Predictive maintenance in the Industry 4.0: A systematic literature review
- Related Documents
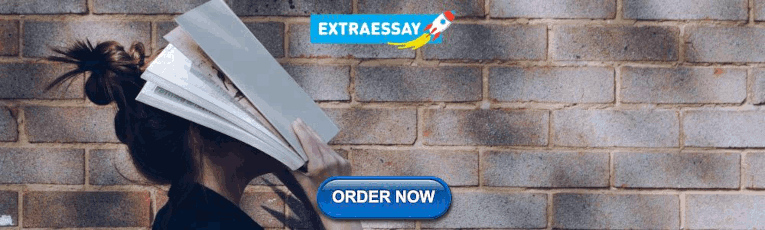
A Systematic Literature Review: Human Roles, Competencies And Skills In Industry 4.0
Improving supply chain resilience through industry 4.0: a systematic literature review under the impressions of the covid-19 pandemic, from supply chain 4.0 to supply chain 5.0: findings from a systematic literature review and research directions.
The main purpose of this paper is to present what the Industry 5.0 phenomenon means in the supply chain context. A systematic literature review method was used to get evidence from the current knowledge linked to this theme. The results have evidenced a strong gap related to Industry 5.0 approaches for the supply chain field. Forty-one (41) publications, including conference and journal papers, have been found in the literature. Nineteen (19) words, which were grouped in four (4) clusters, have been identified in the data analysis. This was the basis to form the four (4) constructs of Industry 5.0: Industry Strategy, Innovation and Technologies, Society and Sustainability, and Transition Issues. Then, an alignment with the supply chain context was proposed, being the basis for the incipient Supply Chain 5.0 framework and its research agenda. Industry 5.0 is still in an embryonic and ideal stage. The literature is scarce and many other concepts and discoveries are going to emerge. Although this literature review is based on few available sources, it provides insightful and novel concepts related to Industry 5.0 in the supply chain context. Moreover, it presents a clear set of constructs and a structured research agenda to encourage researchers in deploying further conceptual and empirical works linked to the subject herein explored. Organizations’ leadership, policymakers, and other practitioners involved in supply chains, and mainly those currently working with Industry 4.0 initiatives, can benefit from this research by having clear guidance regarding the dimensions needed to structurally design and implement an Industry 5.0 strategy. This article adds valuable insights to researchers and practitioners, by approaching the newest and revolutionary concept of the Industry 5.0 phenomenon in the supply chain context, which is still an unexplored theme.
Lean production and Industry 4.0: Strategy/management or technique/implementation? A systematic literature review
Evolution of industry 4.0 and international business: a systematic literature review and a research agenda, industry 4.0 data-related technologies and servitization: a systematic literature review, the applicability of industry 4.0 in geothermal drilling: a systematic literature review, industry 4.0 technologies as enablers of collaboration in circular supply chains: a systematic literature review, looking at energy through the lens of industry 4.0: a systematic literature review of concerns and challenges, organizational learning and industry 4.0: findings from a systematic literature review and research agenda.
PurposeIndustry 4.0 has been one of the most topics of interest by researches and practitioners in recent years. Then, researches which bring new insights related to the subjects linked to the Industry 4.0 become relevant to support Industry 4.0's initiatives as well as for the deployment of new research works. Considering “organizational learning” as one of the most crucial subjects in this new context, this article aims to identify dimensions present in the literature regarding the relation between organizational learning and Industry 4.0 seeking to clarify how learning can be understood into the context of the fourth industrial revolution. In addition, future research directions are presented as well.Design/methodology/approachThis study is based on a systematic literature review that covers Industry 4.0 and organizational learning based on publications made from 2012, when the topic of Industry 4.0 was coined in Germany, using data basis Web of Science and Google Scholar. Also, NVivo software was used in order to identify keywords and the respective dimensions and constructs found out on this research.FindingsNine dimensions were identified between organizational learning and Industry 4.0. These include management, Industry 4.0, general industry, technology, sustainability, application, interaction between industry and the academia, education and training and competency and skills. These dimensions may be viewed in three main constructs which are essentially in order to understand and manage learning in Industry 4.0's programs. They are: learning development, Industry 4.0 structure and technology Adoption.Research limitations/implicationsEven though there are relatively few publications that have studied the relationship between organizational learning and Industry 4.0, this article makes a material contribution to both the theory in relation to Industry 4.0 and the theory of learning - for its unprecedented nature, introducing the dimensions comprising this relation as well as possible future research directions encouraging empirical researches.Practical implicationsThis article identifies the thematic dimensions relative to Industry 4.0 and organizational learning. The understanding of this relation has a relevant contribution to professionals acting in the field of organizational learning and Industry 4.0 in the sense of affording an adequate deployment of these elements by organizations.Originality/valueThis article is unique for filling a gap in the academic literature in terms of understanding the relation between organizational learning and Industry 4.0. The article also provides future research directions on learning within the context of Industry 4.0.
Export Citation Format
Share document.

Predictive maintenance in the Industry 4.0: A systematic literature review
Citation Count
Industry 5.0: A survey on enabling technologies and potential applications
Industry 4.0 ten years on: a bibliometric and systematic review of concepts, sustainability value drivers, and success determinants, predictive maintenance and intelligent sensors in smart factory: review., integration of industry 4.0 technologies into total productive maintenance practices, data science applications for predictive maintenance and materials science in context to industry 4.0, systematic mapping studies in software engineering, industry 4.0: a survey on technologies, applications and open research issues, deep learning for iot big data and streaming analytics: a survey, an introduction to predictive maintenance, recent advances and trends in predictive manufacturing systems in big data environment, related papers (5), a systematic literature review of machine learning methods applied to predictive maintenance, machine learning and reasoning for predictive maintenance in industry 4.0: current status and challenges, big data and stream processing platforms for industry 4.0 requirements mapping for a predictive maintenance use case, machine learning in predictive maintenance towards sustainable smart manufacturing in industry 4.0, trending questions (1).
This paper provides a systematic literature review on predictive maintenance in the context of Industry 4.0, discussing methods, standards, and applications.
The influence of predictive maintenance in industry 4.0: A systematic literature review
Ieee account.
- Change Username/Password
- Update Address
Purchase Details
- Payment Options
- Order History
- View Purchased Documents
Profile Information
- Communications Preferences
- Profession and Education
- Technical Interests
- US & Canada: +1 800 678 4333
- Worldwide: +1 732 981 0060
- Contact & Support
- About IEEE Xplore
- Accessibility
- Terms of Use
- Nondiscrimination Policy
- Privacy & Opting Out of Cookies
A not-for-profit organization, IEEE is the world's largest technical professional organization dedicated to advancing technology for the benefit of humanity. © Copyright 2024 IEEE - All rights reserved. Use of this web site signifies your agreement to the terms and conditions.
The Use of Industry 4.0 Technologies in Maintenance: A Systematic Literature Review
- Conference paper
- First Online: 29 April 2023
- Cite this conference paper
- Safaa Essalih 11 ,
- Zineb El Haouat 11 ,
- Mohamed Ramadany 12 ,
- Fatima Bennouna 13 &
- Driss Amegouz 11
Part of the book series: Lecture Notes in Networks and Systems ((LNNS,volume 668))
Included in the following conference series:
- International Conference on Digital Technologies and Applications
606 Accesses
In the Industry 4.0 era, where competitiveness in the industrial sector is increasingly tough, maintenance optimization is an undeniable tool to stand out in this fierce context. To minimize costs, increase productivity, improve quality and facilitate decision-making in maintenance activities, companies are resorting to the deployment of digital technologies of the fourth industrial revolution, including the Internet of Things (IoT), Big Data, Additive Manufacturing (AM), Augmented Reality (AR), Cloud Computing, etc. The main goal of this paper is to assess the impact of Industry 4.0 in maintenance, to identify which technologies are used by companies in maintenance, what are the reasons that push companies to use these tools, and what are their benefits.
This is a preview of subscription content, log in via an institution to check access.
Access this chapter
- Available as PDF
- Read on any device
- Instant download
- Own it forever
- Available as EPUB and PDF
- Compact, lightweight edition
- Dispatched in 3 to 5 business days
- Free shipping worldwide - see info
Tax calculation will be finalised at checkout
Purchases are for personal use only
Institutional subscriptions
Rødseth, H., Schjølberg, P., Marhaug, A.: Deep digital maintenance. Adv. Manufact. 5 (4), 299–310 (2017)
Article Google Scholar
Wee, D., Kelly, R., Cattel, J., Breunig, M.: Industry 4.0-how to navigate digitization of the manufacturing sector. McKinsey Company 58 , 7–11 (2015)
Google Scholar
Mosyurchak, A., Veselkov, V., Turygin, A., Hammer, M.: Prognosis of behaviour of machine tool spindles, their diagnostics and maintenance. MM Sci. J. 2017 (5), 2100–2104 (2017)
Nunes, D.S., Zhang, P., Silva, J.S.: A survey on human-in-the-loop applications towards an internet of all. IEEE Commun. Surv. Tutorials 17 (2), 944–965 (2015)
Gubbi, J., Buyya, R., Marusic, S., Palaniswami, M.: Internet of Things (IoT): A vision, architectural elements, and future directions. Futur. Gener. Comput. Syst. 29 (7), 1645–1660 (2013)
Kaisler, S., Armour, F., Espinosa, J. A., Money, W.: Big data: Issues and challenges moving forward. In: 2013 46th Hawaii International Conference on System Sciences, pp. 995–1004. IEEE (2013)
Ge, Z., Song, Z., Ding, S.X., Huang, B.: Data mining and analytics in the process industry: The role of machine learning. IEEE Access 5 , 20590–20616 (2017)
Peres, R. S., Rocha, A.D., Leitao, P., Barata, J.: IDARTS–Towards intelligent data analysis and real-time supervision for industry 4.0. Comput. industry 101 , 138–146 (2018)
Chong, S., Pan, G. T., Chin, J., Show, P. L., Yang, T.C.K., Huang, C.M.: Integration of 3D printing and Industry 4.0 into engineering teaching. Sustainability 10 (11), 3960 (2018)
Neto, P.: Demystifying cloud computing. In: Proceeding of Doctoral Symposium on Informatics Engineering, vol. 24, pp. 16–21 (2011)
Marilungo, E., Papetti, A., Germani, M., Peruzzini, M.: From PSS to CPS design: a real industrial use case toward Industry 4.0. Procedia Cirp 64 , 357–362 (2017)
Figueiredo, M. J., Cardoso, P. J., Gonçalves, C. D., Rodrigues, J.M.: Augmented reality and holograms for the visualization of mechanical engineering parts. In: 2014 18th International Conference on Information Visualisation. pp. 368–373. IEEE (2014)
Sadik, A.R., Urban, B.: An ontology-based approach to enable knowledge representation and reasoning in worker–cobot agile manufacturing. Future Internet 9 (4), 90 (2017)
Chua, C. K., Leong, K.F., Lim, C.S.: Rapid prototyping: principles and applications (with companion CD-ROM). World Scientific Publishing Company (2010)
Wells, L.J., Camelio, J.A., Williams, C.B., White, J.: Cyber-physical security challenges in manufacturing systems. Manufact. Lett. 2 (2), 74–77 (2014)
Mayiring, P.: Qualitative content analysis. Companion Qualitative Res. 1 , 159–176 (2004)
Jónasdóttir, H., Dhanani, K., McRae, K., Mehnen, J.: Upgrading legacy equipment to industry 4.0 through a cyber-physical interface. In: IFIP International Conference on Advances in Production Management Systems, pp. 3–10 (2018)
Tortorella, G. L., Silva, E., Vargas, D.: An empirical analysis of total quality management and total productive maintenance in industry 4.0. In: Proceedings of the International Conference on Industrial Engineering and Operations Management, pp. 742–753 (2018)
Caterino, M., Fera, M., Macchiaroli, R., Manco, P.A.: Maintenance policy selection method enhanced by industry 4.0 technologies. In: Proceedings of the Summer School Francesco Turco, vol. 1, pp. 60 - 66 24 (2019)
Burdack, M., Rössle, M.: A concept of an interactive web-based machine learning tool for individual machine and production monitoring. In: Intelligent Decision Technologies, pp. 183–193 (2019)
Justus, A.D.S., Ramos, L.F.P., Loures, E.D.F.R.: A capability assessment model of industry 4.0 technologies for viability analysis of poc (proof of concept) in an automotive company. In: Transdisciplinary Engineering Methods for Social Innovation of Industry 4.0, pp. 936–945 (2018)
Di Bona, G., Cesarotti, V., Arcese, G., Gallo, T.: Implementation of Industry 4.0 technology: New opportunities and challenges for maintenance strategy. Procedia Comput. Sci. 180 , 424–429 (2021)
Onur, K. O. C. A., Kaymakci, O.T., Mercimek, M.: Advanced predictive maintenance with machine learning failure estimation in industrial packaging robots. In: 2020 International Conference on Development and Application Systems (DAS), pp. 1–6 (2020)
Di Capaci, R.B., Scali, C., Vallati, C., Anastasi, G.: A technological demonstrator for cloud-based performance monitoring and assessment of industrial plants: present architecture and future developments. IFAC-PapersOnLine 53 (2), 11656–11661 (2020)
Di Carlo, F., Mazzuto, G., Bevilacqua, M., Ciarapica, F. E. :Retrofitting a process plant in an industry 4.0 perspective for improving safety and maintenance performance. Sustainability 13 (2), 646 (2021)
Forcina, A., Introna, V., Silvestri, A.: Enabling technology for maintenance in a smart factory: A literature review. Proc. Comput. Sci. 180 , 430–435 (2021)
Gallo, T., Santolamazza, A.: Industry 4.0 and human factor: How is technology changing the role of the maintenance operator?. Proc. Comput. Sci. 180 , 388–393 (2021)
Hardt, F., Kotyrba, M., Volna, E., Jarusek, R.: Innovative approach to preventive maintenance of production equipment based on a modified tpm methodology for industry 4.0. Appli. Sci. 11 (15), 6953 (2021)
Titmarsh, R., Harrison, R.: Automated leak testing for cylindrical cell automotive battery modules: enabling data visibility using industry 4.0. In: 2019 23rd International Conference on Mechatronics Technology (ICMT), pp. 1–4 (2019)
Aheleroff, S., et al.:IoT-enabled smart appliances under industry 4.0: A case study. Adv. Eng. Inf. 43 , 101043 (2020)
Aheleroff, S., Xu, X., Zhong, R. Y., Lu, Y.: Digital twin as a service (DTaaS) in industry 4.0: an architecture reference model. Adv. Eng. Inform. 47 , 101225 (2021)
Silvestri, L., Forcina, A., Introna, V., Santolamazza, A., Cesarotti, V. :Maintenance transformation through Industry 4.0 technologies: A systematic literature review. Comput. Industry 123 , 103335 (2020)
Chen, J., Gusikhin, O., Finkenstaedt, W., Liu, Y.N.: Maintenance, repair, and operations parts inventory management in the era of industry 4.0. IFAC-PapersOnLine 52 (13), 171–176 (2019)
Kerin, M., Pham, D. T. :A review of emerging industry 4.0 technologies in remanufacturing. J. Clean. Prod. 237 , 117805 (2019)
Wang, N., Ren, S., Liu, Y., Yang, M., Wang, J., Huisingh, D.: An active preventive maintenance approach of complex equipment based on a novel product-service system operation mode. J. Clean. Prod. 277 , 123365 (2020)
Vargas, D.G.M., Vijayan, K.K., Mork, O.J.: Augmented reality for future research opportunities and challenges in the shipbuilding industry: A literature review. Proc. Manuf. 45 , 497–503 (2020)
Nordal, H., El-Thalji, I.: Assessing the technical specifications of predictive maintenance: A case study of centrifugal compressor. Appl. Sci. 11 (4), 1527 (2021)
Butt, J.: A conceptual framework to support digital transformation in manufacturing using an integrated business process management approach. Designs 4 (3), 17 (2020)
Pech, M., Vrchota, J., Bednář, J.: Predictive maintenance and intelligent sensors in smart factory. Sensors 21 (4), 1470 (2021)
Tortorella, G.L., Fogliatto, F.S., Cauchick-Miguel, P.A., Kurnia, S., Jurburg, D.: Integration of Industry 4.0 technologies into total productive maintenance practices. Int. J. Production Econom. 240 , 108224 (2021)
Turner, C., Okorie, O., Emmanouilidis, C., Oyekan, J.: A digital maintenance practice framework for circular production of automotive parts. IFAC-PapersOnLine 53 (3), 19–24 (2020)
Giada, C.V., Rossella, P.: Barriers to Predictive Maintenance implementation in the Italian machinery industry. IFAC-PapersOnLine 54 (1), 1266–1271 (2021)
Wippel, M., Lucke, D., Jooste, J.L.: An industry 4.0 technology implementation model for rolling stock maintenance. Procedia CIRP 104 , 606–611 (2021)
Nardo, M. D., Madonna, M., Addonizio, P., Gallab, M.: A mapping analysis of maintenance in Industry 4.0. J. Appli. Res. Technol. 19 (6), 653–675 (2021)
Cortés-Leal, A., Del-Valle-Soto, C., Cardenas, C., Valdivia, L.J., Del Puerto-Flores, J.A.: Performance metric analysis for a jamming detection mechanism under collaborative and cooperative schemes in industrial wireless sensor networks. Sensors 22 , 178 (2021)
Drakaki, M., Karnavas, Y. L., Tziafettas, I.A., Linardos, V., Tzionas, P.: Machine learning and deep learning based methods toward industry 4.0 predictive maintenance in induction motors: State of the art survey. J. Industrial Eng. Manag. 15 (1), 31–57 (2022)
Turner, C., Okorie, O., Emmanouilidis, C., Oyekan, J.: Circular production and maintenance of automotive parts: An Internet of Things (IoT) data framework and practice review. Comput. Ind. 136 , 103593 (2022)
Cortés-Leal, A., Del-Valle-Soto, C., Cardenas, C., Valdivia, L.J., Del Puerto-Flores, J.A.: Performance metric analysis for a jamming detection mechanism under collaborative and cooperative schemes in industrial wireless sensor networks. Sensors 22 (1), 178 (2022)
Jasiulewicz-Kaczmarek, M., Antosz, K., Zhang, C., Waszkowski, R. :Assessing the barriers to industry 4.0 implementation from a maintenance management perspective-pilot study results. IFAC-PapersOnLine 55 (2), 223–228 (2022).
Guerroum, M., Zegrari, M., Elmahjoub, A.A., El Alaoui, A., Saadi, J.: The architecture of rotating machines condition-monitoring using model-based system engineering approach. Int. J. Signal Process. Anal. 5 (006) (2022)
Giliyana, S., Salonen, A., & Bengtsson, M. :Perspectives on smart maintenance technologies–a case study in large manufacturing companies. In: SPS 2022, pp. 255–266. IOS Press (2022)
Weerasekara, S., Lu, Z., Ozek, B., Isaacs, J., Kamarthi, S.: Trends in adopting industry 4.0 for asset life cycle management for sustainability: a keyword co-occurrence network review and analysis. Sustainability, 14(19), 12233 (2022)
Ras, E., Wild, F., Stahl, C., Baudet, A.: Bridging the skills gap of workers in Industry 4.0 by human performance augmentation tools: Challenges and roadmap. In: Proceedings of the 10th International Conference on PErvasive Technologies Related to Assistive Environments, pp. 428–432 (2017)
Download references
Author information
Authors and affiliations.
Higher School of Technology Sidi Mohammed Ben Abdellah University, 30050, Fez, Morocco
Safaa Essalih, Zineb El Haouat & Driss Amegouz
Faculty of Sciences and Techniques Sidi Mohammed, Ben Abdellah University, 30050, Fez, Morocco
Mohamed Ramadany
National School of Applied Sciences, Sidi Mohammed Ben Abdellah University, 30050, Fez, Morocco
Fatima Bennouna
You can also search for this author in PubMed Google Scholar
Corresponding author
Correspondence to Safaa Essalih .
Editor information
Editors and affiliations.
Ecole Nationale des Sciences Appliquées, Fez, Morocco
Saad Motahhir
Faculty of Sciences, Sidi Mohamed Ben Abdellah University, Fez, Morocco
Badre Bossoufi
Rights and permissions
Reprints and permissions
Copyright information
© 2023 The Author(s), under exclusive license to Springer Nature Switzerland AG
About this paper
Cite this paper.
Essalih, S., Haouat, Z.E., Ramadany, M., Bennouna, F., Amegouz, D. (2023). The Use of Industry 4.0 Technologies in Maintenance: A Systematic Literature Review. In: Motahhir, S., Bossoufi, B. (eds) Digital Technologies and Applications. ICDTA 2023. Lecture Notes in Networks and Systems, vol 668. Springer, Cham. https://doi.org/10.1007/978-3-031-29857-8_81
Download citation
DOI : https://doi.org/10.1007/978-3-031-29857-8_81
Published : 29 April 2023
Publisher Name : Springer, Cham
Print ISBN : 978-3-031-29856-1
Online ISBN : 978-3-031-29857-8
eBook Packages : Intelligent Technologies and Robotics Intelligent Technologies and Robotics (R0)
Share this paper
Anyone you share the following link with will be able to read this content:
Sorry, a shareable link is not currently available for this article.
Provided by the Springer Nature SharedIt content-sharing initiative
- Publish with us
Policies and ethics
- Find a journal
- Track your research
- Publications / Predictive maintenance approaches in industry 4.0: A systematic literature review
Predictive maintenance approaches in industry 4.0: A systematic literature review
The emergence of Industry 4.0 has heralded notable progress in manufacturing processes, utilizing sophisticated sensing and data analytics technologies to maximize efficiency. A vital component within this model is predictive maintenance, which is instrumental in ensuring the dependability and readiness of production systems. Nonetheless, the heterogeneous characteristics of industrial data present obstacles in realizing effective maintenance decision-making and achieving interoperability among diverse manufacturing systems. This paper addresses these obstacles by introducing a hybrid approach that harnesses the power of ontologies, machine learning techniques, and data mining to identify and predict potential anomalies in manufacturing processes. Our work concentrates on designing an intelligent system with standardized knowledge representation and predictive capacities. By bridging the semantic divide and enhancing interoperability, ontologies enable the amalgamation of various manufacturing systems, thereby optimizing maintenance decision-making in real-time. As demonstrated in the experimental results, this approach not only ensures system reliability but also fosters a seamless, integrated, and efficient production landscape.
Thank you for visiting nature.com. You are using a browser version with limited support for CSS. To obtain the best experience, we recommend you use a more up to date browser (or turn off compatibility mode in Internet Explorer). In the meantime, to ensure continued support, we are displaying the site without styles and JavaScript.
- View all journals
- My Account Login
- Explore content
- About the journal
- Publish with us
- Sign up for alerts
- Review Article
- Open access
- Published: 01 May 2024
Digital technologies for construction sustainability: Status quo, challenges, and future prospects
- Weisheng Lu 1 ,
- Jinfeng Lou 1 ,
- Benjamin Kwaku Ababio 1 ,
- Ray Y. Zhong 2 ,
- Zhikang Bao 3 ,
- Xiao Li 4 &
- Fan Xue 1
npj Materials Sustainability volume 2 , Article number: 10 ( 2024 ) Cite this article
Metrics details
- Civil engineering
- Environmental impact
The nexus between digital technologies (DTs) and sustainability in the built environment has attracted increasing research interest in recent years, yet understanding DT utilization and its impact on construction processes remains fragmented. To address this gap, this study conducts a systematic review of the construction sustainability literature to analyze and synthesize research findings on the application of DTs at various stages of the construction lifecycle. We undertake an in-depth content analysis of 72 articles, with findings revealing that prominent DTs for construction sustainability include building information modeling, the Internet of Things, big data, and artificial intelligence. We also identify that the application of DTs for sustainability across the construction lifecycle is clustered in four areas: namely (1) integration and collaboration; (2) optimization, simulation, and decision-making; (3) tracking, monitoring, and control; and (4) training. Based on existing knowledge gaps, future research opportunities are identified, including the development of integrated and interoperable systems, long-term performance and resilience, and advanced simulation and modeling techniques. This study contributes to the literature on construction digitalization by offering a complete overview of research investigations in relation to construction sustainability and identifying research crucial to advancing a DT-enabled sustainable built environment.
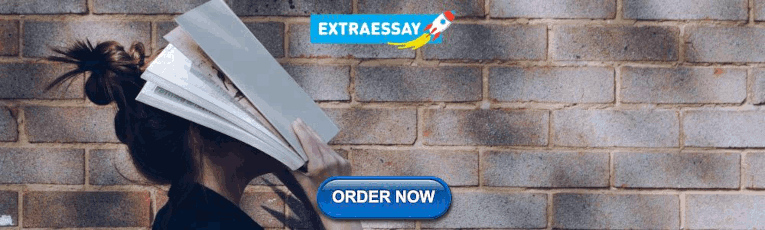
Introduction
The construction industry is not just about erecting buildings and infrastructure; it plays a vital role in economic and social development, contributing approximately USD200 billion to global GDP and providing over 220 million jobs 1 . The sector embodies a continuous lifecycle that integrates design, construction, operation & maintenance (O&M), and end of life, where each stage is interlinked and connected to the overall functionality and sustainability of the built environment 2 . The initial design sets the blueprint for sustainable and efficient use of resources 3 , 4 , and this design is materialized in the construction phase 5 . The O&M period extends over the longest phase of a structure’s life, emphasizing energy efficiency and environmental stewardship 6 . Finally, end-of-life is a critical component in the recycling and repurposing of materials 7 , 8 .
The construction industry has long been associated with significant environmental impacts, including resource consumption, waste generation, and carbon emissions 9 , 10 , 11 . It produces 45–65% of the waste dumped in landfills, contributing 35% of the world’s CO 2 emissions 12 . As the focus of the global community on sustainable development intensifies 13 , the construction sector faces an imperative to evolve. Sustainable practices meet the needs of the present without compromising the ability of future generations to meet their own needs 14 . ‘Construction sustainability’, therefore, is the implementation of such practices in construction industry activities 15 , encompassing three core dimensions: environmental protection, social responsibility, and economic viability 16 .
In responding to sustainability challenges, digital technologies (DTs) are a potentially transformative tool. DTs include information and communication technologies (ICTs) that manage information through digital binary computer language. In construction, they range from standalone systems to integrated and web-based technologies, which aid in data capture, storage, processing, display, and communication during various procurement stages. By streamlining processes, reducing waste, and enabling better decision-making, DTs promise to significantly advance the sustainability agenda in construction.
Despite growing recognition of their potential, the academic landscape reveals a fragmented understanding of DTs’ impact on sustainability in construction. Research studies vary in focus, methodology, and findings, making it challenging for industry stakeholders to form a cohesive view of the role of DTs in promoting sustainability. This fragmentation presents a critical barrier to effectively leveraging DTs for sustainable construction practices. To bridge this knowledge gap, this study conducts a systematic review of the construction sustainability literature. The goal is to compile, analyze, and synthesize research findings to present a clear, coherent picture of how DTs are being used to foster sustainability in construction. By critically examining the interplay between DT application and sustainable practices, we aim to identify successful strategies and highlight areas urging further exploration.
Prominent digital technologies in the construction sustainability literature
Reviewing the content of 72 articles in the construction sustainability literature, DTs frequently referenced and thoroughly examined were collected and organized in a Sankey diagram (see Fig. 1 ). Some DTs have received particular attention in the literature, e.g. building information modeling (BIM) and Internet of Things (IoT), while others have remained under-researched in relation to sustainability, e.g. unmanned aerial vehicles (UAV) and cloud computing. The diagram also reveals that DTs are employed for construction sustainability purposes mostly at the design and O&M stages of the construction lifecycle, typically as a standalone technology or paired with one other technology to achieve better performance.
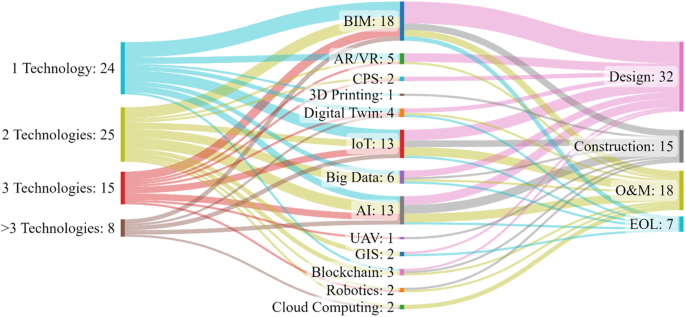
The papers reviewed are categorized according to the number of technologies, the name of the primary technology, and the stage at which the technologies are applied.
Figure 2 presents the distribution in the literature of key DTs for construction sustainability from 2020 to the present. BIM is the most frequently mentioned DT in the sustainability literature, with a peak of 10 research articles published between 2021 and 2022. The results indicate the continuation of a trend, with 17 identifying BIM as a top research theme in the DT-sustainability literature. This is attributable to the function of BIM as a collaborative platform and a means for integrating other DTs at different stages of the construction lifecycle. Studies relating BIM to sustainability in construction processes have seen a steady rise since the inception of BIM, with its application traversing design, construction, and O&M lifecycle stages. This suggests the vital role of BIM in improving sustainability through collaboration, visual representation, and optimization. IoT, with its use of sensors and actuators, has also been extensively discussed in the literature as essential to gathering sustainability-related data. Studies indicate the utilization of IoT in monitoring and controlling energy usage of buildings, optimizing resource use, and enhancing safety on site, all of which enable informed decisions on sustainability-related issues. Big data is also an important DT leveraging the vast amount of data generated throughout the project lifecycle by IoTs and other sources. There is a strong link between big data and IoT in the current body of knowledge, with both concepts often discussed together in optimization studies, environmental assessments, and risk and safety management. Artificial intelligence (AI) and machine learning (ML) in sustainability are gradually gaining momentum. Their ability to process huge amounts of heterogeneous data using computational power is vital for extracting insights into sustainable decision-making that effectively improves construction processes.
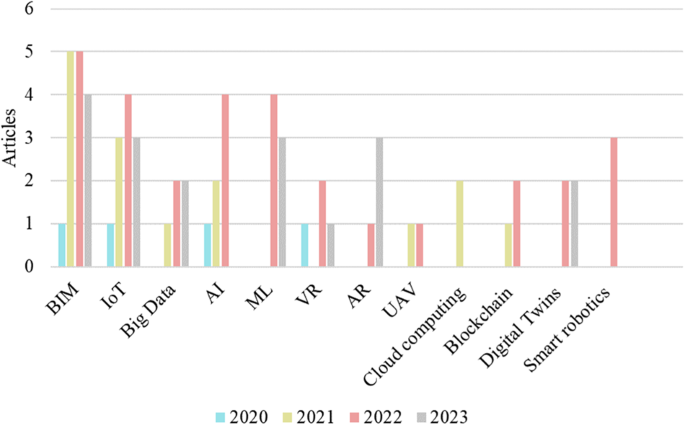
BIM is the most widely used technology for construction sustainability in recent years (2020–2023).
Other prominent DTs in construction sustainability are virtual reality (VR) and augmented reality (AR). Studies involving VR and AR cite their role in construction planning, where these DTs can be used for simulating interactive experiences and reviewing sustainability scenarios such as energy-efficient installations and user comfort. VR and AR technologies have been articulated in existing literature as early design optimization and visualization toolsets promoting a deeper understanding of sustainability features and fostering a sense of commitment towards sustainable outcomes. UAVs, or drones, are another type of DT increasingly used in construction, for surveying, inspection, and monitoring. In the construction sustainability literature, the application of UAVs has been discussed in hazard identification, safe work processes, and waste reduction. Cloud computing has also been identified as relevant to construction sustainability for its potential in providing centralized platforms for information management, communication, and collaboration throughout the project lifecycle. However, many recent studies are shifting their attention to blockchain technology as an enabler of construction sustainability, through providing transparent and immutable records and facilitating the integration of sustainable practices, such as material reuse and tracking. Little attention has been given to digital twins and cyber-physical systems (CPS) in sustainability research, though there has been an increasing trend in recent years for their potential to revolutionize construction sustainability by enabling real-time monitoring, optimization, and performance improvement throughout the project lifecycle.
Based on the results of our review of the literature, the DTs most closely associated with sustainability in the construction industry are BIM, IoT, AI, ML, VR, AR, UAV, robots, blockchain, and CPS. The overall growth in the application of these DTs for construction sustainably is likely to continue as the DT potential progressively permeates the entire lifecycle of construction products and services.
Application of digital technologies in construction sustainability
In examining the application of digital technologies to construction sustainability, it is crucial to analyze their impacts across different stages of the construction lifecycle: design, construction, O&M, and end of life.
In the design stage, recent literature indicates there is a growing nexus of DTs that enhance sustainability. Prominent examples include BIM in its 6D form, AR/VR, and AI for design. Six-dimensional BIM transcends traditional 3D modeling by integrating time (4D), cost (5D), and sustainability (6D) considerations, such as energy performance 18 , resource efficiency 19 , carbon emissions reduction 20 , and retrofit process simulation 21 . The emphasis is on the lifecycle approach 22 , where BIM informs decisions right from the design phase to reduce environmental impact and promote green building practices. AR/VR technologies have evolved to significantly improve design visualization and stakeholder engagement 23 , with discussions extending into the realm of the metaverse, which offers immersive spaces for interaction. The metaverse can benefit sustainability by facilitating the participation of the majority of people in decisions about how to utilize resources more efficiently 24 . AI, particularly in generative design 25 , is leveraged to create multiple sustainable design alternatives based on specific constraints and goals, focusing on material usage reduction 26 and energy efficiency optimization 27 .
The construction stage sees the critical role of blockchain, IoT, radio-frequency identification (RFID), digital twins, and UAVs in advancing sustainability. Blockchain’s potential lies in enhancing transparency and accountability in construction processes 28 , 29 , while smart contracts facilitate complex agreements and compliance with sustainability standards 30 . They can streamline procurement processes 31 , enhance supply chain management 32 , and facilitate more efficient project management 33 , 34 , leading to reduced waste and improved resource allocation. The role of IoT in sustainable construction is growing, with a focus on real-time data collection and monitoring 35 . IoT devices, such as sensors and wearables, are used to track resource utilization and environmental conditions 36 , thus aiding in optimizing resource use and reducing energy consumption 37 . RFID, one of the most powerful IoT technologies, has been extensively employed for effective logistics management, deemed crucial in reducing the carbon footprint of construction activities 38 by enabling accurate tracking of materials, tools, and equipment. RFID systems help in minimizing losses, reducing waste, and ensuring that materials are sourced and used sustainably. In addition, the integration of digital twins in the construction process represents a significant advancement. A digital twin is a virtual replica of a physical building that can be used for monitoring, simulation, and analysis 39 , 40 . Optimized construction efficiency is achievable through digital twins, which help streamline workflows, allocate resources efficiently, and monitor construction progress in real-time. This reduces the risks of delays and material waste by providing active and accurate information 41 . By creating a virtual representation of the construction project, digital twins enable a more dynamic and responsive approach to construction management, further enhancing sustainability. UAVs, commonly known as drones, are increasingly used in construction for various tasks such as site surveying, monitoring, and inspection. They provide a unique vantage point for overseeing construction activities, enabling better management of resources, and ensuring adherence to environmental and safety regulations 42 .
During the O&M phase, human–robot collaboration signifies a shift towards automation, enhancing efficiency and sustainability 43 . In this context, human–robot collaboration is not about replacing human workers, but rather enhancing their capabilities and safety 44 . Robots are used for tasks like cleaning, maintenance, surveillance, and inspection 45 , reducing the workload on human staff, increasing precision, and enhancing safety, particularly in hazardous environments. The concept of digital twins has also gained prominence in the O&M phase 46 . Data management and access post-construction can be complex due to the transition of control and operations among various stakeholders. This technology effectively bridges information gaps, ensuring reliable and convenient project management and facilitating enhanced communication among stakeholders 41 . Moreover, the digital twin provides a dynamic and real-time representation of the building and enables “what-if” analyses in occupant comfort, energy utilization, logistics optimization, asset management, and predictive maintenance, enhancing decision-making capabilities 47 , 48 , 49 . IoT devices and sensors, in addition, are widely used for continuous monitoring and data collection in buildings. This technology trend contributes significantly to the sustainability of facilities by enabling energy efficiency and predictive maintenance. Sensors collect data on aspects such as energy usage, temperature, and occupancy levels 50 , 51 , crucial for optimizing building operations and reducing unnecessary energy consumption 52 . To analyze the vast amount of data generated by IoT devices and other sources, AI and ML algorithms are increasingly applied. These technologies enable predictive maintenance, energy management, and anomaly detection, thereby enhancing the sustainability of building operations 53 , 54 .
At the end-of-life stage, DTs such as big data analytics, computer vision, robotics, and blockchain are key to managing deconstruction and waste processes. Big data analytics assists in material stock assessment, facilitating optimal material reuse and recycling, aligning with circular economy principles 55 , 56 . The role of computer vision in automating waste material sorting, combined with AI algorithms, enhances recycling efficiency 57 , 58 . Robotics in deconstruction focuses on safe material recovery 59 , 60 , while blockchain improves material traceability, ensuring informed decisions for material reuse and recycling 61 .
Prospects for digital technologies in construction sustainability
DTs incorporate several enhancements and modifications to construction value chain processes, increasing efficiency and productivity 19 . The opportunities for adopting these technologies to streamline sustainable production, construction, and operation in the industry are vast. From the analysis of the literature, we find that the driving themes in DT prospects for sustainability are linked with integration and collaboration 62 ; optimization, simulation, and decision-making 63 ; monitoring and control 64 ; and training 24 as presented in Fig. 3 .
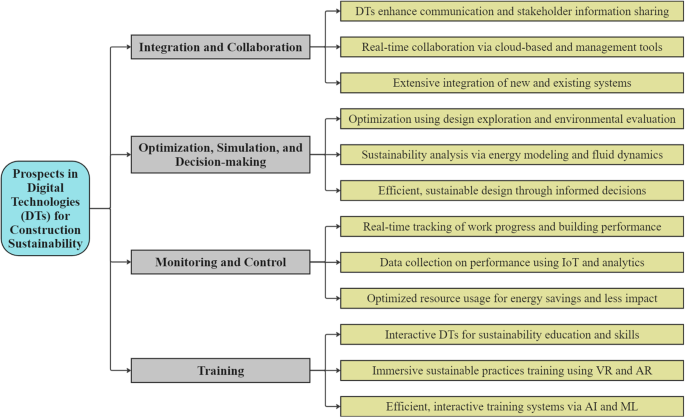
The potential of digital technologies can be explored in four areas, including integration and collaboration, optimization, simulation, and decision-making, monitoring and control, and training.
Integration and collaboration
The task of maintaining profitability while achieving cleaner, low-carbon construction processes, and greener and safer job sites, requires extensive stakeholder buy-in, involvement, and support 65 . To achieve this, there is a need to improve collaboration within and across different building lifecycles. DTs facilitate collaboration among stakeholders involved in the construction process by enabling effective communication and information sharing. According to 66 , cloud-based platforms, project management software, and collaborative tools allow real-time collaboration, document sharing, and coordination among architects, engineers, contractors, and sustainability consultants, among others. This collaboration enhances the integration of sustainability considerations into the project, leading to better-informed decisions and improved sustainability outcomes. Furthermore, DTs facilitate the integration of new and existing systems and processes to enhance sustainability outcomes. Integrating BIM models with energy modeling software, for instance, enables automated energy analysis and interference detection 21 . Integration with supply chain management systems allows for tracking and optimizing material usage, reducing waste, and promoting sustainable sourcing practices 67 . DTs provide a comprehensive approach to sustainability over the whole project lifecycle by combining various data sources and platforms.
Optimization, simulation and decision-making
Making informed decisions as early as feasible assists in efficient and cost-effective sustainable design and construction. Sustainability analysis tools, for example, enable better-informed decisions by analyzing numerous design options and identifying sustainable alternatives 68 , 69 . These evaluations assist professionals at various stages in determining the consequences of their building designs for environmental performance and efficiency. To improve sustainability, DTs enable optimization through the exploration of several design possibilities and evaluate their societal, environmental, and energy performance using BIM, ML, and parametric modeling. This aids in the identification of the most sustainable design solutions, decreasing material waste, energy usage, and environmental impacts 70 . Moreover, DTs provide simulation capabilities to assess the performance of buildings and infrastructure systems. For example, studies have incorporated energy modeling software to simulate and analyze energy consumption, daylighting, and thermal performance 18 . Others such as 71 have used computational fluid dynamics simulations to evaluate indoor air quality, ventilation effectiveness, and natural ventilation strategies. These simulations enable designers to make informed decisions, optimize sustainability performance, and identify potential issues before construction.
Monitoring and control
Some studies have explored mechanisms for monitoring sustainable aspects such as construction-related emissions using DTs that enable real-time tracking and monitoring of work progress and building performance 72 . Building management systems, IoT sensors, digital twins, and data analytics platforms collect data on energy consumption, indoor environmental quality, occupancy patterns, and equipment performance. The data helps identify areas of inefficiencies, pinpoint maintenance needs, and optimize resource usage, leading to energy savings, improved occupant comfort, and reduced environmental impact 73 .
For sustainability education and skill development, DTs provide interactive and immersive training alternatives. Immersive training experiences are made possible by VR, AR, and mixed-reality technologies, which allow users to simulate and implement sustainable construction practices, safety regulations, and energy-efficient operations 24 . The deployment of DTs enables safe demonstration and experimentation, promoting knowledge transfer and capacity building. Additionally, AI and ML platforms enable easy access to more efficient, responsive, and interactive train systems linked to sustainability 74 .
Digital technology application bottlenecks in construction sustainability
Deployment of DTs for construction sustainability faces several challenges across different stages of the project lifecycle (Fig. 4 ). First, accurate data and information are essential for conducting sustainability analysis during the design and planning stages of projects 75 . However, the present challenge of data quality and availability of construction products and services makes it difficult to fully benefit from the prospects of DT application. Data on material properties, energy consumption, and environmental impacts may not be readily available or standardized, making it difficult to assess sustainability performance accurately 76 . Standardized protocols, formats, and data exchange mechanisms that ensure consistent data quality and compatibility across different digital tools and platforms are still largely underdeveloped and unable to fully grasp all aspects of sustainability considerations in the construction industry. Interoperability of various DTs for holistic construction sustainability is missing within and across different lifecycle stages 77 . Within the same lifecycle stage, various digital tools and applications such as BIM, geographic information systems, energy modeling software, and sustainability analysis tools generate different types of data in varying formats and qualities 78 . Across different lifecycle stages, gathering real-time data from various sources, such as sensors and equipment, and integrating it into a unified system can be complex 79 . posit that the challenge lies in integrating these diverse datasets into a unified system that allows for comprehensive analysis and decision-making. Thus, ensuring data accuracy, reliability, and interoperability in different platforms and systems is crucial for enabling construction sustainability.
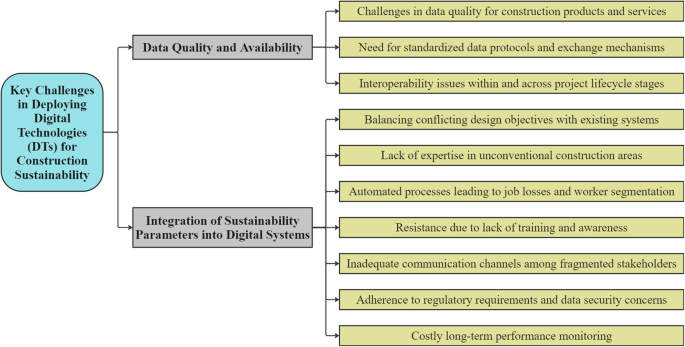
Two categories of challenges are identified: data quality and availability, and integration of sustainability parameters into digital systems.
Another key challenge is the integration of sustainability parameters into digital systems, including balancing conflicting design objectives and ensuring compatibility with already existing systems 80 . The deployment of DTs for sustainable construction requires expertise in certain unconventional areas of construction and given present inadequacies in the construction workforce, such expertise is hard to find 81 . point to many benefits of deploying DTs for construction sustainability, including lowered employment costs for organizations through the application of automated work processes. However, this can lead to significant job losses and worker segmentation, which may in turn result in resistance to change stemming from a lack of training, awareness, and involvement. There is a need to develop innovative and varied instructional approaches, but it is unclear how these challenges faced are being addressed in the present literature 80 . The introduction of both DTs and sustainable practices is nascent in the construction industry but is gaining momentum. Therefore, more conscious planning and capital investment are needed to enhance industry skills and manage behavior change with necessary measures that may completely deviate from existing supply chain operations. Moreover, at the core of DT for construction sustainability is the need for stakeholder collaboration and engagement 82 . Yet, given the vast and fragmented nature of stakeholders along a construction project’s lifecycle, including designers, contractors, facility managers, maintenance personnel, and occupants, it can be challenging to track and foster effective collaboration. This challenge stems from the absence of efficient communication channels, unwillingness to exchange and transmit knowledge and information, and failure by the relevant parties to cultivate proactive sustainability initiatives 83 . Other challenges are linked to adherence to regulatory requirements and data confidence, security, protection, and issues associated with the deployment of DTs 84 . Given the long-term performance monitoring of sustainability features and systems over a building or infrastructure’s lifespan, the need to build trust and efficient systems is critical. Ensuring that digital systems continue to operate optimally, maintaining modern sustainable practices during renovations or retrofits, and monitoring changes in building performance require continuous data collection, analysis, and stakeholder involvement, which can be onerous and costly 85 .
Future research trends and directions
The findings from this critical review of trending topics, prospects, challenges, and application of DTs in promoting construction sustainability offer a full picture of current research activities and reveal relevant gaps and future research needs. These future research needs represent the untapped potential identified from the analysis and evaluation of future works proposed in the existing literature and are explained below.
Limited attention has been paid to the integration of DTs for sustainability in construction 86 . Further research is required to delve into the development of standardized protocols, formats, and data exchange mechanisms to ensure consistent data quality and compatibility across different digital tools and platforms. This includes establishing guidelines for data collection, verification, and validation to enhance the accuracy and reliability of sustainability-related data.
An evolving trend receiving considerable attention has been the utilization of advanced simulation and modeling techniques 21 . Additional study is required to enhance simulation and modeling methodologies in order to achieve more accurate forecasting and analysis of sustainability results. This encompasses the enhancement of energy modeling software, the utilization of computational fluid dynamics simulations, and the implementation of multi-scale modeling techniques to accurately represent intricate interactions and maximize the utilization of resources.
The application of advanced DTs including ML and AI has stimulated conversations on their anticipated threat to social aspects of sustainability. Presently, AI/ML models are used to predict sustainability performance, optimize resource allocation, and identify patterns and trends to support informed decision-making 87 . Though the potential of AI/ML models is much greater, the present utilization of such DTs for sustainable construction is limited. Thus, future research needs to pay attention to their impact at full potential and how risk will be managed among participants in the construction value chain.
The circular economy is an emerging trend in sustainability in construction and would benefit immensely from the application of DT. There is a need to develop digital tools and frameworks that support the principles of the circular economy and material optimization 88 . This includes exploring methods for tracking and managing material flows, assessing the environmental impact of material choices, and optimizing material usage throughout the construction lifecycle 89 . Present research in such areas is still nascent.
An obvious and reoccurring problem in sustainability research is long-term performance and assessment 90 . Despite the application of DTs for sustainable construction, certain inherent challenges such as system resilience and adaptability persist. Therefore, future research may focus on the extended performance and resilience of sustainable construction projects supported by DTs. This could include studying the durability and adaptability of sustainable alternatives, evaluating the performance of digital monitoring systems over extended periods, and exploring the impact of climate change and other external factors on sustainability outcomes.
Studies that look into knowledge-sharing mechanisms and collaboration among stakeholders are crucial and should be promoted. As suggested by Balasubramanian et al. 91 , future research is needed to identify effective mechanisms for sharing best practices, case studies, and lessons learned in the application of DTs for construction sustainability. This can facilitate collective learning, foster innovation, and accelerate the adoption of sustainable practices.
Implications and conclusions
This study provides an overview of current applications of DTs in promoting construction sustainability and highlights opportunities for future research and innovation. It does so by adopting a systematic literature review and content analysis to identify DTs used in relation to construction sustainability. The findings reveal BIM, IoT, and big data to have become prominent technologies over the past 5 years, with digital twins and smart robotics emerging trends in DTs for construction sustainability. The major application areas of the identified DTs are integration and collaboration; optimization, simulation, and decision-making; monitoring and control; and training. Among these application areas, DTs have been extensively researched in optimization and monitoring construction infrastructure for sustainable outcomes. The study also identifies challenges associated with DTs for construction sustainability. Key among these are technical issues of data accuracy, security, and confidence; behavioral and cultural challenges such as resistance to change among stakeholders; and financial barriers due to the high cost of implementation.
The study’s contribution lies in the provision of a holistic perspective on DTs for construction sustainability across the entire construction lifecycle. Its theoretical contribution to the sustainable construction technologies framework is to identify gaps in the existing literature and propose new directions to enable efficient deployment and implementation. It also advances knowledge of how DTs can be applied in different construction scenarios to achieve sustainable outcomes. For industry and practice, the study provides insights into challenges faced in deploying DTs for construction sustainability, enabling practitioners to anticipate and manage risks ahead of implementation. Policymakers can be informed of the landscape of DTs and their application at various stages of construction. This can guide the development of tailored policies and regulations on the efficient advancement of DTs for achieving sustainability in the industry.
Despite these contributions, the study has a few limitations. The analysis is limited to a dataset obtained only from journals in the Web of Science (WoS) database, while the selection criteria and focus on only technical articles present some limitations. While these limitations present opportunities for future research to expand the study’s findings, further studies could also examine the evolution of DTs in the built environment and their connections with sustainable development.
Bibliographic materials collection and processing
Building on the practice of conducting literature reviews within the field of construction research 75 , 92 , this study seeks to elucidate the intersections of DT and construction sustainability through a systematic literature review (SLR), designed to ensure an objective and replicable synthesis of existing research 93 . Such a structured approach mitigates the reliance on simplistic judgment calls, curtails the incidence of subjective inclinations and errors, and upholds the integrity of scholarly research 94 .
The SLR uses the consolidated steps shown in Fig. 5 95 , 96 . First, it defines research objectives and conceptual boundaries to ensure a focused review; specifically, it targets the current and emergent trends of DTs in construction sustainability. Concurrently, conceptual boundaries are defined to delineate the scope of ‘digital technologies’, ‘sustainability’, and the ‘construction industry’. This dual step guarantees that the literature review remains focused and relevant. The second step involves a comprehensive search strategy and its execution. It encompasses selecting a suitable database, which, considering the nascent and interdisciplinary nature of the field, must be expansive. The WoS is apt for this purpose, encompassing a diverse range of scholarly works, including journal articles, conference proceedings, and more 97 . We use the WoS Core Collection, including SCl, SCI-Expanded, SSCl, A&HCI, and ESCI indices. Given the evolving terminology within DTs and sustainability in construction, a detailed search string with diverse terms is formulated and applied to the WoS database. The search is temporally bound to include articles published up to October 2023, ensuring contemporary relevance. It yields 790 articles for further processing. Subsequently, a manual exclusion process is applied, where articles are meticulously screened against predefined criteria. For instance, non-English papers, review articles, and studies that do not synergize sustainability with DT are excluded. A special plan of our SLR is the discussion of excluded papers, where an independent initial assessment by two researchers with at least 7 years of research experience is followed by a mutual discussion to reach a consensus on each paper’s exclusion. This phase ultimately narrowed down the selection to 72 articles. Following this, an independent data encoding and comparative analysis is conducted. To ensure the integrity and impartiality of data extraction, the two researchers independently read articles thoroughly and encoded data from each study. This data encompasses a variety of details, such as bibliographic information, methodologies, DTs examined, stages of concern, and their sustainability implications in construction. The independently derived datasets are compared to resolve discrepancies and to build a consensus on the themes and findings. This comparison fosters a rigorous synthesis of the literature, ensuring that the results are replicable and verifiable. Finally, an analytical synthesis is performed to summarize the current and potential future state of DT in construction sustainability.
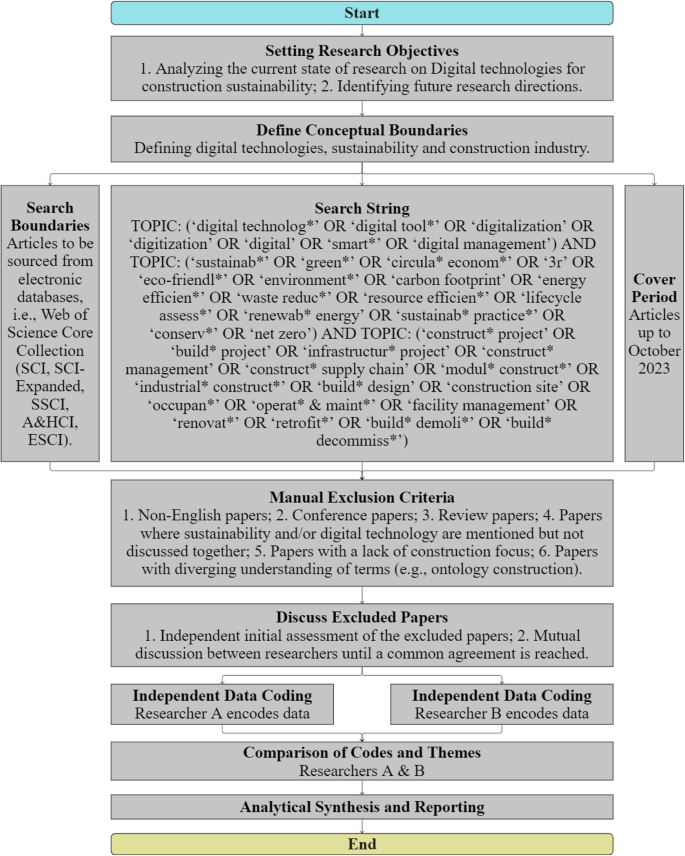
The review process follows a systematic approach.
Data availability
The datasets used and/or analyzed during the current study are available from the corresponding author on reasonable request.
Barbosa, F. et al. Reinventing Construction through a Productivity Revolution (McKinsey Global Institute, 2017).
Zhang, A., Yang, J. & Wang, F. Application and enabling technologies of digital twin in operation and maintenance stage of the AEC industry: a literature review. J. Build. Eng. 80 , 107859 (2023).
Article Google Scholar
Wang, J., Li, Z. & Tam, V. W. Critical factors in effective construction waste minimization at the design stage: a Shenzhen case study, China. Resour. Conserv. Recycl. 82 , 1–7 (2014).
Bao, Z., Laovisutthichai, V., Tan, T., Wang, Q. & Lu, W. Design for manufacture and assembly (DfMA) enablers for offsite interior design and construction. Build. Res. Inf. 50 , 325–338 (2022).
Sánchez-Garrido, A. J., Navarro, I. J. & Yepes, V. Multi-criteria decision-making applied to the sustainability of building structures based on modern methods of construction. J. Cleaner Prod. 330 , 129724 (2022).
Beccali, M., Bonomolo, M., Ciulla, G., Galatioto, A. & Brano, V. L. Improvement of energy efficiency and quality of street lighting in South Italy as an action of Sustainable Energy Action Plans. The case study of Comiso (RG). Energy 92 , 394–408 (2015).
Blengini, G. A. Life cycle of buildings, demolition and recycling potential: a case study in Turin, Italy. Build. Environ. 44 , 319–330 (2009).
Bao, Z. & Lu, W. A decision-support framework for planning construction waste recycling: a case study of Shenzhen, China. J. Clean. Prod. 309 , 127449 (2021).
Bao, Z., Lee, W. M. & Lu, W. Implementing on-site construction waste recycling in Hong Kong: barriers and facilitators. Sci. Total Environ. 747 , 141091 (2020).
Article CAS PubMed PubMed Central Google Scholar
Lu, W. et al. Estimating construction waste generation in the Greater Bay Area, China using machine learning. Waste Manag. 134 , 78–88 (2021).
Article PubMed Google Scholar
Sev, A. How can the construction industry contribute to sustainable development? A conceptual framework. Sustain. Dev. 17 , 161–173 (2009).
Lima, L., Trindade, E., Alencar, L., Alencar, M. & Silva, L. Sustainability in the construction industry: a systematic review of the literature. J. Clean. Prod. 289 , 125730 (2021).
Vinuesa, R. et al. The role of artificial intelligence in achieving the Sustainable Development Goals. Nat. Commun. 11 , 1–10 (2020).
Kuhlman, T. & Farrington, J. What is sustainability? Sustainability 2 , 3436–3448 (2010).
Shelbourn, M. et al. Managing knowledge in the context of sustainable construction. J. Inf. Technol. Constr. 11 , 57–71 (2006).
Google Scholar
Zavadskas, E. K., Šaparauskas, J. & Antucheviciene, J. Sustainability in construction engineering. Sustainability 10 , 2236 (2018).
Jin, R., Zou, Y., Gidado, K., Ashton, P. & Painting, N. Scientometric analysis of BIM-based research in construction engineering and management. Eng. Constr. Archit. Manag. 26 , 1750–1776 (2019).
Gnecco, V. M., Vittori, F. & Pisello, A. L. Digital twins for decoding human-building interaction in multi-domain test-rooms for environmental comfort and energy saving via graph representation. Energy Build. 279 , 112652 (2023).
Wong, J. K. W. & Zhou, J. Enhancing environmental sustainability over building life cycles through green BIM: aA review. Autom. Constr. 57 , 156–165 (2015).
Gan, V. J. et al. Holistic BIM framework for sustainable low carbon design of high-rise buildings. J. Clean. Prod. 195 , 1091–1104 (2018).
Mirpanahi, M. V. & Noorzai, E. Modeling the relationship between critical BIM attributes and environmental sustainability criteria using PLS-SEM technique. J. Archit. Eng. 27 , 04021037 (2021).
Motalebi, M., Rashidi, A. & Nasiri, M. M. Optimization and BIM-based lifecycle assessment integration for energy efficiency retrofit of buildings. J. Build. Eng. 49 , 104022 (2022).
Hajirasouli, A., Banihashemi, S., Drogemuller, R., Fazeli, A. & Mohandes, S. R. Augmented reality in design and construction: thematic analysis and conceptual frameworks. Constr. Innov. 22 , 412–443 (2022).
Allam, Z., Sharifi, A., Bibri, S. E., Jones, D. S. & Krogstie, J. The metaverse as a virtual form of smart cities: opportunities and challenges for environmental, economic, and social sustainability in urban futures. Smart Cities 5 , 771–801 (2022).
Buonamici, F., Carfagni, M., Furferi, R., Volpe, Y. & Governi, L. Generative design: an explorative study. Comput.-Aided Des. Appl. 18 , 144–155 (2020).
Alsakka, F. et al. Generative design for more economical and environmentally sustainable reinforced concrete structures. J. Clean. Prod. 387 , 135829 (2023).
Caldas, L. Generation of energy-efficient architecture solutions applying GENE_ARCH: an evolution-based generative design system. Adv. Eng. Inform. 22 , 59–70 (2008).
Xu, J., Lou, J., Lu, W., Wu, L. & Chen, C. Ensuring construction material provenance using Internet of Things and blockchain: learning from the food industry. J. Ind. Inf. Integr. 33 , 100455 (2023).
Lou, J. & Lu, W. Construction information authentication and integrity using blockchain-oriented watermarking techniques. Autom. Constr. 143 , 104570 (2022).
Salmerón-Manzano, E. & Manzano-Agugliaro, F. The role of smart contracts in sustainability: worldwide research trends. Sustainability 11 , 3049 (2019).
Li, J. & Kassem, M. Applications of distributed ledger technology (DLT) and blockchain-enabled smart contracts in construction. Autom. Constr. 132 , 103955 (2021).
Li, X. et al. Blockchain-enabled IoT-BIM platform for supply chain management in modular construction. J. Constr. Eng. Manag. 148 , 04021195 (2022).
Xu, J., Lu, W., Wu, L., Lou, J. & Li, X. Balancing privacy and occupational safety and health in construction: a blockchain-enabled P-OSH deployment framework. Saf. Sci. 154 , 105860 (2022).
Zhao, R., Chen, Z. & Xue, F. A blockchain 3.0 paradigm for digital twins in construction project management. Autom. Constr. 145 , 104645 (2023).
Rao, A. S. et al. Real-time monitoring of construction sites: Sensors, methods, and applications. Automation in Construction 136 , 104099 (2022).
Lu, W., Lou, J. & Wu, L. Combining smart construction objects-enabled blockchain oracles and signature techniques to ensure information authentication and integrity in construction. J. Comput. Civil Eng. 37 , 04023031 (2023).
Sajjad, M. et al. Evaluation of the success of industry 4.0 digitalization practices for sustainable construction management: Chinese construction industry. Buildings 13 , 1668 (2023).
Tao, X., Mao, C., Xie, F., Liu, G. & Xu, P. Greenhouse gas emission monitoring system for manufacturing prefabricated components. Autom. Constr. 93 , 361–374 (2018).
Zhao, R. et al. Comparison of BIM collaboration paradigms for digital twin readiness: centralized files, decentralized clouds and distributed blockchains. In Proc. Conference on CIB W78 (eds Kubicki, S. & Rezgui, Y.). 11–15 (International Council for Research and Innovation in Building and Construction (CIB), 2021).
Long, W., Bao, Z., Chen, K., Ng, S. T. & Wuni, I. Y. Developing an integrative framework for digital twin applications in the building construction industry: a systematic literature review. Adv. Eng. Inform. 59 , 102346 (2024).
Opoku, D. G. J., Perera, S., Osei-Kyei, R. & Rashidi, M. Digital twin application in the construction industry: a literature review. J. Build. Eng. 40 , 102726 (2021).
Hammad, A. W., da Costa, B. B., Soares, C. A. & Haddad, A. N. The use of unmanned aerial vehicles for dynamic site layout planning in large-scale construction projects. Buildings 11 , 602 (2021).
Wu, M., Lin, J. R. & Zhang, X. H. How human-robot collaboration impacts construction productivity: an agent-based multi-fidelity modeling approach. Adv. Eng. Inform. 52 , 101589 (2022).
Villani, V., Pini, F., Leali, F. & Secchi, C. Survey on human–robot collaboration in industrial settings: safety, intuitive interfaces and applications. Mechatronics 55 , 248–266 (2018).
Halder, S. & Afsari, K. Robots in inspection and monitoring of buildings and infrastructure: a systematic review. Appl. Sci. 13 , 2304 (2023).
Article CAS Google Scholar
Lu, Q., Xie, X., Parlikad, A. K., Schooling, J. M. & Konstantinou, E. Moving from building information models to digital twins for operation and maintenance. Proc. Institution of Civil Engineers-Smart Infrastructure and Construction. 174 , 46–56 (2020).
Yitmen, I., Alizadehsalehi, S., Akıner, İ. & Akıner, M. E. An adapted model of cognitive digital twins for building lifecycle management. Appl. Sci. 11 , 4276 (2021).
Petri, I., Rezgui, Y., Ghoroghi, A. & Alzahrani, A. Digital twins for performance management in the built environment. J. Ind. Inf. Integr. 33 , 100445 (2023).
Chen, J., Lu, W. & Lou, J. Automatic concrete defect detection and reconstruction by aligning aerial images onto semantic‐rich building information model. Comput.‐Aided Civil and Infrastruct. Eng. 38 , 1079–1098 (2023).
Wang, C. et al. Integrated sensor data processing for occupancy detection in residential buildings. Energy Build. 237 , 110810 (2021).
Floris, A., Porcu, S., Girau, R. & Atzori, L. An IoT-based smart building solution for indoor environment management and occupants prediction. Energies 14 , 2959 (2021).
Nesa, N. & Banerjee, I. IoT-based sensor data fusion for occupancy sensing using Dempster–Shafer evidence theory for smart buildings. IEEE Internet Things J. 4 , 1563–1570 (2017).
Tien, P. W., Wei, S., Darkwa, J., Wood, C. & Calautit, J. K. Machine learning and deep learning methods for enhancing building energy efficiency and indoor environmental quality—a review. Energy AI 10 , 100198 (2022).
Sanzana, M. R., Maul, T., Wong, J. Y., Abdulrazic, M. O. M. & Yip, C. C. Application of deep learning in facility management and maintenance for heating, ventilation, and air conditioning. Autom. Constr. 141 , 104445 (2022).
Yuan, L., Lu, W., Xue, F. & Li, M. Building feature‐based machine learning regression to quantify urban material stocks: a Hong Kong study. J. Ind. Ecol. 27 , 336–349 (2023).
Lu, W., Long, W. & Yuan, L. A machine learning regression approach for pre-renovation construction waste auditing. J. Clean. Prod. 397 , 136596 (2023).
Lu, W., Chen, J. & Xue, F. Using computer vision to recognize composition of construction waste mixtures: a semantic segmentation approach. Resour. Conserv. Recycl. 178 , 106022 (2022).
Chen, J., Lu, W. & Xue, F. “Looking beneath the surface”: a visual-physical feature hybrid approach for unattended gauging of construction waste composition. J. Environ. Manag. 286 , 112233 (2021).
Lublasser, E., Hildebrand, L., Vollpracht, A. & Brell-Cokcan, S. Robot assisted deconstruction of multi-layered façade constructions on the example of external thermal insulation composite systems. Constr. Robot. 1 , 39–47 (2017).
Lee, S., Pan, W., Linner, T. & Bock, T. A framework for robot assisted deconstruction: process, sub-systems and modeling. In 32 nd ISARC: Proc. International Symposium on Automation and Robotics in Construction (ed. Fraser, S. J). (International Association for Automation and Robotics in Construction (IAARC), 2015).
Wu, L., Lu, W., Peng, Z. & Webster, C. A blockchain non-fungible token-enabled ‘passport’ for construction waste material cross-jurisdictional trading. Autom. Constr. 149 , 104783 (2023).
Li, L. et al. Sustainability assessment of intelligent manufacturing supported by digital twin. IEEE Access 8 , 174988–175008 (2020).
Akbarzadeh, O. et al. Heating–cooling monitoring and power consumption forecasting using LSTM for energy-efficient smart management of buildings: a computational intelligence solution for smart homes. Tsinghua Sci.Technol. 29 , 143–157 (2023).
Elnour, M. et al. Performance and energy optimization of building automation and management systems: towards smart sustainable carbon-neutral sports facilities. Renew. Sustain. Energy Rev. 162 , 112401 (2022).
Ababio, B. K., Lu, W. & Ghansah, F. A. Transitioning from green to circular procurement in developing countries: a conceptual framework for Ghana’s construction sector. Build. Res. Inf. 51 , 798–815 (2023).
Oke, A. E., Kineber, A. F., Alsolami, B. & Kingsley, C. Adoption of cloud computing tools for sustainable construction: a structural equation modeling approach. J. Facil. Manag. 21 , 334–351 (2023).
Charef, R. & Lu, W. Factor dynamics to facilitate circular economy adoption in construction. J. Clean. Prod. 319 , 128639 (2021).
Kavitha, R. J. et al. Improved Harris Hawks optimization with hybrid deep learning based heating and cooling load prediction on residential buildings. Chemosphere 309 , 136525 (2022).
Article CAS PubMed Google Scholar
Lou, J., Wang, B., Yuan, Z. & Lu, W. Willingness to pay for well-being housing attributes driven by design layout: evidence from Hong Kong. Build. Environ. 251 , 111227 (2024).
Qiu, J. et al. Design of an evaluation system for disruptive technologies to benefit smart cities. Sustainability 15 , 9109 (2023).
Bay, E., Martinez-Molina, A. & Dupont, W. A. Assessment of natural ventilation strategies in historical buildings in a hot and humid climate using energy and CFD simulations. J. Build. Eng. 51 , 104287 (2022).
Zhang, E., He, X. & Xiao, P. Does smart city construction decrease urban carbon emission intensity? Evidence from a difference-in-difference estimation in China. Sustainability 14 , 16097 (2022).
Wei, Y., Lei, Z. & Altaf, S. An off-site construction digital twin assessment framework using wood panelized construction as a case study. Buildings 12 , 566 (2022).
Chen, Y., Huang, D., Liu, Z., Osmani, M. & Demian, P. Construction 4.0, Industry 4.0, and building information modeling (BIM) for sustainable building development within the smart city. Sustainability 14 , 10028 (2022).
Qi, B., Razkenari, M., Costin, A., Kibert, C. & Fu, M. A systematic review of emerging technologies in industrialized construction. J. Build. Eng. 39 , 102265 (2021).
Petrov, I. & Hakimov, A. Digital technologies in construction monitoring and construction control. In IOP Conference Series: Materials Science and Engineering , (ed. Sergeev, V.) 497, 012016 (IOP Publishing, 2019).
Yevu, S. K., Ann, T. W. & Darko, A. Digitalization of construction supply chain and procurement in the built environment: emerging technologies and opportunities for sustainable processes. J. Clean. Prod. 322 , 129093 (2021).
Meng, Q. et al. A review of integrated applications of BIM and related technologies in whole building life cycle. Eng. Constr. Archit. Manag. 27 , 1647–1677 (2020).
Malagnino, A. et al. Building information modeling and Internet of Things integration for smart and sustainable environments: a review. J. Clean. Prod. 312 , 127716 (2021).
Wang, M., Wang, C. C., Sepasgozar, S. & Zlatanova, S. A systematic review of digital technology adoption in off-site construction: current status and future direction towards industry 4.0. Buildings 10 , 204 (2020).
Love, P. E. & Matthews, J. The ‘how’ of benefits management for digital technology: from engineering to asset management. Autom. Constr. 107 , 102930 (2019).
Liao, L. & Teo, E. A. L. Managing critical drivers for building information modeling implementation in the Singapore construction industry: an organizational change perspective. Int. J. Constr. Manag. 19 , 240–256 (2019).
Durdyev, S., Ismail, S., Ihtiyar, A., Bakar, N. F. S. A. & Darko, A. A partial least squares structural equation modeling (PLS-SEM) of barriers to sustainable construction in Malaysia. J. Clean. Prod. 204 , 564–572 (2018).
Das, M., Luo, H. & Cheng, J. C. Securing interim payments in construction projects through a blockchain-based framework. Autom. Constr. 118 , 103284 (2020).
Aghimien, D. et al. Barriers to digital technology deployment in value management practice. Buildings 12 , 731 (2022).
Moshood, T. D., Adeleke, A. Q., Nawanir, G., Ajibike, W. A. & Shittu, R. A. Emerging challenges and sustainability of industry 4.0 era in the Malaysian construction industry. Emerg. Chall. Sustain. Ind. 4 , 1627–1634 (2020).
Pan, Y. & Zhang, L. Roles of artificial intelligence in construction engineering and management: a critical review and future trends. Autom. Constr. 122 , 103517 (2021).
Antwi-Afari, P. et al. Enhancing life cycle assessment for circular economy measurement of different case scenarios of modular steel slab. Build. Environ. 239 , 110411 (2023).
Bao, Z., Lu, W. & Hao, J. Tackling the “last mile” problem in renovation waste management: a case study in China. Sci. Total Environ. 790 , 148261 (2021).
Zhang, Y. et al. A survey of the status and challenges of green building development in various countries. Sustainability 11 , 5385 (2019).
Balasubramanian, S., Shukla, V., Islam, N. & Manghat, S. Construction industry 4.0 and sustainability: an enabling framework. IEEE Trans. Eng. Manag. 71 , 1–19 (2021).
Ibem, E. O. & Laryea, S. Survey of digital technologies in procurement of construction projects. Autom. Constr. 46 , 11–21 (2014).
Tranfield, D., Denyer, D. & Smart, P. Towards a methodology for developing evidence‐informed management knowledge by means of systematic review. Br. J. Manag. 14 , 207–222 (2003).
Petticrew, M. & Roberts, H. Systematic Reviews in the Social Sciences: A Practical Guide (John Wiley & Sons, 2008).
Holzmann, P. & Gregori, P. The promise of digital technologies for sustainable entrepreneurship: a systematic literature review and research agenda. Int. J. Inf. Manag. 68 , 102593 (2023).
Okoli, C. A guide to conducting a standalone systematic literature review. Commun. Assoc. Inf. Syst. 37 , 43 (2015).
Gusenbauer, M. & Haddaway, N. R. Which academic search systems are suitable for systematic reviews or meta‐analyses? Evaluating retrieval qualities of Google Scholar, PubMed, and 26 other resources. Res. Synth. Methods 11 , 181–217 (2020).
Article PubMed PubMed Central Google Scholar
Download references
Author information
Authors and affiliations.
Department of Real Estate and Construction, Faculty of Architecture, The University of Hong Kong, Pokfulam, Hong Kong
Weisheng Lu, Jinfeng Lou, Benjamin Kwaku Ababio & Fan Xue
Department of Industrial and Manufacturing Systems Engineering, The University of Hong Kong, Pokfulam, Hong Kong
Ray Y. Zhong
School of Energy, Geoscience, Infrastructure and Society, Heriot-Watt University, Edinburgh, UK
Zhikang Bao
Department of Civil Engineering, The University of Hong Kong, Pokfulam, Hong Kong
You can also search for this author in PubMed Google Scholar
Contributions
W.L., J.L., and B.A. conducted the review and wrote the manuscript. R.Z., Z.B., X.L., and F.X. made suggestions and revised the manuscript. All authors read and approved the final manuscript.
Corresponding authors
Correspondence to Jinfeng Lou or Benjamin Kwaku Ababio .
Ethics declarations
Competing interests.
The authors declare no competing interests.
Additional information
Publisher’s note Springer Nature remains neutral with regard to jurisdictional claims in published maps and institutional affiliations.
Rights and permissions
Open Access This article is licensed under a Creative Commons Attribution 4.0 International License, which permits use, sharing, adaptation, distribution and reproduction in any medium or format, as long as you give appropriate credit to the original author(s) and the source, provide a link to the Creative Commons licence, and indicate if changes were made. The images or other third party material in this article are included in the article’s Creative Commons licence, unless indicated otherwise in a credit line to the material. If material is not included in the article’s Creative Commons licence and your intended use is not permitted by statutory regulation or exceeds the permitted use, you will need to obtain permission directly from the copyright holder. To view a copy of this licence, visit http://creativecommons.org/licenses/by/4.0/ .
Reprints and permissions
About this article
Cite this article.
Lu, W., Lou, J., Ababio, B.K. et al. Digital technologies for construction sustainability: Status quo, challenges, and future prospects. npj Mater. Sustain. 2 , 10 (2024). https://doi.org/10.1038/s44296-024-00010-2
Download citation
Received : 18 December 2023
Accepted : 04 February 2024
Published : 01 May 2024
DOI : https://doi.org/10.1038/s44296-024-00010-2
Share this article
Anyone you share the following link with will be able to read this content:
Sorry, a shareable link is not currently available for this article.
Provided by the Springer Nature SharedIt content-sharing initiative
Quick links
- Explore articles by subject
- Guide to authors
- Editorial policies
Sign up for the Nature Briefing: Anthropocene newsletter — what matters in anthropocene research, free to your inbox weekly.

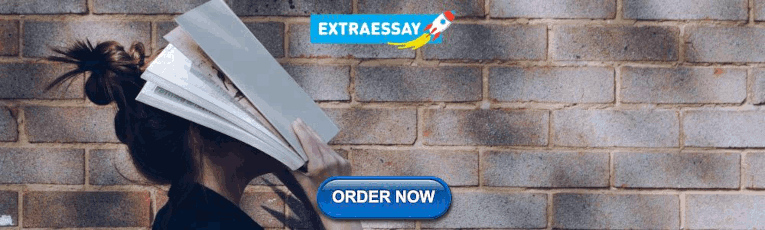
IMAGES
VIDEO
COMMENTS
Thereby, the literature review presented in this article aims to provide an overview of PdM in the context of Industry 4.0. We performed a systematic literature review methodology that resulted in a total of 47 articles on methods, architectures, and technologies aligned with the application of predictive maintenance.
Highlights. We review the state-of-the-art of predictive maintenance in the Industry 4.0. The methodology consisted of a systematic literature review of the area. We consider time-based approaches as the main challenge for predictive maintenance. We present a taxonomy for monitoring in the context of the Industry 4.0.
In this context, this article presents a systematic literature review of initiatives of predictive maintenance in Industry 4.0, identifying and cataloging methods, standards, and applications. As ...
2022. TLDR. An exhaustive literature review of methods and applied tools for intelligent predictive maintenance models in Industry 4.0 is presented by identifying and categorizing the life cycle of maintenance projects and the challenges encountered, and the models associated with this type of maintenance are presented. Expand.
A systematic literature review method was used to get evidence from the current knowledge linked to this theme. The results have evidenced a strong gap related to Industry 5.0 approaches for the supply chain field. Forty-one (41) publications, including conference and journal papers, have been found in the literature.
The advent of industry 4.0 (I4.0) has brought about significant advancements in manufacturing processes, leveraging advanced sensing and data analytics technologies to optimize efficiency. Within this paradigm, predictive maintenance (PdM) plays a crucial role in ensuring the reliability and availability of production systems. There are several existing approaches for PdM in I4.0, each with ...
Jain M, Vasdev D, Pal K, Sharma V (2022) Systematic literature review on predictive maintenance of vehicles and diagnosis of vehicle's health using machine learning techniques. Comput Intell 38(6):1990-2008. Publisher: Wiley Online Library ... Hain M (2022a) The influence of predictive maintenance in industry 4.0: a systematic literature ...
The article focuses on the topics: Predictive maintenance & Industry 4.0. read more. Overview Citations (322) References (64) Related Papers (5) Trending Questions (1) ... This paper provides a systematic literature review on predictive maintenance in the context of Industry 4.0, discussing methods, standards, and applications. ...
The Predictive maintenance has an ability to maintain in real time so it has an advantage over several features such as downtime, costs and production quality. This article is a systematic review of the literature on the study of the challenges of the Predictive maintenance and give a new classification of this field of research.
This paper presents an exhaustive literature review of methods and applied tools for intelligent predictive maintenance models in Industry 4.0 by identifying and categorizing the life cycle of maintenance projects and the challenges encountered, and presents the models associated with this type of maintenance: condition-based maintenance (CBM ...
Request PDF | Predictive Maintenance Approaches in Industry 4.0:A Systematic Literature Review | The advent of industry 4.0 (I4.0) has brought about significant advancements in manufacturing ...
In this context, this article presents a systematic literature review of initiatives of predictive maintenance in Industry 4.0, identifying and cataloging methods, standards, and applications.
Section snippets Literature background: an analysis of extant reviews. To identify the main research concepts and provide a theoretical context for our analysis, a brief discussion of existing maintenance-related and industry-specific review articles that primarily focus on Industry 4.0 PdM solutions was initially conducted (as a response to RQ#1).
The aim of this review was to evaluate and analyze the research conducted in the last decade on predictive maintenance based on Industry 4.0, with special consideration for small- and medium-sized enterprises. ... Hassankhani Dolatabadi, Sepideh, and Ivana Budinska. 2021. "Systematic Literature Review Predictive Maintenance Solutions for SMEs ...
Based on , the majority of firms perceive maintenance management as an early action in the implementation of Industry 4.0, with a significant move from breakdowns and regular maintenance to predictive maintenance policies to reach economic and technical benefits. This study intends to define which Industry 4.0 technologies are currently used by ...
An exhaustive literature review of methods and applied tools for intelligent predictive maintenance models in Industry 4.0 is presented by identifying and categorizing the life cycle of maintenance projects and the challenges encountered, and the models associated with this type of maintenance are presented. In the era of the fourth industrial revolution, several concepts have arisen in ...
Predictive maintenance plays an important role in managing commercial buildings. This article provides a systematic review of the literature on predictive maintenance applications of chilled water systems that are in line with Industry 4.0/Quality 4.0. The review is based on answering two research questions about understanding the mechanism of identifying the system's faults during its ...
The emergence of Industry 4.0 has heralded notable progress in manufacturing processes, utilizing sophisticated sensing and data analytics technologies to maximize efficiency. A vital component within this model is predictive maintenance, which is instrumental in ensuring the dependability and readiness of production systems. Nonetheless, the heterogeneous characteristics of industrial data ...
This chapter presents a systematic literature review (SLR) on Maintenance 4.0, with the aim of outlining the current achievements as well as limitations of maintenance meeting Industry 4.0 demands ...
The Influence of Predictive Maintenance in Industry 4.0: A Systematic Literature Review; Proceedings of the 2022 2nd International Conference on Innovative Research in Applied Science, Engineering and Technology, IRASET; Meknes, Morocco. 3-4 March 2022; New York, NY, USA: IEEE; pp. 1-13.
These technologies enable predictive maintenance, ... I. Y. Developing an integrative framework for digital twin applications in the building construction industry: a systematic literature review. ...
A systematic review of the literature is presented related to the usage of blockchain technology (BCT) for cyber-threats in the context of Industry 4.0, showing that the integration of BCT in industry can ensure data confidentiality and integrity and should be enforced to preserve data availability and privacy. Expand