SSCI Header The online and residential MS in Geographic Information Science and Technology provides state-of-the-art education that draws upon scientific principles and concepts with core geographic information technologies (geographic information systems, global positioning systems and remote sensing, among others). Students choose from three tracks that provide a foundation for professional work in diverse occupations and disciplines that rely on geospatial data, analysis and visualization. Course RequirementsTwenty-eight units of graduate work are required. Core Courses (12 units)All students complete the following courses: - SSCI 581 Concepts for Spatial Thinking Units: 4
- SSCI 587 Spatial Data Acquisition Units: 4
- SSCI 594a Master’s Thesis Units: 2
- SSCI 594b Master’s Thesis Units: 2
Course TracksStudents must choose one of the following tracks: Spatial Data Management (12 units)Students in the Spatial Data Management track must take the following courses: - SSCI 582 Spatial Databases Units: 4
- SSCI 585 Geospatial Technology Project Management Units: 4
- SSCI 588 Remote Sensing for GIS Units: 4
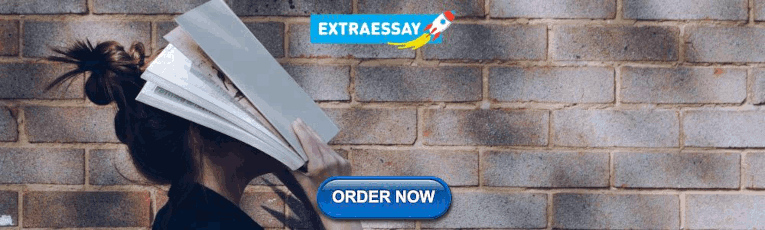 Electives (4 units)Students in the Spatial Data Management track must choose one of the following electives: - SSCI 575 Spatial Data Science Units: 4
- SSCI 576 Remote Sensing Applications and Emerging Technologies Units: 4
- SSCI 591 Web and Mobile GIS Units: 4
Spatial Computing (12 units)Students in the Spatial Computing track must take the following courses: - SSCI 586 GIS Programming and Customization Units: 4
Students in the Spatial Computing track must choose one of the following electives; - SSCI 589 Cartography and Visualization Units: 4
Spatial Analytics (12 units)Students in the Spatial Analytics track must take the following courses: - SSCI 574 Spatial Econometrics Units: 4
- SSCI 583 Spatial Analysis and Modeling Units: 4
Students in the Spatial Analytics track must choose one of the following electives: Additional RequirementsAll electives are chosen in direct consultation with the student’s academic adviser based on background, academic interests, etc. The courses in this program are open to students living and/or working anywhere, including students at USC’s Los Angeles, Orange County, Sacramento and Washington, D.C. centers. The master’s program can be completed in two to three years as long as students take one or two courses in each of the fall, spring and summer semesters. Continuous enrollment in the fall, spring and summer terms is required in this program, including SSCI 594a , SSCI 594b and SSCI 594z summer registration. Admission RequirementsFour groups of students are served by this program: - New students who wish to apply directly to the geographic information science and technology master’s program.
- Students currently enrolled in the geographic information science and technology graduate certificate program since this certificate program may serve as a possible “stepping stone” toward the master’s program.
- Students currently matriculated in a USC master’s or doctoral degree program.
- USC undergraduate students who want to stay for a fifth year and earn both bachelor’s and master’s degrees.
Candidates for admission among the first two groups of students must have: (1) a BA or BS degree or its international equivalent; (2) a minimum 3.0 GPA (A = 4.0). All course work taken at the undergraduate level is used to calculate the GPA. Exceptions will be made in cases of very high GRE scores or some other compelling evidence of potential to excel in graduate studies (e.g., outstanding letters of recommendation). Preference will be given to candidates with significant professional experience working with geographic information systems and related geospatial technologies. Application ProceduresApplicants are required to submit the following documents: (1) completed application for admission, which can be found online at usc.edu/admission/graduate ; (2) statement of purpose; (3) a writing sample; (4) official transcripts from all schools previously attended; and (5) two letters of recommendation. International students must submit TOEFL scores with a minimum score of 100 on the Internet-based examination, or an IELTS score of 7. The statement of purpose should be uploaded into the online application. This statement should: (1) describe the student’s motivation, field of interest and career goals; and (2) identify potential projects that the student might pursue for the master’s thesis project. The master’s program utilizes rolling admissions and enrollment based on the standard academic calendar. This means that students may start the program in either the fall, spring or summer semesters. Those interested in learning more about this program should contact the Spatial Sciences Institute, University of Southern California, 3616 Trousdale Parkway, AHF B55B, Los Angeles, CA 90089-0374. Advertisement  Review of Geospatial Technology for Infectious Disease Surveillance: Use Case on COVID-19- Review Article
- Published: 18 August 2020
- Volume 48 , pages 1121–1138, ( 2020 )
Cite this article - Sameer Saran 1 ,
- Priyanka Singh 1 ,
- Vishal Kumar 1 &
- Prakash Chauhan 1
11k Accesses 32 Citations 3 Altmetric Explore all metrics This paper discusses on the increasing relevancy of geospatial technologies such as geographic information system (GIS) in the public health domain, particularly for the infectious disease surveillance and modelling strategies. Traditionally, the disease mapping tasks have faced many challenges—(1) authors rarely documented the evidence that were used to create map, (2) before evolution of GIS, many errors aroused in mapping tasks which were expanded extremely at global scales, and (3) there were no fidelity assessment of maps which resulted in inaccurate precision. This study on infectious diseases geo-surveillance is divided into four broad sections with emphasis on handling geographical and temporal issues to help in public health decision-making and planning policies: (1) geospatial mapping of diseases using its spatial and temporal information to understand their behaviour across geography; (2) the citizen’s involvement as volunteers in giving health and disease data to assess the critical situation for disease’s spread and prevention in neighbourhood effect; (3) scientific analysis of health-related behaviour using mathematical epidemiological and geo-statistical approaches with (4) capacity building program. To illustrate each theme, recent case studies are cited and case studies are performed on COVID-19 to demonstrate selected models. Similar content being viewed by others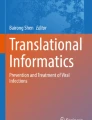 Health-Based Geographic Information Systems for Mapping and Risk Modeling of Infectious Diseases and COVID-19 to Support Spatial Decision-Making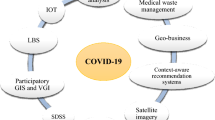 Ten GIS-Based Solutions for Managing and Controlling COVID-19 Pandemic Outbreak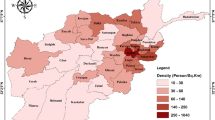 Spatial distribution and mapping of COVID-19 pandemic in Afghanistan using GIS techniqueAvoid common mistakes on your manuscript. IntroductionThe public health sector’s increasing demand for mapping, analytics and visualization had started a date back in the last 20 years, which has resulted in a growing information-age technology for communicable disease surveillance and epidemiology (Baker et al. 1995 ; Bos and Blobel 2007 ; Friede et al. 1993 ; Friede 1995 ; Khan et al. 2010 ; Reeder et al. 2012 ; Yu and Edberg 2005 ). This continuous public health burden with advances in information technology combined with spatial data led to the development of various tools and systems that provides visualization of disease data in space and time (Dredger et al. 2007 ; Kothari et al. 2008 ; Robertson and Nelson 2010 ; Schriml et al. 2009 ). The first integral definition of public health was given by Winslow ( 1920 ) as “science and art of preventing disease, prolonging life, and promoting health through the organized efforts and informed choices of society, organizations, public and private communities, and individuals”. The American Public Health Association (APHA) mentioned public health as a practice of preventing the spread of disease and an aim of promoting good health from small communities to across the world (Turnock 2012 ). Advances in information technology and spatial features resulted in geospatial technology which is acute for mapping, surveillance, predicting outbreaks, detecting clustering and analysing spread patterns of infectious diseases with epidemic or pandemic potential in communities and across territories (AvRuskin et al. 2004 ; Carpenter 2011 ; Castronovo et al. 2009 ; Dominkovics et al. 2011 ; Gao et al. 2008 ; Heymann and Brilliant 2011 ; Hills et al. 2008 ; Klompas et al. 2011 ; Reis et al. 2007 ). Geospatial technology has provided visualization and analytical tools to public health professionals and decision makers to execute diseases control programs in affected and/or suspected regions and make analysis and predictions possible that was once technologically out of reach. Geospatial technology includes geographical information systems (GIS), global positioning systems (GPS) and satellite-based technologies such as remote sensing (RS). GIS is known for geographic data capture, input, update, manipulation, transformation, analysis, query, modelling and visualization of all forms of geographically referenced information through the set of computer programs (Bonham-Carter 2014 ). GPS provides positioning, navigation and timing (PNT) services by capturing data from satellites and providing it to users (Eldredge et al. 2010 ), and RS is an earth observation instrument that delivers regional information on climatic factors and landscape features. Therefore, GPS and RS provide regional and spatial information, while GIS provides geospatial data integration as well as accurate geospatial analysis in real-time manner (Zhen et al. 2010 ). Geospatial Technology and Infectious Disease SurveillanceInfectious diseases mostly adapts anti-microbial and mobility features later formed in a shape of pandemic and/or epidemic (Chen et al. 2019 ; Cheng et al. 2019 ; Lee and Nishiura 2019 ), which forced public health authorities to understand not only the diseases virulence, but also its demographic and environmental factors that helps in making spread patterns though space and time domain (Croner 2004 ). For example, the global spread of highly pathogenic avian influenza (HPAI) H5N1 in 2005–06 with no effective vaccines led to concern among public health decision makers, in spite of many international programs (Rappole and Hubálek 2006 ) 32 . The reason behind their concern was they were lacking of disease surveillance tool in its initial stage which caused inaccessibility to populations at risk, and faced difficulties in implementing immunization strategies at a global scale (Kitler et al. 2002 ; Stoto et al. 2004 ). However, the impact of environmental and demographic factors also plays a major role as this can inform about the interaction between hosts and pathogens, and patterns of spread in space and time. The GIS provides dynamic maps to understand geographical distribution of diseases for analysis on frequency of cases, disease mapping, spatial cluster of diseases, disease association with environmental factors, network analysis, etc. With such a visualization and analytical capabilities, GIS technology is holding a widespread growth in public health (Ahmad et al. 2011a , b ; Booman et al. 2000 ; Hanafi-Bojd et al. 2012 ; Kolivras 2006 ; Martin et al. 2002 ; Nykiforuk and Flaman 2011 ; Abdul Rasam et al. 2011 ; Zhang et al. 2008 ; Zhen et al. 2010 ). The seamless integration of GIS with real-time infectious disease-related diverse datasets through web-based mapping leads to the development of geospatial dashboard, geospatial service framework, for infectious disease surveillance (Dent 2006 ; Gao et al. 2008 ; Yun 2007 ). The infectious disease-related data might include disease surveillance data (active/confirmed cases) and health system data (hospital visits, emergency services availability, nurse/doctor availability, ICU/bed availability). Many open-source geospatial standards of Open Geospatial Consortium (OGC) are used as a Web Map Service (WMS), Web Coverage Service (WCS), Web Processing Service (WPS), Web Feature Service (WFS), etc, (Bulatović et al. 2010 ; Gao et al. 2008 ) to visualize, access, publish and manipulate geospatial resources. Also, many other popular industrial geospatial standards are developed by ESRI, Google, Yahoo, and MapInfo (Granell et al. 2014 ) to fetch location-based data and provide infectious disease surveillance dashboard to monitor and control the geographically spread of disease (Zhang et al. 2007 ). The Geocoded Really Simple Syndication (GeoRSS) tagged XML files from GeoRSS services can also be used to provide geocoded infectious disease news from social media platform (Tolentino et al. 2007 ; Kass-Hout and Alhinnawi 2013a , b ; Kodong et al. 2020 ). Historical ContextThe mapping of infectious diseases using geospatial and information technology to benefit public health is not a new way of tracking the diseases (Ahmad et al. 2017 ; Cui et al. 2011 ; Hirsch 1883 ; Hornsby 2000 ; Matthew et al. 2004 ; May 1951 ; Mujica 2013 ; Nicholson and Mather 1996 ; Noble et al. 2012 ; Perl and Moalem 2006 ; Williams et al. 1986 ). The historical disease mapping has faced many challenges—(1) authors rarely documented the evidence that were used to create map, (2) after mapping had been implemented before the beginning of geographical information systems, many errors arouse which were expanded extremely at global scales, and (3) there were no fidelity assessment of maps which resulted in inaccurate precision. But nowadays, wide range of geospatial applications are available in public health community with a possibilities of visualization, analysis, detection of clusters formed and calculate disease-related metrics such as incidence and prevalence rate (Beck et al. 2000 ; Clarke et al. 1996 ; Hay 2000 ; Jacquez 2000 ; Kleinschmidt et al. 2000 ; Lawson and Leimich 2000 ; Moore and Carpenter 1999 ; Robinson 2000 ; Wilkinson et al. 1998 ). The earliest mapping for visualisation of the link between disease and place was done in 1694 on plague epidemic in Italy (Dent 2006 ). During cholera outbreak in 1854, the study of physician John Snow had made a novel contribution in history of public health and epidemiology by using cartography applications and geographic visualization in fighting cholera. After 225 years, the maps were identified as a communication tool in understanding and tracking of infectious diseases, such as the 1918 influenza pandemic, yellow fever, and cholera. Since then revolution of web-based tools started in applied health geography (Boulos 2008 ). The trend of infectious disease mapping could be seen from 2014 review of the Health GIS literature which demonstrated that 248 research papers out of 865 were focused on infectious disease mapping (Lyseen et al. 2014 ). The ongoing pandemic outbreak targeting humans’ respiratory system was recently discovered in December 2019 by the name of Coronavirus Disease 19 (Covid-19) (World Health Organization) from a cluster of patients with acute respiratory distress syndrome in Wuhan, Hubei Province, China (Huang et al. 2020 ; Lu et al. 2020a , b ) and spread globally by March 2020. This pathogenic disease is structurally related to the Coronavirus (CoV), which belongs to family Coronaviridae and the order Nidovirales . This family is classified into four genera— Alphacoronavirus , Betacoronavirus , Gammacoronavirus , and Deltacoronavirus , on the basis of their phylogenetic and genomic analysis. The species of Alphacoronavirus and Betacoronavirus infect mammals, causes respiratory illness in humans and gastroenteritis in animals, while species of Gammacoronaviruses and Deltacoronaviruses infect birds, but some of them can also infect mammals (Woo et al. 2012 ). The two virus species from Betacoronavirus genus—Severe Acute Respiratory Syndrome (SARS-CoV) or Middle East Respiratory Syndrome (MERS-CoV)—had earlier demonstrated that coronaviruses can cause significant public threat (Ge et al. 2013 ). The COVID-19 is categorized into Betacoronavirus by World Health Organization (WHO) on the basis of genomic sequencing analysis of lower respiratory tract samples, which is obtained from total of nine patients (Huang et al. 2020 ; Lu et al. 2020a , b ). COVID-19 has started behaving like the once-in-a-century pandemic by affecting healthy adults as well as elderly people with some health issues and by infecting others at an exponential rate of increase than SARS or MERS. Geospatial TechnologyDuring occurrence of diseases, geospatial technologies and services could help in representing the spatio-temporal information and in analysing the dynamic spread of diseases. As mentioned by Boulos ( 2004 ), geospatial technologies and services, which performs in real time manner, are tremendously relevant to create a “spatial health information infrastructure”. In this section, a review on many geospatial technologies with enabled IT services is carried out to understand and analyse the spread and outbreak of disease with a case study on COVID-19 pandemic. The expansion of Citizen Science from biodiversity and ecological domain (Haklay 2013 ; Miller-Rushing et al. 2012 ) to public health community across spatial extents made an urgent need to study its different forms (Crowl et al. 2008 ). The in-depth report of EU describes taxonomy of Citizen Science in three levels (European Commission 2013 ), described in Roy et al. ( 2012 ), Wiggins and Crowston ( 2011 ) and Haklay ( 2013 ). Roy et al. ( 2012 ) categorized Citizen Science by participant’s number and of their spread (“local” and “mass”) and “thoroughness” (time and resource investment), or King et al. ( 2016 ) described “for the people, with the people, or by the people” about Citizen Science activities. Wiggins and Crownston ( 2011 ) classified Citizen Science projects in conservation (managing natural resources), action (addressing local issues and concerns), investigation (answering scientific questions), and education (providing knowledge to citizens). Haklay ( 2013 ) classified Citizen Science into four levels based on participant’s engagement—(1) level 1 is crowdsourcing in which citizens with less or no knowledge on activity perform as sensors to complete computing tasks, (2) level 2 is distributed intelligence where citizens are being trained with skills for interpretation of collected data, (3) level 3 is participatory science in which citizens decide about research questions and types of data to be collected, and (4) level 4 is extreme where citizens are fully involved in defining research strategies, data collection, data interpretation, and performing scientific analysis. Apparently, the concept of Citizen Science is rare in public health domain, but some of its contribution seen in some studies which not only helps in predicting disease risks but also in combating the infectious diseases (Curtis-Robles et al. 2015 ; Palmer et al. 2017 ; Smolinski et al. 2015 ; Wilson et al. 2014 ). Another approach similar to Citizen Science is ‘popular epidemiology’, in which experts and laypersons jointly collect environmental data responsible for particular health consequences (Brown 2013 ), or ‘street science’ as a process in which general public communities actively engaged in defining problems, framing of research questions, and decision-making activities about research design (Corburn 2007 ). Crowdsource/VGI Mobile AppsDespite technological and computational developments in GeoWeb, many web technologies (such as jQuery and AJAX), mapping APIs (like Google), and GPS devices resulted in a new revolution of neogeography (Turner 2006 ), where mapping is done by crowd and can be reached by anyone from general public members group. Such revolution brought a trend of Volunteered Geographic Information (VGI), which is first coined and explained by Goodchild ( 2007a ). According to Goodchild ( 2007b ), VGI highlighted the human capabilities in collecting geospatial information by using five senses and then integrating with external sensors of mobile devices like GPS, accelerometer, camera, digital compass and microphone gives valuable datasets which can neither be retrieved from satellite imagery nor collected with any GPS receivers. Another successful term in geospatial mapping using mobile technology is crowdsourcing (Heipke 2010 ; Hudson-Smith et al. 2009 ), which was coined by Howe ( 2006 ), that involves the collection of geospatial information or mapping of any particular activity by an undefined crowd or network of people. Both terms, VGI and crowdsourcing, slightly differ but they are usually recognized as a synonyms or even as a combined term, “crowdsourcing geographic information” (Sui et al. 2013 ). Over the last decade, VGI-oriented open-source mobile apps are EpiCollect (Aanensen et al. 2009 ) for ecology and epidemiology; NoiseTube (Maisonneuve et al. 2010 ) ( http://noisetube.net ) and Noise Battle (Garcia-Martí et al. 2013 ) for noise monitoring; Skywatch Windoo ( http://windoo.ch ) for weather monitoring; Mappiness ( http://www.mappiness.org.uk ) for behavioural analysis (MacKerron and Mourato 2010 ). The open-source mechanism for data collection using Android devices can be performed by Open Data Kit (ODK) suite 107 ( https://opendatakit.org ), which is composed of ODK Collect and ODK Aggregate. ODK Collect ( https://opendatakit.org/use/collect ) provides a customizable framework for geospatial data collection, and ODK Aggregate is a web application that runs on Apache Tomcat server ( http://tomcat.apache.org ) to store collected data through a synchronization with a database, for example, PostgreSQL (Brunette et al. 2013 ). As such, suite’s performance can be seen in various activities like agricultural monitoring (Krosing and Roybal 2013 ), monitoring of deforestation and school attendance, documentation of war crimes, and health programs (Anokwa et al. 2009 ). Digital Contact TracingNowadays, COVID-19 has become the greatest threat for public health in last 100 years, and due to such pandemic, various levels of lockdown are issued across the world to break its chain of infection transmission. However, this is the first approach to invade the contagion, but once it would be lifted, this pandemic would start in a new way and might reach its highest peak by infecting more and more population (Ferguson et al. 2020 ). Therefore, to combat with such a global pandemic threat, another approach is discovered by a group of researchers, known as digital contact tracing. Smartphone-based contact tracing is known as a digital contact tracing which presents a sustainable solution to limit the transmission of infectious disease by tracing their potential transmission routes in a population; however, such an app presents significant concerns regarding privacy. The digital contact tracing works on the principle of ‘crowdsource data’ by measuring the proximity to an infectious person. In previous diseases risk surveillance, the contact tracing apps were used to pool location timestamped data to determine the exposures to risk of infections (Sacks et al. 2015 ). Such data are highly personal and lead many privacy concerns (Smith et al. 2012 ), but they were not always accurate to infer the exposure risks due to noisy data (Farrahi et al. 2014 ). Therefore, various smartphone apps are developed in COVID-19 pandemic in which some apps use location for proximity and some of them are not using location services of mobile device subject to the privacy-preserving nature. COVID-19 Contact TracingIn order to illuminate the epidemiology of COVID-19 and to characterize its severity (Lipsitch et al. 2020 ), there is an urgent need of digital platform that captures real-time accurate information on COVID-19 patients, diseases, diagnosis, treatment, and clinical reports, and whom they get interacted at which place to detect clusters and generate alerts. Such information may help in understanding risk factors of infection and in predicting the next generation of infectious persons (FitzGerald 2020 ). Addressing this unprecedented challenge, many mobile apps have been developed and are being used at large scale, and some of them are as follows: COVID Symptom Study (COVID Symptom Tracker)—This mobile app is developed in collaboration of Zoe Global Ltd., a digital health care company, and a group of academic scientists from Massachusetts General Hospital and King’s College London, which was launched in UK on March 24, 2020, and became available after 5 days in USA. This app enquires about age, location, and other diseases risks, and also, a self-reporting function is enabled which is associated with COVID-19 infection and exposure (Drew et al. 2020 ). This app retrieve updates on healthcare worker’s experiences who are on COVID-19 duty, their stress and anxiety, and use of personal protective equipment (PPE) kits are being surveyed through this app to observe intensity of health care workers (Drew et al. 2020 ). Aarogya Setu—This mobile app is launched on April 2, 2020, by Government of India to aware general public on COVID-19 symptoms, government advisory measures, online consultation facility, and dynamics of disease. This app implemented crowdsourcing approach by which general public members enter their details for self-assessment, and this assessment is then used to trace the infectious contacts or agents as a digital contact tracing concept. This app uses location services to geolocate the users and Bluetooth to maintain the log of contacts when one user/device comes in contact with another user/device, and as such, digital contact tracing activity helps in identifying the cluster of diseases and communities which are at risk of infection. The Aarogya Setu app was downloaded by ~ 100 million users within 40 days of its launch (Upadhyay 2020 ), and by using app’s crowdsource data, the Indian government detected approx. 13,000 positive cases, informed 130,000 probable users of being at risk, and identified 300 potential clusters (The Times of India). Numerous digital contact tracing apps are in use in different parts of world—TrackCOVID (Yasaka et al. 2020 ), TraceTogether (Bay et al. 2020 ), WeTrace (De Carli et al. 2020 ), and Google and Apple’s recently announced joint initiative (Li and Guo 2020 ). COVID-19 Data Visualization and Exploratory Data AnalysisWith early experiences of epidemics such as 2002–2003 SARS-CoV (Boulos 2004 ) and the 2012–2014 MERS-CoV (Gikonyo et al. 2018 ), and other seasonal flu’s, online real-time or near-real-time mapping of diseases’ occurrences using geospatial technologies and web applications have always been used as a pivotal web-based tools in tracking health threats and combating infectious diseases. This section described a range of mapping dashboards based on geospatial technologies for tracking and unfolding the coronavirus disease around the world. Some of the global and national geospatial initiatives with an aim to supply information faster than diseases are as summarized in Table 1 . Infectious Diseases ModellingThe intention of infectious diseases surveillance is to detect epidemics in their early stages so that the countermeasures could be taken for preventing its wide spread. Such surveillance tasks require many epidemiological and statistical methods with geospatial features in investigating epidemics, preferably from localized areas. The reason for preferring the local areas for investigation is because epidemics generally emerged in small areas and then spread widely, if they are not controlled. However, some methods require rigorous conventions in their underlying models and are too problematical to be applied on small areas. Therefore, this section discusses simple methods for detecting diseases prevalence with case studies on small datasets which would be more useful for public health activities. Clustering deals with the study of spatial-temporal patterns of the spread of communicable diseases and identification of other disease-related aspects allied with heterogeneous geographical distribution which might be helpful in elucidating the diseases’ spread mechanism. Such study and analysis on space-time patterns is a kind of disease surveillance which involves detecting the outbreak clusters of active cases, monitoring of localisation and isolation of infectious agents, and relative risks assessment of affected sites at early stage (Clements et al. 2013 ; Cromley 2019 ; Kulldorff 2001 ). This study on geographical clustering of infectious diseases with temporal features helps in making strategies that dynamically update on emergence source of disease outbreak to help epidemiologists and decision makers for identification of spread and risk zones. Thus, clustering helps to enable timely prevention and containment measures and timely resource allocation to mitigate the diffusion of diseases. Based on space-time surveillance of diseases, space-time scan statistic (Kulldorff 1997 ) is one of the cluster detection tools which is widely used in geographical surveillance of diseases during epidemic and/or pandemic. The space-time scan statistic comes with two versions—prospective and retrospective (Desjardins et al. 2018 ; Owusu et al. 2019 ), and difference between both is that prospective neglects historical clusters which may have previously occurred before the most current time period of analysis with no health threat (Kulldorff 2001 ). Therefore, the prospective version of space-time scan statistic is commonly used to detect statistically significant active or evolving clusters of diseases for the present time period, and when more data become available, the tool can be re-run to detect new evolving clusters with update on relative risks for each affected sites. Previously, the prospective space-time scan statistic was used in thyroid cancer (Kulldorff 2001 ), shigellosis (Jones et al. 2006 ), measles (Yin et al. 2007 ), syndromic surveillance (Yih et al. 2010 ), and many other diseases. However, cluster analysis of diseases can be performed through several packages and libraries in R (Gómez-Rubio et al. 2005 ) and Python software (Yeng et al. 2019 ). The contribution of cluster detections and analysis in COVID-19 pandemic is becoming useful nowadays as it detects active and emerging clusters of COVID-19 and notify epidemiologist, decision makers, and public health care officials, which can help in eradicating infections from affected sites, and improving interventions, quarantine, and isolation measures. The significant applications of clustering with respect to infectious diseases modelling are demonstrated across the world (Zarikas et al. 2020 ), for example, India (Bhosale and Shinde 2020 ), USA (Desjardins et al. 2020 ; Hohl et al. 2020 ), Brazil (Martines et al. 2020 ), Italy (Cereda et al. 2020 ), China (Ji et al. 2020 ; Liu et al. 2020a , b ; Qiu et al. 2020 ; Zhang et al. 2020 ), Singapore (Bhosale and Shinde 2020 ; Pung et al. 2020 ), South Korea (Shim et al. 2020 ), French Alps (Danis et al. 2020 ), Germany (Pfefferle et al. 2020 ), Sergipe (Andrade et al. 2020 ), etc. Outlier AnalysisThe outlier is defined by Hawkins ( 1980 ) as “an observation which deviates so much from the other observations as to arouse suspicions that it was generated by a different mechanism.” In other words, when data generation process starts behaving abnormal and reflects the abnormalities or errors in data, such abnormalities are known as outliers (Bansal et al. 2016 ). However, the outliers generally hold advantageous information about the systems unusual characteristics and entities, which impact the data generation process. Some of the useful applications of outliers in diseases are (Cleynen et al. 2016 ; Dai and Bikdash 2016 ; Krishnan et al. 2017 ; Lo et al. 2015 ; Prensner et al. 2011 ; Washington 2007 ; Wu and Krishnan 2010 ). Clustering algorithms are optimized to find clusters rather than outliers, and the accuracy of outlier detection depends on how good the clustering algorithm captures the structure of clusters. Maximum Entropy Modelling (Maxent) ApproachIn context of disease systems, disease transmission risks depend on distribution of pathogens (species) in space and time in some complex environmental conditions (Townsend 2015 ), and as such treatments are focused mainly on spatial dimensions; therefore, diseases transmission risks are purely handled through geographical phenomena. Such geographical link with diseases leads to the challenge of spatial mapping of disease transmission which overcame through the branches of biodiversity science—ecology and biogeography. Such approach of ecological and biogeographical modelling can be seen from various studies on disease transmission risks mapping, for example, Arboleda et al. ( 2009 ), Deka and Morshed ( 2018 ), Ferreira et al. ( 2020 ), Holt et al. ( 2009 ), Mweya et al. ( 2013 ), Nakazawa et al. ( 2013 ), Reeves et al. ( 2015 ), Samy et al. ( 2014 ), Qian et al. ( 2014 ), Zhao et al. ( 2016 ), Zhu et al. ( 2017 ). Following recent studies on geographical mapping of pathogens causing disease transmission, machine learning-based maximum entropy method (Maxent) (Elith et al. 2011 ; Phillips 2010 ) is applied on spatial records of COVID-19 with a set of 19 bioclimatic environmental variables from WorldClim (Poggio et al. 2018 ; Ramírez Villegas and Bueno Cabrera 2009 ) to analyse their favourable environmental conditions (as shown in Fig. 1 and Table 2 ), required in maintaining its population. The Maxent principle is to estimate the target probability distribution by applying the maximum entropy to distribution which is most spread or closest. This study is carried out in R software (Ihaka and Gentleman 1996 ), and a geographical dataset consists of latitude and longitude of those regions which were affected till March, 2020. 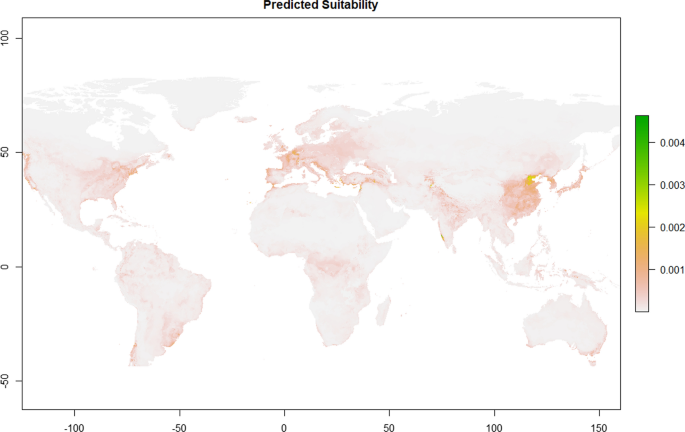 Predicted suitability of Betacoronavirus using data till March, 2020 Figure 1 depicts the habitat suitability map of virus with probability range in colour scale to visualize the high suitability (light and dark green colour), medium suitability (yellow and dark brown), low suitability (light brown colour) and unsuitable (grey colour). Table 2 lists the favourable bioclimatic variables and their contribution in percent in maintaining the suitability of virus. Susceptible-Infectious-Recovered (SIR) ModelEpidemiology deals with the study of pattern and occurrence of diseases in space and time associated with other factors such as environment demography, and the translation of epidemiology into mathematical equations to describe the spread of infectious diseases is known as mathematical epidemiology (Allen et al. 2008 ; Rayner and Bender 1980 ). The mathematical epidemiology model is implemented to understand the transmission dynamics of communicable diseases by categorizing population into susceptible, infectious, and recovered compartments. The first basic model, known as Susceptible-Infectious-Recovered (SIR) model, was proposed by Kermack and McKendrick ( 1991 ) to describe the transmission of epidemic diseases from individual to individual. The SIR model is a set of nonlinear ordinary differential equations, which is mathematically defined as follows: S is the class of susceptible individuals who are not yet contracted to disease, I is the class of infectious people who are now infected with disease and become infectious to infect others, R is class of recovered individuals who have recovered now and are removed from class S , N is a total population size, N = S + I + R , and t is time in days or weeks \(\beta\) is the contact rate of infected person with suspected person per day, \(\gamma\) is the infectious period and average infectious period is 1/ \(\gamma\) , µ is the per capita death rate which is adjusted by birth rate µ N . There are many other compartment models derived from the basic epidemic model, (SIR), with more compartments and transitions—(1) Susceptible-Exposed-Infectious-Recovered (SEIR) (Li and Muldowney 1995 ), (2) Susceptible-Infectious-Exposed-Recovered-Dead (SEIRD) (Piccolomiini and Zama 2020 ), (3) Susceptible-Infectious-Exposed-Recovered-Susceptible (SEIRS) (Liu and Zhang 2011 ), (4) Susceptible-Infectious-Quarantine-Recovered (SIQR) (Erdem et al. 2017 ), etc. The SIR model is implemented for COVID-19 to understand its dynamics of transmission from individual to individual in India for first 25 days of this pandemic. The data on actual cases of COVID-19 are obtained from COVID-19 India ( https://www.covid19india.org/ ) and then used in SIR model in R software. The SIR model is optimized using parameters, \(\beta = 0.00021\) and \(\gamma = \frac{1}{14}\) , against actual cases of disease (Fig. 2 ), and then, the probable SIR model is constructed using the above parameters for next 90 days to understand that how much of the population could be infected in upcoming days, as shown in Fig. 3 . However, the SIR model can be constructed and optimized for more days by increasing the population size and decreasing the contact rate. 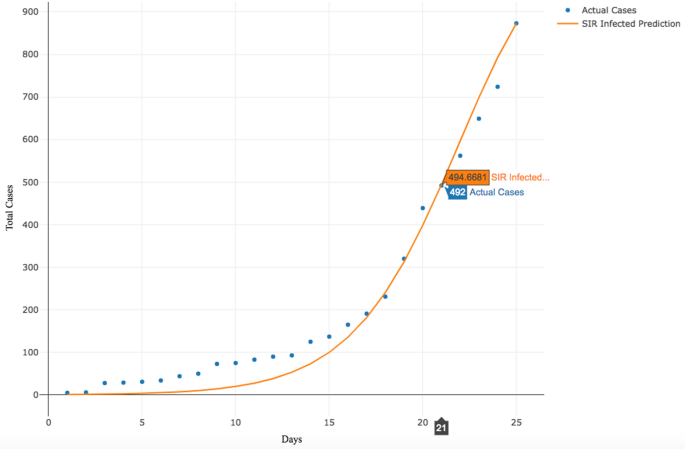 Model optimization as per actual cases for first 25 days 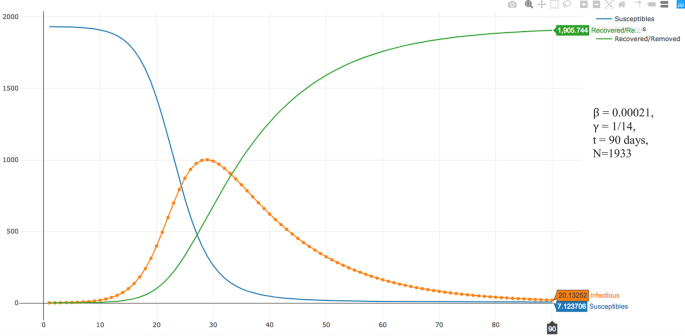 SIR model for 90 days Google Earth EngineGoogle Earth Engine (GEE) is a web-based free cloud computing platform introduced by Google, Inc., to provide a high-performance geospatial computing platform with multi-petabyte analysis-ready remote sensing big data (Casu et al. 2017 ; Gorelick et al. 2017 ). The GEE has opened an intrinsically parallel processing way for the researchers to swiftly process millions of satellite images by reducing massive computations. GEE provides a dynamic web explorer for data cataloguing, visualization, and analysis in JavaScript (Goodman 2007 ) and Python (Oliphant 2007 ). Also, GEE provides a time-lapse video tool for measuring, tracking, and visualizing changes to the Earth’s surface over the past 35 years (1984–2018). Some applications of GEE can be seen in time series analysis of snakebites (Reis et al. 2020 ), forest cover (Hansen et al. 2013 ; Johansen et al. 2015 ), tree cover loss (Tracewski et al. 2016 ), surface water (Donchyts et al. 2016 ; Pekel et al. 2016 ; Tang et al. 2016 ), populated areas (Patela et al. 2015 ), cropland and soils (Padarian et al. 2015 ; Xiong et al. 2017 ) and various applications (Dong et al. 2016 ; Joshi et al. 2016 ; Lee et al. 2016 ). COVID-19 affects respiratory system of humans (Marini and Gattinoni 2020 ; Rothan and Byrareddy 2020 ;Xu et al. 2020 ), and based on previous studies, this can be more prone to those who are in old age (Wu et al. 2020 ), smoking habits (Liu et al. 2020a , b ), respiratory complications (Chen et al. 2020 ; Sohrabi et al. 2020 ) due to long-term exposure to harmful pollutants. For instance, NO 2 is one of the harmful air pollutant gases which might be responsible for causing chronic obstructive pulmonary disease (COPD) (Abbey et al. 1993 ; Euler et al. 1988 ), hypertension (Shin et al. 2020 ), heart diseases (Gan et al. 2012 ; Mann et al. 2002 ), diabetes (Shin et al. 2020 ), lung injuries (Bowatte et al. 2017 ), and even death in humans, which can make them susceptible to various respiratory viruses or syndromes. In the current pandemic situation, many studies are carried out to analyse that NO 2 could be one of the responsible factors for COVID-19 fatality (Baldasano 2020 ; Dutheil et al. 2020 ; Frontera et al. 2020 ; Kumari and Toshniwal 2020 ; Nakada and Urban 2020 ; Ogen 2020 ; Pansini and Fornacca 2020 ; Ranjan et al. 2020 ; Zoran et al. 2020 ). Hotspot and Coldspot AnalysisThe aim of many geosurveillance systems or surveys is to find hazard level of the subject, which can be later converted to risk. The idea of risk mapping is to assign a probability value to the occurrence of defined condition indicating some form of unusual aggregation in spatial information (Jeefoo et al. 2011 ; Osei and Duker 2008 ). The spatial clustering of high values is known as hotspot, whereas the cluster with low values is known as coldspot (Jana and Sar 2016 ). With advancements in GIS, the hotspot (or coldspot) detection method is widely used in disease surveillance system (Ahmad et al. 2015 ; Bhunia et al. 2013 ; Jana and Sar 2016 ; Jeefoo et al. 2011 ; Ng et al. 2018 ; Osei and Duker 2008 ; Panahi et al. 2020 ; Willerson et al. 1981 ). In the current pandemic situation, various studies were carried out on detecting hotspot as well as coldspot in different countries to fight against it, for example, Al-Jumaili and Hamed ( 2020 ), Arab-Mazar et al. 2020 , Bouffanais and Lim ( 2020 ), Eckerle and Meyer ( 2020 ), Mo et al. ( 2020 ). With the help of such disease modelling algorithms, various tasks are carried out for surveillance of current pandemic, and some of them are summarized in Table 3 . Capacity BuildingThe online capacity building and outreach programme was organized at Indian Institute of Remote Sensing (IIRS), Dehradun, to make public aware about application and services of geospatial technology, and various mathematical and statistical modelling tools for automated analysis on infectious disease surveillance and control. The programme on geospatial technology in conjunction with COVID-19 was held during June 15–19, 2020, on the topic “Health GIS: Geoinformatics for COVID-19” with 3601 participants from 494 institutions and universities ( https://www.youtube.com/watch?v=_I09X4jdbfg&t=29s ). The lectures were delivered on role of geospatial technology, mobile and web GIS in COVID 19 pandemic, public health surveillance system, geo-processing for public health, disease risk mapping, exploratory data analysis, and dynamic epidemiology modelling ( https://www.youtube.com/watch?v=u2UOGCgTlo4&t=269s ). ConclusionsThis comprehensive review on geospatial technology and services for infectious diseases surveillance is to illuminate the path for decision makers and public health officials for implementation of web-based spatio-temporal health information system and epidemiological modelling tools. Geospatial technology is having rich set of tools for understanding spatial and temporal aspects in wide range of disciplines, dominantly in geospatial health research for establishing the links between diseases and in its suitable environment. Also, this provides various geo-statistical approaches to perform analysis in finding the interconnectedness spatial and temporal links, outbreak detection, identifying population at risk, movement tracing of infectious agents, investigation of environmental factors responsible in outbreak, and hotspot and coldspot analysis of outbreak location. However, there are many mathematical modelling algorithms also used for estimating the prevalence and incidence rate of disease, proportion of population that would be infected at any particular time, and severity and potential scale of epidemic or pandemic. Such analytical capable information systems are for automated visualization and analysis of every dimensions of infectious disease related to its transmission, demographic and environmental recognitions, and statistics. This review paper concluded that geospatial applications and dynamic modelling algorithms could offer a well-timed solution to all time historic challenge of humankind in understanding the disease outbreaks, vulnerabilities to population health and adaption of upcoming generation. Aanensen, D. M., Huntley, D. M., Feil, E. J., Al-Own, F., & Spratt, B. G. (2009). EpiCollect: Linking smartphones to web applications for epidemiology, ecology and community data collection. PLoS ONE . https://doi.org/10.1371/journal.pone.0006968 . Article Google Scholar Abbey, D. E., Colome, S. D., Mills, P. K., Burchette, R., Beeson, W. L., & Tian, Y. (1993). Chronic disease associated with long-term concentrations of nitrogen dioxide. Journal of Exposure Analysis and Environmental Epidemiology, 3, 181–202. Google Scholar Abdul Rasam, A. R., Mohd Noor, A. M., Ahmad, N., & Ghazali, R. (2011). MyGeoHealth: GIS-based cholera transmission risk system in Sabah, Malaysia. In Proceedings — 2011 IEEE 7th international colloquium on signal processing and its applications, CSPA 2011 . https://doi.org/10.1109/CSPA.2011.5759925 . Ahmad, F., Goparaju, L., & Qayum, A. (2017). Studying malaria epidemic for vulnerability zones: Multi-criteria approach of geospatial tools. Journal of Geoscience and Environment Protection . https://doi.org/10.4236/gep.2017.55003 . Ahmad, R., Ali, W. N. W. M., Nor, Z. M., Ismail, Z., Hadi, A. A., Ibrahim, M. N., et al. (2011a). Mapping of mosquito breeding sites in malaria endemic areas in Pos Lenjang, Kuala Lipis, Pahang, Malaysia. Malaria Journal . https://doi.org/10.1186/1475-2875-10-361 . Ahmad, R., Ali, W. N. W. M., Nor, Z. M., Ismail, Z., Hadi, A. A., Ibrahim, M. N., et al. (2011b). Mapping of mosquito breeding sites in malaria endemic areas in Pos Lenjang, Kuala Lipis, Pahang, Malaysia. Malaria Journal . https://doi.org/10.1186/1475-2875-10-361 . Ahmad, U., Ahmad, S. R., & Luqman, M. (2015). A study of polio disease in Pakistan using GIS approach. International Journal of Scientific Technology and Research, 4, 315–322. Al-Jumaili, M. H. A., & Hamed, A. S. (2020). Coronavirus disease-19: Outbreaks in Iraq. Allen, L. J., Brauer, F., Van den Driessche, P., & Wu, J. (2008). Mathematical epidemiology (Vol. 1945). Berlin: Springer. Book Google Scholar Andrade, L. A., Gomes, D. S., Góes, M. A. D. O., Souza, M. S. F. D., Teixeira, D. C. P., Ribeiro, C. J. N., et al. (2020). Surveillance of the first cases of COVID-19 in Sergipe using a prospective spatiotemporal analysis: the spatial dispersion and its public health implications. Revista da Sociedade Brasileira de Medicina Tropical . https://doi.org/10.1590/0037-8682-0287-2020 . Anokwa, Y., Hartung, C., Brunette, W., Borriello, G., & Lerer, A. (2009). Open source data collection in the developing world. Computer . https://doi.org/10.1109/MC.2009.328 . Arab-Mazar, Z., Sah, R., Rabaan, A. A., Dhama, K., & Rodriguez-Morales, A. J. (2020). Mapping the incidence of the COVID-19 hotspot in Iran—Implications for travellers. Travel Medicine and Infectious Disease . https://doi.org/10.1016/j.tmaid.2020.101630 . Arboleda, S., Jaramillo, O. N., & Peterson, A. T. (2009). Mapping environmental dimensions of dengue fever transmission risk in the Aburrá Valley Colombia. International Journal of Environmental Research and Public Health . https://doi.org/10.3390/ijerph6123040 . AvRuskin, G. A., Jacquez, G. M., Meliker, J. R., Slotnick, M. J., Kaufmann, A. M., & Nriagu, J. O. (2004). Visualization and exploratory analysis of epidemiologic data using a novel space time information system. International Journal of Health Geographics , 3 (1), 26. Baker, E. L., Friede, A., Moulton, A. D., & Ross, D. A. (1995). CDC’s information network for public health officials (INPHO): A framework for integrated public health information and practice. Journal of Public Health Management and Practice . https://doi.org/10.1097/00124784-199500110-00009 . Baldasano, J. M. (2020). COVID-19 lockdown effects on air quality by NO 2 in the cities of Barcelona and Madrid (Spain). Science of the Total Environment . https://doi.org/10.1016/j.scitotenv.2020.140353 . Bansal, R., Gaur, N., & Singh, S. N. (2016). Outlier detection: Applications and techniques in data mining. In Proceedings of the 2016 6th international conference — Cloud system and big data engineering, confluence 2016 . https://doi.org/10.1109/CONFLUENCE.2016.7508146 . Bay, J., Kek, J., Tan, A., Hau, C. S., Yongquan, L., Tan, J., et al. (2020). BlueTrace : A privacy-preserving protocol for community-driven contact tracing across borders . Singapore: Government Technology Agency. Beck, L. R., Lobitz, B. M., & Wood, B. L. (2000). Remote sensing and human health: New sensors and new opportunities. Emerging Infectious Diseases . https://doi.org/10.3201/eid0603.000301 . Bhosale, S. K., & Shinde, N. V. (2020). Outbreak of COVID-19 in India and high risk of cluster containment: A case study. Journal of Applied Pharmaceutical Research, 8 (2), 01–07. Bhunia, G. S., Kesari, S., Chatterjee, N., Kumar, V., & Das, P. (2013). Spatial and temporal variation and hotspot detection of kala-azar disease in Vaishali district (Bihar), India. BMC Infectious Diseases . https://doi.org/10.1186/1471-2334-13-64 . Bonham-Carter, G. (2014). Geographic information systems for geoscientists: Modelling with GIS. In Computer methods in the geosciences . Booman, M., Durrheim, D. N., La Grange, K., Martin, C., Mabuza, A. M., Zitha, A., et al. (2000). Using a geographical information system to plan a malaria control programme in South Africa. Bulletin of the World Health Organization . https://doi.org/10.1590/S0042-96862000001200010 . Bos, L., & Blobel, B. (2007). Semantic interoperability between clinical and public health information systems for improving public health services. Med Care Compunetics Four , 4 , 256. Bouffanais, R., & Lim, S. S. (2020). Cities—Try to predict superspreading hotspots for COVID-19. Boulos, K. (2008). Principles and techniques of interactive Web cartography and Internet GIS. In Manual of geographic information systems. Bethesda, Maryland: ASPRS – American Society for Photogrammetry and Remote Sensing (pp. 935–974). Boulos, M. N. K. (2004). Descriptive review of geographic mapping of severe acute respiratory syndrome (SARS) on the internet. International Journal of Health Geographics . https://doi.org/10.1186/1476-072X-3-2 . Bowatte, G., Erbas, B., Lodge, C. J., Knibbs, L. D., Gurrin, L. C., Marks, G. B., et al. (2017). Traffic-related air pollution exposure over a 5-year period is associated with increased risk of asthma and poor lung function in middle age. The European Respiratory Journal . https://doi.org/10.1183/13993003.02357-2016 . Brown, P. (2013). Integrating medical and environmental sociology with environmental health: Crossing boundaries and building connections through advocacy. Journal of Health and Social Behavior . https://doi.org/10.1177/0022146513484473 . Brunette, W., Sundt, M., Dell, N., Chaudhri, R., Breit, N., & Borriello, G. (2013). Open Data Kit 2.0: Expanding and refining information services for developing regions. In ACM HotMobile 2013: The 14th workshop on mobile computing systems and applications . https://doi.org/10.1145/2444776.2444790 . Bulatović, V., Ninkov, T., & Sušić, Z. (2010). Open geospatial consortium web services in complex distribution systems. Geodetski List . Carpenter, T. E. (2011). The spatial epidemiologic (r)evolution: A look back in time and forward to the future. Spatial and Spatio-Temporal Epidemiology . https://doi.org/10.1016/j.sste.2011.07.002 . Castronovo, D. A., Chui, K. K. H., & Naumova, E. N. (2009). Dynamic maps: A visual-analytic methodology for exploring spatio-temporal disease patterns . Environmental Health: A Global Access Science Source. https://doi.org/10.1186/1476-069X-8-61 . Casu, F., Manunta, M., Agram, P. S., & Crippen, R. E. (2017). Big remotely sensed data: Tools, applications and experiences. Remote Sensing of Environment . https://doi.org/10.1016/j.rse.2017.09.013 . Cereda, D., Tirani, M., Rovida, F., Demicheli, V., Ajelli, M., Poletti, P., et al. (2020). The early phase of the COVID-19 outbreak in Lombardy, Italy. arXiv preprint arXiv:2003.09320 . Chen, N., Zhou, M., Dong, X., Qu, J., Gong, F., Han, Y., et al. (2020). Epidemiological and clinical characteristics of 99 cases of 2019 novel coronavirus pneumonia in Wuhan, China: A descriptive study. The Lancet . https://doi.org/10.1016/S0140-6736(20)30211-7 . Chen, Y., Gao, J., Yang, L., Li, C., Chen, R., Xie, Z., et al. (2019). A predominant dengue virus-1 endemic strain and the vector competence of Aedes albopictus from Guangzhou City, China. Acta Tropica . https://doi.org/10.1016/j.actatropica.2019.03.029 . Cheng, Y., Zhao, H., Song, P., Zhang, Z., Chen, J., & Zhou, Y. H. (2019). Dynamic changes of lymphocyte counts in adult patients with severe pandemic H1N1 influenza A. Journal of Infection and Public Health . https://doi.org/10.1016/j.jiph.2019.05.017 . Clarke, K. C., McLafferty, S. L., & Tempalski, B. J. (1996). On epidemiology and geographic information systems: A review and discussion of future directions. Emerging Infectious Diseases . https://doi.org/10.3201/eid0202.960202 . Clements, A. C. A., Reid, H. L., Kelly, G. C., & Hay, S. I. (2013). Further shrinking the malaria map: How can geospatial science help to achieve malaria elimination? The Lancet Infectious Diseases . https://doi.org/10.1016/S1473-3099(13)70140-3 . Cleynen, I., Boucher, G., Jostins, L., Schumm, L. P., Zeissig, S., Ahmad, T., et al. (2016). Inherited determinants of Crohn’s disease and ulcerative colitis phenotypes: A genetic association study. The Lancet . https://doi.org/10.1016/S0140-6736(15)00465-1 . Corburn, J. (2007). Community knowledge in environmental health science: Co-producing policy expertise. Environmental Science & Policy . https://doi.org/10.1016/j.envsci.2006.09.004 . Cromley, E. K. (2019). Using GIS to address epidemiologic research questions. Current Epidemiology Reports . https://doi.org/10.1007/s40471-019-00193-6 . Croner, C. M. (2004). Public health GIS and the internet. Journal of Map and Geography Libraries . https://doi.org/10.1300/J230v01n01_07 . Crowl, T. A., Crist, T. O., Parmenter, R. R., Belovsky, G., & Lugo, A. E. (2008). The spread of invasive species and infectious disease as drivers of ecosystem change. Frontiers in Ecology and the Environment . https://doi.org/10.1890/070151 . Cui, K., Cao, Z., Zheng, X., Zeng, D., Zeng, K., & Zheng, M. (2011). A geospatial analysis on the potential value of news comments in infectious disease surveillance. Lecture Notes in Computer Science . https://doi.org/10.1007/978-3-642-22039-5_9 . Curtis-Robles, R., Wozniak, E. J., Auckland, L. D., Hamer, G. L., & Hamer, S. A. (2015). Combining public health education and disease ecology research: Using citizen science to assess chagas disease entomological risk in Texas. PLoS Neglected Tropical Diseases . https://doi.org/10.1371/journal.pntd.0004235 . Dai, X., & Bikdash, M. (2016). Distance-based outliers method for detecting disease outbreaks using social media. In Conference proceedings — IEEE SOUTHEASTCON . https://doi.org/10.1109/SECON.2016.7506752 . Danis, K., Epaulard, O., Bénet, T., Gaymard, A., Campoy, S., Bothelo-Nevers, E., et al. (2020). Cluster of coronavirus disease 2019 (Covid-19) in the French Alps, 2020. Clinical Infectious Diseases . https://doi.org/10.1093/cid/ciaa424 . De Carli, A., Franco, M., Gassmann, A., Killer, C., Rodrigues, B., & Scheid, E., et al. (2020). WeTrace–A privacy-preserving mobile COVID-19 tracing approach and application. arXiv preprint arXiv:2004.08812 . Deka, M. A., & Morshed, N. (2018). Mapping disease transmission risk of Nipah virus in South and Southeast Asia. Tropical Medicine and Infectious Disease . https://doi.org/10.3390/tropicalmed3020057 . Dent, D. M. (2006). Cartographies of disease. Maps, mapping, and medicine. Tom Koch, ESRI Press, Redlands, CA, 2005, 388 pp, $28.32. World Journal of Surgery , 30 (8), 1626–1626. Desjardins, M. R., Hohl, A., & Delmelle, E. M. (2020). Rapid surveillance of COVID-19 in the United States using a prospective space-time scan statistic: Detecting and evaluating emerging clusters. Applied Geography . https://doi.org/10.1016/j.apgeog.2020.102202 . Desjardins, M. R., Whiteman, A., Casas, I., & Delmelle, E. (2018). Space-time clusters and co-occurrence of chikungunya and dengue fever in Colombia from 2015 to 2016. Acta Tropica . https://doi.org/10.1016/j.actatropica.2018.04.023 . Dominkovics, P., Granell, C., Pérez-Navarro, A., Casals, M., Orcau, À., & Caylà, J. A. (2011). Development of spatial density maps based on geoprocessing web services: Application to tuberculosis incidence in Barcelona Spain. International Journal of Health Geographics . https://doi.org/10.1186/1476-072X-10-62 . Donchyts, G., Baart, F., Winsemius, H., Gorelick, N., Kwadijk, J., & Van De Giesen, N. (2016). Earth’s surface water change over the past 30 years. Nature Climate Change . https://doi.org/10.1038/nclimate3111 . Dong, E., Du, H., & Gardner, L. (2020). An interactive web-based dashboard to track COVID-19 in real time. The Lancet Infectious Diseases . https://doi.org/10.1016/S1473-3099(20)30120-1 . Dong, J., Xiao, X., Menarguez, M. A., Zhang, G., Qin, Y., Thau, D., et al. (2016). Mapping paddy rice planting area in northeastern Asia with Landsat 8 images, phenology-based algorithm and Google Earth Engine. Remote Sensing of Environment . https://doi.org/10.1016/j.rse.2016.02.016 . Dredger, S. M., Kothari, A., Morrison, J., Sawada, M., Crighton, E. J., & Graham, I. D. (2007). Using participatory design to develop (public) health decision support systems through GIS. International Journal of Health Geographics . https://doi.org/10.1186/1476-072X-6-53 . Drew, D. A., Nguyen, L. H., Steves, C. J., Menni, C., Freydin, M., Varsavsky, T., et al. (2020). Rapid implementation of mobile technology for real-time epidemiology of COVID-19. Science . https://doi.org/10.1126/science.abc0473 . Dutheil, F., Baker, J. S., & Navel, V. (2020). COVID-19 as a factor influencing air pollution? Environmental Pollution . https://doi.org/10.1016/j.envpol.2020.114466 . Eckerle, I., & Meyer, B. (2020). SARS-CoV-2 seroprevalence in COVID-19 hotspots. Lancet. Eldredge, L., Enge, P., Harrison, M., Kenagy, R., Lo, S., Loh, R., et al. (2010). Alternative positioning, navigation & timing (PNT) study. In International civil aviation organisation navigation systems panel (NSP), Working Group Meetings, Montreal, Canada . Elith, J., Phillips, S. J., Hastie, T., Dudík, M., Chee, Y. E., & Yates, C. J. (2011). A statistical explanation of MaxEnt for ecologists. Diversity and Distributions . https://doi.org/10.1111/j.1472-4642.2010.00725.x . Erdem, M., Safan, M., & Castillo-Chavez, C. (2017). Mathematical analysis of an SIQR influenza model with imperfect quarantine. Bulletin of Mathematical Biology . https://doi.org/10.1007/s11538-017-0301-6 . Euler, G. L., Abbey, D. E., & Hodgkin, J. E. (1988). Chronic obstructive pulmonary disease symptom effects of long-term cumulative exposure to ambient levels of total oxidants and nitrogen dioxide in california seventh-day adventist residents. Archives of Environmental Health . https://doi.org/10.1080/00039896.1988.10545950 . European Commission. (2013). Science for Environment Policy In-Depth Report: Environmental Citizen Science. https://ec.europa.eu/environment/integration/research/newsalert/pdf/IR9_en.pdf . Accessed 25 July 2020. Farrahi, K., Emonet, R., & Cebrian, M. (2014). Epidemic contact tracing via communication traces. PLoS ONE . https://doi.org/10.1371/journal.pone.0095133 . Ferguson, N., Laydon, D., Nedjati-Gilani, G., Imai, N., Ainslie, K., & Baguelin, M., et al. (2020). Report 9—Impact of non-pharmaceutical interventions (NPIs) to reduce COVID-19 mortality and healthcare demand | Faculty of Medicine | Imperial College London. Imperial College COVID Response Team . Ferreira, A. A. F., dos Reis, V. P., Boeno, C. N., Evangelista, J. R., Santana, H. M., Serrath, S. N., et al. (2020). Increase in the risk of snakebites incidence due to changes in humidity levels: A time series study in four municipalities of the state of Rondonia. Revista da Sociedade Brasileira de Medicina Tropical . https://doi.org/10.1590/0037-8682-0377-2019 . FitzGerald, G. A. (2020). Misguided drug advice for COVID-19. Science . https://doi.org/10.1126/science.abb8034 . Friede, A. (1995). Public health informatics: How information-age technology can strengthen public health. Annual Review of Public Health . https://doi.org/10.1146/annurev.publhealth.16.1.239 . Friede, A., Reid, J. A., & Ory, H. W. (1993). CDC WONDER: A comprehensive on-line public health information system of the Centers for Disease Control and Prevention. American Journal of Public Health . https://doi.org/10.2105/AJPH.83.9.1289 . Frontera, A., Cianfanelli, L., Vlachos, K., Landoni, G., & Cremona, G. (2020). Severe air pollution links to higher mortality in COVID-19 patients: The “double-hit” hypothesis. Journal of Infection . https://doi.org/10.1016/j.jinf.2020.05.031 . Gan, W. Q., Davies, H. W., Koehoorn, M., & Brauer, M. (2012). Association of long-term exposure to community noise and traffic-related air pollution with coronary heart disease mortality. American Journal of Epidemiology . https://doi.org/10.1093/aje/kwr424 . Gao, S., Mioc, D., Anton, F., Yi, X., & Coleman, D. J. (2008). Online GIS services for mapping and sharing disease information. International Journal of Health Geographics . https://doi.org/10.1186/1476-072X-7-8 . Garcia-Martí, I., Rodríguez-Pupo, L. E., Díaz, L., & Huerta, J. (2013). Noise battle: A Gamified application for environmental noise monitoring in urban areas. In 16th AGILE conference on geographic information science, Leuven, Belgium, 14 – 17 May 2013 . Ge, X. Y., Li, J. L., Yang, X. Lou, Chmura, A. A., Zhu, G., Epstein, J. H., et al. (2013). Isolation and characterization of a bat SARS-like coronavirus that uses the ACE2 receptor. Nature . https://doi.org/10.1038/nature12711 . Gikonyo, S., Kimani, T., Matere, J., Kimutai, J., Kiambi, S. G., Bitek, A. O., et al. (2018). Mapping potential amplification and transmission hotspots for MERS-CoV, Kenya. EcoHealth . https://doi.org/10.1007/s10393-018-1317-6 . Gómez-Rubio, V., Ferrándiz-Ferragud, J., & López-Quílez, A. (2005). Detecting clusters of disease with R. Journal of Geographical Systems . https://doi.org/10.1007/s10109-005-0156-5 . Goodchild, M. F. (2007a). Citizens as sensors: The world of volunteered geography. GeoJournal . https://doi.org/10.1007/s10708-007-9111-y . Goodchild, M. F. (2007b). Citizens as voluntary sensors: Spatial data infrastructure in the world of Web 2.0. International Journal of Spatial Data Infrastructures Research, 2 (2), 24–32. Goodman, D. (2007). JavaScript bible . Hoboken: Wiley. Gorelick, N., Hancher, M., Dixon, M., Ilyushchenko, S., Thau, D., & Moore, R. (2017). Google Earth Engine: Planetary-scale geospatial analysis for everyone. Remote Sensing of Environment . https://doi.org/10.1016/j.rse.2017.06.031 . Granell, C., Fernández, Ó. B., & Díaz, L. (2014). Geospatial information infrastructures to address spatial needs in health: Collaboration, challenges and opportunities. Future Generation Computer Systems . https://doi.org/10.1016/j.future.2013.04.002 . Haklay, M. (2013). Citizen science and volunteered geographic information: Overview and typology of participation. In Crowdsourcing geographic knowledge: volunteered geographic information (VGI) in theory and practice . https://doi.org/10.1007/978-94-007-4587-2_7 . Hanafi-Bojd, A. A., Vatandoost, H., Oshaghi, M. A., Charrahy, Z., Haghdoost, A. A., Zamani, G., et al. (2012). Spatial analysis and mapping of malaria risk in an endemic area, south of Iran: A GIS based decision making for planning of control. Acta Tropica . https://doi.org/10.1016/j.actatropica.2012.01.003 . Hansen, M. C., Potapov, P. V., Moore, R., Hancher, M., Turubanova, S. A., Tyukavina, A., et al. (2013). High-resolution global maps of 21st-century forest cover change. Science . https://doi.org/10.1126/science.1244693 . Hawkins, D. M. (1980). Identification of outliers . London: Chapman and Hall. https://doi.org/10.1007/978-94-015-3994-4 . Hay, S. I. (2000). An overview of remote sensing and geodesy for epidemiology and public health application. Advances in Parasitology . https://doi.org/10.1016/s0065-308x(00)47005-3 . Heipke, C. (2010). Crowdsourcing geospatial data. ISPRS Journal of Photogrammetry and Remote Sensing . https://doi.org/10.1016/j.isprsjprs.2010.06.005 . Heymann, D. L., & Brilliant, L. (2011). Surveillance in eradication and elimination of infectious diseases: A progression through the years. Vaccine . https://doi.org/10.1016/j.vaccine.2011.12.135 . Hills, R. A., Lober, W. B., & Painter, I. S. (2008). Biosurveillance and biosecurity. Lecture Notes in Computer Science . https://doi.org/10.1007/978-3-540-89746-0 . Hirsch, A. (1883). Handbook of geographical and historical pathology (Vol. 1). London: New Sydenham Society. Hohl, A., Delmelle, E., & Desjardins, M. (2020). Rapid detection of COVID-19 clusters in the United States using a prospective space-time scan statistic. SIGSPATIAL Special . https://doi.org/10.1145/3404111.3404116 . Holt, A. C., Salkeld, D. J., Fritz, C. L., Tucker, J. R., & Gong, P. (2009). Spatial analysis of plague in California: Niche modeling predictions of the current distribution and potential response to climate change. International Journal of Health Geographics . https://doi.org/10.1186/1476-072X-8-38 . Hornsby, K. (2000). Shifting granularity over geospatial lifelines. In AAAI Workshop on Spatial and Temporal Granularity . Howe, J. (2006). The rise of crowdsourcing. Wired Magazine . https://doi.org/10.1086/599595 . Huang, C., Wang, Y., Li, X., Ren, L., Zhao, J., Hu, Y., et al. (2020). Clinical features of patients infected with 2019 novel coronavirus in Wuhan, China. The Lancet . https://doi.org/10.1016/S0140-6736(20)30183-5 . Hudson-Smith, A., Batty, M., Crooks, A., & Milton, R. (2009). Mapping for the masses: Accessing web 2.0 through crowdsourcing. Social Science Computer Review . https://doi.org/10.1177/0894439309332299 . Ihaka, R., & Gentleman, R. (1996). R: A language for data analysis and graphics. Journal of Computational and Graphical Statistics . https://doi.org/10.1080/10618600.1996.10474713 . Jacquez, G. M. (2000). Spatial analysis in epidemiology: Nascent science or a failure of GIS? Journal of Geographical Systems . https://doi.org/10.1007/s101090050035 . Jana, M., & Sar, N. (2016). Modeling of hotspot detection using cluster outlier analysis and Getis-Ord Gi* statistic of educational development in upper-primary level . India: Modeling Earth Systems and Environment. https://doi.org/10.1007/s40808-016-0122-x . Jeefoo, P., Tripathi, N. K., & Souris, M. (2011). Spatio-temporal diffusion pattern and hotspot detection of dengue in Chachoengsao province, Thailand. International Journal of Environmental Research and Public Health . https://doi.org/10.3390/ijerph8010051 . Ji, L. N., Chao, S., Wang, Y. J., Li, X. J., Mu, X. D., Lin, M. G., et al. (2020). Clinical features of pediatric patients with COVID-19: a report of two family cluster cases. World Journal of Pediatrics . https://doi.org/10.1007/s12519-020-00356-2 . Johansen, K., Phinn, S., & Taylor, M. (2015). Mapping woody vegetation clearing in Queensland, Australia from Landsat imagery using the Google Earth Engine. Remote Sensing Applications: Society and Environment . https://doi.org/10.1016/j.rsase.2015.06.002 . Jones, R. C., Liberatore, M., Fernandez, J. R., & Gerber, S. I. (2006). Use of a prospective space-time scan statistic to prioritize shigellosis case investigations in an urban jurisdiction. Public Health Reports . https://doi.org/10.1177/003335490612100206 . Joshi, A. R., Dinerstein, E., Wikramanayake, E., Anderson, M. L., Olson, D., Jones, B. S., et al. (2016). Tracking changes and preventing loss in critical tiger habitat. Science Advances . https://doi.org/10.1126/sciadv.1501675 . Kass-Hout, T. A., & Alhinnawi, H. (2013a). Social media in public health. British Medical Bulletin . https://doi.org/10.1093/bmb/ldt028 . Kass-Hout, T. A., & Alhinnawi, H. (2013b). Social media in public health. British Medical Bulletin . https://doi.org/10.1093/bmb/ldt028 . Kermack, W. O., & McKendrick, A. G. (1991). Contributions to the mathematical theory of epidemics-I. Bulletin of Mathematical Biology . https://doi.org/10.1007/BF02464423 . Khan, A. S., Fleischauer, A., Casani, J., & Groseclose, S. L. (2010). The next public health revolution: Public health information fusion and social networks. American Journal of Public Health . https://doi.org/10.2105/AJPH.2009.180489 . King, A. C., Winter, S. J., Sheats, J. L., Rosas, L. G., Buman, M. P., Salvo, D., et al. (2016). Leveraging citizen science and information technology for population physical activity promotion. Translational Journal of the American College of Sports Medicine . https://doi.org/10.1249/TJX.0000000000000003 . Kitler, M. E., Gavinio, P., & Lavanchy, D. (2002). Influenza and the work of the World Health Organization. Vaccine . https://doi.org/10.1016/S0264-410X(02)00121-4 . Kleinschmidt, I., Bagayoko, M., Clarke, G. P. Y., Craig, M., & Le Sueur, D. (2000). A spatial statistical approach to malaria mapping. International Journal of Epidemiology . https://doi.org/10.1093/ije/29.2.355 . Klompas, M., Murphy, M., Lankiewicz, J., McVetta, J., Lazarus, R., Eggleston, E., et al. (2011). Harnessing electronic health records for public health surveillance. Online Journal of Public Health Informatics . https://doi.org/10.5210/ojphi.v3i3.3794 . Kodong, F. R., Shanono, N. M., & AL-Jaberi, M. A. A. (2020). The monitoring infectious diseases diffusion through GIS. SciTech Framework, 2 (1), 23–33. Kolivras, K. N. (2006). Mosquito habitat and dengue risk potential in Hawaii: A conceptual framework and GIS application. Professional Geographer . https://doi.org/10.1111/j.1467-9272.2006.00521.x . Kothari, A., Driedger, S. M., Bickford, J., Morrison, J., Sawada, M., Graham, I. D., et al. (2008). Mapping as a knowledge translation tool for Ontario Early Years Centres: Views from data analysts and managers. Implementation Science . https://doi.org/10.1186/1748-5908-3-4 . Krishnan, A., Xu, T., Hutfless, S., Park, A., Stasko, T., Vidimos, A. T., et al. (2017). Outlier practice patterns in Mohs micrographic surgery: Defining the problem and a proposed solution. JAMA Dermatology . https://doi.org/10.1001/jamadermatol.2017.1450 . Krosing, H., & Roybal, K. (2013). PostgreSQL Server Programming . Community experience distilled . Kulldorff, M. (1997). A spatial scan statistic. Communications in statistics — Theory and methods . https://doi.org/10.1080/03610929708831995 . Kulldorff, M. (2001). Prospective time periodic geographical disease surveillance using a scan statistic. Journal of the Royal Statistical Society. Series A: Statistics in Society . https://doi.org/10.1111/1467-985X.00186 . Kumari, P., & Toshniwal, D. (2020). Impact of lockdown measures during COVID-19 on air quality—A case study of India. International Journal of Environmental Health Research . https://doi.org/10.1080/09603123.2020.1778646 . Lawson, A. B., & Leimich, P. (2000). Approaches to the space-time modelling of infectious disease behaviour. IMA Journal of Mathemathics Applied in Medicine and Biology . https://doi.org/10.1093/imammb17.1.1 . Lee, H., & Nishiura, H. (2019). Sexual transmission and the probability of an end of the Ebola virus disease epidemic. Journal of Theoretical Biology . https://doi.org/10.1016/j.jtbi.2019.03.022 . Lee, J. S. H., Wich, S., Widayati, A., & Koh, L. P. (2016). Detecting industrial oil palm plantations on Landsat images with Google Earth Engine . Remote Sensing Applications: Society and Environment. https://doi.org/10.1016/j.rsase.2016.11.003 . Li, J., & Guo, X. (2020). COVID-19 Contact-tracing apps: A survey on the global deployment and challenges. arXiv preprint arXiv:2005.03599 . Li, M. Y., & Muldowney, J. S. (1995). Global stability for the SEIR model in epidemiology. Mathematical Biosciences . https://doi.org/10.1016/0025-5564(95)92756-5 . Lipsitch, M., Swerdlow, D. L., & Finelli, L. (2020). Defining the epidemiology of Covid-19—Studies needed. New England Journal of Medicine . https://doi.org/10.1056/NEJMp2002125 . Liu, Y. F., Li, J. M., Zhou, P. H., Liu, J., Dong, X. C., Lyu, J., & Zhang, Y. (2020). Analysis on cluster cases of COVID-19 in Tianjin. Zhonghua Liu Xing Bing Xue Za Zhi = Zhonghua Liuxingbingxue Zazhi . https://doi.org/10.3760/cma.j.cn112338-20200225-00165 . Liu, W., Tao, Z. W., Wang, L., Yuan, M. L., Liu, K., Zhou, L., et al. (2020b). Analysis of factors associated with disease outcomes in hospitalized patients with 2019 novel coronavirus disease. Chinese Medical Journal . https://doi.org/10.1097/CM9.0000000000000775 . Liu, J., & Zhang, T. (2011). Epidemic spreading of an SEIRS model in scale-free networks. Communications in Nonlinear Science and Numerical Simulation . https://doi.org/10.1016/j.cnsns.2010.11.019 . Lo, Y. W., Zhao, Q., Ting, Y. H., & Chen, R. C. (2015). Automatic generation and recommendation of recipes based on outlier analysis. In IEEE 7th international conference on awareness science and technology, iCAST 2015 — proceedings . https://doi.org/10.1109/ICAwST.2015.7314050 . Lu, H., Stratton, C. W., & Tang, Y. W. (2020a). Outbreak of pneumonia of unknown etiology in Wuhan, China: The mystery and the miracle. Journal of Medical Virology . https://doi.org/10.1002/jmv.25678 . Lu, R., Zhao, X., Li, J., Niu, P., Yang, B., Wu, H., et al. (2020b). Genomic characterisation and epidemiology of 2019 novel coronavirus: implications for virus origins and receptor binding. The Lancet . https://doi.org/10.1016/S0140-6736(20)30251-8 . Lyseen, A. K., Nøhr, C., Sørensen, E. M., Gudes, O., Geraghty, E. M., Shaw, N. T., & Bivona-Tellez, C. (2014). A review and framework for categorizing current research and development in health related geographical information systems (GIS) Studies. Yearbook of Medical Informatics . https://doi.org/10.15265/IY-2014-0008 . MacKerron, G., & Mourato, S. (2010). LSE’s mappiness project may help us track the national mood: but how much should we consider happiness in deciding public policy?. Maisonneuve, N., Stevens, M., & Ochab, B. (2010). Participatory noise pollution monitoring using mobile phones. Information Polity . https://doi.org/10.3233/IP-2010-0200 . Mann, J. K., Tager, I. B., Lurmann, F., Segal, M., Quesenberry, C. P., Lugg, M. M., et al. (2002). Air pollution and hospital admissions for ischemic heart disease in persons with congestive heart failure or arrhythmia. Environmental Health Perspectives . https://doi.org/10.1289/ehp.021101247 . Marini, J. J., & Gattinoni, L. (2020). Management of COVID-19 respiratory distress. JAMA Journal of the American Medical Association . https://doi.org/10.1001/jama.2020.6825 . Martin, C., Curtis, B., Fraser, C., & Sharp, B. (2002). The use of a GIS-based malaria information system for malaria research and control in South Africa. Health and Place . https://doi.org/10.1016/S1353-8292(02)00008-4 . Martines, M. R., Ferreira, R. V., Toppa, R. H., Assuncao, L., Desjardins, M. R., & Delmelle, E. M. (2020). Detecting space-time clusters of COVID-19 in Brazil: Mortality, inequality, socioeconomic vulnerability, and the relative risk of the disease in Brazilian municipalities. MedRxiv . https://doi.org/10.1101/2020.06.14.20131102 . Matthew, S. R., Peter, H., & Andrew, C. (2004). World atlas of epidemic diseases. Cartographic Perspectives . https://doi.org/10.14714/cp52.381 . May, J. M. (1951). Map of the world distribution of malaria vectors. Geographical Review . https://doi.org/10.2307/210709 . Miller-Rushing, A., Primack, R., & Bonney, R. (2012). The history of public participation in ecological research. Frontiers in Ecology and the Environment . https://doi.org/10.1890/110278 . Mo, C., Tan, D., Mai, T., Bei, C., Qin, J., Pang, W., et al. (2020). An analysis of spatiotemporal pattern for COIVD-19 in China based on space-time cube. Journal of Medical Virology . https://doi.org/10.1002/jmv.25834 . Moore, D. A., & Carpenter, T. E. (1999). Spatial analytical methods and geographic information systems: Use in health research and epidemiology. Epidemiologic Reviews . https://doi.org/10.1093/oxfordjournals.epirev.a017993 . Mujica, F. C. (2013). Disease maps: Epidemics on the ground. Cartography and Geographic Information Science . https://doi.org/10.1080/15230406.2013.776209 . Mweya, C. N., Kimera, S. I., Kija, J. B., & Mboera, L. E. G. (2013). Predicting distribution of Aedes aegypti and Culex pipiens complex, potential vectors of Rift Valley fever virus in relation to disease epidemics in East Africa. Infection Ecology & Epidemiology . https://doi.org/10.3402/iee.v3i0.21748 . Nakada, L. Y. K., & Urban, R. C. (2020). COVID-19 pandemic: Impacts on the air quality during the partial lockdown in São Paulo state, Brazil. Science of the Total Environment, 730, 139087. Nakazawa, Y., Lash, R. R., Carroll, D. S., Damon, I. K., Karem, K. L., Reynolds, M. G., et al. (2013). Mapping monkeypox transmission risk through time and space in the Congo Basin. PLoS ONE . https://doi.org/10.1371/journal.pone.0074816 . Ng, K. C., Chaves, L. F., Tsai, K. H., & Chuang, T. W. (2018). Increased adult aedes aegypti and culex quinquefasciatus (Diptera: Culicidae) abundance in a dengue transmission hotspot, compared to a coldspot, within Kaohsiung city . Taiwan: Insects. https://doi.org/10.3390/insects9030098 . Nicholson, M. C., & Mather, T. N. (1996). Methods for evaluating lyme disease risks using geographic information systems and geospatial analysis. Journal of Medical Entomology . https://doi.org/10.1093/jmedent/33.5.711 . Noble, D., Smith, D., Mathur, R., Robson, J., & Greenhalgh, T. (2012). Feasibility study of geospatial mapping of chronic disease risk to inform public health commissioning. British Medical Journal Open . https://doi.org/10.1136/bmjopen-2011-000711 . Nykiforuk, C. I. J., & Flaman, L. M. (2011). Geographic information systems (GIS) for health promotion and public health: a review. Health Promotion Practice . https://doi.org/10.1177/1524839909334624 . Ogen, Y. (2020). Assessing nitrogen dioxide (NO 2 ) levels as a contributing factor to coronavirus (COVID-19) fatality. Science of the Total Environment . https://doi.org/10.1016/j.scitotenv.2020.138605 . Oliphant, T. E. (2007). Python for scientific computing. Computing in Science & Engineering . https://doi.org/10.1109/MCSE.2007.58 . Osei, F. B., & Duker, A. A. (2008). Spatial and demographic patterns of Cholera in Ashanti region, Ghana. International Journal of Health Geographics . https://doi.org/10.1186/1476-072X-7-44 . Owusu, C., Desjardins, M. R., Baker, K. M., & Delmelle, E. (2019). Residential mobility impacts relative risk estimates of space-time clusters of chlamydia in Kalamazoo county, Michigan. Geospatial Health . https://doi.org/10.4081/gh.2019.812 . Padarian, J., Minasny, B., & McBratney, A. B. (2015). Using Google’s cloud-based platform for digital soil mapping. Computers & Geosciences . https://doi.org/10.1016/j.cageo.2015.06.023 . Palmer, J. R. B., Oltra, A., Collantes, F., Delgado, J. A., Lucientes, J., Delacour, S., et al. (2017). Citizen science provides a reliable and scalable tool to track disease-carrying mosquitoes. Nature Communications . https://doi.org/10.1038/s41467-017-00914-9 . Panahi, M. H., Parsaeian, M., Mansournia, M. A., Khoshabi, M., Gouya, M. M., Hemmati, P., et al. (2020). A spatio-temporal analysis of influenza-like illness in Iran from 2011 to 2016. Medical Journal of The Islamic Republic of Iran (MJIRI), 34 (1), 464–469. Pansini, R., & Fornacca, D. (2020). COVID-19 higher induced mortality in Chinese regions with lower air quality. 10(2020.04), 04-20053595. Patela, N. N., Angiuli, E., Gamba, P., Gaughan, A., Lisini, G., Stevens, F. R., et al. (2015). Multitemporal settlement and population mapping from landsatusing google earth engine. International Journal of Applied Earth Observation and Geoinformation . https://doi.org/10.1016/j.jag.2014.09.005 . Pekel, J. F., Cottam, A., Gorelick, N., & Belward, A. S. (2016). High-resolution mapping of global surface water and its long-term changes. Nature . https://doi.org/10.1038/nature20584 . Perl, D. P., & Moalem, S. (2006). Aluminum, Alzheimer’s disease and the geospatial occurrence of similar disorders. Reviews in Mineralogy and Geochemistry . https://doi.org/10.2138/rmg.2006.64.4 . Pfefferle, S., Kobbe, R., Guenther, T., Noerz, D., Santer, R., Oh, J., et al. (2020). Infection control and virological assessment of the first cluster of COVID-19 in Northern Germany. SSRN Electronic Journal . https://doi.org/10.2139/ssrn.3572733 . Phillips, S. (2010). “A brief tutorial on maxent” in species distribution modeling for educators and practitioners. Lessons in Conservation . Piccolomiini, E. L., & Zama, F. (2020). Monitoring Italian COVID-19 spread by an adaptive SEIRD model. MedRxiv . https://doi.org/10.1101/2020.04.03.20049734 . Poggio, L., Simonetti, E., & Gimona, A. (2018). Enhancing the WorldClim data set for national and regional applications. Science of the Total Environment . https://doi.org/10.1016/j.scitotenv.2017.12.258 . Prensner, J. R., Iyer, M. K., Balbin, O. A., Dhanasekaran, S. M., Cao, Q., Brenner, J. C., et al. (2011). Transcriptome sequencing across a prostate cancer cohort identifies PCAT-1, an unannotated lincRNA implicated in disease progression. Nature Biotechnology . https://doi.org/10.1038/nbt.1914 . Pung, R., Chiew, C. J., Young, B. E., Chin, S., Chen, M. I. C., Clapham, H. E., et al. (2020). Investigation of three clusters of COVID-19 in Singapore: implications for surveillance and response measures. The Lancet . https://doi.org/10.1016/S0140-6736(20)30528-6 . Qian, Q., Zhao, J., Fang, L., Zhou, H., Zhang, W., Wei, L., et al. (2014). Mapping risk of plague in Qinghai-Tibetan Plateau, China. BMC Infectious Diseases . https://doi.org/10.1186/1471-2334-14-382 . Qiu, Y. Y., Wang, S. Q., Wang, X. L., Lu, W. X., Qiao, D., & Li, J. B., et al. (2020). Epidemiological analysis on a family cluster of COVID-19. Zhonghua Liu Xing Bing Xue Za Zhi = Zhonghua Liuxingbingxue Zazhi . https://doi.org/10.3760/cma.j.cn112338-20200221-00147 . Ramírez Villegas, J., & Bueno Cabrera, A. (2009). Working with climate data and niche modeling: I. Creation of bioclimatic variables. Ranjan, A. K., Patra, A. K., & Gorai, A. K. (2020). Effect of lockdown due to SARS COVID-19 on aerosol optical depth (AOD) over urban and mining regions in India . Science of the Total Environment , 141024. Rappole, J. H., & Hubálek, Z. (2006). Birds and influenza H5N1 virus movement to and within North America. Emerging Infectious Diseases . https://doi.org/10.3201/eid1210.051577 . Rayner, M. E., & Bender, E. A. (1980). An introduction to mathematical modelling. The Mathematical Gazette . https://doi.org/10.2307/3615903 . Reeder, B., Revere, D., Hills, R. A., Baseman, J. G., & Lober, W. B. (2012). Public health practice within a health information exchange: Information needs and barriers to disease surveillance. Online Journal of Public Health Informatics . https://doi.org/10.5210/ojphi.v4i3.4277 . Reeves, T., Samy, A. M., & Peterson, A. T. (2015). MERS-CoV geography and ecology in the Middle East: Analyses of reported camel exposures and a preliminary risk map. BMC Research Notes . https://doi.org/10.1186/s13104-015-1789-1 . Reis, B. Y., Kohane, I. S., & Mandl, K. D. (2007). An epidemiological network model for disease outbreak detection. PLoS Medicine . https://doi.org/10.1371/journal.pmed.0040210 . Reis, V. P. D., Boeno, C. N., Evangelista, J. R., Santana, H. M., Serrath, S. N., Lopes, J. A., et al. (2020). Increase in the risk of snakebites incidence due to changes in humidity levels: A time series study in four municipalities of the state of Rondônia. Revista da Sociedade Brasileira de Medicina Tropical , 53. Ren, H., Zhao, L., Zhang, A., Song, L., Liao, Y., Lu, W., et al. (2020). Early forecasting of the potential risk zones of COVID-19 in China’s megacities. Science of the Total Environment . https://doi.org/10.1016/j.scitotenv.2020.138995 . Robertson, C., & Nelson, T. A. (2010). Review of software for space-time disease surveillance. International Journal of Health Geographics . https://doi.org/10.1186/1476-072X-9-16 . Robinson, T. P. (2000). Spatial statistics and geographical information systems in epidemiology and public health. Advances in Parasitology . https://doi.org/10.1016/s0065-308x(00)47007-7 . Rothan, H. A., & Byrareddy, S. N. (2020). The epidemiology and pathogenesis of coronavirus disease (COVID-19) outbreak. Journal of Autoimmunity . https://doi.org/10.1016/j.jaut.2020.102433 . Roy, H. E., Pocock, M. J. O., Preston, C. D., Roy, D. B., Savage, J., Tweddle, J., & Robinson, L. D. (2012). Understanding citizen science and environmental monitoring. Final Report on behalf of UK-EOF. NERC Centre for Ecology & Hydrology. Sacks, J. A., Zehe, E., Redick, C., Bah, A., Cowger, K., Camara, M., et al. (2015). Introduction of mobile health tools to support Ebola surveillance and contact tracing in Guinea. Global Health Science and Practice . https://doi.org/10.9745/GHSP-D-15-00207 . Samy, A. M., Campbell, L. P., & Townsend Peterson, A. (2014). Leishmaniasis transmission: Distribution and coarse-resolution ecology of two vectors and two parasites in Egypt. Revista da Sociedade Brasileira de Medicina Tropical . https://doi.org/10.1590/0037-8682-0189-2013 . Schriml, L. M., Arze, C., Nadendla, S., Ganapathy, A., Felix, V., Mahurkar, A., et al. (2009). GeMInA, genomic metadata for infectious agents, a geospatial surveillance pathogen database. Nucleic Acids Research . https://doi.org/10.1093/nar/gkp832 . Shim, E., Tariq, A., Choi, W., Lee, Y., & Chowell, G. (2020). Transmission potential and severity of COVID-19 in South Korea. International Journal of Infectious Diseases . https://doi.org/10.1016/j.ijid.2020.03.031 . Shin, S., Bai, L., Oiamo, T. H., Burnett, R. T., Weichenthal, S., Jerrett, M., et al. (2020). Association between road traffic noise and incidence of diabetes mellitus and hypertension in Toronto, Canada: A population-based cohort study. Journal of the American Heart Association . https://doi.org/10.1161/JAHA.119.013021 . Smith, M., Szongott, C., Henne, B., & Von Voigt, G. (2012). Big data privacy issues in public social media. IEEE International Conference on Digital Ecosystems and Technologies . https://doi.org/10.1109/DEST.2012.6227909 . Smolinski, M. S., Crawley, A. W., Baltrusaitis, K., Chunara, R., Olsen, J. M., Wójcik, O., et al. (2015). Flu near you: Crowdsourced symptom reporting spanning 2 influenza seasons. American Journal of Public Health . https://doi.org/10.2105/AJPH.2015.302696 . Sohrabi, C., Alsafi, Z., O’Neill, N., Khan, M., Kerwan, A., Al-Jabir, A., et al. (2020). World Health Organization declares global emergency: A review of the 2019 novel coronavirus (COVID-19). International Journal of Surgery . https://doi.org/10.1016/j.ijsu.2020.02.034 . Stoto, M. A., Schonlau, M., & Mariano, L. T. (2004). Syndromic surveillance: Is it worth the effort? CHANCE . https://doi.org/10.1080/09332480.2004.10554882 . Sui, D., Elwood, S., & Goodchild, M. (2013). Crowdsourcing geographic Knowledge: Volunteered geographic information (VGI) in theory and practice. Crowdsourcing Geographic Knowledge: Volunteered Geographic Information (VGI) in Theory and Practice . https://doi.org/10.1007/978-94-007-4587-2 . Tang, Z., Li, Y., Gu, Y., Jiang, W., Xue, Y., Hu, Q., et al. (2016). Assessing Nebraska playa wetland inundation status during 1985–2015 using Landsat data and Google Earth Engine. Environmental Monitoring and Assessment . https://doi.org/10.1007/s10661-016-5664-x . The Times of India. https://timesofindia.indiatimes.com/india/aarogya-setu-app-alerted-govt-to-300-emerging-hotspots-which-could-have-been-missed-out-niti-aayog-ceo/articleshow/75648084.cms . Accessed 18 July 2020. Toda, A. A. (2020). Susceptible-infected-recovered (sir) dynamics of covid-19 and economic impact. arXiv preprint arXiv:2003.11221 . Tolentino, H., Kamadjeu, R., Fontelo, P., Liu, F., Matters, M., Pollack, M., et al. (2007). Scanning the emerging infectious diseases horizon-visualizing ProMED emails using EpiSPIDER. Adv Dis Surveil , 2 , 169. Townsend, J. (2015). Mapping disease transmission risk: enriching models using biogeography and ecology. Emerging Infectious Diseases , 21 (8), 1489. Tracewski, Ł., Butchart, S. H. M., Donald, P. F., Evans, M., Fishpool, L. D. C., & Buchanan, G. M. (2016). Patterns of twenty-first century forest loss across a global network of important sites for biodiversity. Remote Sensing in Ecology and Conservation . https://doi.org/10.1002/rse2.13 . Turner, A. J. (2006). Introduction to neogeography . OReilly Short Cuts . Turnock, B. (2012). Public health . Burlington: Jones & Bartlett Publishers. Upadhyay, H. (2020). Aarogya Setu app crosses 100 million downloads. Resource document. https://entrackr.com/2020/05/aarogya-setu-crosses-100-mn-download-mark/ . Accessed 18 July 2020. Washington, M. K. (2007). Autoimmune liver disease: Overlap and outliers. Modern Pathology . https://doi.org/10.1038/modpathol.3800684 . Wiggins, A., & Crowston, K. (2011). From conservation to crowdsourcing: A typology of citizen science. Proceedings of the Annual Hawaii International Conference on System Sciences . https://doi.org/10.1109/HICSS.2011.207 . Wilkinson, P., Grundy, C., Landon, M., & Stevenson, S. (1998). GIS in public health. GIS and Health, 6, 179–190. Willerson, J. T., Lewis, S. E., Buja, L. M., Bonte, F. J., & Parkey, R. W. (1981). Recent advances in nuclear cardiology. I. “Hot-spot” and “cold-spot” myocardial scintigraphy. Postgraduate Medicine . https://doi.org/10.1080/00325481.1981.11715850 . Williams, C., Curran, A. S., Chen Lee, A., & Sousa, V. O. (1986). Lyme disease: Epidemiologic characteristics of an outbreak in Westchester County, NY. American Journal of Public Health . https://doi.org/10.2105/AJPH.76.1.62 . Wilson, K., Atkinson, K., Pluscauskas, M., & Bell, C. (2014). A mobile-phone immunization record in Ontario: Uptake and opportunities for improving public health. Journal of Telemedicine and Telecare . https://doi.org/10.1177/1357633X14537771 . Winslow, C. E. A. (1920). The untilled fields of public health. Science . https://doi.org/10.1126/science.51.1306.23 . Woo, P. C. Y., Lau, S. K. P., Lam, C. S. F., Lau, C. C. Y., Tsang, A. K. L., Lau, J. H. N., et al. (2012). Discovery of seven novel mammalian and avian coronaviruses in the genus deltacoronavirus supports bat coronaviruses as the gene source of alphacoronavirus and betacoronavirus and avian coronaviruses as the gene source of gammacoronavirus and deltacoronavi. Journal of Virology . https://doi.org/10.1128/jvi.06540-11 . World Health Organization. https://www.who.int/csr/don/05-january-2020-pneumonia-of-unkown-cause-china/en/ . Accessed 21 July 2020. Wu, C., Chen, X., Cai, Y., Xia, J., Zhou, X., Xu, S., et al. (2020). Risk factors associated with acute respiratory distress syndrome and death in patients with coronavirus disease 2019 pneumonia in Wuhan, China. JAMA Internal Medicine . https://doi.org/10.1001/jamainternmed.2020.0994 . Wu, Y., & Krishnan, S. (2010). Statistical analysis of gait rhythm in patients with parkinson’s disease. IEEE Transactions on Neural Systems and Rehabilitation Engineering . https://doi.org/10.1109/TNSRE.2009.2033062 . Xiong, J., Thenkabail, P. S., Gumma, M. K., Teluguntla, P., Poehnelt, J., Congalton, R. G., et al. (2017). Automated cropland mapping of continental Africa using Google Earth Engine cloud computing. ISPRS Journal of Photogrammetry and Remote Sensing . https://doi.org/10.1016/j.isprsjprs.2017.01.019 . Xu, Z., Shi, L., Wang, Y., Zhang, J., Huang, L., Zhang, C., et al. (2020). Pathological findings of COVID-19 associated with acute respiratory distress syndrome. The Lancet Respiratory Medicine . https://doi.org/10.1016/S2213-2600(20)30076-X . Yasaka, T. M., Lehrich, B. M., & Sahyouni, R. (2020). Peer-to-peer contact tracing: Development of a privacy-preserving smartphone app. JMIR MHealth and UHealth . https://doi.org/10.2196/18936 . Yeng, P., Woldaregay, A. Z., & Hartvigsen, G. (2019). K-CUSUM: Cluster detection mechanism in EDMON. In Proceedings of the 17th scandinavian conference on health informatics (pp. 141–147) Oslo Norway . Yih, W. K., Deshpande, S., Fuller, C., Heisey-Grove, D., Hsu, J., Kruskal, B. A., et al. (2010). Evaluating real-time syndromic surveillance signals from ambulatory care data in four states. Public Health Reports . https://doi.org/10.1177/003335491012500115 . Yin, F., Li, X., Ma, J., & Feng, Z. (2007). The early warning system based on the prospective space-time permutation statistic. Wei Sheng Yan Jiu = Journal of Hygiene Research . Yu, V. L., & Edberg, S. C. (2005). Global infectious diseases and epidemiology network (GIDEON): A world wide web-based program for diagnosis and informatics in infectious diseases. Clinical Infectious Diseases . https://doi.org/10.1086/426549 . Yun, S. C. (2007). The user-participated geospatial web as open platform. In The 11th international seminar on GIS Oct. Zarikas, V., Poulopoulos, S. G., Gareiou, Z., & Zervas, E. (2020). Clustering analysis of countries using the COVID-19 cases dataset. Data in Brief . https://doi.org/10.1016/j.dib.2020.105787 . Zhang, A., Qi, Q., & Jiang, L. (2007). GeoRSS based emergency response information sharing and visualization. In 3rd international conference on semantics, knowledge, and grid, SKG 2007 . https://doi.org/10.1109/SKG.2007.80 . Zhang, W., Wang, L., Fang, L., Ma, J., Xu, Y., Jiang, J., et al. (2008). Spatial analysis of malaria in Anhui province, China. Malaria Journal . https://doi.org/10.1186/1475-2875-7-206 . Zhang, Y., Su, X., Chen, W., Fei, C. N., Guo, L. R., Wu, X. L., et al. (2020). Epidemiological investigation on a cluster epidemic of COVID-19 in a collective workplace in Tianjin. Zhonghua Liu Xing Bing Xue Za Zhi = Zhonghua Liuxingbingxue Zazhi . https://doi.org/10.3760/cma.j.cn112338-20200219-00121 . Zhao, J., Liao, J., Huang, X., Zhao, J., Wang, Y., Ren, J., et al. (2016). Mapping risk of leptospirosis in China using environmental and socioeconomic data. BMC Infectious Diseases . https://doi.org/10.1186/s12879-016-1653-5 . Zhen, Z., Jin, J. M., & Liu, F. (2010). The application of geographic information system (GIS) in the field of public health. In 2010 2nd IITA international conference on geoscience and remote sensing, IITA - GRS 2010 . https://doi.org/10.1109/IITA-GRS.2010.5603111 . Zhu, G., Fan, J., & Peterson, A. T. (2017). Schistosoma japonicum transmission risk maps at present and under climate change in mainland China. PLoS Neglected Tropical Diseases . https://doi.org/10.1371/journal.pntd.0006021 . Zoran, M. A., Savastru, R. S., Savastru, D. M., & Tautan, M. N. (2020). Assessing the relationship between ground levels of ozone (O 3 ) and nitrogen dioxide (NO 2 ) with coronavirus (COVID-19) in Milan, Italy. Science of the Total Environment . https://doi.org/10.1016/j.scitotenv.2020.140005 . Download references Author informationAuthors and affiliations. Indian Institute of Remote Sensing, Indian Space Research Organisation, #4, Kalidas Road, Dehradun, 248001, India Sameer Saran, Priyanka Singh, Vishal Kumar & Prakash Chauhan You can also search for this author in PubMed Google Scholar Corresponding authorCorrespondence to Sameer Saran . Additional informationPublisher's note. Springer Nature remains neutral with regard to jurisdictional claims in published maps and institutional affiliations. About this articleSaran, S., Singh, P., Kumar, V. et al. Review of Geospatial Technology for Infectious Disease Surveillance: Use Case on COVID-19. J Indian Soc Remote Sens 48 , 1121–1138 (2020). https://doi.org/10.1007/s12524-020-01140-5 Download citation Received : 28 July 2020 Accepted : 04 August 2020 Published : 18 August 2020 Issue Date : August 2020 DOI : https://doi.org/10.1007/s12524-020-01140-5 Share this articleAnyone you share the following link with will be able to read this content: Sorry, a shareable link is not currently available for this article. Provided by the Springer Nature SharedIt content-sharing initiative - Geospatial technology
- Public health
- Mathematical epidemiology
- Find a journal
- Publish with us
- Track your research
The GEOINT Playbook: Geospatial Industry TrendsAn investment thesis on the future of geospatial intelligence, geospatial industry trends, and our outlook on digitizing the physical world.The global geospatial market is expected to grow from $63.1 billion to $147.6 billion in the next five years. This geospatial industry growth is largely due to the proliferation of spatial analysis; a critical feature of modern enterprise and consumer applications. Geospatial industry growthThe global geospatial market is expected to continue to grow due to several factors that are driving increased demand for geospatial solutions and services. Some of the key factors contributing to this increase in the geospatial industry size: Technological advancements:Rapid developments in technologies such as satellite imagery, remote sensing, GPS, GIS, and 3D mapping have led to more sophisticated and accurate geospatial data collection and analysis. These advancements make it possible to process and analyze large volumes of geospatial data, thus creating more opportunities for various industries to benefit from this GEOINT intelligence information. Growing adoption across industries: Geospatial solutions are increasingly being adopted across various sectors such as agriculture, transportation, energy, defense, urban planning, and telecommunications. This widespread adoption is driven by the need for better decision-making, cost reduction, and improved efficiency. Government initiatives and investments: Governments around the world are investing in geospatial technologies and infrastructure to support national development, disaster management, and public safety. These investments, in turn, contribute to the growth of the geospatial market. Integration with IoT and Big Data: The integration of geospatial data with the Internet of Things (IoT) and Big Data analytics has opened new avenues for businesses to gain insights, optimize processes, and improve decision-making. This has led to an increased demand for geospatial solutions and services. Increasing demand for location-based services: The rise in the popularity of smartphones and other mobile devices has driven the demand for location-based services (LBS) such as navigation, local search, and geo-targeted advertising. This growing demand has spurred the need for more accurate and reliable geospatial data, further contributing to the growth of the market. Climate change and environmental concerns: As climate change and environmental issues become more pressing, governments and organizations are seeking ways to monitor, analyze, and mitigate these challenges. Geospatial technologies can provide valuable insights and help in addressing these concerns, thus driving market growth. Each of these factors has led to a greater demand for geospatial solutions and services, fueling market growth. Opportunities pertaining to geospatial industry market sizeDespite the growth in geospatial data creation and, subsequently, the tools to conduct geospatial analysis, working with this type of information remains complex, and the number of products limited. This is in large part due to the broader industry-wide trend of market consolidation amongst existing incumbents and well-funded technology startups. This points to a conflicting theme within the geospatial market and industry: companies that begin as a modular technology stack (unbundled) and then evolve into a vertically integrated software solution (bundled). The new “As-a-service” paradigm has unlocked scalable modular development across compute, storage, application programming interfaces (APIs), etc, and is accelerating the pace of innovation. What you’ll find in The GEOINT PlaybookIn this playbook we will walk through our thesis, which analyzes geospatial intelligence (GEOINT) through the lens of technology layers: Infrastructure, Distribution, and Applications. This GEOINT analysis framework helps us connect the dots from the origin and constraints of the geospatial stack to evolution and inflection within the market. It also helps us see the larger role that space-based technology plays in an ecosystem that intersects with the modern tech industry and serves customers across a wide variety of markets. For more information, discussion, and news about space and the geospatial industry, visit our INSIGHTS page . Here, you will find our Blog, our Podcasts, and links to vital news and other media that will keep you in the know. Space Capital is a seed-stage venture capital firm investing in the space economy, specifically focused on unlocking the value in space technology stacks such as GPS, geospatial intelligence, and communications. Our team’s 55 years of combined sector experience and the support of our best-in-class operating partners ensure a diverse and profitable portfolio of brilliant, cutting-edge companies developing space technology that impacts our day-to-day world. In the same way that every company today is a technology company, every company of tomorrow will be a space company. THE AUTHORSStay up to date on the latest news., get the report. By filling in this form you agree to be added to our mailing list. You’ll be sent the latest news from Space Capital. 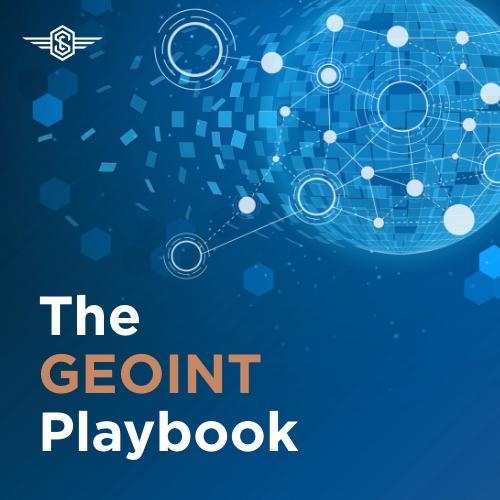 GET THE REPORT If you would like to share your ideas, business, or feedback with us, please email us. All business plan submissions must include a clear description of your operations and current progress.  Nasslackieren und pulverbeschichten Der Kongress am 15. / 16. Oktober 2024 in Heidelberg informiert umfassend über neue lack-, anlagen- und verfahrenstechnische Entwicklungen und deren Umsetzung in die Praxis. Springer Professional2022 | Buch 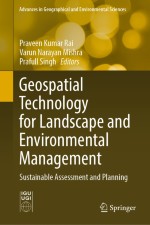 Geospatial Technology for Landscape and Environmental ManagementSustainable assessment and planning. herausgegeben von: Dr. Praveen Kumar Rai, Dr. Varun Narayan Mishra, Dr. Prafull Singh Verlag: Springer Nature Singapore Buchreihe : Advances in Geographical and Environmental Sciences Enthalten in: Springer Professional "Wirtschaft+Technik" , Springer Professional "Technik" , Springer Professional "Wirtschaft" Über dieses BuchGeospatial technology is a combination of state-of-the-art remote sensing and technology for geographic information systems (GIS) and global navigation satellite systems (GNSS) for the mapping and monitoring of landscapes and environment. The main thrust of using geospatial technology is to understand the causes, mechanisms, and consequences of spatial heterogeneity, while its ultimate objective is to provide a scientific basis for developing and maintaining ecologically, economically, and socially sustainable landscapes. This book presents new research on the interdisciplinary applications of geospatial technology for identification, assessment, monitoring, and modelling issues related to landscape, natural resources, and environmental management. The book specifically focuses on the creation, collection, storage, processing, modelling, interpretation, display, and dissemination of spatio-temporal data, which help to resolve environmental management issues including ecosystem change, resource utilization, land use management, and environmental pollution. The positive environmental impacts of information technology advancements with regard to global environmental and climate change are also discussed. The book addresses the interests of a wide spectrum of readers who have a common interest in geospatial science, geology, water resource management, database management, planning and policy making, and resource management. InhaltsverzeichnisFrontmatter, chapter 1. spatio-temporal variability of channel planform dynamics in response to spatial expansion of brick kilns: a case study of the downstream course of ichamati river, west bengal, india, chapter 2. assessment of replenishable groundwater resource and integrated water resource planning for sustainable agriculture, chapter 3. spatial prediction of flood frequency analysis in a semi-arid zone: a case study from the seyad basin (guelmim region, morocco), chapter 4. geospatial modeling in the assessment of environmental resources for sustainable water resource management in a gondia district, india, chapter 5. hydrochemical characteristics of groundwater—assessment of saltwater intrusion along krishna and godavari delta region, andhra pradesh, india, chapter 6. microlevel planning for integrated natural resources management and sustainable development: an approach through a micro watershed using geospatial technology, chapter 7. ecohydrological perspective for environmental degradation of lakes and wetlands in delhi, chapter 8. prioritization and quantitative assessment of dhundsir gad using rs and gis: implications for watershed management, planning and conservation, garhwal himalaya, uttarakhand, chapter 9. assessment of groundwater potential zones and resource sustainability through geospatial techniques: a case study of kamina sub-watershed of bhima river basin, maharashtra, india, chapter 10. morphometric analysis of damodar river sub-watershed, jharkhand, india, using remote sensing and gis techniques, chapter 11. the increasing inevitability of iot in remote disaster monitoring applications, chapter 12. countering challenges of smart cities mission through participatory approach, chapter 13. urban growth modeling and prediction of land use land cover change over nagpur city, india using cellular automata approach, chapter 14. slum categorization for efficient development plan—a case study of udhampur city, jammu and kashmir using remote sensing and gis, chapter 15. urban growth trend analysis using shannon entropy approach—a case study of dehradun city of uttarakhand, india, chapter 16. geospatial approach for mapping of significant land use/land cover changes in andhra pradesh, chapter 17. assessing the impact of delhi metro network towards urbanisation of delhi-ncr, chapter 18. analysis of urban heat island effect in rajkot city using geospatial techniques, chapter 19. multispectral remote sensing for urban planning and development, chapter 20. analysis of urban green spaces using geospatial techniques—a case study of vijayawada urban local body andhra pradesh, india, chapter 21. magnetic susceptibility and heavy metals contamination in agricultural soil of kopargaon area, ahmadnagar district, maharashtra, india, premium partner. 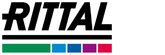 - Webinare Technik
- Webinare Wirtschaft
- Veranstaltungskalender
- MyNewsletter
- Automobil + Motoren
- Bauwesen + Immobilien
- Business IT + Informatik
- Elektrotechnik + Elektronik
- Energie + Nachhaltigkeit
- Finance + Banking
- Management + Führung
- Marketing + Vertrieb
- Maschinenbau + Werkstoffe
- Versicherung + Risiko
- Organisationspsychologie
- Projektmanagement
- Smart Manufacturing
- Zeitschriften
- Jetzt Einzelzugang starten
- Zugang für Unternehmen
- Referenzkunden
- Lackier- und Pulvertreff 2024
Scripps Technical Forum: Into the Deep: A look at the worlds source maritime data with East View GeospatialIn this presentation, East View Geospatial (EVG) will share how they provide a wide range of vector and raster products produced from authoritative maritime and hydrographic sources to support the GIS-fluent research and analytical community. Known to navigators worldwide, vector-based Electronic Navigation Charts (ENCs) and their raster cousins RNCs have long provided unique and useful content to non-navigational users, just like print nautical charts before them. However, the legal requirements and technology evolution connected with supporting the navigation community have come at the expense of researchers and engineers around the world. East View’s data has served researchers from a dynamic base of patrons concerned with the maritime and hydrographic aspects of climate change, boundary disputes and international security, biological and geological natural resources, green energy development, and even the social and historical sciences. To aid researchers and engineers in the above aspects EVG provides numerous forms of maritime mapping information in the form of East View Maritime Vector (EVMV) data, which comes directly from sourcing ENC data from around the world. In addition to EVMV, we provide Print-On-Demand nautical charts, raster nautical charts, bathymetry data, etc. to ensure researchers can successfully implement and or complete their projects. About East View Geospatial For more than 25 years, East View Geospatial has been a leading knowledge center for the world’s maps and geospatial data. From academics to individual explorers, risk assessors to software integrators, East View Geospatial is the trusted source for authoritative, accurate cartographic products and data management solutions. East View Geospatial works as a source provider for numerous institutions and organizations in the maritime industry. Specifically providing data and support for Seabed 2030 and GEBCO throughout the last decade enabling the sustainable protection of marine resources and coastal environments. - Birch Aquarium Special Events
- Education Department
- Equity, Diversity and Inclusion
- Philanthropy
- Public Lectures and Events
- Scripps Public Tours
- - Applied Ocean Science
- - Earth Section
- - Fisheries
- - Geophysics
- - Geoscience/Marine Chemistry & Geochemistry Seminar
- - Marine Biology
- - Polar Seminar
- - Scripps Technical Forum
- - Thesis Defenses
- - Women and Minorities in Science
- Scripps Only
Sign Up For Explorations NowArtificial intelligence can speed-sort satellite photosCould it also recruit an agent.  I n 1957 Frank Rosenblatt , a psychologist, built a machine called the Perceptron. Modelled on the human brain, its neural networks were a forerunner of today’s artificial intelligence ( ai ). It intrigued the cia which was drowning in photos from spy planes and satellites. It funded the Perceptron in the hope of automatically identifying objects of interest. The experiment failed. There was not enough computing power, storage or training data available. But it was a start. Spy agencies used machine learning to sift through images and text in the cold war, and then to identify patterns in billions of phone records after 9/11. Although advances in algorithms and computing power over the past decade have made those models faster and better, most agencies still believe ai will assist humans rather than replace them. However, the Perceptron’s successors, large language models ( llm s) like gpt -4, are beginning to challenge that assumption. Start with geospatial intelligence ( geoint ). Machines have not solved the problem that led to the cia ’s interest in the Perceptron: too many images from space, too few people and too little time to sort through them. Vice-Admiral Frank Whitworth, who runs America’s National Geospatial-Intelligence Agency ( nga ), points out that the number of humans in his agency—around 14,000 today compared with 32,000 in the National Security Agency ( nsa )—will rise more slowly than the “terabytes from space”. Computer vision is helping deal with the deluge. “If you started a shift at 7.30am,” says General Sir Jim Hockenhull, who oversees British defence intelligence, “you might get to the important image at 1pm.” Now algorithms flag up key changes, and analysts are at least twice as productive. “Through my career, intelligence analysts used to spend 80% of their time wrangling the information and 20% adding value,” he says. “In the geospatial world, we’ve been able to flip that.” Eyes in the skyThe war in Ukraine has been an “accelerator” for experimentation, says Trent Maul of America’s Defence Intelligence Agency ( dia ). He points out that data from one sensor now routinely prompt collection from another “in an automated fashion you’ve never seen before”, a process that is enabling the dia and other agencies to process data at a speed, volume and accuracy “that has never been done”. Admiral Whitworth says that “visual transformers”—a subset of the “generative pre-trained transformers” that form the gpt in Chat gpt —hold great promise. They might allow a model to provide context: not just identifying a missile battery, but explaining how it is deployed. British intelligence is experimenting with tools that can produce automatic orders of battle—summaries of the deployment of enemy forces. It helps that armies, especially if conscript-heavy, often position in predictable ways. That was not possible even in early 2022. But the admiral is wary of hype. The nga says humans still outperform algorithms. The models get 70-80% right, says one person familiar with the data, but that depends on the target. Jets on an airfield are easy, smaller or more obscure hardware less so. It was not until the war in Ukraine that the agency realised it would need to train models to recognise destroyed equipment, like mangled tanks. The big difference between computer vision in the civilian and intelligence world, says Admiral Whitworth, is that for facial-recognition algorithms, a face makes up 80% of the field of view. A missile in a forest in the corner of a satellite image is another matter. “We’re looking at two one-hundred-thousandths of a percent.” The fact that geoint is used in military targeting creates a high threshold. “We cannot afford any hallucination”, he says. “The algorithm is always going to be an entry-level analyst.” Similar debates are playing out in adjacent fields. American intelligence officials already have access to Chat gpt -like tools on their mobile phones, which are based on non-classified data. In May Microsoft said it had developed an “air-gapped” version of gpt -4, disconnected from the internet, for American agencies. Some experts are still sceptical. In April Adam “C”, the (semi-anonymous) chief data scientist for Britain’s gchq , described llm s as “a really sketchy technology for analysts, who have a profound national obligation to be right”. In a paper published in 2023 Mr “C” and Richard Carter of the Alan Turing Institute, a think-tank, warned that existing llm s could not be trusted to produce finished intelligence reports, which require lateral thinking and counterfactual (“what if”) reasoning. New hybrid models would be needed for that, they argued, such as neurosymbolic networks, which combine the statistical approach of neural networks with old-fashioned logic-based (“if this, then that”) ai . Until then, the llm s were best confined to early stages of drafting—“an extremely junior analyst”. People working with cutting-edge models contest this and say that agencies are being too conservative. A recent paper by Philipp Schoenegger of the London School of Economics and colleagues found that volunteers given access to llm s made forecasts that were 23% more accurate than a control group. Others hope to go much further. Mark Warner, who chairs the Senate’s intelligence committee, says that as recently as a year ago there was still talk of a “single large language model” which would combine images, intercepts and files acquired by human intelligence. In practice, collecting lots of data requires storage capacity, and running machine-learning models on it requires lots of computing power. The nga is the largest consumer of both in the American intelligence community. Many intelligence agencies are building secret cloud servers to host classified data. But these are turning into “superpower infrastructure”, says a European intelligence official, with only American and Chinese firms capable of building them. “The days of building sovereign technologies in-house are in the past,” he says. Even with a secret cloud, throwing data into a common bucket is not easy. The idea of a unifying model has faded, says Mr Warner: “The nga is going to have its model, nsa is going to have its model, cia may have a third.” There are bureaucratic and technical reasons. “Much of the key work that needs to be done to make the intelligence community ai -ready isn’t very sexy,” says Jason Matheny, who oversaw technology and national-security policy in the White House until 2022. “It’s building systems that modern software can run on. It’s ensuring that databases within and across agencies are interoperable.” The name’s GPT…A larger question is whether llm s can go beyond understanding the world to acting in it. Humint agencies refer to the recruitment cycle of spotting a target, assessing their value, developing a relationship and then recruiting them. In 2023 Sir Richard Moore, head of mi 6, hinted that ai was already helping. “My teams are now using ai to augment, but not replace, their own judgment about how people might act in various situations,” he said. “In future…as AI begins to overtake some aspects of human cognition...digital tools may come to understand, or…predict, human behaviour better than humans.”  In a working paper published in March, researchers at the Swiss Federal Institute of Technology in Lausanne describe an experiment in which participants engaged in short debates against another human, the gpt -4 model or the same model given basic information about the participant, such as age, employment and political affiliation. The personalised model was 82% more persuasive than a human (see chart). The ai players “tend to implement logical and analytical thinking significantly more than humans”. The next frontier could be “agentic” ai , in which llm s can perform actions on a user’s behalf. The Alan Turing Institute recently tested llm _ osint , a model which can build a dossier on someone using open sources, answer questions about them, develop a psychological profile and write convincing phishing emails. If that model were paired with others, it is possible to imagine a virtual case officer performing every step in the intelligence cycle, including recruitment. Sceptics point to a human element that ai cannot replicate. Jack O’Connor, a former cia officer, writes of how an analyst realised the Soviet cruise ships ferrying troops to Cuba in 1962 were the ones with empty swimming pools. Algorithms “may never be able to detect…when something absent from the image may be more important than all the objects that are on the image”, he concludes. “There will always be an extraordinary bond that allows one person genuinely to confide in another,” insists Sir Richard. “However swift and all-encompassing the advance of ai , some relationships are going to stay uniquely, stubbornly human.” ■ 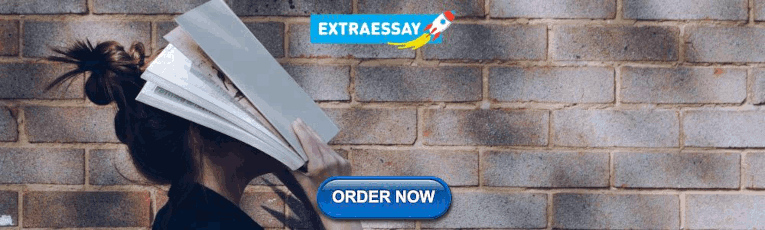 |
IMAGES
VIDEO
COMMENTS
MS, Integrated Geospatial Technology: Thesis Option. This option requires a research thesis prepared under the supervision of the advisor. The thesis describes a research investigation and its results. The scope of the research topic for the thesis should be defined in such a way that a full-time student could complete the requirements for a ...
The integration of geospatial technology opens up opportunities for more engaging and effective contextualized learning in the digital era. Discover the world's research. 25+ million members;
technology. These four factors are the backbone to the geospatial realm. Together, users can create maps, analyze images, and verify their results with accuracy assessments (American Association for the Advancement of Science, 2015). For this thesis, geospatial technologies were used to examine the road and trail network
This thesis reviews the smart city concept in relation to geospatial information and technologies. It aims to do so by defining the ideal smart city information system and comparing this with a prototype geospatial information model. ... (Percivall et al., 2015). Geographic Information Systems (GIS), being ICT technology, can therefore serve as ...
Apply for a Ph.D. in Geospatial Analytics. Ten fully funded Ph.D. graduate assistantships with $30,000 salary, benefits, and tuition waiver are available for Fall 2024 through the Center for Geospatial Analytics. Applications for Fall 2024 admissions are now open. The application deadline is February 1, 2024 - all recommendations and test scores must be received by this date.
3 Scope of Geospatial Technologies and Smart City Integration. In recent years, there have been several initiatives to use geospatial technologies and smart city applications to manage flooding in Malaysia's cities and towns. First example, is the application in creating flood hazard maps in the state of Penang.
Abstract. Geospatial technology helps in the creation, management, analysis, and visualization of spatial data. For Smart citySmart City management and functional applications; geospatial data and ...
MS, Integrated Geospatial Technology: Thesis Option. This option requires a research thesis prepared under the supervision of the advisor. The thesis describes a research investigation and its results. The scope of the research topic for the thesis should be defined in such a way that a full-time student could complete the requirements for a ...
2. Summary. This thesis reviews the smart city concept in relation to geospatial information and technologies. It aims to do so by defining the ideal smart city information system and comparing this with a prototype geospatial information model. Also, an overview is given of geospatial applications in smart city development.
Geospatial Technology and Smart Cities: ICT, Geoscience Modeling, GIS and Remote Sensing. This book presents fundamental and applied research in developing geospatial modeling solutions to manage the challenges that urban areas are facing today. It aims to connect the academics, researchers, experts, town planners, investors and government ...
The level of destruction caused by an earthquake depends on a variety of factors, such as magnitude, duration, intensity, time of occurrence, and underlying geological features, which may be mitigated and reduced by the level of preparedness of risk management measures. Geospatial technologies offer a means by which earthquake occurrence can be predicted or foreshadowed; managed in terms of ...
The Research Master of Science in Geospatial Information Science and Technology gives students the opportunity to develop geospatial research and applied skills. Students complete core geospatial courses and a collaborate with a faculty advisor to complete a research thesis.
Geospatial technology has been recognised as useful tools in ensuring sustainable development (Xiuwan, 2002) Geospatial Technology Geospatial Technologies are the methods used for the measurement, analysis and visualisation of features and phenomena that occur on Earth. The three commonly used technologies are: Global Positioning Systems (GPS ...
Geospatial technology helps in the creation, management, analysis, and visualization of spatial data. For Smart city management and functional applications; geospatial data and geospatial technology are instrumental. In this paper, geospatial technology and its role have been broadly discussed to assess its significance in smart city development. A smart city concept is considered to transform ...
The international Master's program (Master of Science, M.Sc.) in Geospatial Technologies is substantially supported by the EU, European Commission, Erasmus+ programme, Erasmus Mundus action, 2021-2027, project number 101049796. The MSc in Geospatial Technologies is a cooperation of: University of Münster (UM), (ifgi), Münster, Germany.
Application of Geospatial Techniques for Urban Flood Management: A Review. April 2022. DOI: 10.1007/978-3-030-94544-2_13. In book: Spatial Modelling of Flood Risk and Flood Hazards (pp.225-236 ...
We've pulled together seven thesis topics thus far, but may be adding more: Utilizing extended multispectral images for ML/AI classification. Towards global AI/ML coverage. AI/ML based Digital Terrain Model generator. Map open source vector data to high resolution 3D map. AI/ML based Bridge Extraction from 3D-Models.
The online and residential MS in Geographic Information Science and Technology provides state-of-the-art education that draws upon scientific principles and concepts with core geographic information technologies (geographic information systems, global positioning systems and remote sensing, among others). ... SSCI 594a Master's Thesis Units ...
2.1. Descriptions of the study area. Addis Ababa is the capital of Ethiopia. It is located in the heart of the country surrounded by Oromia Special Zones and covers an area about 527 km 2.The city lies to the north of the equator between 8°51′15″and 9°4′15″N latitude and 38°38′0″ and 38°55′30″E longitude (Figure 1).The city has been considered as the center of social ...
The mapping of infectious diseases using geospatial and information technology to benefit public health is not a new way of tracking the diseases (Ahmad et al. 2017; Cui et al. 2011; Hirsch 1883; Hornsby 2000; Matthew et al. 2004; May 1951; Mujica 2013; Nicholson and Mather 1996; Noble et al. 2012; Perl and Moalem 2006; Williams et al. 1986).The historical disease mapping has faced many ...
In this playbook we will walk through our thesis, which analyzes geospatial intelligence (GEOINT) through the lens of technology layers: Infrastructure, Distribution, and Applications. This GEOINT analysis framework helps us connect the dots from the origin and constraints of the geospatial stack to evolution and inflection within the market.
Geospatial technology is a combination of state-of-the-art remote sensing and technology for geographic information systems (GIS) and global navigation satellite systems (GNSS) for the mapping and monitoring of landscapes and environment. The main thrust of using geospatial technology is to understand the causes, mechanisms, and consequences of spatial heterogeneity, while its ultimate ...
The precise topic of a new MSc project will be defined in collaboration with the external animal ecology group. Methods, requirements: Computational movement analysis; machine learning (e.g. using RapidMiner, R, Matlab); statistical analysis (using R); programming in R and/or Matlab (or Python or Java)
In this presentation, East View Geospatial (EVG) will share how they provide a wide range of vector and raster products produced from authoritative maritime and hydrographic sources to support the GIS-fluent research and analytical community. Known to navigators worldwide, vector-based Electronic Navigation Charts (ENCs) and their raster cousins RNCs have long provided unique and useful ...
Abayomi Adesina, a visionary leader in the field of Geospatial Technology, has revealed how he is leveraging his expertise to drive economic growth in Nigeria. Monday, 1st July 2024 .
Start with geospatial intelligence (geoint).Machines have not solved the problem that led to the cia's interest in the Perceptron: too many images from space, too few people and too little time ...