- Data Management Plans: A Comprehensive Guide for Researchers
This guide outlines the main areas that researchers should consider when preparing a Data Management Plan (DMP). It includes information on the data specification, institutional repositories, long-term preservation services, IT infrastructure requirements and more.

Data Management Plans (DMPs) are documents prepared by researchers as they plan a project and write a grant proposal. These plans provide an outline of the types of data that will be collected, the data format, including data and metadata standards, and how the data will be handled throughout the project lifecycle. Many research funders require a data management plan with a grant proposal, so it is important for researchers to understand the main areas to consider when preparing one. This guide outlines the main areas that researchers should consider when preparing a data management plan.
It includes information on the data specification, including size, file format , number of files, data dictionary, and codebook. It also covers the use of institutional data repositories hosted in university libraries as an open access platform for the dissemination and archiving of university research data. Additionally, it provides information on long-term preservation of digital data files using services such as migration (limited format types), secure backups, bit-level checksums, and maintaining a persistent DOI for data sets. When a project includes data about people and organizations, this affects the design of the necessary IT infrastructure.
It is expected that the full dataset will be accessible after the study and all related publications are completed, and will remain accessible for at least 10 years after the data become publicly available. This section includes a description of the format of your data and how it will be created, collected, maintained and delivered. In addition, information from individuals or entities that own the intellectual property rights to the data should be included in this section. SPARC (the Academic Resources and Academic Publications Coalition) has compiled an excellent resource with information on the data management and data exchange requirements of all federal funding agencies.
ICPSR allows several mediated forms of exchange, where individuals interested in obtaining a less de-identified individual level would sign data use agreements before receiving the data, or would need to use special software to access it directly from ICPSR instead of downloading it, for security reasons. Some demographics may not be shareable on an individual level and would therefore only be provided in aggregate form. Finally, all applicants submitting funding proposals to the MRC must include a Data Management Plan (DMP) as an integral part of the application. Through DRUM policies, unidentified data will be accompanied by appropriate documentation, metadata and code to facilitate reuse and provide the potential for interoperability with similar datasets. The main benefit of DRUM is that everything shared through this repository is public; however, a fully open system is not optimal if any of the data can be identified.
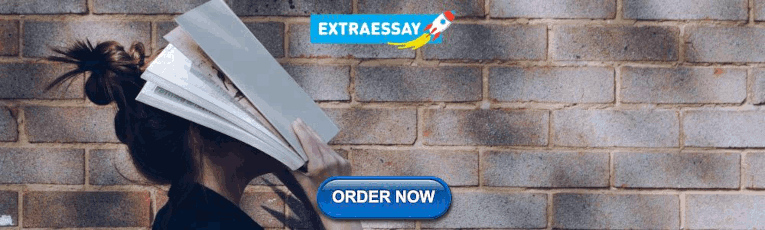
Related Posts
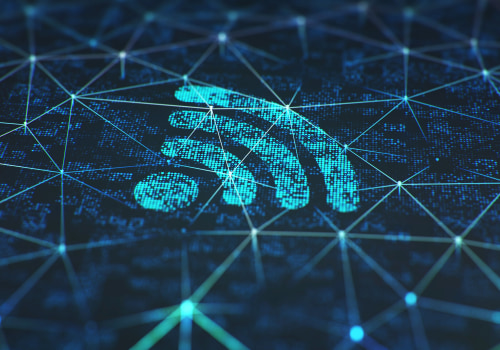
- What are data standards and interoperability?
Data standards support semantic interoperability, that is, the ability of systems that exchange data to interpret them correctly. For example, different health systems may use different terms for the same concept (for example, interoperability refers to the ability of different IT systems with different infrastructures to accurately share data).
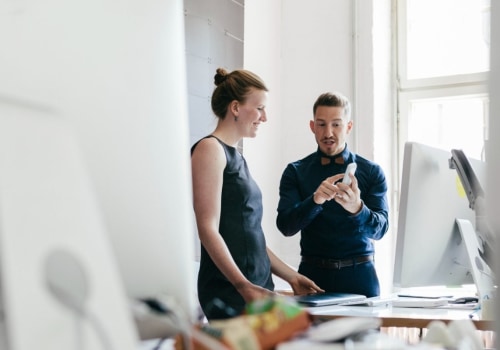
- The Benefits of Enterprise Data Management
Learn about the benefits & advantages of enterprise data management (EDM) for organizations including reducing risk & increasing efficiency.

- Data Management Services: A Comprehensive Guide
Data management services provide businesses with a centralized storage platform for disparate data sources. Learn how to develop a comprehensive approach to data management that complies with regulatory requirements such as the General Data Protection Regulation (G

- What should a data management plan include?
A data management plan (DMP) describes the data that will be acquired or produced during the research; how the data will be managed, described and stored, what standards will be used and how the data will be managed and protected during and after the completion of the project. A data management plan, or DMP, is a formal document that describes what you will do with your data during and after a research project.
More articles

- The Benefits of Understanding Project Data and Processes
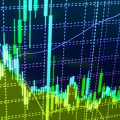
- The Benefits of Data Management in Research

- Data Management: A Comprehensive Guide to the Process

- Best Practices for Managing Big Data Analytics Programs
- Data Management Softwares: A Comprehensive Guide
- What are spreadsheet data tools?
- Why is tools management important?
- Data Collection Methods: An Expert's Guide
- What is a value in data management?
- What are the data management principles?
- Data Management Tools: A Comprehensive Guide
- The Significance of Data Management in Mathematics
- A Comprehensive Guide to Different Types of Data Management
- Types of Data Management Functions Explained
- Who created data management?
- Who will approve data management plan?
Understanding Research Data Management Systems
- What is Data Management and How to Manage it Effectively?
- What is Enterprise Data Management and How Can It Help Your Organization?
- Data Management Standards: A Comprehensive Guide
- What is data standard?
- Is sql a database management system?
- Data Management in Research Studies: An Expert's Guide
- The Benefits of Data Management for Businesses
- The Ultimate Guide to Enterprise Data Management
- Data Management: What is it and How to Use it?
- Do You Need Math to Become a Data Scientist?
- What is Data Management Software and How Does it Work?
- Data Management: A Comprehensive Guide to Activities Involved
- What is a data principle?
- 6 Major Areas for Best Practices in Data Management
- What are the 5 importance of data processing?
- What is Enterprise Data Management and How to Implement It?
- The Essential Role of Data Management in the Digital Economy
- The Benefits of Data Management: Unlocking the Power of Your Data
- What software create and manage data base?
- Data Management: What You Need to Know
- The Essential Role of Data Management in Business
- What is a data standard?
- Data Management Best Practices: A Comprehensive Guide
- 10 Steps to Master Data Management
- Data Management: A Comprehensive Guide
- The Essential Role of Enterprise Data Management
- Data Management: Functions, Storage, Security and More
- What is data processing and its types?
- Organizing IoT Device Data: A Comprehensive Guide
- Data Management in Research: A Comprehensive Guide
- What are database systems?
- Unlocking the Secrets of Big Data Analytics: A Comprehensive Guide
The Ultimate Guide to Data Management
- Types of Management Tools: A Comprehensive Guide
- The Benefits of Enterprise Data Management for Businesses
The Benefits of Research Data Management
- What is a data management tool?
- Data Management: Unlocking the Power of Data Analysis
- The 10 Principles of Data Management for a Successful Organization
- What Are the Different Types of Management Tools?
- Data Management Principles: A Comprehensive Guide
- The Benefits of Using Management Tools for Businesses
- Which of the following is api management tool?
- What tools are used in data analysis?
- What is Enterprise Data Management Framework?
- Six Major Areas for Best Practices in Data Management
- The Benefits of Data Management: Unlocking the Potential of Data
- What are the 5 layers of big data architecture?
- What is the Purpose of a Data Management Plan?
- Four Essential Best Practices for Big Data Governance
- The Benefits of Data Management in Research: A Comprehensive Guide
- Data Management: A Comprehensive Guide to Unlocking Data Potential
- The Benefits of Data Management in Biology
- Data Management: A Comprehensive Guide for Researchers
- Data Management: Unlocking the Potential of Data
- Data Management: An Overview of the 5 Key Functions
- 9 Best Practices of Master Data Management
- The Advantages of Enterprise Data Management
- What is managing the data?
- A Comprehensive Guide to Data Management Plans
- Types of Data Management Tools: A Comprehensive Guide
- What are the 5 basic steps in data analysis?
- Data Management Services: What You Need to Know
- Data Governance vs Data Management: What's the Difference?
- The Benefits of Data Management Tools
- What is data management framework in d365?
- Data Management: What is it and How to Implement it?
New Articles

Which cookies do you want to accept?
Have a language expert improve your writing
Run a free plagiarism check in 10 minutes, generate accurate citations for free.
- Knowledge Base
- Starting the research process
- How to Write a Research Proposal | Examples & Templates
How to Write a Research Proposal | Examples & Templates
Published on October 12, 2022 by Shona McCombes and Tegan George. Revised on November 21, 2023.
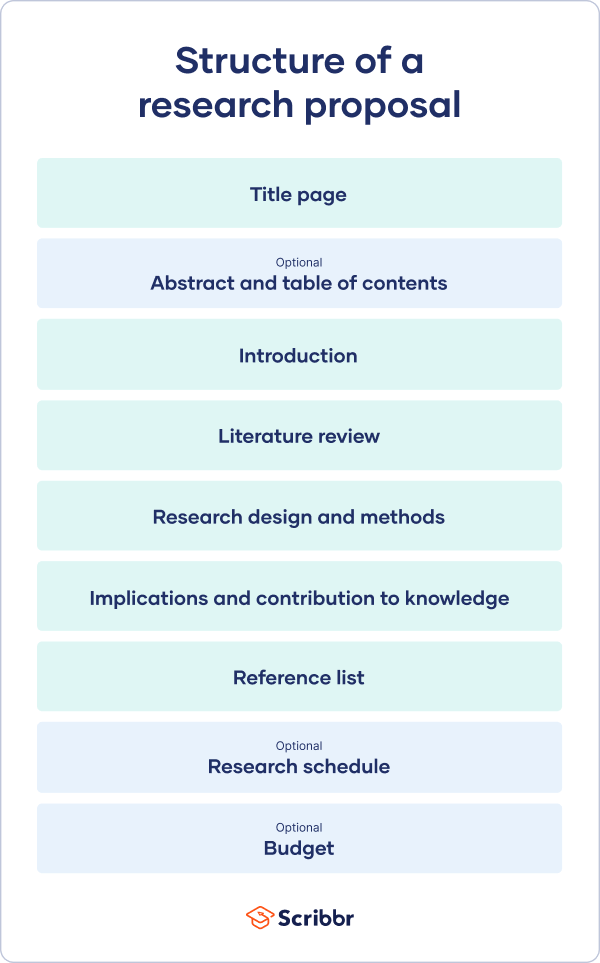
A research proposal describes what you will investigate, why it’s important, and how you will conduct your research.
The format of a research proposal varies between fields, but most proposals will contain at least these elements:
Introduction
Literature review.
- Research design
Reference list
While the sections may vary, the overall objective is always the same. A research proposal serves as a blueprint and guide for your research plan, helping you get organized and feel confident in the path forward you choose to take.
Table of contents
Research proposal purpose, research proposal examples, research design and methods, contribution to knowledge, research schedule, other interesting articles, frequently asked questions about research proposals.
Academics often have to write research proposals to get funding for their projects. As a student, you might have to write a research proposal as part of a grad school application , or prior to starting your thesis or dissertation .
In addition to helping you figure out what your research can look like, a proposal can also serve to demonstrate why your project is worth pursuing to a funder, educational institution, or supervisor.
Research proposal length
The length of a research proposal can vary quite a bit. A bachelor’s or master’s thesis proposal can be just a few pages, while proposals for PhD dissertations or research funding are usually much longer and more detailed. Your supervisor can help you determine the best length for your work.
One trick to get started is to think of your proposal’s structure as a shorter version of your thesis or dissertation , only without the results , conclusion and discussion sections.
Download our research proposal template
Here's why students love Scribbr's proofreading services
Discover proofreading & editing
Writing a research proposal can be quite challenging, but a good starting point could be to look at some examples. We’ve included a few for you below.
- Example research proposal #1: “A Conceptual Framework for Scheduling Constraint Management”
- Example research proposal #2: “Medical Students as Mediators of Change in Tobacco Use”
Like your dissertation or thesis, the proposal will usually have a title page that includes:
- The proposed title of your project
- Your supervisor’s name
- Your institution and department
The first part of your proposal is the initial pitch for your project. Make sure it succinctly explains what you want to do and why.
Your introduction should:
- Introduce your topic
- Give necessary background and context
- Outline your problem statement and research questions
To guide your introduction , include information about:
- Who could have an interest in the topic (e.g., scientists, policymakers)
- How much is already known about the topic
- What is missing from this current knowledge
- What new insights your research will contribute
- Why you believe this research is worth doing
As you get started, it’s important to demonstrate that you’re familiar with the most important research on your topic. A strong literature review shows your reader that your project has a solid foundation in existing knowledge or theory. It also shows that you’re not simply repeating what other people have already done or said, but rather using existing research as a jumping-off point for your own.
In this section, share exactly how your project will contribute to ongoing conversations in the field by:
- Comparing and contrasting the main theories, methods, and debates
- Examining the strengths and weaknesses of different approaches
- Explaining how will you build on, challenge, or synthesize prior scholarship
Following the literature review, restate your main objectives . This brings the focus back to your own project. Next, your research design or methodology section will describe your overall approach, and the practical steps you will take to answer your research questions.
To finish your proposal on a strong note, explore the potential implications of your research for your field. Emphasize again what you aim to contribute and why it matters.
For example, your results might have implications for:
- Improving best practices
- Informing policymaking decisions
- Strengthening a theory or model
- Challenging popular or scientific beliefs
- Creating a basis for future research
Last but not least, your research proposal must include correct citations for every source you have used, compiled in a reference list . To create citations quickly and easily, you can use our free APA citation generator .
Some institutions or funders require a detailed timeline of the project, asking you to forecast what you will do at each stage and how long it may take. While not always required, be sure to check the requirements of your project.
Here’s an example schedule to help you get started. You can also download a template at the button below.
Download our research schedule template
If you are applying for research funding, chances are you will have to include a detailed budget. This shows your estimates of how much each part of your project will cost.
Make sure to check what type of costs the funding body will agree to cover. For each item, include:
- Cost : exactly how much money do you need?
- Justification : why is this cost necessary to complete the research?
- Source : how did you calculate the amount?
To determine your budget, think about:
- Travel costs : do you need to go somewhere to collect your data? How will you get there, and how much time will you need? What will you do there (e.g., interviews, archival research)?
- Materials : do you need access to any tools or technologies?
- Help : do you need to hire any research assistants for the project? What will they do, and how much will you pay them?
If you want to know more about the research process , methodology , research bias , or statistics , make sure to check out some of our other articles with explanations and examples.
Methodology
- Sampling methods
- Simple random sampling
- Stratified sampling
- Cluster sampling
- Likert scales
- Reproducibility
Statistics
- Null hypothesis
- Statistical power
- Probability distribution
- Effect size
- Poisson distribution
Research bias
- Optimism bias
- Cognitive bias
- Implicit bias
- Hawthorne effect
- Anchoring bias
- Explicit bias
Once you’ve decided on your research objectives , you need to explain them in your paper, at the end of your problem statement .
Keep your research objectives clear and concise, and use appropriate verbs to accurately convey the work that you will carry out for each one.
I will compare …
A research aim is a broad statement indicating the general purpose of your research project. It should appear in your introduction at the end of your problem statement , before your research objectives.
Research objectives are more specific than your research aim. They indicate the specific ways you’ll address the overarching aim.
A PhD, which is short for philosophiae doctor (doctor of philosophy in Latin), is the highest university degree that can be obtained. In a PhD, students spend 3–5 years writing a dissertation , which aims to make a significant, original contribution to current knowledge.
A PhD is intended to prepare students for a career as a researcher, whether that be in academia, the public sector, or the private sector.
A master’s is a 1- or 2-year graduate degree that can prepare you for a variety of careers.
All master’s involve graduate-level coursework. Some are research-intensive and intend to prepare students for further study in a PhD; these usually require their students to write a master’s thesis . Others focus on professional training for a specific career.
Critical thinking refers to the ability to evaluate information and to be aware of biases or assumptions, including your own.
Like information literacy , it involves evaluating arguments, identifying and solving problems in an objective and systematic way, and clearly communicating your ideas.
The best way to remember the difference between a research plan and a research proposal is that they have fundamentally different audiences. A research plan helps you, the researcher, organize your thoughts. On the other hand, a dissertation proposal or research proposal aims to convince others (e.g., a supervisor, a funding body, or a dissertation committee) that your research topic is relevant and worthy of being conducted.
Cite this Scribbr article
If you want to cite this source, you can copy and paste the citation or click the “Cite this Scribbr article” button to automatically add the citation to our free Citation Generator.
McCombes, S. & George, T. (2023, November 21). How to Write a Research Proposal | Examples & Templates. Scribbr. Retrieved March 28, 2024, from https://www.scribbr.com/research-process/research-proposal/
Is this article helpful?
Shona McCombes
Other students also liked, how to write a problem statement | guide & examples, writing strong research questions | criteria & examples, how to write a literature review | guide, examples, & templates, unlimited academic ai-proofreading.
✔ Document error-free in 5minutes ✔ Unlimited document corrections ✔ Specialized in correcting academic texts

Research Data Management
- Finding Existing Data
- Data Citation
- Research Proposal
- RDM Requirements
- Collaboration
- Data Security
- GDPR & Privacy
- Data Management Plan
- Policies & Regulations
- Data Collection
- Data Storage
- Data Protection
- Data Provenance
- Data Processing
- Data Analysis
- High Performance Computing
- Selecting Data
- Data Documentation
- Selecting an Archive
- Data Publication
- Dataset Registration
Research Proposal / Grant Office
Grant programmes from organisations like NWO, ZonMW and ERC increasingly require you to not only think about the journey of the data in your research project, but also the method of data collection and how to protect or share data during and after the research project. It is important to bear in mind the specific laws and regulations that apply to the kind of data that is collected. If a project involves data on persons and organisations this impacts the design of the necessary IT infrastructure. A more detailed description of this will later be captured in the data management plan.
When writing your research proposal the following items are important:
- Fill in the data management paragraph (see the four questions below)
- Planning: one of the early deliverables will be a detailed data management plan
- Budget: take into account the costs (labour and material) for data storage during and data archiving after your project.
- Writing: Funders that distribute grants like to maximise the effectiveness of this investment. It is therefore highly recommended that the data will be made Findable, Accessible, Interoperable and Re-usable (FAIRdata). This does not mean that the data have to be open: laws, licenses and contracts regarding personal and sensitive data may limit the possibility to share the data publicly.
Research Data Services provides advice and help when writing a data paragraph as part of the Research proposal. The Library also regularly organises workshops to help you get started. Together with the VU Grants Office and project control we are part of the grant support team offering advice and practical aid for your grant. You will be directed to the specific unit during your support trajectory. Make sure to contact the team as early as possible.
Data Management Paragraph
In order to make data re-usable, funders require researchers to include a data section (= paragraph) in their project proposal, in which they explain whether research data will be collected or generated during the project, and how they plan to structure, archive and share their data. Depending on requirements of the funder, the paragraph can be short or more extensive.
Funders may have different requirements for the Data Management Paragraph in the project proposal. Always check what your funder asks for. For example, in 2016, the NWO formulated four questions that need to be answered in the data paragraph of the research proposal:
- Will data be collected or generated that are suitable for re-use?
- Where will the data be stored during the research?
- After the project has been completed, how will the data be stored for the long term and made available for the use by third parties? To whom will the data be accessible?
- Which facilities (ICT, (secure) archive, refrigerators or legal expertise) do you expect will be needed for the storage of data during the research and after the research? Are these available?
- << Previous: Plan & Design
- Next: RDM Costs >>
- Last Updated: Feb 22, 2024 1:30 PM
- URL: https://libguides.vu.nl/rdm
Manage Research Data
Categories:
- Award Management
- Regulatory Compliance
Introduction
FAIRport Guiding Principles drive the University’s scientific data management. Make sure you understand the type of data you want to share or exchange. Your proposal may require a data management plan and your award may need a data use agreement. Sharing data Stanford provides guidance and resources for research data acquisition, sharing and management. Some data is subject to particular protections which require additional terms and management considerations.
The Data Management Plan
A data management plan (DMP) may be required as part of your proposal documentation to comply with funding agency provisions on data management and to improve the visibility of their research. The DMP is a written document that describes the data you expect to acquire or generate during the course of a research project, how you will manage, describe, analyze, and store those data, and what mechanisms you will use at the end of your project to share and preserve your data.
The DMP Tool (Data Management Planning Tool) provides templates, Stanford-specific guidance, and suggested answer text for creating a data management plan for your next grant submission.
Research Data Management
Stanford University Libraries offer Data Management Services to assist Stanford's researchers with the organization, management, and curation of research data, including:
Understanding and creating data management plans
Organizing and backing up your research data
Acquiring and analyzing data
Assigning metadata to enable future discovery
Preserving your data for long-term access
In addition, the Stanford Digital Repository provides long-term preservation of your important research data in a secure, sustainable stewardship environment, combined with a persistent URL (PURL) that allows for easy data discovery, access, sharing, and reuse.
Data Use Agreements
A Data Use Agreement (“DUA”) is a contract that governs the exchange of specific data between two parties. DUA’s establish who is permitted to use and receive a unique data set, along with the allowable uses and disclosures of the data by the recipient. A DUA also assigns appropriate responsibility to the researcher and recipient for using the data. The DUA must be entered into before there is any use or disclosure of a limited data set to an outside institution or party.
Common terms of a DUA provide that the recipient will:
not use, disclose, or destroy the data set other than as permitted by the DUA, or as required by law;
use appropriate administrative, technical, and physical safeguards to prevent unauthorized uses or disclosures of the data set, including specific data transfer/access/disposition instructions;
report to the provider any uses or disclosures of the data set that are in violation of a DUA;
ensure that anyone to whom it provides the data set agree to the same requirements that apply to the recipient for receiving or accessing the data; and
not re-identify or contact the data subjects (for data related to a human subject).
Clinical Research Data
The Stanford Data Science Resources can help you access the tools, datasets, data platforms and methodologies for conducting innovative clinical and translational research.
The School of Medicine offers a limited initial consultation (underwritten by the Dean’s Office and Spectrum ) to help you identify the resources you need. These consults may lead to longer-term engagements and partnerships with one or more of the consulting groups from across the School of Medicine.
Through these consulting groups, you can access datasets, a variety of platforms and tools and research services, including expert advice on databases and management, study design and implementation, biostatistics, informatics, technology integration, and much more.
Genomic Data Sharing
Effective January 25, 2015, all NIH-supported research that generates large-scale genomic data and uses large scale human or non-human genomic data, as well as the use of these data for subsequent research:
Large-scale data include genome-wide association studies (GWAS), single nucleotide polymorphisms (SNP) arrays, and genome sequence, transcriptomic, metagenomic, epigenomic, and gene expression data, irrespective of funding level and funding mechanism (e.g., grant, contract, cooperative agreement, or intramural support).
Examples include, but are not limited to, sequence data from more than one gene or region of comparable size in the genomes of more than 1000 human research participants; sequence data from more than 100 genes in the genomes of more than 100 human research participants; comparisons of differentially methylated sites genome-wide at single-base resolution within a given sample (e.g. within the same subjects over time or across cell types). Additional examples are available in the Supplemental Information to the NIH GDS Policy. When does the policy NOT apply? Examples of NIH-funded research or research-related activities that are outside the Policy’s scope include, but are not limited to, projects that do not meet the criteria above such as: instrument calibration exercises statistical or technical methods development, or the use of genomic data for control purposes, such as for assay development.
In addition, the following types of funding generally do not fall under the GDS Policy: Institutional Training Grants, K12 Career Awards, Individual Fellowships (Fs) such as the Ruth L. Kirschstein National Research Service Award, Resource Grants and Contracts (Ss), or Facilities and coordinating centers funded to provide genotyping, sequencing, or other core services in support of GDS.
Extramural Institutional Certification is required prior to depositing human genomic data into one of the NIH-supported repositories, even if the research itself is not NIH-supported. NIHsupported repositories include, but are not limited to Database of Genotypes and Phenotypes (dbGaP), Gene Expression Omnibus (GEO), or the Sequence Read Archive (SRA).
The European Union GDPR
The European Union General Data Protection Regulation, or GDPR, is a new and substantial data privacy law that is relevant to 33 countries in the EU and European Economic Area.
GDPR applies to individuals and organizations handling personal data within the EU, transferring data into and out of the EU, and processing of EU data anywhere. It is effective as of May 25th, 2018.
Created: 03.25.2021
Updated: 03.28.2024
Ask Yale Library
My Library Accounts
Find, Request, and Use
Help and Research Support
Visit and Study
Explore Collections
Research Data Management: Plan for Data
- Plan for Data
- Organize & Document Data
- Store & Secure Data
- Validate Data
- Share & Re-use Data
- Data Use Agreements
- Research Data Policies
What is a Data Management Plan?
Data management plans (DMPs) are documents that outline how data will be collected , stored , secured , analyzed , disseminated , and preserved over the lifecycle of a research project. They are typically created in the early stages of a project, and they are typically short documents that may evolve over time. Increasingly, they are required by funders and institutions alike, and they are a recommended best practice in research data management.
Tab through this guide to consider each stage of the research data management process, and each correlated section of a data management plan.
Tools for Data Management Planning
DMPTool is a collaborative effort between several universities to streamline the data management planning process.
The DMPTool supports the majority of federal and many non-profit and private funding agencies that require data management plans as part of a grant proposal application. ( View the list of supported organizations and corresponding templates.) If the funder you're applying to isn't listed or you just want to create one as good practice, there is an option for a generic plan.
Key features:
Data management plan templates from most major funders
Guided creation of a data management plan with click-throughs and helpful questions and examples
Access to public plans , to review ahead of creating your own
Ability to share plans with collaborators as well as copy and reuse existing plans
How to get started:
Log in with your yale.edu email to be directed to a NetID sign-in, and review the quick start guide .
Research Data Lifecycle
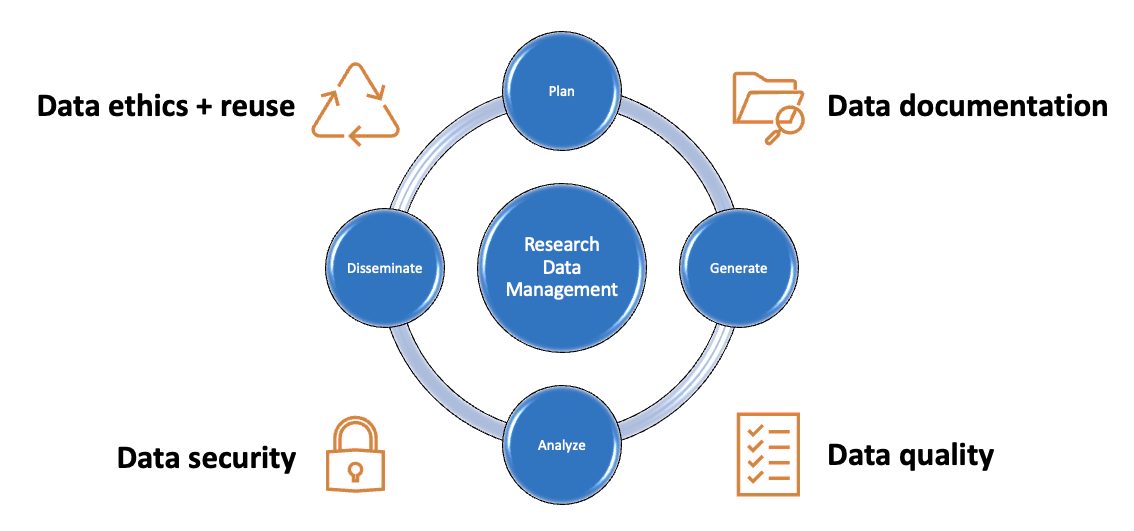
Additional Resources for Data Management Planning
- << Previous: Overview
- Next: Organize & Document Data >>
- Last Updated: Sep 27, 2023 1:15 PM
- URL: https://guides.library.yale.edu/datamanagement

Site Navigation
P.O. BOX 208240 New Haven, CT 06250-8240 (203) 432-1775
Yale's Libraries
Bass Library
Beinecke Rare Book and Manuscript Library
Classics Library
Cushing/Whitney Medical Library
Divinity Library
East Asia Library
Gilmore Music Library
Haas Family Arts Library
Lewis Walpole Library
Lillian Goldman Law Library
Marx Science and Social Science Library
Sterling Memorial Library
Yale Center for British Art
SUBSCRIBE TO OUR NEWSLETTER
@YALELIBRARY

Yale Library Instagram
Accessibility Diversity, Equity, and Inclusion Giving Privacy and Data Use Contact Our Web Team
© 2022 Yale University Library • All Rights Reserved
Loading metrics
Open Access
Perspective
Ten Simple Rules for Creating a Good Data Management Plan
* E-mail: [email protected]
Affiliation College of University Libraries & Learning Sciences, University of New Mexico, Albuquerque, New Mexico, United States of America
- William K. Michener
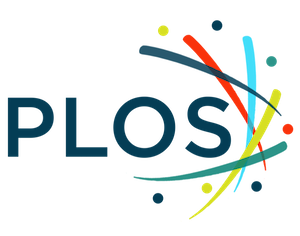
Published: October 22, 2015
- https://doi.org/10.1371/journal.pcbi.1004525
- Reader Comments
Citation: Michener WK (2015) Ten Simple Rules for Creating a Good Data Management Plan. PLoS Comput Biol 11(10): e1004525. https://doi.org/10.1371/journal.pcbi.1004525
Editor: Philip E. Bourne, National Institutes of Health, UNITED STATES
Copyright: © 2015 William K. Michener. This is an open access article distributed under the terms of the Creative Commons Attribution License , which permits unrestricted use, distribution, and reproduction in any medium, provided the original author and source are credited
Funding: This work was supported by NSF IIA-1301346, IIA-1329470, and ACI-1430508 ( http://nsf.gov ). The funders had no role in study design, data collection and analysis, decision to publish, or preparation of the manuscript.
Competing interests: The author has declared that no competing interests exist.
Introduction
Research papers and data products are key outcomes of the science enterprise. Governmental, nongovernmental, and private foundation sponsors of research are increasingly recognizing the value of research data. As a result, most funders now require that sufficiently detailed data management plans be submitted as part of a research proposal. A data management plan (DMP) is a document that describes how you will treat your data during a project and what happens with the data after the project ends. Such plans typically cover all or portions of the data life cycle—from data discovery, collection, and organization (e.g., spreadsheets, databases), through quality assurance/quality control, documentation (e.g., data types, laboratory methods) and use of the data, to data preservation and sharing with others (e.g., data policies and dissemination approaches). Fig 1 illustrates the relationship between hypothetical research and data life cycles and highlights the links to the rules presented in this paper. The DMP undergoes peer review and is used in part to evaluate a project’s merit. Plans also document the data management activities associated with funded projects and may be revisited during performance reviews.
- PPT PowerPoint slide
- PNG larger image
- TIFF original image
As part of the research life cycle (A), many researchers (1) test ideas and hypotheses by (2) acquiring data that are (3) incorporated into various analyses and visualizations, leading to interpretations that are then (4) published in the literature and disseminated via other mechanisms (e.g., conference presentations, blogs, tweets), and that often lead back to (1) new ideas and hypotheses. During the data life cycle (B), researchers typically (1) develop a plan for how data will be managed during and after the project; (2) discover and acquire existing data and (3) collect and organize new data; (4) assure the quality of the data; (5) describe the data (i.e., ascribe metadata); (6) use the data in analyses, models, visualizations, etc.; and (7) preserve and (8) share the data with others (e.g., researchers, students, decision makers), possibly leading to new ideas and hypotheses.
https://doi.org/10.1371/journal.pcbi.1004525.g001
Earlier articles in the Ten Simple Rules series of PLOS Computational Biology provided guidance on getting grants [ 1 ], writing research papers [ 2 ], presenting research findings [ 3 ], and caring for scientific data [ 4 ]. Here, I present ten simple rules that can help guide the process of creating an effective plan for managing research data—the basis for the project’s findings, research papers, and data products. I focus on the principles and practices that will result in a DMP that can be easily understood by others and put to use by your research team. Moreover, following the ten simple rules will help ensure that your data are safe and sharable and that your project maximizes the funder’s return on investment.
Rule 1: Determine the Research Sponsor Requirements
Research communities typically develop their own standard methods and approaches for managing and disseminating data. Likewise, research sponsors often have very specific DMP expectations. For instance, the Wellcome Trust, the Gordon and Betty Moore Foundation (GBMF), the United States National Institutes of Health (NIH), and the US National Science Foundation (NSF) all fund computational biology research but differ markedly in their DMP requirements. The GBMF, for instance, requires that potential grantees develop a comprehensive DMP in conjunction with their program officer that answers dozens of specific questions. In contrast, NIH requirements are much less detailed and primarily ask that potential grantees explain how data will be shared or provide reasons as to why the data cannot be shared. Furthermore, a single research sponsor (such as the NSF) may have different requirements that are established for individual divisions and programs within the organization. Note that plan requirements may not be labeled as such; for example, the National Institutes of Health guidelines focus largely on data sharing and are found in a document entitled “NIH Data Sharing Policy and Implementation Guidance” ( http://grants.nih.gov/grants/policy/data_sharing/data_sharing_guidance.htm ).
Significant time and effort can be saved by first understanding the requirements set forth by the organization to which you are submitting a proposal. Research sponsors normally provide DMP requirements in either the public request for proposals (RFP) or in an online grant proposal guide. The DMPTool ( https://dmptool.org/ ) and DMPonline ( https://dmponline.dcc.ac.uk/ ) websites are also extremely valuable resources that provide updated funding agency plan requirements (for the US and United Kingdom, respectively) in the form of templates that are usually accompanied with annotated advice for filling in the template. The DMPTool website also includes numerous example plans that have been published by DMPTool users. Such examples provide an indication of the depth and breadth of detail that are normally included in a plan and often lead to new ideas that can be incorporated in your plan.
Regardless of whether you have previously submitted proposals to a particular funding program, it is always important to check the latest RFP, as well as the research sponsor’s website, to verify whether requirements have recently changed and how. Furthermore, don’t hesitate to contact the responsible program officer(s) that are listed in a specific solicitation to discuss sponsor requirements or to address specific questions that arise as you are creating a DMP for your proposed project. Keep in mind that the principle objective should be to create a plan that will be useful for your project. Thus, good data management plans can and often do contain more information than is minimally required by the research sponsor. Note, though, that some sponsors constrain the length of DMPs (e.g., two-page limit); in such cases, a synopsis of your more comprehensive plan can be provided, and it may be permissible to include an appendix, supplementary file, or link.
Rule 2: Identify the Data to Be Collected
Every component of the DMP depends upon knowing how much and what types of data will be collected. Data volume is clearly important, as it normally costs more in terms of infrastructure and personnel time to manage 10 terabytes of data than 10 megabytes. But, other characteristics of the data also affect costs as well as metadata, data quality assurance and preservation strategies, and even data policies. A good plan will include information that is sufficient to understand the nature of the data that is collected, including:
- Types. A good first step is to list the various types of data that you expect to collect or create. This may include text, spreadsheets, software and algorithms, models, images and movies, audio files, and patient records. Note that many research sponsors define data broadly to include physical collections, software and code, and curriculum materials.
- Sources. Data may come from direct human observation, laboratory and field instruments, experiments, simulations, and compilations of data from other studies. Reviewers and sponsors may be particularly interested in understanding if data are proprietary, are being compiled from other studies, pertain to human subjects, or are otherwise subject to restrictions in their use or redistribution.
- Volume. Both the total volume of data and the total number of files that are expected to be collected can affect all other data management activities.
- Data and file formats. Technology changes and formats that are acceptable today may soon be obsolete. Good choices include those formats that are nonproprietary, based upon open standards, and widely adopted and preferred by the scientific community (e.g., Comma Separated Values [CSV] over Excel [.xls, xlsx]). Data are more likely to be accessible for the long term if they are uncompressed, unencrypted, and stored using standard character encodings such as UTF-16.
The precise types, sources, volume, and formats of data may not be known beforehand, depending on the nature and uniqueness of the research. In such case, the solution is to iteratively update the plan (see Rule 9 ).
Rule 3: Define How the Data Will Be Organized
Once there is an understanding of the volume and types of data to be collected, a next obvious step is to define how the data will be organized and managed. For many projects, a small number of data tables will be generated that can be effectively managed with commercial or open source spreadsheet programs like Excel and OpenOffice Calc. Larger data volumes and usage constraints may require the use of relational database management systems (RDBMS) for linked data tables like ORACLE or mySQL, or a Geographic Information System (GIS) for geospatial data layers like ArcGIS, GRASS, or QGIS.
The details about how the data will be organized and managed could fill many pages of text and, in fact, should be recorded as the project evolves. However, in drafting a DMP, it is most helpful to initially focus on the types and, possibly, names of the products that will be used. The software tools that are employed in a project should be amenable to the anticipated tasks. A spreadsheet program, for example, would be insufficient for a project in which terabytes of data are expected to be generated, and a sophisticated RDMBS may be overkill for a project in which only a few small data tables will be created. Furthermore, projects dependent upon a GIS or RDBMS may entail considerable software costs and design and programming effort that should be planned and budgeted for upfront (see Rules 9 and 10 ). Depending on sponsor requirements and space constraints, it may also be useful to specify conventions for file naming, persistent unique identifiers (e.g., Digital Object Identifiers [DOIs]), and versioning control (for both software and data products).
Rule 4: Explain How the Data Will Be Documented
Rows and columns of numbers and characters have little to no meaning unless they are documented in some fashion. Metadata—the details about what, where, when, why, and how the data were collected, processed, and interpreted—provide the information that enables data and files to be discovered, used, and properly cited. Metadata include descriptions of how data and files are named, physically structured, and stored as well as details about the experiments, analytical methods, and research context. It is generally the case that the utility and longevity of data relate directly to how complete and comprehensive the metadata are. The amount of effort devoted to creating comprehensive metadata may vary substantially based on the complexity, types, and volume of data.
A sound documentation strategy can be based on three steps. First, identify the types of information that should be captured to enable a researcher like you to discover, access, interpret, use, and cite your data. Second, determine whether there is a community-based metadata schema or standard (i.e., preferred sets of metadata elements) that can be adopted. As examples, variations of the Dublin Core Metadata Initiative Abstract Model are used for many types of data and other resources, ISO (International Organization for Standardization) 19115 is used for geospatial data, ISA-Tab file format is used for experimental metadata, and Ecological Metadata Language (EML) is used for many types of environmental data. In many cases, a specific metadata content standard will be recommended by a target data repository, archive, or domain professional organization. Third, identify software tools that can be employed to create and manage metadata content (e.g., Metavist, Morpho). In lieu of existing tools, text files (e.g., readme.txt) that include the relevant metadata can be included as headers to the data files.
A best practice is to assign a responsible person to maintain an electronic lab notebook, in which all project details are maintained. The notebook should ideally be routinely reviewed and revised by another team member, as well as duplicated (see Rules 6 and 9 ). The metadata recorded in the notebook provide the basis for the metadata that will be associated with data products that are to be stored, reused, and shared.
Rule 5: Describe How Data Quality Will Be Assured
Quality assurance and quality control (QA/QC) refer to the processes that are employed to measure, assess, and improve the quality of products (e.g., data, software, etc.). It may be necessary to follow specific QA/QC guidelines depending on the nature of a study and research sponsorship; such requirements, if they exist, are normally stated in the RFP. Regardless, it is good practice to describe the QA/QC measures that you plan to employ in your project. Such measures may encompass training activities, instrument calibration and verification tests, double-blind data entry, and statistical and visualization approaches to error detection. Simple graphical data exploration approaches (e.g., scatterplots, mapping) can be invaluable for detecting anomalies and errors.
Rule 6: Present a Sound Data Storage and Preservation Strategy
A common mistake of inexperienced (and even many experienced) researchers is to assume that their personal computer and website will live forever. They fail to routinely duplicate their data during the course of the project and do not see the benefit of archiving data in a secure location for the long term. Inevitably, though, papers get lost, hard disks crash, URLs break, and tapes and other media degrade, with the result that the data become unavailable for use by both the originators and others. Thus, data storage and preservation are central to any good data management plan. Give careful consideration to three questions:
- How long will the data be accessible?
- How will data be stored and protected over the duration of the project?
- How will data be preserved and made available for future use?
The answer to the first question depends on several factors. First, determine whether the research sponsor or your home institution have any specific requirements. Usually, all data do not need to be retained, and those that do need not be retained forever. Second, consider the intrinsic value of the data. Observations of phenomena that cannot be repeated (e.g., astronomical and environmental events) may need to be stored indefinitely. Data from easily repeatable experiments may only need to be stored for a short period. Simulations may only need to have the source code, initial conditions, and verification data stored. In addition to explaining how data will be selected for short-term storage and long-term preservation, remember to also highlight your plans for the accompanying metadata and related code and algorithms that will allow others to interpret and use the data (see Rule 4 ).
Develop a sound plan for storing and protecting data over the life of the project. A good approach is to store at least three copies in at least two geographically distributed locations (e.g., original location such as a desktop computer, an external hard drive, and one or more remote sites) and to adopt a regular schedule for duplicating the data (i.e., backup). Remote locations may include an offsite collaborator’s laboratory, an institutional repository (e.g., your departmental, university, or organization’s repository if located in a different building), or a commercial service, such as those offered by Amazon, Dropbox, Google, and Microsoft. The backup schedule should also include testing to ensure that stored data files can be retrieved.
Your best bet for being able to access the data 20 years beyond the life of the project will likely require a more robust solution (i.e., question 3 above). Seek advice from colleagues and librarians to identify an appropriate data repository for your research domain. Many disciplines maintain specific repositories such as GenBank for nucleotide sequence data and the Protein Data Bank for protein sequences. Likewise, many universities and organizations also host institutional repositories, and there are numerous general science data repositories such as Dryad ( http://datadryad.org/ ), figshare ( http://figshare.com/ ), and Zenodo ( http://zenodo.org/ ). Alternatively, one can easily search for discipline-specific and general-use repositories via online catalogs such as http://www.re3data.org/ (i.e., REgistry of REsearch data REpositories) and http://www.biosharing.org (i.e., BioSharing). It is often considered good practice to deposit code in a host repository like GitHub that specializes in source code management as well as some types of data like large files and tabular data (see https://github.com/ ). Make note of any repository-specific policies (e.g., data privacy and security, requirements to submit associated code) and costs for data submission, curation, and backup that should be included in the DMP and the proposal budget.
Rule 7: Define the Project’s Data Policies
Despite what may be a natural proclivity to avoid policy and legal matters, researchers cannot afford to do so when it comes to data. Research sponsors, institutions that host research, and scientists all have a role in and obligation for promoting responsible and ethical behavior. Consequently, many research sponsors require that DMPs include explicit policy statements about how data will be managed and shared. Such policies include:
- licensing or sharing arrangements that pertain to the use of preexisting materials;
- plans for retaining, licensing, sharing, and embargoing (i.e., limiting use by others for a period of time) data, code, and other materials; and
- legal and ethical restrictions on access and use of human subject and other sensitive data.
Unfortunately, policies and laws often appear or are, in fact, confusing or contradictory. Furthermore, policies that apply within a single organization or in a given country may not apply elsewhere. When in doubt, consult your institution’s office of sponsored research, the relevant Institutional Review Board, or the program officer(s) assigned to the program to which you are applying for support.
Despite these caveats, it is usually possible to develop a sound policy by following a few simple steps. First, if preexisting materials, such as data and code, are being used, identify and include a description of the relevant licensing and sharing arrangements in your DMP. Explain how third party software or libraries are used in the creation and release of new software. Note that proprietary and intellectual property rights (IPR) laws and export control regulations may limit the extent to which code and software can be shared.
Second, explain how and when the data and other research products will be made available. Be sure to explain any embargo periods or delays such as publication or patent reasons. A common practice is to make data broadly available at the time of publication, or in the case of graduate students, at the time the graduate degree is awarded. Whenever possible, apply standard rights waivers or licenses, such as those established by Open Data Commons (ODC) and Creative Commons (CC), that guide subsequent use of data and other intellectual products (see http://creativecommons.org/ and http://opendatacommons.org/licenses/pddl/summary/ ). The CC0 license and the ODC Public Domain Dedication and License, for example, promote unrestricted sharing and data use. Nonstandard licenses and waivers can be a significant barrier to reuse.
Third, explain how human subject and other sensitive data will be treated (e.g., see http://privacyruleandresearch.nih.gov/ for information pertaining to human health research regulations set forth in the US Health Insurance Portability and Accountability Act). Many research sponsors require that investigators engaged in human subject research approaches seek or receive prior approval from the appropriate Institutional Review Board before a grant proposal is submitted and, certainly, receive approval before the actual research is undertaken. Approvals may require that informed consent be granted, that data are anonymized, or that use is restricted in some fashion.
Rule 8: Describe How the Data Will Be Disseminated
The best-laid preservation plans and data sharing policies do not necessarily mean that a project’s data will see the light of day. Reviewers and research sponsors will be reassured that this will not be the case if you have spelled out how and when the data products will be disseminated to others, especially people outside your research group. There are passive and active ways to disseminate data. Passive approaches include posting data on a project or personal website or mailing or emailing data upon request, although the latter can be problematic when dealing with large data and bandwidth constraints. More active, robust, and preferred approaches include: (1) publishing the data in an open repository or archive (see Rule 6 ); (2) submitting the data (or subsets thereof) as appendices or supplements to journal articles, such as is commonly done with the PLOS family of journals; and (3) publishing the data, metadata, and relevant code as a “data paper” [ 5 ]. Data papers can be published in various journals, including Scientific Data (from Nature Publishing Group), the GeoScience Data Journal (a Wiley publication on behalf of the Royal Meteorological Society), and GigaScience (a joint BioMed Central and Springer publication that supports big data from many biology and life science disciplines).
A good dissemination plan includes a few concise statements. State when, how, and what data products will be made available. Generally, making data available to the greatest extent and with the fewest possible restrictions at the time of publication or project completion is encouraged. The more proactive approaches described above are greatly preferred over mailing or emailing data and will likely save significant time and money in the long run, as the data curation and sharing will be supported by the appropriate journals and repositories or archives. Furthermore, many journals and repositories provide guidelines and mechanisms for how others can appropriately cite your data, including digital object identifiers, and recommended citation formats; this helps ensure that you receive credit for the data products you create. Keep in mind that the data will be more usable and interpretable by you and others if the data are disseminated using standard, nonproprietary approaches and if the data are accompanied by metadata and associated code that is used for data processing.
Rule 9: Assign Roles and Responsibilities
A comprehensive DMP clearly articulates the roles and responsibilities of every named individual and organization associated with the project. Roles may include data collection, data entry, QA/QC, metadata creation and management, backup, data preparation and submission to an archive, and systems administration. Consider time allocations and levels of expertise needed by staff. For small to medium size projects, a single student or postdoctoral associate who is collecting and processing the data may easily assume most or all of the data management tasks. In contrast, large, multi-investigator projects may benefit from having a dedicated staff person(s) assigned to data management.
Treat your DMP as a living document and revisit it frequently (e.g., quarterly basis). Assign a project team member to revise the plan, reflecting any new changes in protocols and policies. It is good practice to track any changes in a revision history that lists the dates that any changes were made to the plan along with the details about those changes, including who made them.
Reviewers and sponsors may be especially interested in knowing how adherence to the data management plan will be assessed and demonstrated, as well as how, and by whom, data will be managed and made available after the project concludes. With respect to the latter, it is often sufficient to include a pointer to the policies and procedures that are followed by the repository where you plan to deposit your data. Be sure to note any contributions by nonproject staff, such as any repository, systems administration, backup, training, or high-performance computing support provided by your institution.
Rule 10: Prepare a Realistic Budget
Creating, managing, publishing, and sharing high-quality data is as much a part of the 21st century research enterprise as is publishing the results. Data management is not new—rather, it is something that all researchers already do. Nonetheless, a common mistake in developing a DMP is forgetting to budget for the activities. Data management takes time and costs money in terms of software, hardware, and personnel. Review your plan and make sure that there are lines in the budget to support the people that manage the data (see Rule 9 ) as well as pay for the requisite hardware, software, and services. Check with the preferred data repository (see Rule 6 ) so that requisite fees and services are budgeted appropriately. As space allows, facilitate reviewers by pointing to specific lines or sections in the budget and budget justification pages. Experienced reviewers will be on the lookout for unfunded components, but they will also recognize that greater or lesser investments in data management depend upon the nature of the research and the types of data.
A data management plan should provide you and others with an easy-to-follow road map that will guide and explain how data are treated throughout the life of the project and after the project is completed. The ten simple rules presented here are designed to aid you in writing a good plan that is logical and comprehensive, that will pass muster with reviewers and research sponsors, and that you can put into practice should your project be funded. A DMP provides a vehicle for conveying information to and setting expectations for your project team during both the proposal and project planning stages, as well as during project team meetings later, when the project is underway. That said, no plan is perfect. Plans do become better through use. The best plans are “living documents” that are periodically reviewed and revised as necessary according to needs and any changes in protocols (e.g., metadata, QA/QC, storage), policy, technology, and staff, as well as reused, in that the most successful parts of the plan are incorporated into subsequent projects. A public, machine-readable, and openly licensed DMP is much more likely to be incorporated into future projects and to have higher impact; such increased transparency in the research funding process (e.g., publication of proposals and DMPs) can assist researchers and sponsors in discovering data and potential collaborators, educating about data management, and monitoring policy compliance [ 6 ].
Acknowledgments
This article is the outcome of a series of training workshops provided for new faculty, postdoctoral associates, and graduate students.
- View Article
- PubMed/NCBI
- Google Scholar
Thank you for visiting nature.com. You are using a browser version with limited support for CSS. To obtain the best experience, we recommend you use a more up to date browser (or turn off compatibility mode in Internet Explorer). In the meantime, to ensure continued support, we are displaying the site without styles and JavaScript.
- View all journals
- Explore content
- About the journal
- Publish with us
- Sign up for alerts
- CAREER FEATURE
- 13 March 2018
Data management made simple
- Quirin Schiermeier
You can also search for this author in PubMed Google Scholar
When Marjorie Etique learnt that she had to create a data-management plan for her next research project, she was not sure exactly what to do.
Access options
Access Nature and 54 other Nature Portfolio journals
Get Nature+, our best-value online-access subscription
24,99 € / 30 days
cancel any time
Subscribe to this journal
Receive 51 print issues and online access
185,98 € per year
only 3,65 € per issue
Rent or buy this article
Prices vary by article type
Prices may be subject to local taxes which are calculated during checkout
Nature 555 , 403-405 (2018)
doi: https://doi.org/10.1038/d41586-018-03071-1
See Editorial: Everyone needs a data-management plan
Related Articles
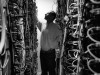
The FAIR Guiding Principles for scientific data management and stewardship
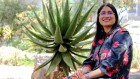
Overcoming low vision to prove my abilities under pressure
Career Q&A 28 MAR 24
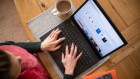
How a spreadsheet helped me to land my dream job
Career Column 28 MAR 24
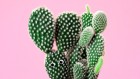
Maple-scented cacti and pom-pom cats: how pranking at work can lift lab spirits
Career Feature 27 MAR 24
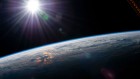
Divisive Sun-dimming study at Harvard cancelled: what’s next?
News Explainer 27 MAR 24
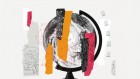
How AI is improving climate forecasts
News Feature 26 MAR 24
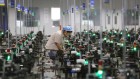
China’s medical-device industry gets a makeover
Spotlight 20 MAR 24
ECUST Seeking Global Talents
Join Us and Create a Bright Future Together!
Shanghai, China
East China University of Science and Technology (ECUST)
World-Class Leaders for Research in Materials Science
National Institute for Materials Science (NIMS, Japan) calls for outstanding researchers who can drive world-class research in materials science.
Tsukuba, Japan (JP)
National Institute for Materials Science
Professor of Experimental Parasitology (Leishmania)
To develop an innovative and internationally competitive research program, to contribute to educational activities and to provide expert advice.
Belgium (BE)
Institute of Tropical Medicine
PhD Candidate (m/f/d)
We search the candidate for the subproject "P2: targeting cardiac macrophages" as part of the DFG-funded Research Training Group "GRK 2989: Targeti...
Dortmund, Nordrhein-Westfalen (DE)
Leibniz-Institut für Analytische Wissenschaften – ISAS – e.V.
At our location in Dortmund we invite applications for a DFG-funded project. This project will aim to structurally and spatially resolve the altere...
Sign up for the Nature Briefing newsletter — what matters in science, free to your inbox daily.
Quick links
- Explore articles by subject
- Guide to authors
- Editorial policies

Library Services
Writing a Data Management Plan

When developing a project or applying for funding you are likely to need a Data Management Plan.
What are research data at UCL?
According to the UCL Research Data policy , data are: “facts, observations or experiences on which an argument or theory is constructed or tested. Data may be numerical, descriptive, aural or visual. Data may be raw, abstracted or analysed, experimental or observational. Data include but are not limited to: laboratory notebooks; field notebooks; questionnaires; texts; audio files; video files; models; photographs; test responses”.
Three kinds of research data
There are three kinds of research data:
- open - data which are freely available online;
- controlled - data access is restricted on the basis of there being ethical, legal and/or commercial reasons prohitbiting their open release. Potential secondary users must meet certain criteria before access is given;
- closed - data which are permanently embargoed due to their nature.
What is a Data Management Plan?
A Data Management Plan (DMP) describes your data management and sharing activities. It is generally 1-3 pages in length and should cover the four phases of the research data lifecycle:
- Planning and preparing for your research project;
- Actively researching;
- Archiving, preserving and curating;
- Discovery, access and sharing.
If you are, or plan to be, in receipt of external funding, check your funder's policies and requirements when writing your DMP.
A range of how-to guides have been created categorised according to the phase of the resesearch data lifecycle they cover. For research domain-specific support, guides are also available.
Download the UCL Data Management Plan Template here:
ucl_dmp_template_.docx

Why are Data Management Plans useful?
In addition to often being a prerequisite to receiving certain grants, DMPs are useful for:
- maximising the research potential of existing research outputs by reusing and repurposing them
- thinking about and developing your strategy for issues such as data storage and long-term preservation , handling of sensitive data , data retention and sharing , early on in your research.
- anticipating legal, ethical and commercial exceptions to releasing data ; deciding who can have access to data in the short and long term.
- estimating the costs of your research project , which can then be included in your project budget.
Before you get started
Here are a few tips to help you start writing a DMP:
- Verify which data management and data sharing policies apply - these could be institutional, funder or journal publisher-led.
- Identify whether you will need to enter into a data sharing agreement before datasets and other study materials may be shared. There could also be legal frameworks and copyright issues to be mindful of. There is more information about material transfer agreements .
- Where research involves living human participants, it is recommended you speak with the Data Protection team to confirm which data protection legislation apply. Where you are collaborating with partners based globally, confirm whether international data protection legislation apply to your research.
- Verify submission deadlines.
DMP Training and Review Service
The RDM team offers both face-to-face and online training courses on how to write a data management plan. Using the UCL DMP template, attendees have the opportnity to write a data management plan which they can take away with them and use as a basis for a more detailed plan of their data management and sharing activities.
For more help and advice, contact your Research Data Support Officers who can also review drafted UCL Data Management Plans if you send them in advance of submission (allow 1 to 2 weeks at least before your submission deadline).
UCL Research Data policy
The UCL Research Data policy describes UCL's expectations relating to data management and sharing within the wider Open Science context.
DMPonline , a free tool created by the DCC, provides a framework for creating your Data Management Plan. UCL guidance is now incorporated into DMPonline; see our further guidance on using the tool.
The Sheridan Libraries
- Data Management and Sharing
- Sheridan Libraries
Write a Data Management and Sharing Plan
- Write A Proposal
NIH DMSP guide
Find funder requirements for data sharing, data management plan components, what are research data, fair principles, allowable costs for data management and sharing, more resources for writing data management plans.
- Human Participant Research Considerations
- JHU Policies for Data Management and Sharing
- Access and Collect Data
- Manage Data
- Analyze and Visualize Data
- Document Data
- Store and Preserve Data
- Overview of Data Sharing
- Prepare Your Data For Sharing
- How to Find a Data Repository
- Share Code or Software
Conditions for Access and Reuse
U.S. Federal funders, and many private funders, require making data associated with grants available for further research. Data are shared through public online data repositories when possible or with restricted access. Grant proposals may require data management plans (or Data Management and Sharing plans) that describe how the proposal will meet those requirements. JHU Data Services provides resources and consultation on writing data management plans using the DMPTool . This section provides an overview of plan components and resources for most funder requirements.
If writing an NIH Data Management and Data Sharing plan, visit this guide for direct guidance. As of January 2023, NIH requires data sharing plans for most funding opportunities.
Funders Data-related Mandates and Public Access Plans
Most US public funders and many private funders require data management and sharing plans for funded projects. These links provide databases of data-related requirements and public access policies for U.S. and many international and private funders. Also listed are direct links to a few major funders.
- DMPTool : up-to-date requirements with funder-specific templates. (See Guidelines within templates for links to policies)
- FAIRsharing.org : searchable database includes many international and private funder requirements, in addition to U.S. funders.
- Sherpa Juliet : database with U.S., international and private funder requirements. May be less frequently updated.
- National Institutes of Health (NIH) Policy for Data Management and Sharing
- National Science Foundation (NSF) Data Management Plan requirements
- United States Agency for International Development (USAID) Data Policy
- Gates Foundation Open Data Policy
We recommend the DMPTool for writing plans from funder-specific templates, with associated guidance and examples. JHU users can log in with their JHU emails and credentials. JHU Data Service will provide direct feedback on drafts that can be sent within the DMPTool or directly to [email protected] for feedback.

Here is an overview of typical components of a proposal data management plan for U.S. funders. The elements described in this section includes links within this guide and external resources for more details and guidance.
Funders, generally, look for the following in a DMP:
- What type of data will be produced?
- What are the standards of organization and metadata for documenting data?
- How will privacy, security, confidentiality and intellectual property be protected?
- How will data be accessed and shared to allow others to use it?
- How will data be archived and preserved and for how long?
Data Description
Consider listing all the products of research, both "raw" and processed data used to support results. All types require management during the project. The list could include sources, file types, format and size. Also indicate which data will be made accessible.
Data Sharing
Indicate which research products will be shared, ideally indicating the value to a range of research communities. Sharing policies prefer unmediated distribution through an online repository or database. Include where the data will be accessed and when it is available, such as accompanying publications. More guidance on data sharing
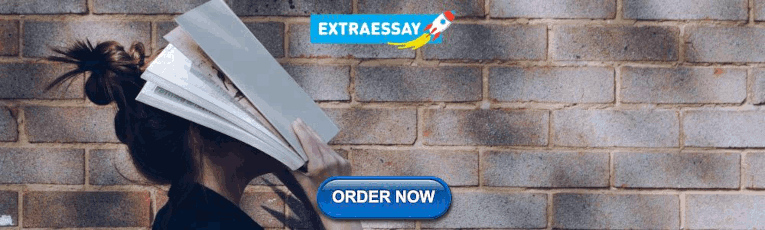
Documentation and Metadata
Shared data should be accompanied by sufficient documentation to be understood and ideally reused. Guidelines prefer use of metadata standards of one's research community, such as accepted descriptors of common data elements. Formatting that facilitates machine readability is ideal. More guidance on documentation
Plans should indicate any requirements for those accessing data, such as citing datasets, and restrictions on use such as intellectual property or proprietary data that might limit what is shared. Plans should also indicate privacy conditions, whether data will be de-identified or require restricted access through an approval process such as IRB reviews. More guidance on usage conditions
Storage and Preservation
Many plans ask for brief details on how data will be stored, especially data requiring high-capacity storage, special collaborative access, or security such as JHU's SAFE Desktop secure data enclave. Also indicate which data will be preserved and for how long after the grant period. Some plans ask who will be responsible for preservation and long-term access to shared data. More guidance on storage and preservation
Researchers often ask what constitutes their data. Johns Hopkins University defines research data “records that would be used for the reconstruction and evaluation of reported or otherwise published results” in the policy on access and retention of research data and materials . Examples include laboratory notebooks, numerical raw experimental results and instrumental outputs.
The FAIR Guiding Principles for scientific data management and stewardship, published in 2016 , outlined methods for broadening access to shared data, focusing particularly on better discovery and open access through data repositories, and better reuse through documentation and machine-readable metadata standards. FAIR Principles fit within the wider promotion of Open Science and reproducible research. Data sharing policies by funders often cite these principles as a goal for making publicly funded data more widely available.
- FAIR Principles : overview provided by the GO FAIR Initiative
- FAIR Sharing Standards : A registry of terminology artefacts, models/formats, reporting guidelines, and identifier schemas.
- FAIR Data Repositories & Knowledgebases: A registry of knowledgebases and repositories of data and other digital assets
- FAIRsharing.org Data Policies database : A registry of data preservation, management and sharing policies from international funding agencies, regulators, journals, and other organisations.
- CARE Principles for Indigenous Data Governance : discussing special considerations for sharing data from indigenous populations
- FASEB Science Policy and Advocacy : Federation of American Societies for Experimental Biology's collection of policy statements and best practices regarding data management and sharing, including the DataWorks! initiative promoting data sharing and exemplary data management plans.
See also, JHU Data Services Online training on Open Science and the Open Access Guide by the Sheridan Libraries
Most US funders allow certain costs for data management and sharing to be included in grant budgets. It can be challenging to estimate costs at the time of proposal. For example, a plan might requires annual funding of repository fees for 10 years on a 5 year grant. Anonymizing data for public access might require hiring a statistician. JHU's Research Administration offices can advise on some of these costs. Funder program officers should also be aware of allowable costs. JHU Data Services can help investigate costs associated with data repositories. Here are additional resources from funders and others:
National Science Foundation (NSF):
Proposal & Award Policies & Procedures Guide Policy of allowable costs. NSF allows certain data management costs, such as covering fees deposits of data ( see FAQ ) but program officers may need to advise on applicable categories for budgeting.
National Institutes of Health (NIH):
- Summary on NIH's Data Sharing site: Budgeting for Data Management and Sharing
- Supplemental Information to the NIH Policy for Data Management and Sharing: Allowable Costs for Data Management and Sharing :
- NIH Grants Policy Statement on Allowable and Unallowable costs
- Costing guidance from COGR's NIH Data Sharing Readiness Guide (coming soon)
- NIMH NDA cost estimator
An infographic from USC Department of Budget and Grants listing a range of costs for data management and sharing.
Examples of Data Management Plans
- DMPTool's ongoing collection of publicly shared data management plans that can be filtered by funder, institution and subject
- DMP examples from University of Arizona
- Sample NIH Data Management and Sharing plans for Clinical, Secondary, and Genomic research from NIHM
- Examples DMPs on GitHub
NIH Scientific Data Sharing
NIH's website for the policy, guidance, and resources for data management and sharing.
Grant Reviewer’s Guide
A user-friendly page of tables and checklists that reviewers or writers of plans can use to quickly evaluate data management plans. More information about the worksheet can be found here .
JHU Data Services Online Training: Preparing a Data Management Plan
This one-hour online training course contains 10 mini-modules, created by JHU Data Services.
ICPSR's Framework for Creating a Data Management Plan
- << Previous: Write A Proposal
- Next: Human Participant Research Considerations >>
- Last Updated: Mar 8, 2024 10:57 AM
- URL: https://guides.library.jhu.edu/dataservices

An official website of the United States government
Here's how you know
Official websites use .gov A .gov website belongs to an official government organization in the United States.
Secure .gov websites use HTTPS A lock ( ) or https:// means you’ve safely connected to the .gov website. Share sensitive information only on official, secure websites.
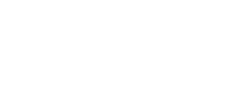
- Digg
Latest Earthquakes | Chat Share Social Media
Data Management Plans
Planning for a project involves making decisions about data resources and potential products. A Data Management Plan (DMP) describes data that will be acquired or produced during research; how the data will be managed, described, and stored, what standards you will use, and how data will be handled and protected during and after the completion of the project.
Data Management Plan Checklist
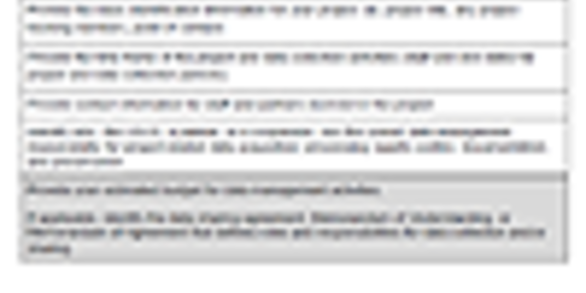
This USGS checklist provides guidance in what must be considered in developing a DMP for all new USGS projects.
Science Center Data Management Strategy
To help standardize or provide guidance on DMPs, a science center or funding source may choose to document their own Data Management strategy. Click the link below for a template for developing a Science Center Data Management Strategy.
Table of Contents
This page is a guide to help you develop a DMP. Find answers to frequently asked questions, checkout templates and DMP examples, learn about tools for creating DMPs, and understand USGS DMP requirements.
- Getting started
- Frequently Asked Questions
- Templates and Examples
Reviewing Data Management Plans
Related training modules.
- What the U.S. Geological Survey Manual Requires
Getting Started
The resources in this section will help you understand how to develop your DMP. The checklist outlines the minimum USGS requirements. The FAQ and DMP Writing Best Practices list below will help you understand other important considerations when developing your own DMP. To help standardize or provide guidance on DMPs, a science center or funding source may choose to document their own Data Management strategy. Click here for a template for developing a Science Center Data Management Strategy [DOCX] .
DMP Writing Best Practices
Create a DMP prior to initiating research as required by USGS policy.
Consider available DMP tools and templates, along with their intended use.
Write DMP content that is descriptive of the project's data acquisition, processing, analysis, preservation, publishing, and sharing (public access) of products as described by the USGS Science Data Lifecycle.
Identify any proprietary or sensitive data in the DMP prior to data acquisition or collection to legally justify the need to withhold them from public access if necessary.
Define roles and responsibilities for management, distribution and ownership of data and subsequent metadata or, if available, reference existing Memoranda of Understanding, Memoranda of Agreement, and/or Data Sharing agreements.
Add content to supplement a DMP template provided by a funding source if that template does not allow you to fully describe your project, data assets, and products and the required investments needed for any software (developed or purchased) and any hardware that are needed to support the research.
Establish a schedule for reviewing and updating a DMP in combination with project events such as funding approval, project review, and publication.
Ensure DMP content contains a level of detail that enables stakeholders (funders, project staff, and repository managers) to understand the reality of the project activities.
Ensure that DMP content and outlined procedures reflect USGS Fundamental Science Practices (FSP) requirements and Science Center guidance.
Frequently Asked Questions
The following FAQ's were developed to extend the information provided by the USGS Fundamental Science Practices DMP FAQ Page . This list also presents exemplary solutions from USGS science centers that are currently in practice.
Note: Always refer to specific guidance that may be provided by your funding source or science center to understand their requirements first and foremost.
Business practices that affect project workflows vary among science centers and funding sources; however, in general terms, DMP creation should occur between the proposal stage and accepted funding stage of the project. SM 502.6 requires "The project work plan (SM 502.2) for every research project funded or managed by the USGS must include a data management plan prior to initiation of the project." Below are example project workflow diagrams showing when a DMP is required to be completed; however, you should use the workflow established by your center or program, if applicable. The DMP may need to be updated at various other project milestones. WARC Example Alaska Science Center Example
A DMP developed to meet the requirements of a funding source is usually acceptable if it captures, at a minimum, the same information as the science center format. Deficiencies should be addressed as an addendum to the funding source DMP.
There are numerous users of a DMP. The author uses the DMP to plan how data will be handled throughout its lifecycle, updating the document throughout the project. Additionally, the author uses a DMP to capture and record relevant information in a timely manner that can be used later on for other requirements such as metadata. Project staff use the DMP to help understand roles and responsibilities of various team members, especially in teams involving partners from different organizations. Data managers and communication teams can use the information to ensure that preservation and data sharing activities are done appropriately.
Funding sources can use DMPs to promote transparent, high quality, and discoverable products. Lastly, in the event of a Freedom of Information Act (FOIA) request, your FOIA officer can use the DMP as substantiating material. The DMP, considered part of a formally agreed upon project work plan, legally establishes who is responsible for providing free public access to the data and what data are proprietary if they are used by the USGS.
You may need to develop your DMP throughout your project to maintain accurate and useful content. Understanding the USGS Science Data Lifecycle will help you develop DMP content; however, specific guidance may also be provided by your funding source or science center.
A Single Document with Color Coding The National Regional Climate Adaptation Science Centers template uses a color coded approach within a single document. Fields shaded gray are not required for proposals. If a project is funded, all fields are required.
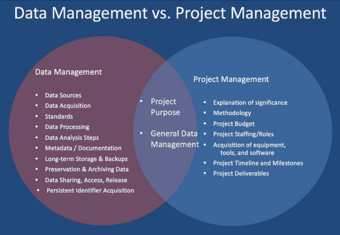
DMPs are focused on the data-related aspects of the project and work together with other descriptive project documents such as a proposal, project plan, or BASIS+ entry. Often DMPs contain planning, roles and responsibilities sections that collect similar information to that found in other documents, but this "duplicate" content is necessary for anyone outside of your project to understand your DMP.
How can I manage all of my project and data documentation including a DMP?
There are many ways to organize and store DMP files. It's most important that you simply develop a consistent strategy. Organization and naming conventions can be associated with other useful elements of a project such as project IDs, project stages, fiscal year, or any combination. Storage options to consider include databases, single files, or folders of content. Online data management and documentation tools can also affect the management of your documents. You may choose to create content or use forms that can be loaded and stored in the software tool.
The Great Lakes Science Center and the Northern Rocky Mountain Science Center (NOROCK) are two examples of centers that conceptualize project documentation as a bundle, where a project folder comprises many documents and forms that describe the project and data. The bundle includes documents such as a Study Plan, a DMP, and a metadata questionnaire. NOROCK additionally uses SharePoint to house research documentation (proposals, Project Work Plans, DMPs, etc.). Document sets have managed metadata. Automated workflows help to streamline the review and approval process, as well as facilitate records management.
Templates and Examples
Below is a selection of DMP templates provided by USGS science centers and programs. Each template was designed with specific needs and use cases in mind. When developing your own or choosing an existing DMP template consider your own project needs.
* Some DMPs listed below are for educational purposes only and are subject to change. Please contact your USGS center or program for more information on their specific DMP requirements and process.
USGS Powell Center
- Proposal evaluation
- Understand data and IT support needs
- Download Data Management Plan [DOCX] (Template)
National and Regional Climate Adaptation Science Centers
- Share and manage data and information products
- DMP Template [DOCX] (Template)
- Data Input Existing Collection [PDF] (Example)
- Biochar Cost-Benefit assessment tool [PDF] (Example)
USGS California Water Science Center
- Example Microsoft questionnaire form
- Coordination needed with center data manager team to develop actual DMP
- Example Form [PDF] * (Template)
USGS Wetlands and Aquatic Research Center
- Example google questionnaire form
- Bathythermograph [PDF] ( Note: This example is not a DMP from this center )
USGS Coastal and Marine Geology Program
- Example database form
USGS Fort Collins Science Center
- Example template
- Example Template [PDF] * (Template)
USGS Water Mission Area
- Excel-based Data Management Planning tool (DMTool)
- Example template maintained by the Office of Quality Assurance
- Template is used by the WMA. Template should be used by researchers in Water Science Centers if the Center does not have a DMP template containing the minimum elements outlined in Survey Manual Fundamental Science Practices ( SM 502.6, Section 4 ) and on this page.
Tools
Below is a selection of tools available to USGS staff. Each tool was designed with specific needs and use cases in mind.
- DMP Tool - https://dmptool.org/
- DMPEditor - https://my.usgs.gov/dmpeditor/
- ezDMP - https://ezdmp.org (for writing NSF DMPs)
- Microsoft Word Templates - See CASC template above as an example
- Microsoft Forms - See California Water Science Center and Wetland and Aquatic Research Center templates as examples
An important aspect of data management planning is having someone knowledgeable about data management and USGS policies review a project's DMP to flag any potential oversights or challenges before they become an issue. The USGS Data Management Working Group has developed a USGS Data Management Plan Review Checklist to help facilitate these types of reviews.
- Planning for Data Management Part 2: Using the DMPTool to Create Data Management Plans
What the U.S. Geological Survey Manual Requires:
Effective October 1, 2016 the USGS Survey Manual chapter SM 502.6 - Fundamental Science Practices: Scientific Data Management Foundation , requires the project work plan ( SM 502.2 ) for every research project funded or managed by the USGS must include a data management plan prior to initiation of the project.
SM 502.6 further specifies, a data management plan will include standards and intended actions as appropriate to the project for acquiring, processing, analyzing, preserving, publishing/sharing, describing, and managing the quality of, backing up, and securing the data holdings.
For more information about data management planning as it pertains to the USGS policy, visit the Fundamental Science Practices FAQs: Data Management Planning .
References
- Chatfield, T., Selbach, R. February, 2011. Data Management for Data Stewards. Data Management Training Workshop. Bureau of Land Management (BLM).
- DataONE Data Management Skillbuilding Hub .
- Digital Curation Centre. Checklist for a Data Management Plan .
- UK Data Archive. Data Management Costing Tool and Checklist .
- Inter-university Consortium for Political and Social Research (ICPSR). Guide to Social Science Data Preparation and Archiving. [PDF]
- UK Data Archive. Plan to Share.
Page last updated 6/21/21.

- RIT Libraries
- Data Analytics Resources
- Writing a Research Proposal
- Electronic Books
- Print Books
- Data Science: Journals
- More Journals, Websites
- Alerts, IDS Express
- Readings on Data
- Sources with Data
- Google Scholar Library Links
- Zotero-Citation Management Tool
- Writing a Literature Review
- ProQuest Research Companion
- Thesis Submission Instructions
- Associations
Writing a Rsearch Proposal
A research proposal describes what you will investigate, why it’s important, and how you will conduct your research. Your paper should include the topic, research question and hypothesis, methods, predictions, and results (if not actual, then projected).
Research Proposal Aims
The format of a research proposal varies between fields, but most proposals will contain at least these elements:
- Introduction
Literature review
- Research design
Reference list
While the sections may vary, the overall objective is always the same. A research proposal serves as a blueprint and guide for your research plan, helping you get organized and feel confident in the path forward you choose to take.
Proposal Format
The proposal will usually have a title page that includes:
- The proposed title of your project
- Your supervisor’s name
- Your institution and department
Introduction The first part of your proposal is the initial pitch for your project. Make sure it succinctly explains what you want to do and why.. Your introduction should:
- Introduce your topic
- Give necessary background and context
- Outline your problem statement and research questions To guide your introduction , include information about:
- Who could have an interest in the topic (e.g., scientists, policymakers)
- How much is already known about the topic
- What is missing from this current knowledge
- What new insights will your research contribute
- Why you believe this research is worth doing
As you get started, it’s important to demonstrate that you’re familiar with the most important research on your topic. A strong literature review shows your reader that your project has a solid foundation in existing knowledge or theory. It also shows that you’re not simply repeating what other people have done or said, but rather using existing research as a jumping-off point for your own.
In this section, share exactly how your project will contribute to ongoing conversations in the field by:
- Comparing and contrasting the main theories, methods, and debates
- Examining the strengths and weaknesses of different approaches
- Explaining how will you build on, challenge, or synthesize prior scholarship
Research design and methods
Following the literature review, restate your main objectives . This brings the focus back to your project. Next, your research design or methodology section will describe your overall approach, and the practical steps you will take to answer your research questions. Write up your projected, if not actual, results.
Contribution to knowledge
To finish your proposal on a strong note, explore the potential implications of your research for your field. Emphasize again what you aim to contribute and why it matters.
For example, your results might have implications for:
- Improving best practices
- Informing policymaking decisions
- Strengthening a theory or model
- Challenging popular or scientific beliefs
- Creating a basis for future research
Lastly, your research proposal must include correct citations for every source you have used, compiled in a reference list . To create citations quickly and easily, you can use free APA citation generators like BibGuru. Databases have a citation button you can click on to see your citation. Sometimes you have to re-format it as the citations may have mistakes.
- << Previous: ProQuest Research Companion
- Next: DIR >>
Edit this Guide
Log into Dashboard
Use of RIT resources is reserved for current RIT students, faculty and staff for academic and teaching purposes only. Please contact your librarian with any questions.

Help is Available

Email a Librarian
A librarian is available by e-mail at [email protected]
Meet with a Librarian
Call reference desk voicemail.
A librarian is available by phone at (585) 475-2563 or on Skype at llll
Or, call (585) 475-2563 to leave a voicemail with the reference desk during normal business hours .
Chat with a Librarian
Data analytics resources infoguide url.
https://infoguides.rit.edu/DA
Use the box below to email yourself a link to this guide
An official website of the United States government
Here's how you know
Official websites use .gov A .gov website belongs to an official government organization in the United States.
Secure .gov websites use HTTPS. A lock ( Lock Locked padlock ) or https:// means you've safely connected to the .gov website. Share sensitive information only on official, secure websites.
Preparing Your Data Management Plan
The two-page data management plan is a required part of a proposal to the U.S. National Science Foundation. It describes how a proposal will follow NSF policy on managing, disseminating and sharing research results.
This page provides an overview of requirements for the data management plan. See the Proposal and Award Policies and Procedures Guide (PAPPG) XI.D.4 for full guidance and for NSF's data sharing policy.
On this page
Nsf's data sharing policy.
NSF-funded investigators are expected to share with other researchers, at no more than incremental cost and within a reasonable time, the primary data, samples, physical collections and other supporting materials created or gathered in the course of work under NSF awards.
General guidance
General guidelines for data management plans are explained in PAPPG II.D.2(ii) .
Content that may be included under the general guidelines is as follows:
- The types of data, samples, physical collections, software, curriculum materials and other materials to be produced in the course of the project.
- The standards to be used for data and metadata format and content. In cases where existing standards are absent or deemed inadequate, this should be documented along with any proposed solutions or remedies.
- Policies for data access and sharing, including provisions for appropriate protection of privacy, confidentiality, security, intellectual property or other rights or requirements.
- Policies and provisions for data reuse, redistribution and the production of derivatives.
- Plans for archiving data, samples and other research products, and for preserving access to them.
If your proposed project will not produce data, you must include a document justifying this in place of the data management plan.
Directorate and/or division guidance
Links to data management requirements and plans relevant to specific NSF directorates, offices, divisions or programs are provided below. If guidance specific to a directorate, division or program is not provided, follow the general requirements detailed in PAPPG II.D.2(ii) :
- Biological Sciences (BIO)
- Engineering (ENG)
- Geosciences (GEO)
- Division of Astronomical Sciences (AST)
- Division of Chemistry (CHE)
- Division of Materials Research (DMR) | DMR template
- Division of Mathematical Sciences (DMS)
- Division of Physics (PHY)
- Office of Polar Programs (OPP)
- Social, Behavioral and Economic Sciences (SBE)
- STEM Education (EDU)
Program-specific guidance:
- Designing Materials to Revolutionize and Engineer our Future (DMREF)
Individual program officers can offer additional information, especially for program solicitations with specific guidance. You may also consult the Public Access FAQ .
Examples of data management plans
These examples of data management plans (DMPs) were provided by University of Minnesota researchers. They feature different elements. One is concise and the other is detailed. One utilizes secondary data, while the other collects primary data. Both have explicit plans for how the data is handled through the life cycle of the project.
School of Public Health featuring data use agreements and secondary data analysis
All data to be used in the proposed study will be obtained from XXXXXX; only completely de-identified data will be obtained. No new data collection is planned. The pre-analysis data obtained from the XXX should be requested from the XXX directly. Below is the contact information provided with the funding opportunity announcement (PAR_XXX).
Types of data : Appendix # contains the specific variable list that will be used in the proposed study. The data specification including the size, file format, number of files, data dictionary and codebook will be documented upon receipt of the data from the XXX. Any newly created variables from the process of data management and analyses will be updated to the data specification.
Data use for others : The post-analysis data may be useful for researchers who plan to conduct a study in WTC related injuries and personal economic status and quality of life change. The Injury Exposure Index that will be created from this project will also be useful for causal analysis between WTC exposure and injuries among WTC general responders.
Data limitations for secondary use : While the data involve human subjects, only completely de-identified data will be available and used in the proposed study. Secondary data use is not expected to be limited, given the permission obtained to use the data from the XXX, through the data use agreement (Appendix #).
Data preparation for transformations, preservation and sharing : The pre-analysis data will be delivered in Stata format. The post-analysis data will also be stored in Stata format. If requested, other data formats, including comma-separated-values (CSV), Excel, SAS, R, and SPSS can be transformed.
Metadata documentation : The Data Use Log will document all data-related activities. The proposed study investigators will have access to a highly secured network drive controlled by the University of Minnesota that requires logging of any data use. For specific data management activities, Stata “log” function will record all activities and store in relevant designated folders. Standard file naming convention will be used with a format: “WTCINJ_[six letter of data indication]_mmddyy_[initial of personnel]”.
Data sharing agreement : Data sharing will require two steps of permission. 1) data use agreement from the XXXXXX for pre-analysis data use, and 2) data use agreement from the Principal Investigator, Dr. XXX XXX ([email protected] and 612-xxx-xxxx) for post-analysis data use.
Data repository/sharing/archiving : A long-term data sharing and preservation plan will be used to store and make publicly accessible the data beyond the life of the project. The data will be deposited into the Data Repository for the University of Minnesota (DRUM), http://hdl.handle.net/11299/166578. This University Libraries’ hosted institutional data repository is an open access platform for dissemination and archiving of university research data. Date files in DRUM are written to an Isilon storage system with two copies, one local to each of the two geographically separated University of Minnesota Data Centers. The local Isilon cluster stores the data in such a way that the data can survive the loss of any two disks or any one node of the cluster. Within two hours of the initial write, data replication to the 2nd Isilon cluster commences. The 2nd cluster employs the same protections as the local cluster, and both verify with a checksum procedure that data has not altered on write. In addition, DRUM provides long-term preservation of digital data files for at least 10 years using services such as migration (limited format types), secure backup, bit-level checksums, and maintains a persistent DOIs for data sets, facilitating data citations. In accordance to DRUM policies, the de-identified data will be accompanied by the appropriate documentation, metadata, and code to facilitate reuse and provide the potential for interoperability with similar data sets.
Expected timeline : Preparation for data sharing will begin with completion of planned publications and anticipated data release date will be six months prior.
Back to top
College of Education and Human Development featuring quantitative and qualitative data
Types of data to be collected and shared The following quantitative and qualitative data (for which we have participant consent to share in de-identified form) will be collected as part of the project and will be available for sharing in raw or aggregate form. Specifically, any individual level data will be de-identified before sharing. Demographic data may only be shared at an aggregated level as needed to maintain confidentiality.
Student-level data including
- Pre- and posttest data from proximal and distal writing measures
- Demographic data (age, sex, race/ethnicity, free or reduced price lunch status, home language, special education and English language learning services status)
- Pre/post knowledge and skills data (collected via secure survey tools such as Qualtrics)
- Teacher efficacy data (collected via secure survey tools such as Qualtrics)
- Fidelity data (teachers’ accuracy of implementation of Data-Based Instruction; DBI)
- Teacher logs of time spent on DBI activities
- Demographic data (age, sex, race/ethnicity, degrees earned, teaching certification, years and nature of teaching experience)
- Qualitative field notes from classroom observations and transcribed teacher responses to semi-structured follow-up interview questions.
- Coded qualitative data
- Audio and video files from teacher observations and interviews (participants will sign a release form indicating that they understand that sharing of these files may reveal their identity)
Procedures for managing and for maintaining the confidentiality of the data to be shared
The following procedures will be used to maintain data confidentiality (for managing confidentiality of qualitative data, we will follow additional guidelines ).
- When participants give consent and are enrolled in the study, each will be assigned a unique (random) study identification number. This ID number will be associated with all participant data that are collected, entered, and analyzed for the study.
- All paper data will be stored in locked file cabinets in locked lab/storage space accessible only to research staff at the performance sites. Whenever possible, paper data will only be labeled with the participant’s study ID. Any direct identifiers will be redacted from paper data as soon as it is processed for data entry.
- All electronic data will be stripped of participant names and other identifiable information such as addresses, and emails.
- During the active project period (while data are being collected, coded, and analyzed), data from students and teachers will be entered remotely from the two performance sites into the University of Minnesota’s secure BOX storage (box.umn.edu), which is a highly secure online file-sharing system. Participants’ names and any other direct identifiers will not be entered into this system; rather, study ID numbers will be associated with the data entered into BOX.
- Data will be downloaded from BOX for analysis onto password protected computers and saved only on secure University servers. A log (saved in BOX) will be maintained to track when, at which site, and by whom data are entered as well as downloaded for analysis (including what data are downloaded and for what specific purpose).
Roles and responsibilities of project or institutional staff in the management and retention of research data
Key personnel on the project (PIs XXXXX and XXXXX; Co-Investigator XXXXX) will be the data stewards while the data are “active” (i.e., during data collection, coding, analysis, and publication phases of the project), and will be responsible for documenting and managing the data throughout this time. Additional project personnel (cost analyst, project coordinators, and graduate research assistants at each site) will receive human subjects and data management training at their institutions, and will also be responsible for adhering to the data management plan described above.
Project PIs will develop study-specific protocols and will train all project staff who handle data to follow these protocols. Protocols will include guidelines for managing confidentiality of data (described above), as well as protocols for naming, organizing, and sharing files and entering and downloading data. For example, we will establish file naming conventions and hierarchies for file and folder organization, as well as conventions for versioning files. We will also develop a directory that lists all types of data and where they are stored and entered. As described above, we will create a log to track data entry and downloads for analysis. We will designate one project staff member (e.g., UMN project coordinator) to ensure that these protocols are followed and documentation is maintained. This person will work closely with Co-Investigator XXXXX, who will oversee primary data analysis activities.
At the end of the grant and publication processes, the data will be archived and shared (see Access below) and the University of Minnesota Libraries will serve as the steward of the de-identified, archived dataset from that point forward.
Expected schedule for data access
The complete dataset is expected to be accessible after the study and all related publications are completed, and will remain accessible for at least 10 years after the data are made available publicly. The PIs and Co-Investigator acknowledge that each annual report must contain information about data accessibility, and that the timeframe of data accessibility will be reviewed as part of the annual progress reviews and revised as necessary for each publication.
Format of the final dataset
The format of the final dataset to be available for public access is as follows: De-identified raw paper data (e.g., student pre/posttest data) will be scanned into pdf files. Raw data collected electronically (e.g., via survey tools, field notes) will be available in MS Excel spreadsheets or pdf files. Raw data from audio/video files will be in .wav format. Audio/video materials and field notes from observations/interviews will also be transcribed and coded onto paper forms and scanned into pdf files. The final database will be in a .csv file that can be exported into MS Excel, SAS, SPSS, or ASCII files.
Dataset documentation to be provided
The final data file to be shared will include (a) raw item-level data (where applicable to recreate analyses) with appropriate variable and value labels, (b) all computed variables created during setup and scoring, and (c) all scale scores for the demographic, behavioral, and assessment data. These data will be the de-identified and individual- or aggregate-level data used for the final and published analyses.
Dataset documentation will consist of electronic codebooks documenting the following information: (a) a description of the research questions, methodology, and sample, (b) a description of each specific data source (e.g., measures, observation protocols), and (c) a description of the raw data and derived variables, including variable lists and definitions.
To aid in final dataset documentation, throughout the project, we will maintain a log of when, where, and how data were collected, decisions related to methods, coding, and analysis, statistical analyses, software and instruments used, where data and corresponding documentation are stored, and future research ideas and plans.
Method of data access
Final peer-reviewed publications resulting from the study/grant will be accompanied by the dataset used at the time of publication, during and after the grant period. A long-term data sharing and preservation plan will be used to store and make publicly accessible the data beyond the life of the project. The data will be deposited into the Data Repository for the University of Minnesota (DRUM), http://hdl.handle.net/11299/166578 . This University Libraries’ hosted institutional data repository is an open access platform for dissemination and archiving of university research data. Date files in DRUM are written to an Isilon storage system with two copies, one local to each of the two geographically separated University of Minnesota Data Centers. The local Isilon cluster stores the data in such a way that the data can survive the loss of any two disks or any one node of the cluster. Within two hours of the initial write, data replication to the 2nd Isilon cluster commences. The 2nd cluster employs the same protections as the local cluster, and both verify with a checksum procedure that data has not altered on write. In addition, DRUM provides long-term preservation of digital data files for at least 10 years using services such as migration (limited format types), secure backup, bit-level checksums, and maintains persistent DOIs for datasets, facilitating data citations. In accordance to DRUM policies, the de-identified data will be accompanied by the appropriate documentation, metadata, and code to facilitate reuse and provide the potential for interoperability with similar datasets.
The main benefit of DRUM is whatever is shared through this repository is public; however, a completely open system is not optimal if any of the data could be identifying (e.g., certain types of demographic data). We will work with the University of MN Library System to determine if DRUM is the best option. Another option available to the University of MN, ICPSR ( https://www.icpsr.umich.edu/icpsrweb/ ), would allow us to share data at different levels. Through ICPSR, data are available to researchers at member institutions of ICPSR rather than publicly. ICPSR allows for various mediated forms of sharing, where people interested in getting less de-identified individual level would sign data use agreements before receiving the data, or would need to use special software to access it directly from ICPSR rather than downloading it, for security proposes. ICPSR is a good option for sensitive or other kinds of data that are difficult to de-identify, but is not as open as DRUM. We expect that data for this project will be de-identifiable to a level that we can use DRUM, but will consider ICPSR as an option if needed.
Data agreement
No specific data sharing agreement will be needed if we use DRUM; however, DRUM does have a general end-user access policy ( conservancy.umn.edu/pages/drum/policies/#end-user-access-policy ). If we go with a less open access system such as ICPSR, we will work with ICPSR and the Un-funded Research Agreements (UFRA) coordinator at the University of Minnesota to develop necessary data sharing agreements.
Circumstances preventing data sharing
The data for this study fall under multiple statutes for confidentiality including multiple IRB requirements for confidentiality and FERPA. If it is not possible to meet all of the requirements of these agencies, data will not be shared.
For example, at the two sites where data will be collected, both universities (University of Minnesota and University of Missouri) and school districts have specific requirements for data confidentiality that will be described in consent forms. Participants will be informed of procedures used to maintain data confidentiality and that only de-identified data will be shared publicly. Some demographic data may not be sharable at the individual level and thus would only be provided in aggregate form.
When we collect audio/video data, participants will sign a release form that provides options to have data shared with project personnel only and/or for sharing purposes. We will not share audio/video data from people who do not consent to share it, and we will not publicly share any data that could identify an individual (these parameters will be specified in our IRB-approved informed consent forms). De-identifying is also required for FERPA data. The level of de-identification needed to meet these requirements is extensive, so it may not be possible to share all raw data exactly as collected in order to protect privacy of participants and maintain confidentiality of data.
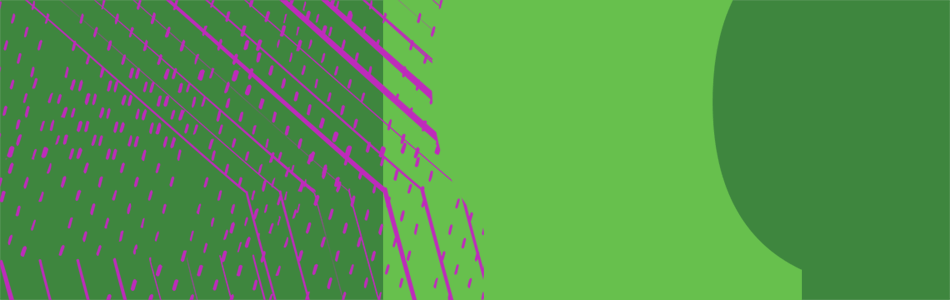
MRC data management plan template
This template should be used to develop a data management plan, part of a research proposal, for the Medical Research Council (MRC).
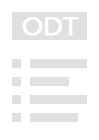
MRC data management plan template (ODT)
ODT , 25 KB
A data management plan should be submitted as part of a research proposal.
You should use this template to make sure that the right information is included.
- 1 March 2024 The data management plan has been updated in line with the revised MRC data sharing policy. It must also include considerations in relation to the UKRI principles and guidance on trusted research and innovation.
This is the website for UKRI: our seven research councils, Research England and Innovate UK. Let us know if you have feedback or would like to help improve our online products and services .
Research and Innovation
Search form.
- Research News
- Poster Session 2024
- Research Day
- Research On Tap
- Huron Migration Announcement
- Research Roadmap
- Reports and Statistics
- Proposals Dashboard
- Awards Dashboard
- Expenditures Dashboard
- Get started
- Student Research Events
- Engage with Research
- Search Opportunities
- Grants and Other Funding
- Get Published
- Sponsored Project Handbook
- Steps to Proposal Submission
- Request a Grants and Contracts Specialist
- Application Guides & Resources
- GRAMS Guide & Resources
- Proposal Submission FAQ
- Budget Basics & Cost Categories
- Fringe Benefits Rates
- Indirect Cost Rates
- Subrecipient vs Vendor Determination
- Data Management Planning
- Available College Resources
- No-Cost Extensions (NCE)
- Programmatic Reporting
- Effort Reporting
- Announcements
- Online PI Training
- Institutional Information
- IRB Protocol Submission
- IRB Forms and Templates
- Training and Education
- Multi-Institutional or Collaborative Research
- IRB Deadlines, Review, and Roster
- Human Subjects Research Determinations
- IRB Policies and Procedures
- External Resources
- Reporting a Concern
- Student Research Guidance
- Participant Compensation
- Human Subjects Recruitment
- IBC Protocol Submission
- IBC Forms and Templates
- Training determination table
- Meeting Dates & Deadline 2023
- References and Resources
- IACUC Protocol Submission
- IACUC Procedures
- IACUC Forms and Templates
- Occupational Health and Safety for Animal Workers
- Field Research
- Resources and Guidance
- Tissue Protocols
- Reporting Animal Welfare Concerns
- Submit a Conflict of Interest Disclosure
- PHS Investigators
- Department of Energy Interim COI Policy
- Conflict of Interest Information Requests
- Scope of Research Requiring Oversight Under Dual Use Research of Concern
- Categories of experiments
- Protocol Review Process
- Export Control
- Federal Research Regulations
- International Affiliations in Research
- Responsible Conduct of Research (RCR)
- International Visiting Scholars
- Countries of Concern
- Foreign Travel
- Foreign Talent Recruitment
- Cybersecurity
- Request a Contract
- Commercialization Process
- Technologies Available For Licensing
- Proposal Managers
- NSF Broader Impacts Review Process
- Partner with UNT Programs
- Additional External Broader Impacts Resources
- Hispanic Serving Institution Proposal Guidance
- Workshops & Information Sessions
- Research BREAKS
- Washington D.C. Fellows Program
- Research Development Resources
- Hispanic Serving Institution Funding Opportunities
- Limited Submissions Program
- External Funding Announcements
- Intramural Seed Funding
- Research and Creativity Awards
- Institutes of Research Excellence
- Instrumentation
- Sample Submission
- Targeted Metabolomics
- Untargeted Metabolomics
- 13C-Stable Isotope Labeling
- Macromolecules
- Policies and Guidelines
- Education and Resources
- About Genomics Center
- Services and Instrumentation
- Questions and Answers: RNAseq
- Designing Your Run
- UNT Greenhouse Core Research Facilities
- User Information
- Request Space
- Materials Research Facility
- Research Computing Services
- Center for Agile and Adaptive Additive Manufacturing (CAAAM)
- Outcome and Broader Impacts
- NSF - Participation Organizations
- Emerging Research Centers
- Annual Report
- Research Magazine
- Research Radar
- Postdoctoral Affairs Program
- Student Research
- Research Councils and Committees
- Research Seminars
Public Access to Research Using Data Management Plans
Public access to research and data management plans.
Federally funded researchers are required to make their published results available and manage their data.
Background: On February 22, 2013, the Office of Science and Technology Policy (OSTP) released a memorandum entitled “Increasing Access to the Results of Federally Funded Research.” It directed Federal agencies with more than $100 million in research and development (R&D) expenditures to develop plans to make the published results of federally funded research freely available to the public within one year of publication, and it required researchers to better account for and manage the digital data resulting from federally funded scientific research.
UNT developed a framework and plan for PIs and staff to implement that will increase public access to publications and data per the OSTP directive.
- The concept of using a Data Management Plan (DMP) as the vehicle to implement Public Access became a widely shared result. The DMP becomes the obligation to those who receive these grants, the obligation of what the institution must meet.
- Awards to institutions will include conditions to implement this public access requirement. Principal Investigators must ensure that all researchers who work on projects funded in whole or in part by federal grants or cooperative agreements comply with the public access requirements, including public access to data being maintained by the university and maintained going forward. Even though this is a shared responsibility, it is the primary responsibility of the PI to execute the public access to the data and publications.
- The Grants and Contracts Administration office created a process for Public Access to Research using the DMP, in partnership with the library. The philosophy is that data and publications be placed in the appropriate location for a designated discipline with the location of the data and publications housed at the library.
- This Public Access to Research process will be fully implemented by Jan 1, 2021. Every outgoing federal grant will have a DMP associated with it, and every incoming federal grant will have a direct link to a library record and where the information is located, based on the DMP.
This process is required for Researchers with Awards at all Federal Agencies as well as any other non-federal award listing “data management” or “public access” on an RFP, an award document, or on a sponsor’s website.
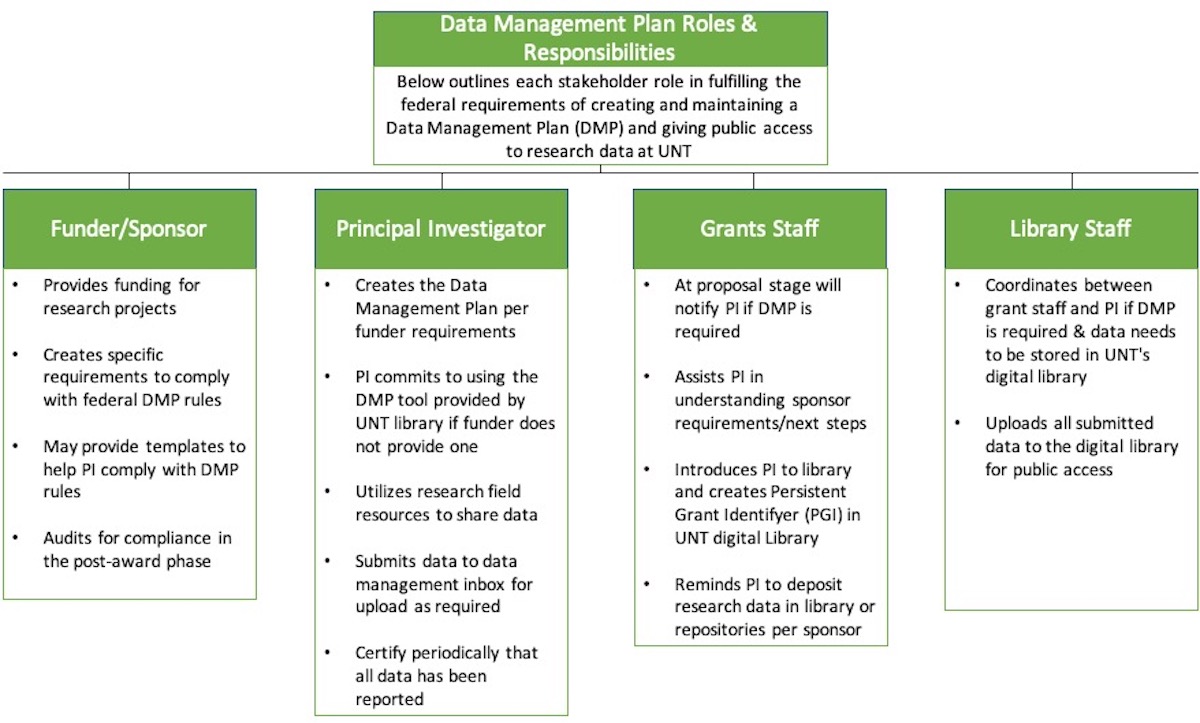
Data Management Procedure
At the proposal stage, the Proposal Team will email the PI letting them know a Data Management Plan (DMP) is required, and that the PI is responsible for developing the plan. Resources to assist PI with DMP:
Follow sponsor agency guidelines on data management storage (example: upload data to NIH PMC database)
Know what the PI’s field/societies utilize
Follow expectations of PI's field and discipline
If a form/format is not provided by the sponsor, it is expected the PI use the tool provided by the UNT Library.
By this process, UNT declares to all UNT university principal investigators that UNT will only recognize the distribution plan for data as detailed in the DMP. The DMP is the formal obligation. regarding public access to data and no other parts of the proposal document will obligate this institution to alternative requirements.
The Proposal Team will set up an email introduction between [email protected] and the PI, and suggest they discuss the Data Management Plan and the availability of the Data Management Plan (DMP) tool. Library staff receiving email to [email protected] and the GCA Specialist should be copied on the email introduction.
If the Proposal/award’s funding source is Federal Flow Through, then a separate DMP is not required by our office, unless required by the sponsor.
Other situations that do not require a DMP:
“Export Control” associated Proposals/Awards
Proposals/Awards that have research formally designated as “Controlled Unclassified” or otherwise deemed by the sponsor as “sensitive.”
Proposals/Awards carrying an IRB protocol with personally identifiable information.
Proposals/Awards with a PI declared “expected intellectual property” exception Requires approval from Research Commercial Agreements (RCA)
In these situations, a DMP would be submitted listing the appropriate exception.
If the grant is awarded, but before GCA sets up the award in their recordkeeping systems, Proposal Team will send the DMP to the GCA Specialist stating an award with a DMP requirement has been received. Included with the DMP should be:
PI name
Federal Agency (or non-fed sponsor requiring a DMP)
Project Title
Agency grant number
GCA Specialist will send email to PI and to [email protected] with DMP and award information attached, congratulating PI on receiving award and telling the PI that we are working to post an online copy of the DMP and establish a Persistent Grant Identifier (PGI) in the UNT Digital Library. The email will include a reminder that the establishment of the DMP and the PGI is required before an award can be set up.
Library staff receiving email to [email protected] creates a record in the UNT Digital Library that contains the PGI and associated copy of the DMP; once the PGI is established, library staff will email the PI, and copy the GCA Specialist, that the DMP and PGI have been set up. The email will include the blank inventory template, with the award information inserted, which is to be used when submitting data and/or publications to the UNT Libraries, with a reminder to include the PGI, or agency grant number with all data and/or publications submitted to the UNT Libraries.
- The DMP and PGI will be saved in the institutional record for these awards.
- GCA Specialist will email PI, copying [email protected] , with the reminder to deposit data and publications and with a recommendation to the PI to submit all available data, publications, and permalinks to content hosted elsewhere (as required by the DMP). If the PI has not yet reported any data or publications to the UNT Digital Library, the attached template can be used. Otherwise, the PI will be asked to add to the last version of the inventory template that was submitted to [email protected] , and to make any applicable submissions at this time rather than waiting until grant closeout. PIs are reminded that they must include the PGI, or agency grant number when submitting data, publications, and permalinks to content elsewhere.
- PI submits any data, publications, and permalinks, including the PGI, Project ID#, or agency grant number, to [email protected] .
If the PI has not yet reported any data or publications to the UNT Digital Library, the attached template can be used. Otherwise, the PI will be asked to add to the last version of the inventory template that was submitted to [email protected] .
The GCA Specialist will also let PI know there will be 2 additional reminders sent, one 12 months after closeout and the final reminder 36 months after closeout, as final opportunities to submit data, publications, and/or permalinks.
PI will be required to submit a statement confirming all data and publications have been disclosed to the library and all conditions of the DMP policy have been fulfilled. This statement can be submitted at any time after closeout and would end future reminders about required data and publications from GCA Specialist.
Following the process described under section (6) above, the PI submits data, publications, and permalinks, to [email protected] .
- GCA Specialist will email PI and copy [email protected] as in the previous sections. Then, following the process described under section (6) above, the PI submits data, publications, and permalinks to [email protected] .
GCA Specialist will email PI copying [email protected] as in the previous sections. Then, following the process described under section (6) above, the PI submits data, publications, and permalinks to [email protected].
A confirmation email from the PI is required at this time stating all data, publications and permalinks have been submitted.
- Should UNT Post Award specialist determine that the PI failed, after 36 months post award closeout, to post the data as stipulated in the formal DMP, the PI will be restricted from access to the UNT pre-award proposal submission process until and unless the VPRI approves a reconciliation.
Note : If there is a change to the primary PI on an award with a federal agency, or other award requiring a DMP, the GCA Specialist will contact the new PI about the institutional obligations of the DMP.
Updated 12/23/20
- Printer-friendly version
Frequently Used Resources
- Disclose an Invention
- UNT Research Roadmap
University Libraries
Library news, get expert help with your data management plan.
Do you need a data management and sharing plan (DMSP) for your grant proposal? The University of Iowa Libraries Research Data Services can help! Brian Westra, data services librarian, is available to help you create a data management plan in alignment with funding agency requirements. If you have questions about:
- Which repository is most appropriate for my data?
- What data standards apply to my data?
- What metadata should be used?
- Are there other agency-specific requirements to include in my plan?
We can help you create a data management plan to support your proposal. Research Data Services monitors funding agency policies and guidance, including the NIH policy.
Resources for NIH plans:
- NIH DMSP Checklist
- UI NIH Data Management and Sharing website
- NIH: Writing a Data Management and Sharing Plan
We can also assist with plans for the NSF, CDC, NOAA, and other funders.
Please contact Brian at [email protected] as early as possible to make sure you have plenty of time to submit your proposal.
2022 Impact Factor
- About Publication Information Subscriptions Permissions Advertising Journal Rankings Best Article Award Press Releases
- Resources Access Options Submission Guidelines Reviewer Guidelines Sample Articles Paper Calls Contact Us Submit & Review
- Browse Current Issue All Issues Featured Latest Topics Videos
California Management Review
California Management Review is a premier academic management journal published at UC Berkeley
CMR INSIGHTS
The new data management model: effective data management for ai systems.
by Luca Collina, Mostafa Sayyadi, and Michael Provitera
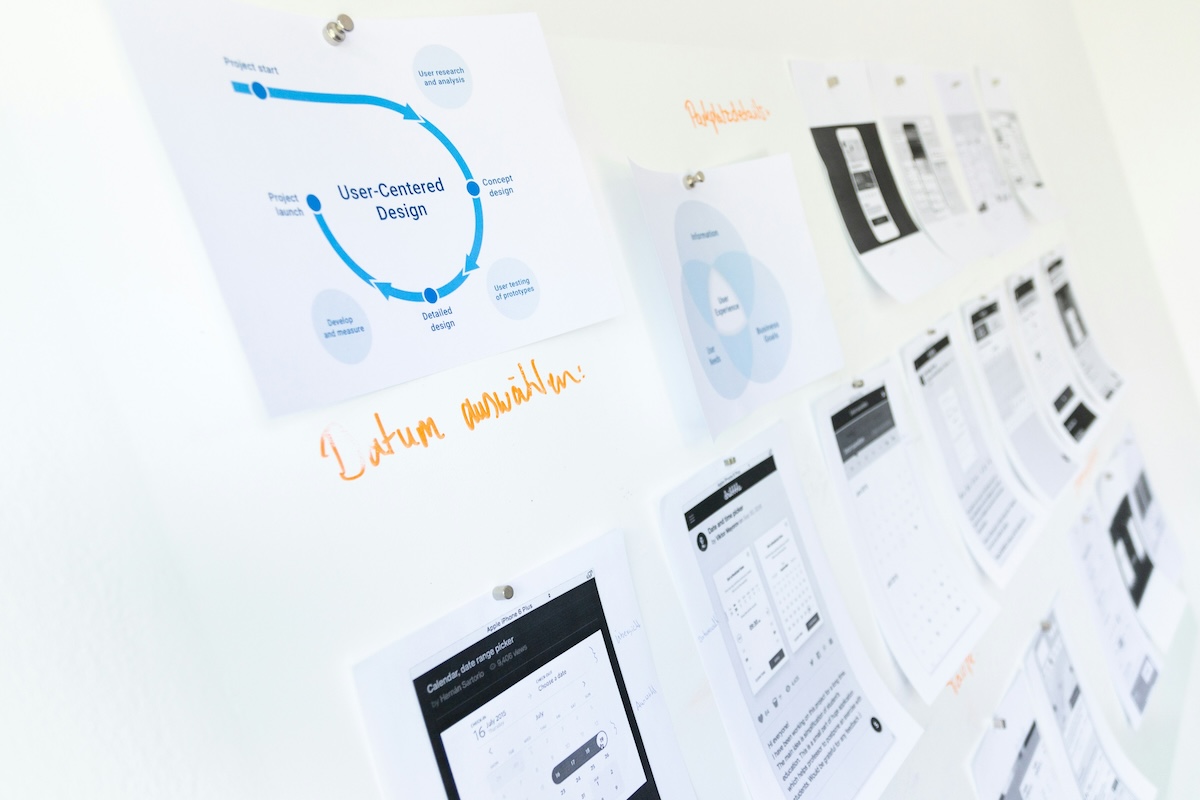
Image Credit | New Data Services
The research presents the Data Quality Funnel Model to improve business decision-making and flexibility, by making data more accurate, reliable, and valuable data for AI systems. This model talks about the critical role of machine learning and predictive analytics. They can effectively enable business strategy and, thus, growth when companies can control the quality of the data that goes into them.
Related CMR Articles
“Getting AI Implementation Right: Insights from a Global Survey” by Rebecka C. Ångström, Michael Björn, Linus Dahlander, Magnus Mähring, & Martin W. Wallin
“Organizational Decision-Making Structures in the Age of Artificial Intelligence” by Yash Raj Shrestha, Shiko M. Ben-Menahem, & Georg von Krogh
All companies have to deal with messy, fragmented data from different silos within their organization. 1, 2 However, prior studies indicate that most companies do not understand the economic impacts of bad data. For example, 60% of companies in the US did not grasp the effects of poor-quality data. 3 Inaccurate or incomplete information costs the US over $3 trillion per year. Poor data quality also costs large organizations an average of $12.9 million annually. 4 Therefore, the business costs of bad data are systemic and substantial.
The Data Quality Funnel Model is a new data management model that can improve the performance of machine learning and artificial intelligence (AI), so companies clean the data they train and operationalize machine learning to help them run actions faster and more informed. With machine learning, explainable AI, cloud computing, and robust data governance, executives take these advanced technologies to bring them to decision-making. Looking at the Data Quality Funnel, executives can see how technology innovation and the company’s culture must join together. This funnel considers high-tech solutions to solve a genuine business need to get high-quality data that drives business growth and keeps companies ahead of others in the digital world.
The Potential Issues and Opportunities of Data Quality
Data quality should always be the initial point of consideration before any machine learning model implementation. Companies can implement data governance and management policies to more effectively handle information. Companies can then maintain data integrity while increasing output quality with such policies. 5
Effetive Data Management for AI Systems
Data Pre-processing or Cleansing: Data cleansing is the critical first step in creating machine learning models. Data cleansing entails eliminating errors or inconsistencies from data to make it reliable for analysis; normalizing brings it all into a standard format to make comparison easier; integration brings in data from various sources in ways that make sense for analysis; finally, data fusion represents merging multiple sources into one coherent analysis. 6
Data-as-a-Service (DaaS): Recent efforts and proposals attempting to ensure data quality from raw sources for Machine Learning and Artificial Intelligence have resulted in the concept of Data-as-a-Service (DaaS), where users receive data without knowing its source, hence requiring continuous Data Quality Management processes using Machine learning models for quality management. 7
Synthetic Data: Synthetic data or pre-fabricated data is data that has been generated using a purpose-built mathematical model or algorithm to solve a (set of) data science task(s). 8 Synthetic data are meant to imitate real data and reuse it for privacy, ethics, and overall quality data. Several applications can be supported by synthetic data: Machine learning for training and privacy and internal business uses like software testing and training models. 9
AI Trust and Governance
Explainable AI (XAI): A lack of clarity around AI can reduce trust in automated decisions. 10 Corporate leaders can use Explainable AI (XAI) to explain AI recommendations. Popular XAI methods like LIME quickly explain individual AI predictions via basic models. SHAP more accurately explains predictions using global data patterns. Companies must train all employees to understand AI outputs and explanations to fully benefit from XAI, empowering people to use AI more confidently.
Algorithms Governance: Studies are developing guidance for companies and governments to get AI’s benefits while minimizing downsides. 11 Recent studies have been focused on healthcare and industry. However, simple processes for responsible AI governance are needed more broadly. This research area is still exploratory. Leaders need plain guidelines to govern AI development. A recent white paper released by HM Guidelines for AI indicates how generative AI requires governance to guarantee high-quality information, accountability, oversight, and privacy, which is a further step ahead.
We propose a specific structure that highlights roles with different levels of responsibility and accountability. A compelling proposal elaborates on the potential strategies to consider to validate the results of elaborations through algorithms, their processes, and XAI. Companies can create oversight to ensure artificial intelligence (AI) is used properly, specifically for algorithms.
Institutional Challenging: Institutions, by creating committees, including AI specialists and non-executive directors, may establish overarching rules to guide decisions with both artificial intelligence technology and human expertise.
Consultancy Challenging: These challenges may be tackled by external professionals who utilize critical assessment to produce more substantial and sustainable outcomes through independent and impartial opinions.
Operational Challenging: These challenges are for the operations staff who watch directly how the AI systems work on tasks. They can run checks and raise issues about problems to rectify algorithms and improve them through an escalation process, but they don’t intervene in modifying the algorithms.
There can also be high-level rules, outside audits, and day-to-day monitoring of the AI. Working together, these can help make AI accountable and catch problems early. The goal is to have people with different views in place to develop and use AI responsibly. Our proposed model requires integration between AI experts, managers, and executives. These responsibilities are diverse and different before and after the outcomes of AI’s decision-making processes. The visualization of the possible roles following the algorithms’ governance and auditing is shown in Figure 1 .
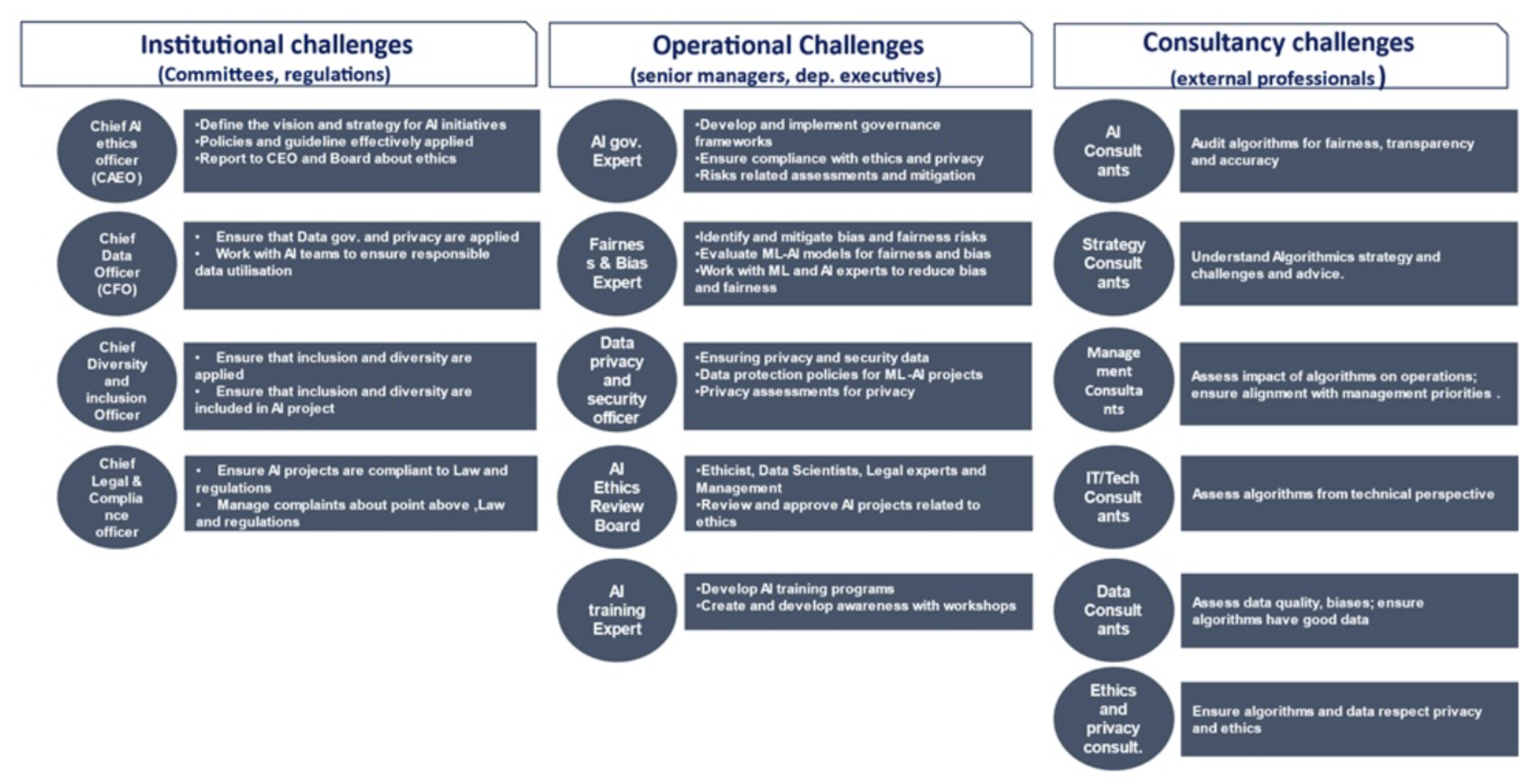
Figure 1: The Roles of AI Experts, Managers, Executives, and Consultants
The Moderating Factors
Data Culture and Leadership: Establishing a data culture within an organizational culture is vital in creating successful business strategies, particularly considering start-ups rely heavily on data from day one. 12, 13
Trust in AI and Machine Learning Outcomes: Using AI and machine learning in business decisions has benefits and risks. AI can improve decision-making, especially regarding customers and marketing. However, AI could also damage value and privacy and models might expose private data, be unfair (show bias), or lack interpretability and transparency. These issues are severe in healthcare. More work is needed to make AI trustworthy and to balance accuracy, avoiding harm and bias while protecting privacy. Technology cannot just focus on performance; it needs collaboration to ensure systems are safe, fair, accountable, and compliant with regulations. 14
XAI (Explainable Artificial Intelligence): There is no consensus on what makes an AI explanation valid or valuable. Some research suggests using logical, step-by-step approaches to build trust in explanations and objective ways to measure explanation quality. 15, 16 But critics say more work is needed so AI explanations are accurate, fair, and genuinely understandable to ordinary people. Overall, explainable AI lacks clear standards for defining and assessing explanations.
Cloud: Using machine learning and AI to make cloud computing more flexible for businesses has been researched and studied extensively. machine learning and AI can enhance resource management in cloud computing.
The Data Quality Funnel Model
Leaders must take responsibility for the AI technology their companies use, even if it is unclear who is accountable when machine learning causes harm. Rather than trying to force accountability despite messy data inputs, fixing problems earlier is more efficient. Carefully checking training data, removing errors, and standardizing inconsistencies builds trust in AI systems while avoiding extra work later. Putting good data practices naturally enables accountable AI systems down the road. Clean data flowing into algorithms pays forward accountability. Therefore, different ideas, good data management, and responsible AI reinforce each other.
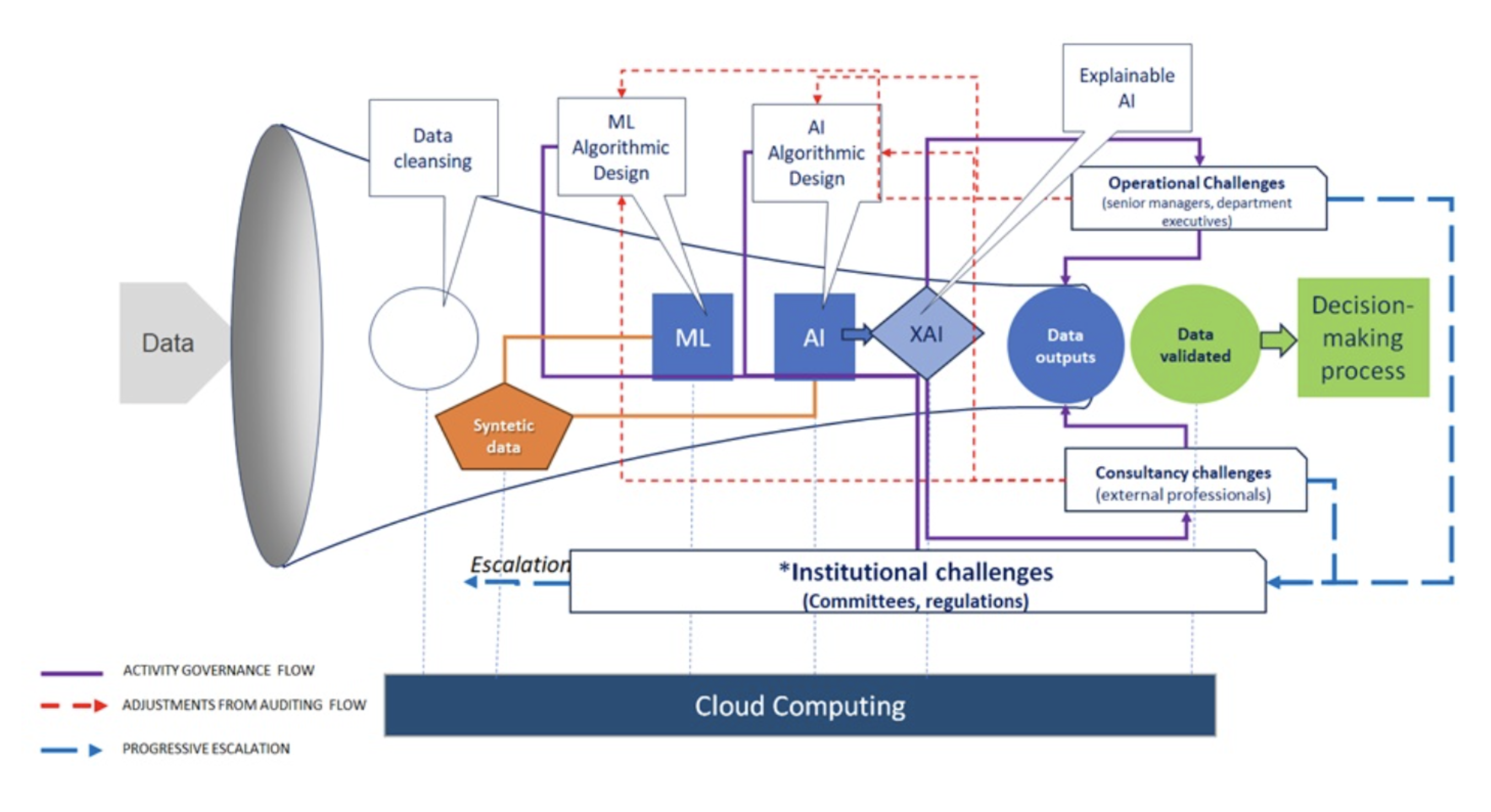
Figure 2: The Data Quality Funnel Model
In the following table, the integration between data quality and accountability is shown:
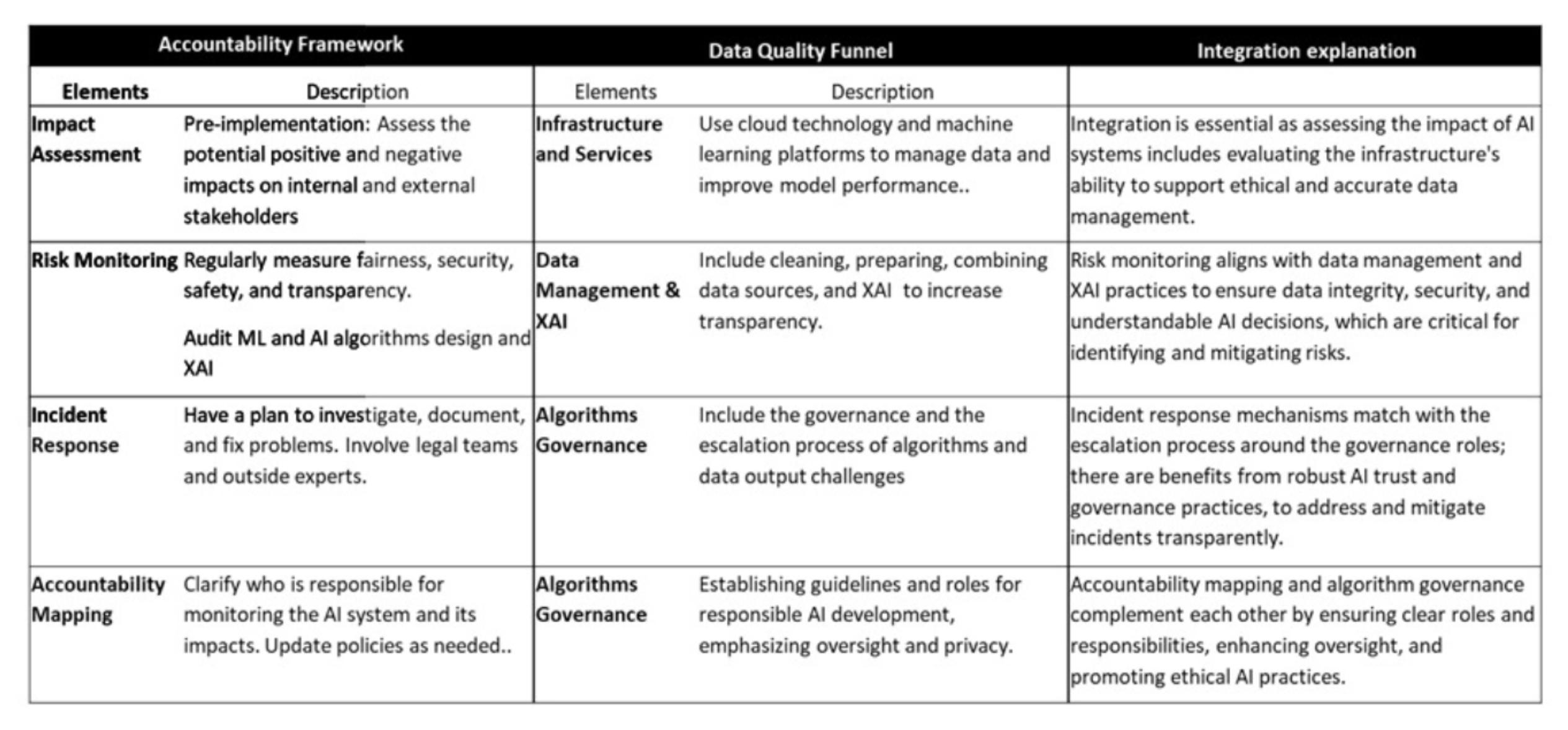
Table 1: Data Quality and Accountability
In Conclusion
This article shows how vital good data is for companies making choices and plans in our tech world. As AI and data become more critical to businesses, ensuring the data used in AI systems is correct and secure is challenging. This paper gives a way to manage these issues - the Data Quality Funnel Model. This model lays out steps to check data is reliable, easy to access, and safe before using it to guide major choices. Clearly showing how to check data at each point helps avoid mistakes or problems. Using this model lets businesses apply AI well to keep up with the competition. The Data Quality Funnel Model fills a gap by showing companies how to handle data troubles posed by new tech. This model gives clear guidance on preparing quality data for strategy and choices that are current real business needs. By lighting the way for accuracy, our proposal displays a route for success in navigating the intricate, tech-driven business world today.
Fan, W., & Geerts, F. (2022 ). Foundations of data quality management. Switzerland: Springer Nature.
Ghasemaghaei, M., & Calic, G. (2019). Does big data enhance firm innovation competency? The mediating role of data-driven insights. Journal of Business Research, 104(C), 69-84.
Moore, S. (2018). How to Stop Data Quality Undermining Your Business. Retrieved 02 02, 2024, from https://www.gartner.com/smarterwithgartner/how-to-stop-data-quality-undermining-your-business
Sakpal, M. (2021). How to Improve Your Data Quality. Retrieved 02 02, 2024, from https://www.gartner.com/smarterwithgartner/how-to-improve-your-data-quality
Khatri, V., & Brown, C. V. (2010). Designing data governance. Communications of the ACM, 53(1), pp. 148-152.
Allamanis, M., & Brockschmidt, M. (2021, 12 8). Finding and fixing bugs with deep learning. Retrieved from Microsoft Research Blog: https://www.microsoft.com/en-us/research/blog/finding-and-fixing-bugs-with-deep-learning/
Azimi, S., & Pahl, C. (2021). Continuous Data Quality Management for Machine Learning based Data-as-a-Service Architectures. International Conference on Cloud Computing and Services Science. 328-335.
Jordon, J., Szpruch, L., Houssiau, F., Bottarelli, M., Cherubin, G., Maple, C. & Weller, A. (2022). Synthetic Data – what, why and how?,. arXiv:, arXiv:2205.03257v1, 5.
James, S., Harbron, C., Branson, J., & Sundler, M. (2021). Synthetic data use: exploring use cases to optimize data utility. Discover Artificial Intelligence, 1, 15. https://doi.org/10.1007/s44163-021-00016-y
Tiwari, R. (2023). Explainable AI (XAI) and its Applications in Building Trust and Understanding in AI Decision Making. International Journal of Management Science and Engineering Management, 7(1), 1-13.
Nikitaeva, A., & Salem, A. (2022.). Institutional Framework for The Development of Artificial Intelligence in The Industry. Journal of Institutional Studies, 14(1), 108-126.
Antonopoulou, H., Halkiopoulos, C., Barlou, O., & Beligiannis, G. (2020). Leadership Types and Digital Leadership in Higher Education: Behavioural Data Analysis from University of Patras in Greece. International Journal of Learning, International Journal of Learning Teaching and Educational Research, 19(4), 110-129.
Denning, S. (2020). Why a culture of experimentation requires management transformation. Strategy & Leadership,(48), 11-16.
Strobel, M., & Shokri, R. (2022). Data Privacy and Trustworthy Machine Learning. IEEE Security & Privacy, 20(5), 44-49.
Ignatiev, A. (2020). Towards Trustable Explainable AI. Proceedings of the Twenty-Ninth International Joint Conference on Artificial IntelligenceJanuary, 5154–5158.
Yang, C., Sinning, R., Lewis, G., Kastner, C., & T., W. (2022). Capabilities for better ML engineering. Retrieved from ARXIV: https://arxiv.org/abs/2211.06409
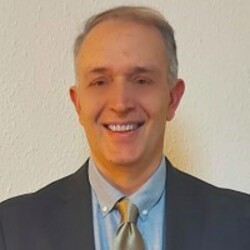
Recommended
Current issue.
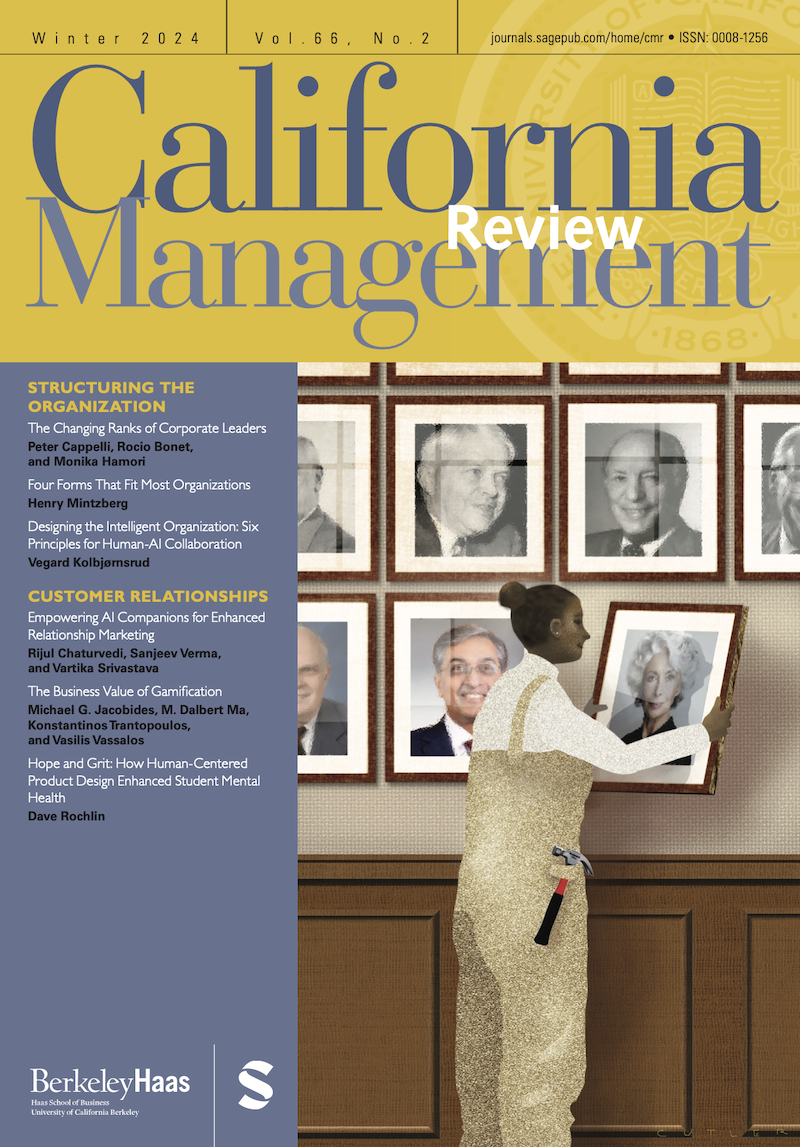
Volume 66, Issue 2 Winter 2024
Recent CMR Articles
The Changing Ranks of Corporate Leaders
The Business Value of Gamification
Hope and Grit: How Human-Centered Product Design Enhanced Student Mental Health
Four Forms That Fit Most Organizations
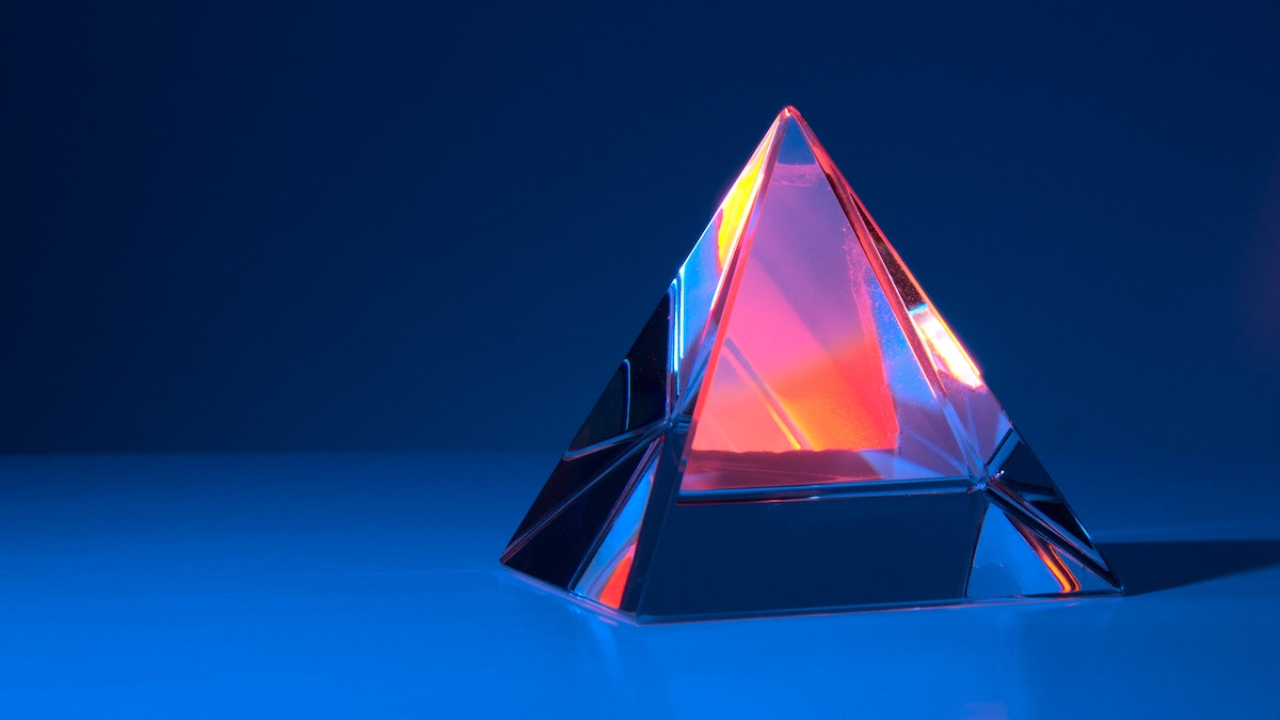
Managing Multi-Sided Platforms: Platform Origins and Go-to-Market Strategy
Berkeley-Haas's Premier Management Journal
Published at Berkeley Haas for more than sixty years, California Management Review seeks to share knowledge that challenges convention and shows a better way of doing business.

An official website of the United States government
The .gov means it’s official. Federal government websites often end in .gov or .mil. Before sharing sensitive information, make sure you’re on a federal government site.
The site is secure. The https:// ensures that you are connecting to the official website and that any information you provide is encrypted and transmitted securely.
- Publications
- Account settings
Preview improvements coming to the PMC website in October 2024. Learn More or Try it out now .
- Advanced Search
- Journal List
- PLoS Comput Biol
- v.11(10); 2015 Oct

Ten Simple Rules for Creating a Good Data Management Plan
William k. michener.
College of University Libraries & Learning Sciences, University of New Mexico, Albuquerque, New Mexico, United States of America
Introduction
Research papers and data products are key outcomes of the science enterprise. Governmental, nongovernmental, and private foundation sponsors of research are increasingly recognizing the value of research data. As a result, most funders now require that sufficiently detailed data management plans be submitted as part of a research proposal. A data management plan (DMP) is a document that describes how you will treat your data during a project and what happens with the data after the project ends. Such plans typically cover all or portions of the data life cycle—from data discovery, collection, and organization (e.g., spreadsheets, databases), through quality assurance/quality control, documentation (e.g., data types, laboratory methods) and use of the data, to data preservation and sharing with others (e.g., data policies and dissemination approaches). Fig 1 illustrates the relationship between hypothetical research and data life cycles and highlights the links to the rules presented in this paper. The DMP undergoes peer review and is used in part to evaluate a project’s merit. Plans also document the data management activities associated with funded projects and may be revisited during performance reviews.

As part of the research life cycle (A), many researchers (1) test ideas and hypotheses by (2) acquiring data that are (3) incorporated into various analyses and visualizations, leading to interpretations that are then (4) published in the literature and disseminated via other mechanisms (e.g., conference presentations, blogs, tweets), and that often lead back to (1) new ideas and hypotheses. During the data life cycle (B), researchers typically (1) develop a plan for how data will be managed during and after the project; (2) discover and acquire existing data and (3) collect and organize new data; (4) assure the quality of the data; (5) describe the data (i.e., ascribe metadata); (6) use the data in analyses, models, visualizations, etc.; and (7) preserve and (8) share the data with others (e.g., researchers, students, decision makers), possibly leading to new ideas and hypotheses.
Earlier articles in the Ten Simple Rules series of PLOS Computational Biology provided guidance on getting grants [ 1 ], writing research papers [ 2 ], presenting research findings [ 3 ], and caring for scientific data [ 4 ]. Here, I present ten simple rules that can help guide the process of creating an effective plan for managing research data—the basis for the project’s findings, research papers, and data products. I focus on the principles and practices that will result in a DMP that can be easily understood by others and put to use by your research team. Moreover, following the ten simple rules will help ensure that your data are safe and sharable and that your project maximizes the funder’s return on investment.
Rule 1: Determine the Research Sponsor Requirements
Research communities typically develop their own standard methods and approaches for managing and disseminating data. Likewise, research sponsors often have very specific DMP expectations. For instance, the Wellcome Trust, the Gordon and Betty Moore Foundation (GBMF), the United States National Institutes of Health (NIH), and the US National Science Foundation (NSF) all fund computational biology research but differ markedly in their DMP requirements. The GBMF, for instance, requires that potential grantees develop a comprehensive DMP in conjunction with their program officer that answers dozens of specific questions. In contrast, NIH requirements are much less detailed and primarily ask that potential grantees explain how data will be shared or provide reasons as to why the data cannot be shared. Furthermore, a single research sponsor (such as the NSF) may have different requirements that are established for individual divisions and programs within the organization. Note that plan requirements may not be labeled as such; for example, the National Institutes of Health guidelines focus largely on data sharing and are found in a document entitled “NIH Data Sharing Policy and Implementation Guidance” ( http://grants.nih.gov/grants/policy/data_sharing/data_sharing_guidance.htm ).
Significant time and effort can be saved by first understanding the requirements set forth by the organization to which you are submitting a proposal. Research sponsors normally provide DMP requirements in either the public request for proposals (RFP) or in an online grant proposal guide. The DMPTool ( https://dmptool.org/ ) and DMPonline ( https://dmponline.dcc.ac.uk/ ) websites are also extremely valuable resources that provide updated funding agency plan requirements (for the US and United Kingdom, respectively) in the form of templates that are usually accompanied with annotated advice for filling in the template. The DMPTool website also includes numerous example plans that have been published by DMPTool users. Such examples provide an indication of the depth and breadth of detail that are normally included in a plan and often lead to new ideas that can be incorporated in your plan.
Regardless of whether you have previously submitted proposals to a particular funding program, it is always important to check the latest RFP, as well as the research sponsor’s website, to verify whether requirements have recently changed and how. Furthermore, don’t hesitate to contact the responsible program officer(s) that are listed in a specific solicitation to discuss sponsor requirements or to address specific questions that arise as you are creating a DMP for your proposed project. Keep in mind that the principle objective should be to create a plan that will be useful for your project. Thus, good data management plans can and often do contain more information than is minimally required by the research sponsor. Note, though, that some sponsors constrain the length of DMPs (e.g., two-page limit); in such cases, a synopsis of your more comprehensive plan can be provided, and it may be permissible to include an appendix, supplementary file, or link.
Rule 2: Identify the Data to Be Collected
Every component of the DMP depends upon knowing how much and what types of data will be collected. Data volume is clearly important, as it normally costs more in terms of infrastructure and personnel time to manage 10 terabytes of data than 10 megabytes. But, other characteristics of the data also affect costs as well as metadata, data quality assurance and preservation strategies, and even data policies. A good plan will include information that is sufficient to understand the nature of the data that is collected, including:
- Types. A good first step is to list the various types of data that you expect to collect or create. This may include text, spreadsheets, software and algorithms, models, images and movies, audio files, and patient records. Note that many research sponsors define data broadly to include physical collections, software and code, and curriculum materials.
- Sources. Data may come from direct human observation, laboratory and field instruments, experiments, simulations, and compilations of data from other studies. Reviewers and sponsors may be particularly interested in understanding if data are proprietary, are being compiled from other studies, pertain to human subjects, or are otherwise subject to restrictions in their use or redistribution.
- Volume. Both the total volume of data and the total number of files that are expected to be collected can affect all other data management activities.
- Data and file formats. Technology changes and formats that are acceptable today may soon be obsolete. Good choices include those formats that are nonproprietary, based upon open standards, and widely adopted and preferred by the scientific community (e.g., Comma Separated Values [CSV] over Excel [.xls, xlsx]). Data are more likely to be accessible for the long term if they are uncompressed, unencrypted, and stored using standard character encodings such as UTF-16.
The precise types, sources, volume, and formats of data may not be known beforehand, depending on the nature and uniqueness of the research. In such case, the solution is to iteratively update the plan (see Rule 9 ).
Rule 3: Define How the Data Will Be Organized
Once there is an understanding of the volume and types of data to be collected, a next obvious step is to define how the data will be organized and managed. For many projects, a small number of data tables will be generated that can be effectively managed with commercial or open source spreadsheet programs like Excel and OpenOffice Calc. Larger data volumes and usage constraints may require the use of relational database management systems (RDBMS) for linked data tables like ORACLE or mySQL, or a Geographic Information System (GIS) for geospatial data layers like ArcGIS, GRASS, or QGIS.
The details about how the data will be organized and managed could fill many pages of text and, in fact, should be recorded as the project evolves. However, in drafting a DMP, it is most helpful to initially focus on the types and, possibly, names of the products that will be used. The software tools that are employed in a project should be amenable to the anticipated tasks. A spreadsheet program, for example, would be insufficient for a project in which terabytes of data are expected to be generated, and a sophisticated RDMBS may be overkill for a project in which only a few small data tables will be created. Furthermore, projects dependent upon a GIS or RDBMS may entail considerable software costs and design and programming effort that should be planned and budgeted for upfront (see Rules 9 and 10 ). Depending on sponsor requirements and space constraints, it may also be useful to specify conventions for file naming, persistent unique identifiers (e.g., Digital Object Identifiers [DOIs]), and versioning control (for both software and data products).
Rule 4: Explain How the Data Will Be Documented
Rows and columns of numbers and characters have little to no meaning unless they are documented in some fashion. Metadata—the details about what, where, when, why, and how the data were collected, processed, and interpreted—provide the information that enables data and files to be discovered, used, and properly cited. Metadata include descriptions of how data and files are named, physically structured, and stored as well as details about the experiments, analytical methods, and research context. It is generally the case that the utility and longevity of data relate directly to how complete and comprehensive the metadata are. The amount of effort devoted to creating comprehensive metadata may vary substantially based on the complexity, types, and volume of data.
A sound documentation strategy can be based on three steps. First, identify the types of information that should be captured to enable a researcher like you to discover, access, interpret, use, and cite your data. Second, determine whether there is a community-based metadata schema or standard (i.e., preferred sets of metadata elements) that can be adopted. As examples, variations of the Dublin Core Metadata Initiative Abstract Model are used for many types of data and other resources, ISO (International Organization for Standardization) 19115 is used for geospatial data, ISA-Tab file format is used for experimental metadata, and Ecological Metadata Language (EML) is used for many types of environmental data. In many cases, a specific metadata content standard will be recommended by a target data repository, archive, or domain professional organization. Third, identify software tools that can be employed to create and manage metadata content (e.g., Metavist, Morpho). In lieu of existing tools, text files (e.g., readme.txt) that include the relevant metadata can be included as headers to the data files.
A best practice is to assign a responsible person to maintain an electronic lab notebook, in which all project details are maintained. The notebook should ideally be routinely reviewed and revised by another team member, as well as duplicated (see Rules 6 and 9 ). The metadata recorded in the notebook provide the basis for the metadata that will be associated with data products that are to be stored, reused, and shared.
Rule 5: Describe How Data Quality Will Be Assured
Quality assurance and quality control (QA/QC) refer to the processes that are employed to measure, assess, and improve the quality of products (e.g., data, software, etc.). It may be necessary to follow specific QA/QC guidelines depending on the nature of a study and research sponsorship; such requirements, if they exist, are normally stated in the RFP. Regardless, it is good practice to describe the QA/QC measures that you plan to employ in your project. Such measures may encompass training activities, instrument calibration and verification tests, double-blind data entry, and statistical and visualization approaches to error detection. Simple graphical data exploration approaches (e.g., scatterplots, mapping) can be invaluable for detecting anomalies and errors.
Rule 6: Present a Sound Data Storage and Preservation Strategy
A common mistake of inexperienced (and even many experienced) researchers is to assume that their personal computer and website will live forever. They fail to routinely duplicate their data during the course of the project and do not see the benefit of archiving data in a secure location for the long term. Inevitably, though, papers get lost, hard disks crash, URLs break, and tapes and other media degrade, with the result that the data become unavailable for use by both the originators and others. Thus, data storage and preservation are central to any good data management plan. Give careful consideration to three questions:
- How long will the data be accessible?
- How will data be stored and protected over the duration of the project?
- How will data be preserved and made available for future use?
The answer to the first question depends on several factors. First, determine whether the research sponsor or your home institution have any specific requirements. Usually, all data do not need to be retained, and those that do need not be retained forever. Second, consider the intrinsic value of the data. Observations of phenomena that cannot be repeated (e.g., astronomical and environmental events) may need to be stored indefinitely. Data from easily repeatable experiments may only need to be stored for a short period. Simulations may only need to have the source code, initial conditions, and verification data stored. In addition to explaining how data will be selected for short-term storage and long-term preservation, remember to also highlight your plans for the accompanying metadata and related code and algorithms that will allow others to interpret and use the data (see Rule 4 ).
Develop a sound plan for storing and protecting data over the life of the project. A good approach is to store at least three copies in at least two geographically distributed locations (e.g., original location such as a desktop computer, an external hard drive, and one or more remote sites) and to adopt a regular schedule for duplicating the data (i.e., backup). Remote locations may include an offsite collaborator’s laboratory, an institutional repository (e.g., your departmental, university, or organization’s repository if located in a different building), or a commercial service, such as those offered by Amazon, Dropbox, Google, and Microsoft. The backup schedule should also include testing to ensure that stored data files can be retrieved.
Your best bet for being able to access the data 20 years beyond the life of the project will likely require a more robust solution (i.e., question 3 above). Seek advice from colleagues and librarians to identify an appropriate data repository for your research domain. Many disciplines maintain specific repositories such as GenBank for nucleotide sequence data and the Protein Data Bank for protein sequences. Likewise, many universities and organizations also host institutional repositories, and there are numerous general science data repositories such as Dryad ( http://datadryad.org/ ), figshare ( http://figshare.com/ ), and Zenodo ( http://zenodo.org/ ). Alternatively, one can easily search for discipline-specific and general-use repositories via online catalogs such as http://www.re3data.org/ (i.e., REgistry of REsearch data REpositories) and http://www.biosharing.org (i.e., BioSharing). It is often considered good practice to deposit code in a host repository like GitHub that specializes in source code management as well as some types of data like large files and tabular data (see https://github.com/ ). Make note of any repository-specific policies (e.g., data privacy and security, requirements to submit associated code) and costs for data submission, curation, and backup that should be included in the DMP and the proposal budget.
Rule 7: Define the Project’s Data Policies
Despite what may be a natural proclivity to avoid policy and legal matters, researchers cannot afford to do so when it comes to data. Research sponsors, institutions that host research, and scientists all have a role in and obligation for promoting responsible and ethical behavior. Consequently, many research sponsors require that DMPs include explicit policy statements about how data will be managed and shared. Such policies include:
- licensing or sharing arrangements that pertain to the use of preexisting materials;
- plans for retaining, licensing, sharing, and embargoing (i.e., limiting use by others for a period of time) data, code, and other materials; and
- legal and ethical restrictions on access and use of human subject and other sensitive data.
Unfortunately, policies and laws often appear or are, in fact, confusing or contradictory. Furthermore, policies that apply within a single organization or in a given country may not apply elsewhere. When in doubt, consult your institution’s office of sponsored research, the relevant Institutional Review Board, or the program officer(s) assigned to the program to which you are applying for support.
Despite these caveats, it is usually possible to develop a sound policy by following a few simple steps. First, if preexisting materials, such as data and code, are being used, identify and include a description of the relevant licensing and sharing arrangements in your DMP. Explain how third party software or libraries are used in the creation and release of new software. Note that proprietary and intellectual property rights (IPR) laws and export control regulations may limit the extent to which code and software can be shared.
Second, explain how and when the data and other research products will be made available. Be sure to explain any embargo periods or delays such as publication or patent reasons. A common practice is to make data broadly available at the time of publication, or in the case of graduate students, at the time the graduate degree is awarded. Whenever possible, apply standard rights waivers or licenses, such as those established by Open Data Commons (ODC) and Creative Commons (CC), that guide subsequent use of data and other intellectual products (see http://creativecommons.org/ and http://opendatacommons.org/licenses/pddl/summary/ ). The CC0 license and the ODC Public Domain Dedication and License, for example, promote unrestricted sharing and data use. Nonstandard licenses and waivers can be a significant barrier to reuse.
Third, explain how human subject and other sensitive data will be treated (e.g., see http://privacyruleandresearch.nih.gov/ for information pertaining to human health research regulations set forth in the US Health Insurance Portability and Accountability Act). Many research sponsors require that investigators engaged in human subject research approaches seek or receive prior approval from the appropriate Institutional Review Board before a grant proposal is submitted and, certainly, receive approval before the actual research is undertaken. Approvals may require that informed consent be granted, that data are anonymized, or that use is restricted in some fashion.
Rule 8: Describe How the Data Will Be Disseminated
The best-laid preservation plans and data sharing policies do not necessarily mean that a project’s data will see the light of day. Reviewers and research sponsors will be reassured that this will not be the case if you have spelled out how and when the data products will be disseminated to others, especially people outside your research group. There are passive and active ways to disseminate data. Passive approaches include posting data on a project or personal website or mailing or emailing data upon request, although the latter can be problematic when dealing with large data and bandwidth constraints. More active, robust, and preferred approaches include: (1) publishing the data in an open repository or archive (see Rule 6 ); (2) submitting the data (or subsets thereof) as appendices or supplements to journal articles, such as is commonly done with the PLOS family of journals; and (3) publishing the data, metadata, and relevant code as a “data paper” [ 5 ]. Data papers can be published in various journals, including Scientific Data (from Nature Publishing Group), the GeoScience Data Journal (a Wiley publication on behalf of the Royal Meteorological Society), and GigaScience (a joint BioMed Central and Springer publication that supports big data from many biology and life science disciplines).
A good dissemination plan includes a few concise statements. State when, how, and what data products will be made available. Generally, making data available to the greatest extent and with the fewest possible restrictions at the time of publication or project completion is encouraged. The more proactive approaches described above are greatly preferred over mailing or emailing data and will likely save significant time and money in the long run, as the data curation and sharing will be supported by the appropriate journals and repositories or archives. Furthermore, many journals and repositories provide guidelines and mechanisms for how others can appropriately cite your data, including digital object identifiers, and recommended citation formats; this helps ensure that you receive credit for the data products you create. Keep in mind that the data will be more usable and interpretable by you and others if the data are disseminated using standard, nonproprietary approaches and if the data are accompanied by metadata and associated code that is used for data processing.
Rule 9: Assign Roles and Responsibilities
A comprehensive DMP clearly articulates the roles and responsibilities of every named individual and organization associated with the project. Roles may include data collection, data entry, QA/QC, metadata creation and management, backup, data preparation and submission to an archive, and systems administration. Consider time allocations and levels of expertise needed by staff. For small to medium size projects, a single student or postdoctoral associate who is collecting and processing the data may easily assume most or all of the data management tasks. In contrast, large, multi-investigator projects may benefit from having a dedicated staff person(s) assigned to data management.
Treat your DMP as a living document and revisit it frequently (e.g., quarterly basis). Assign a project team member to revise the plan, reflecting any new changes in protocols and policies. It is good practice to track any changes in a revision history that lists the dates that any changes were made to the plan along with the details about those changes, including who made them.
Reviewers and sponsors may be especially interested in knowing how adherence to the data management plan will be assessed and demonstrated, as well as how, and by whom, data will be managed and made available after the project concludes. With respect to the latter, it is often sufficient to include a pointer to the policies and procedures that are followed by the repository where you plan to deposit your data. Be sure to note any contributions by nonproject staff, such as any repository, systems administration, backup, training, or high-performance computing support provided by your institution.
Rule 10: Prepare a Realistic Budget
Creating, managing, publishing, and sharing high-quality data is as much a part of the 21st century research enterprise as is publishing the results. Data management is not new—rather, it is something that all researchers already do. Nonetheless, a common mistake in developing a DMP is forgetting to budget for the activities. Data management takes time and costs money in terms of software, hardware, and personnel. Review your plan and make sure that there are lines in the budget to support the people that manage the data (see Rule 9 ) as well as pay for the requisite hardware, software, and services. Check with the preferred data repository (see Rule 6 ) so that requisite fees and services are budgeted appropriately. As space allows, facilitate reviewers by pointing to specific lines or sections in the budget and budget justification pages. Experienced reviewers will be on the lookout for unfunded components, but they will also recognize that greater or lesser investments in data management depend upon the nature of the research and the types of data.
A data management plan should provide you and others with an easy-to-follow road map that will guide and explain how data are treated throughout the life of the project and after the project is completed. The ten simple rules presented here are designed to aid you in writing a good plan that is logical and comprehensive, that will pass muster with reviewers and research sponsors, and that you can put into practice should your project be funded. A DMP provides a vehicle for conveying information to and setting expectations for your project team during both the proposal and project planning stages, as well as during project team meetings later, when the project is underway. That said, no plan is perfect. Plans do become better through use. The best plans are “living documents” that are periodically reviewed and revised as necessary according to needs and any changes in protocols (e.g., metadata, QA/QC, storage), policy, technology, and staff, as well as reused, in that the most successful parts of the plan are incorporated into subsequent projects. A public, machine-readable, and openly licensed DMP is much more likely to be incorporated into future projects and to have higher impact; such increased transparency in the research funding process (e.g., publication of proposals and DMPs) can assist researchers and sponsors in discovering data and potential collaborators, educating about data management, and monitoring policy compliance [ 6 ].
Acknowledgments
This article is the outcome of a series of training workshops provided for new faculty, postdoctoral associates, and graduate students.
Funding Statement
This work was supported by NSF IIA-1301346, IIA-1329470, and ACI-1430508 ( http://nsf.gov ). The funders had no role in study design, data collection and analysis, decision to publish, or preparation of the manuscript.

An official website of the United States government
Here’s how you know
Official websites use .gov A .gov website belongs to an official government organization in the United States.
Secure .gov websites use HTTPS A lock ( ) or https:// means you’ve safely connected to the .gov website. Share sensitive information only on official, secure websites.
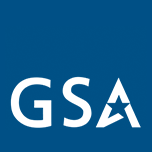
- Explore sell to government
- Ways you can sell to government
- How to access contract opportunities
- Conduct market research
- Register your business
- Certify as a small business
- Become a schedule holder
- Market your business
- Research active solicitations
- Respond to a solicitation
- What to expect during the award process
- Comply with contractual requirements
- Handle contract modifications
- Monitor past performance evaluations
- Explore real estate
- 3D-4D building information modeling
- Art in architecture | Fine arts
- Computer-aided design standards
- Commissioning
- Design excellence
- Engineering
- Project management information system
- Spatial data management
- Facilities operations
- Smart buildings
- Tenant services
- Utility services
- Water quality management
- Explore historic buildings
- Heritage tourism
- Historic preservation policy, tools and resources
- Historic building stewardship
- Videos, pictures, posters and more
- NEPA implementation
- Courthouse program
- Land ports of entry
- Prospectus library
- Regional buildings
- Renting property
- Visiting public buildings
- Real property disposal
- Reimbursable services (RWA)
- Rental policy and procedures
- Site selection and relocation
- For businesses seeking opportunities
- For federal customers
- For workers in federal buildings
- Explore policy and regulations
- Acquisition management policy
- Aviation management policy
- Information technology policy
- Real property management policy
- Relocation management policy
- Travel management policy
- Vehicle management policy
- Federal acquisition regulations
- Federal management regulations
- Federal travel regulations
- GSA acquisition manual
- Managing the federal rulemaking process
- Explore small business
- Explore business models
- Research the federal market
- Forecast of contracting opportunities
- Events and contacts
- Explore travel
- Per diem rates
- Transportation (airfare rates, POV rates, etc.)
- State tax exemption
- Travel charge card
- Conferences and meetings
- E-gov travel service (ETS)
- Travel category schedule
- Federal travel regulation
- Travel policy
- Explore technology
- Cloud computing services
- Cybersecurity products and services
- Data center services
- Hardware products and services
- Professional IT services
- Software products and services
- Telecommunications and network services
- Work with small businesses
- Governmentwide acquisition contracts
- MAS information technology
- Software purchase agreements
- Cybersecurity
- Digital strategy
- Emerging citizen technology
- Federal identity, credentials, and access management
- Mobile government
- Technology modernization fund
- Explore about us
- Annual reports
- Mission and strategic goals
- Role in presidential transitions
- Get an internship
- Launch your career
- Elevate your professional career
- Discover special hiring paths
- Events and training
- Agency blog
- Congressional testimony
- GSA does that podcast
- News releases
- Leadership directory
- Staff directory
- Office of the administrator
- Federal Acquisition Service
- Public Buildings Service
- Staff offices
- Board of Contract Appeals
- Office of Inspector General
- Region 1 | New England
- Region 2 | Northeast and Caribbean
- Region 3 | Mid-Atlantic
- Region 4 | Southeast Sunbelt
- Region 5 | Great Lakes
- Region 6 | Heartland
- Region 7 | Greater Southwest
- Region 8 | Rocky Mountain
- Region 9 | Pacific Rim
- Region 10 | Northwest/Arctic
- Region 11 | National Capital Region
- Per Diem Lookup
Privately owned vehicle (POV) mileage reimbursement rates
GSA has adjusted all POV mileage reimbursement rates effective January 1, 2024.
* Airplane nautical miles (NMs) should be converted into statute miles (SMs) or regular miles when submitting a voucher using the formula (1 NM equals 1.15077945 SMs).
For calculating the mileage difference between airports, please visit the U.S. Department of Transportation's Inter-Airport Distance website.
QUESTIONS: For all travel policy questions, email [email protected] .
Have travel policy questions? Use our ' Have a Question? ' site
PER DIEM LOOK-UP
1 choose a location.
Error, The Per Diem API is not responding. Please try again later.
No results could be found for the location you've entered.
Rates for Alaska, Hawaii, U.S. Territories and Possessions are set by the Department of Defense .
Rates for foreign countries are set by the State Department .
2 Choose a date
Rates are available between 10/1/2021 and 09/30/2024.
The End Date of your trip can not occur before the Start Date.
Traveler reimbursement is based on the location of the work activities and not the accommodations, unless lodging is not available at the work activity, then the agency may authorize the rate where lodging is obtained.
Unless otherwise specified, the per diem locality is defined as "all locations within, or entirely surrounded by, the corporate limits of the key city, including independent entities located within those boundaries."
Per diem localities with county definitions shall include "all locations within, or entirely surrounded by, the corporate limits of the key city as well as the boundaries of the listed counties, including independent entities located within the boundaries of the key city and the listed counties (unless otherwise listed separately)."
When a military installation or Government - related facility(whether or not specifically named) is located partially within more than one city or county boundary, the applicable per diem rate for the entire installation or facility is the higher of the rates which apply to the cities and / or counties, even though part(s) of such activities may be located outside the defined per diem locality.
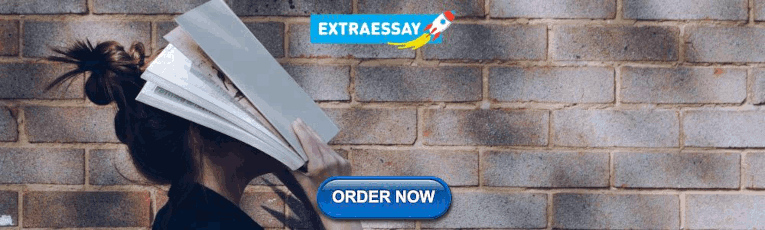
IMAGES
VIDEO
COMMENTS
5 3.5k. Data Management Plans (DMPs) are documents prepared by researchers as they plan a project and write a grant proposal. These plans provide an outline of the types of data that will be collected, the data format, including data and metadata standards, and how the data will be handled throughout the project lifecycle.
Research proposal examples. Writing a research proposal can be quite challenging, but a good starting point could be to look at some examples. We've included a few for you below. Example research proposal #1: "A Conceptual Framework for Scheduling Constraint Management".
When writing your research proposal the following items are important: Fill in the data management paragraph (see the four questions below) Planning: one of the early deliverables will be a detailed data management plan. Budget: take into account the costs (labour and material) for data storage during and data archiving after your project.
A Data Management Cautionary Tale. Why Manage Data? Research today not only has to be rigorous, innovative, and insightful - it also has to be organized! As improved technology creates more capacity to create and store data, it increases the challenge of making data FAIR: Findable, Accessible, Interoperable, and Reusable (The FAIR Guiding ...
For this reason, your plan should describe data management and sharing during your research and, importantly, after your research is complete. Many funding agencies and sponsors require a data management plan with each proposal, but any researcher or team will benefit from developing a data management plan at the beginning of a project.
Sharing data Stanford provides guidance and resources for research data acquisition, sharing and management. Some data is subject to particular protections which require additional terms and management considerations. The Data Management Plan. A data management plan (DMP) may be required as part of your proposal documentation to comply with ...
Tab through this guide to consider each stage of the research data management process, and each correlated section of a data management plan. ... The DMPTool supports the majority of federal and many non-profit and private funding agencies that require data management plans as part of a grant proposal application.
Write A Proposal - Data Management and Sharing - Guides at Johns Hopkins University. Milton S. Eisenhower Library. 7:30am - 6pm. M-level Service Desk. Closed. Online Research Consultation. Checked One Time. Non-Jcard Holder Access. 7:30am - 6pm.
Research papers and data products are key outcomes of the science enterprise. Governmental, nongovernmental, and private foundation sponsors of research are increasingly recognizing the value of research data. As a result, most funders now require that sufficiently detailed data management plans be submitted as part of a research proposal.
What are data-management plans? A data-management plan explains how researchers will handle their data during and after a project, and encompasses creating, sharing and preserving research data of ...
A Data Management Plan (DMP) describes your data management and sharing activities. It is generally 1-3 pages in length and should cover the four phases of the research data lifecycle: Discovery, access and sharing. If you are, or plan to be, in receipt of external funding, check your funder's policies and requirements when writing your DMP.
This guide gathers overviews and resources for data management and sharing following the research workflow for data, from preparing data management and sharing plans for grant proposals, conducting research, to sharing research data. ... Here is an overview of typical components of a proposal data management plan for U.S. funders. The elements ...
A Data Management Plan (DMP) describes data that will be acquired or produced during research; how the data will be managed, described, and stored, what standards you will use, and how data will be handled and protected during and after the completion of the project. ... in general terms, DMP creation should occur between the proposal stage and ...
Show that you understand the current state of research on your topic. Approach: Make a case for your methodology. Demonstrate that you have carefully considered the data, tools, and procedures necessary to conduct your research: Achievability: Confirm that your project is feasible within the timeline of your program or funding deadline
The two-page data management plan is a required part of a proposal to the U.S. National Science Foundation. It describes how a proposal will follow NSF policy on managing, disseminating and sharing research results. This page provides an overview of requirements for the data management plan. See the Proposal and Award Policies and Procedures ...
proposals, making it seem like it is not a true priority 27. In interviews with successful NSF PIs, the sentiment . ... Research Data Management (RDM) is a complex process and to ensure good RDM ...
Examples of data management plans. These examples of data management plans (DMPs) were provided by University of Minnesota researchers. They feature different elements. One is concise and the other is detailed. One utilizes secondary data, while the other collects primary data. Both have explicit plans for how the data is handled through the ...
Plans for data management and sharing of the products of research. Proposals must include a document of no more than two pages uploaded under "Data Management Plan" in the supplementary documentation section of FastLane. This supplementary document should describe how the proposal will conform to NSF policy on the dissemination and sharing ...
A data management plan should be submitted as part of a research proposal. You should use this template to make sure that the right information is included. This is the website for UKRI: our seven research councils, Research England and Innovate UK. Let us know if you have feedback or would like to help improve our online products and services.
Public Access to Research and Data Management Plans. Federally funded researchers are required to make their published results available and manage their data. Background: On February 22, 2013, the Office of Science and Technology Policy (OSTP) released a memorandum entitled "Increasing Access to the Results of Federally Funded Research.".
Step 4: Visualize Data. Data visualization is a powerful tool for your research proposal. With Visme's data visualization software, you can communicate complex ideas and provide context. To access data visualization features in Visme, click "Data" on the left tab.
We can help you create a data management plan to support your proposal. Research Data Services monitors funding agency policies and guidance, including the NIH policy. Resources for NIH plans: NIH DMSP Checklist; UI NIH Data Management and Sharing website; NIH: Writing a Data Management and Sharing Plan
If you are using research data in your research, you must include a data management plan in your research proposal and your research data must be stored in a secure environment, in a tamper-free form, and with sufficient detail (as metadata). You will be asked to upload your research data management plan in the PeopleSoft Ethics Application and ...
Data-as-a-Service (DaaS): Recent efforts and proposals attempting to ensure data quality from raw sources for Machine Learning and Artificial Intelligence have resulted in the concept of Data-as-a-Service (DaaS), where users receive data without knowing its source, hence requiring continuous Data Quality Management processes using Machine ...
Research papers and data products are key outcomes of the science enterprise. Governmental, nongovernmental, and private foundation sponsors of research are increasingly recognizing the value of research data. As a result, most funders now require that sufficiently detailed data management plans be submitted as part of a research proposal.
Vendor support center Research the federal market, report sales, and upload contract information. ... Spatial data management. Facilities management expand menu. Facilities operations. Security. Smart buildings. ... Federal identity, credentials, and access management. Mobile government. Technology modernization fund. Training. IT policy. About ...