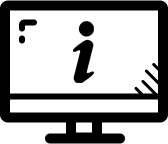
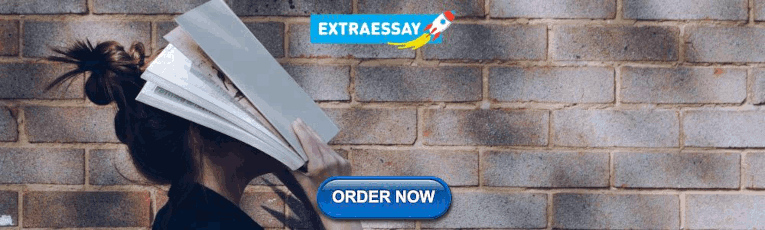
Request Brochure
- 877-650-9054
- The Value of the M.S. GIST Master’s Thesis
The M.S. GIST master’s thesis gives students the opportunity to design and produce an original, independent, professional work on a compelling topic of their choice. Students work closely with a faculty advisor who serves as the thesis committee chair and two committee members from the Spatial Sciences Institute faculty to produce an abstract and the thesis.
Thesis topics have ranged across the full suite of geographic information science and technology issues, including novel applications of GIS as well as implementations of web and mobile technology. Specific projects span a wide range of fields, including architecture, biogeography, business, geology, history , human geography, natural hazards, planetary sciences, planning, public health and sustainability.
Read previous GIS master’s theses >
Outstanding Thesis Examples
Students have produced thesis projects of publishable and award-winning caliber. Here are some examples of how our students learn the fundamental science of spatial thinking and analysis, and apply that science through technology to produce new discoveries and knowledge:
- For her thesis entitled “The Bottom Trawl Fishery in the Bering Sea, Aleutian Islands and Gulf of Alaska: A GIS-based Spatiotemporal Analysis,” Carrie Steves received the 2018 USC Spatial Sciences Institute M.S. in GIST Thesis Prize. In her thesis, she outlined that the Bering Sea, Aleutian Islands and Gulf of Alaska yield one of the largest sustainable fishing industries in the world. To ensure continued sustainable practices, the effects of fishing activity on the health of the ecosystem should be actively studied. Bottom-trawl gear is a sustainability concern due to its direct interaction with the benthic layer. Impact from bottom-trawl fisheries is difficult to assess, particularly over the long-term. Using fishery-dependent observer data from National Marine Fisheries (NMFS) provides insight on the location and the intensity of fishing effort, which can identify areas most exposed to fishing pressure. She explored the spatial and temporal extent of fishing effort in the Bering Sea and Aleutian Islands in a space-time cube in ArcGIS Pro v1.4.1 using NMFS data collected between 1993 and 2015, using various statistical techniques to examine spatio-temporal autocorrelation and clustering in this data.
- Katherine “Kelly” Wright’s thesis “The Chigoe Flea Eradication Project (CFEP) and Tungiasis eLibrary Web Mapping Applications” outlines her program to raise awareness about and to actively combat tungiasis, a painful, debilitating and disfiguring disease of poverty caused by the microscopic Chigoe flea tunga penetrans. For her thesis project, Wright used the efficacy of web GIS as a disease management strategy by establishing a collaborative virtual workspace for aid workers, aid organizations and governments of afflicted regions. She created an app for humanitarian aid workers to gather epidemiological data using volunteered geographic information (VGI) approaches. This information is enabling the medical community to develop treatment options and to help qualify tungiasis as a “neglected tropical disease” by the World Health Organization (WHO). The project’s global map provides the first-ever authoritative global spatial distribution of tungiasis. Wright was awarded the Jacques May Thesis Prize for 2018 from the Health and Medical Geography Specialty Group of the American Association of Geographers and was the 2017 USC Spatial Sciences Institute Esri Development Center Student of the Year. She co-authored the article “ Control of Tungiasis in Absence of a Roadmap: Grassroots and Global Approaches ” published in July 2017 in the journal Tropical Medicine and Infectious Disease.
- For her thesis “Quantifying Changes in Glacier Thickness and Area Using Remote Sensing and GIS: Taku Glacier System, AK,” Laura Hughes-Allen won the 2017 USC M.S. in GIST Thesis Prize. Her thesis establishes the effectiveness of using remote sensing to quantify long-term changes in glacier parameters including surface area, equilibrium line altitude (ELA) and accumulation area ratio (AAR) by combining a digitized historical topographic map, Landsat images and a USGS DEM. The results of the remote sensing analysis demonstrate significant downwasting and loss of mass at the margins of the Taku Glacier and areas of the glacier that are bounded by bedrock. Her study quantified a substantial up glacier migration of the ELA and a corresponding reduction in AAR. Comparison of the AAR associated with each Landsat scene to the established equilibrium AAR for the Taku Glacier indicated that the Taku glacier has transitioned from a long period of positive mass balance to relative equilibrium, likely presaging a new period of retreat for the Taku glacier.
- Nathan Novak received the 2016 USC Spatial Sciences Institute M.S. in GIST Thesis Prize and the 2016 UNIGIS International Association Academic Excellence competition second place prize for his thesis entitled “Predictive Habitat Modeling of Sperm Whale (Physeter macroceaphalus) within the Central Gulf of Alaska utilizing Passive Acoustic Monitoring.” In his thesis, Novak proposed an elaborate modeling of sperm whale habitats within the Central Gulf of Alaska using a range of geospatial data. His novel research also was performed in association with Bio-Waves, where he worked together with leading experts in the field of marine mammal acoustics.
- For his thesis “Geosocial Footprint (2013): Social Media Location Privacy Web Map,” Chris Weidemann developed a novel geospatial application called Twitter2GIS to analyze what locational information Twitter users may inadvertently give away and investigated how third parties could make use of this information. His study was published in the International Journal of Geoinformatics in June 2013.
- In his thesis “Delimiting the PostModern Urban Center: An Analysis of Urban Amenity Clusters in Los Angeles,” Samuel Krueger mapped the location of urban amenities in the Los Angeles metropolitan area and calculated centrality scores, which identified a strong urban core running from Santa Monica to downtown Los Angeles. The path, which he dubs “The Wilshire/Santa Monica Corridor,” is named for the two main arteries along which the city’s center is concentrated, scientifically dismissing writer Dorothy Parker’s characterization of Los Angeles as “72 suburbs in search of a city.” The Los Angeles Times covered his findings . Krueger’s thesis also earned him two prestigious recognitions, one as first place winner of the 2012 UNIGIS International Academic Excellence competition and winner of the North American Regional Science Association’s 2012 Graduate Student-Author Paper Competition.
Preparing and Defending the Thesis
First Semester: During the one-week Catalina field excursion in SSCI 587, the instructor gives a presentation on the thesis process. Students begin to consider their thesis topic, prepare a thesis abstract and prepare a thesis prospectus. They also start identifying a thesis advisor from among the faculty and two other faculty members to serve as members of their thesis guidance committee.
Third Semester: Students either improve their first-semester thesis prospectus, or they may select a new topic and prepare a new thesis prospectus.
Fourth Semester: In SSCI 594a, students finalize their thesis prospectus and the composition of their thesis guidance committee. Students schedule their presentations and meet with their committees, and they work with the SSCI 594a faculty member, their thesis advisor and writing instructor to prepare the first drafts of chapters 1-3. Students conduct research, which may entail writing code, building an app, gathering data, performing analysis or other elements germane to their topics.
Fifth Semester: In SSCI 594b, students schedule their presentations and meetings with their thesis guidance committee. They complete their research and drafts of chapters 4-5. They work with their advisor and writing instructor to prepare the final draft of their thesis, which they distribute to their committee for feedback. Students and their advisors schedule and conduct the thesis defense. Once students incorporate final changes into their thesis document, they are deemed to have successfully completed their thesis when the USC Libraries accepts it.
Writing Support
Master’s students requiring writing support can work with USC GIST writing faculty from preparation of their statement of research interest in SSCI 587 through development of their thesis in SSCI 594a. Writing faculty work individually with students to discuss strategies for better writing, to examine the strength of ideas and to ensure the integrity of analysis and discussion. While students are responsible for editing their own work, writing instructors will guide students through particular areas of difficulty, working on both global issues — idea generation, clarity of hypothesis, focus, specificity of study — as well as surface-level issues, including paragraphing, sentence structure, grammar and mechanics. GIST writing instructors are faculty members of the USC Writing Program.
Thesis Format Guidelines
Turabian’s (2018) Ninth Edition of A Manual for Writers of Research Papers, Theses, and Dissertations is used as the standard reference for style and format for the SSI M.S. programs.
- GIST Program Thesis Writing Rubric
- USC MS GIST Program Thesis Style Guide Effective Spring 2023
- USC MS GIST Program Thesis Document Template Effective Spring 2023
- Dornsife SSI PowerPoint template
Fill out the information below to learn more about the University of Southern California’s GIS Graduate Programs and download a free brochure . If you have any additional questions, please call 877-650-9054 to speak to an enrollment advisor.
University of Southern California has engaged AllCampus to help support your educational journey. AllCampus will contact you shortly in response to your request for information. About AllCampus . Privacy Policy . You may opt out of receiving communications at any time.
* All Fields are Required. Your Privacy is Protected.
In this section
- GIS Fieldwork on Catalina Island
- Master’s in Geographic Information Science and Technology
- Master’s in Human Security and Geospatial Intelligence
- Master’s in Spatial Data Science
- Master’s in Spatial Economics and Data Analysis
- Master’s in Global Security Studies
- Graduate Certificate in Geographic Information Science and Technology
- Graduate Certificate in Geospatial Intelligence
- Graduate Certificate in Geospatial Leadership
- Graduate Certificate in Remote Sensing for Earth Observation
Catalina Island Excursion
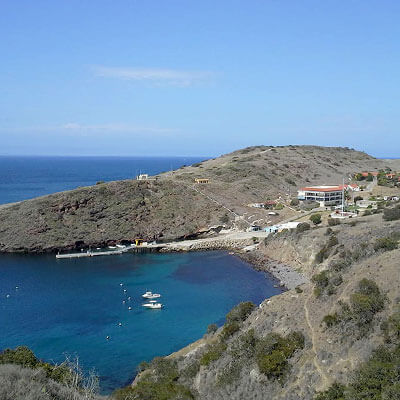
Students in the M.S. in GIST, M.S. in HSGI and Graduate Certificate in GIST programs will participate in a week-long fieldwork excursion...
Connect with us
- [email protected]
- Chat with us
- Skip to Content
- Catalog Home
- Cartography and Geographic Information Systems: Thesis, MS
This is a named option within the Cartographic and Geographic Information Systems MS .
The Department of Geography offers a thesis-based master of science in Cartography and Geographic Information Systems, titled Thesis . In addition, it offers two professional non-thesis options in the Cartography and Geographic Information Systems MS titled: GIS Development (online program) and Accelerated/ Non-Thesis (in-residence program).
Information about facilities, supporting faculty and staff, and program requirements for all graduate programs in the department can be found in the Geography listing in this catalog.
The MS (Thesis) in Cartography and Geographic Information Systems provides a broad foundation in the theory and application of mapping and geographic information sciences. Students who earn the MS (Thesis) degree are prepared to continue on for the PhD in geography or for positions in government agencies, planning organizations, environmental agencies, nongovernmental organizations, and private industry.
Please consult the table below for key information about this degree program’s admissions requirements. The program may have more detailed admissions requirements, which can be found below the table or on the program’s website.
Graduate admissions is a two-step process between academic programs and the Graduate School. Applicants must meet the minimum requirements of the Graduate School as well as the program(s). Once you have researched the graduate program(s) you are interested in, apply online .
Breadth Requirements
This program requires students to have taken coursework in several areas of Cartography to show breadth of knowledge in the field. Most students have completed the coursework for the breadth requirements prior to entering the program. Typically, these courses are not seminars. Students must have completed the equivalent of one undergraduate-level course in quantitative methods, two courses in mathematics, and two intermediate or advanced geography courses. Students who begin the program lacking one or more of the breadth courses are expected to complete such coursework during the master’s program. One course taken for breadth can also be used to fulfill degree requirements.
Graduate School Resources
Resources to help you afford graduate study might include assistantships, fellowships, traineeships, and financial aid. Further funding information is available from the Graduate School. Be sure to check with your program for individual policies and restrictions related to funding.
Program Resources
See "How Does Funding Work?" at the Geography Master's FAQ .
Minimum Graduate School Requirements
Named option requirements.
Review the Graduate School minimum academic progress and degree requirements , in addition to the program requirements listed below.
Mode of Instruction
Mode of instruction definitions.
Accelerated: Accelerated programs are offered at a fast pace that condenses the time to completion. Students typically take enough credits aimed at completing the program in a year or two.
Evening/Weekend: Courses meet on the UW–Madison campus only in evenings and/or on weekends to accommodate typical business schedules. Students have the advantages of face-to-face courses with the flexibility to keep work and other life commitments.
Face-to-Face: Courses typically meet during weekdays on the UW-Madison Campus.
Hybrid: These programs combine face-to-face and online learning formats. Contact the program for more specific information.
Online: These programs are offered 100% online. Some programs may require an on-campus orientation or residency experience, but the courses will be facilitated in an online format.
Curricular Requirements
Required courses.
GEOG 970 must be taken for 3 credits.
Graduate School Policies
The Graduate School’s Academic Policies and Procedures provide essential information regarding general university policies. Program authority to set degree policies beyond the minimum required by the Graduate School lies with the degree program faculty. Policies set by the academic degree program can be found below.
Named Option-Specific Policies
Prior coursework, graduate credits earned at other institutions.
With program approval, students are allowed to transfer no more than 6 credits of graduate coursework from other institutions. Coursework earned ten or more years prior to admission to a master’s degree is not allowed to satisfy requirements.
Undergraduate Credits Earned at Other Institutions or UW-Madison
No credits from a UW–Madison undergraduate degree are allowed to transfer toward the degree.
Credits Earned as a Professional Student at UW-Madison (Law, Medicine, Pharmacy, and Veterinary careers)
Refer to the Graduate School: Transfer Credits for Prior Coursework policy.
Credits Earned as a University Special Student at UW–Madison
With program approval, students are allowed to transfer no more than 6 credits of graduate coursework as defined above taken as a University Special student. Coursework earned ten or more years prior to admission to a master’s degree is not allowed to satisfy requirements.
The Department of Geography expects graduate students to progress through a sequence of benchmarks within prescribed time periods. These benchmarks constitute a reasonable rate of accomplishment for full-time students holding teaching or research appointments. The department recognizes that individual circumstances vary, and not all students progressing toward their academic goals will hit the benchmarks exactly. Thus, a student’s progress is considered unsatisfactory only after a period of time elapses following an unmet benchmark. A student not making satisfactory progress is placed on probation. For detailed information about these benchmarks and triggers for probationary status, please see the department's Criteria for Satisfactory Progress .
Advisor / Committee
The chair (or co-chair) of a master's student's committee is the student's advisor. This individual must be graduate faculty in Geography or affiliated with Geography. The committee must have at least three members, two of whom must be graduate faculty (or former graduate faculty up to one year after resignation/retirement). Two of the three members must be affiliated with the Geography Department. The third member may be a qualified individual from within or outside UW-Madison. Inclusion of committee members who are not UW-Madison graduate faculty must be approved by the student's advisor.
Credits Per Term Allowed
Time limits.
Refer to the Graduate School: Time Limits policy.
Grievances and Appeals
These resources may be helpful in addressing your concerns:
- Bias or Hate Reporting
- Graduate Assistantship Policies and Procedures
- Office of the Provost for Faculty and Staff Affairs
- Employee Assistance (for personal counseling and workplace consultation around communication and conflict involving graduate assistants and other employees, post-doctoral students, faculty and staff)
- Employee Disability Resource Office (for qualified employees or applicants with disabilities to have equal employment opportunities)
- Graduate School (for informal advice at any level of review and for official appeals of program/departmental or school/college grievance decisions)
- Office of Compliance (for class harassment and discrimination, including sexual harassment and sexual violence)
- Office Student Assistance and Support (OSAS) (for all students to seek grievance assistance and support)
- Office of Student Conduct and Community Standards (for conflicts involving students)
- Ombuds Office for Faculty and Staff (for employed graduate students and post-docs, as well as faculty and staff)
- Title IX (for concerns about discrimination)
Students should contact the department chair or program director with questions about grievances. They may also contact the L&S Academic Divisional Associate Deans, the L&S Associate Dean for Teaching and Learning Administration, or the L&S Director of Human Resources.
- Professional Development
Take advantage of the Graduate School's professional development resources to build skills, thrive academically, and launch your career.
Department Chair: John (Jack) Williams
Professors: Ian Baird, Erika Marin-Spiotta, Joseph Mason, Lisa Naughton, Kristopher Olds, Morgan Robertson, Robert Roth, Matthew Turner, John (Jack) Williams, Keith Woodward, A-Xing Zhu
Associate Professors: Song Gao, Asligül Göçmen, Qunying Huang, Jenna Loyd, Sarah Moore, Stephen Young
Assistant Professors: Christian Andresen, Ken Keefover-Ring, Bill Limpisathian, Almita Miranda, Jonathan Nelson
- Requirements
Contact Information
Department of Geography College of Letters & Science geography.wisc.edu
Marguerite Roulet, Graduate Program Director [email protected]
Sarah Moore, Director of Graduate Studies [email protected]
Graduate Program Handbook View Here
Graduate School grad.wisc.edu
- /api/
- /pdf/
- Explore Graduate Opportunities
- Explore UW-Madison's Undergraduate Opportunities
- Accounting and Information Systems
- African American Studies
- African Cultural Studies
- Agricultural and Applied Economics
- Agricultural and Life Sciences - College-Wide
- Animal and Dairy Sciences
- Anthropology
- Art History
- Asian Languages and Cultures
- Atmospheric and Oceanic Sciences
- Bacteriology
- Biochemistry
- Biological Systems Engineering
- Biomedical Engineering
- Biostatistics and Medical Informatics
- Business - School-Wide
- Cell and Regenerative Biology
- Chemical and Biological Engineering
- Chicana/o and Latina/o Studies
- Civil and Environmental Engineering
- Civil Society & Community Studies
- Classical and Ancient Near Eastern Studies
- Communication Arts
- Communication Sciences and Disorders
- Community and Environmental Sociology
- Computer Sciences
- Counseling Psychology
- Curriculum and Instruction
- Educational Leadership and Policy Analysis
- Educational Policy Studies
- Educational Psychology
- Electrical and Computer Engineering
- Engineering - College-Wide
- Food Science
- Forest and Wildlife Ecology
- French and Italian
- Gaylord Nelson Institute for Environmental Studies
- Gender and Women's Studies
- Cartography and Geographic Information Systems, Doctoral Minor
- Cartography and Geographic Information Systems: Accelerated/Non-Thesis, MS
- Cartography and Geographic Information Systems: GIS Development, MS
- Geography, Doctoral Minor
- Geography, MS
- Geography, PhD
- German, Nordic, and Slavic
- Graduate - School-Wide
- Human Ecology - School-Wide
- Industrial and Systems Engineering
- Information School
- Institute for Clinical and Translational Research
- Institute for Regional and International Studies
- Integrative Biology
- Journalism and Mass Communication
- Kinesiology
- La Follette School of Public Affairs
- Language Institute
- Language Sciences
- Law - School-Wide
- Life Sciences Communication
- Management and Human Resources
- Materials Science and Engineering
- Mathematics
- Mead Witter School of Music
- Mechanical Engineering
- Medical Physics
- Medicine and Public Health - School-Wide
- Nuclear Engineering and Engineering Physics
- Nursing - School-Wide
- Nutritional Sciences
- Operations and Information Management
- Pharmacy - School-Wide
- Planning and Landscape Architecture
- Plant and Agroecosystem Sciences
- Plant Pathology
- Political Science
- Population Health Sciences
- Real Estate and Urban Land Economics
- Rehabilitation Psychology and Special Education
- Religious Studies
- Risk and Insurance
- Sandra Rosenbaum School of Social Work
- Soil and Environmental Sciences
- Soil Science
- Spanish and Portuguese
- Veterinary Medicine - School-Wide
- Nondegree/Visiting Student Guide
- Pharmacy Guide
- School of Medicine and Public Health Guide
- Undergraduate Guide
- Veterinary Guide
Advertisement
GIS-based analysis of landslides susceptibility mapping: a case study of Lushoto district, north-eastern Tanzania
- Original Paper
- Published: 02 June 2023
- Volume 118 , pages 1085–1115, ( 2023 )
Cite this article
- Michael Makonyo ORCID: orcid.org/0000-0003-2124-1578 1 &
- Zahor Zahor 2
437 Accesses
3 Citations
Explore all metrics
Landslides are becoming increasingly widespread, claiming tens of thousands of fatalities, hundreds of thousands of injuries, and billions of dollars in economic losses each year. Thus, studies for geographically locating landslides, vulnerable areas have been increasingly relevant in recent decades. This research is aimed at integrating Geographical Information Systems (GIS) and Remote Sensing (RS) techniques to delineate landslides susceptibility areas of Lushoto district, Tanzania. RS assisted in providing remote datasets including; Digital Elevation Models (DEMs), Landsat 8 OLI imageries, and past spatially distributed landslides coordinate with the use of a handheld Global Position System (GPS) receiver, while various GIS analysis techniques were used in the preparation and analysis of landslides influencing factors hence, generating landslides susceptibility areas index values. However, rainfall, slope angle, elevation, soil type, lithology, proximity to roads, rivers, faults, and Normalized Difference Vegetation Index (NDVI) factors were found to have a direct influence on the occurrence of landslides in the study area. These factors were evaluated, weighted, and ranked using Analytical Hierarchy Process (AHP) technique in which a 0.086 (8.6%) Consistency Ratio (CR) was attained (highly accepted). Findings reveal that rainfall (29.97%), slopes’ angle (21.72%), elevation (15.68%), and soil types (11.77%) were found to have high influence on the occurrence of landslides, while proximity to faults (8.35%), lithology (4.94%), proximity to roads (3.41%), rivers (2.48%), and NDVI (1.69%) had very low influences, respectively. The overall results, obtained through Weighted Linear Combination (WLC) analysis techniques indicate that about 97669.65 Hectares (ha) of land are under very low levels of landslides susceptibility, which accounts for 24.03% of the total study area. Low susceptibility levels had 123105.84 ha (30.28%), moderate landslides susceptibility areas were found to have 140264.79 ha (34.50%), while high and very high susceptibility areas were found to cover about 45423.43 ha (11.17%) and 57.78 ha (0.01%), respectively. Furthermore, 81% overall model accuracy was obtained as computed from the Area Under the Curve (AUC) using Receiver Operating Characteristic (ROC) curve.
This is a preview of subscription content, log in via an institution to check access.
Access this article
Price includes VAT (Russian Federation)
Instant access to the full article PDF.
Rent this article via DeepDyve
Institutional subscriptions
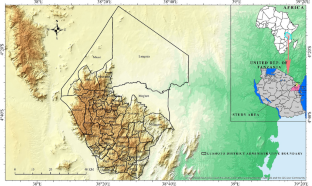
Similar content being viewed by others
Landslide susceptibility mapping using gis and weighted overlay method: a case study from nw himalayas, pakistan.
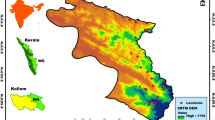
A framework employing the AHP and FR methods to assess the landslide susceptibility of the Western Ghats region in Kollam district
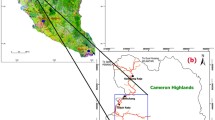
Landslide susceptibility mapping using GIS-based statistical models and Remote sensing data in tropical environment
Abdo H (2022) Assessment of landslide susceptibility zonation using frequency ratio and statistical index: a case study of Al-Fawar basin, Tartous, Syria. Int J Environ Sci Technol 19(4):2599–2618
Article Google Scholar
Abedini M, Ghasemian B, Shirzadi A, Shahabi H, Chapi K, Pham BT, Ahmad B, B., Bui T, D (2019) A novel hybrid approach of bayesian logistic regression and its ensembles for landslide susceptibility assessment. Geocarto Int 34(13):1427–1457. https://doi.org/10.1080/10106049.2018.1499820
Agliardi F, Crosta GB, Frattini P (2012) 18 slow rock-slope deformation. Landslides: Types mechanisms modeling Earth Systems and Environment, 207
Ayalew L, Yamagishi H (2005) The application of GIS-based logistic regression for landslide susceptibility mapping in the Kakuda-Yahiko Mountains, Central Japan. Geomorphology 65(1–2):15–31. https://doi.org/10.1016/j.geomorph.2004.06.010
Ayalew L, Yamagishi H, Ugawa N (2004) Landslide susceptibility mapping using GIS-based weighted linear combination, the case in Tsugawa area of Agano River, Niigata Prefecture, Japan. Landslides 1(1):73–81. https://doi.org/10.1007/s10346-003-0006-9
Baharvand S, Rahnamarad J, Soori S, Saadatkhah N (2020) Landslide susceptibility zoning in a catchment of Zagros mountains using fuzzy logic and GIS. Environ Earth Sci 79:1–10. https://doi.org/10.1007/s12665-020-08957-w
Bahrami Y, Hassani H, Maghsoudi A (2020) Landslide susceptibility mapping using AHP and fuzzy methods in the Gilan province. Iran Geoj 1–20. https://doi.org/10.1007/s10708-020-10162-y
Berhane G, Kebede M, Alfarah N, Hagos E, Grum B, Giday A, Abera T (2020) Landslide susceptibility zonation mapping using GIS-based frequency ratio model with multi-class spatial data-sets in the Adwa-Adigrat mountain chains, northern Ethiopia. J Afr Earth Sc 164:103795. https://doi.org/10.1016/j.jafrearsci.2020.103795
Biswas S, Mukhopadhyay BP, Bera A (2020) Delineating groundwater potential zones of agriculture dominated landscapes using GIS based AHP techniques: a case study from Uttar Dinajpur district, West Bengal. Environ Earth Sci 79(12):1–25
Bostjančić I, Filipović M, Gulam V, Pollak D (2021) Regional-Scale Landslide Susceptibility Mapping using limited LiDAR-Based landslide inventories for Sisak-Moslavina County, Croatia. Sustainability 13(8):4543. https://doi.org/10.3390/su13084543
Braslow J, Cordingley J (2016) Participatory mapping in Lushoto district, Tanzania. International Center for Tropical Agriculture (CIAT)
Brenning A (2005) Spatial prediction models for landslide hazards: review, comparison and evaluation. Nat Hazards Earth Syst Sci 5(6):853–862. https://doi.org/10.5194/nhess-5-853-2005
CRED (2018) The human cost of natural disasters, a global perspective. http://repo.floodalliance.net/jspui/44111/1165
Chalise D, Kumar L, Kristiansen P (2019) Land degradation by soil erosion in Nepal: a review. Soil Syst 3(1):12. https://doi.org/10.3390/soilsystems3010012
Chen X, Chen W (2021) GIS-based landslide susceptibility assessment using optimized hybrid machine learning methods. CATENA 196:104833. https://doi.org/10.1016/j.catena.2020.104833
Chen W, Pradhan B, Li S, Shahabi H, Rizeei HM, Hou E, Wang S (2019a) Novel hybrid integration approach of bagging-based fisher’s linear discriminant function for groundwater potential analysis. Nat Resour Res 28(4):1239–1258. https://doi.org/https://doi.org/10.1007/s11053-019-09465-w
Chen W, Shahabi H, Shirzadi A, Hong H, Akgun A, Tian Y, Liu J, Zhu A, Li S (2019b) Novel hybrid artificial intelligence approach of bivariate statistical-methods-based kernel logistic regression classifier for landslide susceptibility modeling. Bull Eng Geol Environ 78(6):4397–4419
Chen Z, Song D, Juliev M, Pourghasemi HR (2021) Landslide susceptibility mapping using statistical bivariate models and their hybrid with normalized spatial-correlated scale index and weighted calibrated landslide potential model. Environ Earth Sci 80(8):1–19. https://doi.org/10.1007/s12665-021-09603-9
Chen W, Sun Z, Han JJA (2019c) s. Landslideusceptibility modeling using integrated ensemble weights of evidence with logistic regression and random forest models. Appliedciences, 9(1), 171
Collins BD, Jibson RW (2015) Assessment of existing and potential landslide hazards resulting from the April 25, 2015 Gorkha, Nepal earthquake sequence (2331 – 1258)
Dang V-H, Dieu TB, Tran X-L, Hoang N-D (2019) Enhancing the accuracy of rainfall-induced landslide prediction along mountain roads with a GIS-based random forest classifier. Bull Eng Geol Environ 78(4):2835–2849. https://doi.org/10.1007/s10064-018-1273-y
Das G, Lepcha K (2019) Application of logistic regression (LR) and frequency ratio (FR) models for landslide susceptibility mapping in Relli Khola river basin of Darjeeling Himalaya, India. Appl Sci 1(11):1–22. https://doi.org/10.1007/s42452-019-1499-8
Das IC (2011) Spatial statistical modelling for assessing landslide hazard and vulnerability
Delcamp A, Kwelwa S, Macheyeki A, De Kervyn M (2013) Multiple collapses at Mt Meru volcano, Tanzania: remote sensing and field evidences from debris avalanche deposits. EGU General Assembly Conference Abstracts
Demir G, Aytekin M, Akgün A, Ikizler SB, Tatar O (2013) A comparison of landslide susceptibility mapping of the eastern part of the North Anatolian Fault Zone (Turkey) by likelihood-frequency ratio and analytic hierarchy process methods. Nat Hazards 65(3):1481–1506. https://doi.org/10.1007/s11069-012-0418-8
Desalegn H, Mulu A, Damtew B (2022) Landslide susceptibility evaluation in the Chemoga watershed, upper Blue Nile, Ethiopia. Nat Hazards 113(2):1391–1417
Devkota KC, Regmi AD, Pourghasemi HR, Yoshida K, Pradhan B, Ryu IC, Dhital MR, Althuwaynee OF (2013) Landslide susceptibility mapping using certainty factor, index of entropy and logistic regression models in GIS and their comparison at Mugling–Narayanghat road section in Nepal Himalaya. Nat Hazards 65(1):135–165. https://doi.org/10.1007/s11069-012-0347-6
Dhamija S, Joshi H (2022) Prediction of Groundwater Arsenic Hazard employing Geostatistical Modelling for the Ganga Basin, India. Water 14(15):2440
Dou J, Yunus AP, Bui DT, Merghadi A, Sahana M, Zhu Z, Chen C-W, Khosravi K, Yang Y, Pham BT (2019) Assessment of advanced random forest and decision tree algorithms for modeling rainfall-induced landslide susceptibility in the Izu-Oshima Volcanic Island, Japan. Sci Total Environ 662:332–346. https://doi.org/10.1016/j.scitotenv.2019.01.221
Díaz SR, Cadena E, Adame S, Dávila N (2020) Landslides in Mexico: their occurrence and social impact since 1935. Landslides 17(2):379–394. https://doi.org/10.1007/s10346-019-01285-6
Faber B (2003) Slope and aspect effect on evaporation as measured by atmometer. IV International Symposium on Irrigation of Horticultural Crops 664
Fontijn K, Williamson D, Mbede E, Ernst GG (2012) The rungwe volcanic province, Tanzania–a volcanological review. ournal of African Earth Sciences 63:12–31. https://doi.org/10.1016/j.jafrearsci.2011.11.005
Froude MJ, Petley DN (2018) Global fatal landslide occurrence from 2004 to 2016. Nat Hazards Earth Syst Sci 18(8):2161–2181. https://doi.org/10.5194/nhess-18-2161-2018
Gheshlaghi HA, Feizizadeh B (2021) GIS-based ensemble modelling of fuzzy system and bivariate statistics as a tool to improve the accuracy of landslide susceptibility mapping. Nat Hazards https://doi.org/10.1007/s11069-021-04673-1
Guha-Sapir D, Below R, Hoyois P (2020) EM-DAT: international disaster database. Brussels, Belgium: Université Catholique de Louvain http://www.emdat.be
Gupta V, Paul A, Kumar S, Dash B (2021) Spatial distribution of landslides vis-à-vis epicentral distribution of earthquakes in the vicinity of the Main Central Thrust zone, Uttarakhand Himalaya. India Curr Sci 120(12):1927–1932
Hamzeh S, Amiri A (2020) Landslide hazard zoning using weighted overlay and Analytic Network process methods-case study: Nasrabad region in Golestan Province. Scientific-Research Q Geographical Data 29(114):117–132. https://doi.org/10.22131/SEPEHR.2020.44597
Haque U, Da Silva PF, Devoli G, Pilz J, Zhao B, Khaloua A, Wilopo W, Andersen P, Lu P, Lee J (2019) The human cost of global warming: deadly landslides and their triggers (1995–2014). Sci Total Environ 682:673–684. https://doi.org/10.1016/j.scitotenv.2019.03.415
Hong H, Liu J, Bui DT, Pradhan B, Acharya TD, Pham BT, Zhu A-X, Chen W, Ahmad BB (2018) Landslide susceptibility mapping using J48 decision tree with AdaBoost, Bagging and Rotation Forest ensembles in the Guangchang area (China). CATENA 163:399–413. https://doi.org/10.1016/j.catena.2018.01.005
Hong H, Pourghasemi HR, Pourtaghi ZS (2016) Landslide susceptibility assessment in Lianhua County (China): a comparison between a random forest data mining technique and bivariate and multivariate statistical models. Geomorphology 259:105–118. https://doi.org/10.1016/j.geomorph.2016.02.012
Igwe O (2018) The characteristics and mechanisms of the recent catastrophic landslides in Africa under IPL and WCoE projects. Landslides 15(12):2509–2519. https://doi.org/10.1007/s10346-018-1064-3
Iqbal J, Cui P, Hussain ML, Pourghasemi HR, Cheng D-Q, Shah SU, Pradhan B (2021) Landslide Susceptibility Assessment Along The Dubair-Dudishal Section of The Karakoram Higway, Northwestern Himalayas, Pakistan. Acta Geodyn. Geomater, Vol. 18, No. 2 ((202),), 137–155, 2021. https://doi.org/10.13168/AGG.2021.0010
Jam AS, Mosaffaie J, Sarfaraz F, Shadfar S, Akhtari R (2021) GIS-based landslide susceptibility mapping using hybrid MCDM models. Nat Hazards. https://doi.org/10.1007/s11069-021-04718-5
Jennifer JJ, Saravanan S, Abijith D (2021) Application of frequency ratio and logistic regression model in the Assessment of Landslide susceptibility mapping for Nilgiris District, Tamilnadu, India. Indian Geotech J. https://doi.org/10.1007/s40098-021-00520-z
Kamugisha M, Gesase S, Minja D, Mgema S, Mlwilo T, Mayala B (2007) Pattern and spatial distribution of plague in Lushoto, north-eastern Tanzania. Tanzan J Health Res 9(1):12–18
Kavzoglu T, Sahin EK, Colkesen I (2014) Landslide susceptibility mapping using GIS-based multi-criteria decision analysis, support vector machines, and logistic regression. Landslides 11(3):425–439. https://doi.org/10.1007/s10346-013-0391-7
Kawabata D, Bandibas J (2009) Landslide susceptibility mapping using geological data, a DEM from ASTER images and an Artificial neural network (ANN). Geomorphology 113(1–2):97–109. https://doi.org/10.1016/j.geomorph.2009.06.006
Kayastha P, Dhital MR, De Smedt F (2013) Application of the analytical hierarchy process (AHP) for landslide susceptibility mapping: a case study from the Tinau watershed, west Nepal. Computers Geosci 52:398–408. https://doi.org/10.1016/j.cageo.2012.11.003
Kervyn M, Ernst GG, Klaudius J, Keller J, Kervyn F, Mattsson HB, Belton F, Mbede E, Jacobs P (2008) Voluminous lava flows at Oldoinyo Lengai in 2006: chronology of events and insights into the shallow magmatic system. Bull Volcanol 70(9):1069–1086. https://doi.org/10.1007/s00445-007-0190-x
Kirschbaum D, Stanley T, Zhou Y (2015) Spatial and temporal analysis of a global landslide catalog. Geomorphology 249:4–15. https://doi.org/10.1016/j.geomorph.2015.03.016
Knapen A, Kitutu MG, Poesen J, Breugelmans W, Deckers J, Muwanga A (2006) Landslides in a densely populated county at the footslopes of Mount Elgon (Uganda): characteristics and causal factors. Geomorphology 73(1–2):149–165. https://doi.org/10.1016/j.geomorph.2005.07.004
Lai J-S, Tsai F (2019) Improving GIS-based landslide susceptibility assessments with multi-temporal remote sensing and machine learning. Sensors 19(17):3717. https://doi.org/10.3390/s19173717
Lee S, Min K (2001) Statistical analysis of landslide susceptibility at Yongin, Korea. Environ Geol 40(9):1095–1113
Lepore C, Kamal SA, Shanahan P, Bras RL (2012) Rainfall-induced landslide susceptibility zonation of Puerto Rico. Environ Earth Sci 66(6):1667–1681. https://doi.org/10.1007/s12665-011-0976-1
Li Y, Chen W (2020) Landslide susceptibility evaluation using hybrid integration of evidential belief function and machine learning techniques. Water 12(1):113. https://doi.org/10.3390/w12010113
Löbmann MT, Geitner C, Wellstein C, Zerbe S (2020) The influence of herbaceous vegetation on slope stability–A review. Earth Sci Rev, 103328
Makonyo M, Msabi MM (2021a) Identification of Groundwater potential recharge zones using GIS-Based Multi-Criteria decision analysis: a Case Study of Semi-Arid Midlands Manyara fractured Aquifer, North-Eastern Tanzania. Remote Sens Appl Soc Environ Asia. https://doi.org/10.1016/j.rsase.2021.100544
Makonyo M, Msabi MM (2021b) Potential landfill sites selection using GIS-based multi-criteria decision analysis in Dodoma capital city, central Tanzania. GeoJournal. https://doi.org/10.1007/s10708-021-10414-5
Malczewski J (1999) GIS and multicriteria decision analysis. John Wiley & Sons
Massawe HJB (2011) Landform and soil analysis for predicting distribution of plague reservoirs and vectors in Mavumo area, Lushoto District, Tanzania. Sokoine University of Agriculture]
Meena SR, Mishra BK, Tavakkoli Piralilou S (2019) A hybrid spatial multi-criteria evaluation method for mapping landslide susceptible areas in kullu valley, himalayas. Geosciences 9(4):156. https://doi.org/10.3390/geosciences9040156
Melese T, Belay T, Andemo A (2022) Application of analytical hierarchal process, frequency ratio, and Shannon entropy approaches for landslide susceptibility mapping using geospatial technology: the case of Dejen district, Ethiopia. Arab J Geosci 15(5):424
Meng Q, Miao F, Zhen J, Wang X, Wang A, Peng Y, Fan Q (2016) GIS-based landslide susceptibility mapping with logistic regression, analytical hierarchy process, and combined fuzzy and support vector machine methods: a case study from Wolong Giant Panda Natural Reserve, China. Bull Eng Geol Environ 75(3):923–944. https://doi.org/10.1007/s10064-015-0786-x
Michael EA, Samanta S (2016) Landslide vulnerability mapping (LVM) using weighted linear combination (WLC) model through remote sensing and GIS techniques. Model Earth Syst Environ 2(2):1–15
Moragues S, Lenzano MG, Lanfri M, Moreiras S, Lannutti E, Lenzano L (2021) Analytic hierarchy process applied to landslide susceptibility mapping of the North Branch of Argentino Lake, Argentina. Nat Hazards 105(1):915–941. https://doi.org/10.1007/s11069-020-04343-8
Morandi DT, de Jesus França LC, Menezes ES, Machado ELM, da Silva MD, Mucida DP (2020) Delimitation of ecological corridors between conservation units in the brazilian cerrado using a GIS and AHP approach. Ecol Ind 115:106440. https://doi.org/10.1016/j.ecolind.2020.106440
Moreiras SM (2009) Análisis estadístico probabilístico de las variables que condicionan la inestabilidad de las laderas en los valles de los ríos Las Cuevas y Mendoza. Revista de la Asociación Geológica Argentina 65(4):780–790
Google Scholar
Motamedi M (2013) Quantitative landslide Hazard Assessment in Regional Scale using statistical modeling techniques. University of Akron]
Msabi MM, Makonyo M (2021) Flood susceptibility mapping using GIS and multi-criteria decision analysis: a case of Dodoma region, central Tanzania. Remote Sens Applications: Soc Environ Asia 21:100445
Mugagga F, Kakembo V, Buyinza M (2012) Land use changes on the slopes of Mount Elgon and the implications for the occurrence of landslides. CATENA 90:39–46. https://doi.org/10.1016/j.catena.2011.11.004
Mücher CA (2009) Geo-spatial modelling and monitoring of european landscapes and habitats using remote sensing and field surveys. Wageningen University and Research
NBS NB, o. S (2012) Tanzania – Population and Housing Census 2012. Dar es Salaam: Ministry of Finance Office of Chief Government Statistician President’s Office – Finance, Economy and Development Planning
Nama E (2020) Modelling variables to predict landslides in the south west flank of the Cameroon volcanic line, Cameroon, West Africa. Unsaturated Soils for Asia. CRC Press, pp 813–817
Neerinckx S, Peterson AT, Gulinck H, Deckers J, Kimaro D, Leirs H (2010) Predicting potential risk areas of human plague for the western Usambara Mountains, Lushoto District, Tanzania. Am J Trop Med Hyg 82(3):492
Nguyen VV, Pham BT, Vu BT, Prakash I, Jha S, Shahabi H, Shirzadi A, Ba DN, Kumar R, Chatterjee JM (2019) Hybrid machine learning approaches for landslide susceptibility modeling. Forests 10(2):157. https://doi.org/10.3390/f10020157
Nyssen J, Moeyersons J, Poesen J, Deckers J, Haile M (2003) The environmental significance of the remobilisation of ancient mass movements in the atbara–tekeze headwaters, Northern Ethiopia. Geomorphology 49(3–4):303–322. https://doi.org/10.1016/S0169-555X(02)00192-7
Okuwaki R, Fan W, Yamada M, Osawa H, Wright TJ (2021) Identifying landslides from continuous seismic surface waves: a case study of multiple small-scale landslides triggered by Typhoon Talas, 2011. Geophys J Int 226(2):729–741
Ozioko O, Igwe O (2020) GIS-based landslide susceptibility mapping using heuristic and bivariate statistical methods for Iva Valley and environs Southeast Nigeria. Environ Monit Assess 192(2):1–19. https://doi.org/10.1007/s10661-019-7951-9
Panchal S, Shrivastava AK (2020) Application of analytic hierarchy process in landslide susceptibility mapping at regional scale in GIS environment. J Stat Manage Syst 23(2):199–206. https://doi.org/10.1080/09720510.2020.1724620
Rahaman SA, Aruchamy (2017) Geoinformatics based landslide vulnerable zonation mapping using analytical hierarchy process (AHP), a study of Kallar river sub watershed, Kallar watershed, Bhavani basin, Tamil Nadu. Model Earth Syst Environ Asia 3(1):41. https://doi.org/10.1007/s40808-017-0298-8
Regmi AD, Dhital MR, Zhang J-q, Su L-j, Chen X-q (2016) Landslide susceptibility assessment of the region affected by the 25 April 2015 Gorkha earthquake of Nepal. J Mt Sci 13(11):1941–1957. https://doi.org/10.1007/s11629-015-3688-2
Reichenbach P, Rossi M, Malamud BD, Mihir M, Guzzetti F (2018) A review of statistically-based landslide susceptibility models. Earth Sci Rev 180:60–91. https://doi.org/10.1016/j.earscirev.2018.03.001
Riaz MT, Basharat M, Hameed N, Shafique M, Luo J (2018) A data-driven approach to landslide-susceptibility mapping in mountainous terrain: case study from the Northwest Himalayas, Pakistan. Nat Hazards Rev 19(4):05018007
Roccati A, Paliaga G, Luino F, Faccini F, Turconi L (2021) GIS-Based landslide susceptibility mapping for Land Use Planning and Risk Assessment. Land 10(2):162. https://doi.org/10.3390/land10020162
Rossi M, Reichenbach P (2016) LAND-SE: a software for statistically based landslide susceptibility zonation, version 1.0. Geosci Model Dev 9(10):3533–3543. https://doi.org/10.5194/gmd-9-3533-2016
Saaty TL (1997) That is not the analytic hierarchy process: what the AHP is and what it is not. J Multi-Criteria Decis Anal 6(6):324–335
Saaty T (1980) The analytic Hierarchy Process. McGrawHill international. New York, NY, USA
Saaty TL, Vargas LG (2001) How to make a decision. In: Models, methods, concepts & applications of the analytic hierarchy process (pp. 1–25). Springer. https://doi.org/10.1007/978-1-4615-1665-1_1
Sarkar S, Kanungo DP (2017) GIS application in landslide susceptibility mapping of Indian Himalayas. In GIS Landslide (pp. 211–219). Springer. https://doi.org/10.1007/978-4-431-54391-6_12
Shano L, Raghuvanshi TK, Meten M (2021) Landslide susceptibility mapping using frequency ratio model: the case of Gamo highland, South Ethiopia. Arab J Geosci 14(7):1–18
Sharma A, Sur U, Singh P, Rai PK, Srivastava PK (2020) Probabilistic landslide hazard assessment using statistical information value (SIV) and GIS techniques: a case study of Himachal Pradesh, India. Tech Dis Risk Manag Mitigation 197:208. https://doi.org/10.1002/9781119359203.ch15
Silalahi FES, Arifianti Y, Hidayat F (2019) Landslide susceptibility assessment using frequency ratio model in Bogor, West Java, Indonesia. Geoscience Lett 6(1):1–17. https://doi.org/10.1186/s40562-019-0140-4
Singh N, Chakrapani G (2015) ANN modelling of sediment concentration in the dynamic glacial environment of Gangotri in Himalaya. Environ Monit Assess 187(8):494
Singh P, Sharma A, Sur U, Rai PK (2021) Comparative landslide susceptibility assessment using statistical information value and index of entropy model in Bhanupali-Beri region, Himachal Pradesh, India. Environ Dev Sustain 23(4):5233–5250. https://doi.org/10.1007/s10668-020-00811-0
Singh RG (2009) Landslide classification, characterization and susceptibility modeling in KwaZulu-Natal. University of the Witwatersrand]
Swetha T, Gopinath G (2020) Landslides susceptibility assessment by analytical network process: a case study for Kuttiyadi river basin (western ghats, southern India). SN Appl Sci 2(11):1–12. https://doi.org/10.1007/s42452-020-03574-5
Tegeje J (2017) Review of spatial and temporal distribution of Landslides in Tanzania. Ecosyst Ecography 7(243):2. https://doi.org/10.4172/2157-7625.1000243
Tian Y, Xu C, Hong H, Zhou Q, Wang D, Risk (2019) Mapping earthquake-triggered landslide susceptibility by use of artificial neural network (ANN) models: an example of the 2013 Minxian (China) Mw 5.9 event. Geomatics Nat Hazards 10(1):1–25. https://doi.org/10.1080/19475705.2018.1487471
Tofelde S, Düsing W, Schildgen TF, Wittmann H, Alonso RN, Strecker MR (2017) Changes in denudation rates and erosion processes in the transition from a low-relief, arid orogen interior to a high-relief, humid mountain-front setting, Toro Basin, southern Central Andes. AGU Fall Meeting Abstracts
Torizin J (2011) Bivariate statistical method for landslide susceptibility analysis using ArcGis. Project of technical cooperation ‘mitigation of georisks’. BGR-Report publication, Hannover
Turconi L, Luino F, Gussoni M, Faccini F, Giardino M, Casazza M (2019) Intrinsic environmental vulnerability as shallow landslide susceptibility in Environmental Impact Assessment. Sustainability 11(22):6285. https://doi.org/10.3390/su11226285
Turner AK (2018) Social and environmental impacts of landslides. Innovative Infrastructure Solutions 3(1):1–25. https://doi.org/10.1007/s41062-018-0175-y
Wang L, Wei S, Horton R (2011) Effects of vegetation and slope aspect on water budget in the hill and gully region of the Loess Plateau of China. CATENA 87(1):90–100. https://doi.org/10.1016/j.catena.2011.05.010
Westerberg L-O, Christiansson C (1999) Highlands in East Africa: unstable slopes, unstable environments? Ambio , 419–429
Wubalem A, Meten M (2020) Landslide susceptibility mapping using information value and logistic regression models in Goncha Siso Eneses area, northwestern Ethiopia. SN Appl Sci 2(5):1–19. https://doi.org/10.1007/s42452-020-2563-0
Youssef AMJE (2015) e. s. Landslide susceptibility delineation in the Ar-Rayth area, Jizan, Kingdom of Saudi Arabia, using analytical hierarchy process, frequency ratio, and logistic regression models. 73 (12), 8499–8518
Zhu A-X, Miao Y, Yang L, Bai S, Liu J, Hong H (2018) Comparison of the presence-only method and presence-absence method in landslide susceptibility mapping. Catena 171(222):233. https://doi.org/10.1016/j.catena.2018.07.012
Download references
Acknowledgements
We thank the University of Dodoma (UDOM) for providing financial support in facilitating this project.
Author information
Authors and affiliations.
Department of Geology, College of Earth Sciences and Engineering, The University of Dodoma, Dodoma, Tanzania
Michael Makonyo
Department of Geography, University of Dar es Salaam, Dar es Salaam, Tanzania
Zahor Zahor
You can also search for this author in PubMed Google Scholar
Contributions
MM performed funding acquisition, collected and analysed data, as well as drafted this manuscript. ZZ guided the research procedures, reviewed, and edited the manuscript. Both authors read and approved the final manuscript
Corresponding author
Correspondence to Michael Makonyo .
Ethics declarations
Conflict of interest.
There is no potential conflict of interest
Additional information
Publisher’s note.
Springer Nature remains neutral with regard to jurisdictional claims in published maps and institutional affiliations.
Rights and permissions
Springer Nature or its licensor (e.g. a society or other partner) holds exclusive rights to this article under a publishing agreement with the author(s) or other rightsholder(s); author self-archiving of the accepted manuscript version of this article is solely governed by the terms of such publishing agreement and applicable law.
Reprints and permissions
About this article
Makonyo, M., Zahor, Z. GIS-based analysis of landslides susceptibility mapping: a case study of Lushoto district, north-eastern Tanzania. Nat Hazards 118 , 1085–1115 (2023). https://doi.org/10.1007/s11069-023-06038-2
Download citation
Received : 30 November 2022
Accepted : 19 May 2023
Published : 02 June 2023
Issue Date : September 2023
DOI : https://doi.org/10.1007/s11069-023-06038-2
Share this article
Anyone you share the following link with will be able to read this content:
Sorry, a shareable link is not currently available for this article.
Provided by the Springer Nature SharedIt content-sharing initiative
- Find a journal
- Publish with us
- Track your research
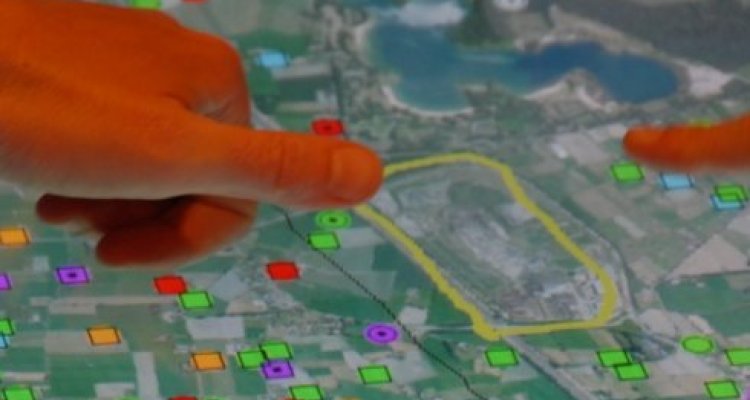
Thesis - Geo-information Science and Remote Sensing
The thesis is a compulsory part of every Master study programme of Wageningen University & Research. A major thesis is between 24 and 39 Ects and is at least 36 Ects for the master programmes Geo-information Science (MGI), Urban Environmental Management (MUE) and Biosystems Engineering (MBE).
Geo-information science thesis topics are narrowly related to the research programme of the Laboratory of Geo-informationt Science and Remote Sensing (GIRS). This research program covers a wide range of subjects. The following main themes have been selected to delineate the laboratory's identity:
- Sensing & measuring
- Modelling & visualization
- Integrated land monitoring
- Human - space interaction
- Empowering & engaging communities
The thesis research is conducted under supervision of a staff member of the GIRS group, but might also take place in another institute or company.
- Students have to follow the GRS thesis procedure. Complete guidelines for doing a thesis Geo-information Science are available via Brightspace.
- Required documents and forms are available via Brightspace. Contact us for access to this Brightspace page.
- For the planning of the thesis research an overview with dates for the midterm presentations and colloquia are scheduled.
- Thesis topics can be selected from the GRS thesis topic list or under conditions be proposed by individual students.
- Past thesis projects can be consulted.
Compulsory course in
- Master Geo-Information Science (MGI)
Restricted Optional in
- Master Biosystems Engineering (MBE)
- Master Urban Environmental Management (MUE)
- Bibliography
- More Referencing guides Blog Automated transliteration Relevant bibliographies by topics
- Automated transliteration
- Relevant bibliographies by topics
- Referencing guides
Dissertations / Theses on the topic 'Geographical Information Systems; GIS Geography Education'
Create a spot-on reference in apa, mla, chicago, harvard, and other styles.
Consult the top 44 dissertations / theses for your research on the topic 'Geographical Information Systems; GIS Geography Education.'
Next to every source in the list of references, there is an 'Add to bibliography' button. Press on it, and we will generate automatically the bibliographic reference to the chosen work in the citation style you need: APA, MLA, Harvard, Chicago, Vancouver, etc.
You can also download the full text of the academic publication as pdf and read online its abstract whenever available in the metadata.
Browse dissertations / theses on a wide variety of disciplines and organise your bibliography correctly.
West, Bryan A. "Conceptions of geographic information systems (GIS) held by senior geography students in Queensland." Queensland University of Technology, 2008. http://eprints.qut.edu.au/16682/.
Parsons, Edward Michael. "Parental choice in secondary education : socio-spatial dynamics and motivation." Thesis, University of Plymouth, 1999. http://hdl.handle.net/10026.1/435.
Tse, To-fun. "Integrating GIS into the geography curriculum of Hong Kong schools." Click to view the E-thesis via HKUTO, 2005. http://sunzi.lib.hku.hk/hkuto/record/B31940390.
Tse, To-fun, and 謝道勳. "Integrating GIS into the geography curriculum of Hong Kong schools." Thesis, The University of Hong Kong (Pokfulam, Hong Kong), 2005. http://hub.hku.hk/bib/B31940390.
Austin, Brittany Grace. "Investigating the Influence of Esri Story Map Design on Partcipation in Sustainability-Related Activities." TopSCHOLAR®, 2018. https://digitalcommons.wku.edu/theses/2571.
Joyce, Kerry Eloise. "Public health decision making : the value of geographical information systems (GIS) mapping." Thesis, Northumbria University, 2007. http://nrl.northumbria.ac.uk/2652/.
Chaudhry, Omair. "Modelling geographic phenomena at multiple levels of detail : a model generalisation approach based on aggregation." Thesis, University of Edinburgh, 2008. http://hdl.handle.net/1842/2385.
Williams, Jeremy Hugh. "Monitoring the condition of semi-natural vegetation : the application of remote sensing and geographical information systems (GIS)." Thesis, Bangor University, 1992. https://research.bangor.ac.uk/portal/en/theses/monitoring-the-condition-of-seminatural-vegetation--the-application-of-remote-sensing-and-geographical-information-systems-gis(c90702cb-be4f-454f-aba1-1c74484c0093).html.
Wu, Changshan. "Remote sensing, geographical information systems, and spatial modeling for analyzing public transit services." Columbus, Ohio : Ohio State University, 2003. http://rave.ohiolink.edu/etdc/view?acc%5Fnum=osu1060071466.
Ousley, Chris. "A Geographic-Information-Systems-Based Approach to Analysis of Characteristics Predicting Student Persistence and Graduation." Diss., The University of Arizona, 2010. http://hdl.handle.net/10150/194256.
Simmons, Matthew Earl. "Studies on bottomland hardwood forest restoration and teaching with geographic information systems (GIS) in ecology labs." [College Station, Tex. : Texas A&M University, 2007. http://hdl.handle.net/1969.1/ETD-TAMU-1374.
Seremet, Mehmet. "Geographic Information Systems (GIS) in higher education : a study of provision, pedagogy and employability in the United Kingdom and Turkey." Thesis, University of Plymouth, 2013. http://hdl.handle.net/10026.1/1489.
Naibbi, Ali Ibrahim. "Fuelwood and vegetation change in northern Nigeria : an exploration using remote sensing (RS), geographical information systems (GIS) and field reports." Thesis, University of Portsmouth, 2013. https://researchportal.port.ac.uk/portal/en/theses/fuelwood-and-vegetation-change-in-northern-nigeria(0b2c79ea-0943-4a21-aa22-cdd9b6e6fd64).html.
Klinsky, Sonja. "Making and using environmental information : an analysis of the development and use of two GIS tools for public environmental engagement." Thesis, McGill University, 2004. http://digitool.Library.McGill.CA:80/R/?func=dbin-jump-full&object_id=83115.
Samdadia, Viren V. "Integration of 3 dimensional parametric building model with geographic information systems in educational facilities planning and management." Link to electronic thesis, 2004. http://www.wpi.edu/Pubs/ETD/Available/etd-0608104-113001/.
Langley, Robert James. "The use and development of geographical information systems (GIS) and spatial modelling for educational planning." Thesis, University of Leeds, 1997. http://etheses.whiterose.ac.uk/491/.
Sivertun, Åke. "Geographical Information Systems (GIS) as a tool for analysis and communications of multidimensional data." Doctoral thesis, Umeå universitet, Institutionen för geografi och ekonomisk historia, 1993. http://urn.kb.se/resolve?urn=urn:nbn:se:umu:diva-100703.
Diss. (sammanfattning) Umeå : Umeå universitet, 1993, härtill 6 uppsatser.
Ayhan, Saglam. "Developing A Geographical Information System For The Gallipoli Campaign." Master's thesis, METU, 2005. http://etd.lib.metu.edu.tr/upload/12606263/index.pdf.
McGrory, Sean. "The influence social problems have with violent crime and its impact on society: An investigation on the five boroughs of New York." Thesis, Umeå universitet, Kulturgeografi, 2018. http://urn.kb.se/resolve?urn=urn:nbn:se:umu:diva-150006.
Stott, Debbie. "A framework for evaluating instructional design models resulting in a model for designing and developing computer based learning tools with GIS technologies." Thesis, Rhodes University, 2005. http://hdl.handle.net/10962/d1003430.
Fleischmann, Elfrieda Marie-Louise. "The viability of an Interactive Geographic Information System Tutor (I-GIS-T) application within the FET phase / Elfrieda Marie-Louise Fleischmann." Thesis, North-West University, 2012. http://hdl.handle.net/10394/8738.
Yun, David In. "Utilization of Geographic Information System for Research, Management, and Education in the Natural Resources Management Department at California Polytechnic State University, San Luis Obispo." DigitalCommons@CalPoly, 2011. https://digitalcommons.calpoly.edu/theses/449.
Cates, Sarah Elizabeth. "An Analysis of National HIV/AIDS Education Efforts Among 15-39 Year Olds and Health Care Workers Applying the Health Belief Model (HBM) in Six Cities in Sonsonate, El Salvador." Diss., Virginia Tech, 2009. http://hdl.handle.net/10919/26201.
Geise, Gregory. "Application of Geographical Information Systems to Determine Human Population Impact on Water Resources of Yellow Springs, Ohio, and the Use of LiDAR Intensities in Land Use Classification." Wright State University / OhioLINK, 2016. http://rave.ohiolink.edu/etdc/view?acc_num=wright1464399610.
Wawro, Patrick Richard. "Gendered Distances: A Methodological Inquiry into Spatial Analysis as an Instrument for Assessing Gender Equality in Access to Secondary Schools in Mukono District, Uganda." BYU ScholarsArchive, 2010. https://scholarsarchive.byu.edu/etd/2320.
Gillman, Malin. "Eco-route modelling using GIS : How to find the most sustainable route option." Thesis, Umeå universitet, Institutionen för geografi, 2021. http://urn.kb.se/resolve?urn=urn:nbn:se:umu:diva-185860.
Snider, Scott. "Predicting Infestations of Hemlock Woolly Adelgid ( Adelges tsugae ) in Great Smoky Mountains National Park, Tennessee/North Carolina, USA." Ohio University / OhioLINK, 2004. http://rave.ohiolink.edu/etdc/view?acc_num=ohiou1103233070.
Tyrie, Elizabeth Katharyn. "Combining Quantitative Eye-Tracking and GIS Techniques with Qualitative Research Methods to Evaluate the Effectiveness of 2D and Static, 3D Karst Visualizations: Seeing Through the Complexities of Karst Environments." TopSCHOLAR®, 2014. http://digitalcommons.wku.edu/theses/1351.
Steer, Lorn Adam. "Site selection for the Small-Scale Aquaculture Farming Systems in the Western Cape : a GIS application." Thesis, Link to the online version, 2006. http://hdl.handle.net/10019/1653.
McCullough, Christina M. "Building a Replicable Flood Forecast Mitigation Support System to Simplify Emergency Decision-Making." University of Toledo / OhioLINK, 2011. http://rave.ohiolink.edu/etdc/view?acc_num=toledo1301756439.
Ozcan, Yavuzoglu Seyma. "An Evaluation Of Clustering And Districting Models For Household Socio-economic Indicators In Address-based Population Register System." Master's thesis, METU, 2009. http://etd.lib.metu.edu.tr/upload/12611471/index.pdf.
Tengbeh, Sahr. "Crime analysis and police station location in Swaziland : a case study in Manzini." Thesis, Stellenbosch : University of Stellenbosch, 2006. http://hdl.handle.net/10019.1/1013.
Colaninno, Nicola. "Semi-automatic land cover classification and urban modelling based on morphological features : remote sensing, geographical information systems, and urban morphology : defining models of land occupation along the Mediterranean side of Spain." Doctoral thesis, Universitat Politècnica de Catalunya, 2016. http://hdl.handle.net/10803/396219.
Davis, Ashley Michelle. "Integrating GIS In A Statewide Medical Education Administrative System." Thesis, 2019. http://hdl.handle.net/1805/19508.
White, Shannon Hill. "Geographic Information Systems (GIS) and Instructional Technology (IT) diffusion K-12 student and educator conceptualizations /." 2005. http://www.lib.ncsu.edu/theses/available/etd-09092005-050901/unrestricted/etd.pdf.
Skelton, Craig. "The Implementation and Education of Geographic Information Systems in a Local Government for Municipal Planning: A Case Study of Dangriga, Belize." 2014. http://scholarworks.gsu.edu/geosciences_theses/77.
George, David Paul. "Environmental Health and Safety data integration using Geographical Information Systems." Thesis, 2008. http://hdl.handle.net/10012/3654.
Marais, Hester J. W. "The status quo of GIS in municipal town planning departments in Gauteng and in the education of town planners." Thesis, 2008. http://hdl.handle.net/10210/858.
Ball, Kendall C. "A CASE STUDY ON USING GIS AS A TOOL TO ENABLE LEARNING OF SPATIAL PROBLEM SOLVING ABILITIES IN AN ONLINE ENVIRONMENT." 2009. http://hdl.handle.net/1969.1/ETD-TAMU-2009-05-265.
Καλησπέρη, Αγγελική. "Συμβολή των Συστημάτων Γεωγραφικών Πληροφοριών (GIS) και της περιβαλλοντικής εκπαίδευσης στην διαχείριση φυσικών περιοχών." Thesis, 2013. http://hdl.handle.net/10889/7799.
Chuang, Chia-Ling, and 莊佳綾. "A Study of the Disabled Children Education Placed and the Distribution of Special Education Teachers — by use of Geographic Information System (GIS) to analyze the primary schools of Nantou County." Thesis, 2008. http://ndltd.ncl.edu.tw/handle/97558210617466499712.
Copithorne, Dana. "A GIS approach for improving transportation and mobility in Iqaluit, Nunavut Territory." Thesis, 2011. http://hdl.handle.net/1828/3770.
NERAD, Jiří. "Návrhy využití geografických informačních systémů v hodinách zeměpisu na základních a středních školách." Master's thesis, 2018. http://www.nusl.cz/ntk/nusl-371745.
Mundia, Lisho Christoh. "Participatory mapping and the use of GIS for sustainable land use planning in the Hardap Region, Namibia." Thesis, 2015. http://hdl.handle.net/10500/19223.
- Open access
- Published: 15 June 2020
GIS-based landslide susceptibility mapping and assessment using bivariate statistical methods in Simada area, northwestern Ethiopia
- Tilahun Mersha 1 , 2 &
- Matebie Meten 1
Geoenvironmental Disasters volume 7 , Article number: 20 ( 2020 ) Cite this article
33k Accesses
97 Citations
1 Altmetric
Metrics details
Simada area is found in the South Gondar Zone of Amhara National Regional State and it is 780Km far from Addis Ababa. Physiographically, it is part of the northwestern highlands of Ethiopia. This area is part of the Guna Mountain which is characterized by weathered volcanic rocks, rugged morphology with deeply incised gorges, heavy rainfall and active surface processes. Many landslides have occurred on August 2018 after a period of heavy rainfall and they caused many damages to the local people. In this study, Frequency Ratio (FR) and Weights of Evidence (WoE) models were applied to evaluate the landslide causative factors and generate landslide susceptibility maps (LSMs). The landslide inventory map that consists of 576 active and passive landslide scarps was prepared from intensive fieldwork and Google Earth image interpretation. These landslide locations were randomly divided into 80% training and 20% validation datasets. Seven landslide causal factors including aspect, slope, curvature, lithology, land use, rainfall and distance to stream were combined with a training dataset using GIS tools to generate the LSMs of the study area. Then the area was divided into five landslide susceptibility zones of very low, low, moderate, high and very high. Later, the resulting maps have been validated by using area under the curve and landslide density index methods. The result showed that the predictive rate of FR and WoE models were 88.2% and 84.8%, respectively. This indicated that the LSM produced by FR model showed a better performance than that of WoE model. Finally, the LSMs produced by FR and WoE models can be used by decision-makers for land use planning and landslide mitigation purpose.
Introduction
Landslides are one of the recurrent natural problems that are widespread throughout the world, especially in mountainous areas which caused a significant injury and loss of human life, damage in properties and infrastructures (Parise and Jibson 2000 ; Dai et al. 2002 ; Glade et al. 2005 ; Kanungo et al. 2006 ; Pan et al. 2008 ; Girma et al. 2015 ). The term “landslide” is the movement of a mass of rock, debris or earth down a slope under the influence of gravity (Varnes 1978 ; Hutchinson 1989 ; WP/WLI - International Geotechnical Societies’ UNESCO Working Party on World Landslide Inventory 1990 ; Cruden 1991 ; Cruden and Varnes 1996 ). Landslides are caused by different triggering factors such as heavy or prolonged precipitation, earthquakes, rapid snow melting and a variety of anthropogenic activities. Landslides can involve flowing, sliding, toppling or falling movements and many landslides exhibit a combination of two or more types of movements (Crozier 1986 ; Cruden and Varnes 1996 ; Dikau et al. 1996 ).
Landslide in Ethiopia is a common phenomenon which often causes significant damage to people and property. Almost 60% of the total population in Ethiopia lives in the highland areas (Ayalew 1999 ) which is characterized by high relief, complex geology, high rainfall, rugged morphology, very deep valleys and gorges with active river incision. The rapid population growth demanded the use of areas which were not previously used for settlement, urban expansion, agricultural and other purposes thereby exposing these areas to landslide problems after rainy seasons (Temesgen et al. 2001 ; Abebe et al. 2010 ; Woldearegay 2013 ).
In recent years landslide incidences are increasing in the Ethiopian highlands due to man-made and natural causes (Meten et al. 2015b ). For instance, from 1960 to 2010 alone, Landslides have killed 388 people, injured 24 people, and damaged agricultural lands, houses and infrastructures (Ayalew 1999 ; Temesgen et al. 1999 ; Woldearegay 2008 and (Ibrahim: Landslide assessment and hazard zonation in Mersa and Wurgessa, North Wollo, Ethiopia, unpublished)). According to Abebe et al. ( 2010 ), the highlands and mountainous area of Ethiopia like the Blue Nile Gorge, the Lower Wabe-Shebele River valley, Gilgel Gibe River, Tarmaber, Kombolcha - Dessie road, Uba Dema village in Sawla, Wondogenet area and many other parts of Ethiopia are repeatedly facing problems associated with landslides. The landslides in these areas are affecting human lives, infrastructures, agricultural lands and the natural environment. As a result of this, the study of the landslide has drawn global attention to increase awareness about its socioeconomic impacts and the pressure of increasing population and urbanization on mountainous areas (Kanungo et al. 2006 ).
The current study area is found in Simada District of South Gondar Zone in the Amhara National Regional State of Northwestern Ethiopia. It is part of the northwestern Ethiopian highlands. This area is severely affected by landslide incidences in recent years. Landslide incidence in the study area occurred on August, 2018 after a heavy and prolonged rainfall that caused the death of animals, destruction of houses and wide areas of cultivated and non-cultivated lands. Therefore, this area requires a detailed investigation to evaluate the causes, types and failure mechanisms of landslides and to prepare the landslide susceptibility maps. A systematic landslide study helps to reduce the damages in infrastructures, houses and cultivated lands and loss of lives. This importance will be noticed when these landslide susceptibility maps are used by decision-makers in regional land use planning, landslide prevention and mitigation measures.
For proper and strategic land use planning, it is important to evaluate and delineate landslide prone areas using different landslide susceptibility mapping techniques. Preparing a landslide susceptibility map of a certain area is a useful tool in landslide hazard management as it shows the degree of susceptibility of an area to landslide occurrence. It is obvious that landslide susceptibility maps can be generated based on the assumption that future landslide will occur under the same condition as in the past (Pham et al. 2015 ). Interpretation of future landslide occurrence needs an understanding of conditions and processes that control landslides in the study area. Past landslides and different conditioning factors such as slope morphology, hydrogeology and geology of the area are the main parameters to assess and evaluate landslide susceptibility by integrating these conditioning factors and past landslides in a GIS environment.
GIS-based landslide susceptibility mapping techniques have been used by several researchers (Aleotti and Chowdhury 1999 ; Kanungo et al. 2009 ) which can be classified into qualitative and quantitative ones (Yalcin et al. 2011 ; Felicisimo et al. 2012 ; Peng et al. 2014 ; Wang and Li 2017 ). Qualitative techniques include geomorphological analyses and inventory methods. These are based on expert judgment and are more subjective than quantitative methods. Quantitative methods such as deterministic analyses, probabilistic approaches and statistical techniques closely rely on mathematical models which have much less personal bias but still needs experience to produce and run these models (Aleotti and Chowdhury 1999 ; Kanungo et al. 2009 ). In recent years, many landslide susceptibility maps were produced using GIS-based statistical approaches like Frequency Ratio (FR) and Weights of Evidence (WoE) models. This is because the result from these models showed good performance with high accuracy and these models are very simple to implement and can provide the contribution of each causative factor class for landslide occurrence (Lee and Pradhan 2007 ; Akgun et al. 2007 ; Dahal et al. 2008 ; Işık Yilmaz 2009 ; Pradhan, Lee and Buchroithner 2010 ; Choi et al. 2012 ; Park et al. 2012 ; Vakhshoori and Zare 2016 ; Fayez et al. 2018 ).
Several researchers have used Frequency ratio model on landslide studies (Bahrain et al. 2014 ; Meten et al. 2015a ; Haoyuan Hong et al. 2015 ; Pham et al. 2015 ; Pirasteh and Li 2017 ; Fayez et al. 2018 ; Khan et al. 2019 ) and in comparison with a few methods (Akgun et al. 2007 ; Lee and Pradhan 2007 ; Işık Yilmaz 2009 ; Choi et al. 2012 ; Park et al. 2012 ; Meten et al. 2015b ; Wang and Li 2017 ). A combination of both FR and WoE models have been applied for landslide susceptibility mapping (Regmi et al. 2013 ; Rahmati et al. 2016 ). Gholami et al. ( 2019 ) also compared the prediction capability of frequency ratio, fuzzy gamma and landslide index models. Each GIS-based statistical method requires data on past landslides, preparatory causative factors and triggering factors. To prevent or mitigate any damage from landslides, it is essential to assess the landslide prone areas. The current study aims to carryout landslide susceptibility mapping by applying FR and WoE models in order to highlight critically high and very high hazard zones. This will help to reduce and mitigate any hazard associated with future landslide occurrence.
The study area is 185.7 square kilometers which is located in Simada District of South Gondar Zone, Amhara National Regional State, Ethiopia (Fig. 1 ). The area is bounded between 38 0 11' E and 38 0 20' E longitudes and 11 0 30' N and 11 0 41' N latitudes. The typical drainage pattern of the study area is dendritic and parallel. Atkus and Kostet Rivers are the main rivers that affect the study area by eroding the banks of rivers leading to slope instability. The confluence of these rivers forms Bijena River which is the largest river in the study area. Most of the rivers in the study area flow towards the southeast direction. The physiography of the study area forms the rugged topography of Guna Mountain (Fig. 2 ) which is part of the northwestern Ethiopian highlands. The area can be classified into two main physiographic regions. These are the plateau area and the rugged terrain. The plateau areas are characterized by volcanic landscapes that represent the high flatlands of the Kefoye, Agona and Jinjero Gedel areas. These areas are water divide zones in which rivers are flowing to Abay Basin in the west and to Bashilo Basin in the south. In this area, the slopes are ranging from flat slopes on the top to steeper slopes at the plateau scarp. The rugged terrain is highly dissected by major rivers and streams which are characterized by deep narrow valleys and gorges. Slopes in these areas are steep to vertical and susceptible to erosional and landslide phenomena. The elevation of the study area ranges from 2067m to 3586 m which comprises of medium to very high relief hills. The presence of steep scarps, rugged slope faces, deep gorges and steep ridges showed that this area is prone to active surface processes and landslide incidences. Based on elevation, the climatic zones of the study area are mostly falling under the highland climatic zone. The primary wet season extends from June to September. There is great variation in the rainfall amounts with maximum rainfall occurring during the wet season which starts in June and ends in September with the heaviest rainfall occurring during the months of July and August.
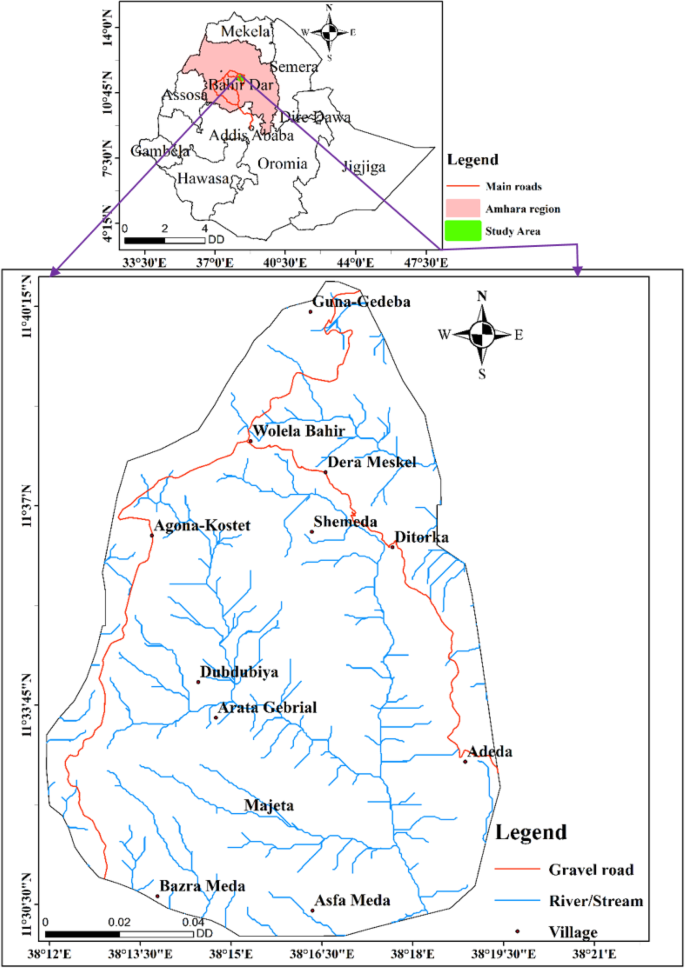
Location map of the study area
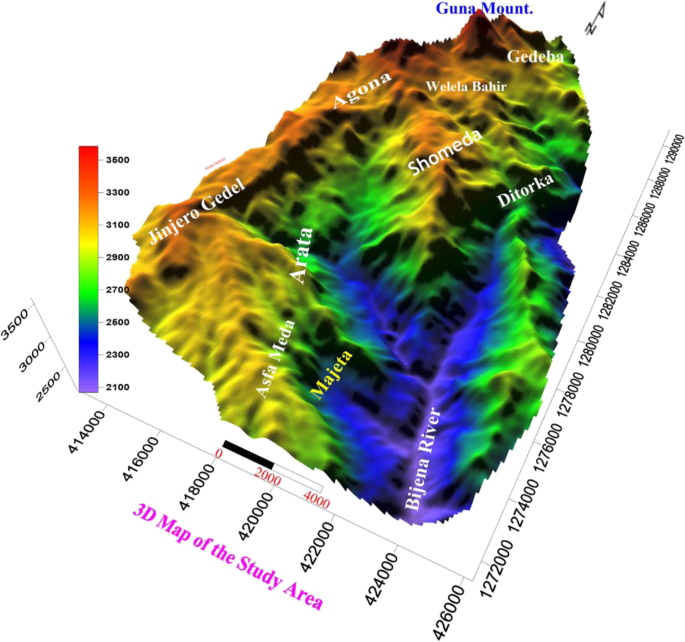
Three dimensional map of the study area
In order to achieve the objectives of this research, data collection and organization, preparation of landslide inventory datasets, database construction of landslide causative factors and application of FR and WoE models were carried out to prepare the landslide susceptibility maps and validate them.
Data collection and organization
The necessary data for this study were collected from various sources. These include collecting relevant literatures from published and unpublished papers, DEM data from USGS, a regional geological map from Geological Survey of Ethiopia at a scale of 1:250000, rainfall data from National Metrological Agency of Ethiopia, a topographic map from Ethiopian Geospatial Information Agency at a scale of 1:50000 and Google Earth image from Google Earth. During field work, data collection was carried out on different rock types by describing their character, the relative degree of weathering, slope steepness, location of springs and swamps, landslide inventory mapping on both active landslide and scarp areas by measuring their length, width, accumulation zone and depth (if possible), land use and land cover, man-made activities including farming practice. After compilation of the actual field investigation, the data has been systematically processed and analyzed first in ArcGIS followed by Microsoft Excel and then finally in ArcGIS.
Preparation of landslide inventory dataset
The quality of the landslide inventories depends on the accuracy, type and certainty of the information shown in the maps. New and emerging mapping methods, based chiefly on satellite, aerial and terrestrial remote sensing technologies, can greatly facilitate the production and the update of landslide maps. Literature review has shown that the most promising approaches exploit VHR optical, monoscopic and stereoscopic satellite images, analyzed visually or through semi-automatic procedures, and VHR digital representations of surface topography captured by LiDAR sensors. A combination of satellite, aerial and terrestrial remote sensing data represents the optimal solution for landslide detection and mapping, in different physiographic, climatic and land cover conditions (Guzzetti et al. 2012 ). Ye et al. ( 2019 ) detected landslides from hyperspectral remote sensing data using a deep learning technique.
The landslide inventory dataset in the current study consist a total of 576 landslides which were identified from Google Earth image interpretation and intensive field survey. For landslide susceptibility mapping landslide polygons can be divided into training and validation datasets. The training dataset is used for constructing the predictive model while the validation dataset is used for validating the model. In this study, the specific date of landslide occurrence is not well known. Hence, the landslide polygons were randomly split into two classes with 80% for training and 20% for validation by keeping their spatial distribution into account (Fig. 3 and 4 ). In addition, the validation data sets for most of the landslide susceptibility or hazard assessments were chosen in between 20% and 30% of the total landslide inventory.
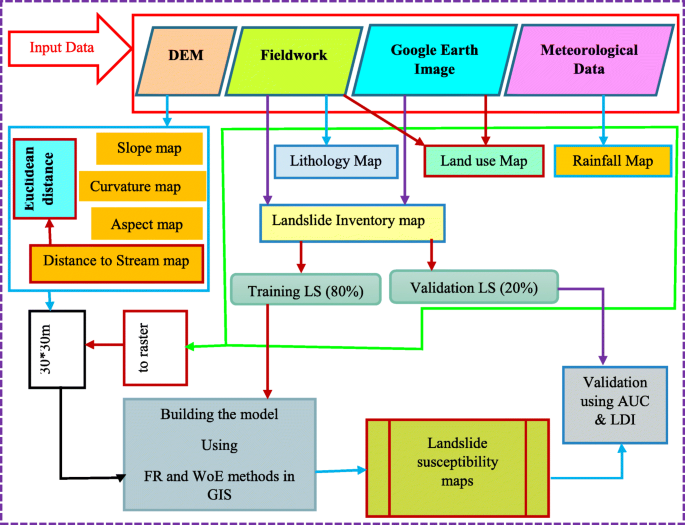
Flowchart of the research work (Note: LS = landslide)
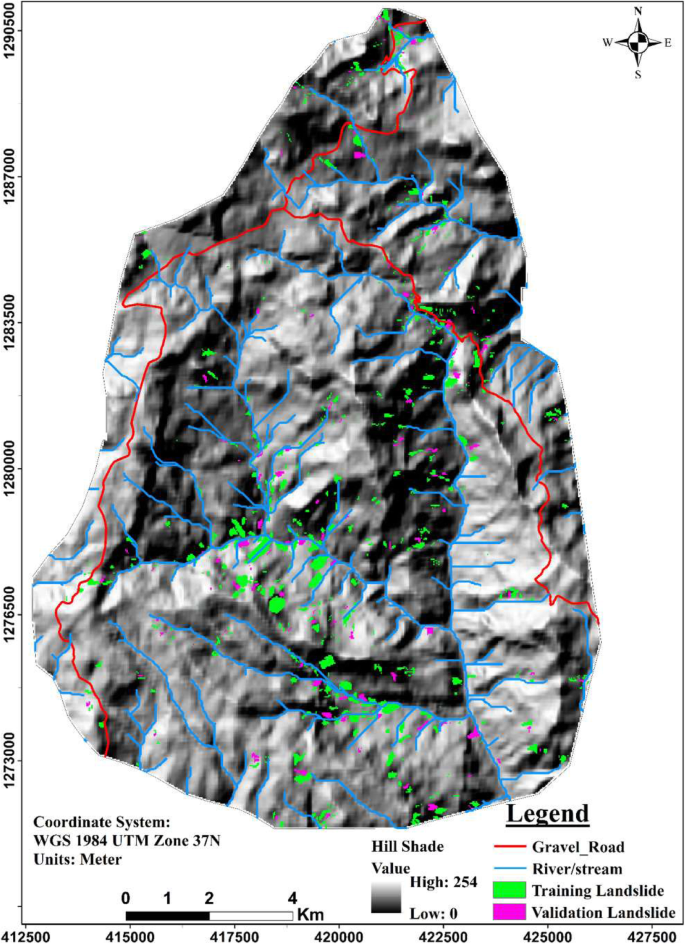
Landslide inventory map showing the distribution of landslides
Database for landslide causative factors
To undertake landslide susceptibility analysis in the study area, a spatial database was first constructed for the causative factors within the spatial analysis tools of ArcGIS 10.4 software. The database consists of the landslide inventory datasets (training and validation) and the landslide causative factors (slope, aspect, curvature; land use, lithology, rainfall and distance from stream). These factors were subsequently evaluated by calculating their weights from the relationship between the landslide and landslide causative factors and then these results were verified. There are no strict rules or guidelines for the triggering factors to be used in different statistical approaches for landslide susceptibility mapping. Instead, the chosen factors should be operative and measurable depending on a particular area’s characteristics (Ayalew and Yamagishi 2005 ). One parameter may be an important controlling factor for landslide occurrence in a certain area but in most cases a combination of two or more landslide causative factors may be effective in addition to the triggering factor for landslide occurrence.
In this study, the triggering factor was heavy and prolonged rainfall. During the fieldwork, landslide locations were identified and marked with GPS, land use (land cover) types around the landslide scar, drainage networks and spring locations, lithological units and human activities were investigated to prepare the landslide susceptibility maps.
Generally, the selection of landslide causative factors should consider the nature of the study area and the availability of data. In this regard, a total of seven parameters were selected including slope, aspect, curvature, lithology, rainfall, land use and distance to stream. All causative factor maps were converted into raster maps with the same coordinate system (WGS 1984 UTM zone 37N) and the same pixel size (30mx30m). The rasterized training (80%) landslide map and all the causative factor maps have been overlaid and the information was extracted using the spatial analyst tool of ArcGIS to calculate the ratings or weights of all factor classes for FR and WoE models. The summation of these ratings or weights of each landslide factor will help to evaluate the spatial relationship between them and the probability of landslide occurrence in the study area.
Topographic parameters like slope, aspect, curvature and distance to stream maps were derived from Digital Elevation Model (DEM) with a cell size of 30 m by 30 m. Lithology and land use maps were prepared from intensive fieldwork and Google Earth image interpretations. The rainfall map was generated using IDW interpolation technique of the spatial analyst tool in ArcGIS from four rain gage stations near to the study area using the rainfall data from National Meteorological Agency of Ethiopia.
Frequency ratio (FR) model
Frequency Ratio model is a well-known and widely used bivariate statistical method that is used for landslide susceptibility mapping (Lee and Talib 2005 ; Akgun 2012 ; Demir et al. 2013 ; Mezughi et al. 2011 ; Yalcin et al. 2011 ; Abay and Barbieri 2012 ; Mondal and Maiti 2013 ; Paulin et al. 2014 ). In this model, processing the input data, computations and output-processes are very simple and can be easily understood. It is simple and relatively flexible to use and implement a landslide susceptibility map with accurate results (Lee and Pradhan 2007 ; Yilmaz 2009 ; Choi et al. 2012 ; Mohammady et al. 2012 ; Park et al. 2012 ). The frequency ratio model is one of the probabilistic models which are based on the observed relationship between the distribution of landslides and each landslide related factor (Lee and Talib 2005 ). To evaluate the contribution of each factor towards landslide susceptibility, the training landslide group was combined with thematic data layers separately and then the frequency ratio of each factor’s class was calculated according to the following procedures.
First, the number of pixels for landslide occurrence and non-occurrence in each factor’s class was calculated. Second, the percentage of each factor’s class having landslide to the total pixels containing landslide of the factor was calculated and the percentage of each factor class’s number of pixels to the total number of pixels in the study area was calculated. Finally, the frequency ratio of each factor class was obtained by dividing the percentage of landslide pixels to the percentage of area pixels in each factor classes (Equation 1 ).
Where; Npix ( S i , j ) = the number of pixels containing landslide within class j in factor i; Npix ( N i , j ) = the number of pixels of class j in factor i; ∑ j NPix ( S i , j ) is the number of total pixels containing landslide in the study area; ∑ j NPix ( N i , j ) is the number of total pixels in the study area.
The calculated FR value represents the degree of correlation between landslide and a certain class of the causative factor. A value of 1 is an average value for the landslide occurrence of a specific landslide causative factor class. A value more than 1 indicates a strong and positive correlation and a high probability of landslide occurrence, while a value of less than 1 indicates a negative relationship and low probability of landslide occurrence in a certain class of a landslide causative factor. The FR map of each causative factor is prepared with the help of ArcGIS by assigning the calculated FR values. Then the FR values of all the causative factor maps were overlaid and numerically added using the raster calculator of the spatial analyst tool in ArcGIS 10.4 to prepare the Landslide Susceptibility Index (LSI) map. LSI is computed by summing the FR values of all the landslide causative factor maps (Equation 2 ) and then the resulting LSI map was further reclassified in to very low, low, moderate, high and very high landslide susceptibility classes.
Where: LSI = Landslide susceptibility index, FR is the frequency ratio and n is the number of selected causative factors. The calculated values of FR for each pixel in the LSI indicate the relative susceptibility to landslide occurrence. The higher LSI pixel values have high susceptibility to landslide occurrence while the lower LSI pixel values have lower susceptibility (Akgun et al. 2007 ).
Weights of evidence (WoE) model
WoE model is a log-linear form of the Bayesian probability model for landslide susceptibility assessment that uses landslide occurrence as a training point to drive prediction outputs. It calculates both unconditional and conditional probability of landslide hazards. This method is based on the calculation of positive and negative weights to define the degree of spatial association between landslide occurrence and each explanatory variable class (Pardeshi et al. 2013 ). The positive weights (W+) indicate the occurrence of an event while the negative weight (W-) indicates the non-occurrence of an event. To evaluate W + and W - , calculating the following parameters is important.
Nmap = total number of pixels in the map
Nslide = number of pixels with landslides in the class
Nclass = number of pixels in the class
NSLclass = number of pixels with landslides in the class
The values needed for the weight of evidence formula are:
Npix1 = NSLclass
Npix2 = Nslide – NSLclass
Npix3 = Nclass – NSLclass
Npix4 = Nmap – Nslide – Nclass + NSLclass
Then the positive and negative weights are calculated as follows (Equations 3 and 4 ).
Where Npix 1 is the number of landslide pixels present on a given factor class, Npix 2 is the number of landslides pixels not present in a given factor class, Npix 3 is the number of pixels in a given factor class in which no landslide pixels are present and Npix 4 is the number of pixels in which neither landslide nor the given factor is present (Van Westen 2002 ; Dahal et al. 2008 ; Regmi et al. 2010 ). These weights are used to calculate a weight of contrast value (C) for the particular susceptibility variable (Equation 5 ).
The contrast value (C) measures the strength of a relationship between the causative factors and landslides. If the contrast value is positive, it will have a positive spatial association while the negative one will have a negative spatial association. The weighted map ( Wmap ) for each landslide causative factor can be prepared by summing the weights of contrast(C) values of each factor class. Similarly, the final landslide susceptibility index (LSI) map was prepared by summing all the weighted maps (∑ Wmap ) of each landslide causative factor through a raster calculator of map algebra in the spatial analyst tool of ArcGIS as follows (Equations 6 and 7 ).
Landslide inventory
During August, 2018, an intense rainfall in Simada area triggered many landslides that occurred mostly in rural areas. The damage was severe in the villages of Dubdubiya, Asfa Meda, Gedeba, Ditorka and at several other sites along the river courses. Particularly, in Dubdubiya and Asfa Meda villages, landslides damaged 81 dwellings, killed 14 goats, affected thousands of people, damaged hundreds of hectares of farmlands and dislocated 486 people. These problems occurred in these villages as the settlement areas are mostly located at the foot of a steep slope that is covered by weathered volcanic rocks as well as the presence of stream accumulated debris and earth flows that can suddenly burst out at the at the outlets of a mountain. Landslide inventory map of the study area (Fig. 4 ) was prepared from the combination of an intensive field survey and Google Earth image interpretations. Extensive field studies conducted from mid-November to mid-December of 2018 helped us to map known landslides using GPS and check the size and shape of these landslides in order to identify the type of movements, materials involved and to determine the state and activity of landslides (active, reactivated, dormant, etc.). This inventory data was mapped as vector-based polygon data and then converted to the raster format with a pixel size of 30m by 30m in ArcGIS 10.4.
In the present study area, a total of 576 landslides that contain 6304 pixels were identified and divided randomly into training and validation landslides by keeping their spatial distributions into account. The training landslides that accounted 80% of landslides with 5126 pixels were used for building the predictive model while the validation landslides that accounted 20% of landslides with 1178 pixels were used for validating purpose. From the total landslide polygons, 117 landslides were active landslides collected from field investigations while the remaining 459 landslide polygons were collected from time serious Google Earth image interpretations.
Landslide locations are predominantly distributed in the south-central, in the north and in the eastern parts of the study area with decreasing order of landslide density, damage on agricultural land and infrastructures. This area consists of a rugged and mountainous terrain which is characterized by steep slopes, deep gorges, high relief and fractured and weathered rocks. The common types of landslide occurrence in the study area include rock slide, rockfall, earth slide, debris slide and debris flow, rotational and translational soil slide, translational debris slide, rotational debris slide and complex types of slides. Generally, these landslides predominantly affected the rural areas in which the type of landslides and their probable causes and damages are described below.
Most prominent landslides occurred in Asfa Meda, Dubdubiya, Tej Wuha-Gedeba and Ditorka-Megersum Villages. Landslides in Asfa Meda Village occurred at the interface between thin residual soils and rhyolitic rock and most of the landslides are shallow rotational and/or translational earth slides. Most of Dubdubiya village was highly affected by stream undercutting, erosion of the slope surface, riverbank erosion and improper farming practice (Fig. 5 ). The slope materials are dominantly covered by weathered basalt and colluvial deposits. Erosional opening surfaces and tension cracks were observed during field investigation indicating that seeping water might have brought instability of the slope through internal erosion of the weathered materials. A typical example of a landslide in this village was the landslide that occurred near Arata Gabriel Church. The main causes of this landslide were stream/river undercutting, presence of spring on top of the slope and colluvial soil slope materials. The slope material in Teji Wuha and Gedeba Villages is dominantly covered with weathered tuff and thin residual soils. In this village, there is an indication of shallow groundwater since the swamp area and many springs are observed with rotational and soil creep. Creeping of soil was identified by tilting of powerlines and fences (Fig. 6 d). The common types of landslides that were observed in Ditorka and Megersum villages were rockslide (Fig. 6 a), rock fall, debris slide (Fig. 6 b) and rotational slide.
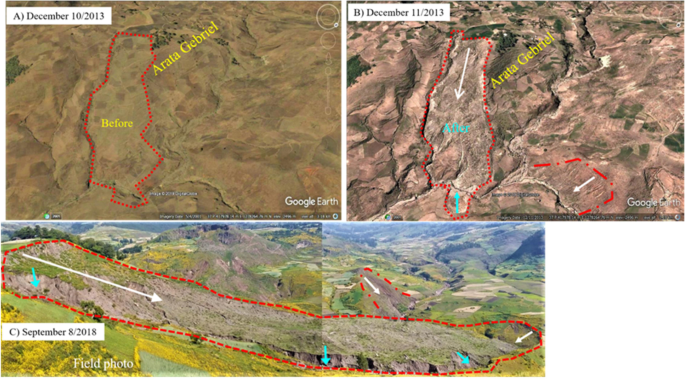
Historical landslide data in Dubdubiya Village as detected from Google Earth image. The white arrow and the red broken line in the figures indicate the direction of the slope movement and a landslide boundary respectively. a Image before the landslide event. b Image after landslide occurrence and, c Photo of the current condition
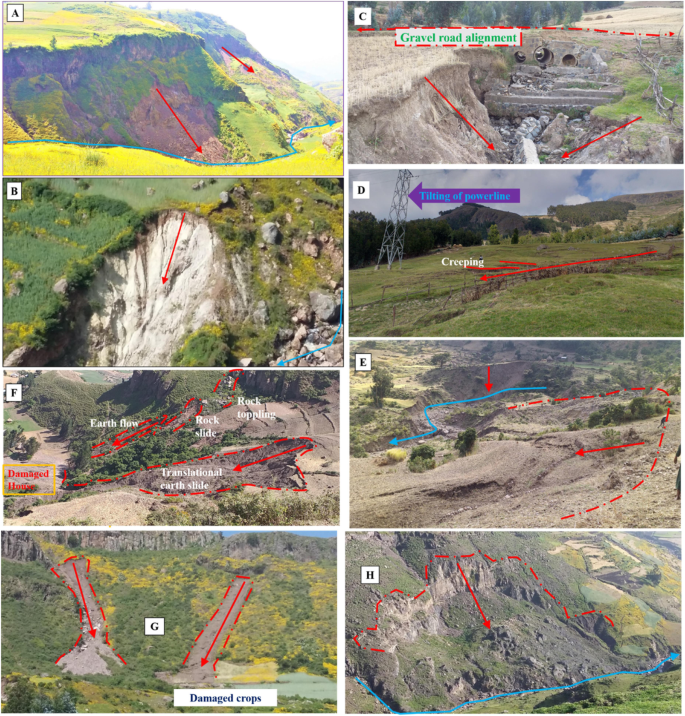
Field photo of landslide and their damage in different villages of the study area (Red and blue colored arrow indicates the direction of slope movements and River/stream flows respectively). In Ditorka and Megersum Villages- ( a ) Rockslide ( b ) Debris slide; Teji Wuha and Gedeba Villages- ( c ) Earth slide on the gravel road (damaged culvert) ( d ) Creep (tilting of powerline); in Asfa Meda Village- ( e ) and ( f ) Complex type landslides; in Dubdubiya Village- ( g ) Shallow debris flow that damaged wheat crop and ( h ) Rockslide
Landslide causative factors
The spatial distribution and density of landslides are mainly controlled by topography of an area, weather condition, geology, land use/land cover and anthropogenic factors (Khan et al. 2019 ). Consequently, evaluating the impact of these causative factors on the spatial distribution of landslides is very important in order to understand their failure mechanism and to prepare the landslide susceptibility map. In this study, seven causative factors that have been used for the preparation of landslide susceptibility maps include slope, aspect, curvature, lithology, land use/ land cover, rainfall and distance to stream. The roles played by each of these causative factors will be discussed in the following sections.
Slope is a very important parameter for landslide study as it has a direct relation with landslide occurrence. As a result, it is frequently used in preparing a landslide susceptibility map (Yalcin and Bulut 2007 ). It is well known that landslide occurs more frequently on steeper slopes due to gravity stress. The slope map (Fig. 7 a) of the study area was prepared from DEM data. It was divided into five classes such of 0 – 5 0 , 5 0 – 12 0 , 12 0 – 30 0 , 30 0 – 45 0 , and > 45 0 . For slope classes above 12 0 , the frequency ratio is increasing which indicate the higher probability of landslide occurrence in these classes (Table 1 ).
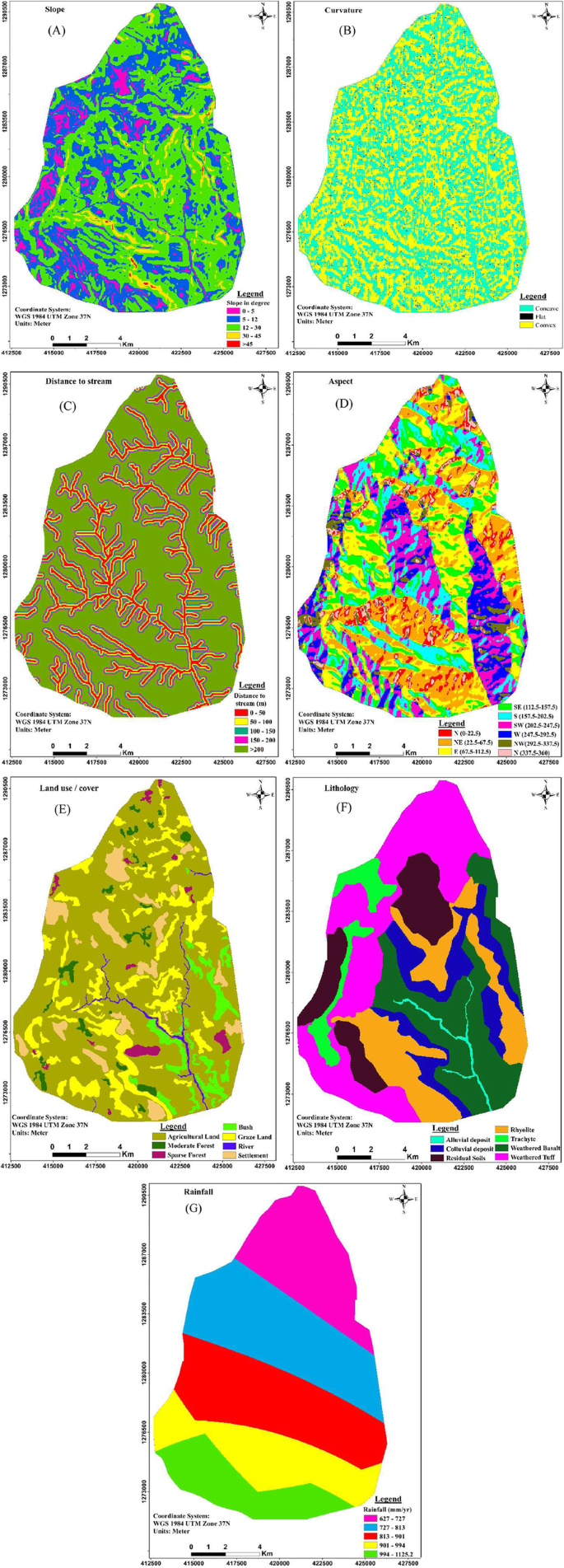
Landslide causative factor maps ( a ) Slope, ( b ) Curvature, ( c ) Distance to stream ( d ) Aspect ( e ) Land use, ( f ) Lithology and ( g ) Rainfall
Curvature map of the study area was generated from DEM data and it was classified into 3 classes of concave, convex and flat surfaces (Fig. 7 b). Following heavy rainfall, a convex or concave slope contains more water and retains this water for a longer period (Lee and Talib 2005 ). The more positive or negative values indicate the higher probability of landslide occurrence. In the flat area, the probability of landslide occurrence is very low. A positive curvature indicates that the surface was upwardly convex at that grid. A negative curvature indicates that the surface was upwardly concave at that grid and a value of zero indicates that the surface is flat.
Aspect refers to the slope orientation which is generally expressed in terms of degree from 0 0 – 360 0 . It is considered as an important factor in landslide studies as it controls slope’s exposure to sunlight, wind direction, rainfall (degree of saturation) and discontinuity conditions (Komac 2006 ). Slope aspect map (Figure 8 c) in this study area was derived from DEM data and it was divided into nine classes, namely; north (0 – 22.5, 337.5 - 360,), northeast, east, southeast, south, southwest, west and northwest (Fig. 7 d).
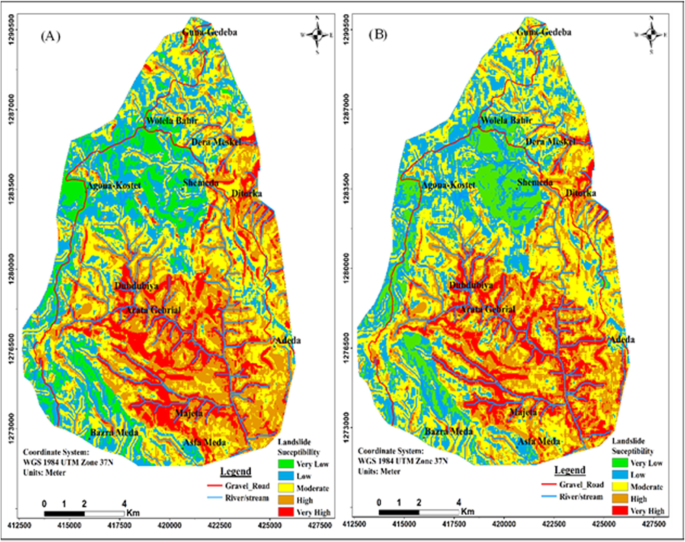
Landslide Susceptibility Map of the study area using: a FR and b WoE Models
Distance to stream
The proximity of the slope to the stream course is an important factor that dictates the landscape evolution of the area and an indicator of the landslide and related erosional aspects. Rivers with a number of drainage networks have a high probability of landslide occurrence as they erode the slope base and saturate the underwater section of the slope forming material (Akgun and Turk 2011 ).
Since there are many streams in the study area which flow into Kostet, Atkus and Bijena Rivers, many landslides occurred in the close vicinity of these rivers. Hence, this parameter was considered as one causal factor in landslide susceptibility analysis. Zones with parallel pattern of drainage in steep slopes are the most probable landside sites. Drainage often plays its own role in developing pore-water pressure which reduces the shear strength of slope materials. Streamlines were derived from DEM data and it was classified based on stream order.
Landslide in this area is mostly associated with 1 st , 2 nd , and 3 rd order streams. Distance from stream map was developed from Euclidean distance buffering method in the spatial analyst tool of ArcGIS 10.4. This map was classified in to five subclasses: 0 – 50, 50 – 100, 100 – 150, 150 – 200 and > 200 meter (Fig. 7 c).
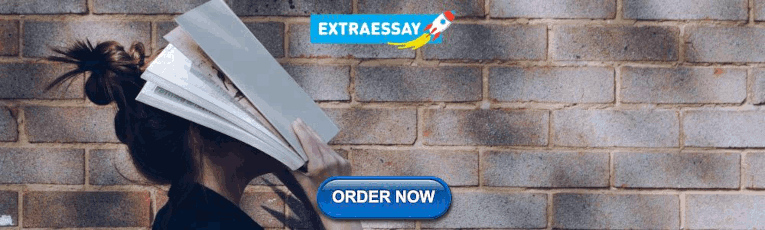
Land use / land cover
Land-use change has been recognized throughout the world as one of the most important factor influencing the occurrence of rainfall-triggered landslides. Changes in land use/cover resulted from man-made activities such as deforestation, overgrazing, intensive farming and cultivation on steep slope can initiate slope instability (Glade 2003 ). Vegetation has a major contribution to resist slope movements. Vegetation having a well-spread network of root systems increases shearing resistance of the slope material. This is due to the natural anchoring of slope materials. In addition to this, it reduces the action of erosion and adds the stability of the slope. In another way, barren or sparsely vegetated slopes are usually exposed to erosion and thus it has the effect of increasing slope instability. The land use map of the study area was prepared from the Google Earth image of 2016 and the analysis was done in ArcGIS. About seven land-use types were identified including moderate forest, sparse forest, bush, grazing land, agricultural land, settlement and river (Fig. 7 e). The area is predominantly covered by agricultural land and grazing land.
Lithology is one of the most controlling parameters in slope stability since each class of materials has different shear strength and permeability characteristics (Yalcin and Bulut 2007 ). Different rock types have varied composition and structure which contribute to the strength of the slope material in a positive or negative way. The stronger rock units give more resistance to the driving forces as compared to the softer/ weaker rocks. Lithological map of the study area was prepared from existing regional geological map (with a scale of 1:250,000) as a preliminary map for further improvement of a lithologic map into a scale of 1:50,000 based on a detailed field survey. The study area contains seven lithological units namely Trachyte, Weathered tuff, Rhyolite, Weathered basalt, Residual soils, Colluvial and Alluvial Deposits (Fig. 7 f).
Rainfall is considered as an influencing factor to cause slope instability. Precipitation, particularly intense and prolonged rains are controlling factors that trigger landslides by providing water thereby increasing underground hydrostatic level and pore water pressure. When the soil undergoes such pressure changes, water within it will create negative or upward pressure, as it cannot drain quickly. When the pore water pressure is equivalent to the upper pressure, the shearing resistance of the material decrease and will lead to failure of the material. The rainfall data of the four stations that surround the study area were collected from National Metrology Agency of Ethiopia. There are various interpolation techniques in ArcGIS to interpolate rainfall over a large area based on few point data. These include Thiessen polygon, Isohyetal, average arithmetic, inverse distance weight (IDW) and Kriging. The general assumption of the IDW method of interpolation is that the value of unsampled point is the weighted average of known values within the neighborhood. Therefore, the values from a scattered set of known points can be utilized to assign rainfall values to unknown points. It can be used to compute the unknown spatial rainfall data from the known sites that are adjacent to the unknown sites (Chen and Liu 2012 ). The rainfall map of the study area was prepared using the IDW interpolation method in GIS. The rainfall data analysis showed that the maximum monthly rainfall occurs in June, July, August and September which coincides with the landslide occurrence in this area. The rainfall map of the study area was divided into five annual rainfall classes of 627 – 727, 727 – 813, 813 – 901, 901 – 994 and 994 - 1125.2 millimeters (Fig. 7 g) by the natural breaks method.
Result and discussion
Relationship between landslide and causative factors.
This study has analyzed the relationship between seven causative factors and landslide occurrence. Using the FR and WoE models, the relative frequency values and the weights of values were calculated respectively. The causative factors were classified into different classes and weights were assigned to them for both FR and WoE models as presented in Table 1 and 2 respectively. These results showed that the relative susceptibility of each class is almost similar for both models but the parameters and results are different from each other. This implies that if a factor class has lower and higher values in both models, the susceptibility will also be lower and higher respectively. In case of FR model, the spatial relationship between the causative factors and landslide is determined by FR values. The causative factor classes with FR value > 1 will have a high degree of landslide occurrence. On the other hand, for the WoE model, C describes the correlation and spatial association of the landslide with the causative factors. The positive C values indicate a positive association with more landslide occurrence and vice versa for negative C values. The weights with higher values indicate a higher degree of influence on landslide occurrence. Generally, the factor class values derived from each model showed the spatial relationship of the causative factors in their contribution to landslide occurrence. The association is more or less the same in both models.
The slope classes > 12 0 have higher contribution for landslide occurrence. The area with a slope class > 45 0 is the most landslide prone class while the area with a slope class < 5 0 is the least one. Generally, as the slope increases, the probability of landslide occurrence also increases. In case of aspect classes, the FR values of slope classes facing towards the northeast (22.5 – 67.5), east (67.5 – 112.5) and north (0 – 22.5) are greater than one indicating a higher probability of landslide occurrence. The northeast facing aspect class has got the maximum weight or rating followed by the east facing ones. The curvature range of (-3.6) - (-0.001) has a greater contribution to the slope failures. In case of lithology, three units i.e. colluvial deposit, weathered basalt and rhyolite have high probability of landslide occurrence. Colluvial deposit and weathered basalts have less strength and hence susceptible to landslides. Rhyolitic rocks in the study area formed a cliff underlying thin residual soils. As a result, most of the landslides occurred at the contact between rhyolite and thin residual soils.
The type of land use also controls the occurrence of landslide in the study area. The highest weights or ratings were observed in the land use types of grazing land, river, sparse forest and bushes indicating a high probability of landslide occurrence. The highest weighted value of grazing land is due to its exposure to erosion and weathering. In case of the relationship between landslide occurrence and the distance from stream, as the distance from stream increases, the occurrence of landslide generally decreases. Landslide occurrence is higher in the first three classes of 0 – 50m, 50 – 100m and 100 – 150m (Table 1 and 2 ). With regard to the causative factor rainfall, two classes with 813 – 901mm and 901 - 994 mm have a higher C and FR values than the other classes and are the most susceptible classes (Table 1 and 2 ). Generally, slope classes > 20 0 , land use classes of grazing land, sparse forest, river and bush; lithology of colluvial deposit, weathered basalt, alluvial deposit and rhyolite and distance to stream classes of < 150 m buffers are the most contributing factor classes among the seven landslide factor classes.
Landslide susceptibility mapping using FR and WoE models
Frequency ratio model.
Map of each causative factor is prepared with the help of ArcGIS and then the frequency ratio values were calculated. The calculated FR values for each pixel in the LSI indicate the relative susceptibility to landslide occurrence. The higher pixel values of LSI have the higher landslide susceptibility while the lower pixel values will have lower susceptibility (Akgun et al. 2007 ). The landslide susceptibility index was calculated based on the frequency ratio values that have been determined in the training process that can be added in a raster calculator of ArcGIS as follows (Equation 8 ).
Where FR sl = frequency ratio value of slope, Fr as = frequency ratio value of aspect, = FR cu = frequency ratio value of curvature, FR li = frequency ratio value of lithology, FRlu = frequency ratio value of land use, FR rf = frequency ratio value of rainfall, FR ds = frequency ratio value of distance to stream.
The LSI values for the frequency ratio model in the study area range from 2.89 to 15.09. The LSI map is reclassified to prepare the landslide susceptibility map of the study area (Fig. 8 a). There are different types of classification methods such as natural break, equal interval, manual, standard deviation and quantile. In the current study, reliable results were obtained from natural breaks method. The result of other classification methods revealed the susceptibility classes with a high degree of exaggeration where large part of the study area fall into the high susceptibility class.
Therefore, the LSI values were classified into five susceptibility classes of very low (2.89 - 5.31), low (5.31 - 6.24), moderate (6.24 - 7.23), high (7.23 - 8.39) and very high (8.39 - 15.09) using the natural breaks method of classification. The result from Table 3 showed that 8.616% (16 km 2 ), 20.474%(38km 2 ), 29.537%(54.9km 2 ), 27.898% (51.8 km 2 ) and 13.474% (25 km 2 ) areas fall into the very low, low, moderate, high and very high susceptibility classes respectively. As Fig. 8 a clearly shows, the very low and low susceptibility classes are dominantly concentrated in the northwestern and southwestern plateau part of the study area including Welela Bahir, Shomeda, Agona and Jinjero Gedel localities. Similarly, the very high and high susceptibility classes are concentrated in the south central, southeastern and eastern part of the study area particularly in Asfa Meda (Majeta), Dubdubiya (Arata Gebriel) and Ditorka-Megersum respectively and scarcely distributed in the northern part of the study area at Guna-Gedeba Village and in the western part. Moderate susceptibility classes are mostly distributed throughout the study area. The high concentrations of landslides in those high and very high susceptibility classes of the aforementioned areas were due to the presence of colluvial and alluvial deposits, stream undercutting, scattered vegetation cover, man-made activities like intensive farming, deforestation and cultivation.
Weights of evidence model
The landslide susceptibility map of the study area by WoE model was produced based on the weighted values from the seven causative factors and the training landslide (Table 2 ). The difference between W + and W - is known as the weight of contrast which is designated by C = W + - W - . This reflects the overall spatial association between the causative factors and landslides. LSI map of the study area was prepared by summing the weight of contrast values (C) of all the seven causative factors using a raster calculator in ArcGIS as follows:
Where LSI = landslide susceptibility index; C sl = weight contrast value of slope, C as = weight contrast value of aspect, C cu = weight contrast value of curvature, C li = weight contrast value of lithology, C lu = weight contrast value of land use, C rf = weight contrast value of rainfall, C ds = weight contrast value of distance to stream.
The LSI values for the WoE model in the study area range from -7.84 to 4.52. The LSI map is reclassified by the natural breaks method of classification technique in order to prepare the landslide susceptibility map of the study area (Fig. 8 a). Then, the LSI values were classified in to five susceptibility zones of very low (-7.84 - -3.72), low (-3.72 - -1.83), moderate (-1.83 - -0.28), high (-0.28 – 1.17) and very high (1.17 – 4.52). The result from Table 4 showed that 8.448%(15.7 km 2 ), 21.408%(39.8km 2 ), 33.140%(61.5km 2 ), 23.787% (44.2 km 2 ) and 13.216% (24.5 km 2 ) area fall in the very low, low, moderate, high and very high susceptibility classes respectively.
Validation of the model
Without model validation, landslide susceptibility maps will not be meaningful. As a result, validation of the predictive model is an important step for landslide susceptibility mapping (Bui et al. 2012 ). A predictive model map was constructed by overlying 80% of the landslides (training) over the causative factors. This model was validated using validation landslides (20%) that were not used for building the model. There are various types of validation techniques for landslide susceptibility maps. In the current study, the performance of the LSMs produced by FR and WoE models were evaluated using Area Under the Curve (AUC) and Landslide Density Index (LDI).
Area under the curve (AUC)
The area-under-curve (AUC) method works by creating success rate and prediction rate curves (Lee 2005 ). Landslide susceptibility maps can be validated by comparing the susceptibility maps with both the training landslide (80%) and validation landslide (20%). The success and predictive rate curves can be created for both FR and WoE models. The success rate curve is based on the comparison between the predictive model and the training landslide. The predictive rate curve is based on the comparison between the predicted map and the validation landslide. The Area Under the Curve (AUC) of the success rate represents the quality of the model to reliably classify the occurrence of existing landslides whereas the AUC of the predictive rate explains the capacity of the proposed landslide model for predicting landslide susceptibility (Pamela et al. 2018 ). AUC was calculated by reclassifying LSI into 50 classes with descending order of the values of pixels in the study area and combined with a landslide inventory. Then the rate curves were drawn through the cumulative percentage of both the training and validation landslide (y-axis) and cumulative area percentage (x-axis). The result showed that both models exhibited very good performances. However, the FR model is better with a success rate of 89.8% and a predictive rate of 88.2% than the WoE model with a success rate of 86.5% and a predictive rate of 84.8% (Fig. 9 ).
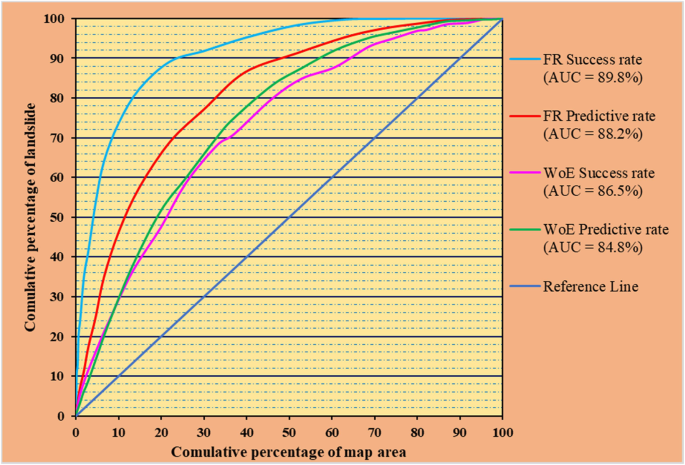
Success and Predictive rate Curves of FR and WoE models
Landslide density index (LDI)
For validation of the model, landslide pixels which have not been used for constructing the models are generally considered as the future landslide area. In this work to check the validation of the landslide susceptibility model, the testing samples that consist of 20% of the landslide pixels were overlaid over the landslide susceptibility map. The landslide density index, which is the ratio between the percentage of landslide pixels and the percentage of class pixels in each class on landslide susceptibility map, was used to validate the model (Pham et al. 2015 ). If the value of the landslide density index is increased from low to a very high susceptibility classes, then the landslide susceptibility map is considered to be valid. LDI can be calculated using the formulae in eq. 10 below and its output was presented in Table 5 . The suitability of any susceptibility map can be validated if more percentages of landslides occur in the high and very high susceptibility zones as compared to other zones (Fayez et al. 2018 ).
From Table 5 , it can be observed that the landslide density values for very high susceptibility classes are 2.743 and 2.993 with respect to WoE and FR models which are remarkably higher than the other classes. In addition to this, there is a gradual decrement in landslide density values from very high to very low susceptibility classes (Fig. 10 ). This indicates the validity of the landslide susceptibility map. Can et al. ( 2005 ) and Bai et al. ( 2010 ) stated that the landslide data should lie in either the high or very high susceptibility classes for successful validation of a LSM. Table 5 , Figs. 10 and 11 illustrate the characteristics of susceptibility classes for validation of both FR and WoE models. In general, the presence of the highest landslide percentage and density in the very high landslide susceptibility class indicates the reliability of the landslide susceptibility maps produced from FR and WoE models.
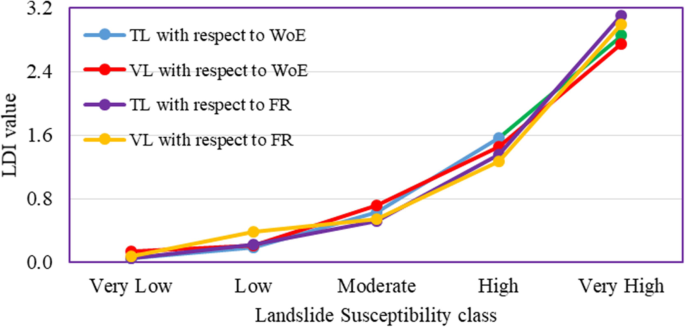
Landslide density of FR and WoE models for both training and validation landslide
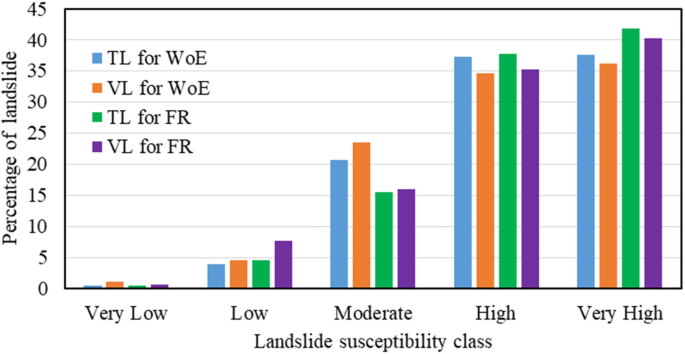
Bar diagram showing the landslide percentages in different landslide susceptibility classes
Landslide posed a significant impact at Simada District of South Gondar Zone in northwestern Ethiopia on human and animal lives, agricultural lands, settlements, infrastructures and also affected the social and economic aspects of the rural community. To investigate this problem, landslide susceptibility mapping has been carried out using FR and WoE models for proper land use planning, development and management of landslide prone areas. For this, a landslide inventory map of the study area with a total of 576 landslides was divided into training and validation landslides with 80 % and 20% respectively. Seven landslide causative factors including slope, aspect, curvature, lithology, land use, rainfall and distance to stream were considered to analyze, evaluate and establish the spatial relation of these factors with landslides. From FR values and WoE contrast values, it was possible to identify which factor classes are playing a significant role for the occurrence of landslides in the study area. The FR values that are greater than 1 and the WoE contrast (C) values that are greater than 0 were found in the factor classes of slope greater than 12°; curvature classes (-3.60) - (-0.001); aspect classes facing towards N (0 – 22.5), NE (22.5 – 67.5) and E (67.5 – 112.5); distance to stream classes (< 150m); land use classes (grazing land, river, sparse forest and bushes); lithology classes (colluvial deposit, alluvial deposit, weathered basalt and rhyolite), rainfall classes (813 – 901mm and 901 – 994 mm). The LSI map of the study area was prepared based on FR values and WoE contrast values in ArcGIS 10.4 using the spatial analyst tools of raster calculator for both FR and WoE models. The LSI map in each model was reclassified into five landslide susceptibility classes of low, low, moderate, high and very high based on the natural breaks method of classification to produce the final landslide susceptibility maps. The performance of the final landslide susceptibility maps produced by FR and WoE models were validated using Landslide Density Index (LDI) and Area Under the Curve (AUC) values. The result revealed that the very low, low, moderate, high and very high values of the landslide susceptibility map are comparable with Landslide Density Index. In case of AUC, the rate curves were drawn using the cumulative percentage of the landslide in the Y-axis and cumulative percentage of map area in the X-axis. The results showed that both models exhibited very good performance. However, the FR model, which showed a success rate of 89.8% and a prediction rate of 88.2%, is better than the WoE model with a success rate of 86.5% and a prediction rate of 84.8%. This study confirmed that the bivariate statistical methods of FR and WoE models were found to be simple and effective models for landslide susceptibility mapping in the Guna mountainous chain of Simada area. The landslide susceptibility maps of the study area were prepared with a scale of 1:50,000 which can be used by civil engineers, geologists, designers and decision-makers for regional land use planning, site selection and landslide prevention and mitigation purposes.
Recommendation
The present study showed the importance of integrating various factors that are responsible for landslide occurrence in the study area. However, the quality of landslide inventory and the causative factor maps should be improved with good quality in time and space. Landslide in the study area has affected the local people who are living near to mountainous area, valleys and gorges. Their animals were died, houses and agricultural lands were destroyed and both social and economic activities were affected. Hence, besides preparing the landslide susceptibility maps of the area, suggesting the necessary preventive measures in the high and very high susceptibility classes is very essential in order to reduce the impact of future landslide hazards in the area. Hence, this study recommends planting trees & vegetation, providing proper drainage, applying gabion and check dam, relocating people and creating public awareness. In order to implement these remedial measures, further study on the geotechnical properties of soils and rocks should be conducted in this area.
Availability of data and materials
Rainfall data was collected from National Metrology Agency of Ethiopia. Topographic Map was purchased from Ethiopian Geospatial Information Agency. DEM data was freely available from http://gdex.cr.usgs.gov/gdex/ website.
Abay A, Barbieri G (2012) Landslide susceptibility and causative factors evaluation of the landslide area of Debresina, in the southwestern Afar escarpment, Ethiopia. J Earth Sci Eng 2:133–144
Google Scholar
Abebe B, Dramis F, Fubelli G, Umar M, Asrat A (2010) Landslides in the Ethiopian highlands and the rift margins. African Earth science 56:131–138
Article Google Scholar
Akgun A, Dag S, Bulut F (2007) Landslide susceptibility mapping for a landslide-prone area (Findikli, NE of Turkey) by likelihood-frequency ratio and weighted linear combination models. Eng Geol V 54:1127–1143
Akgun A, Turk N (2011) Mapping erosion susceptibility by multivariate statistical method: a case study from the Ayvalik region. NW Turkey Computers & Geosciences, Turkey
Akgun AA (2012) Comparison of landslide susceptibility maps produced by logistic regression, multi-criteria decision, and likelihood ratio methods: a case study at İzmir, Turkey. Landslides 9:93–106
Aleotti P, Chowdhury R (1999) Landslide Hazard assessment: summary, review and new perspectives. Bull Eng Geol Environ 58:21–44
Ayalew L (1999) The effect of seasonal rainfall on landslides in the highlands of Ethiopia. Bull Eng Geol Environ 58:9–19
Ayalew L, Yamagishi H (2005) The application of GIS based logistic regression for landslide susceptibility mapping in the KakudaYahiko Mountains Central Japan. Geomorphology 65(1):15–31
Baharin A, Farshid S, Javad M, Barat M (2014) Using Frequency Ratio Method for Spatial Landslide Prediction. Res J App Sci Eng Technol. 7:3174–3180
Bai SB, Wang J, Lu G-N, Zhou P-G, Hou S-S, Xu S-N (2010) GIS-based logistic regression for landslide susceptibility mapping of the Zhongxian segment in the three gorges area, China. Geomorphology 115:23–31 https://doi.org/10.1016/j.geomorph.2009.09.025
Bui DT, Pradhan B, Lofman O, Revhaug I, Dick OB (2012) Spatial prediction of landslide hazards in Hoa Binh province (Vietnam): a comparative assessment of the efficacy of evidential belief functions and fuzzy logic models. Catena 96:28–40
Can, Nefeslioglu HA, Gokceoglu C, Sonmez H, Duman TY (2005) Susceptibility assessments of shallow earthflows triggered by heavy rainfall at three catchments by logistic regression analyses
Book Google Scholar
Chen FW, Liu CW (2012) Estimation of the spatial rainfall distribution using inverse distance weighting (IDW) in the middle of Taiwan. Paddy Water Environ 10:209–222
Choi J, Oh H-J, Lee H-J, Lee C, Lee S (2012) Combining landslide susceptibility maps obtained from frequency ratio, logistic regression, and artificial neural network models using ASTER images and GIS. Eng Geol 124:12–23
Crozier MJ (1986) Landslides: causes, consequences & environment. Croom Helm Pub, London
Cruden DM (1991) A simple definition of a landslide. Bulletin of the International Association of Engineering Geology 43(1):27–29. https://doi.org/10.1007/bf02590167
Cruden DM, Varnes DJ (1996) Landslide types and processes. National Research Council, Washington DC
Dahal RK, Hasegawa S, Nonomura A, Yamanaka M, Dhakal S, Paudyal P (2008) Predictive modelling of rainfall-induced landslide hazard in the Lesser Himalaya of Nepal based on weights-of-evidence. Geomorphology 102:496–510
Dai FC, Lee CF, Ngai YY (2002) Landslide risk assessment and management: an overview. Eng Geol 64:65–87
Demir G, Aytekin M, Akgün A, Ikizler SB, Tatar O (2013) A comparison of landslide susceptibility mapping of the eastern part of the North Anatolian Fault zone (Turkey) by likelihood-frequency ratio and analytic hierarchy process methods. Nat Hazards 65:1481–1506
Dikau R, Brunsden D, Schrott L, Ibsen M-L (eds) (1996) Landslide recognition. Identification, movements and causes. Wiley, Chichester, p 251
Fayez L, Pham BT, Solanki HA, Pazhman D, Dholakia MB, Khalid M, Prakash I (2018) Application of frequency ratio model for the development of landslide susceptibility mapping at part of Uttarakhand state, India. Int J Appl Eng Res 13:6846–6854
Felicisimo ÁM, Cuartero A, Remondo J, Quirós E (2012) Mapping landslide susceptibility with logistic regression, multiple adaptive regression splines, classification and regression trees, and maximum entropy methods: a comparative study. Landslides 10:175–189
Gholami M, Ghachkanlu EN, Khosravi K, Pirasteh S (2019) 2018 Landslide prediction capability by comparison of frequency ratio, fuzzy gamma and landslide index methods. J Earth Syst Sci 128:42 https://doi.org/10.1007/s12040-018-1047-8 Indian Academy of Sciences
Girma F, Raghuvanshi TK, Ayenew T, Hailemariam T (2015) Landslide hazard zonation in Ada Berga district, Central Ethiopia – a GIS-based statistical approach. J Geom 90:25–38
Glade T (2003) Landslide occurrence as a response to land-use change: a review of evidence from New Zealand. Catena 51(3–4):297–314
Glade T, Anderson M, Crozier MJ (eds) (2005) Landslide Hazard and Risk. https://doi.org/10.1002/9780470012659
Guzzetti F, Mondini AC, Cardinali M, Fiorucci F, Santangelo M, ChangM KT (2012) Landslide inventory maps: new tools for an old problem. Earth Sci Rev 112:42–66 Elsevier
Hong H, Xu C, Bui DT (2015) Landslide susceptibility assessment at the Xiushui area (China) using frequency ratio model. Procedia Earth and Planetary Science 15:513–517
Hutchinson JN (1989) General report: morphological and geotechnical parameters of landslides in relation to geology and hydrogeology. International Journal of Rock Mechanics and Mining Sciences & Geomechanics Abstracts 26(2):88. https://doi.org/10.1016/0148-9062(89)90310-0
Kanungo D, Arora M, Sarkar S, Gupta R (2009) Landslide susceptibility zonation (LSZ) mapping-a review. J South Asia Disaster Stud 2:81–105
Kanungo DP, Arora MK, Sarkar S, Gupta RP (2006) A comparative study of conventional, ANN black box, fuzzy and combined neural and fuzzy weighting procedures for landslide susceptibility zonation in Darjeeling Himalayas. Eng Geol 85:347–366
Khan H, Shafique M, Khan M, Bacha M, Shah S, Calligaris C (2019) Landslide susceptibility assessment using Frequency Ratio, a case study of northern Pakistan. Egyptian J Remote Sensing Space Sci 22(1):11–24. https://doi.org/10.1016/j.ejrs.2018.03.004
Komac M (2006) A landslide susceptibility model using the analytical hierarchy process method and multivariate statistics in perialpine Slovenia. Geomorphology 74(1–4):17–28
Lee S (2005) Application of logistic regression model and its validation for landslide susceptibility mapping using GIS and remote sensing data. Int J Remote Sens 26(7):1477–1491. https://doi.org/10.1080/01431160412331331012
Lee S, Pradhan B (2007) Landslide hazard mapping at Selangor, Malaysia using frequency ratio and logistic regression models. Landslides. 4:33–41
Lee S, Talib JA (2005) Probabilistic landslide susceptibility and factor effect analysis. Environ Geol 47:982–990
Article CAS Google Scholar
Meten M, Bhandary NP, Yatabe R (2015a) Effect of landslide factor combinations on the prediction accuracy of landslide susceptibility maps in the Blue Nile gorge of Central Ethiopia. Geoenviron Disast 2:9 https://doi.org/10.1186/s40677-015-0016-7
Meten M, Bhandary NP, Yatabe R (2015b) GIS-based frequency ratio and logistic regression modeling for landslide susceptibility mapping of Debre Sina area in Central Ethiopia. J Mt Sci 12(6). https://doi.org/10.1007/s11629-015-3464-3
Mezughi TH, Akhir JM, Rafek AG, Abdullah I (2011) Landslide susceptibility assessment using frequency ratio model applied to an area along the E-W highway (Gerik-Jeli). Am J Environ Sci 7:43–50
Mohammady M, Pourghasemi HR, Pradhan B (2012) Landslide susceptibility mapping at Golestan Province, Iran: a comparison between frequency ratio, Dempster-Shafer, and weights-of-evidence models. J Asian Earth Sci. 61:221–236
Mondal S, Maiti R (2013) Integrating the analytical hierarchy process (AHP) and the frequency ratio (FR) model in landslide susceptibility mapping of shiv-khola watershed, Darjeeling Himalaya. Int J Disaster Risk Sci 4:200–212
Pamela, Sadisun IA, Arifianti Y (2018) Weights of evidence method for landslide susceptibility mapping in Takengon, Central Aceh, Indonesia. IOP Conference Series: Earth and Environmental Science, 118:012037. https://doi.org/10.1088/1755-1315/118/1/012037
Pan X, Nakamura H, Nozaki T, Huang X (2008) A GIS-based landslide hazard assessment by multivariate analysis landslides. J Jpn Landslide Soc 45(3):187–195
Pardeshi SD, Autade SE, Pardeshi SS (2013) Landslide hazard assessment: recent trends and techniques. SpringerPlus 2(1). https://doi.org/10.1186/2193-1801-2-523
Parise M, Jibson RW (2000) A seismic landslide susceptibility rating of geologic units based on analysis of characteristics of landslides triggered by the 17 January, 1994 Northridge, California earthquake. Eng Geol 58:251–270
Park S, Choi C, Kim B, Kim J (2012) Landslide susceptibility mapping using frequency ratio, analytic hierarchy process, logistic regression, and artificial neural network methods at the Inje area, Korea. Environ Earth Sci 68:1443–1464
Paulin L, Bursik G, Hubp M, Mejía JL, Aceves Quesada FLMP (2014) A GIS method for landslide inventory and susceptibility mapping in the Río El Estado watershed, Pico de Orizaba volcano, México. Nat Hazards 71:229–241
Peng L, Niu R, Huang B, Wu X, Zhao Y, Ye R (2014) Landslide susceptibility mapping based on rough set theory and support vector machines: a case of the three gorges area, China. Geomorphology 204:287–301 Elsevier B.V
Pham BT, Tien Bui D, Prakash I, Dholakia M (2015) Landslide susceptibility assessment at a part of Uttarakhand Himalaya, India using GIS–based statistical approach frequency ratio method. Int J Eng Res Technol 4:338–344
Pirasteh S, Li J (2017) Probabilistic frequency ratio (PFR) model for quality improvement of landslide susceptibility mapping from LiDAR-derived DEMs. Pirasteh Li Geoenviron Dis 4:19 https://doi.org/10.1186/s40677-017-0083-z
Pradhan B, Lee S, Buchroithner MF (2010) Remote Sensing and GIS-based Landslide Susceptibility Analysis and its Cross-validation in Three Test Areas Using a Frequency Ratio Model. Photogrammetrie-FernerkundungGeoinformation 2010(1):17–32. https://doi.org/10.1127/1432-8364/2010/0037
Rahmati O, Haghizadeh A, Pourghasemi HR, Noormohamadi F (2016) Gully erosion susceptibility mapping: the role of GIS-based bivariate statistical models and their comparison. Nat Hazards 82(2):1231–1258 https://doi.org/10.1007/s11069-016-2239-7
Regmi AD, Devkota KC, Yoshida K, Pradhan B, Pourghasemi HR, Kumamoto T, Akgun A (2013) Application of frequency ratio, statistical index, and weights-of-evidence models and their comparison in landslide susceptibility mapping in Central Nepal Himalaya. Arab J Geosci 7(2):725–742 https://doi.org/10.1007/s12517-012-0807-z
Regmi NR, Giardino JR, Vitek JD (2010) Modeling susceptibility to landslides using the weight of evidence approach: Western Colorado, USA. Geomorphology 115(1-2):172–187. https://doi.org/10.1016/j.geomorph.2009.10.002
Temesgen B, Mohammed U, Asfawosen A (1999) Landslide hazard on the Dabicho, Wondo genet area: the case of June 18, 1996 event. SENET: Ethiop J Sci 22(l):l27–l140
Temesgen B, Mohammed U, Korme T (2001) Natural Hazard assessment using GIS and remote sensing methods, with particular reference to the landslides in the Wondogenet area, Ethiopia. Phys Chem Earth Part C: Solar Terrestr Planet Sci (C) 26(9):665–615
Vakhshoori V, Zare M (2016) Landslide susceptibility mapping by comparing the weight of evidence, fuzzy logic, and frequency ratio methods. Geomatics Nat Hazards Risk. 7(5):1731–1752
Van Westen CJ (2002) Use of weights of evidence modeling for landslide susceptibility mapping. International Institute for Geoinformation Science and Earth Observation (ITC), Enscheda
Varnes DJ (1978) Slope movements: types and processes. In: Schuster, R.L. and Krizek, R.J. (eds.) Landslide analysis and control, National Academy of Sciences, Transportation Research Board Special Report 176, Washington, 11-33.
Wang Q, Li W (2017) A GIS-based comparative evaluation of analytical hierarchy process and frequency ratio models for landslide susceptibility mapping. Phys Geogr 38(4):318–337
Woldearegay K (2008) Characteristics of a large-scale landslide triggered by heavy rainfall in Tarmaber area, central highlands of Ethiopia. Geophys Res Abstr 10:EGU2008-A-04506 2008, SRef – ID:16077962/gra/EGU2008-A-04506
Woldearegay K (2013) Review of the occurrences and influencing factors of landslides in the highlands of Ethiopia. Momona Ethiop J Sci (MEJS) 5(1):3–31
WP/WLI - International Geotechnical Societies’ UNESCO Working Party on World Landslide Inventory (1990) A suggested method for reporting a landslide. Int Assoc Eng Geol Bull 41:5–12
Yalcin A, Bulut F (2007) Landslide susceptibility mapping using GIS and digital photogrammetric techniques: a case study from Ardesen (NE-Turkey). Nat Hazards 41:201–226
Yalcin A, Reis S, Aydinoglu AC, Yomralioglu T (2011) A GIS-based comparative study of frequency ratio, analytical hierarchy process, bivariate statistics and logistics regression methods for landslide susceptibility mapping in Trabzon, NE Turkey. CATENA 85(3):274–287 https://doi.org/10.1016/j.catena.2011.01.014
Ye C, Li Y, Cui P, Liang L, Pirasteh S, Marcato J et al (2019) Landslide Detection of Hyperspectral Remote Sensing Data Based on Deep Learning With Constrains. IEEE J Selected Topics Appl Earth Observ Remote Sensing 12(12):5047–5060. https://doi.org/10.1109/jstars.2019.2951725
Yilmaz I (2009) Landslide susceptibility mapping using frequency ratio, logistic regression, artificial neural networks and their comparison: A case study from Kat landslides (Tokat—Turkey). Comput Geosci 35(6):1125–1138
Download references
Acknowledgments
The first author would like to thank Dr. Kifle Woldearegay, Mr. Azmeraw Wubalem and Mr. Leulalem Shano for thier useful Comments and suggestions to improve this research work. He would also like to thank Mizan Tepi University for providing the scholarship opportunity to pursue his MSc study at Addis Ababa Science and Technology University.
Mizan Tepi University partially funded the first author’s MSc thesis research work.
Author information
Authors and affiliations.
Department of Geology, College of Applied Sciences, Addis Ababa Science and Technology University, Addis Ababa, Ethiopia
Tilahun Mersha & Matebie Meten
Department of Geology, College of Natural and Computational Science, Mizan Tepi University, Tepi, Ethiopia
Tilahun Mersha
You can also search for this author in PubMed Google Scholar
Contributions
TM as a first author has mostly participated in the whole process of this research work including the field-work, data collection, database preparation and in compiling the results by himself. MM, as an advisor, commented in each phase of the research for further improvement. TM addressed the comments given from his advisor in terms of its scientific justification, methodological aspect and English correction before the final submission and finally the two authors approved this paper submission.
Corresponding author
Correspondence to Tilahun Mersha .
Ethics declarations
Competing interests.
The authors declare that we do not have any competing interests with any individual or organization.
Additional information
Publisher’s note.
Springer Nature remains neutral with regard to jurisdictional claims in published maps and institutional affiliations.
Rights and permissions
Open Access This article is licensed under a Creative Commons Attribution 4.0 International License, which permits use, sharing, adaptation, distribution and reproduction in any medium or format, as long as you give appropriate credit to the original author(s) and the source, provide a link to the Creative Commons licence, and indicate if changes were made. The images or other third party material in this article are included in the article's Creative Commons licence, unless indicated otherwise in a credit line to the material. If material is not included in the article's Creative Commons licence and your intended use is not permitted by statutory regulation or exceeds the permitted use, you will need to obtain permission directly from the copyright holder. To view a copy of this licence, visit http://creativecommons.org/licenses/by/4.0/ .
Reprints and permissions
About this article
Cite this article.
Mersha, T., Meten, M. GIS-based landslide susceptibility mapping and assessment using bivariate statistical methods in Simada area, northwestern Ethiopia. Geoenviron Disasters 7 , 20 (2020). https://doi.org/10.1186/s40677-020-00155-x
Download citation
Received : 09 October 2019
Accepted : 11 May 2020
Published : 15 June 2020
DOI : https://doi.org/10.1186/s40677-020-00155-x
Share this article
Anyone you share the following link with will be able to read this content:
Sorry, a shareable link is not currently available for this article.
Provided by the Springer Nature SharedIt content-sharing initiative
- Frequency ratio
- Weights of evidence
- Landslide susceptibility
Academia.edu no longer supports Internet Explorer.
To browse Academia.edu and the wider internet faster and more securely, please take a few seconds to upgrade your browser .
Enter the email address you signed up with and we'll email you a reset link.
- We're Hiring!
- Help Center
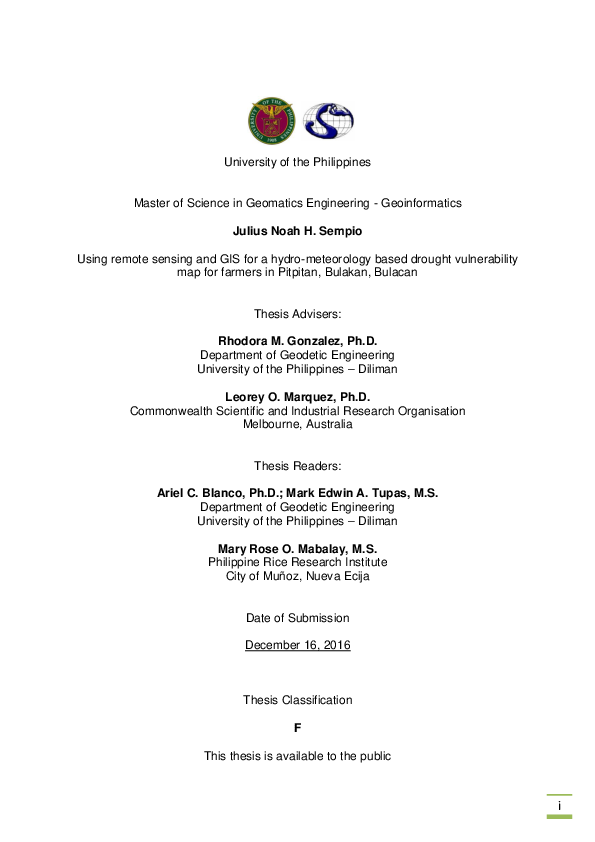
Remote sensing and GIS for drought vulnerability for farmers (ed.1)

For agriculture to withstand the adverse effects of climate change and remain productive in spite of unusual weather phenomena, active adaptation is a practicable option for farmers and government agencies alike. Other strategies, such as the construction of water retention ponds and developing climate-adaptable varieties, require heavy funding that, without institutional support, are beyond the Filipino farmers’ financial capabilities. Also, these strategies take many years to build and bear fruit. In view of these shortcomings and vulnerability to extreme weather events, immediate and low-cost adaptation measures are needed for farmers to consider while waiting for the long-term solutions. This paper presents the development of a methodology using remote sensing and GIS techniques in determining the vulnerability to climate irregularities of a farming community in Pitpitan, Bulakan, Bulacan. This methodology is intended to become part of a GIS-based climate adaptation recommender system that, in consideration of farmers’ customary practices, will help to inform them of alternative adaptive measures in cases of imminent climate issues such as drought and floods. Search of related literature, personal visits to concerned local government units, and discussions with Pitpitan’s farmers reveal that, in general, farmers are more concerned with drought than with heavy flooding. Thus focus was given on helping farmers cope with drought conditions, and the developed methodology made use of a reduced version of the agricultural drought vulnerability index (ADVI), which is a data-intensive climatic vulnerability assessment index developed in India. ADVI is modified in order to take into account current data shortcomings of the Philippines, and still come up with a potentially useful analysis tool for extreme climate vulnerability of farm parcels in Pitpitan. The resulting thematic maps are then validated by farmers for being accurate and informative. A prototype table of recommendations was also developed with the reduced ADVI (rADVI) methodology, which aims to provide farmers with suggested adaptation measures based on their parcels’ rADVI ranking and the expected rainfall situation in the Pitpitan area. These recommendations at parcel level were arrived at using GIS that incorporates both established agricultural strategies and local farmers’ knowledge of time-tested strategies.
Related Papers
Julius Sempio , Leorey O Marquez
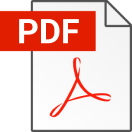
Venkataraman Balaji
Drought occurs due to a deficiency of precipitation over an extended period of time, usually for a season or more, affecting virtually all climate regimes. This deficiency results in water shortage for some activities, group or environmental sector, causing economic losses and significant damage to human lives. Furthermore, water demand for growing human population, industrial development and agriculture has increased significantly in developing countries threatening the outcome of major environmental, social and economic problems. With natural calamities such as drought becoming a recurrent phenomenon, science-based interventions using GIS-based tools to predict the severity of drought in an area can potentially contribute to mitigation efforts.
Wilfredo Uy , Venus Leopardas
Remote Sensing
Bayes Ahmed
Agriculture is one of the fundamental economic activities in most countries; however, this sector suffers from various natural hazards including flood and drought. The determination of drought-prone areas is essential to select drought-tolerant crops in climate sensitive vulnerable areas. This study aims to enhance the detection of agricultural areas with vulnerability to drought conditions in a heterogeneous environment, taking Bangladesh as a case study. The normalized difference vegetation index (NDVI) and land cover products from the Moderate Resolution Imaging Spectroradiometer (MODIS) satellite images have been incorporated to compute the vegetation index. In this study, a modified vegetation condition index (mVCI) is proposed to enhance the estimation of agricultural drought. The NDVI values ranging between 0.44 to 0.66 for croplands are utilized for the mVCI. The outcomes of the mVCI are compared with the traditional vegetation condition index (VCI). Precipitation and crop yield data are used for the evaluation. The mVCI maps from multiple years (2006-2018) have been produced to compute the drought hazard index (DHI) using a weighted sum overlay method. The results show that the proposed mVCI enhances the detection of agricultural drought compared to the traditional VCI in a heterogeneous environment. The "Aus" rice-growing season (sown in mid-March to mid-April and harvested in mid-July to early August) receives the highest average precipitation (>400 mm), and thereby this season is less vulnerable to drought. A comparison of crop yields reveals the lowest productivity in the drought year (2006) compared to the non-drought year (2018), and the DHI map presents that the northwest region of Bangladesh is highly vulnerable to agricultural drought. This study has undertaken a large-scale analysis that is important to prioritize agricultural zones and initiate development projects based on the associated level of vulnerability.
Biswajit Das
Sumanta Das, Malini Roy Choudhury & Sachikanta Nanda
TJPRC Publication
Geospatial techniques have played a key role in studying different types of hazards either natural or manmade. Temporal satellite data of three years 2000, 2005 and 2010 are used to monitor and assess the drought severity and the impact of agricultural drought on crop production. In Bankura District, agricultural drought and crop failure have been common and spatial variability of rainfall in cropping season with frequent and longer dry spells. This makes them vulnerable to the risk of agricultural drought. This study is conducting with the objective of assessing agricultural drought risk and it’s impacts on yield reduction using RS and GIS techniques. The digital indices using satellite data namely, NDVI (Normalized difference Vegetation index) and NDVI anomaly can be prepared from the long term mean values of maximum NDVI to assess the severity of drought. VCI(Vegetation condition Index), TCI(Temperature condition Index), SPI(Standardized Precipitation Index), MSI(Moisture Stress Index) and YVI(Yellowness vegetation Index) is very essential to Assess the agricultural drought. The impact of agricultural drought on crop production was measured through estimation of yield reduction(in %). Compared to other cropping seasons of the analysis period, yield reduction for the year 2005 was lesser than the highly drought years 2000 and 2010. Simple regression analyses has performed between Land surface temperature with NDVI, TCI with VCI and SPI,MSI, NDVI Anomaly, VCI with Yield reduction(%). Finally, a resultant drought vulnerability map was obtained by integrating NDVI Anomaly, MSI, SPI, VCI, TCI and YVI which indicates the area facing a combined drought. The combined vulnerability map shows that 6% area has no risk, 53 % area face moderate risk and 41 % area face high risk within the entire geographical area. Thus, this agricultural drought risk mapping can be useful to guide decision making process in drought monitoring and to reduce the risk of drought on agricultural production and productivity.
Philippine Geomatics Symposium (PhilGEOS) 2017
Julius Sempio
This paper proposes a reduced form of the agricultural drought vulnerability index (Murthy, Laxman, & Sesha Sai, 2015), that is, some of the contributing indicators for the composite indices were set aside due to data restrictions in the Philippine setting, focusing instead on what data were readily available for the study area: a barangay-level farming community in Pitpitan, Bulakan, Bulacan. The vulnerability map produced by rADVI for the community’s irrigated season was then subjected to validation purposes to see if its output was acceptable to the farmers.
Bhawna Sharma
As part of a pilot project, a decision making assistance tool was developed; this was done considering the high sensitivity to rainfall variability in rice crops over the coastal region of Ecuador. Through land cover, demographic distribution, topography, flooding geospatial information at 1:50.000 scale, and socio economic impacts statistics, vulnerability estimation was performed. This approach was based on the combination of “exposure to risk” with “coping capacity”. Processed vulnerability maps during a rainy season in Ecuador (January-May 2005), were compared with the documented impacts in rice crops in the area during the same period showed a fairly acceptable correlation between them (more than 65%).Risk maps were distributed to community leaders and other local contacts. Distribution of vulnerability maps to potential users in Ecuador has lead to the increasing of a distribution list of more than 200 direct users of the information with the capacity to spread this informatio...
Firoz Ahmad
Agroforestry has the capacity to reduce the poverty, enhance livelihood, improve food security and mitigate the climate change impact. The agroforestry suitability mapping is performed using geospatial technology based on similar guideline documented by FAO for Land suitability mapping. The study aims for applying remote sensing and GIS technology towards various spatial (soil and environmental) datasets in Dumka district of Jharkhand, India to achieve a nutrient availability and agroforestry suitability map. The Landsat satellite data, ASTER DEM and decadal annual rainfall datasets were utilized to generate wetness, landuse/ land cover (LULC), elevation, slope, and rainfall map. LULC map was utilized to identify the open area land in which agroforestry suitability was evaluated. Various thematic layers such as soil nitrogen (N), phosphorus (P), potassium (K), organic carbon (C), pH and sulphur (S) were used for generating nutrient availability map. The agroforestry suitability map was generated utilizing the layers viz. nutrient availability, slope, wetness, rainfall and elevation by GIS integration/modeling in ArcGIS 10.1 software by providing different weight to each thematic layer as per its importance in the study area. The thematic layer weight was calculated based on pairwise comparisons (a standard statistical procedure). Finally agroforestry suitability maps were generated in the form of high, medium and low grid with spacing (1km × 1km). We also generated poverty map, one of the complete watersheds in the part of the study area and drainage map in whole study area to understand its proximity toward high agroforestry suitability grid. The study shows that the total open area land grids were 3421 out of which 50% of grid was found to be highly suitable towards agroforestry. Furthermore a watershed, drainage pattern and poverty map were analyzed based on its proximity toward high agroforestry suitability. The study reveals high agroforestry suitability land grid proximity towards high poverty grid was approximately more than 70%, whereas the proximity to the drainage pattern was roughly 60%. One of the completed watershed in the part of the study area evaluation reveals approximately 50% of the watershed area grid has the proximity of high agroforestry suitable land grid. The high agroforestry suitability and its proximity/relationship with high poverty, drainage pattern and in watershed based analysis provides golden opportunity to the local poor people to harness agroforestry practices by adopting intensive soil and water conservation measures if supported by sufficient funds/technology will largely help in mitigating poverty and enhancing the livelihood. The ongoing agroforestry projects can be further extended in the open areas of high agroforestry suitability grid for long term benefits of diversified output.
RELATED PAPERS
Yuri Moreira
Bulletin of University of Agricultural Sciences and Veterinary Medicine Cluj-Napoca. Veterinary Medicine
Marina Spinu
Celal Bayar Üniversitesi Fen Bilimleri Dergisi
beril ertınmaz
Lentera Perawat
M Agung Akbar
Alfredo Cano
Wuri Handoko
hyghjgjh liioiyt
Frontiers in Medical Technology
Frances Hsia
Water science and technology : a journal of the International Association on Water Pollution Research
Gordon Yang
Celestial Mechanics & Dynamical Astronomy
Paolo Paolicchi , Alberto Cellino
VOLUME-8 ISSUE-10, AUGUST 2019, REGULAR ISSUE
Prof A K Verma
Frontiers in Behavioral Neuroscience
The American Journal of Cardiology
Francesco Pozzar
Geoffrey Kane
New Directions in Elite Studies
Johan Heilbron
Zeitschrift f�r Physik A Atomic Nuclei
Thomas Massey
Revista da Jornada de Pós-Graduação e Pesquisa - Congrega Urcamp
Jaison Luiz
agungbs Hefram
Scientific Reports
Seng Ghee Tan
Food ScienTech Journal
Nia Gusti Eka Putri
RELATED TOPICS
- We're Hiring!
- Help Center
- Find new research papers in:
- Health Sciences
- Earth Sciences
- Cognitive Science
- Mathematics
- Computer Science
- Academia ©2024
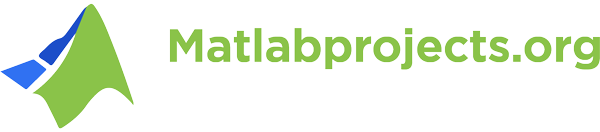
Thesis on GIS and Remote Sensing
Remote sensing is a technology that investigates the earth with help of satellites which is deployed with cameras and sensors. ...
- matlabprojects.org
- [email protected]
- +91 9790238391
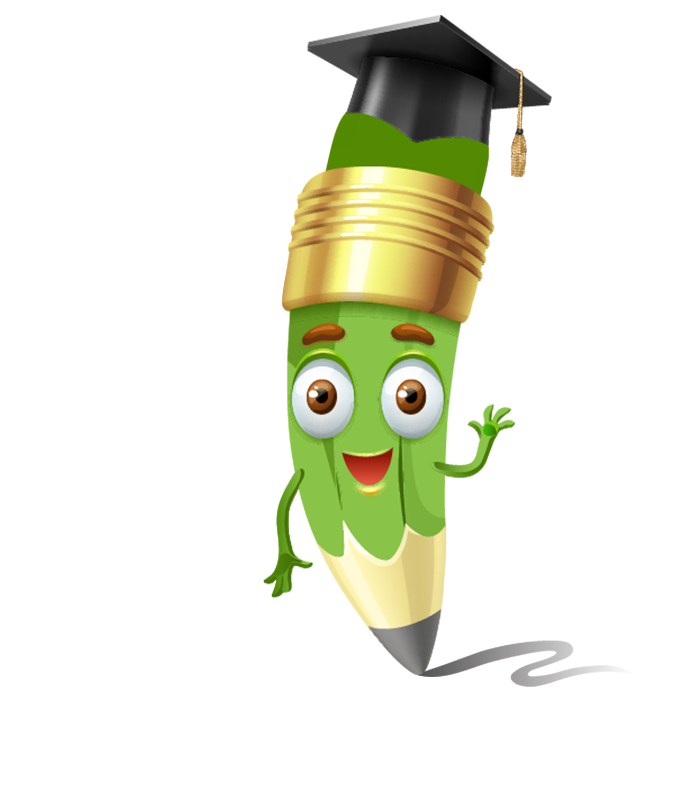
Remote sensing is a technology that investigates the earth with help of satellites which is deployed with cameras and sensors. Generally, the manual observation of natural resources is quite difficult. In the event of this challenge developers and technology engineers have developed a mapping tool called GIS.
The acronym stands for the Geographic Information System. GIS will gather data like economic improvement of the nation, population information, and the plant types in the farmlands. The effective regulation of the tool is based on the assimilation of the general server information and their arithmetical investigations corresponding with the remote sensing road maps.
This article will enumerate the general thesis on GIS and Remote Sensing in detail!!
Now we will see about remote sensing and their thesis on GIS. Every technology supports some tools to enhance the process effectively. For the ease of your understanding, the developers of our concern made the key points very short and sweet. Let us have an understanding of the thesis on GIS and remote sensing.
Overview of GIS and Remote Sensing
- The data gathered by the remote sensing tool will be used in the Geographical Information System (GIS) assimilation
- The gathered data are always represented in the form of images
- The GIS Geographical Information System, itself indicates that it is a location-oriented information gathering tool
- It is the advanced graphic visualization technology that eliminates the traditional spreadsheet in capabilities
- Furthermore it the visualization of the issues will lead to safety measures in an earlier stage
This is all about remote sensing and Geographical Information systems in general. Usually, every researcher needs an expert’s guidance for effective outcomes. Now we will see about the road maps of remote sensing.
Steps involved in the Remote Sensing
- The sunlight rays directly explore the environmental atmosphere
- The earth surface reflected by the rays
- The reflected rays will be gathered by the cameras
- The captured rays will be turned into images
- The sensor will gather the images
- The gathered images will be stored in the database
- Then it will be displayed for the analytical purpose
These are the main steps involved in remote sensing as they are presented in hierarchical this will be very easy to understand. As of now, we had seen the Geographical Information System, remote sensing, and its road map in detail to formulate Thesis on GIS and Remote Sensing. It is time to discuss the source of remote sensing in brief.
What is the Data Source for the Remote Sensing?
- Data of the streets
- Data of the buildings
- Data regarding vegetation
- Integration of the data
The above-mentioned key points are the sources of remote sensing. We hope that it will be very easy to remember. Furthermore, we will see about the application of the Geographical Information System in remote sensing.
- The gathered information of the living and non-living creatures on the earth surface is subject to the zero interaction
- Sensors in the remote sensing are assimilated with the Geographical Information System for visualization and analytical purpose which will be very understandable
How does Remote Sensing works?
- It is the primary source of the energy that is produced by the environment
- Direct solar radiance of the energy by the direct transmission of the primary source
- The intensity of the radiance is related to the improvement in the viewpoint of the related scene renewable energy
- This is where the creatures of the earth is living, this surface is subject to the reflection of the radiance and the light produced
- Determination of the temperature and the radiation produced in the surface
- The terrain is the 3D landscape tool used to handle the queries and the generation of the mirrored reflection
- Visibility of the atmosphere
- The type of the atmosphere will be stated by the model on each particle presented in each layer
- The commotion of the images generated a lack of quality (Blurriness)
- The high volume of the particles leads to the haze in the absorption
- Transmission of the radiance which is produced in the atmosphere is subject to the remote sensing
- Upwelling and Downwelling Radiance
This is the way how the surface, atmosphere, and the ultimate source of remote sensing are integrated. The above-explained aspects will be effective for understanding. As our researchers made this article for ease of understanding which will lead the readers to explore the things in it.
We would like to share our victories with you, the company that served around 120+ countries in the world with our project and research ideas for Thesis on GIS and Remote Sensing. Additionally, we are having 5000+ happy customers who are served by our ideas. Without wasting time we will move to the next phase about the features of remote sensing.
Important Characteristics of Remote Sensing
- This is all about the least particles in the scenery which will be filtered out
- This is all about the sensors bandwidth and the specimen rate
- The satellites used in the sensors will repeatedly visit the Geolocation for several days based on their range
- The limitations of the SNR (Signal to Noise Ratio) and capacity lies on the high spatial or the low spectral ratio
- This has capable of tracking the isolated and vibrant range signals and systems
- The satellites used here is Landsat 7 which has the capacity of 8-bit images, 256 unique grey values produced by the energy, and 2048 radiometric grey values which leads to immediate monitoring
We are doing researches in the various fields that are emerging now a day. They are mentioned below for your understanding. It is a worthy note to be taken as of now. Without wasting time we will have a quick summary of our research areas Thesis on GIS and Remote Sensing.
The researchers in our concern are habitually exploring them and transferring the technical facts in the research guidance!!
So far, we gave you a basic overview of remote sensing and the GIS in brief now is the time to understand the applications of the GIS in remote sensing. They come under the environmental, economic, and social development of sustainable cities.
What are the important aspects of GIS in Remote Sensing?
- Detecting the slum areas
- Population data and the computation of the quality of the life
- Assessment of challenges involved in the environment
- Observation of the rural and urban areas
- Assessing the remaining usable land
- Evaluating the lands and their allocation
- Discovering the impaired building infrastructures
- Evaluation of the GDP (Gross Domestic Product)
- Computation of the energy landscapes
- Renewable energy assessment
- Observing the land-use changes
- Evaluating the accurate population
- Evaluating the expansion of an urban area
- Evaluation of the pollution matrix
- Assessment of temperature conditions
Now we will see about the procedures or methods oriented with the Geographical Information System and Remote Sensing. The following techniques will let you know the key factors very keenly.
Methods for GIS and Remote Sensing
- DNA computing
- Cellular Automated Membrane computing
- Evolutionary computing
- Neuro-computing
- Charge system search
- Gravitational search
- Bee huddling algorithm
- An artificial flock of fishes
- Bacteria hunting
- Firefly optimization
- Intelligent water drops
- Cuckoo search
- Swarm particle optimization
- Biogeography optimization
- Dynamics of the river formation
- Ant colony optimization
- Wisdom technology
- Anticipatory computing
- Granular computing
- Rough set theory
- Fuzzy set theory
- Perception-based computing
These are the methods involved in the Geographical Information System and remote sensing. We hope that you will understand the aspects explained. Our developers and researchers have done this article with essential aspects which are very needy in technology. Next, we will see about the latest ideas oriented with Thesis on GIS and remote sensing in detail.
Ideologies are the eminent thing while researching a technology. We are there for you to assist in the research areas!!
As we are providing research and project-related assistance to our scholars and the college students get our expert’s assistance in research areas. We assist in the fields of thesis writing, conference paper writing, system development, paper publication, and so on by well-organized experts. Do you surf for research guidance or thesis writing and then this is the right place to do it. We are providing 24/7 supports with a clear understanding.
Current trends on GIS and Remote Sensing
- Planning of urban development
- Disaster Alleviation and organization
- Earth observation and plotting following the remote sensing systems
- Organization of the water resources
- Impervious earthquake model
- Planning on traffic aspects and transportation construction
- Reintegration of the structures
- Ecological improvement and creation
These are the emerging ideas that are currently in trend. Knowing about the databases used in GIS and remote sensing is very important. To this, we have been mentioned the databases in the following passage. By observing the earth aspects, enormous volumes of the multi-spectrum, multi-temporal, multi-resolution pictures are produced which results in performance enhancements.
Databases used in the GIS and Remote Sensing
- This database represents the geospatial information with the help of NEO4J libraries
- These libraries collect the logs and
- stores them for analysis
- Layers of the NEO4J consisted of the geometrics and geo indices
- The data acquisition is pillared with the help of Open Street Map and Shapefiles
- This easy approach stores the geometric indices in their well-known text or format (WKT)
- This has a format called coordinate pairs which will be added in the planners, for instance, JSON planner will be denoted as GeoJSON
- This database represents the geospatial vectors which are built in the form of spatial queries
- The coordinate pairs indicate the different geographic data structures
Performance Metrics in GIS and Remote Sensing
- This is all about how the platform plotting the relevant scenes in the
- Evaluating the collection of the picture in each second to run a video
- The time taken for the capturing will result in the effective object
- This is about the distance between the platform range and the earth surface
- For the view of the geometric aspects
- Casual regulation of the camera
- This is about the time taken for the capturing of scenarios by per pixel
Master / doctoral thesis is a research study that is done by the students following real observation and experiments. This is an empirical research study that focuses on the student’s execution of the research till the climax. Now we will see about the components involved in the master thesis writing .
What are the chapters of Thesis Writing?
- Introduction of the Thesis
- Literature remarks
- Methods and modes
These are the aspects involved in the master thesis writing in general. Our researchers made this as simplest for ease of your understanding. It is time to write the thesis. There are four steps involved in effective thesis writing.
What are the phases in Thesis writing?
- Identify the theme and do a literature
- Identify the research gaps in the subject
- Enrich the results and give them reasons
- Frame the main theme
So far we gave you the overall perspective on the Thesis on GIS and Remote Sensing in wide. For any queries and guidance feel free to approach us.
“Let’s put hands together with us for the enrichment of the research and project ideas and grow with us”
Similar Pages
- 275 words per page
- Free revisions1
- Topic/subject mastery
- Perfect citations
- Editorial review
- Money back guarantee2
- 1-on-1 writer chat
- 24/7 support
- A top writer
- Satisfaction guarantee
Subscribe Our Youtube Channel
You can Watch all Subjects Matlab & Simulink latest Innovative Project Results
Watch The Results
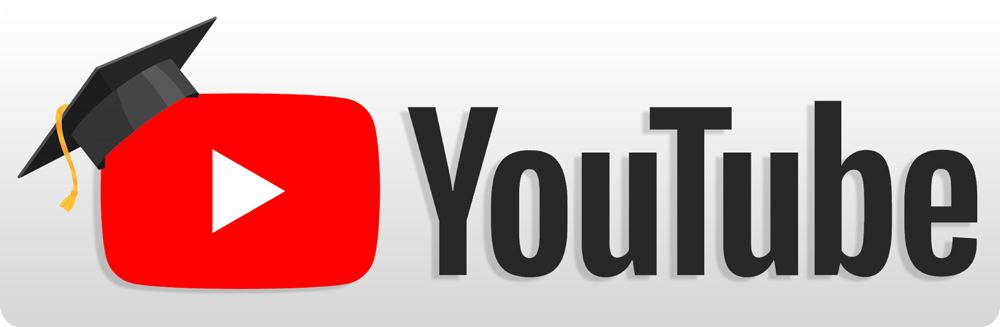
Our services
We want to support Uncompromise Matlab service for all your Requirements Our Reseachers and Technical team keep update the technology for all subjects ,We assure We Meet out Your Needs.
Our Services
- Matlab Research Paper Help
- Matlab assignment help
- Matlab Project Help
- Matlab Homework Help
- Simulink assignment help
- Simulink Project Help
- Simulink Homework Help
- NS3 Research Paper Help
- Omnet++ Research Paper Help
Our Benefits
- Customised Matlab Assignments
- Global Assignment Knowledge
- Best Assignment Writers
- Certified Matlab Trainers
- Experienced Matlab Developers
- Over 400k+ Satisfied Students
- Ontime support
- Best Price Guarantee
- Plagiarism Free Work
- Correct Citations
Expert Matlab services just 1-click
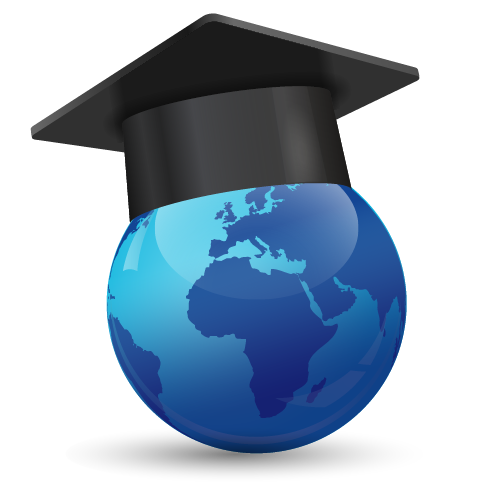
Delivery Materials
Unlimited support we offer you.
For better understanding purpose we provide following Materials for all Kind of Research & Assignment & Homework service.
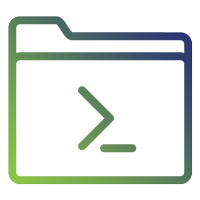
Matlab Projects
Matlab projects innovators has laid our steps in all dimension related to math works.Our concern support matlab projects for more than 10 years.Many Research scholars are benefited by our matlab projects service.We are trusted institution who supplies matlab projects for many universities and colleges.
Reasons to choose Matlab Projects .org???
Our Service are widely utilized by Research centers.More than 5000+ Projects & Thesis has been provided by us to Students & Research Scholars. All current mathworks software versions are being updated by us.
Our concern has provided the required solution for all the above mention technical problems required by clients with best Customer Support.
- Ontime Delivery
- Best Prices
- Unique Work
Simulation Projects Workflow

Embedded Projects Workflow

This Service will be usefull for
Share us your Matlab needs our technical team will get it done Ontime with Detailed Explanations .All Matlab assignments , routine matlab homeworks and Matlab academic Tasks completed at affordable prices. You get Top Grade without any Tension .Upload your Matlab requirements and see your Marks improving.Our Matlab Tutors are from US, UK, CANADA, Australia, UAE , china and India.If you need guidance in MATLAB ,assignments or Thesis and want to chat with experts or any related queries and Research issues feel free contact us.
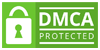
- OnTime Delivery
- Customized Works
- Plagiarism Free
- Unique works
- Detailed Explanations
- Multiple Revisions
- MATLAB Simulink
- 90, Pretham Street, Duraisamy Nagar Madurai – 625001 Tamilnadu, India
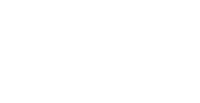
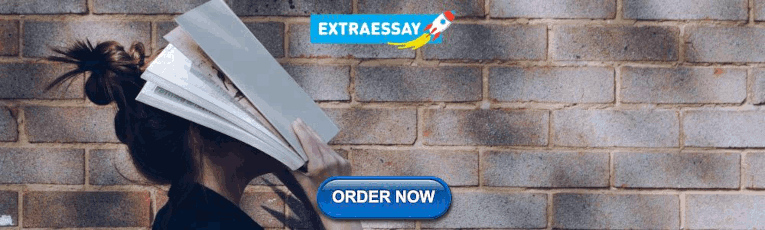
IMAGES
VIDEO
COMMENTS
The thesis has f ive chapters as Int roduction, Litera ture review, Met hodology, Work s Carried out and co nclu sio n . Chapter 1 is Introduction in which Background, Scope of GIS, Transportation ...
Sample Theses: Burgess 2013 Using imagery to identify lost gas in a natural gas gathering system. Casey 2012 Assessing impact on turkeys of forest fragmentation due to natural gas development. Jumper 2013 Social media integration for open source GIS applications. Reed 2012 Locating suitable replacement property for wildlife refuge using GIS ...
Recommendations by the American Academy of Pediatrics to prevent childhood obesity. include getting at least 1 hour of physical activity per day, limiting high sugar beverage and high. fat fast food consumption, and switching dietary habits to include low-fat dairy products, and. high fiber and calcium rich foods49.
Geovisualisation is a developing field of computing science with the fundamental approach that displaying visual representations of data assists humans in generating ideas and hypotheses about the data set (e.g., [3, 4, 9]).In the applied sciences, coupling remote sensing and GIS-based mapping are helpful for data visualisation, spatial analysis, and a better understanding of the functioning ...
D. thesis, Anna University, Madras. 29. ... 2015). The use of GIS technology can be employed not only for spatial data management, but they can also be applied to support decision ...
Suitability analysis is difficult - requiring large factors and data. Five factors (slope, soil, LULC, distance from road infrastructure and urbanization) were identified. The overall suitability map reveals that, 16.8%, 35% and 48.2% of the area is highly suitable, moderately suitable and not suitable respectively.
Amanda Suzzi 1, * and Kadambari Devaraj an 2. 1 University of Massachusetts Amherst, Dept of Environmental Conservation, , ORCID: 0000-0001-7640-0842. 2 University of Rhode Island, Department of ...
Topics for MSc Theses, GIS Unit March 2017 General Overview: Research in the GIS Unit Our group develops and utilizes methods that seek structure in spatio-temporal data, thus turning raw data into geographic information, ultimately aiming at generating knowledge that leads to a better understanding of geographic patterns and processes.
1. Introduction. Since its birth in the 1960s, GIS has played an important role in the cognitive living space and social development of human society (Goodchild 2018 ). The development of GIS is accompanied by the progress of information technology. On one hand, the development of information technology promotes the progress of GIS.
Consult the top 50 dissertations / theses for your research on the topic 'GIS and Remote Sensing.'. Next to every source in the list of references, there is an 'Add to bibliography' button. Press on it, and we will generate automatically the bibliographic reference to the chosen work in the citation style you need: APA, MLA, Harvard, Chicago ...
The M.S. GIST master's thesis gives students the opportunity to design and produce an original, independent, professional work on a compelling topic of their choice. Students work closely with a faculty advisor who serves as the thesis committee chair and two committee members from the Spatial Sciences Institute faculty to produce an abstract ...
This is a named option within the Cartographic and Geographic Information Systems M.S.. The Department of Geography offers a thesis-based master of science in Cartography and Geographic Information Systems, titled Thesis.In addition, it offers two professional non-thesis options in the Cartography and Geographic Information Systems M.S. titled: GIS Development (online program) and Accelerated ...
This thesis is an exploration of how predictive modeling and GIS could make the identification of slave wrecks plausible, and an overview of the ethical issues that surround the use of GIS within the context of the African Diaspora. Using documented non-slaving wrecks off the coast of Rhode Island, a sample workflow was generated,
Making (MCDM) technique, could be used for urban land-use planning with support of GIS technology. The aim of this thesis is preparing urban land-use planning using GIS and AHP, where the case study is Dhaka city. Dhaka, which is one of the fastest growing mega cities in the world and is the capital city of
Consult the top 50 dissertations / theses for your research on the topic 'GIS (Geographic Information System).'. Next to every source in the list of references, there is an 'Add to bibliography' button. Press on it, and we will generate automatically the bibliographic reference to the chosen work in the citation style you need: APA, MLA ...
Landslides are becoming increasingly widespread, claiming tens of thousands of fatalities, hundreds of thousands of injuries, and billions of dollars in economic losses each year. Thus, studies for geographically locating landslides, vulnerable areas have been increasingly relevant in recent decades. This research is aimed at integrating Geographical Information Systems (GIS) and Remote ...
This thesis adopts C# plug-in application development and uses a set of complete embedded GIS component libraries and tools libraries provided by GIS Engine to finish the core of plug-in GIS application framework, namely, ... GIS is a system for assembling, storing, analyzing, and displaying geographically referenced disaster. The information ...
Geo-information science thesis topics are narrowly related to the research programme of the Laboratory of Geo-informationt Science and Remote Sensing (GIRS). This research program covers a wide range of subjects. The following main themes have been selected to delineate the laboratory's identity: Sensing & measuring. Modelling & visualization.
This thesis will outline how GIS brings to EHS the advantages of traditional IT methods with the added benefit of spatial analytical operations such as map overlay, relationships and querying, and informative visual presentation through maps, floor plans, and imagery through the implementation of a GIS database for EHS called GeoSpatial ...
GIS-based landslide susceptibility mapping techniques have been used by several researchers (Aleotti and Chowdhury 1999; ... Mizan Tepi University partially funded the first author's MSc thesis research work. Author information. Authors and Affiliations. Department of Geology, College of Applied Sciences, Addis Ababa Science and Technology ...
GIS in land use planning: Lessons from critical theory and the Gulf Islands. Journal of Plannin g Education and Research Vol. 19 Issue 3, p p 287 - 296.
The study aims for applying remote sensing and GIS technology towards various spatial (soil and environmental) datasets in Dumka district of Jharkhand, India to achieve a nutrient availability and agroforestry suitability map. The Landsat satellite data, ASTER DEM and decadal annual rainfall datasets were utilized to generate wetness, landuse ...
We assist in the fields of thesis writing, conference paper writing, system development, paper publication, and so on by well-organized experts. Do you surf for research guidance or thesis writing and then this is the right place to do it. We are providing 24/7 supports with a clear understanding. Current trends on GIS and Remote Sensing
LSU Department of Geography & Anthropology 227 Howe-Russell-Kniffen Geoscience Complex Louisiana State University Phone: 225-578-5942