Melanoma and Other Skin Cancers Research Results and Study Updates
See Advances in Melanoma and Other Skin Cancers Research for an overview of recent findings and progress, plus ongoing projects supported by NCI.
In an event more than three decades in the making, FDA has approved lifileucel (Amtagvi), the first cancer treatment that uses immune cells called tumor-infiltrating lymphocytes, or TILs.
People with desmoplastic melanoma, a rare form of skin cancer, are likely to benefit from treatment with a single immunotherapy drug, pembrolizumab (Keytruda), according to new results from a small clinical trial.
For melanoma that can be treated with surgery, a few doses of pembrolizumab (Keytruda) beforehand looks to be a good choice. In a clinical trial, people who got the presurgical immunotherapy were much less likely to have their cancer come back than those who only received it after surgery.
Male patients with metastatic melanoma don’t live as long as females, and their tumors are more likely to become resistant to commonly used treatments. A new study may help explain why: the androgen receptor.
Regular skin cancer screening leads to many diagnoses of very early-stage melanomas, results from a new study suggest. The results add to a debate about whether screening is fueling an overdiagnosis of melanoma in the United States.
The immunotherapy treatment, which combines the LAG-3 inhibitor relatlimab and PD-1 inhibitor nivolumab, becomes the first new immune checkpoint inhibitor approved in 8 years. Both drugs are given to patients via a single infusion to treat advanced melanoma.
Melanoma cells that travel to the brain produce their own amyloid beta, helping the cells survive and form metastases, a new study in mice shows. The Alzheimer’s-linked proteins appear to tamp down the brain’s immune response to the cancer cells.
NCI researchers have found that a diet rich in fiber may help some people being treated for melanoma respond to immunotherapy treatment by influencing the gut microbiome. The new findings come from an analysis of people with melanoma and mouse models of the disease.
Clinical trial finds that ipilimumab (Yervoy) and nivolumab (Opdivo) combo is superior to a combination of the targeted therapies dabrafenib (Tafinlar) and trametinib (Mekinist) as the first treatment for metastatic BRAF-positive melanoma.
Trial results show patients who received the immunotherapy pembrolizumab (Keytruda) after surgery to remove high-risk stage II melanomas were less likely to have the cancer come back than those who received no treatment after surgery.
People with advanced melanoma treated with two immunotherapy drugs—nivolumab (Opdivo) and a new drug called relatlimab—lived longer without their cancer getting worse than those treated only with nivolumab, results from a large clinical trial show.
While doctors are familiar with the short-term side effects of immune checkpoint inhibitors, less is known about potential long-term side effects. A new study details the chronic side effects of these drugs in people who received them as part of treatment for melanoma.
In a large trial, tebentafusp helped patients with uveal melanoma live longer than patients who received other treatments for the disease. Uveal melanoma is an aggressive cancer of the eye, and many patients do not survive for a year once it has spread.
For patients with cancers that do not respond to immunotherapy drugs, the use of fecal transplants to modify the gut microbiome may help some of these patients respond to the immunotherapy drugs.
Melanoma cells that pass through the lymphatic system before entering the bloodstream are more resistant to cell death and spread more readily than cells that enter the bloodstream directly. The finding could lead to new treatment approaches.
After rising steadily for decades, the number of people in the United States who die each year from the skin cancer melanoma has dramatically dropped in recent years, results from a new study show. Learn what has contributed to the dramatic decline.
Melanoma cells that metastasize to other parts of the body produce high levels of a protein called MCT1, a new study in mice has found. Blocking MCT1 with an investigational drug, AZD3965, led to fewer and smaller metastatic tumors.
Researchers have developed a device that uses lasers and sound waves to scan circulating blood for melanoma cells. In a small study, the device accurately detected and reduced the amount of cancer cells in participants’ blood.
FDA has approved pembrolizumab (Keytruda) to treat people with Merkel cell carcinoma, a rare and deadly form of skin cancer. The approval covers use of the drug to treat locally advanced or metastatic forms of the disease.
The Food and Drug Administration approved the immunotherapy drug cemiplimab (Libtayo) for an advanced form of cutaneous squamous cell carcinoma (SCC), a common type of skin cancer. It is the first agent to be approved specifically for advanced SCC.
Results from a clinical trial show that the combination of nivolumab (Opdivo) and ipilimumab (Yervoy) halted the growth of or shrank metastatic brain tumors in more than half of participants with melanoma that had spread to the brain.
In a new study, NCI-led researchers developed a gene expression predictor that can indicate whether melanoma in a specific patient is likely to respond to treatment with immune checkpoint inhibitors, a type of immunotherapy.
A new study has linked age with how well patients with melanoma responded to treatment with immune checkpoint inhibitors. Experiments in mice suggested that the response pattern may be due to an age-related shift in the kinds of immune cells in tumors.
FDA recently approved the targeted-drug combination to treat patients with advanced melanoma and a subset of patients with a rare and aggressive form of thyroid cancer whose tumors have a specific mutation in the BRAF gene.
A new study suggests that patients with a rare form of melanoma, called desmoplastic melanoma, may be particularly likely to benefit from treatments known as immune checkpoint inhibitors. An NCI-sponsored clinical trial is already testing one such drug in patients with this cancer.
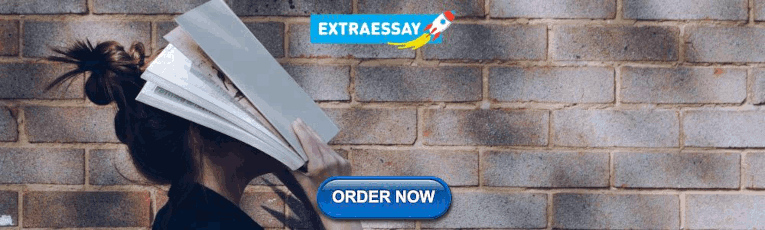
Melanoma Mirage: Unmasking Skin Cancer with Deep Learning
Ieee account.
- Change Username/Password
- Update Address
Purchase Details
- Payment Options
- Order History
- View Purchased Documents
Profile Information
- Communications Preferences
- Profession and Education
- Technical Interests
- US & Canada: +1 800 678 4333
- Worldwide: +1 732 981 0060
- Contact & Support
- About IEEE Xplore
- Accessibility
- Terms of Use
- Nondiscrimination Policy
- Privacy & Opting Out of Cookies
A not-for-profit organization, IEEE is the world's largest technical professional organization dedicated to advancing technology for the benefit of humanity. © Copyright 2024 IEEE - All rights reserved. Use of this web site signifies your agreement to the terms and conditions.
Cookies on this website
We use cookies to ensure that we give you the best experience on our website. If you click 'Accept all cookies' we'll assume that you are happy to receive all cookies and you won't see this message again. If you click 'Reject all non-essential cookies' only necessary cookies providing core functionality such as security, network management, and accessibility will be enabled. Click 'Find out more' for information on how to change your cookie settings.

- Accessibility
Ludwig Oxford looks back at Melanoma research in the Branch for Skin Cancer Awareness Month
Goding White
In May, Skin Cancer Awareness Month, Ludwig Oxford looks at recent research into Melanoma from Professors Richard White and Colin Goding
May is Skin Cancer Awareness month, and at Ludwig Oxford, multiples researchers focus on the different types of skin cancer. In particular, Professors Richard White and Colin Goding run research groups both with a focus towards melanoma research. The largest rick factor for developing Melanoma is exposure to ultraviolet light.
Colin Goding’s research lab uses melanoma as a model to study the role of the Microphthalmia-associated transcription factor (MITF) in microenvironment-driven phenotype-switching in melanoma biology. The team look to understand how MITF is regulated, and how it integrates microenvironmental signals to determine melanoma phenotype. They also look to determine the role of MITF-related factors in non-melanoma cancers. Their recent paper in Genes and Development shows that MITF plays a non-transcriptional role in shaping the DNA Damage Response programmes.
Richard White’s research lab also use melanoma as a model for their research into tumour initiation and development. By using a transgenic model of BRAFV600E-induced melanoma, the team have discovered that tumour initiation requires establishment of a permissive neural crest programme. The group ask why some developmental stages were more susceptible to BRAF, and have recently found that neural crest and melanoblast cells were more susceptible to BRAF compared to melanocytes. Richard has recently co-authored an editorial celebrating 20 years of progress in melanoma research in Pigment Cell Melanoma Research .
Further details of the research undertaken by the Goding and White research teams can be found in their respective research overviews.
Similar stories
Ludwig oxford at 2024 aacr.
17 April 2024
Ludwig Oxford staff and students alike share their research in San Diego at the 2024 AACR Annual Meeting
Melanoma Genomic Instability increased by DNA damage remodelling the MITF interactome
Cutaneous melanoma
Affiliations.
- 1 Melanoma Institute Australia, Sydney, NSW, Australia; Charles Perkins Centre, The University of Sydney, Sydney, NSW, Australia; Faculty of Medicine and Health, The University of Sydney, Sydney, NSW, Australia; Department of Medical Oncology, Royal North Shore Hospital, Sydney, NSW, Australia; Department of Medical Oncology, Mater Hospital, Sydney, NSW, Australia. Electronic address: [email protected].
- 2 Department of Dermatology and Pigmented Lesion and Melanoma Program, Stanford University Medical Center and Cancer Institute, Stanford, CA, USA; Department of Dermatology, VA Palo Alto Health Care System, Palo Alto, CA, USA.
- 3 Melanoma Institute Australia, Sydney, NSW, Australia; Faculty of Medicine and Health, The University of Sydney, Sydney, NSW, Australia; Department of Medical Oncology, Royal North Shore Hospital, Sydney, NSW, Australia; Department of Medical Oncology, Mater Hospital, Sydney, NSW, Australia.
- 4 Department of Surgical Oncology and Department of Cancer Biology, The University of Texas MD Anderson Cancer Center, Houston, TX, USA.
- 5 Melanoma Institute Australia, Sydney, NSW, Australia; Charles Perkins Centre, The University of Sydney, Sydney, NSW, Australia; Faculty of Medicine and Health, The University of Sydney, Sydney, NSW, Australia; Tissue Pathology and Diagnostic Oncology, Royal Prince Alfred Hospital, Sydney, NSW, Australia; NSW Health Pathology, Sydney, NSW, Australia.
- PMID: 37499671
- DOI: 10.1016/S0140-6736(23)00821-8
Cutaneous melanoma is a malignancy arising from melanocytes of the skin. Incidence rates are rising, particularly in White populations. Cutaneous melanoma is typically driven by exposure to ultraviolet radiation from natural sunlight and indoor tanning, although there are several subtypes that are not related to ultraviolet radiation exposure. Primary melanomas are often darkly pigmented, but can be amelanotic, with diagnosis based on a combination of clinical and histopathological findings. Primary melanoma is treated with wide excision, with margins determined by tumour thickness. Further treatment depends on the disease stage (following histopathological examination and, where appropriate, sentinel lymph node biopsy) and can include surgery, checkpoint immunotherapy, targeted therapy, or radiotherapy. Systemic drug therapies are recommended as an adjunct to surgery in patients with resectable locoregional metastases and are the mainstay of treatment in advanced melanoma. Management of advanced melanoma is complex, particularly in those with cerebral metastasis. Multidisciplinary care is essential. Systemic drug therapies, particularly immune checkpoint inhibitors, have substantially increased melanoma survival following a series of landmark approvals from 2011 onward.
Copyright © 2023 Elsevier Ltd. All rights reserved.
Publication types
- Lymph Node Excision
- Melanoma* / diagnosis
- Melanoma* / epidemiology
- Melanoma* / therapy
- Melanoma, Cutaneous Malignant
- Sentinel Lymph Node Biopsy
- Skin Neoplasms* / diagnosis
- Skin Neoplasms* / epidemiology
- Skin Neoplasms* / therapy
- Ultraviolet Rays
Thank you for visiting nature.com. You are using a browser version with limited support for CSS. To obtain the best experience, we recommend you use a more up to date browser (or turn off compatibility mode in Internet Explorer). In the meantime, to ensure continued support, we are displaying the site without styles and JavaScript.
- View all journals
- My Account Login
- Explore content
- About the journal
- Publish with us
- Sign up for alerts
- Open access
- Published: 28 April 2024
Early automated detection system for skin cancer diagnosis using artificial intelligent techniques
- Nourelhoda M. Mahmoud 1 &
- Ahmed M. Soliman 2
Scientific Reports volume 14 , Article number: 9749 ( 2024 ) Cite this article
58 Accesses
4 Altmetric
Metrics details
- Biomedical engineering
- Skin cancer
Recently, skin cancer is one of the spread and dangerous cancers around the world. Early detection of skin cancer can reduce mortality. Traditional methods for skin cancer detection are painful, time-consuming, expensive, and may cause the disease to spread out. Dermoscopy is used for noninvasive diagnosis of skin cancer. Artificial Intelligence (AI) plays a vital role in diseases’ diagnosis especially in biomedical engineering field. The automated detection systems based on AI reduce the complications in the traditional methods and can improve skin cancer’s diagnosis rate. In this paper, automated early detection system for skin cancer dermoscopic images using artificial intelligent is presented. Adaptive snake (AS) and region growing (RG) algorithms are used for automated segmentation and compared with each other. The results show that AS is accurate and efficient (accuracy = 96%) more than RG algorithm (accuracy = 90%). Artificial Neural networks (ANN) and support vector machine (SVM) algorithms are used for automated classification compared with each other. The proposed system with ANN algorithm shows high accuracy (94%), precision (96%), specificity (95.83%), sensitivity (recall) (92.30%), and F1-score (0.94). The proposed system is easy to use, time consuming, enables patients to make early detection for skin cancer and has high efficiency.
Similar content being viewed by others
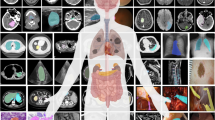
Segment anything in medical images
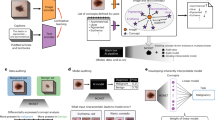
Transparent medical image AI via an image–text foundation model grounded in medical literature
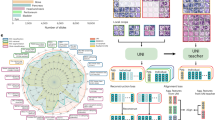
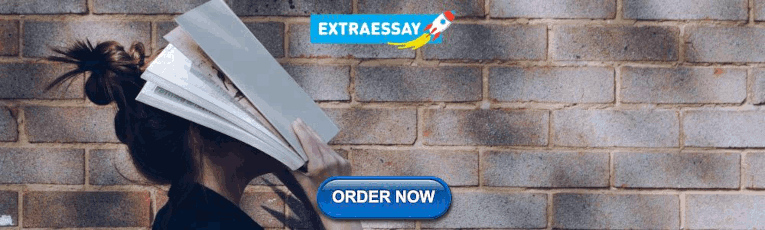
Towards a general-purpose foundation model for computational pathology
Introduction.
In the last decades, skin cancer is considered one of the most common and spread cancers around the world. It is extremely important to detect skin cancer in the early stage to reduce mortality 1 , 2 . The skin protects the body from infection, viruses such as coronavirus 3 , heat and dangerous UV radiation 4 , 5 , 6 . It also can store water and fat, maintain body temperature, and form vitamin D 7 , 8 . The estimation of the World Health Organization is recorded as approximately 132,000 skin melanoma cases per year 9 . Middle East has the highest skin cancer rates, Egypt has 1.52 rate, and its world rank is 117 10 .
Skin consists of two basic layers; the top layer is called epidermis which is made of flat cells called squamous cells. Basal cells are under the squamous cell, they are round cells. Among the basal cells, there are melanocytes cells which are responsible for pigment for skin color. Under the epidermis, there is dermis which contains blood cells and glands such as sweat gland 8 . Skin cancer is the abnormal growth of skin cells. This growth can be benign, or it can be malignant such as melanoma. Types of skin cancer are classified according to the type of cells that are subjected to cancer itself. Melanoma skin cancer, Basal skin cancer, and Squamous skin cancer are the most common types of skin cancer 11 , 12 . Melanoma skin cancer is the most fatal and dangerous type of skin cancer. It originated from melanocytes on the skin surface 13 , 14 . Basal skin cancer is originated from basal cells. Squamous skin cancer originated from Squamous cells 15 .
There are many methods to detect skin cancer. Traditional methods for skin cancer detection such as BIOPSY and naked eye 16 , 17 (visual inspection by dermatologists or general practitioners), have several challenges and limitations. BIOPSY is an invasive detection method, it is painful way and time-consuming method, it also may cause the disease to spread out 18 , 19 , 20 . In cases where a lesion appears suspicious, BIOPSY is required for definitive diagnosis, it may cause scarring, infection, and discomfort for the patient, also it can be expensive. The naked eye is another method where doctors use their eyes and experience to determine if there is a cancer or not. Visual inspection relies heavily on the expertise and subjective judgment of the healthcare provider. The same lesion may be interpreted differently by different practitioners, leading to variability in diagnosis. Errors can occur even when skilled professionals diagnose skin lesions. It can result in both overdiagnosis (identifying benign lesions as malignant) and underdiagnosis (missing malignant lesions), therefore, visual inspection method is a non-dependable way 11 , 21 , 22 , 23 . Inaccuracy in differentiating lesions is considered one of traditional methods limitations. Distinguishing between benign and malignant lesions based solely on visual inspection can be challenging, especially for lesions that exhibit atypical features or are in early stages of development. In addition, another limitation is accessibility to dermatologists or specialized healthcare providers in skin cancer diagnosis in many regions, especially in rural or underserved areas. This limitation can lead to delays in diagnosis and treatment. To overcome these limitations, there is ongoing research and development of technologies such as dermoscopy, teledermatology and computer aided diagnosis systems, which aim to improve the accuracy, efficiency, and accessibility of skin cancer detection. Dermoscopy technique is used for diagnosing skin cancer, it is a noninvasive skin imaging technique. It acquires a magnified image of a region of skin. It has higher accuracy than evaluation by naked eyes 22 , 24 .
Digital skin cancer microscopic images can be improved by Machine Learning (ML) 25 , 26 , 27 and Deep Learning (DL) 28 , 29 techniques. Artificial intelligence and adaptation of the technology to the human service are used for different diseases detection 30 , 31 , 32 , 33 , 34 . The computer-based detection systems can improve the diagnosis rate of skin cancer in comparison with the traditional methods. The computer aided diagnoses system is identifying the skin images and detects the skin cancer. Image segmentation, features extraction/selection and lesion classification are used for analyzing the automated dermoscopic images 30 , 35 , 36 .
The purpose of the proposed paper is implementing automated skin cancer detection system using dermoscopic images to identify benign and malignant skin lesions using AI. In this paper, automated segmentation algorithms based on AS and RG algorithms are proposed and compared with each other. Automated classification algorithms based on ANN and SVM algorithms are proposed and compared with each other. ANN algorithm is employed to identify the most discriminative features for benign and malignant skin lesion classification to improve classification accuracy. System efficiency is evaluated using the following metrics: accuracy, specificity, precision, recall and F1-score are presented. The proposed system is easy to use, time consuming and enables patients to make early detection for skin cancer. GUI for this system is implemented.
Related work
There are many researches in automatic detection for skin cancer with different methods and techniques. In this section, the detailed analysis of related work, including preprocessing, segmentation, and classification is illustrated.
Kang Hao Cheong et al. 23 proposed an automated skin melanoma detection system with melanoma-index based on entropy features. The system used image pre-processing, image enhancement, entropy and energy feature mining. 600 benign and 600 digital dermoscopy malignant images from benchmark databases were examined. The classification performance assessment with the combination of Support Vector Machine (SVM) and Radial Basis Function (RBF) offered a classification accuracy about 97.50%.
Lyer et al. 37 developed hybrid quantum mechanical system to classify cancerous and non-cancerous pigmented skin-lesions. The hybrid approach consisted of quantum classification and classical optimization using gradient descent methods. HAM10000 dataset was used, and the system achieved accuracies of 52% for training and 60% for validation.
Arora et al. 38 developed computer-aided detection and diagnosis systems for classifying a lesion into cancer or non-cancer. They proposed feature extractor and quadratic support vector machine for skin lesions classification. The PH 2 dataset was used, and the model achieved accuracy of 85.7%, sensitivity of 100%, specificity of 60% and training time of 0.8507 S.
Senan et al. 39 applied the ABCD (Asymmetry, Border, Color and Diameter) rules for automatic skin cancer detection. They used PH 2 standard dataset. Gaussian filter was applied to enhance the images. The contour method was applied for extracting the Region of Interest (RoI) from dermoscopy images. Morphology method was applied for increasing the quality of skin lesions. The ABCD rules were implemented for features extraction. Accuracy, specificity and sensitivity of the system were calculated. Accuracy was 84%, specificity was 89.50% and sensitivity was 60.50%.
Rajib Chakravorty et al. 40 introduced a system for improving asymmetry classification in PH 2 database using dermatologist-like feature extraction from skin lesion. Early diagnosis of melanoma was performed using asymmetry according to medical algorithms such as ABCD and CASH. They presented the performance of several classifiers using these features on PH 2 dataset. The obtained result shows better asymmetry classification than available literature. The results for full asymmetry using SVM were accuracy (81%), precision (63%), recall (62%), specificity (87%) and F1-score (0.87).
Alan Lima et al. 41 evaluated the melanoma diagnosis using deep features. Different classifiers were tested with the characteristic vectors extracted by the networks on the dataset PH 2 Database. Combination of the VGG19 network and Logistic Regression (LR) was applied. The results showed accuracy (92.5%), precision (85.71%), recall (75%), specificity (96.88%) and F1-score (0.80). When applying VGG19 network and SVM linear, the results were accuracy (90.5%), precision (81.82%), recall (67.50%), specificity (96.25%) and F1-score (0.7397).
Vasconcelos et al. 42 performed analysis of skin lesion images using principal axes-based asymmetry assessment for both dermoscopic and mobile acquired images. Two databases dermoscopic images set were used. The first is the CD-ROM Interactive Atlas of Dermoscopy and the second is the PH 2 database. For dermoscopic images, the developed methodology using SVM achieved high accuracy (83.1%), sensitivity (75.8%), specificity (88.1%), roc (82%). While, for mobile acquired images the accuracy reached 73.1%.
Barata et al. 43 developed two systems for the detection of melanomas in dermoscopy images using texture and color features. The best system was determined. Data set from Hospital Pedro Hispano Matosinhos was used. Both methods achieved very good results. Sensitivity was 96% and specificity was 80% for global methods using AdaBoost algorithm. Sensitivity was 100% and specificity was 75% for local methods using histogram and KNN algorithms.
Zhang et al. 44 developed an intelligent decision support system for skin cancer detection from dermoscopic images. The system was developed to identify malignant and benigin using genetic algorithm and SVM. Dermofit dataset was used. The results showed accuracy (88%), recall (83%) and specificity (89%).
Vasconcelos et al. 45 applied Deep Convolutional Neural Networks using small amount of data of ISBI dataset for melanoma detection. The results showed that the proposed approach improved the final classifier invariance for common melanoma variations, common skin patterns and markers, and dermatoscope capturing conditions. The results were accuracy (83.6%), precision (69.9%), recall (76%) and specificity (86.5%).
Lopez et al. 46 introduced deep learning techniques for skin lesion classification from ISIC dermoscopic images. The proposed system determined whether the lesion was a melanoma or a benign lesion. The system was built using the VGGNet convolutional neural network architecture and used the transfer learning paradigm. The results were accuracy (81.33%), precision (79.74%) and recall (78.66%).
Materials and methods
This paper proposed an intelligent system for skin cancer detection. The implemented system is developed to detect benign and malignant skin lesions. Multiple steps, including pre-processing, different methods for segmentation, features extraction/features selection, and different methods of classification are used for analyzing the automated dermoscopic images.
The dermoscopic images dataset used in this paper is from PH 2 Dataset 47 . This dataset is publicly available 47 . PH 2 Dataset is a widely used dataset in the field of dermatology and skin cancer detection. PH 2 dataset contains dermoscopic images that were obtained at the Dermatology Service of Hospital Pedro Hispano (Matosinhos, Portugal) under the same conditions through Tuebinger Mole Analyzer system using 20× magnification. The dermoscopic images are 8-bit RGB color images with a resolution of 768 × 560 pixels 47 . PH 2 Dataset is a widely used dataset in the field of dermatology and skin cancer detection. PH 2 dataset contains three types of skin diseases, they are Atypical Nevi, Melanoma and Common Nevus. This dataset includes 200 dermoscopic images (80 common nevus, 80 atypical nevi, and 40 melanomas). The dermoscopic images were selected randomly for training and test, as 80% for training and 20% for test. GUI for this study was implemented. The system configuration used for the proposed models was Intel Core i5 with processor 1.80 GHz and 8 GB of RAM. The system was implemented using MATLAB program. Figure 1 shows the flowchart of the implemented proposed system.
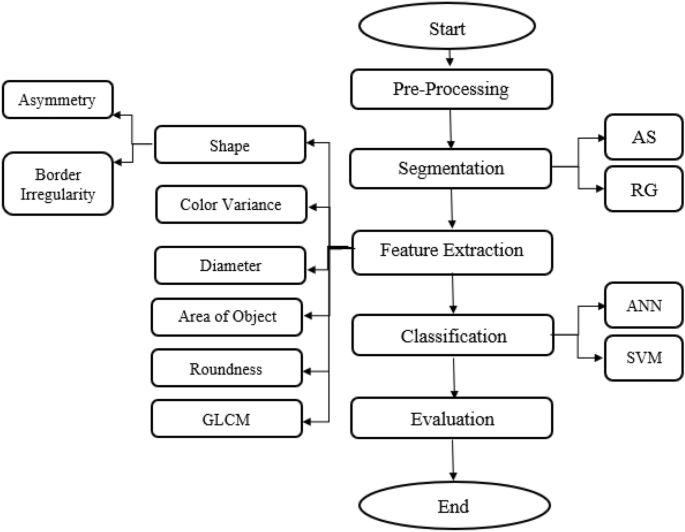
Flowchart for the implemented proposed system.
Pre-processing
Each image has several noises, therefore, the noise that appeared in the images should be eradicated for improving identification process. Pre-processing includes reading the image and applying sequence of hair removal filters using DullRazor algorithm 48 ; negative the image; enhancing the contrast of image; gray scale transformation; converting it to grayscale; noise filtering by median filter; and finding the negative of image by finding maximum of this image. The proposed system is characterized by using series of filters to enhance the contrast of the image. These filters are median, Gaussian and lee filters. Negative of enhanced image is applied. Smooth images are obtained after pre-processing step.
Segmentation
Segmentation is a technique to separate the objects from their background. It can adapt to the complex morphology of biological structures. In the step of segmentation, Adaptive snake and Region growing algorithms are used and modified.
In the segmentation process for skin cancer detection, AS and RG algorithms offer valuable advantages including efficiency, flexibility, incorporation of prior knowledge, adaptation to image variability, and exploitation of local homogeneity. The choice between these two algorithms is dependent on factors such as the complexity of the lesion, the quality of the input images, and computational resources available.
Segmentation using adaptive snake 49 includes finding the size of interested image; creating a mask of zeros by the same size of the interested image; assigning one value for a specific region in the created mask to snake around lesion; resizing all images to be the same; and applying the mask on the interested image. The result will be the interesting lesion.
AS algorithm is deformable model that can adapt to the contours of skin lesions. This flexibility enables AS to accurately identify irregularly shaped lesions, which is common in skin cancer cases. In addition, AS often incorporate prior knowledge about the shape and appearance of skin lesions, including color, texture, and gradient information. It can help improve the accuracy of segmentation by guiding the snake towards relevant features. Their shape and position based on the characteristics of the image can be adjusted, such as intensity gradients and edges. This flexibility makes AS robust to variations in image quality, lighting conditions, and skin types.
Segmentation using region growing 50 includes finding the size of interested image; specifying the lesion coordinates; determining the point form where the machine begin to grow by knowing X & Y Coordinates; applying opening and closing morphological methods.
RG starts from one or more seed points within the lesion and iteratively grows the region by adding adjacent pixels that satisfy certain criteria, such as similarity in intensity or color. This seed-based approach allows for the segmentation of lesions without requiring explicit boundary information. RG exploits the concept of local homogeneity within skin lesions, meaning that pixels within the lesion exhibit similar characteristics in terms of color, texture, or intensity. Therefore, even in the presence of noise or variations in illumination, RG algorithms can accurately segment lesions. In addition, it is computationally efficient and can produce segmentation results quickly, making them suitable for real-time or large-scale applications. Compared to other traditional image segmentation techniques, such as thresholding or clustering, RG offers unique characteristics and advantages including boundary sensitivity and it considers local pixel relationships and can capture fine-grained details and complex boundaries.
The evaluation of AS and RG algorithms is achieved using accuracy as evaluation metric. Accuracy measures the overall correctness of the segmentation results, considering both true positive and true negative pixels.
Features extraction
The next important step is deriving the features from the affected region. The extracted feature is reflection of the affected area information 51 .
Gray Level Co-occurrence matrix (GLCM) is used for features extraction. GLCM is a statistical method to examine and characterize the texture of the image. It is a second-order statistical texture analysis method. It is also known as the gray-level spatial dependence matrix 52 , 53 , 54 , 55 .
GLCM Parameters include Autocorrelation, Contrast, Correlation, Cluster-Prominence, Cluster Shade, Dissimilarity, Energy, Entropy, Homogeneity, Maximum probability, Sum of squares, Sum average, Sum variance, Sum entropy, Difference variance, Difference entropy, Information measure of correlation1, Information measure of correlation, Inverse difference normalized (INN), Inverse difference moment normalized 52 , 53 , 54 , 55 .
ABCDE rules of dermatology are used for features extraction. ABCDE rules are the commonly approved approaches for skin lesions detection. The selected features are shape including asymmetry and border irregularity, color variance, diameter, area of object, and roundness 39 , 56 , 57 .
ABCDE rules for features extraction are as the following:
Asymmetry: The lesion asymmetry was evaluated by calculating the area with inner and outer of the lesion, as follows 58 , 59 :
where, ASI is asymmetry index. ΔAK is the area between the two halves of the lesion and AL is lesion area.
Border irregularity: The edge of a malignant lesion usually exhibits four factors of interest, density, fractal dimension, radial variability and the extent to which its contour exhibits small irregularities. Border Irregularity is evaluated as follows 60 :
where, I denotes irregularity with a and b representing the lengths of major and minor axes of the lesions. P is the perimeter of the lesion and ΔA is the area of corresponding.
Color Variance
This feature helps in finding the variations in colors among several types of images by converting images from RGB to HSV 56 .
Area of Object
Lesion images can be classified by finding area of segmented lesion or area of interest. This can be done by converting image to black and white and segment lesion and find diameter from 'regionprops' 60 .
Lesion images can be classified by finding the diameter of segmented lesion or area of interest. This can be done by converting image to Black & White and segment lesion and find diameter from 'regionprops'. Diameter is evaluated as follows 46 , 51 :
Roundness of lesion can be calculated after finding area of object to distinguish common lesions from diseased one.
Roundness is evaluated as follows 60 :
Classification
ANN and SVM algorithms are used for classification of skin cancer. Classification includes specifying all image's features; entering input data and target data; applying validation and performance on it; and using train data and test data.
ANN is also known as Neural Networks. ANN are trained using a supervised learning approach. There are three types of computational nodes. There is input layer, nodes in it have a connection with the hidden layer. A typical ANN consists of multiple hidden layers. The number of input units connected to the hidden layer depends on the dataset. Output nodes produce the final output of the neural network after receiving the processed data from the hidden layers. The number of output nodes depends on the nature of the task that the network is designed for. ANN is particularly useful for skin cancer detection because ANN is excellent in learning complex representations from raw data. It can learn complex patterns and relationships from the input data, which is crucial for accurately classifying skin lesions.
SVM is used for classification, it is supervised learning model with associated learning algorithms that analyze data and recognize patterns, used for classification and regression analysis. SVM takes a set of input data and predicts, for each given input, which of two possible classes forms the output. SVM aims to find the hyperplane that best separates different classes in the input space. It works by mapping input data into a higher-dimensional feature space and finding the optimal separating hyperplane with the maximum margin between classes. SVM is effective in skin cancer detection because it can handle high-dimensional data and is robust to overfitting. SVM can also efficiently handle nonlinear relationships in the data by using kernel functions, allowing it to classify skin lesions accurately. SVM is widely used to classify digital dermoscope images 61 .
Both ANN and SVM offer advantages for skin cancer detection. By learning from a variety of training data, ANN can adjust to variations in imaging conditions, lesion characteristics, and patient demographics. In real clinical settings, this adaptability can enhance generalization performance. Compared to other ML models, SVM is less prone to overfitting, especially when using appropriate regularization techniques and kernel functions. This robustness is helpful in the detection of skin cancer, where the ability to generalize to new data is essential. SVM can perform well even with relatively small training datasets such as PH 2 dataset, making it suitable for skin cancer detection where collecting large amounts of labeled data may be challenging.
In the classification process of skin cancer detection, both ANN and SVM algorithms are typically trained using labeled datasets containing various features extracted from images of skin lesions, such as color, texture, and shape information. Once trained, these algorithms can classify new skin lesion images into different categories, such as benign or malignant, based on the learned patterns and relationships in the data. The choice between ANN and SVM depends on factors such as the size and complexity of the dataset, computational resources available, and the desired performance metrics. Both algorithms have demonstrated promising results in skin cancer detection and are actively used in research and clinical applications.
The accuracy of skin cancer detection depends on the efficiency of classification.
Evaluation metrics are very important in the development, validation, and deployment of skin cancer detection algorithms. They provide critical insights into algorithm performance, guiding improvements, supporting clinical decision-making, and ultimately contributing to better patient outcomes.
The efficiency of the proposed system is evaluated using the following metrics:
Accuracy: This measure records the correct and incorrect recognized samples of each class according to confusion matrix to evaluate the classification quality. A confusion matrix is a binary classification which is determined as TP: true positive, FP: false positive, FN: false negative, TN: the true negative amount(s). Accuracy is evaluated as follows 62 :
Sensitivity or Recall is called true positive rate and it measures the proportion of actual positives that are correctly identified as such (e.g., the percentage of sick people who are correctly identified as having the condition). Sensitivity is evaluated as follows 32 :
Specificity is called true negative rate and it measures the proportion of actual negatives that are correctly identified as such (e.g., the percentage of healthy people who are correctly identified as not having the condition). Specificity is evaluated as follows 63 :
Precision is called positive predictive value. It is the fraction of relevant instances among the retrieved instances. Precision is evaluated as follows 32 , 63 :
Dice or F1 Score is a measure of a test's accuracy. It considers both the precision and the recall of the test to compute the score. F1 score will be best value at 1 when the system achieves perfect precision and recall; and F1 will be worst at 0. F1 score is evaluated as follows 23 :
The Jaccard index is known as Intersection over Union (IoU) and the Jaccard similarity coefficient. It is a statistic used for comparing the similarity and diversity of sample sets. The Jaccard coefficient measures similarity between finite sample sets. It is defined as the size of the intersection divided by the size of the union of the sample sets. Jaccard is evaluated as follows 64 :
Matthews correlation coefficient (MCC) is calculated as follows 65 :
Results and discussion
In this section, the results obtained are presented. The results from the proposed methodology compared with the previously published research.
AS and RG algorithms are used for segmentation. Figure 2 shows segmentation using AS, while Fig. 3 shows segmentation using RG. Accuracy for AS is 96% while accuracy for RG is 90%.
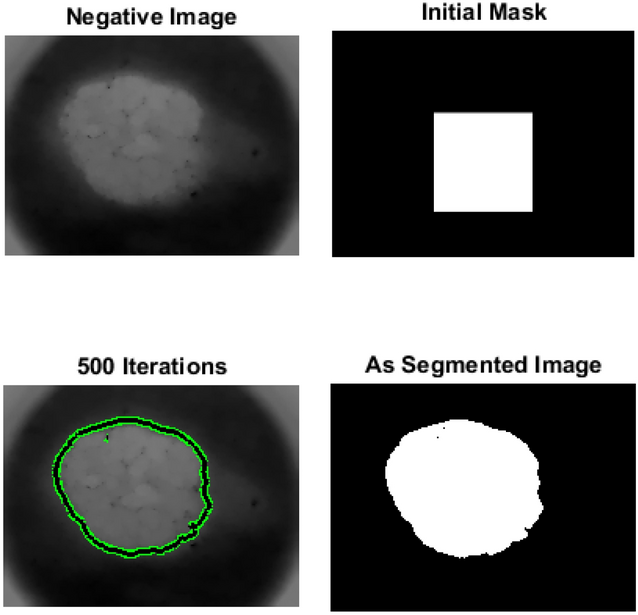
Adaptive snake segmentation.
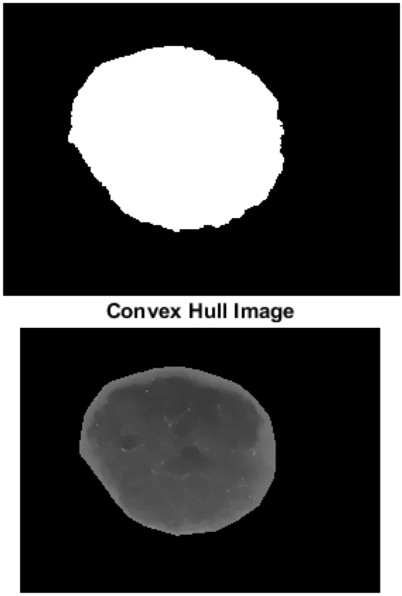
Region growing segmentation.
Results show that both AS and RG have their strengths in the segmentation process for skin cancer detection. AS is more efficient than RG due to its flexibility, ability to incorporate prior knowledge and ability to handle complex lesions. AS provides more precise boundaries compared to RG, especially for objects with irregular boundaries or complex shapes. AS is more suitable for a wider range of images. Compared to RG, AS optimizes the contour globally based on the entire image.
It is clear that, AS is simple, more accurate and segment specified region, but it needs to specify mask size. RG can segment image if it is symmetric, but it is slower than AS and it can’t segment image if it is Asymmetric.
Classification using NN and SVM
The classification results are presented in terms of seven metrics: precision, accuracy, sensitivity, specificity, F1 score, jaccard and MCC.
Figure 4 shows GUI for the proposed system. Table 1 summarizes the performances obtained by the proposed system in comparison with the results from literature review, including the methods used, datasets, algorithms and results achieved for the diagnosis of skin cancer dermatoscopic images. It is important to notice that a comparison would imply implementation of all methods and validation with the same dataset. However, it could be noticed that the obtained results are comparable with the best results in the literature.
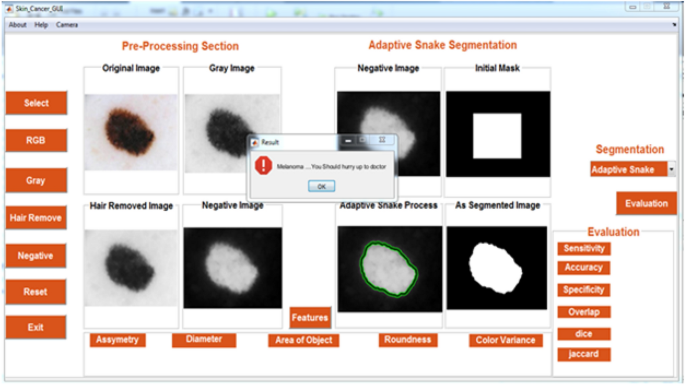
GUI for the proposed system.
As shown in Table 1 , the proposed system with ANN algorithm has maximum efficiency [accuracy (94%), precision (96%), specificity (95.83%), sensitivity (recall) (92.30%), and F1-score (0.94)] compared to the proposed system with SVM algorithm. Also, the proposed system with ANN algorithm has maximum efficiency compared to other research using PH2, Hospital Pedro Hispano (PH2), HAM10000, Dermofit, and ISIC datasets.
The proposed methodology outperforms the previously published result. Consistent classification performance in all the metrics across various classifiers indicates the suitability of the proposed features and methodologies.
Therefore, the proposed system is easy to use, time consuming, enables patients to monitor remotely and make early detection for skin cancer and has high efficiency. It also improves skin cancer’s diagnosis rate. Automated early detection system for skin cancer dermoscopic images using artificial intelligent accelerates the time of dermatologists and improves diagnosis performance.
In this work, automated early detection system for skin cancer dermoscopic images using artificial intelligent is presented. The proposed system accelerates the time of dermatologists and improves diagnosis performance. All the images in the PH 2 database are used, divided into 80 common nevus, 80 atypical nevi, and 40 melanomas images. The system was implemented using MATLAB program.
The proposed system is developed to detect benign and malignant skin lesions using multiple steps, including pre-processing, different methods for segmentation, features extraction/features selection, and different methods of classification are used for analyzing the automated dermoscopic images.
From the study of literature, it is concluded that various methods are employed for detecting skin cancer. The results show that AS is more accurate and efficient than RG algorithm. Accuracy for AS is 96% while accuracy for RG is 90%. Artificial Neural networks (ANN) and support vector machine (SVM) algorithms are used for automated classification which are applied and compared with each other. The proposed system with ANN classifier has high performances, accuracy (94%), precision (96%), specificity (95.83%), sensitivity (recall) (92.30%), and F1-score (0.94). The proposed system improves skin cancer’s diagnosis rate.
This study utilized two ML models (ANN and SVM); they offer advantages for skin cancer detection using the PH 2 dataset. ANN is excellent in learning complex representations from raw data and SVM provides robustness and efficiency. The implemented proposed system is efficient, accurate and easy to use by different users (doctors and patients). Early detection and diagnosis of skin cancer can lead to more successful treatment outcomes and potentially save lives, in addition, it can reduce the overall cost of treatment.
In the future work, the proposed system can be operated on a real-time diagnosis application after obtaining required approval and declarations. Additionally, more methods such as another ML models or deep learning models will be implemented aiming to enhance the performance level. Other available datasets can be used to test the suggested framework's ability to categorize skin cancer.
Data availability
The dermoscopic images dataset used in this paper is from PH 2 Dataset. This dataset is publicly available from the website: https://www.fc.up.pt/addi/ph2%20database.html .
Apalla, Z., Nashan, D., Weller, R. B. & Castellsagué, X. Skin cancer: Epidemiology, disease burden, pathophysiology, diagnosis, and therapeutic approaches. Dermatol. Ther. (Heidelb). 7 (Suppl 1), 5–19 (2017).
Article PubMed PubMed Central Google Scholar
Garcovich, S. et al. Skin cancer epidemics in the elderly as an emerging issue in geriatric oncology. Aging Dis. 8 (5), 643–661 (2017).
Yan, Y. et al. Consensus of Chinese experts on protection of skin and mucous membrane barrier for health-care workers fighting against coronavirus disease. Dermatol. Ther. 33 (4), e13310 (2020).
Article CAS PubMed PubMed Central Google Scholar
Bustamante, M. et al. Dose and time effects of solar-simulated ultraviolet radiation on the in vivo human skin transcriptome. Br. J. Dermatol. 182 (6), 1458–1468 (2020).
Article CAS PubMed Google Scholar
Nicolaï, M. P. J., Shawkey, M. D., Porchetta, S., Claus, R. & D’Alba, L. Exposure to UV radiance predicts repeated evolution of concealed black skin in birds. Nat. Commun. 11 , 2414–2421 (2020).
Article ADS PubMed PubMed Central Google Scholar
Linnér, A. et al. Immediate skin-to-skin contact is feasible for very preterm infants but thermal control remains a challenge. Acta Paediatr. 109 (4), 697–704 (2020).
Article PubMed Google Scholar
Bikle, D. & Christakos, S. New aspects of vitamin D metabolism and action—Addressing the skin as source and target. Nat. Rev. Endocrinol. 16 (4), 234–252 (2020).
Reichrath, J. Sunlight, Vitamin D and Skin Cancer . 1268 (Springer, 2020).
https://www.who.int/news-room/q-a-detail/radiation-ultraviolet-(uv)-radiation-and-skin-cancer . Accessed 27 Feb 2024.
https://www.worldlifeexpectancy.com/middle-east/skin-cancers-cause-of-death-female . Accessed 27 Feb 2024.
Jones, O. T., Ranmuthu, C. K. I., Hall, P. N., Funston, G. & Walter, F. M. Recognising skin cancer in primary care. Adv. Ther. 37 (1), 603–616 (2020).
Hamilton, W. et al. For which cancers might patients benefit most from expedited symptomatic diagnosis? Construction of a ranking order by a modified Delphi technique. BMC Cancer. 15 (1), 820 (2015).
Rangwala, S. & Tsai, K. Y. Roles of the immune system in skin cancer. Br. J. Dermatol. 165 (5), 953–965 (2011).
Leiter, U., Eigentler, T. & Garbe, C. Epidemiology of skin cancer. Adv. Exp. Med. Biol. 810 , 120–140 (2014).
PubMed Google Scholar
Lanoy, E. & Engels, E. A. Skin cancers associated with autoimmune conditions among elderly adults. Br. J. Cancer. 103 (1), 112–114 (2010).
Sreedhar, B., Swamy, M., Kumar, S. A comparative study of melanoma skin cancer detection in traditional and current image processing techniques. In Fourth International Conference on I-SMAC 654–658 (2020).
Andre, G. C. & Renato, A. The impact of patient clinical information on automated skin cancer detection. Comput. Biol. Med. 116 , 103545 (2020).
Article Google Scholar
Heibel, H. D., Hooey, L. & Cockerell, C. J. A review of noninvasive techniques for skin cancer detection in dermatology. Am. J. Clin. Dermatol. 21 (4), 513–524 (2020).
Ferris, L. K. et al. Utility of a noninvasive 2-gene molecular assay for cutaneous melanoma and effect on the decision to biopsy. JAMA Dermatol. 153 (7), 675–680 (2017).
Markowitz, O. et al. Evaluation of optical coherence tomography as a means of identifying earlier stage basal cell carcinomas while reducing the use of diagnostic biopsy. J. Clin. Aesthet. Dermatol. 8 (10), 14–20 (2015).
PubMed PubMed Central Google Scholar
Akilandasowmya, G., Nirmaladevi, G., Suganthi, S. U. & Aishwariya, A. Skin cancer diagnosis: Leveraging deep hidden features and ensemble classifiers for early detection and classification. Biomed. Signal Process. Control 88 , 105306 (2024).
Dinnes, J. et al. Visual inspection and dermoscopy, alone or in combination, for diagnosing keratinocyte skin cancers in adults. Cochrane Database Syst. Rev. 12 (12), CD011901 (2018).
Cheong, K. et al. An automated skin melanoma detection system with melanoma-index based on entropy features. Biocybern. Biomed. Eng. 41 (3), 997–1012 (2021).
Conforti, C. et al. Dermoscopy and the experienced clinicians. Int. J. Dermatol. 59 (1), 16–22 (2020).
Masood, M. et al. Multi-class skin cancer detection and classification using hybrid features extraction techniques. J. Med. Imaging Health Inform. 10 (10), 2466–2472 (2020).
Zghal, N. S. & Derbel, N. Melanoma skin cancer detection based on image processing. Curr. Med. Imaging. 16 (1), 50–58 (2020).
Rajinikanth, V., Satapathy, S. C., Dey, N., Fernandes, S. L. & Manic, K. S. Skin melanoma assessment using kapur’s entropy and level set’a study with bat algorithm. Smart Intell. Comput. Appl. Proc. Second Int. Conf. SCI. 1 , 193–202 (2019).
Google Scholar
Adegun, A. & Viriri, S. Deep learning techniques for skin lesion analysis and melanoma cancer detection: a survey of stateof- the-art. Artif. Intell. Rev. 54 , 1–31 (2020).
Albahar, M. A. Skin lesion classification using convolutional neural network with novel regularizer. IEEE Access. 7 , 38306–38313 (2019).
Goyal, M., Knackstedt, T., Yan, S. & Hassanpour, S. Artificial intelligence-based image classification methods for diagnosis of skin cancer: Challenges and opportunities. Comput. Biol. Med. 127 , 104065 (2020).
Mahmoud, N. M., Mahmoud, M. H., Alamery, S. & Fouad, H. Structural modeling and phylogenetic analysis for infectious disease transmission pattern based on maximum likelihood tree approach. J. Ambient Intell. Hum. Comput. 12 , 3479–3492 (2021).
Mahmoud, N. M., Fouad, H., Alsadon, O. & Soliman, A. M. Detecting dental problem related brain disease using intelligent bacterial optimized associative deep neural network. Cluster Comput. 23 , 1647–1657 (2020).
Alsiddiky, A., Fouad, H., Soliman, A. M., Altinawi, A. & Mahmoud, N. M. Vertebral tumor detection and segmentation using analytical transform assisted statistical characteristic decomposition model. IEEE Access. 8 , 145278–145289 (2020).
Mahmoud, N. M., Fouad, H. & Soliman, A. M. Smart healthcare solutions using the internet of medical things for hand gesture recognition system. Complex Intell. Syst. 7 , 1253–1264 (2021).
Han, S. S. et al. Deep neural networks show an equivalent and often superior performance to dermatologists in onychomycosis diagnosis: Automatic construction of onychomycosis datasets by region-based convolutional deep neural network. PLoS One. 13 (1), e0191493 (2018).
Mehtaa, P. & Shahb, B. Review on techniques and steps of computer aided skin cancer diagnosis. Proc. Comput. Sci. 85 , 309–316 (2016).
Iyer, V., Ganti, B., Hima Vyshnavi, A., Krishnan Namboori, P. & Iyer, S. Hybrid quantum computing based early detection of skin cancer. J. Interdiscip. Math. 23 (2), 347–355 (2020).
Arora, G., Dubey, A. K., Jaffery, Z. A. & Rocha, A. Bag of feature and support vector machine based early diagnosis of skin cancer. Neural Comput. Appl. 34 , 8385–8392 (2022).
Senan, E. M. & Jadhav, M. E. Analysis of dermoscopy images by using ABCD rule for early detection of skin cancer. Glob. Transit. Proc. 2 (1), 1–7 (2021).
Chakravorty, R., Sisi, L., Abedini, M., Garnavi, R. Dermatologist-like feature extraction from skin lesion for improved asymmetry classification in PH 2 database. Annu. Int. Conf. IEEE Eng. Med. Biol. Soc. 3855–3858 (2016).
Maia, L. B., Lima, A., Pinheiro, R. M., Junior, G. B., Dallyson, J., Paiva, A. C. Evaluation of melanoma diagnosis using deep features. In IEEE 25th International Conference on Systems Signals and Image Processing, IWSSIP 1–4 (2018).
Vasconcelos, M. J. M., Rosado, L. & Ferreira, M. Principal axes-based asymmetry assessment methodology for skin lesion image analysis. Adv. Vis. Comput. ISVC 2014 , 21–31 (2014).
Barata, C., Ruela, M., Francisco, M., Mendonça, T. & Marques, J. S. Two systems for the detection of melanomas in dermoscopy images using texture and color features. IEEE Syst. J. 8 (3), 965–979 (2014).
Article ADS Google Scholar
Tan, T. Y., Zhang, L., Jiang, M. An intelligent decision support system for skin cancer detection from dermoscopic images. In 12th International Conference on Natural Computation, Fuzzy Systems and Knowledge Discovery (ICNC-FSKD) . 2194–2199 (2016).
Vasconcelos, C. N. & Vasconcelos, B. N. Experiments using deep learning for dermoscopy image analysis. Pattern Recogn. Lett. 139 , 95–103 (2017).
Lopez, A. R., Giro, X., Burdick, J., Marques, O. Skin lesion classification from dermoscopic images using deep learning techniques. In 13th IASTED International Conference on . 49–54 (IEEE, 2017).
https://www.fc.up.pt/addi/ph2%20database.html . Accessed 27 Feb 2024.
Tim, L., Vincent, N., Richard, G., Andrew, C. & David, M. A software approach to hair removal from images. Comput. Biol. Med. 27 (6), 533–543 (1997).
Nascimento, J. C. & Marques, J. S. Adaptive snakes using the EM algorithm. IEEE Trans. Image Process. 14 (11), 1678–1686 (2005).
Article ADS PubMed Google Scholar
Jun, T. A color image segmentation algorithm based on region growing. In 2nd International Conference on Computer Engineering and Technology . V6-634–V6-637 (2010).
Cao, L., Lu, Y., Li, C., Yang, W. Automatic segmentation of pathological glomerular basement membrane in transmission electron microscopy images with random forest stacks. Comput. Math. Methods Med . 1684218 (2019).
Mohanaiah, P., Sathyanarayana, P. & GuruKumar, L. Image texture feature extraction using GLCM approach. Int. J. Sci. Res. Publ. 3 (5), 290–294 (2013).
Öztürk, Ş & Akdemir, B. Application of feature extraction and classification methods for histopathological image using GLCM, LBP, LBGLCM, GLRLM and SFTA. Proc. Comput. Sci. 132 , 40–46 (2018).
Sadique, F. & Haque, R. Content-based image retrieval using color layout descriptor, gray-level co-occurrence matrix and K-nearest neighbors. Int. J. Inf. Technol. Comput. Sci. 3 , 19–25 (2020).
Li, Y., Cui, W., Luo, M., Li, K. & Wang, L. Epileptic seizure detection based on time-frequency images of EEG signals using gaussian mixture model and gray level co-occurrence matrix features. Int. J. Neural Syst. 28 (7), 1850003 (2018).
Aljanabi, M. et al. A review ABCDE evaluated the model for decision by dermatologists for skin lesions using bee colony. IOP Conf. Ser. Mater. Sci. Eng. 745 , 012098 (2020).
Tsao, H. et al. Early detection of melanoma: Reviewing the ABCDEs. J. Am. Acad. Dermatol. 72 , 717–723 (2015).
Zghal, N. S. Derbel1 N melanoma skin cancer detection based on image processing. Curr. Med. Imaging. 16 , 50–58 (2020).
Article ADS MathSciNet Google Scholar
Moussa, R., Gerges, F., Salem, C., Akiki, R., Falou, O., Azar, D. Computer-aided detection of Melanoma using geometric features. In 3rd Middle East Conference on Biomedical Engineering (MECBME) . 125–128 (2016).
Abbes, W. & Sellami, D. High-Level Features for Automatic Skin Lesions Neural Network Based Classification 1–7 (International Image Processing, 2016).
Lu, C. & Mandal, M. Automated analysis and diagnosis of skin melanoma on whole slide histopathological images. Pattern Recogn. 48 (8), 2738–2750 (2015).
Sun, X., Hu, C., Lei, G., Guo, Y. & Zhu, J. State feedback control for a PM hub motor based on grey wolf optimization algorithm. IEEE Trans. Power Electr. 35 , 1136–1146 (2019).
Mahmoud, M. H., Alamery, S., Altinawi, A., Fouad, H. & Mahmoud, N. M. Cohesive prediction model for analyzing the recurrent attributes of infectious cryptosporidiosis disease. J. Ambient Intell. Hum. Comput. https://doi.org/10.1007/s12652-020-02861-8 (2021).
Starovoitov, V. & Golub, Y. Comparative study of quality estimation of binary classification. Informatics. 17 (1), 87–101 (2020).
Saqlain, S. M. et al. Fisher score and Matthews correlation coefficient-based feature subset selection for heart disease diagnosis using support vector machines. Knowl. Inf. Syst. 58 , 139–167 (2019).
Download references
Open access funding provided by The Science, Technology & Innovation Funding Authority (STDF) in cooperation with The Egyptian Knowledge Bank (EKB).
Author information
Authors and affiliations.
Biomedical Engineering Department, Faculty of Engineering, Minia University, Minya, Egypt
Nourelhoda M. Mahmoud
Biomedical Engineering Department, Faculty of Engineering, Helwan University, Cairo, Egypt
Ahmed M. Soliman
You can also search for this author in PubMed Google Scholar
Contributions
N.M.M. wrote the main manuscript text, built program, and prepared figures. A.M.S. analyzed the results, reviewed and updated the manuscript.
Corresponding author
Correspondence to Nourelhoda M. Mahmoud .
Ethics declarations
Competing interests.
The authors declare no competing interests.
Additional information
Publisher's note.
Springer Nature remains neutral with regard to jurisdictional claims in published maps and institutional affiliations.
Rights and permissions
Open Access This article is licensed under a Creative Commons Attribution 4.0 International License, which permits use, sharing, adaptation, distribution and reproduction in any medium or format, as long as you give appropriate credit to the original author(s) and the source, provide a link to the Creative Commons licence, and indicate if changes were made. The images or other third party material in this article are included in the article's Creative Commons licence, unless indicated otherwise in a credit line to the material. If material is not included in the article's Creative Commons licence and your intended use is not permitted by statutory regulation or exceeds the permitted use, you will need to obtain permission directly from the copyright holder. To view a copy of this licence, visit http://creativecommons.org/licenses/by/4.0/ .
Reprints and permissions
About this article
Cite this article.
Mahmoud, N.M., Soliman, A.M. Early automated detection system for skin cancer diagnosis using artificial intelligent techniques. Sci Rep 14 , 9749 (2024). https://doi.org/10.1038/s41598-024-59783-0
Download citation
Received : 27 February 2024
Accepted : 15 April 2024
Published : 28 April 2024
DOI : https://doi.org/10.1038/s41598-024-59783-0
Share this article
Anyone you share the following link with will be able to read this content:
Sorry, a shareable link is not currently available for this article.
Provided by the Springer Nature SharedIt content-sharing initiative
- Artificial intelligent
- Artificial neural networks
- Support vector machine
- Adaptive snake
- Region growing
By submitting a comment you agree to abide by our Terms and Community Guidelines . If you find something abusive or that does not comply with our terms or guidelines please flag it as inappropriate.
Quick links
- Explore articles by subject
- Guide to authors
- Editorial policies
Sign up for the Nature Briefing: Cancer newsletter — what matters in cancer research, free to your inbox weekly.

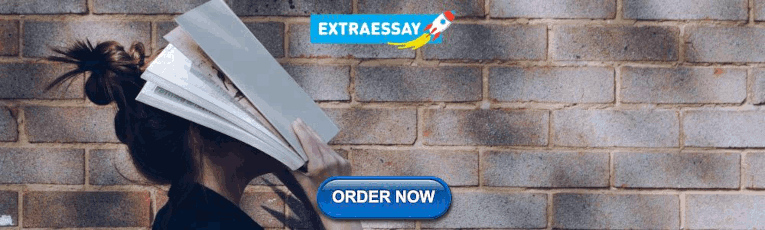
COMMENTS
Cutaneous melanoma (CM) is a malignant tumor formed from pigment-producing cells called melanocytes. It is one of the most aggressive and fatal forms of skin malignancy. In the last decades, CM's incidence has gradually risen, with 351,880 new cases in 2015. Since the 1960s, its incidence has increased steadily, in 2019, with approximately ...
Melanoma, a malignant tumor arising from melanocytes, is a rare disease, affecting only 22.1 out of 100,000 people in the US (Cancer statistics from the Center for Disease Control and Prevention). However, it is also a very deadly disease accounting for 75% of skin cancer deaths though it only accounts for 4% of skin cancer cases.
This paper presents a detailed systematic review of deep learning techniques for the early detection of skin cancer. Research papers published in well-reputed journals, relevant to the topic of skin cancer diagnosis, were analyzed. Research findings are presented in tools, graphs, tables, techniques, and frameworks for better understanding.
The recent research on the application of CNN in predicting skin cancer has identified significant challenges in incorporating the entire spectrum of the patient population and various melanoma ...
There are associations between skin cancers and those viral infectious diseases like acquired immune deficiency syndrome (AIDS). It has been observed that the risk of progression of non-melanoma skin cancer increases 3 to 5 times in AIDS patients [21]. Moreover, it has been documented that the incidence of BCC is 11.4 times more common in HIV ...
Human skin cancer is the most common and potentially life-threatening form of cancer. Melanoma skin cancer, in particular, exhibits a high mortality rate. Early detection is crucial for effective treatment. Traditionally, melanoma is detected through painful and time-consuming biopsies. This research introduces a computer-aided detection technique for early melanoma diagnosis-sis. In this ...
Cutaneous melanoma causes 55 500 deaths annually. The incidence and mortality rates of the disease differ widely across the globe depending on access to early detection and primary care. Once melanoma has spread, this type of cancer rapidly becomes life-threatening. For more than 40 years, few treatment options were available, and clinical ...
Melanoma is the most serious type of skin cancer. It develops when skin cells multiply rapidly as a consequence of mutations in their DNA caused by UV exposure. Melanomas originate in the pigment ...
Melanoma Research is a well established international forum for the dissemination of new findings relating to melanoma. The aim of the Journal is to promote the level of informational exchange between those engaged in the field. Melanoma Research aims to encourage an informed and balanced view of experimental and clinical research and extend and stimulate communication and exchange of ...
The Skin SPORE program's main focus is on melanoma research activities, but it also includes projects in other skin cancer types, such as cutaneous T-cell lymphoma. NCI's National Clinical Trials Network (NCTN) is a collection of organizations and clinicians that coordinates and supports cancer clinical trials at more than 3,000 sites across ...
Skin cancer, the most common human malignancy1,2,3, is primarily diagnosed visually, beginning with an initial clinical screening and followed potentially by dermoscopic analysis, a biopsy and ...
This study presents the use of recent deep CNN approaches to detect melanoma skin cancer and investigate suspicious lesions. Tests were conducted using a set of more than 36,000 images extracted from multiple datasets. The obtained results show that the best performing deep learning approach achieves high scores with an accuracy and Area Under ...
Identifying melanoma at the early stages of diagnosis is imperative as early detection can exponentially increase one's chances of cure. The paper first proposes a literature survey of multiple methods used for performing skin cancer classification. Our methodology consists of using Convolutional Neural Network (CNN) to identify and diagnose the skin cancer using the IS IC dataset containing ...
Through evaluation of research on skin cancer detection using ANN and CNN, the effectiveness and performance of these techniques in early and efficient diagnosis of skin cancer were established. ... deep learning, melanoma, lesions etc. The 11 papers published show that the merging of patient data with CNN classifier has improved accuracy and ...
Lesion parameters such as symmetry, color, size, shape, etc. are used to detect skin cancer and to distinguish benign skin cancer from melanoma. This paper presents a detailed systematic review of deep learning techniques for the early detection of skin cancer. Research papers published in well-reputed journals, relevant to the topic of skin ...
Review. Epidemiology and risk factors. Malignant melanoma is the most aggressive skin cancer, and its incidence is rising [8-11].Malignant melanoma is the 19th most common cancer diagnosis worldwide [] and is the leading cause of death due to cutaneous malignancy.In spite of only accounting for less than 5% of skin cancer diagnosis, melanoma is responsible for 65% of skin cancer-related deaths [].
Study Adds to Debate about Screening for Melanoma. Posted: May 4, 2022. Regular skin cancer screening leads to many diagnoses of very early-stage melanomas, results from a new study suggest. The results add to a debate about whether screening is fueling an overdiagnosis of melanoma in the United States. Opdualag Becomes First FDA-Approved ...
This article presents research on automated skin cancer detection using image processing and machine learning techniques. This paper focuses on melanoma, the deadliest form of skin cancer, and highlights the limitations of clinical observation. This study uses Convolutional Neural Networks (CNNs) and feature extraction to accurately classify skin cancers as malignant or benign. The proposed ...
Melanoma is an aggressive disease that accounts for approximately 75% of skin cancer-related deaths. 1 The World Health Organization reports that the incidence of metastatic melanoma has increased over the past 3 decades—estimating 66 000 deaths worldwide every year are due to skin cancer, with approximately 80% due to melanoma. Most cases of malignant melanoma are diagnosed at an early ...
Melanoma vaccines: Vaccines to treat melanoma are being studied in clinical trials. These vaccines are, in some ways, like the vaccines used to prevent diseases such as polio, measles, and mumps that are caused by viruses. Such vaccines usually contain weakened viruses or parts of a virus that can't cause the disease.
In the past few years many researches investigated algorithms to diagnose skin cancer lesions where melanoma is the deadliest type of skin cancer. In this paper, we propose three different methods for binary classification (melanoma and nevus) using smartphone images since there is a lack in research on it.
The incidence of melanoma is increasing at a greater rate than other types of cancer. The mean age at diagnosis is 57 years with higher incidence in women in the younger age groups while the ratio reverses in old age with higher incidence in men. Estimates from the US report a lifetime risk of melanoma of 1 in 56 for women and 1 in 37 for men.
May is Skin Cancer Awareness month, and at Ludwig Oxford, multiples researchers focus on the different types of skin cancer. In particular, Professors Richard White and Colin Goding run research groups both with a focus towards melanoma research. The largest rick factor for developing Melanoma is exposure to ultraviolet light.
Cutaneous melanoma is a malignancy arising from melanocytes of the skin. Incidence rates are rising, particularly in White populations. Cutaneous melanoma is typically driven by exposure to ultraviolet radiation from natural sunlight and indoor tanning, although there are several subtypes that are not related to ultraviolet radiation exposure.
A comparative study of melanoma skin cancer detection in traditional and current image processing techniques. In Fourth International Conference on I-SMAC 654-658 (2020).
Melanoma, by definition, is a skin cancer that impacts ... of a staple and raised to the height of 60 sheets of paper above the skin, per the Cleveland Clinic. ... Research Alliance. (2024 ...
The study was supported by National Cancer Institute grants R01 CA201376 (JGE), R01 CA151306 (JGE), R01 CA201376 (BB), and R35 CA220481 (BB), the National Health and Medical Research Council (NHMRC) Australian Government, grant APP1141295 (RAS), Melanoma Research Alliance (grant 622600; JGE), and Department of Defense award W81XWH2010797 (JGE).
Non-melanoma skin cancer includes several types of cutaneous tumors, with basal cell carcinoma (BCC) and cutaneous squamous cell carcinoma (cSCC) as the commonest. Among the available therapeutic options, surgical excision is the mainstay of treatment for both tumors. However, tumor features and patients' comorbidities may limit the use of these techniques, making the treatment challenging.
Abstract. Melanoma is a relentless type of skin cancer which involves myriad signaling pathways which regulate many cellular processes. This makes melanoma difficult to treat, especially when identified late. At present, therapeutics include chemotherapy, surgical resection, biochemotherapy, immunotherapy, photodynamic and targeted approaches.
The management of non-melanoma skin cancer during pregnancy requires a multidisciplinary and individualized approach, considering the risks and benefits for the mother and fetus. Pregnancy presents unique challenges in diagnosing and treating non-melanoma skin cancer, the most common type of skin cancer. Hormonal and immunological changes during pregnancy can influence the development and ...