
An official website of the United States government
The .gov means it’s official. Federal government websites often end in .gov or .mil. Before sharing sensitive information, make sure you’re on a federal government site.
The site is secure. The https:// ensures that you are connecting to the official website and that any information you provide is encrypted and transmitted securely.
- Publications
- Account settings
Preview improvements coming to the PMC website in October 2024. Learn More or Try it out now .
- Advanced Search
- Journal List
- BMC Public Health

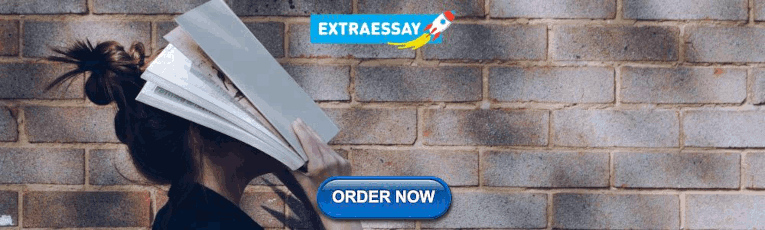
A review of the quantitative effectiveness evidence synthesis methods used in public health intervention guidelines
Ellesha a. smith.
Department of Health Sciences, University of Leicester, Lancaster Road, Leicester, UK
Nicola J. Cooper
Alex j. sutton, keith r. abrams, stephanie j. hubbard, associated data.
The dataset supporting the conclusions of this article is included within the article.
The complexity of public health interventions create challenges in evaluating their effectiveness. There have been huge advancements in quantitative evidence synthesis methods development (including meta-analysis) for dealing with heterogeneity of intervention effects, inappropriate ‘lumping’ of interventions, adjusting for different populations and outcomes and the inclusion of various study types. Growing awareness of the importance of using all available evidence has led to the publication of guidance documents for implementing methods to improve decision making by answering policy relevant questions.
The first part of this paper reviews the methods used to synthesise quantitative effectiveness evidence in public health guidelines by the National Institute for Health and Care Excellence (NICE) that had been published or updated since the previous review in 2012 until the 19th August 2019.The second part of this paper provides an update of the statistical methods and explains how they address issues related to evaluating effectiveness evidence of public health interventions.
The proportion of NICE public health guidelines that used a meta-analysis as part of the synthesis of effectiveness evidence has increased since the previous review in 2012 from 23% (9 out of 39) to 31% (14 out of 45). The proportion of NICE guidelines that synthesised the evidence using only a narrative review decreased from 74% (29 out of 39) to 60% (27 out of 45).An application in the prevention of accidents in children at home illustrated how the choice of synthesis methods can enable more informed decision making by defining and estimating the effectiveness of more distinct interventions, including combinations of intervention components, and identifying subgroups in which interventions are most effective.
Conclusions
Despite methodology development and the publication of guidance documents to address issues in public health intervention evaluation since the original review, NICE public health guidelines are not making full use of meta-analysis and other tools that would provide decision makers with fuller information with which to develop policy. There is an evident need to facilitate the translation of the synthesis methods into a public health context and encourage the use of methods to improve decision making.
Supplementary Information
The online version contains supplementary material available at (10.1186/s12889-021-10162-8).
To make well-informed decisions and provide the best guidance in health care policy, it is essential to have a clear framework for synthesising good quality evidence on the effectiveness and cost-effectiveness of health interventions. There is a broad range of methods available for evidence synthesis. Narrative reviews provide a qualitative summary of the effectiveness of the interventions. Meta-analysis is a statistical method that pools evidence from multiple independent sources [ 1 ]. Meta-analysis and more complex variations of meta-analysis have been extensively applied in the appraisals of clinical interventions and treatments, such as drugs, as the interventions and populations are clearly defined and tested in randomised, controlled conditions. In comparison, public health studies are often more complex in design, making synthesis more challenging [ 2 ].
Many challenges are faced in the synthesis of public health interventions. There is often increased methodological heterogeneity due to the inclusion of different study designs. Interventions are often poorly described in the literature which may result in variation within the intervention groups. There can be a wide range of outcomes, whose definitions are not consistent across studies. Intermediate, or surrogate, outcomes are often used in studies evaluating public health interventions [ 3 ]. In addition to these challenges, public health interventions are often also complex meaning that they are made up of multiple, interacting components [ 4 ]. Recent guidance documents have focused on the synthesis of complex interventions [ 2 , 5 , 6 ]. The National Institute for Health and Care Excellence (NICE) guidance manual provides recommendations across all topics that are covered by NICE and there is currently no guidance that focuses specifically on the public health context.
Research questions
A methodological review of NICE public health intervention guidelines by Achana et al. (2014) found that meta-analysis methods were not being used [ 3 ]. The first part of this paper aims to update and compare, to the original review, the meta-analysis methods being used in evidence synthesis of public health intervention appraisals.
The second part of this paper aims to illustrate what methods are available to address the challenges of public health intervention evidence synthesis. Synthesis methods that go beyond a pairwise meta-analysis are illustrated through the application to a case study in public health and are discussed to understand how evidence synthesis methods can enable more informed decision making.
The third part of this paper presents software, guidance documents and web tools for methods that aim to make appropriate evidence synthesis of public health interventions more accessible. Recommendations for future research and guidance production that can improve the uptake of these methods in a public health context are discussed.
Update of NICE public health intervention guidelines review
Nice guidelines.
The National Institute for Health and Care Excellence (NICE) was established in 1999 as a health authority to provide guidance on new medical technologies to the NHS in England and Wales [ 7 ]. Using an evidence-based approach, it provides recommendations based on effectiveness and cost-effectiveness to ensure an open and transparent process of allocating NHS resources [ 8 ]. The remit for NICE guideline production was extended to public health in April 2005 and the first recommendations were published in March 2006. NICE published ‘Developing NICE guidelines: the manual’ in 2006, which has been updated since, with the most recent in 2018 [ 9 ]. It was intended to be a guidance document to aid in the production of NICE guidelines across all NICE topics. In terms of synthesising quantitative evidence, the NICE recommendations state: ‘meta-analysis may be appropriate if treatment estimates of the same outcome from more than 1 study are available’ and ‘when multiple competing options are being appraised, a network meta-analysis should be considered’. The implementation of network meta-analysis (NMA), which is described later, as a recommendation from NICE was introduced into the guidance document in 2014, with a further update in 2018.
Background to the previous review
The paper by Achana et al. (2014) explored the use of evidence synthesis methodology in NICE public health intervention guidelines published between 2006 and 2012 [ 3 ]. The authors conducted a systematic review of the methods used to synthesise quantitative effectiveness evidence within NICE public health guidelines. They found that only 23% of NICE public health guidelines used pairwise meta-analysis as part of the effectiveness review and the remainder used a narrative summary or no synthesis of evidence at all. The authors argued that despite significant advances in the methodology of evidence synthesis, the uptake of methods in public health intervention evaluation is lower than other fields, including clinical treatment evaluation. The paper concluded that more sophisticated methods in evidence synthesis should be considered to aid in decision making in the public health context [ 3 ].
The search strategy used in this paper was equivalent to that in the previous paper by Achana et al. (2014)[ 3 ]. The search was conducted through the NICE website ( https://www.nice.org.uk/guidance ) by searching the ‘Guidance and Advice List’ and filtering by ‘Public Health Guidelines’ [ 10 ]. The search criteria included all guidance documents that had been published from inception (March 2006) until the 19th August 2019. Since the original review, many of the guidelines had been updated with new documents or merged. Guidelines that remained unchanged since the previous review in 2012 were excluded and used for comparison.
The guidelines contained multiple documents that were assessed for relevance. A systematic review is a separate synthesis within a guideline that systematically collates all evidence on a specific research question of interest in the literature. Systematic reviews of quantitative effectiveness, cost-effectiveness evidence and decision modelling reports were all included as relevant. Qualitative reviews, field reports, expert opinions, surveillance reports, review decisions and other supporting documents were excluded at the search stage.
Within the reports, data was extracted on the types of review (narrative summary, pairwise meta-analysis, network meta-analysis (NMA), cost-effectiveness review or decision model), design of included primary studies (randomised controlled trials or non-randomised studies, intermediate or final outcomes, description of outcomes, outcome measure statistic), details of the synthesis methods used in the effectiveness evaluation (type of synthesis, fixed or random effects model, study quality assessment, publication bias assessment, presentation of results, software). Further details of the interventions were also recorded, including whether multiple interventions were lumped together for a pairwise comparison, whether interventions were complex (made up of multiple components) and details of the components. The reports were also assessed for potential use of complex intervention evidence synthesis methodology, meaning that the interventions that were evaluated in the review were made up of components that could potentially be synthesised using an NMA or a component NMA [ 11 ]. Where meta-analysis was not used to synthesis effectiveness evidence, the reasons for this was also recorded.
Search results and types of reviews
There were 67 NICE public health guidelines available on the NICE website. A summary flow diagram describing the literature identification process and the list of guidelines and their reference codes are provided in Additional files 1 and 2 . Since the previous review, 22 guidelines had not been updated. The results from the previous review were used for comparison to the 45 guidelines that were either newly published or updated.
The guidelines consisted of 508 documents that were assessed for relevance. Table 1 shows which types of relevant documents were available in each of the 45 guidelines. The median number of relevant articles per guideline was 3 (minimum = 0, maximum = 10). Two (4%) of the NICE public health guidelines did not report any type of systematic review, cost-effectiveness review or decision model (NG68, NG64) that met the inclusion criteria. 167 documents from 43 NICE public health guidelines were systematic reviews of quantitative effectiveness, cost-effectiveness or decision model reports and met the inclusion criteria.
Contents of the NICE public health intervention guidelines
Narrative reviews of effectiveness were implemented in 41 (91%) of the NICE PH guidelines. 14 (31%) contained a review that used meta-analysis to synthesise the evidence. Only one (1%) NICE guideline contained a review that implemented NMA to synthesise the effectiveness of multiple interventions; this was the same guideline that used NMA in the original review and had been updated. 33 (73%) guidelines contained cost-effectiveness reviews and 34 (76%) developed a decision model.
Comparison of review types to original review
Table 2 compares the results of the update to the original review and shows that the types of reviews and evidence synthesis methodologies remain largely unchanged since 2012. The proportion of guidelines that only contain narrative reviews to synthesise effectiveness or cost-effectiveness evidence has reduced from 74% to 60% and the proportion that included a meta-analysis has increased from 23% to 31%. The proportion of guidelines with reviews that only included evidence from randomised controlled trials and assessed the quality of individual studies remained similar to the original review.
Comparison of methods of original review. RCT: randomised controlled trial
Characteristics of guidelines using meta-analytic methods
Table 3 details the characteristics of the meta-analytic methods implemented in 24 reviews of the 14 guidelines that included one. All of the reviews reported an assessment of study quality, 12 (50%) reviews included only data from randomised controlled trials, 4 (17%) reviews used intermediate outcomes (e.g. uptake of chlamydia screening rather than prevention of chlamydia (PH3)), compared to the 20 (83%) reviews that used final outcomes (e.g. smoking cessation rather than uptake of a smoking cessation programme (NG92)). 2 (8%) reviews only used a fixed effect meta-analysis, 19 (79%) reviews used a random effects meta-analysis and 3 (13%) did not report which they had used.
Meta-analytic methods used in the NICE public health intervention appraisals to synthesise the effectiveness evidence
Notation: E: effectiveness, CE: cost-effectiveness, DM: decision model, RR: risk ratio, MD: mean difference, OR: odds ratio, SMD: standardised mean difference, HR: hazard ratio, MA: meta-analysis, NMA: network meta-analysis, nr: not reported, R: random effects, F: fixed effect, Txt: text, T: table, FP: forest plot
An evaluation of the intervention information reported in the reviews concluded that 12 (50%) reviews had lumped multiple (more than two) different interventions into a control versus intervention pairwise meta-analysis. Eleven (46%) of the reviews evaluated interventions that are made up of multiple components (e.g. interventions for preventing obesity in PH47 were made up of diet, physical activity and behavioural change components).
21 (88%) of the reviews presented the results of the meta-analysis in the form of a forest plot and 22 (92%) presented the results in the text of the report. 20 (83%) of the reviews used two or more forms of presentation for the results. Only three (13%) reviews assessed publication bias. The most common software to perform meta-analysis was RevMan in 14 (58%) of the reviews.
Reasons for not using meta-analytic methods
The 143 reviews of effectiveness and cost effectiveness that did not use meta-analysis methods to synthesise the quantitative effectiveness evidence were searched for reasons behind this decision. 70 reports (49%) did not give a reason for not synthesising the data using a meta-analysis and 164 reasons were reported which are displayed in Fig. 1 . Out of the remaining reviews, multiple reasons for not using a meta-analysis were given. 53 (37%) of the reviews reported at least one reason due to heterogeneity. 30 (21%) decision model reports did not give a reason and these are categorised separately. 5 (3%) reviews reported that meta-analysis was not applicable or feasible, 1 (1%) reported that they were following NICE guidelines and 5 (3%) reported that there were a lack of studies.

Frequency and proportions of reasons reported for not using statistical methods in quantitative evidence synthesis in NICE PH intervention reviews
The frequency of reviews and guidelines that used meta-analytic methods were plotted against year of publication, which is reported in Fig. 2 . This showed that the number of reviews that used meta-analysis were approximately constant but there is some suggestion that the number of meta-analyses used per guideline increased, particularly in 2018.

Number of meta-analyses in NICE PH guidelines by year. Guidelines that were published before 2012 had been updated since the previous review by Achana et al. (2014) [ 3 ]
Comparison of meta-analysis characteristics to original review
Table 4 compares the characteristics of the meta-analyses used in the evidence synthesis of NICE public health intervention guidelines to the original review by Achana et al. (2014) [ 3 ]. Overall, the characteristics in the updated review have not much changed from those in the original. These changes demonstrate that the use of meta-analysis in NICE guidelines has increased but remains low. Lumping of interventions still appears to be common in 50% of reviews. The implications of this are discussed in the next section.
Meta-analysis characteristics: comparison to original review
Application of evidence synthesis methodology in a public health intervention: motivating example
Since the original review, evidence synthesis methods have been developed and can address some of the challenges of synthesising quantitative effectiveness evidence of public health interventions. Despite this, the previous section shows that the uptake of these methods is still low in NICE public health guidelines - usually limited to a pairwise meta-analysis.
It has been shown in the results above and elsewhere [ 12 ] that heterogeneity is a common reason for not synthesising the quantitative effectiveness evidence available from systematic reviews in public health. Statistical heterogeneity is the variation in the intervention effects between the individual studies. Heterogeneity is problematic in evidence synthesis as it leads to uncertainty in the pooled effect estimates in a meta-analysis which can make it difficult to interpret the pooled results and draw conclusions. Rather than exploring the source of the heterogeneity, often in public health intervention appraisals a random effects model is fitted which assumes that the study intervention effects are not equivalent but come from a common distribution [ 13 , 14 ]. Alternatively, as demonstrated in the review update, heterogeneity is used as a reason to not undertake any quantitative evidence synthesis at all.
Since the size of the intervention effects and the methodological variation in the studies will affect the impact of the heterogeneity on a meta-analysis, it is inappropriate to base the methodological approach of a review on the degree of heterogeneity, especially within public health intervention appraisal where heterogeneity seems inevitable. Ioannidis et al. (2008) argued that there are ‘almost always’ quantitative synthesis options that may offer some useful insights in the presence of heterogeneity, as long as the reviewers interpret the findings with respect to their limitations [ 12 ].
In this section current evidence synthesis methods are applied to a motivating example in public health. This aims to demonstrate that methods beyond pairwise meta-analysis can provide appropriate and pragmatic information to public health decision makers to enable more informed decision making.
Figure 3 summarises the narrative of this part of the paper and illustrates the methods that are discussed. The red boxes represent the challenges in synthesising quantitative effectiveness evidence and refers to the section within the paper for more detail. The blue boxes represent the methods that can be applied to investigate each challenge.

Summary of challenges that are faces in the evidence synthesis of public health interventions and methods that are discussed to overcome these challenges
Evaluating the effect of interventions for promoting the safe storage of cleaning products to prevent childhood poisoning accidents
To illustrate the methodological developments, a motivating example is used from the five year, NIHR funded, Keeping Children Safe Programme [ 15 ]. The project included a Cochrane systematic review that aimed to increase the use of safety equipment to prevent accidents at home in children under five years old. This application is intended to be illustrative of the benefits of new evidence synthesis methods since the previous review. It is not a complete, comprehensive analysis as it only uses a subset of the original dataset and therefore the results are not intended to be used for policy decision making. This example has been chosen as it demonstrates many of the issues in synthesising effectiveness evidence of public health interventions, including different study designs (randomised controlled trials, observational studies and cluster randomised trials), heterogeneity of populations or settings, incomplete individual participant data and complex interventions that contain multiple components.
This analysis will investigate the most effective promotional interventions for the outcome of ‘safe storage of cleaning products’ to prevent childhood poisoning accidents. There are 12 studies included in the dataset, with IPD available from nine of the studies. The covariate, single parent family, is included in the analysis to demonstrate the effect of being a single parent family on the outcome. In this example, all of the interventions are made up of one or more of the following components: education (Ed), free or low cost equipment (Eq), home safety inspection (HSI), and installation of safety equipment (In). A Bayesian approach using WinBUGS was used and therefore credible intervals (CrI) are presented with estimates of the effect sizes [ 16 ].
The original review paper by Achana et al. (2014) demonstrated pairwise meta-analysis and meta-regression using individual and cluster allocated trials, subgroup analyses, meta-regression using individual participant data (IPD) and summary aggregate data and NMA. This paper firstly applies NMA to the motivating example for context, followed by extensions to NMA.
Multiple interventions: lumping or splitting?
Often in public health there are multiple intervention options. However, interventions are often lumped together in a pairwise meta-analysis. Pairwise meta-analysis is a useful tool for two interventions or, alternatively in the presence of lumping interventions, for answering the research question: ‘are interventions in general better than a control or another group of interventions?’. However, when there are multiple interventions, this type of analysis is not appropriate for informing health care providers which intervention should be recommended to the public. ‘Lumping’ is becoming less frequent in other areas of evidence synthesis, such as for clinical interventions, as the use of sophisticated synthesis techniques, such as NMA, increases (Achana et al. 2014) but lumping is still common in public health.
NMA is an extension of the pairwise meta-analysis framework to more than two interventions. Multiple interventions that are lumped into a pairwise meta-analysis are likely to demonstrate high statistical heterogeneity. This does not mean that quantitative synthesis could not be undertaken but that a more appropriate method, NMA, should be implemented. Instead the statistical approach should be based on the research questions of the systematic review. For example, if the research question is ‘are any interventions effective for preventing obesity?’, it would be appropriate to perform a pairwise meta-analysis comparing every intervention in the literature to a control. However, if the research question is ‘which intervention is the most effective for preventing obesity?’, it would be more appropriate and informative to perform a network meta-analysis, which can compare multiple interventions simultaneously and identify the best one.
NMA is a useful statistical method in the context of public health intervention appraisal, where there are often multiple intervention options, as it estimates the relative effectiveness of three or more interventions simultaneously, even if direct study evidence is not available for all intervention comparisons. Using NMA can help to answer the research question ‘what is the effectiveness of each intervention compared to all other interventions in the network?’.
In the motivating example there are six intervention options. The effect of lumping interventions is shown in Fig. 4 , where different interventions in both the intervention and control arms are compared. There is overlap of intervention and control arms across studies and interpretation of the results of a pairwise meta-analysis comparing the effectiveness of the two groups of interventions would not be useful in deciding which intervention to recommend. In comparison, the network plot in Fig. 5 illustrates the evidence base of the prevention of childhood poisonings review comparing six interventions that promote the use of safety equipment in the home. Most of the studies use ‘usual care’ as a baseline and compare this to another intervention. There are also studies in the evidence base that compare pairs of the interventions, such as ‘Education and equipment’ to ‘Equipment’. The plot also demonstrates the absence of direct study evidence between many pairs of interventions, for which the associated treatment effects can be indirectly estimated using NMA.

Network plot to illustrate how pairwise meta-analysis groups the interventions in the motivating dataset. Notation UC: Usual care, Ed: Education, Ed+Eq: Education and equipment, Ed+Eq+HSI: Education, equipment, and home safety inspection, Ed+Eq+In: Education, equipment and installation, Eq: Equipment

Network plot for the safe storage of cleaning products outcome. Notation UC: Usual care, Ed: Education, Ed+Eq: Education and equipment, Ed+Eq+HSI: Education, equipment, and home safety inspection, Ed+Eq+In: Education, equipment and installation, Eq: Equipment
An NMA was fitted to the motivating example to compare the six interventions in the studies from the review. The results are reported in the ‘triangle table’ in Table 5 [ 17 ]. The top right half of the table shows the direct evidence between pairs of the interventions in the corresponding rows and columns by either pooling the studies as a pairwise meta-analysis or presenting the single study results if evidence is only available from a single study. The bottom left half of the table reports the results of the NMA. The gaps in the top right half of the table arise where no direct study evidence exists to compare the two interventions. For example, there is no direct study evidence comparing ‘Education’ (Ed) to ‘Education, equipment and home safety inspection’ (Ed+Eq+HSI). The NMA, however, can estimate this comparison through the direct study evidence as an odds ratio of 3.80 with a 95% credible interval of (1.16, 12.44). The results suggest that the odds of safely storing cleaning products in the Ed+Eq+HSI intervention group is 3.80 times the odds in the Ed group. The results demonstrate a key benefit of NMA that all intervention effects in a network can be estimated using indirect evidence, even if there is no direct study evidence for some pairwise comparisons. This is based on the consistency assumption (that estimates of intervention effects from direct and indirect evidence are consistent) which should be checked when performing an NMA. This is beyond the scope of this paper and details on this can be found elsewhere [ 18 ].
Results of an NMA expressed as odds ratios with 95% CrIs
NMA results are in the bottom left half of the table. Pairwise meta-analysis or single study results, where no other direct evidence is available, are in the top right half of the table.
Notation UC: Usual care, Ed: Education, Ed+Eq: Education and equipment, Ed+Eq+HSI: Education, equipment, and home safety inspection, Ed+Eq+In: Education, equipment and installation, Eq: Equipment
NMA can also be used to rank the interventions in terms of their effectiveness and estimate the probability that each intervention is likely to be the most effective. This can help to answer the research question ‘which intervention is the best?’ out of all of the interventions that have provided evidence in the network. The rankings and associated probabilities for the motivating example are presented in Table 6 . It can be seen that in this case the ‘education, equipment and home safety inspection’ (Ed+Eq+HSI) intervention is ranked first, with a 0.87 probability of being the best intervention. However, there is overlap of the 95% credible intervals of the median rankings. This overlap reflects the uncertainty in the intervention effect estimates and therefore it is important that the interpretation of these statistics clearly communicates this uncertainty to decision makers.
Results of the NMA: probability that each intervention is the best and their ranks
Notation P(best): probability that intervention is the best, CrI: Credible interval, UC: Usual care, Ed: Education, Ed+Eq: Education and equipment, Ed+Eq+HSI: Education, equipment, and home safety inspection, Ed+Eq+In: Education, equipment and installation, Eq: Equipment
NMA has the potential to be extremely useful but is underutilised in the evidence synthesis of public health interventions. The ability to compare and rank multiple interventions in an area where there are often multiple intervention options is invaluable in decision making for identifying which intervention to recommend. NMA can also include further literature in the analysis, compared to a pairwise meta-analysis, by expanding the network to improve the uncertainty in the effectiveness estimates.
Statistical heterogeneity
When heterogeneity remains in the results of an NMA, it is useful to explore the reasons for this. Strategies for dealing with heterogeneity involve the inclusion of covariates in a meta-analysis or NMA to adjust for the differences in the covariates across studies [ 19 ]. Meta-regression is a statistical method developed from meta-analysis that includes covariates to potentially explain the between-study heterogeneity ‘with the aim of estimating treatment-covariate interactions’ (Saramago et al. 2012). NMA has been extended to network meta-regression which investigates the effect of trial characteristics on multiple intervention effects. Three ways have been suggested to include covariates in an NMA: single covariate effect, exchangeable covariate effects and independent covariate effects which are discussed in more detail in the NICE Technical Support Document 3 [ 14 ]. This method has the potential to assess the effect of study level covariates on the intervention effects, which is particularly relevant in public health due to the variation across studies.
The most widespread method of meta-regression uses study level data for the inclusion of covariates into meta-regression models. Study level covariate data is when the data from the studies are aggregated, e.g. the proportion of participants in a study that are from single parent families compared to dual parent families. The alternative to study level data is individual participant data (IPD), where the data are available and used as a covariate at the individual level e.g. the parental status of every individual in a study can be used as a covariate. Although IPD is considered to be the gold standard for meta-analysis, aggregated level data is much more commonly used as it is usually available and easily accessible from published research whereas IPD can be hard to obtain from study authors.
There are some limitations to network meta-regression. In our motivating example, using the single parent covariate in a meta-regression would estimate the relative difference in the intervention effects of a population that is made up of 100% single parent families compared to a population that is made up of 100% dual parent families. This interpretation is not as useful as the analysis that uses IPD, which would give the relative difference of the intervention effects in a single parent family compared to a dual parent family. The meta-regression using aggregated data would also be susceptible to ecological bias. Ecological bias is where the effect of the covariate is different at the study level compared to the individual level [ 14 ]. For example, if each study demonstrates a relationship between a covariate and the intervention but the covariate is similar across the studies, a meta-regression of the aggregate data would not demonstrate the effect that is observed within the studies [ 20 ].
Although meta-regression is a useful tool for investigating sources of heterogeneity in the data, caution should be taken when using the results of meta-regression to explain how covariates affect the intervention effects. Meta-regression should only be used to investigate study characteristics, such as the duration of intervention, which will not be susceptible to ecological bias and the interpretation of the results (the effect of intervention duration on intervention effectiveness) would be more meaningful for the development of public health interventions.
Since the covariate of interest in this motivating example is not a study characteristic, meta-regression of aggregated covariate data was not performed. Network meta-regression including IPD and aggregate level data was developed by Samarago et al. (2012) [ 21 ] to overcome the issues with aggregated data network meta-regression, which is discussed in the next section.
Tailored decision making to specific sub-groups
In public health it is important to identify which interventions are best for which people. There has been a recent move towards precision medicine. In the field of public health the ‘concept of precision prevention may [...] be valuable for efficiently targeting preventive strategies to the specific subsets of a population that will derive maximal benefit’ (Khoury and Evans, 2015). Tailoring interventions has the potential to reduce the effect of inequalities in social factors that are influencing the health of the population. Identifying which interventions should be targeted to which subgroups can also lead to better public health outcomes and help to allocate scarce NHS resources. Research interest, therefore, lies in identifying participant level covariate-intervention interactions.
IPD meta-analysis uses data at the individual level to overcome ecological bias. The interpretation of IPD meta-analysis is more relevant in the case of using participant characteristics as covariates since the interpretation of the covariate-intervention interaction is at the individual level rather than the study level. This means that it can answer the research question: ‘which interventions work best in subgroups of the population?’. IPD meta-analyses are considered to be the gold standard for evidence synthesis since it increases the power of the analysis to identify covariate-intervention interactions and it has the ability to reduce the effect of ecological bias compared to aggregated data alone. IPD meta-analysis can also help to overcome scarcity of data issues and has been shown to have higher power and reduce the uncertainty in the estimates compared to analysis including only summary aggregate data [ 22 ].
Despite the advantages of including IPD in a meta-analysis, in reality it is often very time consuming and difficult to collect IPD for all of the studies [ 21 ]. Although data sharing is becoming more common, it remains time consuming and difficult to collect IPD for all studies in a review. This results in IPD being underutilised in meta-analyses. As an intermediate solution, statistical methods have been developed, such as the NMA in Samarago et al. (2012), that incorporates both IPD and aggregate data. Methods that simultaneously include IPD and aggregate level data have been shown to reduce uncertainty in the effect estimates and minimise ecological bias [ 20 , 21 ]. A simulation study by Leahy et al. (2018) found that an increased proportion of IPD resulted in more accurate and precise NMA estimates [ 23 ].
An NMA including IPD, where it is available, was performed, based on the model presented in Samarago et al. (2012) [ 21 ]. The results in Table 7 demonstrates the detail that this type of analysis can provide to base decisions on. More relevant covariate-intervention interaction interpretations can be obtained, for example the regression coefficients for covariate-intervention interactions are the individual level covariate intervention interactions or the ‘within study interactions’ that are interpreted as the effect of being in a single parent family on the effectiveness of each of the interventions. For example, the effect of Ed+Eq compared to UC in a single parent family is 1.66 times the effect of Ed+Eq compared to UC in a dual parent family but this is not an important difference as the credible interval crosses 1. The regression coefficients for the study level covariate-intervention interactions or the ‘between study interactions’ can be interpreted as the relative difference in the intervention effects of a population that is made up of 100% single parent families compared to a population that is made up of 100% dual parent families.
Results of network meta-regression including IPD and summary aggregate data
Notation CrI: Credible interval, UC: Usual care, Ed: Education, Ed+Eq: Education and equipment, Ed+Eq+HSI: Education, equipment, and home safety inspection, Ed+Eq+In: Education, equipment and installation, Eq: Equipment
Complex interventions
In many public health research settings the complex interventions are comprised of a number of components. An NMA can compare all of the interventions in a network as they are implemented in the original trials. However, NMA does not tell us which components of the complex intervention are attributable to this effect. It could be that particular components, or the interacting effect of multiple components, are driving the effectiveness and other components are not as effective. Often, trials have not directly compared every combination of components as there are so many component combination options, it would be inefficient and impractical. Component NMA was developed by Welton et al. (2009) to estimate the effect of each component of the complex interventions and combination of components in a network, in the absence of direct trial evidence and answers the question: ‘are interventions with a particular component or combination of components effective?’ [ 11 ]. For example, for the motivating example, in comparison to Fig. 5 , which demonstrates the interventions that an NMA can estimate effectiveness, Fig. 6 demonstrates all of the possible interventions of which the effectiveness can be estimated in a component NMA, given the components present in the network.

Network plot that illustrates how component network meta-analysis can estimate the effectiveness of intervention components and combinations of components, even when they are not included in the direct evidence. Notation UC: Usual care, Ed: Education, Eq: Equipment, Installation, Ed+Eq: Education and equipment, Ed+HSI: Education and home safety inspection, Ed+In: Education and installation, Eq+HSI: Equipment and home safety inspection, Eq+In: equipment and installation, HSI+In: Home safety inspection and installation, Ed+Eq+HSI: Education, equipment, and home safety inspection, Ed+Eq+In: Education, equipment and installation, Eq+HSI+In: Equipment, home safety inspection and installation, Ed+Eq+HSI+In: Education, equipment, home safety inspection and installation
The results of the analyses of the main effects, two way effects and full effects models are shown in Table 8 . The models, proposed in the original paper by Welton et al. (2009), increase in complexity as the assumptions regarding the component effects relax [ 24 ]. The main effects component NMA assumes that the components in the interventions each have separate, independent effects and intervention effects are the sum of the component effects. The two-way effects models assumes that there are interactions between pairs of the components, so the effects of the interventions are more than the sum of the effects. The full effects model assumes that all of the components and combinations of the components interact. Component NMA did not provide further insight into which components are likely to be the most effective since all of the 95% credible intervals were very wide and overlapped 1. There is a lot of uncertainty in the results, particularly in the 2-way and full effects models. A limitation of component NMA is that there are issues with uncertainty when data is scarce. However, the results demonstrate the potential of component NMA as a useful tool to gain better insights from the available dataset.
Results of the complex interventions analysis. All results are presented as OR (95% CrI)
Notation CrI: Credible interval, UC: Usual care, Ed: Education, Eq: Equipment, Installation, Ed+Eq: Education and equipment, Ed+HSI: Education and home safety inspection, Ed+In: Education and installation, Eq+HSI: Equipment and home safety inspection, Eq+In: equipment and installation, HSI+In: Home safety inspection and installation, Ed+Eq+HSI: Education, equipment, and home safety inspection, Ed+Eq+In: Education, equipment and installation, Eq+HSI+In: Equipment, home safety inspection and installation, Ed+Eq+HSI+In: Education, equipment, home safety inspection and installation, τ 2 : between study variance
In practice, this method has rarely been used since its development [ 24 – 26 ]. It may be challenging to define the components in some areas of public health where many interventions have been studied. However, the use of meta-analysis for planning future studies is rarely discussed and component NMA would provide a useful tool for identifying new component combinations that may be more effective [ 27 ]. This type of analysis has the potential to prioritise future public health research, which is especially useful where there are multiple intervention options, and identify more effective interventions to recommend to the public.
Further methods / other outcomes
The analysis and methods described in this paper only cover a small subset of the methods that have been developed in meta-analysis in recent years. Methods that aim to assess the quality of evidence supporting a NMA and how to quantify how much the evidence could change due to potential biases or sampling variation before the recommendation changes have been developed [ 28 , 29 ]. Models adjusting for baseline risk have been developed to allow for different study populations to have different levels of underlying risk, by using the observed event rate in the control arm [ 30 , 31 ]. Multivariate methods can be used to compare the effect of multiple interventions on two or more outcomes simultaneously [ 32 ]. This area of methodological development is especially appealing within public health where studies assess a broad range of health effects and typically have multiple outcome measures. Multivariate methods offer benefits over univariate models by allowing the borrowing of information across outcomes and modelling the relationships between outcomes which can potentially reduce the uncertainty in the effect estimates [ 33 ]. Methods have also been developed to evaluate interventions with classes or different intervention intensities, known as hierarchical interventions [ 34 ]. These methods were not demonstrated in this paper but can also be useful tools for addressing challenges of appraising public health interventions, such as multiple and surrogate outcomes.
This paper only considered an example with a binary outcome. All of the methods described have also been adapted for other outcome measures. For example, the Technical Support Document 2 proposed a Bayesian generalised linear modelling framework to synthesise other outcome measures. More information and models for continuous and time-to-event data is available elsewhere [ 21 , 35 – 38 ].
Software and guidelines
In the previous section, meta-analytic methods that answer more policy relevant questions were demonstrated. However, as shown by the update to the review, methods such as these are still under-utilised. It is suspected from the NICE public health review that one of the reasons for the lack of uptake of methods in public health could be due to common software choices, such as RevMan, being limited in their flexibility for statistical methods.
Table 9 provides a list of software options and guidance documents that are more flexible than RevMan for implementing the statistical methods illustrated in the previous section to make these methods more accessible to researchers.
Software for fitting meta-analysis models (full references in bibliography)
In this paper, the network plot in Figs. 5 and and6 6 were produced using the networkplot command from the mvmeta package [ 39 ] in Stata [ 61 ]. WinBUGS was used to fit the NMA in this paper by adapting the code in the book ‘Evidence Synthesis for Decision Making in Healthcare’ which also provides more detail on Bayesian methods and assessing convergence of Bayesian models [ 45 ]. The model for including IPD and summary aggregate data in an NMA was based on the code in the paper by Saramago et al. (2012). The component NMA in this paper was performed in WinBUGS through R2WinBUGS, [ 47 ] using the code in Welton et al. (2009) [ 11 ].
WinBUGS is a flexible tool for fitting complex models in a Bayesian framework. The NICE Decision Support Unit produced a series of Evidence Synthesis Technical Support Documents [ 46 ] that provide a comprehensive technical guide to methods for evidence synthesis and WinBUGS code is also provided for many of the models. Complex models can also be performed in a frequentist framework. Code and commands for many models are available in R and STATA (see Table 9 ).
The software, R2WinBUGS, was used in the analysis of the motivating example. Increasing numbers of researchers are using R and so packages that can be used to link the two softwares by calling BUGS models in R, packages such as R2WinBUGS, can improve the accessibility of Bayesian methods [ 47 ]. The new R package, BUGSnet, may also help to facilitate the accessibility and improve the reporting of Bayesian NMA [ 48 ]. Webtools have also been developed as a means of enabling researchers to undertake increasingly complex analyses [ 52 , 53 ]. Webtools provide a user-friendly interface to perform statistical analyses and often help in the reporting of the analyses by producing plots, including network plots and forest plots. These tools are very useful for researchers that have a good understanding of the statistical methods they want to implement as part of their review but are inexperienced in statistical software.
This paper has reviewed NICE public health intervention guidelines to identify the methods that are currently being used to synthesise effectiveness evidence to inform public health decision making. A previous review from 2012 was updated to see how method utilisation has changed. Methods have been developed since the previous review and these were applied to an example dataset to show how methods can answer more policy relevant questions. Resources and guidelines for implementing these methods were signposted to encourage uptake.
The review found that the proportion of NICE guidelines containing effectiveness evidence summarised using meta-analysis methods has increased since the original review, but remains low. The majority of the reviews presented only narrative summaries of the evidence - a similar result to the original review. In recent years, there has been an increased awareness of the need to improve decision making by using all of the available evidence. As a result, this has led to the development of new methods, easier application in standard statistical software packages, and guidance documents. Based on this, it would have been expected that their implementation would rise in recent years to reflect this, but the results of the review update showed no such increasing pattern.
A high proportion of NICE guideline reports did not provide a reason for not applying quantitative evidence synthesis methods. Possible explanations for this could be time or resource constraints, lack of statistical expertise, being unaware of the available methods or poor reporting. Reporting guidelines, such as the Preferred Reporting Items for Systematic Reviews and Meta-Analyses (PRISMA), should be updated to emphasise the importance of documenting reasons for not applying methods, as this can direct future research to improve uptake.
Where it was specified, the most common reported reason for not conducting a meta-analysis was heterogeneity. Often in public health, the data is heterogeneous due to the differences between studies in population, design, interventions or outcomes. A common misconception is that the presence of heterogeneity implies that it is not possible to pool the data. Meta-analytic methods can be used to investigate the sources of heterogeneity, as demonstrated in the NMA of the motivating example, and the use of IPD is recommended where possible to improve the precision of the results and reduce the effect of ecological bias. Although caution should be exercised in the interpretation of the results, quantitative synthesis methods provide a stronger basis for making decisions than narrative accounts because they explicitly quantify the heterogeneity and seek to explain it where possible.
The review also found that the most common software to perform the synthesis was RevMan. RevMan is very limited in its ability to perform advanced statistical analyses, beyond that of pairwise meta-analysis, which might explain the above findings. Standard software code is being developed to help make statistical methodology and application more accessible and guidance documents are becoming increasingly available.
The evaluation of public health interventions can be problematic due to the number and complexity of the interventions. NMA methods were applied to a real Cochrane public health review dataset. The methods that were demonstrated showed ways to address some of these issues, including the use of NMA for multiple interventions, the inclusion of covariates as both aggregated data and IPD to explain heterogeneity, and the extension to component network meta-analysis for guiding future research. These analyses illustrated how the choice of synthesis methods can enable more informed decision making by allowing more distinct interventions, and combinations of intervention components, to be defined and their effectiveness estimated. It also demonstrated the potential to target interventions to population subgroups where they are likely to be most effective. However, the application of component NMA to the motivating example has also demonstrated the issues around uncertainty if there are a limited number of studies observing the interventions and intervention components.
The application of methods to the motivating example demonstrated a key benefit of using statistical methods in a public health context compared to only presenting a narrative review – the methods provide a quantitative estimate of the effectiveness of the interventions. The uncertainty from the credible intervals can be used to demonstrate the lack of available evidence. In the context of decision making, having pooled estimates makes it much easier for decision makers to assess the effectiveness of the interventions or identify when more research is required. The posterior distribution of the pooled results from the evidence synthesis can also be incorporated into a comprehensive decision analytic model to determine cost-effectiveness [ 62 ]. Although narrative reviews are useful for describing the evidence base, the results are very difficult to summarise in a decision context.
Although heterogeneity seems to be inevitable within public health interventions due to their complex nature, this review has shown that it is still the main reported reason for not using statistical methods in evidence synthesis. This may be due to guidelines that were originally developed for clinical treatments that are tested in randomised conditions still being applied in public health settings. Guidelines for the choice of methods used in public health intervention appraisals could be updated to take into account the complexities and wide ranging areas in public health. Sophisticated methods may be more appropriate in some cases than simpler models for modelling multiple, complex interventions and their uncertainty, given the limitations are also fully reported [ 19 ]. Synthesis may not be appropriate if statistical heterogeneity remains after adjustment for possible explanatory covariates but details of exploratory analysis and reasons for not synthesising the data should be reported. Future research should focus on the application and dissemination of the advantages of using more advanced methods in public health, identifying circumstances where these methods are likely to be the most beneficial, and ways to make the methods more accessible, for example, the development of packages and web tools.
There is an evident need to facilitate the translation of the synthesis methods into a public health context and encourage the use of methods to improve decision making. This review has shown that the uptake of statistical methods for evaluating the effectiveness of public health interventions is slow, despite advances in methods that address specific issues in public health intervention appraisal and the publication of guidance documents to complement their application.
Acknowledgements
We would like to acknowledge Professor Denise Kendrick as the lead on the NIHR Keeping Children Safe at Home Programme that originally funded the collection of the evidence for the motivating example and some of the analyses illustrated in the paper.
Abbreviations
Authors’ contributions.
ES performed the review, analysed the data and wrote the paper. SH supervised the project. SH, KA, NC and AS provided substantial feedback on the manuscript. All authors have read and approved the manuscript.
ES is funded by a National Institute for Health Research (NIHR), Doctoral Research Fellow for this research project. This paper presents independent research funded by the National Institute for Health Research (NIHR). The views expressed are those of the authors and not necessarily those of the NHS, the NIHR or the Department of Health and Social Care. The funding bodies played no role in the design of the study and collection, analysis, and interpretation of data and in writing the manuscript.
Availability of data and materials
Ethics approval and consent to participate.
Not applicable.
Consent for publication
Competing interests.
KA is supported by Health Data Research (HDR) UK, the UK National Institute for Health Research (NIHR) Applied Research Collaboration East Midlands (ARC EM), and as a NIHR Senior Investigator Emeritus (NF-SI-0512-10159). The views expressed are those of the author(s) and not necessarily those of the NIHR or the Department of Health and Social Care. KA has served as a paid consultant, providing unrelated methodological advice, to; Abbvie, Amaris, Allergan, Astellas, AstraZeneca, Boehringer Ingelheim, Bristol-Meyers Squibb, Creativ-Ceutical, GSK, ICON/Oxford Outcomes, Ipsen, Janssen, Eli Lilly, Merck, NICE, Novartis, NovoNordisk, Pfizer, PRMA, Roche and Takeda, and has received research funding from Association of the British Pharmaceutical Industry (ABPI), European Federation of Pharmaceutical Industries & Associations (EFPIA), Pfizer, Sanofi and Swiss Precision Diagnostics. He is a Partner and Director of Visible Analytics Limited, a healthcare consultancy company.
Publisher’s Note
Springer Nature remains neutral with regard to jurisdictional claims in published maps and institutional affiliations.
Contributor Information
Ellesha A. Smith, Email: ku.ca.el@42sae .
Nicola J. Cooper, Email: ku.ca.retseciel@12cjn .
Alex J. Sutton, Email: ku.ca.retseciel@22sja .
Keith R. Abrams, Email: ku.ca.el@ark .
Stephanie J. Hubbard, Email: ku.ca.el@26hjs .
Click through the PLOS taxonomy to find articles in your field.
For more information about PLOS Subject Areas, click here .
Loading metrics
Open Access
Peer-reviewed
Research Article
Recent quantitative research on determinants of health in high income countries: A scoping review
Roles Conceptualization, Data curation, Formal analysis, Investigation, Methodology, Project administration, Software, Visualization, Writing – original draft, Writing – review & editing
* E-mail: [email protected]
Affiliation Centre for Health Economics Research and Modelling Infectious Diseases, Vaccine and Infectious Disease Institute, University of Antwerp, Antwerp, Belgium

Roles Conceptualization, Data curation, Funding acquisition, Project administration, Resources, Supervision, Validation, Visualization, Writing – review & editing
- Vladimira Varbanova,
- Philippe Beutels
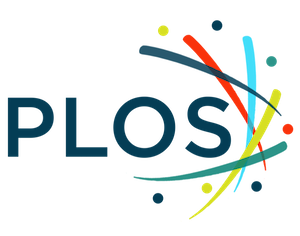
- Published: September 17, 2020
- https://doi.org/10.1371/journal.pone.0239031
- Peer Review
- Reader Comments
Identifying determinants of health and understanding their role in health production constitutes an important research theme. We aimed to document the state of recent multi-country research on this theme in the literature.
We followed the PRISMA-ScR guidelines to systematically identify, triage and review literature (January 2013—July 2019). We searched for studies that performed cross-national statistical analyses aiming to evaluate the impact of one or more aggregate level determinants on one or more general population health outcomes in high-income countries. To assess in which combinations and to what extent individual (or thematically linked) determinants had been studied together, we performed multidimensional scaling and cluster analysis.
Sixty studies were selected, out of an original yield of 3686. Life-expectancy and overall mortality were the most widely used population health indicators, while determinants came from the areas of healthcare, culture, politics, socio-economics, environment, labor, fertility, demographics, life-style, and psychology. The family of regression models was the predominant statistical approach. Results from our multidimensional scaling showed that a relatively tight core of determinants have received much attention, as main covariates of interest or controls, whereas the majority of other determinants were studied in very limited contexts. We consider findings from these studies regarding the importance of any given health determinant inconclusive at present. Across a multitude of model specifications, different country samples, and varying time periods, effects fluctuated between statistically significant and not significant, and between beneficial and detrimental to health.
Conclusions
We conclude that efforts to understand the underlying mechanisms of population health are far from settled, and the present state of research on the topic leaves much to be desired. It is essential that future research considers multiple factors simultaneously and takes advantage of more sophisticated methodology with regards to quantifying health as well as analyzing determinants’ influence.
Citation: Varbanova V, Beutels P (2020) Recent quantitative research on determinants of health in high income countries: A scoping review. PLoS ONE 15(9): e0239031. https://doi.org/10.1371/journal.pone.0239031
Editor: Amir Radfar, University of Central Florida, UNITED STATES
Received: November 14, 2019; Accepted: August 28, 2020; Published: September 17, 2020
Copyright: © 2020 Varbanova, Beutels. This is an open access article distributed under the terms of the Creative Commons Attribution License , which permits unrestricted use, distribution, and reproduction in any medium, provided the original author and source are credited.
Data Availability: All relevant data are within the manuscript and its Supporting Information files.
Funding: This study (and VV) is funded by the Research Foundation Flanders ( https://www.fwo.be/en/ ), FWO project number G0D5917N, award obtained by PB. The funder had no role in study design, data collection and analysis, decision to publish, or preparation of the manuscript.
Competing interests: The authors have declared that no competing interests exist.
Introduction
Identifying the key drivers of population health is a core subject in public health and health economics research. Between-country comparative research on the topic is challenging. In order to be relevant for policy, it requires disentangling different interrelated drivers of “good health”, each having different degrees of importance in different contexts.
“Good health”–physical and psychological, subjective and objective–can be defined and measured using a variety of approaches, depending on which aspect of health is the focus. A major distinction can be made between health measurements at the individual level or some aggregate level, such as a neighborhood, a region or a country. In view of this, a great diversity of specific research topics exists on the drivers of what constitutes individual or aggregate “good health”, including those focusing on health inequalities, the gender gap in longevity, and regional mortality and longevity differences.
The current scoping review focuses on determinants of population health. Stated as such, this topic is quite broad. Indeed, we are interested in the very general question of what methods have been used to make the most of increasingly available region or country-specific databases to understand the drivers of population health through inter-country comparisons. Existing reviews indicate that researchers thus far tend to adopt a narrower focus. Usually, attention is given to only one health outcome at a time, with further geographical and/or population [ 1 , 2 ] restrictions. In some cases, the impact of one or more interventions is at the core of the review [ 3 – 7 ], while in others it is the relationship between health and just one particular predictor, e.g., income inequality, access to healthcare, government mechanisms [ 8 – 13 ]. Some relatively recent reviews on the subject of social determinants of health [ 4 – 6 , 14 – 17 ] have considered a number of indicators potentially influencing health as opposed to a single one. One review defines “social determinants” as “the social, economic, and political conditions that influence the health of individuals and populations” [ 17 ] while another refers even more broadly to “the factors apart from medical care” [ 15 ].
In the present work, we aimed to be more inclusive, setting no limitations on the nature of possible health correlates, as well as making use of a multitude of commonly accepted measures of general population health. The goal of this scoping review was to document the state of the art in the recent published literature on determinants of population health, with a particular focus on the types of determinants selected and the methodology used. In doing so, we also report the main characteristics of the results these studies found. The materials collected in this review are intended to inform our (and potentially other researchers’) future analyses on this topic. Since the production of health is subject to the law of diminishing marginal returns, we focused our review on those studies that included countries where a high standard of wealth has been achieved for some time, i.e., high-income countries belonging to the Organisation for Economic Co-operation and Development (OECD) or Europe. Adding similar reviews for other country income groups is of limited interest to the research we plan to do in this area.
In view of its focus on data and methods, rather than results, a formal protocol was not registered prior to undertaking this review, but the procedure followed the guidelines of the PRISMA statement for scoping reviews [ 18 ].
We focused on multi-country studies investigating the potential associations between any aggregate level (region/city/country) determinant and general measures of population health (e.g., life expectancy, mortality rate).
Within the query itself, we listed well-established population health indicators as well as the six world regions, as defined by the World Health Organization (WHO). We searched only in the publications’ titles in order to keep the number of hits manageable, and the ratio of broadly relevant abstracts over all abstracts in the order of magnitude of 10% (based on a series of time-focused trial runs). The search strategy was developed iteratively between the two authors and is presented in S1 Appendix . The search was performed by VV in PubMed and Web of Science on the 16 th of July, 2019, without any language restrictions, and with a start date set to the 1 st of January, 2013, as we were interested in the latest developments in this area of research.
Eligibility criteria
Records obtained via the search methods described above were screened independently by the two authors. Consistency between inclusion/exclusion decisions was approximately 90% and the 43 instances where uncertainty existed were judged through discussion. Articles were included subject to meeting the following requirements: (a) the paper was a full published report of an original empirical study investigating the impact of at least one aggregate level (city/region/country) factor on at least one health indicator (or self-reported health) of the general population (the only admissible “sub-populations” were those based on gender and/or age); (b) the study employed statistical techniques (calculating correlations, at the very least) and was not purely descriptive or theoretical in nature; (c) the analysis involved at least two countries or at least two regions or cities (or another aggregate level) in at least two different countries; (d) the health outcome was not differentiated according to some socio-economic factor and thus studied in terms of inequality (with the exception of gender and age differentiations); (e) mortality, in case it was one of the health indicators under investigation, was strictly “total” or “all-cause” (no cause-specific or determinant-attributable mortality).
Data extraction
The following pieces of information were extracted in an Excel table from the full text of each eligible study (primarily by VV, consulting with PB in case of doubt): health outcome(s), determinants, statistical methodology, level of analysis, results, type of data, data sources, time period, countries. The evidence is synthesized according to these extracted data (often directly reflected in the section headings), using a narrative form accompanied by a “summary-of-findings” table and a graph.
Search and selection
The initial yield contained 4583 records, reduced to 3686 after removal of duplicates ( Fig 1 ). Based on title and abstract screening, 3271 records were excluded because they focused on specific medical condition(s) or specific populations (based on morbidity or some other factor), dealt with intervention effectiveness, with theoretical or non-health related issues, or with animals or plants. Of the remaining 415 papers, roughly half were disqualified upon full-text consideration, mostly due to using an outcome not of interest to us (e.g., health inequality), measuring and analyzing determinants and outcomes exclusively at the individual level, performing analyses one country at a time, employing indices that are a mixture of both health indicators and health determinants, or not utilizing potential health determinants at all. After this second stage of the screening process, 202 papers were deemed eligible for inclusion. This group was further dichotomized according to level of economic development of the countries or regions under study, using membership of the OECD or Europe as a reference “cut-off” point. Sixty papers were judged to include high-income countries, and the remaining 142 included either low- or middle-income countries or a mix of both these levels of development. The rest of this report outlines findings in relation to high-income countries only, reflecting our own primary research interests. Nonetheless, we chose to report our search yield for the other income groups for two reasons. First, to gauge the relative interest in applied published research for these different income levels; and second, to enable other researchers with a focus on determinants of health in other countries to use the extraction we made here.
- PPT PowerPoint slide
- PNG larger image
- TIFF original image
https://doi.org/10.1371/journal.pone.0239031.g001
Health outcomes
The most frequent population health indicator, life expectancy (LE), was present in 24 of the 60 studies. Apart from “life expectancy at birth” (representing the average life-span a newborn is expected to have if current mortality rates remain constant), also called “period LE” by some [ 19 , 20 ], we encountered as well LE at 40 years of age [ 21 ], at 60 [ 22 ], and at 65 [ 21 , 23 , 24 ]. In two papers, the age-specificity of life expectancy (be it at birth or another age) was not stated [ 25 , 26 ].
Some studies considered male and female LE separately [ 21 , 24 , 25 , 27 – 33 ]. This consideration was also often observed with the second most commonly used health index [ 28 – 30 , 34 – 38 ]–termed “total”, or “overall”, or “all-cause”, mortality rate (MR)–included in 22 of the 60 studies. In addition to gender, this index was also sometimes broken down according to age group [ 30 , 39 , 40 ], as well as gender-age group [ 38 ].
While the majority of studies under review here focused on a single health indicator, 23 out of the 60 studies made use of multiple outcomes, although these outcomes were always considered one at a time, and sometimes not all of them fell within the scope of our review. An easily discernable group of indices that typically went together [ 25 , 37 , 41 ] was that of neonatal (deaths occurring within 28 days postpartum), perinatal (fetal or early neonatal / first-7-days deaths), and post-neonatal (deaths between the 29 th day and completion of one year of life) mortality. More often than not, these indices were also accompanied by “stand-alone” indicators, such as infant mortality (deaths within the first year of life; our third most common index found in 16 of the 60 studies), maternal mortality (deaths during pregnancy or within 42 days of termination of pregnancy), and child mortality rates. Child mortality has conventionally been defined as mortality within the first 5 years of life, thus often also called “under-5 mortality”. Nonetheless, Pritchard & Wallace used the term “child mortality” to denote deaths of children younger than 14 years [ 42 ].
As previously stated, inclusion criteria did allow for self-reported health status to be used as a general measure of population health. Within our final selection of studies, seven utilized some form of subjective health as an outcome variable [ 25 , 43 – 48 ]. Additionally, the Health Human Development Index [ 49 ], healthy life expectancy [ 50 ], old-age survival [ 51 ], potential years of life lost [ 52 ], and disability-adjusted life expectancy [ 25 ] were also used.
We note that while in most cases the indicators mentioned above (and/or the covariates considered, see below) were taken in their absolute or logarithmic form, as a—typically annual—number, sometimes they were used in the form of differences, change rates, averages over a given time period, or even z-scores of rankings [ 19 , 22 , 40 , 42 , 44 , 53 – 57 ].
Regions, countries, and populations
Despite our decision to confine this review to high-income countries, some variation in the countries and regions studied was still present. Selection seemed to be most often conditioned on the European Union, or the European continent more generally, and the Organisation of Economic Co-operation and Development (OECD), though, typically, not all member nations–based on the instances where these were also explicitly listed—were included in a given study. Some of the stated reasons for omitting certain nations included data unavailability [ 30 , 45 , 54 ] or inconsistency [ 20 , 58 ], Gross Domestic Product (GDP) too low [ 40 ], differences in economic development and political stability with the rest of the sampled countries [ 59 ], and national population too small [ 24 , 40 ]. On the other hand, the rationales for selecting a group of countries included having similar above-average infant mortality [ 60 ], similar healthcare systems [ 23 ], and being randomly drawn from a social spending category [ 61 ]. Some researchers were interested explicitly in a specific geographical region, such as Eastern Europe [ 50 ], Central and Eastern Europe [ 48 , 60 ], the Visegrad (V4) group [ 62 ], or the Asia/Pacific area [ 32 ]. In certain instances, national regions or cities, rather than countries, constituted the units of investigation instead [ 31 , 51 , 56 , 62 – 66 ]. In two particular cases, a mix of countries and cities was used [ 35 , 57 ]. In another two [ 28 , 29 ], due to the long time periods under study, some of the included countries no longer exist. Finally, besides “European” and “OECD”, the terms “developed”, “Western”, and “industrialized” were also used to describe the group of selected nations [ 30 , 42 , 52 , 53 , 67 ].
As stated above, it was the health status of the general population that we were interested in, and during screening we made a concerted effort to exclude research using data based on a more narrowly defined group of individuals. All studies included in this review adhere to this general rule, albeit with two caveats. First, as cities (even neighborhoods) were the unit of analysis in three of the studies that made the selection [ 56 , 64 , 65 ], the populations under investigation there can be more accurately described as general urban , instead of just general. Second, oftentimes health indicators were stratified based on gender and/or age, therefore we also admitted one study that, due to its specific research question, focused on men and women of early retirement age [ 35 ] and another that considered adult males only [ 68 ].
Data types and sources
A great diversity of sources was utilized for data collection purposes. The accessible reference databases of the OECD ( https://www.oecd.org/ ), WHO ( https://www.who.int/ ), World Bank ( https://www.worldbank.org/ ), United Nations ( https://www.un.org/en/ ), and Eurostat ( https://ec.europa.eu/eurostat ) were among the top choices. The other international databases included Human Mortality [ 30 , 39 , 50 ], Transparency International [ 40 , 48 , 50 ], Quality of Government [ 28 , 69 ], World Income Inequality [ 30 ], International Labor Organization [ 41 ], International Monetary Fund [ 70 ]. A number of national databases were referred to as well, for example the US Bureau of Statistics [ 42 , 53 ], Korean Statistical Information Services [ 67 ], Statistics Canada [ 67 ], Australian Bureau of Statistics [ 67 ], and Health New Zealand Tobacco control and Health New Zealand Food and Nutrition [ 19 ]. Well-known surveys, such as the World Values Survey [ 25 , 55 ], the European Social Survey [ 25 , 39 , 44 ], the Eurobarometer [ 46 , 56 ], the European Value Survey [ 25 ], and the European Statistics of Income and Living Condition Survey [ 43 , 47 , 70 ] were used as data sources, too. Finally, in some cases [ 25 , 28 , 29 , 35 , 36 , 41 , 69 ], built-for-purpose datasets from previous studies were re-used.
In most of the studies, the level of the data (and analysis) was national. The exceptions were six papers that dealt with Nomenclature of Territorial Units of Statistics (NUTS2) regions [ 31 , 62 , 63 , 66 ], otherwise defined areas [ 51 ] or cities [ 56 ], and seven others that were multilevel designs and utilized both country- and region-level data [ 57 ], individual- and city- or country-level [ 35 ], individual- and country-level [ 44 , 45 , 48 ], individual- and neighborhood-level [ 64 ], and city-region- (NUTS3) and country-level data [ 65 ]. Parallel to that, the data type was predominantly longitudinal, with only a few studies using purely cross-sectional data [ 25 , 33 , 43 , 45 – 48 , 50 , 62 , 67 , 68 , 71 , 72 ], albeit in four of those [ 43 , 48 , 68 , 72 ] two separate points in time were taken (thus resulting in a kind of “double cross-section”), while in another the averages across survey waves were used [ 56 ].
In studies using longitudinal data, the length of the covered time periods varied greatly. Although this was almost always less than 40 years, in one study it covered the entire 20 th century [ 29 ]. Longitudinal data, typically in the form of annual records, was sometimes transformed before usage. For example, some researchers considered data points at 5- [ 34 , 36 , 49 ] or 10-year [ 27 , 29 , 35 ] intervals instead of the traditional 1, or took averages over 3-year periods [ 42 , 53 , 73 ]. In one study concerned with the effect of the Great Recession all data were in a “recession minus expansion change in trends”-form [ 57 ]. Furthermore, there were a few instances where two different time periods were compared to each other [ 42 , 53 ] or when data was divided into 2 to 4 (possibly overlapping) periods which were then analyzed separately [ 24 , 26 , 28 , 29 , 31 , 65 ]. Lastly, owing to data availability issues, discrepancies between the time points or periods of data on the different variables were occasionally observed [ 22 , 35 , 42 , 53 – 55 , 63 ].
Health determinants
Together with other essential details, Table 1 lists the health correlates considered in the selected studies. Several general categories for these correlates can be discerned, including health care, political stability, socio-economics, demographics, psychology, environment, fertility, life-style, culture, labor. All of these, directly or implicitly, have been recognized as holding importance for population health by existing theoretical models of (social) determinants of health [ 74 – 77 ].
https://doi.org/10.1371/journal.pone.0239031.t001
It is worth noting that in a few studies there was just a single aggregate-level covariate investigated in relation to a health outcome of interest to us. In one instance, this was life satisfaction [ 44 ], in another–welfare system typology [ 45 ], but also gender inequality [ 33 ], austerity level [ 70 , 78 ], and deprivation [ 51 ]. Most often though, attention went exclusively to GDP [ 27 , 29 , 46 , 57 , 65 , 71 ]. It was often the case that research had a more particular focus. Among others, minimum wages [ 79 ], hospital payment schemes [ 23 ], cigarette prices [ 63 ], social expenditure [ 20 ], residents’ dissatisfaction [ 56 ], income inequality [ 30 , 69 ], and work leave [ 41 , 58 ] took center stage. Whenever variables outside of these specific areas were also included, they were usually identified as confounders or controls, moderators or mediators.
We visualized the combinations in which the different determinants have been studied in Fig 2 , which was obtained via multidimensional scaling and a subsequent cluster analysis (details outlined in S2 Appendix ). It depicts the spatial positioning of each determinant relative to all others, based on the number of times the effects of each pair of determinants have been studied simultaneously. When interpreting Fig 2 , one should keep in mind that determinants marked with an asterisk represent, in fact, collectives of variables.
Groups of determinants are marked by asterisks (see S1 Table in S1 Appendix ). Diminishing color intensity reflects a decrease in the total number of “connections” for a given determinant. Noteworthy pairwise “connections” are emphasized via lines (solid-dashed-dotted indicates decreasing frequency). Grey contour lines encircle groups of variables that were identified via cluster analysis. Abbreviations: age = population age distribution, associations = membership in associations, AT-index = atherogenic-thrombogenic index, BR = birth rate, CAPB = Cyclically Adjusted Primary Balance, civilian-labor = civilian labor force, C-section = Cesarean delivery rate, credit-info = depth of credit information, dissatisf = residents’ dissatisfaction, distrib.orient = distributional orientation, EDU = education, eHealth = eHealth index at GP-level, exch.rate = exchange rate, fat = fat consumption, GDP = gross domestic product, GFCF = Gross Fixed Capital Formation/Creation, GH-gas = greenhouse gas, GII = gender inequality index, gov = governance index, gov.revenue = government revenues, HC-coverage = healthcare coverage, HE = health(care) expenditure, HHconsump = household consumption, hosp.beds = hospital beds, hosp.payment = hospital payment scheme, hosp.stay = length of hospital stay, IDI = ICT development index, inc.ineq = income inequality, industry-labor = industrial labor force, infant-sex = infant sex ratio, labor-product = labor production, LBW = low birth weight, leave = work leave, life-satisf = life satisfaction, M-age = maternal age, marginal-tax = marginal tax rate, MDs = physicians, mult.preg = multiple pregnancy, NHS = Nation Health System, NO = nitrous oxide emissions, PM10 = particulate matter (PM10) emissions, pop = population size, pop.density = population density, pre-term = pre-term birth rate, prison = prison population, researchE = research&development expenditure, school.ref = compulsory schooling reform, smoke-free = smoke-free places, SO = sulfur oxide emissions, soc.E = social expenditure, soc.workers = social workers, sugar = sugar consumption, terror = terrorism, union = union density, UR = unemployment rate, urban = urbanization, veg-fr = vegetable-and-fruit consumption, welfare = welfare regime, Wwater = wastewater treatment.
https://doi.org/10.1371/journal.pone.0239031.g002
Distances between determinants in Fig 2 are indicative of determinants’ “connectedness” with each other. While the statistical procedure called for higher dimensionality of the model, for demonstration purposes we show here a two-dimensional solution. This simplification unfortunately comes with a caveat. To use the factor smoking as an example, it would appear it stands at a much greater distance from GDP than it does from alcohol. In reality however, smoking was considered together with alcohol consumption [ 21 , 25 , 26 , 52 , 68 ] in just as many studies as it was with GDP [ 21 , 25 , 26 , 52 , 59 ], five. To aid with respect to this apparent shortcoming, we have emphasized the strongest pairwise links. Solid lines connect GDP with health expenditure (HE), unemployment rate (UR), and education (EDU), indicating that the effect of GDP on health, taking into account the effects of the other three determinants as well, was evaluated in between 12 to 16 studies of the 60 included in this review. Tracing the dashed lines, we can also tell that GDP appeared jointly with income inequality, and HE together with either EDU or UR, in anywhere between 8 to 10 of our selected studies. Finally, some weaker but still worth-mentioning “connections” between variables are displayed as well via the dotted lines.
The fact that all notable pairwise “connections” are concentrated within a relatively small region of the plot may be interpreted as low overall “connectedness” among the health indicators studied. GDP is the most widely investigated determinant in relation to general population health. Its total number of “connections” is disproportionately high (159) compared to its runner-up–HE (with 113 “connections”), and then subsequently EDU (with 90) and UR (with 86). In fact, all of these determinants could be thought of as outliers, given that none of the remaining factors have a total count of pairings above 52. This decrease in individual determinants’ overall “connectedness” can be tracked on the graph via the change of color intensity as we move outwards from the symbolic center of GDP and its closest “co-determinants”, to finally reach the other extreme of the ten indicators (welfare regime, household consumption, compulsory school reform, life satisfaction, government revenues, literacy, research expenditure, multiple pregnancy, Cyclically Adjusted Primary Balance, and residents’ dissatisfaction; in white) the effects on health of which were only studied in isolation.
Lastly, we point to the few small but stable clusters of covariates encircled by the grey bubbles on Fig 2 . These groups of determinants were identified as “close” by both statistical procedures used for the production of the graph (see details in S2 Appendix ).
Statistical methodology
There was great variation in the level of statistical detail reported. Some authors provided too vague a description of their analytical approach, necessitating some inference in this section.
The issue of missing data is a challenging reality in this field of research, but few of the studies under review (12/60) explain how they dealt with it. Among the ones that do, three general approaches to handling missingness can be identified, listed in increasing level of sophistication: case-wise deletion, i.e., removal of countries from the sample [ 20 , 45 , 48 , 58 , 59 ], (linear) interpolation [ 28 , 30 , 34 , 58 , 59 , 63 ], and multiple imputation [ 26 , 41 , 52 ].
Correlations, Pearson, Spearman, or unspecified, were the only technique applied with respect to the health outcomes of interest in eight analyses [ 33 , 42 – 44 , 46 , 53 , 57 , 61 ]. Among the more advanced statistical methods, the family of regression models proved to be, by and large, predominant. Before examining this closer, we note the techniques that were, in a way, “unique” within this selection of studies: meta-analyses were performed (random and fixed effects, respectively) on the reduced form and 2-sample two stage least squares (2SLS) estimations done within countries [ 39 ]; difference-in-difference (DiD) analysis was applied in one case [ 23 ]; dynamic time-series methods, among which co-integration, impulse-response function (IRF), and panel vector autoregressive (VAR) modeling, were utilized in one study [ 80 ]; longitudinal generalized estimating equation (GEE) models were developed on two occasions [ 70 , 78 ]; hierarchical Bayesian spatial models [ 51 ] and special autoregressive regression [ 62 ] were also implemented.
Purely cross-sectional data analyses were performed in eight studies [ 25 , 45 , 47 , 50 , 55 , 56 , 67 , 71 ]. These consisted of linear regression (assumed ordinary least squares (OLS)), generalized least squares (GLS) regression, and multilevel analyses. However, six other studies that used longitudinal data in fact had a cross-sectional design, through which they applied regression at multiple time-points separately [ 27 , 29 , 36 , 48 , 68 , 72 ].
Apart from these “multi-point cross-sectional studies”, some other simplistic approaches to longitudinal data analysis were found, involving calculating and regressing 3-year averages of both the response and the predictor variables [ 54 ], taking the average of a few data-points (i.e., survey waves) [ 56 ] or using difference scores over 10-year [ 19 , 29 ] or unspecified time intervals [ 40 , 55 ].
Moving further in the direction of more sensible longitudinal data usage, we turn to the methods widely known among (health) economists as “panel data analysis” or “panel regression”. Most often seen were models with fixed effects for country/region and sometimes also time-point (occasionally including a country-specific trend as well), with robust standard errors for the parameter estimates to take into account correlations among clustered observations [ 20 , 21 , 24 , 28 , 30 , 32 , 34 , 37 , 38 , 41 , 52 , 59 , 60 , 63 , 66 , 69 , 73 , 79 , 81 , 82 ]. The Hausman test [ 83 ] was sometimes mentioned as the tool used to decide between fixed and random effects [ 26 , 49 , 63 , 66 , 73 , 82 ]. A few studies considered the latter more appropriate for their particular analyses, with some further specifying that (feasible) GLS estimation was employed [ 26 , 34 , 49 , 58 , 60 , 73 ]. Apart from these two types of models, the first differences method was encountered once as well [ 31 ]. Across all, the error terms were sometimes assumed to come from a first-order autoregressive process (AR(1)), i.e., they were allowed to be serially correlated [ 20 , 30 , 38 , 58 – 60 , 73 ], and lags of (typically) predictor variables were included in the model specification, too [ 20 , 21 , 37 , 38 , 48 , 69 , 81 ]. Lastly, a somewhat different approach to longitudinal data analysis was undertaken in four studies [ 22 , 35 , 48 , 65 ] in which multilevel–linear or Poisson–models were developed.
Regardless of the exact techniques used, most studies included in this review presented multiple model applications within their main analysis. None attempted to formally compare models in order to identify the “best”, even if goodness-of-fit statistics were occasionally reported. As indicated above, many studies investigated women’s and men’s health separately [ 19 , 21 , 22 , 27 – 29 , 31 , 33 , 35 , 36 , 38 , 39 , 45 , 50 , 51 , 64 , 65 , 69 , 82 ], and covariates were often tested one at a time, including other covariates only incrementally [ 20 , 25 , 28 , 36 , 40 , 50 , 55 , 67 , 73 ]. Furthermore, there were a few instances where analyses within countries were performed as well [ 32 , 39 , 51 ] or where the full time period of interest was divided into a few sub-periods [ 24 , 26 , 28 , 31 ]. There were also cases where different statistical techniques were applied in parallel [ 29 , 55 , 60 , 66 , 69 , 73 , 82 ], sometimes as a form of sensitivity analysis [ 24 , 26 , 30 , 58 , 73 ]. However, the most common approach to sensitivity analysis was to re-run models with somewhat different samples [ 39 , 50 , 59 , 67 , 69 , 80 , 82 ]. Other strategies included different categorization of variables or adding (more/other) controls [ 21 , 23 , 25 , 28 , 37 , 50 , 63 , 69 ], using an alternative main covariate measure [ 59 , 82 ], including lags for predictors or outcomes [ 28 , 30 , 58 , 63 , 65 , 79 ], using weights [ 24 , 67 ] or alternative data sources [ 37 , 69 ], or using non-imputed data [ 41 ].
As the methods and not the findings are the main focus of the current review, and because generic checklists cannot discern the underlying quality in this application field (see also below), we opted to pool all reported findings together, regardless of individual study characteristics or particular outcome(s) used, and speak generally of positive and negative effects on health. For this summary we have adopted the 0.05-significance level and only considered results from multivariate analyses. Strictly birth-related factors are omitted since these potentially only relate to the group of infant mortality indicators and not to any of the other general population health measures.
Starting with the determinants most often studied, higher GDP levels [ 21 , 26 , 27 , 29 , 30 , 32 , 43 , 48 , 52 , 58 , 60 , 66 , 67 , 73 , 79 , 81 , 82 ], higher health [ 21 , 37 , 47 , 49 , 52 , 58 , 59 , 68 , 72 , 82 ] and social [ 20 , 21 , 26 , 38 , 79 ] expenditures, higher education [ 26 , 39 , 52 , 62 , 72 , 73 ], lower unemployment [ 60 , 61 , 66 ], and lower income inequality [ 30 , 42 , 53 , 55 , 73 ] were found to be significantly associated with better population health on a number of occasions. In addition to that, there was also some evidence that democracy [ 36 ] and freedom [ 50 ], higher work compensation [ 43 , 79 ], distributional orientation [ 54 ], cigarette prices [ 63 ], gross national income [ 22 , 72 ], labor productivity [ 26 ], exchange rates [ 32 ], marginal tax rates [ 79 ], vaccination rates [ 52 ], total fertility [ 59 , 66 ], fruit and vegetable [ 68 ], fat [ 52 ] and sugar consumption [ 52 ], as well as bigger depth of credit information [ 22 ] and percentage of civilian labor force [ 79 ], longer work leaves [ 41 , 58 ], more physicians [ 37 , 52 , 72 ], nurses [ 72 ], and hospital beds [ 79 , 82 ], and also membership in associations, perceived corruption and societal trust [ 48 ] were beneficial to health. Higher nitrous oxide (NO) levels [ 52 ], longer average hospital stay [ 48 ], deprivation [ 51 ], dissatisfaction with healthcare and the social environment [ 56 ], corruption [ 40 , 50 ], smoking [ 19 , 26 , 52 , 68 ], alcohol consumption [ 26 , 52 , 68 ] and illegal drug use [ 68 ], poverty [ 64 ], higher percentage of industrial workers [ 26 ], Gross Fixed Capital creation [ 66 ] and older population [ 38 , 66 , 79 ], gender inequality [ 22 ], and fertility [ 26 , 66 ] were detrimental.
It is important to point out that the above-mentioned effects could not be considered stable either across or within studies. Very often, statistical significance of a given covariate fluctuated between the different model specifications tried out within the same study [ 20 , 49 , 59 , 66 , 68 , 69 , 73 , 80 , 82 ], testifying to the importance of control variables and multivariate research (i.e., analyzing multiple independent variables simultaneously) in general. Furthermore, conflicting results were observed even with regards to the “core” determinants given special attention, so to speak, throughout this text. Thus, some studies reported negative effects of health expenditure [ 32 , 82 ], social expenditure [ 58 ], GDP [ 49 , 66 ], and education [ 82 ], and positive effects of income inequality [ 82 ] and unemployment [ 24 , 31 , 32 , 52 , 66 , 68 ]. Interestingly, one study [ 34 ] differentiated between temporary and long-term effects of GDP and unemployment, alluding to possibly much greater complexity of the association with health. It is also worth noting that some gender differences were found, with determinants being more influential for males than for females, or only having statistically significant effects for male health [ 19 , 21 , 28 , 34 , 36 , 37 , 39 , 64 , 65 , 69 ].
The purpose of this scoping review was to examine recent quantitative work on the topic of multi-country analyses of determinants of population health in high-income countries.
Measuring population health via relatively simple mortality-based indicators still seems to be the state of the art. What is more, these indicators are routinely considered one at a time, instead of, for example, employing existing statistical procedures to devise a more general, composite, index of population health, or using some of the established indices, such as disability-adjusted life expectancy (DALE) or quality-adjusted life expectancy (QALE). Although strong arguments for their wider use were already voiced decades ago [ 84 ], such summary measures surface only rarely in this research field.
On a related note, the greater data availability and accessibility that we enjoy today does not automatically equate to data quality. Nonetheless, this is routinely assumed in aggregate level studies. We almost never encountered a discussion on the topic. The non-mundane issue of data missingness, too, goes largely underappreciated. With all recent methodological advancements in this area [ 85 – 88 ], there is no excuse for ignorance; and still, too few of the reviewed studies tackled the matter in any adequate fashion.
Much optimism can be gained considering the abundance of different determinants that have attracted researchers’ attention in relation to population health. We took on a visual approach with regards to these determinants and presented a graph that links spatial distances between determinants with frequencies of being studies together. To facilitate interpretation, we grouped some variables, which resulted in some loss of finer detail. Nevertheless, the graph is helpful in exemplifying how many effects continue to be studied in a very limited context, if any. Since in reality no factor acts in isolation, this oversimplification practice threatens to render the whole exercise meaningless from the outset. The importance of multivariate analysis cannot be stressed enough. While there is no “best method” to be recommended and appropriate techniques vary according to the specifics of the research question and the characteristics of the data at hand [ 89 – 93 ], in the future, in addition to abandoning simplistic univariate approaches, we hope to see a shift from the currently dominating fixed effects to the more flexible random/mixed effects models [ 94 ], as well as wider application of more sophisticated methods, such as principle component regression, partial least squares, covariance structure models (e.g., structural equations), canonical correlations, time-series, and generalized estimating equations.
Finally, there are some limitations of the current scoping review. We searched the two main databases for published research in medical and non-medical sciences (PubMed and Web of Science) since 2013, thus potentially excluding publications and reports that are not indexed in these databases, as well as older indexed publications. These choices were guided by our interest in the most recent (i.e., the current state-of-the-art) and arguably the highest-quality research (i.e., peer-reviewed articles, primarily in indexed non-predatory journals). Furthermore, despite holding a critical stance with regards to some aspects of how determinants-of-health research is currently conducted, we opted out of formally assessing the quality of the individual studies included. The reason for that is two-fold. On the one hand, we are unaware of the existence of a formal and standard tool for quality assessment of ecological designs. And on the other, we consider trying to score the quality of these diverse studies (in terms of regional setting, specific topic, outcome indices, and methodology) undesirable and misleading, particularly since we would sometimes have been rating the quality of only a (small) part of the original studies—the part that was relevant to our review’s goal.
Our aim was to investigate the current state of research on the very broad and general topic of population health, specifically, the way it has been examined in a multi-country context. We learned that data treatment and analytical approach were, in the majority of these recent studies, ill-equipped or insufficiently transparent to provide clarity regarding the underlying mechanisms of population health in high-income countries. Whether due to methodological shortcomings or the inherent complexity of the topic, research so far fails to provide any definitive answers. It is our sincere belief that with the application of more advanced analytical techniques this continuous quest could come to fruition sooner.
Supporting information
S1 checklist. preferred reporting items for systematic reviews and meta-analyses extension for scoping reviews (prisma-scr) checklist..
https://doi.org/10.1371/journal.pone.0239031.s001
S1 Appendix.
https://doi.org/10.1371/journal.pone.0239031.s002
S2 Appendix.
https://doi.org/10.1371/journal.pone.0239031.s003
- View Article
- Google Scholar
- PubMed/NCBI
- 75. Dahlgren G, Whitehead M. Policies and Strategies to Promote Equity in Health. Stockholm, Sweden: Institute for Future Studies; 1991.
- 76. Brunner E, Marmot M. Social Organization, Stress, and Health. In: Marmot M, Wilkinson RG, editors. Social Determinants of Health. Oxford, England: Oxford University Press; 1999.
- 77. Najman JM. A General Model of the Social Origins of Health and Well-being. In: Eckersley R, Dixon J, Douglas B, editors. The Social Origins of Health and Well-being. Cambridge, England: Cambridge University Press; 2001.
- 85. Carpenter JR, Kenward MG. Multiple Imputation and its Application. New York: John Wiley & Sons; 2013.
- 86. Molenberghs G, Fitzmaurice G, Kenward MG, Verbeke G, Tsiatis AA. Handbook of Missing Data Methodology. Boca Raton: Chapman & Hall/CRC; 2014.
- 87. van Buuren S. Flexible Imputation of Missing Data. 2nd ed. Boca Raton: Chapman & Hall/CRC; 2018.
- 88. Enders CK. Applied Missing Data Analysis. New York: Guilford; 2010.
- 89. Shayle R. Searle GC, Charles E. McCulloch. Variance Components: John Wiley & Sons, Inc.; 1992.
- 90. Agresti A. Foundations of Linear and Generalized Linear Models. Hoboken, New Jersey: John Wiley & Sons Inc.; 2015.
- 91. Leyland A. H. (Editor) HGE. Multilevel Modelling of Health Statistics: John Wiley & Sons Inc; 2001.
- 92. Garrett Fitzmaurice MD, Geert Verbeke, Geert Molenberghs. Longitudinal Data Analysis. New York: Chapman and Hall/CRC; 2008.
- 93. Wolfgang Karl Härdle LS. Applied Multivariate Statistical Analysis. Berlin, Heidelberg: Springer; 2015.
Advertisement

- Previous Issue
- Previous Article
- Next Article
Clarifying the Research Purpose
Methodology, measurement, data analysis and interpretation, tools for evaluating the quality of medical education research, research support, competing interests, quantitative research methods in medical education.
Submitted for publication January 8, 2018. Accepted for publication November 29, 2018.
- Split-Screen
- Article contents
- Figures & tables
- Supplementary Data
- Peer Review
- Open the PDF for in another window
- Cite Icon Cite
- Get Permissions
- Search Site
John T. Ratelle , Adam P. Sawatsky , Thomas J. Beckman; Quantitative Research Methods in Medical Education. Anesthesiology 2019; 131:23–35 doi: https://doi.org/10.1097/ALN.0000000000002727
Download citation file:
- Ris (Zotero)
- Reference Manager
There has been a dramatic growth of scholarly articles in medical education in recent years. Evaluating medical education research requires specific orientation to issues related to format and content. Our goal is to review the quantitative aspects of research in medical education so that clinicians may understand these articles with respect to framing the study, recognizing methodologic issues, and utilizing instruments for evaluating the quality of medical education research. This review can be used both as a tool when appraising medical education research articles and as a primer for clinicians interested in pursuing scholarship in medical education.

Image: J. P. Rathmell and Terri Navarette.
There has been an explosion of research in the field of medical education. A search of PubMed demonstrates that more than 40,000 articles have been indexed under the medical subject heading “Medical Education” since 2010, which is more than the total number of articles indexed under this heading in the 1980s and 1990s combined. Keeping up to date requires that practicing clinicians have the skills to interpret and appraise the quality of research articles, especially when serving as editors, reviewers, and consumers of the literature.
While medical education shares many characteristics with other biomedical fields, substantial particularities exist. We recognize that practicing clinicians may not be familiar with the nuances of education research and how to assess its quality. Therefore, our purpose is to provide a review of quantitative research methodologies in medical education. Specifically, we describe a structure that can be used when conducting or evaluating medical education research articles.
Clarifying the research purpose is an essential first step when reading or conducting scholarship in medical education. 1 Medical education research can serve a variety of purposes, from advancing the science of learning to improving the outcomes of medical trainees and the patients they care for. However, a well-designed study has limited value if it addresses vague, redundant, or unimportant medical education research questions.
What is the research topic and why is it important? What is unknown about the research topic? Why is further research necessary?
What is the conceptual framework being used to approach the study?
What is the statement of study intent?
What are the research methodology and study design? Are they appropriate for the study objective(s)?
Which threats to internal validity are most relevant for the study?
What is the outcome and how was it measured?
Can the results be trusted? What is the validity and reliability of the measurements?
How were research subjects selected? Is the research sample representative of the target population?
Was the data analysis appropriate for the study design and type of data?
What is the effect size? Do the results have educational significance?
Fortunately, there are steps to ensure that the purpose of a research study is clear and logical. Table 1 2–5 outlines these steps, which will be described in detail in the following sections. We describe these elements not as a simple “checklist,” but as an advanced organizer that can be used to understand a medical education research study. These steps can also be used by clinician educators who are new to the field of education research and who wish to conduct scholarship in medical education.
Steps in Clarifying the Purpose of a Research Study in Medical Education

Literature Review and Problem Statement
A literature review is the first step in clarifying the purpose of a medical education research article. 2 , 5 , 6 When conducting scholarship in medical education, a literature review helps researchers develop an understanding of their topic of interest. This understanding includes both existing knowledge about the topic as well as key gaps in the literature, which aids the researcher in refining their study question. Additionally, a literature review helps researchers identify conceptual frameworks that have been used to approach the research topic. 2
When reading scholarship in medical education, a successful literature review provides background information so that even someone unfamiliar with the research topic can understand the rationale for the study. Located in the introduction of the manuscript, the literature review guides the reader through what is already known in a manner that highlights the importance of the research topic. The literature review should also identify key gaps in the literature so the reader can understand the need for further research. This gap description includes an explicit problem statement that summarizes the important issues and provides a reason for the study. 2 , 4 The following is one example of a problem statement:
“Identifying gaps in the competency of anesthesia residents in time for intervention is critical to patient safety and an effective learning system… [However], few available instruments relate to complex behavioral performance or provide descriptors…that could inform subsequent feedback, individualized teaching, remediation, and curriculum revision.” 7
This problem statement articulates the research topic (identifying resident performance gaps), why it is important (to intervene for the sake of learning and patient safety), and current gaps in the literature (few tools are available to assess resident performance). The researchers have now underscored why further research is needed and have helped readers anticipate the overarching goals of their study (to develop an instrument to measure anesthesiology resident performance). 4
The Conceptual Framework
Following the literature review and articulation of the problem statement, the next step in clarifying the research purpose is to select a conceptual framework that can be applied to the research topic. Conceptual frameworks are “ways of thinking about a problem or a study, or ways of representing how complex things work.” 3 Just as clinical trials are informed by basic science research in the laboratory, conceptual frameworks often serve as the “basic science” that informs scholarship in medical education. At a fundamental level, conceptual frameworks provide a structured approach to solving the problem identified in the problem statement.
Conceptual frameworks may take the form of theories, principles, or models that help to explain the research problem by identifying its essential variables or elements. Alternatively, conceptual frameworks may represent evidence-based best practices that researchers can apply to an issue identified in the problem statement. 3 Importantly, there is no single best conceptual framework for a particular research topic, although the choice of a conceptual framework is often informed by the literature review and knowing which conceptual frameworks have been used in similar research. 8 For further information on selecting a conceptual framework for research in medical education, we direct readers to the work of Bordage 3 and Irby et al. 9
To illustrate how different conceptual frameworks can be applied to a research problem, suppose you encounter a study to reduce the frequency of communication errors among anesthesiology residents during day-to-night handoff. Table 2 10 , 11 identifies two different conceptual frameworks researchers might use to approach the task. The first framework, cognitive load theory, has been proposed as a conceptual framework to identify potential variables that may lead to handoff errors. 12 Specifically, cognitive load theory identifies the three factors that affect short-term memory and thus may lead to communication errors:
Conceptual Frameworks to Address the Issue of Handoff Errors in the Intensive Care Unit

Intrinsic load: Inherent complexity or difficulty of the information the resident is trying to learn ( e.g. , complex patients).
Extraneous load: Distractions or demands on short-term memory that are not related to the information the resident is trying to learn ( e.g. , background noise, interruptions).
Germane load: Effort or mental strategies used by the resident to organize and understand the information he/she is trying to learn ( e.g. , teach back, note taking).
Using cognitive load theory as a conceptual framework, researchers may design an intervention to reduce extraneous load and help the resident remember the overnight to-do’s. An example might be dedicated, pager-free handoff times where distractions are minimized.
The second framework identified in table 2 , the I-PASS (Illness severity, Patient summary, Action list, Situational awareness and contingency planning, and Synthesis by receiver) handoff mnemonic, 11 is an evidence-based best practice that, when incorporated as part of a handoff bundle, has been shown to reduce handoff errors on pediatric wards. 13 Researchers choosing this conceptual framework may adapt some or all of the I-PASS elements for resident handoffs in the intensive care unit.
Note that both of the conceptual frameworks outlined above provide researchers with a structured approach to addressing the issue of handoff errors; one is not necessarily better than the other. Indeed, it is possible for researchers to use both frameworks when designing their study. Ultimately, we provide this example to demonstrate the necessity of selecting conceptual frameworks to clarify the research purpose. 3 , 8 Readers should look for conceptual frameworks in the introduction section and should be wary of their omission, as commonly seen in less well-developed medical education research articles. 14
Statement of Study Intent
After reviewing the literature, articulating the problem statement, and selecting a conceptual framework to address the research topic, the final step in clarifying the research purpose is the statement of study intent. The statement of study intent is arguably the most important element of framing the study because it makes the research purpose explicit. 2 Consider the following example:
This study aimed to test the hypothesis that the introduction of the BASIC Examination was associated with an accelerated knowledge acquisition during residency training, as measured by increments in annual ITE scores. 15
This statement of study intent succinctly identifies several key study elements including the population (anesthesiology residents), the intervention/independent variable (introduction of the BASIC Examination), the outcome/dependent variable (knowledge acquisition, as measure by in In-training Examination [ITE] scores), and the hypothesized relationship between the independent and dependent variable (the authors hypothesize a positive correlation between the BASIC examination and the speed of knowledge acquisition). 6 , 14
The statement of study intent will sometimes manifest as a research objective, rather than hypothesis or question. In such instances there may not be explicit independent and dependent variables, but the study population and research aim should be clearly identified. The following is an example:
“In this report, we present the results of 3 [years] of course data with respect to the practice improvements proposed by participating anesthesiologists and their success in implementing those plans. Specifically, our primary aim is to assess the frequency and type of improvements that were completed and any factors that influence completion.” 16
The statement of study intent is the logical culmination of the literature review, problem statement, and conceptual framework, and is a transition point between the Introduction and Methods sections of a medical education research report. Nonetheless, a systematic review of experimental research in medical education demonstrated that statements of study intent are absent in the majority of articles. 14 When reading a medical education research article where the statement of study intent is absent, it may be necessary to infer the research aim by gathering information from the Introduction and Methods sections. In these cases, it can be useful to identify the following key elements 6 , 14 , 17 :
Population of interest/type of learner ( e.g. , pain medicine fellow or anesthesiology residents)
Independent/predictor variable ( e.g. , educational intervention or characteristic of the learners)
Dependent/outcome variable ( e.g. , intubation skills or knowledge of anesthetic agents)
Relationship between the variables ( e.g. , “improve” or “mitigate”)
Occasionally, it may be difficult to differentiate the independent study variable from the dependent study variable. 17 For example, consider a study aiming to measure the relationship between burnout and personal debt among anesthesiology residents. Do the researchers believe burnout might lead to high personal debt, or that high personal debt may lead to burnout? This “chicken or egg” conundrum reinforces the importance of the conceptual framework which, if present, should serve as an explanation or rationale for the predicted relationship between study variables.
Research methodology is the “…design or plan that shapes the methods to be used in a study.” 1 Essentially, methodology is the general strategy for answering a research question, whereas methods are the specific steps and techniques that are used to collect data and implement the strategy. Our objective here is to provide an overview of quantitative methodologies ( i.e. , approaches) in medical education research.
The choice of research methodology is made by balancing the approach that best answers the research question against the feasibility of completing the study. There is no perfect methodology because each has its own potential caveats, flaws and/or sources of bias. Before delving into an overview of the methodologies, it is important to highlight common sources of bias in education research. We use the term internal validity to describe the degree to which the findings of a research study represent “the truth,” as opposed to some alternative hypothesis or variables. 18 Table 3 18–20 provides a list of common threats to internal validity in medical education research, along with tactics to mitigate these threats.
Threats to Internal Validity and Strategies to Mitigate Their Effects

Experimental Research
The fundamental tenet of experimental research is the manipulation of an independent or experimental variable to measure its effect on a dependent or outcome variable.
True Experiment
True experimental study designs minimize threats to internal validity by randomizing study subjects to experimental and control groups. Through ensuring that differences between groups are—beyond the intervention/variable of interest—purely due to chance, researchers reduce the internal validity threats related to subject characteristics, time-related maturation, and regression to the mean. 18 , 19
Quasi-experiment
There are many instances in medical education where randomization may not be feasible or ethical. For instance, researchers wanting to test the effect of a new curriculum among medical students may not be able to randomize learners due to competing curricular obligations and schedules. In these cases, researchers may be forced to assign subjects to experimental and control groups based upon some other criterion beyond randomization, such as different classrooms or different sections of the same course. This process, called quasi-randomization, does not inherently lead to internal validity threats, as long as research investigators are mindful of measuring and controlling for extraneous variables between study groups. 19
Single-group Methodologies
All experimental study designs compare two or more groups: experimental and control. A common experimental study design in medical education research is the single-group pretest–posttest design, which compares a group of learners before and after the implementation of an intervention. 21 In essence, a single-group pre–post design compares an experimental group ( i.e. , postintervention) to a “no-intervention” control group ( i.e. , preintervention). 19 This study design is problematic for several reasons. Consider the following hypothetical example: A research article reports the effects of a year-long intubation curriculum for first-year anesthesiology residents. All residents participate in monthly, half-day workshops over the course of an academic year. The article reports a positive effect on residents’ skills as demonstrated by a significant improvement in intubation success rates at the end of the year when compared to the beginning.
This study does little to advance the science of learning among anesthesiology residents. While this hypothetical report demonstrates an improvement in residents’ intubation success before versus after the intervention, it does not tell why the workshop worked, how it compares to other educational interventions, or how it fits in to the broader picture of anesthesia training.
Single-group pre–post study designs open themselves to a myriad of threats to internal validity. 20 In our hypothetical example, the improvement in residents’ intubation skills may have been due to other educational experience(s) ( i.e. , implementation threat) and/or improvement in manual dexterity that occurred naturally with time ( i.e. , maturation threat), rather than the airway curriculum. Consequently, single-group pre–post studies should be interpreted with caution. 18
Repeated testing, before and after the intervention, is one strategy that can be used to reduce the some of the inherent limitations of the single-group study design. Repeated pretesting can mitigate the effect of regression toward the mean, a statistical phenomenon whereby low pretest scores tend to move closer to the mean on subsequent testing (regardless of intervention). 20 Likewise, repeated posttesting at multiple time intervals can provide potentially useful information about the short- and long-term effects of an intervention ( e.g. , the “durability” of the gain in knowledge, skill, or attitude).
Observational Research
Unlike experimental studies, observational research does not involve manipulation of any variables. These studies often involve measuring associations, developing psychometric instruments, or conducting surveys.
Association Research
Association research seeks to identify relationships between two or more variables within a group or groups (correlational research), or similarities/differences between two or more existing groups (causal–comparative research). For example, correlational research might seek to measure the relationship between burnout and educational debt among anesthesiology residents, while causal–comparative research may seek to measure differences in educational debt and/or burnout between anesthesiology and surgery residents. Notably, association research may identify relationships between variables, but does not necessarily support a causal relationship between them.
Psychometric and Survey Research
Psychometric instruments measure a psychologic or cognitive construct such as knowledge, satisfaction, beliefs, and symptoms. Surveys are one type of psychometric instrument, but many other types exist, such as evaluations of direct observation, written examinations, or screening tools. 22 Psychometric instruments are ubiquitous in medical education research and can be used to describe a trait within a study population ( e.g. , rates of depression among medical students) or to measure associations between study variables ( e.g. , association between depression and board scores among medical students).
Psychometric and survey research studies are prone to the internal validity threats listed in table 3 , particularly those relating to mortality, location, and instrumentation. 18 Additionally, readers must ensure that the instrument scores can be trusted to truly represent the construct being measured. For example, suppose you encounter a research article demonstrating a positive association between attending physician teaching effectiveness as measured by a survey of medical students, and the frequency with which the attending physician provides coffee and doughnuts on rounds. Can we be confident that this survey administered to medical students is truly measuring teaching effectiveness? Or is it simply measuring the attending physician’s “likability”? Issues related to measurement and the trustworthiness of data are described in detail in the following section on measurement and the related issues of validity and reliability.
Measurement refers to “the assigning of numbers to individuals in a systematic way as a means of representing properties of the individuals.” 23 Research data can only be trusted insofar as we trust the measurement used to obtain the data. Measurement is of particular importance in medical education research because many of the constructs being measured ( e.g. , knowledge, skill, attitudes) are abstract and subject to measurement error. 24 This section highlights two specific issues related to the trustworthiness of data: the validity and reliability of measurements.
Validity regarding the scores of a measurement instrument “refers to the degree to which evidence and theory support the interpretations of the [instrument’s results] for the proposed use of the [instrument].” 25 In essence, do we believe the results obtained from a measurement really represent what we were trying to measure? Note that validity evidence for the scores of a measurement instrument is separate from the internal validity of a research study. Several frameworks for validity evidence exist. Table 4 2 , 22 , 26 represents the most commonly used framework, developed by Messick, 27 which identifies sources of validity evidence—to support the target construct—from five main categories: content, response process, internal structure, relations to other variables, and consequences.
Sources of Validity Evidence for Measurement Instruments

Reliability
Reliability refers to the consistency of scores for a measurement instrument. 22 , 25 , 28 For an instrument to be reliable, we would anticipate that two individuals rating the same object of measurement in a specific context would provide the same scores. 25 Further, if the scores for an instrument are reliable between raters of the same object of measurement, then we can extrapolate that any difference in scores between two objects represents a true difference across the sample, and is not due to random variation in measurement. 29 Reliability can be demonstrated through a variety of methods such as internal consistency ( e.g. , Cronbach’s alpha), temporal stability ( e.g. , test–retest reliability), interrater agreement ( e.g. , intraclass correlation coefficient), and generalizability theory (generalizability coefficient). 22 , 29
Example of a Validity and Reliability Argument
This section provides an illustration of validity and reliability in medical education. We use the signaling questions outlined in table 4 to make a validity and reliability argument for the Harvard Assessment of Anesthesia Resident Performance (HARP) instrument. 7 The HARP was developed by Blum et al. to measure the performance of anesthesia trainees that is required to provide safe anesthetic care to patients. According to the authors, the HARP is designed to be used “…as part of a multiscenario, simulation-based assessment” of resident performance. 7
Content Validity: Does the Instrument’s Content Represent the Construct Being Measured?
To demonstrate content validity, instrument developers should describe the construct being measured and how the instrument was developed, and justify their approach. 25 The HARP is intended to measure resident performance in the critical domains required to provide safe anesthetic care. As such, investigators note that the HARP items were created through a two-step process. First, the instrument’s developers interviewed anesthesiologists with experience in resident education to identify the key traits needed for successful completion of anesthesia residency training. Second, the authors used a modified Delphi process to synthesize the responses into five key behaviors: (1) formulate a clear anesthetic plan, (2) modify the plan under changing conditions, (3) communicate effectively, (4) identify performance improvement opportunities, and (5) recognize one’s limits. 7 , 30
Response Process Validity: Are Raters Interpreting the Instrument Items as Intended?
In the case of the HARP, the developers included a scoring rubric with behavioral anchors to ensure that faculty raters could clearly identify how resident performance in each domain should be scored. 7
Internal Structure Validity: Do Instrument Items Measuring Similar Constructs Yield Homogenous Results? Do Instrument Items Measuring Different Constructs Yield Heterogeneous Results?
Item-correlation for the HARP demonstrated a high degree of correlation between some items ( e.g. , formulating a plan and modifying the plan under changing conditions) and a lower degree of correlation between other items ( e.g. , formulating a plan and identifying performance improvement opportunities). 30 This finding is expected since the items within the HARP are designed to assess separate performance domains, and we would expect residents’ functioning to vary across domains.
Relationship to Other Variables’ Validity: Do Instrument Scores Correlate with Other Measures of Similar or Different Constructs as Expected?
As it applies to the HARP, one would expect that the performance of anesthesia residents will improve over the course of training. Indeed, HARP scores were found to be generally higher among third-year residents compared to first-year residents. 30
Consequence Validity: Are Instrument Results Being Used as Intended? Are There Unintended or Negative Uses of the Instrument Results?
While investigators did not intentionally seek out consequence validity evidence for the HARP, unanticipated consequences of HARP scores were identified by the authors as follows:
“Data indicated that CA-3s had a lower percentage of worrisome scores (rating 2 or lower) than CA-1s… However, it is concerning that any CA-3s had any worrisome scores…low performance of some CA-3 residents, albeit in the simulated environment, suggests opportunities for training improvement.” 30
That is, using the HARP to measure the performance of CA-3 anesthesia residents had the unintended consequence of identifying the need for improvement in resident training.
Reliability: Are the Instrument’s Scores Reproducible and Consistent between Raters?
The HARP was applied by two raters for every resident in the study across seven different simulation scenarios. The investigators conducted a generalizability study of HARP scores to estimate the variance in assessment scores that was due to the resident, the rater, and the scenario. They found little variance was due to the rater ( i.e. , scores were consistent between raters), indicating a high level of reliability. 7
Sampling refers to the selection of research subjects ( i.e. , the sample) from a larger group of eligible individuals ( i.e. , the population). 31 Effective sampling leads to the inclusion of research subjects who represent the larger population of interest. Alternatively, ineffective sampling may lead to the selection of research subjects who are significantly different from the target population. Imagine that researchers want to explore the relationship between burnout and educational debt among pain medicine specialists. The researchers distribute a survey to 1,000 pain medicine specialists (the population), but only 300 individuals complete the survey (the sample). This result is problematic because the characteristics of those individuals who completed the survey and the entire population of pain medicine specialists may be fundamentally different. It is possible that the 300 study subjects may be experiencing more burnout and/or debt, and thus, were more motivated to complete the survey. Alternatively, the 700 nonresponders might have been too busy to respond and even more burned out than the 300 responders, which would suggest that the study findings were even more amplified than actually observed.
When evaluating a medical education research article, it is important to identify the sampling technique the researchers employed, how it might have influenced the results, and whether the results apply to the target population. 24
Sampling Techniques
Sampling techniques generally fall into two categories: probability- or nonprobability-based. Probability-based sampling ensures that each individual within the target population has an equal opportunity of being selected as a research subject. Most commonly, this is done through random sampling, which should lead to a sample of research subjects that is similar to the target population. If significant differences between sample and population exist, those differences should be due to random chance, rather than systematic bias. The difference between data from a random sample and that from the population is referred to as sampling error. 24
Nonprobability-based sampling involves selecting research participants such that inclusion of some individuals may be more likely than the inclusion of others. 31 Convenience sampling is one such example and involves selection of research subjects based upon ease or opportuneness. Convenience sampling is common in medical education research, but, as outlined in the example at the beginning of this section, it can lead to sampling bias. 24 When evaluating an article that uses nonprobability-based sampling, it is important to look for participation/response rate. In general, a participation rate of less than 75% should be viewed with skepticism. 21 Additionally, it is important to determine whether characteristics of participants and nonparticipants were reported and if significant differences between the two groups exist.
Interpreting medical education research requires a basic understanding of common ways in which quantitative data are analyzed and displayed. In this section, we highlight two broad topics that are of particular importance when evaluating research articles.
The Nature of the Measurement Variable
Measurement variables in quantitative research generally fall into three categories: nominal, ordinal, or interval. 24 Nominal variables (sometimes called categorical variables) involve data that can be placed into discrete categories without a specific order or structure. Examples include sex (male or female) and professional degree (M.D., D.O., M.B.B.S., etc .) where there is no clear hierarchical order to the categories. Ordinal variables can be ranked according to some criterion, but the spacing between categories may not be equal. Examples of ordinal variables may include measurements of satisfaction (satisfied vs . unsatisfied), agreement (disagree vs . agree), and educational experience (medical student, resident, fellow). As it applies to educational experience, it is noteworthy that even though education can be quantified in years, the spacing between years ( i.e. , educational “growth”) remains unequal. For instance, the difference in performance between second- and third-year medical students is dramatically different than third- and fourth-year medical students. Interval variables can also be ranked according to some criteria, but, unlike ordinal variables, the spacing between variable categories is equal. Examples of interval variables include test scores and salary. However, the conceptual boundaries between these measurement variables are not always clear, as in the case where ordinal scales can be assumed to have the properties of an interval scale, so long as the data’s distribution is not substantially skewed. 32
Understanding the nature of the measurement variable is important when evaluating how the data are analyzed and reported. Medical education research commonly uses measurement instruments with items that are rated on Likert-type scales, whereby the respondent is asked to assess their level of agreement with a given statement. The response is often translated into a corresponding number ( e.g. , 1 = strongly disagree, 3 = neutral, 5 = strongly agree). It is remarkable that scores from Likert-type scales are sometimes not normally distributed ( i.e. , are skewed toward one end of the scale), indicating that the spacing between scores is unequal and the variable is ordinal in nature. In these cases, it is recommended to report results as frequencies or medians, rather than means and SDs. 33
Consider an article evaluating medical students’ satisfaction with a new curriculum. Researchers measure satisfaction using a Likert-type scale (1 = very unsatisfied, 2 = unsatisfied, 3 = neutral, 4 = satisfied, 5 = very satisfied). A total of 20 medical students evaluate the curriculum, 10 of whom rate their satisfaction as “satisfied,” and 10 of whom rate it as “very satisfied.” In this case, it does not make much sense to report an average score of 4.5; it makes more sense to report results in terms of frequency ( e.g. , half of the students were “very satisfied” with the curriculum, and half were not).
Effect Size and CIs
In medical education, as in other research disciplines, it is common to report statistically significant results ( i.e. , small P values) in order to increase the likelihood of publication. 34 , 35 However, a significant P value in itself does necessarily represent the educational impact of the study results. A statement like “Intervention x was associated with a significant improvement in learners’ intubation skill compared to education intervention y ( P < 0.05)” tells us that there was a less than 5% chance that the difference in improvement between interventions x and y was due to chance. Yet that does not mean that the study intervention necessarily caused the nonchance results, or indicate whether the between-group difference is educationally significant. Therefore, readers should consider looking beyond the P value to effect size and/or CI when interpreting the study results. 36 , 37
Effect size is “the magnitude of the difference between two groups,” which helps to quantify the educational significance of the research results. 37 Common measures of effect size include Cohen’s d (standardized difference between two means), risk ratio (compares binary outcomes between two groups), and Pearson’s r correlation (linear relationship between two continuous variables). 37 CIs represent “a range of values around a sample mean or proportion” and are a measure of precision. 31 While effect size and CI give more useful information than simple statistical significance, they are commonly omitted from medical education research articles. 35 In such instances, readers should be wary of overinterpreting a P value in isolation. For further information effect size and CI, we direct readers the work of Sullivan and Feinn 37 and Hulley et al. 31
In this final section, we identify instruments that can be used to evaluate the quality of quantitative medical education research articles. To this point, we have focused on framing the study and research methodologies and identifying potential pitfalls to consider when appraising a specific article. This is important because how a study is framed and the choice of methodology require some subjective interpretation. Fortunately, there are several instruments available for evaluating medical education research methods and providing a structured approach to the evaluation process.
The Medical Education Research Study Quality Instrument (MERSQI) 21 and the Newcastle Ottawa Scale-Education (NOS-E) 38 are two commonly used instruments, both of which have an extensive body of validity evidence to support the interpretation of their scores. Table 5 21 , 39 provides more detail regarding the MERSQI, which includes evaluation of study design, sampling, data type, validity, data analysis, and outcomes. We have found that applying the MERSQI to manuscripts, articles, and protocols has intrinsic educational value, because this practice of application familiarizes MERSQI users with fundamental principles of medical education research. One aspect of the MERSQI that deserves special mention is the section on evaluating outcomes based on Kirkpatrick’s widely recognized hierarchy of reaction, learning, behavior, and results ( table 5 ; fig .). 40 Validity evidence for the scores of the MERSQI include its operational definitions to improve response process, excellent reliability, and internal consistency, as well as high correlation with other measures of study quality, likelihood of publication, citation rate, and an association between MERSQI score and the likelihood of study funding. 21 , 41 Additionally, consequence validity for the MERSQI scores has been demonstrated by its utility for identifying and disseminating high-quality research in medical education. 42

Kirkpatrick’s hierarchy of outcomes as applied to education research. Reaction = Level 1, Learning = Level 2, Behavior = Level 3, Results = Level 4. Outcomes become more meaningful, yet more difficult to achieve, when progressing from Level 1 through Level 4. Adapted with permission from Beckman and Cook, 2007. 2
The Medical Education Research Study Quality Instrument for Evaluating the Quality of Medical Education Research

The NOS-E is a newer tool to evaluate the quality of medication education research. It was developed as a modification of the Newcastle-Ottawa Scale 43 for appraising the quality of nonrandomized studies. The NOS-E includes items focusing on the representativeness of the experimental group, selection and compatibility of the control group, missing data/study retention, and blinding of outcome assessors. 38 , 39 Additional validity evidence for NOS-E scores includes operational definitions to improve response process, excellent reliability and internal consistency, and its correlation with other measures of study quality. 39 Notably, the complete NOS-E, along with its scoring rubric, can found in the article by Cook and Reed. 39
A recent comparison of the MERSQI and NOS-E found acceptable interrater reliability and good correlation between the two instruments 39 However, noted differences exist between the MERSQI and NOS-E. Specifically, the MERSQI may be applied to a broad range of study designs, including experimental and cross-sectional research. Additionally, the MERSQI addresses issues related to measurement validity and data analysis, and places emphasis on educational outcomes. On the other hand, the NOS-E focuses specifically on experimental study designs, and on issues related to sampling techniques and outcome assessment. 39 Ultimately, the MERSQI and NOS-E are complementary tools that may be used together when evaluating the quality of medical education research.
Conclusions
This article provides an overview of quantitative research in medical education, underscores the main components of education research, and provides a general framework for evaluating research quality. We highlighted the importance of framing a study with respect to purpose, conceptual framework, and statement of study intent. We reviewed the most common research methodologies, along with threats to the validity of a study and its measurement instruments. Finally, we identified two complementary instruments, the MERSQI and NOS-E, for evaluating the quality of a medical education research study.
Bordage G: Conceptual frameworks to illuminate and magnify. Medical education. 2009; 43(4):312–9.
Cook DA, Beckman TJ: Current concepts in validity and reliability for psychometric instruments: Theory and application. The American journal of medicine. 2006; 119(2):166. e7–166. e116.
Franenkel JR, Wallen NE, Hyun HH: How to Design and Evaluate Research in Education. 9th edition. New York, McGraw-Hill Education, 2015.
Hulley SB, Cummings SR, Browner WS, Grady DG, Newman TB: Designing clinical research. 4th edition. Philadelphia, Lippincott Williams & Wilkins, 2011.
Irby BJ, Brown G, Lara-Alecio R, Jackson S: The Handbook of Educational Theories. Charlotte, NC, Information Age Publishing, Inc., 2015
Standards for Educational and Psychological Testing (American Educational Research Association & American Psychological Association, 2014)
Swanwick T: Understanding medical education: Evidence, theory and practice, 2nd edition. Wiley-Blackwell, 2013.
Sullivan GM, Artino Jr AR: Analyzing and interpreting data from Likert-type scales. Journal of graduate medical education. 2013; 5(4):541–2.
Sullivan GM, Feinn R: Using effect size—or why the P value is not enough. Journal of graduate medical education. 2012; 4(3):279–82.
Tavakol M, Sandars J: Quantitative and qualitative methods in medical education research: AMEE Guide No 90: Part II. Medical teacher. 2014; 36(10):838–48.
Support was provided solely from institutional and/or departmental sources.
The authors declare no competing interests.
Citing articles via
Most viewed, email alerts, related articles, social media, affiliations.
- ASA Practice Parameters
- Online First
- Author Resource Center
- About the Journal
- Editorial Board
- Rights & Permissions
- Online ISSN 1528-1175
- Print ISSN 0003-3022
- Anesthesiology
- ASA Monitor

- Terms & Conditions Privacy Policy
- Manage Cookie Preferences
- © Copyright 2024 American Society of Anesthesiologists
This Feature Is Available To Subscribers Only
Sign In or Create an Account
- Research article
- Open access
- Published: 06 January 2021
Effects of the COVID-19 pandemic on medical students: a multicenter quantitative study
- Aaron J. Harries ORCID: orcid.org/0000-0001-7107-0995 1 ,
- Carmen Lee 1 ,
- Lee Jones 2 ,
- Robert M. Rodriguez 1 ,
- John A. Davis 2 ,
- Megan Boysen-Osborn 3 ,
- Kathleen J. Kashima 4 ,
- N. Kevin Krane 5 ,
- Guenevere Rae 6 ,
- Nicholas Kman 7 ,
- Jodi M. Langsfeld 8 &
- Marianne Juarez 1
BMC Medical Education volume 21 , Article number: 14 ( 2021 ) Cite this article
131k Accesses
148 Citations
37 Altmetric
Metrics details
The COVID-19 pandemic disrupted the United States (US) medical education system with the necessary, yet unprecedented Association of American Medical Colleges (AAMC) national recommendation to pause all student clinical rotations with in-person patient care. This study is a quantitative analysis investigating the educational and psychological effects of the pandemic on US medical students and their reactions to the AAMC recommendation in order to inform medical education policy.
The authors sent a cross-sectional survey via email to medical students in their clinical training years at six medical schools during the initial peak phase of the COVID-19 pandemic. Survey questions aimed to evaluate students’ perceptions of COVID-19’s impact on medical education; ethical obligations during a pandemic; infection risk; anxiety and burnout; willingness and needed preparations to return to clinical rotations.
Seven hundred forty-one (29.5%) students responded. Nearly all students (93.7%) were not involved in clinical rotations with in-person patient contact at the time the study was conducted. Reactions to being removed were mixed, with 75.8% feeling this was appropriate, 34.7% guilty, 33.5% disappointed, and 27.0% relieved.
Most students (74.7%) agreed the pandemic had significantly disrupted their medical education, and believed they should continue with normal clinical rotations during this pandemic (61.3%). When asked if they would accept the risk of infection with COVID-19 if they returned to the clinical setting, 83.4% agreed.
Students reported the pandemic had moderate effects on their stress and anxiety levels with 84.1% of respondents feeling at least somewhat anxious. Adequate personal protective equipment (PPE) (53.5%) was the most important factor to feel safe returning to clinical rotations, followed by adequate testing for infection (19.3%) and antibody testing (16.2%).
Conclusions
The COVID-19 pandemic disrupted the education of US medical students in their clinical training years. The majority of students wanted to return to clinical rotations and were willing to accept the risk of COVID-19 infection. Students were most concerned with having enough PPE if allowed to return to clinical activities.
Peer Review reports
The COVID-19 pandemic has tested the limits of healthcare systems and challenged conventional practices in medical education. The rapid evolution of the pandemic dictated that critical decisions regarding the training of medical students in the United States (US) be made expeditiously, without significant input or guidance from the students themselves. On March 17, 2020, for the first time in modern US history, the Association of American Medical Colleges (AAMC), the largest national governing body of US medical schools, released guidance recommending that medical students immediately pause all clinical rotations to allow time to obtain additional information about the risks of COVID-19 and prepare for safe participation in the future. This decisive action would also conserve scarce resources such as personal protective equipment (PPE) and testing kits; minimize exposure of healthcare workers (HCWs) and the general population; and protect students’ education and wellbeing [ 1 ].
A similar precedent was set outside of the US during the SARS-CoV1 epidemic in 2003, where an initial cluster of infection in medical students in Hong Kong resulted in students being removed from hospital systems where SARS surfaced, including Hong Kong, Singapore and Toronto [ 2 , 3 ]. Later, studies demonstrated that the exclusion of Canadian students from those clinical environments resulted in frustration at lost learning opportunities and students’ inability to help [ 3 ]. International evidence also suggests that medical students perceive an ethical obligation to participate in pandemic response, and are willing to participate in scenarios similar to the current COVID-19 crisis, even when they believe the risk of infection to themselves to be high [ 4 , 5 , 6 ].
The sudden removal of some US medical students from educational settings has occurred previously in the wake of local disasters, with significant academic and personal impacts. In 2005, it was estimated that one-third of medical students experienced some degree of depression or post-traumatic stress disorder (PTSD) after Hurricane Katrina resulted in the closure of Tulane University School of Medicine [ 7 ].
Prior to the current COVID-19 pandemic, we found no studies investigating the effects of pandemics on the US medical education system or its students. The limited pool of evidence on medical student perceptions comes from two earlier global coronavirus surges, SARS and MERS, and studies of student anxiety related to pandemics are also limited to non-US populations [ 3 , 8 , 9 ]. Given the unprecedented nature of the current COVID-19 pandemic, there is concern that students may be missing out on meaningful educational experiences and months of clinical training with unknown effects on their current well-being or professional trajectory [ 10 ].
Our study, conducted during the initial peak phase of the COVID-19 pandemic, reports students’ perceptions of COVID-19’s impact on: medical student education; ethical obligations during a pandemic; perceptions of infection risk; anxiety and burnout; willingness to return to clinical rotations; and needed preparations to return safely. This data may help inform policies regarding the roles of medical students in clinical training during the current pandemic and prepare for the possibility of future pandemics.
We conducted a cross-sectional survey during the initial peak phase of the COVID-19 pandemic in the United States, from 4/20/20 to 5/25/20, via email sent to all clinically rotating medical students at six US medical schools: University of California San Francisco School of Medicine (San Francisco, CA), University of California Irvine School of Medicine (Irvine, CA), Tulane University School of Medicine (New Orleans, LA), University of Illinois College of Medicine (Chicago, Peoria, Rockford, and Urbana, IL), Ohio State University College of Medicine (Columbus, OH), and Zucker School of Medicine at Hofstra/Northwell (Hempstead, NY). Traditional undergraduate medical education in the US comprises 4 years of medical school with 2 years of primarily pre-clinical classroom learning followed by 2 years of clinical training involving direct patient care. Study participants were defined as medical students involved in their clinical training years at whom the AAMC guidance statement was directed. Depending on the curricular schedule of each medical school, this included intended graduation class years of 2020 (graduating 4th year student), 2021 (rising 4th year student), and 2022 (rising 3rd year student), exclusive of planned time off. Participating schools were specifically chosen to represent a broad spectrum of students from different regions of the country (West, South, Midwest, East) with variable COVID-19 prevalence. We excluded medical students not yet involved in clinical rotations. This study was deemed exempt by the respective Institutional Review Boards.
We developed a survey instrument modeled after a survey used in a previously published peer reviewed study evaluating the effects of the COVID-19 pandemic on Emergency Physicians, which incorporated items from validated stress scales [ 11 ]. The survey was modified for use in medical students to assess perceptions of the following domains: perceived impact on medical student education; ethical beliefs surrounding obligations to participate clinically during the pandemic; perceptions of personal infection risk; anxiety and burnout related to the pandemic; willingness to return to clinical rotations; and preparation needed for students to feel safe in the clinical environment. Once created, the survey underwent an iterative process of input and review from our team of authors with experience in survey methodology and psychometric measures to allow for optimization of content and validity. We tested a pilot of our preliminary instrument on five medical students to ensure question clarity, and confirm completion of the survey in approximately 10 min. The final survey consisted of 29 Likert, yes/no, multiple choice, and free response questions. Both medical school deans and student class representatives distributed the survey via email, with three follow-up emails to increase response rates. Data was collected anonymously.
For example, to assess the impact on students’ anxiety, participants were asked, “How much has the COVID-19 pandemic affected your stress or anxiety levels?” using a unipolar 7-point scale (1 = not at all, 4 = somewhat, 7 = extremely). To assess willingness to return to clinical rotations, participants were asked to rate on a bipolar scale (1 = strongly disagree, 2 = disagree, 3 = somewhat disagree, 4 = neither disagree nor agree, 5 = somewhat agree, 6 = agree, and 7 = strongly agree) their agreement with the statement: “to the extent possible, medical students should continue with normal clinical rotations during this pandemic.” (Survey Instrument, Supplemental Table 1 ).
Survey data was managed using Qualtrics hosted by the University of California, San Francisco. For data analysis we used STATA v15.1 (Stata Corp, College Station, TX). We summarized respondent characteristics and key responses as raw counts, frequency percent, medians and interquartile ranges (IQR). For responses to bipolar questions, we combined positive responses (somewhat agree, agree, or strongly agree) into an agreement percentage. To compare differences in medians we used a signed rank test with p value < 0.05 to show statistical difference. In a secondary analysis we stratified data to compare questions within key domains amongst the following sub-groups: female versus male, graduation year, local community COVID-19 prevalence (high, medium, low), and students on clinical rotations with in-person patient care. This secondary analysis used a chi square test with p value < 0.05 to show statistical difference between sub-group agreement percentages.
Of 2511 students contacted, we received 741 responses (29.5% response rate). Of these, 63.9% of respondents were female and 35.1% were male, with 1.0% reporting a different gender identity; 27.7% of responses came from the class of 2020, 53.5% from the class of 2021, and 18.7% from the class of 2022. (Demographics, Table 1 ).
Most student respondents (74.9%) had a clinical rotation that was cut short or canceled due to COVID-19 and 93.7% reported not being involved in clinical rotations with in-person patient contact at the time of the study. Regarding students’ perceptions of cancelled rotations (allowing for multiple reactions), 75.8% felt this was appropriate, 34.7% felt guilty for not being able to help patients and colleagues, 33.5% felt disappointed, and 27.0% felt relieved.
Most students (74.7%) agreed that their medical education had been significantly disrupted by the pandemic. Students also felt they were able to find meaningful learning experiences during the pandemic (72.1%). Free response examples included: taking a novel COVID-19 pandemic elective course, telehealth patient care, clinical rotations transitioned to virtual online courses, research or education electives, clinical and non-clinical COVID-19-related volunteering, and self-guided independent study electives. Students felt their medical schools were doing everything they could to help students adjust (72.7%). Overall, respondents felt the pandemic had interfered with their ability to develop skills needed to prepare for residency (61.4%), though fewer (45.7%) felt it had interfered with their ability to apply to residency. (Educational Impact, Fig. 1 ).
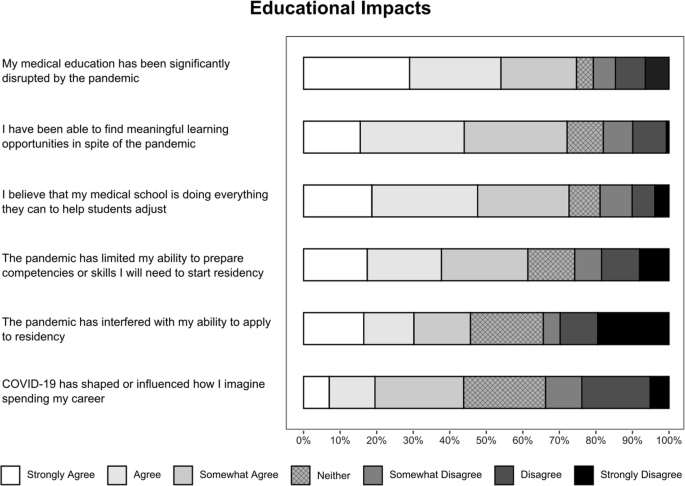
Perceived educational impacts of the COVID-19 pandemic on medical students
A majority of medical students agreed they should be allowed to continue with normal clinical rotations during this pandemic (61.3%). Most students agreed (83.4%) that they accepted the risk of being infected with COVID-19, if they returned. When asked if students should be allowed to volunteer in clinical settings even if there is not a healthcare worker (HCW) shortage, 63.5% agreed; however, in the case of a HCW shortage only 19.5% believed students should be required to volunteer clinically. (Willingness to Participate Clinically, Fig. 2 ).
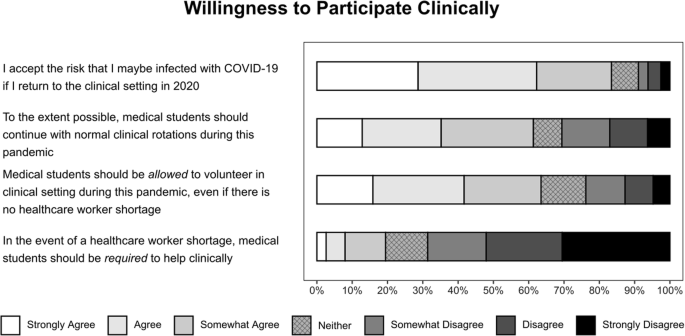
Willingness to participate clinically during the COVID-19 pandemic
When asked if they perceived a moral, ethical, or professional obligation for medical students to help, 37.8% agreed that medical students have such an obligation during the current pandemic. This is in contrast to their perceptions of physicians: 87.1% of students agreed with a physician obligation to help during the COVID-19 pandemic. For both groups, students were asked if this obligation persisted without adequate PPE: only 10.9% of students believed medical students had this obligation, while 34.0% agreed physicians had this obligation. (Ethical Obligation, Fig. 3 ).
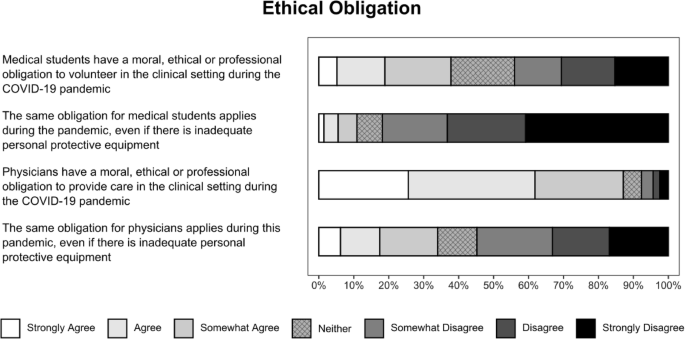
Ethical obligation to volunteer during the COVID-19 pandemic
Given the assumption that there will not be a COVID-19 vaccine until 2021, students felt the single most important factor in a safe return to clinical rotations was having access to adequate PPE (53.3%), followed by adequate testing for infection (19.3%) and antibody testing for possible immunity (16.2%). Few students (5%) stated that nothing would make them feel comfortable until a vaccine is available. On a 1–7 scale (1 = not at all, 4 = somewhat, 7 = extremely), students felt somewhat prepared to use PPE during this pandemic in the clinical setting, median = 4 (IQR 4,6), and somewhat confident identifying symptoms most concerning for COVID-19, median = 4 (IQR 4,5). Students preferred to learn about PPE via video demonstration (76.7%), online modules (47.7%), and in-person or Zoom style conferences (44.7%).
Students believed they were likely to contract COVID-19 in general (75.6%), independent of a return to the clinical environment. Most respondents believed that missing some school or work would be a likely outcome (90.5%), and only a minority of students believed that hospitalization (22.1%) or death (4.3%) was slightly, moderately, or extremely likely.
On a 1–7 scale (1 = not at all, 4 = somewhat, and 7 = extremely), the median (IQR) reported effect of the COVID-19 pandemic on students’ stress or anxiety level was 5 (4, 6) with 84.1% of respondents feeling at least somewhat anxious due to the pandemic. Students’ perceived emotional exhaustion and burnout before the pandemic was a median = 2 (IQR 2,4) and since the pandemic started a median = 4 (IQR 2,5) with a median difference Δ = 2, p value < 0.001.
Secondary analysis of key questions revealed statistical differences between sub-groups. Women were significantly more likely than men to agree that the pandemic had affected their anxiety. Several significant differences existed for the class of 2020 when compared to the classes of 2021 and 2022: they were less likely to report disruptions to their education, to prefer to return to rotations, and to report an effect on anxiety. There were no significant differences with students who were still involved with in-person patient care compared with those who were not. In comparing areas with high COVID-19 prevalence at the time of the survey (New York and Louisiana) with medium (Illinois and Ohio) and low prevalence (California), students were less likely to report that the pandemic had disrupted their education. Students in low prevalence areas were most likely to agree that medical students should return to rotations. There were no differences between prevalence groups in accepting the risk of infection to return, or subjective anxiety effects. (Stratification, Table 2 ).
The COVID-19 pandemic has fundamentally transformed education at all levels - from preschool to postgraduate. Although changes to K-12 and college education have been well documented [ 12 , 13 ], there have been very few studies to date investigating the effects of COVID-19 on undergraduate medical education [ 14 ]. To maintain the delicate balance between student safety and wellbeing, and the time-sensitive need to train future physicians, student input must guide decisions regarding their roles in the clinical arena. Student concerns related to the pandemic, paired with their desire to return to rotations despite the risks, suggest that medical students may take on emotional burdens as members of the patient care team even when not present in the clinical environment. This study offers insight into how best to support medical students as they return to clinical rotations, how to prepare them for successful careers ahead, and how to plan for their potential roles in future pandemics.
Previous international studies of medical student attitudes towards hypothetical influenza-like pandemics demonstrated a willingness (80%) [ 4 ] and a perceived ethical obligation to volunteer (77 and 70%), despite 40% of Canadian students in one study perceiving a high likelihood of becoming infected [ 5 , 6 ]. Amidst the current COVID-19 pandemic, our participants reported less agreement with a medical student ethical obligation to volunteer in the clinical setting at 37.8%, but believed in a higher likelihood of becoming infected at 75.6%. Their willingness to be allowed to volunteer freely (63.5%) may suggest that the stresses of an ongoing pandemic alter students’ perceptions of the ethical requirement more than their willingness to help. Students overwhelmingly agreed that physicians had an ethical obligation to provide care during the COVID-19 pandemic (87.1%), possibly reflecting how they view the ethical transition from student to physician, or differences between paid professionals and paying for an education.
At the time our study was conducted, there were widespread concerns for possible HCW shortages. It was unclear whether medical students would be called to volunteer when residents became ill, or even graduate early to start residency training immediately (as occurred at half of schools surveyed). This timing allowed us to capture a truly unique perspective amongst medical students, a majority of whom reported increased anxiety and burnout due to the pandemic. At the same time, students felt that their medical schools were doing everything possible to support them, perhaps driven by virtual town halls and daily communication updates.
Trends in secondary analysis show important differences in the impacts of the pandemic. Women were more likely to report increased anxiety as compared to men, which may reflect broader gender differences in medical student anxiety [ 15 ] but requires more study to rule out different pandemic stresses by gender. Graduating medical students (class of 2020) overall described less impact on medical education and anxiety, a decreased desire to return to rotations, but equal acceptance of the risk of infection in clinical settings, possibly reflecting a focus on their upcoming intern year rather than the remaining months of undergraduate medical education. Since this class’s responses decreased overall agreement on these questions, educational impacts and anxiety effects may have been even greater had they been assessed further from graduation. Interestingly, students from areas with high local COVID-19 prevalence (New York and Louisiana) reported a less significant effect of the pandemic on their education, a paradoxical result that may indicate that medical student tolerance for the disruptions was greater in high-prevalence areas, as these students were removed at the same, if not higher, rates as their peers. Our results suggest that in future waves of the current pandemic or other disasters, students may be more patient with educational impacts when they have more immediate awareness of strains on the healthcare system.
A limitation of our study was the survey response rate, which was anticipated given the challenges students were facing. Some may not have been living near campus; others may have stopped reading emails due to early graduation or limited access to email; and some would likely be dealing with additional personal challenges related to the pandemic. We attempted to increase response rates by having the study sent directly from medical school deans and leadership, as well as respective class representatives, and by sending reminders for completion. The survey was not incentivized, and a higher response rate in the class of 2021 across all schools may indicate that students who felt their education was most affected were most likely to respond. We addressed this potential source of bias in the secondary analysis, which showed no differences between 2021 and 2022 respondents. Another limitation was the inherent issue with survey data collection of missing responses for some questions that occurred in a small number of surveys. This resulted in slight variability in the total responses received for certain questions, which were not statistically significant. To be transparent about this limitation, we presented our data by stating each total response and denominator in the Tables.
This initial study lays the groundwork for future investigations and next steps. With 72.1% of students agreeing that they were able to find meaningful learning in spite of the pandemic, future research should investigate novel learning modalities that were successful during this time. Educators should consider additional training on PPE use, given only moderate levels of student comfort in this area, which may be best received via video. It is also important to study the long-term effects of missing several months of essential clinical training and identifying competencies that may not have been achieved, since students perceived a significant disruption to their ability to prepare skills for residency. Next steps could be to study curriculum interventions, such as capstone boot camps and targeted didactic skills training, to help students feel more comfortable as they transition into residency. Educators must also acknowledge that some students may not feel comfortable returning to the clinical environment until a vaccine becomes available (5%) and ensure they are equally supported. Lastly, it is vital to further investigate the mental health effects of the pandemic on medical students, identifying subgroups with additional stressors, needs related to anxiety or possible PTSD, and ways to minimize these negative effects.
In this cross-sectional survey, conducted during the initial peak phase of the COVID-19 pandemic, we capture a snapshot of the effects of the pandemic on US medical students and gain insight into their reactions to the unprecedented AAMC national recommendation for removal from clinical rotations. Student respondents from across the US similarly recognized a significant disruption to their medical education, shared a desire to continue with in-person rotations, and were willing to accept the risk of infection with COVID-19. Our novel results provide a solid foundation to help shape medical student roles in the clinical environment during this pandemic and future outbreaks.
Availability of data and materials
The datasets used and/or analyzed during the current study are available from the corresponding author on reasonable request.
Association of American Medical Colleges. Interim Guidance on Medical Students’ Participation in Direct Patient Contact Activities: Principles and Guidelines. https://www.aamc.org/news-insights/press-releases/important-guidance-medical-students-clinical-rotations-during-coronavirus-covid-19-outbreak . Published March 17, 2020. Accessed April 1, 2020.
Clark J. Fear of SARS thwarts medical education in Toronto. BMJ. 2003;326(7393):784. https://doi.org/10.1136/bmj.326.7393.784/c .
Article Google Scholar
Loh LC, Ali AM, Ang TH, Chelliah A. Impact of a spreading epidemic on medical students. Malays J Med Sci. 2006;13(2):30–6.
Google Scholar
Mortelmans LJ, Bouman SJ, Gaakeer MI, Dieltiens G, Anseeuw K, Sabbe MB. Dutch senior medical students and disaster medicine: a national survey. Int J Emerg Med. 2015;8(1):77. https://doi.org/10.1186/s12245-015-0077-0 .
Huapaya JA, Maquera-Afaray J, García PJ, Cárcamo C, Cieza JA. Conocimientos, prácticas y actitudes hacia el voluntariado ante una influenza pandémica: estudio transversal con estudiantes de medicina en Perú [Knowledge, practices and attitudes toward volunteer work in an influenza pandemic: cross-sectional study with Peruvian medical students]. Medwave. 2015;15(4):e6136Published 2015 May 8. https://doi.org/10.5867/medwave.2015.04.6136 .
Herman B, Rosychuk RJ, Bailey T, Lake R, Yonge O, Marrie TJ. Medical students and pandemic influenza. Emerg Infect Dis. 2007;13(11):1781–3. https://doi.org/10.3201/eid1311.070279 .
Kahn MJ, Markert RJ, Johnson JE, Owens D, Krane NK. Psychiatric issues and answers following hurricane Katrina. Acad Psychiatry. 2007;31(3):200–4. https://doi.org/10.1176/appi.ap.31.3.200 .
Al-Rabiaah A, Temsah MH, Al-Eyadhy AA, et al. Middle East respiratory syndrome-Corona virus (MERS-CoV) associated stress among medical students at a university teaching hospital in Saudi Arabia. J Infect Public Health. 2020;13(5):687–91. https://doi.org/10.1016/j.jiph.2020.01.005 .
Wong JG, Cheung EP, Cheung V, et al. Psychological responses to the SARS outbreak in healthcare students in Hong Kong. Med Teach. 2004;26(7):657–9. https://doi.org/10.1080/01421590400006572 .
Stokes DC. Senior medical students in the COVID-19 response: an opportunity to be proactive. Acad Emerg Med. 2020;27(4):343–5. https://doi.org/10.1111/acem.13972 .
Rodriguez RM, Medak AJ, Baumann BM, et al. Academic emergency medicine physicians’ anxiety levels, stressors, and potential mitigation measures during the acceleration phase of the COVID-19 pandemic. Acad Emerg Med. 2020;27(8):700–7. https://doi.org/10.1111/acem.14065 .
Sahu P. Closure of universities due to coronavirus disease 2019 (COVID-19): impact on education and mental health of students and academic staff. Cureus. 2020;12(4):e7541Published 2020 Apr 4. https://doi.org/10.7759/cureus.7541 .
Reimers FM, Schleicher A. A framework to guide an education response to the COVID-19 pandemic of 2020: OECD. https://www.hm.ee/sites/default/files/framework_guide_v1_002_harward.pdf .
Choi B, Jegatheeswaran L, Minocha A, Alhilani M, Nakhoul M, Mutengesa E. The impact of the COVID-19 pandemic on final year medical students in the United Kingdom: a national survey. BMC Med Educ. 2020;20:206–16. https://doi.org/10.1186/s12909-020-02117-1 .
Dyrbye LN, Thomas MR, Shanafelt TD. Systematic review of depression, anxiety, and other indicators of psychological distress among U.S. and Canadian medical students. Acad Med. 2006;81(4):354–73. https://doi.org/10.1097/00001888-200604000-00009 .
Download references
Acknowledgments
The authors wish to thank Newton Addo, UCSF Statistician.
Author information
Authors and affiliations.
Department of Emergency Medicine, University of California San Francisco School of Medicine, San Francisco General Hospital, 1001 Potrero Avenue, Building 5, Room #6A4, San Francisco, California, 94110, USA
Aaron J. Harries, Carmen Lee, Robert M. Rodriguez & Marianne Juarez
University of California San Francisco School of Medicine, San Francisco, California, USA
Lee Jones & John A. Davis
Clinical Emergency Medicine, University of California Irvine School of Medicine, Irvine, CA, USA
Megan Boysen-Osborn
University of Illinois College of Medicine, Chicago, IL, USA
Kathleen J. Kashima
Deming Department of Medicine, Tulane University School of Medicine, New Orleans, Louisiana, USA
N. Kevin Krane
Basic Science Education, Tulane University School of Medicine, New Orleans, Louisiana, USA
Guenevere Rae
Emergency Medicine, Ohio State College of Medicine, Columbus, OH, USA
Nicholas Kman
Department of Science Education, Donald and Barbara Zucker School of Medicine at Hofstra/Northwell, Hempstead, New York, USA
Jodi M. Langsfeld
You can also search for this author in PubMed Google Scholar
Contributions
All authors made substantial contributions to the study and met the specific conditions listed in the BMC Medical Education editorial policy for authorship. All authors have read and approved the manuscript. AH as principal investigator contributed to study design, survey instrument creation, IRB submission for his respective medical school, acquisition of data and recruitment of other participating medical schools, data analysis, writing and editing the manuscript. CL contributed to background literature review, study design, survey instrument creation, acquisition of data, data analysis, writing and editing the manuscript. LJ contributed to study design, survey instrument creation, acquisition of data from his respective medical school and recruitment of other participating medical schools, data analysis, and editing the manuscript. RR contributed to study design, survey instrument creation, data analysis, writing and editing the manuscript. JD contributed to study design, survey instrument creation, recruitment of other participating medical schools, data analysis, and editing the manuscript. MBO contributed as individual site principal investigator obtaining IRB exemption acceptance and acquisition of data from her respective medical school along with editing the manuscript. KK contributed as individual site principal investigator obtaining IRB exemption acceptance and acquisition of data from her respective medical school along with editing the manuscript. NKK contributed as individual site co-principal investigator obtaining IRB exemption acceptance and acquisition of data from his respective medical school along with editing the manuscript. GR contributed as individual site co-principal investigator obtaining IRB exemption acceptance and acquisition of data from her respective medical school along with editing the manuscript. NK contributed as individual site principal investigator obtaining IRB exemption acceptance and acquisition of data from his respective medical school along with editing the manuscript. JL contributed as individual site principal investigator obtaining IRB exemption acceptance and acquisition of data from her respective medical school along with editing the manuscript. MJ contributed to study design, survey instrument creation, data analysis, writing and editing the manuscript.
Corresponding authors
Correspondence to Aaron J. Harries or Marianne Juarez .
Ethics declarations
Ethics approval and consent to participate.
This study was reviewed and deemed exempt by each participating medical school’s Institutional Review Board (IRB): University of California San Francisco School of Medicine, IRB# 20–30712, Reference# 280106, Tulane University School of Medicine, Reference # 2020–331, University of Illinois College of Medicine), IRB Protocol # 2012–0783, Ohio State University College of Medicine, Study ID# 2020E0463, Zucker School of Medicine at Hofstra/Northwell, Reference # 20200527-SOM-LAN-1, University of California Irvine School of Medicine, submitted self-exemption IRB form. In accordance with the IRB exemption approval, each survey participant received an email consent describing the study and their optional participation.
Consent for publication
This manuscript does not contain any individualized person’s data, therefore consent for publication was not necessary according to the IRB exemption approval.
Competing interests
The authors declare that they have no competing interests.
Additional information
Publisher’s note.
Springer Nature remains neutral with regard to jurisdictional claims in published maps and institutional affiliations.
Supplementary Information
Additional file 1: table s1..
Survey Instrument
Rights and permissions
Open Access This article is licensed under a Creative Commons Attribution 4.0 International License, which permits use, sharing, adaptation, distribution and reproduction in any medium or format, as long as you give appropriate credit to the original author(s) and the source, provide a link to the Creative Commons licence, and indicate if changes were made. The images or other third party material in this article are included in the article's Creative Commons licence, unless indicated otherwise in a credit line to the material. If material is not included in the article's Creative Commons licence and your intended use is not permitted by statutory regulation or exceeds the permitted use, you will need to obtain permission directly from the copyright holder. To view a copy of this licence, visit http://creativecommons.org/licenses/by/4.0/ . The Creative Commons Public Domain Dedication waiver ( http://creativecommons.org/publicdomain/zero/1.0/ ) applies to the data made available in this article, unless otherwise stated in a credit line to the data.
Reprints and permissions
About this article
Cite this article.
Harries, A.J., Lee, C., Jones, L. et al. Effects of the COVID-19 pandemic on medical students: a multicenter quantitative study. BMC Med Educ 21 , 14 (2021). https://doi.org/10.1186/s12909-020-02462-1
Download citation
Received : 29 July 2020
Accepted : 16 December 2020
Published : 06 January 2021
DOI : https://doi.org/10.1186/s12909-020-02462-1
Share this article
Anyone you share the following link with will be able to read this content:
Sorry, a shareable link is not currently available for this article.
Provided by the Springer Nature SharedIt content-sharing initiative
- Undergraduate medical education
- COVID-19 pandemic
- Medical student anxiety
BMC Medical Education
ISSN: 1472-6920
- Submission enquiries: [email protected]
- General enquiries: [email protected]
- Research article
- Open access
- Published: 03 February 2021
A review of the quantitative effectiveness evidence synthesis methods used in public health intervention guidelines
- Ellesha A. Smith ORCID: orcid.org/0000-0002-4241-7205 1 ,
- Nicola J. Cooper 1 ,
- Alex J. Sutton 1 ,
- Keith R. Abrams 1 &
- Stephanie J. Hubbard 1
BMC Public Health volume 21 , Article number: 278 ( 2021 ) Cite this article
3409 Accesses
5 Citations
3 Altmetric
Metrics details
The complexity of public health interventions create challenges in evaluating their effectiveness. There have been huge advancements in quantitative evidence synthesis methods development (including meta-analysis) for dealing with heterogeneity of intervention effects, inappropriate ‘lumping’ of interventions, adjusting for different populations and outcomes and the inclusion of various study types. Growing awareness of the importance of using all available evidence has led to the publication of guidance documents for implementing methods to improve decision making by answering policy relevant questions.
The first part of this paper reviews the methods used to synthesise quantitative effectiveness evidence in public health guidelines by the National Institute for Health and Care Excellence (NICE) that had been published or updated since the previous review in 2012 until the 19th August 2019.The second part of this paper provides an update of the statistical methods and explains how they address issues related to evaluating effectiveness evidence of public health interventions.
The proportion of NICE public health guidelines that used a meta-analysis as part of the synthesis of effectiveness evidence has increased since the previous review in 2012 from 23% (9 out of 39) to 31% (14 out of 45). The proportion of NICE guidelines that synthesised the evidence using only a narrative review decreased from 74% (29 out of 39) to 60% (27 out of 45).An application in the prevention of accidents in children at home illustrated how the choice of synthesis methods can enable more informed decision making by defining and estimating the effectiveness of more distinct interventions, including combinations of intervention components, and identifying subgroups in which interventions are most effective.
Conclusions
Despite methodology development and the publication of guidance documents to address issues in public health intervention evaluation since the original review, NICE public health guidelines are not making full use of meta-analysis and other tools that would provide decision makers with fuller information with which to develop policy. There is an evident need to facilitate the translation of the synthesis methods into a public health context and encourage the use of methods to improve decision making.
Peer Review reports
To make well-informed decisions and provide the best guidance in health care policy, it is essential to have a clear framework for synthesising good quality evidence on the effectiveness and cost-effectiveness of health interventions. There is a broad range of methods available for evidence synthesis. Narrative reviews provide a qualitative summary of the effectiveness of the interventions. Meta-analysis is a statistical method that pools evidence from multiple independent sources [ 1 ]. Meta-analysis and more complex variations of meta-analysis have been extensively applied in the appraisals of clinical interventions and treatments, such as drugs, as the interventions and populations are clearly defined and tested in randomised, controlled conditions. In comparison, public health studies are often more complex in design, making synthesis more challenging [ 2 ].
Many challenges are faced in the synthesis of public health interventions. There is often increased methodological heterogeneity due to the inclusion of different study designs. Interventions are often poorly described in the literature which may result in variation within the intervention groups. There can be a wide range of outcomes, whose definitions are not consistent across studies. Intermediate, or surrogate, outcomes are often used in studies evaluating public health interventions [ 3 ]. In addition to these challenges, public health interventions are often also complex meaning that they are made up of multiple, interacting components [ 4 ]. Recent guidance documents have focused on the synthesis of complex interventions [ 2 , 5 , 6 ]. The National Institute for Health and Care Excellence (NICE) guidance manual provides recommendations across all topics that are covered by NICE and there is currently no guidance that focuses specifically on the public health context.
Research questions
A methodological review of NICE public health intervention guidelines by Achana et al. (2014) found that meta-analysis methods were not being used [ 3 ]. The first part of this paper aims to update and compare, to the original review, the meta-analysis methods being used in evidence synthesis of public health intervention appraisals.
The second part of this paper aims to illustrate what methods are available to address the challenges of public health intervention evidence synthesis. Synthesis methods that go beyond a pairwise meta-analysis are illustrated through the application to a case study in public health and are discussed to understand how evidence synthesis methods can enable more informed decision making.
The third part of this paper presents software, guidance documents and web tools for methods that aim to make appropriate evidence synthesis of public health interventions more accessible. Recommendations for future research and guidance production that can improve the uptake of these methods in a public health context are discussed.
Update of NICE public health intervention guidelines review
Nice guidelines.
The National Institute for Health and Care Excellence (NICE) was established in 1999 as a health authority to provide guidance on new medical technologies to the NHS in England and Wales [ 7 ]. Using an evidence-based approach, it provides recommendations based on effectiveness and cost-effectiveness to ensure an open and transparent process of allocating NHS resources [ 8 ]. The remit for NICE guideline production was extended to public health in April 2005 and the first recommendations were published in March 2006. NICE published ‘Developing NICE guidelines: the manual’ in 2006, which has been updated since, with the most recent in 2018 [ 9 ]. It was intended to be a guidance document to aid in the production of NICE guidelines across all NICE topics. In terms of synthesising quantitative evidence, the NICE recommendations state: ‘meta-analysis may be appropriate if treatment estimates of the same outcome from more than 1 study are available’ and ‘when multiple competing options are being appraised, a network meta-analysis should be considered’. The implementation of network meta-analysis (NMA), which is described later, as a recommendation from NICE was introduced into the guidance document in 2014, with a further update in 2018.
Background to the previous review
The paper by Achana et al. (2014) explored the use of evidence synthesis methodology in NICE public health intervention guidelines published between 2006 and 2012 [ 3 ]. The authors conducted a systematic review of the methods used to synthesise quantitative effectiveness evidence within NICE public health guidelines. They found that only 23% of NICE public health guidelines used pairwise meta-analysis as part of the effectiveness review and the remainder used a narrative summary or no synthesis of evidence at all. The authors argued that despite significant advances in the methodology of evidence synthesis, the uptake of methods in public health intervention evaluation is lower than other fields, including clinical treatment evaluation. The paper concluded that more sophisticated methods in evidence synthesis should be considered to aid in decision making in the public health context [ 3 ].
The search strategy used in this paper was equivalent to that in the previous paper by Achana et al. (2014)[ 3 ]. The search was conducted through the NICE website ( https://www.nice.org.uk/guidance ) by searching the ‘Guidance and Advice List’ and filtering by ‘Public Health Guidelines’ [ 10 ]. The search criteria included all guidance documents that had been published from inception (March 2006) until the 19th August 2019. Since the original review, many of the guidelines had been updated with new documents or merged. Guidelines that remained unchanged since the previous review in 2012 were excluded and used for comparison.
The guidelines contained multiple documents that were assessed for relevance. A systematic review is a separate synthesis within a guideline that systematically collates all evidence on a specific research question of interest in the literature. Systematic reviews of quantitative effectiveness, cost-effectiveness evidence and decision modelling reports were all included as relevant. Qualitative reviews, field reports, expert opinions, surveillance reports, review decisions and other supporting documents were excluded at the search stage.
Within the reports, data was extracted on the types of review (narrative summary, pairwise meta-analysis, network meta-analysis (NMA), cost-effectiveness review or decision model), design of included primary studies (randomised controlled trials or non-randomised studies, intermediate or final outcomes, description of outcomes, outcome measure statistic), details of the synthesis methods used in the effectiveness evaluation (type of synthesis, fixed or random effects model, study quality assessment, publication bias assessment, presentation of results, software). Further details of the interventions were also recorded, including whether multiple interventions were lumped together for a pairwise comparison, whether interventions were complex (made up of multiple components) and details of the components. The reports were also assessed for potential use of complex intervention evidence synthesis methodology, meaning that the interventions that were evaluated in the review were made up of components that could potentially be synthesised using an NMA or a component NMA [ 11 ]. Where meta-analysis was not used to synthesis effectiveness evidence, the reasons for this was also recorded.
Search results and types of reviews
There were 67 NICE public health guidelines available on the NICE website. A summary flow diagram describing the literature identification process and the list of guidelines and their reference codes are provided in Additional files 1 and 2 . Since the previous review, 22 guidelines had not been updated. The results from the previous review were used for comparison to the 45 guidelines that were either newly published or updated.
The guidelines consisted of 508 documents that were assessed for relevance. Table 1 shows which types of relevant documents were available in each of the 45 guidelines. The median number of relevant articles per guideline was 3 (minimum = 0, maximum = 10). Two (4%) of the NICE public health guidelines did not report any type of systematic review, cost-effectiveness review or decision model (NG68, NG64) that met the inclusion criteria. 167 documents from 43 NICE public health guidelines were systematic reviews of quantitative effectiveness, cost-effectiveness or decision model reports and met the inclusion criteria.
Narrative reviews of effectiveness were implemented in 41 (91%) of the NICE PH guidelines. 14 (31%) contained a review that used meta-analysis to synthesise the evidence. Only one (1%) NICE guideline contained a review that implemented NMA to synthesise the effectiveness of multiple interventions; this was the same guideline that used NMA in the original review and had been updated. 33 (73%) guidelines contained cost-effectiveness reviews and 34 (76%) developed a decision model.
Comparison of review types to original review
Table 2 compares the results of the update to the original review and shows that the types of reviews and evidence synthesis methodologies remain largely unchanged since 2012. The proportion of guidelines that only contain narrative reviews to synthesise effectiveness or cost-effectiveness evidence has reduced from 74% to 60% and the proportion that included a meta-analysis has increased from 23% to 31%. The proportion of guidelines with reviews that only included evidence from randomised controlled trials and assessed the quality of individual studies remained similar to the original review.
Characteristics of guidelines using meta-analytic methods
Table 3 details the characteristics of the meta-analytic methods implemented in 24 reviews of the 14 guidelines that included one. All of the reviews reported an assessment of study quality, 12 (50%) reviews included only data from randomised controlled trials, 4 (17%) reviews used intermediate outcomes (e.g. uptake of chlamydia screening rather than prevention of chlamydia (PH3)), compared to the 20 (83%) reviews that used final outcomes (e.g. smoking cessation rather than uptake of a smoking cessation programme (NG92)). 2 (8%) reviews only used a fixed effect meta-analysis, 19 (79%) reviews used a random effects meta-analysis and 3 (13%) did not report which they had used.
An evaluation of the intervention information reported in the reviews concluded that 12 (50%) reviews had lumped multiple (more than two) different interventions into a control versus intervention pairwise meta-analysis. Eleven (46%) of the reviews evaluated interventions that are made up of multiple components (e.g. interventions for preventing obesity in PH47 were made up of diet, physical activity and behavioural change components).
21 (88%) of the reviews presented the results of the meta-analysis in the form of a forest plot and 22 (92%) presented the results in the text of the report. 20 (83%) of the reviews used two or more forms of presentation for the results. Only three (13%) reviews assessed publication bias. The most common software to perform meta-analysis was RevMan in 14 (58%) of the reviews.
Reasons for not using meta-analytic methods
The 143 reviews of effectiveness and cost effectiveness that did not use meta-analysis methods to synthesise the quantitative effectiveness evidence were searched for reasons behind this decision. 70 reports (49%) did not give a reason for not synthesising the data using a meta-analysis and 164 reasons were reported which are displayed in Fig. 1 . Out of the remaining reviews, multiple reasons for not using a meta-analysis were given. 53 (37%) of the reviews reported at least one reason due to heterogeneity. 30 (21%) decision model reports did not give a reason and these are categorised separately. 5 (3%) reviews reported that meta-analysis was not applicable or feasible, 1 (1%) reported that they were following NICE guidelines and 5 (3%) reported that there were a lack of studies.
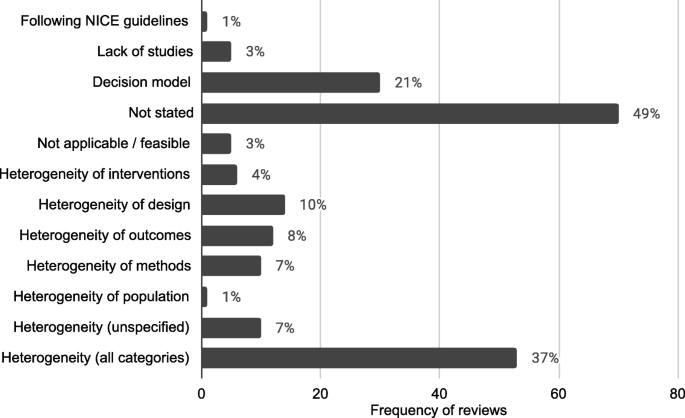
Frequency and proportions of reasons reported for not using statistical methods in quantitative evidence synthesis in NICE PH intervention reviews
The frequency of reviews and guidelines that used meta-analytic methods were plotted against year of publication, which is reported in Fig. 2 . This showed that the number of reviews that used meta-analysis were approximately constant but there is some suggestion that the number of meta-analyses used per guideline increased, particularly in 2018.
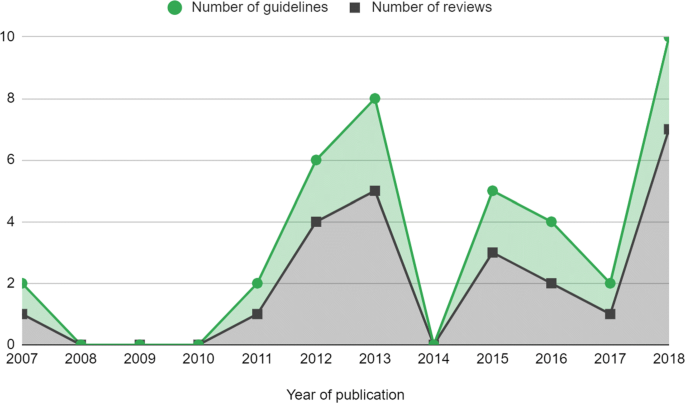
Number of meta-analyses in NICE PH guidelines by year. Guidelines that were published before 2012 had been updated since the previous review by Achana et al. (2014) [ 3 ]
Comparison of meta-analysis characteristics to original review
Table 4 compares the characteristics of the meta-analyses used in the evidence synthesis of NICE public health intervention guidelines to the original review by Achana et al. (2014) [ 3 ]. Overall, the characteristics in the updated review have not much changed from those in the original. These changes demonstrate that the use of meta-analysis in NICE guidelines has increased but remains low. Lumping of interventions still appears to be common in 50% of reviews. The implications of this are discussed in the next section.
Application of evidence synthesis methodology in a public health intervention: motivating example
Since the original review, evidence synthesis methods have been developed and can address some of the challenges of synthesising quantitative effectiveness evidence of public health interventions. Despite this, the previous section shows that the uptake of these methods is still low in NICE public health guidelines - usually limited to a pairwise meta-analysis.
It has been shown in the results above and elsewhere [ 12 ] that heterogeneity is a common reason for not synthesising the quantitative effectiveness evidence available from systematic reviews in public health. Statistical heterogeneity is the variation in the intervention effects between the individual studies. Heterogeneity is problematic in evidence synthesis as it leads to uncertainty in the pooled effect estimates in a meta-analysis which can make it difficult to interpret the pooled results and draw conclusions. Rather than exploring the source of the heterogeneity, often in public health intervention appraisals a random effects model is fitted which assumes that the study intervention effects are not equivalent but come from a common distribution [ 13 , 14 ]. Alternatively, as demonstrated in the review update, heterogeneity is used as a reason to not undertake any quantitative evidence synthesis at all.
Since the size of the intervention effects and the methodological variation in the studies will affect the impact of the heterogeneity on a meta-analysis, it is inappropriate to base the methodological approach of a review on the degree of heterogeneity, especially within public health intervention appraisal where heterogeneity seems inevitable. Ioannidis et al. (2008) argued that there are ‘almost always’ quantitative synthesis options that may offer some useful insights in the presence of heterogeneity, as long as the reviewers interpret the findings with respect to their limitations [ 12 ].
In this section current evidence synthesis methods are applied to a motivating example in public health. This aims to demonstrate that methods beyond pairwise meta-analysis can provide appropriate and pragmatic information to public health decision makers to enable more informed decision making.
Figure 3 summarises the narrative of this part of the paper and illustrates the methods that are discussed. The red boxes represent the challenges in synthesising quantitative effectiveness evidence and refers to the section within the paper for more detail. The blue boxes represent the methods that can be applied to investigate each challenge.
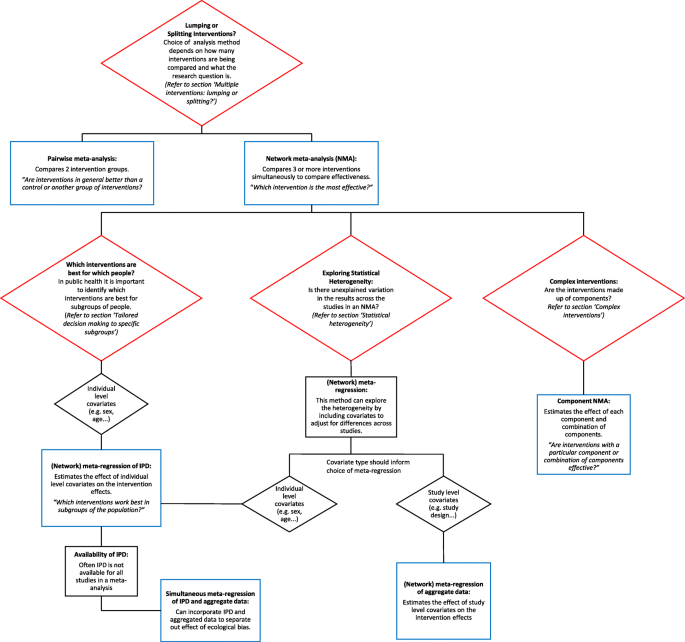
Summary of challenges that are faces in the evidence synthesis of public health interventions and methods that are discussed to overcome these challenges
Evaluating the effect of interventions for promoting the safe storage of cleaning products to prevent childhood poisoning accidents
To illustrate the methodological developments, a motivating example is used from the five year, NIHR funded, Keeping Children Safe Programme [ 15 ]. The project included a Cochrane systematic review that aimed to increase the use of safety equipment to prevent accidents at home in children under five years old. This application is intended to be illustrative of the benefits of new evidence synthesis methods since the previous review. It is not a complete, comprehensive analysis as it only uses a subset of the original dataset and therefore the results are not intended to be used for policy decision making. This example has been chosen as it demonstrates many of the issues in synthesising effectiveness evidence of public health interventions, including different study designs (randomised controlled trials, observational studies and cluster randomised trials), heterogeneity of populations or settings, incomplete individual participant data and complex interventions that contain multiple components.
This analysis will investigate the most effective promotional interventions for the outcome of ‘safe storage of cleaning products’ to prevent childhood poisoning accidents. There are 12 studies included in the dataset, with IPD available from nine of the studies. The covariate, single parent family, is included in the analysis to demonstrate the effect of being a single parent family on the outcome. In this example, all of the interventions are made up of one or more of the following components: education (Ed), free or low cost equipment (Eq), home safety inspection (HSI), and installation of safety equipment (In). A Bayesian approach using WinBUGS was used and therefore credible intervals (CrI) are presented with estimates of the effect sizes [ 16 ].
The original review paper by Achana et al. (2014) demonstrated pairwise meta-analysis and meta-regression using individual and cluster allocated trials, subgroup analyses, meta-regression using individual participant data (IPD) and summary aggregate data and NMA. This paper firstly applies NMA to the motivating example for context, followed by extensions to NMA.
Multiple interventions: lumping or splitting?
Often in public health there are multiple intervention options. However, interventions are often lumped together in a pairwise meta-analysis. Pairwise meta-analysis is a useful tool for two interventions or, alternatively in the presence of lumping interventions, for answering the research question: ‘are interventions in general better than a control or another group of interventions?’. However, when there are multiple interventions, this type of analysis is not appropriate for informing health care providers which intervention should be recommended to the public. ‘Lumping’ is becoming less frequent in other areas of evidence synthesis, such as for clinical interventions, as the use of sophisticated synthesis techniques, such as NMA, increases (Achana et al. 2014) but lumping is still common in public health.
NMA is an extension of the pairwise meta-analysis framework to more than two interventions. Multiple interventions that are lumped into a pairwise meta-analysis are likely to demonstrate high statistical heterogeneity. This does not mean that quantitative synthesis could not be undertaken but that a more appropriate method, NMA, should be implemented. Instead the statistical approach should be based on the research questions of the systematic review. For example, if the research question is ‘are any interventions effective for preventing obesity?’, it would be appropriate to perform a pairwise meta-analysis comparing every intervention in the literature to a control. However, if the research question is ‘which intervention is the most effective for preventing obesity?’, it would be more appropriate and informative to perform a network meta-analysis, which can compare multiple interventions simultaneously and identify the best one.
NMA is a useful statistical method in the context of public health intervention appraisal, where there are often multiple intervention options, as it estimates the relative effectiveness of three or more interventions simultaneously, even if direct study evidence is not available for all intervention comparisons. Using NMA can help to answer the research question ‘what is the effectiveness of each intervention compared to all other interventions in the network?’.
In the motivating example there are six intervention options. The effect of lumping interventions is shown in Fig. 4 , where different interventions in both the intervention and control arms are compared. There is overlap of intervention and control arms across studies and interpretation of the results of a pairwise meta-analysis comparing the effectiveness of the two groups of interventions would not be useful in deciding which intervention to recommend. In comparison, the network plot in Fig. 5 illustrates the evidence base of the prevention of childhood poisonings review comparing six interventions that promote the use of safety equipment in the home. Most of the studies use ‘usual care’ as a baseline and compare this to another intervention. There are also studies in the evidence base that compare pairs of the interventions, such as ‘Education and equipment’ to ‘Equipment’. The plot also demonstrates the absence of direct study evidence between many pairs of interventions, for which the associated treatment effects can be indirectly estimated using NMA.
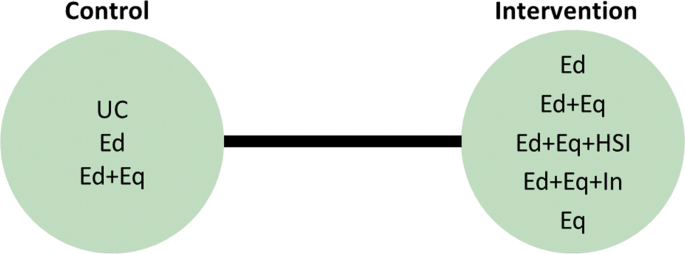
Network plot to illustrate how pairwise meta-analysis groups the interventions in the motivating dataset. Notation UC: Usual care, Ed: Education, Ed+Eq: Education and equipment, Ed+Eq+HSI: Education, equipment, and home safety inspection, Ed+Eq+In: Education, equipment and installation, Eq: Equipment
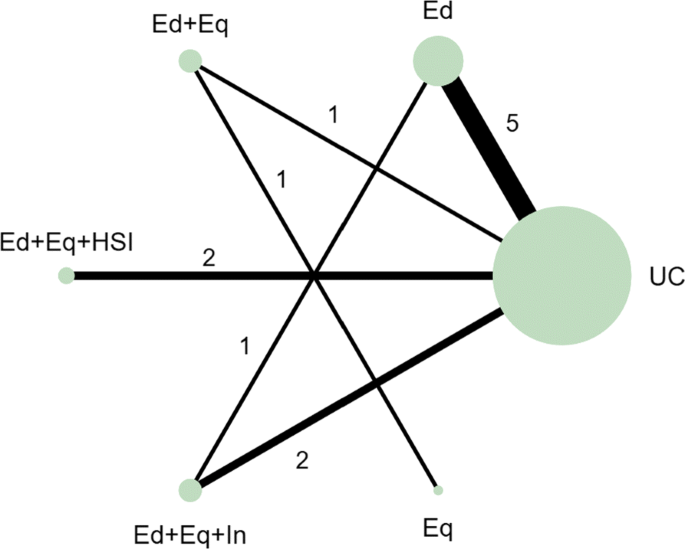
Network plot for the safe storage of cleaning products outcome. Notation UC: Usual care, Ed: Education, Ed+Eq: Education and equipment, Ed+Eq+HSI: Education, equipment, and home safety inspection, Ed+Eq+In: Education, equipment and installation, Eq: Equipment
An NMA was fitted to the motivating example to compare the six interventions in the studies from the review. The results are reported in the ‘triangle table’ in Table 5 [ 17 ]. The top right half of the table shows the direct evidence between pairs of the interventions in the corresponding rows and columns by either pooling the studies as a pairwise meta-analysis or presenting the single study results if evidence is only available from a single study. The bottom left half of the table reports the results of the NMA. The gaps in the top right half of the table arise where no direct study evidence exists to compare the two interventions. For example, there is no direct study evidence comparing ‘Education’ (Ed) to ‘Education, equipment and home safety inspection’ (Ed+Eq+HSI). The NMA, however, can estimate this comparison through the direct study evidence as an odds ratio of 3.80 with a 95% credible interval of (1.16, 12.44). The results suggest that the odds of safely storing cleaning products in the Ed+Eq+HSI intervention group is 3.80 times the odds in the Ed group. The results demonstrate a key benefit of NMA that all intervention effects in a network can be estimated using indirect evidence, even if there is no direct study evidence for some pairwise comparisons. This is based on the consistency assumption (that estimates of intervention effects from direct and indirect evidence are consistent) which should be checked when performing an NMA. This is beyond the scope of this paper and details on this can be found elsewhere [ 18 ].
NMA can also be used to rank the interventions in terms of their effectiveness and estimate the probability that each intervention is likely to be the most effective. This can help to answer the research question ‘which intervention is the best?’ out of all of the interventions that have provided evidence in the network. The rankings and associated probabilities for the motivating example are presented in Table 6 . It can be seen that in this case the ‘education, equipment and home safety inspection’ (Ed+Eq+HSI) intervention is ranked first, with a 0.87 probability of being the best intervention. However, there is overlap of the 95% credible intervals of the median rankings. This overlap reflects the uncertainty in the intervention effect estimates and therefore it is important that the interpretation of these statistics clearly communicates this uncertainty to decision makers.
NMA has the potential to be extremely useful but is underutilised in the evidence synthesis of public health interventions. The ability to compare and rank multiple interventions in an area where there are often multiple intervention options is invaluable in decision making for identifying which intervention to recommend. NMA can also include further literature in the analysis, compared to a pairwise meta-analysis, by expanding the network to improve the uncertainty in the effectiveness estimates.
Statistical heterogeneity
When heterogeneity remains in the results of an NMA, it is useful to explore the reasons for this. Strategies for dealing with heterogeneity involve the inclusion of covariates in a meta-analysis or NMA to adjust for the differences in the covariates across studies [ 19 ]. Meta-regression is a statistical method developed from meta-analysis that includes covariates to potentially explain the between-study heterogeneity ‘with the aim of estimating treatment-covariate interactions’ (Saramago et al. 2012). NMA has been extended to network meta-regression which investigates the effect of trial characteristics on multiple intervention effects. Three ways have been suggested to include covariates in an NMA: single covariate effect, exchangeable covariate effects and independent covariate effects which are discussed in more detail in the NICE Technical Support Document 3 [ 14 ]. This method has the potential to assess the effect of study level covariates on the intervention effects, which is particularly relevant in public health due to the variation across studies.
The most widespread method of meta-regression uses study level data for the inclusion of covariates into meta-regression models. Study level covariate data is when the data from the studies are aggregated, e.g. the proportion of participants in a study that are from single parent families compared to dual parent families. The alternative to study level data is individual participant data (IPD), where the data are available and used as a covariate at the individual level e.g. the parental status of every individual in a study can be used as a covariate. Although IPD is considered to be the gold standard for meta-analysis, aggregated level data is much more commonly used as it is usually available and easily accessible from published research whereas IPD can be hard to obtain from study authors.
There are some limitations to network meta-regression. In our motivating example, using the single parent covariate in a meta-regression would estimate the relative difference in the intervention effects of a population that is made up of 100% single parent families compared to a population that is made up of 100% dual parent families. This interpretation is not as useful as the analysis that uses IPD, which would give the relative difference of the intervention effects in a single parent family compared to a dual parent family. The meta-regression using aggregated data would also be susceptible to ecological bias. Ecological bias is where the effect of the covariate is different at the study level compared to the individual level [ 14 ]. For example, if each study demonstrates a relationship between a covariate and the intervention but the covariate is similar across the studies, a meta-regression of the aggregate data would not demonstrate the effect that is observed within the studies [ 20 ].
Although meta-regression is a useful tool for investigating sources of heterogeneity in the data, caution should be taken when using the results of meta-regression to explain how covariates affect the intervention effects. Meta-regression should only be used to investigate study characteristics, such as the duration of intervention, which will not be susceptible to ecological bias and the interpretation of the results (the effect of intervention duration on intervention effectiveness) would be more meaningful for the development of public health interventions.
Since the covariate of interest in this motivating example is not a study characteristic, meta-regression of aggregated covariate data was not performed. Network meta-regression including IPD and aggregate level data was developed by Samarago et al. (2012) [ 21 ] to overcome the issues with aggregated data network meta-regression, which is discussed in the next section.
Tailored decision making to specific sub-groups
In public health it is important to identify which interventions are best for which people. There has been a recent move towards precision medicine. In the field of public health the ‘concept of precision prevention may [...] be valuable for efficiently targeting preventive strategies to the specific subsets of a population that will derive maximal benefit’ (Khoury and Evans, 2015). Tailoring interventions has the potential to reduce the effect of inequalities in social factors that are influencing the health of the population. Identifying which interventions should be targeted to which subgroups can also lead to better public health outcomes and help to allocate scarce NHS resources. Research interest, therefore, lies in identifying participant level covariate-intervention interactions.
IPD meta-analysis uses data at the individual level to overcome ecological bias. The interpretation of IPD meta-analysis is more relevant in the case of using participant characteristics as covariates since the interpretation of the covariate-intervention interaction is at the individual level rather than the study level. This means that it can answer the research question: ‘which interventions work best in subgroups of the population?’. IPD meta-analyses are considered to be the gold standard for evidence synthesis since it increases the power of the analysis to identify covariate-intervention interactions and it has the ability to reduce the effect of ecological bias compared to aggregated data alone. IPD meta-analysis can also help to overcome scarcity of data issues and has been shown to have higher power and reduce the uncertainty in the estimates compared to analysis including only summary aggregate data [ 22 ].
Despite the advantages of including IPD in a meta-analysis, in reality it is often very time consuming and difficult to collect IPD for all of the studies [ 21 ]. Although data sharing is becoming more common, it remains time consuming and difficult to collect IPD for all studies in a review. This results in IPD being underutilised in meta-analyses. As an intermediate solution, statistical methods have been developed, such as the NMA in Samarago et al. (2012), that incorporates both IPD and aggregate data. Methods that simultaneously include IPD and aggregate level data have been shown to reduce uncertainty in the effect estimates and minimise ecological bias [ 20 , 21 ]. A simulation study by Leahy et al. (2018) found that an increased proportion of IPD resulted in more accurate and precise NMA estimates [ 23 ].
An NMA including IPD, where it is available, was performed, based on the model presented in Samarago et al. (2012) [ 21 ]. The results in Table 7 demonstrates the detail that this type of analysis can provide to base decisions on. More relevant covariate-intervention interaction interpretations can be obtained, for example the regression coefficients for covariate-intervention interactions are the individual level covariate intervention interactions or the ‘within study interactions’ that are interpreted as the effect of being in a single parent family on the effectiveness of each of the interventions. For example, the effect of Ed+Eq compared to UC in a single parent family is 1.66 times the effect of Ed+Eq compared to UC in a dual parent family but this is not an important difference as the credible interval crosses 1. The regression coefficients for the study level covariate-intervention interactions or the ‘between study interactions’ can be interpreted as the relative difference in the intervention effects of a population that is made up of 100% single parent families compared to a population that is made up of 100% dual parent families.
- Complex interventions
In many public health research settings the complex interventions are comprised of a number of components. An NMA can compare all of the interventions in a network as they are implemented in the original trials. However, NMA does not tell us which components of the complex intervention are attributable to this effect. It could be that particular components, or the interacting effect of multiple components, are driving the effectiveness and other components are not as effective. Often, trials have not directly compared every combination of components as there are so many component combination options, it would be inefficient and impractical. Component NMA was developed by Welton et al. (2009) to estimate the effect of each component of the complex interventions and combination of components in a network, in the absence of direct trial evidence and answers the question: ‘are interventions with a particular component or combination of components effective?’ [ 11 ]. For example, for the motivating example, in comparison to Fig. 5 , which demonstrates the interventions that an NMA can estimate effectiveness, Fig. 6 demonstrates all of the possible interventions of which the effectiveness can be estimated in a component NMA, given the components present in the network.
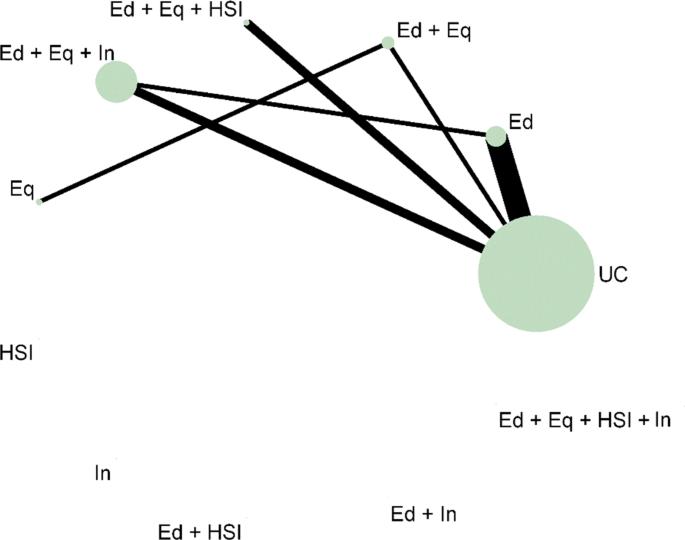
Network plot that illustrates how component network meta-analysis can estimate the effectiveness of intervention components and combinations of components, even when they are not included in the direct evidence. Notation UC: Usual care, Ed: Education, Eq: Equipment, Installation, Ed+Eq: Education and equipment, Ed+HSI: Education and home safety inspection, Ed+In: Education and installation, Eq+HSI: Equipment and home safety inspection, Eq+In: equipment and installation, HSI+In: Home safety inspection and installation, Ed+Eq+HSI: Education, equipment, and home safety inspection, Ed+Eq+In: Education, equipment and installation, Eq+HSI+In: Equipment, home safety inspection and installation, Ed+Eq+HSI+In: Education, equipment, home safety inspection and installation
The results of the analyses of the main effects, two way effects and full effects models are shown in Table 8 . The models, proposed in the original paper by Welton et al. (2009), increase in complexity as the assumptions regarding the component effects relax [ 24 ]. The main effects component NMA assumes that the components in the interventions each have separate, independent effects and intervention effects are the sum of the component effects. The two-way effects models assumes that there are interactions between pairs of the components, so the effects of the interventions are more than the sum of the effects. The full effects model assumes that all of the components and combinations of the components interact. Component NMA did not provide further insight into which components are likely to be the most effective since all of the 95% credible intervals were very wide and overlapped 1. There is a lot of uncertainty in the results, particularly in the 2-way and full effects models. A limitation of component NMA is that there are issues with uncertainty when data is scarce. However, the results demonstrate the potential of component NMA as a useful tool to gain better insights from the available dataset.
In practice, this method has rarely been used since its development [ 24 – 26 ]. It may be challenging to define the components in some areas of public health where many interventions have been studied. However, the use of meta-analysis for planning future studies is rarely discussed and component NMA would provide a useful tool for identifying new component combinations that may be more effective [ 27 ]. This type of analysis has the potential to prioritise future public health research, which is especially useful where there are multiple intervention options, and identify more effective interventions to recommend to the public.
Further methods / other outcomes
The analysis and methods described in this paper only cover a small subset of the methods that have been developed in meta-analysis in recent years. Methods that aim to assess the quality of evidence supporting a NMA and how to quantify how much the evidence could change due to potential biases or sampling variation before the recommendation changes have been developed [ 28 , 29 ]. Models adjusting for baseline risk have been developed to allow for different study populations to have different levels of underlying risk, by using the observed event rate in the control arm [ 30 , 31 ]. Multivariate methods can be used to compare the effect of multiple interventions on two or more outcomes simultaneously [ 32 ]. This area of methodological development is especially appealing within public health where studies assess a broad range of health effects and typically have multiple outcome measures. Multivariate methods offer benefits over univariate models by allowing the borrowing of information across outcomes and modelling the relationships between outcomes which can potentially reduce the uncertainty in the effect estimates [ 33 ]. Methods have also been developed to evaluate interventions with classes or different intervention intensities, known as hierarchical interventions [ 34 ]. These methods were not demonstrated in this paper but can also be useful tools for addressing challenges of appraising public health interventions, such as multiple and surrogate outcomes.
This paper only considered an example with a binary outcome. All of the methods described have also been adapted for other outcome measures. For example, the Technical Support Document 2 proposed a Bayesian generalised linear modelling framework to synthesise other outcome measures. More information and models for continuous and time-to-event data is available elsewhere [ 21 , 35 – 38 ].
Software and guidelines
In the previous section, meta-analytic methods that answer more policy relevant questions were demonstrated. However, as shown by the update to the review, methods such as these are still under-utilised. It is suspected from the NICE public health review that one of the reasons for the lack of uptake of methods in public health could be due to common software choices, such as RevMan, being limited in their flexibility for statistical methods.
Table 9 provides a list of software options and guidance documents that are more flexible than RevMan for implementing the statistical methods illustrated in the previous section to make these methods more accessible to researchers.
In this paper, the network plot in Figs. 5 and 6 were produced using the networkplot command from the mvmeta package [ 39 ] in Stata [ 61 ]. WinBUGS was used to fit the NMA in this paper by adapting the code in the book ‘Evidence Synthesis for Decision Making in Healthcare’ which also provides more detail on Bayesian methods and assessing convergence of Bayesian models [ 45 ]. The model for including IPD and summary aggregate data in an NMA was based on the code in the paper by Saramago et al. (2012). The component NMA in this paper was performed in WinBUGS through R2WinBUGS, [ 47 ] using the code in Welton et al. (2009) [ 11 ].
WinBUGS is a flexible tool for fitting complex models in a Bayesian framework. The NICE Decision Support Unit produced a series of Evidence Synthesis Technical Support Documents [ 46 ] that provide a comprehensive technical guide to methods for evidence synthesis and WinBUGS code is also provided for many of the models. Complex models can also be performed in a frequentist framework. Code and commands for many models are available in R and STATA (see Table 9 ).
The software, R2WinBUGS, was used in the analysis of the motivating example. Increasing numbers of researchers are using R and so packages that can be used to link the two softwares by calling BUGS models in R, packages such as R2WinBUGS, can improve the accessibility of Bayesian methods [ 47 ]. The new R package, BUGSnet, may also help to facilitate the accessibility and improve the reporting of Bayesian NMA [ 48 ]. Webtools have also been developed as a means of enabling researchers to undertake increasingly complex analyses [ 52 , 53 ]. Webtools provide a user-friendly interface to perform statistical analyses and often help in the reporting of the analyses by producing plots, including network plots and forest plots. These tools are very useful for researchers that have a good understanding of the statistical methods they want to implement as part of their review but are inexperienced in statistical software.
This paper has reviewed NICE public health intervention guidelines to identify the methods that are currently being used to synthesise effectiveness evidence to inform public health decision making. A previous review from 2012 was updated to see how method utilisation has changed. Methods have been developed since the previous review and these were applied to an example dataset to show how methods can answer more policy relevant questions. Resources and guidelines for implementing these methods were signposted to encourage uptake.
The review found that the proportion of NICE guidelines containing effectiveness evidence summarised using meta-analysis methods has increased since the original review, but remains low. The majority of the reviews presented only narrative summaries of the evidence - a similar result to the original review. In recent years, there has been an increased awareness of the need to improve decision making by using all of the available evidence. As a result, this has led to the development of new methods, easier application in standard statistical software packages, and guidance documents. Based on this, it would have been expected that their implementation would rise in recent years to reflect this, but the results of the review update showed no such increasing pattern.
A high proportion of NICE guideline reports did not provide a reason for not applying quantitative evidence synthesis methods. Possible explanations for this could be time or resource constraints, lack of statistical expertise, being unaware of the available methods or poor reporting. Reporting guidelines, such as the Preferred Reporting Items for Systematic Reviews and Meta-Analyses (PRISMA), should be updated to emphasise the importance of documenting reasons for not applying methods, as this can direct future research to improve uptake.
Where it was specified, the most common reported reason for not conducting a meta-analysis was heterogeneity. Often in public health, the data is heterogeneous due to the differences between studies in population, design, interventions or outcomes. A common misconception is that the presence of heterogeneity implies that it is not possible to pool the data. Meta-analytic methods can be used to investigate the sources of heterogeneity, as demonstrated in the NMA of the motivating example, and the use of IPD is recommended where possible to improve the precision of the results and reduce the effect of ecological bias. Although caution should be exercised in the interpretation of the results, quantitative synthesis methods provide a stronger basis for making decisions than narrative accounts because they explicitly quantify the heterogeneity and seek to explain it where possible.
The review also found that the most common software to perform the synthesis was RevMan. RevMan is very limited in its ability to perform advanced statistical analyses, beyond that of pairwise meta-analysis, which might explain the above findings. Standard software code is being developed to help make statistical methodology and application more accessible and guidance documents are becoming increasingly available.
The evaluation of public health interventions can be problematic due to the number and complexity of the interventions. NMA methods were applied to a real Cochrane public health review dataset. The methods that were demonstrated showed ways to address some of these issues, including the use of NMA for multiple interventions, the inclusion of covariates as both aggregated data and IPD to explain heterogeneity, and the extension to component network meta-analysis for guiding future research. These analyses illustrated how the choice of synthesis methods can enable more informed decision making by allowing more distinct interventions, and combinations of intervention components, to be defined and their effectiveness estimated. It also demonstrated the potential to target interventions to population subgroups where they are likely to be most effective. However, the application of component NMA to the motivating example has also demonstrated the issues around uncertainty if there are a limited number of studies observing the interventions and intervention components.
The application of methods to the motivating example demonstrated a key benefit of using statistical methods in a public health context compared to only presenting a narrative review – the methods provide a quantitative estimate of the effectiveness of the interventions. The uncertainty from the credible intervals can be used to demonstrate the lack of available evidence. In the context of decision making, having pooled estimates makes it much easier for decision makers to assess the effectiveness of the interventions or identify when more research is required. The posterior distribution of the pooled results from the evidence synthesis can also be incorporated into a comprehensive decision analytic model to determine cost-effectiveness [ 62 ]. Although narrative reviews are useful for describing the evidence base, the results are very difficult to summarise in a decision context.
Although heterogeneity seems to be inevitable within public health interventions due to their complex nature, this review has shown that it is still the main reported reason for not using statistical methods in evidence synthesis. This may be due to guidelines that were originally developed for clinical treatments that are tested in randomised conditions still being applied in public health settings. Guidelines for the choice of methods used in public health intervention appraisals could be updated to take into account the complexities and wide ranging areas in public health. Sophisticated methods may be more appropriate in some cases than simpler models for modelling multiple, complex interventions and their uncertainty, given the limitations are also fully reported [ 19 ]. Synthesis may not be appropriate if statistical heterogeneity remains after adjustment for possible explanatory covariates but details of exploratory analysis and reasons for not synthesising the data should be reported. Future research should focus on the application and dissemination of the advantages of using more advanced methods in public health, identifying circumstances where these methods are likely to be the most beneficial, and ways to make the methods more accessible, for example, the development of packages and web tools.
There is an evident need to facilitate the translation of the synthesis methods into a public health context and encourage the use of methods to improve decision making. This review has shown that the uptake of statistical methods for evaluating the effectiveness of public health interventions is slow, despite advances in methods that address specific issues in public health intervention appraisal and the publication of guidance documents to complement their application.
Availability of data and materials
The dataset supporting the conclusions of this article is included within the article.
Abbreviations
National institute for health and care excellence
- Network meta-analysis
Individual participant data
Home safety inspection
Installation
Credible interval
Preferred reporting items for systematic reviews and meta-analyses
Dias S, Welton NJ, Sutton AJ, Ades A. NICE DSU Technical Support Document 2: A Generalised Linear Modelling Framework for Pairwise and Network Meta-Analysis of Randomised Controlled Trials: National Institute for Health and Clinical Excellence; 2011, p. 98. (Technical Support Document in Evidence Synthesis; TSD2).
Higgins JPT, López-López JA, Becker BJ, et al.Synthesising quantitative evidence in systematic reviews of complex health interventions. BMJ Global Health. 2019; 4(Suppl 1):e000858. https://doi.org/10.1136/bmjgh-2018-000858 .
Article PubMed PubMed Central Google Scholar
Achana F, Hubbard S, Sutton A, Kendrick D, Cooper N. An exploration of synthesis methods in public health evaluations of interventions concludes that the use of modern statistical methods would be beneficial. J Clin Epidemiol. 2014; 67(4):376–90.
Article PubMed Google Scholar
Craig P, Dieppe P, Macintyre S, Michie S, Nazareth I, Petticrew M. Developing and evaluating complex interventions: the new medical research council guidance. Int J Nurs Stud. 2013; 50(5):587–92.
Caldwell DM, Welton NJ. Approaches for synthesising complex mental health interventions in meta-analysis. Evidence-Based Mental Health. 2016; 19(1):16–21.
Melendez-Torres G, Bonell C, Thomas J. Emergent approaches to the meta-analysis of multiple heterogeneous complex interventions. BMC Med Res Methodol. 2015; 15(1):47.
Article CAS PubMed PubMed Central Google Scholar
NICE. NICE: Who We Are. https://www.nice.org.uk/about/who-we-are . Accessed 19 Sept 2019.
Kelly M, Morgan A, Ellis S, Younger T, Huntley J, Swann C. Evidence based public health: a review of the experience of the national institute of health and clinical excellence (NICE) of developing public health guidance in England. Soc Sci Med. 2010; 71(6):1056–62.
NICE. Developing NICE Guidelines: The Manual. https://www.nice.org.uk/process/pmg20/chapter/introduction-and-overview . Accessed 19 Sept 2019.
NICE. Public Health Guidance. https://www.nice.org.uk/guidance/published?type=ph . Accessed 19 Sept 2019.
Welton NJ, Caldwell D, Adamopoulos E, Vedhara K. Mixed treatment comparison meta-analysis of complex interventions: psychological interventions in coronary heart disease. Am J Epidemiol. 2009; 169(9):1158–65.
Ioannidis JP, Patsopoulos NA, Rothstein HR. Reasons or excuses for avoiding meta-analysis in forest plots. BMJ. 2008; 336(7658):1413–5.
Higgins JP, Thompson SG. Quantifying heterogeneity in a meta-analysis. Stat Med. 2002; 21(11):1539–58.
Article Google Scholar
Dias S, Sutton A, Welton N, Ades A. NICE DSU Technical Support Document 3: Heterogeneity: Subgroups, Meta-Regression, Bias and Bias-Adjustment: National Institute for Health and Clinical Excellence; 2011, p. 76.
Kendrick D, Ablewhite J, Achana F, et al.Keeping Children Safe: a multicentre programme of research to increase the evidence base for preventing unintentional injuries in the home in the under-fives. Southampton: NIHR Journals Library; 2017.
Google Scholar
Lunn DJ, Thomas A, Best N, et al.WinBUGS - A Bayesian modelling framework: Concepts, structure, and extensibility. Stat Comput. 2000; 10:325–37. https://doi.org/10.1023/A:1008929526011 .
Dias S, Caldwell DM. Network meta-analysis explained. Arch Dis Child Fetal Neonatal Ed. 2019; 104(1):8–12. https://doi.org/10.1136/archdischild-2018-315224. http://arxiv.org/abs/https://fn.bmj.com/content/104/1/F8.full.pdf.
Dias S, Welton NJ, Sutton AJ, Caldwell DM, Lu G, Ades A. NICE DSU Technical Support Document 4: Inconsistency in Networks of Evidence Based on Randomised Controlled Trials: National Institute for Health and Clinical Excellence; 2011. (NICE DSU Technical Support Document in Evidence Synthesis; TSD4).
Cipriani A, Higgins JP, Geddes JR, Salanti G. Conceptual and technical challenges in network meta-analysis. Ann Intern Med. 2013; 159(2):130–7.
Riley RD, Steyerberg EW. Meta-analysis of a binary outcome using individual participant data and aggregate data. Res Synth Methods. 2010; 1(1):2–19.
Saramago P, Sutton AJ, Cooper NJ, Manca A. Mixed treatment comparisons using aggregate and individual participant level data. Stat Med. 2012; 31(28):3516–36.
Lambert PC, Sutton AJ, Abrams KR, Jones DR. A comparison of summary patient-level covariates in meta-regression with individual patient data meta-analysis. J Clin Epidemiol. 2002; 55(1):86–94.
Article CAS PubMed Google Scholar
Leahy J, O’Leary A, Afdhal N, Gray E, Milligan S, Wehmeyer MH, Walsh C. The impact of individual patient data in a network meta-analysis: an investigation into parameter estimation and model selection. Res Synth Methods. 2018; 9(3):441–69.
Freeman SC, Scott NW, Powell R, Johnston M, Sutton AJ, Cooper NJ. Component network meta-analysis identifies the most effective components of psychological preparation for adults undergoing surgery under general anesthesia. J Clin Epidemiol. 2018; 98:105–16.
Pompoli A, Furukawa TA, Efthimiou O, Imai H, Tajika A, Salanti G. Dismantling cognitive-behaviour therapy for panic disorder: a systematic review and component network meta-analysis. Psychol Med. 2018; 48(12):1945–53.
Rücker G, Schmitz S, Schwarzer G. Component network meta-analysis compared to a matching method in a disconnected network: A case study. Biom J. 2020. https://doi.org/10.1002/bimj.201900339 .
Efthimiou O, Debray TP, van Valkenhoef G, Trelle S, Panayidou K, Moons KG, Reitsma JB, Shang A, Salanti G, Group GMR. GetReal in network meta-analysis: a review of the methodology. Res Synth Methods. 2016; 7(3):236–63.
Salanti G, Del Giovane C, Chaimani A, Caldwell DM, Higgins JP. Evaluating the quality of evidence from a network meta-analysis. PLoS ONE. 2014; 9(7):99682.
Article CAS Google Scholar
Phillippo DM, Dias S, Welton NJ, Caldwell DM, Taske N, Ades A. Threshold analysis as an alternative to grade for assessing confidence in guideline recommendations based on network meta-analyses. Ann Intern Med. 2019; 170(8):538–46.
Dias S, Welton NJ, Sutton AJ, Ades AE. NICE DSU Technical Support Document 5: Evidence Synthesis in the Baseline Natural History Model: National Institute for Health and Clinical Excellence; 2011, p. 29. (NICE DSU Technical Support Document in Evidence Synthesis; TSD5).
Achana FA, Cooper NJ, Dias S, Lu G, Rice SJ, Kendrick D, Sutton AJ. Extending methods for investigating the relationship between treatment effect and baseline risk from pairwise meta-analysis to network meta-analysis. Stat Med. 2013; 32(5):752–71.
Riley RD, Jackson D, Salanti G, Burke DL, Price M, Kirkham J, White IR. Multivariate and network meta-analysis of multiple outcomes and multiple treatments: rationale, concepts, and examples. BMJ (Clinical research ed.) 2017; 358:j3932. https://doi.org/10.1136/bmj.j3932 .
Achana FA, Cooper NJ, Bujkiewicz S, Hubbard SJ, Kendrick D, Jones DR, Sutton AJ. Network meta-analysis of multiple outcome measures accounting for borrowing of information across outcomes. BMC Med Res Methodol. 2014; 14(1):92.
Owen RK, Tincello DG, Keith RA. Network meta-analysis: development of a three-level hierarchical modeling approach incorporating dose-related constraints. Value Health. 2015; 18(1):116–26.
Jansen JP. Network meta-analysis of individual and aggregate level data. Res Synth Methods. 2012; 3(2):177–90.
Donegan S, Williamson P, D’Alessandro U, Garner P, Smith CT. Combining individual patient data and aggregate data in mixed treatment comparison meta-analysis: individual patient data may be beneficial if only for a subset of trials. Stat Med. 2013; 32(6):914–30.
Saramago P, Chuang L-H, Soares MO. Network meta-analysis of (individual patient) time to event data alongside (aggregate) count data. BMC Med Res Methodol. 2014; 14(1):105.
Thom HH, Capkun G, Cerulli A, Nixon RM, Howard LS. Network meta-analysis combining individual patient and aggregate data from a mixture of study designs with an application to pulmonary arterial hypertension. BMC Med Res Methodol. 2015; 15(1):34.
Gasparrini A, Armstrong B, Kenward MG. Multivariate meta-analysis for non-linear and other multi-parameter associations. Stat Med. 2012; 31(29):3821–39.
Chaimani A, Higgins JP, Mavridis D, Spyridonos P, Salanti G. Graphical tools for network meta-analysis in stata. PLoS ONE. 2013; 8(10):76654.
Rücker G, Schwarzer G, Krahn U, König J. netmeta: Network meta-analysis with R. R package version 0.5-0. 2014. R package version 0.5-0. Availiable: http://CRAN.R-project.org/package=netmeta .
van Valkenhoef G, Kuiper J. gemtc: Network Meta-Analysis Using Bayesian Methods. R package version 0.8-2. 2016. Available online at: https://CRAN.R-project.org/package=gemtc .
Lin L, Zhang J, Hodges JS, Chu H. Performing arm-based network meta-analysis in R with the pcnetmeta package. J Stat Softw. 2017; 80(5):1–25. https://doi.org/10.18637/jss.v080.i05 .
Rücker G, Schwarzer G. Automated drawing of network plots in network meta-analysis. Res Synth Methods. 2016; 7(1):94–107.
Welton NJ, Sutton AJ, Cooper N, Abrams KR, Ades A. Evidence Synthesis for Decision Making in Healthcare, vol. 132. UK: Wiley; 2012.
Book Google Scholar
Dias S, Welton NJ, Sutton AJ, Ades AE. Evidence synthesis for decision making 1: introduction. Med Decis Making Int J Soc Med Decis Making. 2013; 33(5):597–606. https://doi.org/10.1177/0272989X13487604 .
Sturtz S, Ligges U, Gelman A. R2WinBUGS: a package for running WinBUGS from R. J Stat Softw. 2005; 12(3):1–16.
Béliveau A, Boyne DJ, Slater J, Brenner D, Arora P. Bugsnet: an r package to facilitate the conduct and reporting of bayesian network meta-analyses. BMC Med Res Methodol. 2019; 19(1):196.
Neupane B, Richer D, Bonner AJ, Kibret T, Beyene J. Network meta-analysis using R: a review of currently available automated packages. PLoS ONE. 2014; 9(12):115065.
White IR. Multivariate random-effects meta-analysis. Stata J. 2009; 9(1):40–56.
Chaimani A, Salanti G. Visualizing assumptions and results in network meta-analysis: the network graphs package. Stata J. 2015; 15(4):905–50.
Owen RK, Bradbury N, Xin Y, Cooper N, Sutton A. MetaInsight: An interactive web-based tool for analyzing, interrogating, and visualizing network meta-analyses using R-shiny and netmeta. Res Synth Methods. 2019; 10(4):569–81. https://doi.org/10.1002/jrsm.1373 .
Freeman SC, Kerby CR, Patel A, Cooper NJ, Quinn T, Sutton AJ. Development of an interactive web-based tool to conduct and interrogate meta-analysis of diagnostic test accuracy studies: MetaDTA. BMC Med Res Methodol. 2019; 19(1):81.
Nikolakopoulou A, Higgins JPT, Papakonstantinou T, Chaimani A, Del Giovane C, Egger M, Salanti G. CINeMA: An approach for assessing confidence in the results of a network meta-analysis. PLoS Med. 2020; 17(4):e1003082. https://doi.org/10.1371/journal.pmed.1003082 .
Viechtbauer W. Conducting meta-analyses in R with the metafor package. J Stat Softw. 2010; 36(3):1–48.
Freeman SC, Carpenter JR. Bayesian one-step ipd network meta-analysis of time-to-event data using royston-parmar models. Res Synth Methods. 2017; 8(4):451–64.
Riley RD, Lambert PC, Staessen JA, Wang J, Gueyffier F, Thijs L, Boutitie F. Meta-analysis of continuous outcomes combining individual patient data and aggregate data. Stat Med. 2008; 27(11):1870–93.
Debray TP, Moons KG, van Valkenhoef G, Efthimiou O, Hummel N, Groenwold RH, Reitsma JB, Group GMR. Get real in individual participant data (ipd) meta-analysis: a review of the methodology. Res Synth Methods. 2015; 6(4):293–309.
Tierney JF, Vale C, Riley R, Smith CT, Stewart L, Clarke M, Rovers M. Individual Participant Data (IPD) Meta-analyses of Randomised Controlled Trials: Guidance on Their Use. PLoS Med. 2015; 12(7):e1001855. https://doi.org/10.1371/journal.pmed.1001855 .
Stewart LA, Clarke M, Rovers M, Riley RD, Simmonds M, Stewart G, Tierney JF. Preferred reporting items for a systematic review and meta-analysis of individual participant data: the prisma-ipd statement. JAMA. 2015; 313(16):1657–65.
StataCorp. Stata Statistical Software: Release 16. College Station: StataCorp LLC; 2019.
Cooper NJ, Sutton AJ, Abrams KR, Turner D, Wailoo A. Comprehensive decision analytical modelling in economic evaluation: a bayesian approach. Health Econ. 2004; 13(3):203–26.
Download references
Acknowledgements
We would like to acknowledge Professor Denise Kendrick as the lead on the NIHR Keeping Children Safe at Home Programme that originally funded the collection of the evidence for the motivating example and some of the analyses illustrated in the paper.
ES is funded by a National Institute for Health Research (NIHR), Doctoral Research Fellow for this research project. This paper presents independent research funded by the National Institute for Health Research (NIHR). The views expressed are those of the authors and not necessarily those of the NHS, the NIHR or the Department of Health and Social Care. The funding bodies played no role in the design of the study and collection, analysis, and interpretation of data and in writing the manuscript.
Author information
Authors and affiliations.
Department of Health Sciences, University of Leicester, Lancaster Road, Leicester, UK
Ellesha A. Smith, Nicola J. Cooper, Alex J. Sutton, Keith R. Abrams & Stephanie J. Hubbard
You can also search for this author in PubMed Google Scholar
Contributions
ES performed the review, analysed the data and wrote the paper. SH supervised the project. SH, KA, NC and AS provided substantial feedback on the manuscript. All authors have read and approved the manuscript.
Corresponding author
Correspondence to Ellesha A. Smith .
Ethics declarations
Ethics approval and consent to participate.
Not applicable.
Consent for publication
Competing interests.
KA is supported by Health Data Research (HDR) UK, the UK National Institute for Health Research (NIHR) Applied Research Collaboration East Midlands (ARC EM), and as a NIHR Senior Investigator Emeritus (NF-SI-0512-10159). The views expressed are those of the author(s) and not necessarily those of the NIHR or the Department of Health and Social Care. KA has served as a paid consultant, providing unrelated methodological advice, to; Abbvie, Amaris, Allergan, Astellas, AstraZeneca, Boehringer Ingelheim, Bristol-Meyers Squibb, Creativ-Ceutical, GSK, ICON/Oxford Outcomes, Ipsen, Janssen, Eli Lilly, Merck, NICE, Novartis, NovoNordisk, Pfizer, PRMA, Roche and Takeda, and has received research funding from Association of the British Pharmaceutical Industry (ABPI), European Federation of Pharmaceutical Industries & Associations (EFPIA), Pfizer, Sanofi and Swiss Precision Diagnostics. He is a Partner and Director of Visible Analytics Limited, a healthcare consultancy company.
Additional information
Publisher’s note.
Springer Nature remains neutral with regard to jurisdictional claims in published maps and institutional affiliations.
Supplementary Information
Additional file 1.
Key for the Nice public health guideline codes. Available in NICEGuidelinesKey.xlsx .
Additional file 2
NICE public health intervention guideline review flowchart for the inclusion and exclusion of documents. Available in Flowchart.JPG .
Rights and permissions
Open Access This article is licensed under a Creative Commons Attribution 4.0 International License, which permits use, sharing, adaptation, distribution and reproduction in any medium or format, as long as you give appropriate credit to the original author(s) and the source, provide a link to the Creative Commons licence, and indicate if changes were made. The images or other third party material in this article are included in the article’s Creative Commons licence, unless indicated otherwise in a credit line to the material. If material is not included in the article’s Creative Commons licence and your intended use is not permitted by statutory regulation or exceeds the permitted use, you will need to obtain permission directly from the copyright holder. To view a copy of this licence, visit http://creativecommons.org/licenses/by/4.0/ . The Creative Commons Public Domain Dedication waiver ( http://creativecommons.org/publicdomain/zero/1.0/ ) applies to the data made available in this article, unless otherwise stated in a credit line to the data.
Reprints and permissions
About this article
Cite this article.
Smith, E.A., Cooper, N.J., Sutton, A.J. et al. A review of the quantitative effectiveness evidence synthesis methods used in public health intervention guidelines. BMC Public Health 21 , 278 (2021). https://doi.org/10.1186/s12889-021-10162-8
Download citation
Received : 22 September 2020
Accepted : 04 January 2021
Published : 03 February 2021
DOI : https://doi.org/10.1186/s12889-021-10162-8
Share this article
Anyone you share the following link with will be able to read this content:
Sorry, a shareable link is not currently available for this article.
Provided by the Springer Nature SharedIt content-sharing initiative
- Meta-analysis
- Systematic review
- Public health
- Decision making
- Evidence synthesis
BMC Public Health
ISSN: 1471-2458
- Submission enquiries: [email protected]
- General enquiries: [email protected]
- Open access
- Published: 25 April 2018
Quantitative study of medicinal plants used by the communities residing in Koh-e-Safaid Range, northern Pakistani-Afghan borders
- Wahid Hussain 1 ,
- Lal Badshah 1 ,
- Manzoor Ullah 2 ,
- Maroof Ali 3 ,
- Asghar Ali 4 &
- Farrukh Hussain 5
Journal of Ethnobiology and Ethnomedicine volume 14 , Article number: 30 ( 2018 ) Cite this article
58k Accesses
38 Citations
1 Altmetric
Metrics details
The residents of remote areas mostly depend on folk knowledge of medicinal plants to cure different ailments. The present study was carried out to document and analyze traditional use regarding the medicinal plants among communities residing in Koh-e-Safaid Range northern Pakistani-Afghan border.
A purposive sampling method was used for the selection of informants, and information regarding the ethnomedicinal use of plants was collected through semi-structured interviews. The collected data was analyzed through quantitative indices viz. relative frequency citation, use value, and family use value. The conservation status of medicinal plants was enumerated with the help of International Union for Conservation of Nature Red List Categories and Criteria (2001). Plant samples were deposited at the Herbarium of Botany Department, University of Peshawar for future reference.
One hundred eight informants including 72 male and 36 female were interviewed. The informants provided information about 92 plants species used in the treatment of 53 ailments. The informant reported maximum number of species used for the treatment of diabetes (16 species), followed by carminatives (12 species), laxatives (11 species), antiseptics (11 species), for cough (10 species), to treat hepatitis (9 species), for curing diarrhea (7 species), and to cure ulcers (7 species), etc. Decoction (37 species, i.e., 40%) was the common method of recipe preparation. Most familiar medicinal plants were Withania coagulans , Caralluma tuberculata , and Artemisia absinthium with relative frequency (0.96), (0.90), and (0.86), respectively. The relative importance of Withania coagulans was highest (1.63) followed by Artemisia absinthium (1.34), Caralluma tuberculata (1.20), Cassia fistula (1.10), Thymus linearis (1.06), etc. This study allows identification of novel uses of plants. Abies pindrow , Artemisia scoparia , Nannorrhops ritchiana , Salvia reflexa , and Vincetoxicum cardiostephanum have not been reported previously for their medicinal importance. The study also highlights many medicinal plants used to treat chronic metabolic conditions in patients with diabetes.
Conclusions
The folk knowledge of medicinal plants species of Koh-e-Safaid Range was unexplored. We, for the first time, conducted this quantitative study in the area to document medicinal plants uses, to preserve traditional knowledge, and also to motivate the local residents against the vanishing wealth of traditional knowledge of medicinal flora. The vast use of medicinal plants reported shows the significance of traditional herbal preparations among tribal people of the area for their health care. Knowledge about the medicinal use of plants is rapidly disappearing in the area as a new generation is unwilling to take interest in medicinal plant use, and the knowledgeable persons keep their knowledge a secret. Thus, the indigenous use of plants needs conservational strategies and further investigation for better utilization of natural resources.
The residents of remote areas mostly depend on folk knowledge of medicinal plants to cure different ailments. Plants not only provide food, shelter, fodder, drugs, timber, and fuel wood, but also provide different other services such as regulating different air gases, water recycling, and control of different soil erosion. Hence, phytodiversity is required to fulfill several human daily livelihood needs. Millions of people in developing countries commonly derive their income from different wild plant products [ 1 ]. Ethnomedicinal plants have been extensively applied in traditional medicine systems to treat various ailments [ 2 ]. This relationship goes back to the Neanderthal man who used plants as a healing agent. In spite of their ancient nature, international community has recognized that many indigenous communities depend on biological resources including medicinal plants [ 3 ]. About 80% of the populations in developing countries rely on medicinal plants to treat diseases, maintaining and improving the lives of their generation [ 4 , 5 ]. The people, in most parts of the world particularly in rural areas, rely on traditional medicinal plants’ remedies due to easy availability, cultural acceptability, and poor economic conditions. Out of the total 422,000 known angiosperms, more than 50,000 are used for medicinal purposes [ 6 ]. Some 75% of the herbal drugs have been developed through research on traditional medicinal plants, and 25% of prescribed drugs belong to higher plants [ 7 ]. Traditional knowledge has a long historical cultural heritage and rich natural resources that have accumulated in the indigenous communities through oral and discipleship practices [ 8 ]. Traditional indigenous knowledge is important in the formulation of herbal remedies and isolates bioactive constituents which are a precursor for semisynthetic drugs. It is the most successful criterion for the development of novelties in drugs [ 9 , 10 , 11 ]. Traditional knowledge can also contribute to conserve and sustain the use of biological diversity. However, traditional knowledge, especially herbal health care system, has declined in remote communities and in younger generations as a result of a shift in attitude and ongoing socio-economic changes [ 12 ]. The human communities are facing health and socio-economic problems due to changing environmental conditions and socio-economic status [ 13 ]. The tribal people have rich unwritten traditional medicinal knowledge. It rests with elders and transfers to younger orally. With rapid economic development and oral transmitted nature of traditional knowledge, there is an urgent need to systematically document traditional medicinal knowledge from these communities confined in rural and tribal areas of the world including Pakistan. The Koh-e-Safaid Range is one of the remote tribal areas of Pakistan having unique and century-old ethnic characteristics. A single hospital with limited insufficient health facilities is out of reach for most inhabitants. Nature has gifted the area with rich diversity of medicinal plants. The current advancement in the use of synthetic medicines has severely affected the indigenous health care system through the use of medicinal traditional practices in the area. The young generation has lost interest in using medicinal plants, and they are reluctant to practice traditional health care system that is one of the causes of the decline in traditional knowledge system. Quantitative approaches can explain and analyze the variables quantitatively. In such approach, authentic information can be used for conservation and development of existing resources. Therefore, the present research was conducted in the area to document medicinal uses of local plants with their relative importance, to record information for future investigation and discovery of novelty in drug use, and to educate the locals about the declining wealth of traditional and medicinal flora from the area.
Ethnographic and socio-economic background of the study area
Koh-e-Safaid Range is a tribal territory banding Pakistan with Afghanistan in Kurram Agency. It lies between 33° 20′ to 34° 10′ N latitudes and 69° 50′ to 70° 50′ E longitudes (Fig. 1 ).This area is federally administered by the Government of Pakistan. The Agency is surrounded on the east by Orakzai and Khyber agencies, in the southeast by Hangu district, and in the south by North Waziristan Agency and Nangarhar and Pukthia of Afghanistan lies on its west. The highest range of Koh-e-Safaid is Sikaram peak with, 4728 m height. The Agency is well-populated with many small fortified villages receiving irrigation water from Kurram River that flows through it. The weather of the Agency is mostly pleasant in summer; however, in winters, freezing temperature is experienced, and sometimes falls to − 10 °C. The weather charts website “Climate-Charts” ranked it as the fourth coldest location in Pakistan. Autumn and winter are usually dry seasons while summer and spring receive much of the precipitation. The total population of the Agency according to the 2017 censuses report is 253,478. Turi, Bangash, Sayed, Maqbal, Mangel, Khushi, Hazara, Kharote, and Jaji are the major tribes in the research area. The joint family system is practiced in the area. Most of the marriages are held within the tribe; however, there is no ban on the marriages outside the tribe. Marriage functions are communal whereby all relatives, friends, and village people participate with songs, music, and dances male and female separately. The death and funeral ceremonies are jointly attended by the friends and relatives. The people of the area follow Jirga to resolve their social and administrative problems. This is one of the most active and strong social institutions in the area. Economically, most people in the area are poor and earning their livelihoods by menial jobs. The professional includes farmers, pastoralists, shopkeepers, horticulturists, local health healers, wood sellers, and government servants. In the adjoining areas of the city, pastorals keep domestic animals and are considered a better source of income.
Map of the study area and area location in Pakistan
Sampling method
The study was conducted through purposive sampling by informants’ selection method. The selection of informants was primary based on the ethnomedicinal plants and their willingness to share the information. The selection criteria include people who prescribe recipes for treatment; people involved in buying, collection, or cultivation of plants; elder members of above 60 years age; and young literate members. The participants were traditional healers, plant collectors, farmers, traders, and selected knowledgeable elders above 60 years age and young ones. The interviews were conducted in local Pashto language in the local dialect. The informants were involved in the gathering of data with a consent of village tribe chieftains called Maliks.
Data collection
Semi-structured open-ended interviews were conducted for the collection of ethnomedicinal information from April 2015 to August 2017. Informants from 19 localities were interviewed including Sultan, Malikhail, Daal, Mali kali, Alam Sher, Kirman, Zeran, Malana, Luqman Khail, Shalozan, Pewar, Teri Mangal, Bughdi, Burki, Kharlachi, Shingak, Nastikot, Karakhila, and Parachinar city (Fig. 1 ). The objectives of this study were thoroughly explained to all the informants before the interview [ 14 ]. Data about medicinal plants and informants including local names of plants, preparation of recipes, storage of plant parts, informant age, occupation, and education were collected during face-to-face interviews. A questionnaire was set with the following information: informant bio-data, medicinal plant use, plant parts used and modes of preparation, and administration of the remedies. Plants were confirmed through repeated group discussion with informants [ 15 , 16 ]. For the identification of plants, informants were requested for transect walks in the field to locate the cited plant for confirmation.
Collection and identification of medicinal plants
The medicinal plants used in traditional treatment of ailments in the study area were collected with the help local knowledgeable persons, traditional healers, and botanists. The plants were pressed, dried, and mounted on herbarium sheet. The field identification was confirmed by a taxonomist in the Herbarium Department of Botany, University of Peshawar. The voucher specimens of all species were numbered and deposited in the Herbarium of Peshawar University (Fig. 2 ).
Landscape of Kurram Valley ( a winter, b summer). c , d Traditional healers selling herbal drugs on footpath. e Trader crushing Artemisia absinthium for marketing. f Principal author in the field during data collection. g , h Plant collectors in subalpine zone. i Lilium polyphyllum rare species distributed in subalpine zone. j Ziziphora tenuior endangered species of subtropical zone
Data analysis
The information about ethnomedicinal uses of plants and informants included in questionnaires such as botanical name, local name, family name, parts used, mode of preparation, use reports, frequency of citation, relative importance, and voucher number were tabulated for all reported plant species. Informants’ use reports for various ailments and frequency of citation were calculated for each species. The relative importance of species was calculated according to use-value formula (UV = UVi/Ni) [ 17 ], where “UVi” is the number of citations for species across all informants and “Ni” the number of informants. The citation probability of each medicinal plant across all informants was equal to avoid researchers’ biasness. Family use value was calculated using the formula FUV = UVs/Ns, where “UVs” represent the sum of use values of species falling within family, and Ns represents the number of species reported for the family. The conservation status of wild medicinal plants species was enumerated by applying International Union for Conservation of Nature (IUCN) criterion (2001) [ 18 ].
Informants’ knowledge about medicinal plants and their demography
A total of 108 including 72 male and 36 female informants were interviewed from 19 locations. The three groups of male respondents were falling in the age groups of 21 to 40, 41 to 60, and 61 to 80 years having the numbers of 19, 19, and 34, respectively. Among the female respondents, 10 aged 21 to 40, 14 aged 41 to 60, and 12 aged 61 to 80 years. Among the informants, 15 males were illiterate, 34 were matriculate, 13 were intermediate, and 10 were graduates. Among the females, 19 were illiterate, 16 were matriculate, and only 1 was graduate (Table 1 ). Informants were shepherd, healers, plant collectors, gardeners, and farmers. Twenty-eight informants of above 60 years age, living a retired life, were also interviewed. It was found that males were more knowledgeable than females. Furthermore, health healers were more knowledgeable.
Diversity of medicinal plants
A total of 92 medicinal species including 91vascular plant species belonging to 50 families and 1 mushroom Morchella of Ascomycetes of family Morchellaceae were reported (Table 2 ) . Asteraceae had eight species followed by seven species of Lamiaceae and Rosaceae. Three species were contributed by each of Moraceae, Asclepiadaceae, Polygonaceae, Brassicaceae, Solanaceae, Cucurbitaceae, and Liliaceae. Of the remaining eight families, namely, Poaceae, Pinaceae, Zingiberaceae, Chenopodiaceae, Plantaginaceae, Apiaceae, Fabaceae, and Zygophyllaceae, each one contributed two species [ 19 , 20 ]. Asteraceae, Lamiaceae, and Rosaceae were also reported with a high number of plants used for medicinal purposes. The reported plants were collected both from the wild (86.9%) and cultivated (13.1%) sources. However, greater percentage of medicinal plants from wild sources indicated higher species’ diversity in the study area. The 62 herbs species, 16 tree, 12 shrubs, and 2 undershrubs species were used in medicinal preparation for remedies.
Plant parts used in preparation of remedies
The plant parts used in the preparation of remedies were root, rhizome, bulbils, stem, branches, leaves, flowers, fruits, seeds, bark, resin, and latex. The relative use of these plant parts is shown in (Fig. 3 ). Fruits were frequently used plant part (26 species), followed by leaves (23 species) and remaining parts (21 species).
Plant parts used in the formulation of remedies
Preparation and mode of administration of remedies
The collection of data for the preparation of remedies from medicinal plants is extremely important. Such information is essential for identification of active ingredients and intake of relevant amount of drug. The present research observed seven methods for preparing recipes. It included decoction, powder, juice, infusions, roast, and ash methods (Fig. 4 ). The 37 species (40%) were most frequently used for the preparation of remedies. A plant part is boiled while infusion is obtained by soaking plant material in cold or hot water overnight. Eleven species (14%) are in powdered form, 11species (14%) in vegetable form, 7 species (9%) in juice form, 7 species (9%) in infusions form, 3 species (4%) in roasted form, and 1 species (2%) in ash form were used. Twenty-seven plant parts were used directly. It included wild fruits that were consumed for their nutritional and medicinal purpose. The most frequently used mode of administration of remedies was oral intake practice of 74 species (79%) followed by both orally and topically practice of 11 species (12%) and topically of 8 species (9%) (Fig. 5 ).
Different modes of drug formulation
Route of administration of drugs
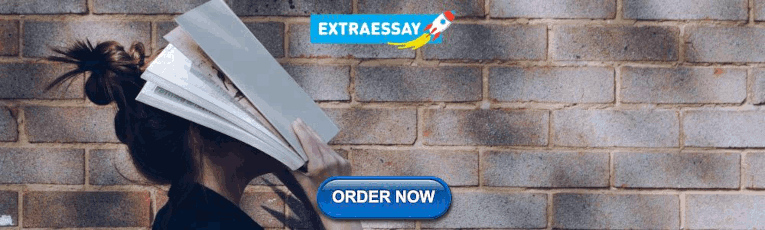
Medicinal plants use categories
The inhabitants used medicinal plants in the treatment of 53 health disorders. The important disorders were cancer, diabetic, diarrhea, dysentery, hepatitis, malaria, and ulcer (Table. 3 ). These disorders were classified into 17 categories. Among the ailments, most plants were used for the treatment of digestive problems mainly as carminative (12 species), diarrhea (11 species), laxative (11 species), ulcer (7 species), appetizer (5 species), colic pain (4 species), and anthelmintic (4 species). Such higher use of plants for the treatment of digestive problems had been reported in ethnobotanical studies conducted in another tribal area of Pakistan [ 21 ]. The other categories (18 species) were used to treat respiratory disorders, followed by endocrine disorders (16 species); antiseptic and anti-inflammatory (15 species); circulatory system disorders (15 species); integumentary problems (15 species); antipyretic, refrigerant, and analgesic (9 species); and hepatic disorders (9 species). However, among the ailments, the highest number of plants were used in the treatment of diabetes (16 species), followed by antiseptic (11 species), cough (10 species), hepatitis (9 species), and ulcer (7 species). Among the remaining species, the informants reported three and two species used against malaria and cancer, respectively (Table 3 ).
Quantitative appraisal of ethnomedicinal use
Based on the quantitative indices, the analyzed data showed that few plants were cited by the majority of the informants for their medicinal value. Seventeen plant species with the highest citation frequency are shown in (Fig. 6 ). The highest citation frequency was calculated for Withania coagulans (0.96), followed by Caralluma tuberculata (0.90), and Artemisia absanthium (0.86). The high values of these species indicated that most of the informants were familiar with their medicinal value. However, the familiarity of these three plants could be linked to their collection for economic purposes [ 22 ]. Withania coagulans (1.63), Artemisia absinthium (1.34), Caralluma tuberculata (1.20), Cassia fistula (1.10), and Thymus linearis (1.06) were reported having the highest used values for medicinal purposes (Fig. 7 ). All these species were used for the cure of three or more diseases. The powdered fruit of Withania coagulans is used for the cure of stomach pain, constipation, diabetes, and ulcer. The next highest use value was calculated for Artemisia absinthium with five medical indications as diabetes, malaria, fever, blood pressure, and urologic problems. Among the remaining three plants, Caralluma tuberculata is used for diabetes, cancer, and stomachic problems, and as blood purifier; Cassia fistula for colic pain and stomach pain and as a carminative agent; and Thymus linearis for cough and as carminative and appetizer. Lowest use value was calculated for Rununculus muricatus (0.04) with next three species having same lowest use value: Abies pindrow (0.05), Lepidium virginicum (0.05), and Oxalis corniculata (0.05). Highest family use value was calculated for Juglandaceae (0.86), followed by Cannabaceae (0.78), Apiaceae (0.75), Asclepiadaceae (0.71), Fumariaceae (0.71), Berberidaceae (0.70), Fabaceae (0.67), Punicaceae (0.65), Solanaceae (0.64), and Asteraceae (0.61). This is the first study that presents a quantitative value of medicinal plants used in the investigated area.
Medicinal plants with highest relative frequency citation
Medicinal plants with highest relative importance
Conservation status of the medicinal flora
Plant preservation means the study of plant declination, their causes, and techniques to protect rare and scarce plants. Plant conservation is a fairly new field that emphasizes the conservation of biodiversity and whole ecosystems as opposed to the conservation of individual species [ 23 ]. The ex situ conservation must be encouraged for the protection of medicinal plants [ 24 ]. In the present case, the area under study is under tremendous anthropogenic pressure as well. Therefore, ex situ conservation of endangered species is recommended. The woody plants, cut down for miscellaneous purposes, are facing conservational problems. Sayer et al. [ 25 ] reported that large investments are being made in the establishment of tree plantation on degraded area in Asia [ 25 ]. Alam and Ali stressed that proper conservation studies are almost negligible in Pakistan [ 26 ]. Same is the case with the study area as no project has been initiated for the conservation of forest or vegetation so far. Anthropogenic activities, small size population, distribution in limited area, and specificity of habitat were observed as the chief threats to endangered species.
According to IUCN Red List Criteria (2001) [ 18 ] conservation status of 80 wild medicinal species have been assessed based on availability, collection status, growth status, and their parts used. The remaining 12 medicinal plants were cultivated species. Of these, 7 (8.7%) species are endangered, 34 (42.5%) species are vulnerable, 29 (36.2%) species are rare, 9 (11.2%) species are infrequent, and only 1 (1.3%) species is dominant. The endangered species were Caralluma tuberculata , Morchella esculenta , Rheum speciforme , Tanacetum artemisioides , Vincetoxicum cardiostephanum , Withania coagulans , and Polygonatum verticillatum.
Traditional medicines are a vital and often underestimated part of health care. Nowadays, it is practiced in almost every country of the world. Its demand is currently increasing rapidly in the form of alternative medicine [ 20 ]. Ethnomedicinal plants have been widely applied in traditional medicine systems to treat various ailments. About 80% of the populations in developing countries rely on medicinal plants to treat diseases, maintaining and improving the lives of their generation [ 19 ]. Traditional knowledge has a long historical cultural heritage and rich natural resources that has accumulated in the indigenous communities through oral and discipleship practices [ 8 ]. Traditional indigenous knowledge is important in the formulation of herbal remedies and isolates bioactive constituents which are a precursor for semisynthetic drugs. It is the most successful criterion for the development of novelties in drugs [ 11 ]. A total of 92 medicinal species including 91 vascular plant species belonging to 50 families and 1 mushroom Morchella of Ascomycetes of family Morchellaceae were reported (Table 2 ) . The current study reveals that the family Asteraceae represents eight species followed by seven species of Lamiaceae and Rosaceae each which showed a higher number of medicinal plants. Three species were contributed by each of Moraceae, Asclepiadaceae, Polygonaceae, Brassicaceae, Solanaceae, Cucurbitaceae, and Amaryllidaceae. While the remaining eight families, namely, Poaceae, Pinaceae, Zingiberaceae, Chenopodiaceae, Plantaginaceae, Apiaceae, Fabaceae, and Zygophylaceae, contributed two species each. Asteraceae, Lamiaceae, and Rosaceae were also reported with a high number of plants used for medicinal purposes. Indigenous use of medicinal plants in the communities residing in Koh-e-Safid Range of Pakistan is evident. Traditional health healers are important to fulfill the basic health needs of the economically poor people of the area. The high dependency on traditional healers is due to limited and inaccessible health facilities. Most people either take recipes from local healers or select wild medicinal plants prescribed by them. Some elders also knew how to preserve medicinal plant parts for future use. Traditional knowledge of medicinal plants is declining in the area due to lack of interest in the young generation to acquire this traditional treasure. Furthermore, most traditional health healers and knowledgeable elders hesitate to disseminate their recipes. Therefore, traditional knowledge in the area is diminishing as aged persons are passing away. Vernacular names of plants are the roots of ethnomedicinal diversity knowledge [ 27 ]. They can clear the ambiguity in the identification of medicinal plants within an area. It also helps in the preservation of indigenous knowledge of medicinal plants. The medicinal plants were mostly reported with one specific vernacular name in the investigated area. While Rosa moschata and Rosa webbiana were known by same single vernacular name as Jangle Gulab. Few species were known by two vernacular names: Curcuma longa as Korkaman or Hildi, Ficus carica as Togh or Anzer, Fumaria indica as Chamtara or Chaptara, Marrubium vulgare as Dorshol or Butaka, Solanum nigrum as Bartang or Kharsobay, Teucrium stocksianum as Harboty or Gulbahar, and Thymus linearis as Paney or Mawory. The informants also mentioned different vernacular names for species even belonging to single genus; Plantago lanceolata as Chamchapan or Ghuyezaba and Plantago major as Ghazaki or Palisepary. Majority of the species commonly had a single name. However, local dialects varied in few species, i. e., Withania coagulans was known by three names: Hapyanaga, Hafyanga, and Shapynga, Caralluma tuberculata as Pamenny or Pawanky, Foeniculum vulgare as Koglany or Khoglany, and Viola canescens was called as Banafsha or Balamsha. The species with high use value need conservation for maintaining biodiversity in the study area. However, in the present case, no project or programs for the conservation of forest or vegetation are operating. Grazing and unsustainable medicinal uses were observed as the chief hazard to highly medicinal plant species. The higher use of herbs can be attributed to their abundance, diversity, and therapeutic potentials as antidiabetic, antimalarial, antipyretic, antiulcerogenic, antipyretic, blood purifier, and emollient and for blood pressure, hepatitis, stomach pain, and itching. Aloe vera , cultivated for ornamental purpose, is used as wound healing agent. Among the plant parts, the higher use of fruit may relate to its nutritional value. The aerial parts of the herbaceous plants were mostly collected in abundance and frequently used for medicinal purposes. In many recipes, more than one part was used. The utilization of roots, rhizomes, and the whole plant is the main threat in the regeneration of the medicinal plants [ 28 ]. In the current study, decoction was found to be the main method of remedy preparation as reported in the ethnopharmacological studies from other parts [ 29 , 30 , 31 ]. Fortunately, we collected important information like preparation of remedies and their mode of administration for all the reported plants. However, the therapeutic potential of few plants are connected to their utilization method. A roasted bulb of Allium cepa is wrapped on the spine-containing wound to release the spine. The leaf of Aloe vera containing viscous juice is scratched and wrapped on a wound. The latex of Calotropis procera is first mixed with flour and then topically applied on the skin for wound healing. Infusion of Cassia fistula fruit’s inner septa is prepared for stomach pain and carminative and colic pain in children. The fruit of Citrullus colocynthis boiled in water is orally taken for the treatment of diabetes. Grains of Hordeum vulgare are kept in water for a day, and its extraction is taken for the treatment of diabetes. The decoction of Seriphidium kurramensis shoots are used as anti-anthelmintic and antimalaria. The leaves of Juglans regia are locally used for cleaning the teeth and to prevent them from decaying. Furthermore, its fruit is used as brain tonic, and its roasted form is useful in the treatment of dysentery. The roots of Pinus wallichiana are cut into small pieces and put into the pot. The cut pieces are boiled, and the extracted liquid is poured into the container. One drop of the extracted liquid is mixed with one glass of milk and taken orally once a day as blood purifier. An infusion of Thymus linearis aerial parts is prepared like hot tea and is drunk for cough and as appetizer and carminative. A decoction of Zingiber officinale rhizome is drunk at night time for relief of cough. Medicinal plants are still practiced in tribal and rural areas as they are considered as main therapeutic agents in maintaining better health. Such practices have been described in the ethnobotanical studies conducted across Pakistan. The current study reveals several plant species with more than one medical use including Artemisia absanthium , Cichorium intybus , Fumaria indica , Punica granatum , Tanacetum artemisioides , Teucrium stocksianum , and Withania coagulans . Their medicinal importance can be validated from indigenous studies conducted in various parts of the country. Amaranthus viridis leaf extract is an emollient and is used for curing cough and asthma as well [ 32 ]. Artemisia absanthium is used for the treatment of malaria and diabetes [ 33 , 34 , 35 , 36 ]. Cichorium intybus is used against diabetes, malaria, and gastric ulcer, and it is also used as digestive and laxative agent [ 28 , 37 , 38 , 39 , 40 , 41 ]. Leaves of Cannabis sativa are used as bandage for wound healing; powdered leaves as anodyne, sedative, tonic, and narcotic; and juice added with milk and nuts as a cold drink [ 42 ]. Whole plant of Fumaria indica [ 36 ] and Tanacetum artemisioides [ 43 ] is used for treating constipation and diabetes, respectively. Dried rind powder and fruit extract of Punica granatum are taken orally for the treatment of anemia, diarrhea, dysentery, and diabetes [ 44 , 45 , 46 , 47 ]. A decoction of aerial parts of Teucrium stocksianum is used for curing diabetes [ 29 , 48 ]. Withania coagulans is known worldwide [ 38 , 49 ] as a medicinal plant, whose fruit decoction is best remedy for skin diseases and diabetes. Its seeds are used against digestive problems, gastritis, diabetes, and constipation [ 21 , 28 , 50 ]. Our results are in line with the traditional uses of plants in the neighboring counties [ 8 ]. For example, Fumaria indica is used as blood purifier, and Hordeum vulgare grains decoction for diabetes; Juglans regia bark for toothaches and scouring teeth; Mangifera indica seed decoction for diarrhea; Solanum nigrum extract for jaundice; and Solanum surattense fruit decoction for cough have been documented in the study (40) . Such agreements strengthen our results and provide good opportunity to evaluate therapeutic potential of the reported plants. Three plants species Adiantum capillus-veneris , Malva parviflora , and Peganum harmala have been documented for their medicinal use in the ethnobotanical study [ 51 ]. According to this, the decoction of the aerial parts of Adiantum capillus-veneris is used for the treatment of asthma and dyspnea. Malva parviflora root and flower are used for stomach ulcers. Peganum harmala fruit powder and decoction are used for toothache, gynecological infections, and menstruation. The dried leaves of Artemisia absanthium is used to cure stomach pain and intestinal worm while an inflorescence paste prepared from its fresh leaves is used as wound healing agent and antidiabetic [ 52 , 53 ]. The bulb of Allium sativum is used in rheumatism while its seed vessel mixed with hot milk is useful for the prevention of tuberculosis and high blood pressure. The fruit bark of Punica granatum is used in herbal mixture for intestinal problems [ 54 ]. Avena sativa decoction is used for skin diseases including eczema, wounds, irritation, inflammation, erythema, burns, itching, and sunburn [ 55 ]. Foeniculum vulgare and Lepidium sativum are used for the treatment of diabetes and renal diseases [ 53 ]. Verbascum thapsus leaves and flowers can be used to reduce mucous formation and stimulate the coughing up of phlegm. Externally, it is used as a good emollient and wound healer. Leaves of Thymus linearis are effective against whooping cough, asthma, and round worms and are an antiseptic agent [ 21 ]. Berberis lycium wood decoction with sugar is the best treatment for jaundice. Chenopodium album has anthelmintic, diuretic, and laxative properties, and its root decoction is effective against jaundice. The whole plant decoction of Fumaria indica is used for blood purification. Dried leaves and flowers of Mentha longifolia are used as a remedy for jaundice, fever, asthma, and high blood pressure [ 36 ]. Morus alba fruit is used to treat constipation and cough [ 42 ]. Oxalis corniculata roots are anthelmintic, and powder of Chenopodium album is used for headache and seminal weakness [ 47 ]. Boiled leaves of Cichorium intybus are used for stomachic pain and laxative while boiled leaves of Plantago major are used against gastralgia [ 56 ]. Viola canescens flower is used as a purgative [ 32 ]. The above ethnomedicinal information confirms the therapeutic importance of the reported plants. The reported plant species show biological activities which suggest their therapeutic uses. The aqueous extract of Allium sativum has been studied for its lipid lowering ability and was found to be effective at the amount of 200 mg/kg of body weight. It also has significant antioxidant effect and normalizes the activities of superoxide dismutase, catalase, glutathione peroxidase, and glutathione reductase in the liver [ 57 ]. An extract of Artemisia absanthium antinociception in mice has been found and was linked to cholinergic, serotonergic, dopaminergic, and opioidergic system [ 58 ]. The ethanolic extract of Artemisia absanthium at a dose of 500 and 1000 mg/kg body weight has reduced blood glucose to significant level [ 59 ]. The hepatoprotective activity of crude extract of aerial parts of Artemisia scoparia was investigated against experimentally produced hepatic damage through carbon tetrachloride. The experimental data showed that crude extract of Artemisia scoparia is hepatoprotective [ 60 ]. Ethanolic and aqueous extracts from Asparagus exhibited strong hypolipidemic and hepatoprotective action when administered at a daily dose of 200 mg/kg for 8 weeks in hyperlipidemic mice [ 61 , 62 ]. The extract of Calotropis procera was evaluated for the antiulcerogenic activity by using different in vivo ulcer in pyloric-ligated rats, and significant protection was observed in histamine-induced duodenal ulcers in guinea pigs [ 63 ]. Cannabidiol of Cannabis sativa was found as anxiolytic, antipsychotic, and schizophrenic agent [ 64 ]. Caralluma tuberculata methanolic extract of aerial parts (500 mg/kg) in fasting blood glucose level in hyperglycemic condition decreased up to 54% at fourth week with concomitant increase in plasma insulin by 206.8% [ 65 ]. The aqueous and methanol crude extract of Celtis australis , traditionally used in Indian system of medicine, was screened for its antibacterial activity [ 66 ]. Cichorium intybus L. whole plant 80% ethanolic extract a percent change in serum glucose has been observed after 30 min in rats administrated with vehicle, 125, 250, and 500 mg notified as 52.1, 25.2, 39, and 30.9%, respectively [ 67 ]. Citrullus colocynthis fruit, pulp, leaves, and root have significantly decreased blood glucose level and restored beta cells [ 30 , 68 , 69 , 70 ]. The two new aromatic esters horizontoates A and B and one new sphingolipid C were isolated from Cotoneaster horizontalis . The compounds A and B showed significant inhibitory effects on acetylcholinesterase and butylcholinesterase in a dose-dependent manner [ 71 ]. The alkaloids found in Datura stramonium are organic esters used clinically as anticholinergic agents [ 72 ]. The methanolic extract of Momordica charantia fruits on gastric and duodenal ulcers was evaluated in pylorus-ligated rats; the extract showed significant decrease in ulcer index [ 73 ]. Antifungal activity of Nannorrhops ritchiana was investigated against fungal strains Aspergillus flavus , Trichophyton longifusis , Trichophyton mentagrophytes , Aspergillus flavus , and Microsporum canis were found susceptible to the extracts with percentage inhibition of 70–80% [ 74 ]. The inhibitory effects of Olea ferruginea crude leaf extract on bacterial and fungal pathogens have been evaluated [ 75 ]. The aqueous extract of Plantago lanceolata showed that higher doses provide an overall better protection against gastro-duodenal ulcers [ 76 ]. The oral and intraperitoneal management of extracts reduced the gastric acidity in pylorus-ligated mice [ 77 ]. The antiulcer effect of Solanum nigrum fruit extract on cold restraint stress, indomethacin, pyloric ligation, and ethanol-induced gastric ulcer models and ulcer healing activity on acetic acid-induced ulcer model in rats [ 78 , 79 ]. The antifungal activity (17.62 mm) of Viola canescens acetone extract 1000 mg/ml against Fusarium oxysporum has been observed [ 80 ]. Leaf methanolic extract of Xanthium strumarium has inhibited eight pathogenic bacteria at a concentration of 50 and 100 mg/ml [ 81 ]. Aqueous extract of the fruits of Withania coagulans in streptozotocin-induced rats at dose of 1 g/kg for 7 days has shown significant decrease ( p < 0.01) in the blood glucose level (52%), triglyceride, total cholesterol, and low density lipoprotein and very significant increase ( p < 0.01) in high density lipoprotein level [ 31 ]. This shows that further investigation on the reported ethnomedicinal plants can lead to the discovery of novel agents with therapeutic properties.
In the current study, conservation status of 80 medicinal species was reported which was growing wild in the area. The information was collected and recorded for different conservation attributes by following International Union for Conservation and Nature (2001) [ 18 ]. It was reported that seven species (8.7%) were endangered due to the much collection, anthropogenic activities, adverse climatic conditions, small size population and distribution in limited area, specificity of habitat, and over grazing in the research area. However, the below-mentioned species were found to be endangered: Caralluma tuberculata , Morchella esculenta , Rheum speciforme , Tanacetum artemisioides , Vincetoxicum cardiostephanum , Withania coagulans , and Polygonatum verticillatum . Unsustainable use and lack of suitable habitat have affected their regeneration and pushed them to endangered category. Traditional knowledge can also contribute to conservation and sustainable use of biological diversity [ 19 , 20 ].
Novelty and future prospects
Ethnomedicinal literature research indicated that five plant species, Abies pindrow , Artemisia scoparia , Nannorrhops ritchiana , Salvia reflexa , and Vincetoxicum cardiostephanum , have not been reported previously for their medicinal importance from this area. The newly documented uses of these plants were Abies pindrow and Salvia reflexa (antidiabetic), Artemisia scoparia (anticancer), Nannorrhops ritchiana (laxative), and Vincetoxicum cardiostephanum (chest problems). Adiantum capillus-veneris is reported for the first time for its use in the treatment of skin problems. These plant species can be further screened for therapeutic agents and their pharmacological activities in search of novel drugs. The study also highlights 16 species of antidiabetic plants Caralluma tuberculata , Momordica charantia , Marrubium vulgare , Artemisia scoparia , Melia azedarach , Salvia reflexa , Citrullus colocynthis , Tanacetum artemisioides , Quercus baloot , Olea ferruginea , Cichorium intybus , Artemisia absinthium , Hordeum vulgare , Teucrium stocksianum , Withania coagulans , and Abies pindrow . Except sole paper from District Attack, Pakistan [ 28 ], such a high number of antidiabetic plants have not been reported previously from any part of Pakistan in the ethnobotanical studies.
Traditional knowledge about medicinal plants and preparation of plant-based remedies is still common in tribal area of Koh-e-Safaid Range. People due to closeness to medicinal plants and inaccessible health facilities still rely on indigenous traditional knowledge of plants. The role of traditional healers in the area is observable in primary health care. The locals used medicinal plants in treatment of important disorders such as cancer, diabetes, hepatitis, malaria, and ulcer. The analyzed data may provide opportunities for extraction of new bioactive constituents and to develop herbal remedies. The study also confirmed that the communities residing in the area have not struggled for conservation of this traditional treasure of indigenous knowledge and medicinal plants. Medicinal plant diversity in the remote and backward area of Koh-e-Safaid Range has great role in maintaining better health conditions of local communities. Therefore, conservation strategies should be adopted for the protection of medicinal plants and traditional knowledge in the study area to sustain them in the future.
Abbreviations
Family use value
International Union for Conservation of Nature
The number of informants
Represent the number of species reported for the family
The number of citations for a species across all informants
Represent sum of use values of species falling within family
Maroyi A. Diversity of use and local knowledge of wild and cultivated plants in the Eastern Cape province. South Africa J Ethnobiol Ethnomed. 2017;13:43.
Article PubMed Google Scholar
El-Seedi HR, Burman R, Mansour A, Turki Z, Boulos L, Gullbo J. The traditional medical uses and cytotoxic activities of sixty-one Egyptian plants: discovery of an active cardiac glycoside from Urginea maritima. J Ethnopharmacol. 2013;145:746–57.
Akerele O. Nature’s medicinal bounty: don’t throw it away. 1993.
Google Scholar
Calixto JB. Twenty-five years of research on medicinal plants in Latin America: a personal view. J Ethnopharmacol. 2005;100:131–4.
Health WHOR. Managing complications in pregnancy and childbirth: a guide for midwives and doctors: World Health Organization; 2003.
Hamilton AC. Medicinal plants, conservation and livelihoods. Biodivers Conserv. 2004;13:1477–517.
Article Google Scholar
Wang M-Y, West BJ, Jensen CJ, Nowicki D, Su C, Palu AK. Morinda citrifolia (Noni): a literature review and recent advances in noni research. Acta Pharmacol Sin. 2002;23:1127–41.
CAS PubMed Google Scholar
Ouelbani R, Bensari S, Mouas TN, Khelifi D. Ethnobotanical investigations on plants used in folk medicine in the regions of Constantine and Mila (north-east of Algeria). J Ethnopharmacol. 2016;194:196–218.
Farnsworth NR. The role of ethnopharmacology in drug development. Bioact Compd from plants. 1990;154:2–21.
CAS Google Scholar
Cox PA, Balick MJ. The ethnobotanical approach to drug discovery. Sci Am. 1994;6:82–7.
Fabricant DS, Farnsworth NR. The value of plants used in traditional medicine for drug discovery. Environ Health Perspect. 2001;109:69.
Article CAS PubMed PubMed Central Google Scholar
Kala CP. Current status of medicinal plants used by traditional Vaidyas in Uttaranchal state of India. 2005.
Abbas Z, Khan SM, Alam J, Khan SW, Abbasi AM. Medicinal plants used by inhabitants of the Shigar Valley, Baltistan region of Karakorum range-Pakistan. J Ethnobiol Ethnomed. 2017;13:53.
Article PubMed PubMed Central Google Scholar
Cunningham AB. Applied ethnobotany: people, wild plant use and conservation. London: Earthscan. Ersity and sustaining local livelihood. Annu Rev Environ Resour. 2001;30:219–52.
Martin GJ. Ethnobotany: a people and plants conservation manual. London: Chapman and Hall CrossRef Google Scholar; 1995.
Book Google Scholar
Maundu P. Methodology for collecting and sharing indigenous knowledge: a case study. Indig Knowl Dev Monit. 1995;3:3–5.
Phillips O, Gentry AH, Reynel C, Wilkin P, Galvez DB. Quantitative ethnobotany and Amazonian conservation. Conserv Biol. 1994;8:225–48.
Anonymous. IUCN Red List Categories and Criteria: version 3.1. IUCN species survival commission IUCN, Gland, witzerland and Cambridge, U.K. 2001.
Tuasha N, Petros B, Asfaw Z. Medicinal plants used by traditional healers to treat malignancies and other human ailments in Dalle District, Sidama Zone, Ethiopia. J Ethnobiol Ethnomed. 2018;14(1):15.
Aziz MA, Khan AH, Adnan M, Ullah H. Traditional uses of medicinal plants used by indigenous communities for veterinary practices at Bajaur Agency, Pakistan. J Ethnobiol Ethnomed. 2018;14(1):11.
Ullah M, Khan MU, Mahmood A, Malik RN, Hussain M, Wazir SM. An ethnobotanical survey of indigenous medicinal plants in Wana district south Waziristan agency. Pakistan J Ethnopharmacol. 2013;3:150–8.
Hussain W, Hussain J, Ali R, Khan I, Shinwari ZK, Nascimento IA. Tradable and conservation status of medicinal plants of KurramValley. Parachinar, Pakistan. 2012;2:66–70.
Soulé ME. What is conservation biology? Bioscience. 1985;35:727–34.
Heywood VH, Iriondo JM. Plant conservation: old problems, new perspectives. Biol Conserv. 2003;113(3):321–35.
Sayer J, Chokkalingam U, Poulsen J. The restoration of forest biodiversity and ecological values. For Ecol Manag. 2004;201(1):3–11.
Alam J, Ali SI. Conservation status of Androsace Russellii Y. Nasir: a critically endangered species in Gilgit District, Pakistan. Pak J Bot. 2010;42(3):1381–93.
Khasbagan S. Indigenous knowledge for plant species diversity: a case study of wild plants’ folk names used by the Mongolians in Ejina desert area, Inner Mongolia. PR China J Ethnobiol Ethnomed. 2008;4:2.
Article CAS PubMed Google Scholar
Ahmad M, Qureshi R, Arshad M, Khan MA, Zafar M. Traditional herbal remedies used for the treatment of diabetes from district Attock (Pakistan). Pak J Bot. 2009;41(6):2777–82.
Ullah R, Iqbal ZHZ, Hussain J, Khan FU, Khan N, Muhammad Z. Traditional uses of medicinal plants in Darra Adam Khel NWFP Pakistan. J Med Plants Res. 2010;4(17):1815–21.
Gurudeeban S, Ramanathan T. Antidiabetic effect of Citrullus colocynthis in alloxon-induced diabetic rats. Inven Rapid Ethno Pharmacol. 2010;1:112.
Hoda Q, Ahmad S, Akhtar M, Najmi AK, Pillai KK, Ahmad SJ. Antihyperglycaemic and antihyperlipidaemic effect of poly-constituents, in aqueous and chloroform extracts, of Withania coagulans Dunal in experimental type 2 diabetes mellitus in rats. Hum Exp Toxicol. 2010;29(8):653–8.
Shinwari MI, Khan MA. Folk use of medicinal herbs of Margalla hills national park. Islamabad J Ethnopharmacol. 2000;69(1):45–56.
Abbas G, Abbas Q, Khan SW, Hussain I, Najumal-ul-Hassan S. Medicinal plants diversity and their utilization in Gilgit region. Northern Pakistan.
Ashraf M, Hayat MQ, Jabeen S, Shaheen N, Khan MA, Yasmin G, Artemisia L. Species recognized by the local community of the northern areas of Pakistan as folk therapeutic plants. J Med Plants Res. 2010;4(2):112–9.
Murad W, Ahmad A, Ishaq G, Saleem KM, Muhammad KA, Ullah I. Ethnobotanical studies on plant resources of Hazar Nao forest, district Malakand, Pakistan. Pakistan J Weed Sci Res. 2012;18(4):509–27.
Khan SW, Khatoon S. Ethnobotanical studies on some useful herbs of Haramosh and Bugrote valleys in Gilgit, northern areas of Pakistan. Pakistan J Bot. 2008;40(1):43.
Mohammad I, Rahmatullah Q, Shinwari ZK, Muhammad A, Mirza SN. Some ethnoecological aspects of the plants of Qalagai hills, Kabal valley, swat. Pakistan Int J Agric Biol. 2013;15(5):801–10.
Jabeen N, Ajaib M, Siddiqui MF, Ulfat M, Khan B. A survey of ethnobotanically important plants of District Ghizer. Gilgit-Baltistan FUUAST J Biol. 2015;5(1):153–60.
Jan G, Khan MA, Jan F. Medicinal value of the Asteraceae of Dir Kohistan Valley, NWFP, Pakistan. Ethnobot Leafl. 2009;13:1205–15.
Ali H, Sannai J, Sher H, Rashid A. Ethnobotanical profile of some plant resources in Malam Jabba valley of Swat. Pakistan J Med Plants Res. 2011;5(18):4676–87.
Jan G, Khan MA, Farhatullah JFG, Ahmad M, Jan M, Zafar M. Ethnobotanical studies on some useful plants of Dir Kohistan valleys, KPK. Pakistan. Pak J Bot. 2011;43(4):1849–52.
Akhtar N, Rashid A, Murad W, Bergmeier E. Diversity and use of ethno-medicinal plants in the region of Swat, north Pakistan. J Ethnobiol Ethnomed. 2013;9(1):25.
Marwat SK. Ethnophytomedicines for treatment of various diseases in DI Khan district. Sarhad J Agric. 2008;24(2):305–15.
Ijaz F, Iqbal Z, Alam J, Khan SM, Afzal A, Rahman IU. Ethno medicinal study upon folk recipes against various human diseases in Sarban Hills, Abbottabad. Pakistan World J Zool. 2015;10(1):41–6.
Abbasi AM, Khan MA, Khan N, Shah MH. Ethnobotanical survey of medicinally important wild edible fruits species used by tribal communities of lesser Himalayas-Pakistan. J Ethnopharmacol. 2013;148(2):528–36.
Haq F, Ahmad H, Alam M. Traditional uses of medicinal plants of Nandiar Khuwarr catchment (district Battagram). Pakistan. J Med Plants Res. 2011;5(1):39–48.
Devi U, Seth MK, Sharma P, Rana JC. Study on ethnomedicinal plants of Kibber wildlife sanctuary: a cold desert in trans Himalaya. India J Med Plants Res. 2013;7(47):3400–19.
Alamgeer TA, Rashid M, Malik MNH, Mushtaq MN. Ethnomedicinal survey of plants of Valley Alladand Dehri, Tehsil Batkhela, District Malakand, Pakistan. Int J Basic Med Sci Pharm. 2013;3(1):23–32.
Khan B, Abdukadir A, Qureshi R, Mustafa G. Medicinal uses of plants by the inhabitants of Khunjerab National Park, Gilgit, Pakistan. Pak J Bot. 2011;43(5):2301–10.
Shah A, Marwat SK, Gohar F, Khan A, Bhatti KH, Amin M. Ethnobotanical study of medicinal plants of semi-tribal area of Makerwal & Gulla Khel (lying between Khyber Pakhtunkhwa and Punjab provinces), Pakistan. Am J Plant Sci. 2013;4(1):98.
Mosaddegh M, Naghibi F, Moazzeni H, Pirani A, Esmaeili S. Ethnobotanical survey of herbal remedies traditionally used in Kohghiluyeh va Boyer Ahmad province of Iran. J Ethnopharmacol. 2012;141(1):80–95.
Malik AH, Khuroo AA, Dar GH, Khan ZS. Ethnomedicinal uses of some plants in the Kashmir Himalaya. 2011.
Jouad H, Haloui M, Rhiouani H, El Hilaly J, Eddouks M. Ethnobotanical survey of medicinal plants used for the treatment of diabetes, cardiac and renal diseases in the north centre region of Morocco (Fez–Boulemane). J Ethnopharmacol. 2001;77(2–3):175–82.
Tumpa SI, Hossain MI, Ishika T. Ethnomedicinal uses of herbs by indigenous medicine practitioners of Jhenaidah district, Bangladesh. J Pharmacogn Phytochem. 2014;3(2):509–27.
Zari ST, Zari TA. A review of four common medicinal plants used to treat eczema. J Med Plants Res. 2015;9(24):702–11.
Dogan Y, Ugulu I. Medicinal plants used for gastrointestinal disorders in some districts of Izmir province, Turkey. Study Ethno-Medicine. 2013;7(3):149–61.
Shrivastava A, Chaturvedi U, Singh SV, Saxena JK, Bhatia G. A mechanism based pharmacological evaluation of efficacy of Allium sativum in regulation of dyslipidemia and oxidative stress in hyperlipidemic rats. Asian J Pharm Clin Res. 2012;5:123–6.
Zeraati F, Esna-Ashari F, Araghchian M, Emam AH, Rad MV, Seif S. Evaluation of topical antinociceptive effect of Artemisia absinthium extract in mice and possible mechanisms. African J Pharm Pharmacol. 2014;8(19):492–6.
Daradka HM, Abas MM, Mohammad MAM, Jaffar MM. Antidiabetic effect of Artemisia absinthium extracts on alloxan-induced diabetic rats. Comp Clin Path. 2014;23(6):1733–42.
Article CAS Google Scholar
Gliani AH, Janbaz KH. Hepatoprotective effects of Artemisia scoparia against carbon tetrachloride: an environmental contaminant. Journal-Pakistan Med Assoc. 1994;44:65.
Zhu X, Zhang W, Zhao J, Wang J, Qu W. Hypolipidaemic and hepatoprotective effects of ethanolic and aqueous extracts from Asparagus officinalis L. by-products in mice fed a high-fat diet. J Sci Food Agric. 2010;90(7):1129–35.
Mathur R, Gupta SK, Mathur SR, Velpandian T. Anti-tumor studies with extracts of Calotropis procera (Ait.) R. Br. Root employing Hep2 cells and their possible mechanism of action. 2009.
Basu A, Sen T, Pal S, Mascolo N, Capasso F, Nag Chaudhuri AK. Studies on the antiulcer activity of the chloroform fraction of Calotropis procera root extract. Phyther Res. 1997;11(2):163–5.
Zuardi AW, Crippa JAS, Hallak JEC, Moreira FA, Guimaraes FS. Cannabidiol, a Cannabis sativa constituent, as an antipsychotic drug. Brazilian J Med Biol Res. 2006;39(4):421–9.
Abdel-Sattar E, Harraz FM, Ghareib SA, Elberry AA, Gabr S, Suliaman MI. Antihyperglycaemic and hypolipidaemic effects of the methanolic extract of Caralluma tuberculata in streptozotocin-induced diabetic rats. Nat Prod Res. 2011;25(12):1171–9.
Ahmad S, Sharma R, Mahajan S, Gupta A. Antibacterial activity of Celtis australis by invitro study. 2012.
Pushparaj PN, Low HK, Manikandan J, Tan BKH, Tan CH. Anti-diabetic effects of Cichorium intybus in streptozotocin-induced diabetic rats. J Ethnopharmacol. 2007;111(2):430–4.
Vinaykumar T, Eswarkumar K, Roy H. Evaluation of antihyperglycemic activity of Citrullus colocynthis fruit pulp in streptozotocin induced diabetic rats.
Abdel-Hassan IA, Abdel-Barry JA, Mohammeda ST. The hypoglycaemic and antihyperglycaemic effect of Citrullus colocynthis fruit aqueous extract in normal and alloxan diabetic rabbits. J Ethnopharmacol. 2000;71(1–2):325–30.
Sebbagh N, Cruciani GC, Ouali F, Berthault MF, Rouch C, Sari DC. Comparative effects of Citrullus colocynthis, sunflower and olive oil-enriched diet in streptozotocin-induced diabetes in rats. Diabetes Metab. 2009;35(3):178–84.
Khan S, Wang Z, Wang R, Zhang L. Horizontoates AC. New cholinesterase inhibitors from Cotoneaster horizontalis. Phytochem Lett 2014;10:204–208.
Soni P, Siddiqui AA, Dwivedi J, Soni V. Pharmacological properties of Datura stramonium L. as a potential medicinal tree: an overview. Asian Pac J Trop Biomed. 2012;2(12):1002–8.
Alam S, Asad M, Asdaq SMB, Prasad VS. Antiulcer activity of methanolic extract of Momordica charantia L. in rats. J Ethnopharmacol. 2009;123(3):464–9.
Rashid R, Mukhtar F, Khan A. Antifungal and cytotoxic activities of Nannorrhops ritchiana roots extract. Acta Pol Pharm. 2014;71(5):789.
PubMed Google Scholar
Amin A, Khan MA, Shah S, Ahmad M, Zafar M, Hameed A. Inhibitory effects of Olea ferruginea crude leaves extract against some bacterial and fungal pathogen. Pak J Pharm Sci. 2013;26(2):251–54.
Melese E, Asres K, Asad M, Engidawork E. Evaluation of the antipeptic ulcer activity of the leaf extract of Plantago lanceolata L. in rodents. Phyther Res. 2011;25(8):1174–80.
Karimi G, Hosseinzadeh H, Ettehad N. Evaluation of the gastric antiulcerogenic effects of Portulaca oleracea L. extracts in mice. Phyther Res. 2004;18(6):484–7.
Jainu M, Devi CSS. Antiulcerogenic and ulcer healing effects of Solanum nigrum (L.) on experimental ulcer models: possible mechanism for the inhibition of acid formation. J Ethnopharmacol. 2006;104(1–2):156–63.
Rashid M, Mushtaq MN, Malik MNH, Ghumman SA, Numan M, Khan AQ. Pharmacological evaluation of antidiabetic effect of ethyl acetate extract of Teucrium stocksianum Boiss in alloxan-induced diabetic rabbits. JAPS, J Anim Plant Sci. 2013;23(2):436–9.
Rawal P, Adhikari R, Tiwari A. Antifungal activity of Viola canescens against fusarium oxysporum f. sp. lycopersici. Int J Curr Microbiol App Sci. 2015;4(5):1025–32.
PSV R. Phytochemical screening and in vitro antimicrobial investigation of the methanolic extract of Xanthium strumarium leaf. Int J Drug Dev Res. 2011;3(4):286–93.
Download references
Acknowledgements
This work is part of the Doctoral research work of the principal (first) author. The authors also acknowledge the participants for sharing their valuable information.
Authors’ contribution
WH conducted the collection of field data and wrote the initial draft of the manuscript. LB supervised the project. MU and MA helped in the field survey, sampling, and identification of taxon. AA and FH helped in the data analysis and revision of the manuscript. All the authors approved the final manuscript after revision.
Availability of data and materials
All the supporting data is available in Additional files 1 and 2 .
Author information
Authors and affiliations.
Department of Botany, University of Peshawar, Peshawar, 25000, Pakistan
Wahid Hussain & Lal Badshah
Department of Botany, University of Science and Technology, Bannu, Pakistan
Manzoor Ullah
Department of Plant Science, Quaid-i-Azam University, Islamabad, Pakistan
Dr. Khan Shaheed Govt. Degree College Kabal, Swat, Pakistan
Institute of Biological Sciences, Sarhad University of Science and Information Technology, Peshawar, Pakistan
Farrukh Hussain
You can also search for this author in PubMed Google Scholar
Corresponding author
Correspondence to Maroof Ali .
Ethics declarations
Ethics approval and consent to participate.
Letters of permission were taken from Peshawar University and local administration office prior to the data collections. Oral agreements were also got from the local informants about the aims and objectives of the study prior to the interviews, and all the field data were collected through their oral consents. No further ethics approval was required.
Competing interests
The authors declare that they have no competing interest.
Publisher’s Note
Springer Nature remains neutral with regard to jurisdictional claims in published maps and institutional affiliations.
Additional files
Additional file 1:.
Field data of the research project Quantitative study of medicinal plants used by the communities residing in Koh-e-Safaid Range northern Pakistani-Afghan border. (XLSX 167 kb)
Additional file 2:
Annexures. (DOCX 27 kb)
Rights and permissions
Open Access This article is distributed under the terms of the Creative Commons Attribution 4.0 International License ( http://creativecommons.org/licenses/by/4.0/ ), which permits unrestricted use, distribution, and reproduction in any medium, provided you give appropriate credit to the original author(s) and the source, provide a link to the Creative Commons license, and indicate if changes were made. The Creative Commons Public Domain Dedication waiver ( http://creativecommons.org/publicdomain/zero/1.0/ ) applies to the data made available in this article, unless otherwise stated.
Reprints and permissions
About this article
Cite this article.
Hussain, W., Badshah, L., Ullah, M. et al. Quantitative study of medicinal plants used by the communities residing in Koh-e-Safaid Range, northern Pakistani-Afghan borders. J Ethnobiology Ethnomedicine 14 , 30 (2018). https://doi.org/10.1186/s13002-018-0229-4
Download citation
Received : 23 November 2017
Accepted : 05 April 2018
Published : 25 April 2018
DOI : https://doi.org/10.1186/s13002-018-0229-4
Share this article
Anyone you share the following link with will be able to read this content:
Sorry, a shareable link is not currently available for this article.
Provided by the Springer Nature SharedIt content-sharing initiative
- Quantitative study
- Medicinal plants
- Traditional knowledge
- Koh-e-Safaid Range
Journal of Ethnobiology and Ethnomedicine
ISSN: 1746-4269
- General enquiries: [email protected]
- Research article
- Open access
- Published: 01 December 2006
Using quantitative and qualitative data in health services research – what happens when mixed method findings conflict? [ISRCTN61522618]
- Suzanne Moffatt 1 ,
- Martin White 1 ,
- Joan Mackintosh 1 &
- Denise Howel 1
BMC Health Services Research volume 6 , Article number: 28 ( 2006 ) Cite this article
83k Accesses
122 Citations
16 Altmetric
Metrics details
In this methodological paper we document the interpretation of a mixed methods study and outline an approach to dealing with apparent discrepancies between qualitative and quantitative research data in a pilot study evaluating whether welfare rights advice has an impact on health and social outcomes among a population aged 60 and over.
Quantitative and qualitative data were collected contemporaneously. Quantitative data were collected from 126 men and women aged over 60 within a randomised controlled trial. Participants received a full welfare benefits assessment which successfully identified additional financial and non-financial resources for 60% of them. A range of demographic, health and social outcome measures were assessed at baseline, 6, 12 and 24 month follow up. Qualitative data were collected from a sub-sample of 25 participants purposively selected to take part in individual interviews to examine the perceived impact of welfare rights advice.
Separate analysis of the quantitative and qualitative data revealed discrepant findings. The quantitative data showed little evidence of significant differences of a size that would be of practical or clinical interest, suggesting that the intervention had no impact on these outcome measures. The qualitative data suggested wide-ranging impacts, indicating that the intervention had a positive effect. Six ways of further exploring these data were considered: (i) treating the methods as fundamentally different; (ii) exploring the methodological rigour of each component; (iii) exploring dataset comparability; (iv) collecting further data and making further comparisons; (v) exploring the process of the intervention; and (vi) exploring whether the outcomes of the two components match.
The study demonstrates how using mixed methods can lead to different and sometimes conflicting accounts and, using this six step approach, how such discrepancies can be harnessed to interrogate each dataset more fully. Not only does this enhance the robustness of the study, it may lead to different conclusions from those that would have been drawn through relying on one method alone and demonstrates the value of collecting both types of data within a single study. More widespread use of mixed methods in trials of complex interventions is likely to enhance the overall quality of the evidence base.
Combining quantitative and qualitative methods in a single study is not uncommon in social research, although, 'traditionally a gulf is seen to exist between qualitative and quantitative research with each belonging to distinctively different paradigms'. [ 1 ] Within health research there has, more recently, been an upsurge of interest in the combined use of qualitative and quantitative methods, sometimes termed mixed methods research [ 2 ] although the terminology can vary. [ 3 ] Greater interest in qualitative research has come about for a number of reasons: the numerous contributions made by qualitative research to the study of health and illness [ 4 – 6 ]; increased methodological rigor [ 7 ] within the qualitative paradigm, which has made it more acceptable to researchers or practitioners trained within a predominantly quantitative paradigm [ 8 ]; and, because combining quantitative and qualitative methods may generate deeper insights than either method alone. [ 9 ] It is now widely recognised that public health problems are embedded within a range of social, political and economic contexts. [ 10 ] Consequently, a range of epidemiological and social science methods are employed to research these complex issues. [ 11 ] Further legitimacy for the use of qualitative methods alongside quantitative has resulted from the recognition that qualitative methods can make an important contribution to randomised controlled trials (RCTs) evaluating complex health service interventions. There is published work on the various ways that qualitative methods are being used in RCTs (e.g. [ 12 , 13 ] but little on how they can optimally enhance the usefulness and policy relevance of trial findings. [ 14 , 15 ]
A number of mixed methods publications outline the various ways in which qualitative and quantitative methods can be combined. [ 1 , 2 , 9 , 16 ] For the purposes of this paper with its focus on mixed methods in the context of a pilot RCT, the significant aspects of mixed methods appear to be: purpose, process and, analysis and interpretation. In terms of purpose, qualitative research may be used to help identify the relevant variables for study [ 17 ], develop an instrument for quantitative research [ 18 ], to examine different questions (such as acceptability of the intervention, rather than its outcome) [ 19 ]; and to examine the same question with different methods (using, for example participant observation or in depth interviews [ 1 ]). Process includes the priority accorded to each method and ordering of both methods which may be concurrent, sequential or iterative. [ 20 ] Bryman [ 9 ] points out that, 'most researchers rely primarily on a method associated with either quantitative or qualitative methods and then buttress their findings with a method associated with the other tradition' (p128). Both datasets may be brought together at the 'analysis/interpretation' phase, often known as 'triangulation' [ 21 ]. Brannen [ 1 ] suggests that most researchers have taken this to mean more than one type of data, but she stresses that Denzin's original conceptualisation involved methods, data, investigators or theories. Bringing different methods together almost inevitably raises discrepancies in findings and their interpretation. However, the investigation of such differences may be as illuminating as their points of similarity. [ 1 , 9 ]
Although mixed methods are now widespread in health research, quantitative and qualitative methods and results are often published separately. [ 22 , 23 ] It is relatively rare to see an account of the methodological implications of the strategy and the way in which both methods are combined when interpreting the data within a particular study. [ 1 ] A notable exception is a study showing divergence between qualitative and quantitative findings of cancer patients' quality of life using a detailed case study approach to the data. [ 13 ]
By presenting quantitative and qualitative data collected within a pilot RCT together, this paper has three main aims: firstly, to demonstrate how divergent quantitative and qualitative data led us to interrogate each dataset more fully and assisted in the interpretation process, producing a greater research yield from each dataset; secondly, to demonstrate how combining both types of data at the analysis stage produces 'more than the sum of its parts'; and thirdly, to emphasise the complementary nature of qualitative and quantitative methods in RCTs of complex interventions. In doing so, we demonstrate how the combination of quantitative and qualitative data led us to conclusions different from those that would have been drawn through relying on one or other method alone.
The study that forms the basis of this paper, a pilot RCT to examine the impact of welfare rights advice in primary care, was funded under the UK Department of Health's Policy Research Programme on tackling health inequalities, and focused on older people. To date, little research has been able to demonstrate how health inequalities can be tackled by interventions within and outside the health sector. Although living standards have risen among older people, a common experience of growing old is worsening material circumstances. [ 24 ] In 2000–01 there were 2.3 million UK pensioners living in households with below 60 per cent of median household income, after housing costs. [ 25 ] Older people in the UK may be eligible for a number of income- or disability-related benefits (the latter could be non-financial such as parking permits or adaptations to the home), but it has been estimated that approximately one in four (about one million) UK pensioner households do not claim the support to which they are entitled. [ 26 ] Action to facilitate access to and uptake of welfare benefits has taken place outside the UK health sector for many years and, more recently, has been introduced within parts of the health service, but its potential to benefit health has not been rigorously evaluated. [ 27 – 29 ]
There are a number of models of mixed methods research. [ 2 , 16 , 30 ] We adopted a model which relies of the principle of complementarity, using the strengths of one method to enhance the other. [ 30 ] We explicitly recognised that each method was appropriate for different research questions. We undertook a pragmatic RCT which aimed to evaluate the health effects of welfare rights advice in primary care among people aged over 60. Quantitative data included standardised outcome measures of health and well-being, health related behaviour, psycho-social interaction and socio-economic status ; qualitative data used semi-structured interviews to explore participants' views about the intervention, its outcome, and the acceptability of the research process.
Following an earlier qualitative pilot study to inform the selection of appropriate outcome measures [ 31 ], contemporaneous quantitative and qualitative data were collected. Both datasets were analysed separately and neither compared until both analyses were complete. The sampling strategy mirrored the embedded design; probability sampling for the quantitative study and theoretical sampling for the qualitative study, done on the basis of factors identified in the quantitative study.
Approval for the study was obtained from Newcastle and North Tyneside Joint Local Research Ethics Committee and from Newcastle Primary Care Trust.
The intervention
The intervention was delivered by a welfare rights officer from Newcastle City Council Welfare Rights Service in participants' own homes and comprised a structured assessment of current welfare status and benefits entitlement, together with active assistance in making claims where appropriate over the following six months, together with necessary follow-up for unresolved claims.
Quantitative study
The design presented ethical dilemmas as it was felt problematic to deprive the control group of welfare rights advice, since there is adequate evidence to show that it leads to significant financial gains. [ 32 ] To circumvent this dilemma, we delivered welfare rights advice to the control group six months after the intervention group. A single-blinded RCT with allocation of individuals to intervention (receipt of welfare rights consultation immediately) and control condition (welfare rights consultation six months after entry into the trial) was undertaken.
Four general practices located at five surgeries across Newcastle upon Tyne took part. Three of the practices were located in the top ten per cent of most deprived wards in England using the Index of Multiple Deprivation (two in the top one percent – ranked 30 th and 36 th most deprived); the other practice was ranked 3,774 out of a total of 8,414 in England. [ 33 ]
Using practice databases, a random sample of 100 patients aged 60 years or over from each of four participating practices was invited to take part in the study. Only one individual per household was allowed to participate in the trial, but if a partner or other adult household member was also eligible for benefits, they also received welfare rights advice. Patients were excluded if they were permanently hospitalised or living in residential or nursing care homes.
Written informed consent was obtained at the baseline interview. Structured face to face interviews were carried out at baseline, six, 12 and 24 months using standard scales covering the areas of demographics, mental and physical health (SF36) [ 34 ], Hospital Anxiety and Depression Scale (HADS) [ 35 ], psychosocial descriptors (e.g. Social Support Questionnaire [ 36 ] and the Self-Esteem Inventory, [ 37 ], and socioeconomic indicators (e.g. affordability and financial vulnerability). [ 38 ] Additionally, a short semi-structured interview was undertaken at 24 months to ascertain the perceived impact of additional resources for those who received them.
All health and welfare assessment data were entered onto customised MS Access databases and checked for quality and completeness. Data were transferred to the Statistical Package for the Social Sciences (SPSS) v11.0 [ 39 ] and STATA v8.0 for analysis. [ 40 ]
Qualitative study
The qualitative findings presented in this paper focus on the impact of the intervention. The sampling frame was formed by those (n = 96) who gave their consent to be contacted during their baseline interview for the RCT. The study sample comprised respondents from intervention and control groups purposively selected to include those eligible for the following resources: financial only; non-financial only; both financial and non financial; and, none. Sampling continued until no new themes emerged from the interviews; until data 'saturation' was reached. [ 21 ]
Initial interviews took place between April and December 2003 in participants' homes after their welfare rights assessment; follow-up interviews were undertaken in January and February 2005. The semi-structured interview schedule covered perceptions of: impact of material and/or financial benefits; impact on mental and/or physical health; impact on health related behaviours; social benefits; and views about the link between material resources and health. All participants agreed to the interview being audio-recorded. Immediately afterwards, observational field notes were made. Interviews were transcribed in full.
Data analysis largely followed the framework approach. [ 41 ] Data were coded, indexed and charted systematically; and resulting typologies discussed with other members of the research team, 'a pragmatic version of double coding'. [ 42 ] Constant comparison [ 43 ] and deviant case analysis [ 44 ] were used since both methods are important for internal validation. [ 7 , 42 ] Finally, sets of categories at a higher level of abstraction were developed.
A brief semi-structured interview was undertaken (by JM) with all participants who received additional resources. These interview data explored the impact data of additional resources on all of those who received them, not just the qualitative sub-sample. The data were independently coded by JM and SM using the same coding frame. Discrepant codes were examined by both researchers and a final code agreed.
One hundred and twenty six people were recruited into the study; there were 117 at 12 month follow-up and 109 at 24 months (five deaths, one moved, the remainder declined).
Table 1 shows the distribution of financial and non-financial benefits awarded as a result of the welfare assessments. Sixty percent of participants were awarded some form of welfare benefit, and just over 40% received a financial benefit. Some households received more than one type of benefit.
Table 2 compares the quantitative and qualitative sub-samples on a number of personal, economic, health and lifestyle factors at baseline. Intervention and control groups were comparable.
Table 3 compares outcome measures by award group, i.e. no award, non-financial and financial and shows only small differences between the mean changes across each group, none of which were statistically significant. Other analyses of the quantitative data compared the changes seen between baseline and six months (by which time the intervention group had received the welfare rights advice but the control group had not) and found little evidence of differences between the intervention and control groups of any practical importance. The only statistically significant difference between the groups was a small decrease in financial vulnerability in the intervention group after six months. [ 45 ]
There was little evidence for differences in health and social outcomes measures as a result of the receipt of welfare advice of a size that would be of major practical or clinical interest. However, this was a pilot study, with only the power to detect large differences if they were present. One reason for a lack of difference may be that the scales were less appropriate for older people and did not capture all relevant outcomes. Another reason for the lack of differences may be that insufficient numbers of people had received their benefits for long enough to allow any health outcomes to have changed when comparisons were made. Fourteen per cent of participants found to be eligible for financial benefits had not started receiving their benefits by the time of the first follow-up interview after their benefit assessment (six months for intervention, 12 months for control); and those who had, had only received them for an average of 2 months. This is likely to have diluted any impact of the intervention effect, and might account, to some extent, for the lack of observed effect.
Twenty five interviews were completed, fourteen of whom were from the intervention group. Ten participants were interviewed with partners who made active contributions. Twenty two follow-up interviews were undertaken between twelve and eighteen months later (three individuals were too ill to take part).
Table 1 (fifth column) shows that 14 of the participants in the qualitative study received some financial award. The median income gain was (€84, $101) (range £10 (€15, $18) -£100 (€148, $178)) representing a 4%-55% increase in weekly income. 18 participants were in receipt of benefit, either as a result of the current intervention or because of claims made prior to this study.
By the follow-up (FU) interviews all but one participant had been receiving their benefits for between 17 and 31 months. The intervention was viewed positively by all interviewees irrespective of outcome. However, for the fourteen participants who received additional financial resources the impact was considerable and accounts revealed a wide range of uses for the extra money. Participants' accounts revealed four linked categories, summarised on Table 4 . Firstly, increased affordability of necessities , without which maintaining independence and participating in daily life was difficult. This included accessing transport, maintaining social networks and social activities, buying better quality food, stocking up on food, paying bills, preventing debt and affording paid help for household activities. Secondly, occasional expenses such as clothes, household equipment, furniture and holidays were more affordable. Thirdly, extra income was used to act as a cushion against potential emergencies and to increase savings . Fourthly, all participants described the easing of financial worries as bringing ' peace of mind' .
Without exception, participants were of the view that extra money or resources would not improve existing health problems. The reasons behind these strongly held views about individual health conditions was generally that their poor health was attributed to specific health conditions and a combination of family history or fate, which were immune to the effects of money. Most participants had more than one chronic condition and felt that because of these conditions, plus their age, additional money would have no effect.
However, a number of participants linked the impact of the intervention with improved ways of coping with their conditions because of what the extra resources enabled them to do:
Mrs T: Having money is not going to improve his health, we could win the lottery and he would still have his health problems.
Mr T: No, but we don't need to worry if I wanted .... Well I mean I eat a lot of honey and I think it's very good, very healthful for you ... at one time we couldn't have afforded to buy these things. Now we can go and buy them if I fancy something, just go and get it where we couldn't before .
Mrs T: Although the Attendance Allowance is actually his [partners], it's made me relax a bit more ... I definitely worry less now (N15, female, 62 and partner)
Despite the fact that no-one expected their own health conditions to improve, most people believed that there was a link between resources and health in a more abstract sense, either because they experienced problems affording necessities such as healthy food or maintaining adequate heat in their homes, or because they empathised with those who lacked money. Participants linked adequate resources to maintaining health and contributing to a sense of well-being.
Money does have a lot to do with health if you are poor. It would have a lot to do with your health ... I don't buy loads and loads of luxuries, but I know I can go out and get the food we need and that sort of thing. I think that money is a big part of how a house, or how people in that house are . (N13, female, 72)
Comparing the results from the two datasets
When the separate analyses of the quantitative and qualitative datasets after the 12 month follow-up structured interviews were completed, the discrepancy in the findings became apparent. The quantitative study showed little evidence of a size that would be of practical or clinical interest, suggesting that the intervention had no impact on these outcome measures. The qualitative study found a wide-ranging impact, indicating that the intervention had a positive effect. The presence of such inter-method discrepancy led to a great deal of discussion and debate, as a result of which we devised six ways of further exploring these data.
(i) Treating the methods as fundamentally different
This process of simultaneous qualitative and quantitative dataset interrogation enables a deeper level of analysis and interpretation than would be possible with one or other alone and demonstrates how mixed methods research produces more than the sum of its parts. It is worth emphasising however, that it is not wholly surprising that each method comes up with divergent findings since each asked different, but related questions, and both are based on fundamentally different theoretical paradigms. Brannen [ 1 ] and Bryman [ 9 ] argue that it is essential to take account of these theoretical differences and caution against taking a purely technical approach to the use of mixed methods, a simple 'bolting together' of techniques. [ 17 ] Combining the two methods for crossvalidation (triangulation) purposes is not a viable option because it rests on the premise that both methods are examining the same research problem. [ 1 ] We have approached the divergent findings as indicative of different aspects of the phenomena in question and searched for reasons which might explain these inconsistencies. In the approach that follows, we have treated the datasets as complementary, rather than attempt to integrate them, since each approach reflects a different view on how social reality ought to be studied.
(ii) Exploring the methodological rigour of each component
It is standard practice at the data analysis and interpretation phases of any study to scrutinise methodological rigour. However, in this case, we had another dataset to use as a yardstick for comparison and it became clear that our interrogation of each dataset was informed to some extent by the findings of the other. It was not the case that we expected to obtain the same results, but clearly the divergence of our findings was of great interest and made us more circumspect about each dataset. We began by examining possible reasons why there might be problems with each dataset individually, but found ourselves continually referring to the results of the other study as a benchmark for comparison.
With regard to the quantitative study, it was a pilot, of modest sample size, and thus not powered to detect small differences in the key outcome measures. In addition there were three important sources of dilution effects: firstly, only 63% of intervention group participants received some type of financial award; secondly, we found that 14% of those in the trial eligible for financial benefits did not receive their money until after the follow up assessments had been carried out; and thirdly, many had received their benefits for only a short period, reducing the possibility of detecting any measurable effects at the time of follow-up. All of these factors provide some explanation for the lack of a measurable effect between intervention and control group and between those who did and did not receive additional financial resources.
The number of participants in the qualitative study who received additional financial resources as a result of this intervention was small (n = 14). We would argue that the fieldwork, analysis and interpretation [ 46 ] were sufficiently transparent to warrant the degree of methodological rigour advocated by Barbour [ 7 , 17 ] and that the findings were therefore an accurate reflection of what was being studied. However, there still remained the possibility that a reason for the discrepant findings was due to differences between the qualitative sub-sample and the parent sample, which led us to step three.
(iii) Exploring dataset comparability
We compared the qualitative and quantitative samples on a number of social and economic factors (Table 2 ). In comparison to the parent sample, the qualitative sub-sample was slightly older, had fewer men, a higher proportion with long-term limiting illness, but fewer current smokers. However, there was nothing to indicate that such small differences would account for the discrepancies. There were negligible differences in SF-36 (Physical and Mental) and HAD (Anxiety and Depression) scores between the groups at baseline, which led us to discount the possibility that those in the quantitative sub sample were markedly different to the quantitative sample on these outcome measures.
(iv) Collection of additional data and making further comparisons
The divergent findings led us to seek further funding to undertake collection of additional quantitative and qualitative data at 24 months. The quantitative and qualitative follow-up data verified the initial findings of each study. [ 45 ] We also collected a limited amount of qualitative data on the perceived impact of resources, from all participants who had received additional resources. These data are presented in figure 1 which shows the uses of additional resources at 24 month follow-up for 35 participants (N = 35, 21 previously in quantitative study only, 14 in both). This dataset demonstrates that similar issues emerged for both qualitative and quantitative datasets: transport, savings and 'peace of mind' emerged as key issues, but the data also showed that the additional money was used on a wide range of items. This follow-up confirmed the initial findings of each study and further, indicated that the perceived impact of the additional resources was the same for a larger sample than the original qualitative sub-sample, further confirming our view that the positive findings extended beyond the fourteen participants in the qualitative sub-sample, to all those receiving additional resources.
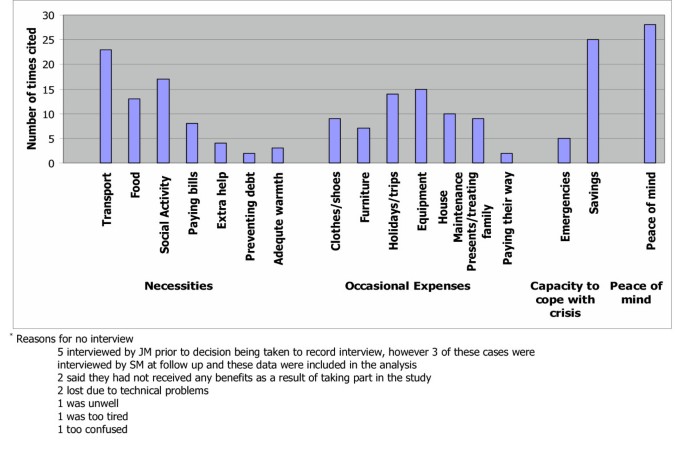
Use of additional resources at 2 year follow up (N = 35)*.
(v) Exploring whether the intervention under study worked as expected
The qualitative study revealed that many participants had received welfare benefits via other services prior to this study, revealing the lack of a 'clean slate' with regard to the receipt of benefits, which we had not anticipated. We investigated this further in the quantitative dataset and found that 75 people (59.5%) had received benefits prior to the study; if the first benefit was on health grounds, a later one may have been because their health had deteriorated further.
(vi) Exploring whether the outcomes of the quantitative and qualitative components match
'Probing certain issues in greater depth' as advocated by Bryman (p134) [ 1 ] focussed our attention on the outcome measures used in the quantitative part of the study and revealed several challenges. Firstly, the qualitative study revealed a number of dimensions not measured by the quantitative study, such as, 'maintaining independence' which included affording paid help, increasing and improving access to facilities and managing better within the home. Secondly, some of the measures used with the intention of capturing dimensions of mental health did not adequately encapsulate participants' accounts of feeling 'less stressed' and 'less depressed' by financial worries. Probing both datasets also revealed congruence along the dimension of physical health. No differences were found on the SF36 physical scale and participants themselves did not expect an improvement in physical health (for reasons of age and chronic health problems). The real issue would appear to be measuring ways in which older people are better able to cope with existing health problems and maintain their independence and quality of life, despite these conditions.
Qualitative study results also led us to look more carefully at the quantitative measures we used. Some of the standardised measures were not wholly applicable to a population of older people. Mallinson [ 47 ] also found this with the SF36 when she demonstrated some of its limitations with this age group, as well as how easy it is to, 'fall into the trap of using questionnaires like a form of laboratory equipment and forget that ... they are open to interpretation'. The data presented here demonstrate the difficulties of trying to capture complex phenomena quantitatively. However, they also demonstrate the usefulness of having alternative data forms on which to draw whether complementary (where they differ but together generate insights) or contradictory (where the findings conflict). [ 30 ] In this study, the complementary and contradictory findings of the two datasets proved useful in making recommendations for the design of a definitive study.
Many researchers understand the importance, indeed the necessity, of combining methods to investigate complex health and social issues. Although quantitative research remains the dominant paradigm in health services research, qualitative research has greater prominence than before and is no longer, as Barbour [ 42 ] points out regarded as the 'poor relation to quantitative research that it has been in the past' (p1019). Brannen [ 48 ] argues that, despite epistemological differences there are 'more overlaps than differences'. Despite this, there is continued debate about the authority of each individual mode of research which is not surprising since these different styles, 'take institutional forms, in relation to cultures of and markets for knowledge' (p168). [ 49 ] Devers [ 50 ] points out that the dominance of positivism, especially within the RCT method, has had an overriding influence on the criteria used to assess research which has had the inevitable result of viewing qualitative studies unfavourably. We advocate treating qualitative and quantitative datasets as complementary rather than in competition for identifying the true version of events. This, we argue, leads to a position which exploits the strengths of each method and at the same time counters the limitations of each. The process of interpreting the meaning of these divergent findings has led us to conclude that much can be learned from scientific realism [ 51 ]which has 'sought to position itself as a model of scientific explanation which avoids the traditional epistemological poles of positivism and relativism' (p64). This stance enables investigators to take account of the complexity inherent in social interventions and reinforces, at a theoretical level, the problems of attempting to measure the impact of a social intervention via experimental means. However, the current focus on evidence based health care [ 52 ] now includes public health [ 53 , 54 ] and there is increased attention paid to the results of trials of public health interventions, attempting as they do, to capture complex social phenomena using standardised measurement tools. We would argue that at the very least, the inclusion of both qualitative and quantitative elements in such studies, is essential and ultimately more cost-effective, increasing the likelihood of arriving at a more thoroughly researched and better understood set of results.
The findings of this study demonstrate how the use of mixed methods can lead to different and sometimes conflicting accounts. This, we argue, is largely due to the outcome measures in the RCT not matching the outcomes emerging from the qualitative arm of the study. Instead of making assumptions about the correct version, we have reported the results of both datasets together rather than separately, and advocate six steps to interrogate each dataset more fully. The methodological strategy advocated by this approach involves contemporaneous qualitative and quantitative data collection, analysis and reciprocal interrogation to inform interpretation in trials of complex interventions. This approach also indicates the need for a realistic appraisal of quantitative tools. More widespread use of mixed methods in trials of complex interventions is likely to enhance the overall quality of the evidence base.
Brannen J: Mixing Methods: qualitative and quantitative research. 1992, Aldershot, Ashgate
Google Scholar
Tashakkori A, Teddlie C: Handbook of Mixed Methods in Social and Behavioural Research. 2003, London, Sage
Morgan DL: Triangulation and it's discontents: Developing pragmatism as an alternative justification for combining qualitative and quantitative methods. Cambridge, 11-12 July.. 2005.
Pill R, Stott NCH: Concepts of illness causation and responsibility: some preliminary data from a sample of working class mothers. Social Science and Medicine. 1982, 16: 43-52. 10.1016/0277-9536(82)90422-1.
Article CAS PubMed Google Scholar
Scambler G, Hopkins A: Generating a model of epileptic stigma: the role of qualitative analysis. Social Science and Medicine. 1990, 30: 1187-1194. 10.1016/0277-9536(90)90258-T.
Townsend A, Hunt K, Wyke S: Managing multiple morbidity in mid-life: a qualitative study of attitudes to drug use. BMJ. 2003, 327: 837-841. 10.1136/bmj.327.7419.837.
Article PubMed PubMed Central Google Scholar
Barbour RS: Checklists for improving rigour in qualitative research: the case of the tail wagging the dog?. British Medical Journal. 2001, 322: 1115-1117.
Article CAS PubMed PubMed Central Google Scholar
Pope C, Mays N: Qualitative Research in Health Care. 2000, London, BMJ Books
Bryman A: Quantity and Quality in Social Research. 1995, London, Routledge
Ashton J: Healthy cities. 1991, Milton Keynes, Open University Press
Baum F: Researching Public Health: Behind the Qualitative-Quantitative Methodological Debate. Social Science and Medicine. 1995, 40: 459-468. 10.1016/0277-9536(94)E0103-Y.
Donovan J, Mills N, Smith M, Brindle L, Jacoby A, Peters T, Frankel S, Neal D, Hamdy F: Improving design and conduct of randomised trials by embedding them in qualitative research: (ProtecT) study. British Medical Journal. 2002, 325: 766-769.
Cox K: Assessing the quality of life of patients in phase I and II anti-cancer drug trials: interviews versus questionnaires. Social Science and Medicine. 2003, 56: 921-934. 10.1016/S0277-9536(02)00100-4.
Article PubMed Google Scholar
Lewin S: Mixing methods in complex health service randomised controlled trials: research 'best practice'. Mixed Methods in Health Services Research Conference 23rd November 2004, Sheffield University.
Cresswell JW: Mixed methods research and applications in intervention studies.: ; Cambridge, July 11-12. 2005.
Cresswell JW: Research Design. Qualitative, Quantitative and Mixed Methods Approaches. 2003, London, Sage
Barbour RS: The case for combining qualitative and quanitative approaches in health services research. Journal of Health Services Research and Policy. 1999, 4: 39-43.
Gabriel Z, Bowling A: Quality of life from the perspectives of older people. Ageing & Society. 2004, 24: 675-691. 10.1017/S0144686X03001582.
Article Google Scholar
Koops L, Lindley RL: Thrombolysis for acute ischaemic stroke: consumer involvement in design of new randomised controlled trial. BMJ. 2002, 325: 415-418. 10.1136/bmj.325.7361.415.
O'Cathain A, Nicholl J, Murphy E: Making the most of mixed methods. Mixed Methods in Health Services Research Conference 23rd November 2004, Sheffield University.
Denzin NK: The Research Act. 1978, New York, McGraw-Hill Book Company
Roberts H, Curtis K, Liabo K, Rowland D, DiGuiseppi C, Roberts I: Putting public health evidence into practice: increasing the prevalance of working smoke alarms in disadvantaged inner city housing. Journal of Epidemiology & Community Health. 2004, 58: 280-285. 10.1136/jech.2003.007948.
Article CAS Google Scholar
Rowland D, DiGuiseppi C, Roberts I, Curtis K, Roberts H, Ginnelly L, Sculpher M, Wade A: Prevalence of working smoke alarms in local authority inner city housing: randomised controlled trial. British Medical Journal. 2002, 325: 998-1001.
Vincent J: Old Age. 2003, London, Routledge
Chapter Google Scholar
Department for Work and Pensions: Households below average income statistics 2000/01. 2002, London, Department for Work and Pensions
National Audit Office: Tackling Pensioner Pverty: Encouraging take-up of entitlements. 2002, London, National Audit Office
Paris JAG, Player D: Citizens advice in general practice. British Medical Journal. 1993, 306: 1518-1520.
Abbott S: Prescribing welfare benefits advice in primary care: is it a health intervention, and if so, what sort?. Journal of Public Health Medicine. 2002, 24: 307-312. 10.1093/pubmed/24.4.307.
Harding R, Sherr L, Sherr A, Moorhead R, Singh S: Welfare rights advice in primary care: prevalence, processes and specialist provision. Family Practice. 2003, 20: 48-53. 10.1093/fampra/20.1.48.
Morgan DL: Practical strategies for combining qualitative and quantitative methods: applications to health research. Qualitative Health Research. 1998, 8: 362-376.
Moffatt S, White M, Stacy R, Downey D, Hudson E: The impact of welfare advice in primary care: a qualitative study. Critical Public Health. 2004, 14: 295-309. 10.1080/09581590400007959.
Thomson H, Hoskins R, Petticrew M, Ogilvie D, Craig N, Quinn T, Lindsey G: Evaluating the health effects of social interventions. British Medical Journal. 2004, 328: 282-285.
Department for the Environment TR: Measuring Multiple Deprivation at the Small Area Level: The Indices of Deprivation. 2000, London, Department for the Envrionment, Trransport and the Regions
Ware JE, Sherbourne CD: The MOS 36 item short form health survey (SF-36). Conceptual framework and item selection. Medical Care. 1992, 30: 473-481.
Snaith RP, Zigmond AS: The hospital anxiety and depression scale. Acta Psychiatrica Scandinivica. 1983, 67: 361-370.
Sarason I, Carroll C, Maton K: Assessing social support: the social support questionnaire. Journal of Personality and Social Psychology. 1983, 44: 127-139. 10.1037//0022-3514.44.1.127.
Ward R: The impact of subjective age and stigma on older persons. Journal of Gerontology. 1977, 32: 227-232.
Ford G, Ecob R, Hunt K, Macintyre S, West P: Patterns of class inequality in health through the lifespan: class gradients at 15, 35 and 55 years in the west of Scotland. Social Science and Medicine. 1994, 39: 1037-1050. 10.1016/0277-9536(94)90375-1.
SPSS.: v. 11.0 for Windows [program]. 2003, Chicago, Illinois.
STATA.: Statistical Software [program]. 8.0 version. 2003, Texas, College Station
Ritchie J, Lewis J: Qualitative Research Practice. A Guide for Social Scientists. 2003, London, Sage
Barbour RS: The Newfound Credibility of Qualitative Research? Tales of Technical Essentialism and Co-Option. Qualitative Health Research. 2003, 13: 1019-1027. 10.1177/1049732303253331.
Silverman D: Doing qualitative research. 2000, London, Sage
Clayman SE, Maynard DW: Ethnomethodology and conversation analysis. Situated Order: Studies in the Social Organisation of Talk and Embodied Activities. Edited by: Have PT and Psathas G. 1994, Washington, D.C., University Press of America
White M, Moffatt S, Mackintosh J, Howel D, Sandell A, Chadwick T, Deverill M: Randomised controlled trial to evaluate the health effects of welfare rights advice in primary health care: a pilot study. Report to the Department of Health, Policy Research Programme. 2005, Newcastle upon Tyne, University of Newcastle upon Tyne
Moffatt S: "All the difference in the world". A qualitative study of the perceived impact of a welfare rights service provided in primary care. 2004, , University College London
Mallinson S: Listening to reposndents: a qualitative assessment of the Short-Form 36 Health Status Questionnaire. Social Science and Medicine. 2002, 54: 11-21. 10.1016/S0277-9536(01)00003-X.
Brannen J: Mixing Methods: The Entry of Qualitative and Quantitative Approaches into the Research Process. International Journal of Social Research Methodology. 2005, 8: 173-184. 10.1080/13645570500154642.
Green A, Preston J: Editorial: Speaking in Tongues- Diversity in Mixed Methods Research. International Journal of Social Research Methodology. 2005, 8: 167-171. 10.1080/13645570500154626.
Devers KJ: How will we know "good" qualitative research when we see it? Beginning the dialogue in Health Services Research. Health Services Research. 1999, 34: 1153-1188.
CAS PubMed PubMed Central Google Scholar
Pawson R, Tilley N: Realistic Evaluation. 2004, London, Sage
Miles A, Grey JE, Polychronis A, Price N, Melchiorri C: Current thinking in the evidence-based health care debate. Journal of Evaluation in Clinical Practice. 2003, 9: 95-109. 10.1046/j.1365-2753.2003.00438.x.
Pencheon D, Guest C, Melzer D, Gray JAM: Oxford Handbook of Public Health Practice. 2001, Oxford, Oxford University Press
Wanless D: Securing Good Health for the Whole Population. 2004, London, HMSO
Pre-publication history
The pre-publication history for this paper can be accessed here: http://www.biomedcentral.com/1472-6963/6/28/prepub
Download references
Acknowledgements
We wish to thank: Rosemary Bell, Jenny Dover and Nick Whitton from Newcastle upon Tyne City Council Welfare Rights Service; all the participants and general practice staff who took part; and for their extremely helpful comments on earlier drafts of this paper, Adam Sandell, Graham Scambler, Rachel Baker, Carl May and John Bond. We are grateful to referees Alicia O'Cathain and Sally Wyke for their insightful comments. The views expressed in this paper are those of the authors and not necessarily those of the Department of Health.
Author information
Authors and affiliations.
Public Health Research Group, School of Population & Health Sciences, Faculty of Medical Sciences, William Leech Building, Framlington Place, Newcastle upon Tyne, NE2 4HH, UK
Suzanne Moffatt, Martin White, Joan Mackintosh & Denise Howel
You can also search for this author in PubMed Google Scholar
Corresponding author
Correspondence to Suzanne Moffatt .
Additional information
Competing interests.
The author(s) declare that they have no competing interests.
Authors' contributions
SM and MW had the original idea for the study, and with the help of DH, Adam Sandell and Nick Whitton developed the proposal and gained funding. JM collected the data for the quantitative study, SM designed and collected data for the qualitative study. JM, DH and MW analysed the quantitative data, SM analysed the qualitative data. All authors contributed to interpretation of both datasets. SM wrote the first draft of the paper, JM, MW and DH commented on subsequent drafts. All authors have read and approved the final manuscript.
Authors’ original submitted files for images
Below are the links to the authors’ original submitted files for images.
Authors’ original file for figure 1
Rights and permissions.
Open Access This article is published under license to BioMed Central Ltd. This is an Open Access article is distributed under the terms of the Creative Commons Attribution License ( https://creativecommons.org/licenses/by/2.0 ), which permits unrestricted use, distribution, and reproduction in any medium, provided the original work is properly cited.
Reprints and permissions
About this article
Cite this article.
Moffatt, S., White, M., Mackintosh, J. et al. Using quantitative and qualitative data in health services research – what happens when mixed method findings conflict? [ISRCTN61522618]. BMC Health Serv Res 6 , 28 (2006). https://doi.org/10.1186/1472-6963-6-28
Download citation
Received : 29 September 2005
Accepted : 08 March 2006
Published : 01 December 2006
DOI : https://doi.org/10.1186/1472-6963-6-28
Share this article
Anyone you share the following link with will be able to read this content:
Sorry, a shareable link is not currently available for this article.
Provided by the Springer Nature SharedIt content-sharing initiative
- Mixed Method
- Welfare Benefit
- Mixed Method Research
- Divergent Finding
- Financial Vulnerability
BMC Health Services Research
ISSN: 1472-6963
- General enquiries: [email protected]
- Skip to main content
- Skip to FDA Search
- Skip to in this section menu
- Skip to footer links

The .gov means it’s official. Federal government websites often end in .gov or .mil. Before sharing sensitive information, make sure you're on a federal government site.
The site is secure. The https:// ensures that you are connecting to the official website and that any information you provide is encrypted and transmitted securely.
U.S. Food and Drug Administration
- Search
- Menu
- Drug Safety and Availability
CDER Establishes New Quantitative Medicine Center of Excellence
[03/25/2024] FDA’s Center for Drug Evaluation and Research (CDER) is pleased to announce the new CDER Quantitative Medicine (QM) Center of Excellence (CoE).
QM involves the development and application of exposure-based, biological, and quantitative modeling and simulation approaches derived from nonclinical, clinical, and real-world sources to inform drug development, regulatory decision-making, and patient care. These approaches contribute to the totality of understanding of a drug's benefits and risks, helping to advance therapeutic medical product development and inform regulatory decision-making.
The goal of this CoE is to facilitate and coordinate the continuous evolution and consistent application of QM across CDER.
“For decades, CDER has been at the forefront of advancing QM approaches to inform premarket product review and post-market product assessment,” said Patrizia Cavazzoni, M.D., director of CDER. “Given the tremendous growth in QM, we see many opportunities to strengthen collaboration across CDER by centrally coordinating outreach, education, scientific and regulatory policy, to facilitate the consistent use of QM approaches during drug development and to inform regulatory decision making.”
CDER’s QM CoE will be a center-wide effort and will:
- Spearhead QM-related policy development and best practices to facilitate the consistent use of QM approaches during drug development and regulatory assessment
- Facilitate systematic outreach to scientific societies, patient advocacy groups, and other key stakeholders
- Coordinate CDER’s efforts around QM education and training
Drug development is a multifaceted, complex, high-risk endeavor involving a range of scientific, financial, and regulatory challenges. Innovative technologies, tools, and approaches are increasingly utilized to improve drug development efficiency throughout the lifecycle, address complex issues and enable optimized treatments reaching patients. By fostering integration of QM approaches across CDER, the CoE can help advance therapeutic medical product development and inform regulatory decision-making. As a result, the QM CoE is expected to help streamline and accelerate drug development and speed the delivery of safe, effective, therapeutically optimized medicines to the public.
On April 25, CDER will host a public workshop on QM and will share more about the new CoE. To register, please visit Streamlining Drug Development and Improving Public Health through Quantitative Medicine: An Introduction to the CDER Quantitative Medicine Center of Excellence .
Advertisement
Quantitative research assessment: using metrics against gamed metrics
- IM - REVIEW
- Open access
- Published: 03 November 2023
- Volume 19 , pages 39–47, ( 2024 )
Cite this article
You have full access to this open access article
- John P. A. Ioannidis ORCID: orcid.org/0000-0003-3118-6859 1 &
- Zacharias Maniadis 2 , 3
2281 Accesses
14 Altmetric
Explore all metrics
Quantitative bibliometric indicators are widely used and widely misused for research assessments. Some metrics have acquired major importance in shaping and rewarding the careers of millions of scientists. Given their perceived prestige, they may be widely gamed in the current “publish or perish” or “get cited or perish” environment. This review examines several gaming practices, including authorship-based, citation-based, editorial-based, and journal-based gaming as well as gaming with outright fabrication. Different patterns are discussed, including massive authorship of papers without meriting credit (gift authorship), team work with over-attribution of authorship to too many people (salami slicing of credit), massive self-citations, citation farms, H-index gaming, journalistic (editorial) nepotism, journal impact factor gaming, paper mills and spurious content papers, and spurious massive publications for studies with demanding designs. For all of those gaming practices, quantitative metrics and analyses may be able to help in their detection and in placing them into perspective. A portfolio of quantitative metrics may also include indicators of best research practices (e.g., data sharing, code sharing, protocol registration, and replications) and poor research practices (e.g., signs of image manipulation). Rigorous, reproducible, transparent quantitative metrics that also inform about gaming may strengthen the legacy and practices of quantitative appraisals of scientific work.
Similar content being viewed by others
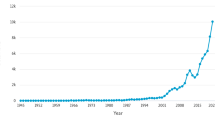
Literature reviews as independent studies: guidelines for academic practice
Sascha Kraus, Matthias Breier, … João J. Ferreira
The Trustworthiness of Content Analysis
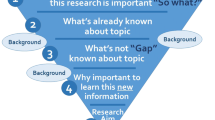
How to Write and Publish a Research Paper for a Peer-Reviewed Journal
Clara Busse & Ella August
Avoid common mistakes on your manuscript.
Introduction
Quantitative bibliometric indicators have become important tools for research assessments. Their advent has elated, frustrated, and haunted investigators, institutions and funding organizations. It is well documented that metrics have both strengths and limitations and that they need to be used with due caution. For example, the Leiden manifesto summarizes such a cautious, judicious approach to the use of bibliometric and other scientometric indicators [ 1 ].
However, misuse and gaming of metrics are rampant [ 2 , 3 ]. The urge to “publish or perish” (or even “get cited or perish”) creates an environment where gaming of metrics is amply incentivized. A whole generation of old and new tricks try to make CVs and their impact look good and impactful—better and more impactful than they really are. Many of these gaming tricks can reach extravagant levels, as in the case of paper mills, massive self-citations, or citation cartels [ 4 , 5 , 6 ].
Concurrently, there are several efforts to try to improve metrics and make them available for all scientists, authors, and papers in ways that allow for proper standardization and more legitimate use [ 7 , 8 , 9 ]. Healthy efforts in bibliometrics and scientometrics should try to counter gaming and flawed practices. In the same way as antivirus software can detect and eliminate software viruses, proper metrics may be used to detect and correct for flawed, biased, gamed metrics. This review examines several gaming practices, including authorship-based, citation-based, editorial-based, and journal-based gaming as well as gaming with outright fabrication. We show how quantitative metrics may be used to detect, correct and hopefully pre-emptively diminish the chances of gaming and other flawed, manipulative research practices. Such an approach may help improve more broadly the standards of worldwide conducted research.
Authorship-based gaming
Authorship of a scientific paper carries credit, responsibility, and accountability. Unfortunately, responsibility and accountability are often forgotten and the focus is placed on how to get maximal credit out of large numbers of coauthored publications (Table 1 ). Gift authorship (honorary authorship) refers to the phenomenon where authors are listed who have not made a sufficient contribution to the work that would justifiably deserve authorship [ 10 , 11 ]. The Vancouver criteria make specific requests for the type of contributions that are necessary for authorship credit. However, it is likely that violations of these criteria are frequent. The exact frequency of gift authorship is difficult to pinpoint, but several surveys suggest high prevalence [ 12 , 13 , 14 , 15 , 16 , 17 , 18 , 19 , 20 ]. Estimates from such surveys may even be gross underestimates, since disclosing and/or admitting gift authorship is a risky disclosure. Gift authorship particularly thrives with specific researcher profiles and situations. The classic stereotype is the department chair placed as an author (often as the senior author) in most/all publications issued from that team, regardless of the level of contribution.
Gift authorship may co-exist with ghost authorship [ 21 , 22 , 23 , 24 , 25 ], where the author(s) who really wrote the paper do not even appear, while one or more gift authors take their place. The classic stereotype is when industry employees do the work and draft manuscripts published with names of academic gift authors, while the industry employees are invisible ghosts. Ghostwriting aims to confer academic prestige to the paper and minimize the perception of sponsor bias.
The advent of the concept of contributorship [ 26 ] has helped to allow provision of more granular information on the type of contributions made by each listed author in a scientific article. Moreover, efforts at standardization of contribution types, in particular the CREDIT system [ 27 , 28 ] may allow some fairer appraisal of contributions. In theory, quantitative approaches can process and analyze in massive scale contributorship from each scientist across his/her papers and place these against the distribution of similar values from other authors in the same field. However, this is meaningful and feasible for papers with standardized (or at least comparable) credit types. More importantly, credit allocation may be gamed in the same way as plain authorship [ 29 ]. There is hardly any way to verify if the listed contributions are genuine, let alone at what level they occurred. Therefore, one may use information on authorship to understand whether some scientists exhibit unusual behavior suggestive of gaming.
In particular, large-scale bibliometric databases [ 30 ] have allowed the detection of hyper-prolific scientists, e.g., those with more than one full article published every 5 days (excluding editorials, commentaries, notes, and letters). This pattern may be particularly suspicious of loose authorship criteria especially when there are massive changes in the productivity of scientists linked to assumption of powerful positions, e.g., one can track that a scientist was in the 20th percentile of productivity in his field, but then moved to the top-0.01% after becoming a powerful administrator (unlikely to have much time for doing research).
In some teams and institutions, inordinate credit does not reflect on a single person, but may diffuse across many team members. This may be common in situations where multi-center work is involved with authorship awarded to many members from each of the participating components or local teams. There is an issue of balance here. On the one hand, credit needs to be given to many people, otherwise those left out would be mistreated. Mistreatment is common and often has other structural societal inequities as contributing factors (e.g., gender bias) [ 31 ]. On the other hand, there may be over-attribution of authorship to too many people, i.e., thin salami slicing of credit. The unfairness becomes more obvious when scientists from a team that over-attributes credit for authorship compete with scientists from teams that are less generous with authorship credit (or are even inappropriately not offering such credit). For example, for the same amount of work, one epidemiological consortium may decide to list 100 authors, while another one may list only 10, and a third one may list only 3.
Quantitative approaches can help sort out the co-author network of each author. They can generate citation metrics that account for co-authorship [ 32 , 33 , 34 ] and/or author position and even for relative contributions (if such information is available) and field-specific practices [ 35 ]. Therefore, two authors may have the same number of authored papers, but they may differ markedly in their relative placement and co-authorship patterns in these papers: one may have many papers as a single author or with few co-authors, while the other may routinely have 50 or more co-authors. On the other hand, they may have the same H-index for overall citations [ 36 ] but they may differ many-fold in a co-authorship-adjusted index, such as Schreiber’s hm index [ 31 ].
Citation-based gaming
Many flawed evaluation systems still emphasize numbers of publications, while this measure should not matter in itself. A good scientist may publish one, few, many or huge number of papers. What should matter is their impact, and citation metrics are used as surrogates of impact. However, these measures can also be gamed, and different metrics differ in their gaming potential.
First, publishing more papers may lead to more citations by itself. Citations are not fully rational, and many scientists cite papers without even having read them [ 37 ]. While some papers are never cited, this proportion has probably decreased over time and the frequent quote that half of the papers are never cited is a myth [ 38 ]. One may penalize publishing large numbers of papers and some have even argued that there should be a cap on how many total words a scientist can publish [ 39 ]. Such penalizing and capping is ill advised, however. It may intensify selective reporting and publication bias, as scientists would struggle to publish only extreme, nice-looking results that may attract more attention. It is probably more appropriate not to pay any attention to the number of publications (except for the extreme tail of hyper-prolific authors) and allow scientists to disseminate their work in whatever way and volume they deem most appropriate. However, one may examine other quantitative metrics such as citations per paper, and place these metrics in a percentile ranking against papers from the same field, e.g., a scientist may have 100 publications and 1000 citations and be at the 25th percentile of his field for citations per paper (1000/100 = 10). Another scientist may also have 1000 citations, but with 1000 publications may be at the bottom 0.1% percentile for citations per paper (1000/1000 = 1), suggesting he/she is publishing very trivial work.
Self-citation is a classic mechanism that increases one’s citation count [ 40 , 41 ]. Self-citations can be defined in different ways. A strict definition includes references to one’s own work. A more inclusive definition includes also references to one’s work by any of the co-authors of that work. Many self-citations are entirely appropriate [ 42 ]. Science requires continuity and linking of the current work to relevant previous work. In fact, avoidance of such linking and not use of self-citations would be inappropriate and even ethically reprehensible—e.g., it may mislead that some work is entirely novel, and/or could lead to undeclared self-plagiarism [ 43 ]. Self-citations may also have both a direct effect in increasing total citations and an indirect effect—when a work is mentioned more frequently, other scientists may notice it and cite it as well [ 44 ] (Table 1 ).
Self-citations would require an impossibly strenuous in-depth evaluation to examine whether each of them is inappropriate or not. However, centralized bibliometric databases [ 5 , 45 ] can allow placing the proportion of self-citations for an author as a percentile ranking against the self-citations of other authors in the same scientific field. Extreme outliers (adjusting for field and possibly also age [ 5 ]) may be characteristic of gaming behavior (Table 2 ).
Self-citation practices may take also complex forms. Occasionally, the authors may collude to cite each other’s works, even though they are not co-authors. Such citation cartels (citation farms) usually involve a small number of authors. Flow of citations may not necessarily be equally towards all members of the cartel. For instance, one or a few members may be cited, while the others may enjoy other repayments. The members of the citation farm may be in different institutions and countries. Again, quantitative metrics is the best way to diagnose a cartel. Usually, a large number of scientists cite one author’s work and citations from each citing author account for a very small portion of the total citations. Conversely, in a citation farm, a handful of citing authors may account for > 50% or even > 80% of the citations received.
Some of the inappropriate self-citing or citation farming behavior may even aim to inflate selectively some specific citation metric considered most important. For example, the Hirsch h-index has enjoyed inordinate popularity since its introduction in 2005 [ 35 ]. H-index can be more easily gamed than the number of total citations. Self-citers preferentially (“strategically”) cite papers that readily boost the H-index [ 44 ]. Again, quantitative metrics can help detect this behavior, for instance by examining the ratio of total citations over the square of the H-index. Average values for this ratio are about 4 [ 35 ]. Very small values suggest that citations have been targeted to papers that boost the H-index while total citations are relatively more difficult to manipulate.
Editorial-based gaming
Journals may not treat equally all the authors who submit their work to them. Some authors may be favored. Proportion of submissions accepted may vary markedly across authors. Often this is entirely normal: some scientists truly submit better work than others. However, difficulties arise when the submitting and publishing authors are directly involved with the journal, as editors-in-chief or as staff members. With the rapid proliferation of journals, including mega-journals [ 46 ], the numbers of editors-in-chief, guest editors and staff members has also increased markedly.
Editors are fully entitled (and even encouraged as part of their job) to write editorials and commentaries on topics that they consider important. This activity is fully legitimate. These editorial pieces may go through no or very limited review and get published quickly on hot matters. Some high-profile journals, such as Nature, Science, and BMJ have numerous staff writers and science journalists (as staff or free lancers) who write news and feature stories, often massively. An empirical analysis [ 47 ] has shown that some of these writers have published 200–2000 papers in these venues where a scientist would consider a career milestone to publish even a single article. Most of these authors are usually not competing in the world of academia. However, exceptions do occur where editorialists publishing massively in one journal may be academics. Other editors may give up their editorial career at some point and move to competitive academia. Another concern is that these editorial publications often have no disclosures of potential conflicts of interest [ 47 ]. Some editors have great power to shape science narratives in good or bad ways. Quantitative metrics can separate the impact of authors due to non-peer-reviewed editorial material versus peer-reviewed full articles.
A more contentious situation arises when an editor-in-chief publishes original full articles in his/her own journal. While this is possible and not reproachable if done sporadically (especially if the paper is handled by another editor), some authors raise concerns about this practice, when it is common. Empirical analyses have shown the prevalence of editorial nepotism practices [ 48 ]: in a survey of 5,468 biomedical journals, 5% of the journals had > 10.6% of their published articles authored by a single person, typically the editor-in-chief and/or other highly preferred members of the editorial board. Quantitative analyses can map the distribution of papers of an author across different journals and identify if there is an inordinate concentration of full, original papers in journals where the author is editor-in-chief.
Journal-based gaming
Most of the gaming at the level of journals involves efforts to boost the journal impact factor [ 49 ]. Detailed description of the multiple well-known problems and gaming practices for this metric is beyond the scope of this paper. Nevertheless, many of the gaming practices used for single scientists have equivalents for gaming at the journal level, e.g., coercive journal self-citation (requests by the editor to cite other papers published by the journal) and citation cartels involving journals rather than single authors (“citation stocking”) [ 50 ]. Multiple processes and gaming tools can be detected by bibliometric analysis at the level of journal self-citation and co-citation patterns. Journal impact factor manipulation may also involve gaming gains for specific researchers as well, in particular for the editors, as described above. Journals with higher impact factors get cited more frequently, even when it comes to papers that are identically published in other journals (e.g., reporting guideline articles) [ 51 ].
Gaming with outright fabrication
The gaming practices described so far typically do not have to involve fabrication. The gamed published and cited material is real, even though its quality may be suboptimal, given the inflated productivity. However, there are also escalating gaming practices that involve entirely fabricated work.
In paper mills, a for-profit company produces papers (typically fraudulent, fabricated ones), which it sells to scientists who want to buy authorship slots in them. The papers are for sale before submission or even after acceptance [ 52 , 53 , 54 ]. An increasing proportion of retractions in the last 7 years has been for paper mill-generated articles [ 55 ]. It is unknown though whether this may be just the tip of the iceberg and these retracted papers are those where the fabrication is more egregious and thus readily discernible. The advent of more powerful large language models may make the paper mill products more sophisticated and difficult to identify [ 56 ]. Software is evolving to detect use of such large language models, but it is unclear whether such detection software would be able to catch up. Involvement of artificial intelligence in writing scientific papers is an evolving challenge for both genuine and fraudulent papers. Several journals have tried to tackle this challenge, but reactions have not been uniform [ 57 , 58 , 59 , 60 ].
There are many other egregious evolutions in the publishing world, a consequence of publish-or-perish pressure. Predatory journals (journals publishing content for a fee but practically without peer review) are widely prevalent, but their exact prevalence is difficult to ascertain, given the difficulty to agree on which journals are predatory [ 61 , 62 , 63 ]. Some of the most notorious phenomena are hijacked journals and publication of spurious content. Hijacking happens when a site belonging formerly to a discontinued serious journal is taken over by a predator who uses the name and the prestige of the previous journal for operating the predatory business [ 64 ]. Some papers also get published in journals with totally unrelated aims/mission/subject matter coverage; such spurious content is indication for fraudulent behavior (e.g., may be associated with both paper mills and predatory publishing).
Again, bibliometric, quantitative indicators can be used to place the prevalence of such behaviors in publication corpora of single authors, teams, institutions, and journals into perspective. Indicators may include frequency of documented paper mill products, hints of inappropriate use of large language models, hints of predatory or other inappropriate journal behavior (e.g., percentage of papers published in journals that lost their journal impact factor), and percentage of papers with content unrelated to the other published content of the journal.
Even in very serious journals, the proportion of fabricated papers may be increasing over time. John Carlisle, an editor of a prestigious specialty journal (Anesthesia) requested the raw data of over 150 randomized trials submitted to his journal and concluded that in 30–40% of them the data were so messed up and/or improbable that he called these trials zombie trials [ 65 , 66 ]. Zombie trials tend to come from particular institutions and countries. Such trials have demanding clinical research designs that are difficult to perform, let alone perform massively. Quantitative bibliometric analysis can allow the detection of sudden, massive production of papers with demanding study designs for which a scientist or team have no prior tradition and resources to run, e.g., massive sudden production of randomized trials in some institutions in less developed countries [ 67 ].
Metrics of best research practices and of poor research practices
Most bibliometric and scientometric indicators to-date have focused on counting numbers and citations of scholarly publications. However, it is very important to capture also information on research practices, good and bad. These research practices may in fact often be well reflected in these publication corpora. For example, good research practices include wide data sharing, code sharing, protocol registration, and replications. It is currently possible to capture for each scientist how often he/she used these standards in his/her published work. For example, a free, publicly available resource covers all the open-access biomedical literature for these indicators [ 68 ].
It is also possible to capture systematically the use of several poor research practices. For example, image manipulation is a common problem across many types of investigation. There are already appraisal efforts that have tried to generate data on signs of image manipulation across large publication corpora rather than doing this exercise painstakingly one paper at a time [ 69 , 70 ].
Another potential sign of poor research practices is retractions. At least one science-wide assessment of top-cited scientists currently excludes from consideration those with retracted papers based on the inclusive Retraction Watch database ( https://retractionwatch.com/2022/11/15/why-misconduct-could-keep-scientists-from-earning-highly-cited-researcher-designations-and-how-our-database-plays-a-part/ ). The majority of retractions may signal some sort of misconduct. However, in a non-negligible proportion of cases, they may actually signal honest acknowledgment of honest error—a sign of a good scientist that should be praised and encouraged if we wish to see better self-correction in the scientific record. Therefore, when retractions are present, they need to be scrutinized on a case-by-case basis regarding their provenance and rationale. Making wider use of available resources, such as the Retraction Watch database, and improving and standardizing the retraction notices [ 71 ] may help add another important dimension to research appraisals.
Putting it together
Table 3 lists a number of quantitative metrics and indicators that are currently readily available (or can be relatively easily obtained) from centralized databases. The examples of scientists shown are entirely hypothetical and do not correspond to specific real individuals; they are provided for illustrative reasons. All three scientists are highly cited, in the top 1.8%, 0.9% and 0.7% of their scientific domain, respectively. However, two of the three scientists show problematic markers and/or score very low for markers of transparency and reproducibility.
Efforts should be devoted to make such datasets more comprehensive, covering routinely such indicators across all scientific investigation, and with percentile rankings adjusted for scientific field. Each metric should be used with full knowledge of its strengths and limitations. Attention should focus particularly on extreme outliers; modest differences between two authors should not be seen as proof that one’s work is necessarily superior to another. Even with extreme values, metrics should not be used to superficially and hastily heroize or demonize people. For example, very high productivity may reflect some of the best, committed, devoted scientists; some recipients of massive gift authorships; and some outright fraudsters. While single metrics may not suffice to fully reliably differentiate these groups, the complete, multi-dimensional picture usually can clearly separate who is who.
Data availability
Not applicable.
Hicks D, Wouters P, Waltman L, De Rijcke S, Rafols I (2015) Bibliometrics: the Leiden Manifesto for research metrics. Nature 520(7548):429–431
Article PubMed Google Scholar
Ioannidis JP, Boyack KW (2020) Citation metrics for appraising scientists: misuse, gaming and proper use. Med J Aust 212(6):247-249.e1
Fire M, Guestrin C (2019) Over-optimization of academic publishing metrics: observing Goodhart’s Law in action. GigaScience 8(6):giz053
Article PubMed PubMed Central Google Scholar
Christopher J (2021) The raw truth about paper mills. FEBS Lett 595(13):1751–1757
Article CAS PubMed Google Scholar
Van Noorden R, Chawla DS (2019) Hundreds of extreme self-citing scientists revealed in new database. Nature 572(7771):578–580
Fister I Jr, Fister I, Perc M (2016) Towards the discovery of citation cartels in citation networks. Front Phys 4:00049
Article Google Scholar
Ioannidis JPA, Baas J, Klavans R, Boyack KW (2019) A standardized citation metrics author database annotated for scientific field. PLoS Biol 17(8):e3000384
Article CAS PubMed PubMed Central Google Scholar
Hutchins BI, Yuan X, Anderson JM, Santangelo GM (2016) Relative citation ratio (RCR): a new metric that uses citation rates to measure influence at the article level. PLoS Biol 14(9):e1002541
Fortunato S, Bergstrom CT, Börner K, Evans JA, Helbing D, Milojević S et al (2018) Science of science. Science 359(6379):eaao0185
Smith J (1994) Gift authorship: a poisoned chalice? BMJ 309(6967):1456–1457
Moffatt B (2011) Responsible authorship: why researchers must forgo honorary authorship. Account Res 18(2):76–90. https://doi.org/10.1080/08989621.2011.557297
Goodman N (1994) Survey of fulfilment of criteria for authorship in published medical research. BMJ 309:1482
Rajasekaran S, Shan RL, Finnoff JT (2014) Honorary authorship: frequency and associated factors in physical medicine and rehabilitation research articles. Arch Phys Med Rehabil 95(3):418–428. https://doi.org/10.1016/j.apmr.2013.09.024
Al-Herz W, Haider H, Al-Bahhar M, Sadeq A (2014) Honorary authorship in biomedical journals: how common is it and why does it exist? J Med Ethics 40(5):346–348. https://doi.org/10.1136/medethics-2012-101311
Kovacs J (2013) Honorary authorship epidemic in scholarly publications? How the current use of citation-based evaluative metrics make (pseudo)honorary authors from honest contributors of every multi-author article. J Med Ethics 39(8):509–512. https://doi.org/10.1136/medethics-2012-100568
Eisenberg RL, Ngo L, Boiselle PM, Bankier AA (2011) Honorary authorship in radiologic research articles: assessment of frequency and associated factors. Radiology 259(2):479–486. https://doi.org/10.1148/radiol.11101500
Kayapa B, Jhingoer S, Nijsten T, Gadjradj PS (2018) The prevalence of honorary authorship in the dermatological literature. Br J Dermatol 178(6):1464–1465. https://doi.org/10.1111/bjd.16678
Shah A, Rajasekaran S, Bhat A, Solomon JM (2018) Frequency and factors associated with honorary authorship in Indian biomedical journals: analysis of papers published from 2012 to 2013. J Empir Res Hum Res Ethics 13(2):187–195. https://doi.org/10.1177/1556264617751475
Elliott KC, Settles IH, Montgomery GM, Brassel ST, Cheruvelil KS, Soranno PA (2017) Honorary authorship practices in environmental science teams: structural and cultural factors and solutions. Account Res 24(2):80–98. https://doi.org/10.1080/08989621.2016.1251320
Eisenberg RL, Ngo LH, Bankier AA (2014) Honorary authorship in radiologic research articles: do geographic factors influence the frequency? Radiology 271(2):472–478. https://doi.org/10.1148/radiol.13131710
Gülen S, Fonnes S, Andresen K, Rosenberg J (2020) More than one-third of Cochrane reviews had gift authors, whereas ghost authorship was rare. J Clin Epidemiol 128:13–19. https://doi.org/10.1016/j.jclinepi.2020.08.004
Vera-Badillo FE, Napoleone M, Krzyzanowska MK, Alibhai SM, Chan AW, Ocana A, Templeton AJ, Seruga B, Amir E, Tannock IF (2016) Honorary and ghost authorship in reports of randomised clinical trials in oncology. Eur J Cancer 66:1–8. https://doi.org/10.1016/j.ejca.2016.06.023
Wislar JS, Flanagin A, Fontanarosa PB, Deangelis CD (2011) Honorary and ghost authorship in high impact biomedical journals: a cross sectional survey. BMJ 25(343):d6128. https://doi.org/10.1136/bmj.d6128
Hargreaves S (2007) Ghost authorship of industry funded drug trials is common, say researchers. BMJ 334(7587):223. https://doi.org/10.1136/bmj.39108.653750.DB
Gøtzsche PC, Hróbjartsson A, Johansen HK, Haahr MT, Altman DG, Chan AW (2007) Ghost authorship in industry-initiated randomised trials. PLoS Med 4(1):e19. https://doi.org/10.1371/journal.pmed.0040019
Rennie D, Yank V, Emanuel L (1997) When authorship fails. A proposal to make contributors accountable. JAMA 278(7):579–585
Brand A, Allen L, Altman M et al (2015) Beyond authorship: attribution, contribution, collaboration, and credit. Learn Publ 28:151–155. https://doi.org/10.1087/20150211
McNutt MK, Bradford M, Drazen JM et al (2018) Transparency in authors’ contributions and responsibilities to promote integrity in scientific publication. Proc Natl Acad Sci USA 115:2557–2560
Ilakovac V, Fister K, Marusic M, Marusic A (2007) Reliability of disclosure forms of authors’ contributions. Can Med Assoc J 176(1):41–46. https://doi.org/10.1503/cmaj.060687
Ioannidis JPA, Klavans R, Boyack KW (2018) Thousands of scientists publish a paper every five days. Nature 561(7722):167–169. https://doi.org/10.1038/d41586-018-06185-8
Ross MB, Glennon BM, Murciano-Goroff R, Berkes EG, Weinberg BA, Lane JI (2022) Women are credited less in science than men. Nature 608(7921):135–145. https://doi.org/10.1038/s41586-022-04966-w
Schreiber M (2008) A modification of the h-index: the hm-index accounts for multi-authored manuscripts. J Informatics 2:211–216
Google Scholar
Batista PD, Campiteli MG, Kinouchi O, Martinez AS (2006) Is it possible to compare researchers with different scientific interests? Scientometrics 68:179–189
Article CAS Google Scholar
Egghe L (2008) Mathematical theory of the h- and g-index in case of fractional counting of authorship. J Am Soc Inform Sci Technol 59:1608–1616
Frandsen TF, Nicolaisen J (2010) What is in a name? Credit assignment practices in different disciplines. J Informetrics 4:608–617
Hirsch JE (2005) An index to quantify an individual’s scientific research output. Proc Natl Acad Sci USA 102(46):16569–16572. https://doi.org/10.1073/pnas.0507655102
Simkin MV, Roychowdhury VP. Read before you cite! https://arxiv.org/abs/cond-mat/0212043 .
Nicolaisen J, Frandsen TF (2019) Zero impact: a large-scale study of uncitedness. Scientometrics 119(2):1227–1254
Martinson B (2017) Give researchers a lifetime word limit. Nature 550:303. https://doi.org/10.1038/550303a
Mishra S, Fegley BD, Diesner J, Torvik VI (2018) Self-citation is the hallmark of productive authors, of any gender. PLoS ONE 13(9):e0195773. https://doi.org/10.1371/journal.pone.0195773
Ioannidis JP (2015) A generalized view of self-citation: direct, co-author, collaborative, and coercive induced self-citation. J Psychosom Res 78(1):7–11. https://doi.org/10.1016/j.jpsychores.2014.11.008
Fowler J, Aksnes D (2007) Does self-citation pay? Scientometrics 72(3):427–437
Bruton SV (2014) Self-plagiarism and textual recycling: legitimate forms of research misconduct. Account Res 21(3):176–197
Bartneck C, Kokkelmans S (2011) Detecting h-index manipulation through self-citation analysis. Scientometrics 87(1):85–98. https://doi.org/10.1007/s11192-010-0306-5
Ioannidis JP, Boyack KW, Baas J (2020) Updated science-wide author databases of standardized citation indicators. PLoS Biol 18(10):e3000918
Ioannidis JP, Pezzullo AM, Boccia S (2023) The rapid growth of mega-journals: threats and opportunities. JAMA 329(15):1253–1254
Ioannidis JPA (2023) Prolific non-research authors in high impact scientific journals: meta-research study. Scientometrics 128(5):3171–3184. https://doi.org/10.1007/s11192-023-04687-5
Scanff A, Naudet F, Cristea IA, Moher D, Bishop DVM, Locher C (2021) A survey of biomedical journals to detect editorial bias and nepotistic behavior. PLoS Biol 19(11):e3001133. https://doi.org/10.1371/journal.pbio.3001133
Ioannidis JPA, Thombs BD (2019) A user’s guide to inflated and manipulated impact factors. EurJ Clin Invest 49(9):e13151. https://doi.org/10.1111/eci.13151
Van Noorden R (2013) Brazilian citation scheme outed. Nature 500:510–511. https://doi.org/10.1038/500510a
Perneger TV (2010) Citation analysis of identical consensus statements revealed journal-related bias. J Clin Epidemiol 63(6):660–664. https://doi.org/10.1016/j.jclinepi.2009.09.012
Christopher J (2021) The raw truth about paper mills. FEBS Lett 595:1751–1757
Else H, Van Noorden R (2021) The fight against fake-paper factories that churn out sham science. Nature 591:516–519
Abalkina A. Publication and collaboration anomalies in academic papers originating from a paper mill: evidence from a Russia-based paper mill. arXiv preprint arXiv:2112.13322 . 2021 Dec 26.
Candal-Pedreira C, Ross JS, Ruano-Ravina A, Egilman DS, Fernández E, Pérez-Ríos M (2022) Retracted papers originating from paper mills: cross sectional study. BMJ 28(379):e071517. https://doi.org/10.1136/bmj-2022-071517
Chen L, Chen P, Lin Z (2020) Artificial intelligence in education: a review. IEEE Access 8:75264–75278. https://doi.org/10.1109/ACCESS.2020.2988510
Flanagin A, Kendall-Taylor J, Bibbins-Domingo K (2023) Guidance for authors, peer reviewers, and editors on use of AI, language models, and chatbots. JAMA 330(8):702–703. https://doi.org/10.1001/jama.2023.12500
Flanagin A, Bibbins-Domingo K, Berkwits M, Christiansen SL (2023) Nonhuman “Authors” and implications for the integrity of scientific publication and medical knowledge. JAMA 329(8):637–639. https://doi.org/10.1001/jama.2023.1344
Brainard J (2023) Journals take up arms against AI-written text. Science 379(6634):740–741. https://doi.org/10.1126/science.adh2762
Thorp HH (2023) ChatGPT is fun, but not an author. Science 379(6630):313. https://doi.org/10.1126/science.adg7879
Ng JY, Haynes RB (2021) “Evidence-based checklists” for identifying predatory journals have not been assessed for reliability or validity: an analysis and proposal for moving forward. J Clin Epidemiol 138:40–48. https://doi.org/10.1016/j.jclinepi.2021.06.015
Cukier S, Lalu M, Bryson GL, Cobey KD, Grudniewicz A, Moher D (2020) Defining predatory journals and responding to the threat they pose: a modified Delphi consensus process. BMJ Open 10(2):e035561. https://doi.org/10.1136/bmjopen-2019-035561
Grudniewicz A, Moher D, Cobey KD, Bryson GL, Cukier S, Allen K, Ardern C, Balcom L, Barros T, Berger M, Ciro JB, Cugusi L, Donaldson MR, Egger M, Graham ID, Hodgkinson M, Khan KM, Mabizela M, Manca A, Milzow K, Mouton J, Muchenje M, Olijhoek T, Ommaya A, Patwardhan B, Poff D, Proulx L, Rodger M, Severin A, Strinzel M, Sylos-Labini M, Tamblyn R, van Niekerk M, Wicherts JM, Lalu MM (2019) Predatory journals: no definition, no defence. Nature 576(7786):210–212. https://doi.org/10.1038/d41586-019-03759-y
Andoohgin Shahri M, Jazi MD, Borchardt G, Dadkhah M (2018) Detecting hijacked journals by using classification algorithms. Sci Eng Ethics 24(2):655–668. https://doi.org/10.1007/s11948-017-9914-2
Ioannidis JPA (2021) Hundreds of thousands of zombie randomised trials circulate among us. Anaesthesia 76(4):444–447. https://doi.org/10.1111/anae.15297
Carlisle JB (2021) False individual patient data and zombie randomised controlled trials submitted to Anaesthesia. Anaesthesia 76(4):472–479. https://doi.org/10.1111/anae.15263
Mol BW, Ioannidis JPA (2023) How do we increase the trustworthiness of medical publications? Fertil Steril 120(3 Pt 1):412–414. https://doi.org/10.1016/j.fertnstert.2023.02.023
Serghiou S, Contopoulos-Ioannidis DG, Boyack KW, Riedel N, Wallach JD, Ioannidis JPA (2021) Assessment of transparency indicators across the biomedical literature: how open is open? PLoS Biol 19(3):e3001107
Bik EM, Casadevall A, Fang FC (2016) The prevalence of inappropriate image duplication in biomedical research publications. MBio 7(3):e00809-16. https://doi.org/10.1128/mBio.00809-16
Fanelli D, Costas R, Fang FC, Casadevall A, Bik EM (2019) Testing hypotheses on risk factors for scientific misconduct via matched-control analysis of papers containing problematic image duplications. Sci Eng Ethics 25(3):771–789
Hwang SY, Yon DK, Lee SW, Kim MS, Kim JY, Smith L, et al. Causes for retraction in the biomedical literature: a systematic review of studies of retraction notices. J Korean Med Sci. 2023;38(41):e33.
Download references
Acknowledgements
Maniadis would like to thank Constantine Sedikides and Marios Demetriadis for inspiring discussions in this research policy area.
The work of John Ioannidis is supported by an unrestricted gift from Sue and Bob O’Donnell to Stanford. Zacharias Maniadis is supported by the project SInnoPSis, funded by Horizon 2020 under grant agreement ID: 857636.
Author information
Authors and affiliations.
Departments of Medicine, of Epidemiology and Population Health, of Biomedical Data Science, and of Statistics, and Meta-Research Innovation Center at Stanford (METRICS), Stanford University, SPRC, MSOB X306, 1265 Welch Rd, Stanford, CA, 94305, USA
John P. A. Ioannidis
SInnoPSis (Science and Innovation Policy and Studies) Unit, Department of Economics, University of Cyprus, Nicosia, Cyprus
Zacharias Maniadis
Department of Economics, University of Southampton, Southampton, UK
You can also search for this author in PubMed Google Scholar
Contributions
JI and ZM contributed equally to conceptual development and writing of the article.
Corresponding author
Correspondence to John P. A. Ioannidis .
Ethics declarations
Conflict of interests.
Ioannidis has coauthored several papers that use commercial bibliometric resources (Scopus) to create freely publicly available science-wide databases, but has received no financial remuneration for this work. Maniadis reports no conflicts of interests related to this study.
Human and animal rights
This article does not include any primary data from human or animal research as it is a review article.
Informed consent
Informed consent for this study there were no participants and thus no informed consent was required.
Additional information
Publisher's note.
Springer Nature remains neutral with regard to jurisdictional claims in published maps and institutional affiliations.
Rights and permissions
Open Access This article is licensed under a Creative Commons Attribution 4.0 International License, which permits use, sharing, adaptation, distribution and reproduction in any medium or format, as long as you give appropriate credit to the original author(s) and the source, provide a link to the Creative Commons licence, and indicate if changes were made. The images or other third party material in this article are included in the article's Creative Commons licence, unless indicated otherwise in a credit line to the material. If material is not included in the article's Creative Commons licence and your intended use is not permitted by statutory regulation or exceeds the permitted use, you will need to obtain permission directly from the copyright holder. To view a copy of this licence, visit http://creativecommons.org/licenses/by/4.0/ .
Reprints and permissions
About this article
Ioannidis, J.P.A., Maniadis, Z. Quantitative research assessment: using metrics against gamed metrics. Intern Emerg Med 19 , 39–47 (2024). https://doi.org/10.1007/s11739-023-03447-w
Download citation
Received : 20 September 2023
Accepted : 26 September 2023
Published : 03 November 2023
Issue Date : January 2024
DOI : https://doi.org/10.1007/s11739-023-03447-w
Share this article
Anyone you share the following link with will be able to read this content:
Sorry, a shareable link is not currently available for this article.
Provided by the Springer Nature SharedIt content-sharing initiative
- Bibliometrics
- Research assessment
- Gift authorship
- Self-citations
- Impact factor
- Find a journal
- Publish with us
- Track your research
CDER Establishes New Quantitative Medicine Center of Excellence
The FDA on Monday announced creation of the new Quantitative Medicine (QM) Center of Excellence to facilitate and coordinate the continuous evolution and consistent application of QM across CDER.
QM relates to the development and application of exposure-based, biological, and quantitative modeling and simulation approaches derived from nonclinical, clinical, and real-world sources to inform drug development, regulatory decision-making, and patient care. These approaches contribute to the totality of understanding of a drug’s benefits and risks, helping to advance the drug through the regulatory process.
CDER’s Quantitative Medicine Center of Excellence will, among other things, lead QM-related policy development and best practices to facilitate the use of QM during drug development and regulatory assessment and facilitate outreach to scientific societies, patient advocacy groups, and other key stakeholders. On April 25 CDER will host a public workshop on the QM Center of Excellence.
Register for the workshop here .
To read the whole story, click here to subscribe.
Related Topics
Upcoming Events
Managing data and documentation for fda inspections and remote assessments, integrating regulatory intelligence into supplier management, magi 2024: the clinical research conference, a masterclass on effective 483 responses, 2024 avoca quality consortium summit, featured products.
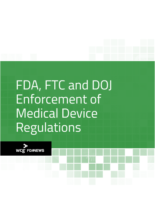
FDA, FTC and DOJ Enforcement of Medical Device Regulations
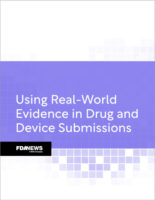
Using Real-World Evidence in Drug and Device Submissions
Featured stories, six fda warning letters prompted by high levels of lidocaine in otc numbing products, comments on benefit-risk of phthalates in medical devices sought by ec, customs teams with fda to improve supply chain traceability, sen. sanders calls for drastically reduced ozempic pricing, rips novo nordisk, the revised ich e8: a guide to new clinical trial requirements.
Advertisement
Supported by
Use of Abortion Pills Has Risen Significantly Post Roe, Research Shows

By Pam Belluck
Pam Belluck has been reporting about reproductive health for over a decade.
- Share full article
On the eve of oral arguments in a Supreme Court case that could affect future access to abortion pills, new research shows the fast-growing use of medication abortion nationally and the many ways women have obtained access to the method since Roe v. Wade was overturned in June 2022.
The Details

A study, published on Monday in the medical journal JAMA , found that the number of abortions using pills obtained outside the formal health system soared in the six months after the national right to abortion was overturned. Another report, published last week by the Guttmacher Institute , a research organization that supports abortion rights, found that medication abortions now account for nearly two-thirds of all abortions provided by the country’s formal health system, which includes clinics and telemedicine abortion services.
The JAMA study evaluated data from overseas telemedicine organizations, online vendors and networks of community volunteers that generally obtain pills from outside the United States. Before Roe was overturned, these avenues provided abortion pills to about 1,400 women per month, but in the six months afterward, the average jumped to 5,900 per month, the study reported.
Overall, the study found that while abortions in the formal health care system declined by about 32,000 from July through December 2022, much of that decline was offset by about 26,000 medication abortions from pills provided by sources outside the formal health system.
“We see what we see elsewhere in the world in the U.S. — that when anti-abortion laws go into effect, oftentimes outside of the formal health care setting is where people look, and the locus of care gets shifted,” said Dr. Abigail Aiken, who is an associate professor at the University of Texas at Austin and the lead author of the JAMA study.
The co-authors were a statistics professor at the university; the founder of Aid Access, a Europe-based organization that helped pioneer telemedicine abortion in the United States; and a leader of Plan C, an organization that provides consumers with information about medication abortion. Before publication, the study went through the rigorous peer review process required by a major medical journal.
The telemedicine organizations in the study evaluated prospective patients using written medical questionnaires, issued prescriptions from doctors who were typically in Europe and had pills shipped from pharmacies in India, generally charging about $100. Community networks typically asked for some information about the pregnancy and either delivered or mailed pills with detailed instructions, often for free.
Online vendors, which supplied a small percentage of the pills in the study and charged between $39 and $470, generally did not ask for women’s medical history and shipped the pills with the least detailed instructions. Vendors in the study were vetted by Plan C and found to be providing genuine abortion pills, Dr. Aiken said.
The Guttmacher report, focusing on the formal health care system, included data from clinics and telemedicine abortion services within the United States that provided abortion to patients who lived in or traveled to states with legal abortion between January and December 2023.
It found that pills accounted for 63 percent of those abortions, up from 53 percent in 2020. The total number of abortions in the report was over a million for the first time in more than a decade.
Why This Matters
Overall, the new reports suggest how rapidly the provision of abortion has adjusted amid post-Roe abortion bans in 14 states and tight restrictions in others.
The numbers may be an undercount and do not reflect the most recent shift: shield laws in six states allowing abortion providers to prescribe and mail pills to tens of thousands of women in states with bans without requiring them to travel. Since last summer, for example, Aid Access has stopped shipping medication from overseas and operating outside the formal health system; it is instead mailing pills to states with bans from within the United States with the protection of shield laws.
What’s Next
In the case that will be argued before the Supreme Court on Tuesday, the plaintiffs, who oppose abortion, are suing the Food and Drug Administration, seeking to block or drastically limit the availability of mifepristone, the first pill in the two-drug medication abortion regimen.
The JAMA study suggests that such a ruling could prompt more women to use avenues outside the formal American health care system, such as pills from other countries.
“There’s so many unknowns about what will happen with the decision,” Dr. Aiken said.
She added: “It’s possible that a decision by the Supreme Court in favor of the plaintiffs could have a knock-on effect where more people are looking to access outside the formal health care setting, either because they’re worried that access is going away or they’re having more trouble accessing the medications.”
Pam Belluck is a health and science reporter, covering a range of subjects, including reproductive health, long Covid, brain science, neurological disorders, mental health and genetics. More about Pam Belluck
Thank you for visiting nature.com. You are using a browser version with limited support for CSS. To obtain the best experience, we recommend you use a more up to date browser (or turn off compatibility mode in Internet Explorer). In the meantime, to ensure continued support, we are displaying the site without styles and JavaScript.
- View all journals
- My Account Login
- Explore content
- About the journal
- Publish with us
- Sign up for alerts
- Open access
- Published: 26 March 2024
Predicting and improving complex beer flavor through machine learning
- Michiel Schreurs ORCID: orcid.org/0000-0002-9449-5619 1 , 2 , 3 na1 ,
- Supinya Piampongsant 1 , 2 , 3 na1 ,
- Miguel Roncoroni ORCID: orcid.org/0000-0001-7461-1427 1 , 2 , 3 na1 ,
- Lloyd Cool ORCID: orcid.org/0000-0001-9936-3124 1 , 2 , 3 , 4 ,
- Beatriz Herrera-Malaver ORCID: orcid.org/0000-0002-5096-9974 1 , 2 , 3 ,
- Christophe Vanderaa ORCID: orcid.org/0000-0001-7443-5427 4 ,
- Florian A. Theßeling 1 , 2 , 3 ,
- Łukasz Kreft ORCID: orcid.org/0000-0001-7620-4657 5 ,
- Alexander Botzki ORCID: orcid.org/0000-0001-6691-4233 5 ,
- Philippe Malcorps 6 ,
- Luk Daenen 6 ,
- Tom Wenseleers ORCID: orcid.org/0000-0002-1434-861X 4 &
- Kevin J. Verstrepen ORCID: orcid.org/0000-0002-3077-6219 1 , 2 , 3
Nature Communications volume 15 , Article number: 2368 ( 2024 ) Cite this article
39k Accesses
749 Altmetric
Metrics details
- Chemical engineering
- Gas chromatography
- Machine learning
- Metabolomics
- Taste receptors
The perception and appreciation of food flavor depends on many interacting chemical compounds and external factors, and therefore proves challenging to understand and predict. Here, we combine extensive chemical and sensory analyses of 250 different beers to train machine learning models that allow predicting flavor and consumer appreciation. For each beer, we measure over 200 chemical properties, perform quantitative descriptive sensory analysis with a trained tasting panel and map data from over 180,000 consumer reviews to train 10 different machine learning models. The best-performing algorithm, Gradient Boosting, yields models that significantly outperform predictions based on conventional statistics and accurately predict complex food features and consumer appreciation from chemical profiles. Model dissection allows identifying specific and unexpected compounds as drivers of beer flavor and appreciation. Adding these compounds results in variants of commercial alcoholic and non-alcoholic beers with improved consumer appreciation. Together, our study reveals how big data and machine learning uncover complex links between food chemistry, flavor and consumer perception, and lays the foundation to develop novel, tailored foods with superior flavors.
Similar content being viewed by others
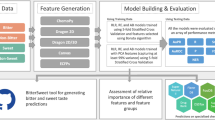
BitterSweet: Building machine learning models for predicting the bitter and sweet taste of small molecules
Rudraksh Tuwani, Somin Wadhwa & Ganesh Bagler
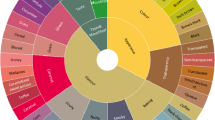
Sensory lexicon and aroma volatiles analysis of brewing malt
Xiaoxia Su, Miao Yu, … Tianyi Du
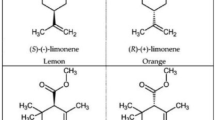
Predicting odor from molecular structure: a multi-label classification approach
Kushagra Saini & Venkatnarayan Ramanathan
Introduction
Predicting and understanding food perception and appreciation is one of the major challenges in food science. Accurate modeling of food flavor and appreciation could yield important opportunities for both producers and consumers, including quality control, product fingerprinting, counterfeit detection, spoilage detection, and the development of new products and product combinations (food pairing) 1 , 2 , 3 , 4 , 5 , 6 . Accurate models for flavor and consumer appreciation would contribute greatly to our scientific understanding of how humans perceive and appreciate flavor. Moreover, accurate predictive models would also facilitate and standardize existing food assessment methods and could supplement or replace assessments by trained and consumer tasting panels, which are variable, expensive and time-consuming 7 , 8 , 9 . Lastly, apart from providing objective, quantitative, accurate and contextual information that can help producers, models can also guide consumers in understanding their personal preferences 10 .
Despite the myriad of applications, predicting food flavor and appreciation from its chemical properties remains a largely elusive goal in sensory science, especially for complex food and beverages 11 , 12 . A key obstacle is the immense number of flavor-active chemicals underlying food flavor. Flavor compounds can vary widely in chemical structure and concentration, making them technically challenging and labor-intensive to quantify, even in the face of innovations in metabolomics, such as non-targeted metabolic fingerprinting 13 , 14 . Moreover, sensory analysis is perhaps even more complicated. Flavor perception is highly complex, resulting from hundreds of different molecules interacting at the physiochemical and sensorial level. Sensory perception is often non-linear, characterized by complex and concentration-dependent synergistic and antagonistic effects 15 , 16 , 17 , 18 , 19 , 20 , 21 that are further convoluted by the genetics, environment, culture and psychology of consumers 22 , 23 , 24 . Perceived flavor is therefore difficult to measure, with problems of sensitivity, accuracy, and reproducibility that can only be resolved by gathering sufficiently large datasets 25 . Trained tasting panels are considered the prime source of quality sensory data, but require meticulous training, are low throughput and high cost. Public databases containing consumer reviews of food products could provide a valuable alternative, especially for studying appreciation scores, which do not require formal training 25 . Public databases offer the advantage of amassing large amounts of data, increasing the statistical power to identify potential drivers of appreciation. However, public datasets suffer from biases, including a bias in the volunteers that contribute to the database, as well as confounding factors such as price, cult status and psychological conformity towards previous ratings of the product.
Classical multivariate statistics and machine learning methods have been used to predict flavor of specific compounds by, for example, linking structural properties of a compound to its potential biological activities or linking concentrations of specific compounds to sensory profiles 1 , 26 . Importantly, most previous studies focused on predicting organoleptic properties of single compounds (often based on their chemical structure) 27 , 28 , 29 , 30 , 31 , 32 , 33 , thus ignoring the fact that these compounds are present in a complex matrix in food or beverages and excluding complex interactions between compounds. Moreover, the classical statistics commonly used in sensory science 34 , 35 , 36 , 37 , 38 , 39 require a large sample size and sufficient variance amongst predictors to create accurate models. They are not fit for studying an extensive set of hundreds of interacting flavor compounds, since they are sensitive to outliers, have a high tendency to overfit and are less suited for non-linear and discontinuous relationships 40 .
In this study, we combine extensive chemical analyses and sensory data of a set of different commercial beers with machine learning approaches to develop models that predict taste, smell, mouthfeel and appreciation from compound concentrations. Beer is particularly suited to model the relationship between chemistry, flavor and appreciation. First, beer is a complex product, consisting of thousands of flavor compounds that partake in complex sensory interactions 41 , 42 , 43 . This chemical diversity arises from the raw materials (malt, yeast, hops, water and spices) and biochemical conversions during the brewing process (kilning, mashing, boiling, fermentation, maturation and aging) 44 , 45 . Second, the advent of the internet saw beer consumers embrace online review platforms, such as RateBeer (ZX Ventures, Anheuser-Busch InBev SA/NV) and BeerAdvocate (Next Glass, inc.). In this way, the beer community provides massive data sets of beer flavor and appreciation scores, creating extraordinarily large sensory databases to complement the analyses of our professional sensory panel. Specifically, we characterize over 200 chemical properties of 250 commercial beers, spread across 22 beer styles, and link these to the descriptive sensory profiling data of a 16-person in-house trained tasting panel and data acquired from over 180,000 public consumer reviews. These unique and extensive datasets enable us to train a suite of machine learning models to predict flavor and appreciation from a beer’s chemical profile. Dissection of the best-performing models allows us to pinpoint specific compounds as potential drivers of beer flavor and appreciation. Follow-up experiments confirm the importance of these compounds and ultimately allow us to significantly improve the flavor and appreciation of selected commercial beers. Together, our study represents a significant step towards understanding complex flavors and reinforces the value of machine learning to develop and refine complex foods. In this way, it represents a stepping stone for further computer-aided food engineering applications 46 .
To generate a comprehensive dataset on beer flavor, we selected 250 commercial Belgian beers across 22 different beer styles (Supplementary Fig. S1 ). Beers with ≤ 4.2% alcohol by volume (ABV) were classified as non-alcoholic and low-alcoholic. Blonds and Tripels constitute a significant portion of the dataset (12.4% and 11.2%, respectively) reflecting their presence on the Belgian beer market and the heterogeneity of beers within these styles. By contrast, lager beers are less diverse and dominated by a handful of brands. Rare styles such as Brut or Faro make up only a small fraction of the dataset (2% and 1%, respectively) because fewer of these beers are produced and because they are dominated by distinct characteristics in terms of flavor and chemical composition.
Extensive analysis identifies relationships between chemical compounds in beer
For each beer, we measured 226 different chemical properties, including common brewing parameters such as alcohol content, iso-alpha acids, pH, sugar concentration 47 , and over 200 flavor compounds (Methods, Supplementary Table S1 ). A large portion (37.2%) are terpenoids arising from hopping, responsible for herbal and fruity flavors 16 , 48 . A second major category are yeast metabolites, such as esters and alcohols, that result in fruity and solvent notes 48 , 49 , 50 . Other measured compounds are primarily derived from malt, or other microbes such as non- Saccharomyces yeasts and bacteria (‘wild flora’). Compounds that arise from spices or staling are labeled under ‘Others’. Five attributes (caloric value, total acids and total ester, hop aroma and sulfur compounds) are calculated from multiple individually measured compounds.
As a first step in identifying relationships between chemical properties, we determined correlations between the concentrations of the compounds (Fig. 1 , upper panel, Supplementary Data 1 and 2 , and Supplementary Fig. S2 . For the sake of clarity, only a subset of the measured compounds is shown in Fig. 1 ). Compounds of the same origin typically show a positive correlation, while absence of correlation hints at parameters varying independently. For example, the hop aroma compounds citronellol, and alpha-terpineol show moderate correlations with each other (Spearman’s rho=0.39 and 0.57), but not with the bittering hop component iso-alpha acids (Spearman’s rho=0.16 and −0.07). This illustrates how brewers can independently modify hop aroma and bitterness by selecting hop varieties and dosage time. If hops are added early in the boiling phase, chemical conversions increase bitterness while aromas evaporate, conversely, late addition of hops preserves aroma but limits bitterness 51 . Similarly, hop-derived iso-alpha acids show a strong anti-correlation with lactic acid and acetic acid, likely reflecting growth inhibition of lactic acid and acetic acid bacteria, or the consequent use of fewer hops in sour beer styles, such as West Flanders ales and Fruit beers, that rely on these bacteria for their distinct flavors 52 . Finally, yeast-derived esters (ethyl acetate, ethyl decanoate, ethyl hexanoate, ethyl octanoate) and alcohols (ethanol, isoamyl alcohol, isobutanol, and glycerol), correlate with Spearman coefficients above 0.5, suggesting that these secondary metabolites are correlated with the yeast genetic background and/or fermentation parameters and may be difficult to influence individually, although the choice of yeast strain may offer some control 53 .
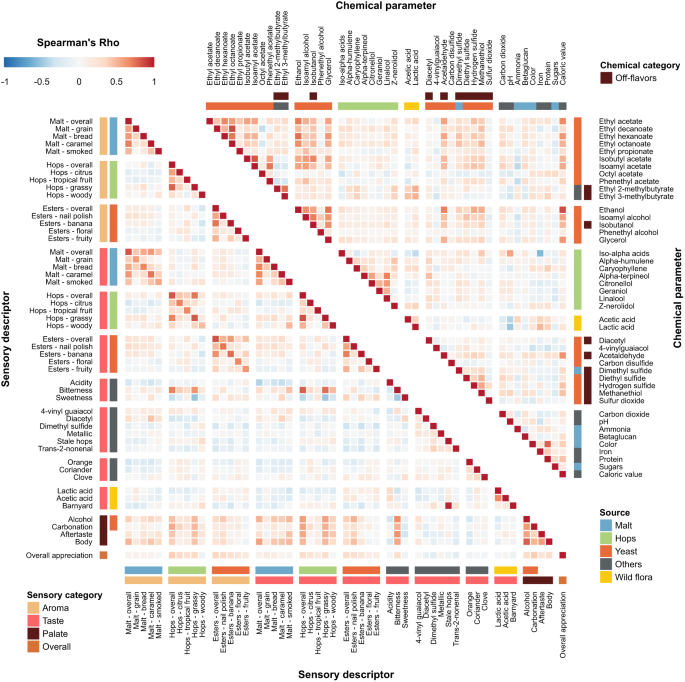
Spearman rank correlations are shown. Descriptors are grouped according to their origin (malt (blue), hops (green), yeast (red), wild flora (yellow), Others (black)), and sensory aspect (aroma, taste, palate, and overall appreciation). Please note that for the chemical compounds, for the sake of clarity, only a subset of the total number of measured compounds is shown, with an emphasis on the key compounds for each source. For more details, see the main text and Methods section. Chemical data can be found in Supplementary Data 1 , correlations between all chemical compounds are depicted in Supplementary Fig. S2 and correlation values can be found in Supplementary Data 2 . See Supplementary Data 4 for sensory panel assessments and Supplementary Data 5 for correlation values between all sensory descriptors.
Interestingly, different beer styles show distinct patterns for some flavor compounds (Supplementary Fig. S3 ). These observations agree with expectations for key beer styles, and serve as a control for our measurements. For instance, Stouts generally show high values for color (darker), while hoppy beers contain elevated levels of iso-alpha acids, compounds associated with bitter hop taste. Acetic and lactic acid are not prevalent in most beers, with notable exceptions such as Kriek, Lambic, Faro, West Flanders ales and Flanders Old Brown, which use acid-producing bacteria ( Lactobacillus and Pediococcus ) or unconventional yeast ( Brettanomyces ) 54 , 55 . Glycerol, ethanol and esters show similar distributions across all beer styles, reflecting their common origin as products of yeast metabolism during fermentation 45 , 53 . Finally, low/no-alcohol beers contain low concentrations of glycerol and esters. This is in line with the production process for most of the low/no-alcohol beers in our dataset, which are produced through limiting fermentation or by stripping away alcohol via evaporation or dialysis, with both methods having the unintended side-effect of reducing the amount of flavor compounds in the final beer 56 , 57 .
Besides expected associations, our data also reveals less trivial associations between beer styles and specific parameters. For example, geraniol and citronellol, two monoterpenoids responsible for citrus, floral and rose flavors and characteristic of Citra hops, are found in relatively high amounts in Christmas, Saison, and Brett/co-fermented beers, where they may originate from terpenoid-rich spices such as coriander seeds instead of hops 58 .
Tasting panel assessments reveal sensorial relationships in beer
To assess the sensory profile of each beer, a trained tasting panel evaluated each of the 250 beers for 50 sensory attributes, including different hop, malt and yeast flavors, off-flavors and spices. Panelists used a tasting sheet (Supplementary Data 3 ) to score the different attributes. Panel consistency was evaluated by repeating 12 samples across different sessions and performing ANOVA. In 95% of cases no significant difference was found across sessions ( p > 0.05), indicating good panel consistency (Supplementary Table S2 ).
Aroma and taste perception reported by the trained panel are often linked (Fig. 1 , bottom left panel and Supplementary Data 4 and 5 ), with high correlations between hops aroma and taste (Spearman’s rho=0.83). Bitter taste was found to correlate with hop aroma and taste in general (Spearman’s rho=0.80 and 0.69), and particularly with “grassy” noble hops (Spearman’s rho=0.75). Barnyard flavor, most often associated with sour beers, is identified together with stale hops (Spearman’s rho=0.97) that are used in these beers. Lactic and acetic acid, which often co-occur, are correlated (Spearman’s rho=0.66). Interestingly, sweetness and bitterness are anti-correlated (Spearman’s rho = −0.48), confirming the hypothesis that they mask each other 59 , 60 . Beer body is highly correlated with alcohol (Spearman’s rho = 0.79), and overall appreciation is found to correlate with multiple aspects that describe beer mouthfeel (alcohol, carbonation; Spearman’s rho= 0.32, 0.39), as well as with hop and ester aroma intensity (Spearman’s rho=0.39 and 0.35).
Similar to the chemical analyses, sensorial analyses confirmed typical features of specific beer styles (Supplementary Fig. S4 ). For example, sour beers (Faro, Flanders Old Brown, Fruit beer, Kriek, Lambic, West Flanders ale) were rated acidic, with flavors of both acetic and lactic acid. Hoppy beers were found to be bitter and showed hop-associated aromas like citrus and tropical fruit. Malt taste is most detected among scotch, stout/porters, and strong ales, while low/no-alcohol beers, which often have a reputation for being ‘worty’ (reminiscent of unfermented, sweet malt extract) appear in the middle. Unsurprisingly, hop aromas are most strongly detected among hoppy beers. Like its chemical counterpart (Supplementary Fig. S3 ), acidity shows a right-skewed distribution, with the most acidic beers being Krieks, Lambics, and West Flanders ales.
Tasting panel assessments of specific flavors correlate with chemical composition
We find that the concentrations of several chemical compounds strongly correlate with specific aroma or taste, as evaluated by the tasting panel (Fig. 2 , Supplementary Fig. S5 , Supplementary Data 6 ). In some cases, these correlations confirm expectations and serve as a useful control for data quality. For example, iso-alpha acids, the bittering compounds in hops, strongly correlate with bitterness (Spearman’s rho=0.68), while ethanol and glycerol correlate with tasters’ perceptions of alcohol and body, the mouthfeel sensation of fullness (Spearman’s rho=0.82/0.62 and 0.72/0.57 respectively) and darker color from roasted malts is a good indication of malt perception (Spearman’s rho=0.54).
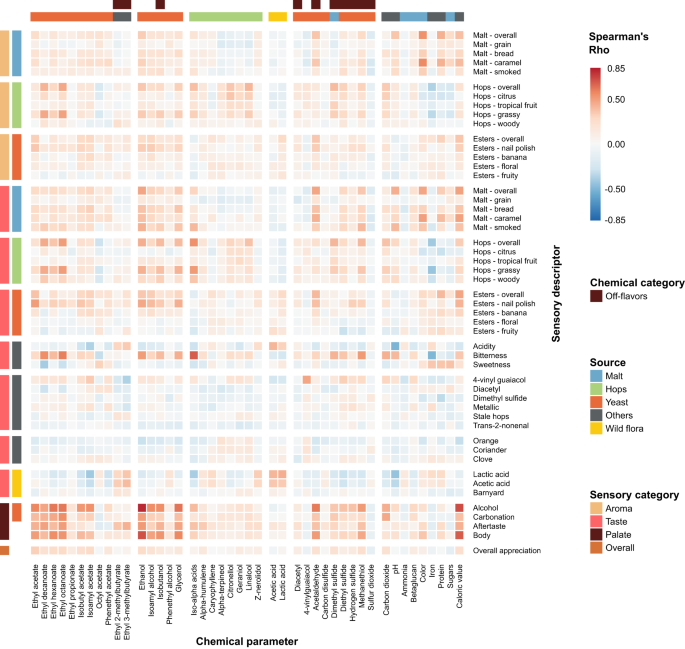
Heatmap colors indicate Spearman’s Rho. Axes are organized according to sensory categories (aroma, taste, mouthfeel, overall), chemical categories and chemical sources in beer (malt (blue), hops (green), yeast (red), wild flora (yellow), Others (black)). See Supplementary Data 6 for all correlation values.
Interestingly, for some relationships between chemical compounds and perceived flavor, correlations are weaker than expected. For example, the rose-smelling phenethyl acetate only weakly correlates with floral aroma. This hints at more complex relationships and interactions between compounds and suggests a need for a more complex model than simple correlations. Lastly, we uncovered unexpected correlations. For instance, the esters ethyl decanoate and ethyl octanoate appear to correlate slightly with hop perception and bitterness, possibly due to their fruity flavor. Iron is anti-correlated with hop aromas and bitterness, most likely because it is also anti-correlated with iso-alpha acids. This could be a sign of metal chelation of hop acids 61 , given that our analyses measure unbound hop acids and total iron content, or could result from the higher iron content in dark and Fruit beers, which typically have less hoppy and bitter flavors 62 .
Public consumer reviews complement expert panel data
To complement and expand the sensory data of our trained tasting panel, we collected 180,000 reviews of our 250 beers from the online consumer review platform RateBeer. This provided numerical scores for beer appearance, aroma, taste, palate, overall quality as well as the average overall score.
Public datasets are known to suffer from biases, such as price, cult status and psychological conformity towards previous ratings of a product. For example, prices correlate with appreciation scores for these online consumer reviews (rho=0.49, Supplementary Fig. S6 ), but not for our trained tasting panel (rho=0.19). This suggests that prices affect consumer appreciation, which has been reported in wine 63 , while blind tastings are unaffected. Moreover, we observe that some beer styles, like lagers and non-alcoholic beers, generally receive lower scores, reflecting that online reviewers are mostly beer aficionados with a preference for specialty beers over lager beers. In general, we find a modest correlation between our trained panel’s overall appreciation score and the online consumer appreciation scores (Fig. 3 , rho=0.29). Apart from the aforementioned biases in the online datasets, serving temperature, sample freshness and surroundings, which are all tightly controlled during the tasting panel sessions, can vary tremendously across online consumers and can further contribute to (among others, appreciation) differences between the two categories of tasters. Importantly, in contrast to the overall appreciation scores, for many sensory aspects the results from the professional panel correlated well with results obtained from RateBeer reviews. Correlations were highest for features that are relatively easy to recognize even for untrained tasters, like bitterness, sweetness, alcohol and malt aroma (Fig. 3 and below).
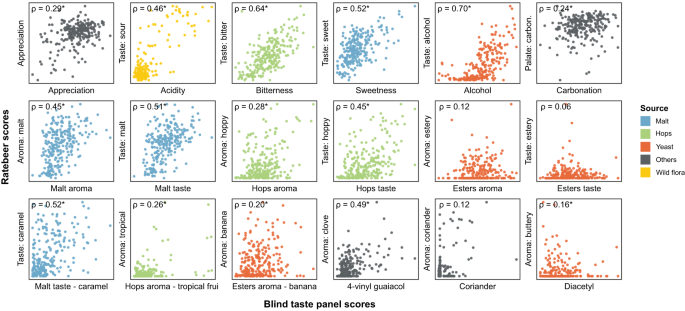
RateBeer text mining results can be found in Supplementary Data 7 . Rho values shown are Spearman correlation values, with asterisks indicating significant correlations ( p < 0.05, two-sided). All p values were smaller than 0.001, except for Esters aroma (0.0553), Esters taste (0.3275), Esters aroma—banana (0.0019), Coriander (0.0508) and Diacetyl (0.0134).
Besides collecting consumer appreciation from these online reviews, we developed automated text analysis tools to gather additional data from review texts (Supplementary Data 7 ). Processing review texts on the RateBeer database yielded comparable results to the scores given by the trained panel for many common sensory aspects, including acidity, bitterness, sweetness, alcohol, malt, and hop tastes (Fig. 3 ). This is in line with what would be expected, since these attributes require less training for accurate assessment and are less influenced by environmental factors such as temperature, serving glass and odors in the environment. Consumer reviews also correlate well with our trained panel for 4-vinyl guaiacol, a compound associated with a very characteristic aroma. By contrast, correlations for more specific aromas like ester, coriander or diacetyl are underrepresented in the online reviews, underscoring the importance of using a trained tasting panel and standardized tasting sheets with explicit factors to be scored for evaluating specific aspects of a beer. Taken together, our results suggest that public reviews are trustworthy for some, but not all, flavor features and can complement or substitute taste panel data for these sensory aspects.
Models can predict beer sensory profiles from chemical data
The rich datasets of chemical analyses, tasting panel assessments and public reviews gathered in the first part of this study provided us with a unique opportunity to develop predictive models that link chemical data to sensorial features. Given the complexity of beer flavor, basic statistical tools such as correlations or linear regression may not always be the most suitable for making accurate predictions. Instead, we applied different machine learning models that can model both simple linear and complex interactive relationships. Specifically, we constructed a set of regression models to predict (a) trained panel scores for beer flavor and quality and (b) public reviews’ appreciation scores from beer chemical profiles. We trained and tested 10 different models (Methods), 3 linear regression-based models (simple linear regression with first-order interactions (LR), lasso regression with first-order interactions (Lasso), partial least squares regressor (PLSR)), 5 decision tree models (AdaBoost regressor (ABR), extra trees (ET), gradient boosting regressor (GBR), random forest (RF) and XGBoost regressor (XGBR)), 1 support vector regression (SVR), and 1 artificial neural network (ANN) model.
To compare the performance of our machine learning models, the dataset was randomly split into a training and test set, stratified by beer style. After a model was trained on data in the training set, its performance was evaluated on its ability to predict the test dataset obtained from multi-output models (based on the coefficient of determination, see Methods). Additionally, individual-attribute models were ranked per descriptor and the average rank was calculated, as proposed by Korneva et al. 64 . Importantly, both ways of evaluating the models’ performance agreed in general. Performance of the different models varied (Table 1 ). It should be noted that all models perform better at predicting RateBeer results than results from our trained tasting panel. One reason could be that sensory data is inherently variable, and this variability is averaged out with the large number of public reviews from RateBeer. Additionally, all tree-based models perform better at predicting taste than aroma. Linear models (LR) performed particularly poorly, with negative R 2 values, due to severe overfitting (training set R 2 = 1). Overfitting is a common issue in linear models with many parameters and limited samples, especially with interaction terms further amplifying the number of parameters. L1 regularization (Lasso) successfully overcomes this overfitting, out-competing multiple tree-based models on the RateBeer dataset. Similarly, the dimensionality reduction of PLSR avoids overfitting and improves performance, to some extent. Still, tree-based models (ABR, ET, GBR, RF and XGBR) show the best performance, out-competing the linear models (LR, Lasso, PLSR) commonly used in sensory science 65 .
GBR models showed the best overall performance in predicting sensory responses from chemical information, with R 2 values up to 0.75 depending on the predicted sensory feature (Supplementary Table S4 ). The GBR models predict consumer appreciation (RateBeer) better than our trained panel’s appreciation (R 2 value of 0.67 compared to R 2 value of 0.09) (Supplementary Table S3 and Supplementary Table S4 ). ANN models showed intermediate performance, likely because neural networks typically perform best with larger datasets 66 . The SVR shows intermediate performance, mostly due to the weak predictions of specific attributes that lower the overall performance (Supplementary Table S4 ).
Model dissection identifies specific, unexpected compounds as drivers of consumer appreciation
Next, we leveraged our models to infer important contributors to sensory perception and consumer appreciation. Consumer preference is a crucial sensory aspects, because a product that shows low consumer appreciation scores often does not succeed commercially 25 . Additionally, the requirement for a large number of representative evaluators makes consumer trials one of the more costly and time-consuming aspects of product development. Hence, a model for predicting chemical drivers of overall appreciation would be a welcome addition to the available toolbox for food development and optimization.
Since GBR models on our RateBeer dataset showed the best overall performance, we focused on these models. Specifically, we used two approaches to identify important contributors. First, rankings of the most important predictors for each sensorial trait in the GBR models were obtained based on impurity-based feature importance (mean decrease in impurity). High-ranked parameters were hypothesized to be either the true causal chemical properties underlying the trait, to correlate with the actual causal properties, or to take part in sensory interactions affecting the trait 67 (Fig. 4A ). In a second approach, we used SHAP 68 to determine which parameters contributed most to the model for making predictions of consumer appreciation (Fig. 4B ). SHAP calculates parameter contributions to model predictions on a per-sample basis, which can be aggregated into an importance score.
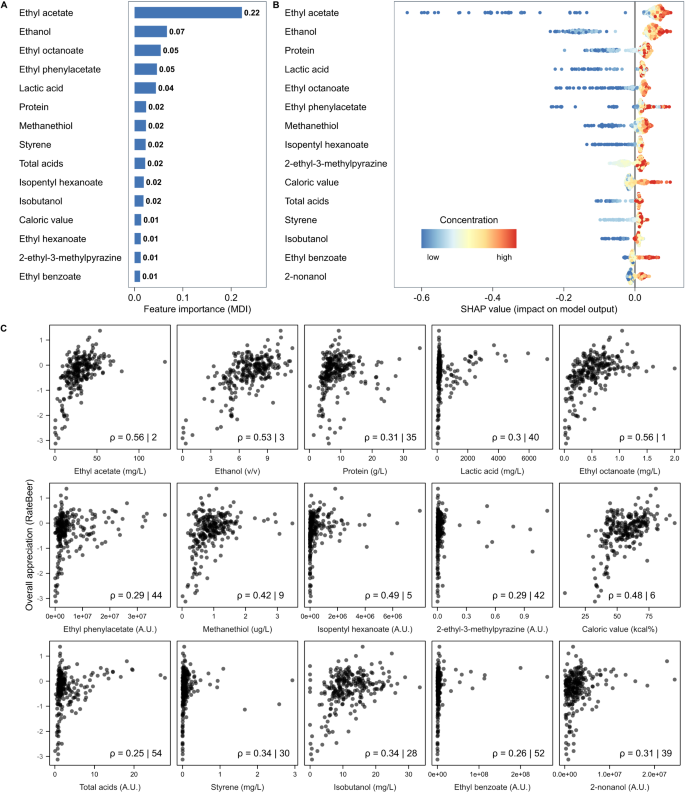
A The impurity-based feature importance (mean deviance in impurity, MDI) calculated from the Gradient Boosting Regression (GBR) model predicting RateBeer appreciation scores. The top 15 highest ranked chemical properties are shown. B SHAP summary plot for the top 15 parameters contributing to our GBR model. Each point on the graph represents a sample from our dataset. The color represents the concentration of that parameter, with bluer colors representing low values and redder colors representing higher values. Greater absolute values on the horizontal axis indicate a higher impact of the parameter on the prediction of the model. C Spearman correlations between the 15 most important chemical properties and consumer overall appreciation. Numbers indicate the Spearman Rho correlation coefficient, and the rank of this correlation compared to all other correlations. The top 15 important compounds were determined using SHAP (panel B).
Both approaches identified ethyl acetate as the most predictive parameter for beer appreciation (Fig. 4 ). Ethyl acetate is the most abundant ester in beer with a typical ‘fruity’, ‘solvent’ and ‘alcoholic’ flavor, but is often considered less important than other esters like isoamyl acetate. The second most important parameter identified by SHAP is ethanol, the most abundant beer compound after water. Apart from directly contributing to beer flavor and mouthfeel, ethanol drastically influences the physical properties of beer, dictating how easily volatile compounds escape the beer matrix to contribute to beer aroma 69 . Importantly, it should also be noted that the importance of ethanol for appreciation is likely inflated by the very low appreciation scores of non-alcoholic beers (Supplementary Fig. S4 ). Despite not often being considered a driver of beer appreciation, protein level also ranks highly in both approaches, possibly due to its effect on mouthfeel and body 70 . Lactic acid, which contributes to the tart taste of sour beers, is the fourth most important parameter identified by SHAP, possibly due to the generally high appreciation of sour beers in our dataset.
Interestingly, some of the most important predictive parameters for our model are not well-established as beer flavors or are even commonly regarded as being negative for beer quality. For example, our models identify methanethiol and ethyl phenyl acetate, an ester commonly linked to beer staling 71 , as a key factor contributing to beer appreciation. Although there is no doubt that high concentrations of these compounds are considered unpleasant, the positive effects of modest concentrations are not yet known 72 , 73 .
To compare our approach to conventional statistics, we evaluated how well the 15 most important SHAP-derived parameters correlate with consumer appreciation (Fig. 4C ). Interestingly, only 6 of the properties derived by SHAP rank amongst the top 15 most correlated parameters. For some chemical compounds, the correlations are so low that they would have likely been considered unimportant. For example, lactic acid, the fourth most important parameter, shows a bimodal distribution for appreciation, with sour beers forming a separate cluster, that is missed entirely by the Spearman correlation. Additionally, the correlation plots reveal outliers, emphasizing the need for robust analysis tools. Together, this highlights the need for alternative models, like the Gradient Boosting model, that better grasp the complexity of (beer) flavor.
Finally, to observe the relationships between these chemical properties and their predicted targets, partial dependence plots were constructed for the six most important predictors of consumer appreciation 74 , 75 , 76 (Supplementary Fig. S7 ). One-way partial dependence plots show how a change in concentration affects the predicted appreciation. These plots reveal an important limitation of our models: appreciation predictions remain constant at ever-increasing concentrations. This implies that once a threshold concentration is reached, further increasing the concentration does not affect appreciation. This is false, as it is well-documented that certain compounds become unpleasant at high concentrations, including ethyl acetate (‘nail polish’) 77 and methanethiol (‘sulfury’ and ‘rotten cabbage’) 78 . The inability of our models to grasp that flavor compounds have optimal levels, above which they become negative, is a consequence of working with commercial beer brands where (off-)flavors are rarely too high to negatively impact the product. The two-way partial dependence plots show how changing the concentration of two compounds influences predicted appreciation, visualizing their interactions (Supplementary Fig. S7 ). In our case, the top 5 parameters are dominated by additive or synergistic interactions, with high concentrations for both compounds resulting in the highest predicted appreciation.
To assess the robustness of our best-performing models and model predictions, we performed 100 iterations of the GBR, RF and ET models. In general, all iterations of the models yielded similar performance (Supplementary Fig. S8 ). Moreover, the main predictors (including the top predictors ethanol and ethyl acetate) remained virtually the same, especially for GBR and RF. For the iterations of the ET model, we did observe more variation in the top predictors, which is likely a consequence of the model’s inherent random architecture in combination with co-correlations between certain predictors. However, even in this case, several of the top predictors (ethanol and ethyl acetate) remain unchanged, although their rank in importance changes (Supplementary Fig. S8 ).
Next, we investigated if a combination of RateBeer and trained panel data into one consolidated dataset would lead to stronger models, under the hypothesis that such a model would suffer less from bias in the datasets. A GBR model was trained to predict appreciation on the combined dataset. This model underperformed compared to the RateBeer model, both in the native case and when including a dataset identifier (R 2 = 0.67, 0.26 and 0.42 respectively). For the latter, the dataset identifier is the most important feature (Supplementary Fig. S9 ), while most of the feature importance remains unchanged, with ethyl acetate and ethanol ranking highest, like in the original model trained only on RateBeer data. It seems that the large variation in the panel dataset introduces noise, weakening the models’ performances and reliability. In addition, it seems reasonable to assume that both datasets are fundamentally different, with the panel dataset obtained by blind tastings by a trained professional panel.
Lastly, we evaluated whether beer style identifiers would further enhance the model’s performance. A GBR model was trained with parameters that explicitly encoded the styles of the samples. This did not improve model performance (R2 = 0.66 with style information vs R2 = 0.67). The most important chemical features are consistent with the model trained without style information (eg. ethanol and ethyl acetate), and with the exception of the most preferred (strong ale) and least preferred (low/no-alcohol) styles, none of the styles were among the most important features (Supplementary Fig. S9 , Supplementary Table S5 and S6 ). This is likely due to a combination of style-specific chemical signatures, such as iso-alpha acids and lactic acid, that implicitly convey style information to the original models, as well as the low number of samples belonging to some styles, making it difficult for the model to learn style-specific patterns. Moreover, beer styles are not rigorously defined, with some styles overlapping in features and some beers being misattributed to a specific style, all of which leads to more noise in models that use style parameters.
Model validation
To test if our predictive models give insight into beer appreciation, we set up experiments aimed at improving existing commercial beers. We specifically selected overall appreciation as the trait to be examined because of its complexity and commercial relevance. Beer flavor comprises a complex bouquet rather than single aromas and tastes 53 . Hence, adding a single compound to the extent that a difference is noticeable may lead to an unbalanced, artificial flavor. Therefore, we evaluated the effect of combinations of compounds. Because Blond beers represent the most extensive style in our dataset, we selected a beer from this style as the starting material for these experiments (Beer 64 in Supplementary Data 1 ).
In the first set of experiments, we adjusted the concentrations of compounds that made up the most important predictors of overall appreciation (ethyl acetate, ethanol, lactic acid, ethyl phenyl acetate) together with correlated compounds (ethyl hexanoate, isoamyl acetate, glycerol), bringing them up to 95 th percentile ethanol-normalized concentrations (Methods) within the Blond group (‘Spiked’ concentration in Fig. 5A ). Compared to controls, the spiked beers were found to have significantly improved overall appreciation among trained panelists, with panelist noting increased intensity of ester flavors, sweetness, alcohol, and body fullness (Fig. 5B ). To disentangle the contribution of ethanol to these results, a second experiment was performed without the addition of ethanol. This resulted in a similar outcome, including increased perception of alcohol and overall appreciation.
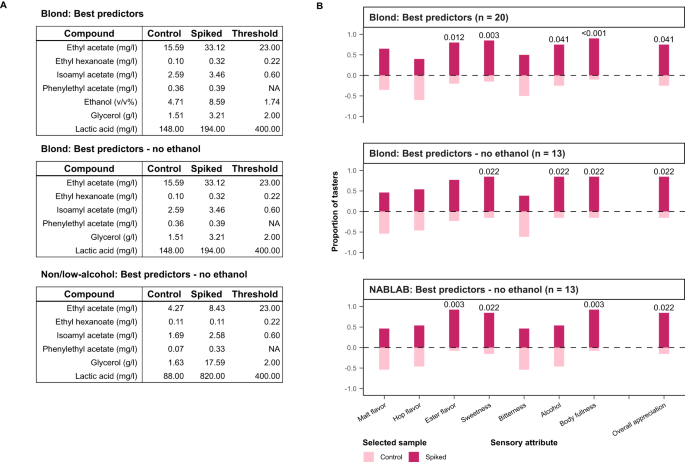
Adding the top chemical compounds, identified as best predictors of appreciation by our model, into poorly appreciated beers results in increased appreciation from our trained panel. Results of sensory tests between base beers and those spiked with compounds identified as the best predictors by the model. A Blond and Non/Low-alcohol (0.0% ABV) base beers were brought up to 95th-percentile ethanol-normalized concentrations within each style. B For each sensory attribute, tasters indicated the more intense sample and selected the sample they preferred. The numbers above the bars correspond to the p values that indicate significant changes in perceived flavor (two-sided binomial test: alpha 0.05, n = 20 or 13).
In a last experiment, we tested whether using the model’s predictions can boost the appreciation of a non-alcoholic beer (beer 223 in Supplementary Data 1 ). Again, the addition of a mixture of predicted compounds (omitting ethanol, in this case) resulted in a significant increase in appreciation, body, ester flavor and sweetness.
Predicting flavor and consumer appreciation from chemical composition is one of the ultimate goals of sensory science. A reliable, systematic and unbiased way to link chemical profiles to flavor and food appreciation would be a significant asset to the food and beverage industry. Such tools would substantially aid in quality control and recipe development, offer an efficient and cost-effective alternative to pilot studies and consumer trials and would ultimately allow food manufacturers to produce superior, tailor-made products that better meet the demands of specific consumer groups more efficiently.
A limited set of studies have previously tried, to varying degrees of success, to predict beer flavor and beer popularity based on (a limited set of) chemical compounds and flavors 79 , 80 . Current sensitive, high-throughput technologies allow measuring an unprecedented number of chemical compounds and properties in a large set of samples, yielding a dataset that can train models that help close the gaps between chemistry and flavor, even for a complex natural product like beer. To our knowledge, no previous research gathered data at this scale (250 samples, 226 chemical parameters, 50 sensory attributes and 5 consumer scores) to disentangle and validate the chemical aspects driving beer preference using various machine-learning techniques. We find that modern machine learning models outperform conventional statistical tools, such as correlations and linear models, and can successfully predict flavor appreciation from chemical composition. This could be attributed to the natural incorporation of interactions and non-linear or discontinuous effects in machine learning models, which are not easily grasped by the linear model architecture. While linear models and partial least squares regression represent the most widespread statistical approaches in sensory science, in part because they allow interpretation 65 , 81 , 82 , modern machine learning methods allow for building better predictive models while preserving the possibility to dissect and exploit the underlying patterns. Of the 10 different models we trained, tree-based models, such as our best performing GBR, showed the best overall performance in predicting sensory responses from chemical information, outcompeting artificial neural networks. This agrees with previous reports for models trained on tabular data 83 . Our results are in line with the findings of Colantonio et al. who also identified the gradient boosting architecture as performing best at predicting appreciation and flavor (of tomatoes and blueberries, in their specific study) 26 . Importantly, besides our larger experimental scale, we were able to directly confirm our models’ predictions in vivo.
Our study confirms that flavor compound concentration does not always correlate with perception, suggesting complex interactions that are often missed by more conventional statistics and simple models. Specifically, we find that tree-based algorithms may perform best in developing models that link complex food chemistry with aroma. Furthermore, we show that massive datasets of untrained consumer reviews provide a valuable source of data, that can complement or even replace trained tasting panels, especially for appreciation and basic flavors, such as sweetness and bitterness. This holds despite biases that are known to occur in such datasets, such as price or conformity bias. Moreover, GBR models predict taste better than aroma. This is likely because taste (e.g. bitterness) often directly relates to the corresponding chemical measurements (e.g., iso-alpha acids), whereas such a link is less clear for aromas, which often result from the interplay between multiple volatile compounds. We also find that our models are best at predicting acidity and alcohol, likely because there is a direct relation between the measured chemical compounds (acids and ethanol) and the corresponding perceived sensorial attribute (acidity and alcohol), and because even untrained consumers are generally able to recognize these flavors and aromas.
The predictions of our final models, trained on review data, hold even for blind tastings with small groups of trained tasters, as demonstrated by our ability to validate specific compounds as drivers of beer flavor and appreciation. Since adding a single compound to the extent of a noticeable difference may result in an unbalanced flavor profile, we specifically tested our identified key drivers as a combination of compounds. While this approach does not allow us to validate if a particular single compound would affect flavor and/or appreciation, our experiments do show that this combination of compounds increases consumer appreciation.
It is important to stress that, while it represents an important step forward, our approach still has several major limitations. A key weakness of the GBR model architecture is that amongst co-correlating variables, the largest main effect is consistently preferred for model building. As a result, co-correlating variables often have artificially low importance scores, both for impurity and SHAP-based methods, like we observed in the comparison to the more randomized Extra Trees models. This implies that chemicals identified as key drivers of a specific sensory feature by GBR might not be the true causative compounds, but rather co-correlate with the actual causative chemical. For example, the high importance of ethyl acetate could be (partially) attributed to the total ester content, ethanol or ethyl hexanoate (rho=0.77, rho=0.72 and rho=0.68), while ethyl phenylacetate could hide the importance of prenyl isobutyrate and ethyl benzoate (rho=0.77 and rho=0.76). Expanding our GBR model to include beer style as a parameter did not yield additional power or insight. This is likely due to style-specific chemical signatures, such as iso-alpha acids and lactic acid, that implicitly convey style information to the original model, as well as the smaller sample size per style, limiting the power to uncover style-specific patterns. This can be partly attributed to the curse of dimensionality, where the high number of parameters results in the models mainly incorporating single parameter effects, rather than complex interactions such as style-dependent effects 67 . A larger number of samples may overcome some of these limitations and offer more insight into style-specific effects. On the other hand, beer style is not a rigid scientific classification, and beers within one style often differ a lot, which further complicates the analysis of style as a model factor.
Our study is limited to beers from Belgian breweries. Although these beers cover a large portion of the beer styles available globally, some beer styles and consumer patterns may be missing, while other features might be overrepresented. For example, many Belgian ales exhibit yeast-driven flavor profiles, which is reflected in the chemical drivers of appreciation discovered by this study. In future work, expanding the scope to include diverse markets and beer styles could lead to the identification of even more drivers of appreciation and better models for special niche products that were not present in our beer set.
In addition to inherent limitations of GBR models, there are also some limitations associated with studying food aroma. Even if our chemical analyses measured most of the known aroma compounds, the total number of flavor compounds in complex foods like beer is still larger than the subset we were able to measure in this study. For example, hop-derived thiols, that influence flavor at very low concentrations, are notoriously difficult to measure in a high-throughput experiment. Moreover, consumer perception remains subjective and prone to biases that are difficult to avoid. It is also important to stress that the models are still immature and that more extensive datasets will be crucial for developing more complete models in the future. Besides more samples and parameters, our dataset does not include any demographic information about the tasters. Including such data could lead to better models that grasp external factors like age and culture. Another limitation is that our set of beers consists of high-quality end-products and lacks beers that are unfit for sale, which limits the current model in accurately predicting products that are appreciated very badly. Finally, while models could be readily applied in quality control, their use in sensory science and product development is restrained by their inability to discern causal relationships. Given that the models cannot distinguish compounds that genuinely drive consumer perception from those that merely correlate, validation experiments are essential to identify true causative compounds.
Despite the inherent limitations, dissection of our models enabled us to pinpoint specific molecules as potential drivers of beer aroma and consumer appreciation, including compounds that were unexpected and would not have been identified using standard approaches. Important drivers of beer appreciation uncovered by our models include protein levels, ethyl acetate, ethyl phenyl acetate and lactic acid. Currently, many brewers already use lactic acid to acidify their brewing water and ensure optimal pH for enzymatic activity during the mashing process. Our results suggest that adding lactic acid can also improve beer appreciation, although its individual effect remains to be tested. Interestingly, ethanol appears to be unnecessary to improve beer appreciation, both for blond beer and alcohol-free beer. Given the growing consumer interest in alcohol-free beer, with a predicted annual market growth of >7% 84 , it is relevant for brewers to know what compounds can further increase consumer appreciation of these beers. Hence, our model may readily provide avenues to further improve the flavor and consumer appreciation of both alcoholic and non-alcoholic beers, which is generally considered one of the key challenges for future beer production.
Whereas we see a direct implementation of our results for the development of superior alcohol-free beverages and other food products, our study can also serve as a stepping stone for the development of novel alcohol-containing beverages. We want to echo the growing body of scientific evidence for the negative effects of alcohol consumption, both on the individual level by the mutagenic, teratogenic and carcinogenic effects of ethanol 85 , 86 , as well as the burden on society caused by alcohol abuse and addiction. We encourage the use of our results for the production of healthier, tastier products, including novel and improved beverages with lower alcohol contents. Furthermore, we strongly discourage the use of these technologies to improve the appreciation or addictive properties of harmful substances.
The present work demonstrates that despite some important remaining hurdles, combining the latest developments in chemical analyses, sensory analysis and modern machine learning methods offers exciting avenues for food chemistry and engineering. Soon, these tools may provide solutions in quality control and recipe development, as well as new approaches to sensory science and flavor research.
Beer selection
250 commercial Belgian beers were selected to cover the broad diversity of beer styles and corresponding diversity in chemical composition and aroma. See Supplementary Fig. S1 .
Chemical dataset
Sample preparation.
Beers within their expiration date were purchased from commercial retailers. Samples were prepared in biological duplicates at room temperature, unless explicitly stated otherwise. Bottle pressure was measured with a manual pressure device (Steinfurth Mess-Systeme GmbH) and used to calculate CO 2 concentration. The beer was poured through two filter papers (Macherey-Nagel, 500713032 MN 713 ¼) to remove carbon dioxide and prevent spontaneous foaming. Samples were then prepared for measurements by targeted Headspace-Gas Chromatography-Flame Ionization Detector/Flame Photometric Detector (HS-GC-FID/FPD), Headspace-Solid Phase Microextraction-Gas Chromatography-Mass Spectrometry (HS-SPME-GC-MS), colorimetric analysis, enzymatic analysis, Near-Infrared (NIR) analysis, as described in the sections below. The mean values of biological duplicates are reported for each compound.
HS-GC-FID/FPD
HS-GC-FID/FPD (Shimadzu GC 2010 Plus) was used to measure higher alcohols, acetaldehyde, esters, 4-vinyl guaicol, and sulfur compounds. Each measurement comprised 5 ml of sample pipetted into a 20 ml glass vial containing 1.75 g NaCl (VWR, 27810.295). 100 µl of 2-heptanol (Sigma-Aldrich, H3003) (internal standard) solution in ethanol (Fisher Chemical, E/0650DF/C17) was added for a final concentration of 2.44 mg/L. Samples were flushed with nitrogen for 10 s, sealed with a silicone septum, stored at −80 °C and analyzed in batches of 20.
The GC was equipped with a DB-WAXetr column (length, 30 m; internal diameter, 0.32 mm; layer thickness, 0.50 µm; Agilent Technologies, Santa Clara, CA, USA) to the FID and an HP-5 column (length, 30 m; internal diameter, 0.25 mm; layer thickness, 0.25 µm; Agilent Technologies, Santa Clara, CA, USA) to the FPD. N 2 was used as the carrier gas. Samples were incubated for 20 min at 70 °C in the headspace autosampler (Flow rate, 35 cm/s; Injection volume, 1000 µL; Injection mode, split; Combi PAL autosampler, CTC analytics, Switzerland). The injector, FID and FPD temperatures were kept at 250 °C. The GC oven temperature was first held at 50 °C for 5 min and then allowed to rise to 80 °C at a rate of 5 °C/min, followed by a second ramp of 4 °C/min until 200 °C kept for 3 min and a final ramp of (4 °C/min) until 230 °C for 1 min. Results were analyzed with the GCSolution software version 2.4 (Shimadzu, Kyoto, Japan). The GC was calibrated with a 5% EtOH solution (VWR International) containing the volatiles under study (Supplementary Table S7 ).
HS-SPME-GC-MS
HS-SPME-GC-MS (Shimadzu GCMS-QP-2010 Ultra) was used to measure additional volatile compounds, mainly comprising terpenoids and esters. Samples were analyzed by HS-SPME using a triphase DVB/Carboxen/PDMS 50/30 μm SPME fiber (Supelco Co., Bellefonte, PA, USA) followed by gas chromatography (Thermo Fisher Scientific Trace 1300 series, USA) coupled to a mass spectrometer (Thermo Fisher Scientific ISQ series MS) equipped with a TriPlus RSH autosampler. 5 ml of degassed beer sample was placed in 20 ml vials containing 1.75 g NaCl (VWR, 27810.295). 5 µl internal standard mix was added, containing 2-heptanol (1 g/L) (Sigma-Aldrich, H3003), 4-fluorobenzaldehyde (1 g/L) (Sigma-Aldrich, 128376), 2,3-hexanedione (1 g/L) (Sigma-Aldrich, 144169) and guaiacol (1 g/L) (Sigma-Aldrich, W253200) in ethanol (Fisher Chemical, E/0650DF/C17). Each sample was incubated at 60 °C in the autosampler oven with constant agitation. After 5 min equilibration, the SPME fiber was exposed to the sample headspace for 30 min. The compounds trapped on the fiber were thermally desorbed in the injection port of the chromatograph by heating the fiber for 15 min at 270 °C.
The GC-MS was equipped with a low polarity RXi-5Sil MS column (length, 20 m; internal diameter, 0.18 mm; layer thickness, 0.18 µm; Restek, Bellefonte, PA, USA). Injection was performed in splitless mode at 320 °C, a split flow of 9 ml/min, a purge flow of 5 ml/min and an open valve time of 3 min. To obtain a pulsed injection, a programmed gas flow was used whereby the helium gas flow was set at 2.7 mL/min for 0.1 min, followed by a decrease in flow of 20 ml/min to the normal 0.9 mL/min. The temperature was first held at 30 °C for 3 min and then allowed to rise to 80 °C at a rate of 7 °C/min, followed by a second ramp of 2 °C/min till 125 °C and a final ramp of 8 °C/min with a final temperature of 270 °C.
Mass acquisition range was 33 to 550 amu at a scan rate of 5 scans/s. Electron impact ionization energy was 70 eV. The interface and ion source were kept at 275 °C and 250 °C, respectively. A mix of linear n-alkanes (from C7 to C40, Supelco Co.) was injected into the GC-MS under identical conditions to serve as external retention index markers. Identification and quantification of the compounds were performed using an in-house developed R script as described in Goelen et al. and Reher et al. 87 , 88 (for package information, see Supplementary Table S8 ). Briefly, chromatograms were analyzed using AMDIS (v2.71) 89 to separate overlapping peaks and obtain pure compound spectra. The NIST MS Search software (v2.0 g) in combination with the NIST2017, FFNSC3 and Adams4 libraries were used to manually identify the empirical spectra, taking into account the expected retention time. After background subtraction and correcting for retention time shifts between samples run on different days based on alkane ladders, compound elution profiles were extracted and integrated using a file with 284 target compounds of interest, which were either recovered in our identified AMDIS list of spectra or were known to occur in beer. Compound elution profiles were estimated for every peak in every chromatogram over a time-restricted window using weighted non-negative least square analysis after which peak areas were integrated 87 , 88 . Batch effect correction was performed by normalizing against the most stable internal standard compound, 4-fluorobenzaldehyde. Out of all 284 target compounds that were analyzed, 167 were visually judged to have reliable elution profiles and were used for final analysis.
Discrete photometric and enzymatic analysis
Discrete photometric and enzymatic analysis (Thermo Scientific TM Gallery TM Plus Beermaster Discrete Analyzer) was used to measure acetic acid, ammonia, beta-glucan, iso-alpha acids, color, sugars, glycerol, iron, pH, protein, and sulfite. 2 ml of sample volume was used for the analyses. Information regarding the reagents and standard solutions used for analyses and calibrations is included in Supplementary Table S7 and Supplementary Table S9 .
NIR analyses
NIR analysis (Anton Paar Alcolyzer Beer ME System) was used to measure ethanol. Measurements comprised 50 ml of sample, and a 10% EtOH solution was used for calibration.
Correlation calculations
Pairwise Spearman Rank correlations were calculated between all chemical properties.
Sensory dataset
Trained panel.
Our trained tasting panel consisted of volunteers who gave prior verbal informed consent. All compounds used for the validation experiment were of food-grade quality. The tasting sessions were approved by the Social and Societal Ethics Committee of the KU Leuven (G-2022-5677-R2(MAR)). All online reviewers agreed to the Terms and Conditions of the RateBeer website.
Sensory analysis was performed according to the American Society of Brewing Chemists (ASBC) Sensory Analysis Methods 90 . 30 volunteers were screened through a series of triangle tests. The sixteen most sensitive and consistent tasters were retained as taste panel members. The resulting panel was diverse in age [22–42, mean: 29], sex [56% male] and nationality [7 different countries]. The panel developed a consensus vocabulary to describe beer aroma, taste and mouthfeel. Panelists were trained to identify and score 50 different attributes, using a 7-point scale to rate attributes’ intensity. The scoring sheet is included as Supplementary Data 3 . Sensory assessments took place between 10–12 a.m. The beers were served in black-colored glasses. Per session, between 5 and 12 beers of the same style were tasted at 12 °C to 16 °C. Two reference beers were added to each set and indicated as ‘Reference 1 & 2’, allowing panel members to calibrate their ratings. Not all panelists were present at every tasting. Scores were scaled by standard deviation and mean-centered per taster. Values are represented as z-scores and clustered by Euclidean distance. Pairwise Spearman correlations were calculated between taste and aroma sensory attributes. Panel consistency was evaluated by repeating samples on different sessions and performing ANOVA to identify differences, using the ‘stats’ package (v4.2.2) in R (for package information, see Supplementary Table S8 ).
Online reviews from a public database
The ‘scrapy’ package in Python (v3.6) (for package information, see Supplementary Table S8 ). was used to collect 232,288 online reviews (mean=922, min=6, max=5343) from RateBeer, an online beer review database. Each review entry comprised 5 numerical scores (appearance, aroma, taste, palate and overall quality) and an optional review text. The total number of reviews per reviewer was collected separately. Numerical scores were scaled and centered per rater, and mean scores were calculated per beer.
For the review texts, the language was estimated using the packages ‘langdetect’ and ‘langid’ in Python. Reviews that were classified as English by both packages were kept. Reviewers with fewer than 100 entries overall were discarded. 181,025 reviews from >6000 reviewers from >40 countries remained. Text processing was done using the ‘nltk’ package in Python. Texts were corrected for slang and misspellings; proper nouns and rare words that are relevant to the beer context were specified and kept as-is (‘Chimay’,’Lambic’, etc.). A dictionary of semantically similar sensorial terms, for example ‘floral’ and ‘flower’, was created and collapsed together into one term. Words were stemmed and lemmatized to avoid identifying words such as ‘acid’ and ‘acidity’ as separate terms. Numbers and punctuation were removed.
Sentences from up to 50 randomly chosen reviews per beer were manually categorized according to the aspect of beer they describe (appearance, aroma, taste, palate, overall quality—not to be confused with the 5 numerical scores described above) or flagged as irrelevant if they contained no useful information. If a beer contained fewer than 50 reviews, all reviews were manually classified. This labeled data set was used to train a model that classified the rest of the sentences for all beers 91 . Sentences describing taste and aroma were extracted, and term frequency–inverse document frequency (TFIDF) was implemented to calculate enrichment scores for sensorial words per beer.
The sex of the tasting subject was not considered when building our sensory database. Instead, results from different panelists were averaged, both for our trained panel (56% male, 44% female) and the RateBeer reviews (70% male, 30% female for RateBeer as a whole).
Beer price collection and processing
Beer prices were collected from the following stores: Colruyt, Delhaize, Total Wine, BeerHawk, The Belgian Beer Shop, The Belgian Shop, and Beer of Belgium. Where applicable, prices were converted to Euros and normalized per liter. Spearman correlations were calculated between these prices and mean overall appreciation scores from RateBeer and the taste panel, respectively.
Pairwise Spearman Rank correlations were calculated between all sensory properties.
Machine learning models
Predictive modeling of sensory profiles from chemical data.
Regression models were constructed to predict (a) trained panel scores for beer flavors and quality from beer chemical profiles and (b) public reviews’ appreciation scores from beer chemical profiles. Z-scores were used to represent sensory attributes in both data sets. Chemical properties with log-normal distributions (Shapiro-Wilk test, p < 0.05 ) were log-transformed. Missing chemical measurements (0.1% of all data) were replaced with mean values per attribute. Observations from 250 beers were randomly separated into a training set (70%, 175 beers) and a test set (30%, 75 beers), stratified per beer style. Chemical measurements (p = 231) were normalized based on the training set average and standard deviation. In total, three linear regression-based models: linear regression with first-order interaction terms (LR), lasso regression with first-order interaction terms (Lasso) and partial least squares regression (PLSR); five decision tree models, Adaboost regressor (ABR), Extra Trees (ET), Gradient Boosting regressor (GBR), Random Forest (RF) and XGBoost regressor (XGBR); one support vector machine model (SVR) and one artificial neural network model (ANN) were trained. The models were implemented using the ‘scikit-learn’ package (v1.2.2) and ‘xgboost’ package (v1.7.3) in Python (v3.9.16). Models were trained, and hyperparameters optimized, using five-fold cross-validated grid search with the coefficient of determination (R 2 ) as the evaluation metric. The ANN (scikit-learn’s MLPRegressor) was optimized using Bayesian Tree-Structured Parzen Estimator optimization with the ‘Optuna’ Python package (v3.2.0). Individual models were trained per attribute, and a multi-output model was trained on all attributes simultaneously.
Model dissection
GBR was found to outperform other methods, resulting in models with the highest average R 2 values in both trained panel and public review data sets. Impurity-based rankings of the most important predictors for each predicted sensorial trait were obtained using the ‘scikit-learn’ package. To observe the relationships between these chemical properties and their predicted targets, partial dependence plots (PDP) were constructed for the six most important predictors of consumer appreciation 74 , 75 .
The ‘SHAP’ package in Python (v0.41.0) was implemented to provide an alternative ranking of predictor importance and to visualize the predictors’ effects as a function of their concentration 68 .
Validation of causal chemical properties
To validate the effects of the most important model features on predicted sensory attributes, beers were spiked with the chemical compounds identified by the models and descriptive sensory analyses were carried out according to the American Society of Brewing Chemists (ASBC) protocol 90 .
Compound spiking was done 30 min before tasting. Compounds were spiked into fresh beer bottles, that were immediately resealed and inverted three times. Fresh bottles of beer were opened for the same duration, resealed, and inverted thrice, to serve as controls. Pairs of spiked samples and controls were served simultaneously, chilled and in dark glasses as outlined in the Trained panel section above. Tasters were instructed to select the glass with the higher flavor intensity for each attribute (directional difference test 92 ) and to select the glass they prefer.
The final concentration after spiking was equal to the within-style average, after normalizing by ethanol concentration. This was done to ensure balanced flavor profiles in the final spiked beer. The same methods were applied to improve a non-alcoholic beer. Compounds were the following: ethyl acetate (Merck KGaA, W241415), ethyl hexanoate (Merck KGaA, W243906), isoamyl acetate (Merck KGaA, W205508), phenethyl acetate (Merck KGaA, W285706), ethanol (96%, Colruyt), glycerol (Merck KGaA, W252506), lactic acid (Merck KGaA, 261106).
Significant differences in preference or perceived intensity were determined by performing the two-sided binomial test on each attribute.
Reporting summary
Further information on research design is available in the Nature Portfolio Reporting Summary linked to this article.
Data availability
The data that support the findings of this work are available in the Supplementary Data files and have been deposited to Zenodo under accession code 10653704 93 . The RateBeer scores data are under restricted access, they are not publicly available as they are property of RateBeer (ZX Ventures, USA). Access can be obtained from the authors upon reasonable request and with permission of RateBeer (ZX Ventures, USA). Source data are provided with this paper.
Code availability
The code for training the machine learning models, analyzing the models, and generating the figures has been deposited to Zenodo under accession code 10653704 93 .
Tieman, D. et al. A chemical genetic roadmap to improved tomato flavor. Science 355 , 391–394 (2017).
Article ADS CAS PubMed Google Scholar
Plutowska, B. & Wardencki, W. Application of gas chromatography–olfactometry (GC–O) in analysis and quality assessment of alcoholic beverages – A review. Food Chem. 107 , 449–463 (2008).
Article CAS Google Scholar
Legin, A., Rudnitskaya, A., Seleznev, B. & Vlasov, Y. Electronic tongue for quality assessment of ethanol, vodka and eau-de-vie. Anal. Chim. Acta 534 , 129–135 (2005).
Loutfi, A., Coradeschi, S., Mani, G. K., Shankar, P. & Rayappan, J. B. B. Electronic noses for food quality: A review. J. Food Eng. 144 , 103–111 (2015).
Ahn, Y.-Y., Ahnert, S. E., Bagrow, J. P. & Barabási, A.-L. Flavor network and the principles of food pairing. Sci. Rep. 1 , 196 (2011).
Article CAS PubMed PubMed Central Google Scholar
Bartoshuk, L. M. & Klee, H. J. Better fruits and vegetables through sensory analysis. Curr. Biol. 23 , R374–R378 (2013).
Article CAS PubMed Google Scholar
Piggott, J. R. Design questions in sensory and consumer science. Food Qual. Prefer. 3293 , 217–220 (1995).
Article Google Scholar
Kermit, M. & Lengard, V. Assessing the performance of a sensory panel-panellist monitoring and tracking. J. Chemom. 19 , 154–161 (2005).
Cook, D. J., Hollowood, T. A., Linforth, R. S. T. & Taylor, A. J. Correlating instrumental measurements of texture and flavour release with human perception. Int. J. Food Sci. Technol. 40 , 631–641 (2005).
Chinchanachokchai, S., Thontirawong, P. & Chinchanachokchai, P. A tale of two recommender systems: The moderating role of consumer expertise on artificial intelligence based product recommendations. J. Retail. Consum. Serv. 61 , 1–12 (2021).
Ross, C. F. Sensory science at the human-machine interface. Trends Food Sci. Technol. 20 , 63–72 (2009).
Chambers, E. IV & Koppel, K. Associations of volatile compounds with sensory aroma and flavor: The complex nature of flavor. Molecules 18 , 4887–4905 (2013).
Pinu, F. R. Metabolomics—The new frontier in food safety and quality research. Food Res. Int. 72 , 80–81 (2015).
Danezis, G. P., Tsagkaris, A. S., Brusic, V. & Georgiou, C. A. Food authentication: state of the art and prospects. Curr. Opin. Food Sci. 10 , 22–31 (2016).
Shepherd, G. M. Smell images and the flavour system in the human brain. Nature 444 , 316–321 (2006).
Meilgaard, M. C. Prediction of flavor differences between beers from their chemical composition. J. Agric. Food Chem. 30 , 1009–1017 (1982).
Xu, L. et al. Widespread receptor-driven modulation in peripheral olfactory coding. Science 368 , eaaz5390 (2020).
Kupferschmidt, K. Following the flavor. Science 340 , 808–809 (2013).
Billesbølle, C. B. et al. Structural basis of odorant recognition by a human odorant receptor. Nature 615 , 742–749 (2023).
Article ADS PubMed PubMed Central Google Scholar
Smith, B. Perspective: Complexities of flavour. Nature 486 , S6–S6 (2012).
Pfister, P. et al. Odorant receptor inhibition is fundamental to odor encoding. Curr. Biol. 30 , 2574–2587 (2020).
Moskowitz, H. W., Kumaraiah, V., Sharma, K. N., Jacobs, H. L. & Sharma, S. D. Cross-cultural differences in simple taste preferences. Science 190 , 1217–1218 (1975).
Eriksson, N. et al. A genetic variant near olfactory receptor genes influences cilantro preference. Flavour 1 , 22 (2012).
Ferdenzi, C. et al. Variability of affective responses to odors: Culture, gender, and olfactory knowledge. Chem. Senses 38 , 175–186 (2013).
Article PubMed Google Scholar
Lawless, H. T. & Heymann, H. Sensory evaluation of food: Principles and practices. (Springer, New York, NY). https://doi.org/10.1007/978-1-4419-6488-5 (2010).
Colantonio, V. et al. Metabolomic selection for enhanced fruit flavor. Proc. Natl. Acad. Sci. 119 , e2115865119 (2022).
Fritz, F., Preissner, R. & Banerjee, P. VirtualTaste: a web server for the prediction of organoleptic properties of chemical compounds. Nucleic Acids Res 49 , W679–W684 (2021).
Tuwani, R., Wadhwa, S. & Bagler, G. BitterSweet: Building machine learning models for predicting the bitter and sweet taste of small molecules. Sci. Rep. 9 , 1–13 (2019).
Dagan-Wiener, A. et al. Bitter or not? BitterPredict, a tool for predicting taste from chemical structure. Sci. Rep. 7 , 1–13 (2017).
Pallante, L. et al. Toward a general and interpretable umami taste predictor using a multi-objective machine learning approach. Sci. Rep. 12 , 1–11 (2022).
Malavolta, M. et al. A survey on computational taste predictors. Eur. Food Res. Technol. 248 , 2215–2235 (2022).
Lee, B. K. et al. A principal odor map unifies diverse tasks in olfactory perception. Science 381 , 999–1006 (2023).
Mayhew, E. J. et al. Transport features predict if a molecule is odorous. Proc. Natl. Acad. Sci. 119 , e2116576119 (2022).
Niu, Y. et al. Sensory evaluation of the synergism among ester odorants in light aroma-type liquor by odor threshold, aroma intensity and flash GC electronic nose. Food Res. Int. 113 , 102–114 (2018).
Yu, P., Low, M. Y. & Zhou, W. Design of experiments and regression modelling in food flavour and sensory analysis: A review. Trends Food Sci. Technol. 71 , 202–215 (2018).
Oladokun, O. et al. The impact of hop bitter acid and polyphenol profiles on the perceived bitterness of beer. Food Chem. 205 , 212–220 (2016).
Linforth, R., Cabannes, M., Hewson, L., Yang, N. & Taylor, A. Effect of fat content on flavor delivery during consumption: An in vivo model. J. Agric. Food Chem. 58 , 6905–6911 (2010).
Guo, S., Na Jom, K. & Ge, Y. Influence of roasting condition on flavor profile of sunflower seeds: A flavoromics approach. Sci. Rep. 9 , 11295 (2019).
Ren, Q. et al. The changes of microbial community and flavor compound in the fermentation process of Chinese rice wine using Fagopyrum tataricum grain as feedstock. Sci. Rep. 9 , 3365 (2019).
Hastie, T., Friedman, J. & Tibshirani, R. The Elements of Statistical Learning. (Springer, New York, NY). https://doi.org/10.1007/978-0-387-21606-5 (2001).
Dietz, C., Cook, D., Huismann, M., Wilson, C. & Ford, R. The multisensory perception of hop essential oil: a review. J. Inst. Brew. 126 , 320–342 (2020).
CAS Google Scholar
Roncoroni, Miguel & Verstrepen, Kevin Joan. Belgian Beer: Tested and Tasted. (Lannoo, 2018).
Meilgaard, M. Flavor chemistry of beer: Part II: Flavor and threshold of 239 aroma volatiles. in (1975).
Bokulich, N. A. & Bamforth, C. W. The microbiology of malting and brewing. Microbiol. Mol. Biol. Rev. MMBR 77 , 157–172 (2013).
Dzialo, M. C., Park, R., Steensels, J., Lievens, B. & Verstrepen, K. J. Physiology, ecology and industrial applications of aroma formation in yeast. FEMS Microbiol. Rev. 41 , S95–S128 (2017).
Article PubMed PubMed Central Google Scholar
Datta, A. et al. Computer-aided food engineering. Nat. Food 3 , 894–904 (2022).
American Society of Brewing Chemists. Beer Methods. (American Society of Brewing Chemists, St. Paul, MN, U.S.A.).
Olaniran, A. O., Hiralal, L., Mokoena, M. P. & Pillay, B. Flavour-active volatile compounds in beer: production, regulation and control. J. Inst. Brew. 123 , 13–23 (2017).
Verstrepen, K. J. et al. Flavor-active esters: Adding fruitiness to beer. J. Biosci. Bioeng. 96 , 110–118 (2003).
Meilgaard, M. C. Flavour chemistry of beer. part I: flavour interaction between principal volatiles. Master Brew. Assoc. Am. Tech. Q 12 , 107–117 (1975).
Briggs, D. E., Boulton, C. A., Brookes, P. A. & Stevens, R. Brewing 227–254. (Woodhead Publishing). https://doi.org/10.1533/9781855739062.227 (2004).
Bossaert, S., Crauwels, S., De Rouck, G. & Lievens, B. The power of sour - A review: Old traditions, new opportunities. BrewingScience 72 , 78–88 (2019).
Google Scholar
Verstrepen, K. J. et al. Flavor active esters: Adding fruitiness to beer. J. Biosci. Bioeng. 96 , 110–118 (2003).
Snauwaert, I. et al. Microbial diversity and metabolite composition of Belgian red-brown acidic ales. Int. J. Food Microbiol. 221 , 1–11 (2016).
Spitaels, F. et al. The microbial diversity of traditional spontaneously fermented lambic beer. PLoS ONE 9 , e95384 (2014).
Blanco, C. A., Andrés-Iglesias, C. & Montero, O. Low-alcohol Beers: Flavor Compounds, Defects, and Improvement Strategies. Crit. Rev. Food Sci. Nutr. 56 , 1379–1388 (2016).
Jackowski, M. & Trusek, A. Non-Alcohol. beer Prod. – Overv. 20 , 32–38 (2018).
Takoi, K. et al. The contribution of geraniol metabolism to the citrus flavour of beer: Synergy of geraniol and β-citronellol under coexistence with excess linalool. J. Inst. Brew. 116 , 251–260 (2010).
Kroeze, J. H. & Bartoshuk, L. M. Bitterness suppression as revealed by split-tongue taste stimulation in humans. Physiol. Behav. 35 , 779–783 (1985).
Mennella, J. A. et al. A spoonful of sugar helps the medicine go down”: Bitter masking bysucrose among children and adults. Chem. Senses 40 , 17–25 (2015).
Wietstock, P., Kunz, T., Perreira, F. & Methner, F.-J. Metal chelation behavior of hop acids in buffered model systems. BrewingScience 69 , 56–63 (2016).
Sancho, D., Blanco, C. A., Caballero, I. & Pascual, A. Free iron in pale, dark and alcohol-free commercial lager beers. J. Sci. Food Agric. 91 , 1142–1147 (2011).
Rodrigues, H. & Parr, W. V. Contribution of cross-cultural studies to understanding wine appreciation: A review. Food Res. Int. 115 , 251–258 (2019).
Korneva, E. & Blockeel, H. Towards better evaluation of multi-target regression models. in ECML PKDD 2020 Workshops (eds. Koprinska, I. et al.) 353–362 (Springer International Publishing, Cham, 2020). https://doi.org/10.1007/978-3-030-65965-3_23 .
Gastón Ares. Mathematical and Statistical Methods in Food Science and Technology. (Wiley, 2013).
Grinsztajn, L., Oyallon, E. & Varoquaux, G. Why do tree-based models still outperform deep learning on tabular data? Preprint at http://arxiv.org/abs/2207.08815 (2022).
Gries, S. T. Statistics for Linguistics with R: A Practical Introduction. in Statistics for Linguistics with R (De Gruyter Mouton, 2021). https://doi.org/10.1515/9783110718256 .
Lundberg, S. M. et al. From local explanations to global understanding with explainable AI for trees. Nat. Mach. Intell. 2 , 56–67 (2020).
Ickes, C. M. & Cadwallader, K. R. Effects of ethanol on flavor perception in alcoholic beverages. Chemosens. Percept. 10 , 119–134 (2017).
Kato, M. et al. Influence of high molecular weight polypeptides on the mouthfeel of commercial beer. J. Inst. Brew. 127 , 27–40 (2021).
Wauters, R. et al. Novel Saccharomyces cerevisiae variants slow down the accumulation of staling aldehydes and improve beer shelf-life. Food Chem. 398 , 1–11 (2023).
Li, H., Jia, S. & Zhang, W. Rapid determination of low-level sulfur compounds in beer by headspace gas chromatography with a pulsed flame photometric detector. J. Am. Soc. Brew. Chem. 66 , 188–191 (2008).
Dercksen, A., Laurens, J., Torline, P., Axcell, B. C. & Rohwer, E. Quantitative analysis of volatile sulfur compounds in beer using a membrane extraction interface. J. Am. Soc. Brew. Chem. 54 , 228–233 (1996).
Molnar, C. Interpretable Machine Learning: A Guide for Making Black-Box Models Interpretable. (2020).
Zhao, Q. & Hastie, T. Causal interpretations of black-box models. J. Bus. Econ. Stat. Publ. Am. Stat. Assoc. 39 , 272–281 (2019).
Article MathSciNet Google Scholar
Hastie, T., Tibshirani, R. & Friedman, J. The Elements of Statistical Learning. (Springer, 2019).
Labrado, D. et al. Identification by NMR of key compounds present in beer distillates and residual phases after dealcoholization by vacuum distillation. J. Sci. Food Agric. 100 , 3971–3978 (2020).
Lusk, L. T., Kay, S. B., Porubcan, A. & Ryder, D. S. Key olfactory cues for beer oxidation. J. Am. Soc. Brew. Chem. 70 , 257–261 (2012).
Gonzalez Viejo, C., Torrico, D. D., Dunshea, F. R. & Fuentes, S. Development of artificial neural network models to assess beer acceptability based on sensory properties using a robotic pourer: A comparative model approach to achieve an artificial intelligence system. Beverages 5 , 33 (2019).
Gonzalez Viejo, C., Fuentes, S., Torrico, D. D., Godbole, A. & Dunshea, F. R. Chemical characterization of aromas in beer and their effect on consumers liking. Food Chem. 293 , 479–485 (2019).
Gilbert, J. L. et al. Identifying breeding priorities for blueberry flavor using biochemical, sensory, and genotype by environment analyses. PLOS ONE 10 , 1–21 (2015).
Goulet, C. et al. Role of an esterase in flavor volatile variation within the tomato clade. Proc. Natl. Acad. Sci. 109 , 19009–19014 (2012).
Article ADS CAS PubMed PubMed Central Google Scholar
Borisov, V. et al. Deep Neural Networks and Tabular Data: A Survey. IEEE Trans. Neural Netw. Learn. Syst. 1–21 https://doi.org/10.1109/TNNLS.2022.3229161 (2022).
Statista. Statista Consumer Market Outlook: Beer - Worldwide.
Seitz, H. K. & Stickel, F. Molecular mechanisms of alcoholmediated carcinogenesis. Nat. Rev. Cancer 7 , 599–612 (2007).
Voordeckers, K. et al. Ethanol exposure increases mutation rate through error-prone polymerases. Nat. Commun. 11 , 3664 (2020).
Goelen, T. et al. Bacterial phylogeny predicts volatile organic compound composition and olfactory response of an aphid parasitoid. Oikos 129 , 1415–1428 (2020).
Article ADS Google Scholar
Reher, T. et al. Evaluation of hop (Humulus lupulus) as a repellent for the management of Drosophila suzukii. Crop Prot. 124 , 104839 (2019).
Stein, S. E. An integrated method for spectrum extraction and compound identification from gas chromatography/mass spectrometry data. J. Am. Soc. Mass Spectrom. 10 , 770–781 (1999).
American Society of Brewing Chemists. Sensory Analysis Methods. (American Society of Brewing Chemists, St. Paul, MN, U.S.A., 1992).
McAuley, J., Leskovec, J. & Jurafsky, D. Learning Attitudes and Attributes from Multi-Aspect Reviews. Preprint at https://doi.org/10.48550/arXiv.1210.3926 (2012).
Meilgaard, M. C., Carr, B. T. & Carr, B. T. Sensory Evaluation Techniques. (CRC Press, Boca Raton). https://doi.org/10.1201/b16452 (2014).
Schreurs, M. et al. Data from: Predicting and improving complex beer flavor through machine learning. Zenodo https://doi.org/10.5281/zenodo.10653704 (2024).
Download references
Acknowledgements
We thank all lab members for their discussions and thank all tasting panel members for their contributions. Special thanks go out to Dr. Karin Voordeckers for her tremendous help in proofreading and improving the manuscript. M.S. was supported by a Baillet-Latour fellowship, L.C. acknowledges financial support from KU Leuven (C16/17/006), F.A.T. was supported by a PhD fellowship from FWO (1S08821N). Research in the lab of K.J.V. is supported by KU Leuven, FWO, VIB, VLAIO and the Brewing Science Serves Health Fund. Research in the lab of T.W. is supported by FWO (G.0A51.15) and KU Leuven (C16/17/006).
Author information
These authors contributed equally: Michiel Schreurs, Supinya Piampongsant, Miguel Roncoroni.
Authors and Affiliations
VIB—KU Leuven Center for Microbiology, Gaston Geenslaan 1, B-3001, Leuven, Belgium
Michiel Schreurs, Supinya Piampongsant, Miguel Roncoroni, Lloyd Cool, Beatriz Herrera-Malaver, Florian A. Theßeling & Kevin J. Verstrepen
CMPG Laboratory of Genetics and Genomics, KU Leuven, Gaston Geenslaan 1, B-3001, Leuven, Belgium
Leuven Institute for Beer Research (LIBR), Gaston Geenslaan 1, B-3001, Leuven, Belgium
Laboratory of Socioecology and Social Evolution, KU Leuven, Naamsestraat 59, B-3000, Leuven, Belgium
Lloyd Cool, Christophe Vanderaa & Tom Wenseleers
VIB Bioinformatics Core, VIB, Rijvisschestraat 120, B-9052, Ghent, Belgium
Łukasz Kreft & Alexander Botzki
AB InBev SA/NV, Brouwerijplein 1, B-3000, Leuven, Belgium
Philippe Malcorps & Luk Daenen
You can also search for this author in PubMed Google Scholar
Contributions
S.P., M.S. and K.J.V. conceived the experiments. S.P., M.S. and K.J.V. designed the experiments. S.P., M.S., M.R., B.H. and F.A.T. performed the experiments. S.P., M.S., L.C., C.V., L.K., A.B., P.M., L.D., T.W. and K.J.V. contributed analysis ideas. S.P., M.S., L.C., C.V., T.W. and K.J.V. analyzed the data. All authors contributed to writing the manuscript.
Corresponding author
Correspondence to Kevin J. Verstrepen .
Ethics declarations
Competing interests.
K.J.V. is affiliated with bar.on. The other authors declare no competing interests.
Peer review
Peer review information.
Nature Communications thanks Florian Bauer, Andrew John Macintosh and the other, anonymous, reviewer(s) for their contribution to the peer review of this work. A peer review file is available.
Additional information
Publisher’s note Springer Nature remains neutral with regard to jurisdictional claims in published maps and institutional affiliations.
Supplementary information
Supplementary information, peer review file, description of additional supplementary files, supplementary data 1, supplementary data 2, supplementary data 3, supplementary data 4, supplementary data 5, supplementary data 6, supplementary data 7, reporting summary, source data, source data, rights and permissions.
Open Access This article is licensed under a Creative Commons Attribution 4.0 International License, which permits use, sharing, adaptation, distribution and reproduction in any medium or format, as long as you give appropriate credit to the original author(s) and the source, provide a link to the Creative Commons licence, and indicate if changes were made. The images or other third party material in this article are included in the article’s Creative Commons licence, unless indicated otherwise in a credit line to the material. If material is not included in the article’s Creative Commons licence and your intended use is not permitted by statutory regulation or exceeds the permitted use, you will need to obtain permission directly from the copyright holder. To view a copy of this licence, visit http://creativecommons.org/licenses/by/4.0/ .
Reprints and permissions
About this article
Cite this article.
Schreurs, M., Piampongsant, S., Roncoroni, M. et al. Predicting and improving complex beer flavor through machine learning. Nat Commun 15 , 2368 (2024). https://doi.org/10.1038/s41467-024-46346-0
Download citation
Received : 30 October 2023
Accepted : 21 February 2024
Published : 26 March 2024
DOI : https://doi.org/10.1038/s41467-024-46346-0
Share this article
Anyone you share the following link with will be able to read this content:
Sorry, a shareable link is not currently available for this article.
Provided by the Springer Nature SharedIt content-sharing initiative
By submitting a comment you agree to abide by our Terms and Community Guidelines . If you find something abusive or that does not comply with our terms or guidelines please flag it as inappropriate.
Quick links
- Explore articles by subject
- Guide to authors
- Editorial policies
Sign up for the Nature Briefing: Translational Research newsletter — top stories in biotechnology, drug discovery and pharma.

Help | Advanced Search
Computer Science > Computation and Language
Title: improving vietnamese-english medical machine translation.
Abstract: Machine translation for Vietnamese-English in the medical domain is still an under-explored research area. In this paper, we introduce MedEV -- a high-quality Vietnamese-English parallel dataset constructed specifically for the medical domain, comprising approximately 360K sentence pairs. We conduct extensive experiments comparing Google Translate, ChatGPT (gpt-3.5-turbo), state-of-the-art Vietnamese-English neural machine translation models and pre-trained bilingual/multilingual sequence-to-sequence models on our new MedEV dataset. Experimental results show that the best performance is achieved by fine-tuning "vinai-translate" for each translation direction. We publicly release our dataset to promote further research.
Submission history
Access paper:.
- HTML (experimental)
- Other Formats
References & Citations
- Google Scholar
- Semantic Scholar
BibTeX formatted citation

Bibliographic and Citation Tools
Code, data and media associated with this article, recommenders and search tools.
- Institution
arXivLabs: experimental projects with community collaborators
arXivLabs is a framework that allows collaborators to develop and share new arXiv features directly on our website.
Both individuals and organizations that work with arXivLabs have embraced and accepted our values of openness, community, excellence, and user data privacy. arXiv is committed to these values and only works with partners that adhere to them.
Have an idea for a project that will add value for arXiv's community? Learn more about arXivLabs .
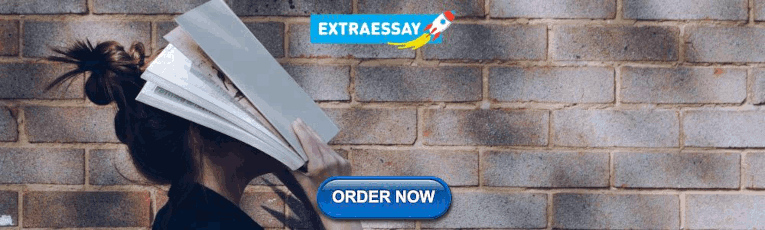
VIDEO
COMMENTS
Methods. The first part of this paper reviews the methods used to synthesise quantitative effectiveness evidence in public health guidelines by the National Institute for Health and Care Excellence (NICE) that had been published or updated since the previous review in 2012 until the 19th August 2019.The second part of this paper provides an update of the statistical methods and explains how ...
Background Identifying determinants of health and understanding their role in health production constitutes an important research theme. We aimed to document the state of recent multi-country research on this theme in the literature. Methods We followed the PRISMA-ScR guidelines to systematically identify, triage and review literature (January 2013—July 2019). We searched for studies that ...
There has been an explosion of research in the field of medical education. A search of PubMed demonstrates that more than 40,000 articles have been indexed under the medical subject heading "Medical Education" since 2010, which is more than the total number of articles indexed under this heading in the 1980s and 1990s combined.
SUBMIT PAPER. SAGE Open Medicine. Impact Factor: 2.3 / 5-Year Impact ... First published online April 20, 2020. Living with a chronic disease: A quantitative study of the views of patients with a chronic disease on the change in their life situation ... Geriatric Medicine and Clinical Osteoporosis Research School, Institute of Medicine ...
The COVID-19 pandemic disrupted the United States (US) medical education system with the necessary, yet unprecedented Association of American Medical Colleges (AAMC) national recommendation to pause all student clinical rotations with in-person patient care. This study is a quantitative analysis investigating the educational and psychological effects of the pandemic on US medical students and ...
Research questions. A methodological review of NICE public health intervention guidelines by Achana et al. (2014) found that meta-analysis methods were not being used . The first part of this paper aims to update and compare, to the original review, the meta-analysis methods being used in evidence synthesis of public health intervention appraisals.
The purpose of this paper is to introduce an overview of the fundamental knowledge, principals and processes in SR. The focus of this paper is on SR especially for the synthesis of quantitative data from primary research studies that examines the effectiveness of healthcare interventions. To activate evidence-based nursing care in various ...
Background Pharmaceutical care is the pharmacist's contribution to the care of individuals to optimize medicines use and improve health outcomes. The primary tool of pharmaceutical care is medication review. Defining and classifying Drug-Related Problems (DRPs) is an essential pillar of the medication review. Our objectives were to perform a pilot of medication review in Hungarian community ...
This paper gives an overview of the main quantitative research designs relevant to nursing and healthcare. It outlines some strengths and weaknesses of the designs, provides examples to illustrate the different designs and examines some of the relevant statistical concepts.
Summary The past three decades of research have seen substantial advances in medical education, ... Quantitative Research Methods in Medical Education. Geoff Norman. Clinical Epidemiology and Biostatistics, McMaster University, Hamilton, Ontario, Canada ... Search for more papers by this author. First published: 05 October 2018. https://doi.org ...
Description. Quantitative Research in Human Biology and Medicine reflects the author's past activities and experiences in the field of medical statistics. The book presents statistical material from a variety of medical fields. The text contains chapters that deal with different aspects of vital statistics. It provides statistical surveys of ...
Quantitative research methods employed in pharmacy practice include nonexperimental research and experimental research methods ( Austin and Sutton, 2018 )( Fig. 1 ).
The residents of remote areas mostly depend on folk knowledge of medicinal plants to cure different ailments. The present study was carried out to document and analyze traditional use regarding the medicinal plants among communities residing in Koh-e-Safaid Range northern Pakistani-Afghan border. A purposive sampling method was used for the selection of informants, and information regarding ...
Background In this methodological paper we document the interpretation of a mixed methods study and outline an approach to dealing with apparent discrepancies between qualitative and quantitative research data in a pilot study evaluating whether welfare rights advice has an impact on health and social outcomes among a population aged 60 and over. Methods Quantitative and qualitative data were ...
Abstract. Sports Medicine or Sport and Exercise Medicine is a rapidly growing speciality, which draws upon basic and applied biomedical, and clinical science for the knowledge to ensure best ...
Evidence-based medicine: what it is and what it isn't. BMJ 1996; 312(7023): 71-72. Crossref, Medline, Google Scholar; 2 Paik S. Development and clinical utility of a 21-gene recurrence score prognostic assay in patients with early breast cancer treated with tamoxifen. Oncologist 2007; 12(6): 631-635. Crossref, Medline, Google Scholar
[03/25/2024] FDA's Center for Drug Evaluation and Research (CDER) is pleased to announce the new CDER Quantitative Medicine (QM) Center of Excellence (CoE). QM involves the development and ...
Quantitative bibliometric indicators are widely used and widely misused for research assessments. Some metrics have acquired major importance in shaping and rewarding the careers of millions of scientists. Given their perceived prestige, they may be widely gamed in the current "publish or perish" or "get cited or perish" environment. This review examines several gaming practices ...
Problem statement. Medical administration is a vital aspect in medical practice as it can determine whether a patient will be exposed to risks such as death. Autocratic oath binds medical practitioners to save life and not to endanger human life and hence medical administration is equally a very sensitive topic of concern in their daily practice.
CDER's Quantitative Medicine Center of Excellence will, among other things, lead QM-related policy development and best practices to facilitate the use of QM during drug development and regulatory assessment and facilitate outreach to scientific societies, patient advocacy groups, and other key stakeholders.
The News. On the eve of oral arguments in a Supreme Court case that could affect future access to abortion pills, new research shows the fast-growing use of medication abortion nationally and the ...
For each beer, we measure over 200 chemical properties, perform quantitative descriptive sensory analysis with a trained tasting panel and map data from over 180,000 consumer reviews to train 10 ...
Machine translation for Vietnamese-English in the medical domain is still an under-explored research area. In this paper, we introduce MedEV -- a high-quality Vietnamese-English parallel dataset constructed specifically for the medical domain, comprising approximately 360K sentence pairs. We conduct extensive experiments comparing Google Translate, ChatGPT (gpt-3.5-turbo), state-of-the-art ...