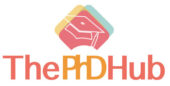
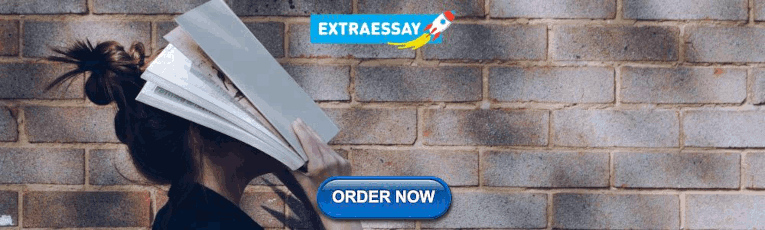
7 Must-Have Thesis Writing Tools in 2021
Writing tools like Grammarly, Mendeley online, Thesaurus, BibMe, Evernote, Plagiarism checker, Hemingway Editor, Stay Focused and Dissertation authors have significant importance in thesis writing.
Traditionally, writing a thesis for either PhD or dissertation was a tougher task, needing huge manual writing, Proofreading, correction, re-writing, and editing things manually.
So, it was tedious, time-consuming and depressive and therefore no one wants to go for a doctorate. However, the recent scenario is totally different, now we have computers, mobile phones and a lot of apps.
Things can be done at a fingertip, with one click and all done! Thanks to high-power computers and apps. “Useful” apps make tasks, work and everyday life easy for us.
We now have apps for writing, proofreading, arranging documents, taking instant and important notes, doing citation & referencing and other stuff during your research. This article explains how a PhD student can utilize apps that are useful. I will also explain how you can use it.
Features of Mendeley:
How to use mendeley , how to cite the work , features of evernote , how to use evernote , features of bibme are: , how to use bibme, how to use thesaurus , how to use dissertationauthers , features of grammarly: .
- How to use Grammarly?
Features of Hemingway Editor:
Wrapping up: , 7 must-have thesis writing tools in 2021, mendeley: .
Citing articles and enlisting references orderly isn’t an easy task; it’s time-consuming and tiresome. But what if a tool can do all this work seamlessly, effectively and precisely? Mendeley is that one tool you need.
Every PhD must have Mendeley- a reference manager tool. This app or web portal is exclusively designed to manage bibliography and citations therefore it must be there on every PhD students’ laptop.
Four amazing features of Mendeley are,
- You can store articles, PhD, journal reads on your Mendeley.
- It automatically creates a reference format.
- It can cite every bit of information seamlessly and precisely when integrated.
Meaning just read the article from Mendeley, write your content in MS word and just do insert a citation. It automatically inserts the related citation and reference at the end of the page.
Besides, several other features are,
- It automatically generates a bibliography
- You can import a pdf and research papers from other sources
- You can find the relevant articles to what you are reading
- You can collab with other researchers online
- You can access all your article libraries from anywhere by logging in to your account.
The Mendeley is developed by Elsevier Publication available on Windows, macOS and Linux.
- Go to www.mendeley.com from your computer
- Click on “create a free account”.
- Enter your email address and continue your work.
- Download the ‘Mendeley for desktop’ software
- Start adding reading materials, pdfs and research articles or create your own library.
- To use Mendeley for citation and referencing, first from your Mendeley app for tools and click on ‘Install MS word Plugin’.
- Go to MS word>> referencing and insert citation using the Mendeley.
Now you are ready to use, write literature from the article and just click on insert citation and your work is ready.
We are planning to write a whole article on the present topic, how to use it and how helpful it is, that article’s we will provide you later.
In summary, the very first software or app or tool every PhD student is required is Mendeley to write a thesis. It’s a citation and reference manager tool.
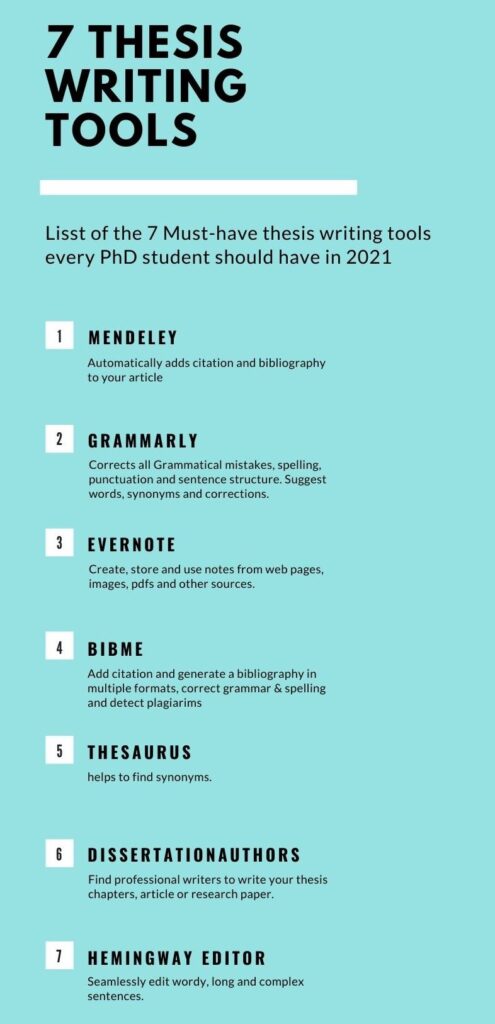
“Notes” as writing, images, pdf, article, news paragraph is an everyday part of a PhD student . Whatever you read, whenever you find things related to your PhD topic, you have to keep a note.
And trust me keeping notes isn’t so easy. If you fail to make it up, you will forget it the next morning. Evernote- is a tool that keeps your notes from desktop, mobile or any other device and syncs them.
- You can scan and save documents, label them, make important sentences underline and create pdf.
- You can even save rich notes, save images, record audio, capture ideas.
- You even can save entire or partial web pages.
- You can mark, highlight, underline, and pinpoint every note you capture.
- And guess what you can excess it from anywhere.
Go to www.evernote.com and click on sign in.
Now click on ‘create account’ and create one using your email address.
Or you can download the desktop apps.
In summary, to keep notes seamlessly and organized, the Evernote tool is required. Moreover, users can create keyboard shortcuts, note links, anchor notes to remember and share notes.
BibMe:
BibMe- The online writing center is the tool or service developed by Chegg. It has many features to improve your academic writing and PhD work.
One of the best features it has is it cites your work using different styles, even using their recent version of the style.
- You can create accurate citations in multiple styles
- Insert citation and references in your article or paper
- Improve your writing by improving sentence structure, grammar and spelling
- It can also detect plagiarism and can correct it.
Go to www.bibme.org .
Click on ‘Create account’. Create your account using your email address and use their features.
In summary, a BibMe app is an excellent tool, however, it isn’t available as a plugin for MS Word. You can use the free version or can use the paid version to explore more features.
Thesaurus:
Academic writing should be professional and precise, one needs to choose the correct word as per the sentence requirement. Using Thesaurus you can find synonyms of various words.
Besides, You can find antonyms and choose acronyms and abbreviations.
Go to www.thesaurus.com .
Click on synonyms and type your word in the search box.
In summary, Thesaurus makes your writing impressive when you use synonyms and transient words.
Disserationauthors:
Writing a research paper, review article or thesis is quite hard for a novice, you may feel helpless sometimes when your writeup is rejected multiple times. Even if you don’t understand what is wrong with your part.
If you feel so, don’t worry you can hire writers, experts or writing assistance who helps you. Dissertationauthors hire expert writers for you basically from the USA and UK.
- Go to www.dissertationauthors.com .
- Fill the order form
- Pay for your write up or paper
- Reach your personal writer
- Download your completed work
In summary, students have to write their own stuff, however, if your writing isn’t of that level you can use this service.
Grammarly:
Grammar, spelling and synonyms are common problems every research student faces, specifically those who aren’t from a pure English background. Imagine, what if some software or tool can do all stuff automatically!
Grammarly is exactly that one tool. They have free and paid plans available, however, the free version is enough for most students.
It has many services in which the Google chrome “add on” extension is the best. When activated it automatically detects and corrects English problems to all documents.
Related article: Grammarly: Your PhD writing assistant
- Correct spelling and Grammer
- Correct wordy sentences
- Suggest punctuations
- Structurize the sentence
- Check plagiarism
- Adds more transient words and synonyms
- And a lot more…
Read more: How to Check Plagiarism for PhD Thesis?- Top 10 Plagiarism Checkers
How to use Grammarly?
- Go to www.grammarly.com
- Sign in or sign up for your account.
- Go to the Google chrome extension search for Grammarly.
- Add Grammarly to your chrome extension.
- Do a quick setting as per your requirement.
In summary, Grammarly is the one tool or software that must be present on every PhD fellow’s laptop. Its technology is based on AI, meaning as you write more, it understands your style more and suggests accordingly.
Moreover, it also mails you your performances, common problems and how you can improve.
Hemingway Editor
This software is somehow similar to Grammarly, however, it has something unique in it. It’s an online editor you can use to quickly edit your article. It finds common errors, sentence structure, and grammatical mistakes.
It corrects it precisely. Several common features are enlisted here,
- Highlight lengthy and complex sentences, and corrections
- Finds common writing errors
- Finds active or passive voices.
- It denotes each error with a different color quote.
- It can also add headings, count the number of words and other things.
In summary, It’s not that impressive software for the students but can help especially to correct complex sentences. It is useful for bloggers.
Apps and Softwares make your everyday life easy and when you are in PhD, it becomes so important to make things easy. You should visit and use all this Softwares and its tools.
However, as per my opinion research candidates should have at least Grammarly, Mendeley and Evernote. These three are enough to do a lot of work.
Use Mendeley for citation, bibliography generation and other PhD stuff, Grammarly for writing assistance and Evernote for keeping notes and important things.
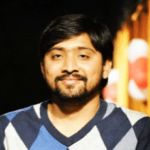
Dr. Tushar Chauhan is a Scientist, Blogger and Scientific-writer. He has completed PhD in Genetics. Dr. Chauhan is a PhD coach and tutor.
Share this:

- Share on Facebook
- Share on Twitter
- Share on Pinterest
- Share on Linkedin
- Share via Email
About The Author
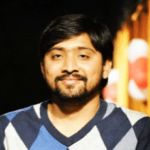
Dr Tushar Chauhan
Related posts.
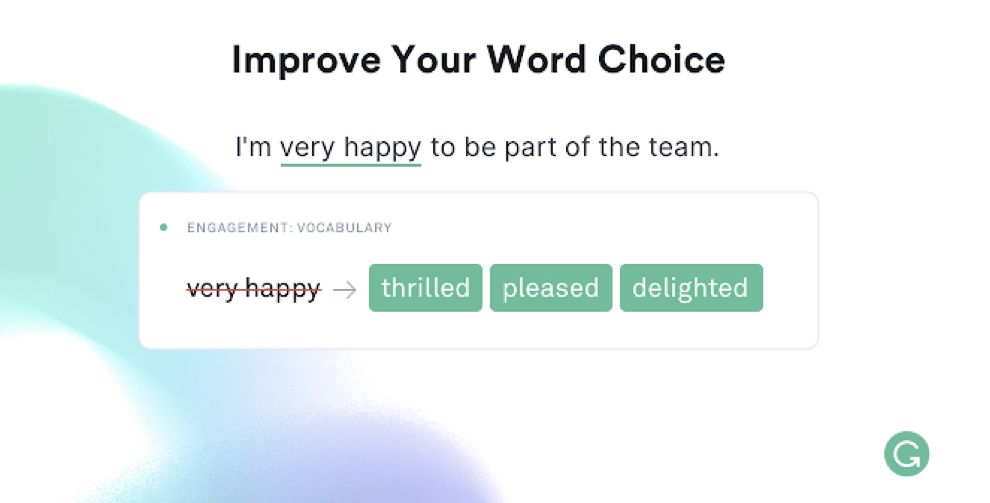
Grammarly: Your PhD writing assistant
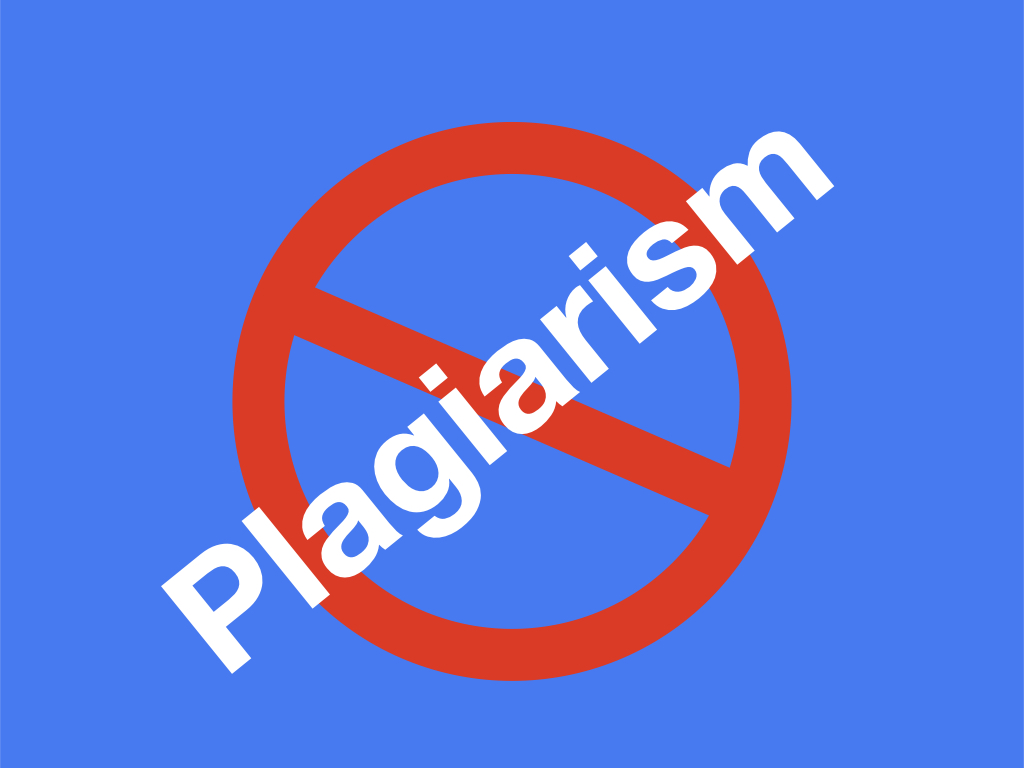
How to Avoid Plagiarism in Your PhD Thesis?
Leave a comment cancel reply.
Your email address will not be published. Required fields are marked *
Save my name, email, and website in this browser for the next time I comment.
Notify me of follow-up comments by email.
Notify me of new posts by email.
- Interesting
- Scholarships
- UGC-CARE Journals
45 Software Tools for Writing Thesis
Boost Your Thesis Writing with Essential Software Tools
Writing a thesis is a significant milestone in any academic journey. It demands an immense amount of dedication, research, and organization. To streamline your thesis writing process and ensure a well-crafted and cohesive document, it’s crucial to equip yourself with the right software tools. In this article, iLovePhD will explore some of the most important software tools you need to effectively write your thesis. These tools encompass a range of areas, including research, writing, organization, and productivity. Let’s dive in!
Discover the must-have software tools for writing a successful thesis. Streamline your research and boost productivity today!
Master Your Thesis Writing with These Essential Software Tools
1. reference management software.
Comprehensive reference management software , such as Zotero, Mendeley, or EndNote, is a must-have tool for any thesis writer. These tools help you organize and manage your references efficiently, generate citations in different formats, and create a bibliography effortlessly. They save time, eliminate the risk of citation errors, and facilitate the seamless integration of references into your thesis. Here is a list of the top ten reference management software tools:
Zotero is a free and open-source reference management tool that helps you collect, organize, and cite research sources. It has a user-friendly interface and offers features like web browser integration and collaboration options.
1.2 EndNote
EndNote is a popular reference management software used by researchers, students, and librarians. It allows you to store, organize, and format bibliographic references, and it offers a wide range of citation styles.
1.3 Mendeley
Mendeley is a reference manager and academic social network. It enables you to organize your references, collaborate with others, and discover new research articles. Mendeley offers both free and premium versions.
1.4 RefWorks
RefWorks is a web-based reference management tool that helps you store and organize your references. It offers features like citation formatting, collaboration options, and document sharing.
Citavi is a comprehensive reference management and knowledge organization tool. It allows you to manage references, create citations, and organize your research materials. Citavi is commonly used by students and researchers.
JabRef is an open-source reference manager that specializes in handling bibliographies in BibTeX format. It is cross-platform compatible and offers features like customizable citation styles and integration with LaTeX editors.
1.7 ReadCube Papers
ReadCube Papers is a reference management tool that focuses on enhancing the reading experience of research papers. It offers features like PDF annotation, article discovery, and cloud-based reference syncing.
1.8 Paperpile
Paperpile is a reference manager designed specifically for Google Docs and Google Scholar. It provides seamless integration with Google services and offers features like citation formatting and collaboration tools.
Colwiz is a reference management, collaboration, and research discovery platform. It allows you to organize your references, collaborate with colleagues, and discover new research articles.
Qiqqa is a reference management software that offers features like PDF management, annotation tools, and citation generation. It also includes advanced features like full-text search and note-taking capabilities.
These are just a few of the popular reference management software tools available. Each tool has its own unique features and capabilities, so it’s worth exploring them further to find the one that best suits your needs.
2. Word Processing Software:
While this might seem obvious, using powerful word-processing software is essential. Microsoft Word and Google Docs are popular choices due to their robust features and compatibility. These tools provide a professional writing environment, including features like spell check, grammar correction, formatting options, and word count tracking. Additionally, they offer collaborative features, making it easier to seek feedback from advisors or peers. Here are the top five word-processing software tools:
2.1 Microsoft Word
Microsoft Word is one of the most popular and widely used word-processing software tools. It offers a comprehensive range of features for creating, editing, and formatting documents. It includes a user-friendly interface, collaboration tools, templates, and advanced formatting options.
2.2 Google Docs
Google Docs is a web-based word-processing tool offered by Google. It allows users to create and edit documents online, collaborate in real-time, and easily share documents with others. Google Docs also provides cloud storage, offline access, and seamless integration with other Google services.
2.3 Apple Pages
Pages is the word processing software included in Apple’s iWork suite. It is designed specifically for macOS and iOS devices and offers a clean and intuitive interface. Apple Pages provide a variety of templates, advanced typography tools, collaboration features, and seamless integration with other Apple products.
2.3 LibreOffice Writer
LibreOffice Writer is a free and open-source word processing software that is part of the larger LibreOffice suite. It offers a range of features comparable to Microsoft Word, including extensive formatting options, styles, templates, and compatibility with various document formats.
2.4 WPS Office Writer
WPS Office Writer is a popular word-processing tool that provides a free alternative to Microsoft Word. It offers a familiar interface, supports a wide range of file formats, and includes features such as document collaboration, templates, and extensive formatting options.
Please note that the popularity and preference for word processing software can vary depending on individual needs and preferences.
3. Research Databases and Search Engines:
Conducting thorough research is a cornerstone of thesis writing. Access to reliable research databases , such as PubMed, JSTOR, or IEEE Xplore, is vital. These platforms provide access to a vast array of scholarly articles, journals, books, and conference papers. Additionally, search engines like Google Scholar and Scopus can help you discover relevant literature and enhance your research capabilities. Here are the top five research databases and search engine tools commonly used:
PubMed is a widely used research database in the field of medicine and life sciences. It provides access to a vast collection of biomedical literature, including articles from scientific journals, books, and conference proceedings.
3.2 Google Scholar
Google Scholar is a freely accessible search engine that indexes scholarly literature across various disciplines. It includes articles, theses, books, conference papers, and more. It provides a convenient way to search for academic resources from multiple sources.
3.3 IEEE Xplore
IEEE Xplore is a digital library that focuses on electrical engineering, computer science, and related disciplines. It provides access to a vast collection of technical documents, including journal articles, conference papers, standards, and ebooks.
3.4 ScienceDirec t
ScienceDirect is a leading full-text scientific database offering access to a wide range of scholarly literature in various fields. It covers subjects such as physical sciences, engineering, life sciences, health sciences, and social sciences. ScienceDirect includes journals, books, and conference proceedings.
JSTOR is a digital library that provides access to a vast collection of academic journals, books, and primary sources across multiple disciplines. It covers subjects such as arts, humanities, social sciences, and more. JSTOR is particularly useful for historical research and accessing older publications.
These tools offer comprehensive access to scholarly literature and can be valuable resources for researchers, academics, and students. However, depending on your specific field or research area, there may be other specialized databases and search engines that are more relevant to your needs.
4. Note-Taking Tools
Keeping track of ideas, concepts, and findings is crucial during the research process. Note-taking tools like Evernote, OneNote, or Notion can be immensely helpful. These tools allow you to create and organize digital notes, attach files, insert images, and even collaborate with others. The ability to access your notes across different devices ensures seamless integration with your thesis writing workflow. Here are the top five note-taking tools commonly used in research:
4.1 Microsoft OneNote
OneNote is a versatile note-taking tool that allows you to create and organize notes in a free-form manner. It offers features such as text formatting, audio and video recording, image embedding, and cross-platform synchronization.
4.2 Evernote
Evernote is a popular note-taking tool that enables users to capture, organize, and search their notes across multiple devices. It offers features like text formatting, web clipping, file attachments, and collaboration options.
Zotero is a powerful research tool specifically designed for academic and research purposes. It allows you to collect, organize, cite, and share research materials such as articles, books, web pages, and more. It also integrates with word processors for easy citation management.
Notion is an all-in-one productivity tool that can be used for note-taking, task management, project planning, and more. It provides a flexible and customizable workspace where you can create and organize notes using a combination of text, tables, lists, media, and more.
4.5 Google Keep
Google Keep is a simple and lightweight note-taking tool that integrates well with other Google services. It offers basic note-taking features, including text, voice, and image notes, as well as checklists and reminders. It also syncs across devices and allows for easy collaboration.
These software tools provide various features and functionalities, so it’s worth exploring them to find the one that best fits your specific research needs and preferences.
5. Plagiarism Checkers
Maintaining academic integrity is of utmost importance when writing a thesis. Reference checkers like Grammarly and Turnitin can help ensure proper citation usage, prevent accidental plagiarism, and improve the overall quality of your writing. These tools provide detailed reports on potential issues and suggest corrections, thereby enhancing the credibility and originality of your work. There are several plagiarism checker tools available for researchers to ensure the originality of their work. Here is a list of ten popular plagiarism checker tools used in research :
5.1 Turnitin
Turnitin is one of the most widely used plagiarism detection tools, often integrated into educational institutions’ systems. It compares submitted work against a vast database of academic content, publications, and internet sources.
5.2 Grammarly
While primarily known as a grammar and writing assistance tool, Grammarly also offers a plagiarism checker feature. It scans the text for similarities against various online sources and provides a detailed report.
5.3 Copyscape
Copyscape is an online plagiarism checker used to detect copied content. It searches the web for matching content and provides a list of URLs where the content has been found.
5.4 Plagscan
Plagscan is a comprehensive plagiarism checker that compares documents against multiple sources, including academic journals, websites, and its extensive database. It offers a detailed report highlighting potential matches.
5.5 DupliChecker
DupliChecker is a free online plagiarism checker that scans the text against various online sources and offers a percentage of similarity along with highlighted matches.
5.6 Unicheck
Unicheck is a cloud-based plagiarism detection tool specifically designed for educational institutions. It compares submitted documents against a massive database to identify potential instances of plagiarism.
5.7 Quetext
Quetext is an AI-powered plagiarism checker that scans documents for similarities against an extensive database of academic content and web sources. It provides a detailed report with highlighted matches.
5.8 Plagiarism CheckerX
Plagiarism CheckerX is a software-based plagiarism detection tool that scans documents against online sources, academic databases, and publications. It offers an easy-to-understand report highlighting similarities.
5.9 WriteCheck
WriteCheck is a plagiarism checker developed by Turnitin. It allows users to submit their work and receive an originality report, indicating potential matches found in the database.
Viper is a free plagiarism checker that scans documents for similarities against online sources. It provides a detailed report and also offers additional features for academic users.
6. Project Management and Task Tracking software Tools:
Thesis writing involves managing numerous tasks, deadlines, and milestones. Employing project management and task tracking tools like Trello, Asana, or Todoist can greatly aid in staying organized. These tools enable you to create task lists, set deadlines, allocate priorities, and collaborate with others. Visualizing your progress and breaking down the writing process into smaller, manageable tasks boosts productivity and helps you meet your targets effectively. When it comes to project management and task tracking tools for thesis writing, there are several options available. Here are five popular ones:
Trello is a highly visual project management tool that allows you to organize tasks and projects on virtual boards. You can create lists, add cards for individual tasks, set due dates, and collaborate with others. Trello’s flexibility makes it suitable for organizing research materials, outlining chapters, and tracking progress.
Asana is a comprehensive project management tool that helps you track tasks, assign responsibilities, set deadlines, and communicate with your team. It provides features such as task dependencies, subtasks, and file attachments, making it useful for managing complex thesis projects with multiple contributors.
6.3 Microsoft Project
Microsoft Project is a powerful project management software that offers robust features for planning, scheduling, and tracking projects. It allows you to create Gantt charts, set milestones, allocate resources, and monitor progress. While it may have a steeper learning curve compared to other tools, it offers extensive functionality for managing large-scale thesis projects.
6.4 Todoist
Todoist is a simple yet effective task-tracking tool that helps you create and manage to-do lists. It allows you to set due dates, prioritize tasks, and create recurring tasks. You can also collaborate with others by sharing tasks and adding comments. Todoist’s intuitive interface and cross-platform availability make it a popular choice for individual thesis writers.
6.5 Evernote
Although not strictly a project management tool, Evernote is a versatile note-taking application that can be invaluable for thesis writing. It allows you to capture and organize research notes, save web clippings, create to-do lists, and attach files. With its powerful search functionality and synchronization across devices, Evernote helps you stay organized and retrieve information quickly.
The choice of the tool ultimately depends on your specific needs and preferences. It’s a good idea to try out a few options and see which one aligns best with your workflow and project requirements.
7. Data Analysis and Visualization Tools:
For research involving data analysis, tools like SPSS, R, or Excel can be invaluable. These tools provide powerful statistical analysis capabilities, allowing you to draw meaningful insights from your data. Additionally, visualization tools like Tableau or Python’s Matplotlib can help present your findings in a visually appealing and easy-to-understand manner. There are several popular data analysis and visualization tools used in thesis writing. Here are the top five tools:
R is a widely used open-source programming language for statistical analysis and data visualization. It provides a vast number of packages and libraries specifically designed for data analysis. R allows you to perform various statistical tests, create visualizations, and generate reports, making it a popular choice among researchers.
Python is another powerful programming language commonly used for data analysis and visualization. It offers libraries like NumPy, Pandas, and Matplotlib, which provide efficient data manipulation, analysis, and plotting capabilities. Python’s versatility and ease of use make it a preferred choice for researchers in various domains.
7.3 Tableau
Tableau is a popular data visualization tool that allows users to create interactive and visually appealing charts, graphs, and dashboards. It provides a user-friendly interface for exploring and analyzing data from various sources. Tableau offers advanced features for storytelling and data presentation , making it suitable for thesis writing and research projects.
SPSS (Statistical Package for the Social Sciences) is a software package widely used in social science research. It provides a comprehensive set of tools for data analysis, including descriptive statistics, regression analysis, and hypothesis testing. SPSS also offers data visualization capabilities to present research findings effectively.
Microsoft Excel is a widely available spreadsheet program that offers basic data analysis and visualization functionalities. It is often used for organizing and manipulating data, performing simple statistical calculations, and creating basic charts. While Excel may not be as powerful as specialized data analysis tools, it remains a popular choice due to its familiarity and ease of use.
These tools provide researchers with a range of options for data analysis and visualization, allowing them to choose the one that best suits their needs and preferences.
Conclusion:
Writing a thesis can be a challenging yet rewarding experience. By equipping yourself with the right tools, you can streamline your workflow, enhance your research capabilities, and produce a well-structured thesis. Remember, the tools mentioned in this article are meant to assist and optimize your writing process, but it’s crucial to adapt them to your specific needs and preferences. Embrace these tools, and let them empower you to create a compelling thesis that showcases your academic prowess. Happy writing!
Various Software Tools for Writing Thesis:
Writing Softwares for Thesis
Research Tools for Thesis Writing
Citation Management Tools for Thesis
Plagiarism Checkers for Thesis Writing
Grammar and Proofreading Tools for Thesis
Formatting Tools for Thesis Writing
Reference Management Tools for Thesis
Data Analysis Tools for Thesis Writing
Literature Review Tools for Thesis
Time Management Tools for Thesis
Collaboration Tools for Thesis Writing
Presentation Tools for Thesis Defense
Thesis Writing Templates and Samples
Tips and Techniques for Thesis Writing
Common Challenges in Thesis Writing
Thesis Writing Resources and Guides
FAQ on Tools for Writing Thesis
- ai thesis generator
- analytical thesis statement generator
- argumentative thesis statement generator
- best paraphrasing tool for thesis
- best paraphrasing tool for thesis free
- paraphrasing tool for thesis
- paraphrasing tool for thesis writing
- persuasive thesis statement generator
- strong thesis statement generator
- thesis outline generator
- thesis paraphrasing tool
- thesis question generator
- thesis sentence generator
- thesis statement finder tool
- thesis statement generator
- thesis statement generator for essay
- thesis statement generator for research paper
- thesis statement tool
- thesis writing software
- thesis writing software free download
Anna’s Archive – Download Research Papers for Free
Fulbright-kalam climate fellowship: fostering us-india collaboration, fulbright specialist program 2024-25, most popular, iitm & birmingham – joint master program, six effective tips to identify research gap, 24 best free plagiarism checkers in 2024, how to check scopus indexed journals 2024, types of research variable in research with example, what is hypothesis in research types, examples, & importance, how to write a research paper a complete guide, best for you, what is phd, popular posts, 480 ugc-care list of journals – science – 2024, popular category.
- POSTDOC 317
- Interesting 259
- Journals 234
- Fellowship 128
- Research Methodology 102
- All Scopus Indexed Journals 92
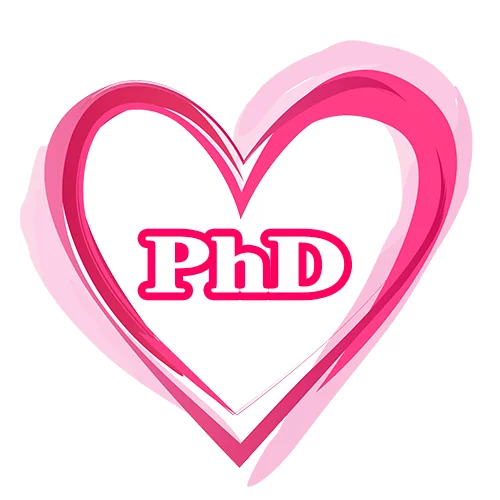
iLovePhD is a research education website to know updated research-related information. It helps researchers to find top journals for publishing research articles and get an easy manual for research tools. The main aim of this website is to help Ph.D. scholars who are working in various domains to get more valuable ideas to carry out their research. Learn the current groundbreaking research activities around the world, love the process of getting a Ph.D.
Contact us: [email protected]
Google News
Copyright © 2024 iLovePhD. All rights reserved
- Artificial intelligence

- Resources Research Proposals --> Industrial Updates Webinar - Research Meet
- Countries-Served
- Add-on-services
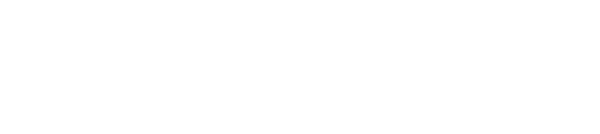
Text particle
feel free to change the value of the variable "message"
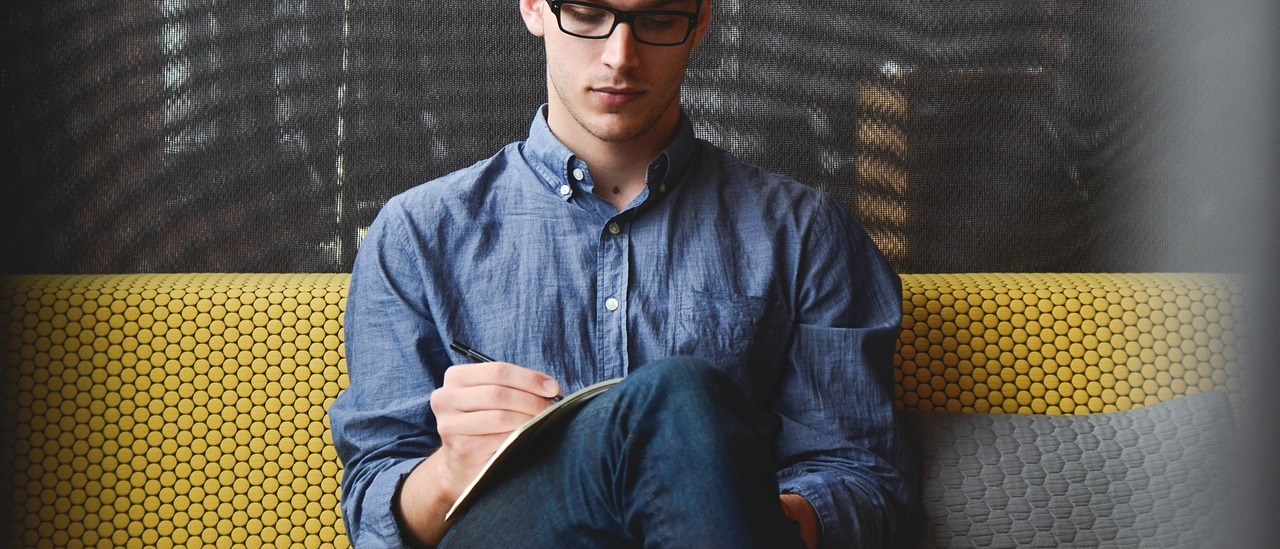
Top 11 Software Tools for Thesis Writing: Boost Your Research
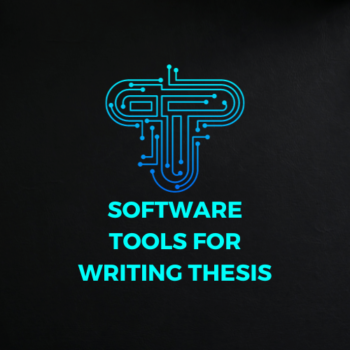
Crafting a thesis may seem challenging, but employing suitable software tools transforms it into a more structured and approachable undertaking. Delve into these potent resources to simplify your research, elevate your writing, and excel in your academic pursuits. This post elaborates on several tools commonly utilized for academic thesis writing.
Citavi is a comprehensive reference management and knowledge organization tool. It allows you to manage references, create citations, and organize your research materials. Citavi is commonly used by students and researchers.
Docear is a unique solution to academic literature management, i.e., it helps you organizing, creating, and discovering academic literature. This unique literature management tool designed specifically for academics when it comes to discovering, organizing and ultimately creating academic literature. A recommender system that helps you to discover new literature: Docear recommends papers which are free, in full-text, instantly to download, and tailored to your information needs.
3. Paperpile
Paperpile is a software for reference management that is just like Zotero and works like an extension for the Google Chrome browser, making it accessible for Linux, Mac, and Windows users. We suggest that you use it to discover and import information from stage platforms such as PubMed, arXiv, and Google Chrome. You can undoubtedly trade all the PDF archives and information to Google Drive, which implies you will have the chance to edit your papers collaboratively. This collaboration will not end, as you can send data back and forth between P Zotero and Paperpile, such as Mendeley.
4. RefWorks
RefWorks is accurate; select from thousands of customizable citation styles to use within authoring tools. Generate bibliographies and citations in a snap with any authoring tool. Use our integrations to insert citations in Word and Google Docs. Leverage thousands of pre-built citation styles and request new styles. Customize citation styles or create your own from scratch with the citation style editor.
5. SpellCheckPlus
SpellCheckPlus applies the same concept to the writing process. Rather than simply correcting your mistakes, it summarizes them into distinct categories. SpellCheckPlus offers feedback that not only improves your current project but also your writing and grammar at large. In other words, SpellCheckPlus is no mere editing tool; rather, it’s a teaching tool. And as even the very best writers know, there’s always room for improvement when it comes to how you deliver your message.
MindMup acts as an online mind-mapping canvas, perfect for representing ideas and concepts visually. Capture ideas at the speed of thought using a mind map maker designed to help you focus on your ideas and remove all the distractions while mindmapping. MindMup is also a terrific collaborative tool.
7. iA Writer
iA Writer gives you the essence of pure writing by providing an unobtrusive interface that lets you concentrate on your text. Despite its minimalistic approach, the application offers a variety of distinctive features that are specifically designed for writing-focused tasks. With iA Writer, you can gain unparalleled insight into your writing structure, detect superfluous words and clichés, and navigate through your documents and notes with ease.
Elicit uses language models to extract data from and summarize research papers. As a new technology, language models sometimes make up inaccurate answers (called hallucinations). They reduce hallucinations and increase accuracy by: training our models on specific tasks; searching over academic papers; and making it easy to double-check answers. Elicit only shows you papers that actually exist and are part of the scientific literature.
9. Lucidchart
It is an online diagramming tool to create flowcharts, diagrams, and visuals. Lucidchart is an intelligent diagramming application that brings teams together to make better decisions and build the future. Lucidchart helps users sketch and share professional flowchart diagrams, providing designs for anything from brainstorming to project management.
10. Trinka AI
Trinka is an online grammar checker and language correction AI tool for academic and technical writing. Trinka finds difficult errors unique to academic writing that other grammar checker tools don’t. From advanced English grammar errors to scientific tone and style, Trinka checks it all! Trinka goes beyond professional grammar checks and enhances your writing for vocabulary, tone, syntax, and much more. Make your point confidently with Trinka.
11. WhiteSmoke
WhiteSmoke renders you with the highest quality proofreading abilities available, correcting not only simple spelling mistakes like old-fashioned word processors but everything from grammar, word choices, and even style mistakes, all without breaking a sweat.
https://www.ref-n-write.com/blog/academic-writing-tools-and-research-software-a-comprehensive-guide/
Leave a Reply Cancel reply
Your email address will not be published. Required fields are marked *
Save my name, email, and website in this browser for the next time I comment.

25+ Dissertation & Thesis Writing Apps
Everything You Need To Optimise Your Research Journey
Contributors: Derek J ansen (MBA), Kerryn Warren (PhD) & David Phair (PhD) | May 2024
Completing your dissertation or thesis requires a hearty investment of time, effort and hard work. There’s no shortcut on the road to research success, but as with anything, there are ways to optimise the process and work smarter.
Here, we’ll share with you a wide range of apps, software and services that will make your life a little easier throughout the research process. While these apps can save you a lot of time, remember that your dissertation or thesis needs to be your own work – no tool should be doing the writing on your behalf. Also, be sure to check your university’s policy regarding AI-based tools and support before using any apps, tools or software.
Overview: Dissertation & Thesis Apps
- Literature review
- Data collection
- Qualitative data analysis
- Quantitative data analysis
- Writing & Plagiarism
- Project management
- Reference management
- Honourable mentions
Literature Review & Search Apps
The following apps and tools can help you discover, analyse, and synthesise scholarly materials, significantly streamlining the literature review process.
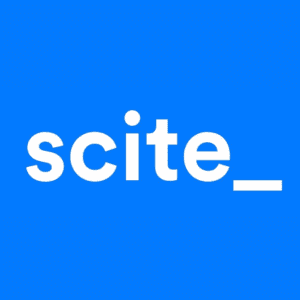
Scite AI – Find & evaluate sources
The Scite AI app uses ‘Smart Citations’ to show how academic papers are discussed—i.e., supported, contradicted, or mentioned. This can help you fast-track the literature review process and source highly relevant papers quickly.
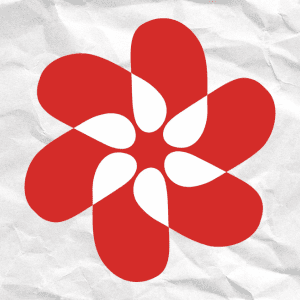
Petal – Chat with your literature
Petal is an AI-driven tool that revolutionises your interaction with documents by enabling direct, context-aware conversations. Upload an article and it will swiftly summarise its contents and allow you to ask questions about the specific study (or studies).
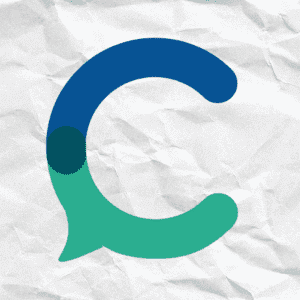
Consensus – ChatGPT for academia
The Consensus app uses a powerful AI engine to connect you to over 200 million scientific papers. It allows precise searches without exact keywords and efficiently summarises key research findings , making your literature review much more manageable.
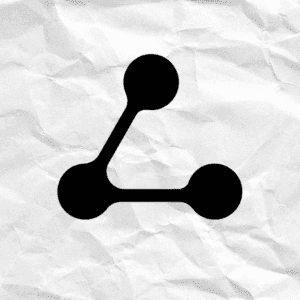
Litmaps – Visualise related papers
This app is great for quickly identifying relevant research. For any given keyword or resource, it will provide you with a visual citation network, showing how studies are interconnected. This reveals both direct and tangential connections to other research, highlighting gaps and key discussions within your field.
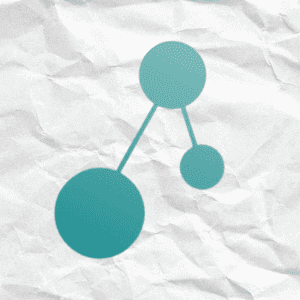
Connected Papers – An alternative
Similar to Litmaps, Connected Papers visually maps academic research, simplifying how you explore related studies. Just input a paper, and it charts connections, helping identify key literature and gaps. It’s ideal for staying updated on emerging research.
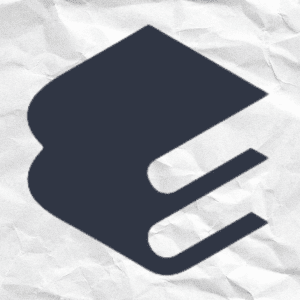
Elicit – An “AI research assistant”
Another AI-powered tool, Elicit automates the discovery, screening, and data extraction from academic papers efficiently. This makes it useful for systematic reviews and meta-analyses, as it allows you to focus on deeper analysis across various fields.
Data Collection & Preparation
These apps and tools can assist you in terms of collecting and organising both qualitative and quantitative data for your dissertation or thesis.
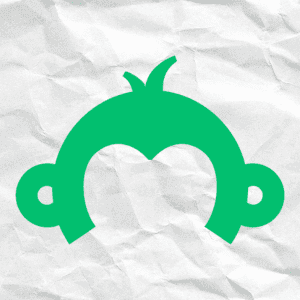
SurveyMonkey – Simple surveys
SurveyMonkey is a versatile tool for creating and distributing surveys. It simplifies collecting and analysing data, helping you craft surveys that generate reliable results. Well suited for the vast majority of postgraduate research projects.
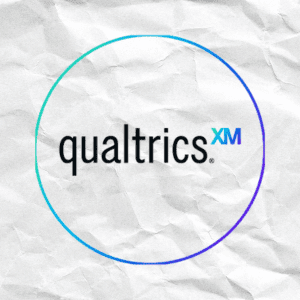
Qualtrics – An alternative
Qualtrics is a comprehensive survey tool with advanced creation, distribution, and analysis capabilities. It supports complex survey designs and robust data analysis, making it ideal for gathering detailed insights and conducting high-quality research.
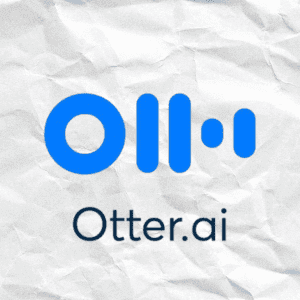
Otter – Easy draft transcription
Otter is an AI-powered transcription tool that converts spoken words into text. It captures and transcribes lectures, interviews, and meetings in real time. Naturally, it’s not 100% accurate (you’ll need to verify), but it can certainly save you some time.
Qualitative Data Analysis
These software packages can help you organise and analyse qualitative data for your dissertation, thesis or research project.
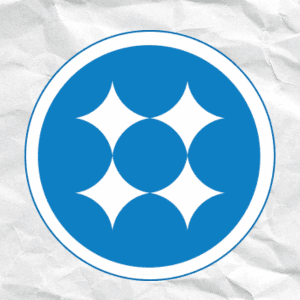
NVivo – All-in-one qual platform
NVivo is a powerful qualitative data analysis software that facilitates data organisation, coding, and analysis. It supports a wide range of data types and methodologies, enabling detailed analysis and helping you extract rich insights from your data.
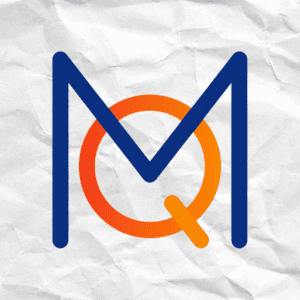
MaxQDA – QDA simplified
MAXQDA is a robust qualitative data analysis software that helps you systematically organise, evaluate, and interpret complex datasets. A little easier to get started with than NVivo, it’s ideal for first-time dissertation and thesis writers.
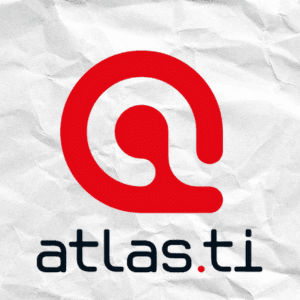
ATLAS.ti – For large datasets
ATLAS.ti offers robust tools for organising, coding, and examining diverse materials such as text, graphics, and multimedia. It’s well-suited for researchers aiming to weave detailed, data-driven narratives as it streamlines complex analysis tasks efficiently.
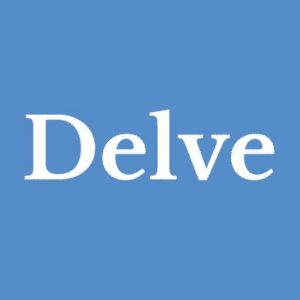
Delve – An intuitive interface
Delve is an intuitive qualitative data analysis tool designed to streamline the qualitative analysis process. Ideal for dissertations, Delve simplifies the process from initial data organisation to in-depth analysis, helping you efficiently manage and interpret complex datasets for clearer insights.
Quantitative (Statistical) Data Analysis
These software packages can help you organise and analyse quantitative (statistical) data for your dissertation, thesis or research project.
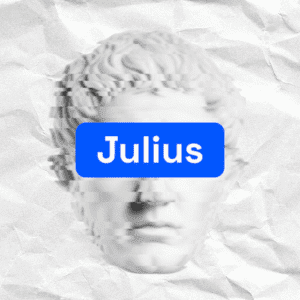
Julius – Your “AI data analyst”
Julius is an AI-powered data analysis tool that simplifies the process of analysing and visualising data for academic research. It allows you to “chat” with your data, create graphs, build forecasting models, and generate comprehensive analyses.
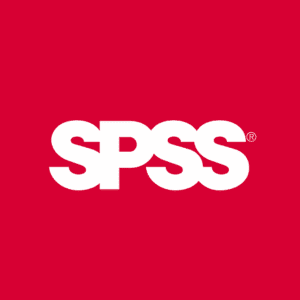
IBM SPSS – The “old faithful”
The OG of statistical analysis software, SPSS is ideal for students handling quantitative data in their dissertations and theses. It simplifies complex statistical testing, data management, and graphical representation, helping you derive robust insights.
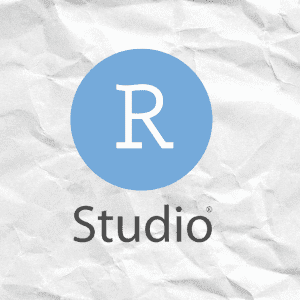
R Studio – For the data wizards
While admittedly a little intimidating at first, R is a versatile software for statistical computing. It’s well-suited for quantitative dissertations and theses, offering a wide range of packages and robust community support to streamline your work.
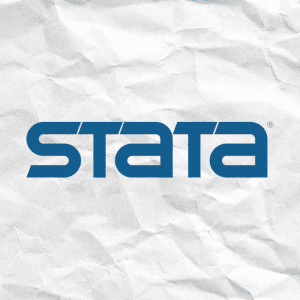
STATA – For the data scientists
Stata is yet another comprehensive statistical software widely used for data management, statistical analysis, and graphical representation. It can efficiently handle large datasets and perform advanced statistical analyses.
Writing Improvement & Plagiarism Tools
These apps and tools can help enhance your writing and proactively identify potential plagiarism issues.
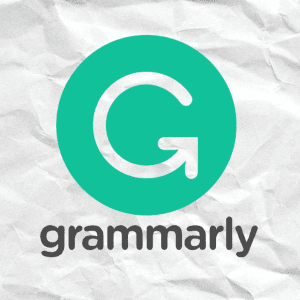
Grammarly – Improve your writing
Grammarly is a writing assistant that can help enhance academic writing by checking for errors in grammar, spelling, and punctuation in real time. It also features a plagiarism detection system , helping you to proactively avoid academic misconduct.
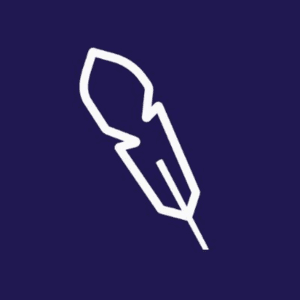
Jenni – An AI “writing assistant”
Jenni AI helps you draft, cite, and edit with ease, streamlining the writing process and tackling writer’s block. Well suited for ESL students and researchers, Jenni helps ensure that your work is both precise, clear and grammatically sound.
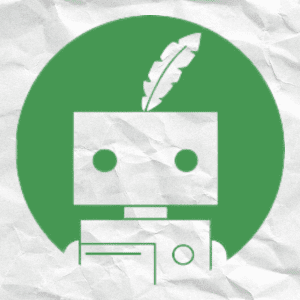
Quillbot – Paraphrasing simplified
Quillbot is yet another AI-powered writing tool that can help streamline the writing process. Specifically, it can assist with paraphrasing , correcting grammar, and improving clarity and flow. It also features a citation generator and plagiarism checker .
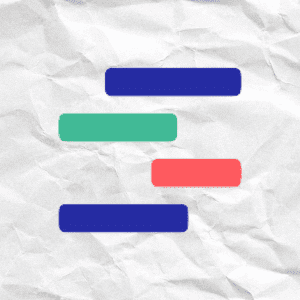
Quetext – Solid plagiarism checking
Quetext is a plagiarism detection tool that helps ensure the originality of your academic work. It cross-references your documents against extensive online databases to highlight potential plagiarism and generate detailed reports.
Project & Time Management
These apps can help you plan your research project and manage your time, so that you can work as efficiently as possible.
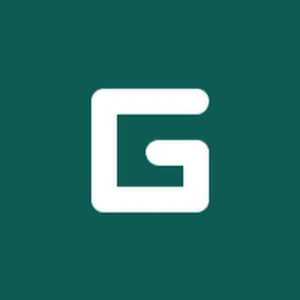
GanttPro – PM simplified
An intuitive project management tool, GanttPro simplifies planning and tracking for dissertations or theses. It offers detailed Gantt charts to visualise task timelines, dependencies, and progress, helping you ensure timely completion of each section.
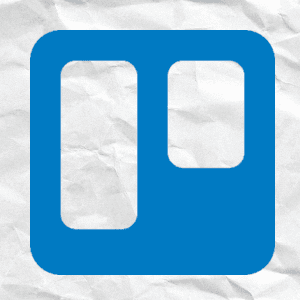
Trello – Drag-and-drop PM
Trello is a versatile project management tool that helps you organise your dissertation or thesis process effectively. By creating boards for each chapter or section, you can track progress, set deadlines, and coordinate tasks efficiently.
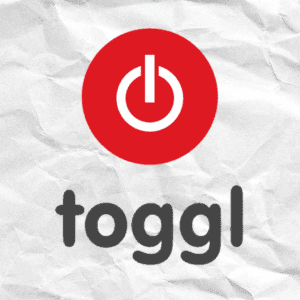
Toggl – Make every minute count
A user-friendly time-tracking app that helps you manage your research project effectively. With Toggl, you can precisely track how much time you spend on specific tasks. This will help you avoid distractions and stay on track throughout your journey.
Reference Management
These apps and tools will help you keep your academic resources well organised and ensure that your citations and references are perfectly formatted, every time.
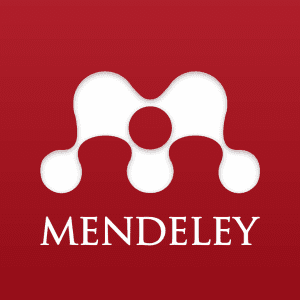
Mendeley – Your citations, sorted
Mendeley is your go-to reference management tool that simplifies academic writing by keeping your sources neatly organised. Perfect for dissertations and theses, it lets you easily store, search, and cite your resources directly in MS Word.
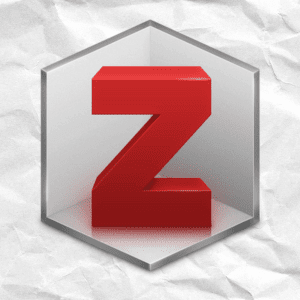
Zotero – Great for Google Docs
Zotero is a free-to-use reference manager that ensures your sources are well-organised and flawlessly cited. It helps you collect, organise, and cite your research sources seamlessly. A great alternative to Mendeley if you’re using Google Docs.
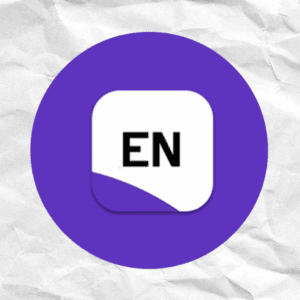
Endnote – A paid option
Yet another reference management option, Endnote is sometimes specifically required by universities. It efficiently organises and stores research materials, making citation and bibliography creation (largely) effortless.
Honourable Mentions
Now that we’ve covered the more “exciting” dissertation apps and tools, it’s worth quickly making one or two mundane but essential mentions before we wrap up.
You’ll need a reliable word processor.
In terms of word processors, Microsoft Word will likely be your go-to, but it’s not the only option. If you don’t have a license for Word, you can certainly consider using Google Docs, which is completely free. Zotero offers a direct integration with Google Docs, making it easy to manage your citations and references. If you want to go to the other extreme, you can consider LaTeX, a professional typesetting software often used in academic documents.
You’ll need cloud storage.
The number of times we’ve seen students lose hours, days or even weeks’ worth of hard work (and even miss the submission deadline) due to corrupted flash drives or hard drives, coffee-soaked laptops, or stolen computers is truly saddening. If you’re not using cloud storage to save your work, you’re running a major risk. Go sign up for any of the following cloud services (most offer a free version) and save your work there:
- Google Drive
- iCloud Drive
Not only will this ensure your work is always safely stored (remember to hit the Save button, though!), but it will make working on multiple devices easier, as your files will be automatically synchronised. No need to have multiple versions between your desktop, laptop, tablet, etc. Everything stays in one place. Safe, secure, happy files.
Need a helping hand?
Key Takeaways: Dissertation & Thesis Apps
And there you have it – a hearty selection of apps, software and services that will undoubtedly make your life easier come dissertation time.
To recap, we’ve covered tools across a range of categories:
Remember, while these apps can help optimise your dissertation or thesis writing journey, you still need to put in the work . Be sure to carefully review your university’s rules and regulations regarding what apps and tools you can use – especially anything AI-related.
Have a suggestion? We’d love to hear your thoughts. Simply leave a comment below and we’ll consider adding your suggested app to the list.
You Might Also Like:
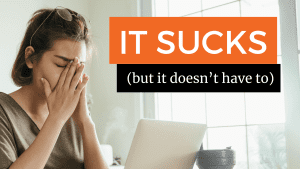
14 Comments
It seems some of the apps mentioned are not android capable. It would be nice if you mentioned items that everyone could use.
Thanks for the feedback, Gale!
Yet to explore some of your recommended apps. I am glad to commend on one app that I have started using, Mendeley. When it comes to referencing it really helps a lot.
Great to hear that, Maggie 🙂
I have got Mendeley and it is fantastic. I have equally downloaded Freemind but I am yet to really understand how to navigate through it.
Based on your YouTube lessons,my literature review and the entire research has been simplified and I am enjoying the flow now,more than ever before.
Thank you so much for your recommendations and guide.It’s working a great deal for me.
Remain blessed!
Thank you for all the amazing help and tutorials. I am in the dissertation research proposal stage having already defended the qualifying paper. I am going to implement some of your advice as I revise chapters 1 & 2 and expand chapter 3 for my research proposal. My question is about the writing– or specifically which software would you recommend. I know MS Word can get glitchy with larger documents. Do the “reference” apps you recommend work with other options such as LaTeX? I understand that for some programs the citations won’t be integrated or automated such as cite while you write etc.. I have a paid version of endnote, and free versions of mendeley and zotero. I have really only used endnote with any fidelity but I’m willing to adapt. What would you advise at this point?
Thanks for your comment and for the kind words – glad to hear that the info is useful.
Generally, Word works reasonably well for most research projects and is our first recommendation. As long as one keeps the document clean (i.e. doesn’t paste in loads of different styles, ultra high-res images, etc), it works fine. But I understand that it’s not perfect for absolutely huge projects.
Regarding referencing with Latex, this article covers how to use Mendeley with Latex – https://blog.mendeley.com/2011/10/25/howto-use-mendeley-to-create-citations-using-latex-and-bibtex/ . Perhaps it will be useful. I hesitate to tell you to adopt software X or Y, or to move from Endnote, as each software has its strengths and weaknesses, and performs better in certain contexts. I’m not familiar with your context, so it’s not possible for me to advise responsibly. Mendeley works well for the vast majority of our students, but if there’s a very specific bit of functionality that draws you to Endnote, then it may be best to stick with it. My generic advice would be to give Mendeley a try with some sample references and see if it has the functionality you need.
I hope this helps! Best of luck with your research 🙂
Thank you for your amazing articles and tips. I have MAC laptop, so would Zotero be as good as Mendeley? Thank you
Derek would you share with me your email please, I need to talk to you urgently.
I need a free Turnitin tool for checking plagiarism as for the tools above
I have been going crazy trying to keep my work polished and thesis or dissertation friendly. My mind said there had to be a better way to do literature reviews. Thank God for these applications. Look out world I am on my way.
Wow Thanks for this write-up i find it hard to track down extremely good guidance out there when it comes to this material appreciate for the publish site
Submit a Comment Cancel reply
Your email address will not be published. Required fields are marked *
Save my name, email, and website in this browser for the next time I comment.
- Print Friendly
Offered MSc Thesis topics
See also our current list of projects on the Research page to get an idea of what is topical in our research. Another list of all our projects is also available in Tuhat, with responsible persons listed (you can ask them about potential thesis topics).
A more exhaustive list of topics from the department is available at CSM Master thesis topics (moodle).
General writing Instructions
We have written some instructions to help the students write their Master's theses, seminar papers and B.Sc. theses. Please, read the guide before starting your thesis work: Scientific Writing – Guide of the Empirical Software Engineering Research Group .
Master's Thesis Topics
Software engineering and technology are prevalent areas for thesis at the department, and many candidates ask for thesis topics every academic year. We do our best to accommodate the requests, but the applicants can smoothen the process by taking an active role in thinking about potential topics based on the themes presented below.
We provide guidance for selecting a suitable topic and the supervision and support needed to complete the work. Please contact Antti-Pekka Tuovinen or Tomi Männistö if you are interested. You can also contact the group members to ask about the subject areas they are working on.
Suppose you, as a student, are working in software development, processes, architecture or something related. In that case, there is a good chance of finding an interesting thesis topic that closely relates to your work. In such a case, the actual work often provides an excellent problem to investigate, propose or try out potential solutions for, or the case can act as a rich source of data about the practice of software development.
We also welcome companies to suggest potential topics for Master's thesis. The topics can be general, based on existing research, or they may require original research and problem-solving. We will help to evaluate and fine-tune the proposals. Depending on the topic, you may also need to be prepared to provide guidance and assistance during the thesis project.
Please contact Antti-Pekka Tuovinen or Tomi Männistö if you have an idea for an industrial thesis and need further information.
The listing below introduces our current research areas and potential topics for the thesis. Each topic has a short description and the names of the researchers working on the topic. Please contact them for more details about the research and thesis work. Note that you can also suggest and discuss other topics within the general area of software engineering research. We encourage creativity and student-centred insight in selecting and defining the topic.
Earlier theses
Some earlier MSc thesis titles below give some idea about the topics. You can try looking up more info from E-thesis , but note that it is up to the author if the actual thesis pdf is available online. Just search using the title (or part of it) in quotation marks. You can also go to the library in person and read all theses (even those without a public pdf) on a kiosk workstation (ask the staff if you need help).
- Exploring study paths and study success in undergraduate Computer Science studies
- EU:n tietosuoja-asetuksen GDPR:n vaikutus suomalaisissa pk-yrityksissä 2018-2020
- Industrial Surveys on Software Testing Practices: A Literature Review
- Laskennallisesti raskaan simulointiohjelmistokomponentin korvaaminen reaaliaikasovelluksessa koneoppimismenetelmällä
- Web service monitoring tool development
- Case study: identifying developer oriented features and capabilities of API developer portals
- Documenting software architecture design decisions in continuous software development – a multivocal literature review
- Elinikäinen oppiminen ohjelmistotuotannon ammattilaisen keskeisenä
- Miten huoltovarmuus toteutuu Ylen verkkouutisissa?
- Utilizing Clustering to Create New Industrial Classifications of Finnish Businesses: Design Science Approach
- Smoke Testing Display Viewer 5
- Modernizing usability and development with microservices
- On the affect of psychological safety, team leader’s behaviour and team’s gender diversity on software team performance: A literature review
- Lean software development and remote working during COVID-19 - a case study
- Julkaisusyklin tihentämisen odotukset, haasteet ja ratkaisut
- Software Development in the Fintech Industry: A Literature Review
- Design of an automated pipeline to improve the process of cross-platform mobile building and deployment
- Haasteet toimijamallin käytössä ohjelmistokehityksessä, systemaattinen kirjallisuuskatsaus
- Light-weight method for detecting API breakages in microservice architectures
- Kirjallisuuskatsaus ja tapaustutkimus API-hallinnasta mikropalveluarkkitehtuurissa
- In-depth comparison of BDD testing frameworks for Java
- Itseohjautuvan auton moraalikoneen kehittämisen haasteet
- Towards secure software development at Neste - a case study
- Etuuspohjaisen eläkejärjestelyn laskennan optimointi vakuutustenhallintajärjestelmässä
- Internal software startup within a university – producing industry-ready graduates
- Applying global software development approaches to building high-performing software teams
- Systemaattinen kirjallisuuskatsaus lääkinnällisistä ohjelmistoista ja ketterästä ohjelmistokehityksestä
- Matalan kynnyksen ohjelmointialustan hyödyntäminen projektinhalinnassa
- Uncertainty Estimation with Calibrated Confidence Scores
- Tool for grouping test log failures using string similarity algorithms
- Design, Implementation, and Validation of a Uniform Control Interface for Drawing Robots with ROS2
- Assuring Model Documentation in Continuous Machine Learning System Development
- Verkkopalvelun saavutettavuuden arviointi ja kehittäminen ohjelmistotuoteyrityksessä
- Methods for API Governance automation: managing interfaces in a microservice system
- Improving Web Performance by Optimizing Cascading Style Sheets (CSS): Literature Review and Empirical Findings
- Implementing continuous delivery for legacy software
- Using ISO/IEC 29110 to Improve Software Testing in an Agile VSE
- An Open-Source and Portable MLOps Pipeline for Continuous Training and Continuous Deployment
- System-level testing with microservice architecture
- Green in software engineering: tools, methods and practices for reducing the environmental impacts of software use – a literature review
- Machine Learning Monitoring and Maintenance: A Multivocal Literature Review
- Green in Software Engineering: A Systematic Literature Review
- Comparison of Two Open Source Feature Stores for Explainable Machine Learning
- Open-source tools for automatic generation of game content
- Verkkosovelluskehysten energiankulutus: vertaileva tutkimus Blazor WebAssembly ja JavaScript
- Infrastruktuuri koodina -toimintatavan tehostaminen
- Geospatial DBSCAN Hyperparameter Optimization with a Novel Genetic Algorithm Method
- Hybrid mobile development using Ionic framework
- Correlation of Unit Test Code Coverage with Software Quality
- Factors affecting productivity of software development teams and individual developers: A systematic literature review
- Case study: Performance of JavaScript on server side
- Reducing complexity of microservices with API-Saga
- Organizing software architecture work in a multi-team, multi-project, agile environment
- Cloud-based visual programming BIM design workflow
- IT SIAM toimintojen kehitysprojekti
- PhyloStreamer: A cloud focused application for integrating phylogenetic command-line tools into graphical interfaces
- Evaluation of WebView Rendering Performance in the Context of React Native
- A Thematic Review of Preventing Bias in Iterative AI Software Development
- Adopting Machine Learning Pipeline in Existing Environment
Current topic areas of interest to the research group (see below for the details)
Open source-related topic areas in collaboration with daimler truck.
- Open Chain: Developing the Journey to Open Chain Compliance at the example of Daimler Truck
- How should an industrial company (for example, Daimler Truck) leverage open source software: Building a framework with different dimensions, from efficient governance to value in inner source and open source projects
- How can an organization efficiently incentivize inner-source activities? (on different levels, culture, infrastructure, governance, regulations & commitments.)
- How can an industrial organization leverage value from actively engaging in FOSS activities (especially on active creation and contribution)
- How can spillovers help Industrial companies to educate the rare resources but also attract and retain talent? Ref: Gandal, N., Naftaliev, P., & Stettner, U. (2017). Following the code: spillovers and knowledge transfer. Review of Network Economics , 16 (3), 243-267. Abstract: Knowledge spillovers in Open Source Software (OSS) can occur via two channels: In the first channel, programmers take knowledge and experience gained from one OSS project they work on and employ it in another OSS project they work on. In the second channel, programmers reuse software code by taking code from an OSS project and employing it in another. We develop a methodology to measure software reuse in a large OSS network at the micro level and show that projects that reuse code from other projects have higher success. We also demonstrate knowledge spillovers from projects connected via common programmers.
If interested, contact Tomi Männistö for further information
Hybrid software development (TOPIC AREA)
The current pandemic has brought many, even radical, changes to almost all software companies and software development organizations. Especially the sudden moves to working from home (WFH) in March 2020 forced them to adapt and even rethink many software engineering practices in order to continue productive software development under the new constraints.
Now (December 2021), various hybrid ways of working appear to become the new "normal" for the software industry in general. For instance, many companies are offering flexible workplace arrangements (WFX).
This thesis theme aims to explore and possibly explain such changes in contemporary software engineering. Potential research questions include the following:
- How has the COVID-19 pandemic affected different software engineering activities (negatively or positively)? What are the mechanisms?
- What adaptations and countermeasures have different software organizations devised to cope with the challenges?
- What could be learned from them for future hybrid software development processes, practices and tools?
Contact: Petri Kettunen
MLOps -- as a derivative of DevOps -- is about practice and tools for ML-based systems that technically enable iterative software engineering practice. We have several funded positions in the area of MLOps in our research projects (IMLE4 https://itea4.org/project/iml4e.html and AIGA https://ai-governance.eu/ ) that can be tailored to the interest of the applicant. For details, contact Mikko Raatikainen ( [email protected] ).
Digital Twin of Yourself
Digital twins are virtual world dynamic models of real-world physical objects. They originate from manufacturing domains. In such environments, they are utilized, for example, for predictive maintenance of equipment based on real-time machine data.
Recently the application domains of digital twins have broadened to cover living objects – especially human beings, for instance, in medical domains (so-called Human Digital Twins). In this thesis topic, the objective is to design a digital twin of yourself. The choice of the digital twin dynamic model is free, and so are the data inputs. One possibility could be, for instance, your real-life physical exercise data (e.g., from a heart-rate monitor). You could also consider your Citizen Digital Twin, following your study data and yourself as a lifelong learner.
Software engineering and climate change (TOPIC AREA)
Global climate change may have various impacts on future software engineering on the one hand, and software engineering may affect climate change directly or indirectly, positively or negatively on the other hand. All that opens up many potentially important research problems. Specific theses in this topic area could be, for instance, the following themes:
- Green IT (e.g., engineering new software with energy-efficiency requirements in order to reduce or limit power consumption and consequently the carbon footprint)
- Carbon neutrality goals of software companies (e.g., software development organizations decreasing physical travelling in order to reduce their greenhouse gas emissions)
- Developing software products or services for measuring climate change-related factors
The thesis could be a literature review, an empirical case study or a scientific design work.
Life-long learning for the modern software engineering profession
Specific intended learning outcomes for computer science (software engineering) graduates are life-long learning skills. Such skills and capabilities are essential in modern industrial software engineering environments. Workplace learning is a vital part of most professional software development jobs. What are the necessary life-long learning skills exactly? Why are those skills and capabilities essential in different software organizations? How can they be learned and improved? How do software professionals learn in their workplaces? What particular skills will be more critical in the future? Why? This topic could be investigated by case studies in real-life software organizations. The specific research questions could be some of the above or possibly focused on particular skills (e.g., assessing one's own and the works of other software developers). Contact: Petri Kettunen
Software development in non-ICT contexts (TOPIC AREA)
Software technology is increasingly applied in non-ICT domains and environments (e.g., healthcare, financial sector, telecommunications systems, industrial automation). Such conditions bring up many considerations for effective and efficient software engineering, such as: What are the key characteristics of different use domains (e.g., complexity, reliability)? What is the scope of the particular software system? How are the software requirements engineered? What are the specific constraints (e.g., regulations) in different domains to be considered in software engineering? How to measure the success of software projects and products? What software development methods (e.g., agile) are applicable in different domains? Why/why not? What particular software-related competencies are needed (e.g., digitalization, IoT, cyber-physical systems)? This research problem could be investigated theoretically (literature study) and empirically in industrial case studies. The actual research questions could be some of the above or formulated individually. Contact: Petri Kettunen
Creatively self-adaptive software architectures (TOPIC AREA)
We have recently started exciting research in the intersection between the research fields of self-adaptive software and computational creativity, intending to develop novel software architectures that can creatively adapt themselves in unforeseen situations. This initiative is a new research collaboration between the Discovery Group of Prof. Hannu Toivonen and ESE. There are different options for thesis work with either of the groups. To get a better idea of the topic, see Linkola et al. 2017. Aspects of Self-awareness: An Anatomy of Metacreative Systems. http://computationalcreativity.net/iccc2017/ICCC_17_accepted_submissions/ICCC-1… Contact: Tomi Männistö
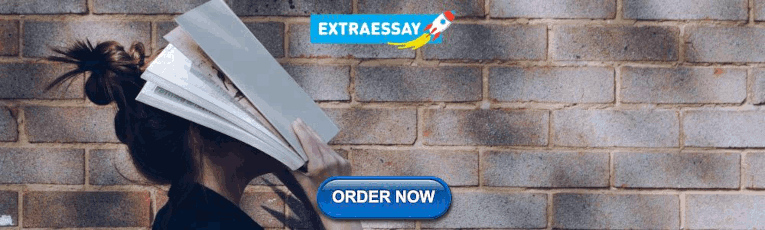
Continuous Experimentation (TOPIC AREA)
Software product and service companies need capabilities to evaluate their development decisions and customer and user value. Continuous experimentation, as an experiment-driven development approach, may reduce such development risks by iteratively testing product and service assumptions critical to the software's success. Experiment-driven development has been a crucial component of software development, especially in the last decade. Companies such as Microsoft, Facebook, Google, Amazon and many others often conduct experiments to base their development decisions on data collected from field usage. Contact: Tomi Männistö
Digitalization and digital transformations: impacts on software engineering and systems development (TOPIC AREA)
Digitalization is nowadays cross-cutting and inherent in most areas of businesses and organizations. Software is increasingly built-in and ubiquitous. Such trends and developments bring up many potential software research problems, such as: What does digitalization entail in different contexts? How should digitalization be taken into account in software development processes? What is the role of customer/user involvement in software-intensive systems development (e.g., digital services)? What are the key quality attributes? What new software engineering skills and competencies may be needed? What is the role of software (and IT) in general in different digital transformations (e.g., vs business process development)? How is digitalization related to traditional software engineering and computer science disciplines in different contexts? What aspects of software development and digital technologies are fundamentally new or different from the past? This research problem could be investigated theoretically (literature study) or empirically in industrial case studies. The actual research questions could be some of the above or formulated individually. Contact: Petri Kettunen
High-performing software teams (TOPIC AREA)
How is (high) performance defined and measured in software development (e.g., productivity)? Which factors affect it - positively or negatively - and how strongly (e.g., development tools, team composition)? Can we "build" high-performing software teams systematically, or do they merely emerge under certain favourable conditions? What are suitable organizational designs and environments for hosting and supporting such teams? See this link and this link for more info. Contact: Petri Kettunen
Software innovation (TOPIC AREA)
How are innovation and creativity taken into account in software development processes and methods (e.g., Agile)? What role do customer/user input and feedback play in software(-intensive) product creation (e.g., open innovation)? How to define and measure 'innovativeness' in software development? What makes software development organizations (more) innovative? See here for more about the topic. How can Open Data Software help innovation? Contact: Petri Kettunen
Streamlined Student Information Systems
High tech and human-centered
Empowering universities of tomorrow.
- For small colleges and universities in the U.S. and Canada
- SAAS/cloud-based SIS software
- Access to financial aid and faculty and student portal
- Utilizes Power BI reporting
- For large colleges and universities in the U.K. and Canada
- SAAS/cloud-based SIS software
- Build your own business logic for custom workflows
- Meets reporting requirements for U.K. and Canada
Supporting universities for the future.
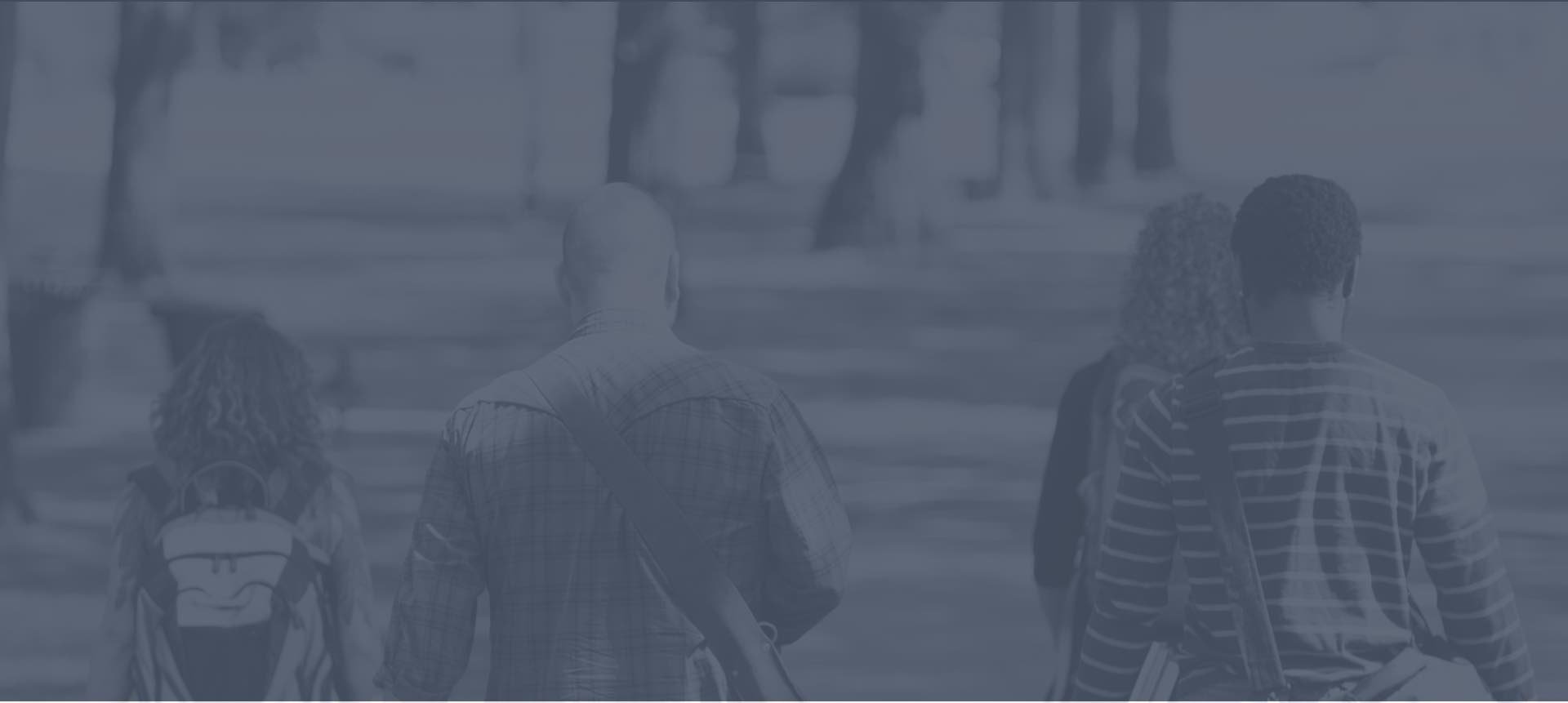
Most SIS software takes 30 months to implement. With Thesis, we’ll help implement new SIS software in 9-24 months.
Our cloud-based SIS will give you easy access to the information you need to make decisions for the future.
We’ll show you how to configure the software to meet your unique needs to support the future of your institution.
Implementation
We will implement a cloud-based SIS that is launched on time and on budget, built for the unique needs of your institution.
Education & Training
Our team will work together to train your faculty and staff to ensure a smooth SIS implementation for your university.
Thesis Community & Customer Support
Submit questions to your peers in Thesis Communities or open a support case. Thesis is here to help!

Build your Next Product with Deski.
Revolutionize Your Business with Thesis Software
Your premier provider of cutting-edge SaaS ERP solutions!

Check why you Should Choose us
Unmatched Expertise
Benefit from our specialized expertise in SaaS ERP solutions
Seamless Integration
Experience a smooth integration process.
Exceptional Customer Support
We believe in building long-lasting relationships with our clients.
Effortless Operations in 3 Simple Steps
Our solutions seamlessly optimize operations with effortless ease
Login to you account
Select Company
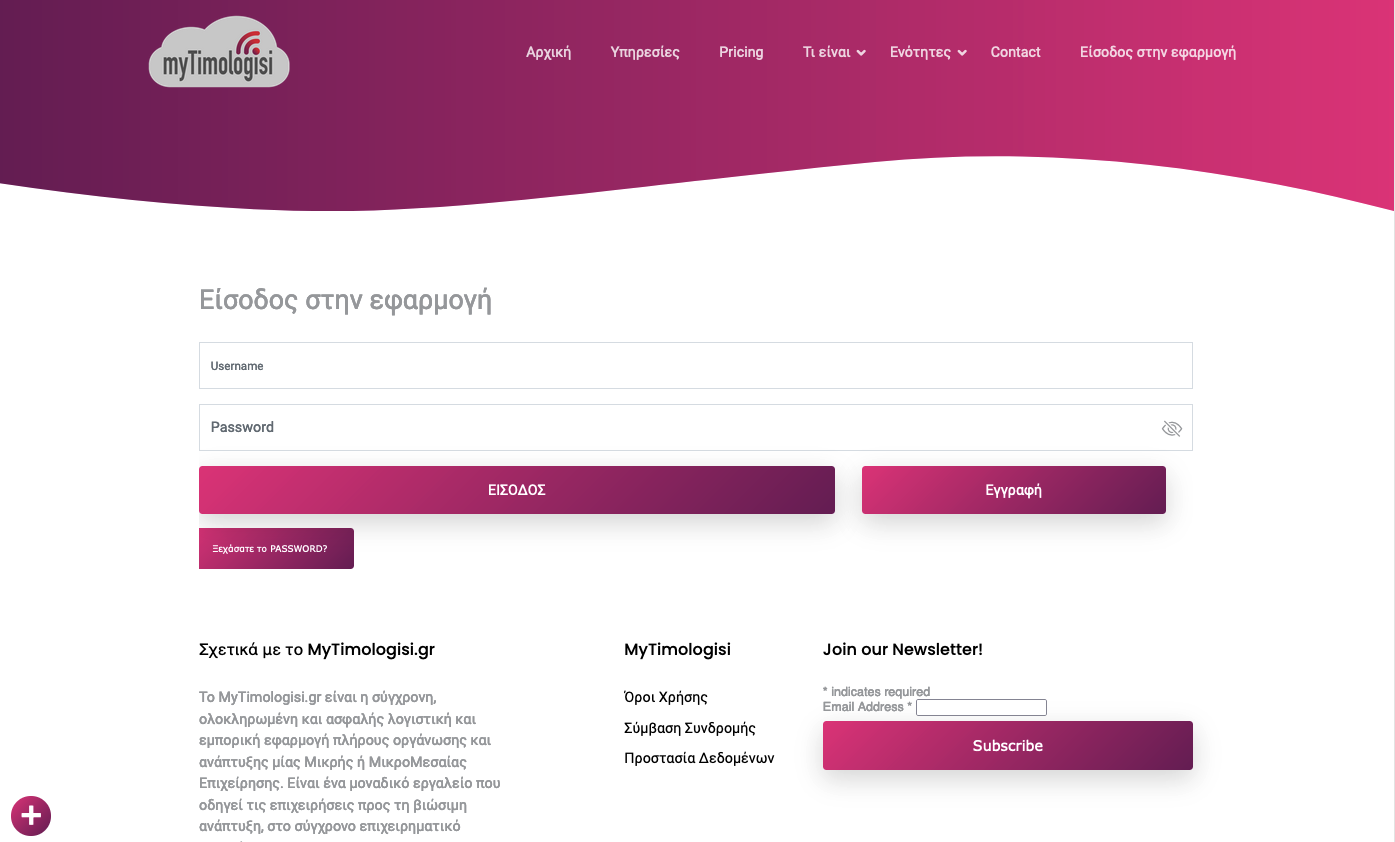
Connect to All Ecommerce Platforms with Ease
Seamlessly Connect to Any Ecommerce Platform and Automate Invoicing with Our API & Plugin Solutions

Our Portfolio

Check what our Customer say about us.
"Working with Thesis has been a game-changer for our business. Their SaaS ERP solution streamlined our operations and improved our efficiency across the board. The seamless integration with our existing systems and the exceptional customer support they provided exceeded our expectations. We highly recommend Thesis for their expertise and dedication."
"We are thrilled with the results we've achieved with Thesis's solution.The dedicated support team has been phenomenal, always available to assist us promptly.Thanks to Thesis, we have experienced significant improvements in our processes and overall business performance."
Our Pricing
Choose your Product.
Lorem ipsum dolor on adipisci elit sed do eiusmod liu.

- Unlimited Email
- 5gb Hosting & Domain
- Email & Live chat.
- 5gb Hosting
love our product? Start Free today.

Reach out to us today and discover how our team can empower your business through tailored

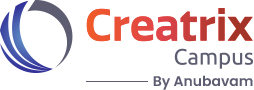
Thesis Management System
Easy-to-use and effective software that easily manages thesis and dissertation processes by minimizing challenges faced by scholars, advisors and committee members.
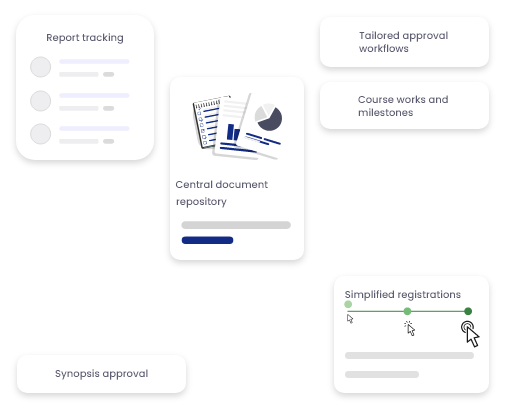
Online Thesis Management System that caters to everyone's needs
Research scholar.
No more frustration - right from initial application, guide selection to DC invite, course works, publications, synopsis, and final thesis submission and viva, everything is automated.
Research Guide
Constructs a bridge between scholars and guides with easy communication channels. Easy acceptance and approval of the coursework, with immediate review processes.
Research Committee Coordinator
Keep track of the entire thesis process in a centralized place. Facilitates research interviews, coordination with external reviewers, and final approvals/rejection in just clicks.
Thesis Management System, for on-the-go thesis, approvals, and submissions
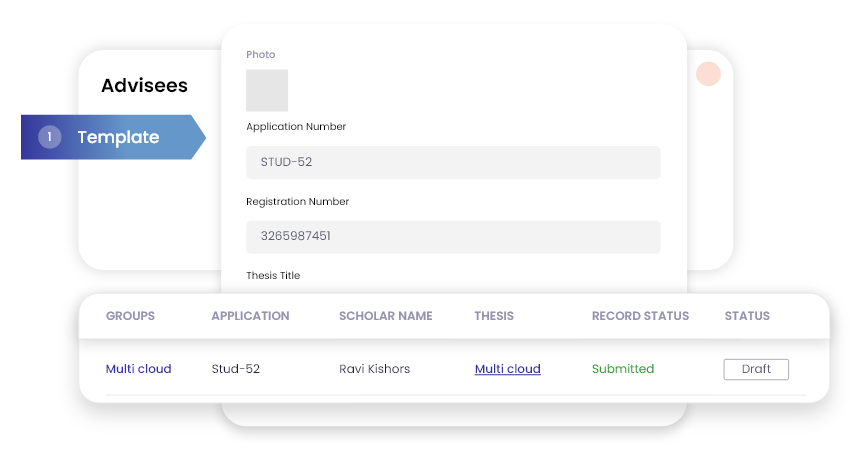
Make thesis registration effortless
Reduce long wait lines of your thesis registration process - from the applications, verification, PET score updation, and interviews to shortlisting and guide allocation, our thesis management system puts your admission committee in charge.
The system is intelligent enough to handle multiple statuses just in a few clicks. With automated processes and reporting tools, your admission team can stay empowered throughout their thesis registration cycle.
Do more with Creatrix:
- Reminders to speed up registrations
- Online thesis registration forms
- Status management for submissions
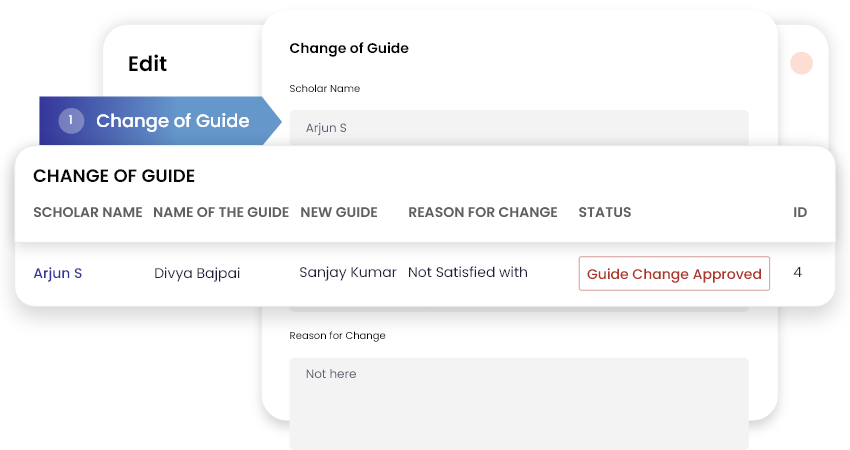
Handle a range of coursework
Supercharge your coursework registrations with an end-to-end thesis management system from Creatrix. Let researchers start any number of coursework related to their thesis, and maintain, and track it to completion.
Get on discussions with the guide, initiate meetings, send emails, create tasks, and follow-up notes. Get a clear understanding of the coursework done, sent for approval, and submitted, all in one view!
- Add/Edit/ Manage courses associated
- Manage documents related to coursework
- Coursework mapping with credit breakup
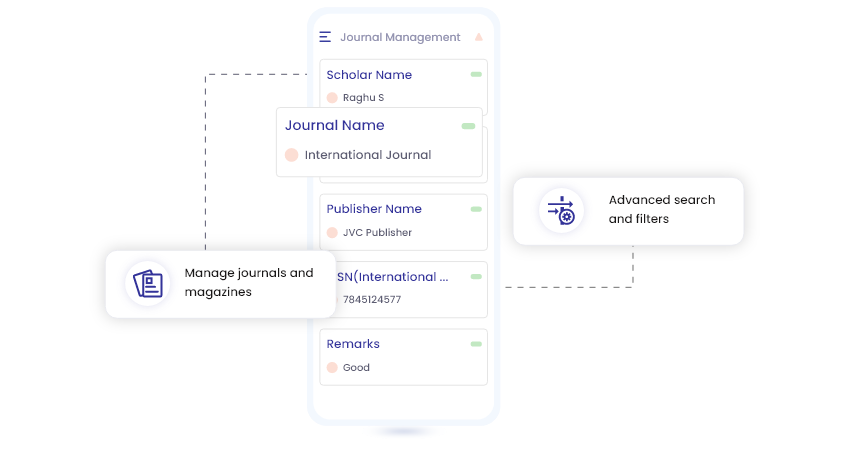
Keep publications on track
Compile your thesis publication process with a high-quality repository that tracks all research publications. Input all details associated with the publication along with remarks, so your scholarly efforts are in one place.
Configure filters to quickly sort out the right publication made from the list. View, edit, and trash them whenever needed. Facilitate effective connections between research scholars and their guides.
- Add/manage journals and magazines online
- Various tools to take action
- Faster communication channels for completion
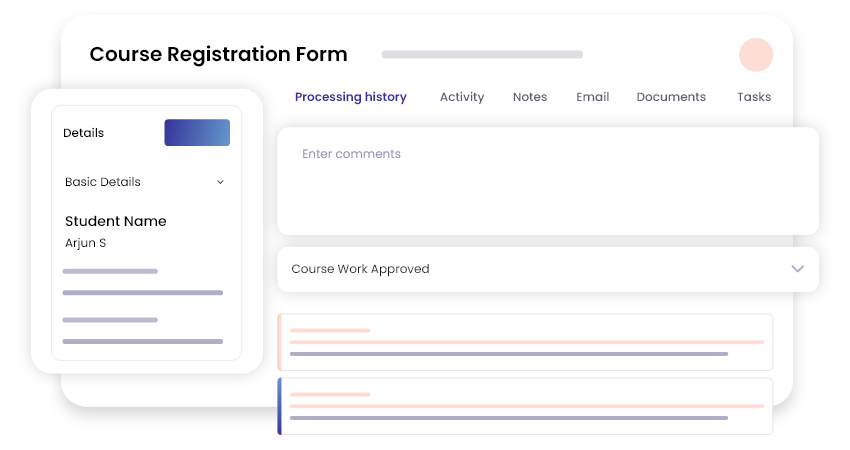
On-the-go approvals
Whether it is topic, thesis, or synopsis approval processes, handle them easily using Creatrix’s configurable workflows. Custom design multiple roles and permissions for RRC, RAC, VC, Referee, and much more.
Expedite thesis submission with individual portal logins, multiple statuses, automatic scheduling, alerts, reports, proper coordination, progress monitoring, and proactive support.
- Integration with third-party fee systems
- Guided thesis support with counsel
- No-code approval workflows for your unique needs

Configure topic approvals
Obtain quicker thesis approvals from your adviser, departmental thesis reviewer, and other committee members at the touch of a button, with our intuitive interface!

Progress report tracking
The progress reporting feature in our thesis management software allows you to track the status of the project, and plan for upcoming milestones in your thesis submission journey.

Extension requests in a snap
Initiate dissertation extension to your thesis panel with all supporting documents with the change of date with our flexible thesis platform

Change of guide requests
Request a change of guide anywhere in the middle of your thesis journey and seek approval through a series of customized steps.

No more extension delays
By inputting new guide details with the old one and the reason, get underway to apply for a change of guide within the specific time using our flexible thesis software.

Enjoy simplicity and efficiency
Empower every one part of the thesis committee with a more functional, easy-to-use, and less code-driven thesis software that dynamically changes as per your needs.
Frequently Asked Questions
What is a thesis management system.
A Thesis Management System is a software solution designed to support the management and administration of theses or dissertations in academic institutions. It provides tools and features to streamline the entire thesis process, including proposal submission, review and approval workflows, document management, and tracking of progress.
What are the key features of a Thesis Management System?
Common features of a Thesis Management System include:
- Online submission of thesis proposals
- Centralized repository for document management
- Review and approval workflows
- Document management and version control
- Communication tools for supervisors and students
- Progress tracking and milestone management
- Plagiarism detection
- Secure storage and access control for thesis documents
- Collaboration tools for co-authors and committee members Integration with other modules or systems
- Customizable templates and forms for thesis management
- Reporting and analytics capabilities for monitoring thesis progress
- User-friendly interface for easy navigation and use.
How does a Thesis Management System benefit academic institutions?
A Thesis Management System offers several benefits to academic institutions, including:
- Streamlined thesis submission and approval processes, reducing paperwork and administrative overhead.
- Reduces administrative burden. Improves efficiency and saves time.
- Improved communication and collaboration between students, supervisors, and committee members.
- Enhanced document management and version control, ensuring proper organization and access to thesis materials.
- Efficient progress tracking, enabling timely feedback and intervention when needed.
- Plagiarism detection tools, ensuring academic integrity in thesis submissions.
- Reporting and analytics for evaluating thesis progress and outcomes.
Can a Thesis Management System handle different types of theses?
Yes, a Thesis Management System can handle different types of theses, including master's theses, doctoral dissertations, research papers, and other scholarly works. The system allows for customization in defining thesis categories and associated workflows.
Is it possible to integrate a Thesis Management System with other systems?
Integration with other systems is possible depending on the capabilities and compatibility of the Thesis Management System. Integration with student information systems, library databases, or plagiarism detection tools can streamline data exchange and provide a seamless experience for users.
How secure is the data in a Thesis Management System?
Data security is crucial for any software system, including a Thesis Management System. These systems employ security measures such as encryption, user authentication, access controls, and regular backups to ensure data protection. It's important to choose a reputable system provider that prioritizes data security and complies with relevant privacy regulations.
Can a Thesis Management System facilitate collaboration among co-authors and committee members?
Yes, many Thesis Management Systems provide collaboration tools to facilitate communication and collaboration among co-authors and committee members. These tools enable online discussions, document sharing, and feedback exchange, ensuring smooth collaboration throughout the thesis process.
Can a Thesis Management System handle multiple reviewers and approval workflows?
Yes, a Thesis Management System can handle multiple reviewers and approval workflows. The system allows for the customization of review and approval processes based on the specific requirements of the academic institution. Different roles and permissions can be assigned to reviewers and approvers to ensure appropriate access and control.
Is it possible to customize a Thesis Management System?
Customization options may vary depending on the system provider. Some providers offer flexibility to customize certain aspects of the system, such as thesis categories, approval workflows, or reporting formats. It's recommended to discuss customization requirements with the system provider to understand the available options and tailor the system to specific institutional needs.
Checkout our more innovative solutions for higher education
Higher education strategic.
Place your long-term and short-term institutional goals efficiently and track them to…
Learning Management System
Most intuitive, user-friendly Learning Management System that augments teaching and…
Student Portfolio
An enriching student ePortfolio that improves and empowers today’s modern students.
Student Assessment Management
An LMS integrated, OBE, CBE, flipped learning supported Assessment platform for modern…
Placement Management System
An integrated campus placement management system that manages everything from student…
Student Advising System
A student planning and advising platform that empowers students to take charge of their…
Student Information System
A comprehensive, mobile-first student information management system that takes care of…
- Skip to main content
- Accessibility information
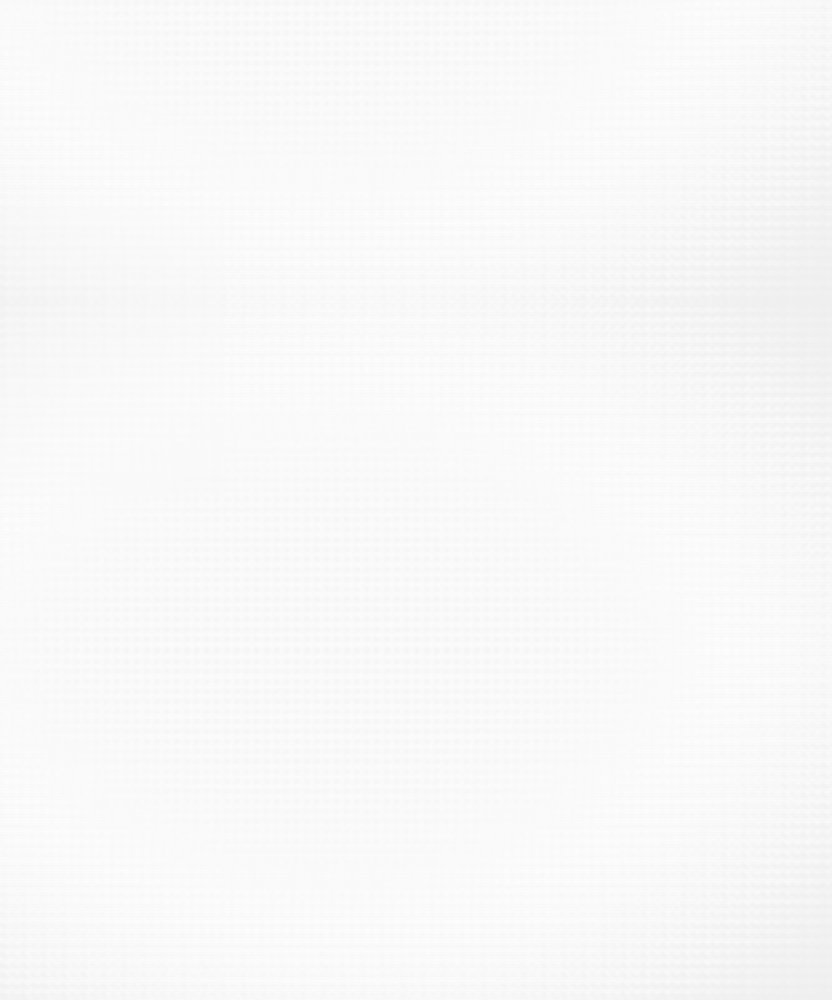
- Enlighten Enlighten
Enlighten Theses
- Latest Additions
- Browse by Year
- Browse by Subject
- Browse by College/School
- Browse by Author
- Browse by Funder
- Login (Library staff only)
In this section
Defining interfaces between hardware and software: Quality and performance
Reid, Alastair David (2019) Defining interfaces between hardware and software: Quality and performance. PhD thesis, University of Glasgow.
One of the most important interfaces in a computer system is the interface between hardware and software. This interface is the contract between the hardware designer and the programmer that defines the functional behaviour of the hardware. This thesis examines two critical aspects of defining the hardware-software interface: quality and performance.
The first aspect is creating a high quality specification of the interface as conventionally defined in an instruction set architecture. The majority of this thesis is concerned with creating a specification that covers the full scope of the interface; that is applicable to all current implementations of the architecture; and that can be trusted to accurately describe the behaviour of implementations of the architecture. We describe the development of a formal specification of the two major types of Arm processors: A-class (for mobile devices such as phones and tablets) and M-class (for micro-controllers). These specifications are unparalleled in their scope, applicability and trustworthiness. This thesis identifies and illustrates what we consider the key ingredient in achieving this goal: creating a specification that is used by many different user groups. Supporting many different groups leads to improved quality as each group finds different problems in the specification; and, by providing value to each different group, it helps justify the considerable effort required to create a high quality specification of a major processor architecture. The work described in this thesis led to a step change in Arm's ability to use formal verification techniques to detect errors in their processors; enabled extensive testing of the specification against Arm's official architecture conformance suite; improved the quality of Arm's architecture conformance suite based on measuring the architectural coverage of the tests; supported earlier, faster development of architecture extensions by enabling animation of changes as they are being made; and enabled early detection of problems created from architecture extensions by performing formal validation of the specification against semi-structured natural language specifications. As far as we are aware, no other mainstream processor architecture has this capability. The formal specifications are included in Arm's publicly released architecture reference manuals and the A-class specification is also released in machine-readable form.
The second aspect is creating a high performance interface by defining the hardware-software interface of a software-defined radio subsystem using a programming language. That is, an interface that allows software to exploit the potential performance of the underlying hardware. While the hardware-software interface is normally defined in terms of machine code, peripheral control registers and memory maps, we define it using a programming language instead. This higher level interface provides the opportunity for compilers to hide some of the low-level differences between different systems from the programmer: a potentially very efficient way of providing a stable, portable interface without having to add hardware to provide portability between different hardware platforms. We describe the design and implementation of a set of extensions to the C programming language to support programming high performance, energy efficient, software defined radio systems. The language extensions enable the programmer to exploit the pipeline parallelism typically present in digital signal processing applications and to make efficient use of the asymmetric multiprocessor systems designed to support such applications. The extensions consist primarily of annotations that can be checked for consistency and that support annotation inference in order to reduce the number of annotations required. Reducing the number of annotations does not just save programmer effort, it also improves portability by reducing the number of annotations that need to be changed when porting an application from one platform to another. This work formed part of a project that developed a high-performance, energy-efficient, software defined radio capable of implementing the physical layers of the 4G cellphone standard (LTE), 802.11a WiFi and Digital Video Broadcast (DVB) with a power and silicon area budget that was competitive with a conventional custom ASIC solution.
The Arm architecture is the largest computer architecture by volume in the world. It behooves us to ensure that the interface it describes is appropriately defined.
Actions (login required)
Downloads per month over past year
View more statistics

The University of Glasgow is a registered Scottish charity: Registration Number SC004401
Academia.edu no longer supports Internet Explorer.
To browse Academia.edu and the wider internet faster and more securely, please take a few seconds to upgrade your browser .
Enter the email address you signed up with and we'll email you a reset link.
- We're Hiring!
- Help Center
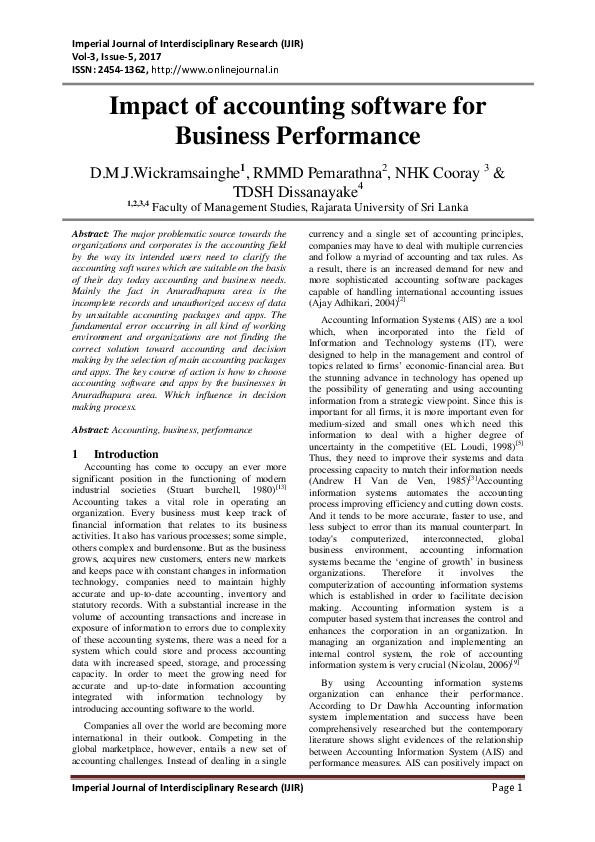
Impact of accounting software for Business Performance

The major problematic source towards the organizations and corporates is the accounting field by the way its intended users need to clarify the accounting soft wares which are suitable on the basis of their day today accounting and business needs. Mainly the fact in Anuradhapura area is the incomplete records and unauthorized access of data by unsuitable accounting packages and apps. The fundamental error occurring in all kind of working environment and organizations are not finding the correct solution toward accounting and decision making by the selection of main accounting packages and apps. The key course of action is how to choose accounting software and apps by the businesses in Anuradhapura area. Which influence in decision making process. Abstract: Accounting, business, performance
Related Papers
Mazedan International Research Academy
Naqeeb Ullah, Ph.D
The aim of the research was to empirically analyse the most effective accounting software for accountants, specifically in SMEs of UK. For the current research, primary quantitative research method was used, and information were gathered from 100 accountants working in SMEs of UK. For data analysis, descriptive and frequency analysis were used though using SPSS software. Findings revealed that Fresh book, Quick book, Zoho, Wave are mostly used in SMEs of UK. Further, it has also been analysed that Fresh book is one of the most effective accounting software due to higher quality, features, and fastest speed. Findings in the current research has significantly contributed in the information body of accounting sphere, and fill a gap in previous studies.
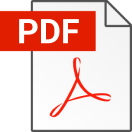
International Scientific days 2016 :: The Agri-Food Value Chain: Challenges for Natural Resources Management and Society :: Proceedings
Zuzana Bigasová
Rahmawati Rahmawati
Company’s management must have the information in order to make business and financial decisions. As management decisions have crucial importance, the information appears as a key instrument of governance. Information produced by the accounting information systems are important for the effective management of business activities, and according to the type of users, its information requests and needs vary. In this paper, particular attention is given to the accounting information systems and their importance for the business and financial decision making of individual users. Key words: application of accounting information system, accounting information system, decision making process, business decision.
Shanlax International Journal of Management
mahmoud manayseh
The purpose of this study is to qualitatively explain the quality of accounting application systems in order to promote the performance of a small, medium and large Sultanate of Oman company’s financial sector. The method used in this study is a semi-structured interview with whistleblowers regarding the quality of accounting information as a corporate budget accountant. As a result, high-quality accounting application systems analyze existing accounting information systems for quality such as security, accessibility, reliability, data integration, and accurate and timely information availability that can support business performance. We found that it was configured. Companies need to include these four elements in their accounting system. The conclusion of this study is that the company used a high quality accounting information system because it could meet all four factors and support the performance of the company’s employees and able to improve the quality of its employees perfo...
Hojjatallah Salari
Ibrahim Saheed
Vysoká škola bezpečnostného manažérstva v Košiciach
Maksym Bezpartochnyi
The book is addressed to those who want to open a business in Romania, to become entrepreneurs or managers and to accumulate necessary accounting information on starting and managing a business. Accounting is the "ace up the sleeve" of any manager because, with the information provided, one can know the past, present and predict the future of a company. This paper presents the theoretical elements of the concepts used in accounting necessary for any manager. The book is intended for those interested in this field whose training can be completed with the ABC of accounting.
IJMSBR Open Access Journal
Regarding to the potential capabilities of accounting information systems (AIS) and software packages as computer AIS, and regarding to the continuous changes and rapid developments of economical units, provision of relevant, reliable, and comparable financial information is necessary. To investigate this, the question is that whether IT affects qualitative features of accounting information in financial statements?To do this research, a sample of 105 confidant auditors from Tehran Stock Exchange and professors of Islamic Azad University, region 5, was selected by Simple Random Sampling (SRS) method as questionnaires. Inferential statistical method, Clemogrov-Smironov test, and t-student test were used. It was found that accounting information systems and softwares highly affect on comparison capability and relevance of financial statement, but they lowly affect on reliability of them. Also, variance analysis was done for three indices of relevance, reliability, comparability. To do this, pair groups were compared by t-test. In this test, first rank was for relevance.
Journal of Applied Science, Information and Computing
Olusegun Alao
Tracking the various business parameters variables related to the business’s goal is a difficult task to do without the right tools. Failure to properly put in place accounting processes that oversee and manage such parameters has led to the demise of many promising small and big organizations. This work looks into how the use of accounting software affects the quality of reporting while focusing on the tools and application modules that make the process hitch-free. Because of the lack of local data and feedback from first-hand operators of accounting software, the study infers knowledge from other works on the effectiveness of accounting software in organizational reporting. It also looks into the tools that come with Microsoft Excel, one of the most widely used accounting software in Nigeria. We make use of sales data gotten online to implement this tool and the result shows that the Microsoft Excel application is capable of minimizing errors, automating tasks, and forecasting. By...
International Journal of Advanced Computer Science and Applications
Agustinus Winoto
RELATED PAPERS
Lori Ashworth
Osman Ferda Beytekin
European Respiratory Journal
Alexandra Borba
Zagazig University Medical Journal
hosni salama
Translational Psychiatry
Yarani Gonzalez
Naučno-tehničeskij Vestnik Informacionnyh Tehnologij, Mehaniki i Optiki
Yuri Matveev
Fitrio Deviantony
Periodico Di Mineralogia
stefania mancuso
Allergologia et Immunopathologia
Historia y Memoria de la Educación
Pablo Cereceda
Annals of Agrarian Science
Milind Sindkhedkar
Clinical Biochemistry
Mohamad Ali
Frontiers in Public Health
Christian Flores
Sonali Mahanta
Frontiers in Physiology
Juan Latorre
Jurnal Mahasiswa Komunikasi Cantrik
Holy R Dhona
Journal of Applied Social Science
Wendy Robeson
International journal of innovative science and research technology
Asita Kulshreshtha
wayan sadyana
Cardiovascular Research
Heleen Van Beusekom
Facta Universitatis
Shahroud Azami
International Journal of Spine Surgery
Isaac Swink
Andreas Niederberger
RELATED TOPICS
- We're Hiring!
- Help Center
- Find new research papers in:
- Health Sciences
- Earth Sciences
- Cognitive Science
- Mathematics
- Computer Science
- Academia ©2024

Automated and Portable Machine Learning Systems
The landscape of ML ecosystem including models, software, and hardware evolves quickly due to phenomenal growth of Machine Learning (ML) and its application. Nevertheless, it remains challenging and labor-intensive to swiftly adapt existing ML systems to new models and hardware to maximize performance. We find that it is attributed to existing ML systems falling short in portability and automatability across several crucial layers of a system stack. However, building a portable ML system requires non-trivial modeling of intricate commonalities and differences of diverse ML models or platforms. In addition, automating ML system layers introduces the challenge of designing practical search space and search algorithms to customize optimizations to a given model and hardware.
In this thesis, we aim to tackle the challenges above of building an automated and portable ML system with a focus on crucial ML system layers. Specifically, the thesis explores ways to build an efficient system that automates 1) integration of ML backends and 2) ML parallelisms and makes them more portable. We develop a user interface and system stack to be more portable across different backends and underlying hardware. We also design practical search space and algorithms to automate backend placement and parallelism.
First, we built Collage, a DL framework that offers seamless integration of DL backends. Collage provides an expressive backend registration interface that allows users to precisely specify the capability of various backends. By leveraging the specifications of available backends, Collage automatically searches for an optimized backend placement strategy for a given workload and execution environment.
Second, we developed GraphPipe, a distributed system that enable performant and scalable DNN training. GraphPipe automatically partitions a DNN into a graph of stages, optimizes micro-batch schedules for these stages, and parallelizes DNN training. This generalizes existing sequential pipeline parallelism and preserves the inherent topology of a DNN, resulting in reduced memory requirement and improved GPU performance.
Lastly, we conducted a comparative analysis of parallelisms in distributed LLM inference for long sequence application. Specifically, we focused on Cache Parallelism (CP), a scheme to parallelize long KV cache in auto-regressive decod?ing step in LLM inference. We investigated trade-offs from different parallelisms for long context scenarios where we need to process tens of thousands tokens.
Degree Type
- Dissertation
- Computer Science
Degree Name
- Doctor of Philosophy (PhD)
Usage metrics
- Distributed Computing

- Quantum Research
Qiskit: The software for quantum performance
Utility-scale quantum computers built by ibm. groundbreaking algorithms applied by you. performance by qiskit..
15 May 2024
Ryan Mandelbaum
Blake Johnson
Share this blog
Last year, we entered a new era of quantum computing: the era of quantum utility . For the first time, quantum computers can run circuits beyond the reach of brute force or exact classical simulation. But our true goal is for the community to demonstrate quantum advantage — using utility-scale quantum processing to deliver value for use cases beyond state-of-the-art classical methods. The crux of our users’ discovery of quantum advantage will be a highly performant and stable software stack to enable the next generation of quantum algorithms. It will require a more performant Qiskit .
What is quantum utility? Read more about how this landmark was achieved .
This year, we introduced the first stable version of the Qiskit open-source software development kit — Qiskit SDK 1.x. But Qiskit is more than just the world’s most popular quantum development software to build and construct quantum circuits. We are redefining Qiskit to represent the full-stack software for quantum at IBM, extending the Qiskit SDK with middleware software and services to write, optimize, and execute programs on IBM Quantum systems — including new generative AI code assistance tools.
Read more about the Qiskit 1.0 release summary .
Qiskit will drive our vision to bring useful quantum computing to the world.
How did we get here?
When we first released Qiskit as an SDK in 2017, it quickly became the software development tool for learning and researching quantum computing. To date, more than 600,000 registered users have run over three trillion circuits on IBM Quantum computers using Qiskit. Researchers have published nearly 2,900 research papers with Qiskit and IBM systems, and over 700 universities around the world have developed quantum computing classes using Qiskit. It has become the preferred open source software development kit for 69% of respondents to the Unitary Fund’s 2023 Open Source Software Survey .
But in the past year, we’ve demonstrated that quantum computers can be used as tools for scientific discovery. And now, with our 100+ qubit quantum computers, users can begin extracting real performance from quantum hardware. Experts can begin doing algorithm discovery in their respective domains with the goal of finding quantum advantage. Qiskit, in turn, must mature to become the most performant software toolset to work alongside the world’s most performant quantum computing hardware.
Qiskit will take users through the entire journey of running utility-scale quantum workloads, from writing code to post-processing the results and everything in between.
Our vision for quantum software
Last year, we introduced a repeatable recipe for developing quantum workloads called the Qiskit pattern — a four-step journey along which users map, optimize, execute, and post-process their quantum circuits. And here’s how Qiskit will bring users along each step of that journey.
The first step of the Qiskit pattern is to map the problem to quantum circuits using the updated Qiskit SDK 1.x. Qiskit SDK 1.x evolves the widely popular open-source development kit to a highly stable, reliable, and utility-scale tool for building and optimizing circuits and operators. It enables working with the increasingly complex quantum circuits being discovered by users — those with 100s of qubits and thousands of gates or more.
The second step is to optimize the circuits with the help of major performance improvements to the Qiskit SDK and the new Qiskit Transpiler Service. Two years ago, we began an effort to improve Qiskit’s performance by refactoring performance-critical code sections from Python into Rust. We are not done yet, but already this effort is yielding impressive gains. For example, binding and transpiling is now 39x faster than it was two years ago with Qiskit SDK 0.33. More recent scrutiny of memory use now allows users to comfortably work with larger circuits thanks to a greater than 3x reduction in memory footprint compared to Qiskit SDK 0.43. More improvements are still to come.
We have also been experimenting with AI-enhanced transpilation methods based upon a reinforcement learning approach. Already this exploration is yielding promising results, with a reduction in both the number of two-qubit gates and circuit depth on a variety of benchmark circuits. We are making these advanced transpilation capabilities available to our Premium users with the beta release of the Qiskit Transpiler Service. It integrates seamlessly into the Qiskit SDK as a plugin.
Read more about the updates to Qiskit SDK 1.x in the release summary here .
Step three of the Qiskit pattern is to execute workloads using the Qiskit Runtime Service. Users will notice an overhaul of the Qiskit Primitives — the Sampler and Estimator — for extracting results from quantum computers. The Primitives now define the interface to quantum systems within Qiskit. The Qiskit Runtime Service executes primitive queries with advanced error mitigation built-in, and now supports dynamic circuits in the Sampler.
Zooming out, we also released Qiskit Serverless in late 2023. Serverless will provision the appropriate resources required to run quantum circuits. But we must continue maturing Qiskit so that users can extend and reuse their code as we begin to think about production workloads.
We’re working to abstract the building blocks we’ve introduced as part of the Qiskit pattern into components called Qiskit Functions in the coming year. Qiskit Functions will be a catalogue of functions in the serverless layer that users can access as part of their workflow. Qiskit Functions will be provided both by IBM and by third-party integrators for certain types of problems — they might include custom error handling routines, post-processing techniques, and more.
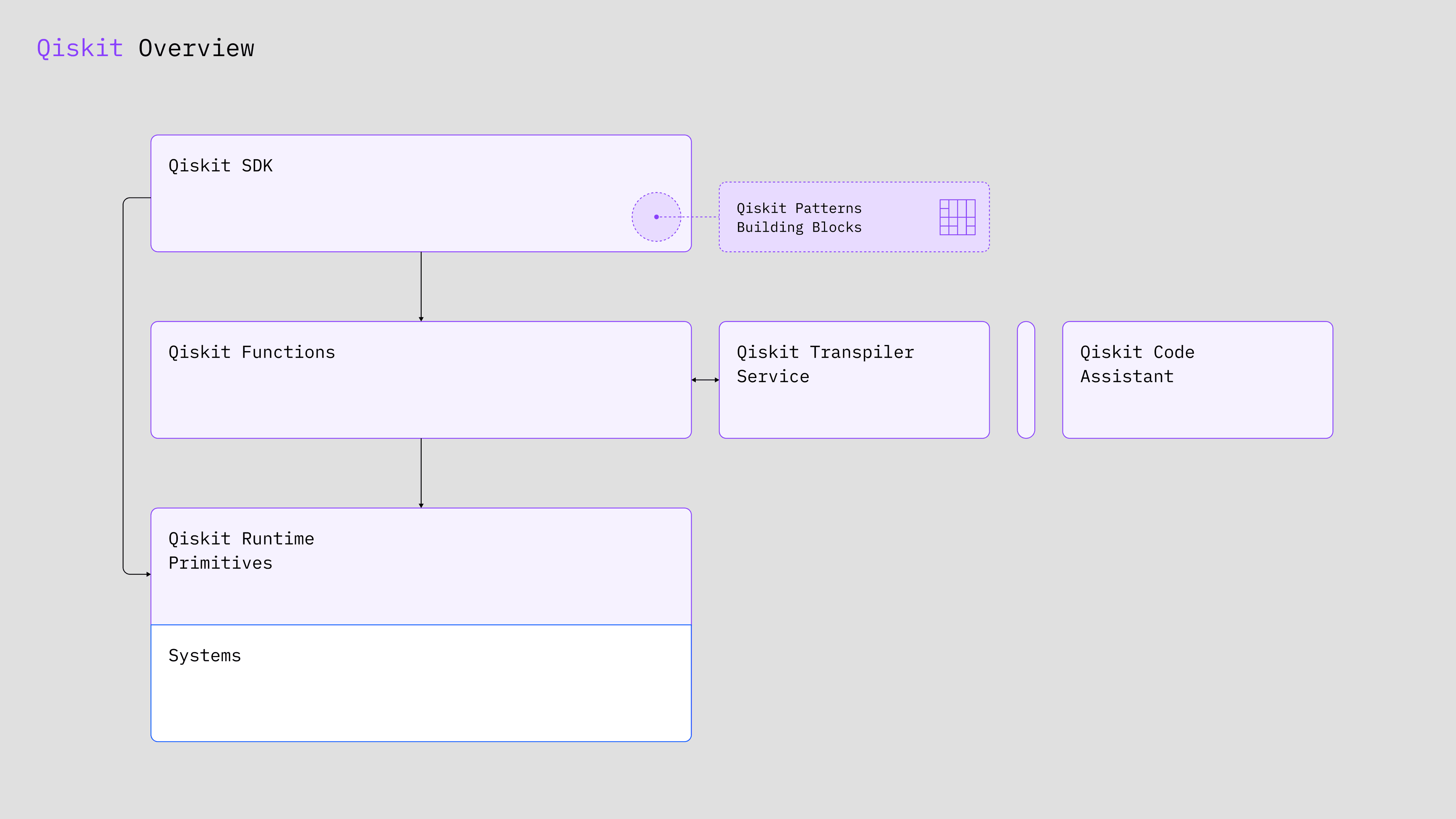
Qiskit stack overview.
Frictionless development with AI + Functions
But you need not take this journey alone. Since our inception, our core objective has been a frictionless developer experience. At the 2023 IBM Quantum Summit , we previewed our Qiskit AI Code Assistant to make programing quantum even easier. And this month, we have now released the Qiskit Code Assistant Service in alpha.
Qiskit Code Assistant is trained on Qiskit SDK 1.x and IBM Quantum features with an LLM model based on IBM Granite, powered by watsonx. It includes a Visual Studio Code extension and Jupyter Lab integration. We’ll release the first Qiskit HumanEval benchmark next month to evaluate Code Assistant’s ability to generate usable code. As we work toward a beta, we’ll hope you’ll get started exploring how Code Assistant alongside Qiskit patterns can accelerate your development workflow.
This is just the start. In the next six months, we’ll be continuing to improve Qiskit’s performance as we make it the highest-performing quantum software stack.
Together, this is part of our broader vision for quantum computing: the IBM Quantum Platform, where Qiskit plus IBM quantum systems equals utility-scale work. Qiskit is as important for running quantum workloads as our hardware is.
Follow along with our IBM Quantum Development Roadmap to see how Qiskit and IBM Quantum systems will evolve as we begin to deploy quantum for utility-scale workflows and realize our vision for useful quantum computing.
Get started with Qiskit
Keep exploring, how execution modes enable efficient, utility-scale workloads.
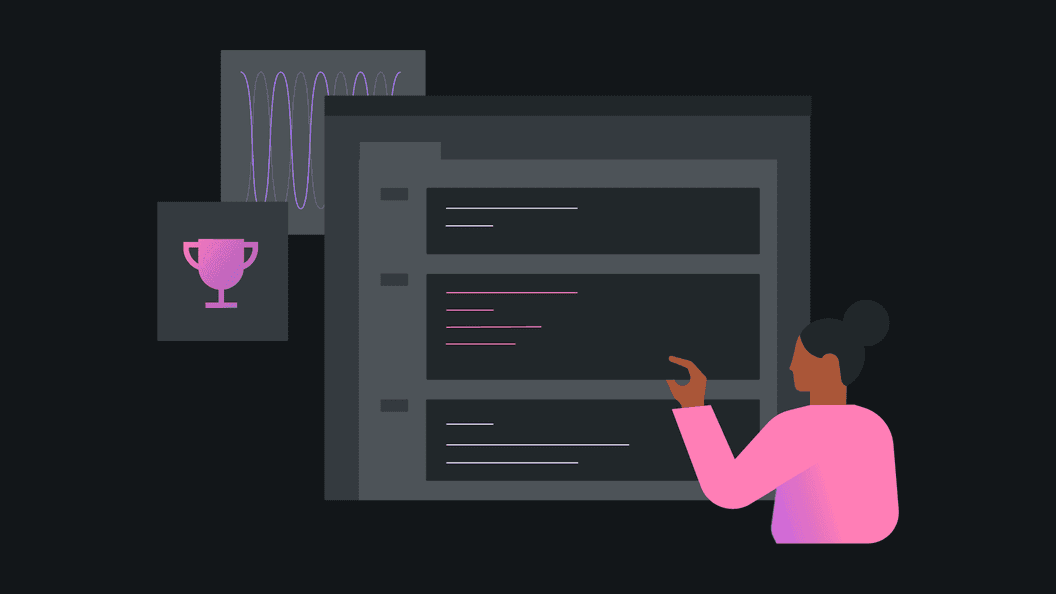
Get started with Qiskit SDK 1.0 at the 2024 IBM Quantum Challenge
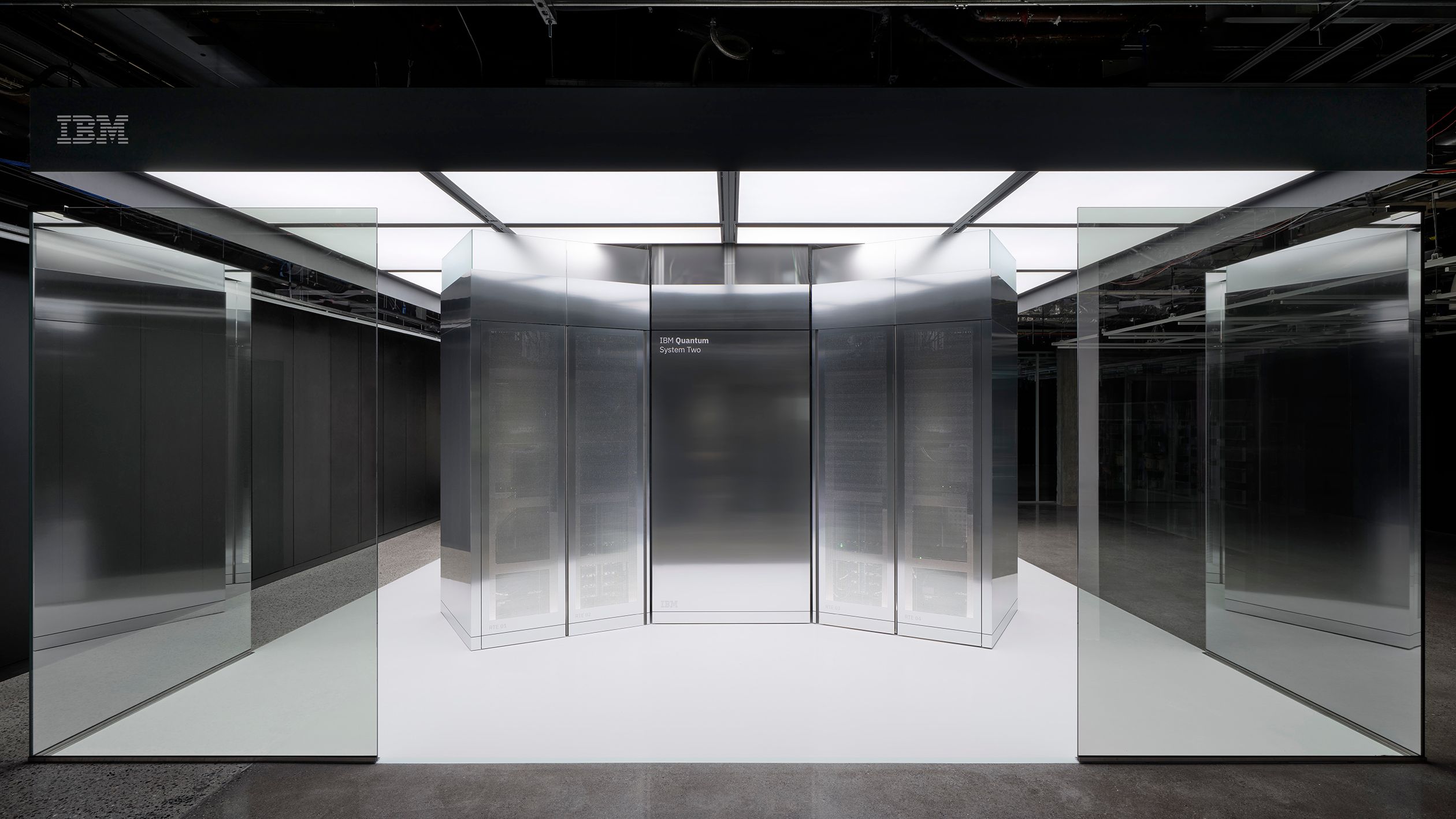
The hardware and software for the era of quantum utility is here
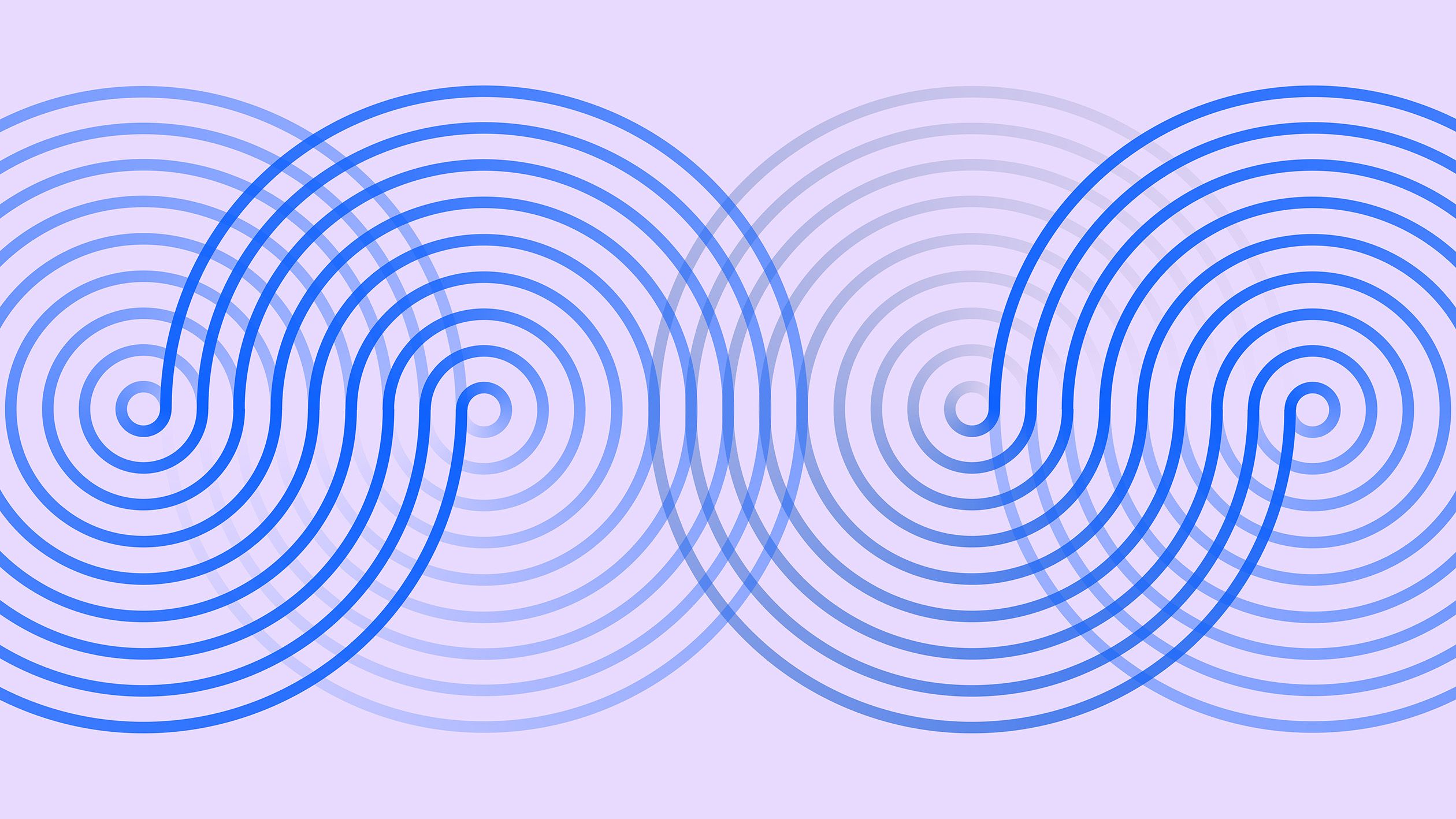
The IBM approach to reliable quantum computing
- Short Report
- Open access
- Published: 13 May 2024
Commonly used software tools produce conflicting and overly-optimistic AUPRC values
- Wenyu Chen 1 na1 ,
- Chen Miao 1 na1 ,
- Zhenghao Zhang 2 ,
- Cathy Sin-Hang Fung 1 ,
- Ran Wang 1 ,
- Yizhen Chen 2 ,
- Yan Qian 3 ,
- Lixin Cheng 4 ,
- Kevin Y. Yip 2 , 5 ,
- Stephen Kwok-Wing Tsui 1 , 6 &
- Qin Cao ORCID: orcid.org/0000-0002-3106-562X 1 , 6 , 7
Genome Biology volume 25 , Article number: 118 ( 2024 ) Cite this article
84 Accesses
4 Altmetric
Metrics details
The precision-recall curve (PRC) and the area under the precision-recall curve (AUPRC) are useful for quantifying classification performance. They are commonly used in situations with imbalanced classes, such as cancer diagnosis and cell type annotation. We evaluate 10 popular tools for plotting PRC and computing AUPRC, which were collectively used in more than 3000 published studies. We find the AUPRC values computed by the tools rank classifiers differently and some tools produce overly-optimistic results.
Introduction
Many problems in computational biology can be formulated as binary classification, in which the goal is to infer whether an entity (e.g., a cell) belongs to a target class (e.g., a cell type). Accuracy, precision, sensitivity (i.e., recall), specificity, and F1 score (Additional file 1 : Fig. S1) are some of the measures commonly used to quantify classification performance, but they all require a threshold of the classification score to assign every entity to either the target class or not. The receiver operating characteristic (ROC) and precision-recall curve (PRC) avoid this problem by considering multiple thresholds [ 1 ], which allows detailed examination of the trade-off between identifying entities of the target class and wrongly including entities not of this class. It is common to summarize these curves by the area under them (AUROC and AUPRC, respectively), which is a value between 0 and 1, with a larger value corresponding to better classification performance.
When the different classes have imbalanced sizes (e.g., the target cell type has few cells), AUPRC is a more sensitive measure than AUROC [ 1 , 2 , 3 , 4 ], especially when there are errors among the top predictions (Additional file 1 : Fig. S2). As a result, AUPRC has been used in a variety of applications, such as reconstructing biological networks [ 5 ], identifying cancer genes [ 6 ] and essential genes [ 7 ], determining protein binding sites [ 8 ], imputing sparse experimental data [ 9 ], and predicting patient treatment response [ 10 ]. AUPRC has also been extensively used as a performance measure in benchmarking studies, such as the ones for comparing methods for analyzing differential gene expression [ 11 ], identifying gene regulatory interactions [ 12 ], and inferring cell-cell communications [ 13 ] from single-cell RNA sequencing data.
Given the importance of PRC and AUPRC, we analyzed commonly used software tools and found that they produce contrasting results, some of which are overly-optimistic.
For each entity, a classifier outputs a score to indicate how likely it belongs to the target (i.e., “positive”) class. Depending on the classifier, the score can be discrete (e.g., random forest) or continuous (e.g., artificial neural network). Using a threshold t , the classification scores can be turned into binary predictions by considering all entities with a score \(\ge t\) as belonging to the positive class and all other entities as not. When these predictions are compared to the actual classes of the entities, precision is defined as the proportion of entities predicted to be positive that are actually positive, while recall is defined as the proportion of actually positive entities that are predicted to be positive (Additional file 1 : Fig. S1).
The PRC is a curve that shows how precision changes with recall. In the most common way to produce the PRC, each unique classification score observed is used as a threshold to compute a pair of precision and recall values, which forms an anchor point on the PRC. Adjacent anchor points are then connected to produce the PRC.
When no two entities have the same score (Fig. 1 a), it is common to connect adjacent anchor points directly by a straight line [ 14 , 15 , 16 , 17 , 18 , 19 ] (Fig. 1 b). Another method uses an expectation formula, which we will explain below, to connect discrete points by piece-wise linear lines [ 20 ] (Fig. 1 c). The third method is to use the same expectation formula to produce a continuous curve between adjacent anchor points [ 17 , 21 ] (Fig. 1 d). A fourth method that has gained popularity, known as Average Precision (AP), connects adjacent anchor points by step curves [ 15 , 19 , 22 , 23 ] (Fig. 1 e). In all four cases, PRC estimates a function of precision in terms of recall based on the observed classification scores of the entities, and AUPRC estimates the integral of this function using trapezoids (in the direct straight line case), interpolation lines/curves (in the expectation cases), or rectangles (in the AP case).
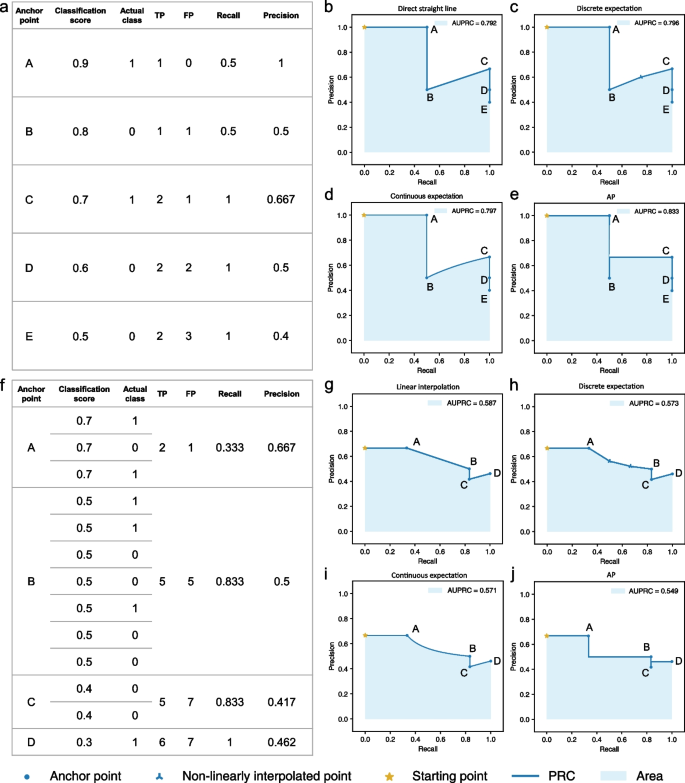
Different methods for connecting adjacent anchor points on the PRC. a An illustrative data set with no two entities receiving the same classification score. b – e Different methods for connecting adjacent anchor points when there are no ties in classification scores, namely b direct straight line, c discrete expectation, d continuous expectation, and e AP. f An illustrative data set with different entities receiving the same classification score. Each group of entities with the same classification score defines a single anchor point (A, B, C, and D, from 3, 7, 2, and 1 entities, respectively). g – j Different methods for connecting anchor point B to its previous anchor point, A, namely g linear interpolation, h discrete expectation, i continuous expectation, and j AP. In c and h , tp is set to 0.5 and 1 in Formula 1, respectively (Additional file 1 : Supplementary Text)
When there are ties with multiple entities having the same score, which happens more easily with classifiers that produce discrete scores, these entities together define only one anchor point (Fig. 1 f). There are again four common methods for connecting such an anchor point to the previous anchor point, which correspond to the four methods for connecting anchor points when there are no ties (details in Additional file 1 : Supplementary Text). The first method is to connect the two anchor points by a straight line [ 15 , 18 , 19 ] (Fig. 1 g). This method is known to easily produce overly-optimistic AUPRC values [ 2 , 24 ], which we will explain below. The second method is to interpolate additional points between the two anchor points using a non-linear function and then connect the points by straight lines [ 14 , 17 , 20 ] (Fig. 1 h). The interpolated points appear at their expected coordinates under the assumption that all possible orders of the entities with the same score have equal probability. The third method uses the same interpolation formula as the second method but instead of creating a finite number of interpolated points, it connects the two anchor points by a continuous curve [ 17 , 21 ] (Fig. 1 i). Finally, the fourth method comes naturally from the AP approach, which uses step curves to connect the anchor points [ 15 , 19 , 22 , 23 ] (Fig. 1 j).
Using the four methods to connect anchor points when there are no ties and the four methods when there are ties can lead to very different AUPRC values (Fig. 1 , Additional file 1 : Fig. S3 and Supplementary Text).
Conceptual and implementation issues of some popular software tools
We analyzed 10 tools commonly used to produce PRC and AUPRC (Additional file 1 : Table S1). Based on citations and keywords, we estimated that these tools have been used in more than 3000 published studies in total ( Methods ).
The 10 tools use different methods to connect anchor points on the PRC and therefore they can produce different AUPRC values (Table 1 , Additional file 1 : Fig. S4–S7 and Supplementary Text). As a comparison, all 10 tools can also compute AUROC, and we found most of them to produce identical values (Additional file 1 : Supplementary Text).
We found five conceptual issues with some of these tools when computing AUPRC values (Table 1 ):
➀ Using the linear interpolation method to handle ties, which can produce overly-optimistic AUPRC values [ 2 , 24 ]. When interpolating between two anchor points, linear interpolation produces higher AUPRC than the other three methods under conditions that can easily happen in real situations (Additional file 1 : Supplementary Text)
➁ Always using (0, 1) as the starting point of the PRC (procedurally produced or conceptually derived, same for ➂ and ➄ below), which is inconsistent with the concepts behind the AP and non-linear expectation methods when the first anchor point with a non-zero recall does not have a precision of one (Additional file 1 : Supplementary Text)
➂ Not producing a complete PRC that covers the full range of recall values from zero to one
➃ Ordering entities with the same classification score by their order in the input and then handling them as if they have distinct classification scores
➄ Not putting all anchor points on the PRC
These issues can lead to overly-optimistic AUPRC values or change the order of two AUPRC values (Additional file 1 : Supplementary Text and Fig. S8-S13).
Some of these tools also produce a visualization of the PRC. We found three types of issues with these visualizations (Table 1 ):
Producing a visualization of PRC that has the same issue(s) as in the calculation of AUPRC
Producing a PRC visualization that does not always start the curve at a point with zero recall
Producing a PRC visualization that always starts at (0, 1)

Inconsistent AUPRC values and contrasting classifier ranks produced by the popular tools
To see how the use of different methods by the 10 tools and their other issues affect PRC analysis in practice, we applied them to evaluate classifiers in four realistic scenarios.
In the first scenario, we analyzed data from a COVID-19 study [ 25 ] in which patient blood samples were subjected to Cellular Indexing of Transcriptomes and Epitopes by Sequencing (CITE-seq) assays [ 26 ]. We constructed a classifier for predicting CD4 \(^+\) T cells, which groups the cells based on their transcriptome data alone and assigns a single cell type label to each group. Using cell type labels defined by the original authors as reference, which were obtained using both antibody-derived tags (ADTs) and transcriptome data, we computed the AUPRC of the classifier. Figure 2 a shows that the 10 tools produced 6 different AUPRC values, ranging from 0.416 to 0.684. In line with the conceptual discussions above, the AP method generally produced the smallest AUPRC values while the linear interpolation method generally produced the largest, although individual issues of the tools created additional variations of the AUPRC values computed.
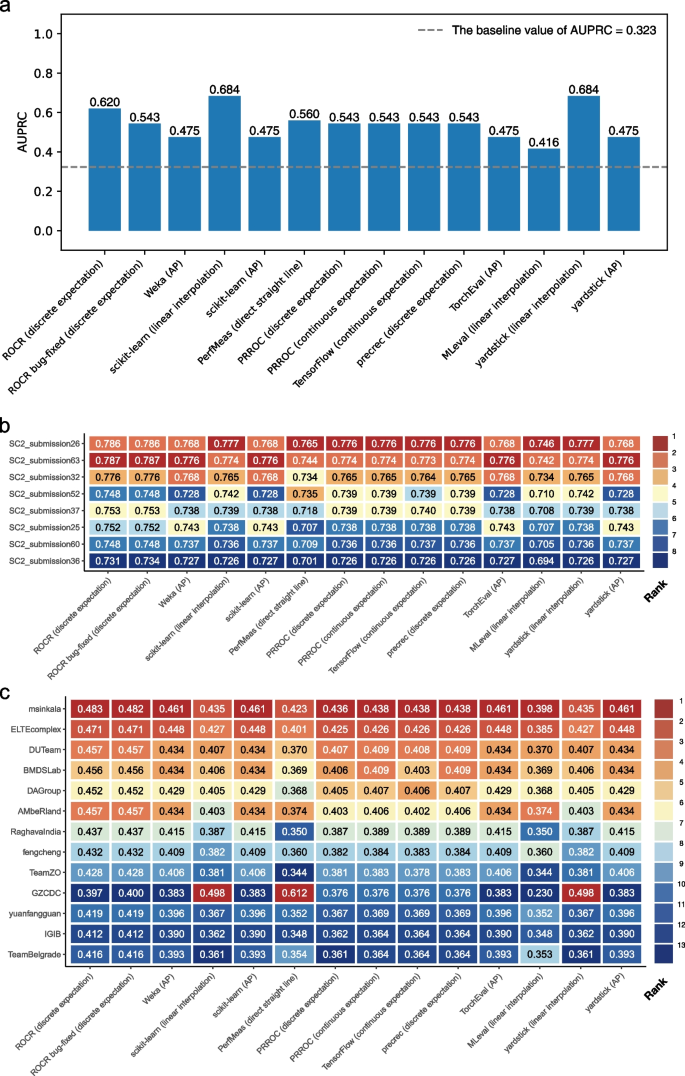
The AUPRC values computed by the 10 tools in several realistic scenarios. a Predicting CD4 \(^+\) T cells from single-cell transcriptomic data. b Predicting inflammatory bowel disease cases that belong to the ulcerative colitis subtype in the sbv IMPROVER Metagenomics Diagnosis for Inflammatory Bowel Disease Challenge. Only the top 8 submissions according to PRROC (discrete expectation) AUPRC values are included. c Predicting cases with preterm prelabor rupture of membranes in the DREAM Preterm Birth Prediction Challenge. In b and c , each entry shows the AUPRC value and the background color indicates its rank among the competitors
In the second scenario, we compared the performance of different classifiers that predict whether a patient has the ulcerative colitis (UC) subtype of inflammatory bowel disease (IBD) or does not have IBD, based on metagenomic data (processed taxonomy-based profile) [ 27 ]. The predictions made by these classifiers were submitted to the sbv IMPROVER Metagenomics Diagnosis for IBD Challenge. Their performance was determined by comparing against diagnosis of these patients based on clinical, endoscopic, and histological criteria. Figure 2 b shows that based on the AUPRC values computed, the 10 tools ranked the classifiers differently. For example, among the top 8 submissions with the highest performance, the classifier in submission 26 was ranked first in 8 cases, sole second place in 2 cases, and tied second place with another classifier in 4 cases (Fig. 2 b and Additional file 1 : Fig. S14). We observed similar rank flips when considering the top 30 submissions (Additional file 1 : Fig. S15 and S16).
In the third scenario, we compared the performance of different classifiers in identifying preterm prelabor rupture of the membranes (PPROM) cases from normal pregnancy in the DREAM Preterm Birth Prediction Challenge [ 28 ]. Based on the AUPRC values produced by the 10 tools, the 13 participating teams were ranked very differently (Fig. 2 c and Additional file 1 : Fig. S17). For example, Team “GZCDC” was ranked first (i.e., highest) in 3 cases, tenth in 4 cases, and thirteenth (i.e., lowest) in 7 cases. In addition to differences in the ranks, some of the AUPRC values themselves are also very different. For example, the AUPRC values computed by PerfMeas and MLeval have a Pearson correlation of − 0.759.
In the fourth scenario, we compared 29 classifiers that predicted target genes of transcription factors in the DREAM5 challenge [ 29 ]. Again, some classifiers received very different ranking based on the AUPRC values computed by the different tools (Additional file 1 : Fig. S18 and S19). For example, the classifier named “Other4” was ranked second based on the AUPRC values computed by PerfMeas but it was ranked twenty-fifth based on the AUPRC values computed by MLeval. In general, tools that use the discrete expectation, continuous expectation, and AP methods are in good agreements in this scenario, but they differ substantially from tools that use the linear interpolation method.
Conclusions
Due to their highly technical nature, it is easy to overlook the inconsistencies and issues of the software tools used for producing PRC and AUPRC. Some possible consequences include reporting overly-optimistic AUPRC, ranking classifiers differently by different tools, and introducing biases to the evaluation process, such as inflating the AUPRC of classifiers that produce discrete scores.
To address the problems, it is crucial to use tools that are free of the bugs described and avoid using the linear interpolation method (Table 1 ). It is also necessary to state clearly in manuscripts both the tool used (with its version number) and the underlying methods implemented by the tool for producing PRC and AUPRC. Whenever feasible, the adoption of multiple tools that implement different methods (e.g., one based on continuous expectation and one based on AP) is recommended, with comprehensive reporting of all their results.
Information about the tools
In this study, we included 12 tools commonly used for PRC and ROC analyses (Additional file 1 : Table S1). For each tool, we analyzed the latest stable version of it as of August 15, 2023. Because TorchEval had not released a stable version, we analyzed the latest version of it, version 0.0.6. Among the 12 tools, ten can compute both AUROC and AUPRC, while the remaining two can only compute AUROC. We focused on these 10 tools in the study of PRC and AUPRC. Some tools provide multiple methods for computing AUROC/AUPRC.
For tools with an associated publication, we obtained its citation count from Google Scholar. If a tool has multiple associated publications, we selected the one with the largest number of citations. As a result, the citation counts we report in Additional file 1 : Table S1 are underestimates if different publications associated with the same tool are not always cited together.
The Comprehensive R Archive Network (CRAN) packages PerfMeas and MLeval did not have an associated formal publication but only release notes. In each of these cases, we used the package name as keyword to search on Google Scholar and then manually checked the publications returned to determine the number of publications that cited these packages.
The CRAN package yardstick also did not have an associated formal publication. However, we were not able to use the same strategy as PerMeas and MLeval to determine the number of publications that cited the yardstick package since “yardstick” is an English word and the search returned too many publications to be verified manually. Therefore, we only counted the number of publications that cited yardstick’s release note, which is likely an underestimate of the number of publications that cited yardstick.
All citation counts were collected on October 9, 2023.
For tools with an associated formal publication, based on our collected lists of publications citing the tools, we further estimated the number of times the tools were actually used in the studies by performing keyword-based filtering. Specifically, if the main text or figure captions of a publication contains either one of the keywords “AUC” and “AUROC,” we assumed that the tool was used in that published study to perform ROC analysis. In the case of PRC, we performed filtering in two different ways and reported both sets of results in Additional file 1 : Table S1. In the first way, we assumed a tool was used in a published study if the main text or figure captions of the publication contains any one of the following keywords: “AUPR,” “AU-PR,” “AUPRC,” “AU-PRC,” “AUCPR,” “AUC-PR,” “PRAUC,” “PR-AUC,” “area under the precision recall,” and “area under precision recall.” In the second way, we assumed a tool was used in a published study if the main text or figure captions of the publication contains both “area under” and “precision recall.”
For the CRAN packages PerfMeas and MLeval, we estimated the number of published studies that actually used them by searching Google Scholar using the above three keyword sets each with the package name appended. We found that for all the publications we considered as using the packages in this way, they were also on our lists of publications that cite these packages. We used the same strategy to identify published studies that used the CRAN package yardstick. We found that some of these publications were not on our original list of publications that cite yardstick, and therefore we added them to the list and updated the citation count accordingly.
TorchEval was officially embedded into PyTorch in 2022. Due to its short history, among the publications that cite the PyTorch publication, we could not find any of them that used the TorchEval library.
Data collection and processing
We used four realistic scenarios to illustrate the issues of the AUPRC calculations.
In the first scenario, we downloaded CITE-seq data produced from COVID-19 patient blood samples by the COVID-19 Multi-Omic Blood ATlas (COMBAT) consortium [ 25 ]. We downloaded the data from Zenodo [ 30 ] and used the data in the “COMBAT-CITESeq-DATA” archive in this study. We then used a standard procedure to cluster the cells based on the transcriptome data and identified CD4 \(^+\) T cells. Specifically, we extracted the raw count matrix of the transcriptome data and ADT features (“X” object) and the annotation data frame (“obs” object) from the H5AD file. We dropped all ADT features (features with names starting with “AB-”) and put the transcriptome data along with the annotation data frame into Seurat (version 4.1.1). We then log-normalized the transcriptome data (method “NormalizeData(),” default parameters), identified highly-variable genes (method “FindVariableFeatures(),” number of variable genes set to 10,000), scaled the data (method “ScaleData(),” default parameters), performed principal component analysis (method “RunPCA(),” number of principal components set to 50), constructed the shared/k-nearest neighbor (SNN/kNN) graph (method “FindNeighbours(),” default parameters), and performed Louvain clustering of the cells (method “FindClusters(),” default parameters). We then extracted the clustering labels generated and concatenated them with cell type, major subtype, and minor subtype annotations provided by the original authors, which were manually curated using both ADT and transcriptome information.
Our procedure produced 29 clusters, which contained 836,148 cells in total. To mimic a classifier that predicts CD4 \(^+\) T cells using the transcriptome data alone, we selected one cluster and “predicted” all cells in it as CD4 \(^+\) T cells and all cells in the other 28 clusters as not, based on which we computed an AUPRC value by comparing these “predictions” with the original authors’ annotations. We repeated this process for each of the 29 clusters in turn, and chose the one that gave the highest AUPRC as the final cluster of predicted CD4 \(^+\) T cells.
For the second scenario, we obtained the data set used in the sbv IMPROVER (Systems Biology Verification combined with Industrial Methodology for PROcess VErification in Research) challenge on inflammatory bowel disease diagnosis based on metagenomics data [ 27 ]. The challenge involved 12 different tasks, and we focused on the task of identifying UC samples from non-IBD samples using the processed taxonomy-based profile as features. The data set contained 32 UC samples and 42 non-IBD samples, and therefore the baseline AUPRC was \(\frac{32}{32+42} = 0.432\) . There were 60 submissions in total, which used a variety of classifiers. We obtained the classification scores in the submissions from Supplementary Information 4 of the original publication [ 27 ]. When we extracted the classification scores of each submission, we put the actual positive entities before the actual negative entities. This ordering did not affect the AUPRC calculations of most tools except those of PerfMeas, which depend on the input order of the entities with the same classification score.
To see how the different tools rank the top submissions, we first computed the AUPRC of each submission using PRROC (option that uses the discrete expectation method to handle ties) since we did not find any issues with its AUPRC calculations (Table 1 ). We then analyzed the AUPRC values produced by the 10 tools based on either the top 8 (Fig. 2 b and Additional file 1 : Fig. S14) or top 30 (Additional file 1 : Fig. S15 and S16) submissions.
For the third scenario, we downloaded the data set used in the Dialogue on Reverse Engineering Assessment and Methods (DREAM) Preterm Birth Prediction Challenge [ 28 ] from https://www.synapse.org/#!Synapse:syn22127152 . We collected the classification scores, from the object “prpile” in each team’s RData file, and the actual classes produced based on clinical evidence, from “anoSC2_v21_withkey.RData” ( https://www.synapse.org/#!Synapse:syn22127343 ). The challenge contained 7 scenarios, each of which had 2 binary classification tasks. For each scenario, 10 different partitioning of the data into training and testing sets were provided. We focused on the task of identifying PPROM cases from the controls under the D2 scenario defined by the challenge. For this task, the baseline AUPRC value averaged across the 10 testing sets was 0.386. There were 13 participating teams in total. For each team, we extracted its classification scores and placed the actual positive entities before the actual negative entities. For submissions that contained negative classification scores, we re-scaled all the scores to the range between 0 and 1 without changing their order since TensorFlow expects all classification scores to be between zero and one (Additional file 1 : Supplementary Text). Finally, for each team, we computed its AUPRC using each of the 10 testing sets and reported their average. We note that the results we obtained by using PRROC (option that uses the continuous expectation method to handle ties) were identical to those reported by the challenge organizer.
For the fourth scenario, we obtained the data set used in the DREAM5 challenge on reconstructing transcription factor-target networks based on gene expression data [ 29 ]. The challenge included multiple networks and we focused on the E. coli in silico Network 1, which has a structure that corresponds to the real E. coli transcriptional regulatory network [ 29 ]. We obtained the data from Supplementary Data of the original publication [ 29 ]. There were 29 submissions in total. For each submission, we extracted the classification scores of the predicted node pairs (each pair involves one potential transcription factor and one gene it potentially regulates) from Supplementary Data 3 and compared them with the actual classes (positive if the transcription factor actually regulates the gene; negative if not) in the gold-standard network from Supplementary Data 1. Both the submissions and the gold-standard were not required to include all node pairs. To handle this, we excluded all node pairs in a submission that were not included in the gold-standard (because we could not judge whether they are actual positives or actual negatives), and assigned a classification score of 0 to all node pairs in the gold-standard that were not included in a submission (because the submission did not give a classification score to them). The gold-standard contained 4012 interacting node pairs and 274,380 non-interacting node pairs, and therefore the baseline AUPRC value was \(\frac{4012}{4012+274380} = 0.014\) .
Availability of data and materials
Our code is written in Java, Python and R. The reproducible code and all the data used in this paper are available at GitHub [ 31 ] and Zenodo [ 32 ].
Saito T, Rehmsmeier M. The precision-recall plot is more informative than the ROC plot when evaluating binary classifiers on imbalanced datasets. PLoS ONE. 2015;10(3):e0118432.
Article PubMed PubMed Central Google Scholar
Davis J, Goadrich M. The relationship between precision-recall and ROC curves. In: Proceedings of the 23rd International Conference on Machine Learning. New York: Association for Computing Machinery; 2006. p. 233–40.
He H, Garcia EA. Learning from imbalanced data. IEEE Trans Knowl Data Eng. 2009;21(9):1263–84.
Article Google Scholar
Lichtnwalter R, Chawla NV. Link prediction: fair and effective evaluation. In: 2012 IEEE/ACM International Conference on Advances in Social Networks Analysis and Mining. New York: IEEE; 2012. p. 376–83.
Cao Q, Anyansi C, Hu X, Xu L, Xiong L, Tang W, et al. Reconstruction of enhancer-target networks in 935 samples of human primary cells, tissues and cell lines. Nat Genet. 2017;49(10):1428–36.
Article CAS PubMed Google Scholar
Schulte-Sasse R, Budach S, Hnisz D, Marsico A. Integration of multiomics data with graph convolutional networks to identify new cancer genes and their associated molecular mechanisms. Nat Mach Intell. 2021;3(6):513–26.
Hong C, Cao Q, Zhang Z, Tsui SKW, Yip KY. Reusability report: Capturing properties of biological objects and their relationships using graph neural networks. Nat Mach Intell. 2022;4(3):222–6.
Sielemann J, Wulf D, Schmidt R, Bräutigam A. Local DNA shape is a general principle of transcription factor binding specificity in Arabidopsis thaliana. Nat Commun. 2021;12(1):6549.
Article CAS PubMed PubMed Central Google Scholar
Li Z, Kuppe C, Ziegler S, Cheng M, Kabgani N, Menzel S, et al. Chromatin-accessibility estimation from single-cell ATAC-Seq data with scOpen. Nat Commun. 2021;12(1):6386.
Chowell D, Yoo SK, Valero C, Pastore A, Krishna C, Lee M, et al. Improved prediction of immune checkpoint blockade efficacy across multiple cancer types. Nat Biotechnol. 2022;40(4):499–506.
Dal Molin A, Baruzzo G, Di Camillo B. Single-cell RNA-sequencing: assessment of differential expression analysis methods. Front Genet. 2017;8:62.
Pratapa A, Jalihal AP, Law JN, Bharadwaj A, Murali T. Benchmarking algorithms for gene regulatory network inference from single-cell transcriptomic data. Nat Methods. 2020;17(2):147–54.
Dimitrov D, Türei D, Garrido-Rodriguez M, Burmedi PL, Nagai JS, Boys C, et al. Comparison of methods and resources for cell-cell communication inference from single-cell RNA-Seq data. Nat Commun. 2022;13(1):3224.
Sing T, Sander O, Beerenwinkel N, Lengauer T. ROCR: visualizing classifier performance in R. Bioinformatics. 2005;21(20):3940–1.
Pedregosa F, Varoquaux G, Gramfort A, Michel V, Thirion B, Grisel O, et al. Scikit-learn: machine learning in Python. J Mach Learn Res. 2011;12:2825–30.
Google Scholar
Valentini G, Re M. PerfMeas: performance measures for ranking and classification tasks. R package. 2014. https://cran.r-project.org/web/packages/PerfMeas .
Grau J, Grosse I, Keilwagen J. PRROC: computing and visualizing precision-recall and receiver operating characteristic curves in R. Bioinformatics. 2015;31(15):2595–7.
John C. MLeval: machine learning model evaluation. R package. 2020. https://cran.r-project.org/web/packages/MLeval .
Kuhn M, Vaughan D. yardstick: tidy characterizations of model performance. R package. 2021. https://cran.r-project.org/web/packages/yardstick .
Saito T, Rehmsmeier M. Precrec: fast and accurate precision-recall and ROC curve calculations in R. Bioinformatics. 2017;33(1):145–7.
Abadi M, Agarwal A, Barham P, Brevdo E, Chen Z, Citro C, et al. TensorFlow: large-scale machine learning on heterogeneous distributed systems. arXiv preprint arXiv:160304467. 2016. https://arxiv.org/abs/1603.04467 .
Hall M, Frank E, Holmes G, Pfahringer B, Reutemann P, Witten IH. The WEKA data mining software: an update. ACM SIGKDD Explor Newsl. 2009;11(1):10–8.
Paszke A, Gross S, Massa F, Lerer A, Bradbury J, Chanan G, et al. PyTorch: an imperative style, high-performance deep learning library. Adv Neural Inf Process Syst. 2019;32:8026–37.
Flach P, Kull M. Precision-recall-gain curves: PR analysis done right. Adv Neural Inf Process Syst. 2015;28:838–46.
COvid-19 Multi-omics Blood ATlas (COMBAT) Consortium. A blood atlas of COVID-19 defines hallmarks of disease severity and specificity. Cell. 2022;185(5):916–938.e58.
Stoeckius M, Hafemeister C, Stephenson W, Houck-Loomis B, Chattopadhyay PK, Swerdlow H, et al. Simultaneous epitope and transcriptome measurement in single cells. Nat Methods. 2017;14(9):865–8.
Khachatryan L, Xiang Y, Ivanov A, Glaab E, Graham G, Granata I, et al. Results and lessons learned from the sbv IMPROVER metagenomics diagnostics for inflammatory bowel disease challenge. Sci Rep. 2023;13:6303.
Tarca AL, Pataki BÁ, Romero R, Sirota M, Guan Y, Kutum R, et al. Crowdsourcing assessment of maternal blood multi-omics for predicting gestational age and preterm birth. Cell Rep Med. 2021;2(6):100323.
Marbach D, Costello JC, Küffner R, Vega NM, Prill RJ, Camacho DM, et al. Wisdom of crowds for robust gene network inference. Nat Methods. 2012;9(8):796–804.
COvid-19 Multi-omics Blood ATlas (COMBAT) Consortium. A blood atlas of COVID-19 defines hallmarks of disease severity and specificity: Associated data. Zenodo. 2022. https://doi.org/10.5281/zenodo.6120249 .
Chen W, Miao C, Zhang Z, Fung CSH, Wang R, Chen Y, et al. Commonly used software tools produce conflicting and overly-optimistic AUPRC values. GitHub. 2024. https://github.com/wychencuhk/AUPRC_project .
Chen W, Miao C, Zhang Z, Fung CSH, Wang R, Chen Y, et al. Commonly used software tools produce conflicting and overly-optimistic AUPRC values. Zenodo. 2024. https://doi.org/10.5281/zenodo.11076192 .
Download references
Review history
The review history is available as Additional file 2 .
Peer review information
Andrew Cosgrove was the primary editor of this article and managed its editorial process and peer review in collaboration with the rest of the editorial team.
QC is supported by National Natural Science Foundation of China under Award Number 32100515. YQ is supported by Guangdong Basic and Applied Basic Research Foundation under Award Number 2021A1515110759 and Natural Science Foundation of Guangdong Province of China under Award Number 2023A1515011187. LC is supported by National Natural Science Foundation of China under Award Number 32370711 and Shenzhen Medical Research Fund under Award Number A2303033. KYY is supported by National Cancer Institute of the National Institutes of Health under Award Number P30CA030199, National Institute on Aging of the National Institutes of Health under Award Numbers U54AG079758 and R01AG085498, and internal grants of Sanford Burnham Prebys Medical Discovery Institute. The content is solely the responsibility of the authors and does not necessarily represent the official views of the National Institutes of Health.
Author information
Wenyu Chen and Chen Miao are co-first authors.
Authors and Affiliations
School of Biomedical Sciences, The Chinese University of Hong Kong, Shatin, New Territories, Hong Kong SAR, China
Wenyu Chen, Chen Miao, Cathy Sin-Hang Fung, Ran Wang, Stephen Kwok-Wing Tsui & Qin Cao
Department of Computer Science and Engineering, The Chinese University of Hong Kong, Shatin, New Territories, Hong Kong SAR, China
Zhenghao Zhang, Yizhen Chen & Kevin Y. Yip
The First Affiliated Hospital, Sun Yat-sen University, Guangzhou, China
Shenzhen People’s Hospital, First Affiliated Hospital of Southern University of Science and Technology, Second Clinical Medical College of Jinan University, Shenzhen, China
Lixin Cheng
Sanford Burnham Prebys Medical Discovery Institute, La Jolla, CA, USA
Kevin Y. Yip
Hong Kong Bioinformatics Centre, The Chinese University of Hong Kong, Shatin, New Territories, Hong Kong SAR, China
Stephen Kwok-Wing Tsui & Qin Cao
Shenzhen Research Institute, The Chinese University of Hong Kong, Shenzhen, China
You can also search for this author in PubMed Google Scholar
Contributions
KYY, SKWT, and QC conceived and supervised the project. WC, CM, KYY, and QC designed the computational experiments and data analyses. WC, CM, and YC surveyed and collected the tools. WC, CM, ZZ, CSHF, and RW prepared the data. WC and CM conducted the computational experiments. WC, CM, ZZ, and RW performed the data analyses. All the authors interpreted the results. WC, CM, KYY, and QC wrote the manuscript.
Corresponding authors
Correspondence to Kevin Y. Yip , Stephen Kwok-Wing Tsui or Qin Cao .
Ethics declarations
Ethics approval and consent to participate.
No ethical approval was required for this study. All utilized public datasets were generated by other organizations that obtained ethical approval.
Competing interests
The authors declare that they have no competing interests.
Additional information
Publisher's note.
Springer Nature remains neutral with regard to jurisdictional claims in published maps and institutional affiliations.
Supplementary Information
Additional file 1. supplementary text, figures and tables., additional file 2. review history., rights and permissions.
Open Access This article is licensed under a Creative Commons Attribution 4.0 International License, which permits use, sharing, adaptation, distribution and reproduction in any medium or format, as long as you give appropriate credit to the original author(s) and the source, provide a link to the Creative Commons licence, and indicate if changes were made. The images or other third party material in this article are included in the article's Creative Commons licence, unless indicated otherwise in a credit line to the material. If material is not included in the article's Creative Commons licence and your intended use is not permitted by statutory regulation or exceeds the permitted use, you will need to obtain permission directly from the copyright holder. To view a copy of this licence, visit http://creativecommons.org/licenses/by/4.0/ . The Creative Commons Public Domain Dedication waiver ( http://creativecommons.org/publicdomain/zero/1.0/ ) applies to the data made available in this article, unless otherwise stated in a credit line to the data.
Reprints and permissions
About this article
Cite this article.
Chen, W., Miao, C., Zhang, Z. et al. Commonly used software tools produce conflicting and overly-optimistic AUPRC values. Genome Biol 25 , 118 (2024). https://doi.org/10.1186/s13059-024-03266-y
Download citation
Received : 07 February 2024
Accepted : 30 April 2024
Published : 13 May 2024
DOI : https://doi.org/10.1186/s13059-024-03266-y
Share this article
Anyone you share the following link with will be able to read this content:
Sorry, a shareable link is not currently available for this article.
Provided by the Springer Nature SharedIt content-sharing initiative
Genome Biology
ISSN: 1474-760X
- Submission enquiries: [email protected]
- General enquiries: [email protected]
Copy-on-Write performance and debugging
Erik Mavrinac
May 14th, 2024 0 0
This is a follow-up to our previous coverage of Dev Drive and copy-on-write (CoW) linking. See our previous articles from May 24, 2023 , October 13, 2023 , and November 2, 2023 .
Dev Drive was released in Windows 11 in October, 2023, and will be part of Windows Server 2025 this fall, along with an enhancement to automatically use copy-on-write linking (CoW-in-Win32). Here, we’ll cover the results of several months of repo build performance testing for several large internal codebases, provide some information on determining whether a file is a CoW link, and share a few tips we found from adding Dev Drive to thousands of Dev Box VMs for daily developer use.
Repo build performance
Let’s start with the chart:
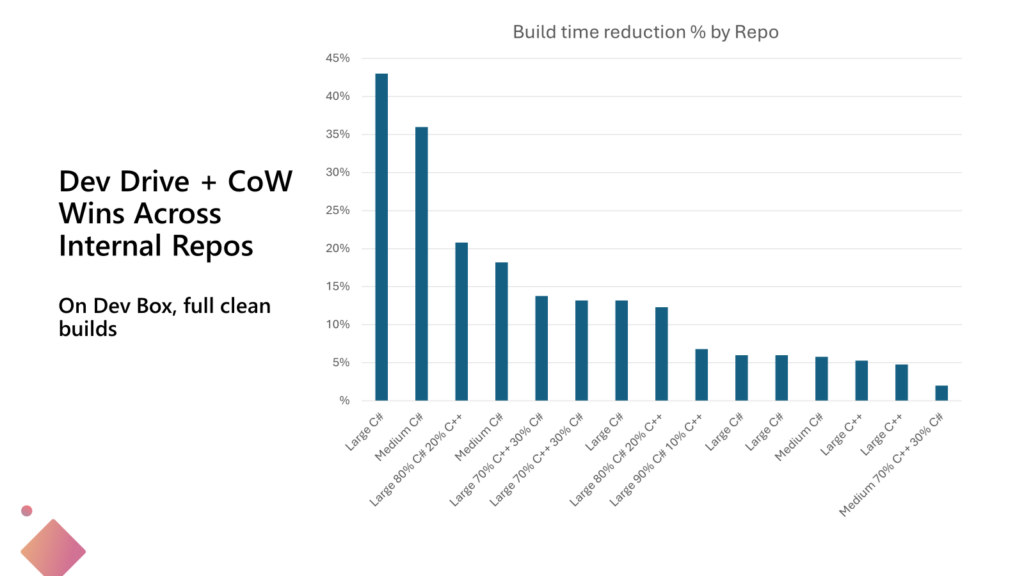
The highest win of 43% did not replicate for all the repos under test. However, many repos did get a reduction of 10% or more. Several patterns stand out when comparing to the underlying repo code:
- Repos containing C# with deep project-to-project dependencies cause MSBuild to copy assemblies many times. These can get a significant benefit from CoW linking.
- Repos that perform lots of additional copying to create microservice layouts as part of the build output also get a strong benefit.
- Repos heavy in C++ showed only a small win except where they were copying files for microservice layouts. C++ builds in MSBuild do not by default copy output files over and over again, and MSVC tends to generate fewer, larger files, where Dev Drive’s reduced file I/O overhead is less effective.
- Two repos with low benefit had a project dependency graph with a lot of initial parallelism that was noticeably faster but with a near-linear chain of large projects at the end that reduced the effect of speeding up I/O in each project. 1
Test methodology notes: Tests for reach repo were run on NTFS and Dev Drive partitions on the same Dev Box VM. NuGet and other package caches were placed in the same partition as the source code. All repos used the Microsoft.Build.CopyOnWrite SDK and, where applicable, an upgraded Microsoft.Build.Artifacts SDK. CoW-in-Win32 was not available at the time of testing, and may produce different results when released this fall. Five or more iterations were run per test case, with the first one dropped to avoid measuring a cold disk cache. Measurements were of the build phase only, with package restore and inline tests separated out. All builds were run with clean repo and output directories to ensure a full build. Typical build times per iteration were selected to be about 20 minutes, which for large repos usually meant building a specific subdirectory.
How to determine whether a file is a CoW link
CoW links are also known as block clones , where blocks of data on disk are referred-to from multiple file entries. fsutil contains subcommands that let us view files from a block clone point of view. Let’s take a look at a block clone of an assembly copied from a package to my MSBuild output directory:
This shows that there are 97 clusters corresponding to the main 400K body of the assembly. Note the Ref: 0x4 meaning the underlying block at Logical Cluster Number 0x1297cf7 has 4 block clones on the disk volume, of which this is one. The last cluster with one reference holds the block clone reference metadata, which means for every cloned file there is one cluster actually used for tracking purposes.
Using ProcMon with Dev Drive
ProcMon uses an included filter driver whose name, e.g. ProcMon24 , changes over time. Attach the filter driver like:
You can generally leave ProcMon24 in the allow list, as it is only attached to the volume when ProcMon is in use. Our internal Dev Box images are generated with the filter always added.
Using Microsoft Performance Recorder (Xperf) with Dev Drive
Attach the FileInfo filter driver:
Then measure. After measurement, it’s important to disable FileInfo as it is always attached to the Dev Drive when allowed, slowing performance.
Finding and fixing leaked CoW references
Dev Drive, which is based on ReFS, allows only 8176 clones of a data block. If you have a file that fails to copy because of an error related to too many clones, e.g. MaxCloneFileLinksExceededException from the CoW library used in the Microsoft.Build.CopyOnWrite and Microsoft.Build.Artifacts SDKs, winerror ERROR_BLOCK_TOO_MANY_REFERENCES = 347, or NTSTATUS STATUS_BLOCK_TOO_MANY_REFERENCES = 0xC000048C, you might have too many actual references, or you might need to clean up orphaned references. We ran into this problem on one machine that had run continuous CoW builds for weeks under a prerelease CoW-in-Win32 implementation, so we don’t expect this to appear in the wild very often.
In an elevated console or PowerShell run the following, where x: is the drive letter of your Dev Drive.
This will scan and fix dangling references. You can add the /d parameter to detect but not fix these references.
Example output from a volume with a significant number of orphaned references:
Copy-on-write will be on by default for Dev Drive in the 24H2 Windows operating system release wave. Dev Drive and CoW will be available in the Server SKU for the first time starting in Server 2025 later this year. These releases will make many builds on Windows notably faster, particularly C# builds. CoW-in-Win32 will avoid the need to integrate the CoW SDKs or modify other build engines or tools.
In the intervening months, consider integrating the CopyOnWrite SDK into your MSBuild repo and creating a Dev Drive partition on your development machine.
We hope you find your build performance notably faster!
We recommended that the repo owners try ReferenceTrimmer to see if any parallelism could be recovered by removing unneeded project dependencies. ↩
Erik Mavrinac Principal Software Engineer, 1ES Engineering

Leave a comment Cancel reply
Log in to start the discussion.

Insert/edit link
Enter the destination URL
Or link to existing content
More From Forbes
Is per-seat licensing sabotaging your software's potential.
- Share to Facebook
- Share to Twitter
- Share to Linkedin
LigoLab Cofounder and Chief Executive Officer.
These days, businesses are no longer as willing to accept the status quo in their relationships with technology companies. Instead, there’s a movement underway where enterprises actively seek partners that align with their business goals of driving substantial and measurable outcomes.
For a good example of this shift, let’s look at the traditional per-seat software license model in which a business is charged a fixed fee for each user to access the software. Although uncomplicated, predictable and still quite common for enterprise and mission-critical software systems, the per-seat model has increasingly been perceived as outdated and problematic compared to the performance-based license model because it fails to align the software provider’s incentives with the needs of the business.
In this article, I make the case for why forward-thinking decision-makers should consider shifting toward performance-based software licensing where cost is ultimately reflected in usage, and why this shift is essential if enterprises are to incorporate automation to mitigate staffing challenges and rising wages.
The Pitfalls Of Per-Seat Licensing
Let’s start by taking a closer look at why software companies are more than happy to offer the per-seat licensing model to their customers. At the top of the list is revenue, or more specifically, a predictable revenue stream based on the number of licenses sold. By tying revenue to the number of individual users, the software company benefits financially every time the customer needs to add a seat because of business growth.
Fallout Dethroned In Amazon Prime Video s Top 10 List By A New Offering
Anya taylor joy messi and more grace the cannes film festival 2024 red carpet, televisaunivision 2024-25 slate touts latino culture, vix growth, juanpa zurita, william levy deals.
Additionally, the software company benefits from the per-seat revenue stream regardless of usage. For example, let’s say your business has five employees who need to access the software system as part of their roles with the company. Four of the five use the system intermittently, while the fifth person uses the system all day, every day of the work week. In this scenario, the business still needs to purchase five seats even though four employees are offline and not using the system most of the time.
Now, let’s examine the negative effects the per-seat license arrangement can have on your business.
Misaligned Incentives
Unfortunately, the core incentive structure of per-seat licensing can financially motivate software companies to increase the number of individual licenses sold over enhancing their software efficiency or automation capabilities. Furthermore, this misalignment can suppress innovation in ways that reduce the need for human intervention and streamlined operations.
Cost Inefficiency
In mission-critical systems where reliability and efficiency are paramount, per-seat fees can also lead to excessive costs without the corresponding corporate benefits. As companies scale, the cost of additional licenses often becomes a significant financial burden, necessitating the diversion of funds from potential investments in innovation.
Limited Scalability And Flexibility
Per-seat licensing restricts flexibility and scalability, two crucial areas for enterprises facing variable workloads and fluctuating user counts. It can force companies to purchase extra seats to accommodate peak periods, even if those seats remain unused most of the time, resulting in wasted resources.
High Upfront Costs And Lowered Service Quality
Adopting a per-seat licensing model often involves significant upfront payments, making it a capital-intensive choice. Once these fees are paid, the business is largely at the vendor's discretion for support and services. Additionally, vendors might be tempted to impose extra charges for essential services, exploiting the financial commitment already made by the client.
The Case For Performance-Based Licensing
Now, let’s turn to performance-based licensing to see why I believe it’s the better alternative for enterprise organizations. I’ll make my case by again focusing on alignment, cost, innovation and flexibility, but this time from the businesses’ perspective.
Alignment With Business Outcomes
Performance-based licensing models tie software costs to the results delivered, such as increased productivity, revenue growth or cost savings. This incentivizes software companies to focus on developing features that contribute directly to their customers’ success.
Cost Transparency
Performance-based licensing offers greater transparency in software costs because pricing is based on measurable informatics rather than blurred pricing structures that only benefit the provider. This transparency helps enterprise companies better understand the value proposition offered by the software and make informed decisions about its usage.
Encouragement Of Innovation And Automation
Shifting the focus to outcomes encourages software providers to maximize automation and reduce the need for continuous human input, a topic especially relevant in today’s business climate as staffing issues and rising wages persist.
Adaptability To Changing Business Needs
A performance-based software license offers greater flexibility, allowing companies to adjust their usage and costs according to their actual business performance and immediate needs. This adaptability is crucial in a dynamic business environment.
Seek Partnership
I believe the shift toward performance-based licensing is beneficial and necessary for modern enterprises because it promotes a better alignment of incentives and encourages software companies to focus on delivering genuine value through innovation and automation. Paying for a per-seat software license rather than a performance-based license mostly leads to a sacrifice in other areas, namely business efficiency and cost-saving automation.
Purchasing a software license is like buying a house, except the partnership and control offered to the business in the performance-based model serve as the foundation needed to turn that house into a hotel.
Your business might only need a house today, but choosing and using the right software licensing model can allow it to grow and evolve to a point where a hotel is required. With the performance-based model, the software company is the one that invests in the build-out of the hotel and your business is only charged based on the number of people checked into the hotel.
On the other side, enterprises that adopt the performance-based model can benefit from far fewer barriers (financial and operational), and they can become more competitive, agile and cost-efficient as they position themselves as leaders in the digital age. I believe that per-seat pricing is dead; long live value-based pricing.
Forbes Business Council is the foremost growth and networking organization for business owners and leaders. Do I qualify?
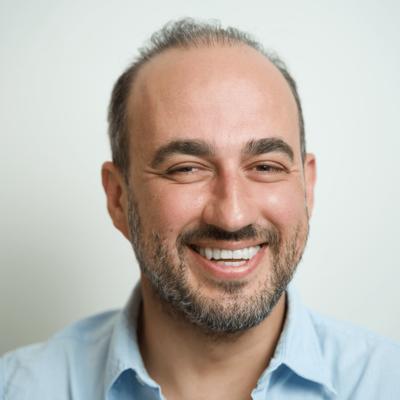
- Editorial Standards
- Reprints & Permissions
You are using an outdated browser. Please upgrade your browser to improve your experience.

UPDATED 15:32 EDT / MAY 15 2024
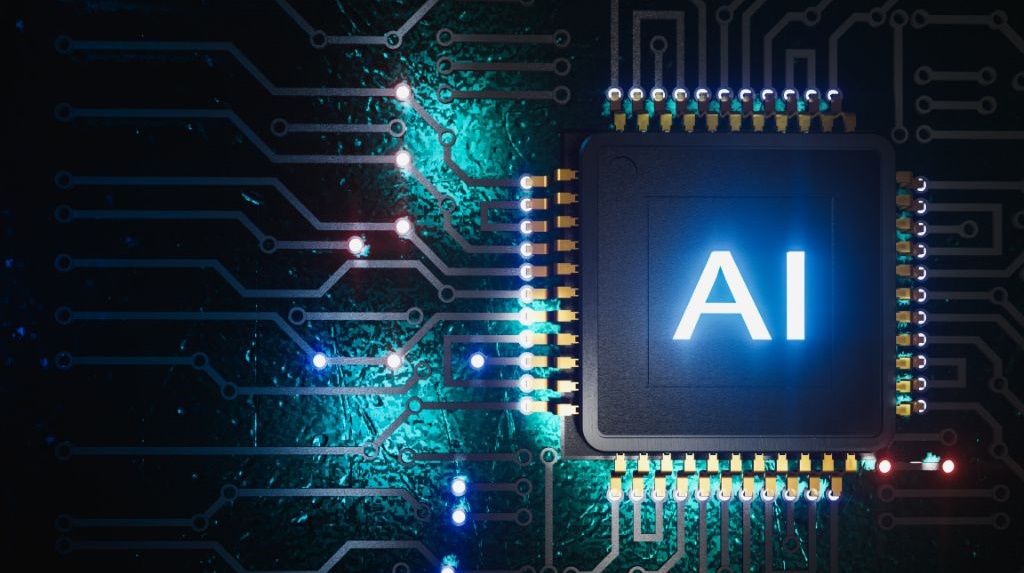
Creating timeless software: How Software AG has remained relevant in the new age of AI

by Mark Albertson
The year Software AG was founded provided two watershed moments in the future growth and direction of the technology industry.
It was 1969, and IBM Corp. announced in June that it would begin pricing its software and services separately from its hardware, laying the groundwork for an emergence of the modern software consulting industry. One month later, U.S. astronauts walked on the moon , a validation that technology could achieve what was once unthinkable.
Software AG, started that year in Germany by six young employees of the Institute of Applied Information Processing, has emerged as a multinational software business that provides enterprise tools for business process management, integration and big data analytics. For more than five and a half decades, the company has shown that it can adapt to the shifting tides of an industry that never stands still.
“Software AG continues to remain relevant by modernizing its legacy technology while moving into new growth areas,” said Paul Gillin , research analyst for theCUBE, SiliconANGLE Media’s livestreaming studio. “The company’s Adabas database management system and Natural development language still have large installed bases, but the company’s growth has shifted to Aris business process management software, which, incidentally, has also been around for more than three decades but is just as relevant today as ever.”
Gillin was on the ground in Dublin, Ireland, for Software AG’s International User Groups Conference in April and spoke with key executives from the company and customers in exclusive interviews about the latest product enhancements for data integration, process management, artificial intelligence and internet of things orchestration for enterprises around the world. (* Disclosure below.)
Software AG Aris plays central role in business optimization
Software AG’s lengthy history in the process management arena has given the company a different perspective on software and its role in enabling enterprise applications. The firm invented its flagship Aris product in 1992, and Aris grew to become a tool used by enterprises for the documentation, design, analysis, assessment and optimization of key processes for running a business.
“When you talk about legacy, I talk about timeless software,” said Stefan Sigg , chief product officer of Software AG, during his conversation with theCUBE . “When software has been around a long time, it becomes timeless and it doesn’t matter anymore how old it is. My favorite example is Microsoft Excel; it’s been around for almost 40 years, but nobody is calling Excel a legacy. Nobody calls Software AG’s Aris legacy. It’s there to keep all those super mission-critical custom applications that were built on top of this technology running because they carry the company. That is what we are doing.”
During the International User Groups Conference in Dublin, Software AG enhanced its Aris process mining and Alfabet portfolio management products with generative AI. By leveraging Microsoft Corp.’s Azure OpenAI, Software AG is now providing the capability to depict existing processes and identify anomalies through the use of graphic formats.
“We continue to innovate … we can use AI to make Aris much more productive where you don’t have to dig into the data so much, but the AI is telling you what’s wrong,” Sigg said. “We mine the data, reconstruct the business processes and have key performance indicators attached to each and every instance. AI creates the summary without a human being needing to spend hours and hours analyzing each process instance.”
Earlier this year, Software AG released a study that identified a common challenge fueled by rapid expansion of technology within many enterprises. Results showed that 89% of organizations rapidly expanded technology over the course of several years, and more than three quarters or 76% indicated that this resulted in increased chaos to be managed. Over 40% pointed to the challenge of managing legacy and new systems together as a key factor in heightened complexity.
“The cloud is promising to make everything easier, but at least in an intermediate time, it’s making things much more complex because no company on this planet is making a 100% shift from being on-premises one day to the cloud,” Sigg explained. “Then there are SaaS services running somewhere and maybe edge cases where I have infrastructure and software running on a factory shop floor. These are four different things that I have to integrate. That is building complexity that you cannot avoid. It’s a question of managing that complexity with state-of-the-art integration software, IoT software, process management software and IT portfolio management software.”
Here’s theCUBE’s complete video interview with Stefan Sigg:
Use cases highlight support for migration and strategic planning
Migration of IT systems to a new platform is not only a complex process, it can also cripple a business dependent on enterprise resource planning and management functions necessary for smooth operation. Add on the sudden impact of a worldwide global COVID-19 pandemic, and this is the scenario that one Software AG customer found itself facing in 2020.
Celulose Nipo-Brasileira S.A., or Cenibra, is a major Brazilian producer of bleached eucalyptus pulp used for making paper and cardboard products. The company designed a plan to upgrade its legacy ERP system to SAP S/4HANA as a key step toward a modern IT infrastructure. Yet with the entire migration team having to work remotely when the upgrade began during the pandemic, the challenges were significant, according to Ronaldo Neves Ribeiro , IT and telecom manager at Cenibra, in his interview on theCUBE .
“It was a huge problem,” Ribeiro said. “We’d never managed projects remotely, but the directors decided to continue. It was planned and completed in 13 months with 178 people. It was the first S/4HANA migration in the world done completely remotely.”
Here’s theCUBE’s complete video interview with Ronaldo Neves Ribeiro:
Announcements made during the conference included news that Software AG would enhance Alfabet 11 , the company’s portfolio of management products, to provide process mining functionality for the IT landscape. New features included a Smart Data Workbench for customizing information displays, Data Quality rules that set parameters for acceptable quality and the application of reasoning-based AI to reduce product configuration effort.
The latest enhancements for Alfabet offered the British financial advisory company St. James Place PLC an opportunity to fine-tune its planning strategy.
“What we find that Alfabet brings for us is it has the ability for us to capture those high-level corporate strategic goals,” said Ian Batty , head of the Office of Architecture at St. James Place, in conversation with theCUBE . “Alfabet supports two distinct areas of usage, which is the enterprise architecture tool and the strategic portfolio management. I’m particularly focused on strategic portfolio management, and that means identifying where to spend your money, how much money you’ve got, what are the priorities you’ve got as a company.”
Here’s theCUBE’s complete video interview with Ian Batty:
Growing complexity of IT environments has fueled interest in Software AG and its portfolio of process management solutions. The company was the subject of acquisition interest last year when the private equity firm Silver Lake competed with Rocket Software Inc. for a majority stake. Silver Lake prevailed and took the company private last fall. Since then, Software AG has sold its StreamSets and webMethods business to IBM Corp.
While Silver Lake has taken an approach to sell off some of Software AG’s assets, the company’s core business remains the same. The firm’s business process management software continues to attract major clients, a sign that despite the rapid pace of change today, enterprises will always need to optimize functions to remain successful.
“Processes are really at the heart of the organization, it’s the DNA of the organization,” said Josèphe Blondaut , head of ARIS product marketing at Software AG, during an interview with theCUBE . “It’s really about learning to learn, creating a culture of optimization and a culture of overall thinking, not thinking only in the specific silo you are working in. In the end, a company that looks at the processes, that looks at the operation will gain some kind of a learning success.”
Here’s theCUBE’s complete video interview with Josèphe Blondaut:
To watch more of theCUBE’s coverage of the International User Groups Conference , here’s our complete event video playlist:
Watch theCUBE’s full coverage of the International User Groups Conference event here:
(* Disclosure: TheCUBE is a paid media partner for the International User Groups Conference. Neither Software AG, the sponsor of theCUBE’s event coverage, nor other sponsors have editorial control over content on theCUBE or SiliconANGLE.)
Image: KENGKAT / Getty Images
A message from john furrier, co-founder of siliconangle:, your vote of support is important to us and it helps us keep the content free., one click below supports our mission to provide free, deep, and relevant content. , join our community on youtube, join the community that includes more than 15,000 #cubealumni experts, including amazon.com ceo andy jassy, dell technologies founder and ceo michael dell, intel ceo pat gelsinger, and many more luminaries and experts..
Like Free Content? Subscribe to follow.
LATEST STORIES
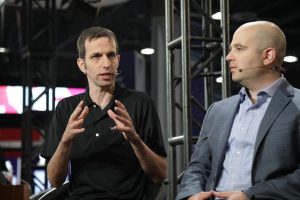
Data management startup Weka nabs $140M at $1.6B valuation
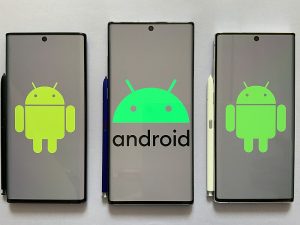
Google releases second Android 15 beta alongside broader ecosystem updates
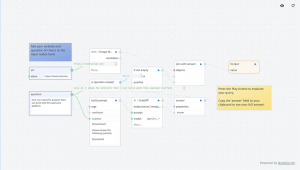
Ubio launches NodeScript low-code visual toolset for data workflow automation
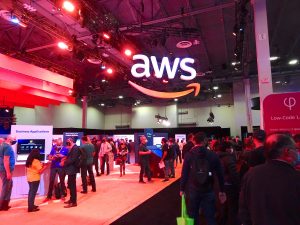
At RSA Conference, AWS brings updates to Amazon Security Lake
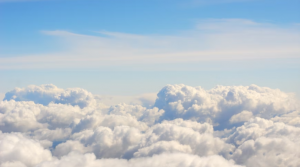
Alkira reels in $100M for its cloud networking platform
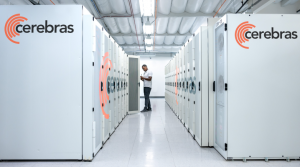
AI chip company Cerebras announces major advances in materials science, sparse training and more
INFRA - BY MARIA DEUTSCHER . 48 MINS AGO
APPS - BY KYT DOTSON . 3 HOURS AGO
APPS - BY KYT DOTSON . 4 HOURS AGO
SECURITY - BY ZEUS KERRAVALA . 4 HOURS AGO
CLOUD - BY MARIA DEUTSCHER . 6 HOURS AGO
AI - BY MIKE WHEATLEY . 7 HOURS AGO
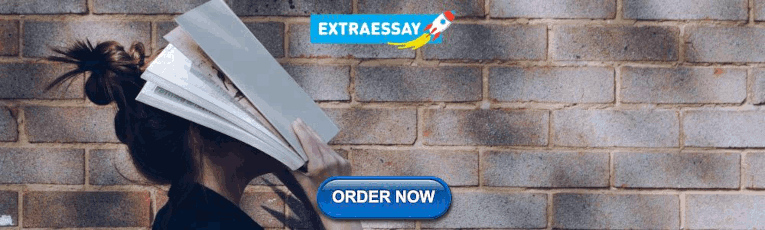
IMAGES
VIDEO
COMMENTS
In summary, the very first software or app or tool every PhD student is required is Mendeley to write a thesis. It's a citation and reference manager tool. Evernote "Notes" as writing, images, pdf, article, news paragraph is an everyday part of a PhD student. Whatever you read, whenever you find things related to your PhD topic, you have ...
1. Reference Management Software. Comprehensive reference management software, such as Zotero, Mendeley, or EndNote, is a must-have tool for any thesis writer. These tools help you organize and manage your references efficiently, generate citations in different formats, and create a bibliography effortlessly.
This post elaborates on several tools commonly utilized for academic thesis writing. 1. Citavi. Citavi is a comprehensive reference management and knowledge organization tool. It allows you to manage references, create citations, and organize your research materials. Citavi is commonly used by students and researchers. 2.
impact of accounting software characteristics on business performance of firms in. Malaysia as 0.711 and adjusted R Square 0.691, indicating that 69.1% of the. variance of accounting software ...
thesis. We introduce the concept of software performance in section 1.1, then we elaborate on the existing related work (section 1.2) and why it is important to observe parametric dependencies (section 1.3). In section 1.4 it is explained why existing related works are not optimal and ˙nally in section 1.5 the goals of the thesis are described ...
Completing your dissertation or thesis requires a hearty investment of time, effort and hard work. There's no shortcut on the road to research success, but as with anything, there are ways to optimise the process and work smarter.. Here, we'll share with you a wide range of apps, software and services that will make your life a little easier throughout the research process.
Performance is another main area of focus for efficient delivery of services. It can become a major reason of poor software quality. Poor delivery of services due to poor performance of software results in economic loss [32]. In this thesis, we perform empirical research to evaluate the maintainability and performance by implementing different ...
the impact of performance management systems on employee's performance, a case study of university of arusha jenifa calisti mbise a dissertation submitted in partial fulfillment of the requirements for the degree of master of human resource management of the open university of tanzania
Help you achieve your academic goals. Whether we're proofreading and editing, checking for plagiarism or AI content, generating citations, or writing useful Knowledge Base articles, our aim is to support students on their journey to become better academic writers. We believe that every student should have the right tools for academic success.
In this thesis the problems associated with software architecture documentation are described, and a new method to document software ... performance or reliability. The right architecture - correctly designed to meet its quality attribute requirements, clearly documented, and conscientiously evaluated - is the ...
Software engineering and technology are prevalent areas for thesis at the department, and many candidates ask for thesis topics every academic year. We do our best to accommodate the requests, but the applicants can smoothen the process by taking an active role in thinking about potential topics based on the themes presented below.
In the literature, studies have evidenced the efforts adopted by firms to develop digital technology with the hope of achieving sustainable decisions and competitive performance. However, studies have yet to provide an extensive explanation of the mechanisms used by firms in their digital technology adoption to impact and enhance value, particularly among small and medium enterprises (SMEs ...
This chapter describes the specific problem that this thesis addresses, the context of the problem, the goals of this thesis project, the research methodology used in this thesis, and outlines the structure of the thesis. 1.1 Background Modern large-scale software systems often need to support concurrent access
Abdel-Hamid (Citation 1984) explored software project performance using interval-based overtime and dynamic hiring through SD modelling. Abdel-Hamid (Citation 1989) ... Li, S. (2008), "A Generic Model of Project Management with Vensim" (master's thesis, Faculty of Engineering and Science, Agder University). Google Scholar.
This thesis presents a series of novel approaches based on empirical insights that attempt to support developers at the task of designing efficient code. We present contributions in three aspects ...
However, the purpose of the study was to. examine the impact of accounting software for. business perf ormance on profitability level of small. scale business. To meas ure the business perform ...
from hardware and limiting the scope of the application domain. This thesis proposes a new software/hardware co-design approach to achieving 3P platforms, called the loop-task accelerator (LTA) platform, that provides high productivity and portability without sacrificing performance or efficiency across a wide range of applications.
Thesis offers streamlined student information systems (SIS) that are cloud-based and personalized to your unique university. ... We'll show you how to configure the software to meet your unique needs to support the future of your institution. Implementation. We will implement a cloud-based SIS that is launched on time and on budget, built for ...
Start Free today. At Thesis Software, we are passionate about creating innovative and tailor-made software solutions to help businesses thrive in the digital age. With our team of skilled professionals and cutting-edge technologies, we are dedicated to transforming your ideas into reality and delivering exceptional results.
A Thesis Management System is a software solution designed to support the management and administration of theses or dissertations in academic institutions. It provides tools and features to streamline the entire thesis process, including proposal submission, review and approval workflows, document management, and tracking of progress.
This thesis examines two critical aspects of defining the hardware-software interface: quality and performance. The first aspect is creating a high quality specification of the interface as conventionally defined in an instruction set architecture. The majority of this thesis is concerned with creating a specification that covers the full scope ...
As a result of that, H2 is accepted. Hypothesis 4(Software package and AIS affect reliability future of information of financial statement.) Table 9. Hypothesis 3 Business Performance 1 User Friendly Business .482** Performance User-Friendly .482** 1 **. Correlation is significant at the 0.01 level (2tailed).
The landscape of ML ecosystem including models, software, and hardware evolves quickly due to phenomenal growth of Machine Learning (ML) and its application. Nevertheless, it remains challenging and labor-intensive to swiftly adapt existing ML systems to new models and hardware to maximize performance. We find that it is attributed to existing ML systems falling short in portability and ...
Qiskit SDK 1.x evolves the widely popular open-source development kit to a highly stable, reliable, and utility-scale tool for building and optimizing circuits and operators. It enables working with the increasingly complex quantum circuits being discovered by users — those with 100s of qubits and thousands of gates or more.
The precision-recall curve (PRC) and the area under the precision-recall curve (AUPRC) are useful for quantifying classification performance. They are commonly used in situations with imbalanced classes, such as cancer diagnosis and cell type annotation. We evaluate 10 popular tools for plotting PRC and computing AUPRC, which were collectively used in more than 3000 published studies. We find ...
Elaine J. Weyuker, Senior Member, IEEE, and Filippos I. Vokolos. Abstract ÐAn approach to software performance testing is discussed. A case study describing the experience of using this approach ...
The HPSF was launched today in Hamburg at ISC'24, by the Linux Foundation, the nonprofit organization that enables mass innovation through open-source. The HPSF has strong support across the HPC landscape. Through a series of technical projects, the HPSF aims to build, promote, and advance a portable core software stack for HPC to increase ...
Copy-on-Write performance and debugging. Erik Mavrinac. May 14th, 2024 0 0. This is a follow-up to our previous coverage of Dev Drive and copy-on-write (CoW) linking. See our previous articles from May 24, 2023, October 13, 2023, and November 2, 2023. Dev Drive was released in Windows 11 in October, 2023, and will be part of Windows Server 2025 ...
Performance-based licensing offers greater transparency in software costs because pricing is based on measurable informatics rather than blurred pricing structures that only benefit the provider.
The year Software AG was founded provided two watershed moments in the future growth and direction of the technology industry.It was 1969, and IBM Corp. announced in June that it would begin prici