quality Rating |
---|---|---|
1 | 9 | 3 |
2 | 2 | 8 |
3 | 1 | 9 |
4 | 0 | 10 |
5 | 1 | 9 |
6 | 3 | 7 |
7 | 4 | 7 |
8 | 5 | 6 |
9 | 5 | 6 |
10 | 7 | 3 |
11 | 10 | 1 |
12 | 6 | 5 |
13 | 7 | 3 |
14 | 8 | 2 |
15 | 9 | 2 |
16 | 4 | 7 |
17 | 5 | 6 |
18 | 4 | 7 |
19 | 9 | 2 |
20 | 6 | 4 |
21 | 3 | 7 |
22 | 10 | 1 |
23 | 2 | 8 |
24 | 5 | 6 |
25 | 3 | 7 |
26 | 1 | 9 |
27 | 8 | 2 |
28 | 4 | 6 |
29 | 7 | 3 |
30 | 2 | 8 |
31 | 7 | 4 |
32 | 9 | 2 |
33 | 10 | 1 |
34 | 10 | 1 |
35 | 10 | 1 |
In the provided example, the x-axis represents Daily Hours of Screen Time, and the y-axis represents the Sleep Quality Rating.
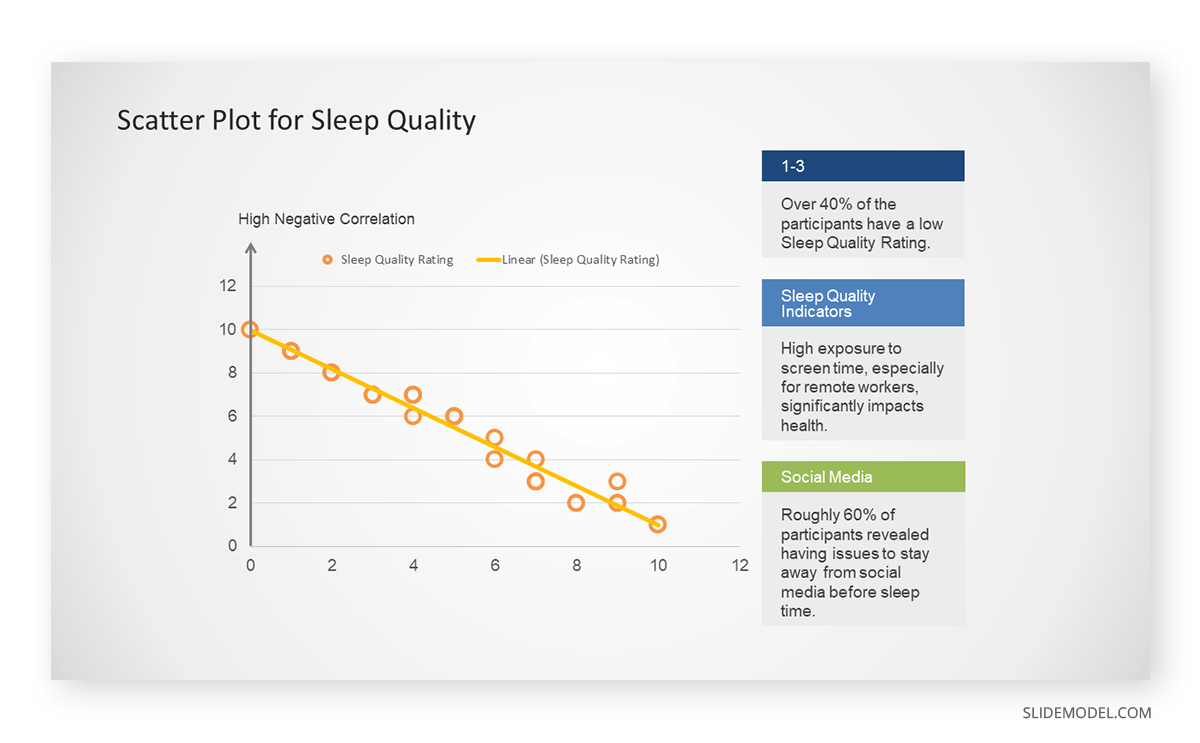
The scientists observe a negative correlation between the amount of screen time and the quality of sleep. This is consistent with their hypothesis that blue light, especially before bedtime, has a significant impact on sleep quality and metabolic processes.
There are a few things to remember when using a scatter plot. Even when a scatter diagram indicates a relationship, it doesn’t mean one variable affects the other. A third factor can influence both variables. The more the plot resembles a straight line, the stronger the relationship is perceived [11] . If it suggests no ties, the observed pattern might be due to random fluctuations in data. When the scatter diagram depicts no correlation, whether the data might be stratified is worth considering.
Choosing the appropriate data presentation type is crucial when making a presentation . Understanding the nature of your data and the message you intend to convey will guide this selection process. For instance, when showcasing quantitative relationships, scatter plots become instrumental in revealing correlations between variables. If the focus is on emphasizing parts of a whole, pie charts offer a concise display of proportions. Histograms, on the other hand, prove valuable for illustrating distributions and frequency patterns.
Bar charts provide a clear visual comparison of different categories. Likewise, line charts excel in showcasing trends over time, while tables are ideal for detailed data examination. Starting a presentation on data presentation types involves evaluating the specific information you want to communicate and selecting the format that aligns with your message. This ensures clarity and resonance with your audience from the beginning of your presentation.
1. Fact Sheet Dashboard for Data Presentation
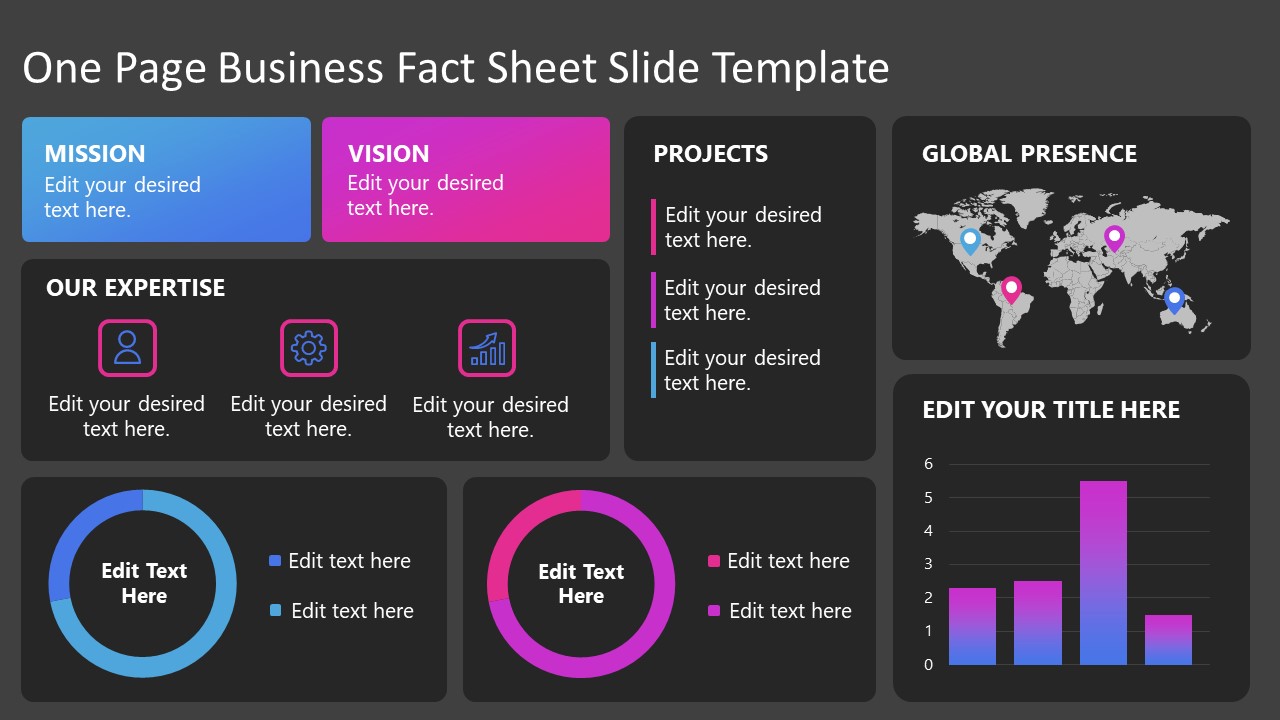
Convey all the data you need to present in this one-pager format, an ideal solution tailored for users looking for presentation aids. Global maps, donut chats, column graphs, and text neatly arranged in a clean layout presented in light and dark themes.
Use This Template
2. 3D Column Chart Infographic PPT Template
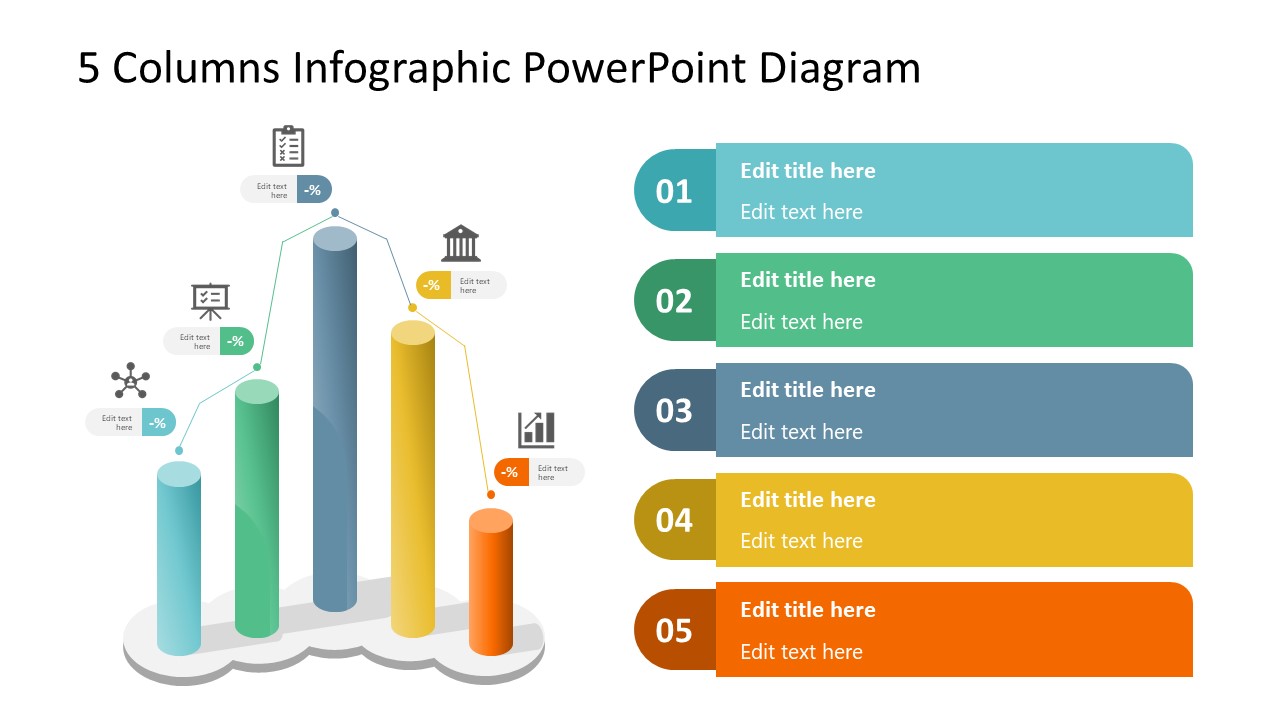
Represent column charts in a highly visual 3D format with this PPT template. A creative way to present data, this template is entirely editable, and we can craft either a one-page infographic or a series of slides explaining what we intend to disclose point by point.
3. Data Circles Infographic PowerPoint Template
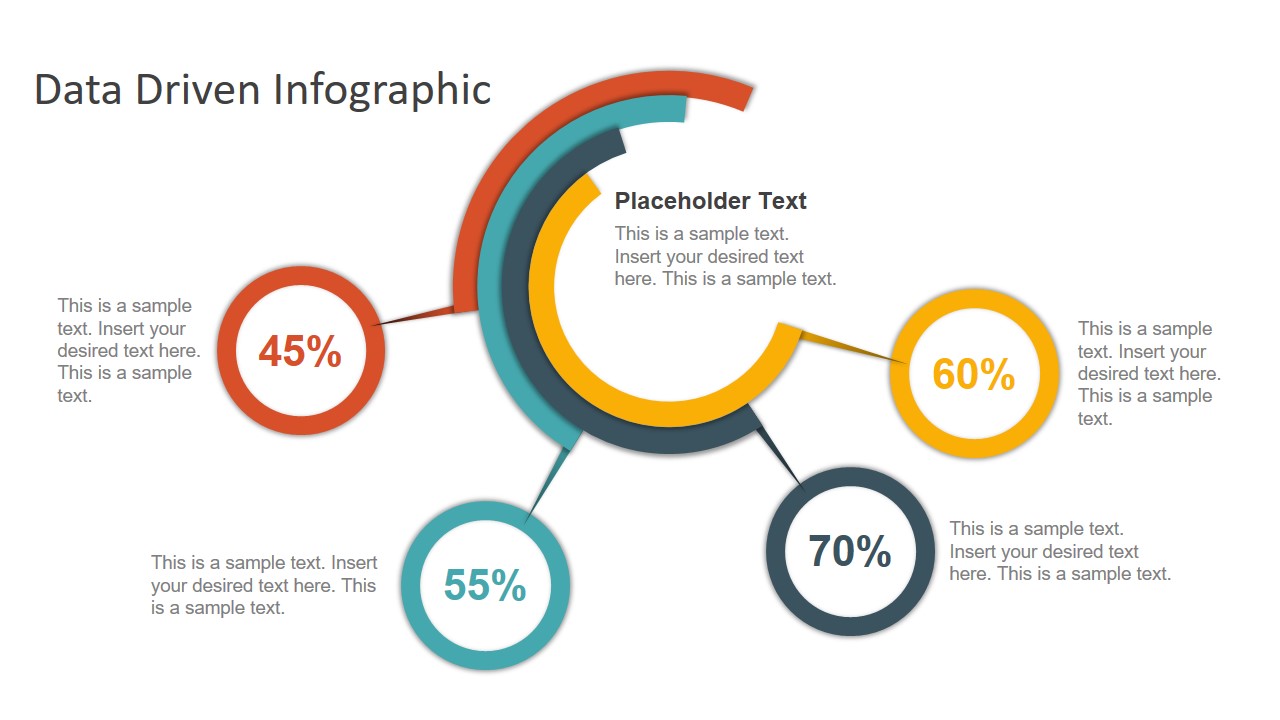
An alternative to the pie chart and donut chart diagrams, this template features a series of curved shapes with bubble callouts as ways of presenting data. Expand the information for each arch in the text placeholder areas.
4. Colorful Metrics Dashboard for Data Presentation
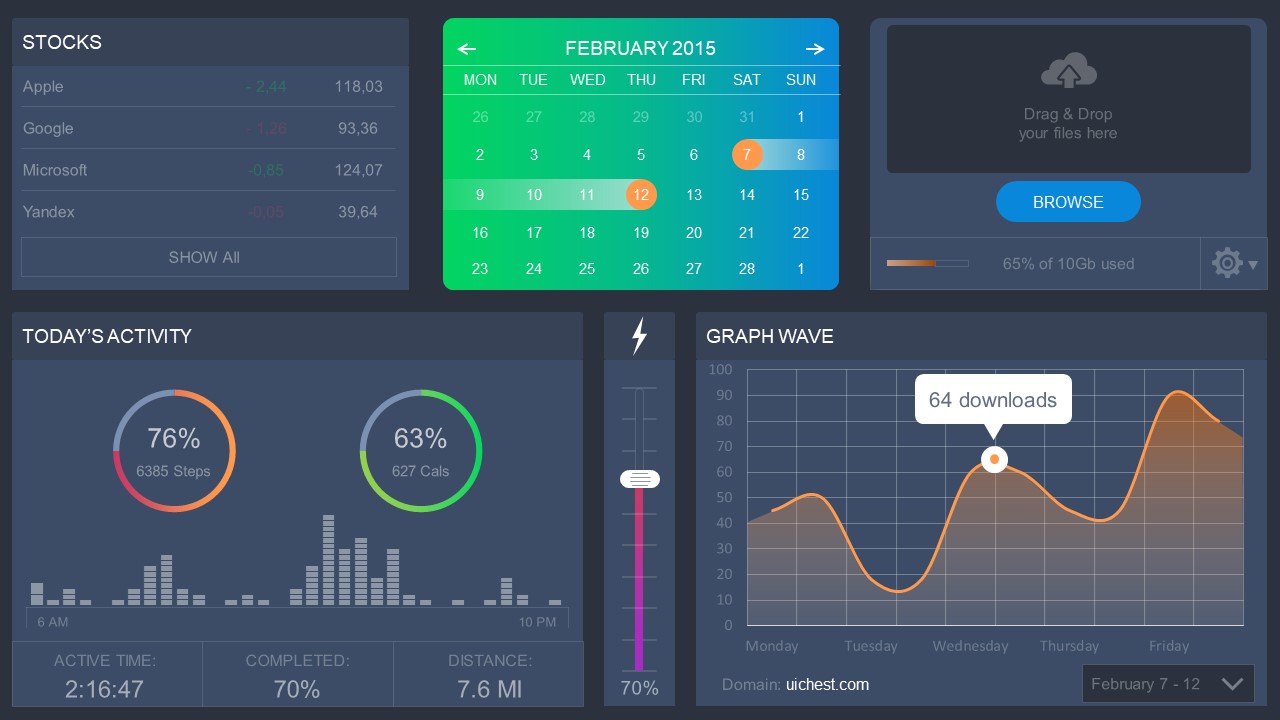
This versatile dashboard template helps us in the presentation of the data by offering several graphs and methods to convert numbers into graphics. Implement it for e-commerce projects, financial projections, project development, and more.
5. Animated Data Presentation Tools for PowerPoint & Google Slides
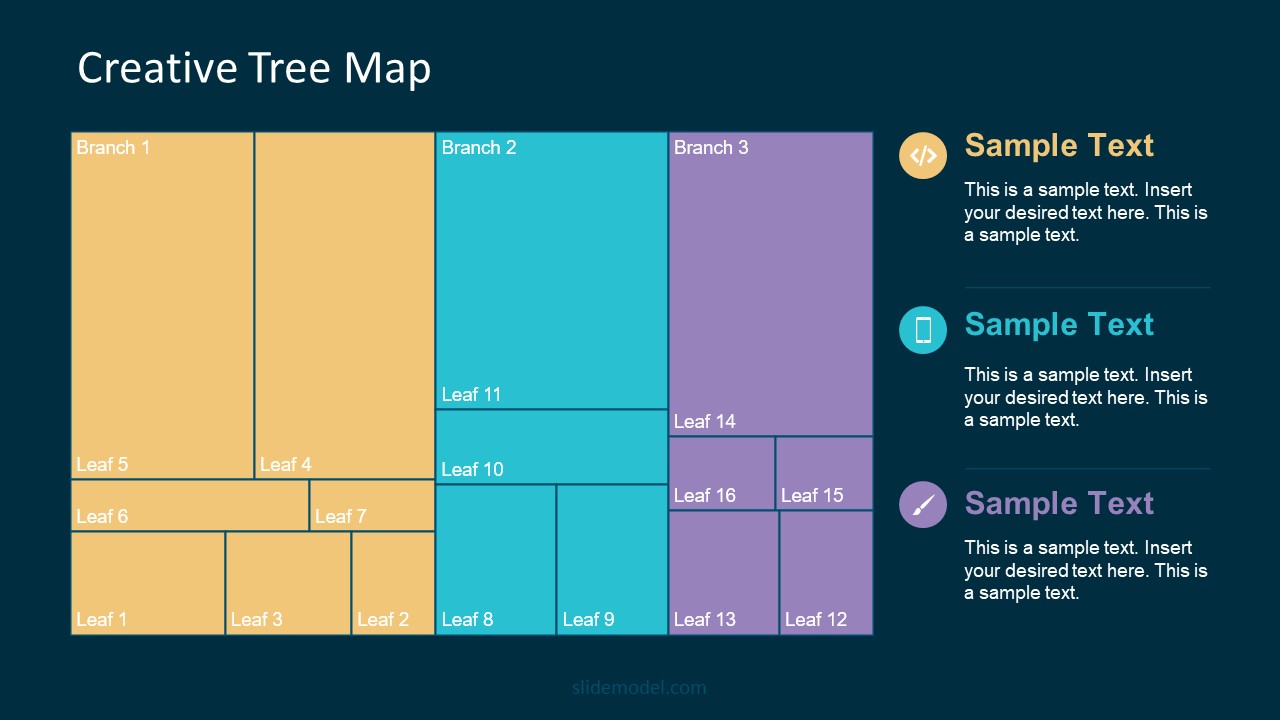
A slide deck filled with most of the tools mentioned in this article, from bar charts, column charts, treemap graphs, pie charts, histogram, etc. Animated effects make each slide look dynamic when sharing data with stakeholders.
6. Statistics Waffle Charts PPT Template for Data Presentations
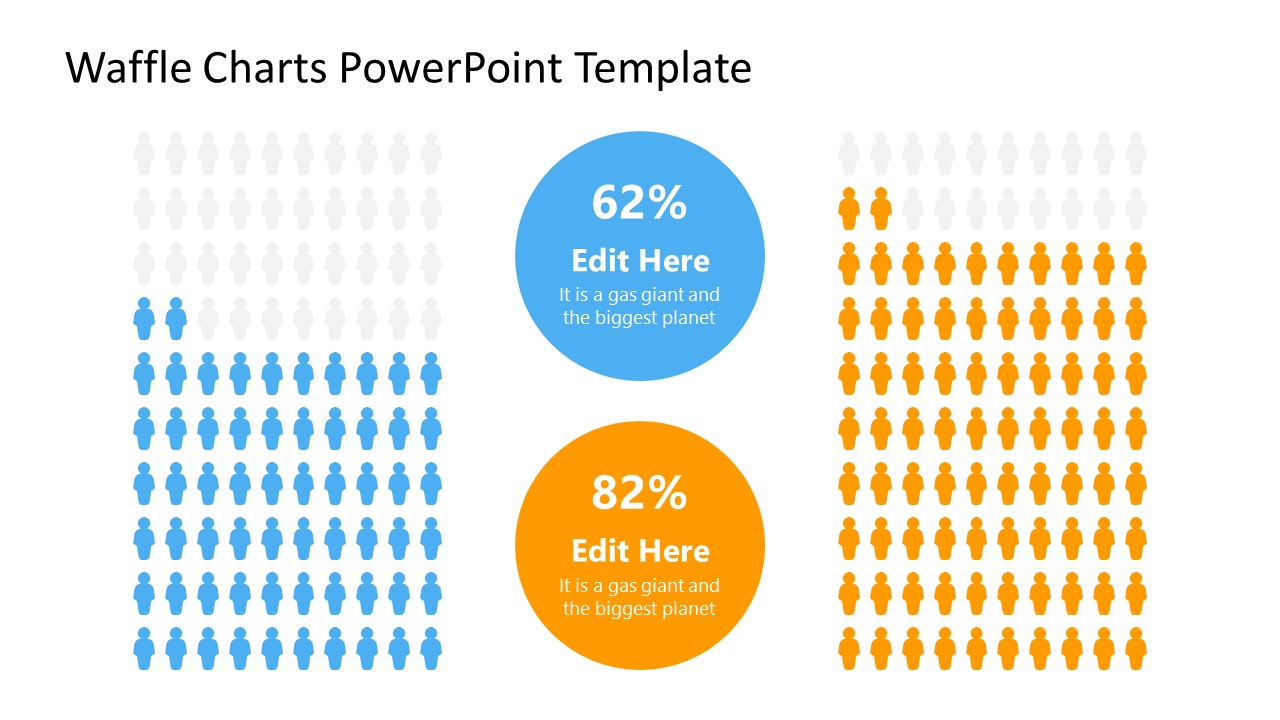
This PPT template helps us how to present data beyond the typical pie chart representation. It is widely used for demographics, so it’s a great fit for marketing teams, data science professionals, HR personnel, and more.
7. Data Presentation Dashboard Template for Google Slides
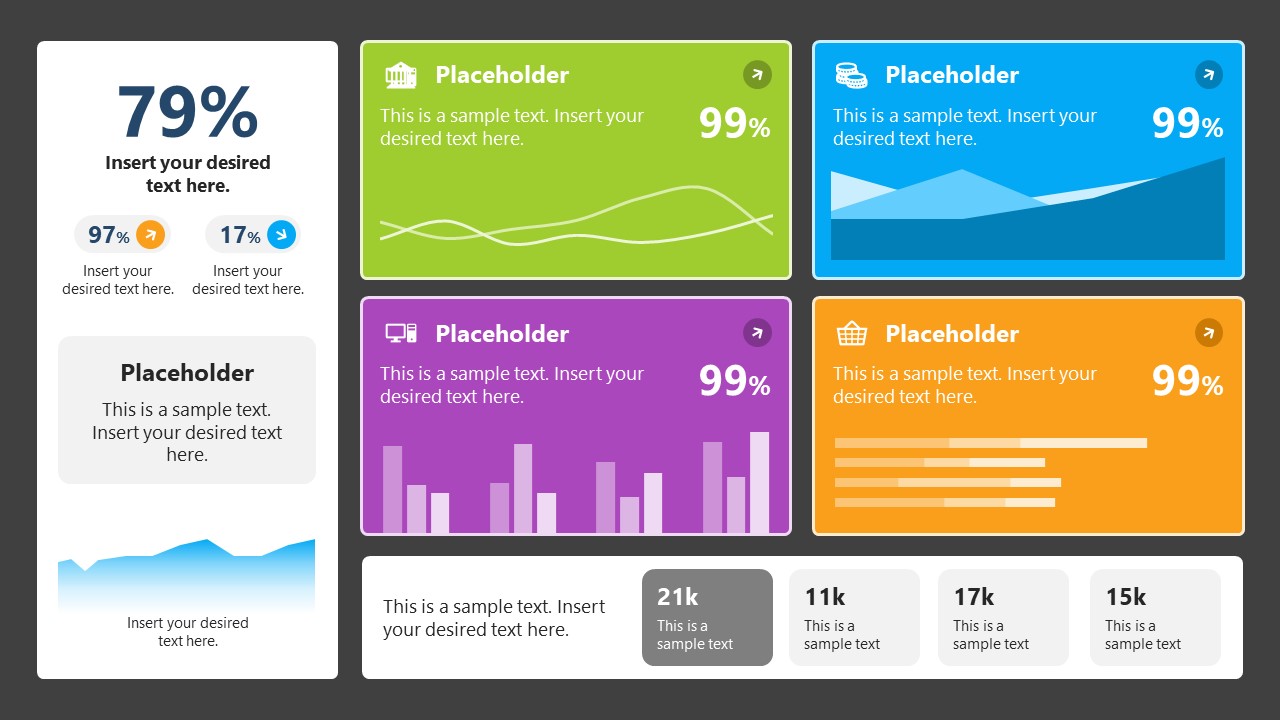
A compendium of tools in dashboard format featuring line graphs, bar charts, column charts, and neatly arranged placeholder text areas.
8. Weather Dashboard for Data Presentation
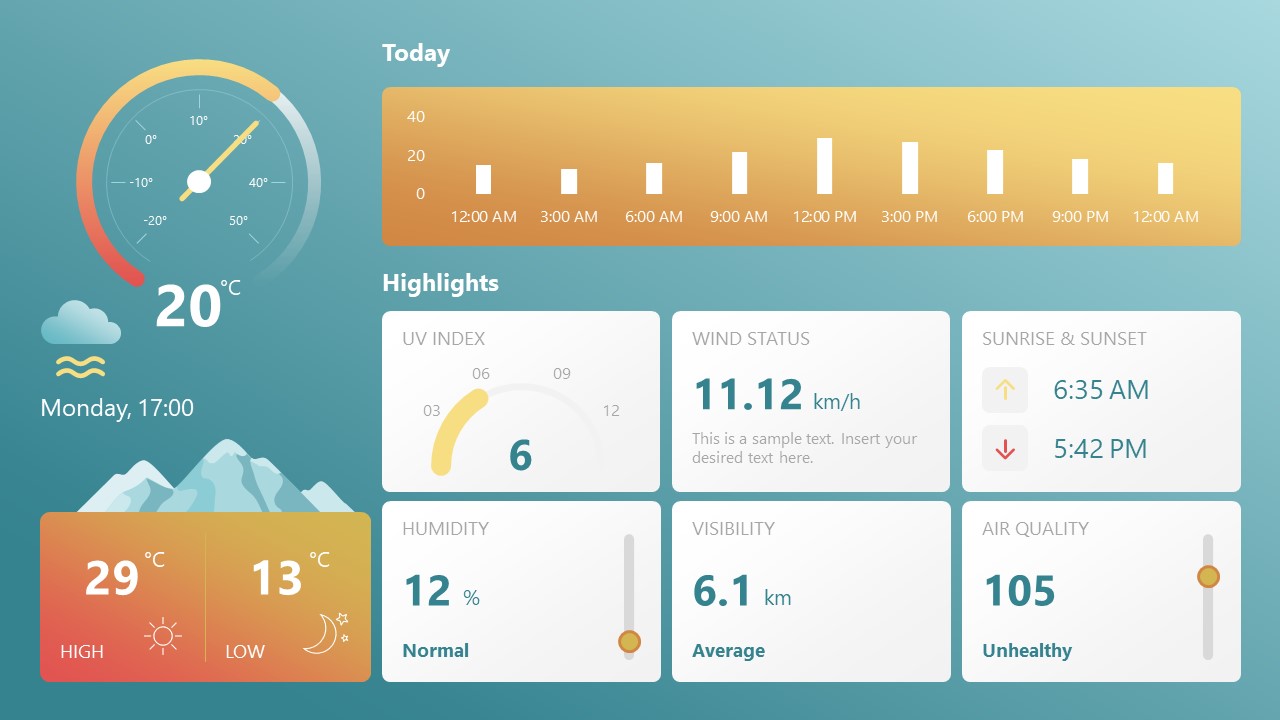
Share weather data for agricultural presentation topics, environmental studies, or any kind of presentation that requires a highly visual layout for weather forecasting on a single day. Two color themes are available.
9. Social Media Marketing Dashboard Data Presentation Template
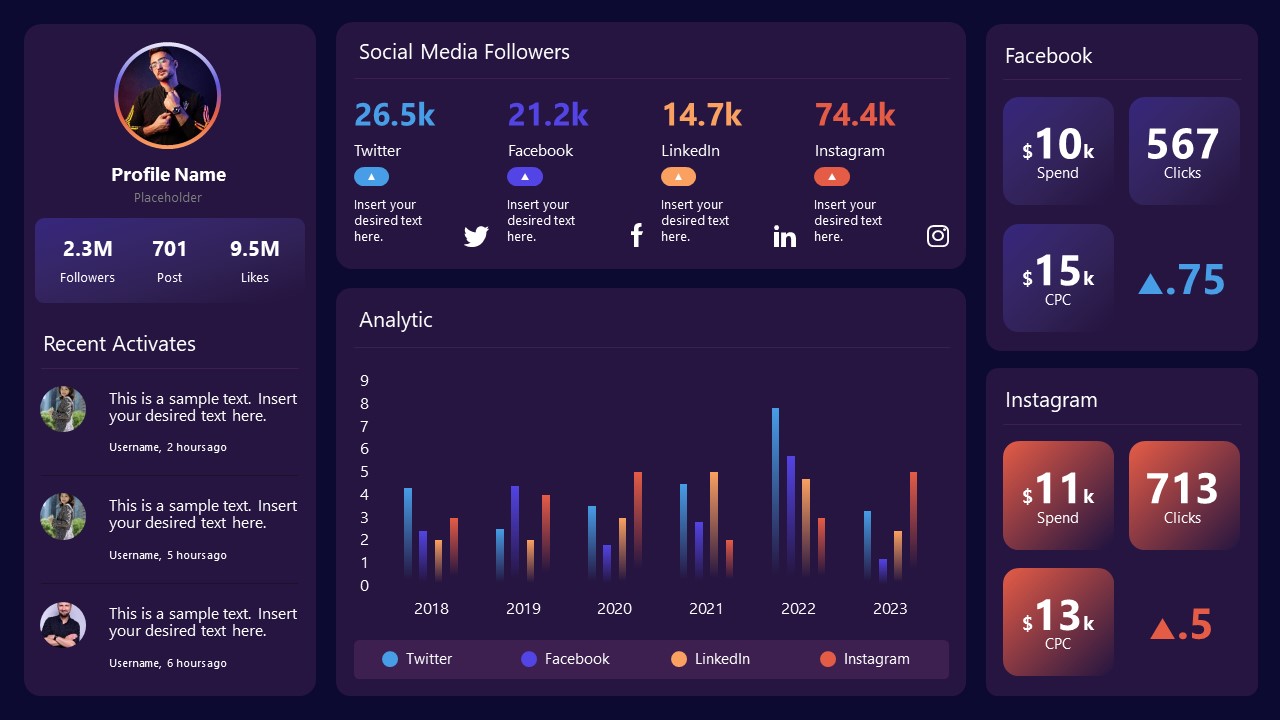
Intended for marketing professionals, this dashboard template for data presentation is a tool for presenting data analytics from social media channels. Two slide layouts featuring line graphs and column charts.
10. Project Management Summary Dashboard Template
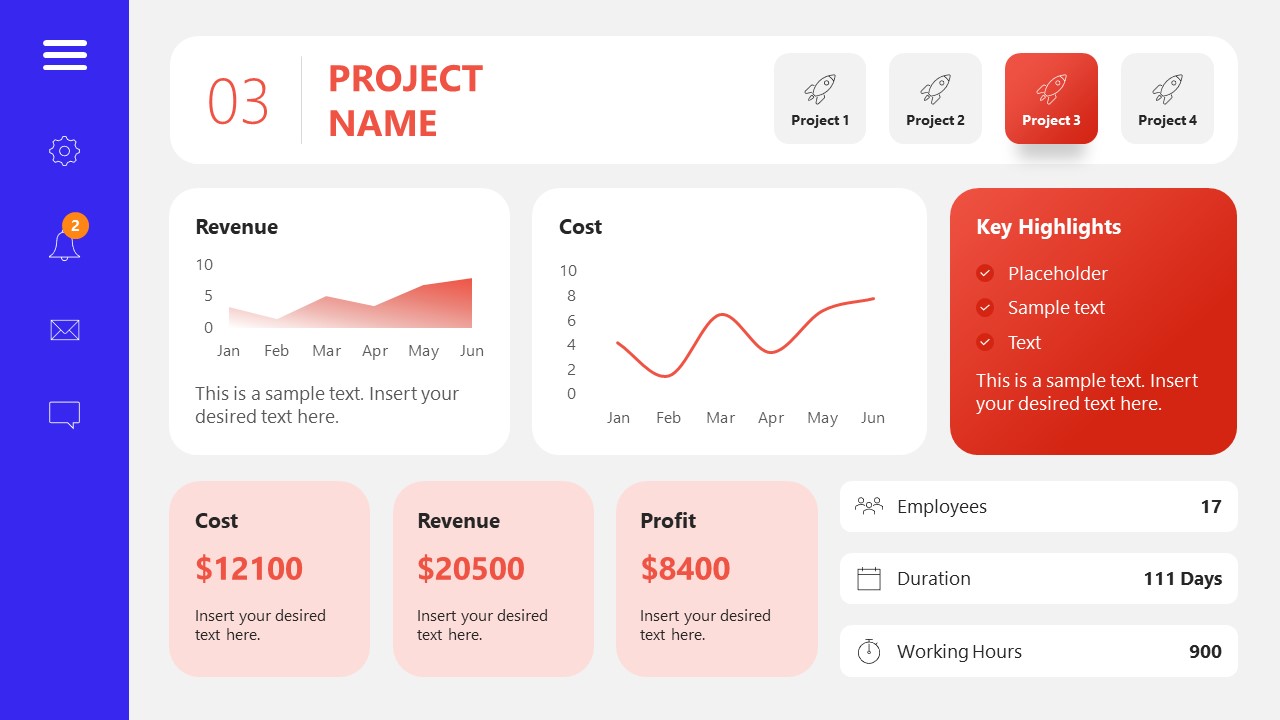
A tool crafted for project managers to deliver highly visual reports on a project’s completion, the profits it delivered for the company, and expenses/time required to execute it. 4 different color layouts are available.
11. Profit & Loss Dashboard for PowerPoint and Google Slides
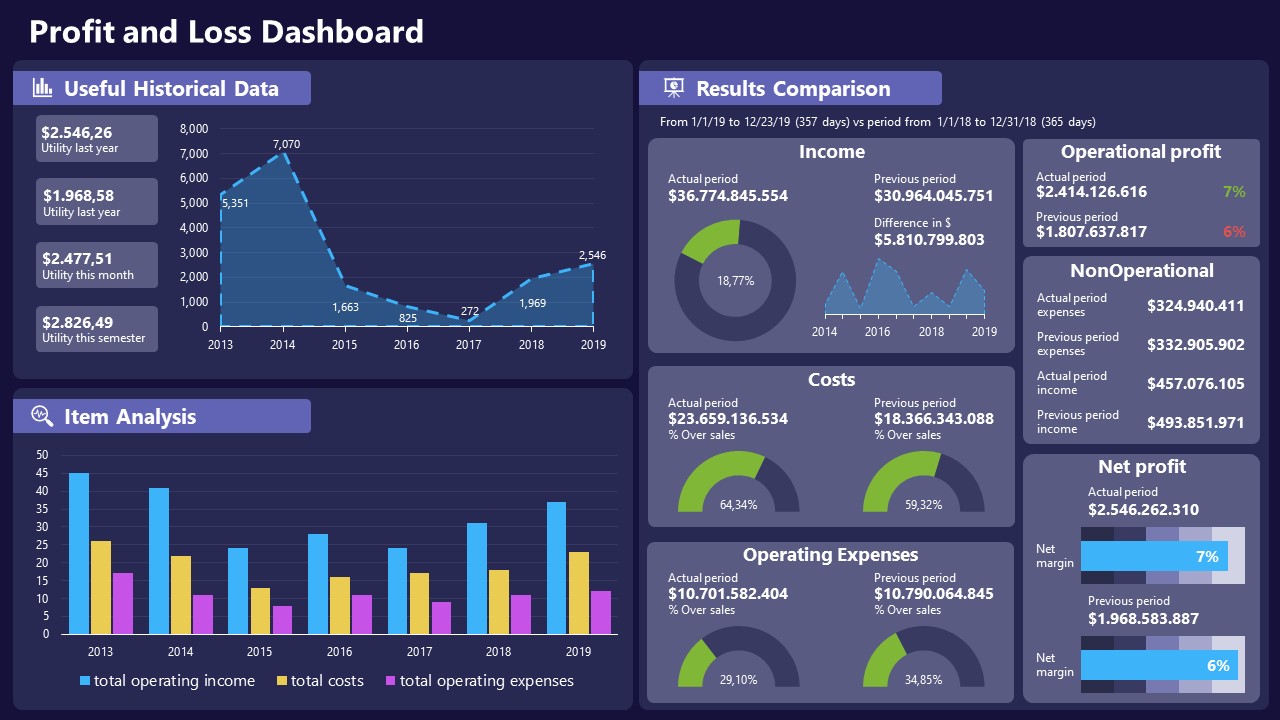
A must-have for finance professionals. This typical profit & loss dashboard includes progress bars, donut charts, column charts, line graphs, and everything that’s required to deliver a comprehensive report about a company’s financial situation.
Overwhelming visuals
One of the mistakes related to using data-presenting methods is including too much data or using overly complex visualizations. They can confuse the audience and dilute the key message.
Inappropriate chart types
Choosing the wrong type of chart for the data at hand can lead to misinterpretation. For example, using a pie chart for data that doesn’t represent parts of a whole is not right.
Lack of context
Failing to provide context or sufficient labeling can make it challenging for the audience to understand the significance of the presented data.
Inconsistency in design
Using inconsistent design elements and color schemes across different visualizations can create confusion and visual disarray.
Failure to provide details
Simply presenting raw data without offering clear insights or takeaways can leave the audience without a meaningful conclusion.
Lack of focus
Not having a clear focus on the key message or main takeaway can result in a presentation that lacks a central theme.
Visual accessibility issues
Overlooking the visual accessibility of charts and graphs can exclude certain audience members who may have difficulty interpreting visual information.
In order to avoid these mistakes in data presentation, presenters can benefit from using presentation templates . These templates provide a structured framework. They ensure consistency, clarity, and an aesthetically pleasing design, enhancing data communication’s overall impact.
Understanding and choosing data presentation types are pivotal in effective communication. Each method serves a unique purpose, so selecting the appropriate one depends on the nature of the data and the message to be conveyed. The diverse array of presentation types offers versatility in visually representing information, from bar charts showing values to pie charts illustrating proportions.
Using the proper method enhances clarity, engages the audience, and ensures that data sets are not just presented but comprehensively understood. By appreciating the strengths and limitations of different presentation types, communicators can tailor their approach to convey information accurately, developing a deeper connection between data and audience understanding.
[1] Government of Canada, S.C. (2021) 5 Data Visualization 5.2 Bar Chart , 5.2 Bar chart . https://www150.statcan.gc.ca/n1/edu/power-pouvoir/ch9/bargraph-diagrammeabarres/5214818-eng.htm
[2] Kosslyn, S.M., 1989. Understanding charts and graphs. Applied cognitive psychology, 3(3), pp.185-225. https://apps.dtic.mil/sti/pdfs/ADA183409.pdf
[3] Creating a Dashboard . https://it.tufts.edu/book/export/html/1870
[4] https://www.goldenwestcollege.edu/research/data-and-more/data-dashboards/index.html
[5] https://www.mit.edu/course/21/21.guide/grf-line.htm
[6] Jadeja, M. and Shah, K., 2015, January. Tree-Map: A Visualization Tool for Large Data. In GSB@ SIGIR (pp. 9-13). https://ceur-ws.org/Vol-1393/gsb15proceedings.pdf#page=15
[7] Heat Maps and Quilt Plots. https://www.publichealth.columbia.edu/research/population-health-methods/heat-maps-and-quilt-plots
[8] EIU QGIS WORKSHOP. https://www.eiu.edu/qgisworkshop/heatmaps.php
[9] About Pie Charts. https://www.mit.edu/~mbarker/formula1/f1help/11-ch-c8.htm
[10] Histograms. https://sites.utexas.edu/sos/guided/descriptive/numericaldd/descriptiven2/histogram/ [11] https://asq.org/quality-resources/scatter-diagram
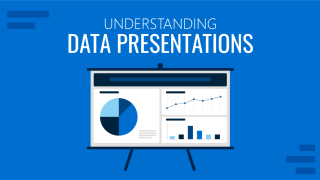
Like this article? Please share
Data Analysis, Data Science, Data Visualization Filed under Design
Related Articles
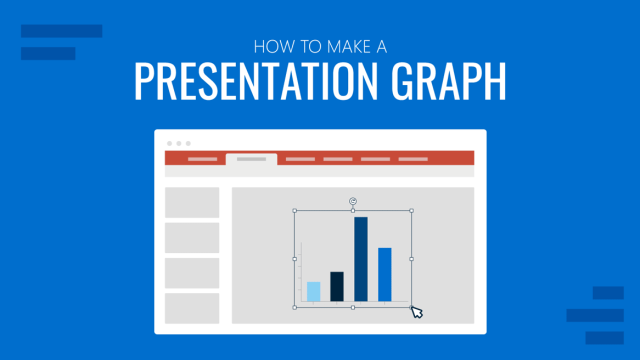
Filed under Design • March 27th, 2024
How to Make a Presentation Graph
Detailed step-by-step instructions to master the art of how to make a presentation graph in PowerPoint and Google Slides. Check it out!
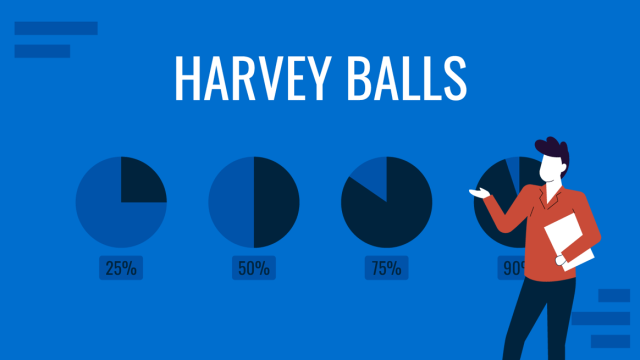
Filed under Presentation Ideas • January 6th, 2024
All About Using Harvey Balls
Among the many tools in the arsenal of the modern presenter, Harvey Balls have a special place. In this article we will tell you all about using Harvey Balls.
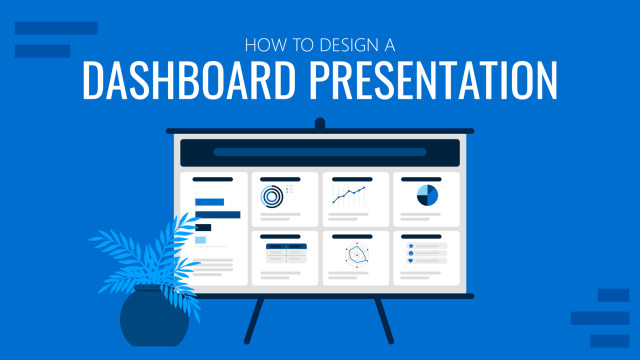
Filed under Business • December 8th, 2023
How to Design a Dashboard Presentation: A Step-by-Step Guide
Take a step further in your professional presentation skills by learning what a dashboard presentation is and how to properly design one in PowerPoint. A detailed step-by-step guide is here!
Leave a Reply

9 Data Presentation Tools for Business Success
- By Judhajit Sen
- May 29, 2024
A data presentation is a slide deck that shares quantitative information with an audience using visuals and effective presentation techniques . The goal is to make complex data easily understandable and actionable using data presentation examples like graphs and charts, tables, dashboards, and clear text explanations.
Data presentations help highlight trends, patterns, and insights, allowing the audience to grasp complicated concepts or trends quickly. This makes it easier for them to make informed decisions or conduct deeper analysis.
Data visualization in presentations is used in every field, from academia to business and industry. Raw data is often too complex to understand directly, so data analysis breaks it down into charts and graphs. These tools help turn raw data into useful information.
Once the information is extracted, it’s presented graphically. A good presentation can significantly enhance understanding and response.
Think of data presentation as storytelling in business presentations with charts. A common mistake is assuming the audience understands the data as well as the presenter. Always consider your audience’s knowledge level and what information they need when you present your data.
To present the data effectively:
1. Provide context to help the audience understand the numbers.
2. Compare data groups using visual aids.
3. Step back and view the data from the audience’s perspective.
Data presentations are crucial in nearly every industry, helping professionals share their findings clearly after analyzing data.
Key Takeaways
- Simplifying Complex Data: Data presentations turn complex data into easy-to-understand visuals and narratives, helping audiences quickly grasp trends and insights for informed decision-making.
- Versatile Tools: Various tools like bar charts, dashboards, pie charts, histograms, scatter plots, pictograms, textual presentations, and tables each serve unique purposes, enhancing the clarity and impact of the data.
- Audience Consideration: Tailor your presentation to the audience’s knowledge level, providing context and using simple visuals to make the information accessible and actionable.
- Effective Data Storytelling: Combining clear context, organized visuals, and thoughtful presentation ensures that the data’s story is conveyed effectively, supporting better business decisions and success.
Following are 9 data presentation tools for business success.
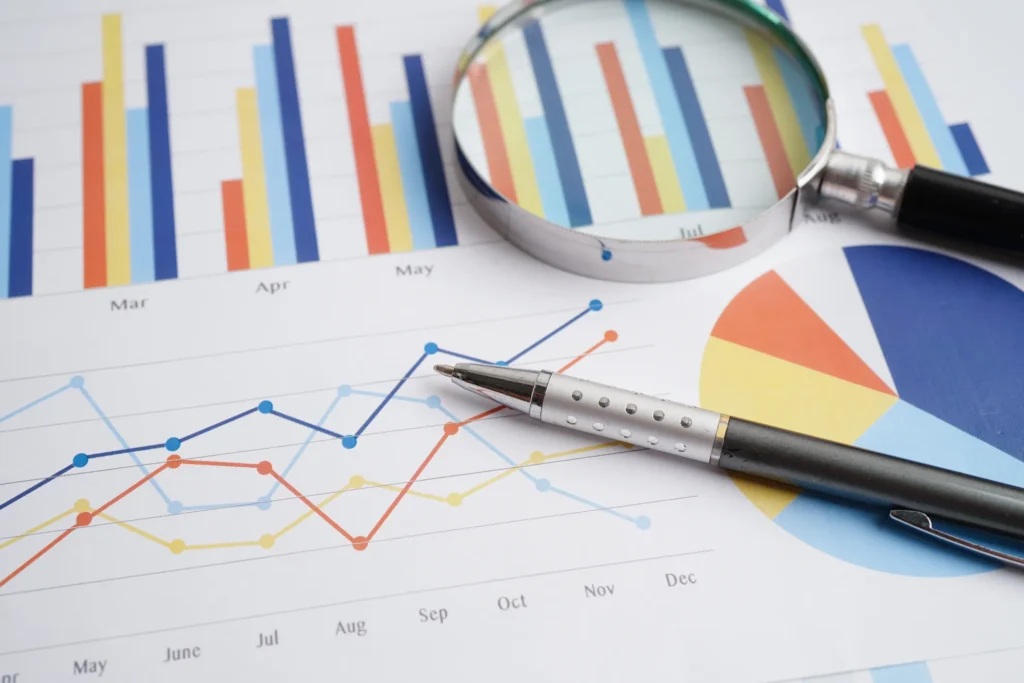
Bar charts are a simple yet powerful method of presentation of the data using rectangular bars to show quantities or frequencies. They make it easy to spot patterns or trends at a glance. Bar charts can be vertical (column charts) or horizontal, depending on how you want to display your data.
In a bar graph, categories are displayed on one axis, usually the x-axis for vertical charts and the y-axis for horizontal ones. The bars’ lengths represent the values or frequencies of these categories, with the scale marked on the opposite axis.
These charts are ideal for comparing data across different categories or showing trends over time. Each bar’s height (or length in a horizontal chart) is directly proportional to the value it represents. This visual representation helps illustrate differences or changes in data.
Bar charts are versatile tools in business reports, academic presentations, and more. To make your bar charts effective:
- Ensure they are concise and have easy-to-read labels.
- Avoid clutter by not including too many categories, making the chart hard to read.
- Keep it simple to maintain clarity and impact, whether your bars go up or sideways.
Line Graphs
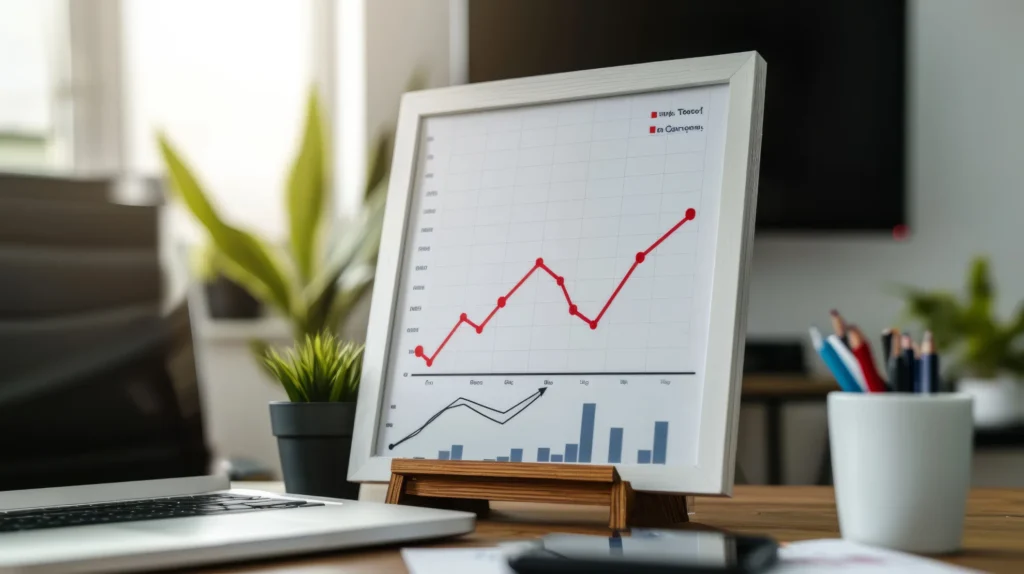
Line graphs show how data changes over time or with continuous variables. They connect points of data with straight lines, making it easy to see trends and fluctuations. These graphs are handy when comparing multiple datasets over the same timeline.
Using line graphs, you can track things like stock prices, sales projections, or experimental results. The x-axis represents time or another continuous variable, while the y-axis shows the data values. This setup allows you to understand the ups and downs in the data quickly.
To make your graphs effective, keep them simple. Avoid overcrowding with too many lines, highlight significant changes, use labels, and give your graph a clear, catchy title. This will help your audience grasp the information quickly and easily.
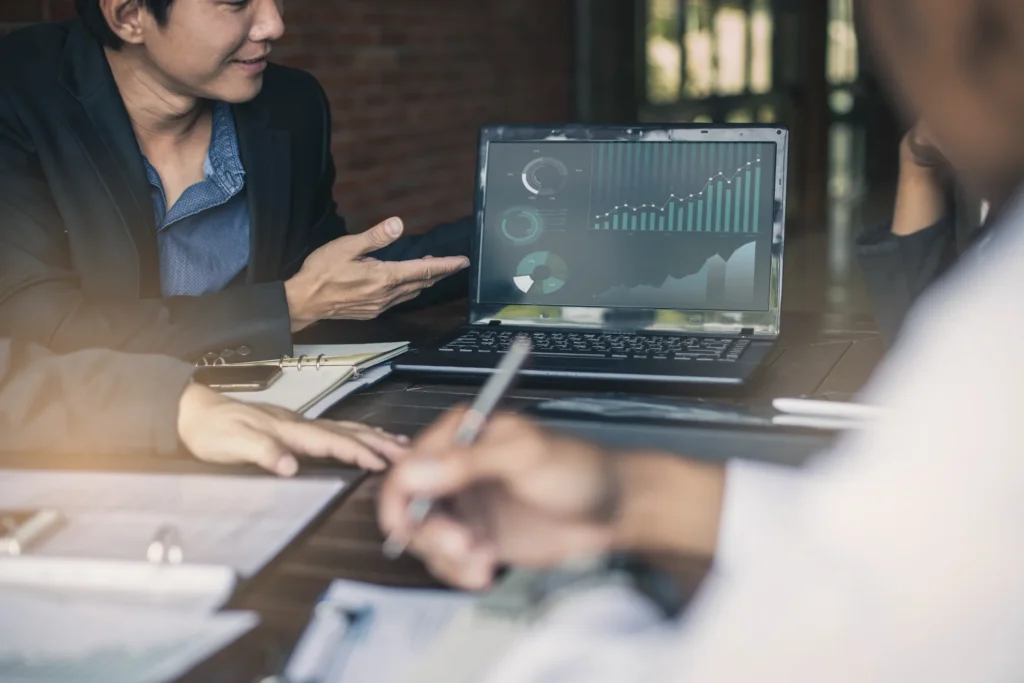
A data dashboard is a data analysis presentation example for analyzing information. It combines different graphs, charts, and tables in one layout to show the information needed to meet one or more objectives. Dashboards help quickly see Key Performance Indicators (KPIs) by displaying visuals you’ve already made in worksheets.
It’s best to keep the number of visuals on a dashboard to three or four. Adding too many can make it hard to see the main points. Dashboards are helpful for business analytics, like analyzing sales, revenue, and marketing metrics. In manufacturing, they help users understand the production scenario and track critical KPIs for each production line.
Dashboards represent vital points of data or metrics in an easy-to-understand way. They are often an interactive presentation idea , allowing users to drill down into the data or view different aspects of it.
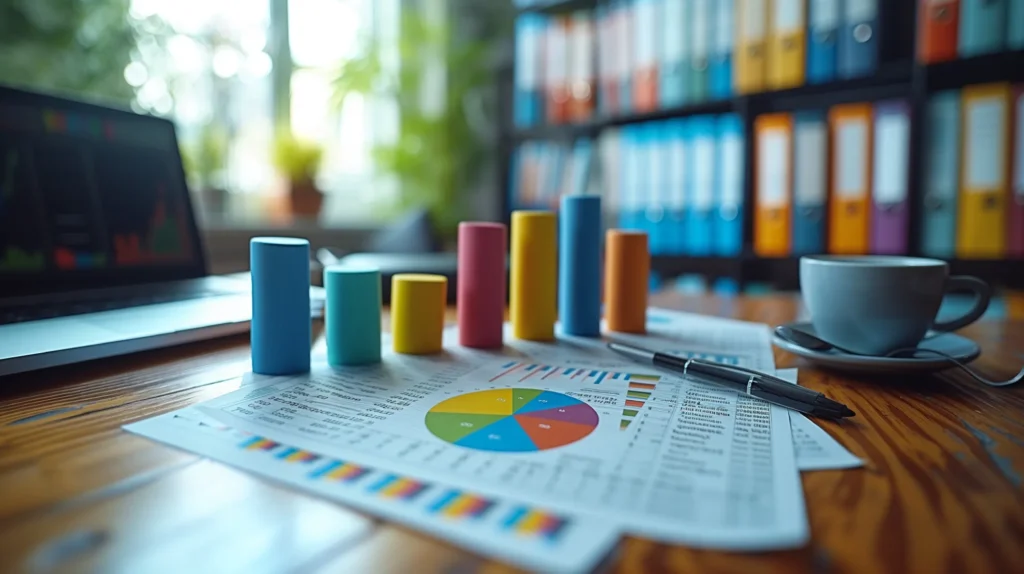
Pie charts are circular graphs divided into parts to show numerical proportions. Each portion represents a part of the whole, making it easy to see each component’s contribution to the total.
The size of each slice is determined by its value relative to the total. A pie chart with more significant points of data will have larger slices, and the whole chart will be more important. However, you can make all pies the same size if proportional representation isn’t necessary.
Pie charts are helpful in business to show percentage distributions, compare category sizes, or present simple data sets where visualizing ratios is essential. They work best with fewer variables.
Each “slice” represents a fraction of the total, and the size of each slice shows its share of the whole. Pie charts are excellent for showing how a whole is divided into parts, such as survey results or demographic data.
While pie charts are great for simple distributions, they can get confusing with too many categories or slight differences in proportions. To keep things clear, label each slice with percentages or values and use a legend if there are many categories. If more detail is needed, consider using a donut chart with a blank center for extra information and a less cluttered look.
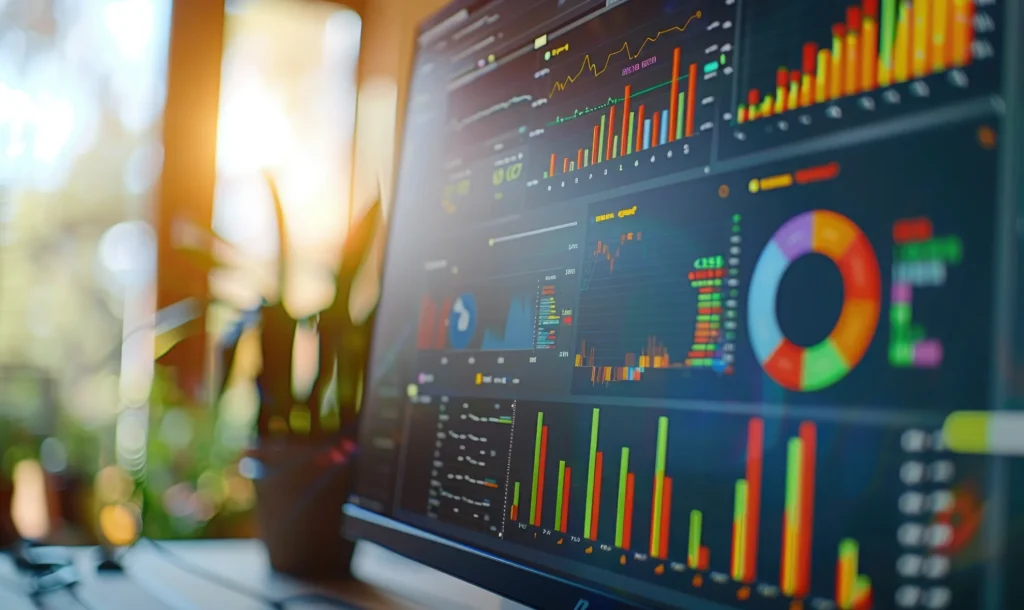
A histogram is a graphical presentation of data to help in understanding the distribution of numerical values. Unlike bar charts that show each response separately, histograms group numeric responses into bins and display the frequency of reactions within each bin. The x-axis denotes the range of values, while the y-axis shows the frequency of those values.
Histograms are useful for understanding your data’s distribution, identifying shared values, and spotting outliers. They highlight the story your data tells, whether it’s exam scores, sales figures, or any other numerical data.
Histograms are great for visualizing the distribution and frequency of a single variable. They divide the data into bins, and the height of each bar indicates how many points of data fall into that bin. This makes it easy to see trends like peaks, gaps, or skewness in your data.
To make your histogram effective, choose bin sizes that capture meaningful patterns. Clear axis labels and titles also help in explaining the data distribution.
Scatter Plot
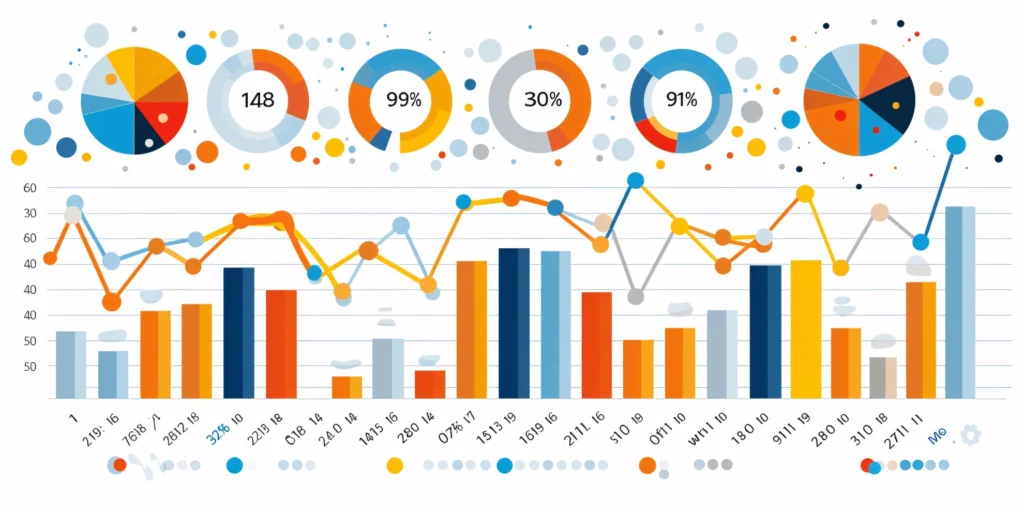
Using individual data points, a scatter plot chart is a presentation of data in visual form to show the relationship between two variables. Each variable is plotted along the x-axis and y-axis, respectively. Each point on the scatter plot represents a single observation.
Scatter plots help visualize patterns, trends, and correlations between the two variables. They can also help identify outliers and understand the overall distribution of data points. The way the points are spread out or clustered together can indicate whether there is a positive, negative, or no clear relationship between the variables.
Scatter plots can be used in practical applications, such as in business, to show how variables like marketing cost and sales revenue are related. They help understand data correlations, which aids in decision-making.
To make scatter plots more effective, consider adding trendlines or regression analysis to highlight patterns. Labeling key data points or tooltips can provide additional information and make the chart easier to interpret.
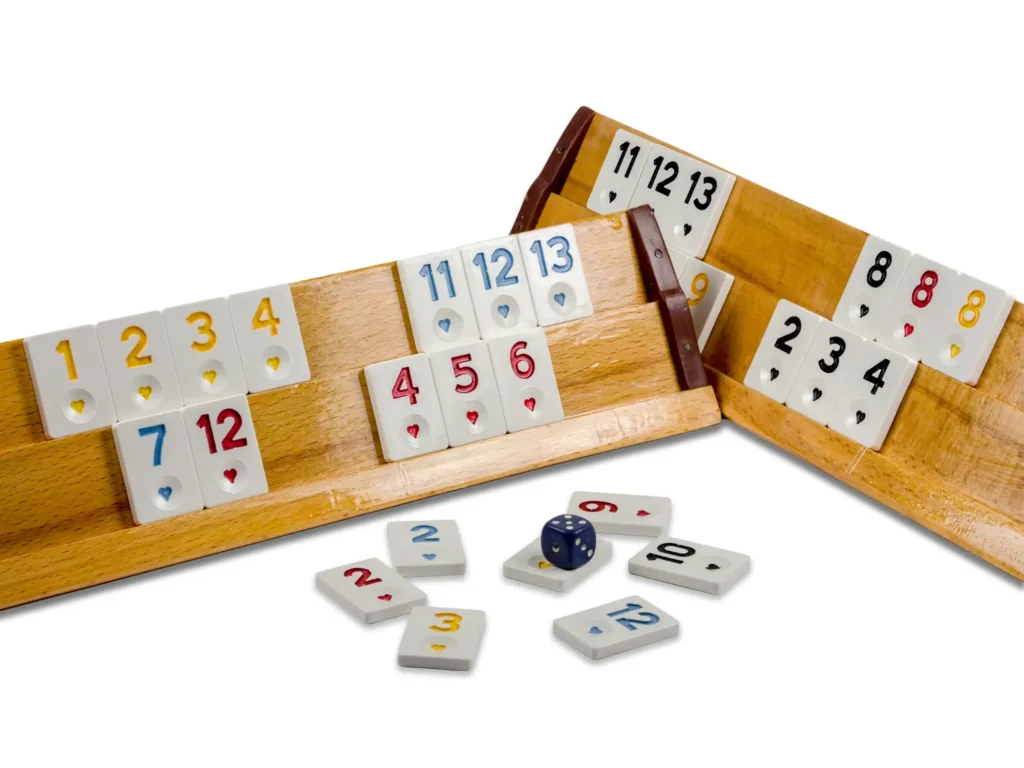
A pictogram is the simplest form of data presentation and analysis, often used in schools and universities to help students grasp concepts more effectively through pictures.
This type of diagram uses images to represent data. For example, you could draw five books to show the number of books sold in the first week of release, with each image representing 1,000 books. If consumers bought 5,000 books, you would display five book images.
Using simple icons or images makes the information visually intuitive. Instead of relying on numbers or complex graphs, pictograms use straightforward symbols to depict data points. For example, a thumbs-up emoji can illustrate customer satisfaction levels, with each emoji representing a different level of satisfaction.
Pictograms are excellent for visual data presentation. Choose symbols that are easy to interpret and relevant to the data to ensure clarity. Consistent scaling and a legend explaining the symbols’ meanings are essential for an effective presentation.
Textual Presentation
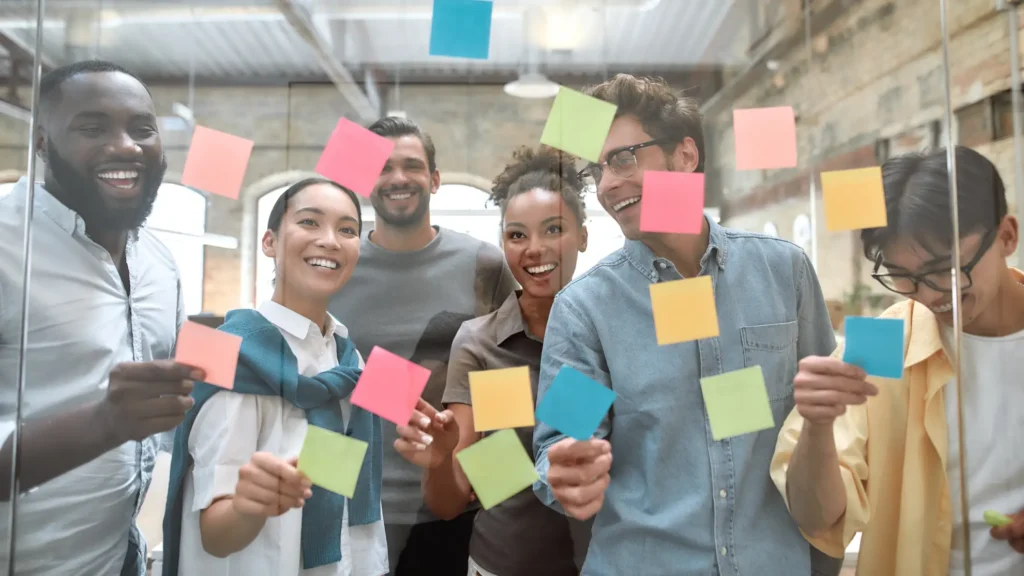
Textual presentation uses words to describe the relationships between pieces of information. This method helps share details that can’t be shown in a graph or table. For example, researchers often present findings in a study textually to provide extra context or explanation. A textual presentation can make the information more transparent.
This type of presentation is common in research and for introducing new ideas. Unlike charts or graphs, it relies solely on paragraphs and words.
Textual presentation also involves using written content, such as annotations or explanatory text, to explain or complement data. While it doesn’t use visual presentation aids like charts, it is a widely used method for presenting qualitative data. Think of it as the narrative that guides your audience through the data.
Adequate textual data may make complex information more accessible. Breaking down complex details into bullet points or short paragraphs helps your audience understand the significance of numbers and visuals. Headings can guide the reader’s attention and tell a coherent story.
Tabular Presentation
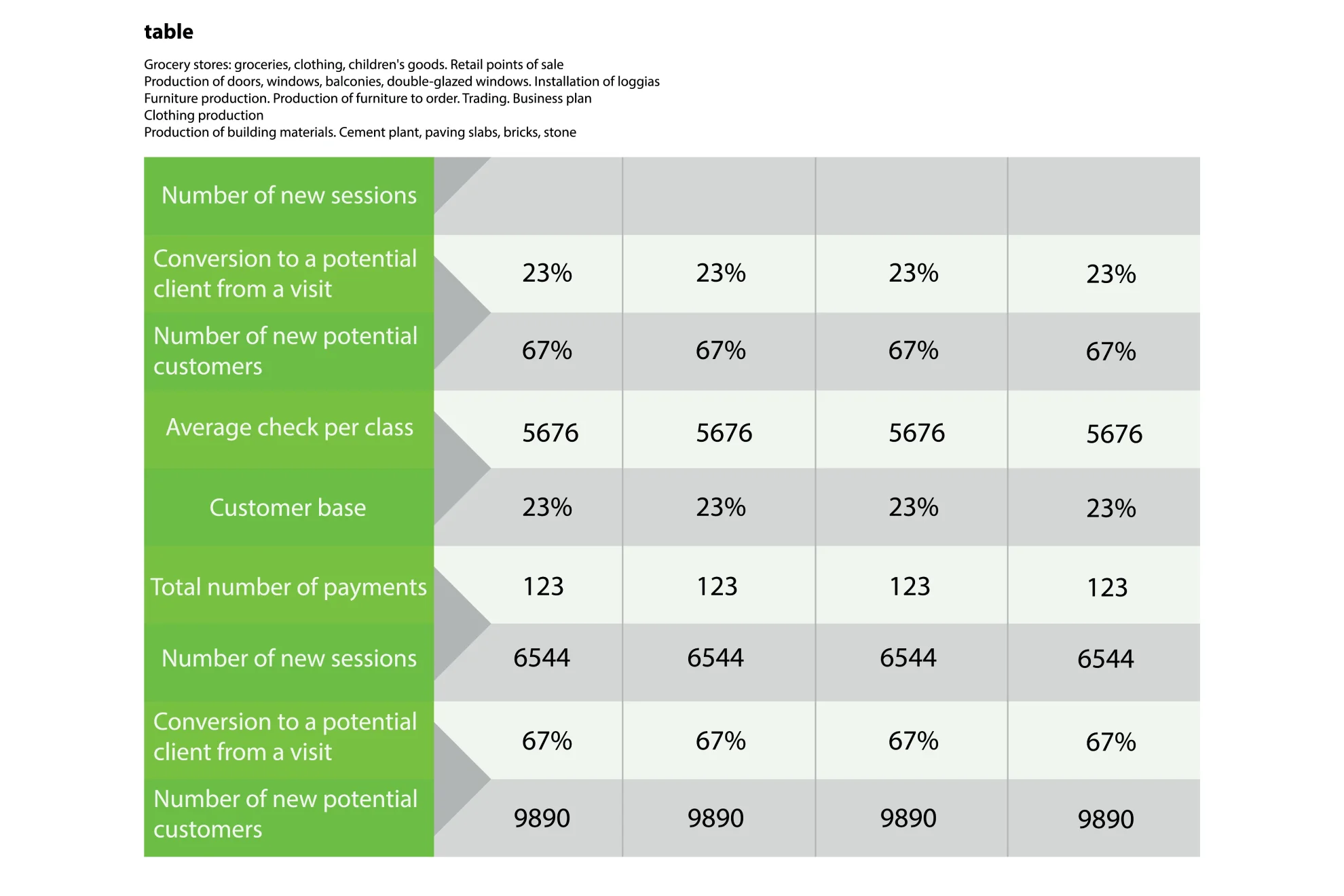
Tabular presentation uses tables to share information by organizing data in rows and columns. This method is useful for comparing data and visualizing information. Researchers often use tables to analyze data in various classifications:
Qualitative classification: This includes qualities like nationality, age, social status, appearance, and personality traits, helping to compare sociological and psychological information.
Quantitative classification: This covers items you can count or number.
Spatial classification: This deals with data based on location, such as information about a city, state, or region.
Temporal classification: This involves time-based data measured in seconds, hours, days, or weeks.
Tables simplify data, making it easily consumable, allow for side-by-side comparisons, and save space in your presentation by condensing information.
Using rows and columns, tabular presentation focuses on clarity and precision. It’s about displaying numerical data in a structured grid, clearly showing individual data points. Tables are invaluable for showcasing detailed data, facilitating comparisons, and presenting exact numerical information. They are commonly used in reports, spreadsheets, and academic papers.
Organize tables neatly with clear headers and appropriate column widths to ensure readability. Highlight important data points or patterns using shading or font formatting. Tables are simple and effective, especially when the audience needs to know precise figures.
Elevate Business Decisions with Effective Data Presentations
Data presentations are essential for transforming complex data into understandable and actionable insights. Data presentations simplify the process of interpreting quantitative information by utilizing data presentation examples like charts, graphs, tables, infographics, dashboards, and clear narratives. This method of storytelling with visuals highlights trends, patterns, and insights, enabling audiences to make informed decisions quickly.
In business, data analysis presentations are invaluable. Different types of presentation tools like bar charts help compare categories and track changes over time, while dashboards consolidate various metrics into a comprehensive view. Pie charts and histograms offer clear views of distributions and proportions, aiding in grasping the bigger picture. Scatter plots reveal relationships between variables, and pictograms make data visually intuitive. Textual presentations and tables provide detailed context and precise figures, which are essential for thorough analysis and comparison.
Consider the audience’s knowledge level to tailor the best way to present data in PowerPoint. Clear context, simple visuals, and thoughtful organization ensure the data’s story is easily understood and impactful. Mastering these nine data presentation types can significantly enhance business success by making data-driven decisions more accessible and practical.
Frequently Asked Questions (FAQs)
1. What is a data presentation?
A data presentation is a slide deck that uses visuals and narrative techniques to make complex data easy to understand and actionable. It includes charts, graphs, tables, infographics, dashboards, and clear text explanations.
2. Why are data presentations important in business?
Data presentations are crucial because they help highlight trends, patterns, and insights, making it easier for the audience to understand complicated concepts. This enables better decision-making and deeper analysis.
3. What types of data presentation tools are commonly used?
Common tools include bar charts, line graphs, dashboards, pie charts, histograms, scatter plots, pictograms, textual presentations, and tables. Each tool has a unique way of representing data to aid understanding.
4. How can I ensure my data presentation is effective?
To ensure effectiveness, provide context, compare data sets using visual aids, consider your audience’s knowledge level, and keep visuals simple. Organizing information thoughtfully and avoiding clutter enhances clarity and impact.
Transform Your Data into Compelling Stories with Prezentium
Unlock the full potential of your business data with Prezentium ‘s expert presentation services. Our AI-powered solutions turn complex data into clear, actionable insights, helping you easily make informed decisions.
Prezentium’s Overnight Presentations ensure you wake up to a stunning, ready-to-use presentation in your inbox by 9:30 am PST. Send your requirements by 5:30 pm PST, and let our team combine business acumen, visual design, and data science to craft a presentation that highlights trends and insights seamlessly.
Our Presentation Specialists transform raw ideas and meeting notes into captivating presentations. Whether you need new designs or bespoke templates, our experts bring your vision to life with precision and creativity.
Enhance your team’s skills with Zenith Learning, our interactive workshops that blend structured problem-solving with visual storytelling. Learn to present data effectively and make a lasting impact in your business communications.
Prezentium’s services are designed to help you make the most of your data, from bar charts to dashboards, ensuring your presentations are informative and visually engaging. Let us help you tell your data’s story in a way that resonates. Contact Prezentium today to elevate your business presentations.
Why wait? Avail a complimentary 1-on-1 session with our presentation expert. See how other enterprise leaders are creating impactful presentations with us.
4 Tips to Write an Effective Presentation Script
12 reasons why business communication is important.
Making Sense of Text and Data
- News & Events
- Case Studies
- Ontotext Applications
- Knowledge Graph Applications
- Text Analysis for Content Management
- Connected Inventory
Learn more about Ontotext’s Applications
Learn more…
Provide consistent unified access to data across different systems by using the flexible and semantically precise structure of the knowledge graph model
- KG Applications Map
- Main Applications

Interlink your organization’s data and content by using knowledge graph powered natural language processing with our Content Management solutions.
- Showcase Demonstrators

Implement a Connected Inventory of enterprise data assets, based on a knowledge graph, to get business insights about the current status and trends, risk and opportunities, based on a holistic interrelated view of all enterprise assets.
- Typical Data Assets

- Ontotext Solutions
- Healthcare & Life Sciences
- Financial Services
- Media & Publishing
- Public Sector
Learn more about Ontotext’s Solutions
Quick and easy discovery in clinical trials, medical coding of patients’ records, advanced drug safety analytics, knowledge graph powered drug discovery, regulatory intelligence and many more
- Business Applications
- LinkedLife Data Inventory
- News, Events & Blog Posts
Make better sense of enterprise data and assets for competitive investment market intelligence, efficient connected inventory management, enhanced regulatory compliance and more
- GraphDB with FIBO Training

Connect and model industry systems and processes for deeper data-driven insights in:
- Manufacturing
- Automotive Industry
- Building Automation
- Infrastructure
- Aerospace & Defense

Improve engagement, discoverability and personalized recommendations for Financial and Business Media, Market Intelligence and Investment Information Agencies, Science, Technology and Medicine Publishers, etc.

Unlock the potential for new intelligent public services and applications for Government, Defence Intelligence, etc.
- Clients Services

Connect and improve the insights from your customer, product, delivery, and location data. Gain a deeper understanding of the relationships between products and your consumers’ intent.
- Ontotext Products
- Ontotext GraphDB
- Ontotext Platform
- Ontotext Metadata Studio
- Ontotext Refine
Learn more about Ontotext’s Products
Link diverse data, index it for semantic search and enrich it via text analysis to build big knowledge graphs.
Download GraphDB
- Release Notes
- Quick Start Guide
- Documentation
Organize your information and documents into enterprise knowledge graphs and make your data management and analytics work in synergy.
- Request a License
Integrate and evaluate any text analysis service on the market against your own ground truth data in a user friendly way.
- Get In Touch
- Installation
- Configuration
Turn strings to things with Ontotext’s free application for automating the conversion of messy string data into a knowledge graph.
- Download Now
- Data Loading
- RDF-izing Tabular Data
- Knowledge Hub
- Fundamentals
What is the Data, Information, Knowledge, Wisdom (DIKW) Pyramid?
The DIKW Pyramid represents the relationships between data, information, knowledge and wisdom. Each building block is a step towards a higher level - first comes data, then is information, next is knowledge and finally comes wisdom. Each step answers different questions about the initial data and adds value to it. The more we enrich our data with meaning and context, the more knowledge and insights we get out of it so we can take better, informed and data-based decisions.

Knowledge Pyramid, Wisdom Hierarchy and Information Hierarchy are some of the names referring to the popular representation of the relationships between data, information, knowledge and wisdom in the Data, Information, Knowledge, Wisdom (DIKW) Pyramid.
Like other hierarchy models, the Knowledge Pyramid has rigidly set building blocks – data comes first, information is next, then knowledge follows and finally wisdom is on the top.
Each step up the pyramid answers questions about the initial data and adds value to it. The more questions we answer, the higher we move up the pyramid. In other words, the more we enrich our data with meaning and context, the more knowledge and insights we get out of it. At the top of the pyramid, we have turned the knowledge and insights into a learning experience that guides our actions.
Climb up the DIKW pyramid with the help of Ontotext!
How to scale data up the knowledge pyramid.
So, let’s have a look at the individual components of the Knowledge Pyramid and how we move from one to the next.

Data is a collection of facts in a raw or unorganized form such as numbers or characters.
However, without context, data can mean little. For example, 12012012 is just a sequence of numbers without apparent importance. But if we view it in the context of ‘this is a date’, we can easily recognize 12th of January, 2012 . By adding context and value to the numbers, they now have more meaning.
In this way, we have transformed the raw sequence of numbers into
Information

Information is the next building block of the DIKW Pyramid. This is data that has been “cleaned” of errors and further processed in a way that makes it easier to measure, visualize and analyze for a specific purpose.
Depending on this purpose, data processing can involve different operations such as combining different sets of data (aggregation), ensuring that the collected data is relevant and accurate (validation), etc. For example, we can organize our data in a way that exposes relationships between various seemingly disparate and disconnected data points. More specifically, we can analyze the Dow Jones index performance by creating a graph of data points for a particular period of time, based on the data at each day’s closing.
By asking relevant questions about ‘who’, ‘what’, ‘when’, ‘where’, etc., we can derive valuable information from the data and make it more useful for us.
But when we get to the question of ‘how’, this is what makes the leap from information to
“How” is the information, derived from the collected data, relevant to our goals? “How” are the pieces of this information connected to other pieces to add more meaning and value? And, maybe most importantly, “how” can we apply the information to achieve our goal?
When we don’t just view information as a description of collected facts, but also understand how to apply it to achieve our goals, we turn it into knowledge. This knowledge is often the edge that enterprises have over their competitors. As we uncover relationships that are not explicitly stated as information, we get deeper insights that take us higher up the DIKW pyramid.
But only when we use the knowledge and insights gained from the information to take proactive decisions, we can say that we have reached the final – ‘wisdom’ – step of the Knowledge Pyramid.

Wisdom is the top of the DIKW hierarchy and to get there, we must answer questions such as ‘why do something’ and ‘what is best’. In other words, wisdom is knowledge applied in action.
We can also say that, if data and information are like a look back to the past, knowledge and wisdom are associated with what we do now and what we want to achieve in the future.
How Enterprises and Organizations Move Up the Knowledge Pyramid
One easy and fast way for enterprises to take the steps from data to information to knowledge and wisdom is to use Semantic Technologies such as Linked Data and Semantic Graph Databases . These technologies can create links between disparate and heterogeneous data and infer new knowledge out of existing facts.
Armed with this new knowledge, enterprises can climb up the mountain of wisdom and gain a competitive advantage by supporting their business decisions with data-driven analytics.
Learn how to climb up the DIKW pyramid with the help of Ontotext!
[schemaapprating]
Ontotext Newsletter

Want to create or adapt books like this? Learn more about how Pressbooks supports open publishing practices.
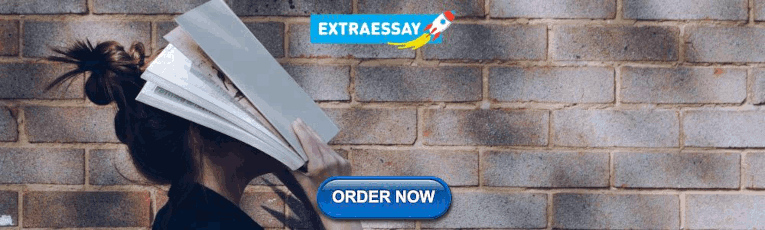
11.2 Data, Information, and Knowledge
Learning objectives.
After studying this section you should be able to do the following:
- Understand the difference between data and information.
- Know the key terms and technologies associated with data organization and management.
Data refers simply to raw facts and figures. Alone it tells you nothing. The real goal is to turn data into information . Data becomes information when it’s presented in a context so that it can answer a question or support decision making. And it’s when this information can be combined with a manager’s knowledge —their insight from experience and expertise—that stronger decisions can be made.
Trusting Your Data
The ability to look critically at data and assess its validity is a vital managerial skill. When decision makers are presented with wrong data, the results can be disastrous. And these problems can get amplified if bad data is fed to automated systems. As an example, look at the series of man-made and computer-triggered events that brought about a billion-dollar collapse in United Airlines stock.
In the wee hours one Sunday morning in September 2008, a single reader browsing back stories on the Orlando Sentinel ’s Web site viewed a 2002 article on the bankruptcy of United Airlines (UAL went bankrupt in 2002, but emerged from bankruptcy four years later). That lone Web surfer’s access of this story during such a low-traffic time was enough for the Sentinel ’s Web server to briefly list the article as one of the paper’s “most popular.” Google crawled the site and picked up this “popular” news item, feeding it into Google News.
Early that morning, a worker in a Florida investment firm came across the Google-fed story, assumed United had yet again filed for bankruptcy, then posted a summary on Bloomberg. Investors scanning Bloomberg jumped on what looked like a reputable early warning of another United bankruptcy, dumping UAL stock. Blame the computers again—the rapid plunge from these early trades caused automatic sell systems to kick in (event-triggered, computer-automated trading is responsible for about 30 percent of all stock trades). Once the machines took over, UAL dropped like a rock, falling from twelve to three dollars. That drop represented the vanishing of $1 billion in wealth, and all this because no one checked the date on a news story. Welcome to the new world of paying attention (Harvey, 2008)!
Understanding How Data Is Organized: Key Terms and Technologies
A database is simply a list (or more likely, several related lists) of data. Most organizations have several databases—perhaps even hundreds or thousands. And these various databases might be focused on any combination of functional areas (sales, product returns, inventory, payroll), geographical regions, or business units. Firms often create specialized databases for recording transactions, as well as databases that aggregate data from multiple sources in order to support reporting and analysis.
Databases are created, maintained, and manipulated using programs called database management systems (DBMS) , sometimes referred to as database software . DBMS products vary widely in scale and capabilities. They include the single-user, desktop versions of Microsoft Access or Filemaker Pro, Web-based offerings like Intuit QuickBase, and industrial strength products from Oracle, IBM (DB2), Sybase, Microsoft (SQL Server), and others. Oracle is the world’s largest database software vendor, and database software has meant big bucks for Oracle cofounder and CEO Larry Ellison. Ellison perennially ranks in the Top 10 of the Forbes 400 list of wealthiest Americans.
The acronym SQL (often pronounced sequel ) also shows up a lot when talking about databases. Structured query language (SQL) is by far the most common language for creating and manipulating databases. You’ll find variants of SQL inhabiting everything from lowly desktop software, to high-powered enterprise products. Microsoft’s high-end database is even called SQL Server. And of course there’s also the open source MySQL (whose stewardship now sits with Oracle as part of the firm’s purchase of Sun Microsystems). Given this popularity, if you’re going to learn one language for database use, SQL’s a pretty good choice. And for a little inspiration, visit Monster.com or another job site and search for jobs mentioning SQL. You’ll find page after page of listings, suggesting that while database systems have been good for Ellison, learning more about them might be pretty good for you, too.
Even if you don’t become a database programmer or database administrator (DBA) , you’re almost surely going to be called upon to dive in and use a database. You may even be asked to help identify your firm’s data requirements. It’s quite common for nontech employees to work on development teams with technical staff, defining business problems, outlining processes, setting requirements, and determining the kinds of data the firm will need to leverage. Database systems are powerful stuff, and can’t be avoided, so a bit of understanding will serve you well.
Figure 11.1 A Simplified Relational Database for a University Course Registration System
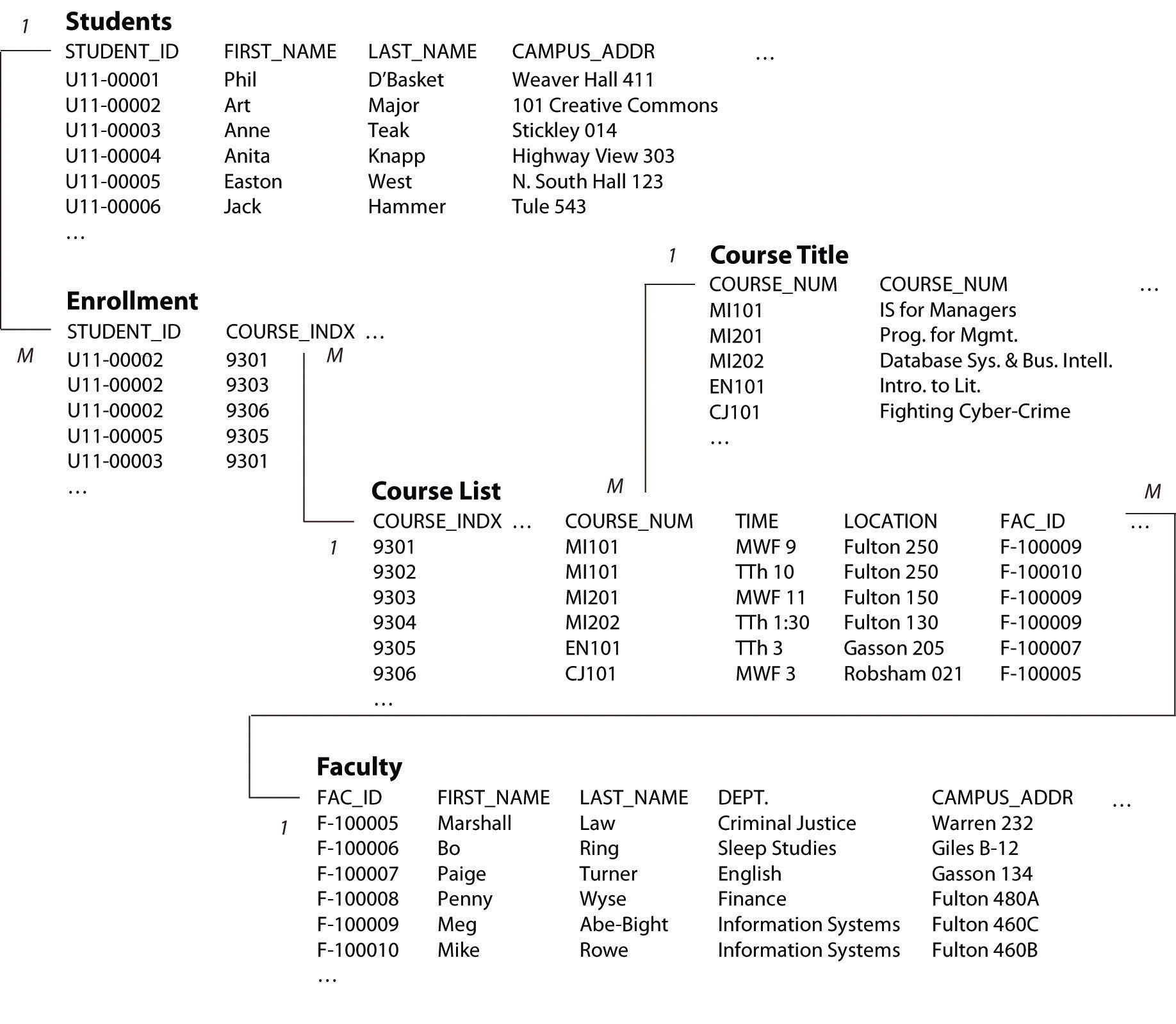
A complete discourse on technical concepts associated with database systems is beyond the scope of our managerial introduction, but here are some key concepts to help get you oriented, and that all managers should know.
- A table or file refers to a list of data.
- A database is either a single table or a collection of related tables. The course registration database above depicts five tables.
- A column or field defines the data that a table can hold. The “Students” table above shows columns for STUDENT_ID, FIRST_NAME, LAST_NAME, CAMPU.S._ADDR (the “…” symbols above are meant to indicate that in practice there may be more columns or rows than are shown in this simplified diagram).
- A row or record represents a single instance of whatever the table keeps track of. In the example above, each row of the “Students” table represents a student, each row of the “Enrollment” table represents the enrollment of a student in a particular course, and each row of the “Course List” represents a given section of each course offered by the University.
- A key is the field used to relate tables in a database. Look at how the STUDENT_ID key is used above. There is one unique STUDENT_ID for each student, but the STUDENT_ID may appear many times in the “Enrollment” table, indicating that each student may be enrolled in many classes. The “1” and “M” in the diagram above indicate the one to many relationships among the keys in these tables.
Databases organized like the one above, where multiple tables are related based on common keys, are referred to as relational databases . There are many other database formats (sporting names like hierarchical , and object-oriented ), but relational databases are far and away the most popular. And all SQL databases are relational databases.
We’ve just scratched the surface for a very basic introduction. Expect that a formal class in database systems will offer you far more detail and better design principles than are conveyed in the elementary example above. But you’re already well on your way!
Key Takeaways
- Data includes raw facts that must be turned into information in order to be useful and valuable.
- Databases are created, maintained, and manipulated using programs called database management systems (DBMS), sometimes referred to as database software.
- All data fields in the same database have unique names, several data fields make up a data record, multiple data records make up a table or data file, and one or more tables or data files make up a database.
- Relational databases are the most common database format.
Questions and Exercises
- Define the following terms: table, record, field. Provide another name for each term along with your definition.
Answer the following questions using the course registration database system, diagramed above:
- Imagine you also want to keep track of student majors. How would you do this? Would you modify an existing table? Would you add new tables? Why or why not?
- Why do you suppose the system needs a “Course Title” table?
- This database is simplified for our brief introduction. What additional data would you need to keep track of if this were a real course registration system? What changes would you make in the database above to account for these needs?
- Research to find additional examples of organizations that made bad decisions based on bad data. Report your examples to your class. What was the end result of the examples you’re citing (e.g., loss, damage, or other outcome)? What could managers have done to prevent problems in the cases that you cited? What role did technology play in the examples that you cite? What role did people or procedural issues play?
- Why is an understanding of database terms and technologies important, even for nontechnical managers and staff? Consider factors associated with both system use and system development. What other skills, beyond technology, may be important when engaged in data-driven decision making?
Information Systems Copyright © 2015 by University of Minnesota is licensed under a Creative Commons Attribution-NonCommercial-ShareAlike 4.0 International License , except where otherwise noted.
Data, information, and knowledge: What’s the difference?
This post was originally published on lessonly.com.
When it comes to knowledge management , all of the industry jargon can easily make your head spin. That’s why we’re going to break down the differences between data, information, and knowledge, the three main building blocks of any effective knowledge management process.
Although they’re closely linked, these terms are often considered interchangeable. But, there are also some important distinctions between them. Recognizing their differences and how they are all connected is the key to understanding how to build a successful knowledge management framework.
So let’s dive straight in.
Data is the starting point of knowledge management and can be best linked to an organization’s raw materials. According to Datarob , data encompasses “plain facts, observations, statistics, characters, symbols, images, numbers, and more that are collected and can be used for analysis.”
There are two types of data: quantitative and qualitative.
Quantitative data is quantifiable, in other words, it is easily measurable as it is numerical by nature. The total revenue a company made in 2020, how often users are clicking on a link, the combined amount of paid leave employees took in any given month – these figures are all examples of quantitative data.
Qualitative data, on the other hand, cannot be measured, as it’s descriptive and linguistic rather than numerical. This type of data includes conversations, observations, and feedback, such as a customer’s conveyed experience using a service, or how employees feel about recent changes implemented in their company.
An isolated piece of data, whether it’s a number or a remark from a customer, can be considered meaningless in itself— it needs to be put in context in order to be useful to an individual or an organization.
Information
So, data vs. information, what’s the difference? Information is a collection of data that has been processed, refined, structured, and/or presented to create relevance and usefulness.
A sequence of prices— $10, $8, $12.50—mean nothing by themselves. It’s only when they’ve been gathered and analyzed that it can be determined that they reflect the range of amounts people in a focus group said they were willing to pay for a particular product.
While data is a stand-alone concept, information doesn’t exist without it. In fact, data is the foundation of information. To turn data into information, organizations use a variety of knowledge management systems , software, and tools. This includes databases, spreadsheets, contact details, key dates, documents, guidelines, strategies, and the list goes on. And since this data has been directed into a recognizable and digestible order, it’s now become information.
Just as information generates relevance from data, knowledge makes meaning from information. When information is analyzed in order to generate insights, draw conclusions, make predictions, and drive change, knowledge is created. What sets knowledge apart from information is that it also is made up of other elements such as experience and intuition. In other words, information is often referred to as the who, what, where, when, and why, but knowledge is more focused on the how.
Organizational knowledge is crucial to a business’s success because it adds a competitive edge. Any company can collect data and process this into information. But, what happens to that information is what can take a company to the next level.
The relationship between data, information, and knowledge is often linked to a pyramid structure, known as the knowledge hierarchy . This concept positions data at the bottom, information in the middle tier, and knowledge at the top, where each rung of the pyramid has gained an extra level of value than the stage before it.
The ultimate goal is that knowledge management tools and processes turn data to information, and then to knowledge, which then is channeled into action. Action can be anything from a change in tactic, a decision being taken, or even a learning experience for an employee or team.
Here at Lessonly, we like to think of the relationship between information and knowledge management not as a pyramid, but more like a cake. Yes, that’s right. Hear us out.
Let’s say data is a cake’s ingredients. A raw egg, a packet of flour, or a teaspoon of baking soda are next to useless by themselves. These individual items gain relevance when they’re combined with the other ingredients on the list, which is like information, where the data has been put into context. Yet, a list of ingredients alone still does not make a cake.
Knowledge is therefore the recipe, where the ingredients list has been transformed into an expertly considered formulation and sequence that results in a valuable outcome (a delicious triple-layered victoria sponge, for example).
Going one step further with this metaphor, almost anybody equipped with a recipe can make a half-decent cake (we hope). Still, an expert baker also taps into their learned experience and intuition to kick their dessert up a notch.
We like to think about data, information, and knowledge in this way, as it means they aren’t measured against each other in a hierarchical structure but regarded as working in tandem towards the desired outcome.
So, why do these definitions matter?
Why should we care about all this jargon anyway? Here’s why.
We’re living in a knowledge-intensive era. Businesses are investing heavily into driving their data and information into “ actionable insights ”, another popular buzzword which is when gained knowledge leads to meaningful action and improvement of your company and its team.
But misunderstandings on the nature of data, information, and knowledge, and how they are interlinked, are leading businesses to fall short of their goals. Many companies mistakenly drown their systems with more and more data and information in a “throw everything at the wall and see what sticks” approach, without considering that generating knowledge actually requires an active and skillful process.
These issues stem from the misconception that information equates to knowledge when this isn’t the case. Just because an employee may have a heap of information about a given subject, this doesn’t mean by default they have the experience and intuition to come to conclusions, value judgments, or informed decisions. That, our friends, is knowledge.
And, even when valuable knowledge is flowing throughout an organization in abundance, businesses’ need a successful knowledge management framework to capture and optimize it. Without that, knowledge runs the risk of not reaching the right people at the right time or isn’t packaged in a way that makes employees understand its use and value to them, getting lost in translation. This is why knowledge management in organizations is so crucial.
After all, knowledge without management is like an ambitious goal without a plan: a missed opportunity.
PROFESSIONAL SERVICES
Expert advisors, at your service
SUBSCRIPTION
Subscribe to our newsletter
Thank you for subscribing
The complete guide to enablement
SEISMIC ROI CALCULATOR
Grow like the sky's the limit.
The 2023 Value of Enablement Report
- Data Science
- Data Analysis
- Data Visualization
- Machine Learning
- Deep Learning
- Computer Vision
- Artificial Intelligence
- AI ML DS Interview Series
- AI ML DS Projects series
- Data Engineering
- Web Scrapping
DIKW Pyramid | Data, Information, Knowledge and Wisdom | Data Science and Big Data Analytics
- Difference between Big Data and Data Analytics
- Difference Between Data Visualization and Data Analytics
- Difference Between Big Data and Predictive Analytics
- Does a Data Scientist/Machine Learning Engineer require in depth knowledge of Data Structures and Algorithms?
- Difference Between Data Science and Business Analytics
- Difference between Cloud Computing and Big Data Analytics
- Difference Between Data Analytics and Predictive Analytics
- Difference Between Big Data and Data Science
- Difference Between Data Science and Data Engineering
- How Do Companies Use Big Data Analytics in Real World?
- Data Preprocessing, Analysis, and Visualization for building a Machine learning model
- Difference between Data Scientist and Data Engineer
- Difference Between Data Science and Data Mining
- How to Use Bard for Data Analysis and Insights
- Power BI - Rise of Microsoft Power BI as a Data Analytics powerhouse
- Does Dark Data Have Any Worth In The Big Data World?
- Difference Between Small Data and Big Data
- Difference Between Data Science and Data Visualization
- Data Science vs Data Analytics
The term DIKW is derived from the field of “data science and big data analytics”. The DIKW model is used for data enrichment. The DIKW model consists of four stages. The full form of every alphabet in the word DIKW has its own meaning. In DIKW, D stands for “Data”, I stands for “Information”, K stands for “Knowledge” and W stands for “Wisdom”. The flow of the DIKW pyramid starts from bottom to up and also with increasing values such as hindsight, insight, and foresight.
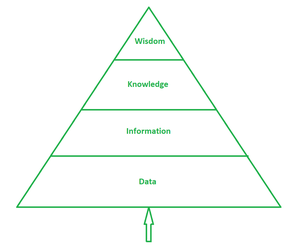
DIKW Pyramid
The DIKW pyramid is a hierarchical model that represents the relationship between different levels of information processing, from raw data to knowledge and wisdom. It is often used in the context of data science and big data analytics to illustrate the transformation of data into actionable insights.
The four levels of the DIKW pyramid are:
- Data: This is the raw, unprocessed facts and figures that are collected from various sources. Data can be structured or unstructured and may include text, numbers, images, audio, and video.
- Information: Data becomes information when it is organized, processed, and interpreted in a meaningful way. The information provides context and relevance to data and enables decision-making and action.
- Knowledge: Knowledge is the understanding gained from information, through analysis, interpretation, and synthesis. Knowledge is often based on experience, expertise, and intuition, and enables more complex decision-making and problem-solving.
- Wisdom: Wisdom is the highest level of the DIKW pyramid, representing the ability to apply knowledge and experience to make sound judgments and decisions. Wisdom requires reflection, insight, and foresight, and is often based on a deep understanding of the broader context and implications of decisions.
In the context of data science and big data analytics, the DIKW pyramid illustrates the process of transforming raw data into actionable insights. Data is collected, processed, and analyzed to generate information, which is then used to develop knowledge and wisdom. By leveraging advanced analytics techniques and technologies, organizations can extract valuable insights from their data and use them to improve their operations, products, and services. The DIKW pyramid is a hierarchical model that illustrates the relationship between four levels of information processing: Data, Information, Knowledge, and Wisdom.
Please Login to comment...
Similar reads, improve your coding skills with practice.
What kind of Experience do you want to share?
Academia.edu no longer supports Internet Explorer.
To browse Academia.edu and the wider internet faster and more securely, please take a few seconds to upgrade your browser .
Enter the email address you signed up with and we'll email you a reset link.
- We're Hiring!
- Help Center
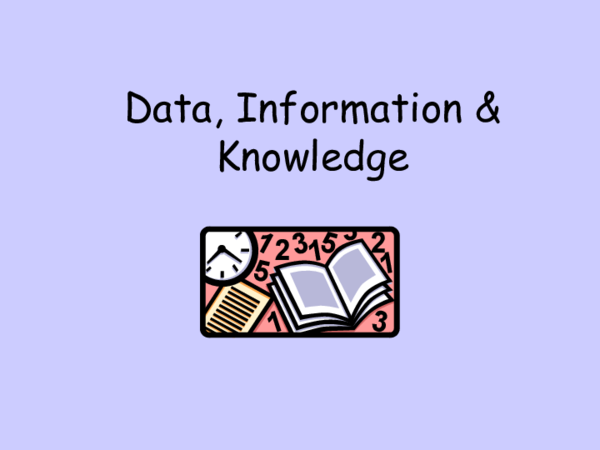
Data, Information and Knowledge

Related Papers
Moffat Bokamoso Jackson
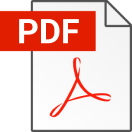
Elder Clair Rudison
Data: Data is nothing but raw facts. The word raw indicates that the facts have not yet been processed to reveal their meaning. For example, suppose that you want to know the number of users of a computer lab think of its services. Typically, you would begin by surveying users to assess the computer lab's performance Refer Figure 1.1 below, Panel A, shows the Web survey form that enables users to respond to your questions. When the survey form has been completed, the form's raw data are saved to a data repository, such as the one shown in below Figure 1.1, Panel B. Although you now have the facts in hand, they are not particularly useful in this format-reading page after page of zeros and ones is not likely to provide much clear information. Therefore, you need transform the raw data into a data summary like the one shown in below Figure 1.1, Panel C. Now it's possible to get quick answers to questions such as "What is the composition of our lab's customer base?" In this case, you can quickly determine that most of your customers are juniors (24.59%) and seniors (53.01%). Because graphics can enhance your ability to quickly extract meaning from data, you show the data summary bar graph in Figure 1.1 below, Panel D. Information: Information is the result of processing raw data to reveal its meaning.Data processing can be simple and it can also be complex. Data processing can be as simple as organizing data to reveal patterns or It can be as complex as making forecasts or drawing inferences using statistical modeling. Information can be used as the foundation for decision making. For example, the data summary for each question on the survey form can point out the lab's strengths and weaknesses, helping us to take decisions and also to better meet the needs of lab customers. So the raw data must be properly formatted for storage, processing, and presentation. In this "information age" production of accurate, relevant, and timely information is the key to good decision making.In turn, good decision making is the key to business survival in a global market.
Valdemar W. Setzer
Corey Anton
The field of Information Science is constantly changing. Therefore, information scientists are required to regularly review and if necessary redefine its fundamental building blocks. This article is one of a group of four articles, which resulted from a Critical Delphi study conducted in 2003-2005. The study, Knowledge Map of Information Science, was aimed at exploring the foundations of information science. The international panel was composed of 57 leading scholars from 16 countries, who represent (almost) all the major subfields and important aspects of the field. This particular article documents 130 definitions of data, information, and knowledge formulated by 45 scholars, and maps the major conceptual approaches for defining these three key concepts.
Lopez Mason
There is a subtle difference between data and information. Data are the facts or details from which information is derived. Individual pieces of data are rarely useful alone. For data to become information, data needs to be put into context. Comparison chart Data versus Information comparison chart Data Information Meaning Data is raw, unorganized facts that need to be processed. Data can be something simple and seemingly random and useless until it is organized. When data is processed, organized, structured or presented in a given context so as to make it useful, it is called information. Example Each student's test score is one piece of data. The average score of a class or of the entire school is information that can be derived from the given data. Etymolog y "Data" comes from a singular Latin word, datum, which originally meant "something given." Its early usage dates back to the 1600s. Over time "data" has become the plural of datum. "Information" is an older word that dates back to the 1300s and has Old French and Middle English origins. It has always referred to "the act of informing, " usually in regard to education, instruction, or other knowledge communication. Data vs. Information-Differences in Meaning "The numbers have no way of speaking for themselves. We speak for them. We imbue them with meaning."-Statistician Nate Silver (Links to an external site.)Links to an external site. in the book The Signal and the NoiseLinks to an external site. Data are simply facts or figures-bits of information, but not information itself. When data are processed, interpreted, organized, structured or presented so as to make them meaningful or useful, they are called information. Information provides context for data. For example, a list of dates-data-is meaningless without the information that makes the dates relevant (dates of holiday). "Data" and "information" are intricately tied together, whether one is recognizing them as two separate words or using them interchangeably, as is common today. Whether they are used interchangeably depends somewhat on the usage of "data"-its context and grammar (Links to an external site.)Links to an external site.. Examples of Data and Information • The history of temperature readings all over the world for the past 100 years is data. If this data is organized and analyzed to find that global temperature is rising, then that is information. • The number of visitors to a website by country is an example of data. Finding out that traffic from the U.S. is increasing while that from Australia is decreasing is meaningful information. • Often data is required to back up a claim or conclusion (information) derived or deduced (Links to an external site.)Links to an external site. from it. For example, before a drug is approved by the FDA, the manufacturer must conduct clinical trials and present a lot of data to demonstrate that the drug is safe.
Tatenda A. Chimusoro
Luciano Floridi
RELATED TOPICS
- We're Hiring!
- Help Center
- Find new research papers in:
- Health Sciences
- Earth Sciences
- Cognitive Science
- Mathematics
- Computer Science
- Academia ©2024

An official website of the United States government
The .gov means it’s official. Federal government websites often end in .gov or .mil. Before sharing sensitive information, make sure you’re on a federal government site.
The site is secure. The https:// ensures that you are connecting to the official website and that any information you provide is encrypted and transmitted securely.
- Publications
- Account settings
Preview improvements coming to the PMC website in October 2024. Learn More or Try it out now .
- Advanced Search
- Journal List
- HHS Author Manuscripts

Transforming clinical data into wisdom
Kenrick d. cato.
Columbia University School of Nursing in New York, NY.
Kathleen McGrow
Microsoft Corporation in Redmond, Wash.
Sarah Collins Rossetti
biomedical informatics and nursing at Columbia University in New York, NY.
Artificial intelligence (AI) is part computer science and part cognitive science, encompassing the phenomena of computers performing tasks that require human intelligence. 1 Current interest in AI is motivated, in part, by recent developments in machine learning, in which algorithms learn from data without human direction. 2 Machine learning is a group of different mathematical methods to build AI rules. Each of these methods has its strengths and weaknesses based on the types of data being analyzed. Some machine learning approaches, such as speech recognition when talking into the speaker of a smart phone or smart home device, are now mainstream.
Although we’ve been on a journey for decades to augment human capabilities, recently there’s been a dramatic rise in AI research in healthcare. AI has been brought to the forefront due to multiple factors, including the tremendous amounts of data that are captured and stored through electronic health records (EHRs), more availability of computer power, and significant innovations in AI tools and algorithms. The increase in AI availability and use in healthcare makes it imperative that both nurse leaders and nurses have a fundamental understanding of AI when used in conjunction with clinical models, critical-thinking skills, and evidence-based practice.
Graves and Corcoran identified the conceptual model that’s pivotal to nursing informatics known as the data, information, knowledge, wisdom (DIKW) framework. 3 This framework has subsequently been used to define how nursing informatics impacts nursing practice and care delivery, and it aligns well with the American Nurses Association Nursing Informatics: Scope and Standards of Practice, which identifies nursing informaticists as assisting in the development of wisdom for all nurses. 4 In addition to the use of knowledge, wisdom includes decision-making and applying nursing actions through the applicable use of data, information, and knowledge. 5 , 6 In the healthcare setting, AI is often paired with a clinical decision support (CDS) application to move from knowledge to wisdom, which involves exercising judgment. 7 The ability to use AI-assisted wisdom to solve problems and identify solutions is key for nurses to enable the delivery of nursing care that’s more precise and efficient, benefiting both nurses and patients. 8
In this article, we explain the DIKW framework, review key concepts of AI and CDS, offer examples of real-world AI/CDS applications within the DIKW framework and how they can be used, and identify relevant questions to ask when investigating AI for use in clinical and operational scenarios.
DIKW, AI, and CDS
The DIKW framework explains that data leads to information, information to knowledge, and knowledge to wisdom. 9 This framework is particularly relevant when designing CDS tools to advance nursing practice to top of licensure for improved nurse decision-making. The DIKW framework can be used to categorize CDS tools, including those that are driven by AI. This framework is pyramidal, which implies that data are profuse and wisdom is rare. As defined in previous publications discussing this framework, data have little or no meaning in isolation, information is data plus meaning, knowledge is derived by discovering patterns and relationships between types of information, and wisdom is understanding and internalization of knowledge patterns and relationships. (See Table 1 .) 10 The DIKW framework isn’t just a way to understand how information can be processed; it’s also a means by which nurse leaders can strategically evaluate the relationship between AI and CDS.
Data-Information-Knowledge-Wisdom Conceptual Framework (DIKW) Definitions 5 , 11
Concept | Definition |
---|---|
Wisdom | Understanding and internalization |
Knowledge | Derived by discovering patterns and relationships between types of information |
Information | Data plus meaning |
Data | Little or no meaning in isolation |
AI generally refers to “machines that respond to stimulation consistent with traditional responses from humans, given the human capacity for contemplation, judgment, and intention...[these machines] make decisions which normally require human level of expertise.” 11 West and Allen describe three characteristics of these systems, which “operate in an intentional, intelligent, and adaptive manner.” 12 Intentionality is defined as algorithms designed to make decisions, typically using real-time data. 12 Intelligent systems use underlying trends or patterns to inform decision-making. 12 And an adaptive manner includes the ability to learn and improve decisions based on new information or pattern recognition. 12 In the healthcare setting, AI is used with analytics to support CDS, improve operational efficiency, and for general cost effectiveness. 13
CDS and AI aren’t one in the same. CDS provides clinicians with computer-generated clinical knowledge and patient-related information that’s intelligently filtered and presented at appropriate times to enhance patient care. 14 In other words, AI can be thought of as a fuel to power more intelligent CDS. For example, imagine having a CDS alert for nurses to help them manage a patient with diabetes based on information from tens of thousands of patients and other nurses in a similar situation. Even though CDS interventions can be used to implement AI at the point of care, they don’t always leverage AI. In fact, a 2017 integrative review identified that only 25% of CDS targeting nurses uses real-time EHR data. 15
An analysis of requests for development of CDS targeted at nurses identified that the majority of requests were information and knowledge related when plotted along the DIKW framework. 16 There’s untapped potential for use of AI within CDS interventions that target risk assessment/risk reduction activities within the nursing process. 16 These areas include AI-based CDS interventions that align with the wisdom concept from the DIKW framework and use, for example, predictive analytics, personalization of information presentation, patient preferences, and social and behavioral determinants of health to guide nurses’ understanding and internalization of knowledge patterns and relationships. 16
Targeted CDS nursing interventions shouldn’t stop at the planning stage of the nursing process as many do currently. 16 To impart wisdom--understanding and internalization of knowledge patterns and relationships--AI designed for nurses should be intentionally designed to use real-time data to recommend decisions, have intelligent recognition of patterns within nursing and patient care workflows and processes, and be adaptive by aligning with the cyclical nature of workflow activities in the nursing process.
In healthcare, there’s been an increase in AI/CDS-based tools. Some of these offerings don’t solve critical nursing decision support needs. However, there are AI/CDS tools that nurse leaders can benefit from; for example, tools that provide predictive analytics for staffing and bed management, to name a few. The DIKW model can help leaders understand which of these AI/CDS tools will be useful in their organization. Figure 1 illustrates the hierarchical nature of AI/CDS applications in which there are data capture, data processing to meet information needs, clinical knowledge-based rules to guide decision-making, and exposed analytics in the form of wisdom to augment nurse decision-making. 16

DIKW strategic plan
From a clinical user perspective, the bottom three levels of the application usually aren’t visible. The user often sees the top, fourth level in the form of an alert, dashboard, risk score, app, or other interface. However, when investigating AI/CDS applications, it’s essential to understand how all of the levels were developed and validated.
Real-world examples
There are numerous examples of AI/CDS applications for nurses, such as the AI-based sepsis CDS alerts used in many EHRs today. 17 – 22 There are also newer AI/CDS tools, such as the Communicating Narrative Concerns Entered by RNs (CONCERN) CDS system. CONCERN is an AI/CDS tool that predicts a patient’s risk of clinical deterioration, which is currently being used at Partners Health System, with a planned rolled out at NewYork-Presbyterian Hospital later this year. 23 Both the sepsis CDS tool and the CONCERN CDS system illustrate how the DIKW approach can be used by nurse leaders to determine if these technologies make sense in their clinical setting.
A number of different types of AI rules have been built to trigger sepsis CDS alerts. 24 The data that most of these sepsis alerts use are vital signs and lab results. Machine learning is then used to build AI-based rules to predict patients who are at risk for sepsis. The information generated is the meaning of different types of lab results and vital signs, and the knowledge is the predicted increased risk. The current sepsis CDS alerts don’t reach the wisdom level for two reasons. First, because the sepsis alerts are delivered by the EHR, there’s a false alarm or fatigue alert issue. This situation happens because no AI is perfect; many patients receive the sepsis alert, disrupting the nurse’s regular workflow when the patient clearly isn’t at risk for sepsis. Eventually, nurses get tired or fatigued by these alert-based disruptions and ignore the CDS altogether. Second, most sepsis alerts trigger 4 hours before it’s clinically clear that the patient is significantly deteriorating. 24 This doesn’t allow clinicians much time to intervene and effectively treat the patient.
The CONCERN CDS identifies nursing documentation patterns that are a proxy of a nurse’s concern for patient deterioration and generates a predictive early warning score. The CONCERN CDS consists of two main components: the decision engine, which uses AI models to analyze the content and patterns of nursing EHR data (such as flowsheet entries and nursing notes), and front-end interfaces that display the predicted CONCERN levels (green, yellow, red), with red indicating that a patient is at the highest risk for deterioration. A web application provides tracking of the CONCERN level over time and facilitates transparency of the AI models by displaying what factors contributed most to the current calculation.
The result of more than 8 years of qualitative and quantitative research involving clinician input at every stage, the CONCERN CDS is built with the DIKW framework in mind. Data capture is automatic from the EHR, with the AI model using data entered during the normal nursing workflow. The nursing data used include patterns and content of nursing notes, medications administration record information, and flowsheet entries. The system continuously monitors data inputs from patterns in nursing documentation and flowsheets to model performance. Alerting tools let the programmers who maintain the system know when expected data feeds are missing or the model output is different than expected.
Information generation is based on focus group and simulation testing with nurses and physicians. An example of knowledge representation is the green, yellow, red scoring system that indicates the patient’s risk of deterioration. When the CONCERN CDS was being developed, nurses and physicians indicated in focus group and simulation testing that colored levels for risk of deterioration would be the most clinically meaningful. Also, the setting-specific (acute care versus ICU) CONCERN AI models are optimized to identify patients who score in the yellow category. Clinicians reported that patients at the lowest and highest risk for deterioration are much easier to identify. Patients at the yellow level are at increased risk for clinical deterioration but haven’t started showing signs yet. Figure 2 shows a sample patient list in which users can quickly see a patient’s CONCERN level in their workflow.

Sample patient list
The CONCERN CDS is designed to augment clinical expertise instead of replacing it. Figure 3 shows the main CONCERN web application, which opens when a clinician clicks on a CONCERN level in the patient list. The main decision support part of the CONCERN CDS system is focused on visualizing trends in patient risk. The factors portion of the CONCERN CDS provides AI transparency, explaining what elements of the patient record contributed to the risk calculation. Additionally, a major goal of CONCERN CDS system is to support interdisciplinary communication by providing the relevant information (notes, medications administered, flowsheet entries) for patients at increased risk for deterioration in a centralized, accessible location many hours before the patient crashes. The CONCERN score has been shown to trigger up to 24 hours before a clear deterioration event.

CONCERN web application
Questions for nurse leaders to ask
With the abundance of data inside and outside of the EHR, AI/CDS applications are being used more frequently by nurses to provide care for patients. 25 However, research indicates that the success of new AI/CDS systems requires careful planning, evaluation, and decision-making. 15 , 26 – 28 AI/CDS applications are often presented as solutions to pressing clinical problems. The DIKW framework can help nurse leaders organize strategic planning and prioritization of potential AI/CDS applications, helping connect clinical practice with informatics solutions and revealing potential nursing practice or informatics gaps for proposed CDS. 16 Table 2 describes a number of questions based on the DIKW levels that nurse leaders should ask about AI/CDS systems.
Questions that a Nurse leader should ask about potential AI/CDS tools
DIKW level | Question | Explanation |
---|---|---|
What data is used in the AI/CDS tool? | It is important to understand the required datasources. Also, what types of transformations that are done on the data that might change it’s clinical meaning. For example, what are the cutoffs for how results are categorized? | |
How is it captured? Does this data capture fit into the existing clinical workflow? | It is important to know if data capture will add any additional burden to clinicians. Also, will clinicians workflow be impacted by required data capture? | |
Is there an appropriate life cycle plan for the CDS? | Data capture is dynamic, with continuous changes in the configuration. For example, forms in the EHR will be updated, created, or retired. Is there a similar life cycle plan for the CDS. How will the CDS handle EHR changes? Is there de-implementation planning? | |
Does the AI/CDS information take into account the clinical context? | The CDS should be agile enough to adapt to changes in clinical settings. For example, the same models should not be applied across settings where workflow and policies are different, for example acute care versus intensive care settings. | |
Does the information produced make clinical sense and have clinical relevance? | You should ensure that clinicians review the decision support and validate the clinical appropriateness and relevance of the CDS recommendations. | |
Does the AI/CDS help to solve a clinical problem. What were the examples that were used to teach the model? | For, AI/CDS, the products of the model are only as good as the data and information that helped create it. | |
Does the clinical decision support fit nursing processes? | Effective CDS should adhere to nursing science and processes. | |
Is the AI/CDS augmenting or taking over decision making? | The goal of effective AI/CDS should be to aid in decision making not replace the clinician particiapation. | |
Is the AI explainable to the clinician? | AI/CDS will not be effective if clinicians don’t understand how the recommendation are produced. | |
Is the required short-term and long-term training in place? | Continuous and effective training is required to be confident that AI/CDS users know what recommendations represent and how to use them in their clinical practice? – |
Strategic implementation
AI-powered CDS tools offer great promise in helping nurses to provide higher quality, safer, and more efficient care. It’s imperative that nurse leaders understand the key concepts of AI and CDS. The DIKW framework and relevant questions support nurse leaders in thinking strategically about current and future implementation of AI/CDS applications.
The CONCERN AI/CDS: An Example
There are a number of exciting examples of these new AI/CDS applications, among them is the Communicating Narrative Concerns Entered by RNs clinical decision support CONCERN CDS system. The CONCERN CDS is an AI/CDS that uses technology that has identified nursing documentation patterns that are a proxy of a nurses concern for deterioration and includes these proxies as features in a predictive early warning score. The CONCERN CDS is consists of two main components. First, the decision engine that uses AI models to analyze the content and patterns of nursing EHR data (e.g. flowsheet entries and nursing notes ). Second, front-end interfaces display the predicted CONCERN levels (green, yellow, red), with red indicating a patient is at highest risk for deterioration. A web application provides tracking of the CONERN level over time and facilitates transparency of the AI models by displaying which factors contributed most to the current calculation.
Acknowledgments
This study was funded by NINR 1R01NR016941-01, Communicating Narrative Concerns Entered by RNs (CONCERN): Clinical Decision Support Communication for Risky Patient States. The content is solely the responsibility of the authors and does not necessarily represent the official views of NIH. The authors have disclosed no financial relationships related to this article.
Contributor Information
Kenrick D. Cato, Columbia University School of Nursing in New York, NY.
Kathleen McGrow, Microsoft Corporation in Redmond, Wash.
Sarah Collins Rossetti, biomedical informatics and nursing at Columbia University in New York, NY.
- Database management
information
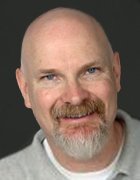
- Robert Sheldon
What is information?
Information is the output that results from analyzing, contextualizing, structuring, interpreting or in other ways processing data. Information infuses meaning and value into the data. It facilitates understanding, communication and learning, and is a key factor in system designs and strategic planning , as well as in problem-solving and decision-making. Information brings context to the data, turning what would otherwise be meaningless content into something comprehensible and usable.
Information has been defined in many ways over the years, and the definitions are not always consistent with each other. For example, one Merriam-Webster definition describes information as "knowledge obtained from investigation, study or instruction." However, the Dictionary of Information Science and Technology, written by Carolyn Watters, defines information as "meaning assigned to data within some context for the use of that data." And the National Institute of Standards and Technology ( NIST ) describes information as "any communication or representation of knowledge such as facts, data or opinions in any medium or form, including textual, numerical, graphic, cartographic, narrative or audiovisual."
Despite the varied definitions, there is still a general consensus that information is different from raw data and that it can be said to bring meaning to the data. Information can also come from many sources, including books, magazines, letters, text messages, emails, websites, classrooms, social networking sites, oral presentations, movies, and audio recordings.
What is the difference between information and data?
The terms data and information are often used interchangeably, but there are important differences between them. Data is made up of raw facts and figures. It is unprocessed and without context. It can include statistics, observations, measures or symbols. For example, a data set might contain dates, ages, inventory levels, eye colors, temperature readings, sports scores or an assortment of other facts.
In the context of computing and information technology ( IT ), data can be thought of as unprocessed electronic content that a software application collects and records. This type of data is typically stored in files , databases or other structured systems. The data can be accessed and manipulated digitally and transferred between systems and across networks.
Digital data is collected from a variety of sources, such as computers, sensors , monitors, smartphones and internet of things ( IoT ) devices. Data is used extensively in business, government, science and engineering, although consumers also store and exchange ample amounts of data. Data can also come in many forms, including numbers, text, videos, graphics and sounds.
Regardless of the source, data on its own has little meaning. For example, a data set might include a list of trees and their ages across multiple geographic regions. Without some type of context, the details are relatively meaningless. However, when the data set is contextualized, it can reveal important information about tree life expectancy, regional differences or environmental patterns. Data provides the necessary facts, but information brings understanding to those facts.
Information is created when data is presented in a way that has meaning to the recipient. To turn data into information, it must be processed and organized. Presenting data in a way that has meaning and value is called information design, which is an important field in both information architecture and human-computer interaction.
The 6 types of information
Information can be categorized in different ways, such as by source, quality or topic. One method that is often used was first proposed in the 1970s by J. H. Shera, a noted librarian and information scientist who identified six categories of information:
- Conceptual. Information that is based on ideas, concepts, theories, hypotheses and other abstract notions or beliefs.
- Empirical . Information that is obtained through observation, experimentation and other verifiable methodologies.
- Procedural. Information that describes how to carry out a procedure or that someone already possesses through knowing how to perform a task.
- Policy. Information that is related to laws, regulations, rules, guidelines, policies and other types of oversights whose purpose is to inform and enable more effective decision-making.
- Directive. Information that provides directions or descriptions to individuals or groups so they can better understand concepts or circumstances.
- Stimulatory. Information that is intended to provoke a response, stimulate a reaction or in other ways motivate individuals or groups to take action.
Another way that information might be categorized is by whether it is factual or analytical . Factual information is based only on known and proven facts, while analytical information is the interpretation of factual data. It considers what is inferred or implied by the data by applying methods of reason or deduction.
Information might also be categorized by whether it is subjective or objective. Subjective information is based on someone's point of view, such as the type of information conveyed through opinions. In contrast, objective information is, in theory, based on facts and free from bias, although there is some debate about whether any knowledge can ever be truly objective.
The data-information-knowledge-wisdom pyramid
The data-information-knowledge-wisdom model illustrates the progression from raw data to wisdom, where insights lead to effective data-driven decisions. Structured as a pyramid, the model was created to show how data can be captured in one form, analyzed and converted into another form. The pyramid includes four levels: data, information, knowledge and wisdom. Each level represents a different perspective or layer of abstraction, as follows:
- Data. The discrete, raw facts about a given situation are collected, but no analysis or interpretation is applied. This level sits at the bottom of the pyramid.
- Information. Structure and meaning are applied to the collected data to make it useful. This layer sits above the data layer in the pyramid.
- Knowledge. Insight, context and a frame of reference are applied to the information so it can be interpreted. This layer sits above the information layer in the pyramid.
- Wisdom. Knowledge is converted into wisdom by applying judgment and action to the information. This level sits at the top of the pyramid.
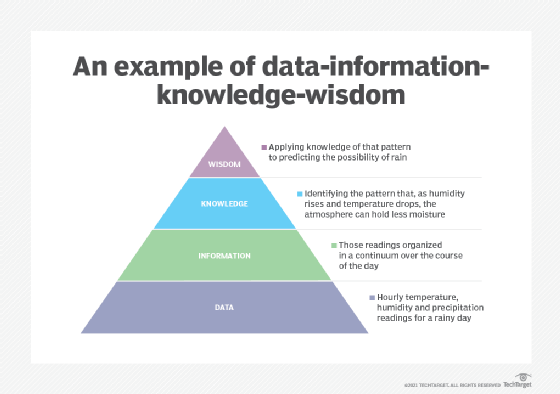
Knowledge is information that has been processed, analyzed and interpreted so it can be used to make decisions. The concept of knowledge involves not just the information, but also the ability to access it. For example, most applications, including models and simulations , contain a form of stored knowledge.
Wisdom is the synthesis of information, knowledge and experience in a way that applies knowledge to real-life situations. The concept of wisdom enables the understanding of patterns and their driving factors. It is also instrumental in predicting future events.
Artificial intelligence ( AI ) has enabled computers to learn, problem-solve and perform tasks that usually require human intelligence. These technologies enable computers to carry out operations based on what the data indicates is the best course of action. AI is used in advanced systems to diagnose disease, buy and sell stocks, and play chess better than a human. However, AI has not yet attained a level of human wisdom.
AI terms are often used interchangeably, but they are not the same. Understand the difference between artificial intelligence, machine learning and deep learning . Also, learn how AI technology is evolving to combine symbolic reasoning and deep learning to capitalize on the power of neural networks.
Continue Reading About information
- Content management vs. knowledge management: What are the differences?
- Treating data as a product a method to grow analytics use
- How to analyze data for better business decisions
- Top business benefits of real-time data analytics
- How generative AI can improve knowledge management
Related Terms
Dig deeper on database management.
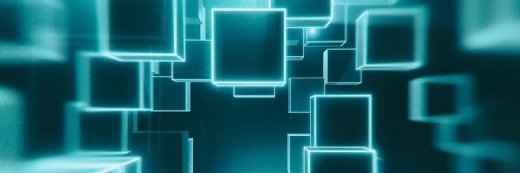
Understanding and implementing the software testing pyramid
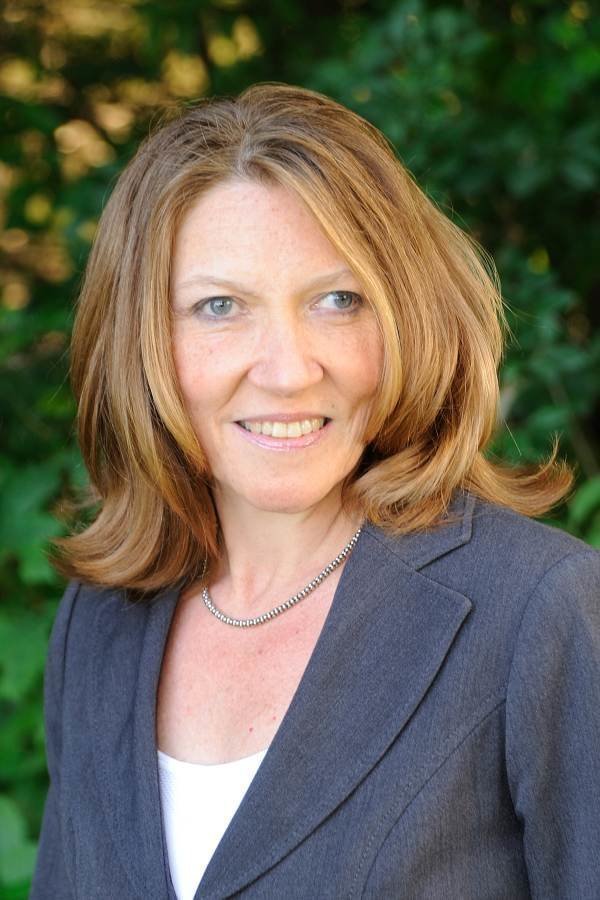
Pyramid Analytics launches integration with OpenAI
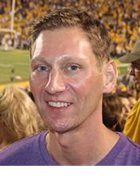
What is an octothorpe? Cool tech words and computer science slang
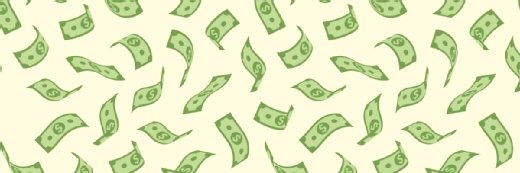
Pyramid Analytics raises $120M, IPO could be next
With trusted data as a foundation, the longtime analytics and data integration vendor has been pragmatic in its creation of an ...
The longtime analytics vendor's latest new features include data integration capabilities targeting data quality and a GenAI ...
The analytics and data integration vendor is focused on providing users with a foundation of trusted data as it develops an ...
Many organizations struggle to manage their vast collection of AWS accounts, but Control Tower can help. The service automates ...
There are several important variables within the Amazon EKS pricing model. Dig into the numbers to ensure you deploy the service ...
AWS users face a choice when deploying Kubernetes: run it themselves on EC2 or let Amazon do the heavy lifting with EKS. See ...
Incorporating consulting services and flexible accommodations for different LLMs, developer-focused Contentstack offers its own ...
As SharePoint 2019 approaches its end of life, users can expect reduced support. Migration to newer platforms like SharePoint ...
Measuring knowledge management effectiveness requires quantitative and qualitative data. Metrics like the balanced scorecard ...
With its Cerner acquisition, Oracle sets its sights on creating a national, anonymized patient database -- a road filled with ...
Oracle plans to acquire Cerner in a deal valued at about $30B. The second-largest EHR vendor in the U.S. could inject new life ...
The Supreme Court ruled 6-2 that Java APIs used in Android phones are not subject to American copyright law, ending a ...
SAP showcases new Business AI applications and continues to make the case for S/4HANA Cloud as the future of SaaS-based ERP ...
SAP acquires the digital adoption platform vendor in a bid to expand its portfolio of applications that helps customers moving ...
On the first day of Sapphire, SAP focused on business AI and the criticality of its GenAI assistant. But analysts say the ...
Lecture 2 Fundamentals of Data, Information, and Knowledge
- First Online: 01 January 2014
Cite this chapter
- Andreas Holzinger 2
2293 Accesses
1 Citations
would be aware of the types and categories of different datasets in biomedical informatics.
This is a preview of subscription content, log in via an institution to check access.
Access this chapter
- Available as PDF
- Read on any device
- Instant download
- Own it forever
- Available as EPUB and PDF
- Compact, lightweight edition
- Dispatched in 3 to 5 business days
- Free shipping worldwide - see info
- Durable hardcover edition
Tax calculation will be finalised at checkout
Purchases are for personal use only
Institutional subscriptions
Refer to: http://physics.nist.gov/cuu/Units/binary.html .
Institute of Medicine, http.//iom.edu.
In Einstein’s theory of Special Relativity, Euclidean 3-space plus time (the “4th-dimension”) are unified into the Minkowski space.
Adler R, Downarowicz T, Misiurewicz M (2008) Topological entropy (Online). http://www.scholarpedia.org/article/Topological_entropy . Accessed 10 Aug 2013
Adler RL, Konheim AG, Mcandrew MH (1965) Topological entropy. Trans Am Math Soc 114(2):309–319
Article MATH MathSciNet Google Scholar
Ahmadian L, Van Engen-Verheul M, Bakhshi-Raiez F, Peek N, Cornet R, De Keizer NF (2011) The role of standardized data and terminological systems in computerized clinical decision support systems: literature review and survey. Int J Med Inform 80(2):81–93
Article Google Scholar
Aho AV, Hopcroft JE, Ullman JD (1983) Data structures and algorithms. Addison-Wesley, Boston, MA
MATH Google Scholar
Bemmel JHV, Musen MA (1997) Handbook of medical informatics. Springer, Heidelberg
Google Scholar
Bessarabova M, Ishkin A, Jebailey L, Nikolskaya T, Nikolsky Y (2012) Knowledge-based analysis of proteomics data. BMC Bioinform 13(Suppl 16):S13
Bleiholder J, Naumann F (2008) Data fusion. ACM Comput Surv (CSUR) 41(1):1
Boisot M, Canals A (2004) Data, information and knowledge: have we got it right? J Evol Econ 14(1):43–67
Bramer M (2013) Principles of data mining, 2nd edn. Springer, Heidelberg
Book MATH Google Scholar
Clausius R (1850) On the motive power of heat, and on the laws which can be deduced from it for the theory of heat (reprint, 1960). Dover, New York
Cormen T (2013) Algorithms unlocked. The MIT Press, Cambridge, MA
Cormen TH, Leiserson CE, Rivest RL, Stein C (2009) Introduction to algorithms, 3rd edn. The MIT Press, Cambridge, MA
Darwin C (1859) On the origin of species by means of natural selection, or the preservation of favoured races in the struggle for life. John Murray, London
De Boer P-T, Kroese DP, Mannor S, Rubinstein RY (2005) A tutorial on the cross-entropy method. Ann Oper Res 134(1):19–67
Dehmer M, Mowshowitz A (2011) A history of graph entropy measures. Inform Sci 181(1):57–78
Dos Santos S, Brodlie K (2002) Visualizing and investigating multidimensional functions. Proceedings of the symposium on data visualisation 2002. Eurographics Association. pp. 173–182
Dos Santos S, Brodlie K (2004) Gaining understanding of multivariate and multidimensional data through visualization. Comput Graph 28(3):311–325
Duda RO, Hart PE, Stork DG (2000) Pattern Classification, 2nd edn. Wiley, New York
Edelsbrunner H, Harer JL (2010) Computational topology: an introduction. American Mathematical Society, Providence, RI
Ghrist R (2008) Barcodes: the persistent topology of data. Bull Am Math Soc 45(1):61–75
Golan A (2008) Information and entropy econometric: a review and synthesis. Found Trends Econ 2(1–2):1–145
MathSciNet Google Scholar
Holzinger A (2003) Basiswissen IT/Informatik. Band 2: Informatik. Vogel Buchverlag, Wuerzburg
Holzinger A (2012) On knowledge discovery and interactive intelligent visualization of biomedical data: challenges in human–computer interaction & biomedical informatics. In: Helfert M, Fancalanci C, Filipe J (eds) DATA—International conference on data technologies and applications. INSTICC, Rome, pp 5–16
Holzinger A (2013) Human–computer interaction & knowledge discovery (HCI-KDD): what is the benefit of bringing those two fields to work together? In: Alfredo Cuzzocrea CK, Simos DE, Weippl E, Xu L (eds) Multidisciplinary Research and practice for information systems, Springer lecture notes in computer science LNCS 8127. Springer, New York, pp 319–328
Hornero R, Aboy M, Abasolo D, Mcnames J, Wakeland W, Goldstein B (2006) Complex analysis of intracranial hypertension using approximate entropy. Crit Care Med 34(1):87–95
Hufford MB, Xu X, Van Heerwaarden J, Pyhajarvi T, Chia J-M, Cartwright RA, Elshire RJ, Glaubitz JC, Guill KE, Kaeppler SM, Lai J, Morrell PL, Shannon LM, Song C, Springer NM, Swanson-Wagner RA, Tiffin P, Wang J, Zhang G, Doebley J, Mcmullen MD, Ware D, Buckler ES, Yang S, Ross-Ibarra J (2012) Comparative population genomics of maize domestication and improvement. Nat Genet 44(7):808–811
Jaynes ET (1957) Information theory and statistical mechanics. Phys Rev 106(4):620
Joyce AR, Palsson BØ (2006) The model organism as a system: integrating “omics” data sets. Nat Rev Mol Cell Biol 7(3):198–210
Kaski S, Peltonen J (2011) Dimensionality reduction for data visualization (applications corner). IEEE Sig Process Mag 28(2):100–104
Körner J (1973) Coding of an information source having ambiguous alphabet and the entropy of graphs. 6th Prague conference on information theory. pp. 411–425
Kreuzthaler M, Bloice MD, Faulstich L, Simonic KM, Holzinger A (2011) A comparison of different retrieval strategies working on medical free texts. J Univ Comput Sci 17(7):1109–1133
Lane N, Martin W (2010) The energetics of genome complexity. Nature 467(7318):929–934
Lanzagorta M, Uhlmann J (2008) Quantum computer science. Morgan & Claypool, San Francisco
Lieberman E, Hauert C, Nowak MA (2005) Evolutionary dynamics on graphs. Nature 433(7023):312–316
Manyika J, Chui M, Brown B, Bughin J, Dobbs R, Roxburgh C, Byers AH (2011) Big data: the next frontier for innovation, competition, and productivity. McKinsey Global Institute, Washington, DC
Marinescu DC (2011) Classical and quantum information. Academic, Burlington, MA
Mowshowitz A (1968) Entropy and the complexity of graphs: I. An index of the relative complexity of a graph. Bull Math Biol 30(1):175–204
MATH MathSciNet Google Scholar
Ottmann T, Widmayer P (2012) Algorithmen und Datenstrukturen (5. Auflage). Spektrum Akademischer Verlag, Heidelberg
Book Google Scholar
Patel VL, Arocha JF, Zhang J (2004) Thinking and reasoning in medicine Key. In: Holyoak K (ed) Cambridge handbook of thinking and reasoning. Cambridge University Press, Cambridge
Patel VL, Ramoni MF (1997) Cognitive models of directional inference in expert medical reasoning. In: Feltovich PJ, Ford KM (eds) Expertise in context: human and machine. The MIT Press, Cambridge, MA, pp 67–99
Peirce CS (1955) Abduction and induction. In: Peirce CS, Buchler J (eds) Philosophical writings of Peirce. Dover Publications, New York, pp 150–156
Pincus SM (1991) Approximate Entropy as a measure of system complexity. Proc Natl Acad Sci U S A 88(6):2297–2301
Posner E (1975) Random coding strategies for minimum entropy. IEEE Trans Inform Theory 21(4):388–391
Richman JS, Moorman JR (2000) Physiological time-series analysis using approximate entropy and sample entropy. Am J Physiol Heart Circ Physiol 278(6):H2039–H2049
Riffle M, Eng JK (2009) Proteomics data repositories. Proteomics 9(20):4653–4663
Rubinstein RY (1997) Optimization of computer simulation models with rare events. Eur J Oper Res 99(1):89–112
Shannon CE (1948) A mathematical theory of communication. Bell Syst Tech J 27:379–423
Simon HA (1973) The structure of ill structured problems. Artif Intell 4(3–4):181–201
Simonic KM, Holzinger A, Bloice M, Hermann J (2011) Optimizing long-term treatment of rheumatoid arthritis with systematic documentation. Proceedings of pervasive health—5th international conference on pervasive computing technologies for healthcare. IEEE, Dublin. pp. 550–554
Stevens SS (1946) On the theory of scales of measurement. Science 103:677–680
Article MATH Google Scholar
Thomas JJ, Cook KA (2005) Illuminating the path: the research and development agenda for visual analytics. IEEE Computer Society Press, New York
Wickens CD (1984) Engineering psychology and human performance. Charles Merrill, Columbus, OH
Yuan L, Kesavan H (1998) Minimum entropy and information measure. IEEE Trans Syst Man Cybern C Appl Rev 28(3):488–491
Zomorodian AJ (2005) Topology for computing. Cambridge University Press, Cambridge, MA
Download references
Author information
Authors and affiliations.
Medical University Graz and Graz University of Technology, Graz, Austria
Andreas Holzinger
You can also search for this author in PubMed Google Scholar
1 Supplementary Materials
Below is the link to the electronic supplementary material.
2_LV444_152_FUNDAMENTALS_HOLZINGER_WS2013_14_lowres.pdf (PDF 5.08 MB)
Rights and permissions.
Reprints and permissions
Copyright information
© 2014 Springer International Publishing Switzerland
About this chapter
Holzinger, A. (2014). Lecture 2 Fundamentals of Data, Information, and Knowledge. In: Biomedical Informatics. Springer, Cham. https://doi.org/10.1007/978-3-319-04528-3_2
Download citation
DOI : https://doi.org/10.1007/978-3-319-04528-3_2
Published : 14 March 2014
Publisher Name : Springer, Cham
Print ISBN : 978-3-319-04527-6
Online ISBN : 978-3-319-04528-3
eBook Packages : Engineering Engineering (R0)
Share this chapter
Anyone you share the following link with will be able to read this content:
Sorry, a shareable link is not currently available for this article.
Provided by the Springer Nature SharedIt content-sharing initiative
- Publish with us
Policies and ethics
- Find a journal
- Track your research
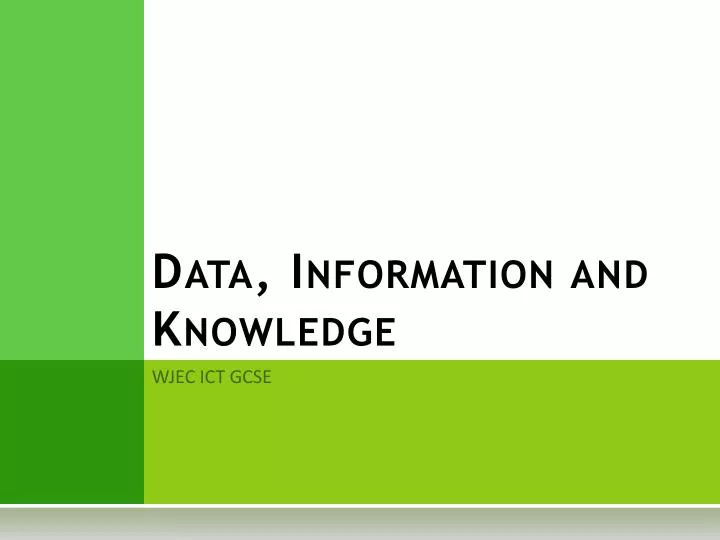
Data, Information and Knowledge
Sep 09, 2014
1.45k likes | 3.36k Views
Data, Information and Knowledge. WJEC ICT GCSE. Starter Activity. Open the PowerPoint: L Drive Miss Pickles Year 10 Data, Information and Knowledge Complete Task 1: Find a definition for these terms: Data Information Knowledge. Lesson Objectives.
Share Presentation
- coding data
- encoding data
- data accurate
- resulting information output
- wjec ict gcse
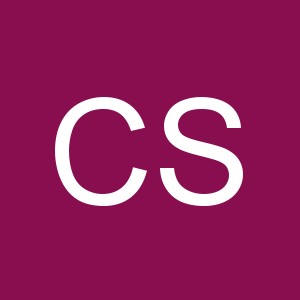
Presentation Transcript
Data, Information and Knowledge WJEC ICT GCSE
Starter Activity • Open the PowerPoint: • L Drive Miss Pickles Year 10 Data, Information and Knowledge • Complete Task 1: • Find a definition for these terms: • Data • Information • Knowledge
Lesson Objectives • Understand the difference between data and information. • Be able to identify data, information and knowledge. • Suggest why data, information and knowledge is used.
Data • Data are raw facts and figures that on their own have no meaning. (e.g. readings from sensors, survey facts, etc) • Data can be numbers, words, letters, images, sound etc.
Information • Information is data that has been processed by a computer system to give it meaning. • Processed can mean: • having calculations performed on it • converted to give it meaning • organized in some way 21041926 Date of Birth - 21st April 1926 A,A,A,A,B,B,C,C,C,D,D A student’s predicted GCSE grades 2010, 93, digital TV In 2010, 93% of UK homes had digital TV
Knowledge • Knowledge is derived from information by applying rules to it. • Draw conclusions, make judgements or opinions. Make predictions and decisions. Example Knowledge If we apply knowledge that the speed limit on the motorway is 70 mph we now know that the driver is breaking the speed limit. Information A vehicle is travelling at 100 mph on the M4. Data 100, M4
Collecting Data Data can be collected ...
Turning Data into Information To turn data into information it needs to be processed. Data Processing Information Data Processing Information
Turning Information into Knowledge Understand the information and learn from it. We apply rules to it to learn. We can then draw conclusions, make judgements or opinions, make predictions and decisions.
Summary Data Raw facts and figures Information Data Context Meaning Processing Information Knowledge Rules
Quality of Data • GIGO (Garbage In Garbage Out) • If data input is poor the resulting information output will be poor i.e. corrupt, inaccurate etc. Garbage In Garbage Out
Measuring the Quality of Data • Is the data fit for purpose? • Is the data suitable for doing the job for which it was collected? • Is there enough data? • Has the correct data been collected? • Is the data accurate? • Has the data been made up? • Is some data missing? • Are their any mistakes in the data? • Is the data bias? • Are questions worded to prompt a certain answer? • Has a broad sample of data been collected?
Encoding Data • Data is often coded when it is collected on input into an ICT system. e.g. • M or F • Mo, Tu, We, Th, Fr, Sa, Su • S, M, L, XL, XXL
Advantages of coding Data • Coded data is quicker to type in • It takes up less storage space • It is easier to check a code using validation checks • It is faster to access data that is coded
Disadvantages ofcoding Data • Precision of data can be lost • In the example all shades of blue are coded as “blue” • The user needs to know the codes used Data in Pink Blue Black Blue Stored data Pink Blue Black Blue
Let’s Recap • What is data? • What is Information? • What is knowledge? • What does GIGO stand for? • What does encoding mean?
- More by User
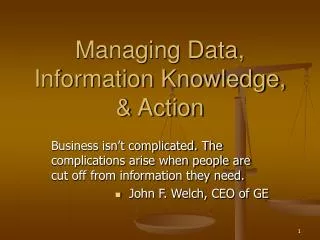
Managing Data, Information Knowledge, & Action
Managing Data, Information Knowledge, & Action. Business isn’t complicated. The complications arise when people are cut off from information they need. John F. Welch, CEO of GE. 1. Myths. More data is better. Information is a commodity Information is knowledge. 2. D-I-K-A Model.
423 views • 12 slides
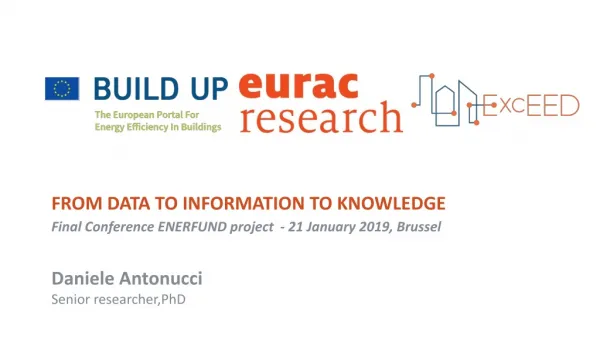
FROM DATA TO INFORMATION TO KNOWLEDGE
FROM DATA TO INFORMATION TO KNOWLEDGE. Final Conference ENERFUND project - 21 January 2019, Brussel. Daniele Antonucci Senior researcher,PhD. AGENDA OF THE PRESENTATION. THE BUILD UP PLATFORM BRIEF PRESENTATION OF EURAC RESEARCH ExCEED PROJECT.
287 views • 20 slides
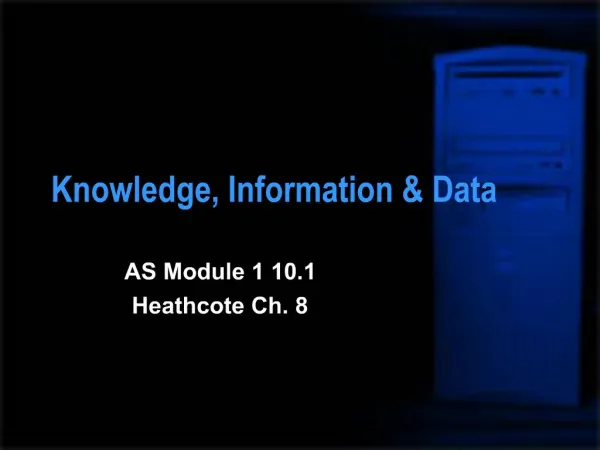
Knowledge, Information Data
Input-process-output. Input incoming data that computers read Process the operation that turns data into information Output displayed or printed information, or perhaps sound. INPUT. PROCESS. OUTPUT. . . Data, Information
364 views • 9 slides
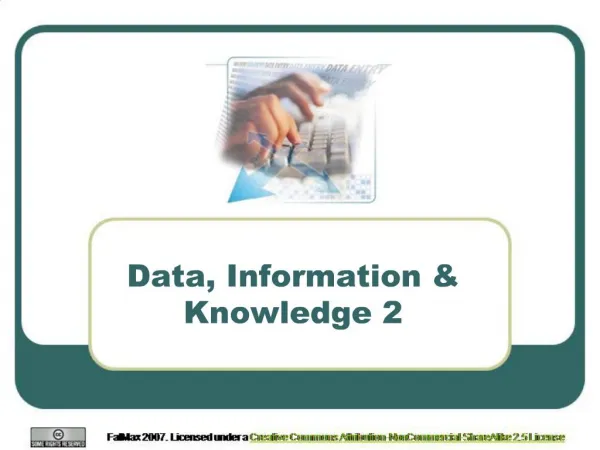
Data, Information Knowledge 2
292 views • 14 slides
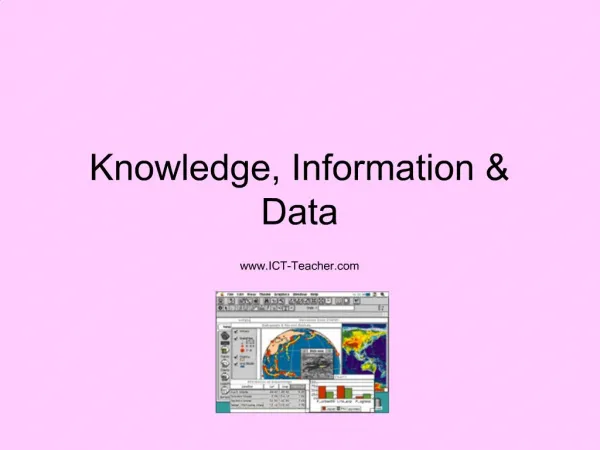
Understand the distinction between knowledge, information and data.2.Information has context and its meaning is determined by that context.3.Understand the nature of data, e.g. recorded facts, events or transactions.Understand the different ways in which data can arise: direct capture or as
294 views • 14 slides
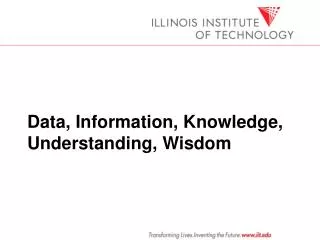
Data, Information, Knowledge, Understanding, Wisdom
Data, Information, Knowledge, Understanding, Wisdom. Overview. Data is just symbols Information is data that are processed to be useful; provides answers to "who", "what", "where", and "when" questions Knowledge is application of data and information; answers "how" questions
605 views • 10 slides
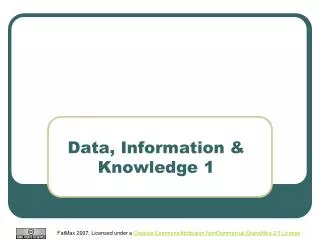
Data, Information & Knowledge 1
Data, Information & Knowledge 1. Data. Data are raw facts and figures that on their own have no meaning These can be any alphanumeric characters i.e. text, numbers, symbols. Note the “ are ” bit above? What does this mean?. Data Examples. Yes, Yes, No, Yes, No, Yes, No, Yes
361 views • 17 slides
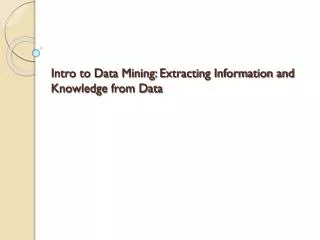
Intro to Data Mining: Extracting Information and Knowledge from Data
Intro to Data Mining: Extracting Information and Knowledge from Data . Topics. Relationships between DSS/BI, database, data management DSS/BI : transforming data into info to support decision making How operational data and DSS/BI data differ
1.08k views • 83 slides
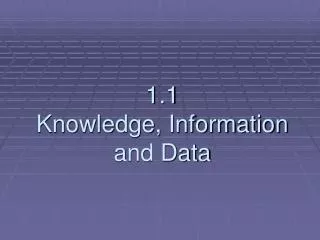
1.1 Knowledge, Information and Data
1.1 Knowledge, Information and Data. AIMS :. To understand the distinction between knowledge, information and data. To understand that data can arise from direct capture, or as a by-product of another operation.
293 views • 16 slides
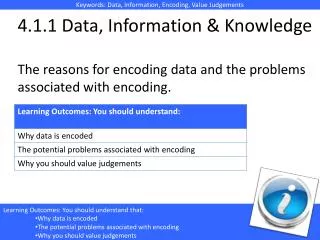
4.1.1 Data, Information & Knowledge
4.1.1 Data, Information & Knowledge The reasons for encoding data and the problems associated with encoding. Starter: Examples. What are the codes used for and where have you seen examples of their use? 10/12/76 M or F L23 5TA 01519307766 90-12-45 LPL, MAN, LGW, LHR GB, F CH, D
597 views • 19 slides
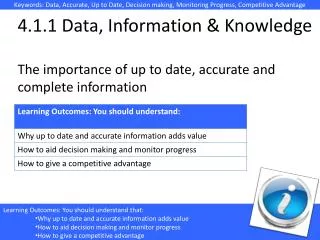
4.1.1 Data, Information & Knowledge The importance of up to date, accurate and complete information. Starter: Spider Diagram. Get into groups of 4 Draw a spider diagram of everything you can think of relating to data, information, knowledge and encoding (5 mins - Groups).
438 views • 21 slides
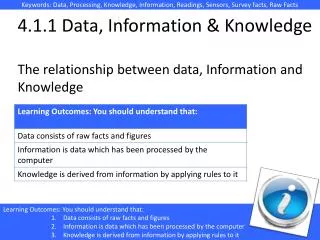
4.1.1 Data, Information & Knowledge The relationship between data, Information and Knowledge
4.1.1 Data, Information & Knowledge The relationship between data, Information and Knowledge. Starter: List example of. Data? Information?. Task: Questions. In groups discuss: What is data, information and knowledge? What makes them different from each other? Think of an example
1.85k views • 29 slides
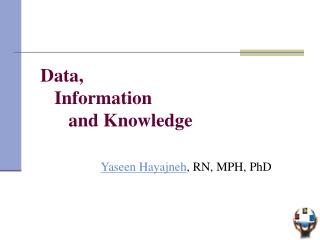
Data, Information and Knowledge. Yaseen Hayajneh , RN, MPH, PhD. Quotes…. Data is not information, Information is not knowledge, ….. Cliff Stoll & Gary Schubert
865 views • 35 slides
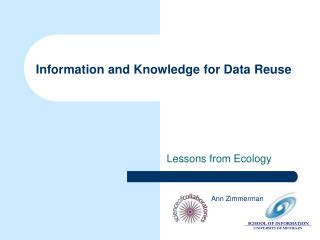
Information and Knowledge for Data Reuse
Information and Knowledge for Data Reuse. Lessons from Ecology. Ann Zimmerman. What do ecologists and organizations have in common when it comes to sharing data? A lot!. Ecology. Ecology is a “craft” science Single investigators conduct small scale studies Data sets are highly diverse
228 views • 13 slides
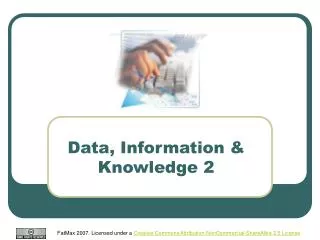
Data, Information & Knowledge 2
Data, Information & Knowledge 2. Introduction. Previous presentation covered what data is* In this presentation we cover where data comes from and factors we need to take into account when gathering data for processing. * Should really be data “are” but nobody talks like this!. Data Sources.
275 views • 14 slides
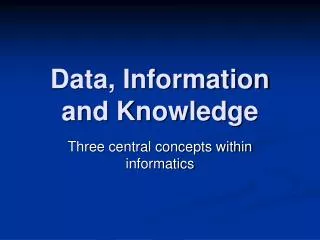
Data, Information and Knowledge. Three central concepts within informatics. My background. 13 years at Lund university 10 years at Copenhagen Business School 6 years at Växjö university Ideal: Maxium user influence Strong philosophical interest Certain tendencies to provoke.
678 views • 32 slides
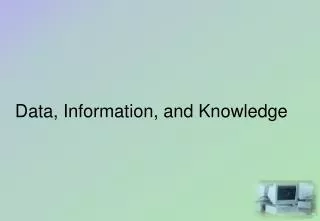
Data, Information, and Knowledge
Data, Information, and Knowledge. Data. Raw facts and figures Letters, numbers, combination of both letters and numbers Values which on their own have no meaning. Examples of Data. 150170 English 23 1066. The above data has no meaning. Context. Information.
411 views • 24 slides
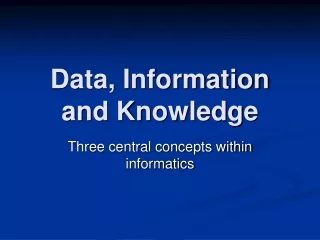
366 views • 32 slides
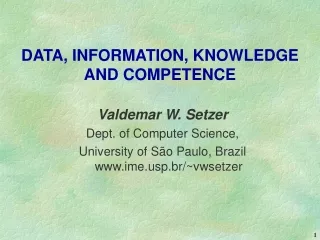
DATA, INFORMATION, KNOWLEDGE AND COMPETENCE
DATA, INFORMATION, KNOWLEDGE AND COMPETENCE. Valdemar W. Setzer Dept. of Computer Science, University of São Paulo, Brazil www.ime.usp.br/~vwsetzer. TOPICS. 1. Introduction 2. Concepts 3. Competence matrices 4. Uses of a competence system 5. Example of a system
517 views • 48 slides
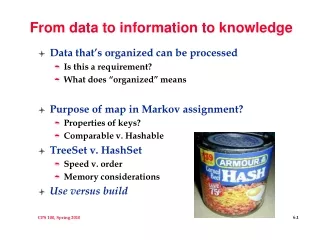
From data to information to knowledge
From data to information to knowledge. Data that’s organized can be processed Is this a requirement? What does “organized” means Purpose of map in Markov assignment? Properties of keys? Comparable v. Hashable TreeSet v. HashSet Speed v. order Memory considerations Use versus build.
253 views • 24 slides
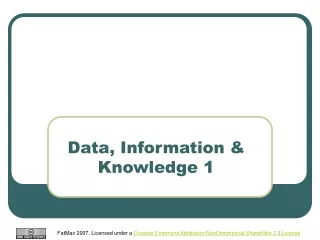
299 views • 17 slides
- Accessibility Policy
- Skip to content
- QUICK LINKS
- Oracle Cloud Infrastructure
- Oracle Fusion Cloud Applications
- Download Java
- Careers at Oracle
What Is Big Data?
Sherry Tiao | Senior Manager, AI & Analytics, Oracle | March 11, 2024

In This Article
Big Data Defined
The three “vs” of big data, the value—and truth—of big data, the history of big data, big data use cases, big data challenges, how big data works, big data best practices.
What exactly is big data?
The definition of big data is data that contains greater variety, arriving in increasing volumes and with more velocity. This is also known as the three “Vs.”
Put simply, big data is larger, more complex data sets, especially from new data sources. These data sets are so voluminous that traditional data processing software just can’t manage them. But these massive volumes of data can be used to address business problems you wouldn’t have been able to tackle before.
Volume | The amount of data matters. With big data, you’ll have to process high volumes of low-density, unstructured data. This can be data of unknown value, such as X (formerly Twitter) data feeds, clickstreams on a web page or a mobile app, or sensor-enabled equipment. For some organizations, this might be tens of terabytes of data. For others, it may be hundreds of petabytes. |
Velocity | Velocity is the fast rate at which data is received and (perhaps) acted on. Normally, the highest velocity of data streams directly into memory versus being written to disk. Some internet-enabled smart products operate in real time or near real time and will require real-time evaluation and action. |
Variety | Variety refers to the many types of data that are available. Traditional data types were structured and fit neatly in a . With the rise of big data, data comes in new unstructured data types. Unstructured and semistructured data types, such as text, audio, and video, require additional preprocessing to derive meaning and support metadata. |
Two more Vs have emerged over the past few years: value and veracity . Data has intrinsic value. But it’s of no use until that value is discovered. Equally important: How truthful is your data—and how much can you rely on it?
Today, big data has become capital. Think of some of the world’s biggest tech companies. A large part of the value they offer comes from their data, which they’re constantly analyzing to produce more efficiency and develop new products.
Recent technological breakthroughs have exponentially reduced the cost of data storage and compute, making it easier and less expensive to store more data than ever before. With an increased volume of big data now cheaper and more accessible, you can make more accurate and precise business decisions.
Finding value in big data isn’t only about analyzing it (which is a whole other benefit). It’s an entire discovery process that requires insightful analysts, business users, and executives who ask the right questions, recognize patterns, make informed assumptions, and predict behavior.
But how did we get here?
Although the concept of big data itself is relatively new, the origins of large data sets go back to the 1960s and ‘70s when the world of data was just getting started with the first data centers and the development of the relational database.
Around 2005, people began to realize just how much data users generated through Facebook, YouTube, and other online services. Hadoop (an open source framework created specifically to store and analyze big data sets) was developed that same year. NoSQL also began to gain popularity during this time.
The development of open source frameworks, such as Hadoop (and more recently, Spark) was essential for the growth of big data because they make big data easier to work with and cheaper to store. In the years since then, the volume of big data has skyrocketed. Users are still generating huge amounts of data—but it’s not just humans who are doing it.
With the advent of the Internet of Things (IoT), more objects and devices are connected to the internet, gathering data on customer usage patterns and product performance. The emergence of machine learning has produced still more data.
While big data has come far, its usefulness is only just beginning. Cloud computing has expanded big data possibilities even further. The cloud offers truly elastic scalability, where developers can simply spin up ad hoc clusters to test a subset of data. And graph databases are becoming increasingly important as well, with their ability to display massive amounts of data in a way that makes analytics fast and comprehensive.

Discover the Insights in Your Data
- Who are the criminals passing dirty money around and committing financial services fraud?
- Who has been in contact with an infected person and needs to go into quarantine?
- How can feature engineering for data science be made simpler and more efficient?
Click below to access the 17 Use Cases for Graph Databases and Graph Analytics ebook.
Big Data Benefits
- Big data makes it possible for you to gain more complete answers because you have more information.
- More complete answers mean more confidence in the data—which means a completely different approach to tackling problems.
Big data can help you address a range of business activities, including customer experience and analytics. Here are just a few.
Product development | Companies like Netflix and Procter & Gamble use big data to anticipate customer demand. They build predictive models for new products and services by classifying key attributes of past and current products or services and modeling the relationship between those attributes and the commercial success of the offerings. In addition, P&G uses data and analytics from focus groups, social media, test markets, and early store rollouts to plan, produce, and launch new products. |
Predictive maintenance | Factors that can predict mechanical failures may be deeply buried in structured data, such as the year, make, and model of equipment, as well as in unstructured data that covers millions of log entries, sensor data, error messages, and engine temperature. By analyzing these indications of potential issues before the problems happen, organizations can deploy maintenance more cost effectively and maximize parts and equipment uptime. |
Customer experience | The race for customers is on. A clearer view of customer experience is more possible now than ever before. Big data enables you to gather data from social media, web visits, call logs, and other sources to improve the interaction experience and maximize the value delivered. Start delivering personalized offers, reduce customer churn, and handle issues proactively. |
Fraud and compliance | When it comes to security, it’s not just a few rogue hackers—you’re up against entire expert teams. Security landscapes and compliance requirements are constantly evolving. Big data helps you identify patterns in data that indicate fraud and aggregate large volumes of information to make regulatory reporting much faster. |
Machine learning | Machine learning is a hot topic right now. And data—specifically big data—is one of the reasons why. We are now able to teach machines instead of program them. The availability of big data to train machine learning models makes that possible. |
Operational efficiency | Operational efficiency may not always make the news, but it’s an area in which big data is having the most impact. With big data, you can analyze and assess production, customer feedback and returns, and other factors to reduce outages and anticipate future demands. Big data can also be used to improve decision-making in line with current market demand. |
Drive innovation | Big data can help you innovate by studying interdependencies among humans, institutions, entities, and process and then determining new ways to use those insights. Use data insights to improve decisions about financial and planning considerations. Examine trends and what customers want to deliver new products and services. Implement dynamic pricing. There are endless possibilities. |

Download your free ebook to learn about:
- New ways you can use your data
- Ways the competition could be innovating
- Benefits and challenges of different use cases
While big data holds a lot of promise, it is not without its challenges.
First, big data is…big. Although new technologies have been developed for data storage, data volumes are doubling in size about every two years. Organizations still struggle to keep pace with their data and find ways to effectively store it.
But it’s not enough to just store the data. Data must be used to be valuable and that depends on curation. Clean data, or data that’s relevant to the client and organized in a way that enables meaningful analysis, requires a lot of work. Data scientists spend 50 to 80 percent of their time curating and preparing data before it can actually be used.
Finally, big data technology is changing at a rapid pace. A few years ago, Apache Hadoop was the popular technology used to handle big data. Then Apache Spark was introduced in 2014. Today, a combination of the two frameworks appears to be the best approach. Keeping up with big data technology is an ongoing challenge.
Discover more big data resources:
Big data gives you new insights that open up new opportunities and business models. Getting started involves three key actions:
1. Integrate Big data brings together data from many disparate sources and applications. Traditional data integration mechanisms, such as extract, transform, and load (ETL) generally aren’t up to the task. It requires new strategies and technologies to analyze big data sets at terabyte, or even petabyte, scale.
During integration, you need to bring in the data, process it, and make sure it’s formatted and available in a form that your business analysts can get started with.
2. Manage Big data requires storage. Your storage solution can be in the cloud, on premises, or both. You can store your data in any form you want and bring your desired processing requirements and necessary process engines to those data sets on an on-demand basis. Many people choose their storage solution according to where their data is currently residing. The cloud is gradually gaining popularity because it supports your current compute requirements and enables you to spin up resources as needed.
3. Analyze Your investment in big data pays off when you analyze and act on your data. Get new clarity with a visual analysis of your varied data sets. Explore the data further to make new discoveries. Share your findings with others. Build data models with machine learning and artificial intelligence. Put your data to work.
To help you on your big data journey, we’ve put together some key best practices for you to keep in mind. Here are our guidelines for building a successful big data foundation.
Align big data with specific business goals | More extensive data sets enable you to make new discoveries. To that end, it is important to base new investments in skills, organization, or infrastructure with a strong business-driven context to guarantee ongoing project investments and funding. To determine if you are on the right track, ask how big data supports and enables your top business and IT priorities. Examples include understanding how to filter web logs to understand ecommerce behavior, deriving sentiment from social media and customer support interactions, and understanding statistical correlation methods and their relevance for customer, product, manufacturing, and engineering data. |
Ease skills shortage with standards and governance | One of the biggest obstacles to benefiting from your investment in big data is a skills shortage. You can mitigate this risk by ensuring that big data technologies, considerations, and decisions are added to your IT governance program. Standardizing your approach will allow you to manage costs and leverage resources. Organizations implementing big data solutions and strategies should assess their skill requirements early and often and should proactively identify any potential skill gaps. These can be addressed by training/cross-training existing resources, hiring new resources, and leveraging consulting firms. |
Optimize knowledge transfer with a center of excellence | Use a center of excellence approach to share knowledge, control oversight, and manage project communications. Whether big data is a new or expanding investment, the soft and hard costs can be shared across the enterprise. Leveraging this approach can help increase big data capabilities and overall information architecture maturity in a more structured and systematic way. |
Top payoff is aligning unstructured with structured data | It is certainly valuable to analyze big data on its own. But you can bring even greater business insights by connecting and integrating low density big data with the structured data you are already using today. Whether you are capturing customer, product, equipment, or environmental big data, the goal is to add more relevant data points to your core master and analytical summaries, leading to better conclusions. For example, there is a difference in distinguishing all customer sentiment from that of only your best customers. Which is why many see big data as an integral extension of their existing business intelligence capabilities, data warehousing platform, and information architecture. Keep in mind that the big data analytical processes and models can be both human- and machine-based. Big data analytical capabilities include statistics, spatial analysis, semantics, interactive discovery, and visualization. Using analytical models, you can correlate different types and sources of data to make associations and meaningful discoveries. |
Plan your discovery lab for performance | Discovering meaning in your data is not always straightforward. Sometimes we don’t even know what we’re looking for. That’s expected. Management and IT needs to support this “lack of direction” or “lack of clear requirement.” At the same time, it’s important for analysts and data scientists to work closely with the business to understand key business knowledge gaps and requirements. To accommodate the interactive exploration of data and the experimentation of statistical algorithms, you need high-performance work areas. Be sure that sandbox environments have the support they need—and are properly governed. |
Align with the cloud operating model | Big data processes and users require access to a broad array of resources for both iterative experimentation and running production jobs. A big data solution includes all data realms including transactions, master data, reference data, and summarized data. Analytical sandboxes should be created on demand. Resource management is critical to ensure control of the entire data flow including pre- and post-processing, integration, in-database summarization, and analytical modeling. A well-planned private and public cloud provisioning and security strategy plays an integral role in supporting these changing requirements. |
Learn More About Big Data at Oracle
- Try a free big data workshop
- Infographic: How to Build Effective Data Lakes
Introducing Microsoft 365 Copilot – your copilot for work
Mar 16, 2023 | Jared Spataro - CVP, AI at Work
- Share on Facebook (opens new window)
- Share on Twitter (opens new window)
- Share on LinkedIn (opens new window)
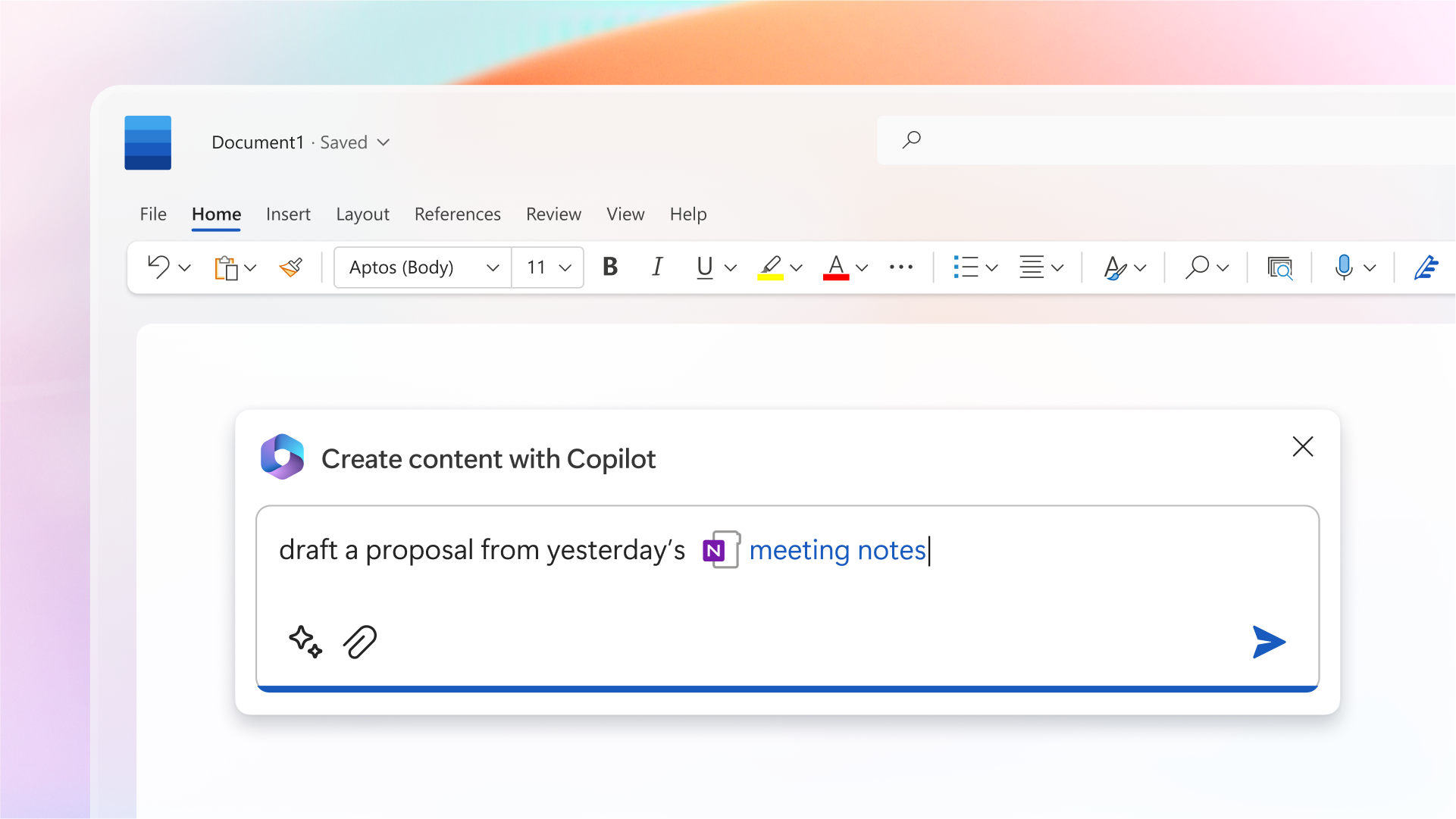
Humans are hard-wired to dream, to create, to innovate. Each of us seeks to do work that gives us purpose — to write a great novel, to make a discovery, to build strong communities, to care for the sick. The urge to connect to the core of our work lives in all of us. But today, we spend too much time consumed by the drudgery of work on tasks that zap our time, creativity and energy. To reconnect to the soul of our work, we don’t just need a better way of doing the same things. We need a whole new way to work.
Today, we are bringing the power of next-generation AI to work. Introducing Microsoft 365 Copilot — your copilot for work . It combines the power of large language models (LLMs) with your data in the Microsoft Graph and the Microsoft 365 apps to turn your words into the most powerful productivity tool on the planet.
“Today marks the next major step in the evolution of how we interact with computing, which will fundamentally change the way we work and unlock a new wave of productivity growth,” said Satya Nadella, Chairman and CEO, Microsoft. “With our new copilot for work, we’re giving people more agency and making technology more accessible through the most universal interface — natural language.”
Copilot is integrated into Microsoft 365 in two ways. It works alongside you, embedded in the Microsoft 365 apps you use every day — Word, Excel, PowerPoint, Outlook, Teams and more — to unleash creativity, unlock productivity and uplevel skills. Today we’re also announcing an entirely new experience: Business Chat . Business Chat works across the LLM, the Microsoft 365 apps, and your data — your calendar, emails, chats, documents, meetings and contacts — to do things you’ve never been able to do before. You can give it natural language prompts like “Tell my team how we updated the product strategy,” and it will generate a status update based on the morning’s meetings, emails and chat threads.
With Copilot, you’re always in control. You decide what to keep, modify or discard. Now, you can be more creative in Word, more analytical in Excel, more expressive in PowerPoint, more productive in Outlook and more collaborative in Teams.
Microsoft 365 Copilot transforms work in three ways:
Unleash creativity. With Copilot in Word, you can jump-start the creative process so you never start with a blank slate again. Copilot gives you a first draft to edit and iterate on — saving hours in writing, sourcing, and editing time. Sometimes Copilot will be right, other times usefully wrong — but it will always put you further ahead. You’re always in control as the author, driving your unique ideas forward, prompting Copilot to shorten, rewrite or give feedback. Copilot in PowerPoint helps you create beautiful presentations with a simple prompt, adding relevant content from a document you made last week or last year. And with Copilot in Excel, you can analyze trends and create professional-looking data visualizations in seconds.
Unlock productivity. We all want to focus on the 20% of our work that really matters, but 80% of our time is consumed with busywork that bogs us down. Copilot lightens the load. From summarizing long email threads to quickly drafting suggested replies, Copilot in Outlook helps you clear your inbox in minutes, not hours. And every meeting is a productive meeting with Copilot in Teams. It can summarize key discussion points — including who said what and where people are aligned and where they disagree — and suggest action items, all in real time during a meeting. And with Copilot in Power Platform, anyone can automate repetitive tasks, create chatbots and go from idea to working app in minutes.
GitHub data shows that Copilot promises to unlock productivity for everyone. Among developers who use GitHub Copilot, 88% say they are more productive, 74% say that they can focus on more satisfying work, and 77% say it helps them spend less time searching for information or examples.
But Copilot doesn’t just supercharge individual productivity. It creates a new knowledge model for every organization — harnessing the massive reservoir of data and insights that lies largely inaccessible and untapped today. Business Chat works across all your business data and apps to surface the information and insights you need from a sea of data — so knowledge flows freely across the organization, saving you valuable time searching for answers. You will be able to access Business Chat from Microsoft 365.com, from Bing when you’re signed in with your work account, or from Teams.
Uplevel skills. Copilot makes you better at what you’re good at and lets you quickly master what you’ve yet to learn. The average person uses only a handful of commands — such as “animate a slide” or “insert a table” — from the thousands available across Microsoft 365. Now, all that rich functionality is unlocked using just natural language. And this is only the beginning.
Copilot will fundamentally change how people work with AI and how AI works with people. As with any new pattern of work, there’s a learning curve — but those who embrace this new way of working will quickly gain an edge.
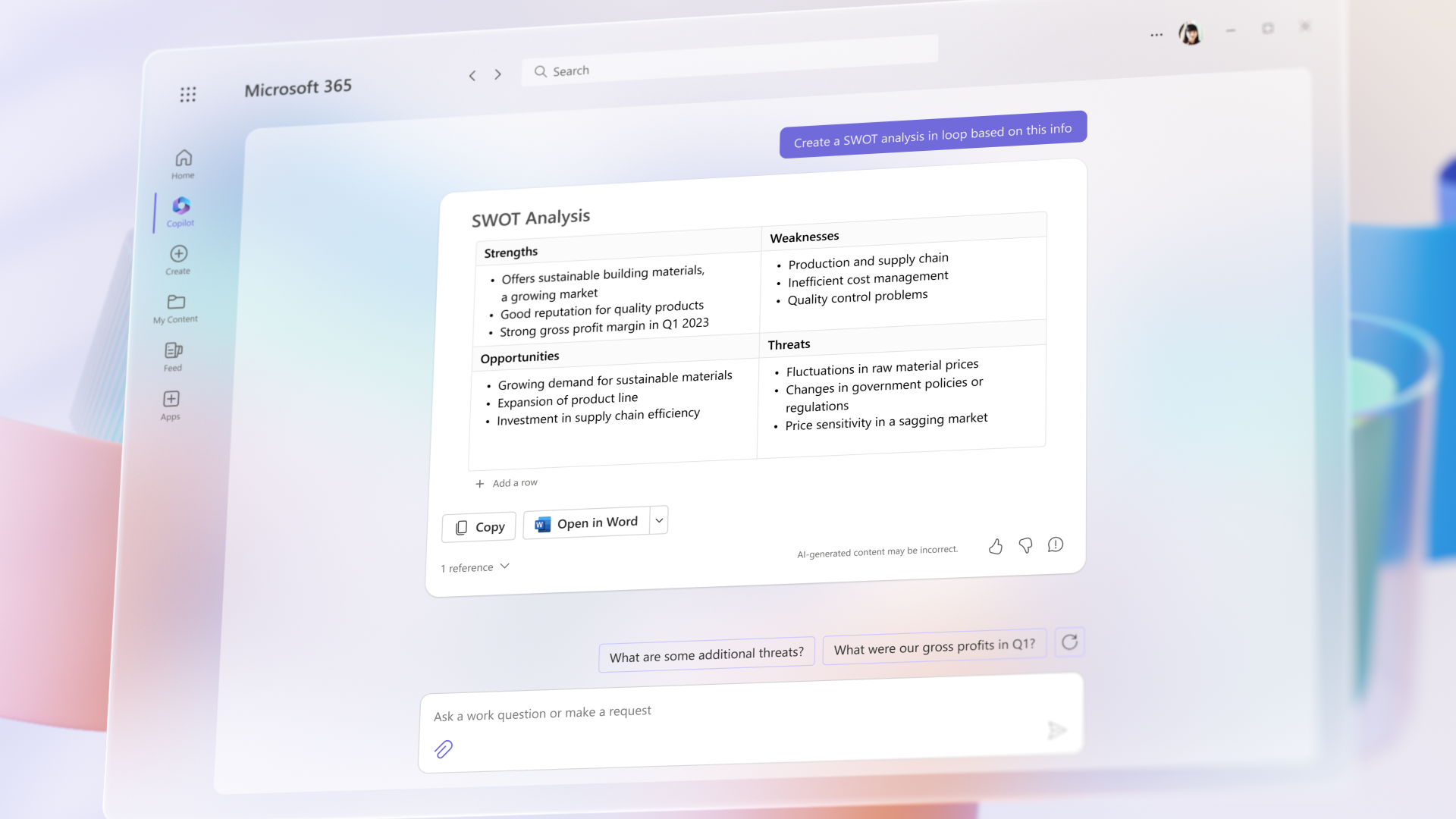
The Copilot System: Enterprise-ready AI
Microsoft is uniquely positioned to deliver enterprise-ready AI with the Copilot System . Copilot is more than OpenAI’s ChatGPT embedded into Microsoft 365. It’s a sophisticated processing and orchestration engine working behind the scenes to combine the power of LLMs, including GPT-4, with the Microsoft 365 apps and your business data in the Microsoft Graph — now accessible to everyone through natural language.
Grounded in your business data. AI-powered LLMs are trained on a large but limited corpus of data. The key to unlocking productivity in business lies in connecting LLMs to your business data — in a secure, compliant, privacy-preserving way. Microsoft 365 Copilot has real-time access to both your content and context in the Microsoft Graph. This means it generates answers anchored in your business content — your documents, emails, calendar, chats, meetings, contacts and other business data — and combines them with your working context — the meeting you’re in now, the email exchanges you’ve had on a topic, the chat conversations you had last week — to deliver accurate, relevant, contextual responses.
Built on Microsoft’s comprehensive approach to security, compliance and privacy. Copilot is integrated into Microsoft 365 and automatically inherits all your company’s valuable security, compliance, and privacy policies and processes. Two-factor authentication, compliance boundaries, privacy protections, and more make Copilot the AI solution you can trust.
Architected to protect tenant, group and individual data. We know data leakage is a concern for customers. Copilot LLMs are not trained on your tenant data or your prompts. Within your tenant, our time-tested permissioning model ensures that data won’t leak across user groups. And on an individual level, Copilot presents only data you can access using the same technology that we’ve been using for years to secure customer data.
Integrated into the apps millions use every day. Microsoft 365 Copilot is integrated in the productivity apps millions of people use and rely on every day for work and life — Word, Excel, PowerPoint, Outlook, Teams and more. An intuitive and consistent user experience ensures it looks, feels and behaves the same way in Teams as it does in Outlook, with a shared design language for prompts, refinements and commands.
Designed to learn new skills. Microsoft 365 Copilot’s foundational skills are a game changer for productivity: It can already create, summarize, analyze, collaborate and automate using your specific business content and context. But it doesn’t stop there. Copilot knows how to command apps (e.g., “animate this slide”) and work across apps, translating a Word document into a PowerPoint presentation. And Copilot is designed to learn new skills. For example, with Viva Sales, Copilot can learn how to connect to CRM systems of record to pull customer data — like interaction and order histories — into communications. As Copilot learns about new domains and processes, it will be able to perform even more sophisticated tasks and queries.
Committed to building responsibly
At Microsoft, we are guided by our AI principles and Responsible AI Standard and decades of research on AI, grounding and privacy-preserving machine learning. A multidisciplinary team of researchers, engineers and policy experts reviews our AI systems for potential harms and mitigations — refining training data, filtering to limit harmful content, query- and result-blocking sensitive topics, and applying Microsoft technologies like InterpretML and Fairlearn to help detect and correct data bias. We make it clear how the system makes decisions by noting limitations, linking to sources, and prompting users to review, fact-check and adjust content based on subject-matter expertise.
Moving boldly as we learn
In the months ahead, we’re bringing Copilot to all our productivity apps—Word, Excel, PowerPoint, Outlook, Teams, Viva, Power Platform, and more. We’ll share more on pricing and licensing soon. Earlier this month we announced Dynamics 365 Copilot as the world’s first AI Copilot in both CRM and ERP to bring the next-generation AI to every line of business.
Everyone deserves to find purpose and meaning in their work — and Microsoft 365 Copilot can help. To serve the unmet needs of our customers, we must move quickly and responsibly, learning as we go. We’re testing Copilot with a small group of customers to get feedback and improve our models as we scale, and we will expand to more soon.
Learn more on the Microsoft 365 blog and visit WorkLab to get expert insights on how AI will create a brighter future of work for everyone.
And for all the blogs, videos and assets related to today’s announcements, please visit our microsite .
Tags: AI , Microsoft 365 , Microsoft 365 Copilot
- Check us out on RSS
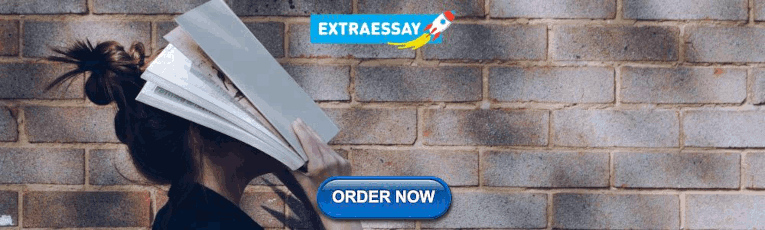
IMAGES
VIDEO
COMMENTS
Understanding Data Presentations (Guide + Examples) Design • March 20th, 2024. In this age of overwhelming information, the skill to effectively convey data has become extremely valuable. Initiating a discussion on data presentation types involves thoughtful consideration of the nature of your data and the message you aim to convey.
Always consider your audience's knowledge level and what information they need when you present your data. To present the data effectively: 1. Provide context to help the audience understand the numbers. 2. Compare data groups using visual aids. 3. Step back and view the data from the audience's perspective.
The DIKW Pyramid represents the relationships between data, information, knowledge and wisdom. Each building block is a step towards a higher level - first comes data, then is information, next is knowledge and finally comes wisdom. Each step answers different questions about the initial data and adds value to it. The more we enrich our data ...
Data refers simply to raw facts and figures. Alone it tells you nothing. The real goal is to turn data into information. Data becomes information when it's presented in a context so that it can answer a question or support decision making. And it's when this information can be combined with a manager's knowledge —their insight from ...
Just as information generates relevance from data, knowledge makes meaning from information. When information is analyzed in order to generate insights, draw conclusions, make predictions, and drive change, knowledge is created. What sets knowledge apart from information is that it also is made up of other elements such as experience and intuition.
History. Danny P. Wallace, a professor of library and information science, explained that the origin of the DIKW pyramid is uncertain: . The presentation of the relationships among data, information, knowledge, and sometimes wisdom in a hierarchical arrangement has been part of the language of information science for many years.
Data, information, and knowledge are central concepts in health informatics and data science. It is not always clear how authors define these entities and how they envision the transition from data to knowledge to work. ... Mensah & Goderre further suggest that "information is the collection, aggregation, analysis, and presentation of data that ...
Data Presentation Earn a sharable certificate Share what you've learned, and be a standout professional in your desired industry with a certificate showcasing your knowledge gained from the course.
Overview of Your Journey. Why Good Presentations Matter. Tip 1 — Understand Your Audience. Tip 2 — Use Visuals Religiously. Tip 3 — Avoid Jargon and Keep it Simple Silly. Tip 4 — Relate Your Work to the Bigger Picture. Tip 5 — Have a Memorable Bottom Line. Wrapping Up.
some of the fundamental concepts used in topic 1 Data, information, knowledge and processing, as well as in the syllabus as a whole . Section 1 lists some key terms used in this topic and their definitions. Section 2 Theory provides the basic theory that you will need for teaching this topic, including illustrative examples. Section 3
It defines data as raw facts and figures that have no meaning on their own. Information is defined as data that has been processed and given context or meaning. Knowledge is defined as understanding the rules for how to interpret and apply information. It provides examples of how data can be processed into meaningful information by giving it ...
Data represents unorganized facts, while information aggregates data to make decision making easier. Knowledge is human understanding acquired through experience dealing with information. Data becomes information through interpretation, and information can lead to knowledge. The presentation discusses how data is collected and analyzed to ...
The process of data collection, storage, coding, transmission utilization and presentation is discussed in other sections. Creation of Information ... Data, information and knowledge form a complex continuum, a dynamic state of interactions where data become information, information is used to create knowledge, and knowledge is applied to ...
Here are five steps on how to present complex data effectively with data visualization. 1. Determine Who The Audience Is. The first step when creating a data visualization is to fully determine who the audience is, their knowledge level, and their technical expertise. If you know the individuals well then you may also have an understanding of ...
Information: Data becomes information when it is organized, processed, and interpreted in a meaningful way. The information provides context and relevance to data and enables decision-making and action. Knowledge: Knowledge is the understanding gained from information, through analysis, interpretation, and synthesis.
Data, information, knowledge. Putting the three concepts. ("data," "information," and "knowledge") as done here, gives the impression of a logical hierarchy: Information is. set ...
The Data-Information-Knowledge-Wisdom (DIKW) hierarchy, or pyramid, relates data, information, knowledge, and wisdom as four layers in a pyra-mid. Data is the foundation of the pyramid, infor-mation is the next layer, then knowledge, and, finally, wisdom is the apex. DIKW is a model or construct that has been used widely within Infor-mation ...
Written by Coursera Staff • Updated on Apr 19, 2024. Data analysis is the practice of working with data to glean useful information, which can then be used to make informed decisions. "It is a capital mistake to theorize before one has data. Insensibly one begins to twist facts to suit theories, instead of theories to suit facts," Sherlock ...
Data are simply facts or figures-bits of information, but not information itself. When data are processed, interpreted, organized, structured or presented so as to make them meaningful or useful, they are called information. Information provides context for data. For example, a list of dates-data-is meaningless without the information that ...
DIKW, AI, and CDS. The DIKW framework explains that data leads to information, information to knowledge, and knowledge to wisdom. 9 This framework is particularly relevant when designing CDS tools to advance nursing practice to top of licensure for improved nurse decision-making. The DIKW framework can be used to categorize CDS tools, including those that are driven by AI.
The data-information-knowledge-wisdom model illustrates the progression from raw data to wisdom, where insights lead to effective data-driven decisions. Structured as a pyramid, the model was created to show how data can be captured in one form, analyzed and converted into another form. The pyramid includes four levels: data, information ...
The principle what we can infer from entropy values is: 1. Low entropy values mean high probability, high certainty, and hence a high degree of structurization in the data. 2. High entropy values mean low probability, low certainty (≅ high uncertainty ;-), and hence a low degree of structurization in the data.
Slide 1 of 2. 8 point infographic stages for data information and knowledge template. Slide 1 of 2. 12 month timeline stages for data information and knowledge infographic template. Slide 1 of 2. Eight step process slide for data information and knowledge infographic template. Slide 1 of 2.
Data analysts gather, clean, and study data to help guide business decisions. If you're considering a career in this in-demand field, here's one path to getting started: Get a foundational education. Build your technical skills. Work on projects with real data. Develop a portfolio of your work. Practice presenting your findings.
Presentation Transcript. Data, Information and Knowledge WJEC ICT GCSE. Starter Activity • Open the PowerPoint: • L Drive Miss Pickles Year 10 Data, Information and Knowledge • Complete Task 1: • Find a definition for these terms: • Data • Information • Knowledge. Lesson Objectives • Understand the difference between data and ...
Data engineering is the practice of designing and building systems for collecting, storing, and analyzing data at scale. It is a broad field with applications in just about every industry. Organizations have the ability to collect massive amounts of data, and they need the right people and technology to ensure it is in a highly usable state by ...
Data and AI Summit — the premier event for the global data, analytics and AI community. Register now to level up your skills. June 10-13, 2024 San Francisco + Virtual / Agenda / WHY ATTEND ... Shipping LLMs with New Knowledge. Sharon Zhou / Lamini. See Details. Generative AI 90 min. Building Production RAG Over Complex Documents. Jerry Liu ...
The definition of big data is data that contains greater variety, arriving in increasing volumes and with more velocity. This is also known as the three "Vs.". Put simply, big data is larger, more complex data sets, especially from new data sources. These data sets are so voluminous that traditional data processing software just can't ...
Copilot in PowerPoint helps you create beautiful presentations with a simple prompt, ... Business Chat works across all your business data and apps to surface the information and insights you need from a sea of data — so knowledge flows freely across the organization, saving you valuable time searching for answers. You will be able to access ...