Thank you for visiting nature.com. You are using a browser version with limited support for CSS. To obtain the best experience, we recommend you use a more up to date browser (or turn off compatibility mode in Internet Explorer). In the meantime, to ensure continued support, we are displaying the site without styles and JavaScript.
- View all journals
- My Account Login
- Explore content
- About the journal
- Publish with us
- Sign up for alerts
- Review Article
- Open access
- Published: 22 February 2021
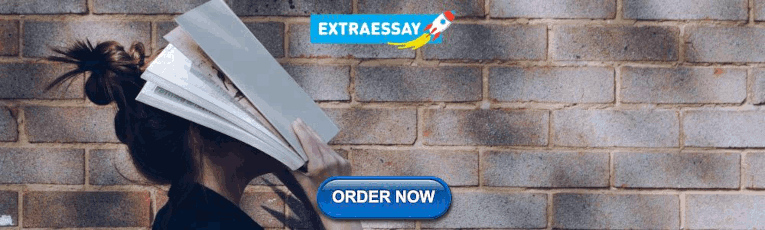
Addiction as a brain disease revised: why it still matters, and the need for consilience
- Markus Heilig 1 ,
- James MacKillop ORCID: orcid.org/0000-0003-4118-9500 2 , 3 ,
- Diana Martinez 4 ,
- Jürgen Rehm ORCID: orcid.org/0000-0001-5665-0385 5 , 6 , 7 , 8 ,
- Lorenzo Leggio ORCID: orcid.org/0000-0001-7284-8754 9 &
- Louk J. M. J. Vanderschuren ORCID: orcid.org/0000-0002-5379-0363 10
Neuropsychopharmacology volume 46 , pages 1715–1723 ( 2021 ) Cite this article
86k Accesses
101 Citations
320 Altmetric
Metrics details
The view that substance addiction is a brain disease, although widely accepted in the neuroscience community, has become subject to acerbic criticism in recent years. These criticisms state that the brain disease view is deterministic, fails to account for heterogeneity in remission and recovery, places too much emphasis on a compulsive dimension of addiction, and that a specific neural signature of addiction has not been identified. We acknowledge that some of these criticisms have merit, but assert that the foundational premise that addiction has a neurobiological basis is fundamentally sound. We also emphasize that denying that addiction is a brain disease is a harmful standpoint since it contributes to reducing access to healthcare and treatment, the consequences of which are catastrophic. Here, we therefore address these criticisms, and in doing so provide a contemporary update of the brain disease view of addiction. We provide arguments to support this view, discuss why apparently spontaneous remission does not negate it, and how seemingly compulsive behaviors can co-exist with the sensitivity to alternative reinforcement in addiction. Most importantly, we argue that the brain is the biological substrate from which both addiction and the capacity for behavior change arise, arguing for an intensified neuroscientific study of recovery. More broadly, we propose that these disagreements reveal the need for multidisciplinary research that integrates neuroscientific, behavioral, clinical, and sociocultural perspectives.
Similar content being viewed by others
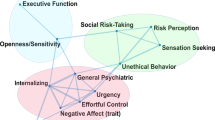
Subtypes in addiction and their neurobehavioral profiles across three functional domains
Gunner Drossel, Leyla R. Brucar, … Anna Zilverstand
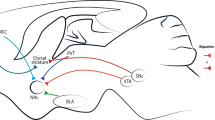
Drug addiction: from bench to bedside
Julian Cheron & Alban de Kerchove d’Exaerde
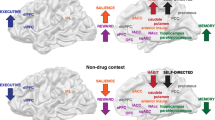
The neurobiology of drug addiction: cross-species insights into the dysfunction and recovery of the prefrontal cortex
Ahmet O. Ceceli, Charles W. Bradberry & Rita Z. Goldstein
Introduction
Close to a quarter of a century ago, then director of the US National Institute on Drug Abuse Alan Leshner famously asserted that “addiction is a brain disease”, articulated a set of implications of this position, and outlined an agenda for realizing its promise [ 1 ]. The paper, now cited almost 2000 times, put forward a position that has been highly influential in guiding the efforts of researchers, and resource allocation by funding agencies. A subsequent 2000 paper by McLellan et al. [ 2 ] examined whether data justify distinguishing addiction from other conditions for which a disease label is rarely questioned, such as diabetes, hypertension or asthma. It concluded that neither genetic risk, the role of personal choices, nor the influence of environmental factors differentiated addiction in a manner that would warrant viewing it differently; neither did relapse rates, nor compliance with treatment. The authors outlined an agenda closely related to that put forward by Leshner, but with a more clinical focus. Their conclusion was that addiction should be insured, treated, and evaluated like other diseases. This paper, too, has been exceptionally influential by academic standards, as witnessed by its ~3000 citations to date. What may be less appreciated among scientists is that its impact in the real world of addiction treatment has remained more limited, with large numbers of patients still not receiving evidence-based treatments.
In recent years, the conceptualization of addiction as a brain disease has come under increasing criticism. When first put forward, the brain disease view was mainly an attempt to articulate an effective response to prevailing nonscientific, moralizing, and stigmatizing attitudes to addiction. According to these attitudes, addiction was simply the result of a person’s moral failing or weakness of character, rather than a “real” disease [ 3 ]. These attitudes created barriers for people with substance use problems to access evidence-based treatments, both those available at the time, such as opioid agonist maintenance, cognitive behavioral therapy-based relapse prevention, community reinforcement or contingency management, and those that could result from research. To promote patient access to treatments, scientists needed to argue that there is a biological basis beneath the challenging behaviors of individuals suffering from addiction. This argument was particularly targeted to the public, policymakers and health care professionals, many of whom held that since addiction was a misery people brought upon themselves, it fell beyond the scope of medicine, and was neither amenable to treatment, nor warranted the use of taxpayer money.
Present-day criticism directed at the conceptualization of addiction as a brain disease is of a very different nature. It originates from within the scientific community itself, and asserts that this conceptualization is neither supported by data, nor helpful for people with substance use problems [ 4 , 5 , 6 , 7 , 8 ]. Addressing these critiques requires a very different perspective, and is the objective of our paper. We readily acknowledge that in some cases, recent critiques of the notion of addiction as a brain disease as postulated originally have merit, and that those critiques require the postulates to be re-assessed and refined. In other cases, we believe the arguments have less validity, but still provide an opportunity to update the position of addiction as a brain disease. Our overarching concern is that questionable arguments against the notion of addiction as a brain disease may harm patients, by impeding access to care, and slowing development of novel treatments.
A premise of our argument is that any useful conceptualization of addiction requires an understanding both of the brains involved, and of environmental factors that interact with those brains [ 9 ]. These environmental factors critically include availability of drugs, but also of healthy alternative rewards and opportunities. As we will show, stating that brain mechanisms are critical for understanding and treating addiction in no way negates the role of psychological, social and socioeconomic processes as both causes and consequences of substance use. To reflect this complex nature of addiction, we have assembled a team with expertise that spans from molecular neuroscience, through animal models of addiction, human brain imaging, clinical addiction medicine, to epidemiology. What brings us together is a passionate commitment to improving the lives of people with substance use problems through science and science-based treatments, with empirical evidence as the guiding principle.
To achieve this goal, we first discuss the nature of the disease concept itself, and why we believe it is important for the science and treatment of addiction. This is followed by a discussion of the main points raised when the notion of addiction as a brain disease has come under criticism. Key among those are claims that spontaneous remission rates are high; that a specific brain pathology is lacking; and that people suffering from addiction, rather than behaving “compulsively”, in fact show a preserved ability to make informed and advantageous choices. In the process of discussing these issues, we also address the common criticism that viewing addiction as a brain disease is a fully deterministic theory of addiction. For our argument, we use the term “addiction” as originally used by Leshner [ 1 ]; in Box 1 , we map out and discuss how this construct may relate to the current diagnostic categories, such as Substance Use Disorder (SUD) and its different levels of severity (Fig. 1) .
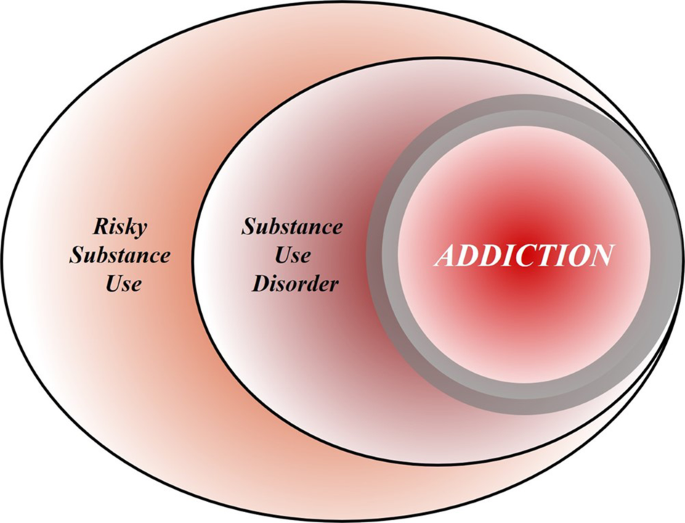
Risky (hazardous) substance use refers to quantity/frequency indicators of consumption; SUD refers to individuals who meet criteria for a DSM-5 diagnosis (mild, moderate, or severe); and addiction refers to individuals who exhibit persistent difficulties with self-regulation of drug consumption. Among high-risk individuals, a subgroup will meet criteria for SUD and, among those who have an SUD, a further subgroup would be considered to be addicted to the drug. However, the boundary for addiction is intentionally blurred to reflect that the dividing line for defining addiction within the category of SUD remains an open empirical question.
Box 1 What’s in a name? Differentiating hazardous use, substance use disorder, and addiction
Although our principal focus is on the brain disease model of addiction, the definition of addiction itself is a source of ambiguity. Here, we provide a perspective on the major forms of terminology in the field.
Hazardous Substance Use
Hazardous (risky) substance use refers to quantitative levels of consumption that increase an individual’s risk for adverse health consequences. In practice, this pertains to alcohol use [ 110 , 111 ]. Clinically, alcohol consumption that exceeds guidelines for moderate drinking has been used to prompt brief interventions or referral for specialist care [ 112 ]. More recently, a reduction in these quantitative levels has been validated as treatment endpoints [ 113 ].
Substance Use Disorder
SUD refers to the DSM-5 diagnosis category that encompasses significant impairment or distress resulting from specific categories of psychoactive drug use. The diagnosis of SUD is operationalized as 2 or more of 11 symptoms over the past year. As a result, the diagnosis is heterogenous, with more than 1100 symptom permutations possible. The diagnosis in DSM-5 is the result of combining two diagnoses from the DSM-IV, abuse and dependence, which proved to be less valid than a single dimensional approach [ 114 ]. Critically, SUD includes three levels of severity: mild (2–3 symptoms), moderate (4–5 symptoms), and severe (6+ symptoms). The International Classification of Diseases (ICD) system retains two diagnoses, harmful use (lower severity) and substance dependence (higher severity).
Addiction is a natural language concept, etymologically meaning enslavement, with the contemporary meaning traceable to the Middle and Late Roman Republic periods [ 115 ]. As a scientific construct, drug addiction can be defined as a state in which an individual exhibits an inability to self-regulate consumption of a substance, although it does not have an operational definition. Regarding clinical diagnosis, as it is typically used in scientific and clinical parlance, addiction is not synonymous with the simple presence of SUD. Nowhere in DSM-5 is it articulated that the diagnostic threshold (or any specific number/type of symptoms) should be interpreted as reflecting addiction, which inherently connotes a high degree of severity. Indeed, concerns were raised about setting the diagnostic standard too low because of the issue of potentially conflating a low-severity SUD with addiction [ 116 ]. In scientific and clinical usage, addiction typically refers to individuals at a moderate or high severity of SUD. This is consistent with the fact that moderate-to-severe SUD has the closest correspondence with the more severe diagnosis in ICD [ 117 , 118 , 119 ]. Nonetheless, akin to the undefined overlap between hazardous use and SUD, the field has not identified the exact thresholds of SUD symptoms above which addiction would be definitively present.
Integration
The ambiguous relationships among these terms contribute to misunderstandings and disagreements. Figure 1 provides a simple working model of how these terms overlap. Fundamentally, we consider that these terms represent successive dimensions of severity, clinical “nesting dolls”. Not all individuals consuming substances at hazardous levels have an SUD, but a subgroup do. Not all individuals with a SUD are addicted to the drug in question, but a subgroup are. At the severe end of the spectrum, these domains converge (heavy consumption, numerous symptoms, the unambiguous presence of addiction), but at low severity, the overlap is more modest. The exact mapping of addiction onto SUD is an open empirical question, warranting systematic study among scientists, clinicians, and patients with lived experience. No less important will be future research situating our definition of SUD using more objective indicators (e.g., [ 55 , 120 ]), brain-based and otherwise, and more precisely in relation to clinical needs [ 121 ]. Finally, such work should ultimately be codified in both the DSM and ICD systems to demarcate clearly where the attribution of addiction belongs within the clinical nosology, and to foster greater clarity and specificity in scientific discourse.
What is a disease?
In his classic 1960 book “The Disease Concept of Alcoholism”, Jellinek noted that in the alcohol field, the debate over the disease concept was plagued by too many definitions of “alcoholism” and too few definitions of “disease” [ 10 ]. He suggested that the addiction field needed to follow the rest of medicine in moving away from viewing disease as an “entity”, i.e., something that has “its own independent existence, apart from other things” [ 11 ]. To modern medicine, he pointed out, a disease is simply a label that is agreed upon to describe a cluster of substantial, deteriorating changes in the structure or function of the human body, and the accompanying deterioration in biopsychosocial functioning. Thus, he concluded that alcoholism can simply be defined as changes in structure or function of the body due to drinking that cause disability or death. A disease label is useful to identify groups of people with commonly co-occurring constellations of problems—syndromes—that significantly impair function, and that lead to clinically significant distress, harm, or both. This convention allows a systematic study of the condition, and of whether group members benefit from a specific intervention.
It is not trivial to delineate the exact category of harmful substance use for which a label such as addiction is warranted (See Box 1 ). Challenges to diagnostic categorization are not unique to addiction, however. Throughout clinical medicine, diagnostic cut-offs are set by consensus, commonly based on an evolving understanding of thresholds above which people tend to benefit from available interventions. Because assessing benefits in large patient groups over time is difficult, diagnostic thresholds are always subject to debate and adjustments. It can be debated whether diagnostic thresholds “merely” capture the extreme of a single underlying population, or actually identify a subpopulation that is at some level distinct. Resolving this issue remains challenging in addiction, but once again, this is not different from other areas of medicine [see e.g., [ 12 ] for type 2 diabetes]. Longitudinal studies that track patient trajectories over time may have a better ability to identify subpopulations than cross-sectional assessments [ 13 ].
By this pragmatic, clinical understanding of the disease concept, it is difficult to argue that “addiction” is unjustified as a disease label. Among people who use drugs or alcohol, some progress to using with a quantity and frequency that results in impaired function and often death, making substance use a major cause of global disease burden [ 14 ]. In these people, use occurs with a pattern that in milder forms may be challenging to capture by current diagnostic criteria (See Box 1 ), but is readily recognized by patients, their families and treatment providers when it reaches a severity that is clinically significant [see [ 15 ] for a classical discussion]. In some cases, such as opioid addiction, those who receive the diagnosis stand to obtain some of the greatest benefits from medical treatments in all of clinical medicine [ 16 , 17 ]. Although effect sizes of available treatments are more modest in nicotine [ 18 ] and alcohol addiction [ 19 ], the evidence supporting their efficacy is also indisputable. A view of addiction as a disease is justified, because it is beneficial: a failure to diagnose addiction drastically increases the risk of a failure to treat it [ 20 ].
Of course, establishing a diagnosis is not a requirement for interventions to be meaningful. People with hazardous or harmful substance use who have not (yet) developed addiction should also be identified, and interventions should be initiated to address their substance-related risks. This is particularly relevant for alcohol, where even in the absence of addiction, use is frequently associated with risks or harm to self, e.g., through cardiovascular disease, liver disease or cancer, and to others, e.g., through accidents or violence [ 21 ]. Interventions to reduce hazardous or harmful substance use in people who have not developed addiction are in fact particularly appealing. In these individuals, limited interventions are able to achieve robust and meaningful benefits [ 22 ], presumably because patterns of misuse have not yet become entrenched.
Thus, as originally pointed out by McLellan and colleagues, most of the criticisms of addiction as a disease could equally be applied to other medical conditions [ 2 ]. This type of criticism could also be applied to other psychiatric disorders, and that has indeed been the case historically [ 23 , 24 ]. Today, there is broad consensus that those criticisms were misguided. Few, if any healthcare professionals continue to maintain that schizophrenia, rather than being a disease, is a normal response to societal conditions. Why, then, do people continue to question if addiction is a disease, but not whether schizophrenia, major depressive disorder or post-traumatic stress disorder are diseases? This is particularly troubling given the decades of data showing high co-morbidity of addiction with these conditions [ 25 , 26 ]. We argue that it comes down to stigma. Dysregulated substance use continues to be perceived as a self-inflicted condition characterized by a lack of willpower, thus falling outside the scope of medicine and into that of morality [ 3 ].
Chronic and relapsing, developmentally-limited, or spontaneously remitting?
Much of the critique targeted at the conceptualization of addiction as a brain disease focuses on its original assertion that addiction is a chronic and relapsing condition. Epidemiological data are cited in support of the notion that large proportions of individuals achieve remission [ 27 ], frequently without any formal treatment [ 28 , 29 ] and in some cases resuming low risk substance use [ 30 ]. For instance, based on data from the National Epidemiologic Survey on Alcohol and Related Conditions (NESARC) study [ 27 ], it has been pointed out that a significant proportion of people with an addictive disorder quit each year, and that most afflicted individuals ultimately remit. These spontaneous remission rates are argued to invalidate the concept of a chronic, relapsing disease [ 4 ].
Interpreting these and similar data is complicated by several methodological and conceptual issues. First, people may appear to remit spontaneously because they actually do, but also because of limited test–retest reliability of the diagnosis [ 31 ]. For instance, using a validated diagnostic interview and trained interviewers, the Collaborative Studies on Genetics of Alcoholism examined the likelihood that an individual diagnosed with a lifetime history of substance dependence would retain this classification after 5 years. This is obviously a diagnosis that, once met, by definition cannot truly remit. Lifetime alcohol dependence was indeed stable in individuals recruited from addiction treatment units, ~90% for women, and 95% for men. In contrast, in a community-based sample similar to that used in the NESARC [ 27 ], stability was only ~30% and 65% for women and men, respectively. The most important characteristic that determined diagnostic stability was severity. Diagnosis was stable in severe, treatment-seeking cases, but not in general population cases of alcohol dependence.
These data suggest that commonly used diagnostic criteria alone are simply over-inclusive for a reliable, clinically meaningful diagnosis of addiction. They do identify a core group of treatment seeking individuals with a reliable diagnosis, but, if applied to nonclinical populations, also flag as “cases” a considerable halo of individuals for whom the diagnostic categorization is unreliable. Any meaningful discussion of remission rates needs to take this into account, and specify which of these two populations that is being discussed. Unfortunately, the DSM-5 has not made this task easier. With only 2 out of 11 symptoms being sufficient for a diagnosis of SUD, it captures under a single diagnostic label individuals in a “mild” category, whose diagnosis is likely to have very low test–retest reliability, and who are unlikely to exhibit a chronic relapsing course, together with people at the severe end of the spectrum, whose diagnosis is reliable, many of whom do show a chronic relapsing course.
The NESARC data nevertheless show that close to 10% of people in the general population who are diagnosed with alcohol addiction (here equated with DSM-IV “dependence” used in the NESARC study) never remitted throughout their participation in the survey. The base life-time prevalence of alcohol dependence in NESARC was 12.5% [ 32 ]. Thus, the data cited against the concept of addiction as a chronic relapsing disease in fact indicate that over 1% of the US population develops an alcohol-related condition that is associated with high morbidity and mortality, and whose chronic and/or relapsing nature cannot be disputed, since it does not remit.
Secondly, the analysis of NESARC data [ 4 , 27 ] omits opioid addiction, which, together with alcohol and tobacco, is the largest addiction-related public health problem in the US [ 33 ]. This is probably the addictive condition where an analysis of cumulative evidence most strikingly supports the notion of a chronic disorder with frequent relapses in a large proportion of people affected [ 34 ]. Of course, a large number of people with opioid addiction are unable to express the chronic, relapsing course of their disease, because over the long term, their mortality rate is about 15 times greater than that of the general population [ 35 ]. However, even among those who remain alive, the prevalence of stable abstinence from opioid use after 10–30 years of observation is <30%. Remission may not always require abstinence, for instance in the case of alcohol addiction, but is a reasonable proxy for remission with opioids, where return to controlled use is rare. Embedded in these data is a message of literally vital importance: when opioid addiction is diagnosed and treated as a chronic relapsing disease, outcomes are markedly improved, and retention in treatment is associated with a greater likelihood of abstinence.
The fact that significant numbers of individuals exhibit a chronic relapsing course does not negate that even larger numbers of individuals with SUD according to current diagnostic criteria do not. For instance, in many countries, the highest prevalence of substance use problems is found among young adults, aged 18–25 [ 36 ], and a majority of these ‘age out’ of excessive substance use [ 37 ]. It is also well documented that many individuals with SUD achieve longstanding remission, in many cases without any formal treatment (see e.g., [ 27 , 30 , 38 ]).
Collectively, the data show that the course of SUD, as defined by current diagnostic criteria, is highly heterogeneous. Accordingly, we do not maintain that a chronic relapsing course is a defining feature of SUD. When present in a patient, however, such as course is of clinical significance, because it identifies a need for long-term disease management [ 2 ], rather than expectations of a recovery that may not be within the individual’s reach [ 39 ]. From a conceptual standpoint, however, a chronic relapsing course is neither necessary nor implied in a view that addiction is a brain disease. This view also does not mean that it is irreversible and hopeless. Human neuroscience documents restoration of functioning after abstinence [ 40 , 41 ] and reveals predictors of clinical success [ 42 ]. If anything, this evidence suggests a need to increase efforts devoted to neuroscientific research on addiction recovery [ 40 , 43 ].
Lessons from genetics
For alcohol addiction, meta-analysis of twin and adoption studies has estimated heritability at ~50%, while estimates for opioid addiction are even higher [ 44 , 45 ]. Genetic risk factors are to a large extent shared across substances [ 46 ]. It has been argued that a genetic contribution cannot support a disease view of a behavior, because most behavioral traits, including religious and political inclinations, have a genetic contribution [ 4 ]. This statement, while correct in pointing out broad heritability of behavioral traits, misses a fundamental point. Genetic architecture is much like organ structure. The fact that normal anatomy shapes healthy organ function does not negate that an altered structure can contribute to pathophysiology of disease. The structure of the genetic landscape is no different. Critics further state that a “genetic predisposition is not a recipe for compulsion”, but no neuroscientist or geneticist would claim that genetic risk is “a recipe for compulsion”. Genetic risk is probabilistic, not deterministic. However, as we will see below, in the case of addiction, it contributes to large, consistent probability shifts towards maladaptive behavior.
In dismissing the relevance of genetic risk for addiction, Hall writes that “a large number of alleles are involved in the genetic susceptibility to addiction and individually these alleles might very weakly predict a risk of addiction”. He goes on to conclude that “generally, genetic prediction of the risk of disease (even with whole-genome sequencing data) is unlikely to be informative for most people who have a so-called average risk of developing an addiction disorder” [ 7 ]. This reflects a fundamental misunderstanding of polygenic risk. It is true that a large number of risk alleles are involved, and that the explanatory power of currently available polygenic risk scores for addictive disorders lags behind those for e.g., schizophrenia or major depression [ 47 , 48 ]. The only implication of this, however, is that low average effect sizes of risk alleles in addiction necessitate larger study samples to construct polygenic scores that account for a large proportion of the known heritability.
However, a heritability of addiction of ~50% indicates that DNA sequence variation accounts for 50% of the risk for this condition. Once whole genome sequencing is readily available, it is likely that it will be possible to identify most of that DNA variation. For clinical purposes, those polygenic scores will of course not replace an understanding of the intricate web of biological and social factors that promote or prevent expression of addiction in an individual case; rather, they will add to it [ 49 ]. Meanwhile, however, genome-wide association studies in addiction have already provided important information. For instance, they have established that the genetic underpinnings of alcohol addiction only partially overlap with those for alcohol consumption, underscoring the genetic distinction between pathological and nonpathological drinking behaviors [ 50 ].
It thus seems that, rather than negating a rationale for a disease view of addiction, the important implication of the polygenic nature of addiction risk is a very different one. Genome-wide association studies of complex traits have largely confirmed the century old “infinitisemal model” in which Fisher reconciled Mendelian and polygenic traits [ 51 ]. A key implication of this model is that genetic susceptibility for a complex, polygenic trait is continuously distributed in the population. This may seem antithetical to a view of addiction as a distinct disease category, but the contradiction is only apparent, and one that has long been familiar to quantitative genetics. Viewing addiction susceptibility as a polygenic quantitative trait, and addiction as a disease category is entirely in line with Falconer’s theorem, according to which, in a given set of environmental conditions, a certain level of genetic susceptibility will determine a threshold above which disease will arise.
A brain disease? Then show me the brain lesion!
The notion of addiction as a brain disease is commonly criticized with the argument that a specific pathognomonic brain lesion has not been identified. Indeed, brain imaging findings in addiction (perhaps with the exception of extensive neurotoxic gray matter loss in advanced alcohol addiction) are nowhere near the level of specificity and sensitivity required of clinical diagnostic tests. However, this criticism neglects the fact that neuroimaging is not used to diagnose many neurologic and psychiatric disorders, including epilepsy, ALS, migraine, Huntington’s disease, bipolar disorder, or schizophrenia. Even among conditions where signs of disease can be detected using brain imaging, such as Alzheimer’s and Parkinson’s disease, a scan is best used in conjunction with clinical acumen when making the diagnosis. Thus, the requirement that addiction be detectable with a brain scan in order to be classified as a disease does not recognize the role of neuroimaging in the clinic.
For the foreseeable future, the main objective of imaging in addiction research is not to diagnose addiction, but rather to improve our understanding of mechanisms that underlie it. The hope is that mechanistic insights will help bring forward new treatments, by identifying candidate targets for them, by pointing to treatment-responsive biomarkers, or both [ 52 ]. Developing innovative treatments is essential to address unmet treatment needs, in particular in stimulant and cannabis addiction, where no approved medications are currently available. Although the task to develop novel treatments is challenging, promising candidates await evaluation [ 53 ]. A particular opportunity for imaging-based research is related to the complex and heterogeneous nature of addictive disorders. Imaging-based biomarkers hold the promise of allowing this complexity to be deconstructed into specific functional domains, as proposed by the RDoC initiative [ 54 ] and its application to addiction [ 55 , 56 ]. This can ultimately guide the development of personalized medicine strategies to addiction treatment.
Countless imaging studies have reported differences in brain structure and function between people with addictive disorders and those without them. Meta-analyses of structural data show that alcohol addiction is associated with gray matter losses in the prefrontal cortex, dorsal striatum, insula, and posterior cingulate cortex [ 57 ], and similar results have been obtained in stimulant-addicted individuals [ 58 ]. Meta-analysis of functional imaging studies has demonstrated common alterations in dorsal striatal, and frontal circuits engaged in reward and salience processing, habit formation, and executive control, across different substances and task-paradigms [ 59 ]. Molecular imaging studies have shown that large and fast increases in dopamine are associated with the reinforcing effects of drugs of abuse, but that after chronic drug use and during withdrawal, brain dopamine function is markedly decreased and that these decreases are associated with dysfunction of prefrontal regions [ 60 ]. Collectively, these findings have given rise to a widely held view of addiction as a disorder of fronto-striatal circuitry that mediates top-down regulation of behavior [ 61 ].
Critics reply that none of the brain imaging findings are sufficiently specific to distinguish between addiction and its absence, and that they are typically obtained in cross-sectional studies that can at best establish correlative rather than causal links. In this, they are largely right, and an updated version of a conceptualization of addiction as a brain disease needs to acknowledge this. Many of the structural brain findings reported are not specific for addiction, but rather shared across psychiatric disorders [ 62 ]. Also, for now, the most sophisticated tools of human brain imaging remain crude in face of complex neural circuit function. Importantly however, a vast literature from animal studies also documents functional changes in fronto-striatal circuits, as well their limbic and midbrain inputs, associated with addictive behaviors [ 63 , 64 , 65 , 66 , 67 , 68 ]. These are circuits akin to those identified by neuroimaging studies in humans, implicated in positive and negative emotions, learning processes and executive functions, altered function of which is thought to underlie addiction. These animal studies, by virtue of their cellular and molecular level resolution, and their ability to establish causality under experimental control, are therefore an important complement to human neuroimaging work.
Nevertheless, factors that seem remote from the activity of brain circuits, such as policies, substance availability and cost, as well as socioeconomic factors, also are critically important determinants of substance use. In this complex landscape, is the brain really a defensible focal point for research and treatment? The answer is “yes”. As powerfully articulated by Francis Crick [ 69 ], “You, your joys and your sorrows, your memories and your ambitions, your sense of personal identity and free will, are in fact no more than the behavior of a vast assembly of nerve cells and their associated molecules”. Social and interpersonal factors are critically important in addiction, but they can only exert their influences by impacting neural processes. They must be encoded as sensory data, represented together with memories of the past and predictions about the future, and combined with representations of interoceptive and other influences to provide inputs to the valuation machinery of the brain. Collectively, these inputs drive action selection and execution of behavior—say, to drink or not to drink, and then, within an episode, to stop drinking or keep drinking. Stating that the pathophysiology of addiction is largely about the brain does not ignore the role of other influences. It is just the opposite: it is attempting to understand how those important influences contribute to drug seeking and taking in the context of the brain, and vice versa.
But if the criticism is one of emphasis rather than of principle—i.e., too much brain, too little social and environmental factors – then neuroscientists need to acknowledge that they are in part guilty as charged. Brain-centric accounts of addiction have for a long time failed to pay enough attention to the inputs that social factors provide to neural processing behind drug seeking and taking [ 9 ]. This landscape is, however, rapidly changing. For instance, using animal models, scientists are finding that lack of social play early in life increases the motivation to take addictive substances in adulthood [ 70 ]. Others find that the opportunity to interact with a fellow rat is protective against addiction-like behaviors [ 71 ]. In humans, a relationship has been found between perceived social support, socioeconomic status, and the availability of dopamine D2 receptors [ 72 , 73 ], a biological marker of addiction vulnerability. Those findings in turn provided translation of data from nonhuman primates, which showed that D2 receptor availability can be altered by changes in social hierarchy, and that these changes are associated with the motivation to obtain cocaine [ 74 ].
Epidemiologically, it is well established that social determinants of health, including major racial and ethnic disparities, play a significant role in the risk for addiction [ 75 , 76 ]. Contemporary neuroscience is illuminating how those factors penetrate the brain [ 77 ] and, in some cases, reveals pathways of resilience [ 78 ] and how evidence-based prevention can interrupt those adverse consequences [ 79 , 80 ]. In other words, from our perspective, viewing addiction as a brain disease in no way negates the importance of social determinants of health or societal inequalities as critical influences. In fact, as shown by the studies correlating dopamine receptors with social experience, imaging is capable of capturing the impact of the social environment on brain function. This provides a platform for understanding how those influences become embedded in the biology of the brain, which provides a biological roadmap for prevention and intervention.
We therefore argue that a contemporary view of addiction as a brain disease does not deny the influence of social, environmental, developmental, or socioeconomic processes, but rather proposes that the brain is the underlying material substrate upon which those factors impinge and from which the responses originate. Because of this, neurobiology is a critical level of analysis for understanding addiction, although certainly not the only one. It is recognized throughout modern medicine that a host of biological and non-biological factors give rise to disease; understanding the biological pathophysiology is critical for understanding etiology and informing treatment.
Is a view of addiction as a brain disease deterministic?
A common criticism of the notion that addiction is a brain disease is that it is reductionist and in the end therefore deterministic [ 81 , 82 ]. This is a fundamental misrepresentation. As indicated above, viewing addiction as a brain disease simply states that neurobiology is an undeniable component of addiction. A reason for deterministic interpretations may be that modern neuroscience emphasizes an understanding of proximal causality within research designs (e.g., whether an observed link between biological processes is mediated by a specific mechanism). That does not in any way reflect a superordinate assumption that neuroscience will achieve global causality. On the contrary, since we realize that addiction involves interactions between biology, environment and society, ultimate (complete) prediction of behavior based on an understanding of neural processes alone is neither expected, nor a goal.
A fairer representation of a contemporary neuroscience view is that it believes insights from neurobiology allow useful probabilistic models to be developed of the inherently stochastic processes involved in behavior [see [ 83 ] for an elegant recent example]. Changes in brain function and structure in addiction exert a powerful probabilistic influence over a person’s behavior, but one that is highly multifactorial, variable, and thus stochastic. Philosophically, this is best understood as being aligned with indeterminism, a perspective that has a deep history in philosophy and psychology [ 84 ]. In modern neuroscience, it refers to the position that the dynamic complexity of the brain, given the probabilistic threshold-gated nature of its biology (e.g., action potential depolarization, ion channel gating), means that behavior cannot be definitively predicted in any individual instance [ 85 , 86 ].
Driven by compulsion, or free to choose?
A major criticism of the brain disease view of addiction, and one that is related to the issue of determinism vs indeterminism, centers around the term “compulsivity” [ 6 , 87 , 88 , 89 , 90 ] and the different meanings it is given. Prominent addiction theories state that addiction is characterized by a transition from controlled to “compulsive” drug seeking and taking [ 91 , 92 , 93 , 94 , 95 ], but allocate somewhat different meanings to “compulsivity”. By some accounts, compulsive substance use is habitual and insensitive to its outcomes [ 92 , 94 , 96 ]. Others refer to compulsive use as a result of increasing incentive value of drug associated cues [ 97 ], while others view it as driven by a recruitment of systems that encode negative affective states [ 95 , 98 ].
The prototype for compulsive behavior is provided by obsessive-compulsive disorder (OCD), where compulsion refers to repeatedly and stereotypically carrying out actions that in themselves may be meaningful, but lose their purpose and become harmful when performed in excess, such as persistent handwashing until skin injuries result. Crucially, this happens despite a conscious desire to do otherwise. Attempts to resist these compulsions result in increasing and ultimately intractable anxiety [ 99 ]. This is in important ways different from the meaning of compulsivity as commonly used in addiction theories. In the addiction field, compulsive drug use typically refers to inflexible, drug-centered behavior in which substance use is insensitive to adverse consequences [ 100 ]. Although this phenomenon is not necessarily present in every patient, it reflects important symptoms of clinical addiction, and is captured by several DSM-5 criteria for SUD [ 101 ]. Examples are needle-sharing despite knowledge of a risk to contract HIV or Hepatitis C, drinking despite a knowledge of having liver cirrhosis, but also the neglect of social and professional activities that previously were more important than substance use. While these behaviors do show similarities with the compulsions of OCD, there are also important differences. For example, “compulsive” substance use is not necessarily accompanied by a conscious desire to withhold the behavior, nor is addictive behavior consistently impervious to change.
Critics question the existence of compulsivity in addiction altogether [ 5 , 6 , 7 , 89 ], typically using a literal interpretation, i.e., that a person who uses alcohol or drugs simply can not do otherwise. Were that the intended meaning in theories of addiction—which it is not—it would clearly be invalidated by observations of preserved sensitivity of behavior to contingencies in addiction. Indeed, substance use is influenced both by the availability of alternative reinforcers, and the state of the organism. The roots of this insight date back to 1940, when Spragg found that chimpanzees would normally choose a banana over morphine. However, when physically dependent and in a state of withdrawal, their choice preference would reverse [ 102 ]. The critical role of alternative reinforcers was elegantly brought into modern neuroscience by Ahmed et al., who showed that rats extensively trained to self-administer cocaine would readily forego the drug if offered a sweet solution as an alternative [ 103 ]. This was later also found to be the case for heroin [ 103 ], methamphetamine [ 104 ] and alcohol [ 105 ]. Early residential laboratory studies on alcohol use disorder indeed revealed orderly operant control over alcohol consumption [ 106 ]. Furthermore, efficacy of treatment approaches such as contingency management, which provides systematic incentives for abstinence [ 107 ], supports the notion that behavioral choices in patients with addictions remain sensitive to reward contingencies.
Evidence that a capacity for choosing advantageously is preserved in addiction provides a valid argument against a narrow concept of “compulsivity” as rigid, immutable behavior that applies to all patients. It does not, however, provide an argument against addiction as a brain disease. If not from the brain, from where do the healthy and unhealthy choices people make originate? The critical question is whether addictive behaviors—for the most part—result from healthy brains responding normally to externally determined contingencies; or rather from a pathology of brain circuits that, through probabilistic shifts, promotes the likelihood of maladaptive choices even when reward contingencies are within a normal range. To resolve this question, it is critical to understand that the ability to choose advantageously is not an all-or-nothing phenomenon, but rather is about probabilities and their shifts, multiple faculties within human cognition, and their interaction. Yes, it is clear that most people whom we would consider to suffer from addiction remain able to choose advantageously much, if not most, of the time. However, it is also clear that the probability of them choosing to their own disadvantage, even when more salutary options are available and sometimes at the expense of losing their life, is systematically and quantifiably increased. There is a freedom of choice, yet there is a shift of prevailing choices that nevertheless can kill.
Synthesized, the notion of addiction as a disease of choice and addiction as a brain disease can be understood as two sides of the same coin. Both of these perspectives are informative, and they are complementary. Viewed this way, addiction is a brain disease in which a person’s choice faculties become profoundly compromised. To articulate it more specifically, embedded in and principally executed by the central nervous system, addiction can be understood as a disorder of choice preferences, preferences that overvalue immediate reinforcement (both positive and negative), preferences for drug-reinforcement in spite of costs, and preferences that are unstable ( “I’ll never drink like that again;” “this will be my last cigarette” ), prone to reversals in the form of lapses and relapse. From a contemporary neuroscience perspective, pre-existing vulnerabilities and persistent drug use lead to a vicious circle of substantive disruptions in the brain that impair and undermine choice capacities for adaptive behavior, but do not annihilate them. Evidence of generally intact decision making does not fundamentally contradict addiction as a brain disease.
Conclusions
The present paper is a response to the increasing number of criticisms of the view that addiction is a chronic relapsing brain disease. In many cases, we show that those criticisms target tenets that are neither needed nor held by a contemporary version of this view. Common themes are that viewing addiction as a brain disease is criticized for being both too narrow (addiction is only a brain disease; no other perspectives or factors are important) or too far reaching (it purports to discover the final causes of addiction). With regard to disease course, we propose that viewing addiction as a chronic relapsing disease is appropriate for some populations, and much less so for others, simply necessitating better ways of delineating the populations being discussed. We argue that when considering addiction as a disease, the lens of neurobiology is valuable to use. It is not the only lens, and it does not have supremacy over other scientific approaches. We agree that critiques of neuroscience are warranted [ 108 ] and that critical thinking is essential to avoid deterministic language and scientific overreach.
Beyond making the case for a view of addiction as a brain disease, perhaps the more important question is when a specific level of analysis is most useful. For understanding the biology of addiction and designing biological interventions, a neurobiological view is almost certainly the most appropriate level of analysis, in particular when informed by an understanding of the behavioral manifestations. In contrast, for understanding the psychology of addiction and designing psychological interventions, behavioral science is the natural realm, but one that can often benefit from an understanding of the underlying neurobiology. For designing policies, such as taxation and regulation of access, economics and public administration provide the most pertinent perspectives, but these also benefit from biological and behavioral science insights.
Finally, we argue that progress would come from integration of these scientific perspectives and traditions. E.O. Wilson has argued more broadly for greater consilience [ 109 ], unity of knowledge, in science. We believe that addiction is among the areas where consilience is most needed. A plurality of disciplines brings important and trenchant insights to bear on this condition; it is the exclusive remit of no single perspective or field. Addiction inherently and necessarily requires multidisciplinary examination. Moreover, those who suffer from addiction will benefit most from the application of the full armamentarium of scientific perspectives.
Funding and disclosures
Supported by the Swedish Research Council grants 2013-07434, 2019-01138 (MH); Netherlands Organisation for Health Research and Development (ZonMw) under project number 912.14.093 (LJMJV); NIDA and NIAAA intramural research programs (LL; the content is solely the responsibility of the author and does not necessarily represent the official views of the National Institutes of Health); the Peter Boris Chair in Addictions Research, Homewood Research Institute, and the National Institute on Alcohol Abuse and Alcoholism grants AA025911, AA024930, AA025849, AA027679 (JM; the content is solely the responsibility of the author and does not necessarily represent the official views of the National Institutes of Health).
MH has received consulting fees, research support or other compensation from Indivior, Camurus, BrainsWay, Aelis Farma, and Janssen Pharmaceuticals. JM is a Principal and Senior Scientist at BEAM Diagnostics, Inc. DM, JR, LL, and LJMJV declare no conflict of interest.
Leshner AI. Addiction is a brain disease, and it matters. Science. 1997;278:45–7.
Article CAS PubMed Google Scholar
McLellan AT, Lewis DC, O’Brien CP, Kleber HD. Drug dependence, a chronic medical illness: implications for treatment, insurance, and outcomes evaluation. JAMA. 2000;284:1689–95.
Schomerus G, Lucht M, Holzinger A, Matschinger H, Carta MG, Angermeyer MC. The stigma of alcohol dependence compared with other mental disorders: a review of population studies. Alcohol Alcohol. 2011;46:105–12.
Heyman GM. Addiction and choice: theory and new data. Front Psychiatry. 2013;4:31.
Article PubMed PubMed Central Google Scholar
Heather N, Best D, Kawalek A, Field M, Lewis M, Rotgers F, et al. Challenging the brain disease model of addiction: European launch of the addiction theory network. Addict Res Theory. 2018;26:249–55.
Article Google Scholar
Pickard H, Ahmed SH, Foddy B. Alternative models of addiction. Front Psychiatr.y 2015;6:20.
Google Scholar
Hall W, Carter A, Forlini C. The brain disease model of addiction: is it supported by the evidence and has it delivered on its promises? Lancet Psychiatr. 2015;2:105–10.
Hart CL. Viewing addiction as a brain disease promotes social injustice. Nat Hum Behav. 2017;1:0055.
Heilig M, Epstein DH, Nader MA, Shaham Y. Time to connect: bringing social context into addiction neuroscience. Nat Rev Neurosc.i 2016;17:592–9.
Article CAS Google Scholar
Jellinek EM. The disease concept of alcoholism. Hillhouse Press on behalf of the Christopher J. Smithers Foundation: New Haven, CT; 1960.
Stevenson A. Oxford dictionary of English. 3 ed. New York, NY: Oxford University Press; 2010.
Fan J, May SJ, Zhou Y, Barrett-Connor E. Bimodality of 2-h plasma glucose distributions in whites: the Rancho Bernardo study. Diabetes Care 2005;28:1451–6.
King AC, Vena A, Hasin D, De Wit D, O’Connor CJ, Cao D. Subjective responses to alcohol in the development and maintenance of alcohol use disorder (AUD). Am J Psychiatry. 2021. https://doi.org/10.1176/appi.ajp.2020.20030247 .
GBD. 2016 Alcohol and Drug Use Collaborators. The global burden of disease attributable to alcohol and drug use in 195 countries and territories, 1990-2016: a systematic analysis for the Global Burden of Disease Study 2016. Lancet Psychiatry. 2018;5:987–1012.
Edwards G, Gross MM. Alcohol dependence: provisional description of a clinical syndrome. Br Med J. 1976;1:1058–61.
Article CAS PubMed PubMed Central Google Scholar
Epstein DH, Heilig M, Shaham Y. Science-based actions can help address the opioid crisis. Trends Pharm Sci. 2018;39:911–16.
Amato L, Davoli M, Perucci CA, Ferri M, Faggiano F, Mattick RP. An overview of systematic reviews of the effectiveness of opiate maintenance therapies: available evidence to inform clinical practice and research. J Subst Abus Treat. 2005;28:321–9.
Cahill K, Stevens S, Perera R, Lancaster T. Pharmacological interventions for smoking cessation: an overview and network meta-analysis. Cochrane Datab System Rev. 2013;5:CD009329.
Jonas DE, Amick HR, Feltner C, Bobashev G, Thomas K, Wines R, et al. Pharmacotherapy for adults with alcohol use disorders in outpatient settings a systematic review and meta-analysis. JAMA. 2014;311:1889–900.
Article PubMed CAS Google Scholar
Mark TL, Kranzler HR, Song X. Understanding US addiction physicians’ low rate of naltrexone prescription. Drug Alcohol Depend. 2003;71:219–28.
Article PubMed Google Scholar
Nutt DJ, King LA, Phillips LD. Drug harms in the UK: a multicriteria decision analysis. Lancet. 2010;376:1558–65.
Wilk AI, Jensen NM, Havighurst TC. Meta-analysis of randomized control trials addressing brief interventions in heavy alcohol drinkers. J Gen Intern Med. 1997;12:274–83.
Laing RD. The divided self; a study of sanity and madness. London: Tavistock Publications; 1960.
Foucault M, Khalfa J. History of madness. New York: Routledge; 2006.
Regier DA, Farmer ME, Rae DS, Locke BZ, Keith SJ, Judd LL. et al.Comorbidity of mental disorders with alcohol and other drug abuse. Results Epidemiologic Catchment Area (ECA) study.JAMA. 1990;264:2511–8.
Grant BF, Stinson FS, Dawson DA, Chou SP, Dufour MC, Compton W, et al. Prevalence and co-occurrence of substance use disorders and independent mood and anxiety disorders: results from the National Epidemiologic Survey on Alcohol and Related Conditions. Arch Gen Psychiatry. 2004;61:807–16.
Lopez-Quintero C, Hasin DS, de Los Cobos JP, Pines A, Wang S, Grant BF, et al. Probability and predictors of remission from life-time nicotine, alcohol, cannabis or cocaine dependence: results from the National Epidemiologic Survey on Alcohol and Related Conditions. Addiction. 2011;106:657–69.
Humphreys K. Addiction treatment professionals are not the gatekeepers of recovery. Subst Use Misuse. 2015;50:1024–7.
Cohen E, Feinn R, Arias A, Kranzler HR. Alcohol treatment utilization: findings from the National Epidemiologic Survey on Alcohol and Related Conditions. Drug Alcohol Depend. 2007;86:214–21.
Sobell LC, Cunningham JA, Sobell MB. Recovery from alcohol problems with and without treatment: prevalence in two population surveys. Am J Public Health. 1996;86:966–72.
Culverhouse R, Bucholz KK, Crowe RR, Hesselbrock V, Nurnberger JI Jr, Porjesz B, et al. Long-term stability of alcohol and other substance dependence diagnoses and habitual smoking: an evaluation after 5 years. Arch Gen Psychiatry. 2005;62:753–60.
Hasin DS, Stinson FS, Ogburn E, Grant BF. Prevalence, correlates, disability, and comorbidity of DSM-IV alcohol abuse and dependence in the United States: results from the National Epidemiologic Survey on Alcohol and Related Conditions. Arch Gen Psychiatry. 2007;64:830–42.
Skolnick P. The opioid epidemic: crisis and solutions. Annu Rev Pharm Toxicol. 2018;58:143–59.
Hser YI, Evans E, Grella C, Ling W, Anglin D. Long-term course of opioid addiction. Harv Rev Psychiatry. 2015;23:76–89.
Mathers BM, Degenhardt L, Bucello C, Lemon J, Wiessing L, Hickman M. Mortality among people who inject drugs: a systematic review and meta-analysis. Bull World Health Organ. 2013;91:102–23.
Grant BF, Goldstein RB, Saha TD, Chou SP, Jung J, Zhang H, et al. Epidemiology of DSM-5 alcohol use disorder: results from the National Epidemiologic Survey on Alcohol and Related Conditions III. JAMA Psychiatry. 2015;72:757–66.
Article PubMed PubMed Central CAS Google Scholar
Lee MR, Sher KJ. “Maturing Out” of binge and problem drinking. Alcohol Res: Curr Rev. 2018;39:31–42.
Dawson DA, Grant BF, Stinson FS, Chou PS, Huang B, Ruan WJ. Recovery from DSM-IV alcohol dependence: United States, 2001–2002. Addiction. 2005;100:281–92.
Berridge V. The rise, fall, and revival of recovery in drug policy. Lancet. 2012;379:22–23.
Parvaz MA, Moeller SJ, d’Oleire Uquillas F, Pflumm A, Maloney T, Alia-Klein N, et al. Prefrontal gray matter volume recovery in treatment-seeking cocaine-addicted individuals: a longitudinal study. Addict Biol. 2017;22:1391–401.
Korponay C, Kosson DS, Decety J, Kiehl KA, Koenigs M. Brain volume correlates with duration of abstinence from substance abuse in a region-specific and substance-specific manner. Biol Psychiatry Cogn Neurosci Neuroimaging. 2017;2:626–35.
PubMed PubMed Central Google Scholar
Janes AC, Datko M, Roy A, Barton B, Druker S, Neal C, et al. Quitting starts in the brain: a randomized controlled trial of app-based mindfulness shows decreases in neural responses to smoking cues that predict reductions in smoking. Neuropsychopharmacology. 2019;44:1631–38.
Humphreys K, Bickel WK. Toward a neuroscience of long-term recovery from addiction. JAMA Psychiatry. 2018;75:875–76.
Verhulst B, Neale MC, Kendler KS. The heritability of alcohol use disorders: a meta-analysis of twin and adoption studies. Psychol Med. 2015;45:1061–72.
Goldman D, Oroszi G, Ducci F. The genetics of addictions: uncovering the genes. Nat Rev Genet. 2005;6:521–32.
Kendler KS, Jacobson KC, Prescott CA, Neale MC. Specificity of genetic and environmental risk factors for use and abuse/dependence of cannabis, cocaine, hallucinogens, sedatives, stimulants, and opiates in male twins. AJ Psychiatry. 2003;160:687–95.
Schizophrenia Working Group of the Psychiatric Genomics C. Biological insights from 108 schizophrenia-associated genetic loci. Nature. 2014;511:421–7.
Wray NR, Ripke S, Mattheisen M, Trzaskowski M, Byrne EM, Abdellaoui A, et al. Genome-wide association analyses identify 44 risk variants and refine the genetic architecture of major depression. Nat Genet. 2018;50:668–81.
Wray NR, Lin T, Austin J, McGrath JJ, Hickie IB, Murray GK, et al. From basic science to clinical application of polygenic risk scores: a primer. JAMA Psychiatry. 2021;78:101–9.
Walters RK, Polimanti R, Johnson EC, McClintick JN, Adams MJ, Adkins AE, et al. Transancestral GWAS of alcohol dependence reveals common genetic underpinnings with psychiatric disorders. Nat Neurosci. 2018;21:1656–69.
Visscher PM, Wray NR. Concepts and misconceptions about the polygenic additive model applied to disease. Hum Hered. 2015;80:165–70.
Heilig M, Leggio L. What the alcohol doctor ordered from the neuroscientist: theragnostic biomarkers for personalized treatments. Prog Brain Res. 2016;224:401–18.
Rasmussen K, White DA, Acri JB. NIDA’s medication development priorities in response to the Opioid Crisis: ten most wanted. Neuropsychopharmacology. 2019;44:657–59.
Insel T, Cuthbert B, Garvey M, Heinssen R, Pine DS, Quinn K, et al. Research domain criteria (RDoC): toward a new classification framework for research on mental disorders. AJ Psychiatry. 2010;167:748–51.
Kwako LE, Schwandt ML, Ramchandani VA, Diazgranados N, Koob GF, Volkow ND, et al. Neurofunctional domains derived from deep behavioral phenotyping in alcohol use disorder. AJ Psychiatry. 2019;176:744–53.
Kwako LE, Bickel WK, Goldman D. Addiction biomarkers: dimensional approaches to understanding addiction. Trends Mol Med. 2018;24:121–28.
Xiao P, Dai Z, Zhong J, Zhu Y, Shi H, Pan P. Regional gray matter deficits in alcohol dependence: a meta-analysis of voxel-based morphometry studies. Drug Alcohol Depend. 2015;153:22–8.
Ersche KD, Williams GB, Robbins TW, Bullmore ET. Meta-analysis of structural brain abnormalities associated with stimulant drug dependence and neuroimaging of addiction vulnerability and resilience. Curr Opin Neurobiol. 2013;23:615–24.
Klugah-Brown B, Di X, Zweerings J, Mathiak K, Becker B, Biswal B. Common and separable neural alterations in substance use disorders: a coordinate-based meta-analyses of functional neuroimaging studies in humans. Hum Brain Mapp. 2020;41:4459–77.
Volkow ND, Fowler JS, Wang GJ. The addicted human brain: insights from imaging studies. J Clin Investig. 2003;111:1444–51.
Goldstein RZ, Volkow ND. Dysfunction of the prefrontal cortex in addiction: neuroimaging findings and clinical implications. Nat Rev Neurosci. 2011;12:652–69.
Goodkind M, Eickhoff SB, Oathes DJ, Jiang Y, Chang A, Jones-Hagata LB, et al. Identification of a common neurobiological substrate for mental illness. JAMA Psychiatry. 2015;72:305–15.
Scofield MD, Heinsbroek JA, Gipson CD, Kupchik YM, Spencer S, Smith AC, et al. The nucleus accumbens: mechanisms of addiction across drug classes reflect the importance of glutamate homeostasis. Pharm Rev. 2016;68:816–71.
Korpi ER, den Hollander B, Farooq U, Vashchinkina E, Rajkumar R, Nutt DJ, et al. Mechanisms of action and persistent neuroplasticity by drugs of abuse. Pharm Rev. 2015;67:872–1004.
Luscher C, Malenka RC. Drug-evoked synaptic plasticity in addiction: from molecular changes to circuit remodeling. Neuron. 2011;69:650–63.
Everitt BJ. Neural and psychological mechanisms underlying compulsive drug seeking habits and drug memories–indications for novel treatments of addiction. Eur J Neurosci. 2014;40:2163–82.
Lesscher HM, Vanderschuren LJ. Compulsive drug use and its neural substrates. Rev Neurosci. 2012;23:731–45.
Cruz FC, Koya E, Guez-Barber DH, Bossert JM, Lupica CR, Shaham Y, et al. New technologies for examining the role of neuronal ensembles in drug addiction and fear. Nat Rev Neurosci. 2013;14:743–54.
Crick F. The astonishing hypothesis: the scientific search for the soul. Scribner; Maxwell Macmillan International: New York, NY; 1994.
Vanderschuren LJ, Achterberg EJ, Trezza V. The neurobiology of social play and its rewarding value in rats. Neurosci Biobehav Rev. 2016;70:86–105.
Venniro M, Zhang M, Caprioli D, Hoots JK, Golden SA, Heins C, et al. Volitional social interaction prevents drug addiction in rat models. Nat Neurosci. 2018;21:1520–29.
Martinez D, Orlowska D, Narendran R, Slifstein M, Liu F, Kumar D, et al. Dopamine type 2/3 receptor availability in the striatum and social status in human volunteers. Biol Psychiatry. 2010;67:275–8.
Wiers CE, Shokri-Kojori E, Cabrera E, Cunningham S, Wong C, Tomasi D, et al. Socioeconomic status is associated with striatal dopamine D2/D3 receptors in healthy volunteers but not in cocaine abusers. Neurosci Lett. 2016;617:27–31.
Morgan D, Grant KA, Gage HD, Mach RH, Kaplan JR, Prioleau O, et al. Social dominance in monkeys: dopamine D2 receptors and cocaine self-administration. Nat Neurosci. 2002;5:169–74.
Hughes K, Bellis MA, Hardcastle KA, Sethi D, Butchart A, Mikton C, et al. The effect of multiple adverse childhood experiences on health: a systematic review and meta-analysis. Lancet Public Health. 2017;2:e356–e66.
Gilbert PA, Zemore SE. Discrimination and drinking: a systematic review of the evidence. Soc Sci Med 2016;161:178–94.
Oshri A, Gray JC, Owens MM, Liu S, Duprey EB, Sweet LH, et al. Adverse childhood experiences and amygdalar reduction: high-resolution segmentation reveals associations with subnuclei and psychiatric outcomes. Child Maltreat. 2019;24:400–10.
Holmes CJ, Barton AW, MacKillop J, Galván A, Owens MM, McCormick MJ, et al. Parenting and salience network connectivity among African Americans: a protective pathway for health-risk behaviors. Biol Psychiatry. 2018;84:365–71.
Brody GH, Gray JC, Yu T, Barton AW, Beach SR, Galván A, et al. Protective prevention effects on the association of poverty with brain development. JAMA Pediatr. 2017;171:46–52.
Hanson JL, Gillmore AD, Yu T, Holmes CJ, Hallowell ES, Barton AW, et al. A family focused intervention influences hippocampal-prefrontal connectivity through gains in self-regulation. Child Dev. 2019;90:1389–401.
Borsboom D, Cramer A, Kalis A. Brain disorders? Not really… why network structures block reductionism in psychopathology research. Behav Brain Sci. 2018;42:1–54.
Field M, Heather N, Wiers RW. Indeed, not really a brain disorder: Implications for reductionist accounts of addiction. Behav Brain Sci. 2019;42:e9.
Pascoli V, Hiver A, Van Zessen R, Loureiro M, Achargui R, Harada M, et al. Stochastic synaptic plasticity underlying compulsion in a model of addiction. Nature. 2018;564:366–71.
James W. The dilemma of determinism. Whitefish, MT: Kessinger Publishing; 2005.
Gessell B. Indeterminism in the brain. Biol Philos. 2017;32:1205–23.
Jedlicka P. Revisiting the quantum brain hypothesis: toward quantum (neuro)biology? Front Mol Neurosci. 2017;10:366.
Heyman GM. Addiction: a disorder of choice. Cambridge, MA: Harvard University Press; 2010.
Heather NQ. Is addiction a brain disease or a moral failing? A: Neither. Neuroethics. 2017;10:115–24.
Ahmed SH, Lenoir M, Guillem K. Neurobiology of addiction versus drug use driven by lack of choice. Curr Opin Neurobiol. 2013;23:581–7.
Hogarth L, Lam-Cassettari C, Pacitti H, Currah T, Mahlberg J, Hartley L, et al. Intact goal-directed control in treatment-seeking drug users indexed by outcome-devaluation and Pavlovian to instrumental transfer: critique of habit theory. Eur J Neurosci. 2019;50:2513–25.
Mathis V, Kenny PJ. From controlled to compulsive drug-taking: the role of the habenula in addiction. Neurosci Biobehav Rev. 2019;106:102–11.
Luscher C, Robbins TW, Everitt BJ. The transition to compulsion in addiction. Nat Rev Neurosci. 2020;21:247–63.
Robinson TE, Berridge KC. Addiction. Annu Rev Psychol. 2003;54:25–53.
Everitt BJ, Robbins TW. Neural systems of reinforcement for drug addiction: from actions to habits to compulsion. Nat Neurosci. 2005;8:1481–89.
Koob GF, Volkow ND. Neurocircuitry of addiction. Neuropsychopharmacology. 2010;35:217–38.
Tiffany ST. A cognitive model of drug urges and drug-use behavior: role of automatic and nonautomatic processes. Psychol Rev. 1990;97:147–68.
Robinson TE, Berridge KC. The neural basis of drug craving: an incentive-sensitization theory of addiction. Brain Res Rev. 1993;18:247–91.
Koob GF, Le Moal M. Plasticity of reward neurocircuitry and the ‘dark side’ of drug addiction. Nat Neurosci. 2005;8:1442–4.
Stein DJ, Costa DLC, Lochner C, Miguel EC, Reddy YCJ, Shavitt RG, et al. Obsessive-compulsive disorder. Nat Rev Dis Prim. 2019;5:52.
Vanderschuren LJ, Everitt BJ. Drug seeking becomes compulsive after prolonged cocaine self-administration. Science 2004;305:1017–9.
American_Psychiatric_Association. Diagnostic and statistical manual of mental disorders: DSM-5™. 5th ed. Arlington, VA, US: American Psychiatric Publishing, Inc; 2013.
Book Google Scholar
Spragg SDS. Morphine addiction in chimpanzees. Comp Psychol Monogr. 1940;15:132–32.
Lenoir M, Cantin L, Vanhille N, Serre F, Ahmed SH. Extended heroin access increases heroin choices over a potent nondrug alternative. Neuropsychopharmacology. 2013;38:1209–20.
Caprioli D, Venniro M, Zeric T, Li X, Adhikary S, Madangopal R, et al. Effect of the novel positive allosteric modulator of metabotropic glutamate receptor 2 AZD8529 on incubation of methamphetamine craving after prolonged voluntary abstinence in a rat model. Biol Psychiatry. 2015;78:463–73.
Augier E, Barbier E, Dulman RS, Licheri V, Augier G, Domi E, et al. A molecular mechanism for choosing alcohol over an alternative reward. Science. 2018;360:1321–26.
Bigelow GE. An operant behavioral perspective on alcohol abuse and dependence. In: Heather N, Peters TJ, Stockwell T, editors. International handbook of alcohol dependence and problems. John Wiley & Sons Ltd; 2001. p. 299–315.
Higgins ST, Heil SH, Lussier JP. Clinical implications of reinforcement as a determinant of substance use disorders. Annu Rev Psychol. 2004;55:431–61.
Satel S, Lilienfeld SO. Brainwashed: the seductive appeal of mindless neuroscience. New York, NY: Basic Books; 2015.
Wilson EO. Consilience: the unity of knowledge. New York, NY: Vintage Books; 1999.
Saunders JB, Degenhardt L, Reed GM, Poznyak V. Alcohol use disorders in ICD-11: past, present, and future. Alcohol Clin Exp Res 2019;43:1617–31.
Organization. WH. ICD-11 for mortality and morbidity statistics. 2018. https://icd.who.int/browse11/l-m/en . Accessed 21 Oct 2020.
Babor TF, McRee BG, Kassebaum PA, Grimaldi PL, Ahmed K, Bray J. Screening, brief intervention, and referral to treatment (SBIRT): toward a public health approach to the management of substance abuse. Subst Abus. 2007;28:7–30.
Witkiewitz K, Hallgren KA, Kranzler HR, Mann KF, Hasin DS, Falk DE, et al. Clinical validation of reduced alcohol consumption after treatment for alcohol dependence using the World Health Organization risk drinking levels. Alcohol Clin Exp Res 2017;41:179–86.
Hasin DS, O’Brien CP, Auriacombe M, Borges G, Bucholz K, Budney A, et al. DSM-5 criteria for substance use disorders: recommendations and rationale. AJ Psychiatry. 2013;170:834–51.
Rosenthal RJ, Faris SB. The etymology and early history of ‘addiction’. Addict Res Theory. 2019;27:437–49.
Martin CS, Steinley DL, Verges A, Sher KJ. The proposed 2/11 symptom algorithm for DSM-5 substance-use disorders is too lenient. Psychol Med. 2011;41:2008–10.
Degenhardt L, Bharat C, Bruno R, Glantz MD, Sampson NA, Lago L, et al. Concordance between the diagnostic guidelines for alcohol and cannabis use disorders in the draft ICD-11 and other classification systems: analysis of data from the WHO’s World Mental Health Surveys. Addiction. 2019;114:534–52.
PubMed Google Scholar
Lago L, Bruno R, Degenhardt L. Concordance of ICD-11 and DSM-5 definitions of alcohol and cannabis use disorders: a population survey. Lancet Psychiatry. 2016;3:673–84.
Lundin A, Hallgren M, Forsman M, Forsell Y. Comparison of DSM-5 classifications of alcohol use disorders with those of DSM-IV, DSM-III-R, and ICD-10 in a general population sample in Sweden. J Stud Alcohol Drugs. 2015;76:773–80.
Kwako LE, Momenan R, Litten RZ, Koob GF, Goldman D. Addictions neuroclinical assessment: a neuroscience-based framework for addictive disorders. Biol Psychiatry. 2016;80:179–89.
Rehm J, Heilig M, Gual A. ICD-11 for alcohol use disorders: not a convincing answer to the challenges. Alcohol Clin Exp Res. 2019;43:2296–300.
Download references
Acknowledgements
The authors want to acknowledge comments by Drs. David Epstein, Kenneth Kendler and Naomi Wray.
Author information
Authors and affiliations.
Center for Social and Affective Neuroscience, Department of Biomedical and Clinical Sciences, Linköping University, Linköping, Sweden
Markus Heilig
Peter Boris Centre for Addictions Research, McMaster University and St. Joseph’s Healthcare Hamilton, Hamilton, ON, Canada
- James MacKillop
Homewood Research Institute, Guelph, ON, Canada
New York State Psychiatric Institute and Columbia University Irving Medical Center, New York, NY, USA
Diana Martinez
Institute for Mental Health Policy Research & Campbell Family Mental Health Research Institute, Centre for Addiction and Mental Health (CAMH), Toronto, ON, Canada
Jürgen Rehm
Dalla Lana School of Public Health and Department of Psychiatry, University of Toronto (UofT), Toronto, ON, Canada
Klinische Psychologie & Psychotherapie, Technische Universität Dresden, Dresden, Germany
Department of International Health Projects, Institute for Leadership and Health Management, I.M. Sechenov First Moscow State Medical University, Moscow, Russia
Clinical Psychoneuroendocrinology and Neuropsychopharmacology Section, Translational Addiction Medicine Branch, National Institute on Drug Abuse Intramural Research Program and National Institute on Alcohol Abuse and Alcoholism Division of Intramural Clinical and Biological Research, National Institutes of Health, Baltimore and Bethesda, MD, USA
Lorenzo Leggio
Department of Population Health Sciences, Unit Animals in Science and Society, Faculty of Veterinary Medicine, Utrecht University, Utrecht, the Netherlands
Louk J. M. J. Vanderschuren
You can also search for this author in PubMed Google Scholar
Contributions
All authors jointly drafted the paper.
Corresponding author
Correspondence to Markus Heilig .
Additional information
Publisher’s note Springer Nature remains neutral with regard to jurisdictional claims in published maps and institutional affiliations.
Rights and permissions
Open Access This article is licensed under a Creative Commons Attribution 4.0 International License, which permits use, sharing, adaptation, distribution and reproduction in any medium or format, as long as you give appropriate credit to the original author(s) and the source, provide a link to the Creative Commons license, and indicate if changes were made. The images or other third party material in this article are included in the article’s Creative Commons license, unless indicated otherwise in a credit line to the material. If material is not included in the article’s Creative Commons license and your intended use is not permitted by statutory regulation or exceeds the permitted use, you will need to obtain permission directly from the copyright holder. To view a copy of this license, visit http://creativecommons.org/licenses/by/4.0/ .
Reprints and permissions
About this article
Cite this article.
Heilig, M., MacKillop, J., Martinez, D. et al. Addiction as a brain disease revised: why it still matters, and the need for consilience. Neuropsychopharmacol. 46 , 1715–1723 (2021). https://doi.org/10.1038/s41386-020-00950-y
Download citation
Received : 10 November 2020
Revised : 11 December 2020
Accepted : 14 December 2020
Published : 22 February 2021
Issue Date : September 2021
DOI : https://doi.org/10.1038/s41386-020-00950-y
Share this article
Anyone you share the following link with will be able to read this content:
Sorry, a shareable link is not currently available for this article.
Provided by the Springer Nature SharedIt content-sharing initiative
This article is cited by
Neuromarkers in addiction: definitions, development strategies, and recent advances.
- Nicholas R. Harp
- Tor D. Wager
Journal of Neural Transmission (2024)
Persistent impacts of smoking on resting-state EEG in male chronic smokers and past-smokers with 20 years of abstinence
- Yoonji Jeon
- Dongil Chung
Scientific Reports (2023)
A contextualized reinforcer pathology approach to addiction
- Samuel F. Acuff
- James G. Murphy
Nature Reviews Psychology (2023)
Functional genomic mechanisms of opioid action and opioid use disorder: a systematic review of animal models and human studies
- Camille Falconnier
- Alba Caparros-Roissard
- Pierre-Eric Lutz
Molecular Psychiatry (2023)
A neuromarker for drug and food craving distinguishes drug users from non-users
- Leonie Koban
Nature Neuroscience (2023)
Quick links
- Explore articles by subject
- Guide to authors
- Editorial policies

Change Password
Your password must have 6 characters or more:.
- a lower case character,
- an upper case character,
- a special character
Password Changed Successfully
Your password has been changed
Create your account
Forget yout password.
Enter your email address below and we will send you the reset instructions
If the address matches an existing account you will receive an email with instructions to reset your password
Forgot your Username?
Enter your email address below and we will send you your username
If the address matches an existing account you will receive an email with instructions to retrieve your username

- April 01, 2024 | VOL. 181, NO. 4 CURRENT ISSUE pp.255-346
- March 01, 2024 | VOL. 181, NO. 3 pp.171-254
- February 01, 2024 | VOL. 181, NO. 2 pp.83-170
- January 01, 2024 | VOL. 181, NO. 1 pp.1-82
The American Psychiatric Association (APA) has updated its Privacy Policy and Terms of Use , including with new information specifically addressed to individuals in the European Economic Area. As described in the Privacy Policy and Terms of Use, this website utilizes cookies, including for the purpose of offering an optimal online experience and services tailored to your preferences.
Please read the entire Privacy Policy and Terms of Use. By closing this message, browsing this website, continuing the navigation, or otherwise continuing to use the APA's websites, you confirm that you understand and accept the terms of the Privacy Policy and Terms of Use, including the utilization of cookies.
Neuroscience of Addiction: Relevance to Prevention and Treatment
- Nora D. Volkow , M.D. ,
- Maureen Boyle , Ph.D.
Search for more papers by this author
Addiction, the most severe form of substance use disorder, is a chronic brain disorder molded by strong biosocial factors that has devastating consequences to individuals and to society. Our understanding of substance use disorder has advanced significantly over the last 3 decades in part due to major progress in genetics and neuroscience research and to the development of new technologies, including tools to interrogate molecular changes in specific neuronal populations in animal models of substance use disorder, as well as brain imaging devices to assess brain function and neurochemistry in humans. These advances have illuminated the neurobiological processes through which biological and sociocultural factors contribute to resilience against or vulnerability for drug use and addiction. The delineation of the neurocircuitry disrupted in addiction, which includes circuits that mediate reward and motivation, executive control, and emotional processing, has given us an understanding of the aberrant behaviors displayed by addicted individuals and has provided new targets for treatment. Most prominent are the disruptions of an individual’s ability to prioritize behaviors that result in long-term benefit over those that provide short-term rewards and the increasing difficulty exerting control over these behaviors even when associated with catastrophic consequences. These advances in our understanding of brain development and of the role of genes and environment on brain structure and function have built a foundation on which to develop more effective tools to prevent and treat substance use disorder.
Drug overdoses claimed more than 63,300 American lives in 2016 ( 1 ), and excessive alcohol use and tobacco use are estimated to contribute to 88,000 ( 2 ) and 480,000 ( 3 ) deaths each year, respectively. More than 20 million Americans suffer with substance use disorder, and overdose deaths represent only a fraction of the resultant health consequences. For example, the rate of emergency department visits from opioid-related overdoses or accidents is 20 times greater than the rate of opioid overdose death ( 4 ). Of every 1,000 babies born, six have neonatal abstinence syndrome ( 5 ) and up to nine have fetal alcohol spectrum disorders ( 6 ). Yearly there are approximately 30,500 new cases of hepatitis C ( 7 ), a virus transmitted by injection drug use. The combined consequences of drug use and addiction also have enormous economic consequences, which include an estimated cost of $249 billion from alcohol ( 8 ), $300 billion from tobacco ( 3 ), and $193 billion from other drugs ( 9 ). And while research has identified many evidence-based prevention and treatment strategies that could help reduce alcohol use and drug use and their consequences, these interventions are highly underutilized and not effective for everyone.
Our deepening understanding of the neurobiological, genetic, epigenetic, and environmental mechanisms underlying addiction is helping researchers identify new targets for prevention and treatment interventions. In addition, advancing technologies—from gene sequencing and manipulation, to increasingly more sensitive imaging technologies, to brain stimulation devices, to information technologies and mobile health tools—are producing unprecedented capacity to interrogate addiction and its causes. This growing knowledge base offers unique opportunities for translation of substance use disorder prevention and treatment strategies.
Neurobiology Of Addiction Risk
The risk for addiction is related to complex interactions between biological factors (genetics, epigenetics, developmental attributes, neurocircuitry) and environmental factors (social and cultural systems, stress, trauma, exposure to alternative reinforcers). Research has started to uncover how psychological traits, emotions, and behaviors are encoded in the brain; how environmental factors influence brain circuits and subsequent behavior; and how genetic and epigenetic factors influence the development and functioning of the brain, all of which are of relevance to addiction risk and resilience.
Genetic factors account for approximately half of the risk for addiction ( 10 ). Thus far, most genes implicated in this risk largely influence an individual’s biological response to substances of abuse or their metabolism ( 11 ). Genetic findings have also provided new insight into the neurobiology of addiction. For example, a variant in the gene encoding for the alpha 5 subunit of the nicotinic receptor, which is highly expressed in the habenula, has been consistently associated with higher vulnerability to nicotine addiction ( 12 , 13 ). This discovery brought attention to the importance of the midbrain habenula-interpeduncular axis in nicotine dependence and withdrawal ( 14 ). Further, studies in transgenic mice have documented that the alpha 5 polymorphism influences the firing pattern of pyramidal neurons in the prefrontal cortex in a way that could help explain the greater vulnerability for smoking among patients with schizophrenia ( 15 ). Ongoing research aims to identify how genes mediate the development of the human brain and the subsequent sensitivity of the brain to environmental factors that influence the risk for substance use disorder.
Epigenetic factors, which orchestrate gene expression and silencing, have been implicated in the long-lasting neuroplastic changes associated with drug taking in animal models of addiction ( 16 ), regulating pathways through which environmental risk factors, such as stress, influence biological drivers of substance use and addiction ( 17 ). For example, early life stress can influence the development of the hypothalamic-pituitary-adrenal (HPA) axis, leading to increased reactivity to stress and susceptibility to addiction. Interestingly, preclinical studies have identified transgenerational epigenetic effects of parental drug taking prior to conception on addiction-related behavior in offspring ( 18 , 19 ), though such transgenerational effects have not been demonstrated yet in humans.
Characterization of human neurodevelopment has allowed us to understand why adolescents are more likely than adults to experiment with drugs and to develop substance use disorders. This understanding in part reflects the fact that the adolescent brain has not completed its development and is more neuroplastic than the adult brain. The human brain continues to develop until the early to mid-20s, and the rate of development differs across neuronal circuits, with development occurring faster for reward/motivation and emotional circuits than for prefrontal top-down control circuits, which are among the last to develop ( 20 ). As a result, during adolescence, the striatal reward/motivation and limbic-emotional circuits are hyperactive, leading to greater emotional reactivity and reward-seeking behaviors. Moreover, the prefrontal cortex cannot fully self-regulate, leading to more impulsivity and risk taking ( 21 ). Early exposure to drugs of abuse may further impair the development of the prefrontal cortex, increasing the long-term risk for addiction ( 16 ). The increased neuroplasticity of the adolescent brain explains why addiction develops faster in an adolescent than in an adult ( 22 ), and it also explains the greater sensitivity of adolescents to environmental stimuli, such as stress, that influence drug taking ( 23 , 24 ). Similarly, studies have started to assess the effects of social stressors on development of the human brain, and these studies are relevant for understanding why social stressors increase the risk for substance use disorder and other mental illnesses. For example, studies evaluating the effects of social deprivation during infancy and early childhood have reported delayed maturation that results in impaired brain connectivity, which could underlie increased impulsivity in these children ( 25 ). Importantly, preliminary studies have reported that interventions that provide social support and care may be able to reverse some of these impairments ( 26 ).
Multiple psychological traits have also been shown to influence risk for addiction, including impulsivity, novelty and sensation seeking, and stress reactivity ( 27 ). Neuroscience has started to delineate the brain circuits that mediate these traits. For example, trait impulsivity is associated with dysregulation of corticostriatal circuits as well as altered activation of, and functional connectivity between, the anterior cingulate cortex and amygdala ( 28 ). High sensation seeking has been associated with reduced thickness of the anterior cingulate cortex and middle frontal gyrus ( 29 ), as well as altered midbrain volumes ( 30 ). Stress reactivity is correlated with prefrontal corticolimbic regulation of HPA axis activity ( 31 ). Understanding the neurobiological basis of traits that influence risk for substance use disorder may lead to development of biomarkers that can be used to target prevention interventions for individuals at high risk. Furthermore, this knowledge will facilitate the development of strategies to strengthen specific circuits to improve resilience and support recovery.
Neurobiology Of The Addicted Brain
Brain imaging research has helped map the neuronal circuits that mediate the relapsing pattern of addictive behaviors, including rewarding responses during intoxication, conditioning to the drug and its cues, negative mood and increased stress reactivity during drug withdrawal, and drug craving during exposure to cues or stressors ( 32 ).
During intoxication, the drug stimulates large bursts of dopamine in the mesolimbic reward system (the nucleus accumbens and dorsal striatum) that reinforce drug taking (bingeing) ( 33 ) and strengthen conditioned associations, which link stimuli that precede drug consumption with the expectation of reward ( 34 ). Counterintuitively, in a person suffering from addiction, the drug-induced dopamine increases are attenuated, an effect that has been observed in both human subjects and animal models ( 35 – 37 ). In humans, the attenuated dopamine response to the drug is associated with reduced subjective experience of reward during intoxication ( 37 ). While major emphasis has been placed on the dopaminergic system in explaining the rewarding and reinforcing effects of drugs, it is also clear that other neurotransmitters, including opioids, cannabinoids, GABA, and serotonin—to a greater or lesser extent, depending on the pharmacological characteristics of the drug—contribute to the pleasurable or euphorigenic responses to drugs and to the neuroadaptations that result in addiction ( 38 ).
As the intoxicating effects of a drug wear off, an addicted individual enters the withdrawal phase, which is associated with negative mood, including anhedonia, increased sensitivity to stress, and significant dysphoria and anxiety. Such a response is not typically observed in an individual with short drug exposure history, and the duration of exposure needed for a response to emerge varies for the different types of drugs, with opioids producing these effects particularly rapidly. The circuits underlying the withdrawal phase comprise basal forebrain areas, including the extended amygdala as well as the habenula, and implicate neurotransmitters and neuropeptides such as corticotropin-releasing factor (CRF), norepinephrine, and dynorphin ( 39 , 40 ). Increased signaling in these circuits triggers aversive symptoms that render the individual vulnerable to cravings and preoccupation with taking the drug as means to counteract this aversive state. In parallel, the dopamine reward/motivation system is hypofunctional, contributing to anhedonia and the aversive state during withdrawal ( 41 ).
During the craving stage, the conditioned stimuli (drug cues) themselves elicit dopamine release in the striatum, triggering the motivation to seek and consume the drug ( 42 ). This phase also involves prefrontal circuits, including the orbitofrontal and anterior cingulate cortex, which underlie salience (or value) attribution ( 43 ), as well as circuits in the hippocampus and amygdala, which mediate conditioned responses ( 44 ). Glutamatergic projections from these regions to the ventral tegmental area and striatum modulate the sensitivity and reactivity to cues and to adverse emotions that trigger the urgent motivation for, and preoccupation with, drug taking ( 32 ).
In a brain not affected by addiction, the circuits controlling desire for a drug are held in check by prefrontal cortical regions that underlie executive functions, which support making rational, healthy decisions, and that regulate emotions. Thus, the awareness that a drug will provide an immediate reward is balanced by consideration of long-term goals, and the individual is able to make a reasonable choice and carry it through. However, when the prefrontal cortical circuits underlying executive functions are hypofunctional—as a result of repeated drug exposure or from an underlying vulnerability—and the limbic circuits underlying conditioned responses and stress reactivity are hyperactive—as a result of drug withdrawal and long-term neuroadaptations that downregulate sensitivity to nondrug rewards—the addicted individual is at a tremendous disadvantage in opposing the strong motivation to take the drug. This explains the difficulty addicted individuals face when trying to stop taking drugs even when they experience negative consequences and have become tolerant to the drug’s pleasurable effects.
The delineation of the various brain circuits and neurotransmitters (dopamine, glutamate, dynorphin, enkephalin, GABA, serotonin) that contribute to addiction has helped identify potential targets for addiction treatment (see below). For example, in animal models, interventions to enhance dopamine signaling through D 2 receptors, which are downregulated in addiction and are associated with impaired prefrontal activity, reduce compulsive drug taking ( 41 ). In addition, interventions that counteract the enhanced reactivity of glutamatergic projections from the prefrontal cortex and amygdala to the ventral tegmental area and striatum have been shown to prevent drug taking following exposures to cues or stressors ( 45 ), and those that counteract the negative mood during the withdrawal state (CRF or kappa antagonists) can prevent escalation of drug use ( 46 , 47 ).
In developing new strategies to prevent and treat substance use disorder, it is also important to recognize the high rate of comorbidity with mental illness ( 48 – 50 ). Patients with these comorbidities often have more severe and treatment-resistant disorders compared with patients who have either disorder alone ( 49 , 51 ). Many overlapping brain regions and circuits ( 49 , 50 , 52 , 53 )—including those that mediate reward, executive function, and emotions—and neurotransmitter systems—including dopamine ( 54 – 56 ), serotonin ( 57 , 58 ), glutamate ( 59 , 60 ), GABA ( 61 ), and norepinephrine ( 56 , 62 , 63 )—have been implicated in substance use disorder and other mental illnesses. There is also overlap in the genetic and environmental risk factors for these disorders. Genes that influence stress reactivity, risk taking, and novelty-seeking behaviors can influence the initiation of substance use as well as the development of substance use disorder and other mental illnesses. And environmental factors such as chronic stress, trauma, and adverse childhood experiences increase risk for both substance use disorder and mental illness ( 49 , 64 ).
Accelerating Development Of New Prevention Interventions
There are several evidence-based prevention interventions for substance use disorder that have been developed on the basis of epidemiological data identifying factors that increase risk for or provide resilience against substance use disorder ( Table 1 ).
a Strategies include decreasing risk factors and enhancing protective factors at the individual, family, community or school, and societal levels. Modified from reference 65 .
TABLE 1. Strategies for Prevention of Substance Use Disorder a
Our increased understanding of the effects of substance use on normal brain development, the deleterious effects of adverse environments, and the role of innate vulnerabilities will allow for the development of personalized intervention to reverse or mitigate some of these deficits. For example, adverse social environments during early childhood can result in delayed prefrontal limbic connectivity ( 25 ), which is associated with impulsivity ( 66 ). In turn, impulsivity predicts greater vulnerability for substance use disorder ( 67 ). However, children can be trained to improve their self-regulation and hence control impulsivity ( 68 ). Furthermore, social isolation and exposure to social environments with limited support are associated with reduced dopamine D 2 receptor expression in the striatum, which is linked with greater vulnerability for impulsivity and compulsive drug use ( 69 – 71 ). Research is also starting to identify changes in brain development triggered by early exposure to drugs, including alcohol and marijuana ( 72 , 73 ). Future access to standardized measures of brain development will support the development of early interventions to mitigate developmental vulnerabilities or counteract negative neuroadaptations. In this respect, the recently launched Adolescent Brain Cognitive Development Study, which aims to study 10,000 children with brain imaging, genotyping, and deep phenotyping across the transition from childhood into adulthood, will provide valuable data for determining normal human variability in brain development and how it is disrupted by drug use and mental illnesses ( 74 ). Similarly, the Baby Connectome Project, a study of brain development in children from birth through 5 years of age ( 75 ), will provide insight into the early development of the human brain at a stage when it is most sensitive to adverse environmental effects, such as neglect and abuse.
Accelerating Development Of New Treatments
The substance use disorder treatment field has seen some important successes, but therapeutic options are still limited. Although the Food and Drug Administration (FDA) has approved medications for the treatment of opioid, alcohol, and tobacco use disorders ( Table 2 ) ( 77 ), which represent meaningful advances in therapeutics for addictions, they are not effective for all patients. Similarly, while evidence-based psychosocial treatments (e.g., cognitive-behavioral therapy, contingency management interventions) are available for substance use disorder, their effectiveness is also limited ( 78 ).
a Modified from reference 65 . DEA=Drug Enforcement Administration.
b One-week buprenorphine injection is being evaluated by the Food and Drug Administration.
TABLE 2. Medications for Addiction Treatment a
Most pharmaceutical companies have been reticent to invest in addiction due in part to stigma ( 79 ) as well as to perceptions that the market is small, that executing clinical trials in patients with substance use disorders is difficult (because of frequent comorbidities, criminal or legal problems, and poor adherence to treatment protocols), and that the regulatory bar required for FDA approval is too high (i.e., abstinence—discussed below [ 80 ]). These factors represent a major challenge in medication development. Consequently, the National Institute on Drug Abuse (NIDA) has focused on partnering with industry to encourage drug development by identifying promising targets and funding research to lessen risks associated with drug development. Examples of such partnerships include helping in the development of medications to treat opioid use disorders, such as a buprenorphine/naloxone combination (Suboxone) ( 81 ), a 1-month extended-release naltrexone (Vivitrol), a 6-month buprenorphine subdermal implant (Probuphine) ( 82 ), and a “user-friendly” intranasal opioid overdose naloxone formulation (Narcan Nasal Spray) ( 83 ). The current opioid crisis has further highlighted the urgency for greater participation of industry in medication development ( 84 ).
In parallel, research is ongoing to translate basic knowledge about the molecular pathways and brain circuits involved in substance use disorders into new treatments. A promising strategy explores the use of pharmacotherapies that target endophenotypes associated with addiction—for example, using cognitive enhancers to improve impulse control, planning, and decision making ( 85 – 87 ), and using medications to reduce stress reactivity and dysphoria to prevent relapses ( 88 , 89 ). As discussed above, various neurotransmitter systems are involved in such cognitive and emotional functions and represent potential therapeutic targets.
Another strategy involves “repurposing” medications already approved for other indications. Because there is overlap in the neuropathology and symptomatology between substance use disorder and other mental illnesses (see the section “Neurobiology of the Addicted Brain”), and many of the medications used to treat mental illnesses bind to multiple therapeutic targets ( 90 ), some of these may be beneficial for substance use disorders. The medications’ existing safety profiles and pharmacology data shorten the timeline for obtaining FDA approval if the drugs are found effective for treating substance use disorders. A notable example is bupropion, which was originally developed as an antidepressant and was subsequently found to be clinically useful in smoking cessation ( 91 ).
Although there is only one approved drug combination for substance use disorders (i.e., the buprenorphine/naloxone combination), the few studies evaluating other drug combinations have shown promising results. For example, the combination of buprenorphine with naltrexone not only decreased cocaine use in individuals with dual cocaine and heroin use disorders ( 92 ) but also reduced heroin use to a greater extent than buprenorphine alone ( 93 ). Similarly, the combination of lofexidine and dronabinol reduced symptoms of cannabis withdrawal significantly more than either medication alone ( 94 ).
Research is also ongoing to define endpoints other than abstinence for measuring treatment efficacy, such as clinically meaningful reductions in drug use associated with improvements in health outcomes ( 95 ). Such adjustments could reduce the regulatory bar for obtaining FDA approval for new therapeutics. For example, research has indicated that reduced use of cocaine led to decreased endothelial dysfunction, a marker of heart-disease risk associated with chronic cocaine use ( 96 ). Importantly, there is precedent for alternative endpoints: the FDA has approved the use of percentage of subjects with no heavy drinking days as an outcome for alcohol use disorder. This measurement is sensitive for detecting differences between medication and placebo, and allowing some days of consumption increases the effect size ( 97 ). In the case of opioid use disorders, a relevant endpoint could be overdose prevention; recently, a study on parolees found reduced overdoses were associated with extended-release naltrexone ( 98 ).
Promising Pharmacological Targets
The preclinical literature includes a wide range of promising strategies for substance use disorders that aim either at the main protein target of specific drugs, at modulators of the brain reward system, or at modulators of downstream circuits disrupted in addiction ( Figure 1 ).
FIGURE 1. The Three Stages of the Addiction Cycle and the Main Brain Regions Implicated a
a Potential medication targets and therapeutic strategies that target each stage are listed. KOR=kappa-opioid receptor; NK1=neurokinin 1; tDCS=transcranial direct current stimulation; TMS=transcranial magnetic stimulation. Modified from reference 65 .
Therapeutic strategies that target the mu-opioid receptor have been most effective for opioid use disorders and are being pursued in the development of nonaddictive analgesics, which could help reduce the risk of addiction as an unintended consequence of pain management ( 84 ). In this respect, the recent identification of the structure of the mu-opioid receptor has provided novel insights into mechanisms of tolerance and is facilitating development of medications that target specific intracellular signaling pathways of the mu-opioid receptor, referred to as biased agonists. Biased opioid agonists that are developed as analgesics target the G-coupled protein “G i ” intracellular pathway, which is believed to underlie analgesia, while not engaging the β-arrestin pathway, which is associated with tolerance and the respiratory-depressing effects of opioid agonists ( 99 ). Phase 3 clinical testing of a mu-opioid receptor biased agonist (TRV130) is under way ( 100 , 101 ). In addition, the orvinol analogue BU08028, a compound similar to buprenorphine, has been shown to be a safe opioid analgesic without abuse liability in nonhuman primates ( 102 ). Research into these and other opioids ( 103 ) is poised to lead to improved treatments for opioid use disorders and pain.
Novel pharmacological approaches for treating opioid use disorders that do not involve the mu-opioid receptor include strategies to modulate the reward circuit via antagonism of the neurokinin 1 receptor ( 104 ) and to counteract withdrawal via antagonism of the kappa-opioid receptor ( 89 ). Lofexidine, an α 2A -adrenergic receptor agonist originally developed as an antihypertensive, also decreases opioid withdrawal symptoms and is undergoing NIDA-funded trials ( 105 , 106 ). Another promising medication is lorcaserin, a selective 5-HT 2C receptor agonist already FDA-approved for weight loss that reduces opioid seeking in rodent models ( 107 ).
Oxytocin, a neuropeptide known for its role in social bonding, is also of interest for substance use disorder. Oxytocin-expressing neurons project to brain regions implicated in reward (including the ventral tegmental area and nucleus accumbens) ( 108 , 109 ) and stress (including the amygdala and hippocampus) ( 108 , 109 ). Preclinical studies have shown that oxytocin decreases self-administration of heroin ( 110 ), cocaine ( 111 , 112 ), methamphetamine ( 113 ), and alcohol ( 114 – 116 ) and also alleviates nicotine withdrawal ( 117 ). Oxytocin treatment during adolescence also reduced methamphetamine ( 118 ) and alcohol ( 119 ) seeking in adult rodents. In addition, oxytocin reduced reinstatement of drug seeking in rodents in response to triggers of drug craving for methamphetamine ( 120 – 122 ) and cocaine ( 112 , 123 , 124 ). In humans, intranasal oxytocin reduces cue-induced craving for nicotine ( 125 ), stress-induced craving for marijuana ( 126 ), and withdrawal and anxiety symptoms.
Cannabinoids may also be useful for treating substance use disorders, and identifying medications that target the endocannabinoid system without producing cognitive impairment and rewarding effects could lead to new treatments for substance use disorders. For example, dronabinol (a synthetic tetrahydrocannabinol [THC] formulation approved for AIDS-related anorexia and chemotherapy-related nausea) reduced withdrawal symptoms associated with cannabis use disorder ( 127 ), and nabilone (a synthetic cannabinoid similar to THC) reduced cannabis withdrawal- and relapse-related measures in human laboratory studies ( 128 ). In addition, a study of nabiximol (an oral mucosal spray containing a THC-to-cannabidiol [CBD] ratio of 1:1) found reductions in severity and duration of cannabis withdrawal and increased retention in treatment ( 129 ). Finally, an ongoing randomized clinical trial will evaluate whether CBD (Epidiolex), when added to medical management, can improve treatment outcomes for cannabis use disorders ( 130 ).
The body’s endogenous cannabinoids (anandamide and 2-arachidonoylglycerol, or 2-AG, which interact with cannabinoid receptors CB1R and CB2R) optimize the inhibitory and excitatory balance in the brain in a state-dependent manner ( 131 ), so side effects might occur with orthosteric ligands that either activate (e.g., dronabinol) or block (e.g., rimonabant) CB1R broadly. For example, rimonabant, which showed efficacy in treating obesity and inhibiting the rewarding effects of cannabis, was also associated with negative affect and suicidal ideation ( 132 ). Instead, the manipulation of cannabinoid receptors (CB1R or CB2R) via allosteric modulators, which simply enhance or inhibit receptor responses to endocannabinoids, maintaining the state dependence of the endocannabinoid system, may be more promising for medication development. Although the development of CB1R allosteric modulator medications is in its infancy ( 133 ), the negative allosteric modulator pregnenolone appears to protect against the intoxicating effects of THC ( 134 ) and is being evaluated for the treatment of cannabis use disorder. In addition, the positive allosteric CB1R modulator ZCZ011 has antinociceptive effects without being reinforcing ( 135 ) and thus holds potential as a nonaddictive analgesic.
Other endocannabinoid system modulators being evaluated for cannabis use disorder include specific inhibitors of fatty acid amide hydrolase or of monoglycerol lipase, which slow the breakdown of endocannabinoids, as these compounds may reduce withdrawal symptoms and/or may lead to new nonaddictive analgesics ( 136 , 137 ).
Stimulant use disorders have been among the most challenging for therapeutics development. The most investigated strategy has been the use of longer-acting stimulant medications (e.g., methylphenidate and amphetamines) for cocaine addiction. Many of these studies have failed to reach significant positive outcomes, except in individuals with comorbid attention deficit hyperactivity disorder. However, a meta-analysis reported evidence of mild benefit for the use of amphetamines in cocaine addiction ( 138 ). Similarly, modafinil, a medication used for narcolepsy that has mild stimulant properties ( 139 ), has been shown by some studies to be beneficial for the treatment of cocaine addiction ( 140 ), though not by others ( 141 ). A different approach, based on studies documenting that enhanced glutamatergic signaling from limbic and ventral prefrontal regions can drive cue-induced cravings and relapse ( 142 ), targets medications that can help restore balance to these glutamatergic projections. For example, N -acetylcysteine, which helps modulate glutamate signals by activating the cystine-glutamate exchange and thereby stimulating extrasynaptic metabotropic glutamate receptors ( 143 ), decreases cocaine seeking in animal models ( 144 ). N -acetylcysteine is well tolerated in cocaine-dependent individuals and may reduce cocaine-related withdrawal symptoms and craving ( 145 ). A clinical trial did not find N -acetylcysteine to be effective for patients actively using cocaine; however, N -acetylcysteine reduced cravings and prevented relapse in patients who had achieved abstinence ( 146 ). The efficacy of N -acetylcysteine is limited by low bioavailability and poor permeability of the blood-brain barrier. Similar compounds with greater potency and bioavailability, such as N -acetylcysteine amide, may prove more effective ( 147 ).
Strategies to deliver degradative enzymes have also been proposed for cocaine use disorders, and research is ongoing to develop stable, long-lasting forms of cocaine-degrading enzymes, including cocaine esterase, cocaine hydrolase ( 148 – 151 ), and butyrylcholinesterase ( 152 ). Preclinical evidence has shown that these fusion proteins increase cocaine’s metabolism ( 153 , 154 ). However, clinical studies have failed to show efficacy ( 152 ).
Antiepileptic drugs have also been evaluated in the management of substance use disorder. A proposed mechanism for their benefit is that in cocaine use disorders, and in other substance use disorders, dysfunction of GABA-ergic signaling contributes to drug taking ( 155 , 156 ). Gabapentin, a widely prescribed anticonvulsant and pain medication, has shown some benefits in the treatment of alcohol use disorders ( 157 ) and might reduce cannabis use and withdrawal symptoms in cannabis use disorders. Topiramate is an antiepileptic medication ( 158 ) for which there is preliminary clinical evidence of reduced cocaine use and improved outcomes in cocaine-dependent individuals ( 159 ). In methamphetamine users, topiramate reduced the amount of drug taken by patients with an active use disorder and reduced relapse rates among those in recovery ( 160 ).
Promising Nonpharmacological Therapies
Alternative nonpharmacological strategies include vaccines and other biologics (i.e., monoclonal antibodies and gene-delivery strategies); neural stimulation technologies, such as transcranial magnetic stimulation (TMS), transcranial direct current stimulation (tDCS), deep-brain stimulation, and peripheral stimulation devices; and behavioral interventions.
Biological therapeutics.
Vaccines and passive immunization with antibodies work by binding to the drug in the blood and preventing it from entering the brain. Preclinical studies have shown encouraging results for vaccines against prescription opioids, heroin, and fentanyl, inducing high-titer antibody responses to opioids ( 161 – 163 ). Clinical studies both with cocaine and nicotine vaccines have resulted in insufficient antibody titers in humans, and further work is needed ( 164 – 166 ).
A similar approach involves passive immunization, or treating patients with monoclonal antibodies. Monoclonal antibodies can be delivered in high concentrations, and the dosing can be more precisely controlled than with vaccines. An anti-methamphetamine monoclonal antibody (ch-mAb7F9) was found to be safe and well tolerated in phase 1 trials ( 167 ). Research is ongoing to develop monoclonal antibodies for fentanyl, nicotine, and cocaine ( 168 , 169 ). Production of long-lasting monoclonal or polyclonal antibodies against very potent synthetic opioids (e.g., fentanyl and its analogs) could be beneficial to prevent relapse and help prevent overdoses, which are currently driving increases in fatalities in the United States ( 1 , 170 ).
Brain and peripheral stimulation therapeutics.
The identification of the neuronal circuits affected in addiction can also suggest therapeutic targets for brain stimulation strategies, such as TMS and tDCS. Examples include the strengthening of prefrontal activity to improve self-regulation or the modulation of limbic pathways to reduce incentive salience and cravings.
TMS uses repetitive pulses of a magnetic field, and tDCS uses weak electrical currents through electrodes placed on the scalp to modulate the activity of targeted brain regions. Preliminary clinical trials have reported positive results with TMS for the treatment of nicotine, alcohol, and cocaine use disorders and with tDCS for nicotine, alcohol, cocaine, methamphetamine, opioid, and cannabis use disorders, with reductions in cravings, use, or both reported ( 171 – 173 ). Most studies have targeted the dorsolateral prefrontal cortex, although some studies have targeted the anterior cingulate cortex, the insular cortex, the frontal-parietal-temporal area, and the ventromedial prefrontal cortex. The small sample sizes, short durations, and heterogeneity in technical protocols (e.g., frequency, duration, hemisphere targeted) limit the conclusions that can be drawn. However, existing research suggests these technologies have significant potential for the treatment of addiction.
Other nonpharmacological strategies include peripheral nerve stimulation and neurofeedback strategies. An example of a peripheral nerve stimulator is the BRIDGE, an FDA-approved device for the treatment of pain, which is being explored as a treatment to reduce opioid withdrawal symptoms and facilitate induction on opioid use disorder medications ( 174 ). Neurofeedback strategies train patients to regulate their own brain activity using real-time feedback from functional magnetic resonance imaging or EEG. These strategies can be used in combination with behavioral interventions to help improve executive function in addicted individuals ( 175 ). A few small studies have found positive results with EEG neurofeedback in individuals with alcohol and cocaine use disorder ( 176 , 177 ). Although these treatments are still in their infancy, these studies are promising and may lead to treatment advances.
Behavioral therapies.
There is also a significant body of research describing the efficacy of behavioral interventions for substance use disorder. Currently, these represent the only interventions available to treat stimulant, cannabis, and hallucinogen use disorders. Understanding the neurobiological mechanisms that underlie their efficacy is important for guiding the refinement of behavioral treatment strategies.
The Promise Of Basic Research
Basic research is the foundation for future advancements in addiction prevention and treatment. A sustained focus in this area will help researchers define the pathways from gene variation to molecular profile, neuron function, brain-circuit activity, and ultimately to disordered behavior, revealing new targets for prevention and treatment interventions.
While research in animal models has contributed to the development of medications for alcohol, opioid, and tobacco use disorders, it often fails to predict efficacy in clinical trials. This may reflect the reliance on abstinence as the primary endpoint in clinical trials for substance use disorder. The use of alternative outcomes may lead to greater correlation of findings. In parallel, researchers are incorporating more complex social environments into experiments testing medications in animal models that might increase their predictive validity.
The prevention and treatment of substance use disorders would also benefit from biomarkers to help classify individuals into biologically based categories that are reproducible and have predictive validity ( 178 ). Biomarkers for the detection of drug exposures in body fluids are valuable but can be used to corroborate only acute or relatively recent drug use. Thus, research is needed to develop and validate biomarkers that reflect chronic drug exposure and that predict disease trajectories and treatment responses. Advances in genetic, epigenetic, and brain imaging tools and technologies offer unprecedented opportunities for the development of such biomarkers.
The same neuroimaging tools that have expanded our understanding of the structural and functional deficits underlying addiction may one day be deployed to monitor, optimize, and personalize addiction treatment ( 175 ). An individual’s environment, experience, and biology combine to determine his or her risk for developing a substance use disorder, the trajectory the substance use disorder will take, and the interventions that will be most effective for treating it. Large, national investments in basic research, including the Brain Research Through Advancing Innovative Neurotechnologies (BRAIN) Initiative ( 179 ), the Adolescent Brain Cognitive Development study ( 74 ), and the Precision Medicine Initiative ( 180 ), a prospective study that aims to genotype and phenotype one million Americans, are poised to bridge the gap between neuroscience, genetics, behavioral research, and personalized interventions for the prevention and treatment of substance use disorder.
Conclusions
Scientific advances have revolutionized our understanding of the biological and psychosocial drivers of addiction. There is tremendous potential to translate this vast knowledge base into meaningful advances in the prevention and treatment of substance use disorder that will benefit not only addiction medicine but the multiplicity of health conditions that are triggered or exacerbated by drug use ( 181 ).
The authors report no financial relationships with commercial interests.
1 Hedegaard H, Warner M, Miniño AM: Drug Overdose Deaths in the United States, 1999–2016. National Center for Health Statistics Data Brief No. 294, December 2017. https://www.cdc.gov/nchs/products/databriefs/db294.htm Google Scholar
2 Stahre M, Roeber J, Kanny D, et al. : Contribution of excessive alcohol consumption to deaths and years of potential life lost in the United States . Prev Chronic Dis 2014 ; 11:E109 Crossref , Medline , Google Scholar
3 National Center for Chronic Disease Prevention and Health Promotion, Office on Smoking and Health : The Health Consequences of Smoking: 50 Years of Progress. A Report of the Surgeon General . Atlanta, Centers for Disease Control and Prevention, 2014 Google Scholar
4 Weiss AJ, Bailey MK, O’Malley L, et al. : Patient Characteristics of Opioid-Related Inpatient Stays and Emergency Department Visits Nationally and by State, 2014 . Rockville, Md, Agency for Healthcare Research and Quality, 2017 , p 224 Google Scholar
5 Patrick SW, Davis MM, Lehmann CU, et al. : Increasing incidence and geographic distribution of neonatal abstinence syndrome: United States 2009 to 2012 . J Perinatol 2015 ; 35:650–655 Crossref , Medline , Google Scholar
6 Centers for Disease Control and Prevention: Fetal Alcohol Spectrum Disorders (FASDs). https://www.cdc.gov/ncbddd/fasd/data.html Google Scholar
7 Centers for Disease Control and Prevention: Surveillance for Viral Hepatitis: United States, 2014. https://www.cdc.gov/hepatitis/statistics/2014surveillance/commentary.htm Google Scholar
8 Sacks JJ, Gonzales KR, Bouchery EE, et al. : 2010 national and state costs of excessive alcohol consumption . Am J Prev Med 2015 ; 49:e73–e79 Crossref , Medline , Google Scholar
9 National Drug Intelligence Center, US Department of Justice: The Economic Impact of Illicit Drug Use on American Society: 2011. https://www.justice.gov/archive/ndic/pubs44/44731/44731p.pdf Google Scholar
10 Volkow N, Li T-K : The neuroscience of addiction . Nat Neurosci 2005 ; 8:1429–1430 Crossref , Medline , Google Scholar
11 Yu C, McClellan J : Genetics of substance use disorders . Child Adolesc Psychiatr Clin N Am 2016 ; 25:377–385 Crossref , Medline , Google Scholar
12 Etter JF, Hoda JC, Perroud N, et al. : Association of genes coding for the alpha-4, alpha-5, beta-2, and beta-3 subunits of nicotinic receptors with cigarette smoking and nicotine dependence . Addict Behav 2009 ; 34:772–775 Crossref , Medline , Google Scholar
13 Bierut LJ, Madden PA, Breslau N, et al. : Novel genes identified in a high-density genome-wide association study for nicotine dependence . Hum Mol Genet 2007 ; 16:24–35 Crossref , Medline , Google Scholar
14 Görlich A, Antolin-Fontes B, Ables JL, et al. : Reexposure to nicotine during withdrawal increases the pacemaking activity of cholinergic habenular neurons . Proc Natl Acad Sci USA 2013 ; 110:17077–17082 Crossref , Medline , Google Scholar
15 Koukouli F, Rooy M, Tziotis D, et al. : Nicotine reverses hypofrontality in animal models of addiction and schizophrenia . Nat Med 2017 ; 23:347–354 Crossref , Medline , Google Scholar
16 Nestler EJ : Epigenetic mechanisms of drug addiction . Neuropharmacology 2014 ;76 Pt B:259–268 Crossref , Medline , Google Scholar
17 Doura MB, Unterwald EM : MicroRNAs modulate interactions between stress and risk for cocaine addiction . Front Cell Neurosci 2016 ; 10:125 Crossref , Medline , Google Scholar
18 Watson CT, Szutorisz H, Garg P, et al. : Genome-wide DNA methylation profiling reveals epigenetic changes in the rat nucleus accumbens associated with cross-generational effects of adolescent THC exposure . Neuropsychopharmacology 2015 ; 40:2993–3005 Crossref , Medline , Google Scholar
19 Vassoler FM, Oliver DJ, Wyse C, et al. : Transgenerational attenuation of opioid self-administration as a consequence of adolescent morphine exposure . Neuropharmacology 2017 ; 113(Pt A):271–280 Crossref , Medline , Google Scholar
20 Giedd JN : The teen brain: insights from neuroimaging . J Adolesc Health 2008 ; 42:335–343 Crossref , Medline , Google Scholar
21 Jordan CJ, Andersen SL : Sensitive periods of substance abuse: early risk for the transition to dependence . Dev Cogn Neurosci 2017 ; 25:29–44 Crossref , Medline , Google Scholar
22 Whitaker LR, Degoulet M, Morikawa H : Social deprivation enhances VTA synaptic plasticity and drug-induced contextual learning . Neuron 2013 ; 77:335–345 Crossref , Medline , Google Scholar
23 Eiland L, Romeo RD : Stress and the developing adolescent brain . Neuroscience 2013 ; 249:162–171 Crossref , Medline , Google Scholar
24 Lewis CR, Olive MF : Early-life stress interactions with the epigenome: potential mechanisms driving vulnerability toward psychiatric illness . Behav Pharmacol 2014 ; 25:341–351 Medline , Google Scholar
25 Govindan RM, Behen ME, Helder E, et al. : Altered water diffusivity in cortical association tracts in children with early deprivation identified with Tract-Based Spatial Statistics (TBSS) . Cereb Cortex 2010 ; 20:561–569 Crossref , Medline , Google Scholar
26 Sheridan MA, Fox NA, Zeanah CH, et al. : Variation in neural development as a result of exposure to institutionalization early in childhood . Proc Natl Acad Sci USA 2012 ; 109:12927–12932 Crossref , Medline , Google Scholar
27 Jasinska AJ, Stein EA, Kaiser J, et al. : Factors modulating neural reactivity to drug cues in addiction: a survey of human neuroimaging studies . Neurosci Biobehav Rev 2014 ; 38:1–16 Crossref , Medline , Google Scholar
28 Bickel WK, Jarmolowicz DP, Mueller ET, et al. : Are executive function and impulsivity antipodes? A conceptual reconstruction with special reference to addiction . Psychopharmacology (Berl) 2012 ; 221:361–387 Crossref , Medline , Google Scholar
29 Holmes AJ, Hollinshead MO, Roffman JL, et al. : Individual differences in cognitive control circuit anatomy link sensation seeking, impulsivity, and substance use . J Neurosci 2016 ; 36:4038–4049 Crossref , Medline , Google Scholar
30 Cheng GLF, Liu Y-P, Chan CCH, et al. : Neurobiological underpinnings of sensation seeking trait in heroin abusers . Eur Neuropsychopharmacol 2015 ; 25:1968–1980 Crossref , Medline , Google Scholar
31 Sinha R : Chronic stress, drug use, and vulnerability to addiction . Ann N Y Acad Sci 2008 ; 1141:105–130 Crossref , Medline , Google Scholar
32 Volkow ND, Koob GF, McLellan AT : Neurobiologic advances from the brain disease model of addiction . N Engl J Med 2016 ; 374:363–371 Crossref , Medline , Google Scholar
33 Di Chiara G : Nucleus accumbens shell and core dopamine: differential role in behavior and addiction . Behav Brain Res 2002 ; 137:75–114 Crossref , Medline , Google Scholar
34 Volkow ND, Baler RD : NOW vs LATER brain circuits: implications for obesity and addiction . Trends Neurosci 2015 ; 38:345–352 Crossref , Medline , Google Scholar
35 Willuhn I, Burgeno LM, Groblewski PA, et al. : Excessive cocaine use results from decreased phasic dopamine signaling in the striatum . Nat Neurosci 2014 ; 17:704–709 Crossref , Medline , Google Scholar
36 Trantham-Davidson H, Chandler LJ : Alcohol-induced alterations in dopamine modulation of prefrontal activity . Alcohol 2015 ; 49:773–779 Crossref , Medline , Google Scholar
37 Volkow ND, Wang G-J, Fowler JS, et al. : Addiction: decreased reward sensitivity and increased expectation sensitivity conspire to overwhelm the brain’s control circuit . BioEssays 2010 ; 32:748–755 Crossref , Medline , Google Scholar
38 Lovinger DM : Neurobiological basis of drug reward and reinforcement , in Addiction Medicine: Science and Practice . Edited by Johnson BA . New York, Springer, 2011 , pp 255–281 Google Scholar
39 Koob GF, Le Moal M : Plasticity of reward neurocircuitry and the ‘dark side’ of drug addiction . Nat Neurosci 2005 ; 8:1442–1444 Crossref , Medline , Google Scholar
40 Batalla A, Homberg JR, Lipina TV, et al. : The role of the habenula in the transition from reward to misery in substance use and mood disorders . Neurosci Biobehav Rev 2017 ; 80:276–285 Crossref , Medline , Google Scholar
41 Volkow ND, Morales M : The brain on drugs: from reward to addiction . Cell 2015 ; 162:712–725 Crossref , Medline , Google Scholar
42 Volkow ND, Wang GJ, Telang F, et al. : Cocaine cues and dopamine in dorsal striatum: mechanism of craving in cocaine addiction . J Neurosci 2006 ; 26:6583–6588 Crossref , Medline , Google Scholar
43 Volkow ND, Wang GJ, Ma Y, et al. : Activation of orbital and medial prefrontal cortex by methylphenidate in cocaine-addicted subjects but not in controls: relevance to addiction . J Neurosci 2005 ; 25:3932–3939 Crossref , Medline , Google Scholar
44 Robbins TW, Ersche KD, Everitt BJ : Drug addiction and the memory systems of the brain . Ann N Y Acad Sci 2008 ; 1141:1–21 Crossref , Medline , Google Scholar
45 Kalivas PW, Volkow ND : New medications for drug addiction hiding in glutamatergic neuroplasticity . Mol Psychiatry 2011 ; 16:974–986 Crossref , Medline , Google Scholar
46 Park PE, Schlosburg JE, Vendruscolo LF, et al. : Chronic CRF1 receptor blockade reduces heroin intake escalation and dependence-induced hyperalgesia . Addict Biol 2015 ; 20:275–284 Crossref , Medline , Google Scholar
47 Schlosburg JE, Whitfield TW Jr, Park PE, et al. : Long-term antagonism of κ opioid receptors prevents escalation of and increased motivation for heroin intake . J Neurosci 2013 ; 33:19384–19392 Crossref , Medline , Google Scholar
48 Kessler RC : The epidemiology of dual diagnosis . Biol Psychiatry 2004 ; 56:730–737 Crossref , Medline , Google Scholar
49 Kelly TM, Daley DC : Integrated treatment of substance use and psychiatric disorders . Soc Work Public Health 2013 ; 28:388–406 Crossref , Medline , Google Scholar
50 Ross S, Peselow E : Co-occurring psychotic and addictive disorders: neurobiology and diagnosis . Clin Neuropharmacol 2012 ; 35:235–243 Crossref , Medline , Google Scholar
51 Torrens M, Rossi PC, Martinez-Riera R, et al. : Psychiatric co-morbidity and substance use disorders: treatment in parallel systems or in one integrated system? Subst Use Misuse 2012 ; 47:1005–1014 Crossref , Medline , Google Scholar
52 Wing VC, Wass CE, Soh DW, et al. : A review of neurobiological vulnerability factors and treatment implications for comorbid tobacco dependence in schizophrenia . Ann N Y Acad Sci 2012 ; 1248:89–106 Crossref , Medline , Google Scholar
53 Tsuang MT, Francis T, Minor K, et al. : Genetics of smoking and depression . Hum Genet 2012 ; 131:905–915 Crossref , Medline , Google Scholar
54 Howes OD, Fusar-Poli P, Bloomfield M, et al. : From the prodrome to chronic schizophrenia: the neurobiology underlying psychotic symptoms and cognitive impairments . Curr Pharm Des 2012 ; 18:459–465 Crossref , Medline , Google Scholar
55 Volkow ND, Fowler JS, Wang GJ, et al. : Dopamine in drug abuse and addiction: results of imaging studies and treatment implications . Arch Neurol 2007 ; 64:1575–1579 Crossref , Medline , Google Scholar
56 Xing B, Li YC, Gao WJ : Norepinephrine versus dopamine and their interaction in modulating synaptic function in the prefrontal cortex . Brain Res 2016 ; 1641(Pt B):217–233 Crossref , Medline , Google Scholar
57 Marazziti D : Understanding the role of serotonin in psychiatric diseases . F1000 Res 2017 ; 6:180 Crossref , Medline , Google Scholar
58 Müller CP, Homberg JR : The role of serotonin in drug use and addiction . Behav Brain Res 2015 ; 277:146–192 Crossref , Medline , Google Scholar
59 Tsapakis EM : Glutamate and psychiatric disorders . Adv Psychiatr Treat 2002 ; 8:189–197 Crossref , Google Scholar
60 Tzschentke TM, Schmidt WJ : Glutamatergic mechanisms in addiction . Mol Psychiatry 2003 ; 8:373–382 Crossref , Medline , Google Scholar
61 Kumar K, Sharma S, Kumar P, et al. : Therapeutic potential of GABA(B) receptor ligands in drug addiction, anxiety, depression, and other CNS disorders . Pharmacol Biochem Behav 2013 ; 110:174–184 Crossref , Medline , Google Scholar
62 Aston-Jones G, Kalivas PW : Brain norepinephrine rediscovered in addiction research . Biol Psychiatry 2008 ; 63:1005–1006 Crossref , Medline , Google Scholar
63 Langer SZ : α2-Adrenoceptors in the treatment of major neuropsychiatric disorders . Trends Pharmacol Sci 2015 ; 36:196–202 Crossref , Medline , Google Scholar
64 Enoch MA : The influence of gene-environment interactions on the development of alcoholism and drug dependence . Curr Psychiatry Rep 2012 ; 14:150–158 Crossref , Medline , Google Scholar
65 US Department of Health and Human Services: Surgeon General’s Report on Alcohol, Drugs, and Health, 2016. https://addiction.surgeongeneral.gov Google Scholar
66 Ersche KD, Jones PS, Williams GB, et al. : Abnormal brain structure implicated in stimulant drug addiction . Science 2012 ; 335:601–604 Crossref , Medline , Google Scholar
67 Moffitt TE, Arseneault L, Belsky D, et al. : A gradient of childhood self-control predicts health, wealth, and public safety . Proc Natl Acad Sci USA 2011 ; 108:2693–2698 Crossref , Medline , Google Scholar
68 Diamond A, Lee K : Interventions shown to aid executive function development in children 4 to 12 years old . Science 2011 ; 333:959–964 Crossref , Medline , Google Scholar
69 Wiers CE, Shokri-Kojori E, Cabrera E, et al. : Socioeconomic status is associated with striatal dopamine D2/D3 receptors in healthy volunteers but not in cocaine abusers . Neurosci Lett 2016 ; 617:27–31 Crossref , Medline , Google Scholar
70 Morgan D, Grant KA, Gage HD, et al. : Social dominance in monkeys: dopamine D2 receptors and cocaine self-administration . Nat Neurosci 2002 ; 5:169–174 Crossref , Medline , Google Scholar
71 Martinez D, Orlowska D, Narendran R, et al. : Dopamine type 2/3 receptor availability in the striatum and social status in human volunteers . Biol Psychiatry 2010 ; 67:275–278 Crossref , Medline , Google Scholar
72 Zalesky A, Solowij N, Yücel M, et al. : Effect of long-term cannabis use on axonal fibre connectivity . Brain 2012 ; 135:2245–2255 Crossref , Medline , Google Scholar
73 Squeglia LM, Tapert SF, Sullivan EV, et al. : Brain development in heavy-drinking adolescents . Am J Psychiatry 2015 ; 172:531–542 Link , Google Scholar
74 Adolescent Brain Cognitive Development Study. https://abcdstudy.org Google Scholar
75 Foundation for the National Institutes of Health: Baby Connectome Project. https://fnih.org/what-we-do/current-research-programs/baby-connectome Google Scholar
76 Braeburn Pharmaceuticals: FDA Acceptance of NDA for CAM2038 for Opioid Use Disorder, 2017. https://www.braeburnpharmaceuticals.com/fda-acceptance-of-nda-for-cam2038-for-opioid-use-disorder/ Google Scholar
77 Klein JW : Pharmacotherapy for substance use disorders . Med Clin North Am 2016 ; 100:891–910 Crossref , Medline , Google Scholar
78 National Institute on Drug Abuse, National Institutes of Health: Principles of Drug Addiction Treatment: A Research Based Guide (Third Edition), 2012. https://www.drugabuse.gov/publications/principles-drug-addiction-treatment/evidence-based-approaches-to-drug-addiction-treatment/behavioral-therapies Google Scholar
79 Institute of Medicine : The Development of Medications for the Treatment of Opiate and Cocaine Addictions: Issues for the Government and Private Sector . Washington, DC, National Academy Press, 1995 Google Scholar
80 Center for Drug Evaluation and Research, US Food and Drug Administration: Alcoholism: Developing Drugs for Treatment: Guidance for Industry, 2015. https://www.fda.gov/ucm/groups/fdagov-public/@fdagov-drugs-gen/documents/document/ucm433618.pdf Google Scholar
81 National Institute on Drug Abuse, National Institutes of Health: Problems of Drug Dependence, 1998: Proceedings of the 66th Annual Scientific Meeting, The College on Problems of Drug Dependence, 1998. http://rzbl04.biblio.etc.tu-bs.de:8080/docportal/servlets/MCRFileNodeServlet/DocPortal_derivate_00001868/NIDA179.pdf Google Scholar
82 National Institute on Drug Abuse, National Institutes of Health: Probuphine: A Game-Changer in Fighting Opioid Dependence, 2016. https://www.drugabuse.gov/about-nida/noras-blog/2016/05/probuphine-game-changer-in-fighting-opioid-dependence Google Scholar
83 National Institute on Drug Abuse, National Institutes of Health: NARCAN Nasal Spray: Life-saving Science at NIDA, 2015. https://www.drugabuse.gov/about-nida/noras-blog/2015/11/narcan-nasal-spray-life-saving-science-nida Google Scholar
84 Volkow ND, Collins FS : The role of science in addressing the opioid crisis . N Engl J Med 2017 ; 377:391–394 Crossref , Medline , Google Scholar
85 Brady KT, Gray KM, Tolliver BK : Cognitive enhancers in the treatment of substance use disorders: clinical evidence . Pharmacol Biochem Behav 2011 ; 99:285–294 Crossref , Medline , Google Scholar
86 Sofuoglu M, DeVito EE, Waters AJ, et al. : Cognitive enhancement as a treatment for drug addictions . Neuropharmacology 2013 ; 64:452–463 Crossref , Medline , Google Scholar
87 Sofuoglu M, DeVito EE, Waters AJ, et al. : Cognitive function as a transdiagnostic treatment target in stimulant use disorders . J Dual Diagn 2016 ; 12:90–106 Crossref , Medline , Google Scholar
88 Verplaetse TL, McKee SA : Targeting stress neuroadaptations for addiction treatment: a commentary on Kaye et al. (2017) . J Stud Alcohol Drugs 2017 ; 78:372–374 Crossref , Medline , Google Scholar
89 Carroll FI, Carlezon WA Jr : Development of κ opioid receptor antagonists . J Med Chem 2013 ; 56:2178–2195 Crossref , Medline , Google Scholar
90 Lee HM, Kim Y : Drug repurposing is a new opportunity for developing drugs against neuropsychiatric disorders . Schizophr Res Treatment 2016 ; 2016:6378137 Crossref , Medline , Google Scholar
91 Goldstein MG : Bupropion sustained release and smoking cessation . J Clin Psychiatry 1998 ; 59(Suppl 4):66–72 Medline , Google Scholar
92 Ling W, Hillhouse MP, Saxon AJ, et al. : Buprenorphine + naloxone plus naltrexone for the treatment of cocaine dependence: the Cocaine Use Reduction with Buprenorphine (CURB) study . Addiction 2016 ; 111:1416–1427 Crossref , Medline , Google Scholar
93 Gerra G, Fantoma A, Zaimovic A : Naltrexone and buprenorphine combination in the treatment of opioid dependence . J Psychopharmacol 2006 ; 20:806–814 Crossref , Medline , Google Scholar
94 Haney M, Hart CL, Vosburg SK, et al. : Effects of THC and lofexidine in a human laboratory model of marijuana withdrawal and relapse . Psychopharmacology (Berl) 2008 ; 197:157–168 Crossref , Medline , Google Scholar
95 Negus SS, Henningfield J : Agonist medications for the treatment of cocaine use disorder . Neuropsychopharmacology 2015 ; 40:1815–1825 Crossref , Medline , Google Scholar
96 Lai H, Stitzer M, Treisman G, et al. : Cocaine abstinence and reduced use associated with lowered marker of endothelial dysfunction in African Americans: a preliminary study . J Addict Med 2015 ; 9:331–339 Crossref , Medline , Google Scholar
97 Falk D, Wang XQ, Liu L, et al. : Percentage of subjects with no heavy drinking days: evaluation as an efficacy endpoint for alcohol clinical trials . Alcohol Clin Exp Res 2010 ; 34:2022–2034 Crossref , Medline , Google Scholar
98 Lee JD, Friedmann PD, Kinlock TW, et al. : Extended-release naltrexone to prevent opioid relapse in criminal justice offenders . N Engl J Med 2016 ; 374:1232–1242 Crossref , Medline , Google Scholar
99 Huang W, Manglik A, Venkatakrishnan AJ, et al. : Structural insights into µ-opioid receptor activation . Nature 2015 ; 524:315–321 Crossref , Medline , Google Scholar
100 DeWire SM, Yamashita DS, Rominger DH, et al. : A G protein-biased ligand at the μ-opioid receptor is potently analgesic with reduced gastrointestinal and respiratory dysfunction compared with morphine . J Pharmacol Exp Ther 2013 ; 344:708–717 Crossref , Medline , Google Scholar
101 Singla N, Minkowitz H, Soergel D, et al. : (432) Respiratory safety signal with oliceridine (TRV130), a novel mu receptor G protein pathway selective modulator (mu-GPS), vs morphine: a safety analysis of a phase 2b randomized clinical trial . J Pain 2016 ; 17:S82 Crossref , Google Scholar
102 Ding H, Czoty PW, Kiguchi N, et al. : A novel orvinol analog, BU08028, as a safe opioid analgesic without abuse liability in primates . Proc Natl Acad Sci USA 2016 ; 113:E5511–E5518 Crossref , Medline , Google Scholar
103 Günther T, Dasgupta P, Mann A, et al. : Targeting multiple opioid receptors: improved analgesics with reduced side effects? Br J Pharmacol (Epub ahead of print, Apr 5, 2017 ) Crossref , Medline , Google Scholar
104 Sandweiss AJ, Vanderah TW : The pharmacology of neurokinin receptors in addiction: prospects for therapy . Subst Abuse Rehabil 2015 ; 6:93–102 Medline , Google Scholar
105 US WorldMeds LLC: Open-Label, Safety Study of Lofexidine. https://clinicaltrials.gov/ct2/show/NCT02363998 Google Scholar
106 Gerra G, Zaimovic A, Giusti F, et al. : Lofexidine versus clonidine in rapid opiate detoxification . J Subst Abuse Treat 2001 ; 21:11–17 Crossref , Medline , Google Scholar
107 Neelakantan H, Holliday ED, Fox RG, et al. : Lorcaserin suppresses oxycodone self-administration and relapse vulnerability in rats . ACS Chem Neurosci 2017 ; 8:1065–1073 Crossref , Medline , Google Scholar
108 Peris J, MacFadyen K, Smith JA, et al. : Oxytocin receptors are expressed on dopamine and glutamate neurons in the mouse ventral tegmental area that project to nucleus accumbens and other mesolimbic targets . J Comp Neurol 2017 ; 525:1094–1108 Crossref , Medline , Google Scholar
109 Meyer-Lindenberg A, Domes G, Kirsch P, et al. : Oxytocin and vasopressin in the human brain: social neuropeptides for translational medicine . Nat Rev Neurosci 2011 ; 12:524–538 Crossref , Medline , Google Scholar
110 Kovács CL, Van Ree JM : Behaviorally active oxytocin fragments simultaneously attenuate heroin self-administration and tolerance in rats . Life Sci 1985 ; 37:1895–1900 Crossref , Medline , Google Scholar
111 Leong KC, Zhou L, Ghee SM, et al. : Oxytocin decreases cocaine taking, cocaine seeking, and locomotor activity in female rats . Exp Clin Psychopharmacol 2016 ; 24:55–64 Crossref , Medline , Google Scholar
112 Bentzley BS, Jhou TC, Aston-Jones G : Economic demand predicts addiction-like behavior and therapeutic efficacy of oxytocin in the rat . Proc Natl Acad Sci USA 2014 ; 111:11822–11827 Crossref , Medline , Google Scholar
113 Cox BM, Bentzley BS, Regen-Tuero H, et al. : Oxytocin acts in nucleus accumbens to attenuate methamphetamine seeking and demand . Biol Psychiatry 2017 ; 81:949–958 Crossref , Medline , Google Scholar
114 MacFadyen K, Loveless R, DeLucca B, et al. : Peripheral oxytocin administration reduces ethanol consumption in rats . Pharmacol Biochem Behav 2016 ; 140:27–32 Crossref , Medline , Google Scholar
115 Peters ST, Bowen MT, Bohrer K, et al. : Oxytocin inhibits ethanol consumption and ethanol-induced dopamine release in the nucleus accumbens . Addict Biol 2017 ; 22:702–711 Crossref , Medline , Google Scholar
116 King CE, Griffin WC, Luderman LN, et al. : Oxytocin reduces ethanol self-administration in mice . Alcohol Clin Exp Res 2017 ; 41:955–964 Crossref , Medline , Google Scholar
117 Manbeck KE, Shelley D, Schmidt CE, et al. : Effects of oxytocin on nicotine withdrawal in rats . Pharmacol Biochem Behav 2014 ; 116:84–89 Crossref , Medline , Google Scholar
118 Hicks C, Cornish JL, Baracz SJ, et al. : Adolescent pre-treatment with oxytocin protects against adult methamphetamine-seeking behavior in female rats . Addict Biol 2016 ; 21:304–315 Crossref , Medline , Google Scholar
119 Bowen MT, Carson DS, Spiro A, et al. : Adolescent oxytocin exposure causes persistent reductions in anxiety and alcohol consumption and enhances sociability in rats . PLoS One 2011 ; 6:e27237 Crossref , Medline , Google Scholar
120 Carson DS, Cornish JL, Guastella AJ, et al. : Oxytocin decreases methamphetamine self-administration, methamphetamine hyperactivity, and relapse to methamphetamine-seeking behaviour in rats . Neuropharmacology 2010 ; 58:38–43 Crossref , Medline , Google Scholar
121 Cox BM, Young AB, See RE, et al. : Sex differences in methamphetamine seeking in rats: impact of oxytocin . Psychoneuroendocrinology 2013 ; 38:2343–2353 Crossref , Medline , Google Scholar
122 Ferland CL, Reichel CM, McGinty JF : Effects of oxytocin on methamphetamine-seeking exacerbated by predator odor pre-exposure in rats . Psychopharmacology (Berl) 2016 ; 233:1015–1024 Crossref , Medline , Google Scholar
123 Zhou L, Sun WL, Young AB, et al. : Oxytocin reduces cocaine seeking and reverses chronic cocaine-induced changes in glutamate receptor function . Int J Neuropsychopharmacol 2015 ; 18:pyu009 Crossref , Google Scholar
124 Morales-Rivera A, Hernández-Burgos MM, Martínez-Rivera A, et al. : Anxiolytic effects of oxytocin in cue-induced cocaine seeking behavior in rats . Psychopharmacology (Berl) 2014 ; 231:4145–4155 Crossref , Medline , Google Scholar
125 Miller MA, Bershad A, King AC, et al. : Intranasal oxytocin dampens cue-elicited cigarette craving in daily smokers: a pilot study . Behav Pharmacol 2016 ; 27:697–703 Crossref , Medline , Google Scholar
126 McRae-Clark AL, Baker NL, Maria MM, et al. : Effect of oxytocin on craving and stress response in marijuana-dependent individuals: a pilot study . Psychopharmacology (Berl) 2013 ; 228:623–631 Crossref , Medline , Google Scholar
127 Levin FR, Mariani JJ, Brooks DJ, et al. : Dronabinol for the treatment of cannabis dependence: a randomized, double-blind, placebo-controlled trial . Drug Alcohol Depend 2011 ; 116:142–150 Crossref , Medline , Google Scholar
128 Haney M, Cooper ZD, Bedi G, et al. : Nabilone decreases marijuana withdrawal and a laboratory measure of marijuana relapse . Neuropsychopharmacology 2013 ; 38:1557–1565 Crossref , Medline , Google Scholar
129 Allsop DJ, Copeland J, Lintzeris N, et al. : Nabiximols as an agonist replacement therapy during cannabis withdrawal: a randomized clinical trial . JAMA Psychiatry 2014 ; 71:281–291 Crossref , Medline , Google Scholar
130 GW Pharmaceuticals: GW Pharmaceuticals Announces Positive Phase 3 Pivotal Study Results for Epidiolex (cannabidiol), Mar 14, 2016. https://www.gwpharm.com/about-us/news/gw-pharmaceuticals-announces-positive-phase-3-pivotal-study-results-epidiolex Google Scholar
131 den Boon FS, Werkman TR, Schaafsma-Zhao Q, et al. : Activation of type-1 cannabinoid receptor shifts the balance between excitation and inhibition towards excitation in layer II/III pyramidal neurons of the rat prelimbic cortex . Pflugers Arch 2015 ; 467:1551–1564 Crossref , Medline , Google Scholar
132 Sam AH, Salem V, Ghatei MA : Rimonabant: from RIO to ban . J Obes 2011 ; 2011:432607 Crossref , Medline , Google Scholar
133 Khajehali E, Malone DT, Glass M, et al. : Biased agonism and biased allosteric modulation at the CB1 cannabinoid receptor . Mol Pharmacol 2015 ; 88:368–379 Crossref , Medline , Google Scholar
134 Vallée M, Vitiello S, Bellocchio L, et al. : Pregnenolone can protect the brain from cannabis intoxication . Science 2014 ; 343:94–98 Crossref , Medline , Google Scholar
135 Ignatowska-Jankowska BM, Baillie GL, Kinsey S, et al. : A cannabinoid CB1 receptor-positive allosteric modulator reduces neuropathic pain in the mouse with no psychoactive effects . Neuropsychopharmacology 2015 ; 40:2948–2959 Crossref , Medline , Google Scholar
136 Schlosburg JE, Carlson BLA, Ramesh D, et al. : Inhibitors of endocannabinoid-metabolizing enzymes reduce precipitated withdrawal responses in THC-dependent mice . AAPS J 2009 ; 11:342–352 Crossref , Medline , Google Scholar
137 Kinsey SG, Long JZ, O’Neal ST, et al. : Blockade of endocannabinoid-degrading enzymes attenuates neuropathic pain . J Pharmacol Exp Ther 2009 ; 330:902–910 Crossref , Medline , Google Scholar
138 Castells X, Cunill R, Pérez-Mañá C, et al. : Psychostimulant drugs for cocaine dependence . Cochrane Database Syst Rev 2016 ; 9:CD007380 Medline , Google Scholar
139 Volkow ND, Fowler JS, Logan J, et al. : Effects of modafinil on dopamine and dopamine transporters in the male human brain: clinical implications . JAMA 2009 ; 301:1148–1154 Crossref , Medline , Google Scholar
140 Kampman KM, Lynch KG, Pettinati HM, et al. : A double blind, placebo controlled trial of modafinil for the treatment of cocaine dependence without co-morbid alcohol dependence . Drug Alcohol Depend 2015 ; 155:105–110 Crossref , Medline , Google Scholar
141 Sangroula D, Motiwala F, Wagle B, et al. : Modafinil treatment of cocaine dependence: a systematic review and meta-analysis . Subst Use Misuse 2017 ; 52:1292–1306 Crossref , Medline , Google Scholar
142 Kauer JA, Malenka RC : Synaptic plasticity and addiction . Nat Rev Neurosci 2007 ; 8:844–858 Crossref , Medline , Google Scholar
143 Moussawi K, Pacchioni A, Moran M, et al. : N-Acetylcysteine reverses cocaine-induced metaplasticity . Nat Neurosci 2009 ; 12:182–189 Crossref , Medline , Google Scholar
144 Reichel CM, See RE : Chronic N-acetylcysteine after cocaine self-administration produces enduring reductions in drug-seeking . Neuropsychopharmacology 2012 ; 37:298 Crossref , Medline , Google Scholar
145 LaRowe SD, Mardikian P, Malcolm R, et al. : Safety and tolerability of N-acetylcysteine in cocaine-dependent individuals . Am J Addict 2006 ; 15:105–110 Crossref , Medline , Google Scholar
146 LaRowe SD, Kalivas PW, Nicholas JS, et al. : A double-blind placebo-controlled trial of N-acetylcysteine in the treatment of cocaine dependence . Am J Addict 2013 ; 22:443–452 Crossref , Medline , Google Scholar
147 Ghitza UE : Needed relapse-prevention research on novel framework (ASPIRE model) for substance use disorders treatment . Front Psychiatry 2015 ; 6:37 Crossref , Medline , Google Scholar
148 Brim RL, Nance MR, Youngstrom DW, et al. : A thermally stable form of bacterial cocaine esterase: a potential therapeutic agent for treatment of cocaine abuse . Mol Pharmacol 2010 ; 77:593–600 Crossref , Medline , Google Scholar
149 Chen X, Zheng X, Zhou Z, et al. : Effects of a cocaine hydrolase engineered from human butyrylcholinesterase on metabolic profile of cocaine in rats . Chem Biol Interact 2016 ; 259(Pt B):104–109 Crossref , Medline , Google Scholar
150 Chen X, Xue L, Hou S, et al. : Long-acting cocaine hydrolase for addiction therapy . Proc Natl Acad Sci USA 2016 ; 113:422–427 Crossref , Medline , Google Scholar
151 Zheng X, Zhou Z, Zhang T, et al. : Effectiveness of a cocaine hydrolase for cocaine toxicity treatment in male and female rats . AAPS J 2017 ; 20:3 Crossref , Medline , Google Scholar
152 Gilgun-Sherki Y, Eliaz RE, McCann DJ, et al. : Placebo-controlled evaluation of a bioengineered, cocaine-metabolizing fusion protein, TV-1380 (AlbuBChE), in the treatment of cocaine dependence . Drug Alcohol Depend 2016 ; 166:13–20 Crossref , Medline , Google Scholar
153 Wood SK, Narasimhan D, Cooper Z, et al. : Prevention and reversal by cocaine esterase of cocaine-induced cardiovascular effects in rats . Drug Alcohol Depend 2010 ; 106:219–229 Crossref , Medline , Google Scholar
154 Zheng F, Zhan C-G : Enzyme-therapy approaches for the treatment of drug overdose and addiction . Future Med Chem 2011 ; 3:9–13 Crossref , Medline , Google Scholar
155 Dewey SL, Morgan AE, Ashby CR Jr, et al. : A novel strategy for the treatment of cocaine addiction . Synapse 1998 ; 30:119–129 Crossref , Medline , Google Scholar
156 Volkow ND, Wang GJ, Fowler JS, et al. : Enhanced sensitivity to benzodiazepines in active cocaine-abusing subjects: a PET study . Am J Psychiatry 1998 ; 155:200–206 Abstract , Google Scholar
157 Leung JG, Hall-Flavin D, Nelson S, et al. : The role of gabapentin in the management of alcohol withdrawal and dependence . Ann Pharmacother 2015 ; 49:897–906 Crossref , Medline , Google Scholar
158 Shinn AK, Greenfield SF : Topiramate in the treatment of substance-related disorders: a critical review of the literature . J Clin Psychiatry 2010 ; 71:634–648 Crossref , Medline , Google Scholar
159 Johnson BA, Ait-Daoud N, Wang XQ, et al. : Topiramate for the treatment of cocaine addiction: a randomized clinical trial . JAMA Psychiatry 2013 ; 70:1338–1346 Crossref , Medline , Google Scholar
160 Elkashef A, Kahn R, Yu E, et al. : Topiramate for the treatment of methamphetamine addiction: a multi-center placebo-controlled trial . Addiction 2012 ; 107:1297–1306 Crossref , Medline , Google Scholar
161 Pravetoni M, Le Naour M, Tucker AM, et al. : Reduced antinociception of opioids in rats and mice by vaccination with immunogens containing oxycodone and hydrocodone haptens . J Med Chem 2013 ; 56:915–923 Crossref , Medline , Google Scholar
162 Bremer PT, Schlosburg JE, Lively JM, et al. : Injection route and TLR9 agonist addition significantly impact heroin vaccine efficacy . Mol Pharm 2014 ; 11:1075–1080 Crossref , Medline , Google Scholar
163 Bremer PT, Kimishima A, Schlosburg JE, et al. : Combatting synthetic designer opioids: a conjugate vaccine ablates lethal doses of fentanyl class drugs . Angew Chem Int Ed Engl 2016 ; 55:3772–3775 Crossref , Medline , Google Scholar
164 Lockner JW, Eubanks LM, Choi JL, et al. : Flagellin as carrier and adjuvant in cocaine vaccine development . Mol Pharm 2015 ; 12:653–662 Crossref , Medline , Google Scholar
165 Kimishima A, Wenthur CJ, Eubanks LM, et al. : Cocaine vaccine development: evaluation of carrier and adjuvant combinations that activate multiple toll-like receptors . Mol Pharm 2016 ; 13:3884–3890 Crossref , Medline , Google Scholar
166 Raupach T, Hoogsteder PHJ, Onno van Schayck CP : Nicotine vaccines to assist with smoking cessation: current status of research . Drugs 2012 ; 72:e1–e16 Crossref , Medline , Google Scholar
167 Stevens MW, Henry RL, Owens SM, et al. : First human study of a chimeric anti-methamphetamine monoclonal antibody in healthy volunteers . MAbs 2014 ; 6:1649–1656 Crossref , Medline , Google Scholar
168 Research Portfolio Online Reporting Tools, National Institutes of Health: Advancing the development of a humanized anti-cocaine monoclonal antibody: project number 5U01DA039550-03. https://projectreporter.nih.gov/project_info_description.cfm?aid=9274949&icde=35785903 Google Scholar
169 Research Portfolio Online Reporting Tools, National Institutes of Health: Lead optimization and preclinical development of human nicotine-specific mAbs: project number 5R01DA038877-03. https://projectreporter.nih.gov/project_info_description.cfm?aid=9113552&icde=35785854 Google Scholar
170 Kimishima A, Wenthur CJ, Zhou B, et al. : An advance in prescription opioid vaccines: overdose mortality reduction and extraordinary alteration of drug half-life . ACS Chem Biol 2017 ; 12:36–40 Crossref , Medline , Google Scholar
171 Terraneo A, Leggio L, Saladini M, et al. : Transcranial magnetic stimulation of dorsolateral prefrontal cortex reduces cocaine use: a pilot study . Eur Neuropsychopharmacol 2016 ; 26:37–44 Crossref , Medline , Google Scholar
172 Grall-Bronnec M, Sauvaget A : The use of repetitive transcranial magnetic stimulation for modulating craving and addictive behaviours: a critical literature review of efficacy, technical and methodological considerations . Neurosci Biobehav Rev 2014 ; 47:592–613 Crossref , Medline , Google Scholar
173 Lupi M, Martinotti G, Santacroce R, et al. : Transcranial direct current stimulation in substance use disorders: a systematic review of scientific literature . J ECT 2017 ; 33:203–209 Crossref , Medline , Google Scholar
174 Miranda A, Taca A : Neuromodulation with percutaneous electrical nerve field stimulation is associated with reduction in signs and symptoms of opioid withdrawal: a multisite, retrospective assessment . Am J Drug Alcohol Abuse 2017 ; 44:56–63 Crossref , Medline , Google Scholar
175 Zilverstand A, Parvaz MA, Moeller SJ, et al. : Cognitive interventions for addiction medicine: understanding the underlying neurobiological mechanisms . Prog Brain Res 2016 ; 224:285–304 Crossref , Medline , Google Scholar
176 Cox WM, Subramanian L, Linden DEJ, et al. : Neurofeedback training for alcohol dependence versus treatment as usual: study protocol for a randomized controlled trial . Trials 2016 ; 17:480 Crossref , Medline , Google Scholar
177 Horrell T, El-Baz A, Baruth J, et al. : Neurofeedback effects on evoked and induced EEG gamma band reactivity to drug-related cues in cocaine addiction . J Neurother 2010 ; 14:195–216 Crossref , Medline , Google Scholar
178 Volkow ND, Koob G, Baler R : Biomarkers in substance use disorders . ACS Chem Neurosci 2015 ; 6:522–525 Crossref , Medline , Google Scholar
179 National Institutes of Health: Brain Research through Advancing Innovative Neurotechnologies (BRAIN) Initiative. https://www.braininitiative.nih.gov Google Scholar
180 National Institutes of Health: Precision Medicine Initiative. https://allofus.nih.gov Google Scholar
181 Schulte MT, Hser Y-I : Substance use and associated health conditions throughout the lifespan . Public Health Rev 2014 ; 35:2 Google Scholar
- Subjective feelings associated with expectations and rewards during risky decision-making in impulse control disorder 4 March 2024 | Scientific Reports, Vol. 14, No. 1
- A Multi-Level Analysis of Biological, Social, and Psychological Determinants of Substance Use Disorder and Co-Occurring Mental Health Outcomes 8 April 2024 | Psychoactives, Vol. 3, No. 2
- Dopamine and entrepreneurship: Unifying entrepreneur personality traits, psychiatric symptoms, entrepreneurial action and outcomes Journal of Business Venturing Insights, Vol. 21
- Can Different Types of Acute Physical Exercise at Moderate Intensity Influence the Inhibitory Control and Craving Levels in Individuals with Substance Use Disorder? 18 April 2024 | Journal of Drug Issues, Vol. 21
- A population health approach to workplace mental health: rationale, implementation and engagement 18 April 2024 | Frontiers in Public Health, Vol. 12
- Neuromarkers in addiction: definitions, development strategies, and recent advances 17 April 2024 | Journal of Neural Transmission, Vol. 35
- Bridging Species and Disciplines: Incorporating Sociological Frameworks into Animal Models of Addiction 10 April 2024
- Behavioural traits related with resilience or vulnerability to the development of cocaine-induced conditioned place preference after exposure of female mice to vicarious social defeat Progress in Neuro-Psychopharmacology and Biological Psychiatry, Vol. 130
- Adolescents flexibly adapt action selection based on controllability inferences 25 March 2024 | Learning & Memory, Vol. 31, No. 3
- Associations Between Family History of Alcohol and/or Substance Use Problems and Frontal Cortical Development From 9 to 13 Years of Age: A Longitudinal Analysis of the ABCD Study Biological Psychiatry Global Open Science, Vol. 4, No. 2
- The role of physical exercise on the brain and cognitive functions of patients in recovery from substance use disorder: A narrative review and recommendations for researchers and practitioners Mental Health and Physical Activity, Vol. 26
- Validation of the Chinese Version of Penn Alcohol Craving Scale for Patients With Alcohol Use Disorder Psychiatry Investigation, Vol. 21, No. 2
- Pleiotropy and genetically inferred causality linking multisite chronic pain to substance use disorders 15 February 2024 | Molecular Psychiatry, Vol. 11
- The Role of Estrogen Signaling and Exercise in Drug Abuse: A Review 8 January 2024 | Clinics and Practice, Vol. 14, No. 1
- The effects of virtual reality environmental enrichments on craving to food in healthy volunteers 11 September 2023 | Psychopharmacology, Vol. 241, No. 1
- The effectiveness of the original anticonvulsant Galodif® — a GABAA receptor modulator for alcohol withdrawal syndrome 2 February 2024 | Reviews on Clinical Pharmacology and Drug Therapy, Vol. 21, No. 4
- Persistent impacts of smoking on resting-state EEG in male chronic smokers and past-smokers with 20 years of abstinence 8 March 2023 | Scientific Reports, Vol. 13, No. 1
- Managing substance use in patients receiving therapeutic repetitive transcranial magnetic stimulation: A scoping review Neuroscience & Biobehavioral Reviews, Vol. 155
- International addiction researchers’ perspectives on the needs of persons with addictions, the use of neuroscientific research for prevention and treatment, and future foci in addictions research 5 April 2023 | Addiction Research & Theory, Vol. 31, No. 6
- 18-Month efficacy and safety analysis of monthly subcutaneous buprenorphine injection for opioid use disorder: Integrated analysis of phase 3 studies Journal of Substance Use and Addiction Treatment, Vol. 154
- Patterns of Familial and Racial Trauma and Their Associations with Substance Use Disorders among Racial/Ethnic Minority Adults 9 October 2023 | Journal of Racial and Ethnic Health Disparities, Vol. 34
- Addictive drugs modify neurogenesis, synaptogenesis and synaptic plasticity to impair memory formation through neurotransmitter imbalances and signaling dysfunction Neurochemistry International, Vol. 169
- A Step Toward Equity: Evidence-Based Policy for Access to Medications for Healthcare Workers With Opioid Use Disorder Journal of Nursing Regulation, Vol. 14, No. 3
- Penal Substitutionary Atonement and the Problem of Shame in Addiction 17 July 2023 | Pastoral Psychology, Vol. 72, No. 5
- Adverse Childhood Experiences and Risk Patterns of Alcohol and Cannabis Co-Use: A Longitudinal Study of Puerto Rican Youth Journal of Adolescent Health, Vol. 73, No. 3
- Non-Opioid Anesthetics Addiction: A Review of Current Situation and Mechanism 30 August 2023 | Brain Sciences, Vol. 13, No. 9
- Substance Use Disorders: Basic Overview for the Anesthesiologist 17 August 2023 | Anesthesia & Analgesia, Vol. 137, No. 3
- Factors associated with changes in alcohol use among medical students during the COVID-19 pandemic 23 August 2023 | Journal of Substance Use, Vol. 199
- A survey of international addiction researchers’ views on implications of brain-based explanations of addiction and the responsibility of affected persons 11 August 2023 | Nordic Studies on Alcohol and Drugs, Vol. 92
- Evolutionary psychiatry: foundations, progress and challenges 9 May 2023 | World Psychiatry, Vol. 22, No. 2
- A multivariate regressor of patterned dopamine release predicts relapse to cocaine Cell Reports, Vol. 42, No. 6
- THC exposure during adolescence increases impulsivity-like behavior in adulthood in a WIN 55,212-2 self-administration mouse model 25 May 2023 | Frontiers in Psychiatry, Vol. 14
- The differential vulnerabilities of Per2 knockout mice to the addictive properties of methamphetamine and cocaine Progress in Neuro-Psychopharmacology and Biological Psychiatry, Vol. 99
- Preventing incubation of drug craving to treat drug relapse: from bench to bedside 16 January 2023 | Molecular Psychiatry, Vol. 28, No. 4
- The importance of translationally evaluating steroid hormone contributions to substance use Frontiers in Neuroendocrinology, Vol. 69
- The Effect of Secondary Sexual Characteristics Outset Time Abnormality on Addiction in Adults: a Mendelian Randomization Study 20 March 2023 | International Journal of Mental Health and Addiction, Vol. 123
- The Role of Physical Exercise in Opioid Substitution Therapy: Mechanisms of Sequential Effects 1 March 2023 | International Journal of Molecular Sciences, Vol. 24, No. 5
- Developmental Patterns of Gambling Participation and Substance use Throughout Adolescence in a Population Birth Cohort 25 February 2022 | Journal of Gambling Studies, Vol. 39, No. 1
- The Potential of Myelin-Sensitive Imaging: Redefining Spatiotemporal Patterns of Myeloarchitecture Biological Psychiatry, Vol. 93, No. 5
- Executive control network resting state fMRI functional and effective connectivity and delay discounting in cocaine dependent subjects compared to healthy controls 23 February 2023 | Frontiers in Psychiatry, Vol. 14
- Therapeutic effect of psilocybin in addiction: A systematic review 9 February 2023 | Frontiers in Psychiatry, Vol. 14
- Subgroups of internet gaming disorder based on addiction‐related resting‐state functional connectivity 24 September 2022 | Addiction, Vol. 118, No. 2
- Effects of β -caryophyllene, A Dietary Cannabinoid, in Animal Models of Drug Addiction Current Neuropharmacology, Vol. 21, No. 2
- The association between naturalistic use of psychedelics and co-occurring substance use disorders 10 January 2023 | Frontiers in Psychiatry, Vol. 13
- The Genetically Informed Neurobiology of Addiction (GINA) model 29 November 2022 | Nature Reviews Neuroscience, Vol. 24, No. 1
- Leveraging circuits to understand addiction
- Assessment of addiction/pain and neurobiology
- Designing and testing population-based prevention programs
- Updated Perspectives on the Neurobiology of Substance Use Disorders Using Neuroimaging 1 August 2023 | Substance Abuse and Rehabilitation, Vol. Volume 14
- The i-frame and the s-frame: How focusing on individual-level solutions has led behavioral public policy astray 5 September 2022 | Behavioral and Brain Sciences, Vol. 46
- Neurobiology of Addiction 1 September 2023
- The Genetics of Alcohol Use Disorder 21 October 2023
- Addiction and Substance Use Recovery 16 May 2023
- Futuristic Exploration of Addiction Neuroscience in the Genomic Era 1 December 2023 | Psychology Research and Behavior Management, Vol. Volume 16
- Thérapies cognitivocomportementales en addictologie
- Major Psychiatric Disorders 25 January 2024
- Articulation de la thérapie d’acceptation et d’engagement à la psychothérapie assistée par psychédéliques en addictologie L'Évolution Psychiatrique, Vol. 382
- Substance Intoxication and Withdrawal 27 March 2024
- Opposing associations of Internet Use Disorder symptom domains with structural and functional organization of the striatum: A dimensional neuroimaging approach Journal of Behavioral Addictions, Vol. 11, No. 4
- Distinct brain structural abnormalities in attention-deficit/hyperactivity disorder and substance use disorders: A comparative meta-analysis 6 September 2022 | Translational Psychiatry, Vol. 12, No. 1
- Addictive and other mental disorders: a call for a standardized definition of dual disorders 13 October 2022 | Translational Psychiatry, Vol. 12, No. 1
- Neurobiological mechanisms and related clinical treatment of addiction: a review 16 December 2022 | Psychoradiology, Vol. 2, No. 4
- Novel role of AMPK in cocaine reinforcement via regulating CRTC1 31 December 2022 | Translational Psychiatry, Vol. 12, No. 1
- Effect of chronic intermittent ethanol vapor exposure on RNA content of brain-derived extracellular vesicles Alcohol, Vol. 105
- Basic psychological needs in gambling and gaming problems Addictive Behaviors Reports, Vol. 16
- Differences in small-world networks between methamphetamine and heroin use disorder patients and their relationship with psychiatric symptoms 26 August 2022 | Brain Imaging and Behavior, Vol. 16, No. 5
- Classic and non‐classic psychedelics for substance use disorder: A review of their historic, past and current research Addiction Neuroscience, Vol. 3
- Neuropsychiatric Model of Addiction Simplified Psychiatric Clinics of North America, Vol. 45, No. 3
- Exploring the Role of DARPP-32 in Addiction: A Review of the Current Limitations of Addiction Treatment Pathways and the Role of DARPP-32 to Improve Them 25 August 2022 | NeuroSci, Vol. 3, No. 3
- Ultrasound combined with glial cell line-derived neurotrophic factor-loaded microbubbles for the targeted treatment of drug addiction 15 August 2022 | Frontiers in Bioengineering and Biotechnology, Vol. 10
- The Significance of NLRP Inflammasome in Neuropsychiatric Disorders 10 August 2022 | Brain Sciences, Vol. 12, No. 8
- Gender-related differences in involvement of addiction brain networks in internet gaming disorder: Relationships with craving and emotional regulation Progress in Neuro-Psychopharmacology and Biological Psychiatry, Vol. 118
- The interaction between Environmental Enrichment and fluoxetine in inhibiting sucrose-seeking renewal in mice depend on social living condition 30 March 2022 | Psychopharmacology, Vol. 239, No. 7
- Clinical judgement versus self‐rating of demoralization in outpatients with a substance‐use disorder: A cross‐sectional study 8 July 2021 | Perspectives in Psychiatric Care, Vol. 58, No. 3
- La N-acetilcisteína como coadyuvante en el manejo del craving por cocaína y tabaco. Revisión narrativa 1 July 2022 | Revista de la Facultad de Ciencias de la Salud Universidad del Cauca, Vol. 24, No. 2
- Drugs and Bugs: The Gut-Brain Axis and Substance Use Disorders 25 October 2021 | Journal of Neuroimmune Pharmacology, Vol. 17, No. 1-2
- A human brain circuit for addiction remission 13 June 2022 | Nature Medicine, Vol. 28, No. 6
- Acid-Sensing Ion Channel 2: Function and Modulation 19 January 2022 | Membranes, Vol. 12, No. 2
- DNA Epigenetics in Addiction Susceptibility 25 January 2022 | Frontiers in Genetics, Vol. 13
- Substance Intoxication and Withdrawal 21 May 2022
- Diagnosis 6 August 2022
- Substance-Related Disorders 31 August 2022
- The Brain in Treatment Settings 23 April 2022
- The Brain in Substance Use Prevention 23 April 2022
- Conclusions 23 April 2022
- Epigenetics of Addiction
- Prepulse inhibition can predict the motivational effects of cocaine in female mice exposed to maternal separation Behavioural Brain Research, Vol. 416
- Pornography Use and Sexting Trends Among American Adolescents: Data to Inform School Counseling Programming and Practice 31 October 2022 | Professional School Counseling, Vol. 26, No. 1
- Repetitive Transcranial Magnetic Stimulation for Comorbid Major Depressive Disorder and Alcohol Use Disorder 30 December 2021 | Brain Sciences, Vol. 12, No. 1
- Comparison of rule- and ordinary differential equation-based dynamic model of DARPP-32 signalling network 15 December 2022 | PeerJ, Vol. 10
- Crossed wires: the hall effect in substance use disorder 3 October 2022 | MOJ Addiction Medicine & Therapy, Vol. 7, No. 1
- The i-Frame and the s-Frame: How Focusing on the Individual-Level Solutions Has Led Behavioral Public Policy Astray SSRN Electronic Journal, Vol. 50
- Pharmacological Management of Heroin Withdrawal Syndrome 1 March 2022 | American Journal of Therapeutics, Vol. 29, No. 2
- Medication overuse and drug addiction: a narrative review from addiction perspective 28 April 2021 | The Journal of Headache and Pain, Vol. 22, No. 1
- Affective Neuroscience Contributions to the Treatment of Addiction: The Role of Social Instincts, Pleasure and SEEKING 23 November 2021 | Frontiers in Psychiatry, Vol. 12
- Genetics of substance use disorders in the era of big data 1 July 2021 | Nature Reviews Genetics, Vol. 22, No. 11
- Social Interaction With Relapsed Partner Facilitates Cocaine Relapse in Rats 4 October 2021 | Frontiers in Pharmacology, Vol. 12
- “Stories of starting”: Understanding the complex contexts of opioid misuse initiation 17 May 2023 | Substance Abuse, Vol. 42, No. 4
- Predicting Success of a Digital Self-Help Intervention for Alcohol and Substance Use With Machine Learning 3 September 2021 | Frontiers in Psychology, Vol. 12
- Sex differences in behavioral traits related with high sensitivity to the reinforcing effects of cocaine Behavioural Brain Research, Vol. 414
- Dissociable neural substrates of opioid and cocaine use identified via connectome-based modelling 12 November 2019 | Molecular Psychiatry, Vol. 26, No. 8
- Epigenetics of addiction Neurochemistry International, Vol. 147
- Ventral pallidum DRD3 potentiates a pallido-habenular circuit driving accumbal dopamine release and cocaine seeking Neuron, Vol. 109, No. 13
- Neuroscience Education Psychiatric Clinics of North America, Vol. 44, No. 2
- Bibliometric Insights in Genetic Factors of Substance-Related Disorders: Intellectual Developments, Turning Points, and Emerging Trends 31 May 2021 | Frontiers in Psychiatry, Vol. 12
- Comparison of Treatments for Cocaine Use Disorder Among Adults 7 May 2021 | JAMA Network Open, Vol. 4, No. 5
- Prenatal opioid exposure and vulnerability to future substance use disorders in offspring Experimental Neurology, Vol. 339
- Social vulnerabilities for substance use: Stressors, socially toxic environments, and discrimination and racism Neuropharmacology, Vol. 188
- Simultaneous HPLC estimation of Amphetamine and Caffeine abuse drugs in Iraqi human addicts 13 December 2021 | Journal of Advanced Sciences and Engineering Technologies, Vol. 4, No. 1
- Recycled Refractory Brick as Aggregate for Eco-friendly Concrete Production 13 December 2021 | Journal of Advanced Sciences and Engineering Technologies, Vol. 4, No. 1
- Microbiome and substances of abuse Progress in Neuro-Psychopharmacology and Biological Psychiatry, Vol. 105
- Substance Use 26 February 2021
- Physical activity: A promising adjunctive treatment for severe alcohol use disorder Addictive Behaviors, Vol. 113
- The Impact of Cannabidiol on Human Brain Function: A Systematic Review 21 January 2021 | Frontiers in Pharmacology, Vol. 11
- Addressing unmet needs in opiate dependence: supporting detoxification and advances in relapse prevention 20 January 2021 | BJPsych Advances, Vol. 191
- Substance Use Disorder 22 April 2021
- Frontiers in Neuroscience Imaging PET Clinics, Vol. 16, No. 1
- Beyond the Tip of the Iceberg: A Narrative Review to Identify Research Gaps on Comorbid Psychiatric Disorders in Adolescents with Methamphetamine use Disorder or Chronic Methamphetamine Use 17 May 2023 | Substance Abuse, Vol. 42, No. 1
- Neurobiology and principles of addiction and tolerance Medicine, Vol. 48, No. 12
- Metabolic and Addiction Indices in Patients on Opioid Agonist Medication-Assisted Treatment: A Comparison of Buprenorphine and Methadone 27 March 2020 | Scientific Reports, Vol. 10, No. 1
- Regulators of G Protein Signaling in Analgesia and Addiction 30 May 2020 | Molecular Pharmacology, Vol. 98, No. 6
- Gambling Dual Disorder: A Dual Disorder and Clinical Neuroscience Perspective 24 November 2020 | Frontiers in Psychiatry, Vol. 11
- Association of Opioid Prescription Initiation During Adolescence and Young Adulthood With Subsequent Substance-Related Morbidity JAMA Pediatrics, Vol. 174, No. 11
- Impact of cannabis on non-medical opioid use and symptoms of posttraumatic stress disorder: a nationwide longitudinal VA study 9 October 2020 | The American Journal of Drug and Alcohol Abuse, Vol. 46, No. 6
- Gray matter alteration in heroin-dependent men: An atlas-based magnetic resonance imaging study Psychiatry Research: Neuroimaging, Vol. 304
- First case report of tDCS efficacy in severe chemsex addiction 1 April 2022 | Dialogues in Clinical Neuroscience, Vol. 22, No. 3
- Bio-Behavioral Indices of Emotion Regulation: Potential Targets for Treatment in Addiction 28 August 2020 | Current Addiction Reports, Vol. 7, No. 3
- Differences in the amplitude of low‐frequency fluctuation between methamphetamine and heroin use disorder individuals: A resting‐state fMRI study 15 July 2020 | Brain and Behavior, Vol. 10, No. 9
- The Neurosciences of Health Communication: An fNIRS Analysis of Prefrontal Cortex and Porn Consumption in Young Women for the Development of Prevention Health Programs 31 August 2020 | Frontiers in Psychology, Vol. 11
- Assessing drug cue-induced brain response in heroin dependents treated by methadone maintenance and protracted abstinence measures 8 February 2019 | Brain Imaging and Behavior, Vol. 14, No. 4
- Toward Addiction Prediction: An Overview of Cross-Validated Predictive Modeling Findings and Considerations for Future Neuroimaging Research Biological Psychiatry: Cognitive Neuroscience and Neuroimaging, Vol. 5, No. 8
- Toward Precision Medicine for Smoking Cessation: Developing a Neuroimaging-Based Classification Algorithm to Identify Smokers at Higher Risk for Relapse 14 November 2019 | Nicotine & Tobacco Research, Vol. 22, No. 8
- One Is Not Enough: Understanding and Modeling Polysubstance Use 16 June 2020 | Frontiers in Neuroscience, Vol. 14
- Postretrieval Microinjection of Baclofen Into the Agranular Insular Cortex Inhibits Morphine-Induced CPP by Disrupting Reconsolidation 19 May 2020 | Frontiers in Pharmacology, Vol. 11
- Sobriety and Satiety: Is NAD+ the Answer? 14 May 2020 | Antioxidants, Vol. 9, No. 5
- Substance Use Disorders Education: Are We Heeding the Call? 27 February 2020 | Academic Psychiatry, Vol. 44, No. 2
- Ankyrin Repeat and Kinase Domain Containing 1 Gene, and Addiction Vulnerability 4 April 2020 | International Journal of Molecular Sciences, Vol. 21, No. 7
- Tetrahydroprotoberberines: A Novel Source of Pharmacotherapies for Substance Use Disorders? Trends in Pharmacological Sciences, Vol. 41, No. 3
- Primary Care Recognition and Treatment of Methamphetamine Use Disorder 1 March 2020 | Osteopathic Family Physician
- Compassionate Care of the Patient Who Uses Substances Journal of Infusion Nursing, Vol. 43, No. 2
- Prepulse Inhibition of the Startle Reflex as a Predictor of Vulnerability to Develop Locomotor Sensitization to Cocaine 3 February 2020 | Frontiers in Behavioral Neuroscience, Vol. 13
- Epidemiology, Genetics, and Neurobiology of Substance Use and Disorders 7 March 2020
- Substance Use Disorder 29 April 2020
- Substance use disorders The Nurse Practitioner, Vol. 45, No. 1
- Distinct effects of cocaine and cocaine + cannabis on neurocognitive functioning and abstinence: A six-month follow-up study Drug and Alcohol Dependence, Vol. 205
- Chapitre 12. Alcool et addiction
- Effects of ketamine treatment on cocaine-induced reinstatement and disruption of functional connectivity in unanesthetized rhesus monkeys 16 March 2019 | Psychopharmacology, Vol. 236, No. 7
- Deficient Functioning of Frontostriatal Circuits During the Resolution of Cognitive Conflict in Cannabis-Using Youth Journal of the American Academy of Child & Adolescent Psychiatry, Vol. 58, No. 7
- Neurocognitive Precursors of Substance Misuse Corresponding to Risk, Resistance, and Resilience Pathways: Implications for Prevention Science 14 June 2019 | Frontiers in Psychiatry, Vol. 10
- Targeting Youth to Prevent Later Substance Use Disorder: An Underutilized Response to the US Opioid Crisis American Journal of Public Health, Vol. 109, No. S3
- Medication-assisted treatment for opioid use disorder The Nurse Practitioner, Vol. 44, No. 3
- The Lancet, Vol. 393, No. 10173
- The Lancet, Vol. 393, No. 10182
- Can neuroimaging help combat the opioid epidemic? A systematic review of clinical and pharmacological challenge fMRI studies with recommendations for future research 3 October 2018 | Neuropsychopharmacology, Vol. 44, No. 2
- International Journal of Neuropsychopharmacology, Vol. 22, No. 3
- PLOS ONE, Vol. 14, No. 3
- Frontiers in Psychiatry, Vol. 10
- Frontiers in Public Health, Vol. 7
- International Archives of Health Sciences, Vol. 6, No. 4
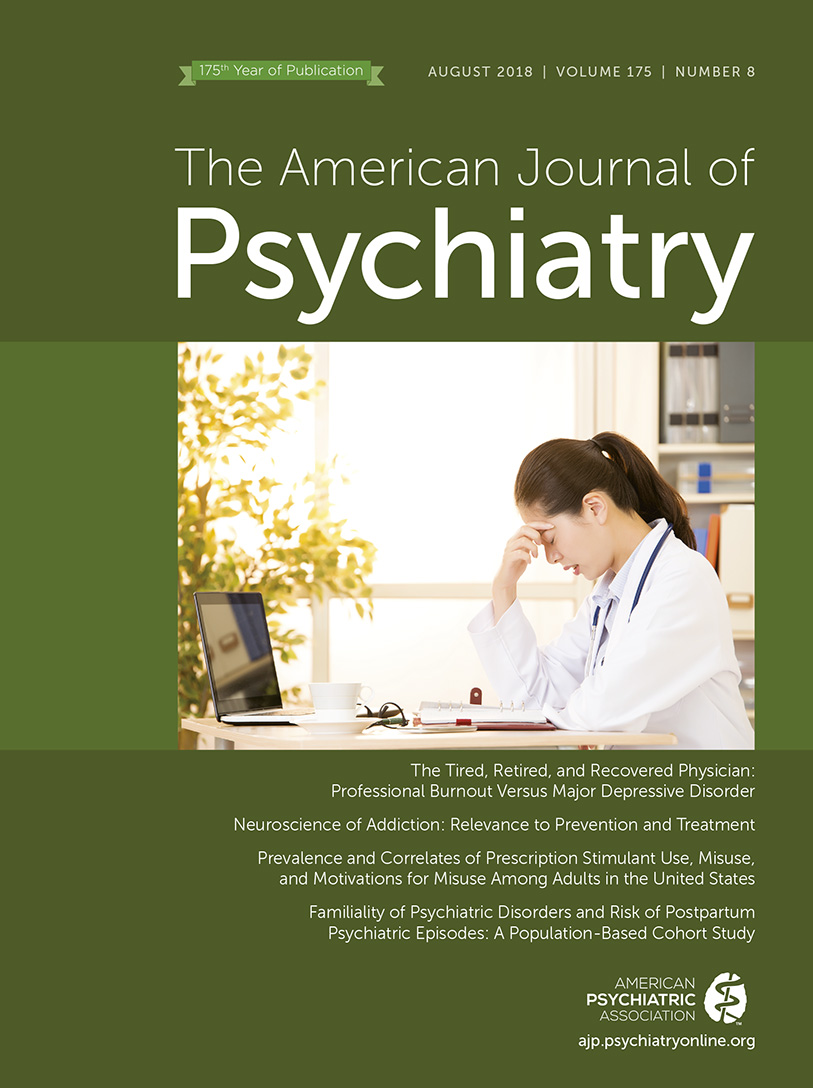
- Substance Use Disorder
- Neurochemistry
- Other Areas Of Neuroscience
- Clinical Drug Studies
- Other Psychosocial Techniques/Treatments
- Open access
- Published: 29 April 2020
Psychological, social, and motivational factors in persons who use drugs
- Sana Shahrabadi 1 ,
- Amir Jalali 2 ,
- Rostam Jalali 3 &
- Ali Gholami 4
Substance Abuse Treatment, Prevention, and Policy volume 15 , Article number: 32 ( 2020 ) Cite this article
10k Accesses
3 Citations
1 Altmetric
Metrics details
Persons who use drug need family and society’s support in the process of treatment and rehabilitation. Therefore, it is imperative to determine the psychological, social, and motivational factors that can help them in the treatment process. The present study was an attempt to determine the relationship between psychological, social, and motivational factors and the demographics of persons who use drugs (PWUD).
An analytical cross-sectional study was carried out. TCU psychological functioning and motivation scales for the PWUD was first translated into Farsi and validated after securing permission from the copyright holder of the tool. Participants were 250 PWUDs under methadone therapy who were selected through convenient sampling. Before analyzing the collected data, validity and reliability of the tool were confirmed using confirmatory and exploratory factor analyses. Given the scale of demographical data, descriptive and analytic statistics were used to analyze the relationship between demographical variables and psychological, social, and motivational factors.
The results of exploratory and confirmatory factor analyses showed that out of 83 statements in the original questionnaire, 55 statements categorized into 11 aspects were usable for Iranian population. The results showed that gender, income, and marital status affect psychological functioning of the PWUD ( P < 0.05). However, education level, place of residence, and type of drug and consumption did not have a significant relationship with social functioning of the participants ( p > 0.05). There was a significant relationship between age, number of children, and history of using drug and psychological functioning of the participants ( P < 0.01). The results showed that the demographics did not have a notable effect on the participants’ motivation for treatment; only marital status had a significant relationship with the participants’ treatment readiness ( P < 0.05).
As the results showed, the demographical variables could affect physical, psychological, and social functioning in the participants.
Drug dependence is one of the main psychosocial challenges in today society all around the world [ 1 ]. Every day, a large number of individuals are lured into drugs [ 2 ]. According to the World Health Organization, about 5.6% of 15–64 years old population in the world have used drugs at least once in their lives. About 31 million in the world suffer the disorders caused by using drugs [ 3 ]. In the case of Iran, the youth are at a high risk of developing drugs dependence due to cultural reasons, wrong beliefs, and neighboring one of the main producing countries of narcotic substances [ 4 ]. The immense losses in the form of lost lives and financial resources and the social outcomes (heavy costs, death, suicide, crime, divorce, sexually transmitted diseases like HIV and hepatitis) caused by using drugs are not negligible [ 5 ]. Shahbazi et al. (2017) reported that mortality rate of PWUDs in Iran was 38.4 individuals per one million, which is higher than the world average rate [ 6 ].
Abusive use of drugs in psychological patients is a prevalent issue that affects social and occupational performance of the individual [ 7 ]. Studies on abusive use of drug have shown that there is a direct relationship between abusive use of drugs and mental health [ 7 , 8 ]. Developing drug dependence hinders fulfillment of social, spiritual, and emotional roles of the user at social and family levels; which causes problems for the society and family [ 9 ]. Psychosocial functioning is a key factor in the treatment and rehabilitation of PWUDs [ 10 , 11 , 12 ]. Personality factors and psychological ones in particular like happiness and self-esteem are of the main factors in the decision to quit [ 13 , 14 ].
Social, psychological, and motivational factors can help the PWUD in making decision to quit drugs [ 12 ]. Physical, psychological, and motivational factors can help the PWUD in the treatment process and afterwards. In addition, demographical factors can be also useful [ 15 ]. As suggested by studies, the variables age, gender, marital status, and education level affect psychological functioning of the PWUD [ 16 ]. In addition, more than one half of the abusive users of drugs suffer psychological disorders [ 17 ]. On the other hand, perceiving the motivations of PWUD is very important for the treatment as it is knowing about the motivational factors when they want to quit [ 18 ]. Motivation has long been considered as a key factor in the treatment of risky behaviors like abusive use of alcoholic drinks and drugs. It is also highly important in the successful treatment of drugs abusive use [ 18 , 19 ]. As showed by studies, there are very important motivational factors in the treatment and rehabilitation of PWUD [ 15 , 20 ].
Given this introduction, it is essential to comprehend the relationship of demographical variables in the PWUD and their psychological, social, and motivational performance. Having a deep insight into the decisive factors, we can take more effective steps to alleviate the damages caused by abusive use of drugs through making more effective decisions to treat the patients. The demographic variables are related to psychosocial aspects, social functions, and tendency to treatment in PWUDs. Therefore, the present study was an attempt to survey the relationship between psychological, social, and motivational factors and some of the demographical factors.
Study design
A cross-sectional and descriptive-analytical study was carried out from Sep 2018 to June 2019.
Participants
The study population consisted of all the PWUD visiting drug abuse clinics (22 clinics) located in Kermanshah City -Iran. Approximately, 100 clients had a file as outpatients in almost every clinic. Following [ 11 , 21 ], 300 participants were selected through convenient sampling based on a set of inclusion criteria (only 250 questionnaire were fully completed and used in the study). For this purpose, the researcher would visit the clinic during business hours. All PWUD treated in the clinics with the inclusion criteria and willing to participate in the study were selected. Inclusion criteria were vising drug dependent treatment centers, both male and female; and willingness to participate in the study. The exclusion criterion was incomplete questionnaires.
Tool translation
At first, modified Texas Christian University (TCU) psychological, social, and motivational performance questionnaire for drug users [ 22 ] was translated into Farsi through forward-backward method (Wild et al. [ 23 ]). Two independent translators translated the tool into Farsi and a translation team checked the translations to spot inconsistencies between the two translations. Two translators translated the draft translation back into English and inconsistencies between the original and translated versions were examined. The draft was designed and arranged as a standard questionnaire and provided to the PWUDs to comment on its understandability and clarity. The patients’ feedbacks were implemented on the questionnaire and vague and unfamiliar terms were corrected. Afterwards, content validity index (CVI), content validity ratio (CVR), and Kappa coefficient were obtained for the questionnaire. Then, data gathering process was started.
At first, the participants were briefed about the questionnaire and how to fill it and they signed a written letter of consent. Inclusion criteria were desire to participate, using herbal narcotic drugs, and at least 2 weeks under methadone therapy. In the tool validation stage, 10 experts gave their opinions about content validity of the tool, and to examine construct validity, the tool was provided to 250 PWUD. To examine content validity, Waltz and Bausell’s content validity index (CVI) was used and to examine correlation between the scores of tests and tools (test-retest reliability) Pearson’s correlation coefficient was used. In addition, Cronbach’s alpha was employed to check internal consistency of the tool. Exploratory and confirmatory factor analyses were used to check the construct validity. The relationship between demographical variables and psychological, social, and motivational performance was examined using independent t test, Pearson Correlation, and one-way ANOVA statistics.
In addition to a demographics form, the modified TCU psychological, social, and motivational functioning form was used. The latter is a self-rating form with 11 sub-scales and 88item that includes four psychological functioning scales, four social functioning scales, and three motivation scales [ 22 ]. The four psychological functioning scales (29 items) include composite measures of self-esteem (SE) - six items, depression (DP) - six items, anxiety (AX) – eight items, and decision-making confidence (DM) - nine items. The four social functioning scales (31 items) includes measures of childhood problems (CP) – eight items, hostility (HS) – eight items, risk-taking behavior (RT) – seven items, and social conformity (SC)- eight items. The three motivational scales (24 items) includes measures of problem recognition (PR)- nine items, desire for help (DH) – seven items, and treatment readiness (TR)- eight items. The scales each consist of 7 to 10 items, with items rated on a 5-point Likert scale (0 = strongly disagree, 1 = disagree, 2 = undecided, 3 = agree, 4 = strongly agree).
Mean age of the participants was 39.24 ± 11.73 and mean history of using drugs was 13.8 ± 11.04. Totally, 86.6% were men, 54.8% were married, 43.6% had an elementary level education, 40.4% had a high school diploma. Moreover, 29.2% had used opiate, 22.4% used heroin and crack heroin, and 48.4% used a combination of natural and industrial opiate. Additionally, 91.6% lived in urban area.
Validation of the tool
The first step to check validity of the tool is content validity check. Waltz and Bausell’s CVI was employed to this end. As the results showed, the CVI and CVR were acceptable for all the statements and no statement was omitted at this stage. To examine reliability of the tool, test-retest technique was used through Pearson’s correlation coefficient, which yielded 0.875.
To examine construct validity, exploratory factor analysis was used followed by confirmatory factor analysis. In the former, correlation coefficients were examined for the statements to make sure that they are in an acceptable range. Kaiser Meyer Olkin (KMO) test and Bartlett’s test of sphericity were used to this end. Given that KMO = 0.858 > 0.7 and that Bartlett’s test was significant (Chi Square = 13,500/19, P -value < 0.01), the presumptions for using exploratory factor analysis on TCU questionnaire with 83 statements were met. Varimax vertical rotation was employed and the factors of which the specific value was above one were selected for exploratory factor analysis through principle components (PC) analysis. In this study, factors with eigenvalues greater than one were selected (Fig. 1 ).
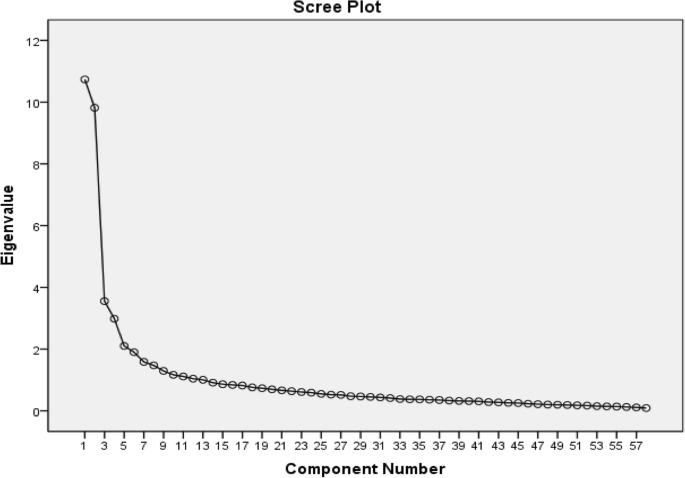
Scree Cattel plot of the extracted elements of the questionnaire
In addition, commonality value of each statement was high (> 0.5) so that none of the questions were omitted in this stage. Still, factor load of the rotated variables showed that some of the variables had factor loading (> 0.3) on two factors at the same time and therefore, they were omitted. In this way, 24 statements (1, 2, 10, 12, 14, 15, 19, 23, 27, 28, 32, 35, 37, 47, 48, 51, 54, 56, 59, 68, 70, 74, 75, and 81) were omitted. In addition, statement No.26 was omitted because of low factor loading (< 0.3) on different factors. Thus, 57 statements remained in the study. Exploratory factor analysis was repeated using the main elements of the analysis and varimax rotation. Scree plot demonstrates factor analysis in SPSS so that 13 factors or elements are fitted for the final analysis (Table 1 ). The questions about each factor, name of each factor, and Cronbach’s alpha coefficients are listed in Table 2 to determine reliability of the elements. Exploratory factor analysis was completed with 11 factors and 56 statements.
First-order confirmatory factor analysis was used in this study in two steps. In the first step, factor loadings of the questionnaire questions were analyzed. Secondly, factor loadings of factors were analyzed (Table 3 ). Only the statement No. 46 had a low factor load (t = 0.26) and eliminated.
Analysis of the relationships
As listed in Table 4 , the variables gender, job, income, and marital status had a relationship with the psychological functioning of the PWUDs ( P < 0.05). However, education level, place of residence, the way of using drugs, and the type of drugs did not have a significant relationship with one’s social functioning ( P > 0.05).
Table 5 lists the Pearson correlation coefficients to examine the relationship between demographical variables, psychological functioning, and its aspects.
As listed in Table 5 , there is a significant relationship between age, number children, and the history of using drugs and psychological functioning ( P < 0.01). In other words, with an increase in the demographical variable, a decrease in psychological functioning takes place. However, there was no relationship between the age of first experience of drugs and psychological functioning ( p = 0.513). Table 6 compares mean score and SD of the aspects of social functioning in terms of demographical variables.
As the findings showed, place of residence, job, income, and marital status had a relationship with social functioning of the patients ( P < 0.05). However, gender ( p = 0.674), education level ( p = 0.432), way of using drug ( p = 0.431), and type of drug ( p = 0.739) did not have a significant relationship with social functioning. Table 7 lists Pearson correlation coefficients for the relationship between demographical variables, psychological functioning, and its aspects.
As listed in Table 7 , there is a negative significant relationship between age and violence in the patients ( p < 0.01). In other words, with an increase in age, violence declines in the patients. There was no significant relationship between other demographical variables and social functioning ( p > 0.05). As listed in Table 8 , none of the demographical variables are related to the motivation for treatment in the subjects ( p > 0.5). Only marital status was significantly related to treatment readiness. So that, the divorces/widows had more motivation to quit. In addition, the type of drug has a significant relationship with treatment readiness ( P < 0.05); so that patients who use only one type of drug have more desire for treatment.
As listed in Table 9 , there is a negative relationship between number of children and motivation for treatment ( p < 0.05, r = − 0.139). That is, with an increase in the number of children, the motivation in patients declines.
Perceiving the effects of demographical variables on psychological, social, and motivational performance of PWUDs may lead to better treatment protocols. The relationship between the demographical variables in PWUDs and their social, psychological, and motivational functioning was examined. Gender had an effect on depression score of the participants – i.e. an aspect of psychological functioning- so that it was higher in females than males. In general, gender affected the psychological functioning of PWUDs so that female drug addicts, being more sensitive than male, were more vulnerable to psychological damages. This finding was more consistent with other studies [ 9 , 24 ]. However, the effect of gender on social functioning of the PWUDs was not significant.
Education level of the participants affected the confidence in decision-making -i.e. an aspect of psychological functioning. In general, however, the effect of education level on psychological and social functioning was not significant. Place of residence was another variable under study and it affected the level of violent behavior -i.e. an aspect of social functioning. That is, urban dwellers were less violent than those living in rural areas. One probable reason for this is that the latter group live in a smaller community and they feel more pressure by their society for being a drug addict. In general, and consistent with Poudel et al. (2016) [ 25 ], the small sample group of rural dwellers and the considerable level of interactions between rural and urban areas in Iran can explain this finding.
Job was another factor in psychological and social functioning. That is, those who had a job had a better social and psychological functioning than those without a job. The results showed that job affected the participants’ self-esteem, depression, and anxiety (psychological functioning) and risk-taking attitudes (social functioning). This is consistent with other studies [ 3 , 9 ]. Many studies have shown that having a decent job is a factor in enabling the PWUD [ 24 ] and it can improve their physical and psychological functioning [ 3 , 5 , 24 , 26 ].
Marital status affected self-esteem, depression, and anxiety (psychological functioning); so that the unmarried individuals had a better psychological functioning. This finding is consistent with other studies like [ 9 , 16 ]. Risk-taking attitude and social conformity in the married individual were higher than the others; which is consistent with Gyawali and Sarkar (2016) [ 27 ].
Individuals with a higher income had higher self-esteem and confidence in decision-making. They also had lower anxiety and depression (psychological functioning). Moreover, the PWUDs with higher income had fewer childhood problems, were less violent, and were more socially adaptable. To explain this, a better economic condition attenuates social problems and improves the quality of life [ 17 ]. Socioeconomic condition of family and proper family support [ 16 ] can be effective in self-esteem, happiness [ 13 ], and even the quality of life [ 18 ] as they play a key role in treatment and prevention of relapse [ 9 ].
There was a negative relationship between age and psychological functioning of PWUD. That is, the older individuals had more psychological functioning problems compared to the younger clients. In addition, the level of violence was lower in the older PWUD. Number of children and the history of using drugs were of other variables effective in psychological functioning of the subjects. These two variables had a negative relationship with psychological functioning of the participants. Drug users with more children or a longer history of using drugs had a lower psychological functioning. These findings are consistent with Poudel et al. (2016) [ 25 ].
Demographical variables did not have a notable effect on motivation for treatment. Only marital status had a significant relationship with treatment readiness; that is, unmarried individuals had more motivation for treatment. One probable reason is that unmarried addicts might have higher hopes for starting a new life. This finding is consistent with German et al. (2006) [ 19 ]. Another explanation for this might be the fact that married PWUD have to spend more time and money on the welfare of their children as the first priority of the family. In addition, desire for treatment was higher in the subjects who only used one type of drug; which is consistent with Targowski et al. (2004) [ 18 ]. Another reason for this finding is that PWUD who only use one type drug have a higher hope for rehabilitation. It appears, however, that the demographical variables are not very effective in the motivation for treatment. Probably, other factors including inner, personal, and family factors are more effective in the motivation for treatment.
Sampling and selection of clients was faced with difficulties. Stratified random sampling was the best method for the study, however, due to inclusion criteria and the unwillingness of many clients to participate in the study, this sampling method was not possible. Also a few of female clients participated in the study after extensive explanations and assurance of confidentiality. At the analysis stage, 50 questionnaires that were not completely filled in were omitted.
Some demographical variables like gender, education level, job, marital state, age, education level, income, number of children, and the experience of drug use were related to psychological functioning. In addition, place of residence, job, marital state, age, income and type of drugs use were related to social functioning. Marital status, number of children, age of the first experience of drugs and type of drugs use were related to the motivation for treatment. Thus, the demographic variables have an effect on the process of treatment and rehabilitation in PWUDs.
Availability of data and materials
The datasets used and analyzed during the current study are available from the corresponding author on reasonable request.
Abbreviations
Content Validity Index
Content Validity Ratio
Person(s) Who Use(s) Drugs
Kaiser Meyer Olkin
Texas Cristian University
Principle Components
Kermanshah University of Medical Sciences
Whittaker E, Swift W, Roxburgh A, Dietze P, Cogger S, Bruno R, et al. Multiply disadvantaged: health and service utilisation factors faced by homeless injecting drug consumers in Australia. Drug Alcohol Rev. 2015;34(4):379–87. https://doi.org/10.1111/dar.12257 .
Article PubMed Google Scholar
Komaromy M, Duhigg D, Metcalf A, Carlson C, Kalishman S, Hayes L, et al. Project ECHO (extension for community healthcare outcomes): a new model for educating primary care providers about treatment of substance use disorders. Subst Abus. 2016;37(1):20–4. https://doi.org/10.1080/08897077.2015.1129388 .
Article PubMed PubMed Central Google Scholar
Guydish J, Passalacqua E, Pagano A, Martínez C, Le T, Chun J, et al. An international systematic review of smoking prevalence in addiction treatment. Addiction. 2016;111(2):220–30. https://doi.org/10.1111/add.13099 .
Urbanoski KA. Coerced addiction treatment: client perspectives and the implications of their neglect. Harm Reduct J. 2010;7(1):13. https://doi.org/10.1186/1477-7517-7-13 .
Tavakoli Ghouchani H, Niknami S, Aminshokravi F, Hojjat SK. Factors related to addiction treatment motivations; validity and reliability of an instrument. J Res Health Sci. 2016;16(3):147–52.
PubMed Google Scholar
Shahbazi F, Mirtorabi SD, Ghadirzadeh MR, Hashemi-Nazari SS, Barzegar A. Characterizing mortality from substance abuse in Iran: an epidemiological study during march 2014 to February 2015. Addict Health. 2017;9(3):166–74.
PubMed PubMed Central Google Scholar
Fadaei MH, Farokhzadian J, Miri S, Goojani R. Promoting drug abuse preventive behaviors in adolescent students based on the health belief model. Int J Adolesc Med Health. 2020. https://doi.org/10.1515/ijamh-2019-0170 .
Henry BF. Adversity, mental health and substance use disorders as predictors and mediators of rule violations in U.S. prisons. Crim Justice Behav. 2020;47(3):271–89. https://doi.org/10.1177/0093854819896844 .
Scorzelli JF, Chaudhry SZ. Relationship between anxiety and addiction to a depressant drug. J Psychoactive Drugs. 2009;41(1):61–6. https://doi.org/10.1080/02791072.2009.10400675 .
Jalali A, Hassanpor-Dehkordi A, Mahvar T, Moradi M, Dinmohammadi M. Psychological needs of men under methadone maintenance treatment: a mixed method study. Heroin Addict Related Clin Problems. 2015;17:23–31.
Google Scholar
Rahimi S, Jalali A, Jalali R. Psychological needs of women treated with methadone: mixed method study. Alcohol Treat Q. 2019;37(3):328–41. https://doi.org/10.1080/07347324.2018.1554982 .
Article Google Scholar
Sau M, Mukherjee A, Manna N, Sanyal S. Sociodemographic and substance use correlates of repeated relapse among patients presenting for relapse treatment at an addiction treatment center in Kolkata. India Afr Health Sci. 2013;13(3):791–9. https://doi.org/10.4314/ahs.v13i3.39 .
Article CAS PubMed Google Scholar
Jalali A, Behrouzi MK, Salari N, Bazrafshan MR, Rahmati M. The effectiveness of group spiritual intervention on self-esteem and happiness among men undergoing methadone maintenance treatment. Curr Drug Abuse Rev. 2018. https://doi.org/10.2174/1874473711666180510164420 .
Jalali A, Shabrandi B, Jalali R, Salari N. Methamphetamine Abusers' personality traits and its relational with spiritual well-being and perceived social support. Curr Drug Res Rev. 2019;11(1):44–50. https://doi.org/10.2174/1874473711666181017121256 .
Heidari J, Jafari H, Hoseini S, Jannati Y, Mohammadpoor R, Mahmoodi G. Study of psychosocial status of addicts in sari. J Mazandaran Univ Med Sci. 2006;16(52):109–16.
West R, McEwen A, Bolling K. L. O. smoking cessation and smoking patterns in the general population: a 1-year follow-up. Addict Res. 2001;96(6):891–902. https://doi.org/10.1046/j.1360-0443.2001.96689110.x .
Article CAS Google Scholar
Battjes RJ, Gordon MS, O'Grady KE, Kinlock TW, Carswell MA. Factors that predict adolescent motivation for substance abuse treatment, journal of substance abuse treatment. J Subst Abus Treat. 2003;24(3):221–32. https://doi.org/10.1016/s0740-5472(03)00022-9 .
Targowski T, Grabowska P, Rozyńska R, From S, Mierzejewska J, Niedziałkowski P. Comparative analysis of nicotin dependence and motivating to quit smoking in patients with bronchial asthma or COPD. Przegl Lek. 2004;61(10):1046–8.
German D, Sherman SG, Sirirojn B, Thomson N, Aramrattana A, Celentano DD. Motivations for methamphetamine cessation among young people in northern Thailand. Addiction. 2006;101(8):1143–52. https://doi.org/10.1111/j.1360-0443.2006.01490.x .
Behravan H, Miranvari S. A Sociological Analysis of Drug Addict's Relapse in the in the center of the therapeutic community (TC) in Mashhad. lran J Soc Problems. 2010;1(1):45–78 (Inpersian).
Munro BH. Statistical methods for health care research. 5th edition ed. Philadelphia: lippincott williams & wilkins; 2005.
Knight K, Holcom M, Simpson DD. TCU Psychosocial Functioning and Motivation Scales: Manual on Psychometric Properties. Fort Worth: Texas Christian University. Institute of Behavioral Research. 1994.
Wild D, Grove A, Martin M, Eremenco S, McElroy S, Verjee-Lorenz A, et al. Principles of good practice for the translation and cultural adaptation process for patient-reported outcomes (PRO) measures: report of the ISPOR task force for translation and cultural adaptation. Value Health. 2005;8(2):94–104. https://doi.org/10.1111/j.1524-4733.2005.04054.x .
Kalivas PW, Volkow ND. The neural basis of addiction: a pathology of motivation and choice. Am J Psychiatr. 2005;162(8):1403–13. https://doi.org/10.1176/appi.ajp.162.8.1403 .
Poudel A, Sharma C, Gautam S, Poudel A. Psychosocial problems among individuals with substance use disorders in drug rehabilitation centers, Nepal. Subst Abuse Treat Prev Policy. 2016;11(1):28. https://doi.org/10.1186/s13011-016-0072-3 .
Simpson D, GW J. Motivation as a predictor of early dropout from drug abuse treatment, Psychotherapy. Theor Res Pract Train. 1993;30(2):357. https://doi.org/10.1037/0033-3204.30.2.357 .
Gyawali S, Sarkar S. Tramadol for maintenance treatment for an elderly "doda" (poppy husk) user. J Geriatr Mental Health. 2016;3(2):179–81. https://doi.org/10.4103/2348-9995.195682 .
Download references
Acknowledgements
We are grateful to the Deputy for Research and Technology, Kermanshah University of Medical Sciences, for cooperating in this research. We thank all the participants in the study.
This study was drawn from a research project (No. 97037) sponsored by deputy of research and technology of KUMS. The payment was spent on the design and implementation of the study.
Author information
Authors and affiliations.
Department of Nursing, School of Nursing, Iran Young Researchers and Elite Club, Gorgan Branch, Islamic Azad University, Gorgan, Iran
Sana Shahrabadi
Substance Abuse Prevention Research Center, Research Institute for Health, Kermanshah University of Medical Sciences, Kermanshah, Iran
Amir Jalali
Department of Nursing, School of Nursing and Midwifery, Kermanshah University of Medical Sciences, Kermanshah, Iran
Rostam Jalali
Department Anesthesiology, Clinical Development Research Center, Kermanshah University of Medical Sciences, Kermanshah, Iran
Ali Gholami
You can also search for this author in PubMed Google Scholar
Contributions
S Sh, contributed in study concept, study design, data collection and manuscript preparation. A J, contributed in study concept, study design, data Analysis, manuscript preparation and submitting the manuscript R J, contributed in study concept, study design and manuscript preparation. A Gh, contributed in study design, data analysis and manuscript preparation. The author(s) read and approved the final manuscript.
Corresponding author
Correspondence to Amir Jalali .
Ethics declarations
Ethics approval and consent to participate.
After issuing the ID code (IR.KUMS.REC.1396.749) by the KUMS Ethics Committee, data collection was initiated. First, the participants were provided with some explanations on the study and its objectives. All participants completed a written consent to participate in the study.
Consent for publication
Not applicable.
Competing interests
The authors declare that they have no conflict of interest about this work.
Additional information
Publisher’s note.
Springer Nature remains neutral with regard to jurisdictional claims in published maps and institutional affiliations.
Rights and permissions
Open Access This article is licensed under a Creative Commons Attribution 4.0 International License, which permits use, sharing, adaptation, distribution and reproduction in any medium or format, as long as you give appropriate credit to the original author(s) and the source, provide a link to the Creative Commons licence, and indicate if changes were made. The images or other third party material in this article are included in the article's Creative Commons licence, unless indicated otherwise in a credit line to the material. If material is not included in the article's Creative Commons licence and your intended use is not permitted by statutory regulation or exceeds the permitted use, you will need to obtain permission directly from the copyright holder. To view a copy of this licence, visit http://creativecommons.org/licenses/by/4.0/ . The Creative Commons Public Domain Dedication waiver ( http://creativecommons.org/publicdomain/zero/1.0/ ) applies to the data made available in this article, unless otherwise stated in a credit line to the data.
Reprints and permissions
About this article
Cite this article.
Shahrabadi, S., Jalali, A., Jalali, R. et al. Psychological, social, and motivational factors in persons who use drugs. Subst Abuse Treat Prev Policy 15 , 32 (2020). https://doi.org/10.1186/s13011-020-00273-7
Download citation
Received : 31 January 2020
Accepted : 15 April 2020
Published : 29 April 2020
DOI : https://doi.org/10.1186/s13011-020-00273-7
Share this article
Anyone you share the following link with will be able to read this content:
Sorry, a shareable link is not currently available for this article.
Provided by the Springer Nature SharedIt content-sharing initiative
- Psychosocial functioning
- Motivation for treatment
- Persons who use drugs
- Demographical variables
Substance Abuse Treatment, Prevention, and Policy
ISSN: 1747-597X
- General enquiries: [email protected]
- Frontiers in Neuroscience
- Neuropharmacology
- Research Topics
Unraveling Vulnerability Factors in Addiction Drug Use and Potential Treatments
Total Downloads
Total Views and Downloads
About this Research Topic
Drug addiction is a chronic mental disorder characterized by escalating compulsive drug use regardless of its negative consequences and by recurrent relapses, that are the main challenge for its treatment. At the initial phase, abused substances activate the reward system causing pleasurable effects that ...
Keywords : Drug use, environment, genetics, inflammation, treatment
Important Note : All contributions to this Research Topic must be within the scope of the section and journal to which they are submitted, as defined in their mission statements. Frontiers reserves the right to guide an out-of-scope manuscript to a more suitable section or journal at any stage of peer review.
Topic Editors
Topic coordinators, recent articles, submission deadlines.
Submission closed.
Participating Journals
Total views.
- Demographics
No records found
total views article views downloads topic views
Top countries
Top referring sites, about frontiers research topics.
With their unique mixes of varied contributions from Original Research to Review Articles, Research Topics unify the most influential researchers, the latest key findings and historical advances in a hot research area! Find out more on how to host your own Frontiers Research Topic or contribute to one as an author.
- Open access
- Published: 13 November 2021
Risk and protective factors of drug abuse among adolescents: a systematic review
- Azmawati Mohammed Nawi 1 ,
- Rozmi Ismail 2 ,
- Fauziah Ibrahim 2 ,
- Mohd Rohaizat Hassan 1 ,
- Mohd Rizal Abdul Manaf 1 ,
- Noh Amit 3 ,
- Norhayati Ibrahim 3 &
- Nurul Shafini Shafurdin 2
BMC Public Health volume 21 , Article number: 2088 ( 2021 ) Cite this article
126k Accesses
89 Citations
21 Altmetric
Metrics details
Drug abuse is detrimental, and excessive drug usage is a worldwide problem. Drug usage typically begins during adolescence. Factors for drug abuse include a variety of protective and risk factors. Hence, this systematic review aimed to determine the risk and protective factors of drug abuse among adolescents worldwide.
Preferred Reporting Items for Systematic Reviews and Meta-Analyses (PRISMA) was adopted for the review which utilized three main journal databases, namely PubMed, EBSCOhost, and Web of Science. Tobacco addiction and alcohol abuse were excluded in this review. Retrieved citations were screened, and the data were extracted based on strict inclusion and exclusion criteria. Inclusion criteria include the article being full text, published from the year 2016 until 2020 and provided via open access resource or subscribed to by the institution. Quality assessment was done using Mixed Methods Appraisal Tools (MMAT) version 2018 to assess the methodological quality of the included studies. Given the heterogeneity of the included studies, a descriptive synthesis of the included studies was undertaken.
Out of 425 articles identified, 22 quantitative articles and one qualitative article were included in the final review. Both the risk and protective factors obtained were categorized into three main domains: individual, family, and community factors. The individual risk factors identified were traits of high impulsivity; rebelliousness; emotional regulation impairment, low religious, pain catastrophic, homework completeness, total screen time and alexithymia; the experience of maltreatment or a negative upbringing; having psychiatric disorders such as conduct problems and major depressive disorder; previous e-cigarette exposure; behavioral addiction; low-perceived risk; high-perceived drug accessibility; and high-attitude to use synthetic drugs. The familial risk factors were prenatal maternal smoking; poor maternal psychological control; low parental education; negligence; poor supervision; uncontrolled pocket money; and the presence of substance-using family members. One community risk factor reported was having peers who abuse drugs. The protective factors determined were individual traits of optimism; a high level of mindfulness; having social phobia; having strong beliefs against substance abuse; the desire to maintain one’s health; high paternal awareness of drug abuse; school connectedness; structured activity and having strong religious beliefs.
The outcomes of this review suggest a complex interaction between a multitude of factors influencing adolescent drug abuse. Therefore, successful adolescent drug abuse prevention programs will require extensive work at all levels of domains.
Peer Review reports
Introduction
Drug abuse is a global problem; 5.6% of the global population aged 15–64 years used drugs at least once during 2016 [ 1 ]. The usage of drugs among younger people has been shown to be higher than that among older people for most drugs. Drug abuse is also on the rise in many ASEAN (Association of Southeast Asian Nations) countries, especially among young males between 15 and 30 years of age. The increased burden due to drug abuse among adolescents and young adults was shown by the Global Burden of Disease (GBD) study in 2013 [ 2 ]. About 14% of the total health burden in young men is caused by alcohol and drug abuse. Younger people are also more likely to die from substance use disorders [ 3 ], and cannabis is the drug of choice among such users [ 4 ].
Adolescents are the group of people most prone to addiction [ 5 ]. The critical age of initiation of drug use begins during the adolescent period, and the maximum usage of drugs occurs among young people aged 18–25 years old [ 1 ]. During this period, adolescents have a strong inclination toward experimentation, curiosity, susceptibility to peer pressure, rebellion against authority, and poor self-worth, which makes such individuals vulnerable to drug abuse [ 2 ]. During adolescence, the basic development process generally involves changing relations between the individual and the multiple levels of the context within which the young person is accustomed. Variation in the substance and timing of these relations promotes diversity in adolescence and represents sources of risk or protective factors across this life period [ 6 ]. All these factors are crucial to helping young people develop their full potential and attain the best health in the transition to adulthood. Abusing drugs impairs the successful transition to adulthood by impairing the development of critical thinking and the learning of crucial cognitive skills [ 7 ]. Adolescents who abuse drugs are also reported to have higher rates of physical and mental illness and reduced overall health and well-being [ 8 ].
The absence of protective factors and the presence of risk factors predispose adolescents to drug abuse. Some of the risk factors are the presence of early mental and behavioral health problems, peer pressure, poorly equipped schools, poverty, poor parental supervision and relationships, a poor family structure, a lack of opportunities, isolation, gender, and accessibility to drugs [ 9 ]. The protective factors include high self-esteem, religiosity, grit, peer factors, self-control, parental monitoring, academic competence, anti-drug use policies, and strong neighborhood attachment [ 10 , 11 , 12 , 13 , 14 , 15 ].
The majority of previous systematic reviews done worldwide on drug usage focused on the mental, psychological, or social consequences of substance abuse [ 16 , 17 , 18 ], while some focused only on risk and protective factors for the non-medical use of prescription drugs among youths [ 19 ]. A few studies focused only on the risk factors of single drug usage among adolescents [ 20 ]. Therefore, the development of the current systematic review is based on the main research question: What is the current risk and protective factors among adolescent on the involvement with drug abuse? To the best of our knowledge, there is limited evidence from systematic reviews that explores the risk and protective factors among the adolescent population involved in drug abuse. Especially among developing countries, such as those in South East Asia, such research on the risk and protective factors for drug abuse is scarce. Furthermore, this review will shed light on the recent trends of risk and protective factors and provide insight into the main focus factors for prevention and control activities program. Additionally, this review will provide information on how these risk and protective factors change throughout various developmental stages. Therefore, the objective of this systematic review was to determine the risk and protective factors of drug abuse among adolescents worldwide. This paper thus fills in the gaps of previous studies and adds to the existing body of knowledge. In addition, this review may benefit certain parties in developing countries like Malaysia, where the national response to drugs is developing in terms of harm reduction, prison sentences, drug treatments, law enforcement responses, and civil society participation.
This systematic review was conducted using three databases, PubMed, EBSCOhost, and Web of Science, considering the easy access and wide coverage of reliable journals, focusing on the risk and protective factors of drug abuse among adolescents from 2016 until December 2020. The search was limited to the last 5 years to focus only on the most recent findings related to risk and protective factors. The search strategy employed was performed in accordance with the Preferred Reporting Items for a Systematic Review and Meta-analysis (PRISMA) checklist.
A preliminary search was conducted to identify appropriate keywords and determine whether this review was feasible. Subsequently, the related keywords were searched using online thesauruses, online dictionaries, and online encyclopedias. These keywords were verified and validated by an academic professor at the National University of Malaysia. The keywords used as shown in Table 1 .
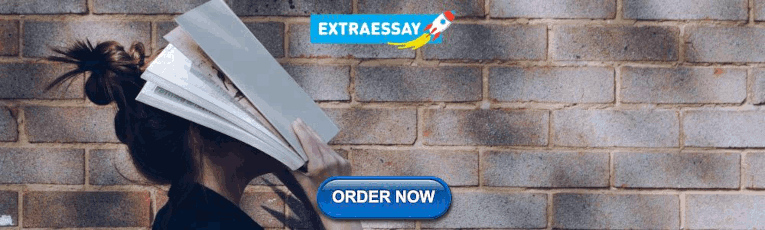
Selection criteria
The systematic review process for searching the articles was carried out via the steps shown in Fig. 1 . Firstly, screening was done to remove duplicate articles from the selected search engines. A total of 240 articles were removed in this stage. Titles and abstracts were screened based on the relevancy of the titles to the inclusion and exclusion criteria and the objectives. The inclusion criteria were full text original articles, open access articles or articles subscribed to by the institution, observation and intervention study design and English language articles. The exclusion criteria in this search were (a) case study articles, (b) systematic and narrative review paper articles, (c) non-adolescent-based analyses, (d) non-English articles, and (e) articles focusing on smoking (nicotine) and alcohol-related issues only. A total of 130 articles were excluded after title and abstract screening, leaving 55 articles to be assessed for eligibility. The full text of each article was obtained, and each full article was checked thoroughly to determine if it would fulfil the inclusion criteria and objectives of this study. Each of the authors compared their list of potentially relevant articles and discussed their selections until a final agreement was obtained. A total of 22 articles were accepted to be included in this review. Most of the excluded articles were excluded because the population was not of the target age range—i.e., featuring subjects with an age > 18 years, a cohort born in 1965–1975, or undergraduate college students; the subject matter was not related to the study objective—i.e., assessing the effects on premature mortality, violent behavior, psychiatric illness, individual traits, and personality; type of article such as narrative review and neuropsychiatry review; and because of our inability to obtain the full article—e.g., forthcoming work in 2021. One qualitative article was added to explain the domain related to risk and the protective factors among the adolescents.
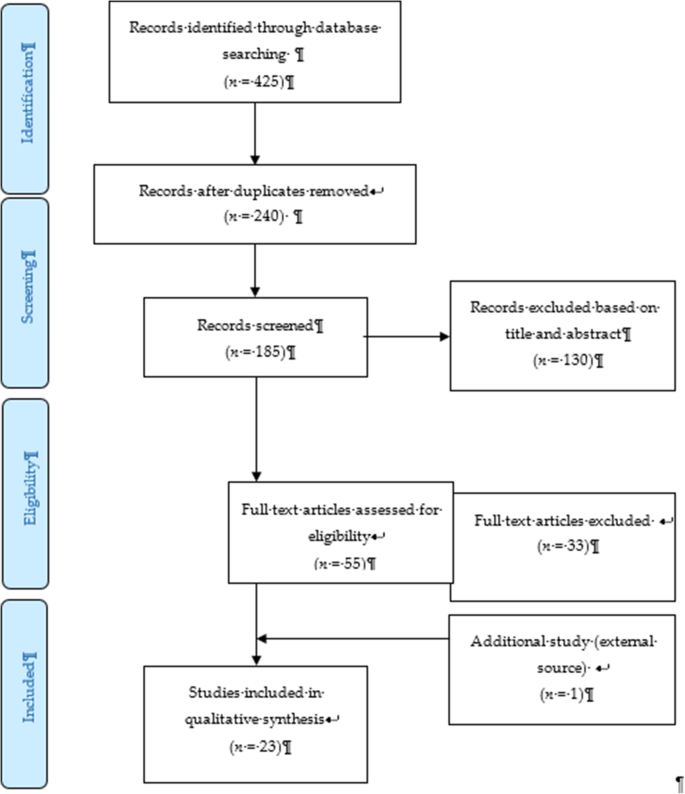
PRISMA flow diagram showing the selection of studies on risk and protective factors for drug abuse among adolescents.2.2. Operational Definition
Drug-related substances in this context refer to narcotics, opioids, psychoactive substances, amphetamines, cannabis, ecstasy, heroin, cocaine, hallucinogens, depressants, and stimulants. Drugs of abuse can be either off-label drugs or drugs that are medically prescribed. The two most commonly abused substances not included in this review are nicotine (tobacco) and alcohol. Accordingly, e-cigarettes and nicotine vape were also not included. Further, “adolescence” in this study refers to members of the population aged between 10 to 18 years [ 21 ].
Data extraction tool
All researchers independently extracted information for each article into an Excel spreadsheet. The data were then customized based on their (a) number; (b) year; (c) author and country; (d) titles; (e) study design; (f) type of substance abuse; (g) results—risks and protective factors; and (h) conclusions. A second reviewer crossed-checked the articles assigned to them and provided comments in the table.
Quality assessment tool
By using the Mixed Method Assessment Tool (MMAT version 2018), all articles were critically appraised for their quality by two independent reviewers. This tool has been shown to be useful in systematic reviews encompassing different study designs [ 22 ]. Articles were only selected if both reviewers agreed upon the articles’ quality. Any disagreement between the assigned reviewers was managed by employing a third independent reviewer. All included studies received a rating of “yes” for the questions in the respective domains of the MMAT checklists. Therefore, none of the articles were removed from this review due to poor quality. The Cohen’s kappa (agreement) between the two reviewers was 0.77, indicating moderate agreement [ 23 ].
The initial search found 425 studies for review, but after removing duplicates and applying the criteria listed above, we narrowed the pool to 22 articles, all of which are quantitative in their study design. The studies include three prospective cohort studies [ 24 , 25 , 26 ], one community trial [ 27 ], one case-control study [ 28 ], and nine cross-sectional studies [ 29 , 30 , 31 , 32 , 33 , 34 , 35 , 36 , 37 , 38 , 39 , 40 , 41 , 42 , 43 , 44 , 45 ]. After careful discussion, all reviewer panels agreed to add one qualitative study [ 46 ] to help provide reasoning for the quantitative results. The selected qualitative paper was chosen because it discussed almost all domains on the risk and protective factors found in this review.
A summary of all 23 articles is listed in Table 2 . A majority of the studies (13 articles) were from the United States of America (USA) [ 25 , 26 , 27 , 29 , 30 , 31 , 34 , 36 , 37 , 38 , 39 , 40 , 41 , 42 , 43 , 44 , 45 ], three studies were from the Asia region [ 32 , 33 , 38 ], four studies were from Europe [ 24 , 28 , 40 , 44 ], and one study was from Latin America [ 35 ], Africa [ 43 ] and Mediterranean [ 45 ]. The number of sample participants varied widely between the studies, ranging from 70 samples (minimum) to 700,178 samples (maximum), while the qualitative paper utilized a total of 100 interviewees. There were a wide range of drugs assessed in the quantitative articles, with marijuana being mentioned in 11 studies, cannabis in five studies, and opioid (six studies). There was also large heterogeneity in terms of the study design, type of drug abused, measurements of outcomes, and analysis techniques used. Therefore, the data were presented descriptively.
After thorough discussion and evaluation, all the findings (both risk and protective factors) from the review were categorized into three main domains: individual factors, family factors, and community factors. The conceptual framework is summarized in Fig. 2 .
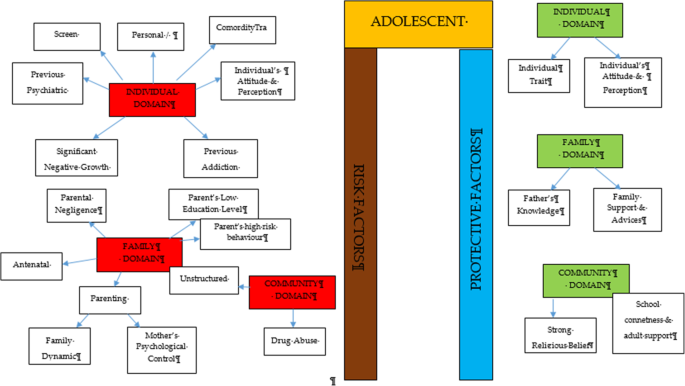
Conceptual framework of risk and protective factors related to adolescent drug abuse
DOMAIN: individual factor
Risk factors.
Almost all the articles highlighted significant findings of individual risk factors for adolescent drug abuse. Therefore, our findings for this domain were further broken down into five more sub-domains consisting of personal/individual traits, significant negative growth exposure, personal psychiatric diagnosis, previous substance history, comorbidity and an individual’s attitude and perception.
Personal/individual traits
Chuang et al. [ 29 ] found that adolescents with high impulsivity traits had a significant positive association with drug addiction. This study also showed that the impulsivity trait alone was an independent risk factor that increased the odds between two to four times for using any drug compared to the non-impulsive group. Another longitudinal study by Guttmannova et al. showed that rebellious traits are positively associated with marijuana drug abuse [ 27 ]. The authors argued that measures of rebelliousness are a good proxy for a youth’s propensity to engage in risky behavior. Nevertheless, Wilson et al. [ 37 ], in a study involving 112 youths undergoing detoxification treatment for opioid abuse, found that a majority of the affected respondents had difficulty in regulating their emotions. The authors found that those with emotional regulation impairment traits became opioid dependent at an earlier age. Apart from that, a case-control study among outpatient youths found that adolescents involved in cannabis abuse had significant alexithymia traits compared to the control population [ 28 ]. Those adolescents scored high in the dimension of Difficulty in Identifying Emotion (DIF), which is one of the key definitions of diagnosing alexithymia. Overall, the adjusted Odds Ratio for DIF in cannabis abuse was 1.11 (95% CI, 1.03–1.20).
Significant negative growth exposure
A history of maltreatment in the past was also shown to have a positive association with adolescent drug abuse. A study found that a history of physical abuse in the past is associated with adolescent drug abuse through a Path Analysis, despite evidence being limited to the female gender [ 25 ]. However, evidence from another study focusing at foster care concluded that any type of maltreatment might result in a prevalence as high as 85.7% for the lifetime use of cannabis and as high as 31.7% for the prevalence of cannabis use within the last 3-months [ 30 ]. The study also found significant latent variables that accounted for drug abuse outcomes, which were chronic physical maltreatment (factor loading of 0.858) and chronic psychological maltreatment (factor loading of 0.825), with an r 2 of 73.6 and 68.1%, respectively. Another study shed light on those living in child welfare service (CWS) [ 35 ]. It was observed through longitudinal measurements that proportions of marijuana usage increased from 9 to 18% after 36 months in CWS. Hence, there is evidence of the possibility of a negative upbringing at such shelters.
Personal psychiatric diagnosis
The robust studies conducted in the USA have deduced that adolescents diagnosed with a conduct problem (CP) have a positive association with marijuana abuse (OR = 1.75 [1.56, 1.96], p < 0.0001). Furthermore, those with a diagnosis of Major Depressive Disorder (MDD) showed a significant positive association with marijuana abuse.
Previous substance and addiction history
Another study found that exposure to e-cigarettes within the past 30 days is related to an increase in the prevalence of marijuana use and prescription drug use by at least four times in the 8th and 10th grades and by at least three times in the 12th grade [ 34 ]. An association between other behavioral addictions and the development of drug abuse was also studied [ 29 ]. Using a 12-item index to assess potential addictive behaviors [ 39 ], significant associations between drug abuse and the groups with two behavioral addictions (OR = 3.19, 95% CI 1.25,9.77) and three behavioral addictions (OR = 3.46, 95% CI 1.25,9.58) were reported.
Comorbidity
The paper by Dash et al. (2020) highlight adolescent with a disease who needs routine medical pain treatment have higher risk of opioid misuse [ 38 ]. The adolescents who have disorder symptoms may have a risk for opioid misuse despite for the pain intensity.
Individual’s attitudes and perceptions
In a study conducted in three Latin America countries (Argentina, Chile, and Uruguay), it was shown that adolescents with low or no perceived risk of taking marijuana had a higher risk of abuse (OR = 8.22 times, 95% CI 7.56, 10.30) [ 35 ]. This finding is in line with another study that investigated 2002 adolescents and concluded that perceiving the drug as harmless was an independent risk factor that could prospectively predict future marijuana abuse [ 27 ]. Moreover, some youth interviewed perceived that they gained benefits from substance use [ 38 ]. The focus group discussion summarized that the youth felt positive personal motivation and could escape from a negative state by taking drugs. Apart from that, adolescents who had high-perceived availability of drugs in their neighborhoods were more likely to increase their usage of marijuana over time (OR = 11.00, 95% CI 9.11, 13.27) [ 35 ]. A cheap price of the substance and the availability of drug dealers around schools were factors for youth accessibility [ 38 ]. Perceived drug accessibility has also been linked with the authorities’ enforcement programs. The youth perception of a lax community enforcement of laws regarding drug use at all-time points predicted an increase in marijuana use in the subsequent assessment period [ 27 ]. Besides perception, a study examining the attitudes towards synthetic drugs based on 8076 probabilistic samples of Macau students found that the odds of the lifetime use of marijuana was almost three times higher among those with a strong attitude towards the use of synthetic drugs [ 32 ]. In addition, total screen time among the adolescent increase the likelihood of frequent cannabis use. Those who reported daily cannabis use have a mean of 12.56 h of total screen time, compared to a mean of 6.93 h among those who reported no cannabis use. Adolescent with more time on internet use, messaging, playing video games and watching TV/movies were significantly associated with more frequent cannabis use [ 44 ].
Protective factors
Individual traits.
Some individual traits have been determined to protect adolescents from developing drug abuse habits. A study by Marin et al. found that youth with an optimistic trait were less likely to become drug dependent [ 33 ]. In this study involving 1104 Iranian students, it was concluded that a higher optimism score (measured using the Children Attributional Style Questionnaire, CASQ) was a protective factor against illicit drug use (OR = 0.90, 95% CI: 0.85–0.95). Another study found that high levels of mindfulness, measured using the 25-item Child Acceptance and Mindfulness Measure, CAMM, lead to a slower progression toward injectable drug abuse among youth with opioid addiction (1.67 years, p = .041) [ 37 ]. In addition, the social phobia trait was found to have a negative association with marijuana use (OR = 0.87, 95% CI 0.77–0.97), as suggested [ 31 ].
According to El Kazdouh et al., individuals with a strong belief against substance use and those with a strong desire to maintain their health were more likely to be protected from involvement in drug abuse [ 46 ].
DOMAIN: family factors
The biological factors underlying drug abuse in adolescents have been reported in several studies. Epigenetic studies are considered important, as they can provide a good outline of the potential pre-natal factors that can be targeted at an earlier stage. Expecting mothers who smoke tobacco and alcohol have an indirect link with adolescent substance abuse in later life [ 24 , 39 ]. Moreover, the dynamic relationship between parents and their children may have some profound effects on the child’s growth. Luk et al. examined the mediator effects between parenting style and substance abuse and found the maternal psychological control dimension to be a significant variable [ 26 ]. The mother’s psychological control was two times higher in influencing her children to be involved in substance abuse compared to the other dimension. Conversely, an indirect risk factor towards youth drug abuse was elaborated in a study in which low parental educational level predicted a greater risk of future drug abuse by reducing the youth’s perception of harm [ 27 , 43 ]. Negligence from a parental perspective could also contribute to this problem. According to El Kazdouh et al. [ 46 ], a lack of parental supervision, uncontrolled pocket money spending among children, and the presence of substance-using family members were the most common negligence factors.
While the maternal factors above were shown to be risk factors, the opposite effect was seen when the paternal figure equipped himself with sufficient knowledge. A study found that fathers with good information and awareness were more likely to protect their adolescent children from drug abuse [ 26 ]. El Kazdouh et al. noted that support and advice could be some of the protective factors in this area [ 46 ].
DOMAIN: community factors
- Risk factor
A study in 2017 showed a positive association between adolescent drug abuse and peers who abuse drugs [ 32 , 39 ]. It was estimated that the odds of becoming a lifetime marijuana user was significantly increased by a factor of 2.5 ( p < 0.001) among peer groups who were taking synthetic drugs. This factor served as peer pressure for youth, who subconsciously had desire to be like the others [ 38 ]. The impact of availability and engagement in structured and unstructured activities also play a role in marijuana use. The findings from Spillane (2000) found that the availability of unstructured activities was associated with increased likelihood of marijuana use [ 42 ].
- Protective factor
Strong religious beliefs integrated into society serve as a crucial protective factor that can prevent adolescents from engaging in drug abuse [ 38 , 45 ]. In addition, the school connectedness and adult support also play a major contribution in the drug use [ 40 ].
The goal of this review was to identify and classify the risks and protective factors that lead adolescents to drug abuse across the three important domains of the individual, family, and community. No findings conflicted with each other, as each of them had their own arguments and justifications. The findings from our review showed that individual factors were the most commonly highlighted. These factors include individual traits, significant negative growth exposure, personal psychiatric diagnosis, previous substance and addiction history, and an individual’s attitude and perception as risk factors.
Within the individual factor domain, nine articles were found to contribute to the subdomain of personal/ individual traits [ 27 , 28 , 29 , 37 , 38 , 39 , 40 , 43 , 44 ]. Despite the heterogeneity of the study designs and the substances under investigation, all of the papers found statistically significant results for the possible risk factors of adolescent drug abuse. The traits of high impulsivity, rebelliousness, difficulty in regulating emotions, and alexithymia can be considered negative characteristic traits. These adolescents suffer from the inability to self-regulate their emotions, so they tend to externalize their behaviors as a way to avoid or suppress the negative feelings that they are experiencing [ 41 , 47 , 48 ]. On the other hand, engaging in such behaviors could plausibly provide a greater sense of positive emotions and make them feel good [ 49 ]. Apart from that, evidence from a neurophysiological point of view also suggests that the compulsive drive toward drug use is complemented by deficits in impulse control and decision making (impulsive trait) [ 50 ]. A person’s ability in self-control will seriously impaired with continuous drug use and will lead to the hallmark of addiction [ 51 ].
On the other hand, there are articles that reported some individual traits to be protective for adolescents from engaging in drug abuse. Youth with the optimistic trait, a high level of mindfulness, and social phobia were less likely to become drug dependent [ 31 , 33 , 37 ]. All of these articles used different psychometric instruments to classify each individual trait and were mutually exclusive. Therefore, each trait measured the chance of engaging in drug abuse on its own and did not reflect the chance at the end of the spectrum. These findings show that individual traits can be either protective or risk factors for the drugs used among adolescents. Therefore, any adolescent with negative personality traits should be monitored closely by providing health education, motivation, counselling, and emotional support since it can be concluded that negative personality traits are correlated with high risk behaviours such as drug abuse [ 52 ].
Our study also found that a history of maltreatment has a positive association with adolescent drug abuse. Those adolescents with episodes of maltreatment were considered to have negative growth exposure, as their childhoods were negatively affected by traumatic events. Some significant associations were found between maltreatment and adolescent drug abuse, although the former factor was limited to the female gender [ 25 , 30 , 36 ]. One possible reason for the contrasting results between genders is the different sample populations, which only covered child welfare centers [ 36 ] and foster care [ 30 ]. Regardless of the place, maltreatment can happen anywhere depending on the presence of the perpetrators. To date, evidence that concretely links maltreatment and substance abuse remains limited. However, a plausible explanation for this link could be the indirect effects of posttraumatic stress (i.e., a history of maltreatment) leading to substance use [ 53 , 54 ]. These findings highlight the importance of continuous monitoring and follow-ups with adolescents who have a history of maltreatment and who have ever attended a welfare center.
Addiction sometimes leads to another addiction, as described by the findings of several studies [ 29 , 34 ]. An initial study focused on the effects of e-cigarettes in the development of other substance abuse disorders, particularly those related to marijuana, alcohol, and commonly prescribed medications [ 34 ]. The authors found that the use of e-cigarettes can lead to more severe substance addiction [ 55 ], possibly through normalization of the behavior. On the other hand, Chuang et al.’s extensive study in 2017 analyzed the combined effects of either multiple addictions alone or a combination of multiple addictions together with the impulsivity trait [ 29 ]. The outcomes reported were intriguing and provide the opportunity for targeted intervention. The synergistic effects of impulsiveness and three other substance addictions (marijuana, tobacco, and alcohol) substantially increased the likelihood for drug abuse from 3.46 (95%CI 1.25, 9.58) to 10.13 (95% CI 3.95, 25.95). Therefore, proper rehabilitation is an important strategy to ensure that one addiction will not lead to another addiction.
The likelihood for drug abuse increases as the population perceives little or no harmful risks associated with the drugs. On the opposite side of the coin, a greater perceived risk remains a protective factor for marijuana abuse [ 56 ]. However, another study noted that a stronger determinant for adolescent drug abuse was the perceived availability of the drug [ 35 , 57 ]. Looking at the bigger picture, both perceptions corroborate each other and may inform drug use. Another study, on the other hand, reported that there was a decreasing trend of perceived drug risk in conjunction with the increasing usage of drugs [ 58 ]. As more people do drugs, youth may inevitably perceive those drugs as an acceptable norm without any harmful consequences [ 59 ].
In addition, the total spent for screen time also contribute to drug abuse among adolescent [ 43 ]. This scenario has been proven by many researchers on the effect of screen time on the mental health [ 60 ] that leads to the substance use among the adolescent due to the ubiquity of pro-substance use content on the internet. Adolescent with comorbidity who needs medical pain management by opioids also tend to misuse in future. A qualitative exploration on the perspectives among general practitioners concerning the risk of opioid misuse in people with pain, showed pain management by opioids is a default treatment and misuse is not a main problem for the them [ 61 ]. A careful decision on the use of opioids as a pain management should be consider among the adolescents and their understanding is needed.
Within the family factor domain, family structures were found to have both positive and negative associations with drug abuse among adolescents. As described in one study, paternal knowledge was consistently found to be a protective factor against substance abuse [ 26 ]. With sufficient knowledge, the father can serve as the guardian of his family to monitor and protect his children from negative influences [ 62 ]. The work by Luk et al. also reported a positive association of maternal psychological association towards drug abuse (IRR 2.41, p < 0.05) [ 26 ]. The authors also observed the same effect of paternal psychological control, although it was statistically insignificant. This construct relates to parenting style, and the authors argued that parenting style might have a profound effect on the outcomes under study. While an earlier literature review [ 63 ] also reported such a relationship, a recent study showed a lesser impact [ 64 ] with regards to neglectful parenting styles leading to poorer substance abuse outcomes. Nevertheless, it was highlighted in another study that the adolescents’ perception of a neglectful parenting style increased their odds (OR 2.14, p = 0.012) of developing alcohol abuse, not the parenting style itself [ 65 ]. Altogether, families play vital roles in adolescents’ risk for engaging in substance abuse [ 66 ]. Therefore, any intervention to impede the initiation of substance use or curb existing substance use among adolescents needs to include parents—especially improving parent–child communication and ensuring that parents monitor their children’s activities.
Finally, the community also contributes to drug abuse among adolescents. As shown by Li et al. [ 32 ] and El Kazdouh et al. [ 46 ], peers exert a certain influence on other teenagers by making them subconsciously want to fit into the group. Peer selection and peer socialization processes might explain why peer pressure serves as a risk factor for drug-abuse among adolescents [ 67 ]. Another study reported that strong religious beliefs integrated into society play a crucial role in preventing adolescents from engaging in drug abuse [ 46 ]. Most religions devalue any actions that can cause harmful health effects, such as substance abuse [ 68 ]. Hence, spiritual beliefs may help protect adolescents. This theme has been well established in many studies [ 60 , 69 , 70 , 71 , 72 ] and, therefore, could be implemented by religious societies as part of interventions to curb the issue of adolescent drug abuse. The connection with school and structured activity did reduce the risk as a study in USA found exposure to media anti-drug messages had an indirect negative effect on substances abuse through school-related activity and social activity [ 73 ]. The school activity should highlight on the importance of developmental perspective when designing and offering school-based prevention programs [75].
Limitations
We adopted a review approach that synthesized existing evidence on the risk and protective factors of adolescents engaging in drug abuse. Although this systematic review builds on the conclusion of a rigorous review of studies in different settings, there are some potential limitations to this work. We may have missed some other important factors, as we only included English articles, and article extraction was only done from the three search engines mentioned. Nonetheless, this review focused on worldwide drug abuse studies, rather than the broader context of substance abuse including alcohol and cigarettes, thereby making this paper more focused.
Conclusions
This review has addressed some recent knowledge related to the individual, familial, and community risk and preventive factors for adolescent drug use. We suggest that more attention should be given to individual factors since most findings were discussed in relation to such factors. With the increasing trend of drug abuse, it will be critical to focus research specifically on this area. Localized studies, especially those related to demographic factors, may be more effective in generating results that are specific to particular areas and thus may be more useful in generating and assessing local control and prevention efforts. Interventions using different theory-based psychotherapies and a recognition of the unique developmental milestones specific to adolescents are among examples that can be used. Relevant holistic approaches should be strengthened not only by relevant government agencies but also by the private sector and non-governmental organizations by promoting protective factors while reducing risk factors in programs involving adolescents from primary school up to adulthood to prevent and control drug abuse. Finally, legal legislation and enforcement against drug abuse should be engaged with regularly as part of our commitment to combat this public health burden.
Data availability and materials
All data generated or analysed during this study are included in this published article.
Nation, U. World Drug Report 2018 (United Nations publication, Sales No. E.18X.XI.9. United Nation publication). 2018. Retrieved from https://www.unodc.org/wdr2018
Google Scholar
Degenhardt L, Stockings E, Patton G, Hall WD, Lynskey M. The increasing global health priority of substance use in young people. Lancet Psychiatry. 2016;3(3):251–64. https://doi.org/10.1016/S2215-0366(15)00508-8 Elsevier Ltd.
Article PubMed Google Scholar
Ritchie H, Roser M. Drug Use - Our World in Data: Global Change Data Lab; 2019. https://ourworldindata.org/drug-use [10 June 2020]
Holm S, Sandberg S, Kolind T, Hesse M. The importance of cannabis culture in young adult cannabis use. J Subst Abus. 2014;19(3):251–6.
Luikinga SJ, Kim JH, Perry CJ. Developmental perspectives on methamphetamine abuse: exploring adolescent vulnerabilities on brain and behavior. Progress Neuro Psychopharmacol Biol Psychiatry. 2018;87(Pt A):78–84. https://doi.org/10.1016/j.pnpbp.2017.11.010 Elsevier Inc.
Article CAS Google Scholar
Ismail R, Ghazalli MN, Ibrahim N. Not all developmental assets can predict negative mental health outcomes of disadvantaged youth: a case of suburban Kuala Lumpur. Mediterr J Soc Sci. 2015;6(1):452–9. https://doi.org/10.5901/mjss.2015.v6n5s1p452 .
Article Google Scholar
Crews F, He J, Hodge C. Adolescent cortical development: a critical period of vulnerability for addiction. Pharmacol Biochem Behav. 2007;86(2):189–99. https://doi.org/10.1016/j.pbb.2006.12.001 .
Article CAS PubMed Google Scholar
Schulte MT, Hser YI. Substance use and associated health conditions throughout the lifespan. Public Health Rev. 2013;35(2). https://doi.org/10.1007/bf03391702 Technosdar Ltd.
Somani, S.; Meghani S. Substance Abuse among Youth: A Harsh Reality 2016. doi: https://doi.org/10.4172/2165-7548.1000330 , 6, 4.
Book Google Scholar
Drabble L, Trocki KF, Klinger JL. Religiosity as a protective factor for hazardous drinking and drug use among sexual minority and heterosexual women: findings from the National Alcohol Survey. Drug Alcohol Depend. 2016;161:127–34. https://doi.org/10.1016/j.drugalcdep.2016.01.022 .
Article PubMed PubMed Central Google Scholar
Goliath V, Pretorius B. Peer risk and protective factors in adolescence: Implications for drug use prevention. Soc Work. 2016;52(1):113–29. https://doi.org/10.15270/52-1-482 .
Guerrero LR, Dudovitz R, Chung PJ, Dosanjh KK, Wong MD. Grit: a potential protective factor against substance use and other risk behaviors among Latino adolescents. Acad Pediatr. 2016;16(3):275–81. https://doi.org/10.1016/j.acap.2015.12.016 .
National Institutes on Drug Abuse. What are risk factors and protective factors? National Institute on Drug Abuse (NIDA); 2003. Retrieved from https://www.drugabuse.gov/publications/preventing-drug-use-among-children-adolescents/chapter-1-risk-factors-protective-factors/what-are-risk-factors
Nguyen NN, Newhill CE. The role of religiosity as a protective factor against marijuana use among African American, White, Asian, and Hispanic adolescents. J Subst Abus. 2016;21(5):547–52. https://doi.org/10.3109/14659891.2015.1093558 .
Schinke S, Schwinn T, Hopkins J, Wahlstrom L. Drug abuse risk and protective factors among Hispanic adolescents. Prev Med Rep. 2016;3:185–8. https://doi.org/10.1016/j.pmedr.2016.01.012 .
Macleod J, Oakes R, Copello A, Crome PI, Egger PM, Hickman M, et al. Psychological and social sequelae of cannabis and other illicit drug use by young people: a systematic review of longitudinal, general population studies. Lancet. 2004;363(9421):1579–88. https://doi.org/10.1016/S0140-6736(04)16200-4 .
Moore TH, Zammit S, Lingford-Hughes A, Barnes TR, Jones PB, Burke M, et al. Cannabis use and risk of psychotic or affective mental health outcomes: a systematic review. Lancet. 2007;370(9584):319–28. https://doi.org/10.1016/S0140-6736(07)61162-3 .
Semple DM, McIntosh AM, Lawrie SM. Cannabis as a risk factor for psychosis: systematic review. J Psychopharmacol. 2005;19(2):187–94. https://doi.org/10.1177/0269881105049040 .
Nargiso JE, Ballard EL, Skeer MR. A systematic review of risk and protective factors associated with nonmedical use of prescription drugs among youth in the united states: A social ecological perspective. J Stud Alcohol Drugs. 2015;76(1):5–20. https://doi.org/10.15288/jsad.2015.76.5 .
Guxensa M, Nebot M, Ariza C, Ochoa D. Factors associated with the onset of cannabis use: a systematic review of cohort studies. Gac Sanit. 2007;21(3):252–60. https://doi.org/10.1157/13106811 .
Susan MS, Peter SA, Dakshitha W, George CP. The age of adolescence. Lancet Child Adolesc Health. 2018;2(Issue 3):223–8. https://doi.org/10.1016/S2352-4642(18)30022-1 .
Hong QN, Fàbregues S, Bartlett G, Boardman F, Cargo M, Dagenais P, et al. The mixed methods appraisal tool (MMAT) version 2018 for information professionals and researchers. Educ Inf. 2018;34(4):285–91. https://doi.org/10.3233/EFI-180221 .
McHugh ML. Interrater reliability: The kappa statistic. Biochem Med. 2012;22(3):276–82. https://doi.org/10.11613/bm.2012.031 .
Cecil CAM, Walton E, Smith RG, Viding E, McCrory EJ, Relton CL, et al. DNA methylation and substance-use risk: a prospective, genome-wide study spanning gestation to adolescence. Transl Psychiatry. 2016;6(12):e976. https://doi.org/10.1038/tp.2016.247 Nature Publishing Group.
Article CAS PubMed PubMed Central Google Scholar
Kobulsky JM. Gender differences in pathways from physical and sexual abuse to early substance use. Child Youth Serv Rev. 2017;83:25–32. https://doi.org/10.1016/j.childyouth.2017.10.027 .
Luk JW, King KM, McCarty CA, McCauley E, Stoep A. Prospective effects of parenting on substance use and problems across Asian/Pacific islander and European American youth: Tests of moderated mediation. J Stud Alcohol Drugs. 2017;78(4):521–30. https://doi.org/10.15288/jsad.2017.78.521 .
Guttmannova K, Skinner ML, Oesterle S, White HR, Catalano RF, Hawkins JD. The interplay between marijuana-specific risk factors and marijuana use over the course of adolescence. Prev Sci. 2019;20(2):235–45. https://doi.org/10.1007/s11121-018-0882-9 .
Dorard G, Bungener C, Phan O, Edel Y, Corcos M, Berthoz S. Is alexithymia related to cannabis use disorder? Results from a case-control study in outpatient adolescent cannabis abusers. J Psychosom Res. 2017;95:74–80. https://doi.org/10.1016/j.jpsychores.2017.02.012 .
Chuang CWI, Sussman S, Stone MD, Pang RD, Chou CP, Leventhal AM, et al. Impulsivity and history of behavioral addictions are associated with drug use in adolescents. Addict Behav. 2017;74:41–7. https://doi.org/10.1016/j.addbeh.2017.05.021 .
Gabrielli J, Jackson Y, Brown S. Associations between maltreatment history and severity of substance use behavior in youth in Foster Care. Child Maltreat. 2016;21(4):298–307. https://doi.org/10.1177/1077559516669443 .
Khoddam R, Jackson NJ, Leventhal AM. Internalizing symptoms and conduct problems: redundant, incremental, or interactive risk factors for adolescent substance use during the first year of high school? Drug Alcohol Depend. 2016;169:48–55. https://doi.org/10.1016/j.drugalcdep.2016.10.007 .
Li SD, Zhang X, Tang W, Xia Y. Predictors and implications of synthetic drug use among adolescents in the gambling Capital of China. SAGE Open. 2017;7(4):215824401773303. https://doi.org/10.1177/2158244017733031 .
Marin S, Heshmatian E, Nadrian H, Fakhari A, Mohammadpoorasl A. Associations between optimism, tobacco smoking and substance abuse among Iranian high school students. Health Promot Perspect. 2019;9(4):279–84. https://doi.org/10.15171/hpp.2019.38 .
Miech RA, O’Malley PM, Johnston LD, Patrick ME. E-cigarettes and the drug use patterns of adolescents. Nicotine Tob Res. 2015;18(5):654–9. https://doi.org/10.1093/ntr/ntv217 .
Schleimer JP, Rivera-Aguirre AE, Castillo-Carniglia A, Laqueur HS, Rudolph KE, Suárez H, et al. Investigating how perceived risk and availability of marijuana relate to marijuana use among adolescents in Argentina, Chile, and Uruguay over time. Drug Alcohol Depend. 2019;201:115–26. https://doi.org/10.1016/j.drugalcdep.2019.03.029 .
Traube DE, Yarnell LM, Schrager SM. Differences in polysubstance use among youth in the child welfare system: toward a better understanding of the highest-risk teens. Child Abuse Negl. 2016;52:146–57. https://doi.org/10.1016/j.chiabu.2015.11.020 .
Wilson JD, Vo H, Matson P, Adger H, Barnett G, Fishman M. Trait mindfulness and progression to injection use in youth with opioid addiction. Subst Use Misuse. 2017;52(11):1486–93. https://doi.org/10.1080/10826084.2017.1289225 .
Dash GF, Feldstein Ewing SW, Murphy C, Hudson KA, Wilson AC. Contextual risk among adolescents receiving opioid prescriptions for acute pain in pediatric ambulatory care settings. Addict Behav. 2020;104:106314. https://doi.org/10.1016/j.addbeh.2020.106314 Epub 2020 Jan 11. PMID: 31962289; PMCID: PMC7024039.
Osborne V, Serdarevic M, Striley CW, Nixon SJ, Winterstein AG, Cottler LB. Age of first use of prescription opioids and prescription opioid non-medical use among older adolescents. Substance Use Misuse. 2020;55(14):2420–7. https://doi.org/10.1080/10826084.2020.1823420 .
Zuckermann AME, Qian W, Battista K, Jiang Y, de Groh M, Leatherdale ST. Factors influencing the non-medical use of prescription opioids among youth: results from the COMPASS study. J Subst Abus. 2020;25(5):507–14. https://doi.org/10.1080/14659891.2020.1736669 .
De Pedro KT, Esqueda MC, Gilreath TD. School protective factors and substance use among lesbian, gay, and bisexual adolescents in California public schools. LGBT Health. 2017;4(3):210–6. https://doi.org/10.1089/lgbt.2016.0132 .
Spillane NS, Schick MR, Kirk-Provencher KT, Hill DC, Wyatt J, Jackson KM. Structured and unstructured activities and alcohol and marijuana use in middle school: the role of availability and engagement. Substance Use Misuse. 2020;55(11):1765–73. https://doi.org/10.1080/10826084.2020.1762652 .
Ogunsola OO, Fatusi AO. Risk and protective factors for adolescent substance use: a comparative study of secondary school students in rural and urban areas of Osun state, Nigeria. Int J Adolesc Med Health. 2016;29(3). https://doi.org/10.1515/ijamh-2015-0096 .
Doggett A, Qian W, Godin K, De Groh M, Leatherdale ST. Examining the association between exposure to various screen time sedentary behaviours and cannabis use among youth in the COMPASS study. SSM Population Health. 2019;9:100487. https://doi.org/10.1016/j.ssmph.2019.100487 .
Afifi RA, El Asmar K, Bteddini D, Assi M, Yassin N, Bitar S, et al. Bullying victimization and use of substances in high school: does religiosity moderate the association? J Relig Health. 2020;59(1):334–50. https://doi.org/10.1007/s10943-019-00789-8 .
El Kazdouh H, El-Ammari A, Bouftini S, El Fakir S, El Achhab Y. Adolescents, parents and teachers’ perceptions of risk and protective factors of substance use in Moroccan adolescents: a qualitative study. Substance Abuse Treat Prevent Policy. 2018;13(1):–31. https://doi.org/10.1186/s13011-018-0169-y .
Sussman S, Lisha N, Griffiths M. Prevalence of the addictions: a problem of the majority or the minority? Eval Health Prof. 2011;34(1):3–56. https://doi.org/10.1177/0163278710380124 .
Aldao A, Nolen-Hoeksema S, Schweizer S. Emotion-regulation strategies across psychopathology: a meta-analytic review. Clin Psychol Rev. 2010;30(2):217–37. https://doi.org/10.1016/j.cpr.2009.11.004 .
Ricketts T, Macaskill A. Gambling as emotion management: developing a grounded theory of problem gambling. Addict Res Theory. 2003;11(6):383–400. https://doi.org/10.1080/1606635031000062074 .
Williams AD, Grisham JR. Impulsivity, emotion regulation, and mindful attentional focus in compulsive buying. Cogn Ther Res. 2012;36(5):451–7. https://doi.org/10.1007/s10608-011-9384-9 .
National Institutes on Drug Abuse. Drugs, brains, and behavior the science of addiction national institute on drug abuse (nida). 2014. Retrieved from https://www.drugabuse.gov/sites/default/files/soa_2014.pdf
Hokm Abadi ME, Bakhti M, Nazemi M, Sedighi S, Mirzadeh Toroghi E. The relationship between personality traits and drug type among substance abuse. J Res Health. 2018;8(6):531–40.
Longman-Mills S, Haye W, Hamilton H, Brands B, Wright MGM, Cumsille F, et al. Psychological maltreatment and its relationship with substance abuse among university students in Kingston, Jamaica, vol. 24. Florianopolis: Texto Contexto Enferm; 2015. p. 63–8.
Rosenkranz SE, Muller RT, Henderson JL. The role of complex PTSD in mediating childhood maltreatment and substance abuse severity among youth seeking substance abuse treatment. Psychol Trauma Theory Res Pract Policy. 2014;6(1):25–33. https://doi.org/10.1037/a0031920 .
Krishnan-Sarin S, Morean M, Kong G, et al. E-Cigarettes and “dripping” among high-school youth. Pediatrics. 2017;139(3). https://doi.org/10.1542/peds.2016-3224 .
Adinoff B. Neurobiologic processes in drug reward and addiction. Harvard review of psychiatry. NIH Public Access. 2004;12(6):305–20. https://doi.org/10.1080/10673220490910844 .
Kandel D, Kandel E. The gateway hypothesis of substance abuse: developmental, biological and societal perspectives. Acta Paediatrica. 2014;104(2):130–7.
Dempsey RC, McAlaney J, Helmer SM, Pischke CR, Akvardar Y, Bewick BM, et al. Normative perceptions of Cannabis use among European University students: associations of perceived peer use and peer attitudes with personal use and attitudes. J Stud Alcohol Drugs. 2016;77(5):740–8.
Cioffredi L, Kamon J, Turner W. Effects of depression, anxiety and screen use on adolescent substance use. Prevent Med Rep. 2021;22:101362. https://doi.org/10.1016/j.pmedr.2021.101362 .
Luckett T, NewtonJohn T, Phillips J, et al. Risk of opioid misuse in people with cancer and pain and related clinical considerations:a qualitative study of the perspectives of Australian general practitioners. BMJ Open. 2020;10(2):e034363. https://doi.org/10.1136/bmjopen-2019-034363 .
Lipari RN. Trends in Adolescent Substance Use and Perception of Risk from Substance Use. The CBHSQ Report. Substance Abuse Mental Health Serv Admin. 2013; Retrieved from http://www.ncbi.nlm.nih.gov/pubmed/27656743 .
Muchiri BW, dos Santos MML. Family management risk and protective factors for adolescent substance use in South Africa. Substance Abuse. 2018;13(1):24. https://doi.org/10.1186/s13011-018-0163-4 .
Becoña E, Martínez Ú, Calafat A, Juan M, Fernández-Hermida JR, Secades-Villa R. Parental styles and drug use: a review. In: Drugs: Education, Prevention and Policy: Taylor & Francis; 2012. https://doi.org/10.3109/09687637.2011.631060 .
Berge J, Sundel K, Ojehagen A, Hakansson A. Role of parenting styles in adolescent substance use: results from a Swedish longitudinal cohort study. BMJ Open. 2016;6(1):e008979. https://doi.org/10.1136/bmjopen-2015-008979 .
Opara I, Lardier DT, Reid RJ, Garcia-Reid P. “It all starts with the parents”: a qualitative study on protective factors for drug-use prevention among black and Hispanic girls. Affilia J Women Soc Work. 2019;34(2):199–218. https://doi.org/10.1177/0886109918822543 .
Martínez-Loredo V, Fernández-Artamendi S, Weidberg S, Pericot I, López-Núñez C, Fernández-Hermida J, et al. Parenting styles and alcohol use among adolescents: a longitudinal study. Eur J Invest Health Psychol Educ. 2016;6(1):27–36. https://doi.org/10.1989/ejihpe.v6i1.146 .
Baharudin MN, Mohamad M, Karim F. Drug-abuse inmates maqasid shariah quality of lifw: a conceotual paper. Hum Soc Sci Rev. 2020;8(3):1285–94. https://doi.org/10.18510/hssr.2020.83131 .
Henneberger AK, Mushonga DR, Preston AM. Peer influence and adolescent substance use: a systematic review of dynamic social network research. Adolesc Res Rev. 2020;6(1):57–73. https://doi.org/10.1007/s40894-019-00130-0 Springer.
Gomes FC, de Andrade AG, Izbicki R, Almeida AM, de Oliveira LG. Religion as a protective factor against drug use among Brazilian university students: a national survey. Rev Bras Psiquiatr. 2013;35(1):29–37. https://doi.org/10.1016/j.rbp.2012.05.010 .
Kulis S, Hodge DR, Ayers SL, Brown EF, Marsiglia FF. Spirituality and religion: intertwined protective factors for substance use among urban American Indian youth. Am J Drug Alcohol Abuse. 2012;38(5):444–9. https://doi.org/10.3109/00952990.2012.670338 .
Miller L, Davies M, Greenwald S. Religiosity and substance use and abuse among adolescents in the national comorbidity survey. J Am Acad Child Adolesc Psychiatry. 2000;39(9):1190–7. https://doi.org/10.1097/00004583-200009000-00020 .
Moon SS, Rao U. Social activity, school-related activity, and anti-substance use media messages on adolescent tobacco and alcohol use. J Hum Behav Soc Environ. 2011;21(5):475–89. https://doi.org/10.1080/10911359.2011.566456 .
Simone A. Onrust, Roy Otten, Jeroen Lammers, Filip smit, school-based programmes to reduce and prevent substance use in different age groups: what works for whom? Systematic review and meta-regression analysis. Clin Psychol Rev. 2016;44:45–59. https://doi.org/10.1016/j.cpr.2015.11.002 .
Download references
Acknowledgements
The authors acknowledge The Ministry of Higher Education Malaysia and The Universiti Kebangsaan Malaysia, (UKM) for funding this study under the Long-Term Research Grant Scheme-(LGRS/1/2019/UKM-UKM/2/1). We also thank the team for their commitment and tireless efforts in ensuring that manuscript was well executed.
Financial support for this study was obtained from the Ministry of Higher Education, Malaysia through the Long-Term Research Grant Scheme-(LGRS/1/2019/UKM-UKM/2/1). The funders had no role in study design, data collection and analysis, decision to publish, or preparation of the manuscript.
Author information
Authors and affiliations.
Department of Community Health, Universiti Kebangsaan Malaysia, Cheras, 56000, Kuala Lumpur, Malaysia
Azmawati Mohammed Nawi, Mohd Rohaizat Hassan & Mohd Rizal Abdul Manaf
Centre for Research in Psychology and Human Well-Being (PSiTra), Faculty of Social Sciences and Humanities, Universiti Kebangsaan Malaysia, 43600, Bangi, Selangor, Malaysia
Rozmi Ismail, Fauziah Ibrahim & Nurul Shafini Shafurdin
Clinical Psychology and Behavioural Health Program, Faculty of Health Sciences, Universiti Kebangsaan Malaysia, Kuala Lumpur, Malaysia
Noh Amit & Norhayati Ibrahim
You can also search for this author in PubMed Google Scholar
Contributions
Manuscript concept, and drafting AMN and RI; model development, FI, NI and NA.; Editing manuscript MRH, MRAN, NSS,; Critical revision of manuscript for important intellectual content, all authors. The authors read and approved the final manuscript.
Corresponding author
Correspondence to Rozmi Ismail .
Ethics declarations
Ethics approval and consent to participate.
This study was approved by the Ethics Committee of the Secretariat of Research Ethics, Universiti Kebangsaan Malaysia, Faculty of Medicine, Cheras, Kuala Lumpur (Reference no. UKMPPI/111/8/JEP-2020.174(2). Dated 27 Mac 2020.
Consent for publication
Not applicable.
Competing interests
The authors AMN, RI, FI, MRM, MRAM, NA, NI NSS declare that they have no conflict of interest relevant to this work.
Additional information
Publisher’s note.
Springer Nature remains neutral with regard to jurisdictional claims in published maps and institutional affiliations.
Rights and permissions
Open Access This article is licensed under a Creative Commons Attribution 4.0 International License, which permits use, sharing, adaptation, distribution and reproduction in any medium or format, as long as you give appropriate credit to the original author(s) and the source, provide a link to the Creative Commons licence, and indicate if changes were made. The images or other third party material in this article are included in the article's Creative Commons licence, unless indicated otherwise in a credit line to the material. If material is not included in the article's Creative Commons licence and your intended use is not permitted by statutory regulation or exceeds the permitted use, you will need to obtain permission directly from the copyright holder. To view a copy of this licence, visit http://creativecommons.org/licenses/by/4.0/ . The Creative Commons Public Domain Dedication waiver ( http://creativecommons.org/publicdomain/zero/1.0/ ) applies to the data made available in this article, unless otherwise stated in a credit line to the data.
Reprints and permissions
About this article
Cite this article.
Nawi, A.M., Ismail, R., Ibrahim, F. et al. Risk and protective factors of drug abuse among adolescents: a systematic review. BMC Public Health 21 , 2088 (2021). https://doi.org/10.1186/s12889-021-11906-2
Download citation
Received : 10 June 2021
Accepted : 22 September 2021
Published : 13 November 2021
DOI : https://doi.org/10.1186/s12889-021-11906-2
Share this article
Anyone you share the following link with will be able to read this content:
Sorry, a shareable link is not currently available for this article.
Provided by the Springer Nature SharedIt content-sharing initiative
- Drug abuse, substance, adolescent
BMC Public Health
ISSN: 1471-2458
- Submission enquiries: [email protected]
- General enquiries: [email protected]
3 Social Determinants of Drug Use, Misuse, and Involvement
Introduction.
Why do some people use drugs, while others do not? Why do patterns of drug use for some people escalate to drug misuse? And, further, why do some individuals become involved in the cultivation, manufacture, distribution and sale of illicit drugs, despite knowing these behaviors carry with them increased risk of involvement in the criminal justice system?
While your own behaviors may contribute to drug use and misuse, the decision to use and misuse drugs is now understood to encompass complex behaviors situated within a matrix of ecological interaction occurring at multiple levels. Individuated treatment approaches centered on individual behavior via health beliefs, self-efficacy, or reasoned action (Rhodes, 2002) are ill equipped to address the generative interactivity across the biological, familial, sociocultural, economic, and physical environments (Thomas, 2007). These social-ecological factors are comprised of determinants that, as we will see throughout this module, disproportionately influence drug-related harms to particular groups of people and communities (World Health Organization, 2010).
Learning Objectives
- Develop familiarity with demographic trends of licit and illicit drug use and misuse.
- Understand the difference between risk factors and protective factors.
- Identify social determinants of health.
- Explain the social and economic impacts of the War on Drugs.
- Demonstrate comprehension of how social and community factors influence disproportionate levels of drug-related harms for specific groups of people.
Image 3.1 – Two Women Smoking Cigarettes

Learning Content
Use and misuse of illicit drugs, alcohol, and tobacco: demographic trends.
As we begin to discuss in detail the social determinants of drug use, misuse, and involvement (e.g., cultivation, manufacture, distribution and sale of illicit drugs), it is important to have a clear sense of the broad demographic patterns of use and misuse of licit and illicit drugs in America.
Mental Illness and Substance Use Disorders in the U.S.
Chart 3.1 – Chart from The National Survey on Drug Use and Health: 2019
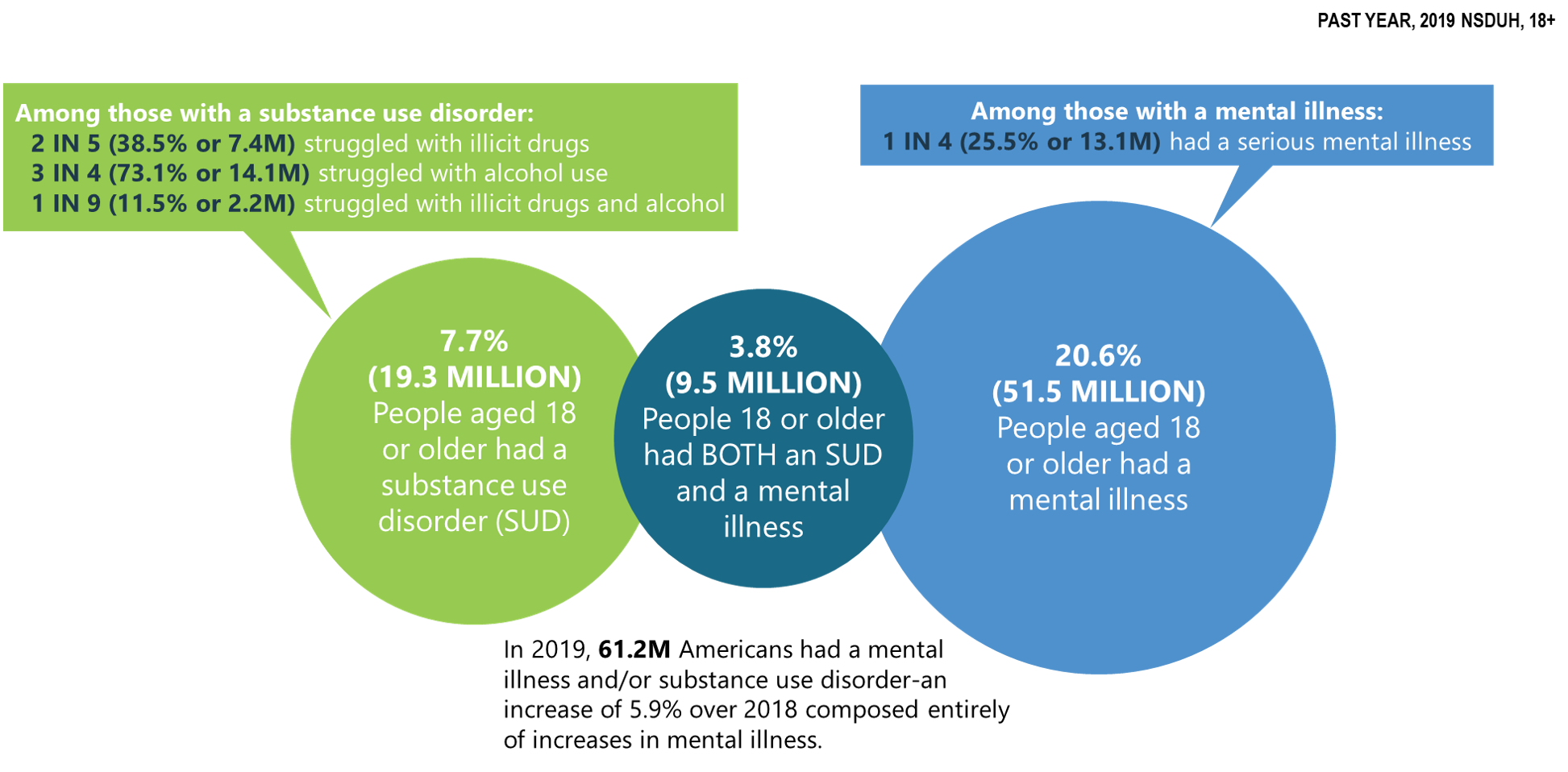
Educational attainment is not generally a reliable predictor of illicit drug use among adults aged 26 or older within the past year. This is demonstrated by the fact that adults who have graduated from college generally have lower rates of illicit drug use (17.4%) than those who have some college experience or an associate’s degree (21.2%), whereas, oddly, both of these “college experience/graduation” groups have higher rates of illicit drug use than adults whose highest level of educational attainment is high school graduate (17.2%) or less than high school (16.3%).
Notably though, with-in group rates, i.e. rates within the same group, of illicit drug use for 3 out of 4 of these educational attainment groups showed statistically significant increases from 2018 to 2019. In that timeframe, illicit drug use increased for adults who did not graduate from high school (13.8% to 16.3%), high school graduates (15.5% to 17.2%), and adults with some college or an associate’s degree (19.6% to 21.2%); whereas the change among college graduates was not statistically significant (Center for Behavioral Health Statistics and Quality, 2020). What this tells us is that although graduating from college does not appear to impact whether or not you will use illicit drugs versus a person with less education, the rates of illicit drug use among college graduates is relatively stable, whereas it seems to be increasing for those with less educational experience.
Adolescents
Chart 3.2 – Alcohol or Illicit Drug Use Among Adolescents in the Past 30 Days in 2017
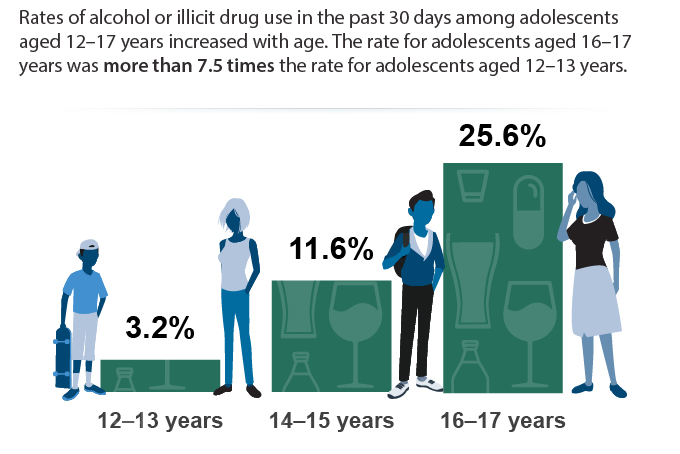
Critical Thinking
Why do you think there are such sharp increases in the rates of alcohol and illicit drug use in later adolescence?
College-Aged Adults
Findings from a 2019 national survey on drug use among college-age adults administered by the Institute on Social Research revealed:
- Cigarette smoking continued a downward trend, with 7.9% of college students reporting having smoked in the past month. Among their peers who are not in college, 16% reported having smoked in the past month, an all-time low.
- Binge drinking (five or more drinks in a row in the past two weeks), which has been declining gradually over the past few decades, showed no significant changes for young adults attending or not attending college. In 2019, 33% of college students and 22% of same-age adults not in college reported binge drinking. High-intensity drinking (10 or more drinks in a row in the past two weeks) has stayed level at about 11% since 2015 for people between the ages of 19 and 22, regardless of college attendance.
- Prescription opioid misuse continued to decline, with 1.5% of college students and 3.3% of those not attending college reporting non-medical use of opioids (narcotic drugs other than heroin) in the past year. This represents a significant five-year decline from rates of 4.8% and 7.7%, respectively, in 2014.
- Amphetamine use continued to decline, with 8.1% of college students and 5.9% of non-college respondents reporting non-medical use of amphetamines in the past year.
- While cigarette use is down, vaping both nicotine and marijuana is increasing among college aged students regardless of whether they are enrolled in college.
Overall, for college-aged adults many drug and alcohol use and misuse categories continue to show downward trends (e.g. cigarette smoking, prescription opioid misuse, amphetamine use), while others remain unchanged (e.g. binge drinking) or are increasing (e.g. vaping both nicotine and marijuana).
Further, there are no clear relationship patterns regarding whether or not college attendance for college-aged adults impacted drug and alcohol use and misuse. In some cases, such as cigarette smoking and prescription opioid misuse, college-aged adults not enrolled in college reported rates of use 2 times higher (16% smoking; 3.3% opioid misuse) than their college-enrolled counterparts (7.9% smoking; 1.5% opioid misuse). In the opposite direction, rates of binge drinking and amphetamine use were higher for college-aged adults enrolled in college (33% binge drinking; 8.1% amphetamine use) when compared with those not enrolled in college (22% binge drinking; 5.9% amphetamine use). (Schulenberg, J. E. et al., 2020).
Employment status affects current illicit drug use, with unemployed adults reporting the highest rates of use.
Among adults aged 26 and older, the rates of illicit drug use within the past year for those who were unemployed (30.3%) were 47.1% higher than those working full time (20.6%), 62% higher than those with part-time (18.7%) employment, and more than twice the rates of those whose employment status was “other” (13.9%), which includes students, persons keeping house or caring for children full time, retired or disabled persons, or other persons not in the labor force.
These data show important findings that those who are unemployed use illicit drugs at much higher rates than all other levels of employment. The severity of the negative impacts of unemployment on illicit drug use, however, becomes much clearer when examining the role of unemployment as a factor for an illicit substance use disorder (substance use disorder involving an illicit drug). When compared with all other levels of employment status, adults 26 or older who are currently unemployed report rates of illicit substance use disorder (9.1%) at more than four times the rate of all other employment levels: full-time (2.1%), part-time (2.1%), or “other” (1.9%) (Center for Behavioral Health Statistics and Quality, 2020).
Generally, unemployment tends to increase the likelihood of illicit drug use and misuse.
As we examine the rates of illicit drug use stratified by poverty, it is important to first understand the poverty thresholds for a specific household size within a particular year, as the poverty threshold increases or decreases depending upon household size and can change year to year with inflation.
The poverty threshold for a household of 4 in 2019 was equal to a total annual household income of $25,750.00. Poverty threshold levels for statistical indices in 2019 were:
- less than 100% if the total annual household income for a household of 4 is below $25,750,
- 100-199% if the total annual household income for a household of 4 is between $25,750.00 and $51,242.50, and
- 200% or more if the total annual household income for a household of 4 is more than $51,500.
Rates of illicit drug use and illicit drug use disorder tracked against poverty thresholds for adults aged 18 or over show a gradient pattern of use and dependence with the highest rates associated with the poorest adults, whereafter the rates decline as poverty decreases (or total annual household income increases). Adults 18 or over living in households at less than 100% of the poverty threshold report rates of illicit drug use in the past year (27.2%) at levels nearly 20% higher than those at 100-199% of the poverty threshold (22.9%), and 40.2% higher than their most affluent peers (2.4% in the 200% or more category).
As observed when fielding patterns of illicit drug use and dependence related to unemployment, increased drug use can lead to increased dependence. This is also the case regarding poverty where the poverty level (less than 100%) reporting the highest use also reported the highest rates of illicit drug-use disorder. In other words, just as drug use and misuse was the highest for those who were unemployed, similarly, it is the highest for those who suffer from poverty. Among adults aged 18 or over, persons living in households at less than 100% of the poverty threshold reported illicit substance use disorder (5.3%) at rates 60.6% more than those at 100-199% of the poverty threshold (3.3%), and more than twice their more affluent peers at 200% or more of the poverty threshold (2.4%). (U.S. Department of Health and Human Services, 2019; Center for Behavioral Health Statistics and Quality; 2020).
Criminal Justice System Involvement
Criminal justice system involvement is also strongly correlated with use and misuse of illicit drugs and alcohol. Adults aged 18 or older who were placed on parole or other supervised release from prison in the past year report using illicit drugs in the past month at more than 2.5 times the rate of individuals who are not on parole or supervised release from prison (30.8% versus 13.3%, respectively). When considering the use of more severe illicit drugs (excluding marijuana from the illicit drug category), this difference (16.7% parole/supervised release) increases to nearly fivefold that of individuals not on parole or supervised release from prison (3.4%). Following these trends, rates of heavy alcohol use for those who are on parole or other supervised release from prison in the past year (12.6%) are twice that of adults who are not on parole or supervised release from prison (6.3%). (Center for Behavioral Health Statistics and Quality, 2020)
Take a moment to consider the important takeaways from these demographic trends; then, respond thoughtfully to the following questions:
What demographic categories are associated with the highest rates of use and misuse? Are there any discernible patterns in these data? What findings surprised you?
Risk and Protective Factors For Drug Use and Misuse
Image 3.2 – Graffiti Art: Risk?

The below information, until Table 3.1, is verbatim derived from Substance Abuse and Mental Health Services Administration, Risk and protective factors . [Fact Sheet] (2019).
Assessing the risk and protective factors that contribute to substance use disorders helps practitioners select appropriate interventions.
Many factors influence a person’s chance of developing a mental and/or substance use disorder. Effective prevention focuses on reducing those risk factors, and strengthening protective factors that are most closely related to the problem being addressed.
Risk factors are characteristics at the biological, psychological, family, community, or cultural level that precede and are associated with a higher likelihood of negative outcomes.
Protective factors are characteristics associated with a lower likelihood of negative outcomes or that reduce a risk factor’s impact. Protective factors may be seen as positive countering events.
Some risk and protective factors are fixed: they don’t change over time. Other risk and protective factors are considered variable and can change over time. Variable risk factors include income level, peer group, adverse childhood experiences (ACEs), and employment status.
Individual-level risk factors may include a person’s genetic predisposition to addiction or exposure to alcohol prenatally.
Individual-level protective factors might include positive self-image, self-control, or social competence.
Image 3.3 – Bulletin Board with Terms Related to Drug Misuse

Key Features of Risk and Protective Factors
Prevention professionals should consider these key features of risk and protective factors when designing and evaluating prevention interventions. Then, prioritize the risk and protective factors that most impact the community. Additionally, prevention professionals should recognize that risk and protective factors exist in multiple contexts.
All people have biological and psychological characteristics that make them vulnerable to, or resilient in the face of, potential behavioral health issues. Because people have relationships within their communities and larger society, each person’s biological and psychological characteristics exist in multiple contexts. A variety of risk and protective factors operate within each of these contexts. These factors also influence one another.
Targeting only one context when addressing a person’s risk or protective factors is unlikely to be successful, because people don’t exist in isolation. For example:
- In relationships , risk factors include parents who use drugs and alcohol or who suffer from mental illness; child abuse and maltreatment; and inadequate supervision. In this context, parental involvement is an example of a protective factor.
- In communities , risk factors include neighborhood poverty and violence. Here, protective factors could include the availability of faith-based resources and after-school activities.
- In society , risk factors can include norms and laws favorable to substance use, as well as racism and a lack of economic opportunity. Protective factors in this context would include hate crime laws or policies limiting the availability of alcohol.
Chart 3.3 – Risk and Protective Factors
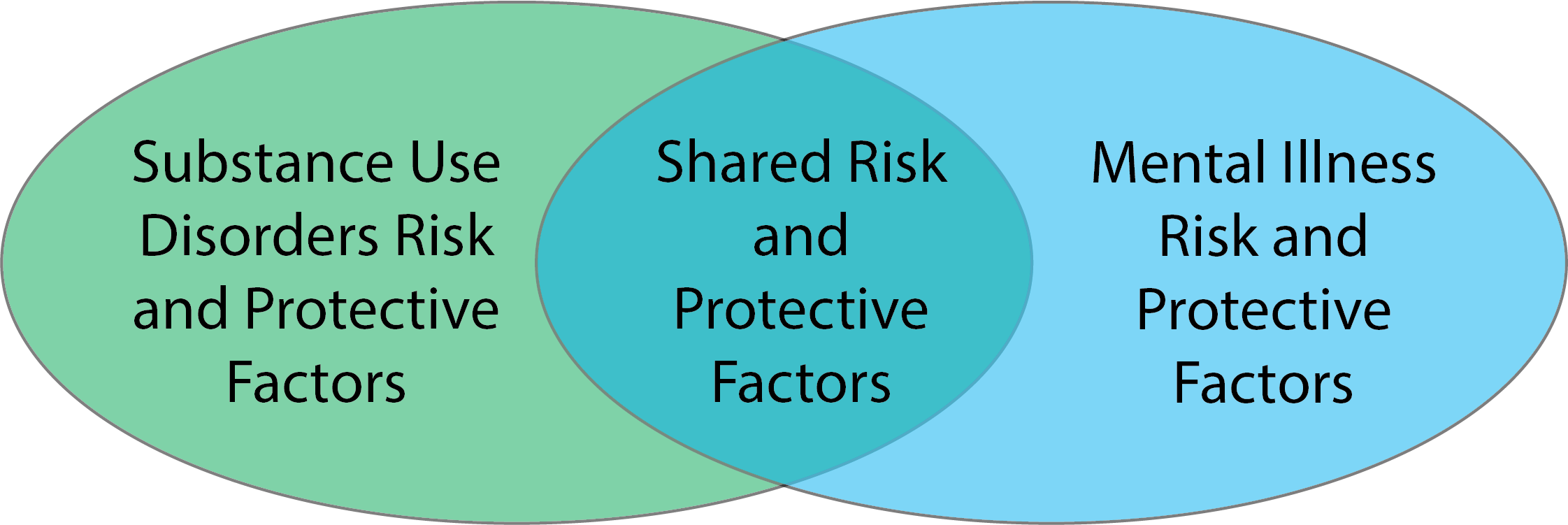
Prevention professionals should recognize that risk and protective factors are correlated and cumulative. Risk factors tend to be positively correlated with one another and negatively correlated to protective factors. In other words, people with some risk factors have a greater chance of experiencing even more risk factors, and they are less likely to have protective factors.
Risk and protective factors also tend to have a cumulative effect on the development—or reduced development—of behavioral health issues. Young people with multiple risk factors have a greater likelihood of developing a condition that impacts their physical or mental health; young people with multiple protective factors are at a reduced risk.
These correlations underscore the importance of:
- early intervention
- interventions that target multiple, not single, factors
Prevention professionals should recognize that individual factors can be associated with multiple outcomes. Though preventive interventions are often designed to produce a single outcome, both risk and protective factors can be associated with multiple outcomes. For example, negative life events are associated with substance use as well as anxiety, depression, and other behavioral health issues. Prevention efforts targeting a set of risk or protective factors have the potential to produce positive effects in multiple areas.
Risk and protective factors can have influence throughout a person’s entire lifespan. For example, risk factors such as poverty and family dysfunction can contribute to the development of mental and/or substance use disorders later in life. Risk and protective factors within one particular context—such as the family—may also influence or be influenced by factors in another context. Effective parenting has been shown to mediate the effects of multiple risk factors, including poverty, divorce, parental bereavement, and parental mental illness. The more we understand how risk and protective factors interact, the better prepared we will be to develop appropriate interventions. (Substance Abuse and Mental Health Services Administration Risk, and Protective Factors. [factsheet] , 2019).
The table below describes how risk and protective factors affect people in five domains, or settings, where interventions can take place (National Institute on Drug Abuse, What are risk factors and protective factors?, 2020; U. S. Dept. of Health and Human Services, Preventing Drug Use Among Children and Adolescents, 2003).
Table 3.1 – Risk, Domain, and Protective Factors
Impact of Socioeconomic Status on Health & Health Behavior
Image 3.4 – Old Apartment Complex

Building upon the demographic data discussed earlier in this module, this section defines socioeconomic status (SES) and describes how the components of SES (e.g., education, income, employment) act as social determinants influencing individual and community-level health and behavior, and, more specifically, how SES intersects with racial-ethnic minority status.
Review this factsheet from the American Psychological Association to learn more about Socioeconomic Status & Ethnic and Racial Minorities (American Psychological Association, Ethnic and Racial Minorities & Socioeconomic Status. [factsheet], 2017).
What are the social determinants of health? Health is influenced by many factors, which may generally be organized into five broad categories known as determinants of health: genetics, behavior, environmental and physical influences, medical care and social factors. These five categories are interconnected.
The fifth category (social factors as a determinant of health) encompasses economic and social conditions that influence the health of people and communities (Commission on Social Determinants of Health, 2008). These conditions are shaped by socioeconomic position, which is the amount of money, power, and resources that people have, all of which are influenced by socioeconomic and political factors (e.g., policies, culture, and societal values) (Commission on Social Determinants of Health, 2007). An individual’s socioeconomic position can be shaped by various factors such as their education, occupation, or income. All of these factors (social determinants) impact the health and well-being of people and the communities they interact with.
Several factors related to health outcomes are listed below:
- early childhood development
- quality of education
- ability to get and hold a job
- type of work a person is engaged in
- food security
- access to health services
- living conditions such as housing status, public safety, clean water and pollution
- individual and household income
- social norms and attitudes (discrimination, racism and distrust of government)
- residential segregation (physical separation of races/ethnicities into different neighborhoods)
- social support
- language and literacy
- incarceration
- culture (general customs and beliefs of a particular group of people)
- access to mass media and emerging technologies (cell phones, internet, and social media)
All of these factors are influenced by social circumstances. Of course, many of the factors in this list are also influenced by the other four determinants of health.
Addressing social determinants of health is a primary approach to achieving health equity. Health equity can be defined in several ways. One commonly used definition of health equity is an environment in which all people have “the opportunity to ‘attain their full health potential’ and no one is ‘disadvantaged from achieving this potential because of their social position or other socially determined circumstance’” (Braveman, 2003). Health equity has also been defined as “the absence of systematic disparities in health between and within social groups that have different levels of underlying social advantages or disadvantages—that is, different positions in a social hierarchy” (Braveman & Gruskin, 2003). Achieving health equity requires valuing everyone equally with focused and ongoing societal efforts to address avoidable inequalities, historical and contemporary injustices, and the elimination of health and healthcare disparities.
Social determinants of health such as poverty, unequal access to health care, lack of education, neighborhood disadvantage, stigma, and racism contribute to broad structural health inequities that shape risky behavior patterns including increased prevalence of drug use and dependence as well as participation in the cultivation, manufacture, distribution and sale of illicit drugs.
In addition to clinical approaches and interventions aimed at modifying behavior, where appropriate, a growing body of research highlights the importance of upstream factors that influence health and the need for policy interventions to address those factors (Gnadinger, 2014). Health organizations, research institutes, governmental agencies, and education programs are encouraged to look beyond behavioral factors and address underlying systemic factors related to social determinants of health (Centers for Disease Control, Adapted from: What is health equity?, 2019).
Check Your Knowledge
The war on drugs.
Image 3.5 – Man in Jail Cell

True Stories: Read an excerpt from Michelle Alexander’s New York Times best-selling book, The New Jim Crow: Mass Incarceration in the Age of Colorblindness , as she shares the story of Erma Faye Stewart, a mother of two children, who was caught up in a drug sweep in Hearne, Texas.
Imagine you are Erma Faye Stewart, a thirty-year-old, single African American mother of two who was arrested as part of a drug sweep in Hearne, Texas (Frontline, 2004; Davis, 2007). All but one of the people arrested were African American. You are innocent. After a week in jail, you have no one to care for your two small children and are eager to get home. Your court-appointed attorney urges you to plead guilty to a drug distribution charge, saying the prosecutor has offered probation. You refuse, steadfastly proclaiming your innocence. Finally, after almost a month in jail, you decide to plead guilty so you can return home to your children. Unwilling to risk a trial and years of imprisonment, you are sentenced to 10 years’ probation and ordered to pay $1,000 in fines, as well as court and probation costs. You are also now branded a drug felon. You are no longer eligible for food stamps; you may be discriminated against in employment; you cannot vote for at least 12 years, and you are about to be evicted from public housing. Once homeless, your children will be taken from you and put in foster care.
A judge eventually dismisses all cases against the defendants who did not plead guilty. At trial, the judge finds that the entire sweep was based on the testimony of a single informant who lied to the prosecution. You, however, are still branded a drug felon, homeless, and desperate to regain custody of your children. Now place yourself in the shoes of Clifford Runoalds, another African American victim of the Hearne drug bust (American Civil Liberties Union, 2002). You returned home to Bryan, Texas, to attend the funeral of your 18-month-old daughter. Before the funeral services begin, the police show up and handcuff you. You beg the officers to let you take one last look at your daughter before she is buried. The police refuse. You are told by prosecutors that you are needed to testify against one of the defendants in a recent drug bust. You deny witnessing any drug transaction; you don’t know what they are talking about. Because of your refusal to cooperate, you are indicted on felony charges. After a month of being held in jail, the charges against you are dropped. You are technically free, but as a result of your arrest and period of incarceration, you lose your job, your apartment, your furniture, and your car. Not to mention the chance to say good-bye to your baby girl.
This is the War on Drugs. The brutal stories described above are not isolated incidents, nor are the racial identities of Erma Faye Stewart and Clifford Runoalds random or accidental. In every state across our nation, African Americans—particularly in the poorest neighborhoods—are subjected to tactics and practices that would result in public outrage and scandal if committed in middle-class white neighborhoods. In the drug war, the enemy is racially defined. The law enforcement methods described [earlier] have been employed almost exclusively in poor communities of color, resulting in jaw-dropping numbers of African Americans and Latinos filling our nation’s prisons and jails every year. We are told by drug warriors that the enemy in this war is a thing—drugs—not a group of people, but the facts prove otherwise (Alexander, M., 2010).
View the press conference on June 18, 1971 , from the East Room of the White House, where President Richard Nixon launched the “War on Drugs.”
Video 3.1 – President Nixon Declares Drug Abuse “Public Enemy Number One”
With the identification of drug misuse as “Public Enemy No. 1,” President Richard Nixon launched the War on Drugs. Though the initial policies included significant resource allocation for substance misuse prevention and treatment programs aimed at “prevention of new addicts, and the rehabilitation of those who are addicted” (The American Presidency Project: Special Message to the Congress in 1971), what followed over the next 40 years was an unprecedented wave of punitive drug policies with increasing incarceration of U.S. citizens for drug related crimes. As of 2017, the U.S. was incarcerating its citizens at 5 to 10 times the rate of other industrialized nations (The Sentencing Project, 2019).
Chart 3.4 – International Rates of Incarceration per 100,000 in 2019
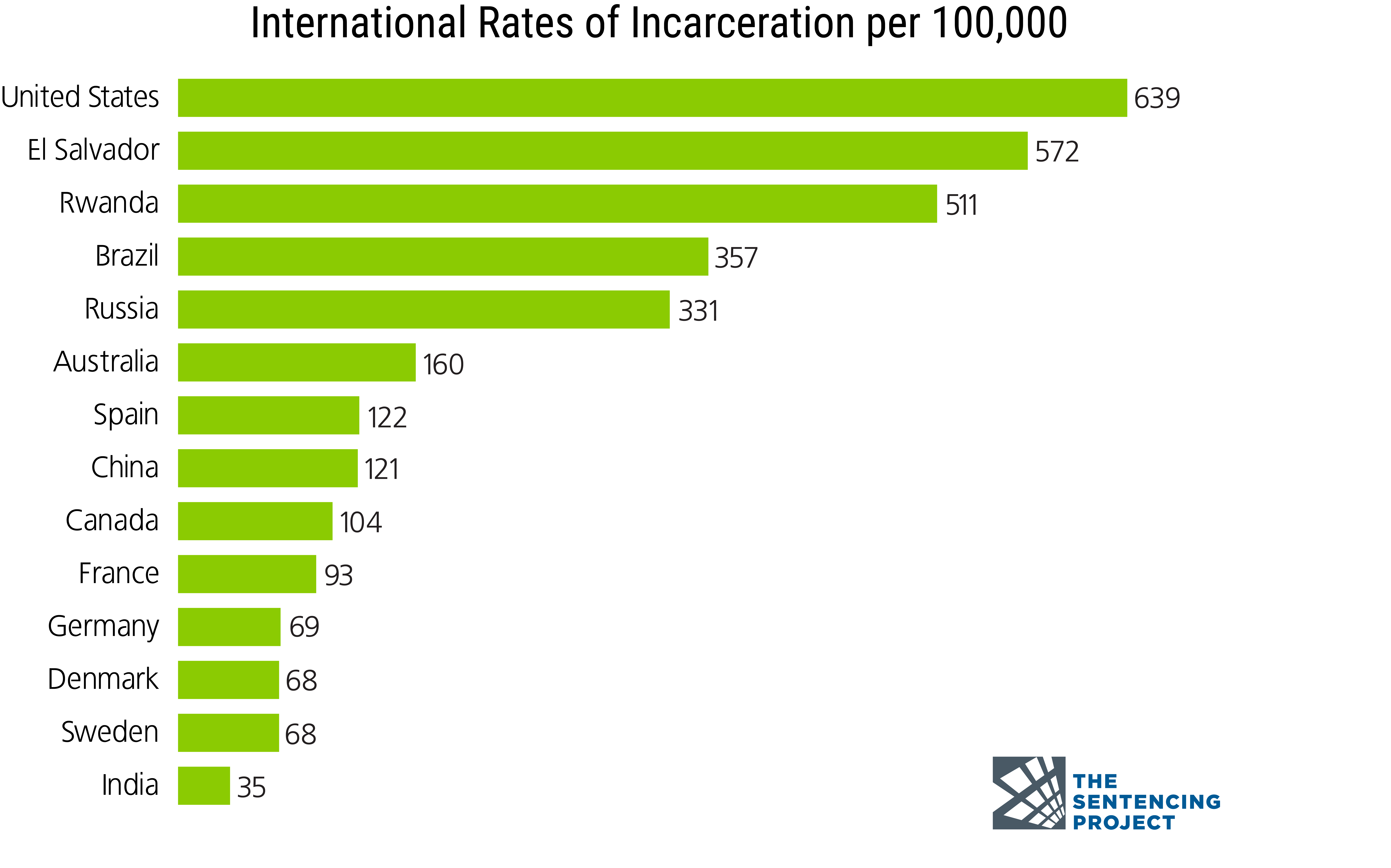
Anti-Drug Abuse Act of 1986
The above graph shows current incarceration rates across the world per 100,000 people. The U.S. incarceration rate is 639 per 100,000 people followed by El Salvador at 572 per 100,000 people. Incarceration rates in the U.S. were not always this high. Prison and jail populations across the country began to escalate sharply in the 1980s with the passage of the Anti-Drug Abuse Act of 1986 under President Ronald Reagan. Passing with strong bipartisan support, the Anti-Drug Abuse Act ushered in harsher penalties, mandatory minimum sentencing for some drug crimes (e.g. those involving crack cocaine), and increased enforcement activities, in particular for low-level drug offenses, by raising overall spending thresholds on drug enforcement by $1.7 billion (Pearl, 2018; Teasley, 2001). Along with the mandatory minimum sentences was a 100:1 sentencing disparity for crack versus powder cocaine. Note that crack was used mainly by the Black community and powder cocaine by Whites. Under this provision of the law, possession of 5 grams of crack cocaine received the same sentence as possession of 500 grams of powder cocaine. The impacts of the Anti-Drug Abuse Act of 1986 were disproportionately levied against low-income, ethnic minority communities nationwide. (Note: In 2010, 24 years after the passage of the Anti-Drug Abuse Act of 1986, Congress passed the Fair Sentencing Act during the Obama Administration reducing the crack versus powder cocaine sentencing disparity from 100:1 to 18:1.)
Violent Crime Control Act and Law Enforcement Act of 1994
The second piece of major legislation introduced during the War on Drugs was the Violent Crime Control Act and Law Enforcement Act of 1994. The 1994 Crime Bill, as it is better known, was passed during the Clinton Administration. Similar to the Anti-Drug Abuse Act of 1986, it received strong bipartisan support. From 1980 to 1992, when urban areas across the nation faced a 27% increase in violent crime rates and overall crime fell just 5% (Block & Twist, 1994), being “tough-on-crime” was viewed by many politicians as an effective strategy to both secure the economic vitality of American cities and protract their own political longevity.
- increased enforcement activities training 100,000 new police officers to patrol American streets as part of an enhanced community policing program that disproportionately centered on marginalized racial-ethnic minority communities;
- large grant mechanisms totaling $9.7 billion to fund new prison construction;
- authorizing the death penalty for 60 new federal offenses;
- making juveniles aged 13 and older eligible to be prosecuted as adults for certain serious violent crimes; and
- expanded mandatory minimum sentencing provisions (“3 Strikes and You Are Out!”) including mandatory life imprisonment without possibility of parole for federal offenders with three or more convictions for serious violent felonies or drug trafficking crimes (U. S. Department of Justice, 1994).
The ramifications of these two major policies enacted during the height of the War on Drugs (Anti-Drug Abuse Act of 1986; 1994 Crime Bill) has shaped the carceral system and low-income minority communities in the U.S. as we know them today. Largely as a result of these policies, marginalized racial-ethnic minority communities in the U.S. are more fragmented than ever before with a new prison opened every 10 days from 1990 to 2005 (Kirchhoff, 2010) and in 2015 approximate 77% of people serving time in federal prisons and about 57% of people in state prison for drug convictions are Black or Latino (Drug Policy Alliance, 2015). Further, when considering the fraying of the nuclear family, 1 in 9 Black children has an incarcerated parent, compared to 1 in 28 Latino children and 1 in 57 white children; totaling 2.7 million children growing up in U.S. households in which one or two parents are incarcerated, where two-thirds of these parents have been convicted of nonviolent offenses (Western & Pettit, 2010).
Collateral Consequence Laws & the Social Costs of a Felony Drug Conviction
In addition to serving time in prison, individuals in the U.S. convicted of felony drug offenses are subject to collateral consequence laws that limit their participation across multiple sectors of mainstream society.
Collateral consequences are defined as “legal and regulatory restrictions that limit or prohibit people convicted of crimes from accessing employment, business and occupational licensing, housing, voting, education, and other rights, benefits, and opportunities” (National Inventory of Collateral Consequences of Conviction, 2021).
Examples of collateral consequences are listed below:
- stigma of being a drug felon
- ineligibility for government sponsored supplemental nutrition assistance programs (SNAP)
- ineligibility for government sponsored student loan programs
- cannot live with individuals who receive housing assistance from government programs (even if they are family members)
- ineligibility to vote (varies by state)
- potential employers can legally discriminate in hiring processes due to felony conviction
True or False
Video 3.2 – What is the Drug War? With Jay-Z & Molly Crabapple
Watch this video from the Drug Policy Alliance titled, “What is the Drug War? With Jay-Z & Molly Crabapple.” In the video, they discuss the War on Drugs and the push to participate in the underground economy when marginalized racial-ethnic minority communities are largely excluded from the mainstream economy.
Race-Ethnicity and Illicit Drug, Alcohol, and Tobacco Use
The disproportionate rates of incarceration for Blacks and Latinos convicted of drug-related crimes in the U.S. could lead people to believe these racial-ethnic groups use drugs at significantly higher rates than other racial-ethnic groups. This, however, is not the case.
In 2017, adolescent alcohol and illicit drug use disparities were observed for a number of racial-ethnic groups.
Asian American adolescents aged 12-17 years reported the lowest rate (7.4%) of using alcohol or illicit drugs within the past 30 days among racial-ethnic groups by a significant margin. Rates for adolescents in other racial-ethnic groups from highest to lowest were:
- 16.3% among adolescents who identify with two or more races (more than twice the lowest group rate);
- 15.5% among non-Hispanic White adolescents (more than twice the lowest group rate);
- 14.4% among American Indian or Alaska Native adolescents (94.6% higher than the lowest group rate);
- 12.2% among Hispanic or Latino adolescents (64.9% higher than the lowest group rate);
- 11.9% among non-Hispanic Black adolescents (60.8% higher than the lowest group rate); and
- 11.9% among Native Hawaiian or Other Pacific Islander adolescents (60.8% higher than the lowest group rate) (Office of Disease Prevention and Health Promotion, Alcohol or Illicit Drug Use Among Adolescents , 2017; Center for Behavioral Health Statistics and Quality, 2017 National Survey on Drug Use and Health , 2018).
Review the below Chart 3.5 to see a timeline of disparities in illicit drug use each month by race-ethnic minorities aged 12 or older between 2002 and 2013. The chart demonstrates that the trend in illicit drug use is higher for Black or African Americans than White counterparts, in contrast to the data from adolescents above, and that Asian Americans still tend to be the lowest users of illicit drugs in America when considering race-ethnicity. However, note that the chart below does not account for persons of two or more races (due to a small sample size), which were the highest users of illicit drugs and alcohol in adolescents above. Also note that the chart below does not consider alcohol use, but only illicit drug use.
Chart 3.5 – Past Month Illicit Drug Use among Persons Aged 12 or Older by Race-Ethnicity in between 2002 and 2013

Moderate Drinking, Binge Drinking, and Excessive Alcohol Use
Image 3.6 – Liquor Store

As discussed in this section, moderate drinking is defined as 2 drinks or less in a day for men and 1 drink or less in a day for women, when alcohol is consumed. Drinking less is better for healthier than drinking more (Dietary Guidelines for American, 2021). Binge drinking is defined as a pattern of alcohol consumption that brings the blood alcohol concentration (BAC) level to 0.08% or more. This pattern of drinking usually corresponds to 5 or more drinks on a single occasion for men or 4 or more drinks on a single occasion for women, generally within about 2 hours (CDC, Alcohol and public health , n. d.). And excessive alcohol use is defined to include binge drinking, heavy drinking, any alcohol use by people under the age of 21 minimum legal drinking age, and any alcohol use by pregnant women (CDC, Alcohol and public health , n. d.).
Rates of alcohol use, binge drinking and excessive alcohol use within the past month among adults aged 21 and older show disparities across multiple racial-ethnic groups.
Whites (60.5%) and those who identify as two or more races (60.5%) use alcohol at rates that outpace other racial-ethnic groups. Rates of alcohol use by Hispanics or Latinos (49.1%), Blacks or African Americans (48.6%), and Native Hawaiians or Other Pacific Islanders (48.7%) are followed by Asian Americans (41.4%) and American Indians or Alaska Natives (35.3%).
Rates of binge drinking alcohol in the past month were lowest among Asian adults (14.4%) by a significant margin. Rates of binge drinking in the past month reported by other racial-ethnic groups were:
- 31.2% among adults who identify with two or more races (more than twice the lowest group rate);
- 27.9% among Hispanic or Latino adults (93.8% higher than the lowest group rate);
- 26.5% among White adults (84.0% higher than the lowest group rate);
- 25.8% among Black or African American adults (79.2% higher than the lowest group rate);
- 22.7% among American Indian or Alaska Native adults (57.6% higher than the lowest group rate); and
- 22.7% among Native Hawaiian or Other Pacific Islander adults (57.6% higher than the lowest group rate).
Rates of excessive alcohol use within the past month were also lowest among Asian American adults (2.6%) at rates significantly lower than that of other racial-ethnic groups, which ranged from 61.5% higher to over 4 times higher. Rates of excessive alcohol use within the past month for other racial-ethnic groups were:
- 11.3% among adults who identify with two or more races (more than 4 times the lowest group rate);
- 7.4% among White adults (nearly 3 times the lowest group rate);
- 5.5% among American Indian or Alaska Native adults (more than twice the lowest group rate);
- 5.1% among Hispanic or Latino adults (nearly twice the lowest group rate);
- 4.7% among Native Hawaiian or Other Pacific Islander adults (80.8% higher than the lowest group rate); and
- 4.2% among Black or African American adults (61.5% higher than the lowest group rate) (Center for Behavioral Health Statistics and Quality, 2020).
Tobacco Use
Rates of tobacco product use within the past month for persons aged 12 or older were lowest among Asian Americans (9.4%), whose rates of use were more than 3 times lower than racial-ethnic groups with the highest rates of use (31.3% American Indians or Alaska Natives; 29.0% two or more races ethnicities), and more than 2 times lower than Blacks or African Americans (23.5%), Whites (23.3%), and Native Hawaiians or Other Pacific Islanders (19.2%). At 1.5 times the lowest rates, Hispanics or Latinos reported the second lowest rates of current tobacco product use at 14.4% (Center for Behavioral Health Statistics and Quality, 2020).
Do the incarceration disparities for drug crimes among racial-ethnic minority groups match the patterns of illicit drug use across racial ethnicities? Discuss similarities and differences.
The Underground Economy: A Survival Strategy for the Urban Poor
Image 3.7 – Street Vendor Selling Found Objects

What is an underground economy? And why would individuals and communities participate in one?
Venkatesh (2006) defines underground economies as “a widespread set of activities, usually scattered and not well integrated, through which people earn money that is not reported to the government and that, in some cases, may entail criminal behavior. In other words, the unreported income can derive from licit exchange, such as selling homemade food or mowing a neighbor’s lawn, and illicit practices, such as advertising sexual favors or selling secondhand guns without a permit [or selling illicit drugs].” These underground exchanges are governed by rules, or codes, to be followed and enforced, with likely consequences of actions.
For the majority of Americans, the existence of an underground economy in the U.S. may seem far-fetched. Further, it may seem unnecessary, even treasonous. But for many people living in concentrated poverty, the underground economy is a lifeline to grab when individuals, families and entire communities are in a social and economic freefall beyond the grasp of the mainstream social structures serving middle-class America.
Watch this video and listen to Ta Nehisi-Coates read a moving passage from his book, “Between the World And Me.”
Neshisi-Coates grew up in the inner city of Baltimore, Maryland. He wrote, ”Between the World And Me,” a National Book Award Winner (2015) and New York Times bestseller, as a letter to his 15 year old son about growing up Black in America.
Video 3.3 – Ta-Nehisi Coates Reads from “Between the World and Me”
When education systems in poor communities are lacking (largely due to policies designed to fund neighborhood schools through local property tax revenue); where much of the buildings and housing stock are either in disrepair or located in areas with high crime and/or unhealthy levels of environmental pollution driving down their value (and subsequently their tax-generating capacity and their ability to produce adequate funding streams to support strong schools); when education infrastructure and poor neighborhoods are impaired in these ways, full-time and living-wage employment becomes difficult to obtain for individuals living in communities with decades of economic disinvestment. With health insurance tied to full-time employment in the U.S., many people born into and living in poverty do not have access to mainstream healthcare, relying instead upon government programs such as Medicaid and CHIP (Children Health Insurance Program), or no healthcare at all. Recent data from 2020-2021 show, over 81 million people were enrolled in either Medicaid or CHIP (Centers for Medicare and Medicaid Services, 2021) along with 30 million people who were uninsured (U. S. Department of Health and Human Services, 2021), totaling 111 million people, or over ⅓ of the U.S. population (33.5%) either on government-sponsored healthcare, or uninsured. Further, consider the impacts of home lending practices, termed “redlining,” initiated by the Federal Housing Administration in the 1930s that actively segregated housing in cities and towns across the country by refusing to insure mortgages for properties located within and around African-American neighborhoods while denying mortgages altogether for people of color in predominantly white, middle-class communities (Rothstein, 2017).
Intersect these systemic socioeconomic factors with the stark incarceration disparities for drug crimes across racial-ethnic groups and one can begin to understand why an underground economy exists.
Listen as Sudhir Venkatesh, William B. Ransford Professor of Sociology & African-American Studies at Columbia University in New York, speaks with Harvard University Press about his book, “Off The Books: The Underground Economy of the Urban Poor.”
Having lived and worked for 10 years in Chicago’s South Side, the community highlighted in this book, while completing graduate studies in sociology at University of Chicago, Off the Books is a true account of the intersectional complexities and inner workings of a modern day underground economy in an impoverished inner city neighborhood.
Audio File 3.1 – Sudhir Venkatesh, William B. Ransford Professor of Sociology & African-American Studies at Columbia University in New York, speaks with Harvard University Press about his book, “Off The Books: The Underground Economy of the Urban Poor”
Marlene Matteson was the person least likely to mourn the death of Johnnie “Big Cat” Williams, leader of the Maquis Park Kings, the local neighborhood gang. Marlene knew firsthand the destruction that gang activity could bring upon families. A mother of three, she was also a widower as a result of the gangland slaying of her own husband. “My husband died like [Big Cat] did,” she said, noting the similarities of Billy Matteson’s murder in 1992 and Big Cat’s fall on a cold, blustery morning in 2003. “Big Cat never knew what hit him. Just like my Billy. Came up on his back, shot him when he wasn’t looking. Probably didn’t feel nothing.” Both Billy Matteson and Big Cat were slain late at night, in the presence of their bodyguards, who were also injured. The killers were never found. Marlene Matteson accepted the news of Big Cat’s murder with a mixture of relief and apprehension. As the president of the 1700 South Maryland Avenue Block Club, she knew that gang activity created persistent safety problems in her area. She saw in Big Cat’s death a sign of difficult times ahead. Life was going to change, sharply and perhaps for the worse, in Maquis Park. She could no longer call on Big Cat to keep rank-and-file members out of parks in the afternoon, when kids came back from school. She could no longer wake him and demand that he put an end to the late-night carousing of younger gang members on her block. With little help from law enforcement, she wondered who would help her police the gang members who overtook public spaces with abandon and whose rhythms and inner clocks did not match those of the residents, like Marlene, who woke each morning to go to work or take children to school.
On that December 2003 morning, a week after Big Cat’s death, Marlene Matteson sat with her thoughts and with a dozen other residents of the community in the back room of the Maquis Park Prayer and Revival Center, a small storefront church on Indiana Avenue in Chicago’s historic Southside black community. She was not the only person in the room struggling to make sense of Big Cat’s death. The others in attendance were unlikely to express great sadness for a gang leader who peddled drugs and brought violence and instability to the neighborhood; but they, too, were touched by sadness, anger, and an uncertainty about what lay ahead.
“You know they’re going to be after each other now,” said Jeremiah Wilkins, a local pastor, referring to the inevitable internecine battles among local gang members to fill Big Cat’s void. “No one knows yet who’s taking [Big Cat’s] place.”
“Yeah, well, it ain’t gonna be pretty, but we been there before,” said Ola Sanders, the proprietor of Ola’s Hair Salon on 16th Street. “Someone’s going to be the leader, but it don’t matter who. We got to stay together. That’s what’s really important, okay?” “Look, whatever these brothers do, we can’t stop them,” chimed in James Arleander, a local handyman who for twenty years had been repairing cars off the books for local residents in the parking lot behind the church. “Let’s not pretend we’re sad or nothing. I mean the man was a killer! You all are acting like he’s your friend. Don’t make any sense to sit here crying. Man was a killer.” James’s voice trailed off. “I agree,” said Dr. J. T. Watkins, director of Paths Ahead, a small social service center that ran programs for Maquis Park’s youth. “Look, we got to go ahead. We all know why we’re here. I mean no offense, Pastor. We’re mourning, but we got to make our money. Do you all agree? Well, am I right or not?”
There was silence. The room was still except for Pastor Wilkins’s finger tapping the wooden table in time with the clock on the wall. For nearly five minutes, no one responded to Dr. Watkins’s question, in part because no one could justifiably dispute his contention—money was the chief reason for the group’s convening that morning. The livelihood of these community leaders was at stake. Yes, they were all concerned about the escalation in violence that was certain to result with Big Cat’s passing: other local gangs would be battling to control the Kings’ drug-trafficking territories in the neighborhood. They were all aware of the chaos that could come as a new hierarchy was chosen. But Big Cat’s death placed in sharp relief their own reliance on dangerous and illegal ways of making ends meet. They were forced to confront their own deep involvement in an outlaw economy.
Although some found it difficult to admit, everyone in the room that morning had benefited materially from Big Cat’s presence. Their motive could have been personal financial gain, political power, or a desire to do their own work more effectively, whether that be preaching or changing a tire. Big Cat not only helped Marlene to police younger gang members; he also gave money to her block club for kids’ parties, and members of his gang patrolled the neighborhood late at night because police presence was a rarity. Dr. Watkins and Pastor Wilkins would need to find a new source of philanthropy, now that Big Cat’s monthly donations to their respective organizations had ended. Big Cat’s gang ensured James Arleander a near monopoly on local off-the-books books car repair by intimidating other mechanics who tried to cut into James’s business. Ola probably would not receive $500 each weekend for letting the gang turn her salon into a thriving nightclub-the weekly “Maquis Park Dee-Jay” contest had been one of Big Cat’s favorite social activities. And others in the room that morning were no different: some received money from Big Cat, others benefited from the customers he sent their way-for everything from homemade meals to fake social security cards-and and still others were hoping to use Big Cat’s influence over two thousand young men and women to win electoral office or organize downtown protests. All of them allowed Big Cat’s gang to operate fluidly, whether that meant tolerating drug selling, presiding over funerals of deceased gang members, participating in citywide gang basketball tournaments, or “cleaning” the gang’s profits through their own businesses…
… Big Cat’s prominent position was the visible tip of the iceberg that is Maquis Park’s shadow economy. He represented a very small part of the innumerable financial exchanges that are not reported ported to the government. From off-the-books day care and domestic work to pimping and prostitution, unreported earnings wove together the social fabric in Maquis Park and surrounding poor neighborhoods. Big Cat was just one of many traders, brokers, hustlers, hawkers, and, of course, customers, who moved about in the streets, homes, and alleyways selling inexpensive labor and goods-or searching for them. He was certainly one of its most famous-and infamous-but he was just one of many who performed functions most Americans associate with the mainstream economy and the government agencies that regulate legitimate exchange. He was only one of many local stakeholders holders who resolved economic disputes because the state had no formal authority. Many other local people enforced contracts, or resolved disputes, or, for a fee, could find you a gun, a social security card, or even a job as a day laborer or a nanny for a wealthy family. Others may have claimed control over parks, alleyways, and street corners; these people would have to be paid if one wanted to fix a car, sell drugs, or panhandle at that spot. And there were many local loan sharks, besides Big Cat, who could loan you cash, or who could find you customers-for stolen stereos or drugs, for prostitutes or home-cooked lunches-in a matter of a few hours (Venkatesh, 2006).
Critical Thinking: In Two Parts
I invite you to take a deep breath before you begin this activity. If you can, be still for a moment and recall this true story you have just read. As best you can, bring to mind as vividly as you can the people in the story: what they may have been wearing, what their voices sound like, what was said, what each person may have been feeling. . . . Now, imagine you live in that-inner city neighborhood. Imagine that meeting is happening right now and you are one of the community members sitting in that room.
Part I : Select one of the people in this story, and describe what the experience might be like for that person (identify the individual in your response). You might consider any of the following: What is that person thinking right now? What emotions is that person feeling? Is that person feeling tight and tense in her/his body? Or loose and relaxed? If that person needs assistance of some kind, where might that person go for help? How will that person make up for the future financial losses resulting from the gang leader’s death? What are the risks? What will happen next? What will happen to their loved ones?
Part II : Continue to focus on what the experience might be like for the person you selected for Part I of this activity. Identify and discuss some of the social-ecological community level factors and determinants that may have influenced that person to participate in an “off the books” financial relationship with a known drug dealer and murderer. Also, describe how that person may feel about Johnnie “Big Cat” Williams, the deceased leader of the Maquis Park Kings street gang.
Other Strategies for Responding to Drug Use and Misuse
Ending the “War on Drugs”
There are three videos below. The first two criticize current or past approaches to drug use and misuse strategies. As you watch the videos, consider whether you agree with the main point the speaker is making. Has the “War on Drugs” been a helpful strategy to stop drug use and misuse? Do you think punishing those addicted to drugs is the correct systemic response to drug misuse? Is there another approach that would be more beneficial to those suffering from drug addiction?
Watch a brief TED Talk from drug policy reformist Ethan Nadelmann titled, “Why we need to end the War on Drugs:”
Video 3.4 – Why We Need to End the War on Drugs
Watch this brief TED Talk titled, “Everything You Think You Know About Addiction is Wrong”, to hear Johann Hari introduce a different perspective in understanding drug addiction:
Video 3.5 – Everything You Think You Know about Addiction is Wrong
Decriminalization: The Unique Story of Portugal
In 2001, Portugal was suffering from record-breaking overdose deaths tied to illicit drug use and addiction.
Portugal is the only country that has decriminalized all drugs. Instead, in Portugal, the country put healthcare and treatment first, rather than criminalization and punishment.
Watch this informational video from Drug Policy Alliance titled, “Decriminalization of Drug Use Explained–Descriminalizacao: A Portuguese Experiment With American Lessons:”
Video 3.6 – Decriminalization of Drug Use Explained–Descriminalizacao: A Portuguese Experiment with American Lessons
Has this policy in Portugal (treating the drug issue as a healthcare concern rather than a criminal one) been beneficial in that country? Should other countries, such as the United States, consider implementing this policy? How would this impact the racial-ethnic impact of the “War on Drugs?” Do you think a policy like this would be welcomed in the United States? Why or why not?
Supplemental Materials
- Democracy Now. (2015, July 22). “Between the World And Me:” Ta-Nehisi Coates Extended Interview on Being Black in America [Video]. https://www.democracynow.org/2015/7/22/between_the_world_and_me_ta
- Families Against Mandatory Minimums [FAMM Video]. Repeal, don’t celebrate, the Anti-Drug Abuse Act of 1986 [Video]. YouTube. https://youtu.be/BhAVJFuPJRc
- Mauer, M., & King, R. S. (2007). A 25-year quagmire: The war on drugs and its impact on American society . The Sentencing Project. https://www.sentencingproject.org/publications/a-25-year-quagmire-the-war-on-drugs-and-its-impact-on-american-society/
- Moyers, B. [Moyers & Company]. (2013, December 20). Michelle Alexander: Locked Out of the American Dream . [Video]. YouTube. https://youtu.be/om2hx6Xm2JE
- TEDx Talks. (2013, October 16). The future of race in America. Michelle Alexander at TEDxColumbus . [Video.] YouTube. https://youtu.be/SQ6H-Mz6hgw
- Tylek, B. (2021, May 24). The multibillion-dollar US prison industry–and how to dismantle it [Video]. TED. https://www.ted.com/talks/bianca_tylek_the_multibillion_dollar_us_prison_industry_and_how_to_dismantle_it?language=en
Interactive Online Applications
- University of Richmond’s Digital Scholarship Lab. (n.d.). Mapping Inequality [Interactive map]. https://dsl.richmond.edu/panorama/redlining/
Alexander, M. (2010). The new Jim Crow: Mass incarceration in the age of colorblindness . The New Press.
- Venkatesh, S. A. (2008). Gang leader for a day: A rogue sociologist takes to the streets . Penguin.
- Venkatesh, S. A. (2006). Off the books: The underground economy of the urban poor . Harvard University Press.
anarchosyn. (2007, May 27). RISK AWR WC T7L LosAngeles Graffiti Art [Digital image]. Flickr. https://www.flickr.com/photos/24293932@N00/530211480
American Civil Liberties Union. (n.d.). Stories of ACLU clients swept up in the Hearne drug bust of November 2000 . www.aclu.org/DrugPolicy/DrugPolicy.cfm?ID=11160&c=80
American Psychological Association. (2017, July). Ethnic and racial minorities & socioeconomic status [Factsheet]. https://www.apa.org/pi/ses/resources/publications/factsheet-erm.pdf
Block, M. & Twist, S. (1994). The Report Card on Crime and Punishment . ALEC Foundation. https://www.ojp.gov/ncjrs/virtual-library-abstracts-report-card-crime-and-punishment
Braveman, P.A. (2003). Monitoring equity in health and healthcare: A conceptual framework. Journal of Health, Population, and Nutrition , 21(3) : 181-92. https://pubmed.ncbi.nlm.nih.gov/14717564/
Braveman, P. & Gruskin, S. (2003). Defining equity in health. Journal of Epidemiology and Community Health , 57(4) : 254-258. https://dx.doi.org/10.1136/jech.57.4.254
Carson, E. A. (2018). Prisoners in 2016 . U.S. Department of Justice, Bureau of Justice Statistics. https://www.bjs.gov/content/pub/pdf/p16.pdf
Center for Behavioral Health Statistics and Quality. (2020). Results from the 2019. National Survey on Drug Use and Health: Detailed tables . Substance Abuse and Mental Health Services Administration. https://www.samhsa.gov/data/report/2019-nsduh-detailed-tables
Centers for Medicare and Medicaid Services. (2021 March). Medicaid & CHIP Enrollment Data Highlights . https://www.medicaid.gov/medicaid/program-information/medicaid-and-chip-enrollment-data/report-highlights/index.html
Commission on Social Determinants of Health. (2008). C losing the gap in a generation: Health equity through action on the social determinants of health. Final report of the Commission on Social Determinants of Health . World Health Organization: Geneva. https://www.who.int/publications/i/item/WHO-IER-CSDH-08.1
David, S. L., Rao, S. A., & Robertson, E. B. (2003). Preventing drug use among children and adolescents: A research-based guide for parents, educators, and community leaders, in brief (2d ed.) (NIH Publication No. 04-4212(A)). U.S. Department of Health and Human Services, National Institutes of Health, National Institute on Drug Abuse. https://nida.nih.gov/sites/default/files/preventingdruguse_2.pdf
Davis, A. (2007). Arbitrary justice: The power of the American prosecutor . Oxford University Press. https://doi.org/10.1093/acprof:oso/9780195384734.001.0001
Drug Policy Alliance. (2015, June). The Drug War, Mass Incarceration, and Race. https://www.unodc.org/documents/ungass2016/Contributions/Civil/DrugPolicyAlliance/DPA_Fact_Sheet_Drug_War_Mass_Incarceration_and_Race_June2015.pdf
Drug Policy Alliance. (2016, September 15). What is the drug war? With Jay-Z & Molly Crabapple [Video]. YouTube. https://youtu.be/HSozqaVcOU8
Drug Policy Alliance. (2019, February 20). How Portugal solved their drug crisis [Video]. YouTube. https://youtu.be/iKe9R_eiIYA
Finegold K., Conmy A., Chu R. C., Bosworth A., & Sommers, B. D. (2021). Issue brief: trends in the U.S. uninsured population, 2010-2020 (Issue Brief No. HP-2021-02). Office of the Assistant Secretary for Planning and Evaluation, U.S. Department of Health and Human Services. https://aspe.hhs.gov/sites/default/files/private/pdf/265041/trends-in-the-us-uninsured.pdf
Frontline. (2004, June 17). Four Stories–Erma Faye Stewart and Regina Kelly. The Plea . www.pbs.org/wgbh/pages/frontline/shows/plea/four/stewart.html
Gnadinger, T. (2014, August 22). Health Policy Brief: The Relative contribution of multiple determinants to health outcomes. Health Affairs Blog. https://doi.org/10.1377/forefront.20140822.040952
Hari, J. (2015, June). Everything you think you know about addiction is wrong [Video]. TED. https://www.ted.com/talks/johann_hari_everything_you_think_you_know_about_addiction_is_wrong
Irwin, A. & Solar, O. (2010). A Conceptual Framework for Action on the Social Determinants of Health . World Health Organization. https://www.who.int/sdhconference/resources/ ConceptualframeworkforactiononSDH_eng.pdf
Kirchhoff, S. M. (2010). Economic impacts of prison growth . Library of Congress Congressional Research Service. https://digital.library.unt.edu/ark:/67531/metadc501951/
Nadelmann, E. (2014, October). Why we need to end the war on drugs [Video]. TED. https://www.ted.com/talks/ethan_nadelmann_why_we_need_to_end_the_war_on_drugs
National Center for HIV, Viral Hepatitis, STD, and TB Prevention. (2019). NCHHSTP Social Determinants of Health: Frequently Asked Questions. Centers for Disease Control and Prevention. https://www.cdc.gov/nchhstp/socialdeterminants/faq.html
National Institute on Drug Abuse. (2011, June). Preventing drug use among children and adolescents (in brief) . U.S. Department of Health and Human Services, National Institutes of Health. https://nida.nih.gov/publications/preventing-drug-use-among-children-adolescents/chapter-1-risk-factors-protective-factors/what-are-risk-factors
National Inventory of Collateral Consequences of Conviction. (n.d.). What are collateral consequences? https://niccc.nationalreentryresourcecenter.org/
Nixon, R. (1971, June 17). Special Message to the Congress on Drug Abuse Prevention and Control [Transcript]. The American Presidency Project . University of California, Santa Barbara. Retrieved August 7, 2021, from https://www.presidency.ucsb.edu/node/240245
Office of Disease Prevention and Health Promotion. (n.d.). Alcohol or illicit drug use among adolescents, 2017 . https://www.healthypeople.gov/2020/leading-health-indicators/2020-lhi-topics/Substance-Abuse/data#SA-13
Pearl, B. (2018, June 27). Ending the war on drugs: By the numbers . Center for American Progress. https://www.americanprogress.org/issues/criminal-justice/reports/2018/06/27/452819/ending-war-drugs-numbers/
Rhodes, T. (2002). The ‘risk environment’: A framework for understanding and reducing drug-related harm. International Journal of Drug Policy , 13(2) , 85-94. https://doi.org/10.1016/S0955-3959(02)00007-5
Richard Nixon Foundation. (2016, April 29). President Nixon declares drug abuse “public enemy number one” [Video]. YouTube. https://youtu.be/y8TGLLQlD9M
Rothstein, R. (2017). The color of law: A forgotten history of how our government segregated America . Liveright Publishing
Shannon, R. (2019, May 10). 3 ways the 1994 crime bill continues to hurt communities of color . Center for American Progress. https://www.americanprogress.org/issues/race/news/2019/05/10/469642/3-ways-1994-crime-bill-continues-hurt-communities-color/
Schulenberg, J. E., Johnston, L. D., O’Malley, P. M., Bachman, J. G., Miech, R. A. & Patrick, M. (2020). Monitoring the Future national survey results on drug use, 1975–2019: Volume II, College students and adults ages 19–60 . Institute for Social Research, The University of Michigan. http://monitoringthefuture.org/pubs.html#monographs
Statista Research Department. (2021, September 29). Reported violent crime rate in the U.S. 1990-2019 . https://www.statista.com/statistics/191219/reported-violent-crime-rate-in-the-usa-since-1990/
Substance Abuse and Mental Health Services Administration. (2014). Results from the 2013 National Survey on Drug Use and Health: Summary of national findings . https://www.samhsa.gov/ data/sites/default/files/NSDUHresultsPDFWHTML2013/Web/NSDUHresults2013.pdf
Substance Abuse and Mental Health Services Administration. (2019). Risk and protective factors [Fact Sheet]. https://www.samhsa.gov/sites/default/files/20190718-samhsa-risk-protective-factors.pdf
Substance Abuse and Mental Health Services Administration. (2020). The national survey on drug use and health: 2019 [Data Release; PowerPoint Slides]. https://www.samhsa.gov/data/sites/default/files/reports/rpt29392/Assistant-Secretary-nsduh2019_presentation/Assistant-Secretary-nsduh2019_presentation.pdf
Teasley, D. (2001, January 24). Crime Control: The Federal Response . Congressional Research Service. https://digital.library.unt.edu/ark:/67531/metacrs1529/
The Atlantic. (2017, August 8). Ta-Nehisi Coates reads from Between the World and Me [Video]. YouTube. https://youtu.be/2Ki2fhQIoSI
The Sentencing Project. (2019). Criminal Justice Facts . https://www.sentencingproject.org/news/new-prison-jail-population-figures-released-u-s-department-justice/
Thomas, Y. F. (2007). The social epidemiology of drug abuse. American Journal of Preventive Medicine , 32(6) , S141-S146. https://doi.org/10.1016/j.amepre.2007.03.007
Office of the Assistant Secretary for Planning and Evaluation. (2019). 2019 poverty guidelines. U.S. Department of Health and Human Services . https://aspe.hhs.gov/topics/poverty-economic-mobility/poverty-guidelines/prior-hhs-poverty-guidelines-federal-register-references/2019-poverty-guidelines
U.S. Department of Justice. (1994). V iolent crime control and law enforcement act of 1994 [Fact Sheet]. National Criminal Justice Reference Service. https://www.ncjrs.gov/txtfiles/billfs.txt
Venkatesh, S. A. (2009). Off the books: The underground economy of the urban poor . Harvard University Press.
Western, B. & Pettit, B. (2010). Collateral costs: Incarceration’s effect on economic mobility . Pew Charitable Trusts. https://www.pewtrusts.org/~/media/legacy/uploadedfiles/pcs_assets/2010/collateralcosts1pdf.pdf
Drug Use and Misuse Copyright © by University of Illinois Board of Trustees is licensed under a Creative Commons Attribution-NonCommercial 4.0 International License , except where otherwise noted.
Share This Book
Numbers, Facts and Trends Shaping Your World
Read our research on:
Full Topic List
Regions & Countries
- Publications
- Our Methods
- Short Reads
- Tools & Resources
Read Our Research On:
What the data says about abortion in the U.S.
Pew Research Center has conducted many surveys about abortion over the years, providing a lens into Americans’ views on whether the procedure should be legal, among a host of other questions.
In a Center survey conducted nearly a year after the Supreme Court’s June 2022 decision that ended the constitutional right to abortion , 62% of U.S. adults said the practice should be legal in all or most cases, while 36% said it should be illegal in all or most cases. Another survey conducted a few months before the decision showed that relatively few Americans take an absolutist view on the issue .
Find answers to common questions about abortion in America, based on data from the Centers for Disease Control and Prevention (CDC) and the Guttmacher Institute, which have tracked these patterns for several decades:
How many abortions are there in the U.S. each year?
How has the number of abortions in the u.s. changed over time, what is the abortion rate among women in the u.s. how has it changed over time, what are the most common types of abortion, how many abortion providers are there in the u.s., and how has that number changed, what percentage of abortions are for women who live in a different state from the abortion provider, what are the demographics of women who have had abortions, when during pregnancy do most abortions occur, how often are there medical complications from abortion.
This compilation of data on abortion in the United States draws mainly from two sources: the Centers for Disease Control and Prevention (CDC) and the Guttmacher Institute, both of which have regularly compiled national abortion data for approximately half a century, and which collect their data in different ways.
The CDC data that is highlighted in this post comes from the agency’s “abortion surveillance” reports, which have been published annually since 1974 (and which have included data from 1969). Its figures from 1973 through 1996 include data from all 50 states, the District of Columbia and New York City – 52 “reporting areas” in all. Since 1997, the CDC’s totals have lacked data from some states (most notably California) for the years that those states did not report data to the agency. The four reporting areas that did not submit data to the CDC in 2021 – California, Maryland, New Hampshire and New Jersey – accounted for approximately 25% of all legal induced abortions in the U.S. in 2020, according to Guttmacher’s data. Most states, though, do have data in the reports, and the figures for the vast majority of them came from each state’s central health agency, while for some states, the figures came from hospitals and other medical facilities.
Discussion of CDC abortion data involving women’s state of residence, marital status, race, ethnicity, age, abortion history and the number of previous live births excludes the low share of abortions where that information was not supplied. Read the methodology for the CDC’s latest abortion surveillance report , which includes data from 2021, for more details. Previous reports can be found at stacks.cdc.gov by entering “abortion surveillance” into the search box.
For the numbers of deaths caused by induced abortions in 1963 and 1965, this analysis looks at reports by the then-U.S. Department of Health, Education and Welfare, a precursor to the Department of Health and Human Services. In computing those figures, we excluded abortions listed in the report under the categories “spontaneous or unspecified” or as “other.” (“Spontaneous abortion” is another way of referring to miscarriages.)
Guttmacher data in this post comes from national surveys of abortion providers that Guttmacher has conducted 19 times since 1973. Guttmacher compiles its figures after contacting every known provider of abortions – clinics, hospitals and physicians’ offices – in the country. It uses questionnaires and health department data, and it provides estimates for abortion providers that don’t respond to its inquiries. (In 2020, the last year for which it has released data on the number of abortions in the U.S., it used estimates for 12% of abortions.) For most of the 2000s, Guttmacher has conducted these national surveys every three years, each time getting abortion data for the prior two years. For each interim year, Guttmacher has calculated estimates based on trends from its own figures and from other data.
The latest full summary of Guttmacher data came in the institute’s report titled “Abortion Incidence and Service Availability in the United States, 2020.” It includes figures for 2020 and 2019 and estimates for 2018. The report includes a methods section.
In addition, this post uses data from StatPearls, an online health care resource, on complications from abortion.
An exact answer is hard to come by. The CDC and the Guttmacher Institute have each tried to measure this for around half a century, but they use different methods and publish different figures.
The last year for which the CDC reported a yearly national total for abortions is 2021. It found there were 625,978 abortions in the District of Columbia and the 46 states with available data that year, up from 597,355 in those states and D.C. in 2020. The corresponding figure for 2019 was 607,720.
The last year for which Guttmacher reported a yearly national total was 2020. It said there were 930,160 abortions that year in all 50 states and the District of Columbia, compared with 916,460 in 2019.
- How the CDC gets its data: It compiles figures that are voluntarily reported by states’ central health agencies, including separate figures for New York City and the District of Columbia. Its latest totals do not include figures from California, Maryland, New Hampshire or New Jersey, which did not report data to the CDC. ( Read the methodology from the latest CDC report .)
- How Guttmacher gets its data: It compiles its figures after contacting every known abortion provider – clinics, hospitals and physicians’ offices – in the country. It uses questionnaires and health department data, then provides estimates for abortion providers that don’t respond. Guttmacher’s figures are higher than the CDC’s in part because they include data (and in some instances, estimates) from all 50 states. ( Read the institute’s latest full report and methodology .)
While the Guttmacher Institute supports abortion rights, its empirical data on abortions in the U.S. has been widely cited by groups and publications across the political spectrum, including by a number of those that disagree with its positions .
These estimates from Guttmacher and the CDC are results of multiyear efforts to collect data on abortion across the U.S. Last year, Guttmacher also began publishing less precise estimates every few months , based on a much smaller sample of providers.
The figures reported by these organizations include only legal induced abortions conducted by clinics, hospitals or physicians’ offices, or those that make use of abortion pills dispensed from certified facilities such as clinics or physicians’ offices. They do not account for the use of abortion pills that were obtained outside of clinical settings .
(Back to top)
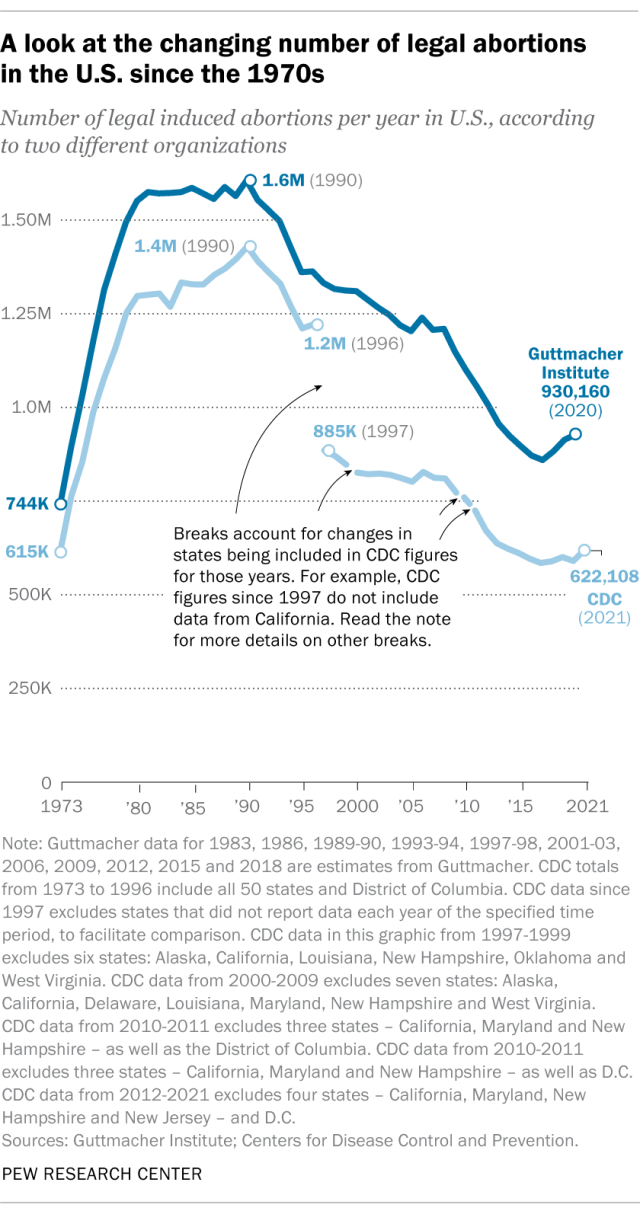
The annual number of U.S. abortions rose for years after Roe v. Wade legalized the procedure in 1973, reaching its highest levels around the late 1980s and early 1990s, according to both the CDC and Guttmacher. Since then, abortions have generally decreased at what a CDC analysis called “a slow yet steady pace.”
Guttmacher says the number of abortions occurring in the U.S. in 2020 was 40% lower than it was in 1991. According to the CDC, the number was 36% lower in 2021 than in 1991, looking just at the District of Columbia and the 46 states that reported both of those years.
(The corresponding line graph shows the long-term trend in the number of legal abortions reported by both organizations. To allow for consistent comparisons over time, the CDC figures in the chart have been adjusted to ensure that the same states are counted from one year to the next. Using that approach, the CDC figure for 2021 is 622,108 legal abortions.)
There have been occasional breaks in this long-term pattern of decline – during the middle of the first decade of the 2000s, and then again in the late 2010s. The CDC reported modest 1% and 2% increases in abortions in 2018 and 2019, and then, after a 2% decrease in 2020, a 5% increase in 2021. Guttmacher reported an 8% increase over the three-year period from 2017 to 2020.
As noted above, these figures do not include abortions that use pills obtained outside of clinical settings.
Guttmacher says that in 2020 there were 14.4 abortions in the U.S. per 1,000 women ages 15 to 44. Its data shows that the rate of abortions among women has generally been declining in the U.S. since 1981, when it reported there were 29.3 abortions per 1,000 women in that age range.
The CDC says that in 2021, there were 11.6 abortions in the U.S. per 1,000 women ages 15 to 44. (That figure excludes data from California, the District of Columbia, Maryland, New Hampshire and New Jersey.) Like Guttmacher’s data, the CDC’s figures also suggest a general decline in the abortion rate over time. In 1980, when the CDC reported on all 50 states and D.C., it said there were 25 abortions per 1,000 women ages 15 to 44.
That said, both Guttmacher and the CDC say there were slight increases in the rate of abortions during the late 2010s and early 2020s. Guttmacher says the abortion rate per 1,000 women ages 15 to 44 rose from 13.5 in 2017 to 14.4 in 2020. The CDC says it rose from 11.2 per 1,000 in 2017 to 11.4 in 2019, before falling back to 11.1 in 2020 and then rising again to 11.6 in 2021. (The CDC’s figures for those years exclude data from California, D.C., Maryland, New Hampshire and New Jersey.)
The CDC broadly divides abortions into two categories: surgical abortions and medication abortions, which involve pills. Since the Food and Drug Administration first approved abortion pills in 2000, their use has increased over time as a share of abortions nationally, according to both the CDC and Guttmacher.
The majority of abortions in the U.S. now involve pills, according to both the CDC and Guttmacher. The CDC says 56% of U.S. abortions in 2021 involved pills, up from 53% in 2020 and 44% in 2019. Its figures for 2021 include the District of Columbia and 44 states that provided this data; its figures for 2020 include D.C. and 44 states (though not all of the same states as in 2021), and its figures for 2019 include D.C. and 45 states.
Guttmacher, which measures this every three years, says 53% of U.S. abortions involved pills in 2020, up from 39% in 2017.
Two pills commonly used together for medication abortions are mifepristone, which, taken first, blocks hormones that support a pregnancy, and misoprostol, which then causes the uterus to empty. According to the FDA, medication abortions are safe until 10 weeks into pregnancy.
Surgical abortions conducted during the first trimester of pregnancy typically use a suction process, while the relatively few surgical abortions that occur during the second trimester of a pregnancy typically use a process called dilation and evacuation, according to the UCLA School of Medicine.
In 2020, there were 1,603 facilities in the U.S. that provided abortions, according to Guttmacher . This included 807 clinics, 530 hospitals and 266 physicians’ offices.
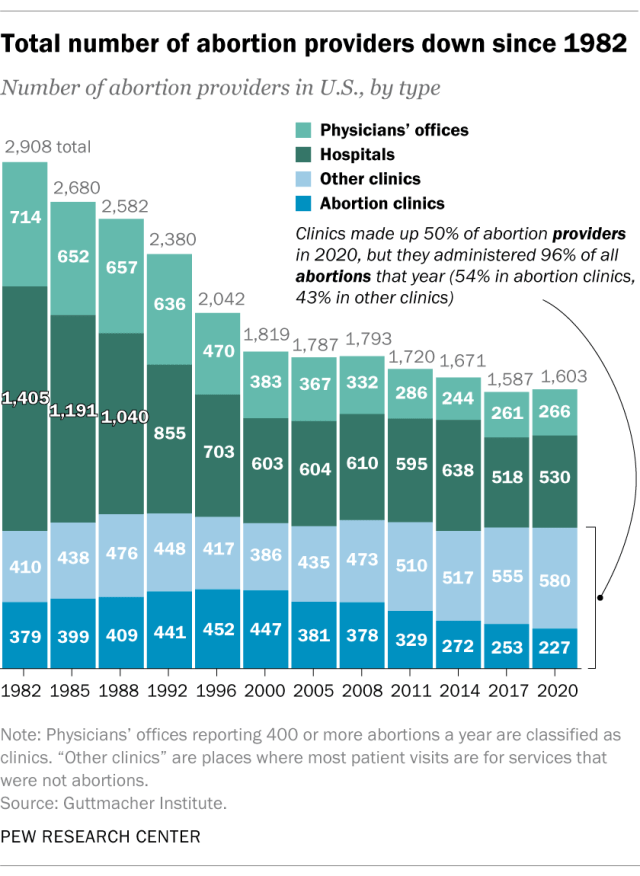
While clinics make up half of the facilities that provide abortions, they are the sites where the vast majority (96%) of abortions are administered, either through procedures or the distribution of pills, according to Guttmacher’s 2020 data. (This includes 54% of abortions that are administered at specialized abortion clinics and 43% at nonspecialized clinics.) Hospitals made up 33% of the facilities that provided abortions in 2020 but accounted for only 3% of abortions that year, while just 1% of abortions were conducted by physicians’ offices.
Looking just at clinics – that is, the total number of specialized abortion clinics and nonspecialized clinics in the U.S. – Guttmacher found the total virtually unchanged between 2017 (808 clinics) and 2020 (807 clinics). However, there were regional differences. In the Midwest, the number of clinics that provide abortions increased by 11% during those years, and in the West by 6%. The number of clinics decreased during those years by 9% in the Northeast and 3% in the South.
The total number of abortion providers has declined dramatically since the 1980s. In 1982, according to Guttmacher, there were 2,908 facilities providing abortions in the U.S., including 789 clinics, 1,405 hospitals and 714 physicians’ offices.
The CDC does not track the number of abortion providers.
In the District of Columbia and the 46 states that provided abortion and residency information to the CDC in 2021, 10.9% of all abortions were performed on women known to live outside the state where the abortion occurred – slightly higher than the percentage in 2020 (9.7%). That year, D.C. and 46 states (though not the same ones as in 2021) reported abortion and residency data. (The total number of abortions used in these calculations included figures for women with both known and unknown residential status.)
The share of reported abortions performed on women outside their state of residence was much higher before the 1973 Roe decision that stopped states from banning abortion. In 1972, 41% of all abortions in D.C. and the 20 states that provided this information to the CDC that year were performed on women outside their state of residence. In 1973, the corresponding figure was 21% in the District of Columbia and the 41 states that provided this information, and in 1974 it was 11% in D.C. and the 43 states that provided data.
In the District of Columbia and the 46 states that reported age data to the CDC in 2021, the majority of women who had abortions (57%) were in their 20s, while about three-in-ten (31%) were in their 30s. Teens ages 13 to 19 accounted for 8% of those who had abortions, while women ages 40 to 44 accounted for about 4%.
The vast majority of women who had abortions in 2021 were unmarried (87%), while married women accounted for 13%, according to the CDC , which had data on this from 37 states.
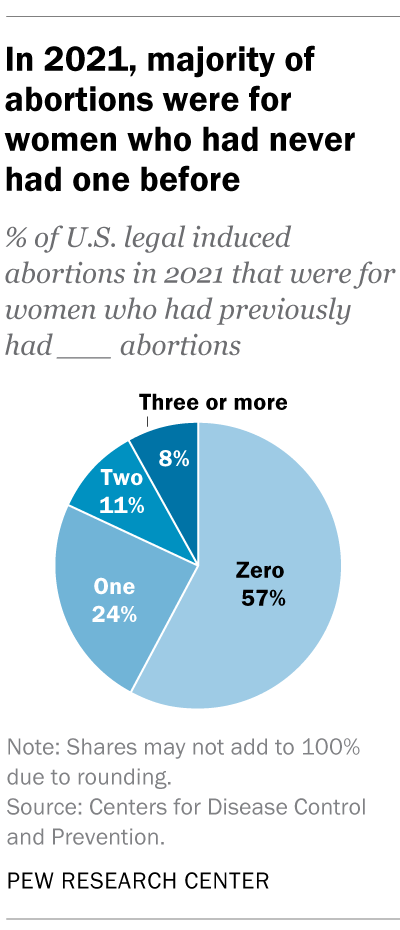
In the District of Columbia, New York City (but not the rest of New York) and the 31 states that reported racial and ethnic data on abortion to the CDC , 42% of all women who had abortions in 2021 were non-Hispanic Black, while 30% were non-Hispanic White, 22% were Hispanic and 6% were of other races.
Looking at abortion rates among those ages 15 to 44, there were 28.6 abortions per 1,000 non-Hispanic Black women in 2021; 12.3 abortions per 1,000 Hispanic women; 6.4 abortions per 1,000 non-Hispanic White women; and 9.2 abortions per 1,000 women of other races, the CDC reported from those same 31 states, D.C. and New York City.
For 57% of U.S. women who had induced abortions in 2021, it was the first time they had ever had one, according to the CDC. For nearly a quarter (24%), it was their second abortion. For 11% of women who had an abortion that year, it was their third, and for 8% it was their fourth or more. These CDC figures include data from 41 states and New York City, but not the rest of New York.
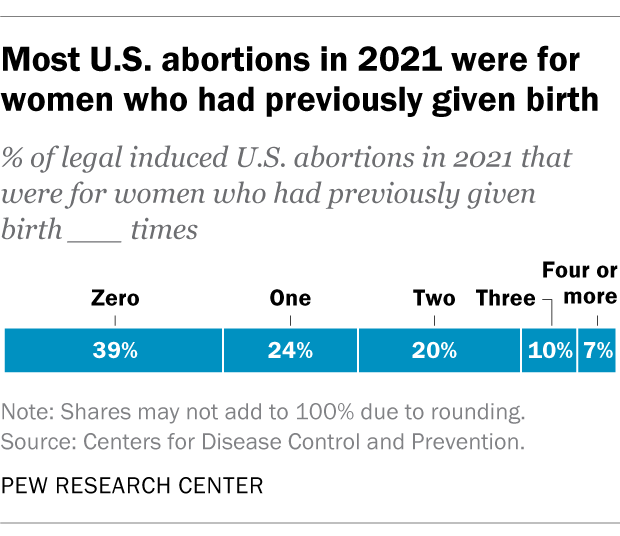
Nearly four-in-ten women who had abortions in 2021 (39%) had no previous live births at the time they had an abortion, according to the CDC . Almost a quarter (24%) of women who had abortions in 2021 had one previous live birth, 20% had two previous live births, 10% had three, and 7% had four or more previous live births. These CDC figures include data from 41 states and New York City, but not the rest of New York.
The vast majority of abortions occur during the first trimester of a pregnancy. In 2021, 93% of abortions occurred during the first trimester – that is, at or before 13 weeks of gestation, according to the CDC . An additional 6% occurred between 14 and 20 weeks of pregnancy, and about 1% were performed at 21 weeks or more of gestation. These CDC figures include data from 40 states and New York City, but not the rest of New York.
About 2% of all abortions in the U.S. involve some type of complication for the woman , according to an article in StatPearls, an online health care resource. “Most complications are considered minor such as pain, bleeding, infection and post-anesthesia complications,” according to the article.
The CDC calculates case-fatality rates for women from induced abortions – that is, how many women die from abortion-related complications, for every 100,000 legal abortions that occur in the U.S . The rate was lowest during the most recent period examined by the agency (2013 to 2020), when there were 0.45 deaths to women per 100,000 legal induced abortions. The case-fatality rate reported by the CDC was highest during the first period examined by the agency (1973 to 1977), when it was 2.09 deaths to women per 100,000 legal induced abortions. During the five-year periods in between, the figure ranged from 0.52 (from 1993 to 1997) to 0.78 (from 1978 to 1982).
The CDC calculates death rates by five-year and seven-year periods because of year-to-year fluctuation in the numbers and due to the relatively low number of women who die from legal induced abortions.
In 2020, the last year for which the CDC has information , six women in the U.S. died due to complications from induced abortions. Four women died in this way in 2019, two in 2018, and three in 2017. (These deaths all followed legal abortions.) Since 1990, the annual number of deaths among women due to legal induced abortion has ranged from two to 12.
The annual number of reported deaths from induced abortions (legal and illegal) tended to be higher in the 1980s, when it ranged from nine to 16, and from 1972 to 1979, when it ranged from 13 to 63. One driver of the decline was the drop in deaths from illegal abortions. There were 39 deaths from illegal abortions in 1972, the last full year before Roe v. Wade. The total fell to 19 in 1973 and to single digits or zero every year after that. (The number of deaths from legal abortions has also declined since then, though with some slight variation over time.)
The number of deaths from induced abortions was considerably higher in the 1960s than afterward. For instance, there were 119 deaths from induced abortions in 1963 and 99 in 1965 , according to reports by the then-U.S. Department of Health, Education and Welfare, a precursor to the Department of Health and Human Services. The CDC is a division of Health and Human Services.
Note: This is an update of a post originally published May 27, 2022, and first updated June 24, 2022.
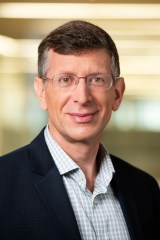
Support for legal abortion is widespread in many countries, especially in Europe
Nearly a year after roe’s demise, americans’ views of abortion access increasingly vary by where they live, by more than two-to-one, americans say medication abortion should be legal in their state, most latinos say democrats care about them and work hard for their vote, far fewer say so of gop, positive views of supreme court decline sharply following abortion ruling, most popular.
1615 L St. NW, Suite 800 Washington, DC 20036 USA (+1) 202-419-4300 | Main (+1) 202-857-8562 | Fax (+1) 202-419-4372 | Media Inquiries
Research Topics
- Age & Generations
- Coronavirus (COVID-19)
- Economy & Work
- Family & Relationships
- Gender & LGBTQ
- Immigration & Migration
- International Affairs
- Internet & Technology
- Methodological Research
- News Habits & Media
- Non-U.S. Governments
- Other Topics
- Politics & Policy
- Race & Ethnicity
- Email Newsletters
ABOUT PEW RESEARCH CENTER Pew Research Center is a nonpartisan fact tank that informs the public about the issues, attitudes and trends shaping the world. It conducts public opinion polling, demographic research, media content analysis and other empirical social science research. Pew Research Center does not take policy positions. It is a subsidiary of The Pew Charitable Trusts .
Copyright 2024 Pew Research Center
Terms & Conditions
Privacy Policy
Cookie Settings
Reprints, Permissions & Use Policy

An official website of the United States government
The .gov means it’s official. Federal government websites often end in .gov or .mil. Before sharing sensitive information, make sure you’re on a federal government site.
The site is secure. The https:// ensures that you are connecting to the official website and that any information you provide is encrypted and transmitted securely.
- Publications
- Account settings
Preview improvements coming to the PMC website in October 2024. Learn More or Try it out now .
- Advanced Search
- Journal List
- Neuropsychopharmacology
- v.46(10); 2021 Sep
Addiction as a brain disease revised: why it still matters, and the need for consilience
Markus heilig.
1 Center for Social and Affective Neuroscience, Department of Biomedical and Clinical Sciences, Linköping University, Linköping, Sweden
James MacKillop
2 Peter Boris Centre for Addictions Research, McMaster University and St. Joseph’s Healthcare Hamilton, Hamilton, ON Canada
3 Homewood Research Institute, Guelph, ON Canada
Diana Martinez
4 New York State Psychiatric Institute and Columbia University Irving Medical Center, New York, NY USA
Jürgen Rehm
5 Institute for Mental Health Policy Research & Campbell Family Mental Health Research Institute, Centre for Addiction and Mental Health (CAMH), Toronto, ON Canada
6 Dalla Lana School of Public Health and Department of Psychiatry, University of Toronto (UofT), Toronto, ON Canada
7 Klinische Psychologie & Psychotherapie, Technische Universität Dresden, Dresden, Germany
8 Department of International Health Projects, Institute for Leadership and Health Management, I.M. Sechenov First Moscow State Medical University, Moscow, Russia
Lorenzo Leggio
9 Clinical Psychoneuroendocrinology and Neuropsychopharmacology Section, Translational Addiction Medicine Branch, National Institute on Drug Abuse Intramural Research Program and National Institute on Alcohol Abuse and Alcoholism Division of Intramural Clinical and Biological Research, National Institutes of Health, Baltimore and Bethesda, MD USA
Louk J. M. J. Vanderschuren
10 Department of Population Health Sciences, Unit Animals in Science and Society, Faculty of Veterinary Medicine, Utrecht University, Utrecht, the Netherlands
The view that substance addiction is a brain disease, although widely accepted in the neuroscience community, has become subject to acerbic criticism in recent years. These criticisms state that the brain disease view is deterministic, fails to account for heterogeneity in remission and recovery, places too much emphasis on a compulsive dimension of addiction, and that a specific neural signature of addiction has not been identified. We acknowledge that some of these criticisms have merit, but assert that the foundational premise that addiction has a neurobiological basis is fundamentally sound. We also emphasize that denying that addiction is a brain disease is a harmful standpoint since it contributes to reducing access to healthcare and treatment, the consequences of which are catastrophic. Here, we therefore address these criticisms, and in doing so provide a contemporary update of the brain disease view of addiction. We provide arguments to support this view, discuss why apparently spontaneous remission does not negate it, and how seemingly compulsive behaviors can co-exist with the sensitivity to alternative reinforcement in addiction. Most importantly, we argue that the brain is the biological substrate from which both addiction and the capacity for behavior change arise, arguing for an intensified neuroscientific study of recovery. More broadly, we propose that these disagreements reveal the need for multidisciplinary research that integrates neuroscientific, behavioral, clinical, and sociocultural perspectives.
Introduction
Close to a quarter of a century ago, then director of the US National Institute on Drug Abuse Alan Leshner famously asserted that “addiction is a brain disease”, articulated a set of implications of this position, and outlined an agenda for realizing its promise [ 1 ]. The paper, now cited almost 2000 times, put forward a position that has been highly influential in guiding the efforts of researchers, and resource allocation by funding agencies. A subsequent 2000 paper by McLellan et al. [ 2 ] examined whether data justify distinguishing addiction from other conditions for which a disease label is rarely questioned, such as diabetes, hypertension or asthma. It concluded that neither genetic risk, the role of personal choices, nor the influence of environmental factors differentiated addiction in a manner that would warrant viewing it differently; neither did relapse rates, nor compliance with treatment. The authors outlined an agenda closely related to that put forward by Leshner, but with a more clinical focus. Their conclusion was that addiction should be insured, treated, and evaluated like other diseases. This paper, too, has been exceptionally influential by academic standards, as witnessed by its ~3000 citations to date. What may be less appreciated among scientists is that its impact in the real world of addiction treatment has remained more limited, with large numbers of patients still not receiving evidence-based treatments.
In recent years, the conceptualization of addiction as a brain disease has come under increasing criticism. When first put forward, the brain disease view was mainly an attempt to articulate an effective response to prevailing nonscientific, moralizing, and stigmatizing attitudes to addiction. According to these attitudes, addiction was simply the result of a person’s moral failing or weakness of character, rather than a “real” disease [ 3 ]. These attitudes created barriers for people with substance use problems to access evidence-based treatments, both those available at the time, such as opioid agonist maintenance, cognitive behavioral therapy-based relapse prevention, community reinforcement or contingency management, and those that could result from research. To promote patient access to treatments, scientists needed to argue that there is a biological basis beneath the challenging behaviors of individuals suffering from addiction. This argument was particularly targeted to the public, policymakers and health care professionals, many of whom held that since addiction was a misery people brought upon themselves, it fell beyond the scope of medicine, and was neither amenable to treatment, nor warranted the use of taxpayer money.
Present-day criticism directed at the conceptualization of addiction as a brain disease is of a very different nature. It originates from within the scientific community itself, and asserts that this conceptualization is neither supported by data, nor helpful for people with substance use problems [ 4 – 8 ]. Addressing these critiques requires a very different perspective, and is the objective of our paper. We readily acknowledge that in some cases, recent critiques of the notion of addiction as a brain disease as postulated originally have merit, and that those critiques require the postulates to be re-assessed and refined. In other cases, we believe the arguments have less validity, but still provide an opportunity to update the position of addiction as a brain disease. Our overarching concern is that questionable arguments against the notion of addiction as a brain disease may harm patients, by impeding access to care, and slowing development of novel treatments.
A premise of our argument is that any useful conceptualization of addiction requires an understanding both of the brains involved, and of environmental factors that interact with those brains [ 9 ]. These environmental factors critically include availability of drugs, but also of healthy alternative rewards and opportunities. As we will show, stating that brain mechanisms are critical for understanding and treating addiction in no way negates the role of psychological, social and socioeconomic processes as both causes and consequences of substance use. To reflect this complex nature of addiction, we have assembled a team with expertise that spans from molecular neuroscience, through animal models of addiction, human brain imaging, clinical addiction medicine, to epidemiology. What brings us together is a passionate commitment to improving the lives of people with substance use problems through science and science-based treatments, with empirical evidence as the guiding principle.
To achieve this goal, we first discuss the nature of the disease concept itself, and why we believe it is important for the science and treatment of addiction. This is followed by a discussion of the main points raised when the notion of addiction as a brain disease has come under criticism. Key among those are claims that spontaneous remission rates are high; that a specific brain pathology is lacking; and that people suffering from addiction, rather than behaving “compulsively”, in fact show a preserved ability to make informed and advantageous choices. In the process of discussing these issues, we also address the common criticism that viewing addiction as a brain disease is a fully deterministic theory of addiction. For our argument, we use the term “addiction” as originally used by Leshner [ 1 ]; in Box 1 , we map out and discuss how this construct may relate to the current diagnostic categories, such as Substance Use Disorder (SUD) and its different levels of severity (Fig. 1) .

Risky (hazardous) substance use refers to quantity/frequency indicators of consumption; SUD refers to individuals who meet criteria for a DSM-5 diagnosis (mild, moderate, or severe); and addiction refers to individuals who exhibit persistent difficulties with self-regulation of drug consumption. Among high-risk individuals, a subgroup will meet criteria for SUD and, among those who have an SUD, a further subgroup would be considered to be addicted to the drug. However, the boundary for addiction is intentionally blurred to reflect that the dividing line for defining addiction within the category of SUD remains an open empirical question.
Box 1 What’s in a name? Differentiating hazardous use, substance use disorder, and addiction
Although our principal focus is on the brain disease model of addiction, the definition of addiction itself is a source of ambiguity. Here, we provide a perspective on the major forms of terminology in the field.
Hazardous Substance Use
Hazardous (risky) substance use refers to quantitative levels of consumption that increase an individual’s risk for adverse health consequences. In practice, this pertains to alcohol use [ 110 , 111 ]. Clinically, alcohol consumption that exceeds guidelines for moderate drinking has been used to prompt brief interventions or referral for specialist care [ 112 ]. More recently, a reduction in these quantitative levels has been validated as treatment endpoints [ 113 ].
Substance Use Disorder
SUD refers to the DSM-5 diagnosis category that encompasses significant impairment or distress resulting from specific categories of psychoactive drug use. The diagnosis of SUD is operationalized as 2 or more of 11 symptoms over the past year. As a result, the diagnosis is heterogenous, with more than 1100 symptom permutations possible. The diagnosis in DSM-5 is the result of combining two diagnoses from the DSM-IV, abuse and dependence, which proved to be less valid than a single dimensional approach [ 114 ]. Critically, SUD includes three levels of severity: mild (2–3 symptoms), moderate (4–5 symptoms), and severe (6+ symptoms). The International Classification of Diseases (ICD) system retains two diagnoses, harmful use (lower severity) and substance dependence (higher severity).
Addiction is a natural language concept, etymologically meaning enslavement, with the contemporary meaning traceable to the Middle and Late Roman Republic periods [ 115 ]. As a scientific construct, drug addiction can be defined as a state in which an individual exhibits an inability to self-regulate consumption of a substance, although it does not have an operational definition. Regarding clinical diagnosis, as it is typically used in scientific and clinical parlance, addiction is not synonymous with the simple presence of SUD. Nowhere in DSM-5 is it articulated that the diagnostic threshold (or any specific number/type of symptoms) should be interpreted as reflecting addiction, which inherently connotes a high degree of severity. Indeed, concerns were raised about setting the diagnostic standard too low because of the issue of potentially conflating a low-severity SUD with addiction [ 116 ]. In scientific and clinical usage, addiction typically refers to individuals at a moderate or high severity of SUD. This is consistent with the fact that moderate-to-severe SUD has the closest correspondence with the more severe diagnosis in ICD [ 117 – 119 ]. Nonetheless, akin to the undefined overlap between hazardous use and SUD, the field has not identified the exact thresholds of SUD symptoms above which addiction would be definitively present.
Integration
The ambiguous relationships among these terms contribute to misunderstandings and disagreements. Figure 1 provides a simple working model of how these terms overlap. Fundamentally, we consider that these terms represent successive dimensions of severity, clinical “nesting dolls”. Not all individuals consuming substances at hazardous levels have an SUD, but a subgroup do. Not all individuals with a SUD are addicted to the drug in question, but a subgroup are. At the severe end of the spectrum, these domains converge (heavy consumption, numerous symptoms, the unambiguous presence of addiction), but at low severity, the overlap is more modest. The exact mapping of addiction onto SUD is an open empirical question, warranting systematic study among scientists, clinicians, and patients with lived experience. No less important will be future research situating our definition of SUD using more objective indicators (e.g., [ 55 , 120 ]), brain-based and otherwise, and more precisely in relation to clinical needs [ 121 ]. Finally, such work should ultimately be codified in both the DSM and ICD systems to demarcate clearly where the attribution of addiction belongs within the clinical nosology, and to foster greater clarity and specificity in scientific discourse.
What is a disease?
In his classic 1960 book “The Disease Concept of Alcoholism”, Jellinek noted that in the alcohol field, the debate over the disease concept was plagued by too many definitions of “alcoholism” and too few definitions of “disease” [ 10 ]. He suggested that the addiction field needed to follow the rest of medicine in moving away from viewing disease as an “entity”, i.e., something that has “its own independent existence, apart from other things” [ 11 ]. To modern medicine, he pointed out, a disease is simply a label that is agreed upon to describe a cluster of substantial, deteriorating changes in the structure or function of the human body, and the accompanying deterioration in biopsychosocial functioning. Thus, he concluded that alcoholism can simply be defined as changes in structure or function of the body due to drinking that cause disability or death. A disease label is useful to identify groups of people with commonly co-occurring constellations of problems—syndromes—that significantly impair function, and that lead to clinically significant distress, harm, or both. This convention allows a systematic study of the condition, and of whether group members benefit from a specific intervention.
It is not trivial to delineate the exact category of harmful substance use for which a label such as addiction is warranted (See Box 1 ). Challenges to diagnostic categorization are not unique to addiction, however. Throughout clinical medicine, diagnostic cut-offs are set by consensus, commonly based on an evolving understanding of thresholds above which people tend to benefit from available interventions. Because assessing benefits in large patient groups over time is difficult, diagnostic thresholds are always subject to debate and adjustments. It can be debated whether diagnostic thresholds “merely” capture the extreme of a single underlying population, or actually identify a subpopulation that is at some level distinct. Resolving this issue remains challenging in addiction, but once again, this is not different from other areas of medicine [see e.g., [ 12 ] for type 2 diabetes]. Longitudinal studies that track patient trajectories over time may have a better ability to identify subpopulations than cross-sectional assessments [ 13 ].
By this pragmatic, clinical understanding of the disease concept, it is difficult to argue that “addiction” is unjustified as a disease label. Among people who use drugs or alcohol, some progress to using with a quantity and frequency that results in impaired function and often death, making substance use a major cause of global disease burden [ 14 ]. In these people, use occurs with a pattern that in milder forms may be challenging to capture by current diagnostic criteria (See Box 1 ), but is readily recognized by patients, their families and treatment providers when it reaches a severity that is clinically significant [see [ 15 ] for a classical discussion]. In some cases, such as opioid addiction, those who receive the diagnosis stand to obtain some of the greatest benefits from medical treatments in all of clinical medicine [ 16 , 17 ]. Although effect sizes of available treatments are more modest in nicotine [ 18 ] and alcohol addiction [ 19 ], the evidence supporting their efficacy is also indisputable. A view of addiction as a disease is justified, because it is beneficial: a failure to diagnose addiction drastically increases the risk of a failure to treat it [ 20 ].
Of course, establishing a diagnosis is not a requirement for interventions to be meaningful. People with hazardous or harmful substance use who have not (yet) developed addiction should also be identified, and interventions should be initiated to address their substance-related risks. This is particularly relevant for alcohol, where even in the absence of addiction, use is frequently associated with risks or harm to self, e.g., through cardiovascular disease, liver disease or cancer, and to others, e.g., through accidents or violence [ 21 ]. Interventions to reduce hazardous or harmful substance use in people who have not developed addiction are in fact particularly appealing. In these individuals, limited interventions are able to achieve robust and meaningful benefits [ 22 ], presumably because patterns of misuse have not yet become entrenched.
Thus, as originally pointed out by McLellan and colleagues, most of the criticisms of addiction as a disease could equally be applied to other medical conditions [ 2 ]. This type of criticism could also be applied to other psychiatric disorders, and that has indeed been the case historically [ 23 , 24 ]. Today, there is broad consensus that those criticisms were misguided. Few, if any healthcare professionals continue to maintain that schizophrenia, rather than being a disease, is a normal response to societal conditions. Why, then, do people continue to question if addiction is a disease, but not whether schizophrenia, major depressive disorder or post-traumatic stress disorder are diseases? This is particularly troubling given the decades of data showing high co-morbidity of addiction with these conditions [ 25 , 26 ]. We argue that it comes down to stigma. Dysregulated substance use continues to be perceived as a self-inflicted condition characterized by a lack of willpower, thus falling outside the scope of medicine and into that of morality [ 3 ].
Chronic and relapsing, developmentally-limited, or spontaneously remitting?
Much of the critique targeted at the conceptualization of addiction as a brain disease focuses on its original assertion that addiction is a chronic and relapsing condition. Epidemiological data are cited in support of the notion that large proportions of individuals achieve remission [ 27 ], frequently without any formal treatment [ 28 , 29 ] and in some cases resuming low risk substance use [ 30 ]. For instance, based on data from the National Epidemiologic Survey on Alcohol and Related Conditions (NESARC) study [ 27 ], it has been pointed out that a significant proportion of people with an addictive disorder quit each year, and that most afflicted individuals ultimately remit. These spontaneous remission rates are argued to invalidate the concept of a chronic, relapsing disease [ 4 ].
Interpreting these and similar data is complicated by several methodological and conceptual issues. First, people may appear to remit spontaneously because they actually do, but also because of limited test–retest reliability of the diagnosis [ 31 ]. For instance, using a validated diagnostic interview and trained interviewers, the Collaborative Studies on Genetics of Alcoholism examined the likelihood that an individual diagnosed with a lifetime history of substance dependence would retain this classification after 5 years. This is obviously a diagnosis that, once met, by definition cannot truly remit. Lifetime alcohol dependence was indeed stable in individuals recruited from addiction treatment units, ~90% for women, and 95% for men. In contrast, in a community-based sample similar to that used in the NESARC [ 27 ], stability was only ~30% and 65% for women and men, respectively. The most important characteristic that determined diagnostic stability was severity. Diagnosis was stable in severe, treatment-seeking cases, but not in general population cases of alcohol dependence.
These data suggest that commonly used diagnostic criteria alone are simply over-inclusive for a reliable, clinically meaningful diagnosis of addiction. They do identify a core group of treatment seeking individuals with a reliable diagnosis, but, if applied to nonclinical populations, also flag as “cases” a considerable halo of individuals for whom the diagnostic categorization is unreliable. Any meaningful discussion of remission rates needs to take this into account, and specify which of these two populations that is being discussed. Unfortunately, the DSM-5 has not made this task easier. With only 2 out of 11 symptoms being sufficient for a diagnosis of SUD, it captures under a single diagnostic label individuals in a “mild” category, whose diagnosis is likely to have very low test–retest reliability, and who are unlikely to exhibit a chronic relapsing course, together with people at the severe end of the spectrum, whose diagnosis is reliable, many of whom do show a chronic relapsing course.
The NESARC data nevertheless show that close to 10% of people in the general population who are diagnosed with alcohol addiction (here equated with DSM-IV “dependence” used in the NESARC study) never remitted throughout their participation in the survey. The base life-time prevalence of alcohol dependence in NESARC was 12.5% [ 32 ]. Thus, the data cited against the concept of addiction as a chronic relapsing disease in fact indicate that over 1% of the US population develops an alcohol-related condition that is associated with high morbidity and mortality, and whose chronic and/or relapsing nature cannot be disputed, since it does not remit.
Secondly, the analysis of NESARC data [ 4 , 27 ] omits opioid addiction, which, together with alcohol and tobacco, is the largest addiction-related public health problem in the US [ 33 ]. This is probably the addictive condition where an analysis of cumulative evidence most strikingly supports the notion of a chronic disorder with frequent relapses in a large proportion of people affected [ 34 ]. Of course, a large number of people with opioid addiction are unable to express the chronic, relapsing course of their disease, because over the long term, their mortality rate is about 15 times greater than that of the general population [ 35 ]. However, even among those who remain alive, the prevalence of stable abstinence from opioid use after 10–30 years of observation is <30%. Remission may not always require abstinence, for instance in the case of alcohol addiction, but is a reasonable proxy for remission with opioids, where return to controlled use is rare. Embedded in these data is a message of literally vital importance: when opioid addiction is diagnosed and treated as a chronic relapsing disease, outcomes are markedly improved, and retention in treatment is associated with a greater likelihood of abstinence.
The fact that significant numbers of individuals exhibit a chronic relapsing course does not negate that even larger numbers of individuals with SUD according to current diagnostic criteria do not. For instance, in many countries, the highest prevalence of substance use problems is found among young adults, aged 18–25 [ 36 ], and a majority of these ‘age out’ of excessive substance use [ 37 ]. It is also well documented that many individuals with SUD achieve longstanding remission, in many cases without any formal treatment (see e.g., [ 27 , 30 , 38 ]).
Collectively, the data show that the course of SUD, as defined by current diagnostic criteria, is highly heterogeneous. Accordingly, we do not maintain that a chronic relapsing course is a defining feature of SUD. When present in a patient, however, such as course is of clinical significance, because it identifies a need for long-term disease management [ 2 ], rather than expectations of a recovery that may not be within the individual’s reach [ 39 ]. From a conceptual standpoint, however, a chronic relapsing course is neither necessary nor implied in a view that addiction is a brain disease. This view also does not mean that it is irreversible and hopeless. Human neuroscience documents restoration of functioning after abstinence [ 40 , 41 ] and reveals predictors of clinical success [ 42 ]. If anything, this evidence suggests a need to increase efforts devoted to neuroscientific research on addiction recovery [ 40 , 43 ].
Lessons from genetics
For alcohol addiction, meta-analysis of twin and adoption studies has estimated heritability at ~50%, while estimates for opioid addiction are even higher [ 44 , 45 ]. Genetic risk factors are to a large extent shared across substances [ 46 ]. It has been argued that a genetic contribution cannot support a disease view of a behavior, because most behavioral traits, including religious and political inclinations, have a genetic contribution [ 4 ]. This statement, while correct in pointing out broad heritability of behavioral traits, misses a fundamental point. Genetic architecture is much like organ structure. The fact that normal anatomy shapes healthy organ function does not negate that an altered structure can contribute to pathophysiology of disease. The structure of the genetic landscape is no different. Critics further state that a “genetic predisposition is not a recipe for compulsion”, but no neuroscientist or geneticist would claim that genetic risk is “a recipe for compulsion”. Genetic risk is probabilistic, not deterministic. However, as we will see below, in the case of addiction, it contributes to large, consistent probability shifts towards maladaptive behavior.
In dismissing the relevance of genetic risk for addiction, Hall writes that “a large number of alleles are involved in the genetic susceptibility to addiction and individually these alleles might very weakly predict a risk of addiction”. He goes on to conclude that “generally, genetic prediction of the risk of disease (even with whole-genome sequencing data) is unlikely to be informative for most people who have a so-called average risk of developing an addiction disorder” [ 7 ]. This reflects a fundamental misunderstanding of polygenic risk. It is true that a large number of risk alleles are involved, and that the explanatory power of currently available polygenic risk scores for addictive disorders lags behind those for e.g., schizophrenia or major depression [ 47 , 48 ]. The only implication of this, however, is that low average effect sizes of risk alleles in addiction necessitate larger study samples to construct polygenic scores that account for a large proportion of the known heritability.
However, a heritability of addiction of ~50% indicates that DNA sequence variation accounts for 50% of the risk for this condition. Once whole genome sequencing is readily available, it is likely that it will be possible to identify most of that DNA variation. For clinical purposes, those polygenic scores will of course not replace an understanding of the intricate web of biological and social factors that promote or prevent expression of addiction in an individual case; rather, they will add to it [ 49 ]. Meanwhile, however, genome-wide association studies in addiction have already provided important information. For instance, they have established that the genetic underpinnings of alcohol addiction only partially overlap with those for alcohol consumption, underscoring the genetic distinction between pathological and nonpathological drinking behaviors [ 50 ].
It thus seems that, rather than negating a rationale for a disease view of addiction, the important implication of the polygenic nature of addiction risk is a very different one. Genome-wide association studies of complex traits have largely confirmed the century old “infinitisemal model” in which Fisher reconciled Mendelian and polygenic traits [ 51 ]. A key implication of this model is that genetic susceptibility for a complex, polygenic trait is continuously distributed in the population. This may seem antithetical to a view of addiction as a distinct disease category, but the contradiction is only apparent, and one that has long been familiar to quantitative genetics. Viewing addiction susceptibility as a polygenic quantitative trait, and addiction as a disease category is entirely in line with Falconer’s theorem, according to which, in a given set of environmental conditions, a certain level of genetic susceptibility will determine a threshold above which disease will arise.
A brain disease? Then show me the brain lesion!
The notion of addiction as a brain disease is commonly criticized with the argument that a specific pathognomonic brain lesion has not been identified. Indeed, brain imaging findings in addiction (perhaps with the exception of extensive neurotoxic gray matter loss in advanced alcohol addiction) are nowhere near the level of specificity and sensitivity required of clinical diagnostic tests. However, this criticism neglects the fact that neuroimaging is not used to diagnose many neurologic and psychiatric disorders, including epilepsy, ALS, migraine, Huntington’s disease, bipolar disorder, or schizophrenia. Even among conditions where signs of disease can be detected using brain imaging, such as Alzheimer’s and Parkinson’s disease, a scan is best used in conjunction with clinical acumen when making the diagnosis. Thus, the requirement that addiction be detectable with a brain scan in order to be classified as a disease does not recognize the role of neuroimaging in the clinic.
For the foreseeable future, the main objective of imaging in addiction research is not to diagnose addiction, but rather to improve our understanding of mechanisms that underlie it. The hope is that mechanistic insights will help bring forward new treatments, by identifying candidate targets for them, by pointing to treatment-responsive biomarkers, or both [ 52 ]. Developing innovative treatments is essential to address unmet treatment needs, in particular in stimulant and cannabis addiction, where no approved medications are currently available. Although the task to develop novel treatments is challenging, promising candidates await evaluation [ 53 ]. A particular opportunity for imaging-based research is related to the complex and heterogeneous nature of addictive disorders. Imaging-based biomarkers hold the promise of allowing this complexity to be deconstructed into specific functional domains, as proposed by the RDoC initiative [ 54 ] and its application to addiction [ 55 , 56 ]. This can ultimately guide the development of personalized medicine strategies to addiction treatment.
Countless imaging studies have reported differences in brain structure and function between people with addictive disorders and those without them. Meta-analyses of structural data show that alcohol addiction is associated with gray matter losses in the prefrontal cortex, dorsal striatum, insula, and posterior cingulate cortex [ 57 ], and similar results have been obtained in stimulant-addicted individuals [ 58 ]. Meta-analysis of functional imaging studies has demonstrated common alterations in dorsal striatal, and frontal circuits engaged in reward and salience processing, habit formation, and executive control, across different substances and task-paradigms [ 59 ]. Molecular imaging studies have shown that large and fast increases in dopamine are associated with the reinforcing effects of drugs of abuse, but that after chronic drug use and during withdrawal, brain dopamine function is markedly decreased and that these decreases are associated with dysfunction of prefrontal regions [ 60 ]. Collectively, these findings have given rise to a widely held view of addiction as a disorder of fronto-striatal circuitry that mediates top-down regulation of behavior [ 61 ].
Critics reply that none of the brain imaging findings are sufficiently specific to distinguish between addiction and its absence, and that they are typically obtained in cross-sectional studies that can at best establish correlative rather than causal links. In this, they are largely right, and an updated version of a conceptualization of addiction as a brain disease needs to acknowledge this. Many of the structural brain findings reported are not specific for addiction, but rather shared across psychiatric disorders [ 62 ]. Also, for now, the most sophisticated tools of human brain imaging remain crude in face of complex neural circuit function. Importantly however, a vast literature from animal studies also documents functional changes in fronto-striatal circuits, as well their limbic and midbrain inputs, associated with addictive behaviors [ 63 – 68 ]. These are circuits akin to those identified by neuroimaging studies in humans, implicated in positive and negative emotions, learning processes and executive functions, altered function of which is thought to underlie addiction. These animal studies, by virtue of their cellular and molecular level resolution, and their ability to establish causality under experimental control, are therefore an important complement to human neuroimaging work.
Nevertheless, factors that seem remote from the activity of brain circuits, such as policies, substance availability and cost, as well as socioeconomic factors, also are critically important determinants of substance use. In this complex landscape, is the brain really a defensible focal point for research and treatment? The answer is “yes”. As powerfully articulated by Francis Crick [ 69 ], “You, your joys and your sorrows, your memories and your ambitions, your sense of personal identity and free will, are in fact no more than the behavior of a vast assembly of nerve cells and their associated molecules”. Social and interpersonal factors are critically important in addiction, but they can only exert their influences by impacting neural processes. They must be encoded as sensory data, represented together with memories of the past and predictions about the future, and combined with representations of interoceptive and other influences to provide inputs to the valuation machinery of the brain. Collectively, these inputs drive action selection and execution of behavior—say, to drink or not to drink, and then, within an episode, to stop drinking or keep drinking. Stating that the pathophysiology of addiction is largely about the brain does not ignore the role of other influences. It is just the opposite: it is attempting to understand how those important influences contribute to drug seeking and taking in the context of the brain, and vice versa.
But if the criticism is one of emphasis rather than of principle—i.e., too much brain, too little social and environmental factors – then neuroscientists need to acknowledge that they are in part guilty as charged. Brain-centric accounts of addiction have for a long time failed to pay enough attention to the inputs that social factors provide to neural processing behind drug seeking and taking [ 9 ]. This landscape is, however, rapidly changing. For instance, using animal models, scientists are finding that lack of social play early in life increases the motivation to take addictive substances in adulthood [ 70 ]. Others find that the opportunity to interact with a fellow rat is protective against addiction-like behaviors [ 71 ]. In humans, a relationship has been found between perceived social support, socioeconomic status, and the availability of dopamine D2 receptors [ 72 , 73 ], a biological marker of addiction vulnerability. Those findings in turn provided translation of data from nonhuman primates, which showed that D2 receptor availability can be altered by changes in social hierarchy, and that these changes are associated with the motivation to obtain cocaine [ 74 ].
Epidemiologically, it is well established that social determinants of health, including major racial and ethnic disparities, play a significant role in the risk for addiction [ 75 , 76 ]. Contemporary neuroscience is illuminating how those factors penetrate the brain [ 77 ] and, in some cases, reveals pathways of resilience [ 78 ] and how evidence-based prevention can interrupt those adverse consequences [ 79 , 80 ]. In other words, from our perspective, viewing addiction as a brain disease in no way negates the importance of social determinants of health or societal inequalities as critical influences. In fact, as shown by the studies correlating dopamine receptors with social experience, imaging is capable of capturing the impact of the social environment on brain function. This provides a platform for understanding how those influences become embedded in the biology of the brain, which provides a biological roadmap for prevention and intervention.
We therefore argue that a contemporary view of addiction as a brain disease does not deny the influence of social, environmental, developmental, or socioeconomic processes, but rather proposes that the brain is the underlying material substrate upon which those factors impinge and from which the responses originate. Because of this, neurobiology is a critical level of analysis for understanding addiction, although certainly not the only one. It is recognized throughout modern medicine that a host of biological and non-biological factors give rise to disease; understanding the biological pathophysiology is critical for understanding etiology and informing treatment.
Is a view of addiction as a brain disease deterministic?
A common criticism of the notion that addiction is a brain disease is that it is reductionist and in the end therefore deterministic [ 81 , 82 ]. This is a fundamental misrepresentation. As indicated above, viewing addiction as a brain disease simply states that neurobiology is an undeniable component of addiction. A reason for deterministic interpretations may be that modern neuroscience emphasizes an understanding of proximal causality within research designs (e.g., whether an observed link between biological processes is mediated by a specific mechanism). That does not in any way reflect a superordinate assumption that neuroscience will achieve global causality. On the contrary, since we realize that addiction involves interactions between biology, environment and society, ultimate (complete) prediction of behavior based on an understanding of neural processes alone is neither expected, nor a goal.
A fairer representation of a contemporary neuroscience view is that it believes insights from neurobiology allow useful probabilistic models to be developed of the inherently stochastic processes involved in behavior [see [ 83 ] for an elegant recent example]. Changes in brain function and structure in addiction exert a powerful probabilistic influence over a person’s behavior, but one that is highly multifactorial, variable, and thus stochastic. Philosophically, this is best understood as being aligned with indeterminism, a perspective that has a deep history in philosophy and psychology [ 84 ]. In modern neuroscience, it refers to the position that the dynamic complexity of the brain, given the probabilistic threshold-gated nature of its biology (e.g., action potential depolarization, ion channel gating), means that behavior cannot be definitively predicted in any individual instance [ 85 , 86 ].
Driven by compulsion, or free to choose?
A major criticism of the brain disease view of addiction, and one that is related to the issue of determinism vs indeterminism, centers around the term “compulsivity” [ 6 , 87 – 90 ] and the different meanings it is given. Prominent addiction theories state that addiction is characterized by a transition from controlled to “compulsive” drug seeking and taking [ 91 – 95 ], but allocate somewhat different meanings to “compulsivity”. By some accounts, compulsive substance use is habitual and insensitive to its outcomes [ 92 , 94 , 96 ]. Others refer to compulsive use as a result of increasing incentive value of drug associated cues [ 97 ], while others view it as driven by a recruitment of systems that encode negative affective states [ 95 , 98 ].
The prototype for compulsive behavior is provided by obsessive-compulsive disorder (OCD), where compulsion refers to repeatedly and stereotypically carrying out actions that in themselves may be meaningful, but lose their purpose and become harmful when performed in excess, such as persistent handwashing until skin injuries result. Crucially, this happens despite a conscious desire to do otherwise. Attempts to resist these compulsions result in increasing and ultimately intractable anxiety [ 99 ]. This is in important ways different from the meaning of compulsivity as commonly used in addiction theories. In the addiction field, compulsive drug use typically refers to inflexible, drug-centered behavior in which substance use is insensitive to adverse consequences [ 100 ]. Although this phenomenon is not necessarily present in every patient, it reflects important symptoms of clinical addiction, and is captured by several DSM-5 criteria for SUD [ 101 ]. Examples are needle-sharing despite knowledge of a risk to contract HIV or Hepatitis C, drinking despite a knowledge of having liver cirrhosis, but also the neglect of social and professional activities that previously were more important than substance use. While these behaviors do show similarities with the compulsions of OCD, there are also important differences. For example, “compulsive” substance use is not necessarily accompanied by a conscious desire to withhold the behavior, nor is addictive behavior consistently impervious to change.
Critics question the existence of compulsivity in addiction altogether [ 5 – 7 , 89 ], typically using a literal interpretation, i.e., that a person who uses alcohol or drugs simply can not do otherwise. Were that the intended meaning in theories of addiction—which it is not—it would clearly be invalidated by observations of preserved sensitivity of behavior to contingencies in addiction. Indeed, substance use is influenced both by the availability of alternative reinforcers, and the state of the organism. The roots of this insight date back to 1940, when Spragg found that chimpanzees would normally choose a banana over morphine. However, when physically dependent and in a state of withdrawal, their choice preference would reverse [ 102 ]. The critical role of alternative reinforcers was elegantly brought into modern neuroscience by Ahmed et al., who showed that rats extensively trained to self-administer cocaine would readily forego the drug if offered a sweet solution as an alternative [ 103 ]. This was later also found to be the case for heroin [ 103 ], methamphetamine [ 104 ] and alcohol [ 105 ]. Early residential laboratory studies on alcohol use disorder indeed revealed orderly operant control over alcohol consumption [ 106 ]. Furthermore, efficacy of treatment approaches such as contingency management, which provides systematic incentives for abstinence [ 107 ], supports the notion that behavioral choices in patients with addictions remain sensitive to reward contingencies.
Evidence that a capacity for choosing advantageously is preserved in addiction provides a valid argument against a narrow concept of “compulsivity” as rigid, immutable behavior that applies to all patients. It does not, however, provide an argument against addiction as a brain disease. If not from the brain, from where do the healthy and unhealthy choices people make originate? The critical question is whether addictive behaviors—for the most part—result from healthy brains responding normally to externally determined contingencies; or rather from a pathology of brain circuits that, through probabilistic shifts, promotes the likelihood of maladaptive choices even when reward contingencies are within a normal range. To resolve this question, it is critical to understand that the ability to choose advantageously is not an all-or-nothing phenomenon, but rather is about probabilities and their shifts, multiple faculties within human cognition, and their interaction. Yes, it is clear that most people whom we would consider to suffer from addiction remain able to choose advantageously much, if not most, of the time. However, it is also clear that the probability of them choosing to their own disadvantage, even when more salutary options are available and sometimes at the expense of losing their life, is systematically and quantifiably increased. There is a freedom of choice, yet there is a shift of prevailing choices that nevertheless can kill.
Synthesized, the notion of addiction as a disease of choice and addiction as a brain disease can be understood as two sides of the same coin. Both of these perspectives are informative, and they are complementary. Viewed this way, addiction is a brain disease in which a person’s choice faculties become profoundly compromised. To articulate it more specifically, embedded in and principally executed by the central nervous system, addiction can be understood as a disorder of choice preferences, preferences that overvalue immediate reinforcement (both positive and negative), preferences for drug-reinforcement in spite of costs, and preferences that are unstable ( “I’ll never drink like that again;” “this will be my last cigarette” ), prone to reversals in the form of lapses and relapse. From a contemporary neuroscience perspective, pre-existing vulnerabilities and persistent drug use lead to a vicious circle of substantive disruptions in the brain that impair and undermine choice capacities for adaptive behavior, but do not annihilate them. Evidence of generally intact decision making does not fundamentally contradict addiction as a brain disease.
Conclusions
The present paper is a response to the increasing number of criticisms of the view that addiction is a chronic relapsing brain disease. In many cases, we show that those criticisms target tenets that are neither needed nor held by a contemporary version of this view. Common themes are that viewing addiction as a brain disease is criticized for being both too narrow (addiction is only a brain disease; no other perspectives or factors are important) or too far reaching (it purports to discover the final causes of addiction). With regard to disease course, we propose that viewing addiction as a chronic relapsing disease is appropriate for some populations, and much less so for others, simply necessitating better ways of delineating the populations being discussed. We argue that when considering addiction as a disease, the lens of neurobiology is valuable to use. It is not the only lens, and it does not have supremacy over other scientific approaches. We agree that critiques of neuroscience are warranted [ 108 ] and that critical thinking is essential to avoid deterministic language and scientific overreach.
Beyond making the case for a view of addiction as a brain disease, perhaps the more important question is when a specific level of analysis is most useful. For understanding the biology of addiction and designing biological interventions, a neurobiological view is almost certainly the most appropriate level of analysis, in particular when informed by an understanding of the behavioral manifestations. In contrast, for understanding the psychology of addiction and designing psychological interventions, behavioral science is the natural realm, but one that can often benefit from an understanding of the underlying neurobiology. For designing policies, such as taxation and regulation of access, economics and public administration provide the most pertinent perspectives, but these also benefit from biological and behavioral science insights.
Finally, we argue that progress would come from integration of these scientific perspectives and traditions. E.O. Wilson has argued more broadly for greater consilience [ 109 ], unity of knowledge, in science. We believe that addiction is among the areas where consilience is most needed. A plurality of disciplines brings important and trenchant insights to bear on this condition; it is the exclusive remit of no single perspective or field. Addiction inherently and necessarily requires multidisciplinary examination. Moreover, those who suffer from addiction will benefit most from the application of the full armamentarium of scientific perspectives.
Funding and disclosures
Supported by the Swedish Research Council grants 2013-07434, 2019-01138 (MH); Netherlands Organisation for Health Research and Development (ZonMw) under project number 912.14.093 (LJMJV); NIDA and NIAAA intramural research programs (LL; the content is solely the responsibility of the author and does not necessarily represent the official views of the National Institutes of Health); the Peter Boris Chair in Addictions Research, Homewood Research Institute, and the National Institute on Alcohol Abuse and Alcoholism grants AA025911, AA024930, AA025849, AA027679 (JM; the content is solely the responsibility of the author and does not necessarily represent the official views of the National Institutes of Health).
MH has received consulting fees, research support or other compensation from Indivior, Camurus, BrainsWay, Aelis Farma, and Janssen Pharmaceuticals. JM is a Principal and Senior Scientist at BEAM Diagnostics, Inc. DM, JR, LL, and LJMJV declare no conflict of interest.
Acknowledgements
The authors want to acknowledge comments by Drs. David Epstein, Kenneth Kendler and Naomi Wray.
Author contributions
All authors jointly drafted the paper.
Publisher’s note Springer Nature remains neutral with regard to jurisdictional claims in published maps and institutional affiliations.
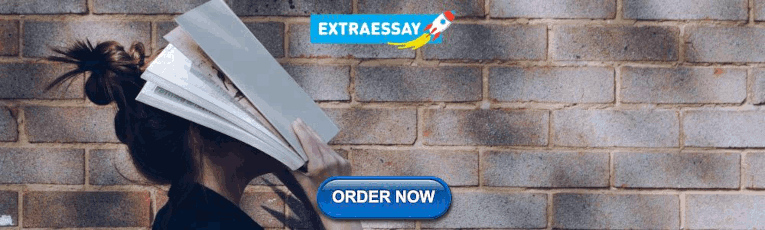
IMAGES
VIDEO
COMMENTS
The numbers for substance use disorders are large, and we need to pay attention to them. Data from the 2018 National Survey on Drug Use and Health suggest that, over the preceding year, 20.3 million people age 12 or older had substance use disorders, and 14.8 million of these cases were attributed to alcohol.When considering other substances, the report estimated that 4.4 million individuals ...
1) No/ Low perceived risk increase the odds of past-month marijuana use by 8.22 times compared to those who perceived moderate/great risk. 2) High perceived availability of drug: consistently associated with higher odds of past-month marijuana use. Protective Factors. 1) Moderate/ High perceived risk of substance use.
The present study was conducted using a protocol-oriented process and all the reliable scientific databases in the world. While, the concept of addiction includes a wide range of addictive behaviors, addiction in this research meant using any drug and alcohol. There are many limitations to the studies used in this research.
Both prevention and treatment of substance abuse are pertinent to public health, as the two approaches need to employ a multilevel perspective to conceptualize and solve fallout generated from drug trafficking, misuse and harm [ 11, 12 ]. This points to the need to investigate human hereditary, biological, and psychological needs, cognitive and ...
Brain-centric accounts of addiction have for a long time failed to pay enough attention to the inputs that social factors provide to neural processing behind drug seeking and taking . This ...
Addiction, the most severe form of substance use disorder, is a chronic brain disorder molded by strong biosocial factors that has devastating consequences to individuals and to society. Our understanding of substance use disorder has advanced significantly over the last 3 decades in part due to major progress in genetics and neuroscience research and to the development of new technologies ...
Background Persons who use drug need family and society's support in the process of treatment and rehabilitation. Therefore, it is imperative to determine the psychological, social, and motivational factors that can help them in the treatment process. The present study was an attempt to determine the relationship between psychological, social, and motivational factors and the demographics of ...
Drug addiction is a chronic mental disorder characterized by escalating compulsive drug use regardless of its negative consequences and by recurrent relapses, that are the main challenge for its treatment. At the initial phase, abused substances activate the reward system causing pleasurable effects that reinforce their consumption. With repeated administration, the rewarding properties of ...
Implications and effects on parents and family. Zimic and Jackic (Citation 2012) aver that the impact that substance abuse has on the family and on the individual family members merit attention.Lander et al. (Citation 2013) maintains that family members are affected by the individual's substance abuse; however, each individual is affected differently together with, but not limited to, having ...
Background Drug abuse is detrimental, and excessive drug usage is a worldwide problem. Drug usage typically begins during adolescence. Factors for drug abuse include a variety of protective and risk factors. Hence, this systematic review aimed to determine the risk and protective factors of drug abuse among adolescents worldwide. Methods Preferred Reporting Items for Systematic Reviews and ...
For much of the past century, scientists studying drugs and drug use labored in the shadows of powerful myths and misconceptions about the nature of addiction. When scientists began to study addictive behavior in the 1930s, people with an addiction were thought to be morally flawed and lacking in willpower. Those views shaped society's ...
Abstract. The current opioid epidemic is one of the most severe public health crisis in US history. Responding to it has been difficult due to its rapidly changing nature and the severity of its associated outcomes. This review examines the origin and evolution of the crisis, the pharmacological properties of opioids, the neurobiology of opioid ...
generation as a global issue. Drug addiction is not just a personal behavior but a social behavior as well [2]. The problem of addiction and drugs is as ominous as the destruction of the environment, nuclear threat, or world famine crisis—one of the four major global disasters [3]. Drug abuse is a predictable process that usually begins
The more risk factors a person has, the greater the chance that taking drugs can lead to addiction. Drug addiction is treatable and can be successfully managed. More good news is that drug use and addiction are preventable. Teachers, parents, and health care providers have crucial roles in educating young people and preventing drug use and ...
Drug addiction is a preventable disease. Results from NIDA-funded research have shown that prevention programs involving families, schools, communities, and the media are effective in reducing drug abuse. Although many events and cultural factors affect drug abuse trends, when youths perceive drug abuse as harmful, they reduce their drug taking.
Prescription opioid misuse continued to decline, with 1.5% of college students and 3.3% of those not attending college reporting non-medical use of opioids (narcotic drugs other than heroin) in the past year. This represents a significant five-year decline from rates of 4.8% and 7.7%, respectively, in 2014. Amphetamine use continued to decline ...
Research paper. Quality of life among people who use drugs living in poor urban communities in the Philippines ... substance use problems are pervasive yet underdiagnosed (Salwan & Katz, 2014), and the demand for addiction treatment, harm reduction, and social welfare services far exceeds the capacity to respond (Lora, Hanna, & Chisholm, 2017 ...
This paper first introduces important conceptual and practical distinctions among three key terms: substance "use," "misuse," and "disorders" (including addiction), and goes on to describe and quantify the important health and social problems associated with these terms. National survey data are presented to summarize the prevalence ...
Comparisons between digital media and narcotic drugs have become increasingly common in the vigorous discussion on smartphone addiction and technology addiction. Commentators have used evocative terms such as "digital heroin," "electronic cocaine," and "virtual drugs" when discussing users' growing dependence on their devices.
Drug addiction is a serious problem that affects mental health and well-being. This research paper examines the objective, methodology, results and implications of a study on the impact of drug ...
Abstract -The present research investigates the relationship between drug addiction and six. factors including peer pressure, media, family negligence, depression, curiosity, a nd ph ysical ...
The CDC says that in 2021, there were 11.6 abortions in the U.S. per 1,000 women ages 15 to 44. (That figure excludes data from California, the District of Columbia, Maryland, New Hampshire and New Jersey.) Like Guttmacher's data, the CDC's figures also suggest a general decline in the abortion rate over time.
The impact of peer pressure on teenagers' behaviour is well documented in literature and is considered as one of the most influential factors in substance abuse. 22 -24 Other studies found that the power of peers in enforcing the behaviour of substance abuse was greater than family's protective role. 25 Literature has also shown that drug ...
Introduction. Close to a quarter of a century ago, then director of the US National Institute on Drug Abuse Alan Leshner famously asserted that "addiction is a brain disease", articulated a set of implications of this position, and outlined an agenda for realizing its promise [].The paper, now cited almost 2000 times, put forward a position that has been highly influential in guiding the ...