- Help & FAQ
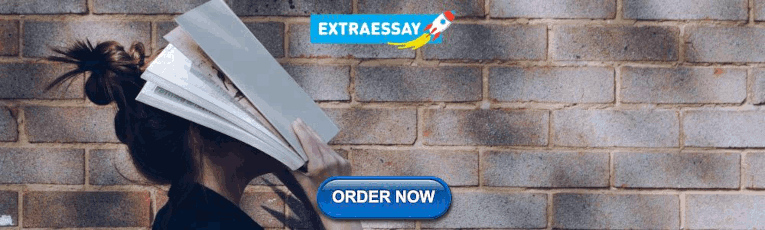
Cost models of additive manufacturing: A literature review
- Mechanical Engineering
Research output : Contribution to journal › Article › peer-review
- Additive manufacturing
- Additive manufacturing cost model
Access to Document
- 10.5267/j.ijiec.2016.9.001 Licence: None: All rights reserved
Fingerprint
- 3D printers Engineering & Materials Science 100%
- Costs Engineering & Materials Science 38%
- Mechanical properties Engineering & Materials Science 32%
- Operations research Engineering & Materials Science 28%
- Industry Engineering & Materials Science 11%
T1 - Cost models of additive manufacturing: A literature review
AU - Costabile, G
AU - Fera, M
AU - Fruggiero, F
AU - Lambiase, Alfredo
AU - Pham, Duc
N2 - From the past decades, increasing attention has been paid to the quality level of technological and mechanical properties achieved by the Additive Manufacturing (AM); these two elements have achieved a good performance, and it is possible to compare this with the results achieved by traditional technology. Therefore, the AM maturity is high enough to let industries adopt this technology in a more general production framework as the mechanical manufacturing industrial one is. Since the technological and mechanical properties are also beneficial for the materials produced with AM, the primary objective of this paper is to focus more on managerial facets,such as the cost control of a production environment, where these new technologies are present. This paper aims to analyse the existing literature about the cost models developed specifically for AM from an operations management point of view and discusses the strengths and weaknesses of all models.
AB - From the past decades, increasing attention has been paid to the quality level of technological and mechanical properties achieved by the Additive Manufacturing (AM); these two elements have achieved a good performance, and it is possible to compare this with the results achieved by traditional technology. Therefore, the AM maturity is high enough to let industries adopt this technology in a more general production framework as the mechanical manufacturing industrial one is. Since the technological and mechanical properties are also beneficial for the materials produced with AM, the primary objective of this paper is to focus more on managerial facets,such as the cost control of a production environment, where these new technologies are present. This paper aims to analyse the existing literature about the cost models developed specifically for AM from an operations management point of view and discusses the strengths and weaknesses of all models.
KW - Additive manufacturing
KW - Additive manufacturing cost model
UR - http://www.m.growingscience.com/ijiec/Vol8/IJIEC_2016_26.pdf
U2 - 10.5267/j.ijiec.2016.9.001
DO - 10.5267/j.ijiec.2016.9.001
M3 - Article
SN - 1923-2926
JO - International Journal of Industrial Engineering Computations
JF - International Journal of Industrial Engineering Computations
From the past decades, increasing attention has been paid to the quality level of technological and mechanical properties achieved by the Additive Manufacturing (AM); these two elements have achieved a good performance, and it is possible to compare this with the results achieved by traditional technology. Therefore, the AM maturity is high enough to let industries adopt this technology in a more general production framework as the mechanical manufacturing industrial one is. Since the technological and mechanical properties are also beneficial for the materials produced with AM, the primary objective of this paper is to focus more on managerial facets, such as the cost control of a production environment, where these new technologies are present. This paper aims to analyse the existing literature about the cost models developed specifically for AM from an operations management point of view and discusses the strengths and weaknesses of all models.
Cost models of additive manufacturing: A literature review
Scheda breve scheda completa scheda completa (dc).
I documenti in IRIS sono protetti da copyright e tutti i diritti sono riservati, salvo diversa indicazione.
Informazioni

social impact
Conferma cancellazione.
Sei sicuro che questo prodotto debba essere cancellato?
simulazione ASN
Additive manufacturing cost estimation models—a classification review
- Related Documents
Designing for RTM using Manufacturing Cost Estimation Models
Additive manufacturing cost estimation for buy scenarios.
Purpose To design for additive manufacturing (AM), the decision to use AM needs to be taken early in the product development process. Therefore, engineers need to be able to estimate AM part cost based on the few parameters available at this point in the process. This paper aims to develop suitable cost estimation models for this purpose, focusing on buy scenarios, as many companies choose to buy parts at service providers. Design/methodology/approach This study applies analogical cost estimation techniques to a data set of price quotations for laser sintering and laser melting parts. Findings The paper proposes easy-to-apply cost estimation models for laser sintering and laser melting for buy scenarios. Further, it generates new insights on the AM service provider market. Research limitations/implications The proposed models are only suitable for buy scenarios and are only a snapshot of cost achievable in 2014. Practical implications The proposed cost estimation models enable engineers to approximate AM part costs early in the product development process and thereby ease the decision to rapid manufacture certain parts. Originality/value This study addresses two gaps in the AM cost literature. It is the first study to take a qualitative approach to AM cost estimation, which is more suitable early in the product development process than the currently available quantitative studies. Further, it develops the first cost estimation for buy scenarios.
Learning Component Size Distributions for Software Cost Estimation: Models Based on Arithmetic and Shifted Geometric Means Rules
Are software cost-estimation models accurate, software cost estimation models, visual comparison of software cost estimation models by regression error characteristic analysis, empirical studies of assumptions that underlie software cost-estimation models, development of open-pit mine reclamation cost estimation models: a regression-based approach, evaluation of the cost estimation models: case study of task manager application, ranking and clustering software cost estimation models through a multiple comparisons algorithm, export citation format, share document.
- All Fields Titles Keywords Authors
- Advanced Search
Growing Science » International Journal of Industrial Engineering Computations » Cost models of additive manufacturing: A literature review
- IJIEC (630)
IJIEC Volumes
- Volume 1 (17)
- Issue 1 (9)
- Issue 2 (8)
- Volume 2 (68)
- Issue 1 (12)
- Issue 2 (20)
- Issue 3 (20)
- Issue 4 (16)
- Volume 3 (76)
- Issue 2 (15)
- Issue 4 (12)
- Issue 5 (20)
- Volume 4 (50)
- Issue 1 (14)
- Issue 2 (10)
- Issue 3 (12)
- Issue 4 (14)
- Volume 5 (47)
- Issue 1 (13)
- Issue 2 (12)
- Issue 4 (10)
- Volume 6 (39)
- Issue 1 (7)
- Issue 3 (10)
- Volume 7 (47)
- Issue 1 (10)
- Issue 2 (14)
- Issue 4 (13)
- Volume 8 (30)
- Issue 2 (7)
- Issue 3 (8)
- Issue 4 (6)
- Volume 9 (32)
- Issue 2 (6)
- Issue 3 (7)
- Volume 10 (34)
- Issue 1 (8)
- Issue 4 (8)
- Volume 11 (36)
- Issue 3 (9)
- Volume 12 (29)
- Volume 13 (41)
- Volume 14 (50)
- Issue 1 (11)
- Issue 4 (15)
- Volume 15 (34)
- Issue 1 (19)
International Journal of Industrial Engineering Computations
Cost models of additive manufacturing: a literature review pages 263-283 download pdf.
Authors: G. Costabile , M. Fera , F. Fruggiero , A. Lambiase , D. Pham
DOI: 10.5267/j.ijiec.2016.9.001
Keywords: Additive manufacturing Additive manufacturing cost model
Journal: International Journal of Industrial Engineering Computations | Year: 2017 | Volume: 8 | Issue: 2 | Views: 8451 | Reviews: 0
- Performance appraisal of Indian state police forces using ARAS method
- Vendor selection and order allocation using an integrated fuzzy mathematica ...
- Ranking periodic ordering models on the basis of minimizing total inventory ...
- A case study to estimate costs using Neural Networks and regression based m ...
- A new approach for product cost estimation using data envelopment analysis
Add Reviews
® 2010-2024 GrowingScience.Com

An official website of the United States government
Here’s how you know
Official websites use .gov A .gov website belongs to an official government organization in the United States.
Secure .gov websites use HTTPS A lock ( Lock A locked padlock ) or https:// means you’ve safely connected to the .gov website. Share sensitive information only on official, secure websites.
https://www.nist.gov/publications/costs-and-cost-effectiveness-additive-manufacturing
Costs and Cost Effectiveness of Additive Manufacturing
Download paper, additional citation formats.
- Google Scholar
If you have any questions about this publication or are having problems accessing it, please contact [email protected] .
Thank you for visiting nature.com. You are using a browser version with limited support for CSS. To obtain the best experience, we recommend you use a more up to date browser (or turn off compatibility mode in Internet Explorer). In the meantime, to ensure continued support, we are displaying the site without styles and JavaScript.
- View all journals
- My Account Login
- Explore content
- About the journal
- Publish with us
- Sign up for alerts
- Review Article
- Open access
- Published: 03 June 2024
A comprehensive review of sustainable materials and toolpath optimization in 3D concrete printing
- Zicheng Zhuang 1 na1 ,
- Fengming Xu 1 na1 ,
- Junhong Ye 1 na1 ,
- Liming Jiang 3 &
- Yiwei Weng 1
npj Materials Sustainability volume 2 , Article number: 12 ( 2024 ) Cite this article
187 Accesses
Metrics details
- Climate sciences
- Environmental sciences
The construction sector has experienced remarkable advancements in recent years, driven by the demand for sustainable and efficient building practices. Among these advancements, 3D concrete printing has emerged as a highly promising technology that holds the potential to revolutionize the construction industry. This review paper aims to provide a comprehensive analysis of the latest developments in three vital areas related to 3D concrete printing: sustainable materials, structural optimization, and toolpath design. A systematic literature review approach is employed based on established practices in additive manufacturing for construction to explore the intersections between these areas. The review reveals that material recycling plays a crucial role in achieving sustainable construction practices. Extensive research has been conducted on structural optimization methodologies to enhance the performance and efficiency of 3D printed concrete structures. In the printing process, toolpath design plays a significant role in ensuring the precise and efficient deposition of concrete. This paper discusses various toolpath generation strategies that take factors such as geometric complexity, printing constraints, and material flow control into account. In summary, the insights presented in this paper may serve as guidelines for researchers, engineers, and industry professionals towards sustainable and efficient construction practices using 3D concrete printing technology.
Similar content being viewed by others
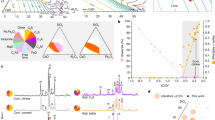
Electric recycling of Portland cement at scale
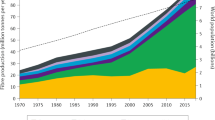
The environmental price of fast fashion
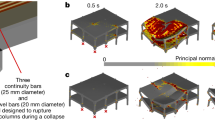
Arresting failure propagation in buildings through collapse isolation
Introduction.
Climate change has emerged as a global challenge due to the substantial carbon emissions and energy consumption. In 2022, the global carbon emissions and energy consumption reached 36.8 gigatons and 14,585 million tonnes of oil equivalent 1 , 2 , respectively. The construction sector is a major contributor to global carbon emission and energy consumption, accounting for 40% and 36% in 2022 3 , respectively. With the urban population estimated to increase to 68% by 2050, the environmental impacts of the construction sector will continuously increase 4 , underscoring the urgent need for developing sustainable construction technologies.
3D concrete printing (3DCP), also known as additive manufacturing (AM) in the construction sector 5 , offers a promising solution for achieving sustainable construction. 3DCP constructs structures by depositing printable concrete materials layer-atop-layer based on a pre-designed building model. The unique construction process possesses the advantages of enhanced sustainability and design flexibility. For example, a prefabricated bathroom unit (PBU) constructed by 3DCP achieved a reduction of 85.9% and 87.1% in carbon emissions and energy consumption, respectively, compared to that of a mold-cast counterpart 6 .
3DCP has gained much attention from both academia and engineering. Figure 1a shows the rapid growth in the publications and citations related to the keywords of “3DCP” based on data obtained from Web of Science. The number of publications reached 444 and 420 in 2022 and 2023, respectively. In these publications, several review works have been conducted in the fields of 3DCP and its potential applications 7 , 8 , 9 , 10 . Wangler et al. 8 present a technical review of 3DCP from fresh materials to hardened materials and further practical applications. Lu et al. 9 provide a comprehensive review of the material behaviors of 3DCP. However, the review articles primarily focus on the technical or material advancements of 3DCP, with less attention given to its sustainability aspects. Figure 1b illustrates the growth of publications related to the keywords of “3DCP and Sustainability”. Despite the growing interest in 3DCP, only 46 publications in 2023, approximately 10% of total 3DCP-related publications, focused on sustainability (Fig. 1a ). Among these publications, Dey et al. 11 provide a comprehensive review of the utilization of industrial wastes in printable materials to improve the sustainability of 3DCP. However, there is a lack of in-depth understanding of how to improve sustainability in 3DCP across its various construction processes.
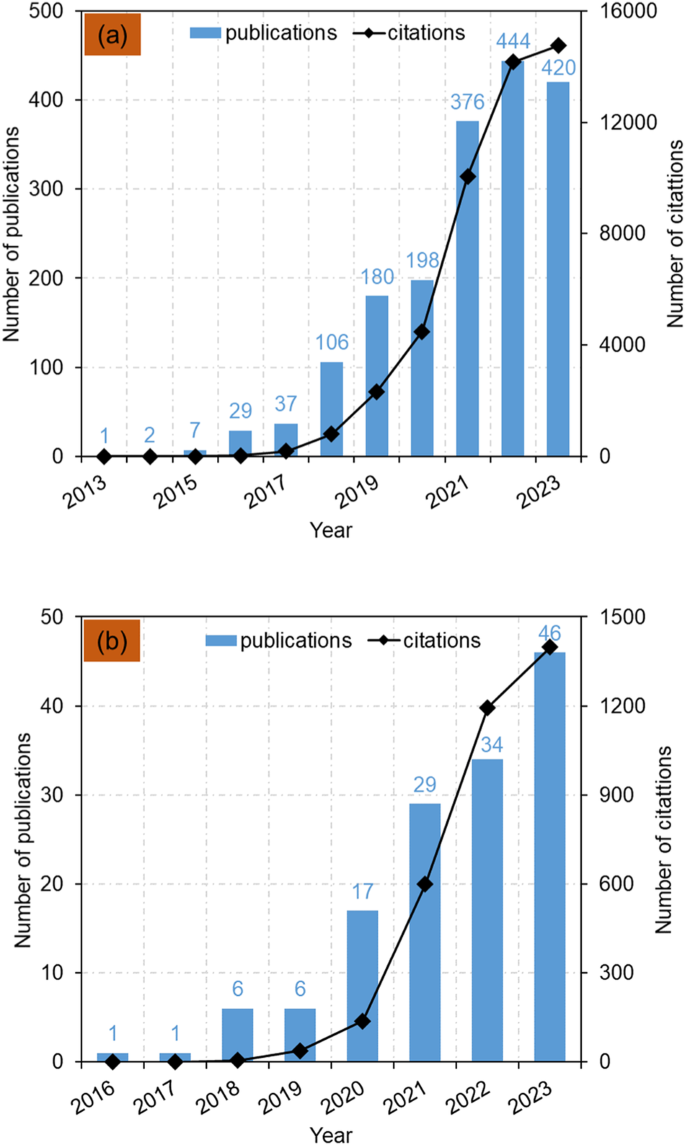
a Keywords of “3DCP”; b keywords of “3DCP and Sustainability”.
The typical construction processes of 3DCP include the development of printable materials, structural optimization, toolpath design, and printing 12 , as shown in Fig. 2 . Each of these processes offers opportunities for enhancing sustainability. At the material level, sustainability can be improved by developing printable materials incorporated with waste materials. The waste materials are used as the substitutions of aggregate and binder contents, thereby reducing the carbon emission associated with the material extraction. At the structural level, the design of hollow structures via topology optimization (TO) 13 , 14 reduces the material usage and thus enhances sustainability. TO involves the optimization of material distribution to achieve the desired performance. In addition, the design flexibility of 3DCP is compatible with the structural TO. Finally, to implement the optimized structure into 3DCP, toolpath design methods 15 , 16 are adopted to determine the efficient path for sustainable concrete printing. The integration of sustainable printable materials, TO, and toolpath design techniques with 3DCP represents a promising synergy for future research and sustainability development in the construction sector. However, comprehensive reviews covering these three aspects are currently lacking in the existing literature.
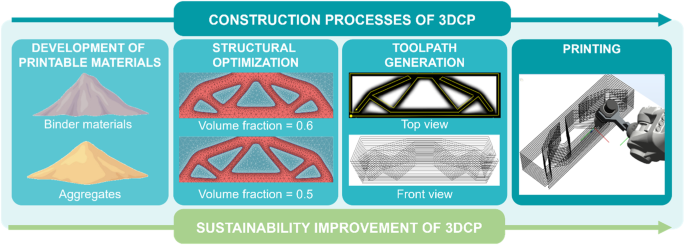
The typical processes include the development of printable materials, structural optimization, toolpath generation, and printing.
This paper aims to fill the abovementioned research gap by providing a comprehensive review of sustainable materials, structural topology optimization, and toolpath planning for the enhancement of sustainability in 3DCP. Based on the findings of these reviewed articles, the perspectives and methods to enhance sustainability with respect to the abovementioned three aspects of 3DCP are highlighted. Finally, Section 5 conclusions are summarized and future research directions are identified.
Sustainable materials in 3D concrete printing
Integrating sustainable materials into 3DCP is a potential strategy for enhancing the sustainability of 3DCP 17 since the construction sector increasingly focuses on the recycling of natural resources, reduction in material waste and carbon emissions. The commonly developed 3D printable cementitious materials consist of binder materials (primarily cement), natural fine aggregates, additives, admixtures, and water 18 . However, two main challenges impede the development of sustainable 3D printable cementitious materials. Firstly, the high usage of ordinary Portland cement (OPC, 700–800 kg/m 3 ) 18 impacts sustainability due to the associated carbon footprint 8 . Secondly, during the printing process, most developed material mixtures only use fine aggregates for 3DCP due to the limitation of the pumping process and nozzle opening 19 , 20 .
Employing sustainable binder and aggregate alternatives is a potential solution to address these challenges 6 , 21 . This section reviews relevant advancements in adopting recycled aggregates and supplementary cementitious materials (SCMs) into 3D printable materials. Figure 3 shows the number of publications associated with the keywords “3D printed concrete”, “Recycled glass”, “Recycled sand”, “Recycled concrete aggregate”, “Recycled plastics”, “Recycled rubber”, “3D printed concrete”, “Silica fume”, “Rice husk”, “Fly ash”, “Limestone”, “Calcined clay”, “Granulated blast-furnace slag” and “Sustainable” from the Web of Science database. As shown in Fig. 3 , a growing academic interest is observed related to recycled aggregates and SCMs. The following sections discuss the performance characteristics and implications of these sustainable materials in 3DCP applications.
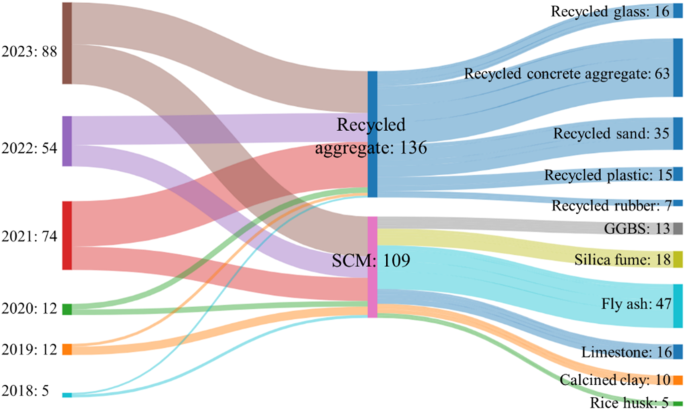
The literature study includes research on two main types of sustainable materials, recycled aggregates and SCM, from 2018 to 2023.
The impact of recycled aggregates, such as recycled glass 22 , concrete 23 , plastics 24 , and rubber 25 , alongside SCMs, such as silica fume 26 , rice husk ash 27 , fly ash 28 , limestone 29 , calcined clay 30 , and granulated blast-furnace slag (GGBS) 31 , on the fresh and hardened properties of 3D printable materials are analyzed. The analysis underscores the importance of these materials in advancing 3DCP sustainability but also reveals the future potential research direction to mitigate environmental impacts and foster sustainable development in 3D printable cementitious materials 19 , 32 , 33 .
3D printable material performance with recycled aggregates
The primary recycled materials in 3D printed concrete for sustainability enhancement include sand 34 , glass 22 , concrete 35 , plastics 24 , and rubber 25 . According to the data from the Hong Kong Environmental Protection Department in 2022 36 , daily waste generation in Hong Kong includes 222.6 tons of glass and 2336.9 tons of plastics. In addition, concrete and sand, derived mainly from construction and demolition debris and construction waste, account for a considerable portion of the waste, with daily production of construction waste reaching 49,865 tons 36 . In the blueprint for Hong Kong 2035 37 , the government proposes a new target concerning “Waste Reduction, Resources Circulation, Zero Landfill”, which presents a significant challenge for the recycling of waste materials in sustainable construction.
In 3DCP, it is essential to achieve a balance of fresh properties and hardened properties for printable materials. Fresh properties such as printability and pumpability, hardened properties such as strength and durability, and sustainability are critical factors for material tailoring 19 , 38 . Recycled aggregates are sustainable alternatives to natural aggregates, helping to conserve natural resources and reduce land waste from landfills 24 , 39 , 40 , 41 . This section discusses the various recycled aggregates in 3DCP (see Table 1 for details) to illustrate their impact on material fresh and hardened performance as well as sustainability.
Impacts of recycled aggregates on fresh properties
Summarizing the findings from Table 1 , the usage of recycled aggregates impacts the fresh properties of cementitious materials. The fresh properties are critical factors, which determine the printability of materials during the printing process. The printability can be characterized by workability, pumpability, extrudability, and buildability 34 . In the 3D printing process, the most essential steps are conveying mixed materials to the nozzle via a delivery system and depositing materials to build the solid object in a layer-by-layer manner 42 . In the conveying step, the materials are required to have good workability and pumpability, which indicates how easily the material can be conveyed. In addition, extrudability indicates the ability of a material to be extruded with minimal energy consumption during the delivery 43 . In the deposition step, the materials are required to have good buildability, which indicates how well the materials can be stacked stably.
With respect to workability, research has indicated that the presence of recycled sand, characterized by its high water absorption rate and irregular shape, tends to reduce the workability of concrete 44 . Similarly, incorporating recycled rubber particles with poor shape and rough surfaces has diminished the workability of 3D printed concrete, resulting in the slow relative motion of rubber particles within the concrete mixture, causing reduced processability 25 . In terms of pumpability, studies conducted by Ting et al. 45 have shown that adding recycled glass to concrete reduces its pumpability. This phenomenon can be attributed to recycled glass particles’ angular and sharp-edged nature, which obstruct flow and decrease pumpability.
Analyzing the extrudability in recycled aggregate concrete, it has been observed that the high water absorption of recycled sand necessitates the addition of extra water and superplasticizers to enhance the extrudability of 3D printed concrete 34 . In addition, the water-absorbing nature of surface cracks in recycled rubber can result in reduced extrudability. However, subjecting recycled rubber to heat treatment can partially close these surface cracks, reducing water absorption and significantly improving extrudability 46 .
Finally, with respect to buildability, increasing the substitution rate of recycled concrete aggregates has been found to improve the buildability of 3D printed concrete. Liu et al.’s 35 research suggests that the buildability increases with the rising substitution rate of recycled concrete aggregates due to the reduction in concrete density. Conversely, studies involving recycled plastics have revealed that while plastic’s hydrophobic nature enhances material flow, it also delays the hydration reaction of calcium silicate, slowing the thixotropic behavior of concrete and ultimately reducing its buildability 24 , 41 .
In summary, recycled aggregates’ influence on cementation materials’ fresh properties is multifaceted and crucial for 3D printing applications. Workability can be compromised by recycled sand and rubber, while pumpability may be hindered when using recycled glass due to its angular characteristics. Extrudability can be improved with additional water and heat treatment for specific recycled materials. In addition, buildability is positively correlated with higher substitution rates of recycled concrete aggregates, while challenges arise from the delayed hydration reaction of calcium silicate when recycled plastics are involved. These insights underscore the need for careful material selection and processing adjustments to optimize the performance of 3D printable materials.
Impacts of recycled aggregates on mechanical properties and sustainability
The mechanical performance of printed structures is paramount for ensuring their structural integrity and safety. Table 1 summarizes the mechanical properties of various types of recycled aggregates, revealing their impact on the mechanical properties of 3D printed concrete. Specifically, incorporating recycled materials such as recycled sand, coarse aggregates, glass, and plastics as sustainable alternatives in concrete leads to decreased compressive strength with increasing substitution rates 22 , 24 , 34 , 35 . This reduction in compressive strength can be attributed to the increased porosity within the concrete resulting from the addition of recycled materials, with higher porosity leading to reduced compressive strength 24 , 39 .
Beyond the problem of increased porosity, the bond between recycled aggregates and the cement matrix plays a significant role in the mechanical performance of 3D printed concrete. The smoother surface and sharper edges of recycled glass particles compared to that of natural sand particles may result in weaker bonding between the particles and the cement matrix at the interface transition zone, decreasing mechanical strength 45 . The inherent properties of recycled aggregates also impact the strength of 3D printed concrete. Recycled concrete aggregates containing old mortar and aggregates with adhering old mortar, which have lower mechanical properties, can serve as weak areas of a structure, decreasing mechanical performance 35 . On the contrary, adding cement-coated modified recycled rubber in 3D printed concrete enhances its compressive strength. This enhancement is primarily attributed to the transformation of the rubber from a hydrophobic material to a hydrophilic material after modification, promoting its interaction with the fresh mortar during mixing and resulting in a more compact interface transition zone within the structure 33 .
These findings emphasize the necessity of incorporating recycled aggregates in 3D printed concrete in appropriate amounts after considering the structural integrity and safety to achieve the desired overall properties of 3D printed concrete. As a type of sustainable material, utilizing recycled aggregates in 3DCP can reduce material costs and mitigate environmental impacts 47 . Han et al. indicate that as the proportion of recycled aggregates increases from 0% to 100%, the CO 2 emissions of 3D printed concrete decrease from 5637.647 kg to 5499.505 kg 48 . Cost analyses demonstrate a downward trend in the total cost of 3D printed concrete with the increasing proportion of recycled aggregates. For instance, the costs of 3D printed concrete with recycling proportions of 0%, 50%, and 100% are 12,913.54 CNY, 12,555.77 CNY, and 12,194.97 CNY, respectively 48 . This underscores that increasing the proportion of recycled aggregates can effectively reduce greenhouse gas emissions during concrete production and enhance building materials’ sustainability in the practical application.
3D printable material performance with supplementary cementitious materials
This section explores the impact of supplementary cementitious materials (SCMs) on the performance of 3D printable cementitious materials. In the area of 3DCP, a significant aspect is its heavy reliance on OPC compared to traditional concrete 18 . Specifically, 3D printable cementitious materials contain more than 20% of OPC, expressed by mass weight due to the requirements of printability 19 . Including SCMs in material mixtures is an alternative solution to address his problem. Various types of SCMs have been adopted for the mixture design of 3D printable concrete in the existing literature, such as fly ash, ground granulated blast furnace slag, and calcined clay from various industrial processes 49 . Fly ash, a residue from coal combustion in power plants 50 , and calcined clay, derived from high-temperature treatment of clay materials 29 , are among these industrially sourced SCM. In addition, GGBS originates from the milling process of waste slag from steel production 51 , while silica fume comes from silicon ferroalloy smelting 52 , and rice husk ash is a by-product of rice milling 27 . Incorporating these SCMs reduces the environmental burden associated with concrete production and addresses the high carbon dioxide emissions from cement production 29 , 53 .
In the selection of SCMs for 3D printable concrete, optimizing characteristics such as fresh properties, mechanical properties, durability, and sustainability is crucial 29 , 30 , 54 . These attributes directly impact the efficiency of the printing process and the performance of the final structure. Table 2 summarizes the material characteristics of individual SCM used in 3DCP and their impacts on the performance of 3D printable concrete by a systematic literature review.
Impacts of SCMs on fresh properties
Based on Table 2 , the utilization of SCMs affects the printability of 3D printed concrete. These parameters serve as crucial indicators of the stability and performance of materials during processes such as pumping, extrusion, and bearing continuous printing layer loads. In terms of workability, adding silica fume reduces the workability of 3D printed concrete. This is primarily attributed to the high surface area of silica fume, which easily aggregates with cement particles to form flocculent structures, partially hindering the free flow of water, and therefore, affecting the workability 26 , 53 .
In terms of pumpability and extrudability, the appropriate addition of fly ash and GGBS can enhance the pumpability and extrudability of cementitious materials. This is primarily attributed to the spherical and smooth surface characteristics of fly ash 55 and GGBS 56 , as shown in Table 2 , and therefore, contribute to improving the extrudability of concrete. However, excessive fly ash and GGBS may diminish extrudability due to increased water absorption. As the dosage increases, the water absorption rate rises, resulting in increased viscosity, thereby impeding the extrusion process during 3D printing 57 . The replacement of cement with silica fume 26 , rice husk ash 27 , limestone, and calcined clay 29 can enhance buildability. For example, torque viscosity rises while flow resistance and thixotropy are decreased with the rise of fly ash-to-cement ratio, negatively impacting the buildability 55 . Conversely, the influence of the silica fume-to-cement ratio shows an opposite trend on rheological properties as compared to that of the fly ash-to-cement ratio. Adding silica fume increases the filler content in concrete, strengthening the interaction between particles and thereby improving the 3D printing performance of the material 50 . Rice husk ash exhibits strong water absorption capability, reducing voids between concrete particles and promoting flocculation and hydration product formation, thereby enhancing the buildability of 3D printed concrete 27 . The addition of limestone and calcined clay can enhance the buildability due to the reduced water film thickness 30 .
In summary, incorporating SCMs significantly impacts the workability, pumpability, extrudability, and buildability of 3D printed concrete. While silica fume reduces workability due to its high surface area 26 , fly ash and GGBS can enhance pumpability and extrudability when added appropriately. However, excessive amounts may hinder extrudability due to increased water absorption 57 . Substituting cement with fly ash, silica fume, rice husk ash, limestone, and calcined clay enhances buildability 26 , 27 , 58 .
Impacts of SCMs on mechanical properties and sustainability
The mechanical performance of 3D printed concrete is crucial for construction practices. Incorporating SCMs can reduce the environmental impact and directly influence the mechanical properties of 3D printed concrete. Studies have shown that materials such as silica fume 26 , limestone, and calcined clay 29 can positively impact the mechanical properties of concrete. Silica fume acts as an inert filler in 3D printed concrete, filling voids, improving pore structure, and therefore, enhancing mechanical performance 50 . Liu et al. 26 attributed the improvement in the mechanical properties of silica fume to the fact that silica fume increases the density of the concrete, which increases the pore densities and reduces the number of connecting and oversized pores.
Moreover, the quantity of SCMs added also affects the mechanical properties of 3D printed concrete. Increasing the content of limestone and calcined clay can increase the amount of fine particles in concrete, promoting microstructure development 54 . However, small additions of fly ash and GGBS can enhance mechanical properties but excessive amounts may compromise concrete strength. This is mainly due to that the high amount of replacement of cement with fly ash or GGBS reduces the initial cement hydration at the early stage 57 . As a result, the mechanical performance of 3D printable concrete decreases. Therefore, when designing formulations for 3D printed concrete, it is essential to consider the type, quantity, and interactions of SCMs to achieve optimal mechanical performance and ensure the sustainability and durability of structures.
In 3DCP, the CO 2 emission in the material production stage is 583.1 kg CO 2 -eq/m 3 , 75% of which is contributed by the production of cement and other binder materials 18 . Therefore, using SCMs as the substitution of binder materials showed possible advantages in enhancing the environmental sustainability of 3D printable concrete 26 , 28 , 54 . Most of the reviewed studies focus on the fresh and hardened properties of 3D printable concrete with SCMs, with limited attention to the quantitative carbon emission assessment of the materials. Long et al. 59 reported that the 3D printable Limestone & Calcined clay cement composites (LC3) reduced carbon emission by 45% and energy consumption by 40%. Conversely, Yao et al. 60 reported that the carbon emission of printable materials was when geopolymer was used as the binder material. The increased carbon emission of geopolymer was due to the use of silicate (alkaline activator). Liu et al. 61 reported that the printable materials with fly ash showed less carbon emission compared to that of the printable geopolymer concrete. Different conclusions were drawn from the existing articles in terms of the carbon emission of 3D printable materials with SCM. Therefore, to comprehensively assess the sustainability effectiveness of SCMs in 3DCP, additional research is necessary in future works by conducting the quantitative carbon emission assessment.
Conventional structural topology optimization methods
Traditional design principles and considerations are being re-evaluated to leverage the unique capabilities provided by 3D printing 62 . This section aims to review the specific structural optimization methods and considerations tailored for 3DCP technology, with a particular focus on the potential to create functional, efficient, and sustainable designs using topology optimization approaches.
Structural topology optimization is the process of arranging the distribution of materials within a specified design domain to maximize specific mechanical or physical properties, while adhering to prescribed constraints. This concept arose in 1904 when Michell proposed a theoretical analysis to obtain the lightest truss 63 . The advent of finite element analysis (FEA) and the development of the widely used homogenization method 64 , 65 in the late 1980s significantly progressed this concept. Since then, the field has seen substantial advancements, thanks to methods such as Solid Isotropic Microstructure with Penalization (SIMP) 66 , Evolutionary Structural Optimization (ESO) 67 , Bi-directional Evolutionary Structural Optimization (BESO) 68 , 69 , and level set method 70 , 71 . These developments have allowed for more sophisticated and efficient designs and further expanded the possibilities of structural topology optimization. As shown in Table 3 , the various topology optimization approaches have continuously evolved to improve their effectiveness and efficiency, which are introduced individually in this section.
After the introduction of the homogenization-based topology optimization method by Bendsoe and Kikuchi 64 and later developments by Bendsoe 72 , the SIMP method was proposed 73 , 74 . Sigmund 75 provided a clear explanation of the numerical implementation of the SIMP method in 2001 using a concise 99-line Matlab code. The SIMP method assumes constant material properties for the solid material within the design domain. The design variables in the optimization process are the relative densities of each element, which range between zero and one. The material properties are modeled as the relative material density raised to a power multiplied by the properties of the solid material. During the early 1990s, Xie and Steven initially put forth the Evolutionary Structural Optimization (ESO) method to attain optimal topologies for continuum structures 67 , 76 , 77 . Subsequently, Querin et al. 68 and Yang et al. 78 advanced the ESO method to develop the Bi-directional Evolutionary Structural Optimization (BESO) method. The level set-based topology optimization method utilizes a higher-dimensional embedded function to implicitly represent solid-void interfaces 79 , 80 . In the traditional level set method, the Hamilton-Jacobi equation (PDE) is solved using the velocity normal to the interface 71 , 81 , 82 . The zero-level contour of the embedded function in the conventional level set method defines the material boundary, serving as the partition between the solid and void domains.
Advanced structural topology optimization methods
In recent years, a variety of innovative optimization algorithms have emerged to tackle the practical challenges associated with flexible design domains, smooth material boundaries, and complex fabrication constraints. One such method is the Reaction diffusion-based level set (RDLS) approach, which was initially introduced in 2014 83 . The RDLS method enables the specification of geometrical complexities within the optimal configuration, thereby facilitating the identification of the desired structure shape through the evolution of the level set function. Another notable advancement is the Floating projection topology optimization (FPTO) method, which was unveiled in 2021 84 . FPTO ensures that design variables take discrete values, resulting in more robust and practical optimization outcomes. Lastly, the Node moving-based topology optimization (NMTO) method, introduced in 2023 85 utilizes a narrowband offset from the structural profile to establish a signed-distance function, which determines the direction of node movement. NMTO aims to optimize the structural topology and enhance its overall performance by manipulating node positions. These cutting-edge methods show great promise for advancing the capabilities of 3DCP and optimizing the production of high-performance structures.
Nowadays, structural topology optimization has become increasingly popular in various fields, including additive manufacturing 69 , 86 , architectural design 87 , 88 , biochemical 89 , 90 , and aerospace engineering 91 , 92 . Among them, the high design flexibility of 3DCP makes it compatible with topology optimization to decrease material usage and improve sustainability. With the integration of these approaches and 3DCP, it becomes possible to create intricate designs that are both structurally sound and resource-efficient.
To find an appropriate method for 3DCP, the benefits and limitations of each topology optimization method should be fully understood, which are introduced and summarized in this subsection. The key scientific differences between the various topology optimization methods include mathematical formulation, optimization algorithms, material models, sensitivity analysis, and post-processing techniques.
The advantages and disadvantages of these topology optimization methods can be concluded to judge whether they can be integrated with 3DCP to fabricate efficient and environmentally friendly structures. For instance, the homogenization method allows for accurate computation of material properties using a systematic approach to obtain optimal topology. However, it may not be suitable for structures with complex material distributions and may struggle with handling geometric complexities. The SIMP method is advantageous as it provides a simple and effective way to model material properties and incorporate manufacturing constraints. Nevertheless, it produces designs with intermediate densities and may suffer from numerical instabilities. Next, the ESO method offers improved utilization of material resources by gradually removing ineffective material but may require a large number of iterations and struggle with complex geometries. Similarly, the BESO method efficiently optimizes structures by employing fundamental strategies but may produce designs with checkerboard patterns and require careful parameter tuning. On the other hand, the conventional level set method utilizes higher-dimensional embedded functions to implicitly represent solid-void interfaces accurately, which can handle topological changes during the optimization process. Nonetheless, it requires careful handling of interface tracking to avoid spurious geometries and may suffer from numerical diffusion and grid-related issues.
On the other hand, the RDLS method allows for specifying geometrical complexity but requires significant computational resources. Besides, this method is sensitive to parameter settings. The FPTO method incorporates floating projection constraints and heuristically simulates 0/1 constraints of design variables, leading to discrete and practical solutions, that provide robust optimization results by considering upper and lower bounds. However, the method’s heuristic nature may not guarantee global optimality, and it may require careful tuning of parameters to balance feasibility and optimality. The NMTO method establishes a signed-distance function to determine node-moving directions, allowing for efficient topology optimization, complex structure design, and flexibility in node manipulation. The disadvantage of the NMTO method is that it may struggle with handling complex boundary conditions and geometric constraints. These are just some general advantages and disadvantages of the topology optimization methods mentioned.
In summary, the suitability of each method regarding 3DCP depends on specific applications and requirements. Different topology optimization methods employ various mathematical formulations to represent and solve the optimization problem. Each formulation has its advantages and limitations in terms of modeling flexibility, convergence behavior, and computational efficiency. Besides, topology optimization methods may differ in the sensitivity analysis approach employed to evaluate the influence of design changes on the objective function and constraints. After obtaining an optimized design, different methods employ various post-processing techniques to interpret and convert the obtained results into manufacturable forms. These techniques can include filtering, mesh smoothing, or shape reconstruction algorithms. The selection of post-processing techniques impacts the final quality, manufacturability, and practicality of the optimized design.
Structural topology optimization in 3D concrete printing
Structural topology optimization has been widely applied in the field of 3DCP, due to the benefits to create efficient and optimized structures. By combining these two techniques, engineers can maximize the use of material, reduce weight, and enhance load-bearing capabilities, resulting in more sustainable and cost-effective structures.
The emergence of 3DCP technology has revolutionized the field of structural design by providing unprecedented freedom in creating intricate geometries and customized structures 93 . This capability opens up new opportunities for designers to push the boundaries of traditional design principles 94 , 95 . By harnessing the inherent freedom of design, 3DCP can create structures that are aesthetically appealing and optimized for performance and functionality 87 . For instance, the optimization of material distribution in 3DCP is a vital research direction to minimize material waste and optimize structural efficiency 14 , 96 . Since the last decade, structural topology optimization has been increasingly applied in 3DCP 97 , 98 . Figure 4 shows the research article number in the last decade integrating different topology optimization approaches and 3DCP using the keywords “3D printed concrete”, “Homogenization method”, “SIMP method”, “ESO method”, “BESO method”, “Level set method”, and “Phase field method” based on data obtained from the Web of Science database. This section focuses on the approaches that have been explored to achieve structural topology optimization in 3DCP. These include using additive manufacturing techniques to build complex geometries and incorporating reinforcement elements during the printing process 14 , 86 , 99 . Existing works 96 , 97 have demonstrated the ability to optimize the internal structure of concrete components, resulting in improved mechanical properties and enhanced performance.
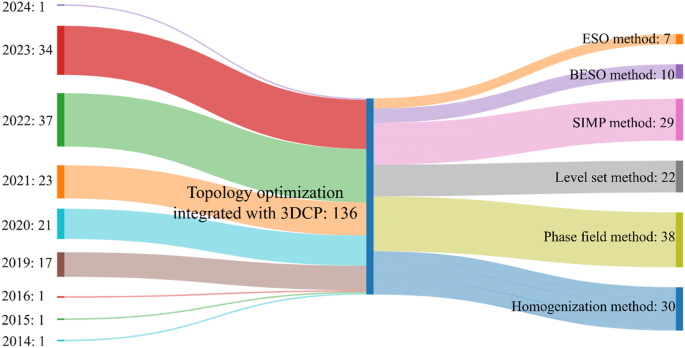
The literature study includes research on the application of six typical optimization methods from 2014 to 2024.
The integration of topology optimization and 3DCP has the potential to enhance the performance and resource efficiency of buildings. With the increasing emphasis on sustainable and eco-friendly practices, optimized structural design has emerged as a critical strategy to reduce material usage while maintaining structural strength 99 , 100 . For instance, the varying physical properties present in functionally graded materials can be customized to meet specific requirements, all while making efficient use of material resources 101 . Building on the multi-material BESO method, a novel approach to 3DCP structural design was introduced 102 . In this approach, 3DPC components primarily experience compression without the need for extra reinforcement. Instead, they synergistically collaborate with tensioned steel cables to create an effective composite structural system. The previous study 96 examined the production process of a topology-optimized 3D printed concrete bridge structure, highlighting its significant deviation from the manufacturing procedures of conventional concrete structures. Yang et al. 103 presented an integrated design method for 3DCP by incorporating extrusion-based manufacturing characteristics into the topology optimization algorithm. Lightweight structures tend to have better seismic performance, increased durability, and reduced energy consumption compared to their heavier counterparts 61 . In addition, lighter structures require less foundation support, resulting in cost savings during construction 104 . Since construction activities are responsible for a significant amount of carbon emissions, reducing the amount of material used can significantly decrease the carbon footprint of a building.
Several examples of a combination of topology optimization and waste materials have been achieved using additive manufacturing 105 , 106 . These technologies provide benefits including minimized waste materials, accelerated construction timelines, and the capacity to create distinctive designs with intricate details. In addition, they classify large-scale 3DCP technologies, emphasizing the importance of optimizing printing ink to enhance economic and environmental results by utilizing waste materials in 3DCP applications. The combination of topology optimization and waste materials offers numerous benefits. Firstly, it promotes sustainable design practices by utilizing recycled or waste materials, contributing to the circular economy and reducing waste. Secondly, it helps reduce costs as waste materials are often less expensive or even available for free compared to conventional materials. In addition, incorporating waste materials into the design improves resource efficiency by minimizing the need for extracting and processing new materials. Moreover, the unique properties of waste materials can enhance the performance of the optimized design, such as strength, durability, or lightweight. This combination also encourages innovation and creativity by exploring unconventional design solutions.
In summary, the integration of topology optimization and 3DCP can enhance the performance and resource efficiency of buildings. The impact of structural lightweighting on seismic performance, durability, and energy consumption makes it a compulsory consideration in achieving resource efficiency. In terms of future research directions, further advancements in structural topology optimization for 3DCP are anticipated. This includes developing advanced algorithms that can handle anisotropic, large-scale optimization problems and integrating multi-material printing capabilities. In addition, research efforts could focus on exploring the potential of bio-inspired design principles and incorporating functional requirements such as interlocking, thermal insulation, and acoustic performance into the optimization process.
Toolpath design and optimization in 3D concrete printing
Toolpath design is a critical aspect of 3DCP as it directly impacts the quality, efficiency, and structural integrity of the printed components. Firstly, toolpath design takes into account material-related problems, such as the flowability and workability of the concrete mixture. By carefully planning the toolpath, engineers can ensure that the material is properly deposited, minimizing problems, such as clogging or inconsistent layering. Toolpath design also addresses process-related concerns, such as the prevention of sagging or deformation during printing. Optimizing the toolpath by the integration of factors such as load-bearing capabilities, stress distribution, and reinforcement placement, can enhance the structural integrity of the printed components.
Toolpath planning determines the success of the 3DCP process. Toolpath design involves mapping out the trajectory and deposition strategy of the printing toolhead to ensure accurate material placement and optimal structural integrity 101 , 107 , 108 . By carefully coordinating the movement of the toolhead, designers can achieve precise layering, intricate geometries, improved sustainability, and desired material properties in the printed structure. Xia et al. 109 proposed an integrated design method to improve the mechanical performance and manufacturability of material extrusion structures according to the technical characteristics of material extrusion. The technical aspects of toolpath planning encompass various considerations, such as path optimization 110 , 111 , 112 , layer sequencing 113 , 114 , manufacturing constraints 14 , 115 , 116 , and support structure generation 86 , 117 , 118 .
Figure 5 illustrates the number of publications during the past decade related to the keywords “3D printed concrete”, “Extrusion-based toolpath design”, “Geometric toolpath design”, “Toolpath visualization”, “Manufacturing constraints”, “Topology optimization-based toolpath design”, “Sliced toolpath design”, and “Toolpath design efficiency/performance” based on data obtained from the Web of Science database. Path optimization algorithms aim to minimize print time, reduce material waste, and enhance printing efficiency by optimizing the toolhead’s movement trajectory. Layer sequencing determines the order in which layers are printed to ensure stability and prevent collapse during the printing process. Material flow control involves adjusting the printing parameters, such as nozzle speed and extrusion rate, to achieve consistent material deposition and avoid defects. Lastly, support structure generation ensures the stability of overhanging or complex geometries during printing.
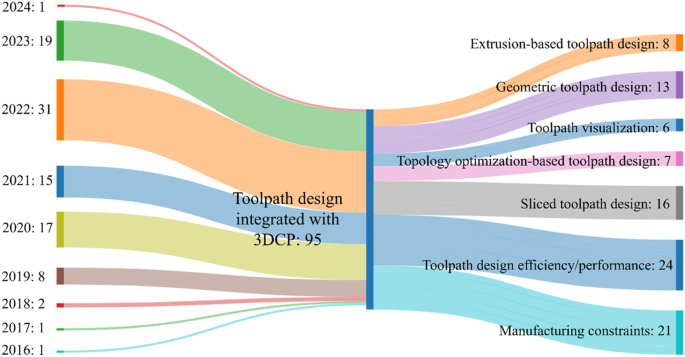
The literature study includes research on different toolpath design approaches from 2016 to 2024.
In recent years, there have been key research developments 14 , 15 , 96 , 119 in toolpath design and optimization. One of the key areas of focus has been on optimizing toolpaths for material efficiency and print time reduction. Researchers have explored various toolpath design methods with the instruction of topology optimization to achieve efficient and environmentally friendly structures. In addition, advancements in path optimization algorithms 110 , 111 , 112 , layer sequencing 113 , 114 , and support structure generation 86 , 117 , 118 have helped to enhance the printing efficiency and accuracy of 3DCP. Two novel printing techniques, “knitting” and “tilting” filaments, were proposed to address the anisotropy inherent in 3D printed ECC, emulating the natural crossed-lamellar structure of conch shells 120 . Three-dimensional spatial paths were devised to distribute tensile and flexural resistance in multiple directions and establish an interwoven interface system to enhance the strength of the structure.
The integration of toolpath design, 3D concrete printing, and topology optimization
Toolpath planning includes the strategic arrangement of the printing toolhead’s movement paths and deposition patterns to achieve the desired structural form 121 , 122 , 123 , 124 . This section aims to highlight the significance of toolpath planning in 3DCP and topology optimization. Existing methods for toolpath design in 3DCP involve a combination of computational algorithms, simulation techniques, and empirical knowledge. These methods consider various constraints and challenges, including printer limitations 14 , geometric complexity 16 , surface finish requirements 125 , overhang (self-support) problem 86 , interlocking 126 , and stability 127 during the printing process. They aim to generate toolpaths that maximize printing efficiency while ensuring the structural integrity and quality of the final product.
The toolpath design methods displayed in Fig. 5 can be integrated with 3DCP to fabricate efficient and high-performance structures depending on the fabrication requirements. Extrusion-based toolpath design in 3D concrete printing refers to the process of planning and creating the specific paths along which the extrusion nozzle will move to deposit layers of concrete material in a three-dimensional printed structure. Extrusion-based toolpath design 128 , 129 offers several advantages. It allows for the generation of toolpaths tailored to the specific material deposition process, resulting in efficient and optimized printing trajectories. By considering the extrusion process, this method can minimize print time, reduce material waste, and enhance printing efficiency. However, it may be limited in its ability to handle complex geometries and struggle with intricate support structure generation. Geometric toolpath design 16 , 130 focuses on creating toolpaths based on the geometric characteristics of the part being printed. This approach can lead to precise toolpaths that align with the part’s geometry, potentially reducing material waste. However, it may be less effective in optimizing toolpaths for overall printing efficiency and may struggle with handling complex layer sequencing. Toolpath visualization 131 , 132 provides a visual representation of the toolpaths, aiding in the identification of potential issues such as collisions, inefficient trajectories, or inadequate support structures. While it can help in identifying and addressing these issues, it may not actively optimize the toolpaths for print time, material waste, or printing efficiency. This method allows for precise control over layer sequencing and material flow control, ensuring stable and accurate printing. However, it may require additional computational resources and not fully optimize toolpaths for overall printing efficiency.
Toolpath design can be integrated with topology optimization to generate better performance 103 , 133 . Topology optimization-based toolpath design integrates the principles of topology optimization into the generation of toolpaths. By considering material deposition constraints and printing process dynamics, this method aims to create toolpaths that are not only geometrically optimized but also aligned with manufacturing constraints and support structure requirements. This approach can lead to highly efficient toolpaths that minimize print time, material waste, and enhance overall printing efficiency.
In summary, each of these toolpath design methods offers unique advantages and considerations. The selection of the most suitable method depends on the specific printing requirements, material characteristics, geometric complexity, and manufacturing constraints of the part being printed.
Benefits and challenges for future applications
The impact of toolpath optimization on the quality and efficiency of 3DCP has garnered significant attention. This section aims to analyze how optimized toolpaths positively influence printing quality and efficiency, emphasizing the reduction of waste and energy consumption. Advanced algorithms and computational models 119 , 127 , 134 are being developed to strategically plan the movement paths and deposition patterns of the printing toolhead, enabling precise material placement and optimized structural performance. A well-planned toolpath can result in structurally sound and aesthetically pleasing printed structures, while inadequate planning can lead to issues like material sagging, poor bonding between layers, or excessive material use 15 , 135 , 136 . Therefore, understanding and optimizing the toolpath planning process is vital for successful and reliable 3DCP 137 , 138 . Furthermore, toolpath planning also provides opportunities for customization and innovation in construction 16 , 125 . With the ability to precisely control the deposition pattern and material properties, designers can explore novel architectural forms, integrate functional features, and optimize performance characteristics.
Through systematic toolpath planning, it becomes possible to mitigate issues such as over-extrusion, uneven material distribution, and inaccuracies in layer deposition, ultimately leading to superior printing quality 112 , 139 . Moreover, the relationship between toolpath planning and material efficiency is paramount in the context of sustainable manufacturing practices. Optimized toolpaths contribute to the reduction of material waste and energy consumption by streamlining the printing process. Efficient toolpaths enable precise material deposition, minimize unnecessary movements, and optimize the use of support structures, thereby reducing material consumption and enhancing overall sustainability in 3DCP 132 , 140 , 141 .
The technical considerations involved in toolpath optimization for 3DCP encompass path optimization algorithms, print speed adjustments, and support structure generation. Path optimization algorithms aim to minimize print time and reduce material waste by optimizing the toolhead’s movement trajectory, while print speed adjustments ensure consistent material flow and deposition 132 . In addition, support structure generation and layer sequencing contribute to the stability and efficiency of the printing process 86 . Real-world case studies provide valuable insights into the benefits and challenges associated with toolpath optimization in construction projects 142 , 143 .
In terms of future research directions, there is a requirement to address additional constraints for the practical usage of 3DCP. For instance, the development of artificial intelligence empowered toolpath design methods for structures with complex geometric features. The integration of real-time monitoring and feedback systems into the toolpath design process can help improve accuracy and adaptability during printing. In addition, considering sustainability aspects, such as the use of recycled materials or minimizing waste, presents another avenue for future research in toolpath design for 3DCP.
Conclusions
This study presents a comprehensive overview of three vital aspects integrated with 3D concrete printing (3DCP) that contribute to enhancing sustainability in the construction sector. The first area of focus is sustainable material, which involves optimizing the constituents of printable materials through the recycling of waste materials into aggregates and supplementary cementitious materials. This approach reduces the environmental impact of the materials but also enhances the economic viability of 3DCP. The second vital area discussed is structural optimization, which plays a crucial role in maximizing structural performance and efficiency by rearranging material distribution. This optimization leads to improved structural integrity, reduced material usage, and minimized construction time and cost. Lastly, advances in toolpath planning have significantly improved the quality and efficiency of 3DCP. By strategically planning the movement paths and deposition patterns of the printing toolhead, toolpath optimization enhances printing accuracy, minimizes defects, and improves overall structural integrity. Furthermore, the review article also explores the influence of printing parameters on the quality and integrity of printed structures, providing valuable insights for future research and development in the field. By investigating the synergies between these three elements, this research aims to provide valuable insights for advancing sustainable and efficient building practices through the implementation of 3DCP technology.
The future of 3DCP in the construction sector is promising, while more systematic works are required to facilitate the practical application and sustainability of 3DCP:
Integration of Advanced Technologies: Future research should focus on integrating advanced technologies such as artificial intelligence and robotic control into toolpath optimization. These technologies can be adopted in the material design, system integration, and real-time optimization of printing processes.
Development of New Algorithms: There is a need for the development of new algorithms for toolpath optimization that can address specific challenges in 3DCP, such as handling complex geometries, optimizing material flow, and managing overhangs. These algorithms should also aim to optimize multiple objectives simultaneously.
Exploration of Novel Applications: Future research should explore novel applications of toolpath optimization in construction, such as printing complex architectural forms, integrating functional features, and creating customized structures. The potential of toolpath optimization in challenging environments, such as underwater or in space, should also be investigated.
Systematic literature review
This review article employs a systematic literature review approach based on established practices in additive manufacturing for construction to explore the intersections between 3DCP, material sustainability, structural topology optimization, and toolpath design. The Web of Science Core Collection, including indices such as SCI, SSCI, SCI-Expanded, and ESCI, is utilized to gather diverse publications until December 2023, encompassing journal articles, conference proceedings, books, and reports. A three-stage review method is meticulously designed to ensure objectivity and reproducibility.
Initially, relevant keywords, including “3D concrete printing,” “sustainable material,” “structural topology optimization,” and “toolpath design,” are defined to ensure a focused review. The literature reviews for sustainable material, TO, and toolpath design sections are conducted independently by different researchers. In the first stage, 1033 papers related to 3DCP are identified, with further breakdowns of 400 papers for sustainable material, 472 for structural topology optimization, and 161 for toolpath design. In the second stage, manual screening is conducted based on predefined criteria, including methodology robustness, published year, bibliographic information, and sustainability considerations. Comparative analysis results in the identification of 476 papers, comprising 245 for sustainable material, 136 for structural topology optimization, and 95 for toolpath design, as displayed in Figs. 3 , 4 , and 5 . In the third stage, the literature was further narrowed down to 160 references for inclusion in this review according to the specific criteria, including published journals, impact in the field, and number of citations. This three-step screening procedure guarantees that the literature review remains focused and relevant.
An analytical synthesis is then performed to summarize the primary studies of additive manufacturing in construction. The 160 studies obtained by the screening procedure are integrated systematically and classified into three sections according to their context, study design, and outcomes. The references cited in the sections on sustainable material, structural topology optimization, and toolpath design are 61, 76, and 23, respectively. In conclusion, the systematic literature review methodology minimizes reliance on subjective judgments, mitigates personal biases and errors, and upholds the integrity of scholarly research 144 .
Data availability
No datasets were generated or analyzed during the current study.
I.E. Agency. CO 2 Emissions in 2022 . https://www.iea.org/reports/co2-emissions-in-2022 (2023).
Enerdata. World Energy & Climate Statistics—Yearbook 2022 . World Energy Consumption Statistics| Enerdata France. https://yearbook.enerdata.net/total-energy/world-consumption-statistics.html (2022).
C.I. Council. Sustainable Construction . https://www.sc.cic.hk/index.php/en/ (2023).
Khan, S. A., Koç, M. & Al-Ghamdi, S. G. Sustainability assessment, potentials and challenges of 3D printed concrete structures: a systematic review for built environmental applications. J. Clean. Prod. 303 , 127027 (2021).
Article Google Scholar
Tay, Y. W. D. et al. 3D printing trends in building and construction industry: a review. Virtual Phys. Prototyp. 12 , 261–276 (2017).
Weng, Y. et al. Comparative economic, environmental and productivity assessment of a concrete bathroom unit fabricated through 3D printing and a precast approach. J. Clean. Prod. 261 , 121245 (2020).
Ngo, T. D. et al. Additive manufacturing (3D printing): a review of materials, methods, applications and challenges. Compos. Part B: Eng. 143 , 172–196 (2018).
Article CAS Google Scholar
Wangler, T. et al. Digital concrete: a review. Cem. Concr. Res. 123 , 105780 (2019).
Lu, B. et al. A systematical review of 3D printable cementitious materials. Constr. Build. Mater. 207 , 477–490 (2019).
Xiao, J. et al. Large-scale 3D printing concrete technology: current status and future opportunities. Cem. Concr. Compos. 122 , 104115 (2021).
Dey, D. et al. Use of industrial waste materials for 3D printing of sustainable concrete: a review. J. Clean. Prod. 340 , 130749 (2022).
Teng, F. et al. BIM-enabled collaborative-robots 3D concrete printing to construct MiC with reinforcement. HKIE Trans. 30 , 106–115 (2023).
Vantyghem, G., De Corte, W., Shakour, E. & Amir, O. 3D printing of a post-tensioned concrete girder designed by topology optimization. Autom. Constr. 112 , 103084 (2020).
Bi, M. et al. Topology optimization for 3D concrete printing with various manufacturing constraints. Addit. Manuf. 57 , 102982 (2022).
Google Scholar
Weng, Y. et al. Extracting BIM information for lattice toolpath planning in digital concrete printing with developed dynamo script: a case study. J. Comput. Civ. Eng. 35 , 05021001 (2021).
Breseghello, L. & Naboni, R. Toolpath-based design for 3D concrete printing of carbon-efficient architectural structures. Addit. Manuf. 56 , 102872 (2022).
CAS Google Scholar
Adaloudis, M. & Roca, J. B. Sustainability tradeoffs in the adoption of 3D Concrete Printing in the construction industry. J. Clean. Prod. 307 , 127201 (2021).
Tinoco, M. P. et al. Life cycle assessment (LCA) and environmental sustainability of cementitious materials for 3D concrete printing: a systematic literature review. J. Build. Eng. 52 , 104456 (2022).
Chen, Y. et al. A review of printing strategies, sustainable cementitious materials and characterization methods in the context of extrusion-based 3D concrete printing. J. Build. Eng. 45 , 103599 (2022).
Lu, B., Li, M., Wong, T. N. & Qian, S. Effect of printing parameters on material distribution in spray-based 3D concrete printing (S-3DCP). Autom. Constr. 124 , 103570 (2021).
Ford, S. & Despeisse, M. Additive manufacturing and sustainability: an exploratory study of the advantages and challenges. J. Clean. Prod. 137 , 1573–1587 (2016).
Liu, J. et al. 3D-printed concrete with recycled glass: effect of glass gradation on flexural strength and microstructure. Constr. Build. Mater. 314 , 125561 (2022).
Wu, Y. et al. Study on the rheology and buildability of 3D printed concrete with recycled coarse aggregates. J. Build. Eng. 42 , 103030 (2021).
Oosthuizen, J. D., Babafemi, A. J. & Walls, R. S. 3D-printed recycled plastic eco-aggregate (Resin8) concrete. Constr. Build. Mater. 408 , 133712 (2023).
Valente, M., Sambucci, M., Chougan, M. & Ghaffar, S. H. Composite alkali-activated materials with waste tire rubber designed for additive manufacturing: an eco-sustainable and energy saving approach. J. Mater. Res. Technol. 24 , 3098–3117 (2023).
Liu, C. et al. Influence of hydroxypropyl methylcellulose and silica fume on stability, rheological properties, and printability of 3D printing foam concrete. Cem. Concr. Compos. 122 , 104158 (2021).
Tinoco, M. Pimentel et al. The use of rice husk particles to adjust the rheological properties of 3D printable cementitious composites through water sorption. Constr. Build. Mater. 365 , 130046 (2023).
Ye, J. et al. Development of 3D printable engineered cementitious composites with incineration bottom ash (IBA) for sustainable and digital construction. J. Clean. Prod. 422 , 138639 (2023).
Ibrahim, K. A., van Zijl, G. P. A. G. & Babafemi, A. J. Influence of limestone calcined clay cement on properties of 3D printed concrete for sustainable construction. J. Build. Eng. 69 , 106186 (2023).
Chen, Y. et al. 3D printing of calcined clay-limestone-based cementitious materials. Cem. Concr. Res. 149 , 106553 (2021).
Qian, H. et al. Synergistic effect of EVA copolymer and sodium desulfurization ash on the printing performance of high volume blast furnace slag mixtures. Addit. Manuf. 46 , 102183 (2021).
Bhattacherjee, S. et al. Sustainable materials for 3D concrete printing. Cem. Concr. Compos. 122 , 104156 (2021).
Liu, J., Setunge, S. & Tran, P. 3D concrete printing with cement-coated recycled crumb rubber: compressive and microstructural properties. Constr. Build. Mater. 347 , 128507 (2022).
Ding, T., Xiao, J., Zou, S. & Wang, Y. Hardened properties of layered 3D printed concrete with recycled sand. Cem. Concr. Compos. 113 , 103724 (2020).
Liu, H. et al. Hardened properties of 3D printed concrete with recycled coarse aggregate. Cem. Concr. Res. 159 , 106868 (2022).
H.K.E.P. Department. Hong Kong Solid Waste Monitoring Report Waste Statistics 2022 (2022).
H.K.E.P. Department. Waste Blueprint for Hong Kong 2035 (2021).
Ahmed, G. H., Askandar, N. H. & Jumaa, G. B. A review of largescale 3DCP: material characteristics, mix design, printing process, and reinforcement strategies. Structures 43 , 508–532 (2022).
Christen, H., van Zijl, G. & de Villiers, W. The incorporation of recycled brick aggregate in 3D printed concrete. Clean. Mater. 4 , 100090 (2022).
Sambucci, M., Biblioteca, I. & Valente, M. Life Cycle Assessment (LCA) of 3D concrete printing and casting processes for cementitious materials incorporating ground waste tire rubber. Recycling 8 , 15 (2023).
Skibicki, S. et al. The effect of using recycled PET aggregates on mechanical and durability properties of 3D printed mortar. Constr. Build. Mater. 335 , 127443 (2022).
Weng, Y., Li, M., Tan, M. J. & Qian, S. Design 3D printing cementitious materials via Fuller Thompson theory and Marson-Percy model. Constr. Build. Mater. 163 , 600–610 (2018).
Nerella, V. N. et al. Inline quantification of extrudability of cementitious materials for digital construction. Cem. Concr. Compos. 95 , 260–270 (2019).
Wu, Y. et al. 3D printed concrete with recycled sand: pore structures and triaxial compression properties. Cem. Concr. Compos. 139 , 105048 (2023).
Ting, G. H. A., Tay, Y. W. D., Qian, Y. & Tan, M. J. Utilization of recycled glass for 3D concrete printing: rheological and mechanical properties. J. Mater. Cycles Waste Manag. 21 , 994–1003 (2019).
Zou, M. et al. Evaluation and control of printability and rheological properties of 3D-printed rubberized concrete. J. Build. Eng. 80 , 107988 (2023).
Ding, T., Xiao, J. & Tam, V. W. Y. A closed-loop life cycle assessment of recycled aggregate concrete utilization in China. Waste Manag. 56 , 367–375 (2016).
Article PubMed Google Scholar
Han, Y., Yang, Z., Ding, T. & Xiao, J. Environmental and economic assessment on 3D printed buildings with recycled concrete. J. Clean. Prod. 278 , 123884 (2021).
Shen, W. et al. Quantifying CO 2 emissions from China’s cement industry. Renew. Sustain. Energy Rev. 50 , 1004–1012 (2015).
Weng, Y. et al. Feasibility study on sustainable magnesium potassium phosphate cement paste for 3D printing. Constr. Build. Mater. 221 , 595–603 (2019).
Gardner, L. J. et al. Characterisation of magnesium potassium phosphate cements blended with fly ash and ground granulated blast furnace slag. Cem. Concr. Res. 74 , 78–87 (2015).
Tangstad, M. in Handbook of Ferroalloys (ed. Gasik, M.) 179–220 (Butterworth-Heinemann, 2013).
Lucen, H. et al. The synergistic effect of greenhouse gas CO 2 and silica fume on the properties of 3D printed mortar. Compos. Part B: Eng. 271 , 111188 (2024).
Chen, Y. et al. Limestone and calcined clay-based sustainable cementitious materials for 3D concrete printing: a fundamental study of extrudability and early-age strength development. Appl. Sci. 9 , 1809 (2019).
Weng, Y. et al. Empirical models to predict rheological properties of fiber reinforced cementitious composites for 3D printing. Constr. Build. Mater. 189 , 676–685 (2018).
Zhao, Y. et al. Development of low-carbon materials from GGBS and clay brick powder for 3D concrete printing. Constr. Build. Mater. 383 , 131232 (2023).
Xu, Z. et al. Effect of FA and GGBFS on compressive strength, rheology, and printing properties of cement-based 3D printing material. Constr. Build. Mater. 339 , 127685 (2022).
Rahul, A. V., Santhanam, M., Meena, H. & Ghani, Z. 3D printable concrete: Mixture design and test methods. Cem. Concr. Compos. 97 , 13–23 (2019).
Long, W.-J. et al. Printability and particle packing of 3D-printable limestone calcined clay cement composites. Constr. Build. Mater. 282 , 122647 (2021).
Yao, Y., Hu, M., Di Maio, F. & Cucurachi, S. Life cycle assessment of 3D printing geo‐polymer concrete: an ex‐ante study. J. Ind. Ecol. 24 , 116–127 (2020).
Liu, S. et al. A comparative study on environmental performance of 3D printing and conventional casting of concrete products with industrial wastes. Chemosphere 298 , 134310 (2022).
Article CAS PubMed Google Scholar
Gao, W. et al. The status, challenges, and future of additive manufacturing in engineering. Comput. Aided Des. 69 , 65–89 (2015).
Michell, A. G. M. The limits of economy of material in frame structures. Philos. Mag. 8 , 589–597 (1904).
Bendsoe, M. P. & Kikuchi, N. Generating optimal topologies in structural design using a homogenization method. Comput. Methods Appl. Mech. Eng. 71 , 197–224 (1988).
Lurie, K. A., Cherkaev, A. V. & Fedorov, A. V. Regularization of optimal design problems for bars and plates, part 1. J. Optim. Theory Appl. 37 , 499–522 (1982).
Suzuki, K. & Kikuchi, N. A homogenization method for shape and topology optimization. Comput. Methods Appl. Mech. Eng. 93 , 291–318 (1991).
Xie, Y. M. & Steven, G. P. A simple evolutionary procedure for structural optimization. Comput. Struct. 49 , 885–896 (1993).
Querin, O. M., Steven, G. P. & Xie, Y. M. Evolutionary structural optimisation (ESO) using a bidirectional algorithm. Eng. Comput. 15 , 1031–1048 (1998).
Zhuang, Z., Xie, Y. M., Li, Q. & Zhou, S. Body-fitted bi-directional evolutionary structural optimization using nonlinear diffusion regularization. Comput. Methods Appl. Mech. Eng. 396 , 115114 (2022).
Sethian, J. A. & Wiegmann, A. Structural boundary design via level set and immersed interface methods. J. Comput. Phys. 163 , 489–528 (2000).
Allaire, G., Jouve, F. & Toader, A.-M. A level set method for shape optimization. C. R. Math. 334 , 1125–1130 (2002).
Bendsoe, M. P. Optimal shape design as a material distribution problem. Struct. Optim. 1 , 193–202 (1989).
Sigmund, O. Materials with prescribed constitutive parameters: an inverse homogenization problem. Int. J. Solids Struct. 31 , 2313–2329 (1994).
Sigmund, O. On the design of compliant mechanisms using topology optimization. Mech. Struct. Mach. 25 , 493–524 (1997).
Sigmund, O. A 99 line topology optimization code written in Matlab. Struct. Multidiscip. Optim. 21 , 120–127 (2001).
Xie, Y. M. & Steven, G. P. Evolutionary Structural Optimization (Springer-Verlag, 1997).
Xie, Y. M. & Steven, G. P. Evolutionary structural optimization for dynamic problems. Comput. Struct. 58 , 1067–1073 (1996).
Yang, X. Y., Xie, Y. M., Steven, G. & Querin, O. Bidirectional evolutionary method for stiffness optimization. AIAA J. 37 , 1483–1488 (1999).
Osher, S. & Sethian, J. A. Fronts propagating with curvature-dependent speed: Algorithms based on Hamilton-Jacobi formulations. J. Comput. Phys. 79 , 12–49 (1988).
Vese, L. A. & Chan, T. F. A multiphase level set framework for image segmentation using the mumford and Shah model. Int. J. Comput. Vis. 50 , 271–293 (2002).
Wang, M. Y., Wang, X. & Guo, D. A level set method for structural topology optimization. Comput. Methods Appl. Mech. Eng. 192 , 227–246 (2003).
Wang, M. Y. & Wang, X. “Color” level sets: a multi-phase method for structural topology optimization with multiple materials. Comput. Methods Appl. Mech. Eng. 193 , 469–496 (2004).
Otomori, M., Yamada, T., Izui, K. & Nishiwaki, S. Matlab code for a level set-based topology optimization method using a reaction diffusion equation. Struct. Multidiscip. Optim. 51 , 1159–1172 (2014).
Huang, X. On smooth or 0/1 designs of the fixed-mesh element-based topology optimization. Adv. Eng. Softw. 151 , 102942 (2021).
Zhuang, Z. et al. A node moving-based structural topology optimization method in the body-fitted mesh. Comput. Methods Appl. Mech. Eng. 419 , 116663 (2024).
Bi, M., Tran, P. & Xie, Y. M. Topology optimization of 3D continuum structures under geometric self-supporting constraint. Addit. Manuf. 36 , 101422 (2020).
Xie, Y. M. Generalized topology optimization for architectural design. Architect. Intell. 1 , 1–11 (2022).
Liu, Y. et al. Reducing the number of different faces in free-form surface approximations through clustering and optimization. Comput. Aided Des. 166 , 103633 (2024).
Zhao, Z.-L., Zhou, S., Feng, X.-Q. & Xie, Y. M. Morphological optimization of scorpion telson. J. Mech. Phys. Solids 135 , 103773 (2020).
Cai, K., Chen, B. S. & Zhang, H. W. Topology optimization of continuum structures based on a new bionics method. Int. J. Computat. Methods Eng. Sci. Mech. 8 , 233–242 (2007).
Zhu, J., Zhang, W. & Xia, L. Topology optimization in aircraft and aerospace structures design. Arch. Comput. Methods Eng. 23 , 595–622 (2016).
Leader, M. K., Chin, T. W. & Kennedy, G. High resolution topology optimization of aerospace structures with stress and frequency constraints, In Proc. 2018 Multidisciplinary Analysis and Optimization Conference, American Institute of Aeronautics and Astronautics (2018).
Menna, C. et al. Opportunities and challenges for structural engineering of digitally fabricated concrete. Cem. Concr. Res. 133 , 106079 (2020).
Chen, Y., Zhou, C. & Lao, J. A layerless additive manufacturing process based on CNC accumulation. Rapid Prototyp. J. 17 , 218–227 (2011).
Pan, Y., Zhou, C., Chen, Y. & Partanen, J. Multi-tool and multi-axis CNC Accumulation for fabricating conformal features on curved surfaces. J. Manuf. Sci. Eng. 136 , 031007 (2014).
Ooms, T. et al. Third RILEM International Conference on Concrete and Digital Fabrication 37–42 (Springer International Publishing, 2022).
Asprone, D., Auricchio, F., Menna, C. & Mercuri, V. 3D printing of reinforced concrete elements: Technology and design approach. Constr. Build. Mater. 165 , 218–231 (2018).
Gebhard, L. et al. Structural behaviour of 3D printed concrete beams with various reinforcement strategies. Eng. Struct. 240 , 112380 (2021).
Martens, P., Mathot, M., Bos, F. & Coenders, J. High Tech Concrete: Where Technology and Engineering Meet 301–309 (Springer International Publishing, 2018).
Ahmed, G. H. A review of “3D concrete printing”: materials and process characterization, economic considerations and environmental sustainability. J. Build. Eng. 66 , 105863 (2023).
Tay, Y. W. D., Lim, J. H., Li, M. & Tan, M. J. Creating functionally graded concrete materials with varying 3D printing parameters. Virtual Phys. Prototyp. 17 , 662–681 (2022).
Li, Y. et al. FloatArch: a cable-supported, unreinforced, and re-assemblable 3D-printed concrete structure designed using multi-material topology optimization. Addit. Manuf. 81 , 104012 (2024).
Yang, W., Wang, L., Ma, G. & Feng, P. An integrated method of topological optimization and path design for 3D concrete printing. Eng. Struct. 291 , 116435 (2023).
Mechtcherine, V. et al. Extrusion-based additive manufacturing with cement-based materials—production steps, processes, and their underlying physics: A review. Cem. Concr. Res. 132 , 106037 (2020).
Tu, H. et al. Recent advancements and future trends in 3D concrete printing using waste materials. Dev. Built Environ. 16 , 100187 (2023).
Heywood, K. & Nicholas, P. Sustainability and 3D concrete printing: identifying a need for a more holistic approach to assessing environmental impacts. Architect. Intell. 2 , 12 (2023).
Wang, L., Jiang, H., Li, Z. & Ma, G. Mechanical behaviors of 3D printed lightweight concrete structure with hollow section. Arch. Civ. Mech. Eng. 20 , 16 (2020).
Geng, Z., Pan, H., Zuo, W. & She, W. Functionally graded lightweight cement-based composites with outstanding mechanical performances via additive manufacturing. Addit. Manuf. 56 , 102911 (2022).
Xia, L. et al. Integrated lightweight design method via structural optimization and path planning for material extrusion. Addit. Manuf. 62 , 103387 (2023).
Jin, Y.-a et al. Optimization of tool-path generation for material extrusion-based additive manufacturing technology. Addit. Manuf. 1-4 , 32–47 (2014).
Jin, Y. et al. An optimization approach for path planning of high-quality and uniform additive manufacturing. Int. J. Adv. Manuf. Technol. 92 , 651–662 (2017).
Ding, D., Pan, Z., Cuiuri, D. & Li, H. A practical path planning methodology for wire and arc additive manufacturing of thin-walled structures. Robot. Comput. Integr. Manuf. 34 , 8–19 (2015).
Sales, E., Kwok, T.-H. & Chen, Y. Function-aware slicing using principal stress line for toolpath planning in additive manufacturing. J. Manuf. Process. 64 , 1420–1433 (2021).
Chakraborty, D., Reddy, B. & Choudhury, A. Extruder path generation for Curved Layer Fused Deposition Modeling. Comput. Aided Des. 40 , 235–243 (2008).
Jiang, J. & Ma, Y. Path planning strategies to optimize accuracy, quality, build time and material use in additive manufacturing: a review. Micromachines 11 , 633 (2020).
Article PubMed PubMed Central Google Scholar
Giberti, H., Sbaglia, L. & Urgo, M. A path planning algorithm for industrial processes under velocity constraints with an application to additive manufacturing. J. Manuf. Syst. 43 , 160–167 (2017).
Jin, Y. et al. A non-retraction path planning approach for extrusion-based additive manufacturing. Robot. Comput. Integr. Manuf. 48 , 132–144 (2017).
Wang, T. et al. Load-dependent path planning method for 3D printing of continuous fiber reinforced plastics. Compos. Part A: Appl. Sci. Manuf. 140 , 106181 (2021).
Chen, X., Fang, G., Liao, W.-H. & Wang, C. C. L. Field-based toolpath generation for 3D printing continuous fibre reinforced thermoplastic composites. Addit. Manuf. 49 , 102470 (2022).
Zhou, W., McGee, W., Gökçe, H. S. & Li, V. C. A bio-inspired solution to alleviate anisotropy of 3D printed engineered cementitious composites (3DP-ECC): Knitting/tilting filaments. Autom. Constr. 155 , 105051 (2023).
Anton, A. et al. A 3D concrete printing prefabrication platform for bespoke columns. Autom. Constr. 122 , 103467 (2021).
Dörrie, R. et al. Automated force-flow-oriented reinforcement integration for Shotcrete 3D Printing. Autom. Constr. 155 , 105075 (2023).
Breseghello, L., Hajikarimian, H., Jørgensen, H. B. & Naboni, R. 3DLightBeam+. Design, simulation, and testing of carbon-efficient reinforced 3D concrete printed beams. Eng. Struct. 292 , 116511 (2023).
Moini, M. et al. Additive manufacturing and performance of architectured cement-based materials. Adv. Mater. 30 , 1802123 (2018).
Lin, Z. et al. Tool path generation for multi-axis freeform surface finishing with the LKH TSP solver. Comput. Aided Des. 69 , 51–61 (2015).
Zareiyan, B. & Khoshnevis, B. Effects of interlocking on interlayer adhesion and strength of structures in 3D printing of concrete. Autom. Constr. 83 , 212–221 (2017).
Bi, M. et al. Continuous contour-zigzag hybrid toolpath for large format additive manufacturing. Addit. Manuf. 55 , 102822 (2022).
Vispute, M., Kumar, N., Taufik, M. & Jain, P. K. Improving surface finish of extrusion based additive manufactured parts using novel triangle based toolpath approach. Int. J. Interact. Des. Manuf. 18 , 433–452 (2024).
Jensen, M. L. et al. Toolpath strategies for 5DOF and 6DOF extrusion-based additive manufacturing, Appl. Sci. 9 , 4168 (2019).
Li, C. L. A geometric approach to boundary-conformed toolpath generation. Comput. Aided Des. 39 , 941–952 (2007).
Breseghello, L. & Naboni, R. Adaptive toolpath: enhanced design and process control for robotic 3DCP. In International Conference on Computer-Aided Architectural Design Futures 301–316 (2022).
Liu, W., Chen, L., Mai, G. & Song, L. Toolpath planning for additive manufacturing using sliced model decomposition and metaheuristic algorithms. Adv. Eng. Softw. 149 , 102906 (2020).
Yang, W. et al. An integrated topology optimization method including manufacturing constraints for 3D printed fiber-reinforced concrete structures. Mater. Lett. 355 , 135442 (2024).
Lin, S. et al. A maze-like path generation scheme for fused deposition modeling. Int. J. Adv. Manuf. Technol. 104 , 1509–1519 (2019).
Jin, G. Q., Li, W. D., Gao, L. & Popplewell, K. A hybrid and adaptive tool-path generation approach of rapid prototyping and manufacturing for biomedical models. Comput. Ind. 64 , 336–349 (2013).
Moini, R. Perspectives in architected infrastructure materials. RILEM Tech. Lett. 8 , 125–140 (2024).
Xia, L., Lin, S. & Ma, G. Stress-based tool-path planning methodology for fused filament fabrication. Addit. Manuf. 32 , 101020 (2020).
Xia, L. et al. Globally continuous hybrid path for extrusion-based additive manufacturing. Autom. Constr. 137 , 104175 (2022).
Li, N. et al. Path-designed 3D printing for topological optimized continuous carbon fibre reinforced composite structures. Compos. Part B: Eng. 182 , 107612 (2020).
Liu, J., Ma, Y., Qureshi, A. J. & Ahmad, D. R. Light-weight shape and topology optimization with hybrid deposition path planning for FDM parts. Int. J. Adv. Manuf. Technol. 97 , 1123–1135 (2018).
Ye, J. et al. Feasibility of using ultra-high ductile concrete to print self-reinforced hollow structures. in Proc. Third RILEM International Conference on Concrete and Digital Fabrication 133–138 (2022).
Jin, G. Q., Li, W. D., Tsai, C. F. & Wang, L. Adaptive tool-path generation of rapid prototyping for complex product models. J. Manuf. Syst. 30 , 154–164 (2011).
Jin, Y.-A., He, Y., Xue, G.-H. & Fu, J.-Z. A parallel-based path generation method for fused deposition modeling. Int. J. Adv. Manuf. Technol. 77 , 927–937 (2015).
Roberts, H. & Petticrew, M. Systematic Reviews in the Social Sciences: A Practical Guide (Wiley, 2006).
Glowinski, R. Trends and Applications of Pure Mathematics to Mechanics 96–145 (Springer Berlin Heidelberg, 1984).
Kikuchi, N., Chung, K. Y., Torigaki, T. & Taylor, J. E. Computer Methods in Applied Mechanics and Engineering 139–169 (1986).
Andreassen, E. et al. Efficient topology optimization in MATLAB using 88 lines of code. Struct. Multidiscip. Optim. 43 , 1–16 (2011).
Ferrari, F. & Sigmund, O. A new generation 99 line Matlab code for compliance topology optimization and its extension to 3D. Struct. Multidiscip. Optim. 62 , 2211–2228 (2020).
Rozvany, G. I. N., Zhou, M. & Birker, T. Generalized shape optimization without homogenization. Struct. Optim. 4 , 250–252 (1992).
Huang, X. & Xie, Y. M. Evolutionary Topology Optimization of Continuum Structures: Methods and Applications (Wiley, 2010).
Zuo, Z. H. & Xie, Y. M. A simple and compact Python code for complex 3D topology optimization. Adv. Eng. Softw. 85 , 1–11 (2015).
Huang, X., Xie, Y. M. & Burry, M. C. A new algorithm for bi-directional evolutionary structural optimization. JSME Int. J. Ser. C. Mech. Syst., Mach. Elem. Manuf. 49 , 1091–1099 (2006).
Challis, V. J. A discrete level-set topology optimization code written in Matlab. Struct. Multidiscip. Optim. 41 , 453–464 (2010).
Allaire, G., Jouve, F. & Toader, A.-M. Structural optimization using sensitivity analysis and a level-set method. J. Comput. Phys. 194 , 363–393 (2004).
Zhuang, Z., Xie, Y. M., Li, Q. & Zhou, S. A 172-line Matlab code for structural topology optimization in the body-fitted mesh. Struct. Multidiscip. Optim. 66 , 11 (2022).
Yamada, T., Izui, K., Nishiwaki, S. & Takezawa, A. A topology optimization method based on the level set method incorporating a fictitious interface energy. Comput. Methods Appl. Mech. Eng. 199 , 2876–2891 (2010).
Li, H. et al. Full-scale 3D structural topology optimization using adaptive mesh refinement based on the level-set method. Finite Elem. Anal. Des. 194 , 103561 (2021).
Zhuang, Z., Xie, Y. M. & Zhou, S. A reaction diffusion-based level set method using body-fitted mesh for structural topology optimization. Comput. Methods Appl. Mech. Eng. 381 , 113829 (2021).
Huang, X. A Matlab code of topology optimization by imposing the implicit floating projection constraint. (2022).
Huang, X. & Li, W. Three-field floating projection topology optimization of continuum structures. Comput. Methods Appl. Mech. Eng. 399 , 115444 (2022).
Zhang, X. et al. A nodal-based optimization method for the design of continuous fiber-reinforced structures. Compos. Struct. 323 , 117455 (2023).
Download references
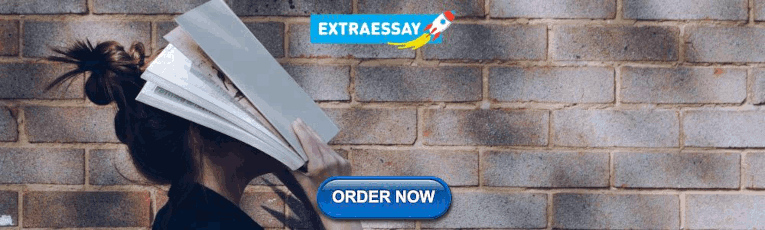
Acknowledgements
This project was funded by National Science and Foundation of China (52308284), Department of Science and Technology of Guangdong Province (306071352047), and Hong Kong Polytechnic University (P0038598, P0038966, P0044299, P0045796). The funder played no role in study design, data collection, analysis and interpretation of data, or the writing of this manuscript.
Author information
These authors contributed equally: Zicheng Zhuang, Fengming Xu, Junhong Ye.
Authors and Affiliations
Department of Building and Real Estate, The Hong Kong Polytechnic University, Hong Kong, China
Zicheng Zhuang, Fengming Xu, Junhong Ye & Yiwei Weng
Department of Civil Engineering and Transportation, South China University of Technology, Hong Kong, China
Department of Building Environment and Energy Engineering, The Hong Kong Polytechnic University, Hong Kong, China
Liming Jiang
You can also search for this author in PubMed Google Scholar
Contributions
Z.Z., M.X., and J.Y. conducted the review and wrote the manuscript. H.N., L.J., and Y.W. made suggestions and revised the manuscript. Y.W. provided the resources and supervision. All authors read and approved the final manuscript.
Corresponding author
Correspondence to Yiwei Weng .
Ethics declarations
Competing interests.
The authors declare no competing interests.
Additional information
Publisher’s note Springer Nature remains neutral with regard to jurisdictional claims in published maps and institutional affiliations.
Rights and permissions
Open Access This article is licensed under a Creative Commons Attribution 4.0 International License, which permits use, sharing, adaptation, distribution and reproduction in any medium or format, as long as you give appropriate credit to the original author(s) and the source, provide a link to the Creative Commons licence, and indicate if changes were made. The images or other third party material in this article are included in the article’s Creative Commons licence, unless indicated otherwise in a credit line to the material. If material is not included in the article’s Creative Commons licence and your intended use is not permitted by statutory regulation or exceeds the permitted use, you will need to obtain permission directly from the copyright holder. To view a copy of this licence, visit http://creativecommons.org/licenses/by/4.0/ .
Reprints and permissions
About this article
Cite this article.
Zhuang, Z., Xu, F., Ye, J. et al. A comprehensive review of sustainable materials and toolpath optimization in 3D concrete printing. npj Mater. Sustain. 2 , 12 (2024). https://doi.org/10.1038/s44296-024-00017-9
Download citation
Received : 30 December 2023
Accepted : 02 March 2024
Published : 03 June 2024
DOI : https://doi.org/10.1038/s44296-024-00017-9
Share this article
Anyone you share the following link with will be able to read this content:
Sorry, a shareable link is not currently available for this article.
Provided by the Springer Nature SharedIt content-sharing initiative
Quick links
- Explore articles by subject
- Guide to authors
- Editorial policies
Sign up for the Nature Briefing: Anthropocene newsletter — what matters in anthropocene research, free to your inbox weekly.

Review: enhancing Additive Digital Manufacturing with supervised classification machine learning algorithms
- Critical Review
- Published: 30 May 2024
Cite this article
- Phan Nguyen Huu 1 ,
- Dong Pham Van 1 ,
- Thinh Hoang Xuan 1 ,
- Mohsen Asghari Ilani 2 ,
- Ly Nguyen Trong 1 ,
- Hai Ha Thanh ORCID: orcid.org/0000-0001-8698-4941 1 &
- Tam Nguyen Chi 1
42 Accesses
Explore all metrics
In the field of in-process monitoring for the production of safety-critical parts, the utilization of Machine Learning (ML) models has demonstrated promising potential for enhancing the manufacturing process. Specifically, Supervised Classification Algorithms, selected based on the complexity of the problem, can significantly improve the performance of ML models. While ML primarily relies on training data to comprehend input-output relationships, its ability to draw rapid conclusions is noteworthy. Deep neural networks have emerged as valuable tools, offering more accurate analytical data compared to Finite Element Method (FEM) models. They present a cost-effective alternative to empirical data by incorporating error compensation in a closed loop. Nevertheless, vision-based techniques, such as those used in this study, necessitate high-quality input images and demand substantial computational power for image classification. To address these challenges, this study proposes the use of a shallow Convolutional Neural Network (CNN)-based architecture for detecting in-process defects in parts manufactured using Additive Manufacturing (AM) technology. Complementing this, Support Vector Machine (SVM) and Extreme Learning Machine (ELM) algorithms are integrated with the CNN architecture. Additionally, the research takes into account the high costs associated with data collection and installation, data unavailability, challenges in data labeling, as well as common difficulties like overfitting and underfitting of ML models. These factors often pose constraints on the application of ML solutions within the context of AM. The study aims to address these issues and shed light on the potential of ML for AM applications. By employing a combination of CNN, SVM, and ELM algorithms, this research delves into the effectiveness of ML models in defect detection during the AM process. The insights derived from this study contribute to mitigating the aforementioned limitations and pave the way for a broader adoption of ML solutions in AM. This optimization aims to enhance performance, reduce costs, and improve the overall quality of manufactured parts.
This is a preview of subscription content, log in via an institution to check access.
Access this article
Price includes VAT (Russian Federation)
Instant access to the full article PDF.
Rent this article via DeepDyve
Institutional subscriptions
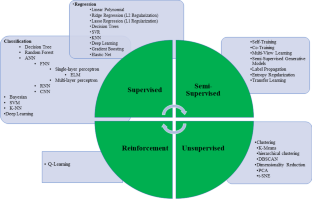
Reprinted with permission [ 24 ]. ( b ) The process involves transitioning from thermal images (input) to porosity predictions (output) for Ti-6Al4V in the L-PBF process. From these thermal images, various geometric features are extracted to train machine learning (ML) models. Subsequently, these trained models possess the capability to classify whether the printed product is porous (considered abnormal) or not (considered normal) [ 13 ]. ( c ) Outline of CNN architecture [ 25 ]
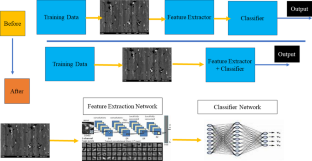
Similar content being viewed by others
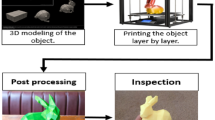
Object and defect detection in additive manufacturing using deep learning algorithms
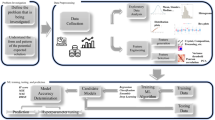
Incorporation of machine learning in additive manufacturing: a review
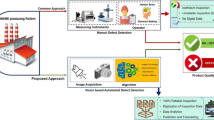
Automated surface defect detection framework using machine vision and convolutional neural networks
Data availability.
There is no need to mention the availability of data and materials in the present study.
Stansbury JW, Idacavage MJ (2016) 3D printing with polymers: challenges among expanding options and opportunities. Dent Mater 32:54–64. https://doi.org/10.1016/J.DENTAL.2015.09.018
Article Google Scholar
Ilani MA, Khoshnevisan M (2022) An evaluation of the surface integrity and corrosion behavior of Ti-6Al-4 V processed thermodynamically by PM-EDM criteria. Int J Adv Manuf Technol 120:5117–5129. https://doi.org/10.1007/S00170-022-09093-4/METRICS
Ilani MA, Khoshnevisan M (2020) Powder mixed-electrical discharge machining (EDM) with the electrode is made by fused deposition modeling (FDM) at Ti-6Al-4V machining procedure. Multiscale Multidisciplinary Model Experiments Des 3:173–186. https://doi.org/10.1007/S41939-020-00070-6/METRICS
Filippou V, Tsoumpas C (2018) Recent advances on the development of phantoms using 3D printing for imaging with CT, MRI, PET, SPECT, and ultrasound. Med Phys 45:e740–e760. https://doi.org/10.1002/MP.13058
Chemali E, Kollmeyer PJ, Preindl M et al (2018) Long short-term memory networks for Accurate State-of-charge estimation of Li-ion batteries. IEEE Trans Industr Electron 65:6730–6739. https://doi.org/10.1109/TIE.2017.2787586
Chua ZY, Ahn IH, Moon SK (2017) Process monitoring and inspection systems in metal additive manufacturing: Status and applications. Int J Precision Eng Manufacturing-Green Technol 2017 4(2):235–245. https://doi.org/10.1007/S40684-017-0029-7
Everton SK, Hirsch M, Stavroulakis PI et al (2016) Review of in-situ process monitoring and in-situ metrology for metal additive manufacturing. Mater Des 95:431–445. https://doi.org/10.1016/J.MATDES.2016.01.099
Berman B (2012) 3-D printing: the new industrial revolution. Bus Horiz 55:155–162. https://doi.org/10.1016/J.BUSHOR.2011.11.003
Yan R, Gao RX, Chen X (2014) Wavelets for fault diagnosis of rotary machines: a review with applications. Sig Process 96:1–15. https://doi.org/10.1016/J.SIGPRO.2013.04.015
Ford S, Despeisse M (2016) Additive manufacturing and sustainability: an exploratory study of the advantages and challenges. J Clean Prod 137:1573–1587. https://doi.org/10.1016/J.JCLEPRO.2016.04.150
Lin YC, Hung MH, Huang HC et al (2017) Development of Advanced Manufacturing Cloud of things (AMCoT)-A Smart Manufacturing platform. IEEE Robot Autom Lett 2:1809–1816. https://doi.org/10.1109/LRA.2017.2706859
Wang KS, Li Z, Braaten J, Yu Q (2015) Interpretation and compensation of backlash error data in machine centers for intelligent predictive maintenance using ANNs. Advances in Manufacturing 2015 3:2 3:97–104. https://doi.org/10.1007/S40436-015-0107-4
Khanzadeh M, Chowdhury S, Marufuzzaman M et al (2018) Porosity prediction: supervised-learning of thermal history for direct laser deposition. J Manuf Syst 47:69–82. https://doi.org/10.1016/J.JMSY.2018.04.001
Rojek I, Mikołajewski D, Kotlarz P et al (2021) Traditional artificial neural networks versus deep learning in optimization of material aspects of 3d printing. Materials 14. https://doi.org/10.3390/MA14247625/S1
Scime L, Beuth J (2019) Using machine learning to identify in-situ melt pool signatures indicative of flaw formation in a laser powder bed fusion additive manufacturing process. Addit Manuf 25:151–165. https://doi.org/10.1016/J.ADDMA.2018.11.010
Zhang W, Li X, Jia XD et al (2020) Machinery fault diagnosis with imbalanced data using deep generative adversarial networks. Measurement 152:107377. https://doi.org/10.1016/J.MEASUREMENT.2019.107377
Li X, Zhang W, Ding Q, Sun JQ (2018) Intelligent rotating machinery fault diagnosis based on deep learning using data augmentation. J Intell Manuf 2018 31:2. https://doi.org/10.1007/S10845-018-1456-1
Li X, Zhang W, Ding Q, Sun JQ (2019) Multi-layer domain adaptation method for rolling bearing fault diagnosis. Sig Process 157:180–197. https://doi.org/10.1016/J.SIGPRO.2018.12.005
Shevchik SA, Kenel C, Leinenbach C, Wasmer K (2018) Acoustic emission for in situ quality monitoring in additive manufacturing using spectral convolutional neural networks. Addit Manuf 21:598–604. https://doi.org/10.1016/J.ADDMA.2017.11.012
Tootooni MS, Dsouza A, Donovan R et al (2017) Classifying the Dimensional Variation in Additive Manufactured Parts from laser-scanned three-Dimensional Point Cloud Data using machine learning approaches. J Manuf Sci Eng Trans ASME 139. https://doi.org/10.1115/1.4036641
Zhang Y, Hong GS, Ye D et al (2018) Extraction and evaluation of melt pool, plume and spatter information for powder-bed fusion AM process monitoring. Mater Des (1980–2015) 156:458–469. https://doi.org/10.1016/J.MATDES.2018.07.002
Liu C, Le Roux L, Ji Z et al (2020) Machine learning-enabled feedback loops for metal powder bed fusion additive manufacturing. Procedia Comput Sci 176:2586–2595. https://doi.org/10.1016/J.PROCS.2020.09.314
Gobert C, Reutzel EW, Petrich J et al (2018) Application of supervised machine learning for defect detection during metallic powder bed fusion additive manufacturing using high resolution imaging. Addit Manuf 21:517–528. https://doi.org/10.1016/J.ADDMA.2018.04.005
Scime L, Beuth J (2018) Anomaly detection and classification in a laser powder bed additive manufacturing process using a trained computer vision algorithm. Addit Manuf 19:114–126. https://doi.org/10.1016/J.ADDMA.2017.11.009
Kim B, Yuvaraj N, Sri Preethaa KR, Arun Pandian R (2021) Surface crack detection using deep learning with shallow CNN architecture for enhanced computation. Neural Computing and Applications 2021 33:15 33:9289–9305. https://doi.org/10.1007/S00521-021-05690-8
Zhang B, Jaiswal P, Rai R et al (2019) Convolutional neural network-based inspection of metal additive manufacturing parts. Rapid Prototyp J 25:530–540. https://doi.org/10.1108/RPJ-04-2018-0096
Han T, Liu C, Yang W, Jiang D (2019) Learning transferable features in deep convolutional neural networks for diagnosing unseen machine conditions. ISA Trans 93:341–353. https://doi.org/10.1016/J.ISATRA.2019.03.017
Tajbakhsh N, Shin JY, Gurudu SR et al (2016) Convolutional Neural Networks for Medical Image Analysis: full training or fine tuning? IEEE Trans Med Imaging 35:1299–1312. https://doi.org/10.1109/TMI.2016.2535302
Thenmozhi K, Srinivasulu Reddy U (2019) Crop pest classification based on deep convolutional neural network and transfer learning. Comput Electron Agric 164:104906. https://doi.org/10.1016/J.COMPAG.2019.104906
Qi X, Chen G, Li Y et al (2019) Applying neural-network-based machine learning to Additive Manufacturing: current applications, challenges, and future perspectives. Engineering 5:721–729. https://doi.org/10.1016/J.ENG.2019.04.012
Chouiekh A, el Haj EHI (2018) ConvNets for Fraud Detection analysis. Procedia Comput Sci 127:133–138. https://doi.org/10.1016/J.PROCS.2018.01.107
Pan SJ, Yang Q (2010) A survey on transfer learning. IEEE Trans Knowl Data Eng 22:1345–1359. https://doi.org/10.1109/TKDE.2009.191
Shen Z, Shang X, Zhao M et al (2019) A learning-based Framework for Error Compensation in 3D Printing. IEEE Trans Cybern 49:4042–4050. https://doi.org/10.1109/TCYB.2019.2898553
Kim P (2017) Convolutional neural network. MATLAB Deep Learn 121–147. https://doi.org/10.1007/978-1-4842-2845-6_6
Valizadeh M, Wolff SJ (2022) Convolutional Neural Network applications in additive manufacturing: a review. Adv Industrial Manuf Eng 4:100072. https://doi.org/10.1016/J.AIME.2022.100072
Lecun Y, Bengio Y, Hinton G (2015) Deep learning. Nature 2015 521:7553 521:436–444. https://doi.org/10.1038/nature14539
Zhu H, Ge W, Liu Z (2019) Deep Learning-Based Classification of Weld Surface Defects. Applied Sciences 2019, Vol 9, Page 3312 9:3312. https://doi.org/10.3390/APP9163312
Salmi M, Paloheimo KS, Tuomi J et al (2013) Accuracy of medical models made by additive manufacturing (rapid manufacturing). J Cranio-Maxillofacial Surg 41:603–609. https://doi.org/10.1016/J.JCMS.2012.11.041
ImageNet Classification with Deep Convolutional Neural Networks https://proceedings.neurips.cc/paper/2012/hash/c399862d3b9d6b76c8436e924a68c45b-Abstract.html . Accessed 27 Apr 2022
van Eijnatten M, van Dijk R, Dobbe J et al (2018) CT image segmentation methods for bone used in medical additive manufacturing. Med Eng Phys 51:6–16. https://doi.org/10.1016/J.MEDENGPHY.2017.10.008
van Eijnatten M, Koivisto J, Karhu K et al (2017) The impact of manual threshold selection in medical additive manufacturing. Int J Comput Assist Radiol Surg 12:607–615. https://doi.org/10.1007/S11548-016-1490-4/FIGURES/7
Shi J, Votruba AR, Farokhzad OC, Langer R (2010) Nanotechnology in drug delivery and tissue engineering: from discovery to applications. Nano Lett 10:3223–3230. https://doi.org/10.1021/NL102184C/ASSET .
Gauvin R, Khademhosseini A (2011) Microscale technologies and modular approaches for tissue engineering: moving toward the fabrication of complex functional structures. ACS Nano 5:4258–4264. https://doi.org/10.1021/NN201826D/ASSET/IMAGES/MEDIUM/NN-2011-01826D_0003.GIF .
Ghaderzadeh M, Asadi F, Jafari R et al (2021) Deep Convolutional Neural Network–Based Computer-Aided Detection System for COVID-19 Using Multiple Lung Scans: Design and Implementation Study. J Med Internet Res 2021;23(4):e27468 https://www.jmir.org/2021/4/e27468 . https://doi.org/10.2196/27468
Bassi PRAS, Attux R (2022) A deep convolutional neural network for COVID-19 detection using chest X-rays. Res Biomedical Eng 38:139–148. https://doi.org/10.1007/S42600-021-00132-9/FIGURES/4
Serj MF, Lavi B, Hoff G, Valls DP (2018) A deep convolutional neural network for Lung Cancer Diagnostic. https://doi.org/10.48550/arxiv.1804.08170
Yang C, Kim Y, Ryu S, Gu GX (2020) Prediction of composite microstructure stress-strain curves using convolutional neural networks. Mater Des 189:108509. https://doi.org/10.1016/J.MATDES.2020.108509
Li Z, Zhang Z, Shi J, Wu D (2019) Prediction of surface roughness in extrusion-based additive manufacturing with machine learning. Robot Comput Integr Manuf 57:488–495. https://doi.org/10.1016/J.RCIM.2019.01.004
Ahn D, Kweon JH, Kwon S et al (2009) Representation of surface roughness in fused deposition modeling. J Mater Process Technol 209:5593–5600. https://doi.org/10.1016/J.JMATPROTEC.2009.05.016
Romano S, Brückner-Foit A, Brandão A et al (2018) Fatigue properties of AlSi10Mg obtained by additive manufacturing: defect-based modelling and prediction of fatigue strength. Eng Fract Mech 187:165–189. https://doi.org/10.1016/J.ENGFRACMECH.2017.11.002
Gordon Jv, Narra SP, Cunningham RW et al (2020) Defect structure process maps for laser powder bed fusion additive manufacturing. Addit Manuf 36:101552. https://doi.org/10.1016/J.ADDMA.2020.101552
Wang J, Wu WJ, Jing W et al (2019) Improvement of densification and microstructure of ASTM A131 EH36 steel samples additively manufactured via selective laser melting with varying laser scanning speed and hatch spacing. Mater Sci Engineering: A 746:300–313. https://doi.org/10.1016/J.MSEA.2019.01.019
Wits WW, Carmignato S, Zanini F, Vaneker THJ (2016) Porosity testing methods for the quality assessment of selective laser melted parts. CIRP Ann 65:201–204. https://doi.org/10.1016/J.CIRP.2016.04.054
Mireles J, Ridwan S, Morton PA et al (2015) Analysis and correction of defects within parts fabricated using powder bed fusion technology. Surf Topogr 3:034002. https://doi.org/10.1088/2051-672X/3/3/034002
Li X, Jia X, Yang Q, Lee J (2020) Quality analysis in metal additive manufacturing with deep learning. J Intell Manuf 2020 31:8. https://doi.org/10.1007/S10845-020-01549-2
Zhang B, Liu S, Shin YC (2019) In-Process monitoring of porosity during laser additive manufacturing process. Addit Manuf 28:497–505. https://doi.org/10.1016/J.ADDMA.2019.05.030
Kwon O, Kim HG, Ham MJ et al (2018) A deep neural network for classification of melt-pool images in metal additive manufacturing. J Intell Manuf 2018 31:2. https://doi.org/10.1007/S10845-018-1451-6
Yuan B, Giera B, Guss G et al (2019) Semi-supervised convolutional neural networks for in-situ video monitoring of selective laser melting. Proceedings – 2019 IEEE Winter Conference on Applications of Computer Vision, WACV 2019 744–753. https://doi.org/10.1109/WACV.2019.00084
Scime L, Beuth J (2018) A multi-scale convolutional neural network for autonomous anomaly detection and classification in a laser powder bed fusion additive manufacturing process. Addit Manuf 24:273–286. https://doi.org/10.1016/J.ADDMA.2018.09.034
Ansari MA, Crampton A, Garrard R et al (2022) A Convolutional Neural Network (CNN) classification to identify the presence of pores in powder bed fusion images. Int J Adv Manuf Technol 1–18. https://doi.org/10.1007/S00170-022-08995-7/FIGURES/16
Chen Y, Zheng WX (2012) Stochastic state estimation for neural networks with distributed delays and Markovian jump. Neural Netw 25:14–20. https://doi.org/10.1016/J.NEUNET.2011.08.002
Xu XZ, Ding SF, Shi ZZ, Zhu H (2012) Optimizing radial basis function neural network based on rough sets and affinity propagation clustering algorithm. J Zhejiang Univ Sci C 13:131–138. https://doi.org/10.1631/JZUS.C1100176
Sebastiani F (2002) Machine learning in automated text categorization. ACM Comput Surv (CSUR) 34:1–47. https://doi.org/10.1145/505282.505283
bin Huang G, Zhu QY, Siew CK (2004) Extreme learning machine: A new learning scheme of feedforward neural networks. IEEE International Conference on Neural Networks - Conference Proceedings 2:985–990. https://doi.org/10.1109/IJCNN.2004.1380068
IEEE Xplore - Page not Found https://ieeexplore.ieee.org/document/1650244/ . Accessed 27 Apr 2022
Ding S, Su C, Yu J (2011) An optimizing BP neural network algorithm based on genetic algorithm. Artif Intell Rev 36:153–162. https://doi.org/10.1007/S10462-011-9208-Z
Fernández-Navarro F, Hervás-Martínez C, Gutiérrez PA, Carbonero-Ruz M (2011) Evolutionary q-Gaussian radial basis function neural networks for multiclassification. Neural Netw 24:779–784. https://doi.org/10.1016/J.NEUNET.2011.03.014
Razavi S, Tolson BA (2011) A new formulation for feedforward neural networks. IEEE Trans Neural Netw 22:1588–1598. https://doi.org/10.1109/TNN.2011.2163169
Xu X, Ding S, Jia W et al (2011) Research of assembling optimized classification algorithm by neural network based on Ordinary Least Squares (OLS). Neural Comput Appl 22:187–193. https://doi.org/10.1007/S00521-011-0694-3
Huang G, Zhu QY, Siew CK (2006) Extreme learning machine: Theory and applications. Neurocomputing 70:489–501
di Angelo L, di Stefano P, Marzola A (2017) Surface quality prediction in FDM additive manufacturing. Int J Adv Manuf Technol 2017 93(9):3655–3662. https://doi.org/10.1007/S00170-017-0763-6
Galantucci LM, Bodi I, Kacani J, Lavecchia F (2015) Analysis of Dimensional Performance for a 3D open-source printer based on fused deposition modeling technique. Procedia CIRP 28:82–87. https://doi.org/10.1016/J.PROCIR.2015.04.014
Zhang S, Sun Z, Long J et al (2019) Dynamic condition monitoring for 3D printers by using error fusion of multiple sparse auto-encoders. Comput Ind 105:164–176. https://doi.org/10.1016/J.COMPIND.2018.12.004
Gao W, Wai RJ, Qiao SP, Guo MF (2019) Mechanical Faults Diagnosis of High-Voltage Circuit Breaker via Hybrid Features and Integrated Extreme Learning Machine. IEEE Access 7:60091–60103. https://doi.org/10.1109/ACCESS.2019.2915252
Tong Q, Cao J, Han B et al (2017) A fault diagnosis approach for rolling element bearings based on dual-tree complex wavelet packet transform-improved intrinsic time-scale decomposition, singular value decomposition, and. https://doi.org/10.1177/1687814017737721 . online sequential extreme learning machine: https://doi.org/101177/1687814017737721 9:
Chen YQ, Fink O, Sansavini G (2018) Combined Fault Location and Classification for Power Transmission Lines Fault Diagnosis with Integrated feature extraction. IEEE Trans Industr Electron 65:561–569. https://doi.org/10.1109/TIE.2017.2721922
Li K, Xiong M, Li F et al (2019) A novel fault diagnosis algorithm for rotating machinery based on a sparsity and neighborhood preserving deep extreme learning machine. Neurocomputing 350:261–270. https://doi.org/10.1016/J.NEUCOM.2019.03.084
Rodriguez N, Lagos C, Cabrera E, Cañete L (2017) Extreme learning machine based on stationary wavelet singular values for bearing failure diagnosis. Stud Inf Control 26:287–249. https://doi.org/10.24846/V26I3Y201704
Guo J, Li X, Liu Z et al (2021) A novel doublet extreme learning machines for Delta 3D printer fault diagnosis using attitude sensor. ISA Trans 109:327–339. https://doi.org/10.1016/J.ISATRA.2020.10.024
Wang S, Huang W, Zhu ZK (2011) Transient modeling and parameter identification based on wavelet and correlation filtering for rotating machine fault diagnosis. Mech Syst Signal Process 25:1299–1320. https://doi.org/10.1016/J.YMSSP.2010.10.013
Luo M, Li C, Zhang X et al (2016) Compound feature selection and parameter optimization of ELM for fault diagnosis of rolling element bearings. ISA Trans 65:556–566. https://doi.org/10.1016/J.ISATRA.2016.08.022
Cui L, Wang X, Wang H, Ma J (2020) Research on remaining useful life prediction of rolling element Bearings based on Time-Varying Kalman Filter. IEEE Trans Instrum Meas 69:2858–2867. https://doi.org/10.1109/TIM.2019.2924509
Lei Y, Lin J, He Z, Zuo MJ (2013) A review on empirical mode decomposition in fault diagnosis of rotating machinery. Mech Syst Signal Process 35:108–126. https://doi.org/10.1016/J.YMSSP.2012.09.015
Shen C, He Q, Kong F, Tse PW (2012) A fast and adaptive varying-scale morphological analysis method for rolling element bearing fault diagnosis 227:1362–1370. https://doi.org/10.1177/0954406212460628
Antoni J (2009) Cyclostationarity by examples. Mech Syst Signal Process 23:987–1036. https://doi.org/10.1016/J.YMSSP.2008.10.010
Cui L, Huang J, Zhang F, Chu F (2019) HVSRMS localization formula and localization law: localization diagnosis of a ball bearing outer ring fault. Mech Syst Signal Process 120:608–629. https://doi.org/10.1016/J.YMSSP.2018.09.043
Lu S, Wang X, He Q et al (2016) Fault diagnosis of motor bearing with speed fluctuation via angular resampling of transient sound signals. J Sound Vib 385:16–32. https://doi.org/10.1016/J.JSV.2016.09.012
Zhang W, Li X, Ding Q (2019) Deep residual learning-based fault diagnosis method for rotating machinery. ISA Trans 95:295–305. https://doi.org/10.1016/J.ISATRA.2018.12.025
Li Y, Cheng G, Liu C, Chen X (2018) Study on planetary gear fault diagnosis based on variational mode decomposition and deep neural networks. Measurement 130:94–104. https://doi.org/10.1016/J.MEASUREMENT.2018.08.002
Zheng J, Jiang Z, Pan H (2018) Sigmoid-based refined composite multiscale fuzzy entropy and t-SNE based fault diagnosis approach for rolling bearing. Measurement 129:332–342. https://doi.org/10.1016/J.MEASUREMENT.2018.07.045
Muralidharan V, Sugumaran V (2012) A comparative study of Naïve Bayes classifier and Bayes net classifier for fault diagnosis of monoblock centrifugal pump using wavelet analysis. Appl Soft Comput 12:2023–2029. https://doi.org/10.1016/J.ASOC.2012.03.021
Panda AK, Rapur JS, Tiwari R (2018) Prediction of flow blockages and impending cavitation in centrifugal pumps using support Vector Machine (SVM) algorithms based on vibration measurements. Measurement 130:44–56. https://doi.org/10.1016/J.MEASUREMENT.2018.07.092
Li C, Tao Y, Ao W et al (2018) Improving forecasting accuracy of daily enterprise electricity consumption using a random forest based on ensemble empirical mode decomposition. Energy 165:1220–1227. https://doi.org/10.1016/J.ENERGY.2018.10.113
Wu C, Jiang P, Ding C et al (2019) Intelligent fault diagnosis of rotating machinery based on one-dimensional convolutional neural network. Comput Ind 108:53–61. https://doi.org/10.1016/J.COMPIND.2018.12.001
Zhang S, Sun Z, Wang M et al (2020) Deep fuzzy Echo State Networks for Machinery Fault diagnosis. IEEE Trans Fuzzy Syst 28:1205–1218. https://doi.org/10.1109/TFUZZ.2019.2914617
Isham MF, Leong MS, Lim MH, bin Ahmad ZA (2019) Intelligent wind turbine gearbox diagnosis using VMDEA and ELM. Wind Energy 22:813–833. https://doi.org/10.1002/WE.2323
Li C, Cerrada M, Cabrera D et al (2018) A comparison of fuzzy clustering algorithms for bearing fault diagnosis. J Intell Fuzzy Syst 34:3565–3580. https://doi.org/10.3233/JIFS-169534
Noble WS (2006) What is a support vector machine? Nat Biotechnol 24:1565–1567. https://doi.org/10.1038/nbt1206-1565
Schölkopf B, Mika S, Burges CJC et al (1999) Input space versus feature space in kernel-based methods. IEEE Trans Neural Netw 10:1000–1017. https://doi.org/10.1109/72.788641
Weckenmann A, Jiang X, Sommer KD et al (2009) Multisensor data fusion in dimensional metrology. CIRP Ann 58:701–721. https://doi.org/10.1016/J.CIRP.2009.09.008
Aminzadeh M, Kurfess T (2015) Layerwise Automated Visual Inspection in Laser Powder. https://doi.org/10.1115/MSEC2015-9393 . -Bed Additive Manufacturing
Joshi MS, Flood A, Sparks T, Liou FW Applications Of Supervised Machine Learning Algorithms In Additive Manufacturing: A Review
Delli U, Chang S (2018) Automated process monitoring in 3D Printing using supervised machine learning. Procedia Manuf 26:865–870. https://doi.org/10.1016/J.PROMFG.2018.07.111
Download references
There is no funding details to be mentioned in the manuscript.
Author information
Authors and affiliations.
Hanoi University of Industry, No. 298 Cau Dien Street, Bac Tu Liem District, Hanoi, Vietnam
Phan Nguyen Huu, Dong Pham Van, Thinh Hoang Xuan, Ly Nguyen Trong, Hai Ha Thanh & Tam Nguyen Chi
School of Mechanical Engineering, College of Engineering, University of Tehran, Tehran, Iran
Mohsen Asghari Ilani
You can also search for this author in PubMed Google Scholar
Contributions
Phan Nguyen Huu- Design of manuscrip; Mohsen Asghari Ilani and Pham Van Dong - Writing a manuscript; Hoang Xuan Thinh, Nguyen Trong Ly, Nguyen Chi Tam and Ha Thanh Hai- Data analysis.
Corresponding author
Correspondence to Phan Nguyen Huu .
Ethics declarations
Ethical approval.
There is no ethical approval needed in the present study.
Consent to participate
There is no consent to participate needed in the present study.
Consent to Publish
There is no consent to publish needed in the present study.
Competing Interests
There is no competing interests to be mentioned in the present study.
Additional information
Publisher’s note.
Springer Nature remains neutral with regard to jurisdictional claims in published maps and institutional affiliations.
Rights and permissions
Springer Nature or its licensor (e.g. a society or other partner) holds exclusive rights to this article under a publishing agreement with the author(s) or other rightsholder(s); author self-archiving of the accepted manuscript version of this article is solely governed by the terms of such publishing agreement and applicable law.
Reprints and permissions
About this article
Huu, P.N., Van, D.P., Xuan, T.H. et al. Review: enhancing Additive Digital Manufacturing with supervised classification machine learning algorithms. Int J Adv Manuf Technol (2024). https://doi.org/10.1007/s00170-024-13781-8
Download citation
Received : 11 July 2023
Accepted : 13 May 2024
Published : 30 May 2024
DOI : https://doi.org/10.1007/s00170-024-13781-8
Share this article
Anyone you share the following link with will be able to read this content:
Sorry, a shareable link is not currently available for this article.
Provided by the Springer Nature SharedIt content-sharing initiative
- Additive Manufacturing
- Machin Learning (ML)
- Convolutional Neural Network (CNN)
- Extreme Learning Machine (ELM)
- Support Vector Machine (SVM)
- Find a journal
- Publish with us
- Track your research
Europe PMC requires Javascript to function effectively.
Either your web browser doesn't support Javascript or it is currently turned off. In the latter case, please turn on Javascript support in your web browser and reload this page.
Search life-sciences literature (44,160,791 articles, preprints and more)
- Available from publisher site using DOI. A subscription may be required. Full text
The Beating Heart of Untapped Business Opportunities for Additive Manufacturing
Preprint from Open Res Europe , 04 Jun 2024 https://doi.org/10.12688/openreseurope.16270.2 PPR: PPR861705
Preprint v2
Preprint version history
- Version 2 [04 Jun 2024]
- Version 1 [07 Sep 2023]
Abstract
Full text links .
Read article at publisher's site: https://doi.org/10.12688/openreseurope.16270.2
Funding
Funders who supported this work.
HORIZON EUROPE European Innovation Council (1)
Grant ID: 101083997
1 publication
Horizon Europe Framework Programme (1)
Grant ID: 870037
Europe PMC is part of the ELIXIR infrastructure
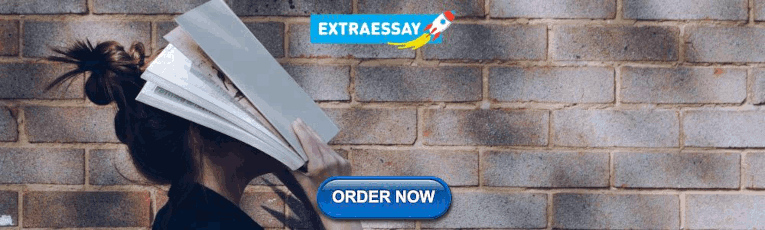
IMAGES
VIDEO
COMMENTS
The work of Schröder et al. (2 015) states the AM business models in the literature review, the development of a business model th at evaluates the process cost s of AM technologies and a ...
Cost models of additive manufacturing: A literature review ... Literature review method Before starting with the literature review, it is important to develop the method being applied in the literature review. The number of papers on AM to examine is very big; therefore, it is possible to
Cost models of additive manufacturing: A literature review. International Journal of Industrial Engineering Computations . 2017;8:263-282. Epub 2016 Sept 5. doi: 10.5267/j.ijiec.2016.9.001
A. A. Kadir Y. Yusof Md Saidin Wahab. Engineering, Materials Science. The International Journal of Advanced…. 2020. With the recent evolution of additive manufacturing (AM), accurate cost prediction models are of increasing importance to assist decision-making during product development tasks. Estimating the cost…. Expand. 3.
Costabile G, Fera M, Fruggiero F, Lambiase A, Pham D (2017) Cost models of additive manufacturing: a literature review. Int J Ind Eng Comput 8(2):263-283. Google Scholar Gisario A, Kazarian M, Martina F, Mehrpouya M (2019) Metal additive manufacturing in the commercial aviation industry: a review. J Manuf Syst 53:124-149
DOI: 10.5267/J.IJIEC.2016.9.001 Corpus ID: 113840130; Cost models of additive manufacturing: A literature review @article{Costabile2017CostMO, title={Cost models of additive manufacturing: A literature review}, author={Gianluca Costabile and Marcello Fera and Fabio Fruggiero and Alfredo Lambiase and Duc Truong Pham}, journal={International Journal of Industrial Engineering Computations}, year ...
4.3 Detailed review of AM cost estimation models utilisation. Additive manufacturing cost estimation models are also utilised for the implementation of architecture and software development studies. The models are related to a certain part of the discussed implementation.
Cost models of additive manufacturing: A literature review Fera M; 2017 Abstract From the past decades, increasing attention has been paid to the quality level of technological and mechanical properties achieved by the Additive Manufacturing (AM); these two elements have achieved a good performance, and it is possible to compare this with the results achieved by traditional technology.
This paper aims to analyse the existing literature about the cost models developed specifically for AM from an operations management point of view and discusses the strengths and weaknesses of all models. Additive manufacturing Additive manufacturing cost model. Additive manufacturing. cost model. From the past decades, increasing attention has ...
The foundation for any useful cost model is the definition of the model's scope. Several general AM processes involving ancillary AM process steps have been defined in the literature (Gibson et al., 2014; Lindemann et al., 2013; Weller et al., 2015).Amalgamating such approaches, Fig. 1 presents a general AM process map showing how the AM build process is situated in an array of pre-build and ...
Cost Estimation Models. Purpose To design for additive manufacturing (AM), the decision to use AM needs to be taken early in the product development process. Therefore, engineers need to be able to estimate AM part cost based on the few parameters available at this point in the process. This paper aims to develop suitable cost estimation models ...
Additive manufacturing cost estimation models—a classification review. Int. J. Adv. Manuf. Technol., 107 (9) (2020) ... Sustainability performance indicators for additive manufacturing: a literature review based on product life cycle studies. Int. J. Adv. Manuf. Technol., 107 (7) (2020) ...
additive manufacturing system. Among other things, this includes energy costs, machine purchase, and associated labor costs to operate the system. The base model has a build rate of 6.3 ccm/hr, a utilization of 4500 h/yr, a material cost of 89 €, and a machine investment cost of 500 000 €.
Additive Manufacturing (AM) is, undoubtedly, one of the most promising and potentially disruptive technologies of the Industry 4.0 era, able to transform the traditional manufacturing paradigm and fuel the generally accepted and necessary shift towards the conceptualisation, design and adoption of sustainable and circular business models. The objective of this paper is to contribute to the ...
Cost models of additive manufacturing: A literature review Pages 263-283 Download PDF. Abstract: From the past decades, increasing attention has been paid to the quality level of technological and mechanical properties achieved by the Additive Manufacturing (AM); these two elements have achieved a good performance, and it is possible to compare ...
The research trend in additive manufacturing (AM) has evolved over the past 30 years, from patents, advances in the design, and layer-by-layer materials, to technologies. However, this evolution is faced with some barriers, such as the implementation of additive manufacturing (AM) in operations, its productivity limitations, and economic and social sustainability. These barriers need to be ...
Costabile G, Fera M, Fruggiero F, Lambiase A, Pham D (2017) Cost models of additive manufacturing: a literature review. Int J Ind Eng Comput 8:263-283. ... Durieux S, Duc E (2020) Sustainability performance indicators for additive manufacturing: a literature review based on product life cycle studies. Int J Adv Manuf Technol 107:3109-3134 ...
A visualisation of future insights into the AM cost-oriented estimation framework from the perspective of various AM users can be better understood and different aspects of the cost estimation classification technique are compiled. With the recent evolution of additive manufacturing (AM), accurate cost prediction models are of increasing importance to assist decision-making during product ...
3. Materials3.1. Metals and alloys. Metal additive manufacturing is showing excellent perspectives of growth. The number of companies selling AM systems went from 49 in 2014 to 97 in 2016, amongst the 49% involved with metal AM [33].This technology has been used predominantly for research, prototyping or advanced applications in the aerospace industry, e.g. manufacturing the F-15 Pylon Rib by ...
Additive manufacturing offers several potentials such as the freedom of design, part consolidation, function integration, or time and cost-savings. These potentials make AM interesting for industries such as aerospace, automotive and medical implants, and are also seen as enables for the creation of entirely new business models.
Currently, however, additive manufactured products represent less than one percent of all manufactured products in the U.S. As the costs of additive manufacturing systems decrease, this technology may change the way that consumers interact with producers. Additive manufacturing technology opens up new opportunities for the economy and society.
This review article employs a systematic literature review approach based on established practices in additive manufacturing for construction to explore the intersections between 3DCP, material ...
This paper is a literature review on business models used in the additive manufacturing industry. ... U., Moi, M., & Koch, R. (2012). Analyzing Product Lifecycle Costs for a Better Understanding of Cost Drivers in Additive Manufacturing. In 23rd Annual International Solid Freeform Fabrication Symposium-An Additive Manufacturing Conference (pp ...
The present work analyses the fundamental cost factors in a real case study of a company dedicated to the supply of spare parts for fluid conduction systems to explore the potential economic impact of the use of AM technologies in the cost levels of manufacturing and stocking of spare part products.
In the field of in-process monitoring for the production of safety-critical parts, the utilization of Machine Learning (ML) models has demonstrated promising potential for enhancing the manufacturing process. Specifically, Supervised Classification Algorithms, selected based on the complexity of the problem, can significantly improve the performance of ML models. While ML primarily relies on ...
Over the past decade, significant research has focused on detecting abnormalities in metal laser powder bed fusion (L-PBF) additive manufacturing. Effective online monitoring systems are crucial for enhancing process stability, repeatability, and the quality of final components. Therefore, the development of in situ detection mechanisms has become essential for metal L-PBF systems, making ...
The model allowed the surgeons to first study and then experiment to determine the most effective operation procedure before the actual operation, which shortened the surgery time by approximately 70%. Reducing surgery time creates two forms of value: improving patient outcomes and reducing costs.
An ecosystem model for AM in the medical sector was developed that helps medical practitioners to better understand the challenges and opportunities associated with AM and AM manufacturers to better identify where to focus their R&D efforts and how this would impact future AM adoption levels. PurposeThis works provides a thorough understanding of the challenges and opportunities associated ...
A comprehensive review of Ti alloys/composites additive manufacturing. • α / β microstructural evolution and properties of AMed Ti alloys and composites.. Beta phase to alpha-/-or martensite (β → α / α ′) transformation in AMed Ti alloys.. Grain growth morphology manipulation by a thermal gradient, and melt pool and cooling rate control.