
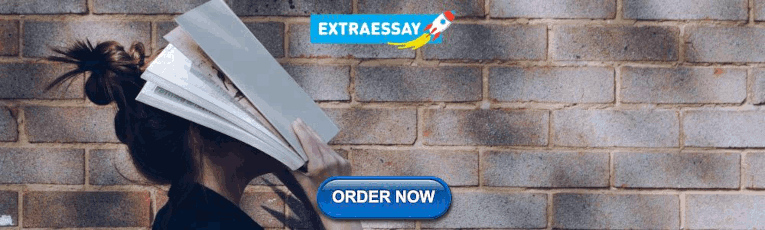
VERTICAL - AI IN HEALTHCARE
AI in wearable sensing, home monitoring, medical imaging, genomics, biomarkers discovery, pharma, digital health, medical devices, personalized medicine, among others and leading to improved patient outcomes, health and care decision-making and efficiencies in healthcare systems.
The advent of genomics and personalized medicine has generated tremendous volumes of data at different biological scales with a need for improved decision-making to assist medical practitioners. Novel approaches using AI in drug design, enable a much faster rate of exploration and discovery of novel molecules and candidate drugs. A growing availability of multimodal digital data enable the understanding of complex relationships between genotype, phenotype, and environment through large-scale AI approaches to tease out critical relationships relative to disease. The digitalization of healthcare data in hospitals and across healthcare systems provides access to vast amounts of real-world data on health, disease, and interventions. Novel AI approaches capitalize on the pre-acquired data to significantly speedup the acquisition, reconstruction, and quantification of medical imaging data. Similarly, real world data open the opportunity to develop novel and decentralized clinical trial as well as to generate virtual populations for in silico clinical trials. However, these opportunities come with concerns about reliability and robustness, explainability, privacy, and data cybersecurity. Furthermore, many other healthcare challenges can benefit from the use of AI, including medical personnel or equipment allocation and scheduling, home monitoring and objective patient outcome monitoring in clinical trials, improved medical devices and manufacturing processes, and supply chain optimization.
CHALLENGE AREAS
- AI in Bioinformatics and Genomics , to improve our understanding of systems biology and disease relationships, leading to novel diagnostics or companion diagnostics for new medical entities with the hope of personalized risk assessments and therapies.
- AI in Drug Discovery , to accelerate lead identification through cognitive molecule research and information sourcing, decision making during the production chain, pharmacovigilance, repurpose failed assets and to improve the rate at which new medical entities move to clinic.
- AI in Clinical Trials , to streamline and improve the quality of clinical trial design and/or patient recruitment, and to automate the capture of clinical data from protocol to submission and reduce costs and timelines.
- AI in Medical Imaging and Sensing , to assist doctors with rapid diagnoses or optimization of therapeutic decisions using complex and heterogenous healthcare data.
- AI in Healthcare Operations , to improve hospital care provision or manufacturing quality and yield using electronic medical records or advanced business analytics.
- AI in Supply Chain Management , to ensure that products are distributed efficiently and effectively despite global dynamics such as pandemics.
- The challenges of regulatory approval for conventional and novel AI approaches.
- Bridging research and financing towards the application and acceptance of new medical opportunities.
ORGANIZING TEAM
Tulay Adali (University of Maryland)
Selin Aviyente (Michigan State University)
Hsun-Hsien Shane Chang (Novartis)
Gary Fogel (Natural Selection, Inc.)
Alejandro F. Frangi (University of Leeds, UK | KU Leuven, Belgium)
Mathews Jacob (University of Iowa)
Alfonso Limon (Oneirix, Inc.)
Karen Panetta (Tufts University)
Ghulam Rasool (Moffett Cancer Center)
Stephen Smith (ClearSky Medical Diagnostics, Ltd.)
For IEEE Members
Ieee spectrum, follow ieee spectrum, support ieee spectrum, enjoy more free content and benefits by creating an account, saving articles to read later requires an ieee spectrum account, the institute content is only available for members, downloading full pdf issues is exclusive for ieee members, downloading this e-book is exclusive for ieee members, access to spectrum 's digital edition is exclusive for ieee members, following topics is a feature exclusive for ieee members, adding your response to an article requires an ieee spectrum account, create an account to access more content and features on ieee spectrum , including the ability to save articles to read later, download spectrum collections, and participate in conversations with readers and editors. for more exclusive content and features, consider joining ieee ., join the world’s largest professional organization devoted to engineering and applied sciences and get access to all of spectrum’s articles, archives, pdf downloads, and other benefits. learn more →, join the world’s largest professional organization devoted to engineering and applied sciences and get access to this e-book plus all of ieee spectrum’s articles, archives, pdf downloads, and other benefits. learn more →, access thousands of articles — completely free, create an account and get exclusive content and features: save articles, download collections, and talk to tech insiders — all free for full access and benefits, join ieee as a paying member., is ai good for health care, industry experts weigh in on inequities and predictive analytics in a new podcast.
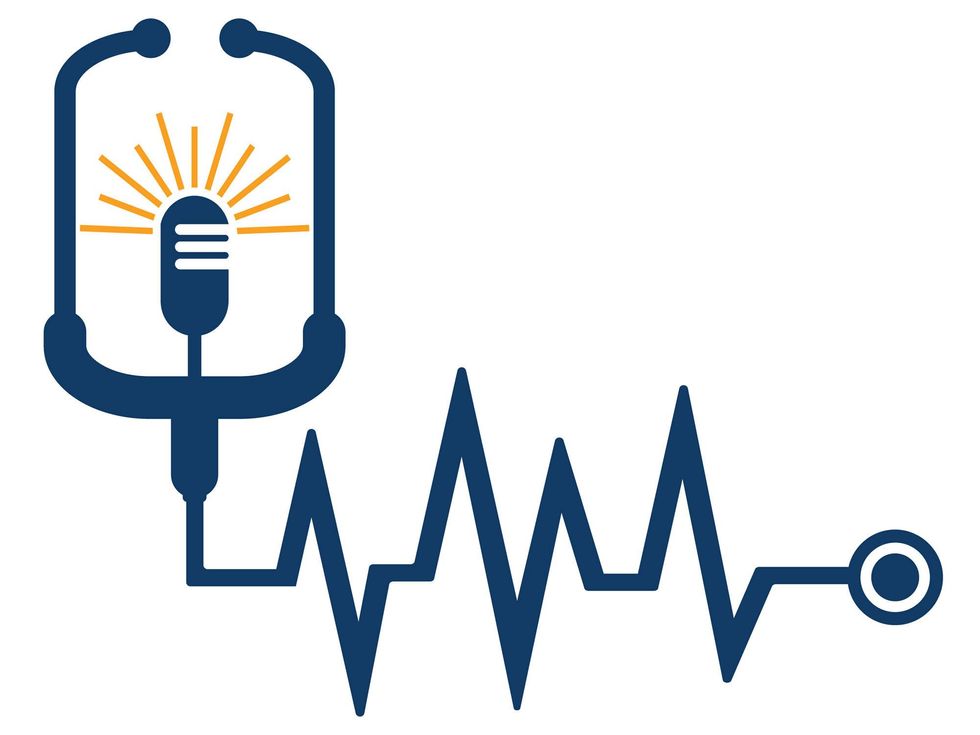
With the rush toward using tools such as artificial intelligence , machine learning, and deep learning technologies to analyze health data for insights, questions are being raised about how good a job the technologies are doing to improve outcomes.
Technologists, clinicians, researchers, scientists, ethicists, policy stewards, and other experts offer their thoughts during the third season of the Re-Think Health Podcast, AI for Good Medicine . The series is part of the IEEE Standards Association’s Healthcare and Life Sciences practice.
Season 3 features these episodes:
The Balance—AI’s Healthcare Goodness for Marginalized Patients. IEEE Senior Member Sampathkumar Veeraraghavan , chair of the IEEE Humanitarian Activities Committee , covers whether AI and machine learning can support fairness, personalization, and inclusiveness or if they create even more inequity.
Riding the Third Wave of AI for Precision Oncology. This episode features Nathan Hayes , founder and CEO of Modal Technology Corp. , and scientist Anthoula Lazaris , director of the biobank at the McGill University Health Center Research Institute . They discuss how AI can improve patient outcomes. The experts also cover whether the full potential for precision oncology will be realized by using the “third wave of AI” with real-world data and practice. In the so-called third wave, AI systems are imagined to have humanlike communication and reasoning capabilities and be able to recognize, classify, and adapt to new situations autonomously.
Advanced AI and Sensors—Reaching the Hardest to Reach Patients at Home. Sumit Kumar Nagpal , cofounder and CEO of Cherish Health —which develops sensors and artificial intelligence—discusses how the technologies can efficiently support the wellness needs of an aging population with dignity and integrity.
AI—The New Pipeline for Targeted Drug Discovery. Maria Luisa Pineda , cofounder and CEO of Envisagenics , covers how splicing RNA using AI and high-performance computing could create a path to targeted drug discovery. RNA splicing is at the forefront of providing insights into diseases that are linked back to RNA errors. Using AI, high-performance computing, and the exponential amount of genetic data, researchers can develop the insights needed for targeted drug discovery in oncology and other genetic conditions faster and more accurately, Pineda says.
Reducing the Healthcare Gap With Explainable AI. Dave DeCaprio , cofounder and CTO of ClosedLoop.ai, discusses health care disparities around the globe. Identifying and leveraging the social determinants of health can close the gap, DeCaprio says. Off-the-shelf AI programs present a new perspective on transparency and the reduction of bias, and they could build trust about the applications among health stakeholders.
Getting Real About Healthcare Data and the Patient’s Journey. The time has come to unleash the value of unstructured data, says Alexandra Ehrlich , principal health innovation scientist at Oracle . AI and machine learning offer opportunities, but first the technologies must be demystified, Ehrlich says, adding that natural language processing can help. Ehrilich explores NLP applications as well as challenges with navigating bias throughout accessible health care data.
Mind Your Data—The First Rule of Predictive Analytics in Clinical Research. Aaron Mann , senior vice president of data science at the Clinical Research Data Sharing Alliance , an IEEE–Industry Standards and Technology Organization alliance, discusses how open data sharing is paving the way for access to better quality, real-world, inclusive data. The sharing of data will enable predictive analytics to be more accurate, resourceful, and utilitarian in clinical research, Mann says.
Can the Health System Benefit From AI as It Stands Today? While focusing on accuracy, ethics, and bias in AI algorithms, we cannot lose sight of the need for more validated data, says Dimitrios Kalogeropoulos . He is a health care expert with the European Commission , UNICEF , the World Bank , and the World Health Organization . Kalogeropoulos explores whether AI is good for medicine and whether medicine is good for AI.
Here are the top 10 insights from the experts:
- Data is a depreciating asset. The longer it sits the less value it has.
- Data is an abyss. If you want AI to make an impact on the health system, then make data reliable by design.
- Fairness is not a math problem. Equity in health care is not about the technology but rather the approaches taken to make health care accessible to all.
- Social determinants of health have significant, if not equal, value to diagnostic health data in closing the health care gap with AI.
- Make explainable AI transparent and off the shelf so that clinicians understand how the algorithms are addressing the questions in the data to help them arrive at the insights needed.
- Going to the same well of data provides the same results. Secondary use of real-world data available in an open, trusted, and validated means can enable predictive analytics to have a material impact on clinical research.
- RNA splicing holds many insights to fighting diseases caused by RNA errors for the development of targeted therapeutics in oncology.
- The trillions of bytes of data in genomes and pathology are no match for AI, which could generate much-needed insights in months, compared with the years it takes oncology researchers using former approaches.
- AI can close the health care gap if it is deployed properly.
- There is a strong disconnect between clinicians and hospital IT system administrators when it comes to implementing, utilizing, and integrating technologies.
This article appears in the December 2022 print issue as “Podcast Explores AI’s Use in Health Care.”
- Q&A: GE Healthcare's Chief Medical Officer for Europe on Which ... ›
- Does AI in Healthcare Need More Emotion? - IEEE Spectrum ›
- How IBM Watson Overpromised and Underdelivered on AI Health ... ›
- A New One-Stop Resource for IEEE Life Members - IEEE Spectrum ›
- Big Data Could Accelerate Drug Approvals - IEEE Spectrum ›
- Artificial intelligence was supposed to transform health care. It hasn't ... ›
- The potential for artificial intelligence in healthcare - PMC ›
IEEE Standards Association facilitates standards development and standards related collaboration among individuals, organizations, and governments.
Ukraine Is the First “Hackers’ War”
Esa satellites to test razor-sharp formation flying, enhance your tech and business skills during ieee education week, more from the institute, how engineers at digital equipment corp. saved ethernet, a novel ieee workshop showcases jamaica’s engineering community, stuart parkin revolutionized disk drive storage, a new alliance is advancing augmented reality, 5 ways to strengthen the ai acquisition process.
Thank you for visiting nature.com. You are using a browser version with limited support for CSS. To obtain the best experience, we recommend you use a more up to date browser (or turn off compatibility mode in Internet Explorer). In the meantime, to ensure continued support, we are displaying the site without styles and JavaScript.
- View all journals
- My Account Login
- Explore content
- About the journal
- Publish with us
- Sign up for alerts
- Perspective
- Open access
- Published: 09 April 2024
The potential for artificial intelligence to transform healthcare: perspectives from international health leaders
- Christina Silcox 1 ,
- Eyal Zimlichmann 2 , 3 ,
- Katie Huber ORCID: orcid.org/0000-0003-2519-8714 1 ,
- Neil Rowen 1 ,
- Robert Saunders 1 ,
- Mark McClellan 1 ,
- Charles N. Kahn III 3 , 4 ,
- Claudia A. Salzberg 3 &
- David W. Bates ORCID: orcid.org/0000-0001-6268-1540 5 , 6 , 7
npj Digital Medicine volume 7 , Article number: 88 ( 2024 ) Cite this article
85 Accesses
2 Altmetric
Metrics details
- Health policy
- Health services
Artificial intelligence (AI) has the potential to transform care delivery by improving health outcomes, patient safety, and the affordability and accessibility of high-quality care. AI will be critical to building an infrastructure capable of caring for an increasingly aging population, utilizing an ever-increasing knowledge of disease and options for precision treatments, and combatting workforce shortages and burnout of medical professionals. However, we are not currently on track to create this future. This is in part because the health data needed to train, test, use, and surveil these tools are generally neither standardized nor accessible. There is also universal concern about the ability to monitor health AI tools for changes in performance as they are implemented in new places, used with diverse populations, and over time as health data may change. The Future of Health (FOH), an international community of senior health care leaders, collaborated with the Duke-Margolis Institute for Health Policy to conduct a literature review, expert convening, and consensus-building exercise around this topic. This commentary summarizes the four priority action areas and recommendations for health care organizations and policymakers across the globe that FOH members identified as important for fully realizing AI’s potential in health care: improving data quality to power AI, building infrastructure to encourage efficient and trustworthy development and evaluations, sharing data for better AI, and providing incentives to accelerate the progress and impact of AI.
Similar content being viewed by others
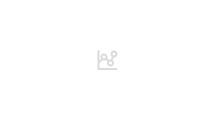
Guiding principles for the responsible development of artificial intelligence tools for healthcare
Kimberly Badal, Carmen M. Lee & Laura J. Esserman
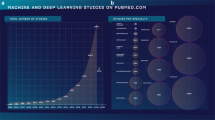
A short guide for medical professionals in the era of artificial intelligence
Bertalan Meskó & Marton Görög
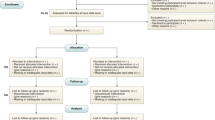
Reporting guidelines for clinical trial reports for interventions involving artificial intelligence: the CONSORT-AI extension
Xiaoxuan Liu, Samantha Cruz Rivera, … The SPIRIT-AI and CONSORT-AI Working Group
Introduction
Artificial intelligence (AI), supported by timely and accurate data and evidence, has the potential to transform health care delivery by improving health outcomes, patient safety, and the affordability and accessibility of high-quality care 1 , 2 . AI integration is critical to building an infrastructure capable of caring for an increasingly aging population, utilizing an ever-increasing knowledge of disease and options for precision treatments, and combatting workforce shortages and burnout of medical professionals. However, we are not currently on track to create this future. This is in part because the health data needed to train, test, use, and surveil these tools are generally neither standardized nor accessible. This is true across the international community, although there is variable progress within individual countries. There is also universal concern about monitoring health AI tools for changes in performance as they are implemented in new places, used with diverse populations, and over time as health data may change.
The Future of Health (FOH) is an international community of senior health care leaders representing health systems, health policy, health care technology, venture funding, insurance, and risk management. FOH collaborated with the Duke-Margolis Institute for Health Policy to conduct a literature review, expert convening, and consensus-building exercise. In total, 46 senior health care leaders were engaged in this work, from eleven countries in Europe, North America, Africa, Asia, and Australia. This commentary summarizes the four priority action areas and recommendations for health care organizations and policymakers that FOH members identified as important for fully realizing AI’s potential in health care: improving data quality to power AI, building infrastructure to encourage efficient and trustworthy development and evaluations, sharing data for better AI, and providing incentives to accelerate the progress and impact of AI.
Powering AI through high-quality data
“Going forward, data are going to be the most valuable commodity in health care. Organizations need robust plans about how to mobilize and use their data.”
AI algorithms will only perform as well as the accuracy and completeness of key underlying data, and data quality is dependent on actions and workflows that encourage trust.
To begin to improve data quality, FOH members agreed that an initial priority is identifying and assuring reliable availability of high-priority data elements for promising AI applications: those with the most predictive value, those of the highest value to patients, and those most important for analyses of performance, including subgroup analyses to detect bias.
Leaders should also advocate for aligned policy incentives to improve the availability and reliability of these priority data elements. There are several examples of efforts across the world to identify and standardize high-priority data elements for AI applications and beyond, such as the multinational project STANDING Together, which is developing standards to improve the quality and representativeness of data used to build and test AI tools 3 .
Policy incentives that would further encourage high-quality data collection include (1) aligned payment incentives for measures of health care quality and safety, and ensuring the reliability of the underlying data, and (2) quality measures and performance standards focused on the reliability, completeness, and timeliness of collection and sharing of high-priority data itself.
Trust and verify
“Your AI algorithms are only going to be as good as the data and the real-world evidence used to validate them, and the data are only going to be as good as the trust and privacy and supporting policies.”
FOH members stressed the importance of showing that AI tools are both effective and safe within their specific patient populations.
This is a particular challenge with AI tools, whose performance can differ dramatically across sites and over time, as health data patterns and population characteristics vary. For example, several studies of the Epic Sepsis Model found both location-based differences in performance and degradation in performance over time due to data drift 4 , 5 . However, real-world evaluations are often much more difficult for algorithms that are used for longer-term predictions, or to avert long-term complications from occurring, particularly in the absence of connected, longitudinal data infrastructure. As such, health systems must prioritize implementing data standards and data infrastructure that can facilitate the retraining or tuning of algorithms, test for local performance and bias, and ensure scalability across the organization and longer-term applications 6 .
There are efforts to help leaders and health systems develop consensus-based evaluation techniques and infrastructure for AI tools, including HealthAI: The Global Agency for Responsible AI in Health, which aims to build and certify validation mechanisms for nations and regions to adopt; and the Coalition for Health AI (CHAI), which recently announced plans to build a US-wide health AI assurance labs network 7 , 8 . These efforts, if successful, will assist manufacturers and health systems in complying with new laws, rules, and regulations being proposed and released that seek to ensure AI tools are trustworthy, such as the EU AI Act and the 2023 US Executive Order on AI.
Sharing data for better AI
“Underlying these challenges is the investment required to standardize business processes so that you actually get data that’s usable between institutions and even within an institution.”
While high-quality internal data may enable some types of AI-tool development and testing, this is insufficient to power and evaluate all AI applications. To build truly effective AI-enabled predictive software for clinical care and predictive supports, data often need to be interoperable across health systems to build a diverse picture of patients’ health across geographies, and reliably shared.
FOH members recommended that health care leaders work with researchers and policymakers to connect detailed encounter data with longitudinal outcomes, and pilot opportunities across diverse populations and systems to help assure valid outcome evaluations as well as address potential confounding and population subgroup differences—the ability to aggregate data is a clear rate-limiting step. The South African National Digital Health Strategy outlined interventions to improve the adoption of digital technologies while complying with the 2013 Protection of Personal Information Act 9 . Although challenges remain, the country has made progress on multiple fronts, including building out a Health Patient Registration System as a first step towards a portable, longitudinal patient record system and releasing a Health Normative Standards Framework to improve data flow across institutional and geographic boundaries 10 .
Leaders should adopt policies in their organizations, and encourage adoption in their province and country, that simplify data governance and sharing while providing appropriate privacy protections – including building foundations of trust with patients and the public as previously discussed. Privacy-preserving innovations include ways to “share” data without movement from protected systems using approaches like federated analyses, data sandboxes, or synthetic data. In addition to exploring privacy-preserving approaches to data sharing, countries and health systems may need to consider broad and dynamic approaches to consent 11 , 12 . As we look to a future where a patient may have thousands of algorithms churning away at their data, efforts to improve data quality and sharing should include enabling patients’ access to and engagement with their own data to encourage them to actively partner in their health and provide transparency on how their data are being used to improve health care. For example, the Understanding Patient Data program in the United Kingdom produces research and resources to explain how the National Health Service uses patients’ data 13 . Community engagement efforts can further assist with these efforts by building trust and expanding understanding.
FOH members also stressed the importance of timely data access. Health systems should work together to establish re-usable governance and privacy frameworks that allow stakeholders to clearly understand what data will be shared and how it will be protected to reduce the time needed for data use agreements. Trusted third-party data coordinating centers could also be used to set up “precertification” systems around data quality, testing, and cybersecurity to support health organizations with appropriate data stewardship to form partnerships and access data rapidly.
Incentivizing progress for AI impact
“Unless it’s tied to some kind of compensation to the organization, the drive to help implement those tools and overcome that risk aversion is going to be very high… I do think that business driver needs to be there.”
AI tools and data quality initiatives have not moved as quickly in health care due to the lack of direct payment, and often, misalignment of financial incentives and supports for high-quality data collection and predictive analytics. This affects both the ability to purchase and safely implement commercial AI products as well as the development of “homegrown” AI tools.
FOH members recommended that leaders should advocate for paying for value in health – quality, safety, better health, and lower costs for patients. This better aligns the financial incentives for accelerating the development, evaluation, and adoption of AI as well as other tools designed to either keep patients healthy or quickly diagnose and treat them with the most effective therapies when they do become ill. Effective personalized health care requires high-quality, standardized, interoperable datasets from diverse sources 14 . Within value-based payments themselves, data are critical to measuring quality of care and patient outcomes, adjusted or contextualized for factors outside of clinical control. Value-based payments therefore align incentives for (1) high-quality data collection and trusted use, (2) building effective AI tools, and (3) ensuring that those tools are improving patient outcomes and/or health system operations.
Data have become the most valuable commodity in health care, but questions remain about whether there will be an AI “revolution” or “evolution” in health care delivery. Early AI applications in certain clinical areas have been promising, but more advanced AI tools will require higher quality, real-world data that is interoperable and secure. The steps health care organization leaders and policymakers take in the coming years, starting with short-term opportunities to develop meaningful AI applications that achieve measurable improvements in outcomes and costs, will be critical in enabling this future that can improve health outcomes, safety, affordability, and equity.
Data availability
Data sharing is not applicable to this article as no datasets were generated or analyzed during the current study.
Abernethy, A. et al. The promise of digital health: then, now, and the future. NAM Perspect. 6 (2022).
Akpakwu, E. Four ways AI can make healthcare more efficient and affordable. World Economic Forum https://www.weforum.org/agenda/2018/05/four-ways-ai-is-bringing-down-the-cost-of-healthcare/ (2018).
STANDING Together. https://www.datadiversity.org/home .
Wong, A. et al. External validation of a widely implemented proprietary sepsis prediction model in hospitalized patients. JAMA Intern Med 181 , 1065–1070 (2021).
Article PubMed Google Scholar
Ross, C. STAT and MIT rooted out the weaknesses in health care algorithms. Here’s how we did it. STAT https://www.statnews.com/2022/02/28/data-drift-machine-learning/ (2022).
Locke, T., Parker, V., Thoumi, A., Goldstein, B. & Silcox, C. Preventing bias and inequities in AI-enabled health tools . https://healthpolicy.duke.edu/publications/preventing-bias-and-inequities-ai-enabled-health-tools (2022).
Introducing HealthAI. The International Digital Health and AI Research Collaborative (I-DAIR) https://www.i-dair.org/news/introducing-healthai (2023).
Shah, N. H. et al. A nationwide network of health AI assurance laboratories. JAMA 331 , 245 (2024).
Singh, V. AI & Data in South Africa’s Health Sector . https://policyaction.org.za/sites/default/files/PAN_TopicalGuide_AIData6_Health_Elec.pdf (2020).
Zharima, C., Griffiths, F. & Goudge, J. Exploring the barriers and facilitators to implementing electronic health records in a middle-income country: a qualitative study from South Africa. Front. Digit. Health 5 , 1207602 (2023).
Article PubMed PubMed Central Google Scholar
Lee, A. R. et al. Identifying facilitators of and barriers to the adoption of dynamic consent in digital health ecosystems: a scoping review. BMC Med. Ethics 24 , 107 (2023).
Article CAS PubMed PubMed Central Google Scholar
Stoeklé, H. C., Hulier-Ammar, E. & Hervé, C. Data medicine: ‘broad’ or ‘dynamic’ consent? Public Health Ethics 15 , 181–185 (2022).
Article Google Scholar
Understanding Patient Data. Understanding Patient Data http://understandingpatientdata.org.uk/ .
Chén, O. Y. & Roberts, B. Personalized health care and public health in the digital age. Front. Digit. Health 3 , 595704 (2021).
Download references
Acknowledgements
The authors acknowledge Oranit Ido and Jonathan Gonzalez-Smith for their contributions to this work. This study was funded by The Future of Health, LLC. The Future of Health, LLC, was involved in all stages of this research, including study design, data collection, analysis and interpretation of data, and the preparation of this manuscript.
Author information
Authors and affiliations.
Duke-Margolis Institute for Health Policy, Duke University, Washington, DC, USA &, Durham, NC, USA
Christina Silcox, Katie Huber, Neil Rowen, Robert Saunders & Mark McClellan
Sheba Medical Center, Ramat Gan, Israel
Eyal Zimlichmann
Future of Health, Washington, DC, USA
Eyal Zimlichmann, Charles N. Kahn III & Claudia A. Salzberg
Federation of American Hospitals, Washington, DC, USA
Charles N. Kahn III
Division of General Internal Medicine, Brigham and Women’s Hospital, Boston, MA, USA
David W. Bates
Harvard Medical School, Boston, MA, USA
Department of Health Policy and Management, Harvard T. H. Chan School of Public Health, Boston, MA, USA
You can also search for this author in PubMed Google Scholar
Contributions
C.S., K.H., N.R., and R.S. conducted initial background research and analyzed qualitative data from stakeholders. All authors (C.S., E.Z., K.H., N.R., R.S., M.M., C.K., C.A.S., and D.B.) assisted with conceptualization of the project and strategic guidance. C.S., K.H., and N.R. wrote initial drafts of the manuscript. All authors contributed to critical revisions of the manuscript and read and approved the final manuscript.
Corresponding author
Correspondence to David W. Bates .
Ethics declarations
Competing interests.
C.S., K.H., N.R., and C.A.S. declare no competing interests. E.Z. reports personal fees from Arkin Holdings, personal fees from Statista and equity from Valera Health, Profility and Hello Heart. R.S. has been an external reviewer for The John A. Hartford Foundation, and is a co-chair for the Health Evolution Summit Roundtable on Value-Based Care for Specialized Populations. M.M. is an independent director on the boards of Johnson & Johnson, Cigna, Alignment Healthcare, and PrognomIQ; co-chairs the Guiding Committee for the Health Care Payment Learning and Action Network; and reports fees for serving as an adviser for Arsenal Capital Partners, Blackstone Life Sciences, and MITRE. C.K. is a Profility Board member and additionally reports equity from Valera Health and MDClone. D.W.B. reports grants and personal fees from EarlySense, personal fees from CDI Negev, equity from Valera Health, equity from Clew, equity from MDClone, personal fees and equity from AESOP, personal fees and equity from Feelbetter, equity from Guided Clinical Solutions, and grants from IBM Watson Health, outside the submitted work. D.W.B. has a patent pending (PHC-028564 US PCT), on intraoperative clinical decision support.
Additional information
Publisher’s note Springer Nature remains neutral with regard to jurisdictional claims in published maps and institutional affiliations.
Rights and permissions
Open Access This article is licensed under a Creative Commons Attribution 4.0 International License, which permits use, sharing, adaptation, distribution and reproduction in any medium or format, as long as you give appropriate credit to the original author(s) and the source, provide a link to the Creative Commons licence, and indicate if changes were made. The images or other third party material in this article are included in the article’s Creative Commons licence, unless indicated otherwise in a credit line to the material. If material is not included in the article’s Creative Commons licence and your intended use is not permitted by statutory regulation or exceeds the permitted use, you will need to obtain permission directly from the copyright holder. To view a copy of this licence, visit http://creativecommons.org/licenses/by/4.0/ .
Reprints and permissions
About this article
Cite this article.
Silcox, C., Zimlichmann, E., Huber, K. et al. The potential for artificial intelligence to transform healthcare: perspectives from international health leaders. npj Digit. Med. 7 , 88 (2024). https://doi.org/10.1038/s41746-024-01097-6
Download citation
Received : 30 October 2023
Accepted : 29 March 2024
Published : 09 April 2024
DOI : https://doi.org/10.1038/s41746-024-01097-6
Share this article
Anyone you share the following link with will be able to read this content:
Sorry, a shareable link is not currently available for this article.
Provided by the Springer Nature SharedIt content-sharing initiative
Quick links
- Explore articles by subject
- Guide to authors
- Editorial policies
Sign up for the Nature Briefing newsletter — what matters in science, free to your inbox daily.


An official website of the United States government
The .gov means it’s official. Federal government websites often end in .gov or .mil. Before sharing sensitive information, make sure you’re on a federal government site.
The site is secure. The https:// ensures that you are connecting to the official website and that any information you provide is encrypted and transmitted securely.
- Publications
- Account settings
Preview improvements coming to the PMC website in October 2024. Learn More or Try it out now .
- Advanced Search
- Journal List
- BMC Med Inform Decis Mak

The role of artificial intelligence in healthcare: a structured literature review
Silvana secinaro.
1 Department of Management, University of Turin, Turin, Italy
Davide Calandra
Aurelio secinaro.
2 Ospedale Pediatrico Bambino Gesù, Rome, Italy
Vivek Muthurangu
3 Institute of Child Health, University College London, London, UK
Paolo Biancone
Associated data.
All the data are retrieved from public scientific platforms.
Background/Introduction
Artificial intelligence (AI) in the healthcare sector is receiving attention from researchers and health professionals. Few previous studies have investigated this topic from a multi-disciplinary perspective, including accounting, business and management, decision sciences and health professions.
The structured literature review with its reliable and replicable research protocol allowed the researchers to extract 288 peer-reviewed papers from Scopus. The authors used qualitative and quantitative variables to analyse authors, journals, keywords, and collaboration networks among researchers. Additionally, the paper benefited from the Bibliometrix R software package.
The investigation showed that the literature in this field is emerging. It focuses on health services management, predictive medicine, patient data and diagnostics, and clinical decision-making. The United States, China, and the United Kingdom contributed the highest number of studies. Keyword analysis revealed that AI can support physicians in making a diagnosis, predicting the spread of diseases and customising treatment paths.
Conclusions
The literature reveals several AI applications for health services and a stream of research that has not fully been covered. For instance, AI projects require skills and data quality awareness for data-intensive analysis and knowledge-based management. Insights can help researchers and health professionals understand and address future research on AI in the healthcare field.
Artificial intelligence (AI) generally applies to computational technologies that emulate mechanisms assisted by human intelligence, such as thought, deep learning, adaptation, engagement, and sensory understanding [ 1 , 2 ]. Some devices can execute a role that typically involves human interpretation and decision-making [ 3 , 4 ]. These techniques have an interdisciplinary approach and can be applied to different fields, such as medicine and health. AI has been involved in medicine since as early as the 1950s, when physicians made the first attempts to improve their diagnoses using computer-aided programs [ 5 , 6 ]. Interest and advances in medical AI applications have surged in recent years due to the substantially enhanced computing power of modern computers and the vast amount of digital data available for collection and utilisation [ 7 ]. AI is gradually changing medical practice. There are several AI applications in medicine that can be used in a variety of medical fields, such as clinical, diagnostic, rehabilitative, surgical, and predictive practices. Another critical area of medicine where AI is making an impact is clinical decision-making and disease diagnosis. AI technologies can ingest, analyse, and report large volumes of data across different modalities to detect disease and guide clinical decisions [ 3 , 8 ]. AI applications can deal with the vast amount of data produced in medicine and find new information that would otherwise remain hidden in the mass of medical big data [ 9 – 11 ]. These technologies can also identify new drugs for health services management and patient care treatments [ 5 , 6 ].
Courage in the application of AI is visible through a search in the primary research databases. However, as Meskò et al. [ 7 ] find, the technology will potentially reduce care costs and repetitive operations by focusing the medical profession on critical thinking and clinical creativity. As Cho et al. and Doyle et al. [ 8 , 9 ] add, the AI perspective is exciting; however, new studies will be needed to establish the efficacy and applications of AI in the medical field [ 10 ].
Our paper will also concentrate on AI strategies for healthcare from the accounting, business, and management perspectives. The authors used the structured literature review (SLR) method for its reliable and replicable research protocol [ 11 ] and selected bibliometric variables as sources of investigation. Bibliometric usage enables the recognition of the main quantitative variables of the study stream [ 12 ]. This method facilitates the detection of the required details of a particular research subject, including field authors, number of publications, keywords for interaction between variables (policies, properties and governance) and country data [ 13 ]. It also allows the application of the science mapping technique [ 14 ]. Our paper adopted the Bibliometrix R package and the biblioshiny web interface as tools of analysis [ 14 ].
The investigation offers the following insights for future researchers and practitioners:
- bibliometric information on 288 peer-reviewed English papers from the Scopus collection.
- Identification of leading journals in this field, such as Journal of Medical Systems, Studies in Health Technology and Informatics, IEEE Journal of Biomedical and Health Informatics, and Decision Support Systems.
- Qualitative and quantitative information on authors’ Lotka’s law, h-index, g-index, m-index, keyword, and citation data.
- Research on specific countries to assess AI in the delivery and effectiveness of healthcare, quotes, and networks within each region.
- A topic dendrogram study that identifies five research clusters: health services management, predictive medicine, patient data, diagnostics, and finally, clinical decision-making.
- An in-depth discussion that develops theoretical and practical implications for future studies.
The paper is organised as follows. Section 2 lists the main bibliometric articles in this field. Section 3 elaborates on the methodology. Section 4 presents the findings of the bibliometric analysis. Section 5 discusses the main elements of AI in healthcare based on the study results. Section 6 concludes the article with future implications for research.
Related works and originality
As suggested by Zupic and Čater [ 15 ], a research stream can be evaluated with bibliometric methods that can introduce objectivity and mitigate researcher bias. For this reason, bibliometric methods are attracting increasing interest among researchers as a reliable and impersonal research analytical approach [ 16 , 17 ]. Recently, bibliometrics has been an essential method for analysing and predicting research trends [ 18 ]. Table 1 lists other research that has used a similar approach in the research stream investigated.
List of research using bibliometric analysis.
Source : Authors’ elaboration
The scientific articles reported show substantial differences in keywords and research topics that have been previously studied. The bibliometric analysis of Huang et al. [ 19 ] describes rehabilitative medicine using virtual reality technology. According to the authors, the primary goal of rehabilitation is to enhance and restore functional ability and quality of life for patients with physical impairments or disabilities. In recent years, many healthcare disciplines have been privileged to access various technologies that provide tools for both research and clinical intervention.
Hao et al. [ 20 ] focus on text mining in medical research. As reported, text mining reveals new, previously unknown information by using a computer to automatically extract information from different text resources. Text mining methods can be regarded as an extension of data mining to text data. Text mining is playing an increasingly significant role in processing medical information. Similarly, the studies by dos Santos et al. [ 21 ] focus on applying data mining and machine learning (ML) techniques to public health problems. As stated in this research, public health may be defined as the art and science of preventing diseases, promoting health, and prolonging life. Using data mining and ML techniques, it is possible to discover new information that otherwise would be hidden. These two studies are related to another topic: medical big data. According to Liao et al. [ 22 ], big data is a typical “buzzword” in the business and research community, referring to a great mass of digital data collected from various sources. In the medical field, we can obtain a vast amount of data (i.e., medical big data). Data mining and ML techniques can help deal with this information and provide helpful insights for physicians and patients. More recently, Choudhury et al. [ 23 ] provide a systematic review on the use of ML to improve the care of elderly patients, demonstrating eligible studies primarily in psychological disorders and eye diseases.
Tran et al. [ 2 ] focus on the global evolution of AI research in medicine. Their bibliometric analysis highlights trends and topics related to AI applications and techniques. As stated in Connelly et al.’s [ 24 ] study, robot-assisted surgeries have rapidly increased in recent years. Their bibliometric analysis demonstrates how robotic-assisted surgery has gained acceptance in different medical fields, such as urological, colorectal, cardiothoracic, orthopaedic, maxillofacial and neurosurgery applications. Additionally, the bibliometric analysis of Guo et al. [ 25 ] provides an in-depth study of AI publications through December 2019. The paper focuses on tangible AI health applications, giving researchers an idea of how algorithms can help doctors and nurses. A new stream of research related to AI is also emerging. In this sense, Choudhury and Asan’s [ 26 ] scientific contribution provides a systematic review of the AI literature to identify health risks for patients. They report on 53 studies involving technology for clinical alerts, clinical reports, and drug safety. Considering the considerable interest within this research stream, this analysis differs from the current literature for several reasons. It aims to provide in-depth discussion, considering mainly the business, management, and accounting fields and not dealing only with medical and health profession publications.
Additionally, our analysis aims to provide a bibliometric analysis of variables such as authors, countries, citations and keywords to guide future research perspectives for researchers and practitioners, as similar analyses have done for several publications in other research streams [ 15 , 16 , 27 ]. In doing so, we use a different database, Scopus, that is typically adopted in social sciences fields. Finally, our analysis will propose and discuss a dominant framework of variables in this field, and our analysis will not be limited to AI application descriptions.
Methodology
This paper evaluated AI in healthcare research streams using the SLR method [ 11 ]. As suggested by Massaro et al. [ 11 ], an SLR enables the study of the scientific corpus of a research field, including the scientific rigour, reliability and replicability of operations carried out by researchers. As suggested by many scholars, the methodology allows qualitative and quantitative variables to highlight the best authors, journals and keywords and combine a systematic literature review and bibliometric analysis [ 27 – 30 ]. Despite its widespread use in business and management [ 16 , 31 ], the SLR is also used in the health sector based on the same philosophy through which it was originally conceived [ 32 , 33 ]. A methodological analysis of previously published articles reveals that the most frequently used steps are as follows [ 28 , 31 , 34 ]:
- defining research questions;
- writing the research protocol;
- defining the research sample to be analysed;
- developing codes for analysis; and
- critically analysing, discussing, and identifying a future research agenda.
Considering the above premises, the authors believe that an SLR is the best method because it combines scientific validity, replicability of the research protocol and connection between multiple inputs.
As stated by the methodological paper, the first step is research question identification. For this purpose, we benefit from the analysis of Zupic and Čater [ 15 ], who provide several research questions for future researchers to link the study of authors, journals, keywords and citations. Therefore, RQ1 is “What are the most prominent authors, journal keywords and citations in the field of the research study?” Additionally, as suggested by Haleem et al. [ 35 ], new technologies, including AI, are changing the medical field in unexpected timeframes, requiring studies in multiple areas. Therefore, RQ2 is “How does artificial intelligence relate to healthcare, and what is the focus of the literature?” Then, as discussed by Massaro et al. [ 36 ], RQ3 is “What are the research applications of artificial intelligence for healthcare?”.
The first research question aims to define the qualitative and quantitative variables of the knowledge flow under investigation. The second research question seeks to determine the state of the art and applications of AI in healthcare. Finally, the third research question aims to help researchers identify practical and theoretical implications and future research ideas in this field.
The second fundamental step of the SLR is writing the research protocol [ 11 ]. Table 2 indicates the currently known literature elements, uniquely identifying the research focus, motivations and research strategy adopted and the results providing a link with the following points. Additionally, to strengthen the analysis, our investigation benefits from the PRISMA statement methodological article [ 37 ]. Although the SLR is a validated method for systematic reviews and meta-analyses, we believe that the workflow provided may benefit the replicability of the results [ 37 – 40 ]. Figure 1 summarises the researchers’ research steps, indicating that there are no results that can be referred to as a meta-analysis.
SLR protocol and results obtained from Scopus.

PRISMA workflow.
Source : Authors’ elaboration on Liberati et al. [ 37 ]
The third step is to specify the search strategy and search database. Our analysis is based on the search string “Artificial Intelligence” OR “AI” AND “Healthcare” with a focus on “Business, Management, and Accounting”, “Decision Sciences”, and “Health professions”. As suggested by [ 11 , 41 ] and motivated by [ 42 ], keywords can be selected through a top-down approach by identifying a large search field and then focusing on particular sub-topics. The paper uses data retrieved from the Scopus database, a multi-disciplinary database, which allowed the researchers to identify critical articles for scientific analysis [ 43 ]. Additionally, Scopus was selected based on Guo et al.’s [ 25 ] limitations, which suggest that “future studies will apply other databases, such as Scopus, to explore more potential papers” . The research focuses on articles and reviews published in peer-reviewed journals for their scientific relevance [ 11 , 16 , 17 , 29 ] and does not include the grey literature, conference proceedings or books/book chapters. Articles written in any language other than English were excluded [ 2 ]. For transparency and replicability, the analysis was conducted on 11 January 2021. Using this research strategy, the authors retrieved 288 articles. To strengthen the study's reliability, we publicly provide the full bibliometric extract on the Zenodo repository [ 44 , 45 ].
The fourth research phase is defining the code framework that initiates the analysis of the variables. The study will identify the following:
- descriptive information of the research area;
- source analysis [ 16 ];
- author and citation analysis [ 28 ];
- keywords and network analysis [ 14 ]; and
- geographic distribution of the papers [ 14 ].
The final research phase is the article’s discussion and conclusion, where implications and future research trends will be identified.
At the research team level, the information is analysed with the statistical software R-Studio and the Bibliometrix package [ 15 ], which allows scientific analysis of the results obtained through the multi-disciplinary database.
The analysis of bibliometric results starts with a description of the main bibliometric statistics with the aim of answering RQ1, What are the most prominent authors, journal keywords and citations in the field of the research study?, and RQ2, How does artificial intelligence relate to healthcare, and what is the focus of the literature? Therefore, the following elements were thoroughly analysed: (1) type of document; (2) annual scientific production; (3) scientific sources; (4) source growth; (5) number of articles per author; (6) author’s dominance ranking; (7) author’s h-index, g-index, and m-index; (8) author’s productivity; (9) author’s keywords; (10) topic dendrogram; (11) a factorial map of the document with the highest contributions; (12) article citations; (13) country production; (14) country citations; (15) country collaboration map; and (16) country collaboration network.
Main information
Table 3 shows the information on 288 peer-reviewed articles published between 1992 and January 2021 extracted from the Scopus database. The number of keywords is 946 from 136 sources, and the number of keywords plus, referring to the number of keywords that frequently appear in an article’s title, was 2329. The analysis period covered 28 years and 1 month of scientific production and included an annual growth rate of 5.12%. However, the most significant increase in published articles occurred in the past three years (please see Fig. 2 ). On average, each article was written by three authors (3.56). Finally, the collaboration index (CI), which was calculated as the total number of authors of multi-authored articles/total number of multi-authored articles, was 3.97 [ 46 ].
Main information.

Annual scientific production.
Table 4 shows the top 20 sources related to the topic. The Journal of Medical Systems is the most relevant source, with twenty-one of the published articles. This journal's main issues are the foundations, functionality, interfaces, implementation, impacts, and evaluation of medical technologies. Another relevant source is Studies in Health Technology and Informatics, with eleven articles. This journal aims to extend scientific knowledge related to biomedical technologies and medical informatics research. Both journals deal with cloud computing, machine learning, and AI as a disruptive healthcare paradigm based on recent publications. The IEEE Journal of Biomedical and Health Informatics investigates technologies in health care, life sciences, and biomedicine applications from a broad perspective. The next journal, Decision Support Systems, aims to analyse how these technologies support decision-making from a multi-disciplinary view, considering business and management. Therefore, the analysis of the journals revealed that we are dealing with an interdisciplinary research field. This conclusion is confirmed, for example, by the presence of purely medical journals, journals dedicated to the technological growth of healthcare, and journals with a long-term perspective such as futures.
Main twenty sources.
The distribution frequency of the articles (Fig. 3 ) indicates the journals dealing with the topic and related issues. Between 2008 and 2012, a significant growth in the number of publications on the subject is noticeable. However, the graph shows the results of the Loess regression, which includes the quantity and publication time of the journal under analysis as variables. This method allows the function to assume an unlimited distribution; that is, feature can consider values below zero if the data are close to zero. It contributes to a better visual result and highlights the discontinuity in the publication periods [ 47 ].

Source growth. Source : Authors’ elaboration
Finally, Fig. 4 provides an analytical perspective on factor analysis for the most cited papers. As indicated in the literature [ 48 , 49 ], using factor analysis to discover the most cited papers allows for a better understanding of the scientific world’s intellectual structure. For example, our research makes it possible to consider certain publications that effectively analyse subject specialisation. For instance, Santosh’s [ 50 ] article addresses the new paradigm of AI with ML algorithms for data analysis and decision support in the COVID-19 period, setting a benchmark in terms of citations by researchers. Moving on to the application, an article by Shickel et al. [ 51 ] begins with the belief that the healthcare world currently has much health and administrative data. In this context, AI and deep learning will support medical and administrative staff in extracting data, predicting outcomes, and learning medical representations. Finally, in the same line of research, Baig et al. [ 52 ], with a focus on wearable patient monitoring systems (WPMs), conclude that AI and deep learning may be landmarks for continuous patient monitoring and support for healthcare delivery.

Factorial map of the most cited documents.
This section identifies the most cited authors of articles on AI in healthcare. It also identifies the authors’ keywords, dominance factor (DF) ranking, h-index, productivity, and total number of citations. Table 5 identifies the authors and their publications in the top 20 rankings. As the table shows, Bushko R.G. has the highest number of publications: four papers. He is the editor-in-chief of Future of Health Technology, a scientific journal that aims to develop a clear vision of the future of health technology. Then, several authors each wrote three papers. For instance, Liu C. is a researcher active in the topic of ML and computer vision, and Sharma A. from Emory University Atlanta in the USA is a researcher with a clear focus on imaging and translational informatics. Some other authors have two publications each. While some authors have published as primary authors, most have published as co-authors. Hence, in the next section, we measure the contributory power of each author by investigating the DF ranking through the number of elements.
Most cited authors.
Authors’ dominance ranking
The dominance factor (DF) is a ratio measuring the fraction of multi-authored articles in which an author acts as the first author [ 53 ]. Several bibliometric studies use the DF in their analyses [ 46 , 54 ]. The DF ranking calculates an author’s dominance in producing articles. The DF is calculated by dividing the number of an author’s multi-authored papers as the first author (Nmf) by the author's total number of multi-authored papers (Nmt). This is omitted in the single-author case due to the constant value of 1 for single-authored articles. This formulation could lead to some distortions in the results, especially in fields where the first author is entered by surname alphabetical order [ 55 ].
The mathematical equation for the DF is shown as:
Table 6 lists the top 20 DF rankings. The data in the table show a low level of articles per author, either for first-authored or multi-authored articles. The results demonstrate that we are dealing with an emerging topic in the literature. Additionally, as shown in the table, Fox J. and Longoni C. are the most dominant authors in the field.
Authors dominance factor.
Authors’ impact
Table 7 shows the impact of authors in terms of the h-index [ 56 ] (i.e., the productivity and impact of citations of a researcher), g-index [ 57 ] (i.e., the distribution of citations received by a researcher's publications), m-index [ 58 ] (i.e., the h-index value per year), total citations, total paper and years of scientific publication. The H-index was introduced in the literature as a metric for the objective comparison of scientific results and depended on the number of publications and their impact [ 59 ]. The results show that the 20 most relevant authors have an h-index between 2 and 1. For the practical interpretation of the data, the authors considered data published by the London School of Economics [ 60 ]. In the social sciences, the analysis shows values of 7.6 for economic publications by professors and researchers who had been active for several years. Therefore, the youthfulness of the research area has attracted young researchers and professors. At the same time, new indicators have emerged over the years to diversify the logic of the h-index. For example, the g-index indicates an author's impact on citations, considering that a single article can generate these. The m-index, on the other hand, shows the cumulative value over the years.
Authors impact.
The analysis, also considering the total number of citations, the number of papers published and the year of starting to publish, thus confirms that we are facing an expanding research flow.
Authors’ productivity
Figure 5 shows Lotka’s law. This mathematical formulation originated in 1926 to describe the publication frequency by authors in a specific research field [ 61 ]. In practice, the law states that the number of authors contributing to research in a given period is a fraction of the number who make up a single contribution [ 14 , 61 ].

Lotka’s law.
The mathematical relationship is expressed in reverse in the following way:
where y x is equal to the number of authors producing x articles in each research field. Therefore, C and n are constants that can be estimated in the calculation.
The figure's results are in line with Lotka's results, with an average of two publications per author in a given research field. In addition, the figure shows the percentage of authors. Our results lead us to state that we are dealing with a young and growing research field, even with this analysis. Approximately 70% of the authors had published only their first research article. Only approximately 20% had published two scientific papers.
Authors’ keywords
This section provides information on the relationship between the keywords artificial intelligence and healthcare . This analysis is essential to determine the research trend, identify gaps in the discussion on AI in healthcare, and identify the fields that can be interesting as research areas [ 42 , 62 ].
Table 8 highlights the total number of keywords per author in the top 20 positions. The ranking is based on the following elements: healthcare, artificial intelligence, and clinical decision support system . Keyword analysis confirms the scientific area of reference. In particular, we deduce the definition as “Artificial intelligence is the theory and development of computer systems able to perform tasks normally requiring human intelligence, such as visual perception, speech recognition, decision-making, and translation between languages” [ 2 , 63 ]. Panch et al. [ 4 ] find that these technologies can be used in different business and management areas. After the first keyword, the analysis reveals AI applications and related research such as machine learning and deep learning.
Author’s keywords in articles on artificial intelligence in healthcare.
Additionally, data mining and big data are a step forward in implementing exciting AI applications. According to our specific interest, if we applied AI in healthcare, we would achieve technological applications to help and support doctors and medical researchers in decision-making. The link between AI and decision-making is the reason why we find, in the seventh position, the keyword clinical decision support system . AI techniques can unlock clinically relevant information hidden in the massive amount of data that can assist clinical decision-making [ 64 ]. If we analyse the following keywords, we find other elements related to decision-making and support systems.
The TreeMap below (Fig. 6 ) highlights the combination of possible keywords representing AI and healthcare.

Keywords treemap.
The topic dendrogram in Fig. 7 represents the hierarchical order and the relationship between the keywords generated by hierarchical clustering [ 42 ]. The cut in the figure and the vertical lines facilitate an investigation and interpretation of the different clusters. As stated by Andrews [ 48 ], the figure is not intended to find the perfect level of associations between clusters. However, it aims to estimate the approximate number of clusters to facilitate further discussion.

Topic dendrogram.
The research stream of AI in healthcare is divided into two main strands. The blue strand focuses on medical information systems and the internet. Some papers are related to healthcare organisations, such as the Internet of Things, meaning that healthcare organisations use AI to support health services management and data analysis. AI applications are also used to improve diagnostic and therapeutic accuracy and the overall clinical treatment process [ 2 ]. If we consider the second block, the red one, three different clusters highlight separate aspects of the topic. The first could be explained as AI and ML predictive algorithms. Through AI applications, it is possible to obtain a predictive approach that can ensure that patients are better monitored. This also allows a better understanding of risk perception for doctors and medical researchers. In the second cluster, the most frequent words are decisions , information system , and support system . This means that AI applications can support doctors and medical researchers in decision-making. Information coming from AI technologies can be used to consider difficult problems and support a more straightforward and rapid decision-making process. In the third cluster, it is vital to highlight that the ML model can deal with vast amounts of data. From those inputs, it can return outcomes that can optimise the work of healthcare organisations and scheduling of medical activities.
Furthermore, the word cloud in Fig. 8 highlights aspects of AI in healthcare, such as decision support systems, decision-making, health services management, learning systems, ML techniques and diseases. The figure depicts how AI is linked to healthcare and how it is used in medicine.

Word cloud.
Figure 9 represents the search trends based on the keywords analysed. The research started in 2012. First, it identified research topics related to clinical decision support systems. This topic was recurrent during the following years. Interestingly, in 2018, studies investigated AI and natural language processes as possible tools to manage patients and administrative elements. Finally, a new research stream considers AI's role in fighting COVID-19 [ 65 , 66 ].

Keywords frequency.
Table 9 represents the number of citations from other articles within the top 20 rankings. The analysis allows the benchmark studies in the field to be identified [ 48 ]. For instance, Burke et al. [ 67 ] writes the most cited paper and analyses efficient nurse rostering methodologies. The paper critically evaluates tangible interdisciplinary solutions that also include AI. Immediately thereafter, Ahmed M.A.'s article proposes a data-driven optimisation methodology to determine the optimal number of healthcare staff to optimise patients' productivity [ 68 ]. Finally, the third most cited article lays the groundwork for developing deep learning by considering diverse health and administrative information [ 51 ].
Authors and sources citations.
This section analyses the diffusion of AI in healthcare around the world. It highlights countries to show the geographies of this research. It includes all published articles, the total number of citations, and the collaboration network. The following sub-sections start with an analysis of the total number of published articles.
Country total articles
Figure 9 and Table 10 display the countries where AI in healthcare has been considered. The USA tops the list of countries with the maximum number of articles on the topic (215). It is followed by China (83), the UK (54), India (51), Australia (54), and Canada (32). It is immediately evident that the theme has developed on different continents, highlighting a growing interest in AI in healthcare. The figure shows that many areas, such as Russia, Eastern Europe and Africa except for Algeria, Egypt, and Morocco, have still not engaged in this scientific debate.
Total number of articles per country.
Country publications and collaboration map
This section discusses articles on AI in healthcare in terms of single or multiple publications in each country. It also aims to observe collaboration and networking between countries. Table 11 and Fig. 10 highlight the average citations by state and show that the UK, the USA, and Kuwait have a higher average number of citations than other countries. Italy, Spain and New Zealand have the most significant number of citations.
Country and their total number of citations.

Articles per country.
Figure 11 depicts global collaborations. The blue colour on the map represents research cooperation among nations. Additionally, the pink border linking states indicates the extent of collaboration between authors. The primary cooperation between nations is between the USA and China, with two collaborative articles. Other collaborations among nations are limited to a few papers.

Collaboration map.
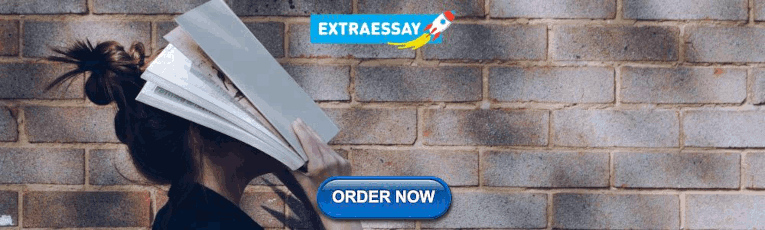
Artificial intelligence for healthcare: applications
This section aims to strengthen the research scope by answering RQ3: What are the research applications of artificial intelligence for healthcare?
Benefiting from the topical dendrogram, researchers will provide a development model based on four relevant variables [ 69 , 70 ]. AI has been a disruptive innovation in healthcare [ 4 ]. With its sophisticated algorithms and several applications, AI has assisted doctors and medical professionals in the domains of health information systems, geocoding health data, epidemic and syndromic surveillance, predictive modelling and decision support, and medical imaging [ 2 , 9 , 10 , 64 ]. Furthermore, the researchers considered the bibliometric analysis to identify four macro-variables dominant in the field and used them as authors' keywords. Therefore, the following sub-sections aim to explain the debate on applications in healthcare for AI techniques. These elements are shown in Fig. 12 .

Dominant variables for AI in healthcare.
Health services management
One of the notable aspects of AI techniques is potential support for comprehensive health services management. These applications can support doctors, nurses and administrators in their work. For instance, an AI system can provide health professionals with constant, possibly real-time medical information updates from various sources, including journals, textbooks, and clinical practices [ 2 , 10 ]. These applications' strength is becoming even more critical in the COVID-19 period, during which information exchange is continually needed to properly manage the pandemic worldwide [ 71 ]. Other applications involve coordinating information tools for patients and enabling appropriate inferences for health risk alerts and health outcome prediction [ 72 ]. AI applications allow, for example, hospitals and all health services to work more efficiently for the following reasons:
- Clinicians can access data immediately when they need it.
- Nurses can ensure better patient safety while administering medication.
- Patients can stay informed and engaged in their care by communicating with their medical teams during hospital stays.
Additionally, AI can contribute to optimising logistics processes, for instance, realising drugs and equipment in a just-in-time supply system based totally on predictive algorithms [ 73 , 74 ]. Interesting applications can also support the training of personnel working in health services. This evidence could be helpful in bridging the gap between urban and rural health services [ 75 ]. Finally, health services management could benefit from AI to leverage the multiplicity of data in electronic health records by predicting data heterogeneity across hospitals and outpatient clinics, checking for outliers, performing clinical tests on the data, unifying patient representation, improving future models that can predict diagnostic tests and analyses, and creating transparency with benchmark data for analysing services delivered [ 51 , 76 ].
Predictive medicine
Another relevant topic is AI applications for disease prediction and diagnosis treatment, outcome prediction and prognosis evaluation [ 72 , 77 ]. Because AI can identify meaningful relationships in raw data, it can support diagnostic, treatment and prediction outcomes in many medical situations [ 64 ]. It allows medical professionals to embrace the proactive management of disease onset. Additionally, predictions are possible for identifying risk factors and drivers for each patient to help target healthcare interventions for better outcomes [ 3 ]. AI techniques can also help design and develop new drugs, monitor patients and personalise patient treatment plans [ 78 ]. Doctors benefit from having more time and concise data to make better patient decisions. Automatic learning through AI could disrupt medicine, allowing prediction models to be created for drugs and exams that monitor patients over their whole lives [ 79 ].
Clinical decision-making
One of the keyword analysis main topics is that AI applications could support doctors and medical researchers in the clinical decision-making process. According to Jiang et al. [ 64 ], AI can help physicians make better clinical decisions or even replace human judgement in healthcare-specific functional areas. According to Bennett and Hauser [ 80 ], algorithms can benefit clinical decisions by accelerating the process and the amount of care provided, positively impacting the cost of health services. Therefore, AI technologies can support medical professionals in their activities and simplify their jobs [ 4 ]. Finally, as Redondo and Sandoval [ 81 ] find, algorithmic platforms can provide virtual assistance to help doctors understand the semantics of language and learning to solve business process queries as a human being would.
Patient data and diagnostics
Another challenging topic related to AI applications is patient data and diagnostics. AI techniques can help medical researchers deal with the vast amount of data from patients (i.e., medical big data ). AI systems can manage data generated from clinical activities, such as screening, diagnosis, and treatment assignment. In this way, health personnel can learn similar subjects and associations between subject features and outcomes of interest [ 64 ].
These technologies can analyse raw data and provide helpful insights that can be used in patient treatments. They can help doctors in the diagnostic process; for example, to realise a high-speed body scan, it will be simpler to have an overall patient condition image. Then, AI technology can recreate a 3D mapping solution of a patient’s body.
In terms of data, interesting research perspectives are emerging. For instance, we observed the emergence of a stream of research on patient data management and protection related to AI applications [ 82 ].
For diagnostics, AI techniques can make a difference in rehabilitation therapy and surgery. Numerous robots have been designed to support and manage such tasks. Rehabilitation robots physically support and guide, for example, a patient’s limb during motor therapy [ 83 ]. For surgery, AI has a vast opportunity to transform surgical robotics through devices that can perform semi-automated surgical tasks with increasing efficiency. The final aim of this technology is to automate procedures to negate human error while maintaining a high level of accuracy and precision [ 84 ]. Finally, the -19 period has led to increased remote patient diagnostics through telemedicine that enables remote observation of patients and provides physicians and nurses with support tools [ 66 , 85 , 86 ].
This study aims to provide a bibliometric analysis of publications on AI in healthcare, focusing on accounting, business and management, decision sciences and health profession studies. Using the SLR method of Massaro et al. [ 11 ], we provide a reliable and replicable research protocol for future studies in this field. Additionally, we investigate the trend of scientific publications on the subject, unexplored information, future directions, and implications using the science mapping workflow. Our analysis provides interesting insights.
In terms of bibliometric variables, the four leading journals, Journal of Medical Systems , Studies in Health Technology and Informatics , IEEE Journal of Biomedical and Health Informatics , and Decision Support Systems , are optimal locations for the publication of scientific articles on this topic. These journals deal mainly with healthcare, medical information systems, and applications such as cloud computing, machine learning, and AI. Additionally, in terms of h-index, Bushko R.G. and Liu C. are the most productive and impactful authors in this research stream. Burke et al.’s [ 67 ] contribution is the most cited with an analysis of nurse rostering using new technologies such as AI. Finally, in terms of keywords, co-occurrence reveals some interesting insights. For instance, researchers have found that AI has a role in diagnostic accuracy and helps in the analysis of health data by comparing thousands of medical records, experiencing automatic learning with clinical alerts, efficient management of health services and places of care, and the possibility of reconstructing patient history using these data.
Second, this paper finds five cluster analyses in healthcare applications: health services management, predictive medicine, patient data, diagnostics, and finally, clinical decision-making. These technologies can also contribute to optimising logistics processes in health services and allowing a better allocation of resources.
Third, the authors analysing the research findings and the issues under discussion strongly support AI's role in decision support. These applications, however, are demonstrated by creating a direct link to data quality management and the technology awareness of health personnel [ 87 ].
The importance of data quality for the decision-making process
Several authors have analysed AI in the healthcare research stream, but in this case, the authors focus on other literature that includes business and decision-making processes. In this regard, the analysis of the search flow reveals a double view of the literature. On the one hand, some contributions belong to the positivist literature and embrace future applications and implications of technology for health service management, data analysis and diagnostics [ 6 , 80 , 88 ]. On the other hand, some investigations also aim to understand the darker sides of technology and its impact. For example, as Carter [ 89 ] states, the impact of AI is multi-sectoral; its development, however, calls for action to protect personal data. Similarly, Davenport and Kalakota [ 77 ] focus on the ethical implications of using AI in healthcare. According to the authors, intelligent machines raise issues of accountability, transparency, and permission, especially in automated communication with patients. Our analysis does not indicate a marked strand of the literature; therefore, we argue that the discussion of elements such as the transparency of technology for patients is essential for the development of AI applications.
A large part of our results shows that, at the application level, AI can be used to improve medical support for patients (Fig. 11 ) [ 64 , 82 ]. However, we believe that, as indicated by Kalis et al. [ 90 ] on the pages of Harvard Business Review, the management of costly back-office problems should also be addressed.
The potential of algorithms includes data analysis. There is an immense quantity of data accessible now, which carries the possibility of providing information about a wide variety of medical and healthcare activities [ 91 ]. With the advent of modern computational methods, computer learning and AI techniques, there are numerous possibilities [ 79 , 83 , 84 ]. For example, AI makes it easier to turn data into concrete and actionable observations to improve decision-making, deliver high-quality patient treatment, adapt to real-time emergencies, and save more lives on the clinical front. In addition, AI makes it easier to leverage capital to develop systems and facilities and reduce expenses at the organisational level [ 78 ]. Studying contributions to the topic, we noticed that data accuracy was included in the debate, indicating that a high standard of data will benefit decision-making practitioners [ 38 , 77 ]. AI techniques are an essential instrument for studying data and the extraction of medical insight, and they may assist medical researchers in their practices. Using computational tools, healthcare stakeholders may leverage the power of data not only to evaluate past data ( descriptive analytics ) but also to forecast potential outcomes ( predictive analytics ) and to define the best actions for the present scenario ( prescriptive analytics ) [ 78 ]. The current abundance of evidence makes it easier to provide a broad view of patient health; doctors should have access to the correct details at the right time and location to provide the proper treatment [ 92 ].
Will medical technology de-skill doctors?
Further reflection concerns the skills of doctors. Studies have shown that healthcare personnel are progressively being exposed to technology for different purposes, such as collecting patient records or diagnosis [ 71 ]. This is demonstrated by the keywords (Fig. 6 ) that focus on technology and the role of decision-making with new innovative tools. In addition, the discussion expands with Lu [ 93 ], which indicates that the excessive use of technology could hinder doctors’ skills and clinical procedures' expansion. Among the main issues arising from the literature is the possible de-skilling of healthcare staff due to reduced autonomy in decision-making concerning patients [ 94 ]. Therefore, the challenges and discussion we uncovered in Fig. 11 are expanded by also considering the ethical implications of technology and the role of skills.
Implications
Our analysis also has multiple theoretical and practical implications.
In terms of theoretical contribution, this paper extends the previous results of Connelly et al., dos Santos et al, Hao et al., Huang et al., Liao et al. and Tran et al. [ 2 , 19 – 22 , 24 ] in considering AI in terms of clinical decision-making and data management quality.
In terms of practical implications, this paper aims to create a fruitful discussion with healthcare professionals and administrative staff on how AI can be at their service to increase work quality. Furthermore, this investigation offers a broad comprehension of bibliometric variables of AI techniques in healthcare. It can contribute to advancing scientific research in this field.
Limitations
Like any other, our study has some limitations that could be addressed by more in-depth future studies. For example, using only one research database, such as Scopus, could be limiting. Further analysis could also investigate the PubMed, IEEE, and Web of Science databases individually and holistically, especially the health parts. Then, the use of search terms such as "Artificial Intelligence" OR "AI" AND "Healthcare" could be too general and exclude interesting studies. Moreover, although we analysed 288 peer-reviewed scientific papers, because the new research topic is new, the analysis of conference papers could return interesting results for future researchers. Additionally, as this is a young research area, the analysis will be subject to recurrent obsolescence as multiple new research investigations are published. Finally, although bibliometric analysis has limited the subjectivity of the analysis [ 15 ], the verification of recurring themes could lead to different results by indicating areas of significant interest not listed here.
Future research avenues
Concerning future research perspectives, researchers believe that an analysis of the overall amount that a healthcare organisation should pay for AI technologies could be helpful. If these technologies are essential for health services management and patient treatment, governments should invest and contribute to healthcare organisations' modernisation. New investment funds could be made available in the healthcare world, as in the European case with the Next Generation EU programme or national investment programmes [ 95 ]. Additionally, this should happen especially in the poorest countries around the world, where there is a lack of infrastructure and services related to health and medicine [ 96 ]. On the other hand, it might be interesting to evaluate additional profits generated by healthcare organisations with AI technologies compared to those that do not use such technologies.
Further analysis could also identify why some parts of the world have not conducted studies in this area. It would be helpful to carry out a comparative analysis between countries active in this research field and countries that are not currently involved. It would make it possible to identify variables affecting AI technologies' presence or absence in healthcare organisations. The results of collaboration between countries also present future researchers with the challenge of greater exchanges between researchers and professionals. Therefore, further research could investigate the difference in vision between professionals and academics.
In the accounting, business, and management research area, there is currently a lack of quantitative analysis of the costs and profits generated by healthcare organisations that use AI technologies. Therefore, research in this direction could further increase our understanding of the topic and the number of healthcare organisations that can access technologies based on AI. Finally, as suggested in the discussion section, more interdisciplinary studies are needed to strengthen AI links with data quality management and AI and ethics considerations in healthcare.
In pursuing the philosophy of Massaro et al.’s [ 11 ] methodological article, we have climbed on the shoulders of giants, hoping to provide a bird's-eye view of the AI literature in healthcare. We performed this study with a bibliometric analysis aimed at discovering authors, countries of publication and collaboration, and keywords and themes. We found a fast-growing, multi-disciplinary stream of research that is attracting an increasing number of authors.
The research, therefore, adopts a quantitative approach to the analysis of bibliometric variables and a qualitative approach to the study of recurring keywords, which has allowed us to demonstrate strands of literature that are not purely positive. There are currently some limitations that will affect future research potential, especially in ethics, data governance and the competencies of the health workforce.
Acknowledgements
The authors are grateful to the Editor-in-Chief for the suggestions and all the reviewers who spend a part of their time ensuring constructive feedback to our research article.
Authors' contributions
SS and PB, Supervision; Validation, writing, AS and VM; Formal analysis, DC and AS; Methodology, DC; Writing; DC, SS and AS; conceptualization, VM, PB; validation, VM, PB. All authors read and approved the final manuscript.
This research received no specific grant from any funding agency in the public, commercial, or not-for-profit sectors.
Availability of data and materials
Declarations.
Not applicable.
The authors declare that they have no known competing financial interests or personal relationships that could have appeared to influence the work reported in this paper.
Publisher's Note
Springer Nature remains neutral with regard to jurisdictional claims in published maps and institutional affiliations.
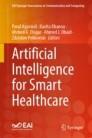
- © 2023
Artificial Intelligence for Smart Healthcare
- Parul Agarwal ORCID: https://orcid.org/0000-0002-7297-335X 0 ,
- Kavita Khanna 1 ,
- Ahmed A Elngar 2 ,
- Ahmed J. Obaid 3 ,
- Zdzislaw Polkowski 4
Computer Science and Engineering, Jamia Hamdard, New Delhi, India
You can also search for this editor in PubMed Google Scholar
Delhi Skill and Entrepreneurship University, Dwarka, India
Faculty of computers and artificial intelligence, beni-suef university, beni-suef city, egypt, faculty of computer science and mathematics, university of kufa, najaf, iraq, jan wyzykowski university, polkowice, poland.
- Provides insight into communication technologies that govern current healthcare sector
- Includes a comprehensive introduction of artificial intelligence in healthcare
- Discusses various machine learning algorithms applied in the field of medicine
Part of the book series: EAI/Springer Innovations in Communication and Computing (EAISICC)
17k Accesses
21 Citations
- Table of contents
About this book
Editors and affiliations, about the editors, bibliographic information.
- Publish with us
Buying options
- Available as EPUB and PDF
- Read on any device
- Instant download
- Own it forever
- Durable hardcover edition
- Dispatched in 3 to 5 business days
- Free shipping worldwide - see info
Tax calculation will be finalised at checkout
Other ways to access
This is a preview of subscription content, log in via an institution to check for access.
Table of contents (30 chapters)
Front matter, fundamentals and applications of ai and enabling technologies in various sectors, a secured data sharing protocol for minimisation of risk in cloud computing and big data in ai application.
- Vijay Prakash Sharma, Kailas S. Patil, G. Pavithra, Sangeetha Krishnan, Anju Asokan
Predictive Modelling for Healthcare Decision-Making Using IoT with Machine Learning Models
- Rajasekar Rangasamy, Thayyaba Khatoon Mohammed, Mageshkumar Chinnaswamy, Ramachandran Veerachamy
Artificial Intelligence for Smart in Match Winning Prediction in Twenty20 Cricket League Using Machine Learning Model
- Maheswari Subburaj, Ganga Rama Koteswara Rao, Binayak Parashar, Indirapriyadharshini Jeyabalan, Hemalatha Semban, Sudhakar Sengan
Comparative Analysis of Handwritten Digit Recognition Investigation Using Deep Learning Model
- Joel Sunny Deol Gosu, Balu Subramaniam, Sasipriyaa Nachimuthu, Kamalanathan Shivasankaran, Arjun Subburaj, Sudhakar Sengan
An Investigation of Machine Learning-Based IDS for Green Smart Transportation in MANET
- C. Edwin Singh, J. Amar Pratap Singh
A Critical Cloud Security Risks Detection Using Artificial Neural Networks at Banking Sector
- R. Velmurugan, R. Kumar, D. Saravanan, Sumagna Patnaik, Siva Kishore Ikkurthi
A Solution to Pose Change Challenge: Real-Time, Robust, and Adaptive Human Tracking Systems Using SURF
- Anshul Pareek, Poonam Kadian
Analysis on Identification and Detection of Forgery in Handwritten Signature Using CNN
- T. Vasudeva Reddy, D. Harikrishna, V. Hindumathi, P. Asha Rani, T. Keerthi
Experimental Analysis of Internet of Technology-Enabled Smart Irrigation System
- Ramachandran Veerachamy, R. Ramalakshmi, C. Mageshkumar, Rajasekar Rangasamy
Analysis on Exposition of Speech Type Video Using SSD and CNN Techniques for Face Detection
- Y. M. Manu, Chetana Prakash, S. Santhosh, Shaik Shafi, K. Shruthi
AI-Enabled Innovations in the Health Sector
Depressive disorder prediction using machine learning-based electroencephalographic signal.
- Govinda Rajulu Ganiga, Kalvikkarasi Subramani, Dilip Kumar Sharma, Sudhakar Sengan, Kalaiyarasi Anbalagan, Priyadarsini Seenivasan
Generation of Masks Using nnU-Net Framework for Brain Tumour Classification
- G. Jaya Lakshmi, Mangesh Ghonge, Ahmed J. Obaid, Muthmainnah
A Brain Seizure Diagnosing Remotely Based on EEG Signal Compression and Encryption: A Step for Telehealth
- Shokhan M. Al-Barzinji, M. N. Saif Al-din, Azmi Shawkat Abdulbaqi, Bharat Bhushan, Ahmed J. Obaid
A Deep Convolutional Neural Network-Based Heart Diagnosis for Smart Healthcare Applications
- K. Saikumar, V. Rajesh
A Dynamic Perceptual Detector Module-Related Telemonitoring for the Intertubes of Health Services
- Vaibhav Rupapara, S. Suman Rajest, Regin Rajan, R. Steffi, T. Shynu, G. Jerusha Angelene Christabel
ConvNet-Based Deep Brain Stimulation for Attack Patterns
- Angel Sajani Joseph, Arokia Jesu Prabhu Lazar, Dilip Kumar Sharma, Anto Bennet Maria, Nivedhitha Ganesan, Sudhakar Sengan
Web-Based Augmented Reality of Smart Healthcare Education for Machine Learning-Based Object Detection in the Night Sky
- Sriram Veeraiya Perumal, Sudhakar Sengan, Dilip Kumar Sharma, Amarendra Kothalanka, Rajesh Iruluappan, Arjun Subburaj
This book provides information on interdependencies of medicine and telecommunications engineering and how the two must rely on each other to effectively function in this era. The book discusses new techniques for medical service improvisation such as clear-cut views on medical technologies. The authors provide chapters on communication essentiality in healthcare, processing of medical amenities using medical images, the importance of data and information technology in medicine, and machine learning and artificial intelligence in healthcare. Authors include researchers, academics, and professionals in the field.
- Smart Health science
- Computational techniques in medicine
- Bio-medical science and AI
- Intelligent systems in healthcare
- Smart healthcare
Parul Agarwal
Kavita Khanna
Ahmed A Elngar
Ahmed J. Obaid
Zdzislaw Polkowski
Parul Agarwal is associated with Jamia Hamdard since 2002. She is currently an Associate Professor at Department of Computer Science and Engineering. Her area of specialization includes Fuzzy Data Mining, Cloud Computing, Sustainable computing, and Soft Computing. Her particular interest includes applications of Sustainable Computing in Agriculture, transportation, and health care. She has published several papers in reputed and SCI, Scopus, and Elsevier indexed journals and many book chapters published by CRC Press, Springer, and IGI-Global are to her credit. She is the Editor of three published edited volumes with reputed publishers: CRC Press (Taylor and Francis), and two books with Springer. Both the books with Springer are SCOPUS indexed. She has chaired several sessions of International/ National Conferences of repute. She has delivered several lectures/talks at the national and international level. Currently, several Ph.D. Scholars are working under her supervision inthe field of Brain Signaling, Energy minimization in the Cloud, Time series forecasting, and Smart Building management for smart cities. She has delivered several lectures on national and international platforms. She has organized a workshop as Principal Investigator in 2017, on “Use of ICT in sustainable Computing” approved by the Department of Science and Technology, Govt. of India, worth 5.75 Lakhs. She has guided more than 70 Masters’s thesis (MCA and M.Tech.). Member of several committees at the university level, life member of ISTE, and senior member of IEEE is to her credit.
Kavita Khanna has more than two decades of teaching, research and administrative experience. Currently she is associated with Delhi Skill and Entrepreneurship University as Campus Director of Dwarka Campus. She has done her PhD from Guru Gobind Singh Indraprastha University, Dwarka, New Delhi. Her research areas include Soft Computing, Machine Learning, Computer Graphics and Algorithm Design. She has to her credit more than 60 publications in reputed journals and conferences including Elsevier, Springer and Taylor & Francis. She has organized multiple International Conferences in collaboration with Elsevier and Springer. She has been a part of many conferences as session chair and have delivered multiple guest lectures at platforms of repute. She has successfully guided 20 MTech & 2 PhD scholars and currently 5 research scholars are working under her supervision. Apart from teaching-learning and research, she has also worked as a Radio Jockey with AIR FM Gold for 16 years. She believes that there is no end to learning and this motivates her to attend various National and International Conferences, Workshops and Faculty Development Programs."
Ahmed A. Elngar is an Associate Professor of Computer Science at the Faculty of Computers and Artificial Intelligence, Beni-Suef University, Egypt. Dr. Elngar is also, an AssociateProfessor of Computer Science at the College of Computer Information Technology, American University in the Emirates, United Arab Emirates. Also, Dr. Elngar is an Adjunct Professor at the School of Technology, Woxsen University, India. Dr. Elngar is the Founder and Head of the Scientific Innovation Research Group (SIRG). Dr. Elngar is a Director of the Technological and Informatics Studies Center (TISC), Faculty of Computers and Artificial Intelligence, Beni-Suef University. Dr. AE has more than 106 scientific research papers published in prestigious international journals and over 25 books covering such diverse topics as data mining, intelligent systems, social networks, and smart environment which were published in Elsevier, Springer and Taylor & Francis. Dr. Elngar is a collaborative researcher He is a member of the Egyptian Mathematical Society (EMS) and International Rough Set Society (IRSS). His other research areas include the Internet of Things (IoT), Network Security, Intrusion Detection, Machine Learning, Data Mining, and Artificial Intelligence. Big Data, Authentication, Cryptology, Healthcare Systems, Automation Systems. Dr. Elngar is an Editor and Reviewer of many international journals around the world. Dr. Elngar won several awards including the Young Researcher in Computer Science Engineering”, from Global Outreach Education Summit and Awards 2019, on 31 January 2019 (Thursday) in Delhi, India. Also, he awards the Best Young Researcher Award (Male) (Below 40 years)”, Global Education and Corporate Leadership Awards (GECL-2018), Plot No-8, Shivaji Park, Alwar 301001, Rajasthan. Dr. Elngar has successfully guided 15 M.Sc & 8 Ph.D. scholars who are working under his supervision. He also serves as an external examiner thesis for Ph. D scholars at many universities all around the world.
Ahmed J. Obaid , is a Asst. Professor at the Department of Computer Science, Faculty of Computer Science and Mathematics, University of Kufa, Iraq. Dr. Ahmed holds a Bachelor in Computer Science, degree in – Information Systems from College of Computers, University of Anbar, Iraq (2001-2005), and a Master Degree (M. TECH) of Computer Science Engineering (CSE) from School of Information Technology, Jawaharlal Nehru Technological University, Hyderabad, India (2010-2013), and a Doctor of Philosophy (PhD) in Web Mining from College of Information Technology, University of University of Babylon, Iraq (2013-2017). He is a Certified Web Mining Consultant with over 14 years of experience in working as Faculty Member in University of Kufa, Iraq. He has taught courses in Web Designing, Web Scripting, JavaScript, VB.Net, MATLAB Toolbox’s, and other courses on PHP, CMC, and DHTML from more than 10 international organizations and institutes from USA, and India. Dr. Ahmed is a member of Statistical and Information Consultation Center (SICC), University of Kufa, Iraq. His main line of research is Web mining Techniques and Application, Image processing in the Web Platforms, Image processing, Genetic Algorithm, information theory, and Medical Health Applications. Ahmed J. is Associated Editor in Brazilian Journal of Operations & Production Management (BJO&PM) and Editorial Board Members in : International Journal of Advance Study and Research Work (IJASRW), Journal of Research in Engineering and Applied Sciences(JREAS), GRD Journal for Engineering (GRDJE), International Research Journal of Multidisciplinary Science & Technology (IRJMST), The International Journal of Technology Information and Computer (IJTIC), Career Point International Journal Research (CPIJR). Ahmed J. was Editor in Many International Conferences such as: ISCPS2020, MAICT 2020, MAICT 2020, IHICPS 2020, IICESAT 2021, etc (Scopus indexed conferences). He has edited Some books, such as Advance Material Science and Engineering (ISBN: 9783035736779, Scientific.net publisher), Computational Intelligence Techniques for Combating COVID-19 (ISBN: 978-3-030-68936-0 EAI/Springer), A Fusion of Artificial Intelligence and Internet of Things for Emerging Cyber Systems (ISBN: 978-3-030-76653-5 Springer). Ahmed J. has supervised several final projects of Bachelor and Master in his main line of work, and authored and co-authored several scientific publications in journals, Books and conferences with more than 65+ Journal Research Articles, 5+ book Chapters, 15+ Conference papers, 10+ Conference proceedings, 8+ Books Editing, 2+ Patent. Ahmed J. also Reviewer in many Scopus, SCI and ESCI Journals e.g., CMC, IETE, IJAACS, IJIPM, IJKBD, IJBSR, IET, IJUFKS and many others. Dr. Ahmed Attend and participate as: Keynote Speakers (40+ Conferences), Webinars (10+), Session Chairs (10+), in many international events in the following countries: India, Turkey, Nepal, Philippines, Vietnam, Thailand, Indonesia and other countries.
Zdzislaw Polkowski is Professor of UJW at Faculty of Technical Sciences and Rector's Representative for International Cooperation and Erasmus+ Program at the Jan Wyzykowski University Polkowice. Since 2019 he is also Adjunct Professor in Department of Business Intelligence in Management, Wroclaw University of Economics and Business, Poland. Moreover, he is visiting professor in Univeristy of Pitesti, Romania, WSG University Bydgoszcz, Poland and adjunct professor in Marwadi University, India. He is the former dean of the Technical Sciences Faculty during the period 2009 -2012 at UZZM in Lubin. He holds a PhD degree in Computer Science and Management from Wroclaw University of Technology, Post Graduate degree in Microcomputer Systems in Management from University of Economics in Wroclaw and Post Graduate degree IT in Education from Economics University in Katowice. He obtained his Engineering degree in Computer Systems in Industry from Technical University of Zielona Gora. He has published more than75 papers in journals, 25 conference proceedings, including more than 20 papers in journals indexed in the Web of Science, Scopus, IEEE. He served as a member of Technical Program Committee in many International conferences in Poland, India, China, Iran, Romania and Bulgaria. Till date he has delivered 24 invited talks at different international conferences across various countries. He is also the member of the Board of Studies and expert member of the doctoral research committee in many universities in India. He is also the member of the editorial board of several journals and served as a reviewer in a wide range of international journals. His area of interests includes IT in Business, IoT in Business and Education Technology. He has successfully completed a research project on Developing the innovative methodology of teaching Business Informatics funded by the European Commission. He also owns an IT SME consultancy company in Polkowice and Lubin, Poland.
Book Title : Artificial Intelligence for Smart Healthcare
Editors : Parul Agarwal, Kavita Khanna, Ahmed A Elngar, Ahmed J. Obaid, Zdzislaw Polkowski
Series Title : EAI/Springer Innovations in Communication and Computing
DOI : https://doi.org/10.1007/978-3-031-23602-0
Publisher : Springer Cham
eBook Packages : Engineering , Engineering (R0)
Copyright Information : The Editor(s) (if applicable) and The Author(s), under exclusive license to Springer Nature Switzerland AG 2023
Hardcover ISBN : 978-3-031-23601-3 Published: 10 June 2023
Softcover ISBN : 978-3-031-23604-4 Due: 11 July 2023
eBook ISBN : 978-3-031-23602-0 Published: 09 June 2023
Series ISSN : 2522-8595
Series E-ISSN : 2522-8609
Edition Number : 1
Number of Pages : XVIII, 529
Number of Illustrations : 1 b/w illustrations
Topics : Communications Engineering, Networks , Artificial Intelligence , Health Informatics , Computational Intelligence
Policies and ethics
- Find a journal
- Track your research
- IEEE Xplore Digital Library
- IEEE Standards
- IEEE Spectrum Online
- More IEEE Sites
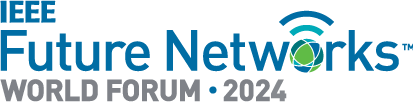
Symposium on Designing the Future RAN Architecture: From Theory to Practice and Beyond
Symposium organizers.
- Mohammad Asif Habibi, University of Kaiserslautern (RPTU), Germany
- Bin Han, University of Kaiserslautern (RPTU), Germany
- Ashutosh Dutta, Johns Hopkins University, USA
- Xavier Costa-Pérez, NEC Laboratories Europe, Germany; i2CAT Research Center, Spain
- Mithun Mukherjee, Khalifa University, UAE
- Hans D. Schotten, University of Kaiserslautern (RPTU), Germany, Germany; German Research Center for Artificial Intelligence, Germany
SCOPE AND MOTIVATION
The radio access network (RAN) is a critical component of wireless communication networks, responsible for connecting user devices to the core network and enabling seamless connectivity and mobility. The RAN also plays a significant role in ensuring the reliability, security, and performance of the network, making it a critical area for research and innovation. With the evolution of 5G and ongoing efforts towards 6G, the RAN is becoming more complex and dynamic, with new challenges and opportunities emerging in areas such as network slicing, virtualization, cloudification, and intelligentization. Furthermore, open interfaces and programmable architectures are gaining traction as a means of achieving greater flexibility, interoperability, and customization in the RAN. As such, there is a need for novel solutions and best practices to address the challenges and exploit the opportunities presented by the next-generation RAN.
The next-generation RAN will play a critical role in shaping the future of communication networks. It is evolving rapidly, fueled by innovations in slicing, virtualization, openness, programmability, standardization, cloudification, intelligentization, and architecture. As the RAN becomes more complex and dynamic, it presents new opportunities and challenges for researchers, practitioners, and industry experts. The IEEE FNWF 2024 symposium on " Designing the Future RAN: Openness, Cloudification, Slicing, Intelligentization, and Architecture" seeks to explore the latest research, innovations, and best practices in RAN design, optimization, and management.
We invite original, high-quality research papers that address the aforementioned aspects of the RAN for beyond 5G and 6G communication networks. The symposium aims to bring together researchers, practitioners, and industry experts to exchange ideas, discuss challenges, and propose solutions for the next-generation RAN design and optimization.
TOPICS OF INTEREST
Topics of interest include, but are not limited to:
- Open RAN architecture
- Slicing and Virtualization of RAN for 5G and 6G
- Open RAN Optimization and Open Interfaces for RAN Evolution
- Intelligent RAN for Efficient and Dynamic Network Management
- Programmable RAN Architectures for Network Customization and Control
- RAN Cloudification and Edge Computing for Low-Latency and High-Performance Services
- Standardization of Open RAN Architecture and Protocols for Interoperability and Scalability
- RAN Security and Privacy for Trustworthy and Reliable Communication
- Novel RAN Architectures for Beyond 5G and 6G Networks
SYMPOSIUM PAPER SUBMISSION
All papers should be submitted via EDAS , Symposium on Designing the Future RAN Architecture: From Theory to Practice and Beyond track. Full instructions on how to submit papers are provided on the IEEE FNWF 2024 website.
Research on Personalized Recommendation Algorithm Based on Cluster Analysis and Artificial Intelligence
Ieee account.
- Change Username/Password
- Update Address
Purchase Details
- Payment Options
- Order History
- View Purchased Documents
Profile Information
- Communications Preferences
- Profession and Education
- Technical Interests
- US & Canada: +1 800 678 4333
- Worldwide: +1 732 981 0060
- Contact & Support
- About IEEE Xplore
- Accessibility
- Terms of Use
- Nondiscrimination Policy
- Privacy & Opting Out of Cookies
A not-for-profit organization, IEEE is the world's largest technical professional organization dedicated to advancing technology for the benefit of humanity. © Copyright 2024 IEEE - All rights reserved. Use of this web site signifies your agreement to the terms and conditions.
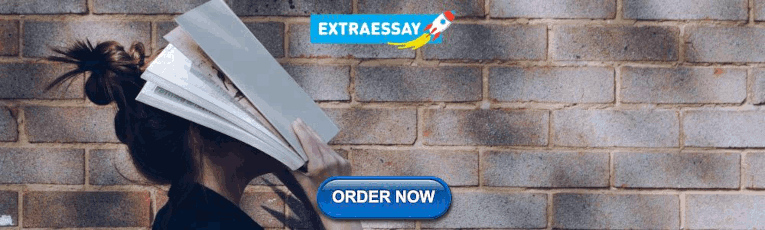
IMAGES
VIDEO
COMMENTS
We have seen during this pandemic, India, there are many dangers to the healthcare system which calls for the need to encourage hospitals o switch an electronic healthcare information system from paper-based medical records to an electronic medical related data that offers e-healthcare information and integrates well with computer-based judgment making systems. With the growing interest in AI ...
The use of artificial intelligence in health is growing rapidly. In this paper, a number of case studies were reviewed to elicit the sensitivity of health impact to parameters of AI design and application context. At a high level, the speed at which breakthroughs are happening is highly encouraging. Whereas application to core science or health preservation is working well, however, things are ...
AI technology based applications are evolving more rapid than ever. End users and customers' expectations are so high and their demands are met with more accuracy and prediction analysis as possible. This article provides insight into research of applying AI based techniques into health care domain for the betterment and ease of doctors and patients. Basic principles are covered in ...
The healthcare industry benefits from computer science. Good technology is necessary for strong medical equipment. Major areas that can be implemented in the healthcare sector. There are many technologies used in healthcare sectors AI, IOT, big data and machine learning. Artificial intelligence (AI) has a wide range of applications, including disease prediction, medication research ...
AI in Healthcare Operations, to improve hospital care provision or manufacturing quality and yield using electronic medical records or advanced business analytics. AI in Supply Chain Management, to ensure that products are distributed efficiently and effectively despite global dynamics such as pandemics. The challenges of regulatory approval ...
The integration of Artificial Intelligence (AI) technology into mental health services has emerged as a promising way to improve the quality and accessibility of mental health services. In the field of analysis, Artificial Intelligence techniques like Natural Language Processing (NLP) and Machine Learning algorithms have shown great potential for the analysis of big data such as electronic ...
Abstract. Artificial intelligence (AI) has been developing rapidly in recent years in terms of software algorithms, hardware implementation, and applications in a vast number of areas. In this review, we summarize the latest developments of applications of AI in biomedicine, including disease diagnostics, living assistance, biomedical ...
Abstract—Artificial intelligence (AI) models are increasingly finding applications in the field of medicine. Concerns have been raised about the explainability of the decisions that are made by these AI models. In this article, we give a systematic analysis of explainable artificial intelligence (XAI), with a primary focus on models that are ...
The potential for artificial intelligence in healthcare - PMC › ieee products services ai artificial intelligence health care IEEE Standards Association ieee-industry standards and technology ...
The goal is to use a bibliographic analysis to show an inclusive ecosystem of artificial intelligence applications in diabetes and to suggest future research directions. Moreover, using COVID-19 to map the landscape of Artificial Intelligence applications is introduced by Bullock et al. (Bullock et al., 2020). The authors offer a summary of ...
Artificial intelligence (AI) has the potential to transform care delivery by improving health outcomes, patient safety, and the affordability and accessibility of high-quality care. AI will be ...
Artificial intelligence (AI) is a powerful and disruptive area of computer science, with the potential to fundamentally transform the practice of medicine and the delivery of healthcare. In this review article, we outline recent breakthroughs in the application of AI in healthcare, describe a roadmap to building effective, reliable and safe AI ...
Research topics. Artificial Intelligence (AI) is a novel topic based on already known knowledge, which has several healthcare implications. Due to its continuous growth, there is the potential for a structured literature review (SLR) investigating how AI can contribute to healthcare implementation. 5069 results.
Artificial intelligence (AI) is the programing of computer systems to analyze, problem-solve, and make decisions just as a human would. 1 AI was first introduced in the 1950s with many limitations which made it difficult to be introduced into healthcare but has since developed into what we use today in modern medicine. 2 AI began as a simple ...
Abstract. Artificial intelligence is to reduce human cognitive functions. It is bringing an approach to healthcare, powdered by increasing the availability of healthcare data and rapid progress of ...
Includes a comprehensive introduction of artificial intelligence in healthcare; ... Member of several committees at the university level, life member of ISTE, and senior member of IEEE is to her credit. ... Faculty of Computers and Artificial Intelligence, Beni-Suef University. Dr. AE has more than 106 scientific research papers published in ...
DOI: 10.1016/j.im.2024.103961 Corpus ID: 268954750; Resistance to artificial intelligence in health care: Literature review, conceptual framework, and research agenda @article{Yang2024ResistanceTA, title={Resistance to artificial intelligence in health care: Literature review, conceptual framework, and research agenda}, author={Yikai Yang and Eric W.T. Ngai and Lei Wang}, journal={Information ...
Chatbot for Healthcare System Using Artificial Intelligence. June 2020. DOI: 10.1109/ICRITO48877.2020.9197833. Conference: 2020 8th International Conference on Reliability, Infocom Technologies ...
This paper establishes an effective early-warning system framework of corporate financial risk. This paper discusses the early-warning model of financial risk. On this basis, Bayes-SVM algorithm is applied to the classification of financial crisis events. We timely detect and alarm the abnormal situation in the company's financial system. Finally, an example is given to prove that the proposed ...
UF Health Research Team Aims to Create AI Algorithm to Improve Prostate Cancer Diagnosis August 14, 2023 Most Recent Select April 2024 September 2023 August 2023 Medical Imaging Research for Translational Healthcare with Artificial Intelligence Laboratory Department of Medicine in the College of Medicine
We invite original, high-quality research papers that address the aforementioned aspects of the RAN for beyond 5G and 6G communication networks. The symposium aims to bring together researchers, practitioners, and industry experts to exchange ideas, discuss challenges, and propose solutions for the next-generation RAN design and optimization.
The demand for personalized recommendation systems is gradually increasing under the backdrop of big data. This paper aims to enhance the effectiveness of personalized recommendation algorithms, better cater to users' individual needs, and provide support for constructing an improved content recommendation system. It proposes that a personalized algorithm based on cluster analysis and ...