Featured Clinical Reviews
- Screening for Atrial Fibrillation: US Preventive Services Task Force Recommendation Statement JAMA Recommendation Statement January 25, 2022
- Evaluating the Patient With a Pulmonary Nodule: A Review JAMA Review January 18, 2022
- Download PDF
- CME & MOC
- Share X Facebook Email LinkedIn
- Permissions
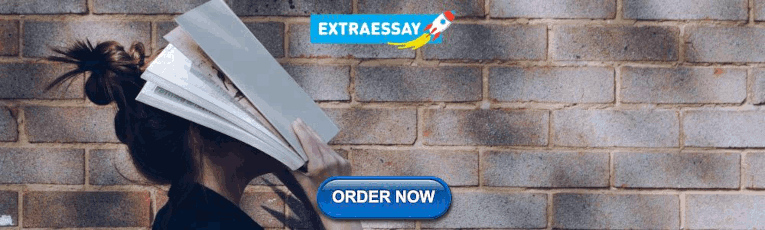
Sequential, Multiple Assignment, Randomized Trial Designs
- 1 Department of Biostatistics, School of Public Health, University of Michigan, Ann Arbor
- 2 Survey Research Center, Institute for Social Research, University of Michigan, Ann Arbor
- 3 Department of Statistics, University of Michigan, Ann Arbor
- JAMA Guide to Statistics and Methods Collider Bias Mathias J. Holmberg, MD, MPH, PhD; Lars W. Andersen, MD, MPH, PhD, DMSc JAMA
- Special Communication Reporting of Factorial Randomized Trials Brennan C. Kahan, PhD; Sophie S. Hall, PhD; Elaine M. Beller, MAppStat; Megan Birchenall, BSc; An-Wen Chan, MD, DPhil; Diana Elbourne, PhD; Paul Little, MD; John Fletcher, MPH; Robert M. Golub, MD; Beatriz Goulao, PhD; Sally Hopewell, DPhil; Nazrul Islam, PhD; Merrick Zwarenstein, MBBCh, PhD; Edmund Juszczak, MSc; Alan A. Montgomery, PhD JAMA
- Original Investigation Comparison of Teleintegrated Care and Telereferral Care for Treating Complex Psychiatric Disorders in Primary Care John C. Fortney, PhD; Amy M. Bauer, MS, MD; Joseph M. Cerimele, MPH, MD; Jeffrey M. Pyne, MD; Paul Pfeiffer, MD; Patrick J. Heagerty, PhD; Matt Hawrilenko, PhD; Melissa J. Zielinski, PhD; Debra Kaysen, PhD; Deborah J. Bowen, PhD; Danna L. Moore, PhD; Lori Ferro, MHA; Karla Metzger, MSW; Stephanie Shushan, MHA; Erin Hafer, MPH; John Paul Nolan, AAS; Gregory W. Dalack, MD; Jürgen Unützer, MPH, MD JAMA Psychiatry
An adaptive intervention is a set of diagnostic, preventive, therapeutic, or engagement strategies that are used in stages, and the selection of the intervention at each stage is based on defined decision rules. At the beginning of each stage in care, treatment may be changed by the clinician to suit the needs of the patient. Typical adaptations include intensifying an ongoing treatment or adding or switching to another treatment. These decisions are made in response to changes in the patient’s status, such as a patient’s early response to, or engagement with, a prior treatment. The patient experiences an adaptive intervention as a sequence of personalized treatments.
Read More About
Kidwell KM , Almirall D. Sequential, Multiple Assignment, Randomized Trial Designs. JAMA. 2023;329(4):336–337. doi:10.1001/jama.2022.24324
Manage citations:
© 2024
Artificial Intelligence Resource Center
Cardiology in JAMA : Read the Latest
Browse and subscribe to JAMA Network podcasts!
Others Also Liked
Select your interests.
Customize your JAMA Network experience by selecting one or more topics from the list below.
- Academic Medicine
- Acid Base, Electrolytes, Fluids
- Allergy and Clinical Immunology
- American Indian or Alaska Natives
- Anesthesiology
- Anticoagulation
- Art and Images in Psychiatry
- Artificial Intelligence
- Assisted Reproduction
- Bleeding and Transfusion
- Caring for the Critically Ill Patient
- Challenges in Clinical Electrocardiography
- Climate and Health
- Climate Change
- Clinical Challenge
- Clinical Decision Support
- Clinical Implications of Basic Neuroscience
- Clinical Pharmacy and Pharmacology
- Complementary and Alternative Medicine
- Consensus Statements
- Coronavirus (COVID-19)
- Critical Care Medicine
- Cultural Competency
- Dental Medicine
- Dermatology
- Diabetes and Endocrinology
- Diagnostic Test Interpretation
- Drug Development
- Electronic Health Records
- Emergency Medicine
- End of Life, Hospice, Palliative Care
- Environmental Health
- Equity, Diversity, and Inclusion
- Facial Plastic Surgery
- Gastroenterology and Hepatology
- Genetics and Genomics
- Genomics and Precision Health
- Global Health
- Guide to Statistics and Methods
- Hair Disorders
- Health Care Delivery Models
- Health Care Economics, Insurance, Payment
- Health Care Quality
- Health Care Reform
- Health Care Safety
- Health Care Workforce
- Health Disparities
- Health Inequities
- Health Policy
- Health Systems Science
- History of Medicine
- Hypertension
- Images in Neurology
- Implementation Science
- Infectious Diseases
- Innovations in Health Care Delivery
- JAMA Infographic
- Law and Medicine
- Leading Change
- Less is More
- LGBTQIA Medicine
- Lifestyle Behaviors
- Medical Coding
- Medical Devices and Equipment
- Medical Education
- Medical Education and Training
- Medical Journals and Publishing
- Mobile Health and Telemedicine
- Narrative Medicine
- Neuroscience and Psychiatry
- Notable Notes
- Nutrition, Obesity, Exercise
- Obstetrics and Gynecology
- Occupational Health
- Ophthalmology
- Orthopedics
- Otolaryngology
- Pain Medicine
- Palliative Care
- Pathology and Laboratory Medicine
- Patient Care
- Patient Information
- Performance Improvement
- Performance Measures
- Perioperative Care and Consultation
- Pharmacoeconomics
- Pharmacoepidemiology
- Pharmacogenetics
- Pharmacy and Clinical Pharmacology
- Physical Medicine and Rehabilitation
- Physical Therapy
- Physician Leadership
- Population Health
- Primary Care
- Professional Well-being
- Professionalism
- Psychiatry and Behavioral Health
- Public Health
- Pulmonary Medicine
- Regulatory Agencies
- Reproductive Health
- Research, Methods, Statistics
- Resuscitation
- Rheumatology
- Risk Management
- Scientific Discovery and the Future of Medicine
- Shared Decision Making and Communication
- Sleep Medicine
- Sports Medicine
- Stem Cell Transplantation
- Substance Use and Addiction Medicine
- Surgical Innovation
- Surgical Pearls
- Teachable Moment
- Technology and Finance
- The Art of JAMA
- The Arts and Medicine
- The Rational Clinical Examination
- Tobacco and e-Cigarettes
- Translational Medicine
- Trauma and Injury
- Treatment Adherence
- Ultrasonography
- Users' Guide to the Medical Literature
- Vaccination
- Venous Thromboembolism
- Veterans Health
- Women's Health
- Workflow and Process
- Wound Care, Infection, Healing
- Register for email alerts with links to free full-text articles
- Access PDFs of free articles
- Manage your interests
- Save searches and receive search alerts
Thank you for visiting nature.com. You are using a browser version with limited support for CSS. To obtain the best experience, we recommend you use a more up to date browser (or turn off compatibility mode in Internet Explorer). In the meantime, to ensure continued support, we are displaying the site without styles and JavaScript.
- View all journals
- Explore content
- About the journal
- Publish with us
- Sign up for alerts
- Review Article
- Published: 26 December 2022
Clinical Studies
Use of Sequential Multiple Assignment Randomized Trials (SMARTs) in oncology: systematic review of published studies
- Giulia Lorenzoni 1 na1 ,
- Elisabetta Petracci 2 na1 ,
- Emanuela Scarpi ORCID: orcid.org/0000-0001-7230-9267 2 ,
- Ileana Baldi 1 ,
- Dario Gregori ORCID: orcid.org/0000-0001-7906-0580 1 &
- Oriana Nanni 2
British Journal of Cancer volume 128 , pages 1177–1188 ( 2023 ) Cite this article
2779 Accesses
1 Citations
1 Altmetric
Metrics details
- Clinical trial design
Sequential multiple assignments randomized trials (SMARTs) are a type of experimental design where patients may be randomised multiple times according to pre-specified decision rules. The present work investigates the state-of-the-art of SMART designs in oncology, focusing on the discrepancy between the available methodological approaches in the statistical literature and the procedures applied within cancer clinical trials. A systematic review was conducted, searching PubMed, Embase and CENTRAL for protocols or reports of results of SMART designs and registrations of SMART designs in clinical trial registries applied to solid tumour research. After title/abstract and full-text screening, 33 records were included. Fifteen were reports of trials’ results, four were trials’ protocols and fourteen were trials’ registrations. The study design was defined as SMART by only one out of fifteen trial reports. Conversely, 13 of 18 study protocols and trial registrations defined the study design SMART. Furthermore, most of the records considered each stage separately in the analysis, without considering treatment regimens embedded in the trial. SMART designs in oncology are still limited. Study powering and analysis is mainly based on statistical approaches traditionally used in single-stage parallel trial designs. Formal reporting guidelines for SMART designs are needed.
Similar content being viewed by others
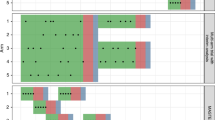
Advantages of multi-arm non-randomised sequentially allocated cohort designs for Phase II oncology trials
Helen Mossop, Michael J. Grayling, … James M. S. Wason
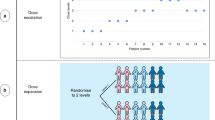
Randomised Phase 1 clinical trials in oncology
Alexia Iasonos & John O’Quigley
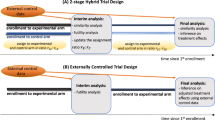
The design and evaluation of hybrid controlled trials that leverage external data and randomization
Steffen Ventz, Sean Khozin, … Lorenzo Trippa
Introduction
Dynamic Treatment Regimens (DTRs), also known as adaptive treatment strategies or adaptive interventions, are a set of sequential decision rules, each one corresponding to a key decision point in the patient’s history [ 1 ]. Each rule establishes the treatment for the patient among the available treatment options according to the information collected until then.
The DTR represents a formalisation of the multi-stage and dynamic decision process followed by clinicians in their everyday clinical practice. The final aim of the decision process is to tailor the treatment to the patients’ characteristics and clinical history, which is the key concept of precision medicine. In this sense, identifying the optimal DTR would be a way to put evidence-based precision medicine into practice, especially in chronic disease management [ 2 ], which is one of the most suitable clinical settings for DTRs. Particularly, cancer research is a promising field of application of SMART designs. Cancer is a chronic disease that requires treatment at multiple stages, according to each patient’s characteristics and clinical status [ 3 ].
However, providing evidence-based DTRs poses relevant methodological challenges to study design and DTRs’ effect estimation. The types of study commonly used for testing and comparing DTRs include observational studies, one-time randomised trials that randomise patients only once to the whole DTR, and sequential multiple assignment randomized trials (SMARTs) [ 2 ]. SMART designs randomise patients at each decision point considering information collected on the patient so far. They are of growing interest in the scientific community, but their use is not well-established yet.
The main difficulty in implementing trials to study DTRs is that there are still several open questions about sample size calculation and identification of the most appropriate method for data analysis [ 4 ]. In oncology, as in many other chronic conditions, it is common that the patient receives a frontline treatment followed by subsequent treatments adaptively chosen by the clinician. Consequently, the patient’s survival depends not only on the frontline treatment but also on the entire treatment strategy. However, the literature seems to be still dominated by trials investigating a single line or stage of the patient clinical history, ignoring previous or subsequent therapies, potentially leading to misleading results [ 5 ].
The present work aims to investigate the state-of-the-art of SMART designs in oncology, focusing on the statistical methods used for the sample size computation and data analysis within cancer clinical trials on solid tumours.
A systematic review was done. The review was conducted according to the Preferred Reporting Items for Systematic Reviews and Meta-Analyses (PRISMA) guidelines [ 6 ].
Information sources and search strategy
The bibliographic search was performed on PubMed, Embase and CENTRAL (Cochrane Trial Registry), without date of publication restrictions. The search string is reported in Table S1 (Supplementary Material).
Eligibility criteria and selection process
Published protocols or results of SMART designs and registrations of SMART designs in clinical trial registries were considered eligible. To be included, the SMART design should be applied to solid tumour research, without restrictions on the intervention type.
The criterion to identify SMART designs was the presence of ≥2 stages in which patients were re-randomised to subsequent treatments according to a set of pre-specified decision rules based on patients’ characteristics and treatment history [ 7 ].
The study selection was done using the COVIDENCE software [ 8 ]. The title/abstract and full-text screening was performed by two independent reviewers (GL and EP). A third independent reviewer (ES) was in charge of solving disagreements.
Conference proceedings, book chapters, systematic reviews and metanalysis were excluded, but they were checked for eligible papers. Papers in the English language were considered.
Data extraction
Information on three domains of interest was considered, i.e. study characteristics, study design and study analysis. Study characteristics included publication year, setting, funding, trial registration (if any), the definition of the study design as SMART, and if the study presented a reanalysis of the original study data. The study design information included the number of stages, the type of intervention administered at each stage, the decision rules employed, the study objectives and endpoints and sample size reporting and calculation information. The study analysis domain included the methods used for data analysis and if specific data analysis techniques were used to account for the adaptive treatment resulting from the multiple sequential assignments. For protocols, such information was extracted from the statistical analysis plan.
A restricted subset of items was employed to extract data from trials’ registrations records to allow a minimum dataset for all trials’ registrations included in the review. The item selection depended on the fact that the detail of information reported slightly changed according to the trial registry type. In most cases, the statistical analysis plan was missing.
Study characteristics were reported for descriptive purposes. Study design information was chosen according to the key SMART designs’ components, e.g. the number of stages, decision rules. Finally, information on study analysis techniques allowed for answering the main research question of the present work, i.e. to describe the statistical methods used within SMART designs to identify potential discrepancies between the available methodological approaches in the statistical literature and the procedures applied.
The data extraction tool was based on an Excel file.
Risk of bias assessment
The Cochrane risk-of-bias tool for randomised trials (RoB 2) [ 9 ] was used to assess the risk of bias in the included studies (articles and protocols). For studies included twice in the review [ 10 , 11 , 12 , 13 ], the assessment of the risk of bias was performed only once.
Search results
The search of the bibliographic databases resulted in the inclusion of 14,586 records (Fig. 1 ). The last search was performed on 9 September 2021.
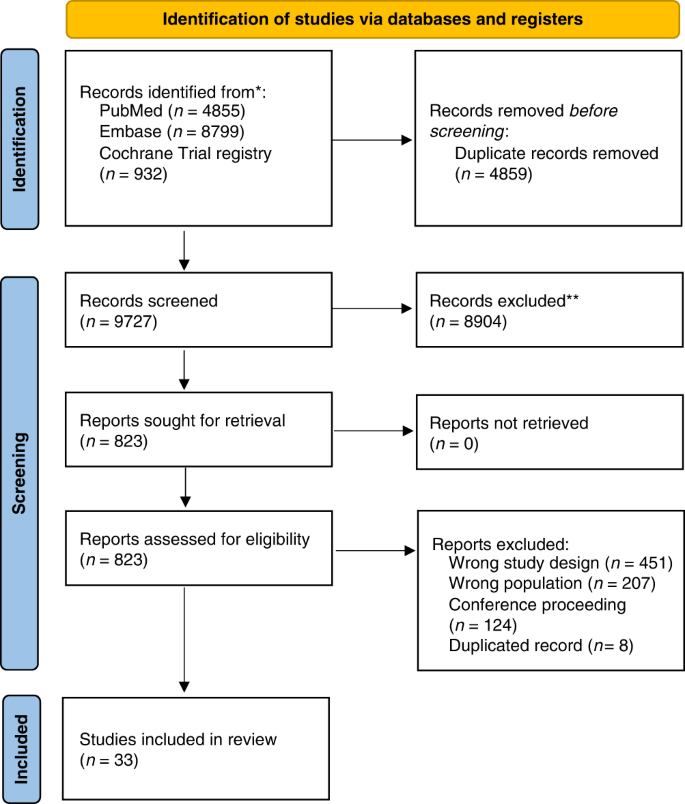
PRISMA flow-chart showing the study selection process.
After duplicate removal, title/abstract screening was performed, resulting in 823 included records which underwent full-text screening. After the full-text screening, 33 results were included in the present systematic review. Fifteen were reports of trials’ results [ 10 , 11 , 12 , 13 , 14 , 15 , 16 , 17 , 18 , 19 , 20 , 21 , 22 , 23 , 24 ], four were trials’ protocols [ 25 , 26 , 27 , 28 ] and fourteen were trials’ registrations.
Among the included records, there was a match between three trials’ protocols [ 26 , 27 , 28 ] and the corresponding trials’ registrations, and between two reports of trials’ results [ 16 , 22 ] and the corresponding trials’ registrations.
Among trials’ results reports and protocols, nine were published in oncology journals [ 11 , 12 , 14 , 15 , 18 , 19 , 22 , 23 , 24 ], three in experimental and research medicine journals [ 25 , 26 , 27 ], two in internal and general medicine journals [ 10 , 16 ]. The other five records were published in specialised journals in other areas of medicine, including clinical neurology [ 17 ], respiratory system [ 20 ] and peripheral vascular diseases [ 21 ]. One study was published in a nursing journal [ 28 ], and only one study was published in a statistics & probability journal [ 13 ].
All studies were found to present with some concerns at risk of bias assessment (Table S2 , Supplementary Material), except for that of Marshall et al. [ 21 ].
Trials’ results
Fifteen studies presenting trials’ results were included in the present work. Table S3 , Supplementary material, presents the detailed characteristics of the studies included.
Eight trials were located in the EU and 5 in North America. Thirteen out of fifteen were multicenter, and half (8 out of 15) received public or private funding. The first study was published in 1992. Six trials were published between 2010–2021, three between 2000–2009 and another six in the period 1990–1999.
Two studies [ 13 , 22 ] presented a reanalysis of previously published data; for what concerns that of Wang et al. [ 13 ], the study presenting the first analysis of the data was included in this review [ 12 ], whereas for the one by Petracci et al. [ 22 ], it was included the one reporting the reanalysis.
Furthermore, two studies presented the same trial’s short- and long-term results [ 10 , 11 ].
All the trials tested chemo/radio/hormone therapy for cancer treatment, including lung cancer, neuroblastoma, glioblastoma, pancreatic cancer, breast cancer, prostate cancer, colorectal cancer and recurrent venous thrombosis in solid tumours.
Interestingly, only one study out of the fifteen included was reported to have a SMART design [ 13 ]. All the trials were characterised by a two-stage design (Table 1 for detailed study design). The decision rule was most frequently based on the response to first-stage treatment. The objectives reported by most of the studies identified were to compare first and second-stage treatments separately or only first or second-stage treatments, except for Petracci et al. [ 22 ], Thall et al. [ 12 ] and Wang et al. [ 13 ]. The authors of these studies explicitly declared in the manuscript that the study’s objective was to identify the best treatment regimen resulting from the multiple assignments.
Eight studies did not report sample size calculation. Those reporting sample size calculations did not take into account the multiple assignments in the sample size estimation. Generally, the sample size was provided for each stage, or the powering of the study was made on one of the two stages and inflating according to the expected proportion of subjects entering the second randomisation. Of notice, Tummarello et al. [ 24 ] declared that the number of people entering the second randomisation was too small to allow groups’ comparison. Marshall et al. [ 21 ] and Bianchi et al. [ 14 ] underwent premature closure because of the low recruitment rate.
Regarding the data analysis (Table 2 for study details), the approaches most frequently used were the Kaplan–Meier method and the Cox Proportional Hazard model since most trials considered a time-to-event endpoint (overall or progression-free survival). Matthay et al. [ 10 , 11 ] used such analysis approaches to compare the treatment regimens resulting from the two-stage randomisation among subjects entering the second randomisation. In all other trials, separate analyses of first and second-stage treatments were carried out, except for Petracci et al. [ 22 ], Thall et al. [ 12 ] and Wang et al. [ 13 ], which were interested in identifying the best treatment regimen. These authors adopted three different strategies of analysis to estimate the treatment effect taking into account patients’ baseline characteristics and outcome history throughout the trial. The analysis of Petracci et al. [ 22 ] involved the estimation of Inverse Probability of Censoring Weighting (IPCW) to account for selection bias resulting from patients’ selection in the second stage. Thall et al. [ 12 ] used a conditional logistic regression approach. Wang et al. [ 13 ] proposed the estimation of Inverse Probability Treatment Weighting (IPTW) for the reanalysis of Thall et al. [ 12 ].
Trials’ protocols
The review included four trial protocols [ 25 , 26 , 27 , 28 ]. They were all located in the USA and published after 2009. Three [ 26 , 27 , 28 ] out of four corresponded to trials’ registrations included in the present review. All the study’s protocols received funding, and two were multicenter (Table S3 for trials’ protocols characteristics).
No trials were aimed at testing chemo/radiotherapy for cancer treatment. Two tested interventions to reduce cancer symptoms in patients with different types of solid tumours [ 28 ] and breast cancer [ 27 ]. One tested pharmacological treatment for depression in melanoma patients undergoing IFN-alpha therapy [ 25 ]. Finally, that of Fu et al. [ 26 ] was aimed at lung cancer prevention through a smoking cessation programme.
In all four protocols included, the design was defined to be SMART. They were all based on two stages, and the decision rule was based on the response to the first-stage treatment (Table 1 ). All the protocols were declared to be aimed at identifying the optimal treatment strategy. However, it is worth pointing out that only one protocol presented the identification of the optimal treatment strategy as the study’s primary objective [ 25 ].
All protocols reported the sample size calculation, but the power analysis was based only on one of the two stages in three out of four records. Only Auyeung et al. [ 25 ] proposed an approach accounting for the two-stage design. Not least, Fu et al. [ 26 ], in the last trial’s update published within the trial registration, declared that a sample size reassessment was done to account for the low enrolment rate.
For data analysis (Table 2 ), all protocols planned to use traditional statistical tests and regression-based analyses to compare first and second-stage treatment separately. Furthermore, Sikorsii et al. [ 28 ], Kelleher et al. [ 27 ] and Auyeung et al. [ 25 ] proposed three different analysis approaches to identify the optimal treatment strategy. Auyeung et al. [ 25 ] proposed using marginal mean models to estimate the mean outcome for each regimen. Sikorsii et al. [ 28 ] declared that the optimal intervention sequence will be identified through Q-learning algorithm, including two Q functions considering patients and their caregivers’ baseline characteristics and history through the two stages. Also, Kelleher et al. [ 27 ] planned the use of the Q-learning algorithm and value search estimation. No technical details about models’ estimations were provided.
Trials’ registrations
Fourteen trials’ registrations were included in the review. Five referred to already included trials’ results [ 16 , 22 ] and protocols [ 26 , 27 , 28 ]. All registrations were made after 2008, twelve were retrieved on clinicaltrials.gov, one from australianclinicaltrials.gov and one from the Clinical Trials Peruvian Registry. Half of the studies were located in North America.
Only five out of 14 trials (36%) were aimed at testing cancer chemo/radio treatments on overall survival or disease-free survival of patients with pancreatic cancer (3 registrations), colorectal cancer (1 registration) and neuroblastoma (1 registration). Six trials tested treatments for cancer and cancer treatment symptoms, such as fatigue, pain, sensory symptoms, depression, anxiety and quality of life, in patients with breast cancer or solid tumours and their caregivers. One trial’s registration was aimed at improving the management of cardiovascular comorbidities in cancer patients, and another one at testing interventions for COVID-19 prevention and treatment in cancer patients. Finally, one trial was aimed at cancer prevention (lung cancer), through a programme for smoking cessation, corresponding to the registration of the trial protocol published by Fu et al. [ 26 ].
Interestingly, all but five registrations referred to the study design as a SMART one. All designs were two-stage based, except for two studies. One included three stages, but only one decision-rule-based randomisation was specified (from the second to the third stage), while in the other trial, the number of stages depended on the patients’ COVID-19 status (no exposure, exposure to COVID-19, moderate or severe COVID-19 infection).
No information is reported regarding sample size calculation and data analysis because the statistical analysis plan was not available in almost all trials’ registrations.
Detailed characteristics of each one of the trials’ registrations included in the review are reported in Table S4 , Supplementary Material.
One of the most relevant findings of the present systematic review is the low number of studies retrieved. Such a low number of records suggests that the use of SMART designs in oncology is still limited, even though the advent of SMART designs offers new opportunities to develop evidence-based personalised treatment regimens, especially in cancer research [ 7 ]. Such findings could be related to the fact that they pose relevant methodological challenges to the sample size and treatment effect estimation and that there is still limited dissemination and perhaps understanding of the methods in the SMART research area. Unsurprisingly, most of the studies employed traditional techniques for study powering and analysis, considering each stage separately instead of comparing DTRs embedded in the trial, maybe because of the lack of formal guidelines for designing and reporting trials employing SMART methodologies.
Noticeably, the study design was defined as SMART in only one out of fifteen trial reports included, which would be one of the main reasons why most trials considered each stage separately from the other. This finding could be related to the fact that the formal introduction of SMART design is relatively new, even though the use of multiple randomisations according to pre-specified decision rules dates back to before the 2000s. Consequently, even though such trials, especially the oldest ones, were not labelled as SMART, they presented all the characteristics to be defined as SMART. Except for five, all study protocols and all trials’ registrations defined the study design SMART. This difference among the record types could be related to the timing of publication. Trial protocols and trials registrations were published within the last fifteen years, while about one-third of trials’ reports were published before 2000.
It is worth pointing out that, despite one of the primary goals of SMART designs is to identify the optimal DTR, only a few records included in the review considered determining the best treatment sequence as the study’s primary outcome. Such an aspect detected in the review is reflected by the approaches employed for sizing the study. It is undoubtedly that power analyses for SMART designs present relevant challenges because of the correlation structure between the embedded DTRs [ 29 ]. Several approaches have been proposed in the last years to undertake such issues in the SMART design [ 29 , 30 , 31 ] without definitive solutions. However, if the primary aim of a SMART study is to identify the best DTR, it follows that the sizing should be done to be able to detect the optimal DTR. However, most of the included trials reporting the power analysis used traditional methods for sample size estimation, since they did not consider the detection of the best DTR as the primary study endpoint. Generally, they estimated the sample size on only one of the trial’s stages and inflated the sample size on the expected proportion of subjects entering the second randomisation, or they estimated the sample size for each stage. The present finding is consistent with the conclusions of a recent review in the field [ 32 ].
For what concerns data analysis, most of the trials’ reports made separate analyses for each trial stage using traditional statistical methods, such as regression-based models, without considering patients’ history throughout the study. Such finding is consistent with the fact that most of the records included did not define the study design as SMART and did not identify the evaluation of the optimal DTR among the study outcomes. Focusing on the few reports aimed at identifying the best treatment regimen, two reports of trials’ results [ 13 , 22 ], both reanalyses of previously published data, tried to account for each subjects’ history through the trial in treatment effect estimation using propensity weighting estimation, while Thall et al. used a conditional regression approach [ 12 ]. On the other side, two study protocols proposed the use of Q-learning algorithm to identify the most promising DTR, which is an approach that has been suggested to be promising for the analysis of data collected using SMART designs [ 33 ]. Furthermore, it is interesting to point out that, even though SMART strategies are well known to suffer from the multiple comparison problem since the number of DTRs embedded in the trial is often large, most of the trials included did not account for such a problem.
Finally, it is noteworthy that the focus of SMART designs in solid tumour research changed over time. The first trials published employing sequential multiple assignments were aimed at testing chemo/radio/hormone therapy for solid tumours. Conversely, they have focused more and more on cancer symptoms and cancers treatments side effects, such as fatigue, depression, anxiety and pain in the last years. SMART designs are particularly suitable for assessing complex or long-term interventions for chronic conditions that require management to adapt to patients’ needs, as is the case of cancer-related symptoms.
For what concerns study limitations, the search strategy is the main one. When the study was done, no index terms referring to SMART designs were available in any one of the thesaurus of the bibliographic databases searched. Not least, as clearly emerged from the systematic review, the term SMART was often not employed by the authors, even though the study design satisfied the criteria for being SMART. We tried to overcome such limitations by including all possible synonymous of the critical aspects of a SMART design in a well-defined research field, that of solid tumours. However, we cannot rule out that relevant reports could not be included in the search. Another study limitation is that most of the trials’ registrations did not report details on the statistical analysis plan. It follows that they contributed to the review only with general information on trial characteristics. However, it would be interesting to update the review to check if reports of these registrations will be published and if the employed methods are consistent with those used in practice.
Conclusions
The present systematic review showed that the use of SMART designs in solid tumour research is still limited; however, the interest in such designs is growing, and it is testified by the increasing number of protocols and trial registrations in the last years. However, the present work clearly showed that despite the SMART designs’ primary aim would be to identify the optimal treatment regimen resulting from the multiple assignments, most of the trials included did not consider the identification of the best DTR as their primary objective. Consequently, they did not employ ad hoc methods for powering and analysing the trial to determine the best DTR; powering and analysing each study’s stage separately is still the approach most widely used. Such aspects could be related to the fact that the SMART designs are relatively new.
Present results highlighted that greater efforts should be put forward to developing formal guidelines for SMART designs’ conduction and reporting. A thorough literature review of methodological papers presenting and discussing statistical approaches for SMART designs would represent the basis for formal guidelines in the field. With such a review, the development of standard guidelines would benefit from the involvement of a panel of experts, i.e. using the Delphi methodology, to improve the use of such design in cancer trials.
Data availability
Not applicable
Chakraborty B, Murphy SA. Dynamic treatment regimes. Annu Rev Stat Appl. 2014;1:447–64.
Article PubMed PubMed Central Google Scholar
Lavori PW, Dawson R. Adaptive treatment strategies in chronic disease. Annu Rev Med. 2008;59:443–53.
Article CAS PubMed PubMed Central Google Scholar
Kidwell KM. SMART designs in cancer research: past, present, and future. Clin Trials. 2014;11:445–56.
Laber EB, Davidian M. Dynamic treatment regimes, past, present, and future: a conversation with experts. Stat Methods Med Res. 2017;26:1605–10.
Wahed AS, Thall PF. Evaluating joint effects of induction–salvage treatment regimes on overall survival in acute leukaemia. J R Stat Soc Ser C (Appl Stat). 2013;62:67–83.
Article PubMed Google Scholar
Page MJ, McKenzie JE, Bossuyt PM, Boutron I, Hoffmann TC, Mulrow CD, et al. The PRISMA 2020 statement: an updated guideline for reporting systematic reviews. Syst Rev. 2021;10:1–11.
Article Google Scholar
Kidwell KM. Chapter 2: Dtrs and smarts: Definitions, designs, and applications. In: Adaptive treatment strategies in practice: Planning trials and analyzing data for personalized medicine. SIAM; 2015. p. 7–23.
Veritas Health Innovation. Covidence Systematic Review Software. 2021. https://www.covidence.org/ .
Higgins JP, Savović J, Page MJ, Elbers RG, Sterne JA. Assessing risk of bias in a randomized trial. In: Cochrane handbook for systematic reviews of interventions. The Cochrane Collaboration and John Wiley & Sons Ltd.; 2019, p. 205–28.
Matthay KK, Villablanca JG, Seeger RC, Stram DO, Harris RE, Ramsay NK, et al. Treatment of high-risk neuroblastoma with intensive chemotherapy, radiotherapy, autologous bone marrow transplantation, and 13-cis-retinoic acid. N Engl J Med. 1999;341:1165–73.
Article CAS PubMed Google Scholar
Matthay KK, Reynolds CP, Seeger RC, Shimada H, Adkins ES, Haas-Kogan D, et al. Long-term results for children with high-risk neuroblastoma treated on a randomized trial of myeloablative therapy followed by 13-cis-retinoic acid: a children’s oncology group study. J Clin Oncol. 2009;27:1007–13.
Thall PF, Logothetis C, Pagliaro LC, Wen S, Brown MA, Williams D, et al. Adaptive therapy for androgen-independent prostate cancer: a randomized selection trial of four regimens. J Natl Cancer Inst. 2007;99:1613–22.
Wang L, Rotnitzky A, Lin X, Millikan RE, Thall PF. Evaluation of viable dynamic treatment regimes in a sequentially randomized trial of advanced prostate cancer. J Am Stat Assoc. 2012;107:493–508.
Bianchi S, Mosca A, Dalla Volta A, Prati V, Ortega C, Buttigliero C, et al. Maintenance versus discontinuation of androgen deprivation therapy during continuous or intermittent docetaxel administration in castration-resistant prostate cancer patients: a multicentre, randomised Phase III study by the Piemonte Oncology Network. Eur J Cancer. 2021;155:127–35.
Fisher B, Dignam J, Bryant J, Wolmark N. Five versus more than five years of tamoxifen for lymph node-negative breast cancer: updated findings from the National Surgical Adjuvant Breast and Bowel Project B-14 randomized trial. J Natl Cancer Inst. 2001;93:684–90.
Hammel P, Huguet F, van Laethem JL, Goldstein D, Glimelius B, Artru P, et al. Effect of chemoradiotherapy vs chemotherapy on survival in patients with locally advanced pancreatic cancer controlled after 4 months of gemcitabine with or without erlotinib: The LAP07 Randomized Clinical Trial. JAMA. 2016;315:1844–53.
Hovey EJ, Field KM, Rosenthal MA, Barnes EH, Cher L, Nowak AK, et al. Continuing or ceasing bevacizumab beyond progression in recurrent glioblastoma: an exploratory randomized phase II trial. Neuro Oncol Pract. 2017;4:171–81.
Joss RA, Alberto P, Bleher EA, Ludwig C, Siegenthaler P, Martinelli G, et al. Combined-modality treatment of small-cell lung cancer: randomized comparison of three induction chemotherapies followed by maintenance chemotherapy with or without radiotherapy to the chest. Ann Oncol. 1994;5:921–8.
Kubota K, Furuse K, Kawahara M, Kodama N, Yamamoto M, Ogawara M, et al. Role of radiotherapy in combined modality treatment of locally advanced non-small-cell lung cancer. J Clin Oncol. 1994;12:1547–52.
Lebeau B, Chastang C, Allard P, Migueres J, Boita F, Fichet D. Six vs twelve cycles for complete responders to chemotherapy in small cell lung cancer: definitive results of a randomized clinical trial. The “Petites Cellules” Group. Eur Respir J. 1992;5:286–90.
Marshall A, Levine M, Hill C, Hale D, Thirlwall J, Wilkie V, et al. Treatment of cancer-associated venous thromboembolism: 12-month outcomes of the placebo versus rivaroxaban randomization of the SELECT-D Trial (SELECT-D: 12m). J Thromb Haemost. 2020;18:905–15.
Petracci E, Scarpi E, Passardi A, Biggeri A, Milandri C, Vecchia S, et al. Effectiveness of bevacizumab in first- and second-line treatment for metastatic colorectal cancer: ITACa randomized trial. Ther Adv Med Oncol. 2020;12:1758835920937427.
Sculier JP, Paesmans M, Bureau G, Giner V, Lecomte J, Michel J, et al. Randomized trial comparing induction chemotherapy versus induction chemotherapy followed by maintenance chemotherapy in small-cell lung cancer. European Lung Cancer Working Party. J Clin Oncol. 1996;14:2337–44.
Tummarello D, Mari D, Graziano F, Isidori P, Cetto G, Pasini F, Santo A, Cellerino R. A randomized, controlled phase III study of cyclophosphamide, doxorubicin, and vincristine with etoposide (CAV-E) or teniposide (CAV-T), followed by recombinant interferon-alpha maintenance therapy or observation, in small cell lung carcinoma patients with complete responses. Cancer. 1997;80:2222–9.
Auyeung SF, Long Q, Royster EB, Murthy S, McNutt MD, Lawson D, et al. Sequential multiple-assignment randomized trial design of neurobehavioral treatment for patients with metastatic malignant melanoma undergoing high-dose interferon-alpha therapy. Clin Trials. 2009;6:480–90.
Fu SS, Rothman AJ, Vock DM, Lindgren B, Almirall D, Begnaud A, et al. Program for lung cancer screening and tobacco cessation: Study protocol of a sequential, multiple assignment, randomized trial. Contemp Clin Trials. 2017;60:86–95.
Kelleher SA, Dorfman CS, Plumb Vilardaga JC, Majestic C, Winger J, Gandhi V, et al. Optimizing delivery of a behavioral pain intervention in cancer patients using a sequential multiple assignment randomized trial SMART. Contemp Clin Trials. 2017;57:51–7.
Sikorskii A, Wyatt G, Lehto R, Victorson D, Badger T, Pace T. Using SMART design to improve symptom management among cancer patients: a study protocol. Res Nurs Health. 2017;40:501–11.
Artman WJ, Nahum-Shani I, Wu T, Mckay JR, Ertefaie A. Power analysis in a SMART design: sample size estimation for determining the best embedded dynamic treatment regime. Biostatistics. 2020;21:432–48.
Almirall D, Lizotte DJ, Murphy SA. SMART Design Issues and the Consideration of Opposing Outcomes: Discussion of “Evaluation of Viable Dynamic Treatment Regimes in a Sequentially Randomized Trial of Advanced Prostate Cancer” by by Wang, Rotnitzky, Lin, Millikan, and Thall. J Am Stat Assoc. 2012;107:509–12.
Kim H, Ionides E, Almirall D. A sample size calculator for smart pilot studies. SIAM Undergrad Res Online. 2016;9:229.
Bigirumurame T, Uwimpuhwe G, Wason J. Sequential multiple assignment randomized trial studies should report all key components: a systematic review. J Clin Epidemiol. 2022;142:152–60.
Almirall D, Nahum-Shani I, Sherwood NE, Murphy SA. Introduction to SMART designs for the development of adaptive interventions: with application to weight loss research. Transl Behav Med. 2014;4:260–74.
Nahum-Shani I, Qian M, Almirall D, Pelham WE, Gnagy B, Fabiano GA, et al. Experimental design and primary data analysis methods for comparing adaptive interventions. Psychol Methods. 2012;17:457.
Download references
Acknowledgements
The work was part of a research project developed in the context of the Master’s Programme in Epidemiology of the University of Turin.
The authors received no specific funding for this work.
Author information
These authors jointly supervised this work: Giulia Lorenzoni, Elisabetta Petracci.
Authors and Affiliations
Unit of Biostatistics, Epidemiology and Public Health, Department of Cardiac Thoracic Vascular Sciences and Public Health, University of Padova, Padova, Italy
Giulia Lorenzoni, Ileana Baldi & Dario Gregori
Unit of Biostatistics and Clinical Trials, IRCCS Istituto Romagnolo per lo Studio dei Tumori (IRST) “Dino Amadori”, Meldola, Italy
Elisabetta Petracci, Emanuela Scarpi & Oriana Nanni
You can also search for this author in PubMed Google Scholar
Contributions
GL designed the work, acquired the data, interpreted the results, drafted the work; EP acquired the data, interpreted the results, drafted the work; ES acquired the data and revised the manuscript; IB designed the work, revised the manuscript; DG conceived the work, revised the manuscript; ON conceived the work, revised the manuscript. All authors approved the final version and agreed to be accountable for all aspects of the work.
Corresponding author
Correspondence to Dario Gregori .
Ethics declarations
Competing interests.
The authors declare no competing interests.
Ethics approval and consent to participate
Consent for publication, additional information.
Publisher’s note Springer Nature remains neutral with regard to jurisdictional claims in published maps and institutional affiliations.
Supplementary information
Supplementary tables, prisma checklist, rights and permissions.
Springer Nature or its licensor (e.g. a society or other partner) holds exclusive rights to this article under a publishing agreement with the author(s) or other rightsholder(s); author self-archiving of the accepted manuscript version of this article is solely governed by the terms of such publishing agreement and applicable law.
Reprints and permissions
About this article
Cite this article.
Lorenzoni, G., Petracci, E., Scarpi, E. et al. Use of Sequential Multiple Assignment Randomized Trials (SMARTs) in oncology: systematic review of published studies. Br J Cancer 128 , 1177–1188 (2023). https://doi.org/10.1038/s41416-022-02110-z
Download citation
Received : 20 July 2022
Revised : 05 December 2022
Accepted : 07 December 2022
Published : 26 December 2022
Issue Date : 30 March 2023
DOI : https://doi.org/10.1038/s41416-022-02110-z
Share this article
Anyone you share the following link with will be able to read this content:
Sorry, a shareable link is not currently available for this article.
Provided by the Springer Nature SharedIt content-sharing initiative
Quick links
- Explore articles by subject
- Guide to authors
- Editorial policies

Advertisement
A Systematic Review of Sequential Multiple-Assignment Randomized Trials in Educational Research
- Review Article
- Published: 09 February 2022
- Volume 34 , pages 1343–1369, ( 2022 )
Cite this article
- Jason C. Chow 1 , 2 &
- Lauren H. Hampton 3
846 Accesses
4 Citations
13 Altmetric
Explore all metrics
The purpose of this systematic review is to describe the state of the art of sequential multiple-assignment randomized trials in education research. An iterative, systematic search strategy yielded thirteen reports for synthesis. We coded eligible reports for study characteristics, population, intervention, outcomes, SMART design components, overall findings, and study quality. Of the thirteen included reports, nine were completed studies at either the full or the pilot design stage, and four were published protocols. All studies measured educational and/or psychosocial outcomes, few studies included measures of achievement, and studies were primarily conducted outside of the classroom setting. We evaluate the current uses of SMARTs in education research and discuss the promise of this design and how it is well-suited for the dynamic nature of education research, diverse student populations, and the field of educational research.
This is a preview of subscription content, log in via an institution to check access.
Access this article
Price includes VAT (Russian Federation)
Instant access to the full article PDF.
Rent this article via DeepDyve
Institutional subscriptions
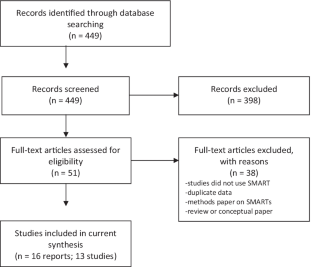
Similar content being viewed by others
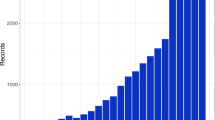
The Impact of Peer Assessment on Academic Performance: A Meta-analysis of Control Group Studies
Kit S. Double, Joshua A. McGrane & Therese N. Hopfenbeck
Ethical Considerations of Conducting Systematic Reviews in Educational Research
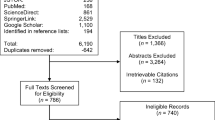
The Gamification of Learning: a Meta-analysis
Michael Sailer & Lisa Homner
Almirall, D., Compton, S. N., Gunlicks-Stoessel, M., Duan, N., & Murphy, S. A. (2012). Designing a pilot sequential multiple assignment randomized trial for developing an adaptive treatment strategy. Statistics in Medicine, 31 (17), 1887–1902.
Article Google Scholar
Almirall, D., DiStefano, C., Chang, Y. C., Shire, S., Kaiser, A., Lu, X., ... & Kasari, C. (2016). Longitudinal effects of adaptive interventions with a speech-generating device in minimally verbal children with ASD. Journal of Clinical Child & Adolescent Psychology, 45 (4), 442–456.
Almirall, D., Kasari, C., McCaffrey, D. F., & Nahum-Shani, I. (2018). Developing optimized adaptive interventions in education. Journal of Research on Educational Effectiveness, 11 (1), 27–34.
August, G. J., Piehler, T. F., & Bloomquist, M. L. (2016). Being “SMART” about adolescent conduct problems prevention: Executing a SMART pilot study in a juvenile diversion agency. Journal of Clinical Child & Adolescent Psychology, 45 (4), 495–509.
August, G. J., Piehler, T. F., & Miller, F. G. (2018). Getting “SMART” about implementing multi-tiered systems of support to promote school mental health. Journal of School Psychology, 66 , 85–96.
Barlow, D. H., & Hersen, M. (1973). Single-case experimental designs: Uses in applied clinical research. Archives of General Psychiatry, 29 (3), 319–325.
Chow JC, Granger KL, Broda MD, & Peterson N (2020). Predictive role of classroom management in literacy development in preschool children at risk for EBD. Behavioral Disorders , 0198742920972322.
Chow, J. C., & Hampton, L. H. (2019). Sequential multiple-assignment randomized trials: Developing and evaluating adaptive interventions in special education. Remedial and Special Education, 40 (5), 267–276.
Chow, J. C. (2020). Commentary–classroom motivation and learning disabilities: Consensus points and recommendations. Learning Disabilities: A Multidisciplinary Journal, 25 (2), 54–60.
Google Scholar
Chronis-Tuscano, A., Wang, C. H., Strickland, J., Almirall, D., & Stein, M. A. (2016). Personalized treatment of mothers with ADHD and their young at-risk children: A SMART pilot. Journal of Clinical Child & Adolescent Psychology, 45 (4), 510–521.
Collins, L. M., Murphy, S. A., & Bierman, K. L. (2004). A conceptual framework for adaptive preventive interventions. Prevention Science, 5 (3), 185–196.
Cook, T. D., Campbell, D. T., & Shadish, W. (2002). Experimental and quasi-experimental designs for generalized causal inference . Houghton Mifflin.
Crank, J. E., Sandbank, M., Dunham, K., Crowley, S., Bottema-Beutel, K., Feldman, J., & Woynaroski, T. G. (2021). Understanding the effects of naturalistic developmental behavioral interventions: A project AIM meta-analysis. Autism Research, 14 (4), 817–834.
Cronbach LJ, & Snow RE (1977). Aptitudes and instructional methods: A handbook for research on interactions. Irvington.
Czyz, E. K., King, C. A., Prouty, D., Micol, V. J., Walton, M., & Nahum-Shani, I. (2021). Adaptive intervention for prevention of adolescent suicidal behavior after hospitalization: a pilot sequential multiple assignment randomized trial. Journal of Child Psychology and Psychiatry, and Allied Disciplines, 62 (8), 1019–1031. https://doi.org/10.1111/jcpp.13383
Fairchild, A. J., & MacKinnon, D. P. (2009). A general model for testing mediation and moderation effects. Prevention Science, 10 (2), 87–99.
Fatori, D., de Bragança Pereira, C. A., Asbahr, F. R., Requena, G., Alvarenga, P. G., de Mathis, M. A., & Miguel, E. C. (2018). Adaptive treatment strategies for children and adolescents with obsessive-compulsive disorder: A sequential multiple assignment randomized trial. Journal of Anxiety Disorders, 58 , 42–50.
Fuchs, D., & Fuchs, L. S. (2006). Introduction to response to intervention: What, why, and how valid is it? Reading Research Quarterly, 41 (1), 93–99.
Fuchs, D., & Fuchs, L. S. (2019). On the importance of moderator analysis in intervention research: An introduction to the special issue. Exceptional Children, 85 (2), 126–128.
Gunlicks-Stoessel, M., Mufson, L., Westervelt, A., Almirall, D., & Murphy, S. (2016). A pilot SMART for developing an adaptive treatment strategy for adolescent depression. Journal of Clinical Child & Adolescent Psychology, 45 (4), 480–494.
Gunlicks-Stoessel, M., Mufson, L., Bernstein, G., Westervelt, A., Reigstad, K., Klimes-Dougan, B., & Vock, D. (2019). Critical decision points for augmenting interpersonal psychotherapy for depressed adolescents: A pilot sequential multiple assignment randomized trial. Journal of the American Academy of Child & Adolescent Psychiatry, 58 (1), 80–91.
Guy, W. (1976) Clinical Global Impressions, ECDEU Assessment Manual for Psychopharmacology, revised (DHEW Publ. No. ADM 76-338). National Institute of Mental Health, Rockville, 218–222.
Hampton, L. H., & Chow, J. C. (2021).Deeply tailoring adaptive interventions: Enhancing knowledge generation of SMARTs in special education. Remedial and Special Education. https://doi.org/10.1177/07419325211030669
Hampton LH, & Rodriguez EM (2021). Preemptive interventions for infants and toddlers with a high likelihood for autism: A systematic review and meta-analysis. Autism, 13623613211050433.
Hayes, A. F. (2017). Introduction to mediation, moderation, and conditional process analysis: A regression-based approach. Guilford publications.
Heppen, J. B., Kurki, A., & Brown, S. (2020). Can texting parents improve attendance in elementary school? A test of an adaptive messaging strategy. Appendix. NCEE 2020-006a. National Center for Education Evaluation and Regional Assistance.
Higgins, J. P. T., Altman, D. G., Gøtzsche, P. C., Jüni, P., Moher, D., Oxman, A. D., ... & Sterne, J. A. C. (2011). The Cochrane Collaboration’s tool for assessing risk of bias in randomised trials. BMJ (Online), 343(7829).
Kasari, C., Kaiser, A., Goods, K., Nietfeld, J., Mathy, P., Landa, R., & Almirall, D. (2014). Communication interventions for minimally verbal children with autism: A sequential multiple assignment randomized trial. Journal of the American Academy of Child & Adolescent Psychiatry, 53 (6), 635–646.
Kasari C, Shire S, Shih W, & Almirall D (2021). Getting SMART about social skills interventions for students with ASD in inclusive classrooms. Exceptional Children , 00144029211007148.
Kilbourne, A. M., Almirall, D., Eisenberg, D., Waxmonsky, J., Goodrich, D. E., Fortney, J. C., & Kyle, J. (2014). Protocol: Adaptive Implementation of Effective Programs Trial (ADEPT): Cluster randomized SMART trial comparing a standard versus enhanced implementation strategy to improve outcomes of a mood disorders program. Implementation Science, 9 (1), 1–14.
Kilbourne, A. M., Smith, S. N., Choi, S. Y., Koschmann, E., Liebrecht, C., Rusch, A., ... & Almirall, D. (2018). Adaptive School-based Implementation of CBT (ASIC): clustered-SMART for building an optimized adaptive implementation intervention to improve uptake of mental health interventions in schools. Implementation Science, 13 (1), 1–15.
Kim, J. S., Asher, C. A., Burkhauser, M., Mesite, L., & Leyva, D. (2019). Using a sequential multiple assignment randomized trial (SMART) to develop an adaptive K–2 literacy intervention with personalized print texts and app-based digital activities. AERA Open, 5 (3), 2332858419872701.
Ledford, J. R., & Gast, D. L. (Eds.). (2018). Single case research methodology . Routledge.
MacKinnon, D. P., Fairchild, A. J., & Fritz, M. S. (2007). Mediation analysis. Annual Review of Psychology , 593–614.
Murphy, S. A., Lynch, K. G., Oslin, D., McKay, J. R., & TenHave, T. (2007). Developing adaptive treatment strategies in substance abuse research. Drug and alcohol dependence, 88, S24–S30.
Nahum-Shani, I., Qian, M., Almirall, D., Pelham, W. E., Gnagy, B., Fabiano, G. A., & Murphy, S. A. (2012). Experimental design and primary data analysis methods for comparing adaptive interventions. Psychological Methods, 17 (4), 457–477.
Nahum-Shani I and Almirall D (2019). An introduction to adaptive interventions and SMART designs in education (NCSER 2020–001). U.S. Department of Education. Washington, DC: National Center for Special Education Research. Retrieved [date] from https://ies.ed.gov/ncser/pubs/ .
O’Keefe, V. M., Haroz, E. E., Goklish, N., Ivanich, J., Cwik, M. F., & Barlow, A. (2019). Employing a sequential multiple assignment randomized trial (SMART) to evaluate the impact of brief risk and protective factor prevention interventions for American Indian youth suicide. BMC Public Health, 19 (1), 1675.
Page, T. F., Pelham, W. E., III., Fabiano, G. A., Greiner, A. R., Gnagy, E. M., Hart, K. C., & Pelham, W. E., Jr. (2016). Comparative cost analysis of sequential, adaptive, behavioral, pharmacological, and combined treatments for childhood ADHD. Journal of Clinical Child & Adolescent Psychology, 45 (4), 416–427.
Pelham, W. E., Jr., Fabiano, G. A., Waxmonsky, J. G., Greiner, A. R., Gnagy, E. M., Pelham, W. E., III., & Karch, K. (2016). Treatment sequencing for childhood ADHD: A multiple-randomization study of adaptive medication and behavioral interventions. Journal of Clinical Child & Adolescent Psychology, 45 (4), 396–415.
Peterson, B. S., West, A. E., Weisz, J. R., Mack, W. J., Kipke, M. D., Findling, R. L., ... & Weersing, R. (2021). A Sequential Multiple Assignment Randomized Trial (SMART) study of medication and CBT sequencing in the treatment of pediatric anxiety disorders. BMC Psychiatry, 21 (1), 1–38.
Raudenbush, S. W., & Schwartz, D. (2020). Randomized experiments in education, with implications for multilevel causal inference. Annual Review of Statistics and Its Application, 7 , 177–208.
Roberts, M. Y., Curtis, P. R., Sone, B. J., & Hampton, L. H. (2019). Association of parent training with child language development: A systematic review and meta-analysis. JAMA Pediatrics, 173 (7), 671–680.
Schoenfelder, E. N., Chronis-Tuscano, A., Strickland, J., Almirall, D., & Stein, M. A. (2019). Piloting a sequential, multiple assignment, randomized trial for mothers with attention-deficit/hyperactivity disorder and their at-risk young children. Journal of Child and Adolescent Psychopharmacology, 29 (4), 256–267.
Snow, R. E. (1980). Aptitude, learner control, and adaptive instruction. Educational Psychologist, 15 (3), 151–158.
Speece, D. L. (1990). Aptitude-treatment interactions: Bad rap or bad idea? The Journal of Special Education, 24 (2), 139–149.
Sugai, G., & Horner, R. R. (2006). A promising approach for expanding and sustaining school-wide positive behavior support. School Psychology Review, 35 (2), 245–259.
Thall, P. F., Logothetis, C., Pagliaro, L. C., Wen, S., Brown, M. A., Williams, D., & Millikan, R. E. (2007). Adaptive therapy for androgen-independent prostate cancer: a randomized selection trial of four regimens. Journal of the National Cancer Institute, 99 (21), 1613–1622.
Yan, X., Matchar, D. B., Sivapragasam, N., Ansah, J. P., Goel, A., & Chakraborty, B. (2021). Sequential Multiple Assignment Randomized Trial (SMART) to identify optimal sequences of telemedicine interventions for improving initiation of insulin therapy: A simulation study. BMC medical research methodology, 21 (1), 1–11.
Download references
Author information
Authors and affiliations.
College of Education, University of Maryland, 3942 Campus Dr, College Park, MD, 20742, USA
Jason C. Chow
College of Behavioral & Social Sciences, University of Maryland, 7343 Preinkert Dr, College Park, MD, 20742, USA
College of Education, The University of Texas at Austin, 1912 Speedway, Stop D5000, Austin, TX, 78712, USA
Lauren H. Hampton
You can also search for this author in PubMed Google Scholar
Corresponding author
Correspondence to Jason C. Chow .
Additional information
Publisher’s note.
Springer Nature remains neutral with regard to jurisdictional claims in published maps and institutional affiliations.
Rights and permissions
Reprints and permissions
About this article
Chow, J.C., Hampton, L.H. A Systematic Review of Sequential Multiple-Assignment Randomized Trials in Educational Research. Educ Psychol Rev 34 , 1343–1369 (2022). https://doi.org/10.1007/s10648-022-09660-x
Download citation
Accepted : 19 January 2022
Published : 09 February 2022
Issue Date : September 2022
DOI : https://doi.org/10.1007/s10648-022-09660-x
Share this article
Anyone you share the following link with will be able to read this content:
Sorry, a shareable link is not currently available for this article.
Provided by the Springer Nature SharedIt content-sharing initiative
- Sequential multiple-assignment randomized trials
- Adaptive interventions
- Find a journal
- Publish with us
- Track your research
Advertisement
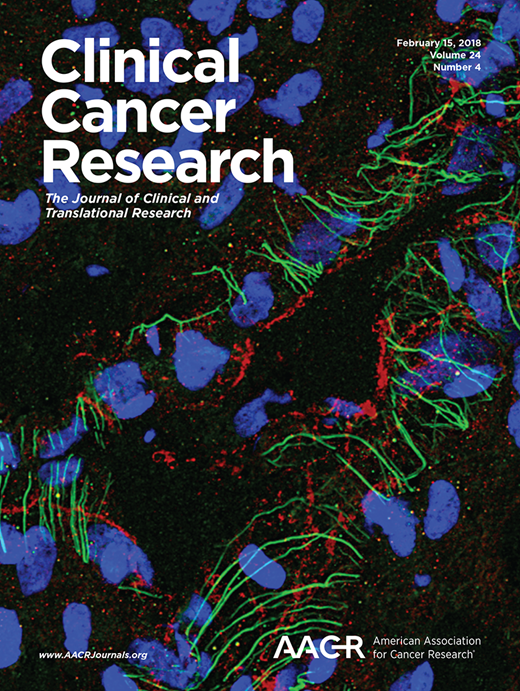
- Previous Article
- Next Article
Introduction
Smart design, hypothetical melanoma smart, disclosure of potential conflicts of interest, authors' contributions, acknowledgments, sequential, multiple assignment, randomized trial designs in immuno-oncology research.
- Funder(s): Memorial Sloan Kettering Cancer Center
- Award Id(s): P30 CA008748
- Principal Award Recipient(s): K.S. M.A. C. Panageas Postow Thompson
- Funder(s): PCORI
- Award Id(s): ME-1507-31108
- Principal Award Recipient(s): K.M. Kidwell
- Split-Screen
- Article contents
- Figures & tables
- Supplementary Data
- Peer Review
- Open the PDF for in another window
- Get Permissions
- Cite Icon Cite
- Search Site
- Version of Record February 14 2018
- Proof January 25 2018
- Accepted Manuscript August 23 2017
Kelley M. Kidwell , Michael A. Postow , Katherine S. Panageas; Sequential, Multiple Assignment, Randomized Trial Designs in Immuno-oncology Research. Clin Cancer Res 15 February 2018; 24 (4): 730–736. https://doi.org/10.1158/1078-0432.CCR-17-1355
Download citation file:
- Ris (Zotero)
- Reference Manager
Clinical trials investigating immune checkpoint inhibitors have led to the approval of anti–CTLA-4 (cytotoxic T-lymphocyte antigen-4), anti–PD-1 (programmed death-1), and anti–PD-L1 (PD-ligand 1) drugs by the FDA for numerous tumor types. In the treatment of metastatic melanoma, combinations of checkpoint inhibitors are more effective than single-agent inhibitors, but combination immunotherapy is associated with increased frequency and severity of toxicity. There are questions about the use of combination immunotherapy or single-agent anti–PD-1 as initial therapy and the number of doses of either approach required to sustain a response. In this article, we describe a novel use of sequential, multiple assignment, randomized trial (SMART) design to evaluate immune checkpoint inhibitors to find treatment regimens that adapt within an individual based on intermediate response and lead to the longest overall survival. We provide a hypothetical example SMART design for BRAF wild-type metastatic melanoma as a framework for investigating immunotherapy treatment regimens. We compare implementing a SMART design to implementing multiple traditional randomized clinical trials. We illustrate the benefits of a SMART over traditional trial designs and acknowledge the complexity of a SMART. SMART designs may be an optimal way to find treatment strategies that yield durable response, longer survival, and lower toxicity. Clin Cancer Res; 24(4); 730–6. ©2017 AACR .
Clinical trials investigating immune checkpoint inhibitors have led to the approval of anti–CTLA-4 (cytotoxic T-lymphocyte antigen-4), anti–PD-1 (programmed death-1), and anti–PD-L1 (PD-ligand 1) drugs by the FDA for numerous tumor types. Immune checkpoint inhibitors are a novel class of immunotherapy agents that block normally negative regulatory proteins on T cells and enable immune system activation. By activating the immune system rather than directly attacking the cancer, immunotherapy drugs differ from cytotoxic chemotherapy and oncogene-directed molecularly targeted agents. Cytotoxic chemotherapy or molecularly targeted agents generally provide clinical benefit during treatment and usually not after treatment discontinuation, whereas immunotherapy benefit may persist after treatment discontinuation.
The anti–CTLA-4 drug ipilimumab was approved for the treatment of metastatic melanoma in 2011 and as adjuvant therapy for resected stage III melanoma in 2015. Inhibition of CTLA-4 is also being tested in other malignancies. In melanoma, ipilimumab improves overall survival but is associated with 20% grade 3/4 immune related adverse events ( 1–6 ). Agents that inhibit PD-1 and PD-L1 have less immune-related adverse events than CTLA-4–blocking agents ( 7 ). PD-1 and PD-L1 agents have been approved by the FDA for use in multiple malignancies including, but not limited to, melanoma (nivolumab and pembrolizumab), non–small cell lung cancer (NSCLC; nivolumab, pembrolizumab, and atezolizumab), renal cell carcinoma (nivolumab), and urothelial carcinoma (atezolizumab; refs. 8–10 ). Combinations of checkpoint inhibitors that block both CTLA-4 and PD-1 are more effective than CTLA-4 blockade alone (ipilimumab) in patients with melanoma, but combination immunotherapy is associated with increased frequency and severity of toxicity. Although we build our framework on the FDA-approved combination of anti–PD-1 therapy and ipilimumab, as this is reflects the current landscape, one could replace the anti–PD-1 and ipilimumab combination with anti–PD-1 and any drug to reflect novel combination agents that may become available down the pipeline, such as inhibitors of indoleamine-2,3-dioxygenase (IDO).
Some individuals may not need combination therapy because they may respond to a single agent, and these individuals should not be subjected to increased toxicities associated with combination therapy. Defining this group of individuals, however, is difficult. Many trials are being proposed to evaluate combinations or sequences of immunotherapy drugs alone in combination with other treatments such as chemotherapy, radiation, and targeted therapies, or with varied doses and schedules (sequential versus concurrent). The goal of these trials is to increase efficacy and decrease toxicity ( 11 ).
The long-term effect of immune activation by these drugs is unknown. It is also unknown whether individuals need continued treatment. Oncologists must optimize a balance in the clinic, incorporating observed efficacy and toxicity, and informally implement treatment pathways so that treatment may change for an individual depending on the individual's status. Many of these treatment pathways are ad hoc , based on the physician's experience and judgement or information pieced together from several randomized clinical trials. Formalized, evidence-based treatment pathways to inform decision-making over the course of care are needed. Formal, evidence-based treatment guidelines that adapt treatment based on a patient's outcomes, including efficacy and toxicity, are known as treatment pathways, dynamic treatment regimens ( 12 ), or adaptive interventions ( 13 ). Specifically, a treatment pathway is a sequence of treatment guidelines or decisions that indicate if, when, and how to modify the dosage or duration of interventions at decision stages throughout clinical care ( 14 ). For example, in treating individuals with stage III or stage IV Hodgkin lymphoma, one treatment pathway is as follows: “Treat with two cycles of doxorubicin, bleomycin, vinblastine and dacarbazine (ABVD). At the end of therapy (6 to 8 weeks), perform positron emission tomography/computed tomography (PET/CT) imaging. Treat with an additional 4 cycles of ABVD if the scan scores 1–3 on the Deauville scale (considered a negative scan). Otherwise, if the scan scores 4–5 on the Deauville scale (considered a positive scan), switch treatment to escalated bleomycin, etoposide, docorubicin, cyclophosphamide, vincreistine, procarbazine and prednisone (eBEACOPP) for 6 cycles ( 15 ).” Note that one treatment pathway includes an initial treatment followed by subsequent treatment that depends on an intermediate outcome for all possibilities of that intermediate outcome.
Treatment pathways are difficult to develop in traditional randomized clinical trial settings because they specify adapting treatments over time for an individual based on response and/or toxicity. Treatments may have delayed effects such that the best initial treatment is not a part of the best overall treatment regimen. For example, one treatment may initially produce the best response rate, but that treatment may also be so aggressive that for those who did not have a response, they cannot tolerate additional treatment; whereas another treatment may produce a lower proportion of responders initially but can be followed by an additional treatment to rescue more nonresponders and lead to a better overall response rate and longer survival. Thus, treatments in combination or sequence do not necessarily result in overall best outcomes. The sequential, multiple assignment, randomized trial (SMART; refs. 16, 17 ) is a multistage trial that is designed to develop and investigate treatment pathways. SMART designs can investigate delayed effects as well as treatment synergies and antagonisms, and provide robust evidence about the timing, sequences, and combinations of immunotherapies. Furthermore, treatment pathways may be individualized to find baseline and time-varying clinical and pathologic characteristics associated with optimal response.
In this article, we describe a novel use of SMART design to evaluate immuno-oncologic agents. We provide a hypothetical example SMART design for metastatic melanoma as a framework for investigating immunotherapy treatment. We compare implementation of a SMART design with implementation of multiple traditional randomized clinical trials. We illustrate the benefits of a SMART over traditional trial designs and acknowledge the complexity of a SMART. SMART designs may be an optimal way to find treatment strategies that yield durable response, longer survival, and lower toxicity.
A SMART is a multistage, randomized trial in which each stage corresponds to an important treatment decision point. Participants are enrolled in a SMART and followed throughout the trial, but each participant may be randomized more than once. Subsequent randomizations allow for unbiased comparisons of post-initial randomization treatments and comparisons of treatment pathways. The goal of a SMART is to develop and find evidence of effective treatment pathways that mimic clinical practice.
In a generic two-stage SMART, participants are randomized between several treatments (usually 2–3; Fig. 1 ). Participants are followed, and an intermediate outcome is assessed over time or at a specific time. On the basis of the intermediate outcome, participants may be classified into groups, and they may be re-randomized to subsequent treatment. The intermediate outcome is a measure of early success or failure that allows the identification of those who may benefit from a treatment change. This intermediate outcome, also known as a tailoring variable, should have only a few categories so that it is a low-dimensional summary that is well defined, agreed upon, implementable in practice and gives early information about the overall endpoint. This intermediate outcome does not need to be defined as response/nonresponse, or more specifically as tumor response, but rather, it may be defined differently, such as adherence to treatment, a composite of efficacy measures, or efficacy and toxicity measures. It is imperative that the intermediate outcome is validated and replicable. Although the two-stage design is most commonly used, SMARTs are not limited to two stages, such as a SMART that investigated treatment strategies in prostate cancer ( 18 ).
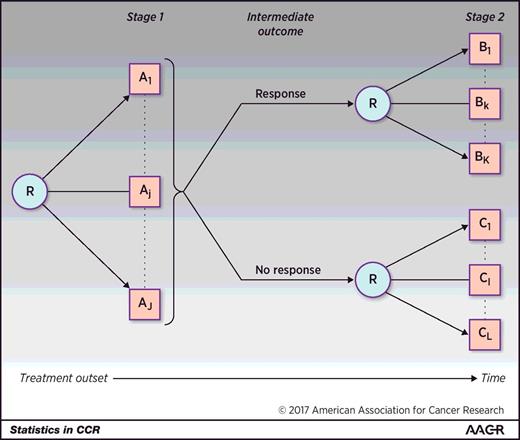
A generic two-stage SMART design where participants are randomized between any number of treatments A 1 to A J . Response is measured at some intermediate time point or over time such that responders are re-randomized in the second stage between any number of treatments B 1 to B K and nonresponders are re-randomized between any number of treatments C 1 to C L . The same participants are followed throughout the trial. R denotes randomization.
A SMART is similar to other commonly used trial designs but has unique features that enable the development of robust evidence of effective treatment strategies. The SMART design is a type of sequential factorial trial design in which the second-stage treatment is restricted based on the previous response. A SMART design is similar to a crossover trial in that the same participants are followed throughout the trial and participants may receive multiple treatments. However, in a SMART, subsequent treatment is based on the response to the previous treatment, and a SMART design takes advantage of treatment interactions as opposed to washing out treatment effects (i.e., a SMART does not require time in between treatments to eliminate carryover effects from the initial treatment on the assessment of the second-stage treatment).
We focus this overview on SMART designs that are nonadaptive. In a nonadaptive SMART, the operating characteristics of the trial, including randomization probabilities and eligibility criteria, are predetermined and fixed throughout the trial. Treatment may adapt within a participant based on intermediate response, but randomization probabilities or other trial-operating characteristics do not change for future participants based on previous participants' results.
By following the same participants over the trial, a SMART enables the development of evidence for treatment pathways that specify an initial treatment, followed by a maintenance treatment for responders and rescue treatment for nonresponders. These treatment pathways are embedded within a SMART design, but within the trial, participants are randomized to treatments based on the intermediate outcome to enable unbiased comparisons and valid causal inference. The end goal of the trial is to provide definitive evidence for treatment pathways to be used in practice. The SMART design has been used in oncology ( 19, 20 ), mental health ( 21 ), and other areas ( 22 ), but to our knowledge, this is the first description of using a SMART in immuno-oncology.
Ipilimumab and anti–PD-1 therapy currently are approved to treat metastatic melanoma. However, combinations of these and other immunotherapy drugs may cause toxic events, and it remains unclear whether patients should start with these combinations or start with single agent anti–PD-1 therapy and receive these additional treatments upon disease progression. There are also questions about the number of doses required to sustain a response for single-agent or combination therapy. The best treatment strategy that may provide enough therapy for sustained response and limit toxicities is unknown. A SMART design may address these questions to provide rigorous evidence for the best immunotherapy treatment pathway for individuals. Our proposed example focuses on patients with BRAF wild-type metastatic melanoma to avoid complexities of additionally considering incorporation of BRAF and MEK inhibitors into the treatment regimen of patients with BRAF-mutant melanoma.
In a hypothetical SMART design to investigate treatment strategies, including anti–PD-1 therapy and ipilimumab, participants may be randomized in the first stage to receive four doses of single-agent anti–PD-1 therapy (pembrolizumab 2 mg/kg or nivolumab 240 mg) or combination nivolumab (1 mg/kg), and ipilimumab (3 mg/kg; Fig. 2 , note these drugs could be replaced with any novel immunotherapy or approved drug). During follow-up, participants would be evaluated for their tumor response; the intermediate outcome in this SMART would be defined by disease response after four doses of immunotherapy (week 12). Although Response Evaluation Criteria in Solid Tumors (RECIST) could be used to define disease response, favorable response could also be defined as any decline in total tumor burden, even in the presence of new lesions, as specified by principles related to immune-related response criteria ( 23 ).
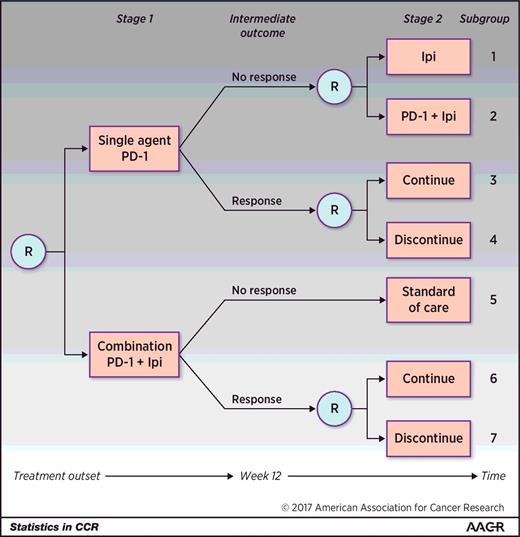
A hypothetical two-stage SMART design in the setting of BRAF wild-type metastatic melanoma. Participants are initially randomized to either single-agent anti–PD-1 therapy or to a combination of anti–PD-1 therapy + ipilimumab (Ipi). Note that Ipi may be replaced by any novel combination agent. After four doses or approximately 12 weeks, response is measured. Those who did not respond to the single agent are re-randomized to receive Ipi or the combination. Those who did respond to single-agent anti–PD-1 are re-randomized to continue the single agent or discontinue therapy. Those who did not respond initially to the combination receive standard of care and those who did respond are re-randomized to continue the combination or discontinue therapy. Subgroups 1 to 7 denote the subgroups that any one participant may fall into. There are six embedded treatment pathways in this SMART, and each one is made up of 2 subgroups: {1,3}, {1,4}, {2,3}, {2,4}, {5,6}, and {5,7}. R denotes randomization.
In the second stage of the trial, responders to either initial treatment would be re-randomized to continue versus discontinue their initial treatment. Specifically, participants who responded to single agent anti–PD-1 would be re-randomized to continue current treatment for additional doses up to 2 years or to discontinue treatment, and participants who responded to the combination of anti–PD-1 + ipilimumab would be re-randomized to continue anti–PD-1 maintenance or discontinue treatment. Participants who did not respond to single-agent anti–PD-1 by 12 weeks would be re-randomized to receive ipilimumab or the combination of anti–PD-1 and ipilimumab. Participants who did not respond to the combination therapy would receive the standard of care (e.g., oncogene-directed targeted therapy if appropriate, chemotherapy, or considered for clinical trials; Fig. 2 ). As newer drugs become available and are promising for nonresponders to combination therapy, we anticipate that there could be an additional randomization for these nonresponders to explore additional treatment pathways. All participants would be followed for at least 28 months. The overall outcome of the trial would be overall survival. Any participant who experienced major toxicity at any time or progressive disease in the second stage would be removed from the study and treated as directed by the treating physician.
Participants belong to one subgroup ( Fig. 2 ) in a SMART. Two subgroups make up one treatment pathway, since a treatment pathway describes the clinical guidelines for initial treatment and subsequent treatment for both responders and nonresponders ( Fig. 2 ). Although there are seven subgroups that a participant may belong to, there are six embedded treatment pathways in this SMART design. The six treatment pathways include the following:
(1) First begin with single-agent anti–PD-1 therapy. If no response to single-agent anti–PD-1 therapy, then switch to single-agent ipilimumab. If response to single-agent anti–PD-1, then continue treatment (subgroups 1 and 3);
(2) First begin with single-agent anti–PD-1 therapy. If no response to single-agent anti–PD-1 therapy, then switch to single-agent ipilimumab. If response to single-agent anti–PD-1, then discontinue treatment (subgroups 1 and 4);
(3) First begin with single-agent anti–PD-1 therapy. If no response to single-agent anti–PD-1 therapy, then add ipilimumab to anti–PD-1 therapy. If response to single-agent anti–PD-1 therapy, then continue treatment (subgroups 2 and 3);
(4) First begin with single-agent anti–PD-1 therapy. If no response to single-agent anti–PD-1 therapy, then add ipilimumab to anti–PD-1 therapy. If response to single-agent anti–PD-1 therapy, then discontinue treatment (subgroups 2 and 4);
(5) First begin with combination anti–PD-1 therapy + ipilimumab. If no response to combination anti–PD-1 therapy + ipilimumab, then receive standard of care. If response to combination anti–PD-1 therapy + ipilimumab, then continue treatment (subgroups 5 and 6); and
(6) First begin with combination anti–PD-1 therapy + ipilimumab. If no response to combination anti–PD-1 therapy + ipilimumab then receive standard of care. If response to combination anti–PD-1 therapy + ipilimumab, then discontinue treatment (subgroups 5 and 7).
A SMART may have several scientific aims, some of which may resemble those of traditional trials and some, pertaining to the treatment pathways, differ. It is important, as in standard trials, to identify and power on a primary aim. Subsequent aims and multiple comparisons may be additionally powered for using any type I error-control method ( 24 ). In metastatic melanoma, the SMART may be interested in answering one of following four questions:
(1) Does a treatment strategy that begins with single-agent anti–PD-1 or combination anti–PD-1 and ipilimumab therapy lead to the longest overall survival?
(2) For responders to initial therapy, does continuing or discontinuing treatment provide the longest overall survival?
(3) For nonresponders to single-agent anti–PD-1 therapy, does ipilimumab or the combination of ipilimumab and anti–PD-1 therapy provide the longest overall survival?
(4) Is there a difference in the overall survival between the six embedded treatment pathways?
Questions similar to numbers 1, 2, and 3 could be answered in three separate, traditional, parallel-arm clinical trials. The traditional paradigm would run a single-stage trial (e.g., single-agent vs. combination therapy) to determine the most effective therapy. A first trial may investigate single agent anti–PD-1 versus the combination of anti–PD-1 and ipilimumab. Another trial with a randomized discontinuation design could identify if continuing or discontinuing treatment leads to longer overall survival for individuals who received the most effective therapy (e.g., anti–PD-1 alone or in combination with ipilimumab). And a third trial could determine for those refractory to anti–PD-1 therapy, if ipilimumab or the combination of ipilimumab and anti–PD-1 therapy results in longer survival. For each of these three traditional trials, power and analyses are standard in terms of powering for and analyzing a two-group comparison with a survival outcome.
If question 1, 2, or 3 is the primary aim of a SMART, the sample size and analysis plan is also standard; however, for questions 2 and 3, the calculated sample size must be inflated. For question 2, the sample size must be inflated on the basis of the assumed response rates to first-stage therapies. Specifically, if 40% respond to single-agent therapy and 55% to combination therapy, the calculated two-group comparison sample size must be increased by these amounts to ensure that in the SMART there will be sufficient responders in the second stage. For question 3, the sample size must also be inflated for the expected percentage of nonresponders to anti–PD-1 therapy. Similarly, in a standard one-stage trial to address question 2 (or 3), more patients would need to be screened to account for the response status, but unlike a SMART, the nonresponders (responders) would not be followed. Furthermore, implementing three separate trials may not provide robust evidence for entire treatment pathways and instead provide evidence for only the best treatments at specific time points.
For a SMART powered on question number 1, 2, or 3, the analysis of treatment pathways would be exploratory and hypothesis generating to be confirmed in a follow-up trial. Instead, the SMART may be powered to compare the embedded treatment pathways (question 4) in contrast with the stage-specific differences. Comparisons of pathways require power calculations and analytic methods specific to SMART designs. Currently, the only sample-size calculator available for a SMART design with a survival outcome compares two specific treatment pathways using a weighted log-rank test. This calculator is only applicable for designs similar to the hypothetical melanoma SMART if the non-responders to anti–PD-1 therapy were not re-randomized (i.e., if there were only 4 embedded treatment pathways instead of 6; ref. 25 ). Any other SMART design (e.g., our hypothetical design in Fig. 2 ) or any other test (e.g., a global test of equality across all treatment pathways or finding the best set of treatment pathways using multiple comparisons with the best) requires statistical simulation. Other sample size calculations exist for survival outcomes but do not have an easy-to-implement calculator ( 26, 27 ). Methods are available to estimate survival ( 28, 29 ) and compare ( 25, 26, 30–32 ) treatment pathways with survival outcomes, and R packages ( 33 ) can aid in the analysis.
In this example, we calculate sample sizes of implementing three single trials versus implementing one trial using a SMART design. For the first single-stage trial, we assume a log-rank test, survival rates of 80% and 68%, respectively, at 1 year for combination and single agent anti–PD-1, exponential survival distributions, 1 year for accrual, and an additional 2.5 years of follow-up. The same assumptions were applied for continuing initial treatment versus discontinuing the initial (this is a conservative sample size for this trial, since the survival rates at 1 year would likely be closer together and require more patients). To have the same assumptions across the single-stage trials and SMART design, the survival rate at 1 year for those who did not respond to single agent anti–PD-1 therapy and received ipilimumab was set at 68% and for those who received the combination anti–PD-1 and ipilimumab was set to 74%. Parameters for the SMART were specified to mimic the single-stage settings with the additional assumptions of a response rate to initial therapy being 40% and 1-year survival rates of 69%, 68%, 75%, 74%, 80%, and 74% for the treatment pathways 1 through 6, respectively. For the SMART, a weighted log-rank test of any difference in the six treatment pathways was used for power via simulation ( 30, 33 ). With these assumptions, 570 participants are required to observe any difference in the six embedded treatment pathways within 1 SMART ( Table 1 ). This sample size is less than the 1,142 participants that are required by summing the sample sizes with the same assumptions using three traditional single-stage trials. We note that using a global test in the SMART allows for less participants, and that potentially, one of the trials in the single-stage trial setting may be dropped on the basis of previous trial results. However, a SMART allows us to answer many questions simultaneously and find optimal treatment pathways potentially ignored in the single-stage setting.
Comparison of the sample sizes needed for three single trials versus one SMART design
NOTE: The trials in approach 1 would require a total of 1,142 participants versus 570 total participants from one SMART.
A SMART would most likely require less time from start to finish than the single-stage trials because it is unlikely that the single-stage trials would run simultaneously (because the trials based on response to initial treatment would require an actionable result from the first trial; ref. 34 ). Furthermore, because participants are followed throughout the trial and offered follow-up treatment, individuals may be more likely to enroll in the SMART (i.e., the sample of participants in a SMART may be more generalizable) and adhere to treatment ( 34 ).
Beyond the sequences of treatments in a SMART design that are tailored to an individual based on intermediate outcome, additional analyses (like subgroup analyses in traditional trials) may evaluate more individualized treatment pathways. Information, including demographic, clinical, and pathologic data collected at baseline and between baseline and the measurement of the intermediate outcome, may be used to further individualize treatment sequences for better overall survival. To further personalize treatment pathways, the analysis requires methods specific for SMART data such as Q-learning or other similar methods ( 35, 36 ). Briefly, Q-learning, borrowed from computer science, is an extension of regression to sequential treatments ( 37 ). Q-learning is a series of regressions used to construct a sequence of treatment guidelines that maximize the outcome (e.g., find more detailed treatment pathways that include baseline and time-varying variables associated with the longest survival). It may be as beneficial for some individuals to receive single-agent as combination therapy even when combination therapy is better when averaged across all individuals. In addition, a subgroup of individuals may benefit more from single-agent therapy because of savings in cost and toxicity compared to combination therapy. These questions are unlikely to be powered for in the SMART, but a priori hypotheses can direct analysis and lead to the identification of more personalized treatment pathways that can be validated in subsequent trials.
This article has focused on an example SMART in BRAF wild-type metastatic melanoma to answer questions about the best treatment pathways, including ipilimumab and anti–PD-1 therapy. As new immunotherapies are available for trials, ipilimumab may ultimately be replaced in this type of design by one of the more novel drugs (e.g., inhibitors of the immunosuppressive enzyme IDO or other checkpoint inhibitors such as drugs targeting lymphocyte-activation gene 3, “LAG-3”). Our proposed SMART design could be considered as a template for testing any number of these potential future possible combinations.
A SMART design may be a more efficient trial design to understand which immunotherapy treatment pathways in BRAF wild-type metastatic melanoma lead to the longest overall survival. SMARTs can definitively evaluate the treatment pathways that many physicians use in practice, leading to the recommendation of treatments over time based on individual response. A single SMART can enroll and continue to follow participants throughout the course of care to provide evidence for beginning treatment with single-agent anti–PD-1 or combination therapy and the optimal number of doses needed to sustain a response while limiting toxicity.
Of course, a SMART design is not limited to providing robust evidence for treatment pathways in BRAF wild-type metastatic melanoma but can help develop and test treatment pathways that lead to optimal outcomes in other melanomas, cancers, and diseases. We acknowledge our SMART proposal is inherently limited by heterogeneity in some of the treatment pathways, such as in the “Standard-of-care” box in subgroup 5. In our melanoma example, this box could include diverse treatments such as chemotherapy, inhibitors of other molecular drivers such as imatinib for patients with KIT mutations, and other potentially effective immunotherapy agents. How the various treatments within this pathway affect overall outcomes remains unknown in our proposed design.
A SMART requires less overall participants and can be implemented and analyzed in a shorter period of time than executing several single-stage, standard two-arm trial designs ( 34 ). However, a commitment to more participants at the initiation of the trial for a SMART is needed than for individual standard trials, and logistics may be more complex in a SMART by re-randomizing participants at an intermediate time point ( 34 ). With current technology that can handle multisite interim randomizations or the ability to randomize participants upfront to follow particular treatment pathways, the increased logistics should not outweigh the benefits of finding optimal immunotherapy treatment pathways from SMART designs.
The SMART design, even when powered on questions regarding the best initial treatment in a pathway or best strategy for responders or nonresponders (i.e., question 1, 2, or 3 from the previous section), may be more beneficial than multiple traditional single-stage designs. A SMART can conclusively answer one question with additional analyses to address questions concerning treatment pathways that may be relevant to clinical practice, such as how long to remain on immunotherapy. Furthermore, SMART designs can identify treatment interactions when treatments differ in the first and second stages (i.e., a SMART design that differs from that in Fig. 2 by re-randomizing to different treatments in the second stage as opposed to continuing or discontinuing initial treatment), and there may be delayed effects of initial treatments that modify the effects of follow-up treatments. Single-stage trials cannot evaluate these interactions between first and second-stage treatments dependent on intermediate outcomes.
More novel trial designs, including the SMART, may be needed to answer pertinent treatment questions and provide robust evidence for effective treatment regimens, especially in immuno-oncology research where novel combinations are frequently being proposed. A SMART can examine treatment sequences and combinations of immunotherapies and other drugs that lead to the longest overall survival with decreased toxicities. SMART designs may be able to verify potential optimal treatment pathways identified from dynamic mathematical modeling ( 38 ). SMARTs may require a paradigm shift for practicing physicians, pharmaceutical companies, and guidance agencies to begin to test and approve treatment regimens that may adapt within an individual along the course of care, as opposed to testing and approving agents at particular snapshots in time and piecing these snapshots together trusting that these pieces tell the full story.
M.A. Postow reports receiving commercial research grants from Bristol-Myers Squibb, speakers bureau honoraria from Bristol-Myers Squibb and Merck, and is a consultant/advisory board member for Array BioPharma, Bristol-Myers Squibb, Merck, and Novartis. No potential conflicts of interest were disclosed by the other authors.
Conception and design: K.M. Kidwell, M.A. Postow, K.S. Panageas
Development of methodology: K.M. Kidwell, K.S. Panageas
Analysis and interpretation of data (e.g., statistical analysis, biostatistics, computational analysis): K.M. Kidwell, K.S. Panageas
Writing, review, and/or revision of the manuscript: K.M. Kidwell, M.A. Postow, K.S. Panageas
Study supervision: K.S. Panageas
This study was support by the Memorial Sloan Kettering Cancer Center Core grant (P30 CA008748; to K.S. Panageas and M.A. Postow; principle investigator: C. Thompson) and PCORI Award (ME-1507-31108; to K.M. Kidwell).
Citing articles via
Email alerts.
- Online First
- Collections
- Online ISSN 1557-3265
- Print ISSN 1078-0432
AACR Journals
- Blood Cancer Discovery
- Cancer Discovery
- Cancer Epidemiology, Biomarkers & Prevention
- Cancer Immunology Research
- Cancer Prevention Research
- Cancer Research
- Cancer Research Communications
- Clinical Cancer Research
- Molecular Cancer Research
- Molecular Cancer Therapeutics
- Info for Advertisers
- Information for Institutions/Librarians

- Privacy Policy
- Copyright © 2023 by the American Association for Cancer Research.
This Feature Is Available To Subscribers Only
Sign In or Create an Account
- Open access
- Published: 30 September 2021
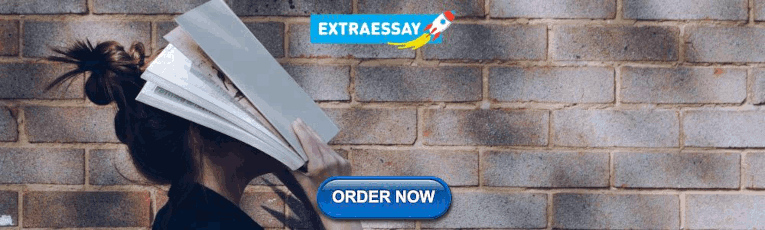
Sequential Multiple Assignment Randomized Trial (SMART) to identify optimal sequences of telemedicine interventions for improving initiation of insulin therapy: A simulation study
- Xiaoxi Yan 1 ,
- David B. Matchar 2 , 3 ,
- Nirmali Sivapragasam 2 ,
- John P. Ansah 2 ,
- Aastha Goel 2 &
- Bibhas Chakraborty 1 , 4 , 5
BMC Medical Research Methodology volume 21 , Article number: 200 ( 2021 ) Cite this article
2822 Accesses
4 Citations
1 Altmetric
Metrics details
To examine the value of a Sequential Multiple Assignment Randomized Trial (SMART) design compared to a conventional randomized control trial (RCT) for telemedicine strategies to support titration of insulin therapy for Type 2 Diabetes Mellitus (T2DM) patients new to insulin.
Microsimulation models were created in R using a synthetic sample based on primary data from 63 subjects enrolled in a pilot study of a smartphone application (App), Diabetes Pal compared to a nurse-based telemedicine strategy (Nurse). For comparability, the SMART and an RCT design were constructed to allow comparison of four (embedded) adaptive interventions (AIs).
In the base case scenario, the SMART has similar overall mean expected HbA1c and cost per subject compared with RCT, for sample size of n = 100 over 10,000 simulations. SMART has lower (better) standard deviations of the mean expected HbA1c per AI, and higher efficiency of choosing the correct AI across various sample sizes. The differences between SMART and RCT become apparent as sample size decreases. For both trial designs, the threshold value at which a subject was deemed to have been responsive at an intermediate point in the trial had an optimal choice (i.e., the sensitivity curve had a U-shape). SMART design dominates the RCT, in the overall mean HbA1c (lower value) when the threshold value is close to optimal.
Conclusions
SMART is suited to evaluating the efficacy of different sequences of treatment options, in addition to the advantage of providing information on optimal treatment sequences.
Peer Review reports
Introduction
A major objective of clinical trials, particularly randomized controlled trials (RCTs) is to identify which of two or more therapies is most effective. However, people often differ in their response to the same intervention. When a treatment that works for most people based on an RCT is not effective for a particular patient, in clinical practice the next step typically is to try something else. The next choice in this “trial and error” process would, ideally, be informed by evidence. However, clinical trials in which individuals are randomized to sequences of treatment strategies are seldom used [ 1 ].
An alternative to an idiosyncratic series of choices are decision rules such as those embodied in guidelines developed by medical professional organizations: a combination of expert opinion, behavioral, psychosocial and biological theories, and observational studies to formulate adaptive treatment algorithms, or adaptive interventions (AIs) [ 2 , 3 ]. While clinical guidelines may reduce variability from practice to practice, they do not alleviate the scientific uncertainty about which sequence is actually optimal. The recommendations become the subject of potential future research.
Experimental trial designs have been proposed for development and optimization of treatment sequences. One such design is the Sequential Multiple Assignment Randomized Trial (SMART) [ 2 , 4 ]. Adaptive interventions are treatment algorithms wherein treatment is sequentially modified over time based on individual’s response. The rationale is that by adjusting the treatment type and level as a function of time-dependent measures such as response to the past treatment, the long-term outcome is optimized [ 2 , 5 ].
Most experience with SMARTs has been limited to mental health and behavioral sciences [ 2 , 4 ], and Phase 2 trials in oncology [ 6 ]. SMART is particularly attractive in cancer therapy as sequential treatment based on intermediate response is already well-established. However, SMART has potential value to scientifically address problems in a wide range of contexts, including the use of technology such as telemedicine to encourage health-promoting behaviors [ 7 ].
Telemedicine is the provision of healthcare services and the exchange of healthcare information using information and communication technology across distances [ 8 , 9 ]. It is used in multiple areas of clinical practice, e.g., surgical practices [ 10 , 11 , 12 ], management of chronic diseases [ 13 ], addiction management [ 14 ] and palliative care [ 15 , 16 ]. The necessity for and utilization of telemedicine has significantly accelerated, when many in-person clinical activities are deferred or suspended, as a result of the on-going coronavirus disease of 2019 (Covid-19) pandemic [ 17 , 18 ]. What is becoming evident in this field is that “one size does not fit all”. Studies have shown that telemedicine interventions are more likely to have a positive effect on users’ self-efficacy, knowledge relevant to their condition, and behavioral and clinical outcomes [ 19 ]. However, not all patients are receptive to a particular mode of delivery. A key to establishing the effective and cost-effective application of telemedicine is understanding how these approaches fit into real-world care, in particular as part of a sequence that maximizes the proportion of patients who ultimately respond to good effect.
With this in mind, we sought to examine the value of a SMART design compared to an RCT for two telemedicine strategies to support titration of insulin therapy for Type 2 Diabetes Mellitus (T2DM) patients new to insulin: (1) a largely self-contained smartphone app, Diabetes Pal [ 20 ] and (2) a nurse-based telephone consultation service, SingHealth Polyclinics’ (SHP) Insulin Initiation Telecare Program (see the Methods section for details about these two telemedicine modalities). For comparability, the SMART and an RCT designs were constructed to allow comparison of various sequences of the two telemedicine strategies. The basis for this comparison is microsimulation using data derived from a pilot clinical trial of Diabetes Pal [ 20 ]. We sought to demonstrate the impact of the two trial designs on improvement in chronic blood glucose control as measured by change in glycated hemoglobin (HbA1c), and trial cost for the study population. In sensitivity analysis we examined how these measures of value were affected by various aspects of trial design, including the operating characteristics of the measure of responsiveness to initial treatment measure used to determine whether to continue or switch treatment.
Overview of the Simulation Study
The purpose of our simulation study was to conduct a head-to-head comparison between two design approaches intended to identify the optimal sequence of the two telemedicine modalities for titration of insulin dose in insulin-naïve diabetic patients. Although it is impractical to compare the design approaches directly using the same set of participants in real-life empirical studies, such comparisons are possible in a computer simulation. Specifically, for this study we developed a microsimulation created in R 3.6.1 [ 21 ]. The synthetic subjects were generated based on the characteristics of the real subjects in the pilot study of the Diabetes Pal app [ 20 ].
Two Telemedicine Intervention Modalities
Here we briefly describe the telemedicine intervention modalities that were compared in the pilot study, and informed by that, were considered in our simulation study.
SingHealth Polyclinics’ Insulin Initiation Telecare Program (Nurse) : The program was designed to support insulin initiation for patients with T2DM at the primary care practices of SingHealth, the largest public healthcare group in Singapore. Designated primary care nurses were trained as care managers, to assist patients with insulin initiation via weekly telephone consultations. These consultations included checking of current insulin dose and presence of symptoms of hypoglycemia, and titration of next insulin dose. Throughout this article, we will refer to this telemedicine intervention as ‘ Nurse ’.
Smartphone Application based Telecare Program (App) : Under this program, self-titration using the smartphone app Diabetes Pal [ 20 ] (Fig. 1 ) with minimal telephone-based support from care managers for insulin initiation, was proposed. Diabetes Pal is a smartphone application that allows a diabetic patient to self-titrate their insulin doses. Patient self-titration of insulin dose based on a prescribed algorithm has been shown to be safe and efficacious in improving glycemic control [ 22 ]. The app was developed by Integrated Health Information Systems Ltd. (IHiS) and has been tested for its feasibility to deliver the insulin titration algorithm in insulin-naïve patients in a pilot study recently conducted at the Singapore General Hospital [ 20 ]. Throughout this article, we will refer to this telemedicine intervention as ‘ App ’.
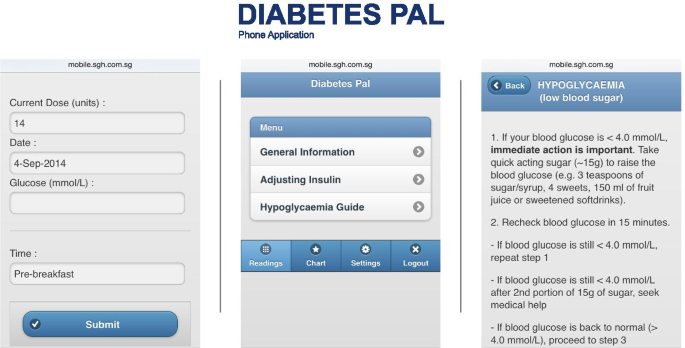
Diabetes pal. From "Diabetes Pal" by Integrated Health Information Systems ( https://www.ihis.com.sg/Project_Showcase/Mobile_Applications/Pages/Diabetes_Pal.aspx ). Copyright 2021 by Integrated Health Information Systems (IHIS) Pte Ltd
Operationalization of Competing Trial Designs
To compare a traditional RCT and SMART for evaluation of effectiveness and cost in the trial context, we implemented a microsimulation of these two trial designs run over a 12-week “study” period.
SMART Design
The SMART design operates in two stages. At stage one, all patients were randomized with a 1:1 ratio between Nurse and App. However, at the end of stage 1 (6 weeks from the initial randomization), patients were categorized as either a responder (R = 1) or a non-responder (R = 0) based on their reduction in HbA1c value in the 6-week period. Based on evidence in literature [ 23 ], insulin therapy is rapidly effective and known to reduce HbA1c levels in the range of 1.5 to 3.5 (conditional on other baseline values). In the pilot study data, the mean reduction in HbA1c after 6 weeks was 0.92 (SD = 0.71). Considering these pieces of information and clinical expert inputs, in the base-case scenario, the threshold for declaring a response was assumed to be 0.5% (i.e., the patient was considered a responder if the reduction in HbA1c from baseline is ≥0.5%, and a non-responder otherwise.).
The value was also varied in sensitivity analysis. The responders to the first-stage intervention continued with the same intervention in stage 2 (weeks 6–12 of the study). However, the non-responders were re-randomized to either a switch to the intervention not tried before for the same patient or a combined intervention (App + Nurse). The non-responders were re-randomized in a 1:1 ratio between the switch option and the combination option. A schematic of the SMART design is presented in Fig. 2 (a). Because of the re-randomization at stage 2, the current SMART design offers a comparison between four embedded adaptive interventions (AIs) (see [ 24 ]), described in Table 1 .
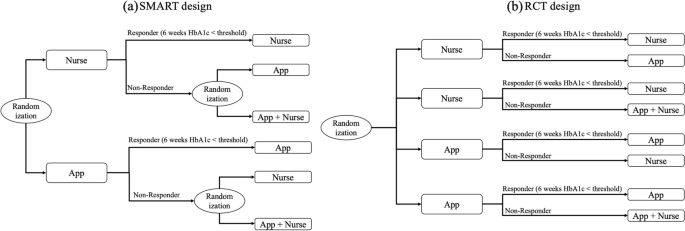
The (a) SMART and (b) RCT design in comparison. Each intervention component (Nurse, App, App + Nurse) is for 6 weeks long. (a) Randomisation happens at baseline for stage 1 intervention and at week 6 for stage 2 intervention, given stage 1 treatment and response. The four AIs are embedded in the design as a result. (b) Randomisation happens at baseline only, so the four arms correspond to the four AIs
The primary outcome (Y) was the HbA1c measurement at the end of the trial, and was recorded for all patients. The expected outcomes corresponding to the four embedded adaptive interventions are denoted E ( Y ) AI 1 , E ( Y ) AI 2 , E ( Y ) AI 3 , and E ( Y ) AI 4 . Also, the average outcome corresponding to the best AI from the SMART is denoted by min[ E ( Y ) AI 1 , E ( Y ) AI 2 , E ( Y ) AI 3 , E ( Y ) AI 4 ] and the best AI being \(\arg \underset{j\in \left\{1,..,4\right\}}{\ \min }E{(Y)}_{AIj}\) ; this is a key performance metric of the SMART design that we compare with the best outcome from the RCT design, in our simulation study. Note that for SMART, E ( Y ) AI 1 , E ( Y ) AI 2 , E( Y ) AI 3 , and E ( Y ) AI 4 need to be estimated using the inverse probability weighting method [ 25 ].
The RCT design (Fig. 2 (b)) is a conventional randomization designed to test the same sequences of treatments as the SMART design with the same intermediate evaluation at 6-weeks for responsiveness to initial treatment. However, for individuals deemed not to respond to the initial treatment, the new treatment was established at the time of initial randomization rather than at 6-weeks. In other words, the four AIs are separate arms in the design, where individuals are assigned to one of the AI arms at the start of the trial. This is different from the SMART design, where the AIs are embedded. As with SMART, effectiveness and cost are assessed based on final HbA1c and cost at the trial end at 12 weeks.
Data Generation Model
Baseline hba1c.
The pilot study [ 20 ] of the Diabetes Pal smartphone app enrolled 66 insulin naïve patients with suboptimal glycemic control of HbA1c ≥ 7.5% despite use of 2 or more oral glucose lowering drugs. These patients were between 30 and 70 years of age. Of these 66 recruited subjects, only 63 patients had complete follow-up data. Based on the baseline HbA1c measurements of these 63 patients, a normal distribution with mean = 9.73 and SD = 1.37 was used to generate the baseline HbA1c values ( Y 0 ) of the hypothetical patients in the simulation model. Detailed description of the model generation and algorithm may be found in the Additional file 1 .
Receptiveness
In addition to the starting level of glycemic control measured by HbA1c, two additional subject characteristics were assigned at baseline: (1) receptiveness to the App ( Rc A ), and (2) receptiveness to the Nurse ( Rc N ). A patient is deemed “receptive” if their engagement with the specific intervention had more than a nominal impact on their tendency to improve glycemic control. This was operationalized as a binary indicator variable (1 = receptive, 0 = non-receptive). Since information on receptiveness was not collected during the pilot study, estimates from the literature were utilized. Specifically, according to Deloitte’s Global Mobile Consumer Survey 2016 for UK [ 26 ], 69% of the smartphone users made standard voice calls weekly. It was assumed that the smartphone users who made standard voice calls were comfortable communicating over telephone and therefore would be receptive to receiving insulin titration information over telephone via the Nurse. The survey also reported that around 51% of the users downloaded more than five apps on their smartphones. Supported by the data in the survey, it was assumed that the users who downloaded more than five apps would be using the apps to carry out activities (other than to communicate) that involved inputting and outputting of information; it was further assumed that these people would also be receptive to using App to carry out insulin titration. We further assumed that the receptiveness to one intervention was independent of the receptiveness to the other intervention. Thus, in our microsimulation study the tendency for Rc A and Rc N were based on the probability of success in Bernoulli trials (receptiveness rates) 0.51 and 0.69, respectively. Furthermore, we assumed that subjects who were receptive to at least one of the two interventions were likely to be receptive to the combined intervention (App + Nurse), which is only given at stage 2 (see Fig. 2 ). The average probability of receptiveness to the combination, assuming they were initially not receptive to the initial intervention, was calculated to be 0.75 (for detailed calculation, refer to Model Development in Additional file 1 ).
Change in HbA1c conditional on being receptive to a received intervention
In the microsimulation, the change in HbA1c for each individual was drawn from a normal distribution corresponding to whether the subject was actually receptive or not (as assigned at baseline). As noted, receptiveness to an intervention, leading to appropriate changes in insulin dose, was assumed to be reflected in improvement in HbA1c over a 6-week period beyond random change. To generate plausible HbA1c change distributions, we stratified data from the actual trial subjects by 6-week change in HbA1c and calculated the means and standard deviations. The mean HbA1c reductions at weeks 6 and 12 were 0.92 (SD = 0.71) and 0.56 (SD = 0.77). To separate these values for receptive and non-receptive participants, we assumed an average receptiveness rate of 60% (e.g., by averaging the receptiveness rates to App and Nurse) in the trial subjects and fixed the mean for non-receptive participants to be zero (i.e., HbA1c reductions at week 6 and 12 for non-receptive participants are assumed to be 0 (SD = 0.71) and 0 (SD = 0.77)). Thus, the mean HbA1c reductions at weeks 6 and 12 for receptive participants are assumed to be 1.53 (SD = 0.71) and 0.94 (SD =0.77), respectively.
Calculation of Trial Costs
In the microsimulation, costs were accumulated for each synthetic subject based on their interventions experienced, including the time costs of providing the interventions, monitoring, and re-randomization when needed. The time and cost components (see Tables A1 and A2 of Additional file 1 ) were based on the actual expenditures incurred during the pilot study and expert inputs. Because costs for the trial are almost entirely personnel time, we did not include cost of the app itself. Cost was calculated in US dollars (USD) by multiplying personnel time by the exchange rate adjusted modal wage rate for Singapore, a country with a gross domestic product per capita comparable to the US, approximately USD 57,000. Based on these, the accumulated cost for each synthetic subject ranges from USD 312.55 to USD 382.00.
In our simulation study, we performed a base case analysis in which the two designs were compared with the key input parameters fixed as follows:
receptiveness rate to the Nurse ( P ( Rc N = 1)) = 69%,
receptiveness rate to the App ( P ( Rc A = 1)) = 51%,
receptiveness rate to the App + Nurse ( P ( Rc A + N = 1)) = 75%,
reduction threshold for response ( δ ) = 0.5%, and
trial size of patient cohort ( n ) = 100.
The Monte Carlo assessments were based on simulation size (B) = 10,000. For each design, the Monte Carlo mean HbA1c estimate ( \(\overset{\sim }{y}=\frac{\sum_{b=1}^B{\overline{y}}_b}{B}\Big)\) and the standard deviation \(\left({s}_{\overset{\sim }{y}}=\sqrt{\frac{\sum_{b=1}^B{\left({\overline{y}}_{b-}\overset{\sim }{y}\right)}^2\ }{\left(B-1\right)}}\right)\) were calculated where \({\overline{y}}_b\) is the overall and per AI mean HbA1c for the b th simulated trial. The probability of selecting the best AI as defined in SMART design over B simulations was calculated. In sensitivity analysis, we varied each parameter over a broad range to determine if there was any change in the sign for the difference in effectiveness or cost, large absolute changes on outcomes for both designs, or non-linear relationships between the parameters and outcomes.
In the base case scenario (Table 2 ), the difference of the overall mean expected HbA1c and per subject cost between the SMART and the RCT design is almost negligible (both HbA1c are 8.28%; per subject cost is USD 343.32 vs USD 343.22). As the sample size increases, the overall effectiveness and cost between SMART and RCT designs are essentially equivalent (Fig. 3 ). This is expected since both designs are unbiased for estimating the above metrics. Although the estimated mean HbA1c by AIs are similar in both designs, the standard deviations are noticeably lower in case of the SMART design. This is true across a wide range of sample sizes, approaching zero as trial size became very large (Fig. 4 ). Given the underlying data generation (see Additional file 1 ), the true optimal AI is AI2. The SMART design is able to outperform the RCT design by having higher probability of choosing AI2 given same sample size n = 100 (48.73% vs 43.38%). The efficiency of the SMART design becomes apparent as we aim to maximize the probability of choosing AI2 by varying the sample sizes (Fig. 5 ). In order to have approximately 70% probability of correctly choosing AI2, SMART requires n = 500 (70.66%), whereas RCT requires n = 1700 (70.72%).
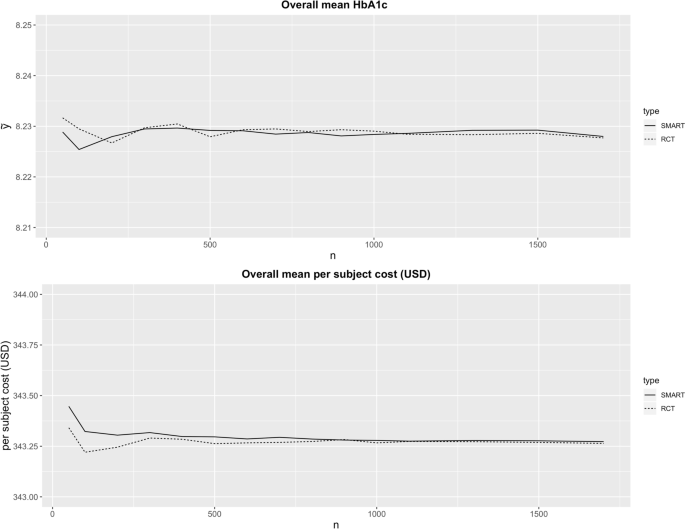
The Monte Carlo means of the final outcome HbA1c and per subject cost (USD) for different sample sizes
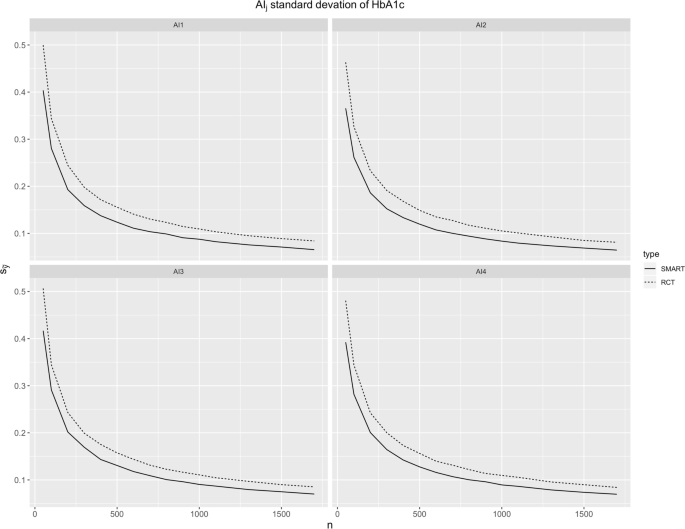
The standard deviations of the Monte Carlo means of the final outcome HbA1c by AIs for different sample sizes
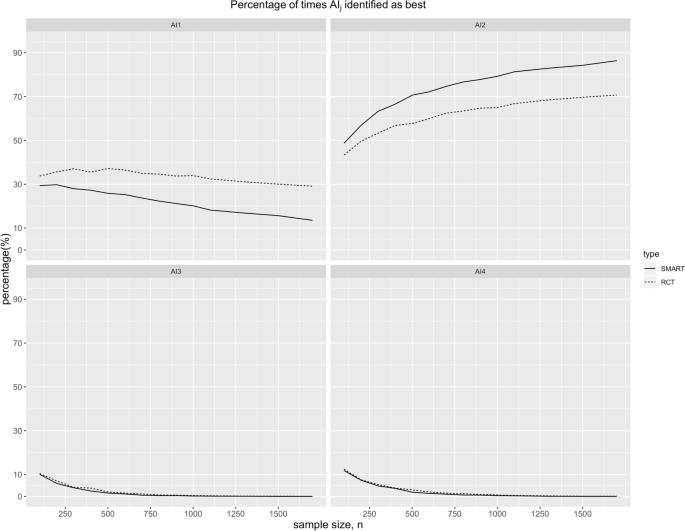
The number of times out of B = 10,000 simulations, the SMART and RCT trial identify the j-th AI (j = 1, … , 4) as the best AI across a wide range of sample sizes. According to the data generation model of the simulation study, AI2 is the truly optimal AI
Sensitivity analyses
For both trial designs the threshold value at which a subject was deemed to have been responsive at an intermediate point in the trial had an optimal value (i.e., the sensitivity curve had a U-shape) (Fig. 6 ). As the threshold moves away in either direction from the optimal value, the mean HbA1c for the trial subjects worsens. Under the optimal threshold, the SMART design becomes more efficient than the RCT design when the sample size is small, because the final overall mean HbA1c performs better (that is, it has a lower value). This change in threshold corresponds to a change in the relationship between the sensitivity and specificity of the interim evaluation of responsiveness. A more negative threshold results in lower sensitivity but greater specificity for responders while a higher threshold results in higher sensitivity but lower specificity.
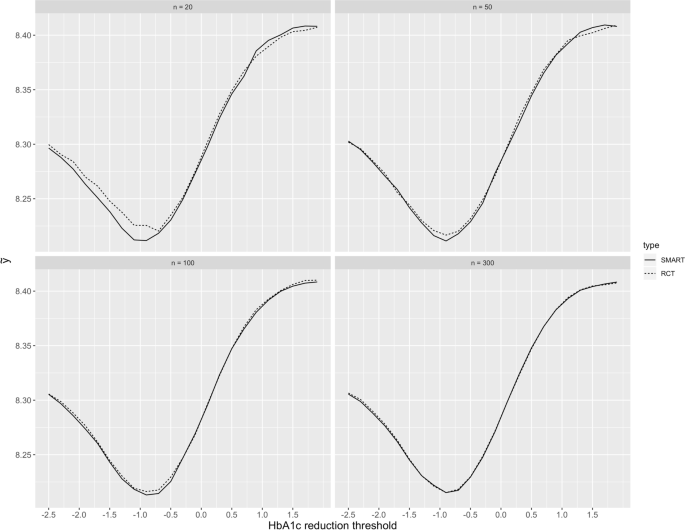
The overall mean expected HbA1c from 10,000 simulated SMART and RCT trials for sample size n = 20, 50, 100, 300 varied across a wide range of reduction threshold values
In this study, we examined the value of the SMART design relative to a comparable RCT design of two telemedicine interventions for insulin initiation: a largely self-contained smartphone app [ 20 ] and a nurse-based telephone consultation service. The designs were comparable in that both had the aim to evaluate the optimal sequencing of these two interventions, including the potential for combining interventions. We did this evaluation using microsimulation drawing on empirical data from a prior conventional trial. Simulation allowed us to perform sensitivity analysis of how diabetes control (as assessed by HbA1c) and trial costs were impacted by various aspects of trial design, including the operating characteristics of the intermediate measure used in the SMART and RCT designs to continue or switch treatment. It should be noted that the RCT design used as the comparator was unconventional, involving both multiple arms and treatment switching based on interim assessment of responsiveness to the initial treatment.
While both designs provide information on the optimal sequencing of therapies, we demonstrated some notable benefits of SMART compared to RCT. First, the SMART design from the perspective of trial population, had consistently smaller variance in the mean HbA1c per AI, which was especially evident at smaller sample sizes, at approximately equivalent cost. For the same sample size, the SMART design has higher probability of identifying the best AI.
Another advantage of SMART is that the design offers the potential to personalize treatment sequences by evaluating features predictive of responsiveness by treatment order. In our present simulation study, this feature of SMART was not examined as subjects were simulated as identical with regard to all features except for responsiveness to one intervention or the other. However, there is a sizable statistical literature that offers methodologies (e.g., Q-learning) for doing such personalization as secondary analysis of SMART data [ 5 , 27 ]. This aspect can be pursued in simulations as an important future work.
In sensitivity analysis, the observed benefits were robust. However, we did note that the value of both designs depended on the threshold value for defining response to treatment at the end of first stage. Average HbA1c control for trial subjects was optimal at an intermediate threshold value: too low and subjects who were unresponsive to their initial treatment were incorrectly maintained on an ineffective therapy; too high and subjects who were responsive to initial therapy would be incorrectly switched from an effective therapy. This suggests that the sensitivity and specificity of the threshold value can be important parameters to consider in SMART design and that the value of the design can be much diminished if the first stage evaluation does not have good operating characteristics.
Most clinical trials aim to conduct formal hypothesis tests in order to determine the superior interventions. However, in case of telemedicine, it may often be of more interest to find out if a cheaper or less burdensome intervention (e.g. App) is non-inferior to an established but more expensive intervention (e.g. Nurse). Such non-inferiority testing methodologies have been applied to conventional RCTs for many years [ 28 ]. Very recently, such non-inferiority testing methods [ 29 ] along with free web-based software [ 30 ], have also been developed in the SMART design context. Availability of such methodology and software tools brings SMARTs to an even playing field as RCTs, in terms of flexibility of hypothesis testing and data analysis. We have not considered non-inferiority testing in the current manuscript.
The primary goal of SMART is to learn – through within-patient adaptation of interventions over stages – an optimal strategy that can benefit future patients beyond the trial, not the trial participants per se . As such, it does not allow between-patient adaptation of interventions within the trial, because the randomization probabilities in a SMART are pre-specified. This fixed allocation scheme in a SMART design (as in conventional RCT) is motivated by the aim to maximize statistical power in order to maximize the scientific information gained from the trial. However, there are settings (e.g., implementation studies) where there is urgent need to translate emerging evidence from ongoing trials into practice, including the remainder of the trial participants, in order to maximize the benefit to the overall population of interest [ 20 ]. This need can be accommodated in both a SMART and an RCT through the machinery of response-adaptive allocation. Such an adaptive SMART or adaptive RCT design would allow modification of the randomization probabilities based on observed outcome data, favoring the treatment sequences that empirically look better (even though not statistically significant), at pre-set interim times during the trial [ 6 , 31 , 32 ]. For simplicity, we chose not to consider such a response-adaptive SMART or RCT in our current simulation study. However, we feel that such designs can potentially be even more attractive in the telemedicine context, optimizing welfare of trial participants while also finding optimal care strategies for future patients. We view more in-depth study of such designs in the telemedicine arena as an important future work.
In light of increasingly complex care management questions, new trial designs have been offered to improve the range of useful inferences that can be derived from clinical trials. SMART is one example that is particularly suited to evaluating the efficacy of different sequences of treatment options. To make better use of SMART, it is important to understand the advantages and disadvantages of SMART relative to a conventional design. This study illustrates the advantages of the SMART design over a comparable RCT for evaluating sequences of therapies. We note that the value of a SMART depends on the accuracy of the intermediate measure of responsiveness as well as the burden and cost of re-randomization.
Availability of data and materials
Relevant code for the simulated data and results is available at https://github.com/xiaoxi-yan/smart-rct-comparison .
Abbreviations
Sequential Multiple Assignment Randomized Trial
Randomized Controlled Trial
Adaptive Interventions
App, a smartphone application, Diabetes Pal
Nurse, a nurse-based telemedicine strategy
Lei H, et al. A "SMART" design for building individualized treatment sequences. Annu Rev Clin Psychol. 2012;8:21–48.
Article CAS Google Scholar
Murphy SA. An experimental design for the development of adaptive treatment strategies. Stat Med. 2005;24(10):1455–81.
2017 ACC/AHA/AAPA/ABC/ACPM/AGS/APhA/ASH/ASPC/NMA/PCNA. Guideline for the Prevention, Detection, Evaluation, and Management of High Blood Pressure in Adults: A Report of the American College of Cardiology/American Heart Association Task Force on Clinical Practice Guidelines. J Am Coll Cardiol. 2018;71(19):e127–248.
Article Google Scholar
Lavori PW, Dawson R. Adaptive Treatment Strategies in Chronic Disease. Annu Rev Med. 2008;59(1):443–53.
Chakraborty B, Moodie EEM. Statistical methods for dynamic treatment regimes: reinforcement learning, causal inference, and personalized medicine. New York: Springer; 2013.
Book Google Scholar
Thall PF, Sung H-G, Estey EH. Selecting Therapeutic Strategies Based on Efficacy and Death in Multicourse Clinical Trials. J Am Stat Assoc. 2002;97(457):29–39.
Kumar S, et al. Mobile health technology evaluation: the mHealth evidence workshop. Am J Prev Med. 2013;45(2):228–36.
Craig J, Patterson V. Introduction to the practice of telemedicine. J Telemed Telecare. 2005;11(1):3–9.
Sood S, et al. What is telemedicine? A collection of 104 peer-reviewed perspectives and theoretical underpinnings. Telemed J E Health. 2007;13(5):573–90.
Gallo G, et al. E-consensus on telemedicine in proctology: A RAND/UCLA-modified study. Surgery. 2021;170(2):405–11.
Huang EY, et al. Telemedicine and telementoring in the surgical specialties: a narrative review. Am J Surg. 2019;218(4):760–6.
Eadie L, Seifalian A, Davidson a. Telemedicine in surgery. J Br Surg. 2003;90(6):647–58.
Wootton R. Twenty years of telemedicine in chronic disease management–an evidence synthesis. J Telemed Telecare. 2012;18(4):211–20.
Molfenter T, et al. Trends in telemedicine use in addiction treatment. Addiction Sci Clin Pract. 2015;10(1):1–9.
Worster B, Swartz K. Telemedicine and palliative care: an increasing role in supportive oncology. Curr Oncol Rep. 2017;19(6):37.
Sirintrapun SJ, Lopez AM. Telemedicine in cancer care. Am Soc Clin Oncol Educ Book. 2018;38:540–5.
Contreras CM, et al. Telemedicine: patient-provider clinical engagement during the COVID-19 pandemic and beyond. J Gastrointest Surg. 2020;24(7):1692–7.
Calton B, Abedini N, Fratkin M. Telemedicine in the time of coronavirus. J Pain Symptom Manag. 2020;60(1):e12–4.
Suter P, Suter WN, Johnston D. Theory-based telehealth and patient empowerment. Popul Health Manag. 2011;14(2):87–92.
Bee YM, et al. A Smartphone Application to Deliver a Treat-to-Target Insulin Titration Algorithm in Insulin-Naive Patients With Type 2 Diabetes: A Pilot Randomized Controlled Trial. Diabetes Care. 2016;39(10):e174–6.
Core Team R. R: A language and environment for statistical computing. R Foundation for Statistical Computing. Vienna; 2020.
Khunti K, Davies MJ, Kalra S. Self-titration of insulin in the management of people with type 2 diabetes: a practical solution to improve management in primary care. Diabetes Obes Metab. 2013;15(8):690–700.
Nathan DM, et al. Medical management of hyperglycemia in type 2 diabetes: a consensus algorithm for the initiation and adjustment of therapy: a consensus statement of the American Diabetes Association and the European Association for the Study of Diabetes. Diabetes Care. 2009;32(1):193–203.
Nahum-Shani I, et al. Experimental design and primary data analysis methods for comparing adaptive interventions. Psychol Methods. 2012;17(4):457–77.
Robins JM, Hernan MA, Brumback B. Marginal structural models and causal inference in epidemiology: LWW; 2000.
Deloitte, Global Mobile Consumer Survey 2016: The UK Cut. 20, Deloitte: London, UK.
Kosorok, M. and E. Moodie, Adaptive Treatment Strategies in Practice. Adaptive Treatment Strategies in Practice.
D'Agostino RB Sr, Massaro JM, Sullivan LM. Non-inferiority trials: design concepts and issues - the encounters of academic consultants in statistics. Stat Med. 2003;22(2):169–86.
Ghosh P, et al. Noninferiority and equivalence tests in sequential, multiple assignment, randomized trials (SMARTs). Psychol Methods. 2020;25(2):182.
Ghosh, P. How_to_use_the_Shiny_App.md. 2019; Available from: https://osf.io/mqpze/ .
Google Scholar
Thall PF, Wathen JK. Covariate-adjusted adaptive randomization in a sarcoma trial with multi-stage treatments. Stat Med. 2005;24(13):1947–64.
Cheung YK, Chakraborty B, Davidson KW. Sequential multiple assignment randomized trial (SMART) with adaptive randomization for quality improvement in depression treatment program. Biometrics. 2015;71(2):450–9.
Download references
Acknowledgements
We will like to acknowledge the support for this project from the Ministry of Education, Singapore grant MOE2015-T2-2-056.
The production of this manuscript was funded by Duke-NUS Medical School.
Author information
Authors and affiliations.
Centre for Quantitative Medicine. Duke-NUS Medical School, 8 College Road, Singapore, 169857, Singapore
Xiaoxi Yan & Bibhas Chakraborty
Health Services and Systems Research Department, Duke-NUS Medical School, 8 College Road, Singapore, 169857, Singapore
David B. Matchar, Nirmali Sivapragasam, John P. Ansah & Aastha Goel
Department of Medicine, Duke University Medical Center, Durham, North Carolina, USA
David B. Matchar
Department of Statistics and Applied Probability, Faculty of Science, National University of Singapore, Singapore, 117546, Singapore
Bibhas Chakraborty
Department of Biostatistics and Bioinformatics, Duke University, Durham, North Carolina, USA
You can also search for this author in PubMed Google Scholar
Contributions
X.Y., D.B.M., N.S., J.P.A., and B.C. were major contributors in writing the manuscript. X.Y., D.B.M., N.S., J.P.A. and B.C. contributed to the concept, design and validation of the simulation. X.Y. produced the code and all the results in the manuscript. A.G. and X.Y. performed the analysis on the pilot study to determine the fixed values used in the data generation model. N.S. provided overall assistance in the project. All authors read and approved the final manuscript.
Corresponding author
Correspondence to Xiaoxi Yan .
Ethics declarations
Ethics approval and consent to participate.
Not applicable.
Consent for publication
Competing interests.
The authors declare that they have no competing interests.
Additional information
Publisher’s note.
Springer Nature remains neutral with regard to jurisdictional claims in published maps and institutional affiliations.
Supplementary Information
Additional file 1..
Details of data generation model and simulation algorithm.
Rights and permissions
Open Access This article is licensed under a Creative Commons Attribution 4.0 International License, which permits use, sharing, adaptation, distribution and reproduction in any medium or format, as long as you give appropriate credit to the original author(s) and the source, provide a link to the Creative Commons licence, and indicate if changes were made. The images or other third party material in this article are included in the article's Creative Commons licence, unless indicated otherwise in a credit line to the material. If material is not included in the article's Creative Commons licence and your intended use is not permitted by statutory regulation or exceeds the permitted use, you will need to obtain permission directly from the copyright holder. To view a copy of this licence, visit http://creativecommons.org/licenses/by/4.0/ . The Creative Commons Public Domain Dedication waiver ( http://creativecommons.org/publicdomain/zero/1.0/ ) applies to the data made available in this article, unless otherwise stated in a credit line to the data.
Reprints and permissions
About this article
Cite this article.
Yan, X., Matchar, D.B., Sivapragasam, N. et al. Sequential Multiple Assignment Randomized Trial (SMART) to identify optimal sequences of telemedicine interventions for improving initiation of insulin therapy: A simulation study. BMC Med Res Methodol 21 , 200 (2021). https://doi.org/10.1186/s12874-021-01395-7
Download citation
Received : 03 May 2021
Accepted : 08 September 2021
Published : 30 September 2021
DOI : https://doi.org/10.1186/s12874-021-01395-7
Share this article
Anyone you share the following link with will be able to read this content:
Sorry, a shareable link is not currently available for this article.
Provided by the Springer Nature SharedIt content-sharing initiative
- Sequential treatment designs
- telemedicine
- optimal adaptive interventions
BMC Medical Research Methodology
ISSN: 1471-2288
- General enquiries: [email protected]
- Remote Access
- Save figures into PowerPoint
- Download tables as PDFs

Sequential, Multiple Assignment, Randomized Trial Designs
Kelley M. Kidwell; Daniel Almirall
- Download Chapter PDF
Disclaimer: These citations have been automatically generated based on the information we have and it may not be 100% accurate. Please consult the latest official manual style if you have any questions regarding the format accuracy.
Download citation file:
- Search Book
Jump to a Section
Introduction, what is a smart design.
- WHY IS A SMART DESIGN USED?
- LIMITATIONS OF SMART DESIGNS
- HOW DID THIS STUDY USE A SMART DESIGN?
- HOW SHOULD RESULTS OF A SMART DESIGN BE INTERPRETED?
- ACKNOWLEDGMENT
- Full Chapter
- Related Content
This JAMA Guide to Statistics and Methods explains sequential, multiple assignment, randomized trial (SMART) study designs, in which some or all participants are randomized at 2 or more decision points depending on the participant’s response to prior treatment.
An adaptive intervention is a set of diagnostic, preventive, therapeutic, or engagement strategies that are used in stages, and the selection of the intervention at each stage is based on defined decision rules. At the beginning of each stage in care, treatment may be changed by the clinician to suit the needs of the patient. Typical adaptations include intensifying an ongoing treatment or adding or switching to another treatment. These decisions are made in response to changes in the patient’s status, such as a patient’s early response to, or engagement with, a prior treatment. The patient experiences an adaptive intervention as a sequence of personalized treatments.
Adaptive interventions are necessary because, for many disorders, the optimal sequence of interventions differs among patients. Not all patients respond the same way or have the same adverse event profile; not all patients engage with treatment in the same way; many disorders have a waxing and waning course; and comorbidities arise or become more salient during the course of care. The trial by Fortney et al 1 constructed a 2-stage, adaptive telecare intervention to treat complex psychiatric disorders in underserved, rural, primary care settings. The investigators used a sequential, multiple assignment, randomized trial (SMART) 2 design to answer questions concerning the most effective mode of intervention delivery at 2 critical decision points in the adaptive telecare intervention.
A SMART is a type of multistage, factorial randomized trial, in which some or all participants are randomized at 2 or more decision points. Whether a patient is randomized at the second or a later decision point, and the available treatment options, may depend on the patient's response to prior treatment.
Sign in or create a free Access profile below to access even more exclusive content.
With an Access profile, you can save and manage favorites from your personal dashboard, complete case quizzes, review Q&A, and take these feature on the go with our Access app.
Pop-up div Successfully Displayed
This div only appears when the trigger link is hovered over. Otherwise it is hidden from view.
Please Wait
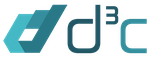
- Advancing the science of adaptive interventions
- Advancing mobile health interventions
- Integrating human-delivered and digital interventions
- Solving implementation challenges
- Intervention Designs
- Trial Designs
- Software Tools
- Training Programs
- Online Courses
- Publications
- CATIE Training for Education Researchers
- Pilot Grant Program
- Postdoctoral Fellowships
Data Analysis for Hybrid Experimental Designs
Home / Code Library
About this code
On this page, we provide example datasets, analysis code in SAS and R, and outputs, for the three kinds of hybrid experimental designs considered in “ Design of Experiments with Sequential Randomizations at Multiple Time Scales: The Hybrid Experimental Design .”
The specific hybrids considered combine: a classic factorial experiment with a sequential multiple assignment randomized trial (SMART), a classic factorial experiment with a micro-randomized trial (MRT), a SMART with an MRT.
How can a behavioral scientist use this code?
A behavioral scientist can use this code to learn how to analyze data for three different types of Hybrid Experimental Designs (HED). They may then repurpose the code to analyze data from their own hybrid design.
Related References
Nahum-Shani, I., Dziak, J. J., Venera, H., Spring, B., & Dempsey W. (2023). Design of Experiments with Sequential Randomizations at Multiple Time Scales: The Hybrid Experimental Design. Behavior Research Methods, doi:10.3758/s13428-023-02119-z.
April 12, 2024, 3 p.m.
Jillian C. Strayhorn
d3center Seminar Series
April 17, 2024, 1 p.m. EDT
Hybrid experimental designs for optimizing digital interventions that adapt on multiple timescales
cadio Webinar Series
April 18-19, 2024
P50 Annual Meetings
April 19, 2024, 3 p.m.
April 30, 2024
Hybrid Experimental Designs
Center for Healthy Minds, UW-Madison
May 3rd, 2024, 3 p.m.
Timothy Lycurgus
May 6, 2024, 11 a.m. EDT
Experimental Designs for Adapting Digital Interventions
mHealth Training Institute 2024
June 3-5, 2024, Day & Time TBA
Missing Data in Micro-Randomized Trials: Challenges and Opportunities
Society for Ambulatory Assessment 2024
June 15, 2024
Innovations in Digital Interventions for Substance Use
College on Problems of Drug Dependence Conference
Join the d3center Mailing List
Keep up to date with the latest news, events, software releases, learning modules, and resources from the d3center.
© 2024 • d3center • Institute for Social Research • University of Michigan

An official website of the United States government
The .gov means it’s official. Federal government websites often end in .gov or .mil. Before sharing sensitive information, make sure you’re on a federal government site.
The site is secure. The https:// ensures that you are connecting to the official website and that any information you provide is encrypted and transmitted securely.
- Publications
- Account settings
Preview improvements coming to the PMC website in October 2024. Learn More or Try it out now .
- Advanced Search
- Journal List
- HHS Author Manuscripts

The Multiphase Optimization Strategy (MOST) and the Sequential Multiple Assignment Randomized Trial (SMART): New Methods for More Potent eHealth Interventions
Linda m. collins.
1 The Methodology Center and Department of Human Development and Family Studies, The Pennsylvania State University, University Park, PA
Susan A. Murphy
2 Institute for Social Research and Department of Statistics, University of Michigan, Ann Arbor, MI
Victor Strecher
3 Department of Health Behavior and Health Education, University of Michigan, Ann Arbor, MI
In this article two new methods for building and evaluating e-health interventions are described. The first is the Multiphase Optimization Strategy (MOST). MOST consists of a screening phase, in which intervention components are efficiently identified for selection for inclusion in an intervention or rejection, based on their performance; a refining phase, in which the selected components are fine-tuned, and questions such as optimal component dosage are investigated; and a confirming phase, in which the optimized intervention, consisting of optimal doses of the selected components, is evaluated in a standard randomized confirmatory trial. The second is the Sequential Multiple Assignment Randomized Trial (SMART) which is an innovative research design especially suited for building time-varying adaptive interventions. A SMART trial can be used to identify the best tailoring variables and decision rules for an adaptive intervention empirically. Both the MOST and SMART approaches use randomized experimentation to enable valid inferences. When properly implemented, these approaches will lead to the development of more potent e-health interventions.
There are good reasons to believe that interventions based on e-health principles have the potential for considerable public health impact. Perhaps the most obvious reason is the reach of these interventions. Once an electronic intervention has been designed and programmed, delivery occurs via methods such as the Internet or by mailing a CD, and therefore is extremely convenient. Moreover, the incremental cost of delivering an intervention to additional people is usually negligible, certainly in comparison to traditional interventions where in order to reach more recipients it becomes necessary to add additional physicians, therapists, health educators, peer counselors, and so on to deliver the program. The limiting factor for reach of an e-intervention is less likely to be a shortage of resources for delivering the program electronically than access to computers on the part of potential recipients. However, access to computers continues to increase in all strata of American society, suggesting that e-health interventions hold growing promise. 1
The broad reach of e-health interventions is particularly exciting in the light of some new methods for building and optimizing behavioral interventions. The purpose of this article is to introduce two of these new methods to e-health scientists. One is the Multiphase Optimization Strategy (MOST) 2 for building and evaluating interventions in such a way that they are made out of active program components delivered at optimal doses. The other is the Sequential Multiple Assignment Randomized Trial (SMART) 3 for building adaptive interventions. We propose that these new methods, although relatively untried at this writing, are eminently practical and hold much potential for e-health research. By using these methods, it is possible to produce more potent interventions which, when coupled with the reach afforded by e-health approaches, will promise considerable overall public health impact.
THE MULTIPHASE OPTIMIZATION STRATEGY (MOST)
The traditional approach to intervention development has involved constructing an intervention a priori and then evaluating it in a standard randomized confirmatory trial (RCT). After the confirmatory trial, post-hoc analyses are done to help explain how the intervention worked, or why it did not work. The results of these analyses may be used to refine the intervention program and construct a second generation version of the program, which is then evaluated in a new RCT.
Collins, Murphy, Nair, and Strecher 2 reviewed shortcomings of this approach. While acknowledging that RCTs are the undisputed gold standard for assessing the effect of an intervention as a package once it has been developed, they pointed out that the post hoc analyses that typically follow a RCT in order to inform further intervention design and evaluation are subject to bias because they are not based on random assignment. As a result the cycle of intervention – RCT – post hoc analyses – revision of intervention – RCT is likely to lead very slowly, if at all, to an optimized intervention.
Collins et al. also pointed out that most behavioral interventions can be considered an aggregation of a set of components. Some intervention components are a part of the program itself (e.g. program content). Others may be more concerned with the delivery of the program (e.g., whether a message is delivered by a lay person or by a physician). Some components may be having the intended effect; others may be having no effect at all; and others may even be reducing the overall potency of the intervention. Because the traditional RCT evaluates the intervention only as a whole, using the RCT alone does not enable isolation of the effects of individual program or delivery components. A different experimental approach is necessary to accomplish this.
We suggest MOST as an alternative way of building, optimizing and evaluating e-health interventions. MOST incorporates the standard RCT, but before the RCT is undertaken also includes a principled method for identifying which components are active in an intervention, and which doses of each component lead to the best outcomes. The principles underlying MOST are drawn from engineering, and emphasize efficiency. MOST consists of three phases, each of which addresses a different set of questions about the intervention by means of randomized experimentation .
Figure 1 offers an outline of the three phases of MOST. The first phase is screening . The starting point for the screening phase is a previously identified finite set of intervention components, made up of program components and/or delivery components. It is assumed that there is some theoretical basis for the choice of these components. It is also assumed that any initial pilot testing necessary to assess feasibility and finalize the details of implementation has been completed prior to the start of the screening phase.

ANOVA, analysis of variance; SMART, sequential multiple assignment randomized trial
The objective of the screening phase is to address questions like the following: Which of the set of program components are active and contributing to positive outcomes, and should be included in the intervention? Which program components are inactive or counterproductive, and should be discarded? Which of the set of delivery components are active and make a difference in the intervention outcome, and thus play a role in maintaining intervention fidelity? Decisions about which program and delivery components are active and should be retained and which are inactive and should be discarded are made based on the results of a randomized experiment. (Experimental design alternatives are discussed below.) The decision may be made on the basis of statistical significance at any alpha level deemed appropriate, or on the basis of estimated effect size. In addition, cost in relation to incremental contribution to the desired outcome may be a consideration. At the conclusion of the screening phase, a set of program and delivery components that are to be retained for further examination has been identified. This set of components constitutes a “first draft” intervention.
This “first draft” intervention is the starting point for the next phase of MOST, the refining phase. In this phase the “first draft” intervention is examined further, with the objective of fine-tuning the intervention and arriving at a “final draft.” The specific activities of the refining phase depend on the intervention being considered, but in general focus on questions such as: Given the components identified in the screening phase, what are the optimal doses? Does the optimal dose vary depending on individual or group characteristics? As in the screening phase, in the refining phase decisions are based on randomized experimentation, and cost may be a consideration. At the conclusion of the refining phase, the investigator has identified an optimized “final draft” intervention consisting of a set of active program and delivery components at the best doses.
The “final draft” intervention provides the starting point for the third phase of MOST, confirming . In the confirming phase this optimized intervention is evaluated in a standard RCT. The confirming phase addresses questions such as: Is this intervention, as a package, efficacious? Is the intervention effect large enough to justify investment in community implementation?
A brief illustrative example
Because MOST is an approach or a perspective rather than an off-the-shelf procedure, exact details about its implementation depend on the application. In order to illustrate MOST, we offer a brief hypothetical example similar to the one in Collins et al. 2 . The example is based on (but not identical to or an account of) the work of one of the authors of the current article (VS).
Suppose the objective is to use MOST to build, optimize and evaluate an e-intervention for smoking cessation, and that six components have been identified for study, four of which program components and two of which are delivery components. The program components are outcome expectation messages (messages addressing an individual’s expectations about what will happen if he or she quits smoking) which may be either present or absent in the intervention; efficacy expectation messages (these address barriers to perceived self-efficacy) which may be present or absent; message framing (this concerns how the persuasive messages about quitting smoking are to be framed), which may be positive or negative; and testimonials (from former smokers), which may be present or absent. The delivery components are exposure schedule, which may be one long message or four smaller ones; and source of message, which may be a primary care physician or the individual’s health maintenance organization (HMO).
In the screening phase randomized experimentation is conducted to isolate the effects of each of the six components. Suppose the experimental results indicate that the active program components are outcome expectation messages, efficacy expectation messages, and testimonials, and that there is one active delivery component, exposure schedule. Once this “first draft” intervention has been identified, the screening phase is concluded. The intervention scientist now proceeds to the refining phase in order to fine-tune the “first draft” and arrive at an optimized intervention. An example of this fine-tuning might be experimentation to pinpoint the best dose of exposure schedule, in other words, the optimal number of messages. The “final draft” intervention would then consist of outcome expectation messages, efficacy expectation messages, and testimonials, with the intervention delivered using the optimal number of messages identified in the refining phase. In the confirming phase, this “final draft” smoking cessation intervention is evaluated in a standard RCT.
Design for the screening and refining phases
The research design to be used in the confirming phase (i.e., the RCT) is straightforward and familiar to most intervention scientists. Usually a simple two-group design consisting of random assignment to either a program condition or a suitable comparison condition would be used. It may be less evident what design would be used in the screening and refining phases. One family of designs that lends itself well to the screening and refining phases is the factorial analysis of variance (ANOVA). In an ANOVA design several independent variables, or factors, are investigated at once. A properly chosen and implemented ANOVA design permits the effects of individual independent variables to be isolated. In the behavioral sciences the factors are usually “fully crossed” which means that each level of a variable is combined with each level of the other variables.
For example, suppose there are just two program components under consideration: outcome expectation messages and efficacy expectation messages. To examine these in the screening phase using a fully crossed factorial ANOVA, subjects would be randomly assigned to one of four experimental conditions: both messages present; outcome expectation messages only; efficacy expectation messages only; and both messages absent (perhaps an information-only control). At the end of the screening phase, after the experiment was completed, the decision about which components to select for further consideration would be based on the main effect and interaction estimates obtained from the ANOVA. The decision may be made by selecting statistically significant effects; it may be made by choosing components associated with an estimated effect size over some threshold level; or it may use the results of the ANOVA in some other way.
Although factorial designs are the most efficient way to assess the effect of several independent variables simultaneously, they have for the most part been eschewed by intervention scientists because of the perception that they are impractical due to the number of conditions that must be implemented. For example, a fully crossed ANOVA design to investigate the six components in our example would involve 64 treatment conditions. This may in fact be too many conditions to manage for interventions delivered by teachers and practitioners in settings like schools and hospitals, but it does not necessarily follow that the field of e-health should be similarly discouraged about factorial ANOVA designs. Because e-health interventions are delivered electronically, the primary cost often will be the computer programming required to construct each of the conditions. Once this task is done, it may be relatively straightforward to assign individuals randomly to experimental conditions and then deliver the corresponding version of the intervention. Thus, factorial ANOVA may be more feasible in e-health than it is in other more traditional areas of intervention science.
However, when there are many factors, the construction of each condition in a fully crossed ANOVA design may be too much for an e-intervention study. In this case, fractional factorial ANOVA designs can be an attractive alternative. When using fractional factorial ANOVA designs, it is not necessary to include every possible experimental condition in the design. Instead, based on working assumptions made by the investigator, a subset of conditions is chosen strategically in order to estimate effects of primary interest. Fractional factorial designs are not new; they go back to Fisher 4 and Box, Hunter, and Hunter, 5 and have been used routinely in engineering and agriculture for many years. Fagerlin et al. 6 recently employed a fractional factorial approach in medical decision making research. Intervention science also can and should benefit from the efficiency and economy these designs provide.
Collins et al. 2 illustrated how a six-factor fully crossed ANOVA design with 64 conditions can be reduced to a fractional factorial ANOVA design with 16 conditions. The reduced design retains the capability to provide main effects estimates for each of the six independent variables, and also the capability to provide estimates for selected interactions. The power associated with the test of each main effect is the same as that for any simple two-group comparison. In the refining phase, variants on fractional factorial designs, such as response surfaces, may be useful for questions involving identification of optimal doses.
As mentioned above, in some situations it may not be necessary or desirable to base decisions strictly on hypothesis tests. 2 If hypothesis testing is to be used, it may be necessary to control the experiment-wise error rate. As a simple expedient, we suggest identifying a priori a limited set of effects predicted to be sizeable, testing those at the desired alpha level without regard to the experiment-wise error rate, and then using a Bonferroni or similar adjustment for the remaining effects. (For more about the experiment-wise error rate see Wu and Hamada. 7 ) Note that in general interaction effect sizes tend to be small, making it important to power a study accordingly if interactions are of particular interest.
Although we propose that MOST is useful in a wide variety of intervention development settings, there are some situations in which investigators may wish to consider a different approach. When applied to the building of new interventions, MOST is based on the idea that it is feasible to identify individual program components that can stand alone, at least enough to assess their individual effects. It may not be sensible to parse an extremely tightly integrated program into separate parts. Even when meaningful individual program components can be identified, it may be expected that each component has a very small, difficult to detect effect that nevertheless contributes to a larger, more readily detectable cumulative effect of the entire package. If in addition it can safely be assumed that none of the components has a deleterious effect or reduces the efficacy of other components, then the effects of individual components may not be of much interest. However, MOST may still be helpful in examining delivery components associated with these interventions.
Even when an intervention can be meaningfully decomposed, it is possible that the list of components cannot be combined at will, in other words, not every combination of program components is sensible to implement. In some cases a fractional factorial design may be chosen that includes only sensible combinations of components. If there is a component that is expected not to operate properly in the absence of another component, it may be more fruitful to consider the two components as one for the purpose of building the intervention.
In the following section, the SMART trial, another type of design that can be used as a stand-alone method or may be useful in the refining phase of MOST, is described. Adaptive interventions and the Sequential Multiple Assignment Randomized Trial (SMART)
In adaptive interventions, 8 which are also called by other names such as stepped care strategies, 9 , 10 treatment algorithms, 11 and expert systems, 12 the dose of intervention components may be varied in response to characteristics of the individual or environment. These characteristics are called tailoring variables. The tailoring variable can be something stable like gender or ethnicity, or something that varies over time, such as stage in the Transtheoretical Model, 12 attitude or even progress toward a treatment goal. When the tailoring variable changes over time and there are repeated opportunities to adapt the intervention, this is called a time-varying adaptive intervention. In adaptive interventions, dosage is assigned to individuals based on a priori decision rules that link values on the tailoring variables to specific intervention dosages. See Collins, Murphy, and Bierman 8 for a discussion of advantages of adaptive interventions as compared to fixed interventions.
For example, suppose a smoking cessation program includes both positively-framed messages (e.g. “Quitting smoking will help you feel healthier”) and negatively-framed messages (e.g. “Continuing to smoke will increase your risk of serious health problems”). Further suppose that it is expected that those in the precontemplation stage of the Transtheoretical Model are more likely to initiate a quit attempt if presented with a negatively framed message, whereas those in the contemplation stage are more likely to try to quit smoking if presented with a positively framed message. In this example, an individual’s stage in the Transtheoretical Model is the tailoring variable. An adaptive intervention would measure the tailoring variable, i.e. assess whether a smoker is a precontemplator or a contemplator, and deliver a negatively or positively framed message accordingly. A time-varying adaptive intervention would assess this at several different occasions, and once the individual moved from precontemplator to contemplator, would switch to delivering positively framed messages. The strategy “If precontemplator, use negative message framing; if contemplator, use positive message framing” is a decision rule.
The e-health approach lends itself naturally to adaptive interventions. One potential difficulty associated with adaptive interventions is that if decision rules are complex, delivery can be more logistically challenging than that of a comparable fixed intervention. However, a great advantage of e-health is that it can make delivery of even complex time-varying adaptive interventions relatively straightforward. When an e-health approach is used, assessments of tailoring variables can be done electronically, for example, by means of on-line questionnaires and immediate scoring algorithms, and programming algorithms can be used to automate variation of intervention program content or aspects of intervention delivery in response to the tailoring variables. The procedure can be repeated periodically, or as often as each time the individual has contact with the computer program.
BUILDING TIME-VARYING ADAPTIVE INTERVENTIONS USING SMART
SMART is a randomized experimental design that has been developed especially for building time-varying adaptive interventions. Developing an adaptive intervention strategy requires addressing questions such as: What is the best sequencing of intervention components? Which tailoring variables should be used? How frequently, and at what times, should tailoring variables be reassessed and an opportunity for changing dosage presented? Is it better to assign treatments to individuals, or to allow them to choose from a menu of treatment options?
The SMART approach enables the intervention scientist to address questions like these in a holistic yet rigorous manner, taking into account the order in which components are presented rather than considering each component in isolation. A SMART trial provides an empirical basis for selecting appropriate decision rules and tailoring variables. The end goal of the SMART approach is the development of evidence-based adaptive intervention strategies, which are then evaluated in a subsequent RCT.
In a SMART trial, each individual may be randomly assigned to conditions several times. For example, suppose the objective of an intervention is to help people who intend to quit smoking to quit successfully. One question might be, is it better to use positively framed messages or negatively framed messages? Another might be, for individuals who return to smoking, what kind of encouragement to continue to try to refrain from smoking is best, daily email messages or daily email messages augmented with weekly phone calls? For those who are successful at not smoking, is it better to encourage them with daily email messages, or to leave them alone? And, does the best adaptive intervention strategy vary depending on whether the individual was originally presented with negatively or positively framed messages? A hypothetical SMART trial addressing these questions is outlined in Table 1 . At the beginning of the trial, individuals are randomly assigned to receive a DVD with quitting strategies framed positively or framed negatively. At the end of the first month, quitting success is assessed. Those who have not been smoking are randomly assigned to receive either daily email encouragement or no encouragement. Those who have been smoking are given daily email encouragement, and are randomly assigned to receive or not to receive a weekly phone call in addition.
Timeline of example SMART trial
Embedded in this SMART trial are eight different adaptive intervention strategies. These are listed in Table 2 . For example, Adaptive Intervention Strategy 3 is, “Begin with a DVD containing smoking cessation strategies framed positively. If at the end of one month the individual is not smoking, no further action is taken. If at the end of one month the individual is smoking, begin sending daily encouraging email messages.” Note that individuals are randomly assigned to adaptive interventions in the SMART approach, but none of the intervention strategies would involve randomization when implemented outside of an experimental setting. In other words, the purpose of the random assignment is to address scientific questions, not to serve as a part of the adaptive intervention.
Adaptive intervention strategies embedded in SMART trial example in Table 1
SMART, sequential multiple assignment randomized trial
Suppose the outcome variable in this example is the number of cigarettes smoked during the last week of the study. To address the question “Is it better to use positively or negatively framed messages?” a statistical analysis can be done comparing the mean across adaptive interventions 1+2+3+4 with the mean across adaptive interventions 5+6+7+8. To address the question “For individuals who return to smoking, what kind of encouragement to continue to try to refrain from smoking is best?” the statistical analysis would involve selecting those who respond that they are smoking, and comparing the mean of 1+3+5+7 with the mean of 2+4+6+8. A comparable analysis can be done for those who do not return to smoking. Note that within the SMART trial all of these questions are addressed by means of randomized experiments. Statistical power for each of these analyses is that of a simple two-group comparison. Other scientific questions can be addressed as well. For more about the statistical analysis of SMART trials, see Murphy. 13 Integration of SMART and MOST
Investigators interested in building a time-varying adaptive intervention may find it advantageous to integrate a SMART trial into the MOST procedure. The screening phase of MOST can be used to identify active program components that will be incorporated into the adaptive intervention. The refining phase can be used initially to provide leads for tailoring variables. This can be done by exploring possible interactions between program components and individual and group characteristics. The active program components identified in the screening phase and any tailoring variables identified in the refining phase up to this point can then be used as the basis for a set of time-varying adaptive intervention strategies. The refining phase can continue with a SMART trial to identify the best of these strategies. The confirming phase will then proceed as usual, with a RCT comparing the best adaptive intervention strategy against a suitable comparison group.
Because of their reach, e-health interventions promise considerable public health impact. It makes sense to maximize this public health impact by developing the most effective interventions we can. This article has described two related methods for building and evaluating e-health interventions. MOST is an approach for systematically and efficiently optimizing behavioral interventions. The SMART trial is an approach for identifying the best time-varying adaptive intervention strategy. Both approaches are based on randomized experimentation, which means that a high degree of confidence can be placed in the results. Used individually or together, these methods enable scientists to increase the potency of behavioral interventions.
ACKNOWLEDGEMENTS
This work has been supported by National Institute on Drug Abuse grants P50 DA10075 (Dr. Collins and Dr. Murphy), K05 DA018206 (Dr. Collins), K02 DA15674 (Dr. Murphy), and National Cancer Institute grant P50 CA101451 (Dr. Strecher and Dr. Murphy).
Publisher's Disclaimer: This is a PDF file of an unedited manuscript that has been accepted for publication. As a service to our customers we are providing this early version of the manuscript. The manuscript will undergo copyediting, typesetting, and review of the resulting proof before it is published in its final citable form. Please note that during the production process errors may be discovered which could affect the content, and all legal disclaimers that apply to the journal pertain.
No financial conflict of interest was reported by the authors of this paper.
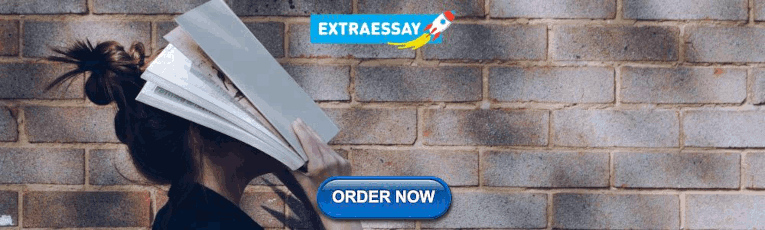
IMAGES
VIDEO
COMMENTS
This JAMA Guide to Statistics and Methods explains sequential, multiple assignment, randomized trial (SMART) study designs, in which some or all participants are randomized at 2 or more decision points depending on the participant's response to prior treatment.
Sequential multiple assignments randomized trials (SMARTs) are a type of experimental design where patients may be randomised multiple times according to pre-specified decision rules. The present ...
A sequential, multiple assignment, randomized trial (SMART) is a type of multistage randomized trial design that aims to answer critical questions in the development of DTRs, such as those described above. In a SMART, all participants move through multiple stages of treatment.
The sequential multiple assignment randomized trial (SMART) is an experimental design consisting of multiple randomization stages. 2 This type of design serves as a promising tool to address scientific questions about constructing effective adaptive interventions for controlling infectious diseases.
In a sequential multiple assignment randomised trial (SMART) design, some or all patients can be randomised more than once, especially nonresponders to the first stage treatment option. One can answer more research questions using a SMART design compared to a classical RCT design.
One type of clinical trial design that is useful for answering such questions is the sequential, multiple assignment, randomized trial, or SMART (Lavori and Dawson 2004; Murphy 2005; Collins et al. 2014). Relative to standard multiarm randomized trials, the SMART is unique in that it involves multiple stages of randomization:
Sequential multiple assignment randomization trial (SMART) is a powerful design to study Dynamic Treatment Regimes (DTRs) and allows causal comparisons of DTRs. To handle practical challenges of SMART, we propose a SMART with Enrichment (SMARTer) design, which performs stage-wise enrichment for SMART. SMARTer can improve design efficiency ...
The types of study commonly used for testing and comparing DTRs include observational studies, one-time randomised trials that randomise patients only once to the whole DTR, and sequential multiple assignment randomized trials (SMARTs) . SMART designs randomise patients at each decision point considering information collected on the patient so far.
A sequential multiple assignment randomized trial (SMART) is an experimental design that scientists can use to develop high-quality dynamic treatment regimens (DTRs), a prespecified treatment plan in which the type(s) or dosage/intensity of an intervention, the delivery of the intervention, or the monitoring schedule is repeatedly adjusted in response to new information collected about the ...
A Sequential Multiple Assignment Randomized Trial (SMART) is a design that involves multiple stages of randomization. Patient's characteristics and treatment history are used to re-randomize them. Many clinically important questions can be answered using SMART designs. •
The purpose of this systematic review is to describe the state of the art of sequential multiple-assignment randomized trials in education research. An iterative, systematic search strategy yielded thirteen reports for synthesis. We coded eligible reports for study characteristics, population, intervention, outcomes, SMART design components, overall findings, and study quality. Of the thirteen ...
The sequential, multiple assignment, randomized trial (SMART; refs. 16, 17 ) is a multistage trial that is designed to develop and investigate treatment pathways. SMART designs can investigate delayed effects as well as treatment synergies and antagonisms, and provide robust evidence about the timing, sequences, and combinations of immunotherapies.
To examine the value of a Sequential Multiple Assignment Randomized Trial (SMART) design compared to a conventional randomized control trial (RCT) for telemedicine strategies to support titration of insulin therapy for Type 2 Diabetes Mellitus (T2DM) patients new to insulin. Microsimulation models were created in R using a synthetic sample based on primary data from 63 subjects enrolled in a ...
The investigators used a sequential, multiple assignment, randomized trial (SMART) 2 design to answer questions concerning the most effective mode of intervention delivery at 2 critical decision points in the adaptive telecare intervention.
Description of a SMART Design. A SMART is a type of multi-stage, factorial, randomized trial, in which some or all participants are randomized at two or more decision points. Whether a patient is randomized at the second or a later decision point, and the available treatment options, may depend on the patient's response to prior treatment.
Primary Aim 1 Examples. Compare initial intervention options. Compare second-stage options among slow-responders. Compare embedded adaptive interventions. First, let's define what we mean by "embedded. Start with Then, at. If response status = responder Then, stage 2 intervention= {add Else if response Then, stage.
To develop adaptive interventions that have sufficient evidence to support decisions, components, and sequences, they must be evaluated as they operate. The sequential multiple-assignment randomized trial is a design that experimentally assesses the efficacy of the decisions, components, and sequence of an adaptive intervention.
A Sequential Multiple Assignment Randomized Trial (SMART) is a design that involves multiple stages of randomization. Patient's characteristics and treatment history are used to re-randomize them. Many clinically important questions can be answered using SMART designs.
A sequential multiple assignment randomized trial (SMART) is a novel trial design that can test these dynamic treatment regimens and lead to treatment guidelines that more closely mimic practice. Aim To characterize a SMART design in comparison to traditional approaches for stroke reperfusion trials.
Strategies to improve implementation of outpatient, primary care goals of care conversations are needed.Methods: We plan a cluster randomized (clinician-level) sequential, multiple assignment randomized trial to evaluate the effectiveness of patient implementation strategies on the outcome of goals of care conversation documentation when ...
Sequential multiple assignment randomized trials (SMARTs, discussed in more detail below) have been proposed to facilitate or accelerate the development of ATSs and represent an important advancement in clinical research methodology.[5, 23-25] SMARTs can be used: (1) to discover which treatments work together in a sequence so as to lead to ...
About this code. On this page, we provide example datasets, analysis code in SAS and R, and outputs, for the three kinds of hybrid experimental designs considered in " Design of Experiments with Sequential Randomizations at Multiple Time Scales: The Hybrid Experimental Design." The specific hybrids considered combine: a classic factorial experiment with a sequential multiple assignment ...
Colorectal adenomas have the potential of malignant transformation if left untreated. Multiple randomized controlled trials have been performed to evaluate the efficacy of aspirin in preventing colorectal adenoma recurrence in a population with a history of colorectal adenoma but not colorectal cancer, however, the relationship between aspirin dose and colorectal adenoma recurrence remains ...
The second is the Sequential Multiple Assignment Randomized Trial (SMART) which is an innovative research design especially suited for building time-varying adaptive interventions. A SMART trial can be used to identify the best tailoring variables and decision rules for an adaptive intervention empirically.