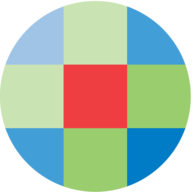
- Subscribe to journal Subscribe
- Get new issue alerts Get alerts
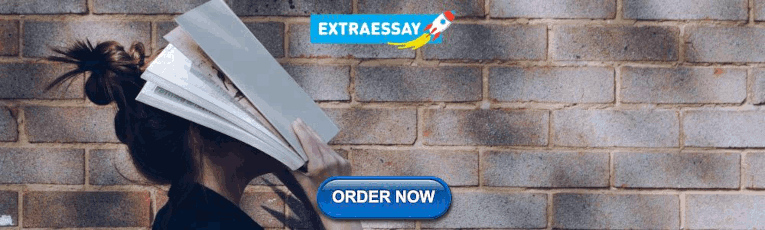
Secondary Logo
Journal logo.
Colleague's E-mail is Invalid
Your message has been successfully sent to your colleague.
Save my selection
A Methodology of Assessment of Air Pollution Health Impact Based on Structural Longitudinal Modeling of Hierarchical Systems and Fuzzy Algorithm: Application to Study of Children Respiratory Functions
Friger, M; Bolotin, A; Peleg, R
Ben-Gurion University Of The Negev, Beersheba.
Introduction:
Assessing the effects of air-pollution is a significant problem in the field of modern environmental epidemiology. When modeling these effects it is important that the models must be epidemiologically meaningful and robust (that is, insensitive to variations in the model parameters). The objective of this paper is to propose a methodology for the assessment of the health impact of air pollution. The proposed methodology involves the construction of models for complex dynamic hierarchical systems in environmental epidemiology and their problem-oriented interpretation.
The principal stages of the proposed methodology are:
- Creation of a multivariate hierarchical structural model based on system analysis.
- Generation of a mathematical formalization for this model.
- Development of a statistical model for a particular study case based on the mathematical formalization, using the generalized estimating equations technique and time-series analysis. At this stage, for a dichotomized dependent variable, a special fuzzy algorithm was used. The algorithm employed fuzzy membership functions instead of the binary variable to obtain robust regression models.
- Use of the “multi-layered” approach for model interpretation developed by the authors. This approach involved the creation of special functional time-dependent coefficients that reflect the effect of air pollutants at a given time. These coefficients allow an epidemiological meaningful model interpretation. Thus, they can be used for air-pollution health effects assessment.
The proposed methodology was used to analyze data collected from lung function measurements in 165 children from February-September 2002 (about 4000 individual daily records). The subject variables were age, gender, body-mass index, and place of residency. The meteorological variables included daily maximum temperature, average humidity and barometric pressure. The air-pollutant variables were suspended particles, ozone, nitrogen and sulphur oxides. In addition, the effects were studied up to a 3-day lag. The results demonstrated a statistically significant effect of air-pollution on lung function. Changes in most of the pollutants did not cause a critical decrease in lung function. However, for the observed period, a 10 mkg/m 3 increase in ozone was associated with a mean decrease in lung function of 6 units for a one-day delay.
Discussion and Conclusions:
The assessment of the health effects of air pollution and their interpretation make epidemiological sense, lending support to the correctness of the proposed methodology. Testing the models by changing the dichotomization cutoff for the lung function variability shows that the models based on the proposed fuzzy algorithm are robust.
- + Favorites
- View in Gallery
Advertisement
Traffic costs of air pollution: the effect of PM 2.5 on traffic violation
- Research Article
- Published: 25 May 2022
- Volume 29 , pages 72699–72717, ( 2022 )
Cite this article
- Tao Wang 1 , 2 ,
- Yu Wang ORCID: orcid.org/0000-0002-2781-2872 1 &
- Nan Cui 1
392 Accesses
Explore all metrics
Although emerging studies have investigated the effect of air pollution on traffic crashes, it is unclear to scholars whether air pollution affects another road safety problem—traffic violations. To address this gap, the current paper constructs a data set from 1,390,221 traffic violation records of 640,971 drivers from the Wuhan Traffic Management Bureau between January 2018 and December 2018. An ordered logistic regression was conducted to verify our hypotheses. The result shows that PM 2.5 has no overall impact on the severity of traffic violations, but each 1% increase in the daily concentration of PM 2.5 leads to a 1.02-fold increase in the odds of serious inexperience-related violations and a 0.99-fold decrease in the odds of serious overconfidence-related violations. This effect is the strongest in PM 2.5 , followed by NO 2 , and has not been observed in CO and O 3 . In addition, robustness tests indicate that the relationship between air pollution and traffic violations is consistent among the different subsets (e.g., clear weather, no rain and snow, and good visibility). We also provide valuable practical advice for drivers and traffic authorities.
This is a preview of subscription content, log in via an institution to check access.
Access this article
Subscribe and save.
- Get 10 units per month
- Download Article/Chapter or Ebook
- 1 Unit = 1 Article or 1 Chapter
- Cancel anytime
Price includes VAT (Russian Federation)
Instant access to the full article PDF.
Rent this article via DeepDyve
Institutional subscriptions
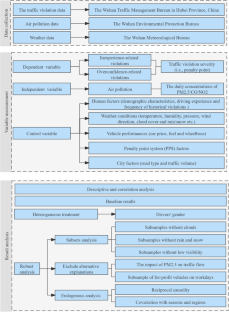
Similar content being viewed by others
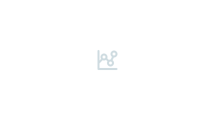
The impact of drivers’ short-term exposure to air pollution on traffic deaths
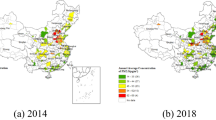
Does air pollution affect traffic safety? Evidence from cities in China
Impact of Traffic-Related Air Pollution on Health
Data availability.
The datasets used and/or analyzed during the current study are available from the corresponding author on reasonable request.
Ahmadi M, Khorsandi B, Mesbah M (2021) The effect of air pollution on drivers’ safety performance. Environ Sci Pollut Res 28:15768–15781. https://doi.org/10.1007/s11356-020-11687-y
Article CAS Google Scholar
Ali Q, Yaseen MR, Khan MTI (2020) The impact of temperature, rainfall, and health worker density index on road traffic fatalities in Pakistan. Environ Sci Pollut Res 27:19510–19529. https://doi.org/10.1007/s11356-020-08233-1
Article Google Scholar
Almetwally AA, Bin-Jumah M, Allam AA (2020) Ambient air pollution and its influence on human health and welfare: an overview. Environ Sci Pollut Res 27:24815–24830. https://doi.org/10.1007/s11356-020-09042-2
Ambo TB, Ma J, Fu C (2020) Investigating influence factors of traffic violation using multinomial logit method. Int J Inj Contr Saf Promot 28:78–85. https://doi.org/10.1080/17457300.2020.1843499
Atkinson RW, Kang S, Anderson HR et al (2014) Epidemiological time series studies of PM2.5 and daily mortality and hospital admissions: a systematic review and meta-analysis. Thorax 69:660–665. https://doi.org/10.1136/thoraxjnl-2013-204492
Atombo C, Wu C, Zhong M, Zhang H (2016) Investigating the motivational factors influencing drivers intentions to unsafe driving behaviours: speeding and overtaking violations. Transp Res Part F Traffic Psychol Behav 43:104–121. https://doi.org/10.1016/j.trf.2016.09.029
Barthwal V, Jain S, Babuta A et al (2022) Health impact assessment of Delhi’s outdoor workers exposed to air pollution and extreme weather events: an integrated epidemiology approach. Environ Sci Pollut Res. https://doi.org/10.1007/s11356-022-18886-9
Beelen R, Raaschou-Nielsen O, Stafoggia M et al (2014) Effects of long-term exposure to air pollution on natural-cause mortality: an analysis of 22 European cohorts within the multicentre ESCAPE project. Lancet 383:785–795. https://doi.org/10.1016/S0140-6736(13)62158-3
Berthelon C, Gineyt G (2014) Effects of alcohol on automated and controlled driving performances. Psychopharmacology 231:2087–2095. https://doi.org/10.1007/s00213-013-3352-x
Castanier C, Deroche T, Woodman T (2013) Theory of planned behaviour and road violations: the moderating influence of perceived behavioural control. Transp Res Part F Traffic Psychol Behav 18:148–158. https://doi.org/10.1016/j.trf.2012.12.014
Chapman EA, Masten SV, Browning KK (2014) Crash and traffic violation rates before and after licensure for novice California drivers subject to different driver licensing requirements. J Safety Res 50:125–138. https://doi.org/10.1016/j.jsr.2014.05.005
Chew SH, Huang W, Li X (2019) Does haze cloud decision making? A natural laboratory experiment. A Nat Lab Exp (July 20, 2019)
Claeson A-S, Lidén E, Nordin M, Nordin S (2013) The role of perceived pollution and health risk perception in annoyance and health symptoms: a population-based study of odorous air pollution. Int Arch Occup Environ Health 86:367–374
Coccaro EF, Sripada CS, Yanowitch RN, Phan KL (2011) Corticolimbic function in impulsive aggressive behavior. Biol Psychiatry 69:1153–1159
Das A, Ghasemzadeh A, Ahmed MM (2019) Analyzing the effect of fog weather conditions on driver lane-keeping performance using the SHRP2 naturalistic driving study data. J Safety Res 68:71–80. https://doi.org/10.1016/j.jsr.2018.12.015
Dockery DW, Pope CA (1994) Acute respiratory effects of particulate air pollution. Annu Rev Public Health 15:107–132
Dong H, Jia N, Tian J, Ma S (2019) The effectiveness and influencing factors of a penalty point system in China from the perspective of risky driving behaviors. Accid Anal Prev 131:171–179. https://doi.org/10.1016/j.aap.2019.06.005
Evans T, Stuckey R, Macdonald W (2020) Young drivers’ perceptions of risk and difficulty: day versus night. Accid Anal Prev 147:105753–105767
FarhadHadadi HS (2017) Assessment of drowsy drivers by fuzzy logic approach based on multinomial logistic regression analysis. IJCSNS Int J Comput Sci Netw Secur 17:298–305
Google Scholar
Festy B (2013) Review of evidence on health aspects of air pollution - REVIHAAP Project. Technical Report. World Health Organization Regional Office for Europe 2013. Pollut Atmos
Forward SE (2009) The theory of planned behaviour: the role of descriptive norms and past behaviour in the prediction of drivers’ intentions to violate. Transp Res Part F Traffic Psychol Behav 12:198–207. https://doi.org/10.1016/j.trf.2008.12.002
Fu L, Chen Y, Yang X et al (2019) The associations of air pollution exposure during pregnancy with fetal growth and anthropometric measurements at birth: a systematic review and meta-analysis. Environ Sci Pollut Res 26:20137–20147. https://doi.org/10.1007/s11356-019-05338-0
Gu H, Yan W, Elahi E, Cao Y (2020) Air pollution risks human mental health: an implication of two-stages least squares estimation of interaction effects. Environ Sci Pollut Res 27:2036–2043. https://doi.org/10.1007/s11356-019-06612-x
Hammad HM, Ashraf M, Abbas F, et al (2019) Environmental factors affecting the frequency of road traffic accidents: a case study of sub-urban area of Pakistan. Environ Sci Pollut Res 11674–11685 https://doi.org/10.1007/s11356-019-04752-8
Hassen A, Godesso A, Abebe L, Girma E (2011) Risky driving behaviors for road traffic accident among drivers in Mekele city, Northern Ethiopia. BMC Res Notes 4:2–7. https://doi.org/10.1186/1756-0500-4-535
Heutel G, Ruhm CJ (2016) Air pollution and procyclical mortality. J Assoc Environ Resour Econ 3:667–706
Huang R, Zhang Y, Bozzetti C, et al (2014) High secondary aerosol contribution to particulate pollution during haze events in China https://doi.org/10.1038/nature13774
Iversen H (2004) Risk-taking attitudes and risky driving behaviour. Transp Res Part F Traffic Psychol Behav 7:135–150
Ju YJ, Lee JE, Lee SY (2021) Perceived environmental pollution and subjective cognitive decline (SCD) or SCD-related functional difficulties among the general population. Environ Sci Pollut Res 28:31289–31300. https://doi.org/10.1007/s11356-021-12831-y
Kang JJ, Ni R, Andersen GJ (2008) Effects of reduced visibility from fog on car-following performance. Transp Res Rec 9–15 https://doi.org/10.3141/2069-02
Kilpeläinen M, Summala H (2007) Effects of weather and weather forecasts on driver behaviour. Transp Res Part F Traffic Psychol Behav 10:288–299. https://doi.org/10.1016/j.trf.2006.11.002
Kim H, Lee K, Park K (2015) Balancing out feelings of risk by playing it safe: the effect of social networking on subsequent risk judgment. Organ Behav Hum Decis Process 131:121–131. https://doi.org/10.1016/j.obhdp.2015.09.002
Laude JR, Fillmore MT (2016) Drivers who self-estimate lower blood alcohol concentrations are riskier drivers after drinking. Psychopharmacology 233:1387–1394. https://doi.org/10.1007/s00213-016-4233-x
Lee S, Lee W, Kim D et al (2019) Short-term PM2. 5 exposure and emergency hospital admissions for mental disease. Environ Res 171:313–320
Lepori GM (2016) Air pollution and stock returns: evidence from a natural experiment. J Empir Financ 35:25–42
Lepp A, Gibson H (2003) Tourist roles, perceived risk and international tourism. Ann Tour Res 30:606–624
Levy T, Yagil J (2011) Air pollution and stock returns in the US. J Econ Psychol 32:374–383
Li H, Cai J, Chen R et al (2017) Particulate matter exposure and stress hormone levels: a randomized, double-blind, crossover trial of air purification. Circulation 136:618–627
Li M, Zhang L (2014) Haze in China: current and future challenges. Environ Pollut 189:85–86
Li X, Yan X, Wong SC (2015) Effects of fog, driver experience and gender on driving behavior on S-curved road segments. Accid Anal Prev 77:91–104. https://doi.org/10.1016/j.aap.2015.01.022
Lima ML (2004) On the influence of risk perception on mental health: living near an incinerator. J Environ Psychol 24:71–84
Liu X, Zhu H, Hu Y et al (2016) Public’s health risk awareness on urban air pollution in Chinese megacities: the cases of Shanghai, Wuhan and Nanchang. Int J Environ Res Public Health 13:845–859
Lo C-C, Liu W-T, Lu Y-H et al (2022) Air pollution associated with cognitive decline by the mediating effects of sleep cycle disruption and changes in brain structure in adults. Environ Sci Pollut Res. https://doi.org/10.1007/s11356-022-19482-7
Losacco C, Perillo A (2018) Particulate matter air pollution and respiratory impact on humans and animals. Environ Sci Pollut Res 25:33901–33910. https://doi.org/10.1007/s11356-018-3344-9
Mackenzie AK, Harris JM (2017) A link between attentional function, effective eye movements, and driving ability. J Exp Psychol Hum Percept Perform 43:381–394
Marques S, Lima ML (2011) Living in grey areas: industrial activity and psychological health. J Environ Psychol 31:314–322
Matthews ML, Moran AR (1986) Age differences in male drivers’ perception of accident risk: the role of perceived driving ability. Accid Anal Prev 18:299–313. https://doi.org/10.1016/0001-4575(86)90044-8
Mbululo Y, Qin J, Yuan Z et al (2019) Boundary layer perspective assessment of air pollution status in Wuhan city from 2013 to 2017. Environ Monit Assess 191:69. https://doi.org/10.1007/s10661-019-7206-9
Mccullagh P (1980) Regression models for ordinal data. J R Stat Soc Ser B 42:109–127. https://doi.org/10.1111/j.2517-6161.1980.tb01109.x
McKnight AJ, McKnight AS (2003) Young novice drivers: careless or clueless? Accid Anal Prev 35:921–925
Ministry of ecology and environment of the People’s Republic of China (2020) National Statistical Bulletin on Ecological Environment (2016–2019). 1–8
Moore M, Dahlen ER (2008) Forgiveness and consideration of future consequences in aggressive driving. Accid Anal Prev 40:1661–1666. https://doi.org/10.1016/j.aap.2008.05.007
Neidell M (2017) Air pollution and worker productivity. IZA World Labor 363:1–10
Özkan T, Lajunen T (2006) What causes the differences in driving between young men and women? The effects of gender roles and sex on young drivers’ driving behaviour and self-assessment of skills. Transp Res Part F Traffic Psychol Behav 9:269–277
Precht L, Keinath A, Krems JF (2017) Identifying the main factors contributing to driving errors and traffic violations – results from naturalistic driving data. Transp Res Part F Traffic Psychol Behav 49:49–92. https://doi.org/10.1016/j.trf.2017.06.002
Ram T, Chand K (2016) Effect of drivers’ risk perception and perception of driving tasks on road safety attitude. Transp Res Part F Traffic Psychol Behav 42:162–176. https://doi.org/10.1016/j.trf.2016.07.012
Ram T, Chand K (2015) Effect of drivers’ risk perception on safe driving attitude. Int J Veh Saf 8:218–232. https://doi.org/10.1504/IJVS.2015.070773
Reason J, Manstead A, Stradling S et al (1990) Errors and violations on the roads: a real distinction? Ergonomics 33:1315–1332
Riis-Vestergaard MI, van Ast V, Cornelisse S et al (2018) The effect of hydrocortisone administration on intertemporal choice. Psychoneuroendocrinology 88:173–182
Roca J, Lupiáñez J, López-Ramón MF, Castro C (2013) Are drivers’ attentional lapses associated with the functioning of the neurocognitive attentional networks and with cognitive failure in everyday life? Transp Res Part F Traffic Psychol Behav 17:98–113. https://doi.org/10.1016/j.trf.2012.10.005
Sager L (2019) Estimating the effect of air pollution on road safety using atmospheric temperature inversions. J Environ Econ Manage 98:1–20. https://doi.org/10.1016/j.jeem.2019.102250
Sani SRH, Tabibi Z, Fadardi JS, Stavrinos D (2017) Aggression, emotional self-regulation, attentional bias, and cognitive inhibition predict risky driving behavior. Accid Anal Prev 109:78–88. https://doi.org/10.1016/j.aap.2017.10.006
Sarnat JA, Schwartz J, Suh HH (2001) Fine particulate air pollution and mortality in 20 U.S. cities. N Engl J Med 344:1253–1254
Satiennam W, Satiennam T, Triyabutra T, Rujopakarn W (2018) Red light running by young motorcyclists: factors and beliefs influencing intentions and behavior. Transp Res Part F Traffic Psychol Behav 55:234–245. https://doi.org/10.1016/j.trf.2018.03.007
Schwarz N (2011) Feelings-as-information theory. Handb Theor. Soc Psychol 1:289–308
Sepandi M, Akbari H, Naseri MH, Alimohamadi Y (2021) Emergency hospital admissions for cardiovascular diseases attributed to air pollution in Tehran during 2016–2019. Environ Sci Pollut Res 28:38426–38433. https://doi.org/10.1007/s11356-021-13377-9
Shabani S (2021) A mechanistic view on the neurotoxic effects of air pollution on central nervous system: risk for autism and neurodegenerative diseases. Environ Sci Pollut Res 28:6349–6373. https://doi.org/10.1007/s11356-020-11620-3
Shang L, Yang L, Yang W et al (2020) Effects of prenatal exposure to NO2 on children’s neurodevelopment: a systematic review and meta-analysis. Environ Sci Pollut Res 27:24786–24798. https://doi.org/10.1007/s11356-020-08832-y
Shi J, Bai Y, Tao L, Atchley P (2011) A model of Beijing drivers’ scrambling behaviors. Accid Anal Prev 43:1540–1546
Shirmohammadi H, Hadadi F, Saeedian M (2019) Clustering analysis of drivers based on behavioral characteristics regarding road safety. Int J Civ Eng 17:1327–1340. https://doi.org/10.1007/s40999-018-00390-2
Siddique HMA, Kiani AK (2020) Industrial pollution and human health: evidence from middle-income countries. Environ Sci Pollut Res 27:12439–12448. https://doi.org/10.1007/s11356-020-07657-z
Stephens AN, Sullman MJM (2014) Development of a short form of the driving anger expression inventory. Accid Anal Prev 72:169–176. https://doi.org/10.1016/j.aap.2014.06.021
Su C-W, Wang K-H, Tao R, Lobonţ O-R (2019) The asymmetric effect of air quality on cross-industries’ stock returns: evidence from China. Environ Sci Pollut Res 26:31422–31433. https://doi.org/10.1007/s11356-019-06283-8
Swietlicki E, Puri S, Hansson H-C, Edner H (1996) Urban air pollution source apportionment using a combination of aerosol and gas monitoring techniques. Atmos Environ 30:2795–2809
Ulleberg P (2001) Personality subtypes of young drivers. Relationship to risk-taking preferences, accident involvement, and response to a traffic safety campaign. Transp Res Part F Traffic Psychol Behav 4:279–297. https://doi.org/10.1016/S1369-8478(01)00029-8
Vallius M, Lanki T, Tiittanen P et al (2003) Source apportionment of urban ambient PM2. 5 in two successive measurement campaigns in Helsinki. Finland Atmos Environ 37:615–623
Verschuur WLG, Hurts K (2008) Modeling safe and unsafe driving behaviour. Accid Anal Prev 40:644–656. https://doi.org/10.1016/j.aap.2007.09.001
Waller PF (2003) The genesis of GDL. J Safety Res 34:17–23
Waller PF, Elliott MR, Shope JT et al (2001) Changes in young adult offense and crash patterns over time. Accid Anal Prev 33:117–128
Wan Y, Li Y, Liu C, Li Z (2020) Is traffic accident related to air pollution? A case report from an island of Taihu Lake, China. Atmos Pollut Res 11:1028–1033. https://doi.org/10.1016/j.apr.2020.02.018
Wang J, Li H, Wang Y, Yang H (2021) A novel assessment and forecasting system for traffic accident economic loss caused by air pollution. Environ Sci Pollut Res 28:49042–49062. https://doi.org/10.1007/s11356-021-13595-1
Wang S, Li Z, Zhang H (2021) Does female labor share reduce embodied carbon in trade? Environ Sci Pollut Res 28:8246–8257. https://doi.org/10.1007/s11356-020-11172-6
Wang T, Mu W, Cui N (2021) Can the effectiveness of driver education be sustained? Effects of driving breaks on novice drivers’ traffic violations. Accid Anal Prev 154. https://doi.org/10.1016/j.aap.2021c.106083
Warshawsky-livne L, Shinar D (2002) Effects of uncertainty, transmission type.pdf. 33:117–128
Wickens CM, Toplak ME, Wiesenthal DL (2008) Cognitive failures as predictors of driving errors, lapses, and violations. Accid Anal Prev 40:1223–1233. https://doi.org/10.1016/j.aap.2008.01.006
Wilde GJS (1982) The theory of risk homeostasis: implications for safety and health. Risk Anal 2:209–225
Wu WS, Wang T (2007) On the performance of a semi-continuous PM2.5 sulphate and nitrate instrument under high loadings of particulate and sulphur dioxide. Atmos Environ 41:5442–5451. https://doi.org/10.1016/j.atmosenv.2007.02.025
Xia X, Yu Y, Zou Y (2022) Air pollution, social engagement and subjective well-being: evidence from the Gallup World Poll. Environ Sci Pollut Res. https://doi.org/10.1007/s11356-022-19451-0
Xie CQ, Parker D (2002) A social psychological approach to driving violations in two Chinese cities. Transp Res Part F Traffic Psychol Behav 5:293–308. https://doi.org/10.1016/S1369-8478(02)00034-7
Xu J, Geng W, Geng X et al (2020) Study on the association between ambient air pollution and daily cardiovascular death in Hefei, China. Environ Sci Pollut Res 27:547–561. https://doi.org/10.1007/s11356-019-06867-4
Yan X, Li X, Liu Y, Zhao J (2014) Effects of foggy conditions on drivers’ speed control behaviors at different risk levels. Saf Sci 68:275–287. https://doi.org/10.1016/j.ssci.2014.04.013
Yan Y, She L, Guo Y et al (2021) Association between ambient air pollution and mortality from chronic obstructive pulmonary disease in Wuhan, China: a population-based time-series study. Environ Sci Pollut Res 28:33698–33706. https://doi.org/10.1007/s11356-021-13180-6
Yaseen MR, Ali Q, Khan MTI (2018) General dependencies and causality analysis of road traffic fatalities in OECD countries. Environ Sci Pollut Res 25:19612–19627. https://doi.org/10.1007/s11356-018-2146-4
Ye B, Ji X, Yang H et al (2003) Concentration and chemical composition of PM2. 5 in Shanghai for a 1-year period. Atmos Environ 37:499–510
Ye R, Cui L, Peng X et al (2019) Effect and threshold of PM2.5 on population mortality in a highly polluted area: a study on applicability of standards. Environ Sci Pollut Res 26:18876–18885. https://doi.org/10.1007/s11356-019-04999-1
Yuan X, Mi M, Mu R, Zuo J (2013) Strategic route map of sulphur dioxide reduction in China. Energy Policy 60:844–851. https://doi.org/10.1016/j.enpol.2013.05.072
Zhai Z, Xu J, Song G, Hatzopoulou M (2022) Comparative analysis of drive-cycles, speed limit violations, and emissions in two cities: Toronto and Beijing. Sci Total Environ 811:152323. https://doi.org/10.1016/j.scitotenv.2021.152323
Zhang G, Yau KKW, Chen G (2013) Risk factors associated with traffic violations and accident severity in China. Accid Anal Prev 59:18–25. https://doi.org/10.1016/j.aap.2013.05.004
Zhang J, Mu Q (2018) Air pollution and defensive expenditures: evidence from particulate-filtering facemasks. J Environ Econ Manage 92:517–536
Zhang K, Batterman S (2013) Air pollution and health risks due to vehicle traffic. Sci Total Environ 450–451:307–316. https://doi.org/10.1016/j.scitotenv.2013.01.074
Zhang X, Zhang X, Chen X (2017) Happiness in the air: how does a dirty sky affect mental health and subjective well-being? J Environ Econ Manage 85:81–94
Zhou J, Zhang R, Cao J et al (2012) Carbonaceous and ionic components of atmospheric fine particles in Beijing and their impact on atmospheric visibility. Aerosol Air Qual Res 12:492–502
Zivin JG, Neidell M (2009) Days of haze: environmental information disclosure and intertemporal avoidance behavior. J Environ Econ Manage 58:119–128
Download references
Tao Wang thanks the National Natural Science Foundation of China 72172107.
Author information
Authors and affiliations.
School of Economics and Management, Wuhan University, Wuhan, People’s Republic of China
Tao Wang, Yu Wang & Nan Cui
Research Center For Organizational Marketing of Wuhan University, Wuhan University, Wuhan, People’s Republic of China
You can also search for this author in PubMed Google Scholar
Contributions
Tao Wang-conceptualization, methodology, resources, data curation, and writing. Yu Wang-software, investigation, original draft, visualization, and project administration. Nan Cui-supervision, conceptualization, methodology, validation, writing-review, and editing data.
Corresponding author
Correspondence to Yu Wang .
Ethics declarations
Ethics approval.
Not applicable.
Consent to participate
Consent for publication, conflict of interest.
The authors declare no competing interests.
Additional information
Responsible Editor: Eyup Dogan
Publisher's note
Springer Nature remains neutral with regard to jurisdictional claims in published maps and institutional affiliations.
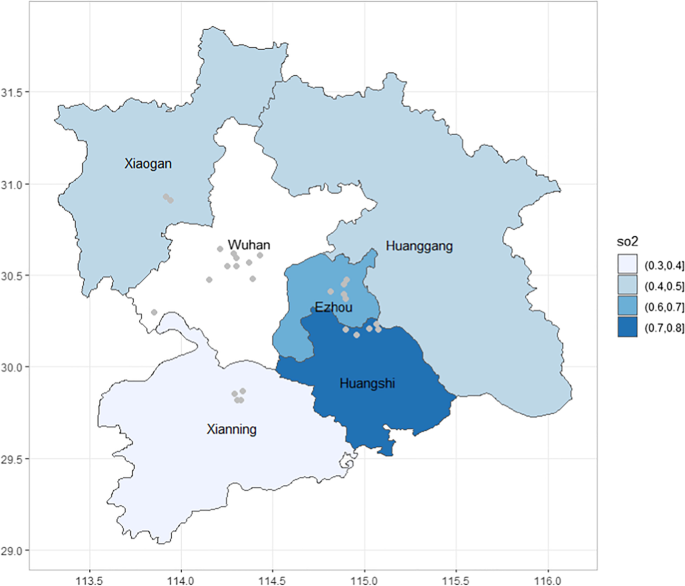
The geographical situation of Wuhan. Note: The geographical distribution of the five cities around Wuhan and the average daily concentration of industrial emissions So 2 in the 2018 year. The dots in the figure represent national-level air monitoring stations
Rights and permissions
Reprints and permissions
About this article
Wang, T., Wang, Y. & Cui, N. Traffic costs of air pollution: the effect of PM 2.5 on traffic violation. Environ Sci Pollut Res 29 , 72699–72717 (2022). https://doi.org/10.1007/s11356-022-20790-1
Download citation
Received : 15 October 2021
Accepted : 09 May 2022
Published : 25 May 2022
Issue Date : October 2022
DOI : https://doi.org/10.1007/s11356-022-20790-1
Share this article
Anyone you share the following link with will be able to read this content:
Sorry, a shareable link is not currently available for this article.
Provided by the Springer Nature SharedIt content-sharing initiative
- Air pollution
- Traffic violations
- Overconfidence
- Inexperience
- Find a journal
- Publish with us
- Track your research
Information
- Author Services
Initiatives
You are accessing a machine-readable page. In order to be human-readable, please install an RSS reader.
All articles published by MDPI are made immediately available worldwide under an open access license. No special permission is required to reuse all or part of the article published by MDPI, including figures and tables. For articles published under an open access Creative Common CC BY license, any part of the article may be reused without permission provided that the original article is clearly cited. For more information, please refer to https://www.mdpi.com/openaccess .
Feature papers represent the most advanced research with significant potential for high impact in the field. A Feature Paper should be a substantial original Article that involves several techniques or approaches, provides an outlook for future research directions and describes possible research applications.
Feature papers are submitted upon individual invitation or recommendation by the scientific editors and must receive positive feedback from the reviewers.
Editor’s Choice articles are based on recommendations by the scientific editors of MDPI journals from around the world. Editors select a small number of articles recently published in the journal that they believe will be particularly interesting to readers, or important in the respective research area. The aim is to provide a snapshot of some of the most exciting work published in the various research areas of the journal.
Original Submission Date Received: .
- Active Journals
- Find a Journal
- Proceedings Series
- For Authors
- For Reviewers
- For Editors
- For Librarians
- For Publishers
- For Societies
- For Conference Organizers
- Open Access Policy
- Institutional Open Access Program
- Special Issues Guidelines
- Editorial Process
- Research and Publication Ethics
- Article Processing Charges
- Testimonials
- Preprints.org
- SciProfiles
- Encyclopedia
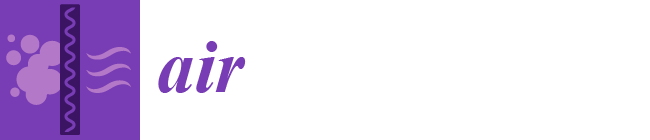
Article Menu
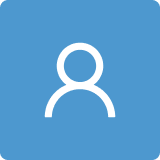
- Subscribe SciFeed
- Recommended Articles
- Google Scholar
- on Google Scholar
- Table of Contents
Find support for a specific problem in the support section of our website.
Please let us know what you think of our products and services.
Visit our dedicated information section to learn more about MDPI.
JSmol Viewer
A study on the impact of air pollution on health status of traffic police personnel in kolkata, india.
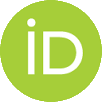
1. Introduction
2. materials and methods, 2.1. selection of study area, 2.2. collection of air quality data, 2.3. questionnaire-based survey, 2.4. data analysis, 2.5. preparation of the geographical map, 3. results and discussion, 3.1. analysis of air quality parameters, 3.2. analysis of questionnaire-based survey data, 4. recommendations.
- Traffic police personnel can be protected from prolonged exposure to air pollution by implementing a roster system. The roster system is a mechanism to schedule traffic police personnels’ shifts to maintain adequate coverage while reducing their exposure to polluted environments on a continuous basis. This is how traffic police personnel will not be permanently stationed in the same high-pollution locations, decreasing their exposure to dangerous pollutants over an extended period. They can obtain enough rest in between shifts, which improves their alertness and effectiveness by allowing them to concentrate and make better decisions when on duty [ 36 ].
- To reduce exposure to peak traffic and pollution, shift timings should be optimized. Off-peak shifts are an approach where shifts can be scheduled during times of the day when traffic congestion is lower. Shift scheduling during peak traffic hours, such as early mornings and late afternoons, when pollution levels are generally higher due to increased vehicular activity, should be avoided. A reasonable shift duration should be maintained. The traffic police personnel should have adequate rest periods between shifts to recover from any potential exposure and stress [ 26 , 39 , 41 , 42 ].
- To keep employees safe while on the job, regular use of personal protective equipment (PPE), like N95 masks and respirators, should be encouraged [ 38 , 62 ].
- Traffic police personnel should be trained in the importance of reducing exposure to air pollution and educated about the health risks associated with prolonged exposure. Information on the proper use of PPE and healthy practices should be provided to minimize exposure during their shifts [ 42 ].
- The awareness campaign should start by arming traffic police personnel with thorough knowledge of the dangers that air pollution poses to their health. Specific high-risk areas with regularly high air pollution levels should be covered in the training. The training course should instruct police personnel on the early warning signs and symptoms of health problems due to air pollution. Because of their alertness, they can spot any health issues early and obtain treatment right away. Coughing, wheezing, shortness of breath, and eye or throat discomfort are some common symptoms. To identify any health difficulties due to exposure to air pollution early on, the government can set up health monitoring programs. A healthy lifestyle, characterized by consistent exercise, maintaining a balanced diet, and refraining from smoking, should be upheld [ 63 ].
- The promotion of green vehicles may reduce emissions and provide a cleaner working environment for traffic police personnel [ 64 , 65 , 66 ]. Incorporating sound urban planning by prioritizing green spaces, proper zoning, and efficient road networks can contribute to an overall improvement in air quality. Encouraging the adoption of public transport as an alternative to private vehicles holds the promise of reducing air pollution [ 67 ]. Organizing a campaign to generate public awareness about the impact of air pollution on traffic police personnel can be thoughtful [ 68 ].
5. Conclusions
Supplementary materials, author contributions, institutional review board statement, informed consent statement, data availability statement, acknowledgments, conflicts of interest.
- Pope, C.A., 3rd; Thun, M.J.; Namboodiri, M.M.; Dockery, D.W.; Evans, J.S.; Speizer, F.E.; Heath, C.W. Particulate air pollution as a predictor of mortality in a prospective study of US adults. Am. J. Respir. Crit. Care Med. 1995 , 151 , 669–674. [ Google Scholar ] [ CrossRef ] [ PubMed ]
- Brunekreef, B.; Holgate, S.T. Air pollution and health. Lancet 2002 , 360 , 1242. [ Google Scholar ] [ CrossRef ] [ PubMed ]
- Organisation for Economic Co-Operation and Development. The Economic Consequences of Outdoor Air Pollution ; Organisation for Economic Co-Operation and Development Publishing: Paris, France, 2016. [ Google Scholar ]
- Kar, S.; Ghosh, I.; Show, S.; Sen, A.; Gupta, T.; Chowdhury, P.; Chatterjee, T.; RoyChowdhury, A. Impact of Coronavirus (COVID-19) Outbreak on Society, Air Quality, and Economy in India: A Study of Three “P”s of Sustainability in India. Sustainability 2021 , 13 , 2873. [ Google Scholar ] [ CrossRef ]
- Smith, K.R.; Ezzati, M. How environmental health risks change with development: The epidemiologic and environmental risk transitions revisited. Annu. Rev. Environ. Resour. 2005 , 30 , 291–333. [ Google Scholar ] [ CrossRef ]
- Global Burden of Disease Study 2015. Global, regional, and national comparative risk assessment of 79 behavioral, environmental and occupational, and metabolic risks or clusters of risks, 1990–2015: A systematic analysis for the Global Burden of Disease Study 2015. Lancet 2016 , 388 , 1659–1724. [ CrossRef ]
- Prüss-Üstun, A.; Wolf, J.; Corvalán, C.; Bos, R.; Neira, M. Preventing Disease through Healthy Environments: A Global Assessment of the Burden of Disease from Environmental Risks ; World Health Organization: Geneva, Switzerland, 2016. [ Google Scholar ]
- Pope, C.A.; Ezzati, M.; Dockery, D.W. Fine-particulate air pollution and life expectancy in the United States. N. Engl. J. Med. 2009 , 360 , 376–386. [ Google Scholar ] [ CrossRef ] [ PubMed ]
- Chowdhury, S.; Dey, S. Cause-specific premature death from ambient PM 2.5 exposure in India: Estimate adjusted for baseline mortality. Environ. Int. 2016 , 91 , 283–290. [ Google Scholar ] [ CrossRef ]
- Gao, M.; Saide, P.E.; Xin, J.; Wang, Y.; Liu, Z.; Wang, Y.; Wang, Z.; Pagowski, M.; Guttikunda, S.K.; Carmichael, G.R. Estimates of health impacts and radiative forcing in winter haze in eastern China through constraints of surface PM 2.5 predictions. Environ. Sci. Technol. 2017 , 51 , 2178–2185. [ Google Scholar ] [ CrossRef ]
- Wang, J.; Xing, J.; Mathur, R.; Pleim Jonathan, E.; Wang, S.; Hogrefe, C.; Gan, C.-M.; Wong, D.C.; Hao, J. Historical trends in PM 2.5 -related premature mortality during 1990–2010 across the northern hemisphere. Environ. Health Perspect. 2017 , 125 , 400–408. [ Google Scholar ] [ CrossRef ]
- Gao, M.; Beig, G.; Song, S.; Zhang, H.; Hu, J.; Ying, Q.; Liang, F.; Liu, Y.; Wang, H.; Lu, X.; et al. The impact of power generation emissions on ambient PM 2.5 pollution and human health in China and India. Environ. Int. 2018 , 121 , 250–259. [ Google Scholar ] [ CrossRef ]
- Huang, J.; Pan, X.; Guo, X.; Li, G. Health impact of China’s Air Pollution Prevention and Control Action Plan: An analysis of national air quality monitoring and mortality data. Lancet Planet. Health 2018 , 2 , e313–e323. [ Google Scholar ] [ CrossRef ] [ PubMed ]
- WHO. WHO Global Urban Ambient Air Pollution Database (Update 2016). 2016. Available online: http://www.who.int/airpollution/data/cities-2016/en/ (accessed on 20 August 2023).
- Atkinson, R.W.; Kang, S.; Anderson, H.R.; Mills, I.C.; Walton, H.A. Epidemiological time series studies of PM 2.5 and daily mortality and hospital admissions: A systematic review and meta-analysis. Thorax 2014 , 69 , 660–665. [ Google Scholar ] [ CrossRef ] [ PubMed ]
- Héroux, M.-E.; Anderson, H.R.; Atkinson, R.; Brunekreef, B.; Cohen, A.; Forastiere, F.; Hurley, F.; Katsouyanni, K.; Krewski, D.; Krzyzanowski, M.; et al. Quantifying the health impacts of ambient air pollutants: Recommendations of a WHO/Europe project. Int. J. Public Health 2015 , 60 , 619–627. [ Google Scholar ] [ CrossRef ] [ PubMed ]
- Johansson, C.; Norman, M.; Gidhagen, L. Spatial and temporal variations of PM 10 and particle number concentrations in urban air. Environ. Monit. Assess. 2007 , 127 , 477–487. [ Google Scholar ] [ CrossRef ] [ PubMed ]
- Zoran, M.; Savastru, D.; Dida, A. Assessing urban air quality and its relation with radon ( 222 Rn). J. Radioanal. Nucl. Chem. 2016 , 309 , 909–922. [ Google Scholar ] [ CrossRef ]
- Wu, C.F.; Shen, F.H.; Li, Y.R.; Tsao, T.M.; Tsai, M.J.; Chen, C.C.; Hwang, J.-S.; Hsu, S.H.-J.; Chao, H.; Chuang, K.-J.; et al. Association of short-term exposure to fine particulate matter and nitrogen dioxide with acute cardiovascular effects. Sci. Total Environ. 2016 , 569–570 , 300–305. [ Google Scholar ] [ CrossRef ]
- Longhin, E.; Holme, J.A.; Gualtieri, M.; Camatini, M.; Øvrevik, J. Milan winter fine particulate matter (PM 2.5 ) induces IL-6 and IL-8 synthesis in human bronchial BEAS-2B cells, but specifically impairs IL-8 release. Toxicol. Vitr. 2018 , 52 , 365–373. [ Google Scholar ] [ CrossRef ] [ PubMed ]
- Prabhu, V.; Shridhar, V. Investigation of potential sources, transport pathway, and health risks associated with respirable suspended particulate matter in Dehradun city, situated in the foothills of the Himalayas. Atmos. Pollut. Res. 2019 , 10 , 187–196. [ Google Scholar ] [ CrossRef ]
- WHO. Air Quality Guidelines for Europe , 2nd ed.; WHO Regional Publications European Series; WHO: Copenhagen, Denmark, 2000; Volume 91, pp. 1–287. ISBN 92-890-1358-3. [ Google Scholar ]
- Holman, C. Sources of Air Pollution. In Air Pollution and Health ; Academic Press: Cambridge, MA, USA, 1999; pp. 115–148. [ Google Scholar ] [ CrossRef ]
- He, M.Z.; Kinney, P.L.; Li, T.; Chen, C.; Sun, Q.; Ban, J.; Wang, J.; Liu, S.; Goldsmith, J.; Kioumourtzoglou, M.-A.; et al. Short-and intermediate-term exposure to NO2 and mortality: A multi-county analysis in China. Environ. Pollut. 2020 , 261 , 114165. [ Google Scholar ] [ CrossRef ]
- Tonne, C.; Beevers, S.; Armstrong, B.; Kelly, F.; Wilkinson, P. Air pollution and mortality benefits of the London Congestion Charge: Spatial and socioeconomic inequalities. Occup. Environ. Med. 2008 , 65 , 620–627. [ Google Scholar ] [ CrossRef ]
- Zhang, D.; Iwasaka, Y. Chlorine deposition on dust particles in marine atmosphere. Geophys. Res. Lett. 2001 , 28 , 3613–3616. [ Google Scholar ] [ CrossRef ]
- Longo, B.; Rossignol, A.; Green, J. Cardiorespiratory Health Effects Associated with Sulphurous Volcanic Air Pollution. Public Health 2008 , 122 , 809–820. [ Google Scholar ] [ CrossRef ] [ PubMed ]
- Alberta Environment. In Sulphur Dioxide: Environmental Effects, Fate and Behaviour ; Alberta Environment: Edmonton, ON, Canada, 2003; ISBN 0-7785-3216-x. (on-line edition).
- Chestnut, L.G. Human Health Benefits from Sulfate Reductions under Title IV of the 1990 Clean Air Act Amendments ; Final Report; US EPA, Office of Atmospheric Programs, Acid Rain Division: Washington, DC, USA, 1995.
- Burgaz, S.; Demircigil, G.C.; Karahalil, B.; Karakaya, A.E. Chromosomal damage in peripheral blood lymphocytes of traffic policemen and taxi drivers exposed to urban air pollution. Chemosphere 2002 , 47 , 57–64. [ Google Scholar ] [ CrossRef ] [ PubMed ]
- Tan, C.; Wang, Y.; Lin, M.; Wang, Z.; He, L.; Li, Z.; Li, Y.; Xu, K. Long-term high air pollution exposure induced metabolic adaptations in traffic policemen. Environ. Toxicol. Pharmacol. 2018 , 58 , 156–162. [ Google Scholar ] [ CrossRef ] [ PubMed ]
- Satapathy, D.M.; Behera, T.R.; Tripathy, R.M. Health status of traffic police personnel in Brahmapur city. Indian J. Community Med. 2009 , 34 , 71. [ Google Scholar ] [ CrossRef ] [ PubMed ]
- Chakraborty, A. Effects of air pollution on public health: The case of vital traffic junctions under Kolkata Municipal Corporation. J. Stud. Dyn. Chang. 2014 , 1 , 125–133. [ Google Scholar ]
- Sharma, H.K.; Dandotiya, B.; Jadon, N. Exposure of air pollution and its health effects in traffic police persons of Gwalior City, India. Environ. Claims J. 2017 , 29 , 305–315. [ Google Scholar ] [ CrossRef ]
- Spiroska, J.; Rahman, A.; Pal, S. Air Pollution in Kolkata: An Analysis of Current Status and Interrelation between Different Factors. SEEU Rev. 2011 , 8 , 182–214. [ Google Scholar ] [ CrossRef ]
- Mukherjee, A.; Mukherjee, G. Occupational exposure of the traffic personnel of Calcutta to lead and carbon monoxide. Pollut. Res. 1998 , 17 , 359–362. [ Google Scholar ]
- Kazimuddin, A.; Banerjee, L. Fighting for Air. Down to Earth. 2000. Available online: https://www.downtoearth.org.in/coverage/fighting-for-air-18428 (accessed on 12 July 2023).
- Delfino, R.J. Epidemiologic evidence for asthma and exposure to air toxics: Linkages between occupational, indoor, and community air pollution research. Environ. Health Perspect. 2002 , 110 (Suppl. S4), 573. [ Google Scholar ] [ CrossRef ]
- EPA. Final Report: Integrated Science Assessment for Sulfur Oxides. 2008. Available online: https://cfpub.epa.gov/ncea/isa/recordisplay.cfm?deid=198843 (accessed on 12 July 2023).
- EPA. Final Report: Integrated Science Assessment for Particulate Matter. 2009. Available online: https://cfpub.epa.gov/ncea/risk/recordisplay.cfm?deid=216546 (accessed on 14 July 2023).
- Ling, S.H.; van Eeden, S.F. Particulate matter air pollution exposure: Role in the development and exacerbation of chronic obstructive pulmonary disease. Int. J. Chronic Obstr. Pulm. Dis. 2009 , 4 , 233–243. [ Google Scholar ] [ CrossRef ] [ PubMed ]
- Ghorani-Azam, A.; Riahi-Zanjani, B.; Balali-Mood, M. Effects of air pollution on human health and practical measures for prevention in Iran. J. Res. Med. Sci. 2016 , 21 , 65. [ Google Scholar ] [ CrossRef ] [ PubMed ]
- Brenes, G.A. Anxiety and chronic obstructive pulmonary disease: Prevalence, impact, and treatment. Psychosom. Med. 2003 , 65 , 963–970. [ Google Scholar ] [ CrossRef ] [ PubMed ]
- Scott, K.M.; Von Korff, M.; Ormel, J.; Zhang, M.Y.; Bruffaerts, R.; Alonso, J.; Kessler, R.C.; Tachimori, H.; Karam, E.; Levinson, D.; et al. Mental disorders among adults with asthma: Results from the World Mental Health Survey. Gen. Hosp. Psychiatry 2011 , 29 , 123–133. [ Google Scholar ] [ CrossRef ] [ PubMed ]
- Yohannes, A.M.; Willgoss, T.G.; Baldwin, R.C.; Connolly, M.J. Depression and anxiety in chronic heart failure and chronic obstructive pulmonary disease: Prevalence, relevance, clinical implications and management principles. Int. J. Geriatr. Psychiatry 2010 , 25 , 1209–1221. [ Google Scholar ] [ CrossRef ]
- Spitzer, C.; Gläser, S.; Grabe, H.J.; Ewert, R.; Barnow, S.; Felix, S.B.; Freyberger, H.J.; Völzke, H.; Koch, B.; Schäper, C. Mental health problems, obstructive lung disease and lung function: Findings from the general population. J. Psychosom. Res. 2007 , 71 , 174–179. [ Google Scholar ] [ CrossRef ]
- Ingle, S.T.; Pachpande, B.G.; Wagh, N.D.; Patel, V.S.; Attarde, S.B. Exposure to Vehicular Pollution and Respiratory Impairment of Traffic Policemen in Jalgaon City, India. Ind. Health 2005 , 43 , 656–662. [ Google Scholar ] [ CrossRef ]
- Ritz, B.; Lee, P.C.; Hansen, J.; Lassen, C.F.; Ketzel, M.; Sørensen, M.; Raaschou-Nielsen, O. Traffic-Related Air Pollution and Parkinson’s Disease in Denmark: A Case–Control Study. Environ. Health Perspect. 2016 , 124 , 351–356. [ Google Scholar ] [ CrossRef ]
- Fathi Najafi, T.; Latifnejad Roudsari, R.; Namvar, F.; Ghavami Ghanbarabadi, V.; Hadizadeh Talasaz, Z.; Esmaeli, M. Air Pollution and Quality of Sperm: A Meta-Analysis. Iran. Red Crescent Med. J. 2015 , 17 , e26930. [ Google Scholar ] [ CrossRef ]
- Dhiman, V.; Trushna, T.; Raj, D.; Tiwari, R.R. Is ambient air pollution a risk factor for Parkinson’s disease? A meta-analysis of epidemiological evidence. Int. J. Environ. Health Res. 2022 , 33 , 733–750. [ Google Scholar ] [ CrossRef ]
- Central Pollution Control Board. 2023. Available online: https://cpcb.nic.in/ (accessed on 15 June 2023).
- Cichowicz, R.; Wielgosiński, G.; Fetter, W. Dispersion of atmospheric air pollution in summer and winter season. Environ. Monit. Assess. 2017 , 189 , 605. [ Google Scholar ] [ CrossRef ]
- Jeż, M. Ochrona Atmosfery ; Oficyna Wydawnicza Wyższej Szkoły Ekologii i Zarządzania: Warszawa, Poland, 2009. [ Google Scholar ]
- Nemitz, E.; Hargreaves, K.J.; McDonald, A.G.; Dorsey, J.R.; Fowler, D. Micrometeorological measurements of the urban heat budget and CO2 emissions on a city scale. Environ. Sci. Technol. 2002 , 36 , 3139–3146. [ Google Scholar ] [ CrossRef ] [ PubMed ]
- Lin, W.; Xu, X.; Ge, B.; Liu, X. Gaseous pollutants in Beijing urban area during the heating period 2007–2008: Variability, sources, meteorological, and chemical impacts. Atmos. Chem. Phys. 2011 , 11 , 8157–8170. [ Google Scholar ] [ CrossRef ]
- Gurney, K.R.; Razlivanov, I.; Song, Y.; Zhou, Y.; Benes, B.; Massih, M.A. Quantification of fossil fuel CO2 emissions on the building/street scale for a large U.S. City. Environ. Sci. Technol. 2012 , 46 , 12194–12202. [ Google Scholar ] [ CrossRef ] [ PubMed ]
- Lelieveld, J.; Evans, J.S.; Fnais, M.; Giannadaki, D.; Pozzer, A. The contribution of outdoor air pollution sources to premature mortality on a global scale. Nature 2015 , 525 , 367–371. [ Google Scholar ] [ CrossRef ] [ PubMed ]
- Yusuf, A.A.; Inambao, F.L. Effect of cold start emissions from gasoline-fueled engines of light-duty vehicles at low and high ambient temperatures: Recent trends. Case Stud. Therm. Eng. 2019 , 14 , 100417. [ Google Scholar ] [ CrossRef ]
- Watson, A.Y.; Bates, R.R.; Kennedy, D. (Eds.) Atmospheric Transport and Dispersion of Air Pollutants Associated with Vehicular Emissions, Air Pollution, the Automobile, and Public Health ; National Academies Press (US): Cambridge, MA, USA, 1988. Available online: https://www.ncbi.nlm.nih.gov/books/NBK218142/ (accessed on 16 July 2023).
- Cichowicz, R.; Wielgosiński, G. Effect of meteorological conditions and building location on CO2 concentration in the university campus. Ecol. Chem. Eng. S 2015 , 22 , 513–525. [ Google Scholar ] [ CrossRef ]
- Won, W.S.; Oh, R.; Lee, W.; Ku, S.; Su, P.C.; Yoon, Y.J. Hygroscopic properties of particulate matter and effects of their interactions with weather on visibility. Sci. Rep. 2021 , 11 , 16401. [ Google Scholar ] [ CrossRef ]
- Long, Y.; Hu, T.; Liu, L.; Chen, R.; Guo, Q.; Yang, L.; Cheng, Y.; Huang, J.; Du, L. Effectiveness of N95 respirators versus surgical masks against influenza: A systematic review and meta-analysis. J. Evid.-Based Med. 2020 , 13 , 93–101. [ Google Scholar ] [ CrossRef ]
- Ramírez, A.S.; Ramondt, S.; Van Bogart, K.; Perez-Zuniga, R. Public awareness of air pollution and health threats: Challenges and opportunities for communication strategies to improve environmental health literacy. J. Health Commun. 2019 , 24 , 641–649. [ Google Scholar ] [ CrossRef ]
- Pandey, A.; Shukla, K.; Ahlawat, J.; Negi, R.; Pandey, D.K. Environmental Issues and Policies ; Orange Books Publication: Bhilai, India; Available online: https://www.researchgate.net/publication/373074937_Environmental_Issues_And_Policies (accessed on 16 July 2023).
- Acharya, S.; Aithal, P.S. Green Fueling for the Future Electric Vehicle. Available online: https://books.google.ie/books?id=GYHezwEACAAJ&dq=Green+fueling+for+the+future+electric+vehicle&hl=&cd=1&source=gbs_api (accessed on 16 July 2023).
- Sanguesa, J.A.; Torres-Sanz, V.; Garrido, P.; Martínez, F.J.; Marquez-Barja, J.M. A Review on Electric Vehicles: Technologies and Challenges. Smart Cities 2020 , 4 , 372–404. [ Google Scholar ] [ CrossRef ]
- Nurdden, A.; Rahmat, R.A.O.K.; Ismail, A. Effect of transportation policies on modal shift from private car to public transport in Malaysia. J. Appl. Sci. 2007 , 7 , 1013–1018. [ Google Scholar ] [ CrossRef ]
- Suwal, R.; Ale, B.B. Idling fuel consumption and emissions of public vehicles run by Bhaktapur Minibus Sewa Samiti and its reduction possibilities. J. Inst. Eng. 2019 , 15 , 155–164. [ Google Scholar ] [ CrossRef ]
- Saxena, J.; Yadav, R.; Singh, S.K. Remote Traffic Monitoring & Control System. In Proceedings of the IEEE 2018 International Conference on Advances in Computing, Communication Control and Networking (ICACCCN), Greater Noida, India, 12–13 October 2018; pp. 552–557. [ Google Scholar ] [ CrossRef ]
Click here to enlarge figure
Sl. No. | Location Details | Location Characteristics |
---|---|---|
1. | Ballygunge | The site is enclosed by Syed Amir Ali Ave., Circus Ave., Gurusaday Rd., and Ballygunge Circular Rd. There is a significant amount of traffic on Syed Amir Ali Rd., leading to frequent congestion. The location is also close to the major Park Circus 7-point crossing, an important intersection in Kolkata, with vehicles being the primary source of pollution. |
The area represents a blend of commercial and residential spaces, encompassing commercial buildings, highrises, apartments, scattered residences, and intermittent green areas. | ||
2. | Fort William | The site is situated near the western side of Kolkata, along the banks of the Hooghly River. This location is intersected by significant roads with heavy traffic throughout the day. |
The area consistently receives pollutants from the traffic moving between Howrah and Kolkata via the Vidyasagar Setu, as well as vehicles commuting between Barabazar and Kolkata. The site’s vicinity to the Hooghly River and its surroundings, including the greenery of Fort William and Maidan, further characterize its environment. | ||
3. | Jadavpur | Situated alongside Jadavpur Main Rd., a crucial thoroughfare for accessing Central Kolkata, this site contends with pollutants from multiple sources. While vehicular emissions constitute a major contributor, considerable pollutants stem from both the roadside eateries and the neighboring residential area. |
4. | Rabindra Bharati University | This site is situated alongside B.T. Rd., a vital connection between Kolkata and the northern regions of West Bengal and other states. The road experiences heavy traffic, including goods and heavy-duty vehicles, leading to frequent congestion and subsequently higher pollutant emissions. |
The surroundings consist of buildings, highrises, and apartments, causing pollutants to disperse at a slower rate. | ||
5. | Rabindra Sarobar | Nestled beside Rabindra Sarobar Lake, this site is bordered by the significant Southern Ave., connecting Golpark, Gariahat, and Shyama Prasad Mukherjee Rd. Throughout the day, S.P. Mukherjee Rd. remains busy with traffic, while Southern Avenue experiences a surge in morning and evening traffic as officegoers travel to and from work. |
The site benefits from the presence of Rabindra Sarobar Lake and its lush green surroundings, providing relief from pollutants. | ||
6. | Victoria Memorial | Positioned near the Exide crossing, a pivotal junction linking north, central, and south Kolkata, this site assumes great importance. Its surroundings witness constant heavy traffic flow through major routes such as Asutosh Mukherjee Rd., Circus Ave., AJC Bose Rd. Flyover, Cathedral Rd., Hospital Rd., and Queens Way. |
Although vehicular pollutants have a significant impact on this site, its advantage lies in the ample greenery and open spaces nearby, including The Maidan and Racecourse Ground. These areas not only absorb pollutants but also aid in dispersing them. |
The statements, opinions and data contained in all publications are solely those of the individual author(s) and contributor(s) and not of MDPI and/or the editor(s). MDPI and/or the editor(s) disclaim responsibility for any injury to people or property resulting from any ideas, methods, instructions or products referred to in the content. |
Share and Cite
Kar, S.; Chowdhury, S.; Gupta, T.; Hati, D.; De, A.; Ghatak, Z.; Tinab, T.; Rahman, I.T.; Chatterjee, S.; RoyChowdhury, A. A Study on the Impact of Air Pollution on Health Status of Traffic Police Personnel in Kolkata, India. Air 2024 , 2 , 1-23. https://doi.org/10.3390/air2010001
Kar S, Chowdhury S, Gupta T, Hati D, De A, Ghatak Z, Tinab T, Rahman IT, Chatterjee S, RoyChowdhury A. A Study on the Impact of Air Pollution on Health Status of Traffic Police Personnel in Kolkata, India. Air . 2024; 2(1):1-23. https://doi.org/10.3390/air2010001
Kar, Sayanti, Santanu Chowdhury, Tanya Gupta, Dipsita Hati, Arindam De, Ziniya Ghatak, Tahsin Tinab, Iffa Tasnim Rahman, Shreyashi Chatterjee, and Abhishek RoyChowdhury. 2024. "A Study on the Impact of Air Pollution on Health Status of Traffic Police Personnel in Kolkata, India" Air 2, no. 1: 1-23. https://doi.org/10.3390/air2010001
Article Metrics
Article access statistics, supplementary material.
ZIP-Document (ZIP, 112 KiB)
Further Information
Mdpi initiatives, follow mdpi.
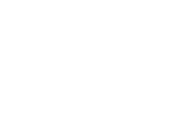
Subscribe to receive issue release notifications and newsletters from MDPI journals
You are here
Rfa 23-1, assessing health effects of traffic-related air pollution in a changing urban transportation landscape.
RFA 23-1 is seeking to fund studies to assess health effects of long-term exposure to TRAP. Studies should propose novel or improved methods and approaches to evaluate exposure to and health effects of traffic-related air pollutants as technologies and fuels change, the fleet turns over, mobility transforms, and electrification makes greater inroads.
SPECIFIC OBJECTIVES
RFA 23-1 seeks to fund studies that can accomplish at least one of the objectives listed below. Note that in meeting the first three objectives, investigators should consider whether their work can effectively include effects in marginalized communities in high-income countries.
- In the proposed health studies, develop, validate, and apply novel or improved exposure assessment methods suitable for estimating exposures to traffic-related air pollutants that (1) account for other air pollution sources in urban areas (such as airports, (sea)ports, industries, and other local point sources), (2) could distinguish between tailpipe and non-tailpipe traffic emissions, to the maximum extent possible, and (3) take into consideration the overall impact of (new) transportation and mobility trends on air quality and exposure.
- Evaluate the effectiveness of key measures to reduce TRAP and improve public health, as well as to assess the health benefits of measures designed to mitigate traffic or achieve other policy objectives.
- Estimate the impacts on urban air quality and health of various new transportation and mobility scenarios, including a baseline (status quo or "business as usual") scenario.
- Investigate health effects of long-term exposure to TRAP in understudied low- and middle-income countries.
STUDY DURATION AND BUDGET GUIDELINES
Overall, a total of $5 million will be available for this RFA. HEI expects to fund a small number of studies (2 to 3 years in duration).
Please consult the Instructions and Forms and FAQs pages.

Hanna Boogaard
Ongoing studies funded under this RFA
An agent-based model for evaluating long-term impacts of trap on disadvantaged communities under multiple scenarios.
The University of British Columbia, Canada
This project will link and extend several models to create a framework for full-chain assessment of transportation systems and impacts of traffic-related air pollution on population health in the San Francisco Bay Area. The investigators will evaluate impacts of three policy scenarios — federal/California long-term transportation electrification, telecommuting impact from COVID-19, and a community-led scenario — on ambient air pollution and associated mortality and morbidity outcomes, including environmental justice disparities.
Traffic‐related air pollution, lipoproteins, and cardiovascular disease risk in the VITamin D and OmegA‐3 TriaL (VITAL)
Harvard T.H. Chan School of Public Health
This project will use an existing study (VITAL) to examine both cross‐sectional and longitudinal associations of traffic-related air pollution with a panel of standard lipid risk factors and novel apolipoprotein and lipoprotein subfractions, and with incident cardiovascular disease events, and explore potential mediating pathways of risk.
- Open access
- Published: 04 November 2013
Mobile phone tracking: in support of modelling traffic-related air pollution contribution to individual exposure and its implications for public health impact assessment
- Hai-Ying Liu 1 ,
- Erik Skjetne 2 &
- Mike Kobernus 1
Environmental Health volume 12 , Article number: 93 ( 2013 ) Cite this article
6796 Accesses
4 Altmetric
Metrics details
We propose a new approach to assess the impact of traffic-related air pollution on public health by mapping personal trajectories using mobile phone tracking technology in an urban environment. Although this approach is not based on any empirical studies, we believe that this method has great potential and deserves serious attention. Mobile phone tracking technology makes it feasible to generate millions of personal trajectories and thereby cover a large fraction of an urban population. Through analysis, personal trajectories are not only associated to persons, but it can also be associated with vehicles, vehicle type, vehicle speed, vehicle emission rates, and sources of vehicle emissions. Pollution levels can be estimated by dispersion models from calculated traffic emissions. Traffic pollution exposure to individuals can be estimated based on the exposure along the individual human trajectories in the estimated pollution concentration fields by utilizing modelling tools. By data integration, one may identify trajectory patterns of particularly exposed human groups. The approach of personal trajectories may open a new paradigm in understanding urban dynamics and new perspectives in population-wide empirical public health research. This new approach can be further applied to individual commuter route planning, land use planning, urban traffic network planning, and used by authorities to formulate air pollution mitigation policies and regulations.
Peer Review reports
According to the United Nations (UN), more than 50% of the world’s population lives in urban areas and this number will increase to 70% by 2050 [ 1 ]. Road traffic is the main source of pollution in well-developed urban regions worldwide. Traffic-related air pollution may have diverse impacts on health including exacerbation of asthma, onset of childhood and adult asthma, non-asthma respiratory symptoms, impaired lung function, total and cardiovascular mortality, and cardiovascular morbidity, hospitalizations, various cancers, birth outcomes, diabetes and life expectancy [ 2 ], etc. Based on a synthesis of the best available evidence, the HEI (Health Effects Institute) identified that an exposure zone within a range of up to 300 to 500 metres from a major road as the area most highly affected by traffic emissions [ 2 ]. According to the United States Environmental Protection Agency (U.S. EPA) [ 3 ], more than one-third of Nitrogen Oxides (NOx) and one-quarter of Particulate Matter (PM) emissions in the U.S. are from roadways, and about 45 million Americans live within 100 metres of a road. A study by researchers at the University of Southern California (USC) and the California Air Resources Board found that up to half of Los Angeles’ residents total exposure to ultrafine air pollutants occurs while people are travelling in their vehicles [ 4 ]. It has frequently been recognised that car drivers are highly exposed to airborne pollution [ 5 , 6 ], and are at an increased risk of developing several types of cancer [ 7 ]. A report by the World Health Organization (WHO) showed that long-term air pollution from traffic in Austria, France and Switzerland triggered 300,000 additional cases of bronchitis in children, 15,000 hospital admissions for heart disease and a further 162,000 asthma attacks in children [ 8 ].
Different methods have been used to estimate exposure of participants to urban traffic-related air pollution in previous studies. Exposure of participants is often estimated by the concentration either measured at the monitoring site nearest to the participants’ residential address [ 9 , 10 ], or generated by applying dispersion model [ 11 , 12 ] or Land Use Regression (LUR) techniques to the participants’ residential address [ 13 , 14 ], and by accounting for indicators of traffic and other factors such as geospatial location, meteorology and nearby urbanization. Dispersion models can provide information that satisfies both the spatial coverage and temporal variation, but good performance from dispersion modelling requires good input data. In the approach of LUR models it is relatively simple to interpolate a limited number of measurements to a larger population [ 15 ]. However, both approaches do not account for personal activities such as time spent in traffic and activity level which will influence exposure considerably [ 16 ].
Methods accounting for personal movement include the Personal Environmental Impact Report (PEIR) [ 17 ]. A platform that uses location data sampled from everyday mobile phones to calculate personalized estimates of environmental impact and exposure was presented in 2009 [ 18 ]. The authors also proposed using mobile phones with a built-in GPS (Global Positioning System) receiver and an accelerometer for identifying individual transportation modes including whether an individual is stationary, walking, running, biking, or in motorized transport [ 19 , 20 ]. This was further explored in the EU-FP7 project ENVIROFI, where the Personal Environmental Information System (mobile phone based application) provided accurate air quality data for the user’s current location based on the GPS coordinates supplied by the user’s phone [ 21 ]. The CitiSense project [ 22 ] had a mobile air quality system that enabled users to track their personal air quality exposure [ 23 , 24 ]. The above studies did not target population level exposure. Studies using mobile air pollution sensors are only active on a relatively small number of vehicles for which the estimated pollution levels will be derived. Through this brief review of the health effects of traffic, we identified: (i) exposure to traffic-related emissions may cause major adverse health effects on the population at or near air polluted roadways; (ii) existing exposure models do not accurately characterize the exposure fields; and (iii) these disadvantages in exposure estimation can attenuate health effect estimates. To address these issues, studies are needed to characterize the personal exposure to traffic-related air pollution, and to better understand the linkages between traffic-related air pollution and public health effects.
In this study, we propose a conceptual framework to improve exposure assessment by using existing, low cost mobile phone data to obtain individual trajectories to further estimate the concentration of the traffic emission in the roadway network and its contribution to exposure for the urban population. We analyse each element along the work process (Figure 1 ) from human mobility, through traffic-related air pollution modelling, to exposure and its implications for public health impact assessment. Our proposed methodology is based upon recent advances in social and information networks using Statistical Physics [ 25 ] and the techniques of Traffic Engineering in mobile phone networks [ 26 ]. The advantage of the proposed study is that we target the entire network of mobile phone users to calculate pollution levels, exposure and health effects. We believe that this has the potential to open up new perspectives in public health research.
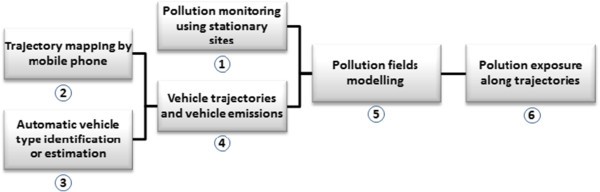
An overview of work process for modelling traffic-related air pollution contribution to individual exposure by mobile phone tracking. It is composed of six parts: ① Pollution monitoring using stationary sites; ② Trajectory mapping by mobile phone; ③ Automatic vehicle type identification or estimation; ④ Vehicle trajectories and vehicle emissions; ⑤ Pollution field modelling; and ⑥ Pollution exposure along trajectories.
The science of individual human trajectories is a new research field based on the recent availability of empirical data on human mobility. Developments in this field have been influenced by results in the multidisciplinary field of network physics [ 27 , 28 ]. Databases and technologies that capture human mobility by mobile phone traces are now available and many interesting features have been discovered including the characteristics of individual human trajectories [ 29 ], the interaction between individual mobility and social networks [ 30 ], and meso-scopic structure (i.e., the detailed substructures and spatiotemporal flows of mobility) and social aspects of human mobility [ 31 ].
Modern network physics is starting to influence public health research. For instance, Health InfoScape, part of the Massachusetts Institute of Technology (MIT) SENSEable Cities and the General Electric Company (GE), aim to create new ways of understanding human health in the U.S. [ 32 ]. By analysing data from over 7.2 million anonymous electronic medical records taken from across the U.S., they seek to uncover statistical relationships between space, geography and health.
There is a recent development in Location-Based Services (LBS) [ 33 ] through mobile phone technology. Basic mobile phone based positioning systems include: location of nearest base station; trilateration of two or more neighbouring base stations by signal strength or Time Of Arrival (TOA); multilateration (hyperbolic lateration) by Time Difference Of Arrival (TDOA); and network based triangulation by Angle Of Arrival (AOA) using multiple antennas at a base station. More methods are available with third generation mobile systems [ 34 ]. Thiragarajan et al. [ 35 – 37 ] used a Hidden Markov Model (HMM) to map noisy phone location data to road segments using mobile phone data from both the GPS and the Global System for Mobile Communications (GSM) systems. The road segments are the hidden states of the system and the noisy data is the input to the algorithm. The mobile phone data are run through the algorithm twice, first to find the mobile phone area sequence and then to find the road segment sequence [ 38 ]. Processed position data provided by mobile phones are as accurate as GPS reading intervals of 2 minutes and have much lower energy consumption [ 39 ]. According to studies on probabilistic models for mobile phone trajectory estimation from Thiagarajan [ 39 ], the misplaced road segment error is approximately only 45 metres. While, on average, mobile phone tracking can retrieve over 75% of a user’s drive accurately, even from highly inaccurate (175 metres raw position error) GSM data.
There are several companies providing individual trajectory mobile phone based traffic monitoring services including: Cellint [ 40 ], Intelligent Mechatronic Systems Inc (IMS) [ 41 ], ITIS [ 42 ], and Urban Informatics (UI) [ 43 ], etc. The car navigation company TomTom [ 44 ], has one million GPS users and uses about 80 million other data sources such as GSM to monitor real time traffic and give advice on the best route, taking into account traffic congestion. Google Maps [ 45 ] gets live traffic information displaying road way traffic conditions for main roads in many countries updated every five to ten minutes. Close to real-time traffic maps are provided by all larger search companies including Yahoo, Bing, Baidu and Yandex. For example Yandex also provide real time video cameras of selected junctions in Moscow, and traffic map forecast at 15 minutes intervals up to one hour. The Mobile Millennium project at the University of California (UC), Berkeley, in collaboration with Nokia Research Centre, and NAVTEQ Company uses GPS in cellular phones to gather traffic information, process it, and distribute it back to the phones in real time [ 46 ].
Microscopic traffic simulation [ 47 ] is a field closely related to trajectory mapping. In this context 'microscopic’ means modelling and simulation of the movement of individual vehicles. The vehicles are typically modelled taking into account the distance to the vehicles in front; this allows realistic simulation of congestion waves that move upstream with a group velocity of about 15 km / h . Several simulation models combining microscopic traffic modelling with simulation of air pollution concentrations in street canyons [ 48 – 51 ] make it feasible to calculate traffic-related air pollution by mapped personal trajectories.
This paper combines the above presented unrelated technologies to form a new approach in urban traffic-related air pollution and public health impact research. We develop a concept for air pollution research based on trajectory mapping by using an empirical model that articulate the overall work process, system architecture, traffic-related air pollution and exposure modelling. We also discuss the main challenges that might be faced by applying such an approach to study the traffic-related air pollution to individual exposure and its public health impact assessment.
Work processes and system architecture
The work process is composed of six parts. Figure 1 illustrates the key elements of the work process. Following the work process in Figure 1 , one can both construct the pollution field and estimate the pollution exposure by fixed site data from relatively high density of monitoring stations (steps 1, 5 and 6), or alternatively by mobile phone tracking (steps 2–6), or through combining both mobile phone tracking and fixed site monitoring stations (steps 1–6). The work process is described in detail below.
Pollution monitoring using stationary sites
In the suggested approach, the air pollution data can be directly gathered from a static network of pollution monitoring sites across the city and air pollution maps are generated by interpolating between them. People can then check their exposure levels by mapping their individual trajectory into the pollution map. However, there are some limitations of using static monitoring networks, i.e., mainly the total number of air quality monitoring sites within a city is often limited by practical constraints, and possibly other limitations (e.g., reporting hourly averages, possibly not at real time in some regions, etc.). As a possible complementing source of information, high spatial resolution satellite observations can be used. While satellite measurements have many advantages and are developing rapidly, they do have some major limitations, particularly when considering intra-urban resolution. Although most operational satellite products relevant for air quality applications currently do not yet possess the spatial resolution necessary to directly resolve fine spatial detail at the urban scale, their output can nonetheless be very valuable for improving the output of local and regional chemical transport models through data assimilation schemes, and as such indirectly improve urban-scale air quality estimates. Furthermore, research is currently ongoing on producing higher-resolution (300 m) satellite-based PM measurements at the urban scale [ 52 ]. Finally, significant progress with regards to spatial resolution achievable from space-based platforms will further be made in the near future with new instruments such as TROPOMI (The TROPOspheric Monitoring Instrument) on the Sentinel-5 precursor platform, which will open up entirely new applications for space-based air quality monitoring, in particular over megacities and other large urban agglomerations.
Trajectory mapping by mobile phone
Trajectory mapping uses individual human trajectories generated by advanced mobile phone GSM (GPS-free) tracking systems. The tracking system should be capable of accurately locating mobile phones on road segments in a roadway network by using traffic engineering tracking systems. From frequent location updates, it is possible to track the approximate real-time speed of the mobile phones. This technology is partly qualified, but to date has not been implemented for large populations. Computational capacity may also be a limitation as one PC calculates a one hour individual trajectory in about two minutes [ 39 ]. There are ways to use one individual trajectory for calculating other trajectories on the same road segment at approximately the same time to make the computation of a large number of trajectories feasible. Commercial algorithms are likely to be faster on commuter freeway systems where the vehicles, on average, are travelling on a small set of roadways and stay on the same roadway for quite a long time.
Automatic vehicle type identification or estimation
A mobile phone signal does not identify a vehicle. The vehicle type can be estimated by drawing randomly or conditioned to trajectories such as bus stop patterns to identify that it is a bus from a national vehicle type distribution. Modern Automatic Vehicle Identification (AVI) systems cover or will soon cover most vehicles in a country such as Autopass [ 53 ] in Norway, SINIAV in Brazil [ 54 ], and EVR in Mexico [ 55 ]. These systems are based on Radio Frequency Identification (RFID) [ 56 ] and give the exact date and time for passing a certain check point. Cross correlation of AVI and mobile phone data results in one-to-one assignment of a mobile phone to an identified vehicle. The vehicle type, age, etc., will determine its emission class which is a key to building up a picture of emissions.
Vehicle trajectories and vehicle emissions
Individual human trajectories can be further used to generate vehicle trajectories on road segments in a roadway network by using traffic engineering tracking systems. Emissions from each individual vehicle can be calculated along the vehicle trajectories.
Pollution field modelling
Traffic pollution is not just a product of vehicle exhaust, but also tyre wear, brakes wear, oil leaks, as well as erosion of the road surface itself and deposited sand and salts at icy/snowy winter roads. Dispersion of traffic air pollution in urban areas can be modelled numerically by taking into account the recirculation effect of street canyons, meteorological conditions, pollution type, traffic counts and types, and other parameters. In this case, various dispersion models (e.g., CALINE3/4 [ 57 ] and CAL3QHC/R [ 58 ]), CFD (Computational Fluid Dynamics) models [ 58 , 59 ], geo-statistical interpolation models and hybrid models that are specially developed for or simply used in street canyon applications can be applied. To realize this, an increase in computational power is needed, especially if the model is run over long time periods of weeks and even months; and/or to model air pollution level on a street canyon for an entire city could also be computational intensive. Moreover, there is a need for high resolution input data for the models about the meteorology in the city, including temperature and wind speed and directions.
Pollution exposure along trajectories
Exposure data can be acquired by evaluating the pollution field along the trajectories in space and time. In this case, a micro-environment based exposure model can be applied [ 60 ].
Mobile phone-based mobility tracking used to model traffic pollution contributions to both individual chronic and acute exposure can open new fields of research. This approach can be further developed to estimate the accumulated dose of air pollutants in the human body, and to assess the health impact by coupling individual trajectories to individual physiological information (e.g., age, gender, inhalation rate, body length and weight, health records, etc.) [ 61 , 62 ]. Field studies with personal exposure monitors have found that traditional exposures estimated from average pollution levels in a city based upon residence postal codes are inadequate for pollutants which are spatially variable [ 16 ] and tend to underestimate variability in exposure, in particular when a person’s location is highly correlated to narrow peaks in the pollution field. The trajectory based system proposed in this paper will pick up such correlations. Therefore, the individual trajectory approach would be expected to more accurately identify the individuals with the greatest health risk. The most highly exposed groups may share common trajectory patterns, for example those with commuter/work patterns that have the highest health risk (e.g., professional drivers, traffic police and construction workers, etc.). By coupling mobile phones to electronic health records (EHR) and/or personal health records (PHR), one may identify highly vulnerable/pollution-sensitive groups (for example those with respiratory diseases) among the most risky trajectory patterns. Thus, this may open up new perspectives in empirical public health research.
Figure 2 illustrates the potential to characterize traffic-related microenvironments, e.g., in-vehicle exposure in congestion as opposed to non-congestion: (a) mapping a mobile phone trajectory; (b) associating mobile phone with vehicles, and linking vehicles to air pollution; and (c) estimating exposure for people travelling through the pollution fields [ 63 ]. It needs to be noted that the mobile phone is used to identify the person and a person-related vehicle.
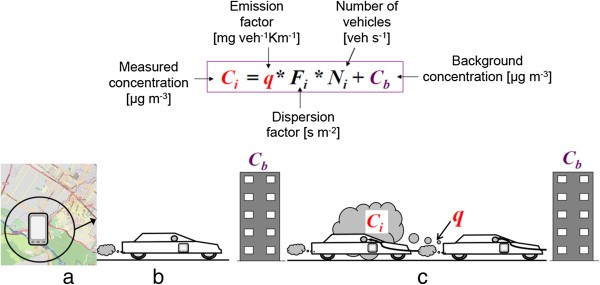
The potential to characterize traffic-related microenvironments, e.g., in-vehicle exposure in congestion as opposed to non-congestion. (a) Mapping a mobile phone trajectory; (b) Associate mobile phones with vehicles, and associate vehicles to air pollution which enables construction of a continuous air pollution field in space and time; (c) Estimate exposure for those people who travelling through the pollution field (the equation is from Reference No. 89).
Figure 3 illustrates the system architecture of a complete system following the work process in Figure 1 . On the Information Communication Technology (ICT) infrastructure part, the raw data are the mobile phone base stations in communication with individual mobile phones. These raw data are used to efficiently generate individual mapped trajectories showing the positions of individual mobile phones through time. Mapping algorithms are used to locate the moving positions onto road segments. The mapped trajectories are used as input to algorithms to construct the pollution field and estimate exposure for people moving in the pollution field.
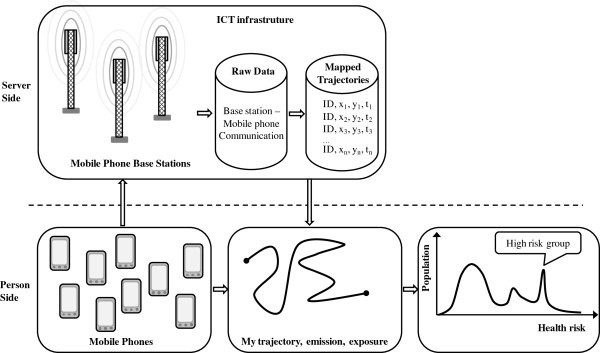
System architecture for obtaining mapped trajectories and public health impact. It consists of a two-way communication: server side (mobile phone base stations – raw data – mapped trajectories) and person side (mobile phone – individual trajectory, emission, exposure - health effect).
At the population-scale one may investigate high risk groups and check if they share common travel patterns. Note that on the lower right graph in Figure 3 the term 'population’ indicates the population density or frequency in a population histogram. This may be used to identify the population fraction (i.e., area below the curve) and the risk of the high risk group.
Existing traffic air pollution exposure models
The goal of this section is to review some modelling approaches that can be used in this proposed approach, to calculate the traffic-related air pollution and its contribution to individual exposure. Each given model is kept simple and current at a conceptual level, so that each model can be replaced by more advanced models in specific studies.
Dispersion models
A simplifying concept of a roadway air pollution model is to treat the emission from many vehicles as one line source. The pollution is dispersed away from the line source to the entire model domain (and not just near roads) by the mechanisms of diffusion and convection (move with the local velocity of the air). However, due to the dispersive spreading the highest pollution levels are found at the line source. For simpler, roadway-only sources, the CALINE3/4 [ 58 ] and CAL3QHC/R [ 58 ] series of dispersion models explicitly address traffic-induced emissions. AERMOD is also broadly used for traffic-related complex source mixtures, e.g., terminals, ports and roadways considered in combination, large highway corridors and urban scale modelling [ 58 ].
Geo-statistical interpolation models
Geo-statistical interpolation models can be implemented to estimate the traffic-related air pollution based on a statistical interpolation of a dense, well-distributed, monitoring work [ 64 ]. These models allow the estimation of pollution concentrations over several time intervals, but this is limited only by the number of available measurement periods [ 64 ].
Hybrid models
The hybrid models are those that combine personal or regional monitoring with other air pollution exposure methods [ 64 ]. Most studies were conducted in European cities, and in San Diego [ 65 ], e.g., by using personal exposure measurements in conjunction with fixed outdoor stations to estimate hourly exposure for each day, to calculate a traffic-density index [ 66 ]; and by using regional monitoring in conjunction with another modelling scheme to assume long-term exposure to NO 2 and Black Smoke (BS) [ 67 ], etc.
Essential factors to estimate traffic air pollution exposure in the mobile phone tracking approach
No matter which of the above given models is applied, in order to calculate real-time varying traffic-related air pollution, the methods for estimating the traffic emission, emission rates, emission factors, traffic flows, and individual exposure to dynamic traffic air pollution need to be addressed explicitly.
Estimation of traffic-related air pollution
The roadway air dispersion models are usually used to estimate traffic-related air pollution. It generally relies on Gaussian plume equation [ 68 ], including wind speed, wind direction, traffic wakes and meteorological air stability.
Many tools have been developed to estimate the vehicle emission factors covering a broad range of pollutants and allow multiple scale analysis, such as the U.S. EPA MOVES (Motor Vehicle Emission Simulator) [ 69 ] for HC, NOx, CO, gaseous SO2, ammonia, direct PM; the UK Defra (Department of environment, food and rural affairs) EFT (Emission factor toolkit) Version 5.2 for NOx, PM10, PM2.5, CO2 and Hydrocarbons [ 70 ]; and the HBEFA (The Handbook Emission Factors for Road Transport) for atmospheric emission factors for regulated and important non-regulated pollutants from road vehicles in Germany, Austria, Switzerland, Sweden, Norway [ 71 , 72 ]; and the EMFAC (EMission FACtors) for HC, CO, NOx, CO2, PM, fuel consumption, oxides of sulphur (SOx), and lead (Pb) developed and used in California [ 73 ], etc.
Estimation of traffic flows
One challenge in roadway air dispersion modelling is the transformation from a Lagrangian formulation [ 74 ] where each vehicle is a particle with a given speed to an Eulerian formulation [ 75 ] where each road segment has a vehicle density with a local flow velocity. This particular challenge has been investigated by the UC Berkeley Mobile Millennium project [ 72 ] and an Eulerian–Lagrangian cell transmission model for air traffic flow has been developed [ 76 ]. In traffic flow conditions, the two most important densities are the critical density ( n c ) and the traffic jam density ( n jam ). The maximum density achievable under free flow is n c , while the minimum density achieved under jamming congestion is n jam . In general, traffic jam density is about seven times the critical density. Traffic is, however, a complex phenomenon that is described by physics concepts such as synchronization and self-organized criticality (SOC). Kerner [ 77 ] divided traffic flow into three phases from low to high density: 1) free flow, 2) synchronized congested flow, and 3) wide moving jam (wide jam that moves upstream).
The traffic density-interaction between the vehicle wakes (i.e., isolated vehicles, interacting vehicle wakes, strong interacting vehicle wakes) have been studied by Gokhale and Khare [ 78 ], and their results showed that in 'unstable’ conditions, the Vehicle Wake Factor (VWF) varies between 1.63 and 0.3 (for wind direction, θ = 90°) and 2.5 and 0.8 (for wind direction, θ = 180°). During 'neutral’ and 'stable’ conditions, it is in the range of 0.84 – 0.4, 1.91 – 0.85 (for wind direction θ = 270°) and 1.7 – 0.7, 3.1 – 0.3 for wind direction θ = 360°, respectively.
Not all citizens carry a mobile phone and this makes the mapped population an incomplete set of mapped persons/vehicles. One may fill in these gaps using statistical distributions. For simplicity, we assume 100% mobile phone coverage, that is, each individual has a mobile phone that is tracked. Linking individual trajectories to vehicles can be done as follows: (i) Identify bus drivers and passengers by their characteristic trajectory pattern. They follow a pre-defined route with a given stopping pattern. It is important to correctly estimate the number of persons travelling by bus in order to avoid over estimating the number of vehicles. (ii) Identify private car commuters by their regular trajectory patterns from the suburb to the city, and vice versa . (iii) Identify other group travellers (e.g., subway commuters, pedestrians, motorcycles, bicycles riders) by having an identical trajectory. This is to avoid overestimating the number of vehicles with passengers. (iv) The rest of the trajectories are individual drivers and their vehicles may be stochastically drawn from the vehicle type (e.g., electric car, truck or lorry) including the vehicle age, probability density function.
The road network is discretized into piecewise linear road segments. The pollution from each segment is modelled by the linear source equation. The pollutant concentration for people on the road segment is given by the characteristic mid-segment pollution. The vehicle densities (number per length) are estimated by the procedure above. The average speed of traffic in the segment is calculated by the trajectory data (∆X,∆Y,∆t) and ∆Z from terrain/map data.
If the road is a freeway with many lanes one may consider splitting the two driving directions into two line sources and sum the pollution from the two directions taking into account the centre-to-centre distance between the lane directions. For near roadway exposure one may select bands parallel to the roadway network and take into account overlapping contributions from the nearest neighbouring road(s). Traffic also contributes to background pollution, and this may be calculated by longer term smoothing of the total city pollution from stationary sites’ data.
To model individual exposure to traffic air pollution
Human exposure refers to an individual’s contact with a pollutant concentration. Given a close to real-time continuous pollution field, it is straightforward to calculate the direct exposure to air pollution in the transport network. In this context, a microenvironment (e.g., the interior of a car/bus, inside a house, or urban, suburban, and rural areas, etc.) based integrated exposure model can be applied and expressed as [ 60 ]:
Where X i is the total exposure for person i over the specified period of time, C j is the pollutant concentration in microenvironment j , t ij is the residence time of the person i in microenvironment j , and J is the total number of microenvironments. The total exposure is, therefore, the sum of exposures during a given time and has thus the same dimension as exposure in general: (mass) × (time) / (volume) or (concentration) × (time), for example, with units μg d m –3 .
In the case where M persons are exposed to the same concentration for the same time, the exposure in this case is M times that for one person and, for example, with the units (concentration) × (time). The resulting population exposure may then be used to relate the number of hospital admissions to exposure.
A constraint for using the microenvironment based exposure models (see equation above) is that the residence time of the person (termed the time–activity pattern) needs to be known together with the pollution concentrations in each of the microenvironments at the time when the person is present. In this article, however, by using mobile phone tracking we could get both individual time-activity pattern and real time continuous pollution concentration along the traffic network.
Two alternatives to an individual trajectory based system are: (1) one may measure real-time air pollution by large-scale implementation of a Wireless Sensor Network (WSN) [ 79 – 82 ], in which hundreds or thousands of small personal environmental sensors carried by the public rely on cell phones to shuttle information to central computers where it will be analysed, made anonymous and reflected back to individuals, authorities and public health agencies [ 83 ]; and (2) one may rely on stationary based, monitored pollution data together with geo-statistical models and use GPS information [ 84 – 86 ] provided by smart phone applications to track air pollution exposure of a sub-population [ 87 – 89 ] as mentioned earlier in this article. Alternative (1) uses individual trajectories. One may expect that an actual measurement of the air pollution exposure by WSN would be somewhat better than a calculated individual trajectory based system as proposed in this article. This is a point in favour of the WSN-based micro sensor approach. However, the cost of equipping a population with environmental micro sensors limits this method to small-scale studies (Table 1 ). Alternative (2) for an entire city would require stationary measurements at nearly all road segments in the city. Although this could provide high quality data, it is certainly extremely costly due to the extremely high density of measurement stations. It scales with the size of the road network as shown in Table 1 . The cost of up-scaling a mobile phone trajectory system is only the cost of incremental computational power. This is very low since the individual data already exists and can be uploaded from the mobile phone or downloaded from the mobile phone service provider. Less computational challenging studies have already included six million phone users of a Call Detailed Records (CDR) data base [ 90 ]. The detailed traffic trajectories need more computational power, but the algorithms are cost-efficient and are likely very well suited for parallel algorithms, since each trajectory is calculated independently of the other trajectories. Trajectory based data have many opportunities for reducing costs by building on synergies with commercial traffic monitoring systems. A commercial traffic monitoring system may add a health impact service that not only optimizes commuting routes based on the time spent from home to work, but may also propose alternative eco-driving routes that have a positive impact on one’s personal health.
Traffic monitoring companies, including the largest search engine companies, may want to show their social responsibility by releasing their data for public health research in the same way as mobile phone tracking data are released for human mobility research. In this way the public health research could be obtained at almost no extra cost. More realistic, we believe that traffic monitoring companies will offer new free services to calculate real time pollution exposure and health risk maps in large cities that can be used by traffic map users with GPS smart phones. We have developed a model to estimate traffic emissions from traffic map data. This modelling is planned to be published in another article. Based on this approach a mobile phone tracking system has the potential of being highly competitive economically for population-wide traffic pollution health studies.
Trajectory based individual exposure analysis opens up new perspectives in public health research. Instead of analysing either the average health risk given by average city pollution levels or analysing a limited number of citizens’ mobility, we are now in a position to analyse the probability distribution density of individual risk for relevant diseases. Furthermore, by linking to HER and/or PHR, the proposed approach can help to better understand the impact of environmental exposure on human health at both individual and population levels, and might enhance environmental public health tracking as well [ 91 – 93 ]. Natural questions that arise include: Who is most at health risk? Is it the large majority of the population with moderate pollution exposure or a small group of highly sensitive/vulnerable persons with unusual exposure trajectories? A natural extension of the trajectory based individual exposure analysis is to do research on optimal regulatory, policy change and urban planning to reduce air pollution. What are the most efficient ways to transform an existing urban environment into a city with a low air pollution health risk?
In polluted cities there are many different options for improving air quality. One may develop trajectory based dynamic computer simulation models where urban planning concepts are evaluated based on simulated performance with regard to cost and improved public health. This approach is similar to dynamic petroleum reservoir simulation by streamlines [ 94 ].
A vision for mobile phone tracking technology is to do global studies that incorporate the global gaps in population of mobile phone users. The gaps in the network, that is, those that do not have a mobile phone, may be inferred directly from the trajectories from home to kindergarten, to school, to work, and scale this number of trajectories to estimate the effect from the whole population. An advantage of this approach is that it is effortless for citizens as the global population does not need to adjust their activity pattern or wear any measurement devices to participate in the study.
There are some limitations to our approach that need to be addressed when used in real practice. First, the proposed approach is mainly targeted at the person who has a mobile phone. This is a limitation for those people that do not tend to have mobile phones (e.g., children, elderly, the poor, etc.). The biased samples are not easily worked out with statistical methods if they are not randomly distributed, which is the case here. Second, the risks arise first from the fact that in order to get the individual human trajectory, the data we need is mostly about people, where they have been, at what times, how often. Therefore privacy and security is a major concern, and needs to be addressed before the opportunities of trajectory mapping can be created. Furthermore, there are also huge issues with privacy and access to link to HER and/or PHR. Encryption and anonymization can provide a level of privacy [ 95 ]. In this study, anonymous data are enough to construct individual human trajectories, pertaining to groups of people. However, to guarantee anonymity is hard in the domain to link/compare the spatio-temporal individual trajectory based exposure-dose modelling with real medical patient data. Many studies that address privacy issues in a spatio-temporal data mining context have already been published. In the Geographic Privacy-Aware Knowledge Discovery and Delivery (GeoPKDD) project, Giannotti and Pedreschi [ 96 ] investigated the various scientific and technological issues of mobility data, open problems, roadmap, and concluded that the privacy issues related to the ICTs can only be addressed through an alliance of technology, legal regulations and social norms. In the meantime, increasingly sophisticated privacy-preserving data mining techniques are being studied and further developed [ 97 ]. Their aim is to achieve appropriate levels of anonymity by means of controlled transformation of data and/or patterns – limited distortion that avoids the undesired side effect on privacy while preserving the possibility of discovering useful knowledge. The project Citisense designed a permissioning system that allows users to configure publicly accessible time Windows of their data [ 98 ]. Mun et al. [ 18 ] suggested a selective sharing and hiding mechanism in PEIR that enable users to share their location traces with people they trust, and generates believable proxy traces for times a user does not want their real location revealed. Furthermore, an alternative to deal with privacy issues related to tracking of individual trajectories is to collect a population-wide data in the form of willing participation, as done by PEIR [ 17 , 18 ] mentioned above, where only individuals that are motivated will take part in the data collection. In this approach, the methods to empower citizens at population level to participate in such studies may need to be further developed.
Another limitation of using mobile phone tracking approach to study individual exposure to traffic-related air pollution and its public health impact assessment is that the external data/information and models needed, such as vehicle type identification, emission dispersion models, emission factors and meteorology data needs to be known together with the vehicle trajectories to model pollution fields; individual physiological information (e.g., height, weight, inhalation rate) is needed in each of the microenvironments at the time when the person is present in order to estimate the accumulated dose in the human body, etc. So this approach also suffers from similar data problems to the more conventional dispersion models. On the other hand, such challenges may indicate that the approach of modelling traffic-related air pollution and its implications for public health impact assessment through mobile phone tracking will require broad cooperation within inter- and multidisciplinary disciplines.
We conclude that there is an opportunity to calculate traffic air pollution and its public health impact by mobile phone trajectory mapping. This approach is promising due to the following characteristics: (i) Low cost : There is no cost from the observation part. The cost is mainly in data power to compute mobile phone trajectories and emission dispersion modelling in real time. Combination of low cost and standardized mobile phone data makes it is feasible to do population-wide traffic pollution studies. The system is suited for developing countries that have a low fraction of mobile phones with GPS. (ii) Near real-time : The system is based on fully automatic calculation and real-time data. One may obtain real-time pollution/exposure fields. This allows identification of high vehicle density congestion events that may contribute significantly to health risk (iii) Effortless citizen participation : Each trajectory reflects one individual citizen. Therefore, this system opens up the possibility of providing information to each individual citizen. One may develop applications for automatic web server feedback to citizens who want to get the data of their own trajectories or want to contribute toward a training data set of true ground GPS measurements. We believe that using the trajectories of individual mobile phones opens up new perspectives on urban dynamics and public health research.
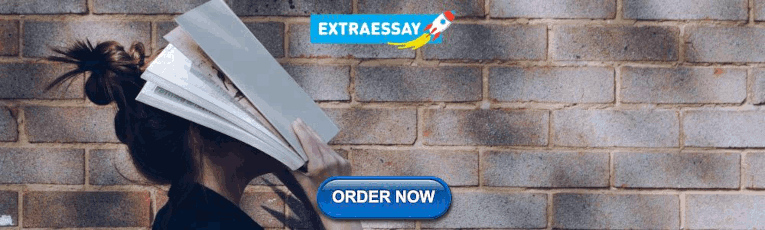
Abbreviations
Angle of arrival
Aerosol optical depth
Automatic vehicle identification
Call detailed records
Adaptive services for community-driven behavioural and environmental monitoring to induce change
Carbon oxides
Emission factors toolkit
Electronic health records
EMission FACtors
GEOS aerosol/smoke product
The general electric company
Geographic privacy-aware knowledge discovery and delivery
Geostationary operational environmental satellite
The global positioning system
The global system for mobile communications
The handbook emission factors for road transport
Hydrogen carbon
Hidden markov model
Information communication technology
Intelligent mechatronic systems inc
Land use regression
Location-based services
The massachusetts institute of technology
Moderate resolution imaging spectro-radiometer
Motor vehicle emission simulator
Nitrogen dioxides
Nitrogen oxides
Ozone monitoring instrument
The personal environmental impact report
Personal health records
Particulate matter
Radio frequency identification
Sulphur dioxides
Oxides of sulphur
Time difference of arrival
Time of arrival
The TROPOspheric Monitoring Instrument
Total suspended particulates
University of California
Urban informatics
The United Nations
The University of Southern California
The United State environmental protection agency
Vehicle wake factor
The World Health Organization
Wireless sensor network.
UN report: World’s biggest cities merging into 'mega-regions’. [ http://www.guardian.co.uk/world/2010/mar/22/un-cities-mega-regions ]
HEI Panel on the Health Effects of Traffic-Related Air Pollution: Traffic-related air pollution: a critical review of the literature on emissions, exposure, and health effects. 2010, Boston, Massachusetts: Special Report 17
Google Scholar
Padmanabhan N, Glenn B: EPA research focus – health effects of near-roadway air pollution. [ http://pubs.awma.org/gsearch/em/2009/8/padmanabhan.pdf ]
Fruin S, Westerdahl D, Sax T, Sioutas C, Fine PM: Measurements and predictors of on-road ultrafine particle concentrations and associated pollutants in Los Angeles. Atmos Environ. 2008, 42: 207-219. 10.1016/j.atmosenv.2007.09.057.
Article CAS Google Scholar
Rank J, Folke J, Jespersen PH: Differences in cyclists and car drivers’ exposure to air pollution from traffic in the city of Copenhagen. Sci Total Environ. 2001, 279: 131-136. 10.1016/S0048-9697(01)00758-6.
van Wijnen JH, Verhoeff AP, Jans HW, van Bruggen M: The exposure of cyclists, car drivers and pedestrians to traffic-related air pollutants. Int Arch Occup Environ Health. 1995, 67: 187-93.
Soll-Johanning H, Bach E, Olsen JH, Tuchsen F: Cancer incidence in urban bus drivers and tramway employees: a retrospective cohort study. Occup Environ Med. 1998, 55: 594-598. 10.1136/oem.55.9.594.
WHO: More die from car pollution than road accidents. [ http://www.ibike.org/environment/air-pollution.htm ]
Pope CA, Dockery DW: Health effects of fine particulate air pollution: lines that connect. J Air Waste Manage Assoc. 2006, 56: 709-742. 10.1080/10473289.2006.10464485.
Halonen JI, Lanki T, Yli-Tuomi T, Kulmala M, Tiittanen P, Pekkanen J: Urban air pollution and asthma and COPD hospital emergency room visits. Thorax. 2008, 63: 574-476. 10.1136/thx.2008.096073.
Article Google Scholar
Sørensen M, Hoffmann B, Hvidberg M, Ketzel M, Jensen SS, Andersen ZJ, Tjønneland A, Overvad K, Raaschou-Nielsen O: Long-term exposure to traffic-related air pollution associated with blood pressure and self-reported hypertension in a Danish cohort. Environ Health Perspect. 2012, 120: 418-24. 10.1289/ehp.1103631.
Raducan GM: Pollutant dispersion modelling with OSPM in a street canyon from Bucharest. Romanian Report in Physics. 2008, 60: 1099-1114.
CAS Google Scholar
Clougherty JE, Wright RJ, Baxter LK, Levy JI: Land use regression modelling of intra-urban residential variability in multiple traffic-related air pollutants. Environ Health. 2008, 7: 17-10.1186/1476-069X-7-17.
Henderson SB, Beckerman B, Jerrett M, Brauer M: Application of land use regression to estimate long-term concentrations of traffic-related nitrogen oxides and fine particulate matter. Environ Sci Technol. 2007, 41: 2422-2428. 10.1021/es0606780.
Marshall JD, Nethery E, Brauer M: Within-urban variability in ambient air pollution: comparison of estimation methods. Atmos Environ. 2008, 42: 1359-1369. 10.1016/j.atmosenv.2007.08.012.
Dijkema M, Traffic Related Air Pollution: PhD thesis. Spatial Variation, Health Effects and Mitigation Measures. 2011, Utrecht University: Institute for Risk Assessment Sciences
UCLA CENS: PEIR: personal environmental impact report. [ http://www.viewingspace.com/peir.html ]
Mun M, Reddy S, Shilton K, Yau N, Burke J, Estrin D, Hansen M, Howard E, West R, Boda P: PEIR, the personal environmental impact report, as a platform for participatory sensing systems research. Proceedings of MobiSys 2009: the 7th international conference on mobile systems, applications and services: 22–25, June, 2009; Wroclaw, Poland. Edited by: Zielinski K, Wolisz A, Flinn J, LaMarca A. 2009, New York, USA: ACM, 55-68.
Reddy S, Mun M, Burke J, Estrin D, Hansen M, Srivastava M: Using mobile phones to determine transportation modes. ACM Transactions on Sensor Networks (TOSN). 2010, 6 (13): 1-13.
Stenneth L, Wolfson O, Yu PS, Xu B: Transportation mode detection using mobile phones and GIS information. Proceedings of GIS '11: the 19th ACM SIGSPATIAl international conference on advances in geographic information systems: 1–4, November, 2011; Chicago, IL, USA. Edited by: Agrawal D, Cruz I, Jensen CS, Ofek E, Tanin E. 2011, New York, USA: ACM, 54-63.
ENVIROFI: The environmental observation web and its service applications within the future internet. [ http://www.envirofi.eu/ ]
CitiSense: Adaptive services for community-driven behavioural and environmental monitoring to induce change. [ https://sosa.ucsd.edu/confluence/display/CitiSensePublic/CitiSense ]
Pau G, Tse R: Challenges and opportunities in immersive vehicular sensing: lessons from urban deployments. Signal Process Image Commun. 2012, 27: 900-908. 10.1016/j.image.2012.01.015.
Bales E, Nikzad N, Quick N, Ziftci C, Patrick K, Griswold W: Citisense: mobile air quality sensing for individuals and communities design and deployment of the citisense mobile air-quality system. Proceedings of 6th international conference on pervasive computing technologies for healthcare (Pervasive Health): 21–24, May, 2012; San Diego, California, USA. Edited by: Chlamtac I, Mayora-Ibarra O, Varshney U, Osmani V. 2012, New York: IEEE Xplore Digital Library, 155-158.
Yeung CH, Saad D: Networking – a statistical physics perspective. J Phys A Math Theor. 2013, 46: 103001-10.1088/1751-8113/46/10/103001.
Paul U, Subramanian AP, Buddhikot MM, Das SR: Understanding traffic dynamics in cellular data networks. Proceedings of INFOCOM: 10–15 April 2011; Shanghai, P.R. China. Edited by: Gopalan K, Striegel AD. 2011, : IEEE Xplore Digital Librry, 882-890.
Chapter Google Scholar
Barabási A-L, Albert R: Emergence of scaling in random networks. Science. 1999, 286: 509-512. 10.1126/science.286.5439.509.
Barabási A-L, Albert R: Statistical mechanics of complex networks. Rev Mod Phys. 2002, 74: 47-97. 10.1103/RevModPhys.74.47.
Song C, Koren T, Wang P, Barabási A-L: Modelling the scaling properties of human mobility. Nat Phys. 2010, 10: 818-823.
Wang D, Pedreschi D, Song C, Giannotti F, Barabasi AL: Human mobility, social ties, and link prediction. Proceedings of KDD 11, the 17th ACM SIGKDD international conference on Knowledge discovery and data mining: 21–24 August 2011; San Diego, California, USA. Edited by: Chid A, Joydeep G, Padhraic S. 2011, : ACM Digital Library, 1100-1108.
Bagrow JP, Lin Y-R: Mesoscopic structure and social aspects of human mobility. PLoS One. 2012, 7: 1-6.
Girardin F, Vaccari A, Gerber A, Biderman A, Ratti C: Towards estimating the presence of visitors from the aggregate mobile phone network activity they generate. Proceedings of the 11th international conference on computers in urban planning and urban management (CUPUM2009): 16–18 June 2009; Hong Kong, P.R. China. Edited by: Yeh AGO, Zhang F. 2009, Hong Kong: University of Hong Kong, 1-11.
Wang S, Min J, Yi BK: Location based services for mobiles i: technologies and standards. [ http://blue-penguin.org/cache/location-based-services-for-mobiles.pdf ]
Wang G, van den Bosch FHM, Kuffer M: Modelling urban traffic air pollution dispersion. Int Arch Photogramm Remote Sens Spat Inf Sci. 2008, XXXVII: 153-158.
Thiragarajan A, Biagioni J, Gerlich T, Eriksson J: Cooperative Transit Tracking Using GPS-enabled Smart-phones. Proceedings of the 8th ACM conference on embedded networked sensor systems: 3–5 November 2010; Zurich, Switzerland. Edited by: Beutel J, Ganesan D, Stankovic J. 2010, New York: ACM, 85-98.
Thiragarajan A, Madden S: Querying continuous functions in a database system. Proceedings of the ACM SIGMOD international conference on management of data, SIGMOD 2008: 10–12 June 2008; Vancouver, BC, Canada. Edited by: Wang JTL. 2008, New York: ACM, 791-804.
Thiragarajan A, Ravindranath L, LaCurts K, Madden S, Balakrishnan H, Toledo S, Eriksson J: Accurate, low-energy trajectory mapping for mobile devices. NSDI’11Proceedings of 8th USENIX Symposium on Networked Systems Design and Implementation (NSDI). Edited by: USENIX Association. 2011, Boston, MA, USA: Berkeley: USENIX Association
Thiragarajan A, Ravindranath L, LaCurts K, Toledo S, Eriksson J, Madden S: VTrack: accurate, energy-aware road traffic delay estimation using mobile phones. Proceedings of the 7th international conference on embedded networked sensor systems, SenSys 2009: 4–6 November 2009; Berkeley, California, USA. Edited by: David E, Culler , Jie L, Matt W. 2009, New York: ACM, 85-98.
Thiragarajan A: Dissertation. Probabilistic models for mobile phone trajectory estimation. 2011, : Massachusetts Institute of Technology, Department of Electrical Engineering and Computer Science
Cellint: Cellient traffic solutions making it work for you. [ http://www.cellint.com ]
IMS: Connected car solutions for life. [ http://www.intellimec.com ]
ITIS: Integrated transport information system. [ http://www.itis.com.my/atis/index.jsf ]
UI: Urban informatics. [ http://www.uinformatics.com ]
TOMTOM. [ http://www.tomtom.com/en_gb/localechange.jsp ]
Google Maps. [ https://maps.google.com/ ]
Herrera JC, Work D, Ban X, Herring R, Jacobson Q, Bayen A: Evaluation of traffic data obtained via GPS-enabled mobile phones: the mobile century field experiment. Transportation Research C. 2010, 18: 568-583. 10.1016/j.trc.2009.10.006.
Treibner M, Kresting A: An open source microscopic traffic simulator. IEEE Intell Transp Syst Mag. 2010, 2: 6-13.
Khalesian M, Pahlavani P, Delavar MR: GIS-based multi-agent traffic micro simulation for modelling the local air pollution. Int Arch Photogramm Remote Sens Spat Inf Sci. 2008, XXXVII: 491-496.
López-Neri E, Ramírez-Treviño A, López-Mellado E: A modelling framework for urban traffic systems microscopic simulation. Simul Model Pract Theory. 2010, 18: 1145-1161. 10.1016/j.simpat.2009.09.007.
Nökel K, Schimdt M, van Vuren T: An integrated dynamic traffic simulation and air pollution decision support system. Proceedings of 8th world conference on transport research; volume 2: planning, operation, management and control: 12–17 July 1998; Antwerp, Belgium. Edited by: Meersman H, van de Voorde E, Winkelmans W. 1999, Victoria: ARRB Group Limited, 237-250.
Vardoulaki S, Fisher BEA, Pericleous K, Gonzalez-Flesca N: Modelling air quality in street canyons: a review. Atmos Environ. 2003, 37: 155-182. 10.1016/S1352-2310(02)00857-9.
Glantz P, Kokhanovsky A, von Hoyningen-Huene W, Johansson C: Estimating PM2.5 over southern Sweden using space-borne optical measurements. Atmos Environ. 2009, 43 (36): 5838-5846. 10.1016/j.atmosenv.2009.05.017.
AutoPASS. [ http://www.autopass.no ]
Siniav. [ http://siniav.net ]
EVR. [ http://www.electronicvehicleregistration.com ]
RFID. [ http://en.wikipedia.org/wiki/Radio-frequency_identification ]
Bailey C: Dispersion modelling for mobile source applications. [ http://www.cleanairinfo.com/regionalstatelocalmodelingworkshop/archive/2009/presentations/06%20Thurs%20AM/Bailey_Dispersion%20Modeling%20for%20Mobile%20Source%20Applications.pdf ]
Wang YJ, Zhang KM: Modelling near-road air quality using a computational fluid dynamics model, CFD-VIT-RIT. Environ Sci Technol. 2009, 43: 7778-7783. 10.1021/es9014844.
Vardoulakis S, Fisher BEA, Pericleous K, Gonzalez-Flesca N: Modelling air quality in street canyons: a review. Atmos Environ. 2003, 37: 155-182. 10.1016/S1352-2310(02)00857-9.
Time micro-environment activity modeling. [ http://www.integrated-assessment.eu/node/204 ]
U.S. EPA: Exposure factors handbook 2011 edition (Final). 2011, Washington, DC:
U.S. EPA report: Extrapolation of the benzene inhalation unit risk estimate to the oral route of exposure. http://www.epa.gov/iris/supdocs/benzsup.pdf .
Belalcazar LC, Clappier A: An alternative technique to estimate road traffic emissions. [ http://www.epa.gov/ttnchie1/conference/ei20/session7/lbelalcazar_pres.pdf ]
Jerrett M, Arain A, Kanaroglou P, Beckerman B, Potoglou D, Sahsuvaroglu T, Morrison J, Giovis C: A review and evaluation of intraurban air pollution exposure models. J Expo Anal Environ Epidemiol. 2005, 15: 185-204. 10.1038/sj.jea.7500388.
Liu LJ, Delfino R, Koutrakis P: Ozone exposure assessment in a southern California community. Environ Health Perspect. 1997, 105: 58-65. 10.1289/ehp.9710558.
Zmirou D, Gauvin S, Pin I: Five epidemiological studies on transport and asthma: objectives, design and descriptive results. J Expos Anal Environ Epidemiol. 2002, 12: 186-196. 10.1038/sj.jea.7500217.
Hoek G, Fischer P, Van Den BP, Goldbohm S, Brunekreef B: Estimation of long-term average exposure to outdoor air pollution for a cohort study on mortality. J Expos Anal Environ Epidemiol. 2001, 11: 459-469. 10.1038/sj.jea.7500189.
Bellander T, Berglind N, Gustavsson P, Jonson T, Nyberg F, Pershagen G, Järup L: Using geographic information systems to assess individual historical exposure to air pollution from traffic and house heating in Stockholm. Environmental Health Perspective. 2001, 109: 633-639.
U.S. EPA: MOVES (Motor Vehicle Emission Simulator). [ http://www.epa.gov/otaq/models/moves/index.htm ]
Defra: Emissions. [ http://laqm.defra.gov.uk/review-and-assessment/tools/emissions.html#eft ]
IEHIAS: emission factors. [ http://www.integrated-assessment.eu/guidebook/emission_factors ]
Handbook emission factor for road transport (HEEFA). http://www.hbefa.net/e/index.html ,
California Air Resources Board: EMFAC software. [ http://www.dot.ca.gov/hq/env/air/pages/emfac.htm ]
Park JY: PhD thesis. Microscopic modelling of air pollution from road traffic. 2005, Imperial College London: University of London Centre for Transport Studies
Wang Y, Zhang KM: Modelling near-road air quality using computational fluid dynamics (CFD) model. ES&T. 2009, 43: 7778-7783.
Bartonova A, Clench-Aas J, Gram F, Gronskei KE, Guerreiro C, Larssen S, Tønnesen DA, Walker SE: Air pollution exposure monitoring and estimation: Part V. Traffic exposure in adults. J Environ Monit. 1999, 1: 337-340. 10.1039/a902780g.
Kerner BS: Introduction to modern traffic flow theory and control. 2009, Springer: The Long Road to Three-Phase Traffic Theory
Gokhale S, Khare M: Vehicle wake factor for heterogeneous traffic in urban environments. Int J Environ Pollut. 2007, 30: 97-105. 10.1504/IJEP.2007.014505.
Ma Y, Richards M, Ghanem M, Guo Y, Hassard J: Air pollution monitoring and mining based on sensor grid in London. Sensors. 2008, 8: 3601-3623. 10.3390/s8063601.
Khedo KK, Perseedoss R, Mungur A: A wireless sensor network air pollution monitoring system. Int J Wireless Mobile Network. 2010, 2: 31-45.
Mishra SA, Tijare DS, Asutkar GM: Design of energy aware air pollution monitoring system using WSW. Int J Adv Eng Tech. 2011, 1: 107-116.
Demin Wang D, Agrawal DP, Toruksa W, Chaiwatpongsakorn C, Lu M, Keener TC: Monitoring ambient air quality with carbon monoxide sensor-based wireless network. Commun ACM. 2010, 53: 138-141.
Tracking Air Pollution by Cell Phone. [ http://pollutionfree.wordpress.com/2009/12/15/tracking-air-pollution-by-cell-phone/ ]
Schreiner C, Branzila M, Trandabat A, Ciobanu RC: Air quality and pollution mapping system, using remote measurements and GPS technology. Global NEST Journal. 2006, 8: 315-323.
Trandabăţ A, Branzila M, Donciu C, Pîslaru M, Ciobanu RC: Using GPS technology and distributed measurement system for air quality mapping of residential area. Environ Eng Manag J. 2007, 6: 545-548.
Al-Ali AR: A mobile GPRS-sensors array for air pollution monitoring. Sensors Journal, IEEE. 2010, 10: 1666-1671.
Sherwood CH: Using GPS and a smart phone to track air pollution exposure. [ http://www.smartplanet.com/blog/pure-genius/using-gps-and-a-smartphone-to-track-air-pollution-exposure/5578 ]
Science Daily: Smartphone app measures particulate air pollution. [ http://www.sciencedaily.com/releases/2010/09/100920135137.htm ]
Bayir MA, Demirbas M, Cosar A: A web-based personalized mobility service for smartphone applications. Comput J. 2011, 54: 800-814. 10.1093/comjnl/bxq027.
Wang D, Pedreschi D, Song C, Giannotti F, Barabasi AL: Human mobility, social ties, and link prediction. Proceedings of KDD '11 the 17th ACM SIGKDD international conference on Knowledge discovery and data mining: 21–24 August 2011; San Diego, California, USA. Edited by: Apte C, Ghosh J, Smyth P. 2011, New York: ACM, 1100-1108.
Fitzgerald E, Wartenberg D, Thompson WD, Houston A: Birth and fetal death records and environmental exposures: promising data elements for environmental public health tracking of reproductive outcomes. Public Health Rep. 2009, 124 (6): 825-830.
Environmental Exposure Modules for EHR and PHR Systems. [ http://www.hoise.com/vmw/10/articles/vmw/LV-VM-08-10-6.html ]
Environmental Exposure & EHR Systems. [ http://www.openhealthnews.com/blogs/groenpj/2011-04-11/environmental-exposure-ehr-systems ]
Fanchi J: Principles of Applied Reservoir Simulation. 2006, Burlington: Elsevier GPP, 3
Nikzad N, Verma N, Ziftci C: CitiSense: Improving Geospatial Environmental Assessment of Air Quality Using a Wireless Personal Exposure Monitoring System. Proceedings of Wireless Health: 23-25 October 2012; San Diego, Califonia, USA. Edited by: Raij A, Venkatasubramanian K. 2012, New York: ACM, 1-8.
Giannotti F, Pedreschi D: Mobility, data mining and privacy: a vision of convergence. Mobility, data mining and privacy: geographic knowledge discovery. Edited by: Giannotti F, Pedreschi D. 2008, New York: Springer, 1-14.
Bonchi F, Saygin Y, Verykios VS, Atzori M, Gkoulalas-Divanis A, Kaya SV, Savas E: Privacy in Spatiotemporal Data Mining. Mobility, data mining and privacy: geographic knowledge discovery. Edited by: Giannotti F, Pedreschi D. 2010, New York: Springer, 297-334.
Ziftci C, Nikzad N, Verma N: Citisense: mobile air quality sensing for individuals and communities. Proceedings of the third annual conference on systems, programming, and applications: software for humanity (SPLASH’12): 19-26 October 2012;Tucson, Arizona. Edited by: Leavens GT. 2012, New York: ACM Digital Library, 23-24.
Download references
Acknowledgements
This study was inspired by the EC FP7 Work Program 2012 Cooperation Theme 6 Environment (including climate change), call ENV.2012.6.5-1-Developing community-based environmental monitoring and information systems using innovative and novel earth observation applications. We would like to thank the referees IIan Levy and Michael Jerrett for their very valuable comments. Special thanks to William A. Lahoz, Alice Newton, Sonja Grossberndt at NILU (Norwegian Institute for Air Research) for helping us with the language. Thanks to Philipp Schneider at NILU for his help to revise the paragraph on how remote sensing from satellite improve the spatial resolution of air pollutants in urban areas. The initial proof of this manuscript benefited from the suggestions and comments from Alena Bartonova and Li Liu from NILU. Any remaining errors are the responsibility of the authors.
Author information
Authors and affiliations.
NILU - Norwegian Institute for Air Research, Instituttveien 18, Kjeller, 2027, Norway
Hai-Ying Liu & Mike Kobernus
Statoil Research Center, Arkitekt Ebbells Veg 10, Rotvoll, Trondheim, 7005, Norway
Erik Skjetne
You can also search for this author in PubMed Google Scholar
Corresponding author
Correspondence to Hai-Ying Liu .
Additional information
Competing interests.
The authors declare that they have no competing interests.
Authors’ contributions
HYL and ES planed this work and wrote the manuscript. MJK contributed content and provided editorial input. All authors approved the final version.
Authors’ original submitted files for images
Below are the links to the authors’ original submitted files for images.
Authors’ original file for figure 1
Authors’ original file for figure 2, authors’ original file for figure 3, rights and permissions.
This article is published under license to BioMed Central Ltd. This is an open access article distributed under the terms of the Creative Commons Attribution License ( http://creativecommons.org/licenses/by/2.0 ), which permits unrestricted use, distribution, and reproduction in any medium, provided the original work is properly cited.
Reprints and permissions
About this article
Cite this article.
Liu, HY., Skjetne, E. & Kobernus, M. Mobile phone tracking: in support of modelling traffic-related air pollution contribution to individual exposure and its implications for public health impact assessment. Environ Health 12 , 93 (2013). https://doi.org/10.1186/1476-069X-12-93
Download citation
Received : 20 November 2012
Accepted : 23 October 2013
Published : 04 November 2013
DOI : https://doi.org/10.1186/1476-069X-12-93
Share this article
Anyone you share the following link with will be able to read this content:
Sorry, a shareable link is not currently available for this article.
Provided by the Springer Nature SharedIt content-sharing initiative
- Citizen participation
- Health impact assessment
- Individual exposure
- Mobile phone tracking
- Public health
- Social network
- Traffic-related air pollution
Environmental Health
ISSN: 1476-069X
- General enquiries: [email protected]

An official website of the United States government
The .gov means it’s official. Federal government websites often end in .gov or .mil. Before sharing sensitive information, make sure you’re on a federal government site.
The site is secure. The https:// ensures that you are connecting to the official website and that any information you provide is encrypted and transmitted securely.
- Publications
- Account settings
- My Bibliography
- Collections
- Citation manager
Save citation to file
Email citation, add to collections.
- Create a new collection
- Add to an existing collection
Add to My Bibliography
Your saved search, create a file for external citation management software, your rss feed.
- Search in PubMed
- Search in NLM Catalog
- Add to Search
The effects of air pollution on the lipid balance of traffic police personnel
Affiliation.
- 1 Center of Aeromedical Evaluation and Occupational Medicine, Municipality of Rome, Rome, Italy.
- PMID: 17146243
- DOI: 10.5144/0256-4947.2002.287
Background: The purpose of our study was to evaluate the effects of urban pollution on the lipid balance of members of a municipal police force in a big city.
Subjects and methods: Our case-control study comprised 118 male members of the police force performing traffic duties, and 118 blood donors who perform office work, and was paired by age and length of service. Total cholesterol, HDL and triglycerides were studied.
Results: The comparison of the average values of HDL cholesterol and triglycerides between the exposed traffic police group and the control group showed significant differences. The difference in the frequency distributions of HDL cholesterol and triglycerides between the exposed group and control group was significant as well. The results suggest the possibility of an alteration in the lipid balance among asymptomatic people who are exposed to air pollution.
Conclusion: The results suggest that some chemical agents (such as carbon dioxide) contained in the urban pollution of a big city could cause dyslipidemia among people exposed to such air pollution.
PubMed Disclaimer
Similar articles
- Cadmium and lipid balance in outdoor workers exposed to urban stressor. Grazia G, Serafino R, Benedetta P, Gianfranco T, Carmina S, Anastasia S, Federica M, Pompeo Donato C, Francesco T, Carlo M. Grazia G, et al. Environ Toxicol Pharmacol. 2022 Feb;90:103793. doi: 10.1016/j.etap.2021.103793. Epub 2021 Dec 28. Environ Toxicol Pharmacol. 2022. PMID: 34971798
- Global review of studies on traffic police with special focus on environmental health effects. Patil RR, Chetlapally SK, Bagavandas M. Patil RR, et al. Int J Occup Med Environ Health. 2014 Aug;27(4):523-35. doi: 10.2478/s13382-014-0285-5. Epub 2014 Jul 18. Int J Occup Med Environ Health. 2014. PMID: 25034905 Review.
- Environmental and biological monitoring of arsenic in outdoor workers exposed to urban air pollutants. Ciarrocca M, Tomei G, Palermo P, Caciari T, Cetica C, Fiaschetti M, Gioffrè PA, Tasciotti Z, Tomei F, Sancini A. Ciarrocca M, et al. Int J Hyg Environ Health. 2012 Nov;215(6):555-61. doi: 10.1016/j.ijheh.2011.11.014. Epub 2011 Dec 24. Int J Hyg Environ Health. 2012. PMID: 22197513
- Effects of long-term exposure to traffic-related air pollution on respiratory and cardiovascular mortality in the Netherlands: the NLCS-AIR study. Brunekreef B, Beelen R, Hoek G, Schouten L, Bausch-Goldbohm S, Fischer P, Armstrong B, Hughes E, Jerrett M, van den Brandt P. Brunekreef B, et al. Res Rep Health Eff Inst. 2009 Mar;(139):5-71; discussion 73-89. Res Rep Health Eff Inst. 2009. PMID: 19554969
- Harm to the liver among employees of the Municipal Police Force. Tomao E, Baccolo TP, Sacchi L, DE Sio S, Tomei F. Tomao E, et al. Int J Environ Health Res. 2002 Jun;12(2):145-51. doi: 10.1080/09603120220129300. Int J Environ Health Res. 2002. PMID: 12396531
- Chronic Occupational Exposure to Traffic Pollution Is Associated with Increased Carotid Intima-Media Thickness in Healthy Urban Traffic Control Police. Balogun AO, Weigel MM, Estévez E, Armijos RX. Balogun AO, et al. Int J Environ Res Public Health. 2023 Sep 1;20(17):6701. doi: 10.3390/ijerph20176701. Int J Environ Res Public Health. 2023. PMID: 37681841 Free PMC article.
- Effects of particulate matter on atherosclerosis: a link via high-density lipoprotein (HDL) functionality? Holme SAN, Sigsgaard T, Holme JA, Holst GJ. Holme SAN, et al. Part Fibre Toxicol. 2020 Aug 4;17(1):36. doi: 10.1186/s12989-020-00367-x. Part Fibre Toxicol. 2020. PMID: 32753036 Free PMC article. Review.
- Coexistence of High Fibrinogen and Low High-density Lipoprotein Cholesterol Levels Predicts Recurrent Cerebral Venous Thrombosis. Ma X, Ji XM, Fu P, Ding YC, Xue Q, Huang Y. Ma X, et al. Chin Med J (Engl). 2015 Jul 5;128(13):1732-7. doi: 10.4103/0366-6999.159345. Chin Med J (Engl). 2015. PMID: 26112712 Free PMC article.
- Outdoor temperature is associated with serum HDL and LDL. Halonen JI, Zanobetti A, Sparrow D, Vokonas PS, Schwartz J. Halonen JI, et al. Environ Res. 2011 Feb;111(2):281-7. doi: 10.1016/j.envres.2010.12.001. Epub 2010 Dec 18. Environ Res. 2011. PMID: 21172696 Free PMC article.
- Association of cardiac and vascular changes with ambient PM2.5 in diabetic individuals. Schneider A, Neas LM, Graff DW, Herbst MC, Cascio WE, Schmitt MT, Buse JB, Peters A, Devlin RB. Schneider A, et al. Part Fibre Toxicol. 2010 Jun 2;7:14. doi: 10.1186/1743-8977-7-14. Part Fibre Toxicol. 2010. PMID: 20525188 Free PMC article.
Related information
- PubChem Compound
- PubChem Substance
LinkOut - more resources
Full text sources.
- Citation Manager
NCBI Literature Resources
MeSH PMC Bookshelf Disclaimer
The PubMed wordmark and PubMed logo are registered trademarks of the U.S. Department of Health and Human Services (HHS). Unauthorized use of these marks is strictly prohibited.

- Login To RMS System
- About JETIR URP
- About All Approval and Licence
- Conference/Special Issue Proposal
- Book and Dissertation/Thesis Publication
- How start New Journal & Software
- Best Papers Award
- Mission and Vision
- Reviewer Board
- Join JETIR URP
- Call For Paper
- Research Areas
- Publication Guidelines
- Sample Paper Format
- Submit Paper Online
- Processing Charges
- Hard Copy and DOI Charges
- Check Your Paper Status
- Current Issue
- Past Issues
- Special Issues
- Conference Proposal
- Recent Conference
- Published Thesis
Contact Us Click Here
Whatsapp contact click here, published in:.
Volume 9 Issue 2 February-2022 eISSN: 2349-5162
UGC and ISSN approved 7.95 impact factor UGC Approved Journal no 63975
Unique identifier.
Published Paper ID: JETIR2202171
Registration ID: 320088
Page Number
Post-publication.
- Downlaod eCertificate, Confirmation Letter
- editor board member
- JETIR front page
- Journal Back Page
- UGC Approval 14 June W.e.f of CARE List UGC Approved Journal no 63975
Share This Article
Important links:.
- Call for Paper
- Submit Manuscript online
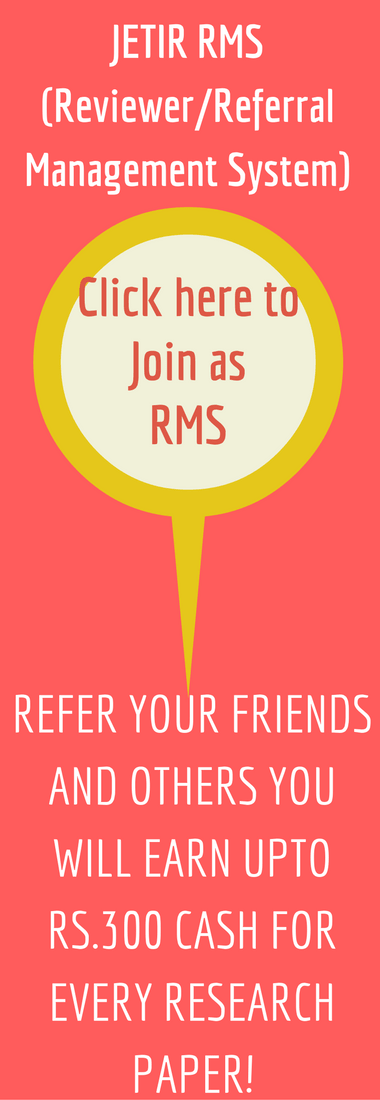
- Manasi Mane
- Dr. Divya Jethwani (PT)
- Dr. Vaibhavi Mahendrakar (PT)
Cite This Article
2349-5162 | Impact Factor 7.95 Calculate by Google Scholar An International Scholarly Open Access Journal, Peer-Reviewed, Refereed Journal Impact Factor 7.95 Calculate by Google Scholar and Semantic Scholar | AI-Powered Research Tool, Multidisciplinary, Monthly, Multilanguage Journal Indexing in All Major Database & Metadata, Citation Generator
Publication Details
Download paper / preview article.

Download Paper
Preview this article, download pdf, print this page.

Impact Factor:
Impact factor calculation click here current call for paper, call for paper cilck here for more info important links:.
--> |
|
|
|
- Follow Us on

- Developed by JETIR
- DOI: 10.5144/0256-4947.2002.287
- Corpus ID: 34238639
The effects of air pollution on the lipid balance of traffic police personnel.
- E. Tomao , Paola Baccolo Tiziana , +2 authors F. Tomei
- Published in Annals of Saudi Medicine 1 September 2002
- Environmental Science, Medicine
39 Citations
Respiratory parameters in traffic policemen exposed to urban pollution, chronic occupational exposure to traffic pollution is associated with increased carotid intima-media thickness in healthy urban traffic control police, exposure to urban stressor and effects on free testosterone in female workers., evaluation of lipid parameters in outdoor and indoor workers: preliminary results.
- Highly Influenced
Luteinizing hormone (LH) levels in male workers exposed to urban stressors.
Follicle-stimulating hormone levels in male workers exposed to urban chemical, physical, and psychosocial stressors, plasma dopamine in workers exposed to urban stressor, [meta-analysis: cardiovascular effects in workers occupationally exposed to urban pollution]., effects on androstenedione in male workers exposed to urban stressors, exposure to traffic pollutants and effects on 17-β-estradiol (e2) in female workers, 11 references, [an epidemiological study of the health conditions of milan traffic police with respect to pollution from vehicular traffic]., exposure to benzene in urban workers: environmental and biological monitoring of traffic police in rome, early health effects and biological monitoring in persons occupationally exposed to tetraethyl lead, particulate air pollution and acute health effects, hypertension and chronic exposure to noise, cigarette smoking impairs hepatic uptake of high density lipoproteins., carbon monoxide, tobacco smoking, and the pathogenesis of atherosclerosis., air pollution and incidence of cardiac arrhythmia., identifying populations potentially exposed to agricultural pesticides using remote sensing and a geographic information system., teneur en cholestérol des lipoprotéines légères chez le lapin hypercholestérolémique intoxiqué ou non par l’oxyde de carbone, related papers.
Showing 1 through 3 of 0 Related Papers

An official website of the United States government
The .gov means it’s official. Federal government websites often end in .gov or .mil. Before sharing sensitive information, make sure you’re on a federal government site.
The site is secure. The https:// ensures that you are connecting to the official website and that any information you provide is encrypted and transmitted securely.
- Publications
- Account settings
Preview improvements coming to the PMC website in October 2024. Learn More or Try it out now .
- Advanced Search
- Journal List
- Environ Health

Mobile phone tracking: in support of modelling traffic-related air pollution contribution to individual exposure and its implications for public health impact assessment
Hai-ying liu.
1 NILU - Norwegian Institute for Air Research, Instituttveien 18, Kjeller 2027, Norway
Erik Skjetne
2 Statoil Research Center, Arkitekt Ebbells Veg 10, Rotvoll, Trondheim 7005, Norway
Mike Kobernus
We propose a new approach to assess the impact of traffic-related air pollution on public health by mapping personal trajectories using mobile phone tracking technology in an urban environment. Although this approach is not based on any empirical studies, we believe that this method has great potential and deserves serious attention. Mobile phone tracking technology makes it feasible to generate millions of personal trajectories and thereby cover a large fraction of an urban population. Through analysis, personal trajectories are not only associated to persons, but it can also be associated with vehicles, vehicle type, vehicle speed, vehicle emission rates, and sources of vehicle emissions. Pollution levels can be estimated by dispersion models from calculated traffic emissions. Traffic pollution exposure to individuals can be estimated based on the exposure along the individual human trajectories in the estimated pollution concentration fields by utilizing modelling tools. By data integration, one may identify trajectory patterns of particularly exposed human groups. The approach of personal trajectories may open a new paradigm in understanding urban dynamics and new perspectives in population-wide empirical public health research. This new approach can be further applied to individual commuter route planning, land use planning, urban traffic network planning, and used by authorities to formulate air pollution mitigation policies and regulations.
According to the United Nations (UN), more than 50% of the world’s population lives in urban areas and this number will increase to 70% by 2050 [ 1 ]. Road traffic is the main source of pollution in well-developed urban regions worldwide. Traffic-related air pollution may have diverse impacts on health including exacerbation of asthma, onset of childhood and adult asthma, non-asthma respiratory symptoms, impaired lung function, total and cardiovascular mortality, and cardiovascular morbidity, hospitalizations, various cancers, birth outcomes, diabetes and life expectancy [ 2 ], etc. Based on a synthesis of the best available evidence, the HEI (Health Effects Institute) identified that an exposure zone within a range of up to 300 to 500 metres from a major road as the area most highly affected by traffic emissions [ 2 ]. According to the United States Environmental Protection Agency (U.S. EPA) [ 3 ], more than one-third of Nitrogen Oxides (NOx) and one-quarter of Particulate Matter (PM) emissions in the U.S. are from roadways, and about 45 million Americans live within 100 metres of a road. A study by researchers at the University of Southern California (USC) and the California Air Resources Board found that up to half of Los Angeles’ residents total exposure to ultrafine air pollutants occurs while people are travelling in their vehicles [ 4 ]. It has frequently been recognised that car drivers are highly exposed to airborne pollution [ 5 , 6 ], and are at an increased risk of developing several types of cancer [ 7 ]. A report by the World Health Organization (WHO) showed that long-term air pollution from traffic in Austria, France and Switzerland triggered 300,000 additional cases of bronchitis in children, 15,000 hospital admissions for heart disease and a further 162,000 asthma attacks in children [ 8 ].
Different methods have been used to estimate exposure of participants to urban traffic-related air pollution in previous studies. Exposure of participants is often estimated by the concentration either measured at the monitoring site nearest to the participants’ residential address [ 9 , 10 ], or generated by applying dispersion model [ 11 , 12 ] or Land Use Regression (LUR) techniques to the participants’ residential address [ 13 , 14 ], and by accounting for indicators of traffic and other factors such as geospatial location, meteorology and nearby urbanization. Dispersion models can provide information that satisfies both the spatial coverage and temporal variation, but good performance from dispersion modelling requires good input data. In the approach of LUR models it is relatively simple to interpolate a limited number of measurements to a larger population [ 15 ]. However, both approaches do not account for personal activities such as time spent in traffic and activity level which will influence exposure considerably [ 16 ].
Methods accounting for personal movement include the Personal Environmental Impact Report (PEIR) [ 17 ]. A platform that uses location data sampled from everyday mobile phones to calculate personalized estimates of environmental impact and exposure was presented in 2009 [ 18 ]. The authors also proposed using mobile phones with a built-in GPS (Global Positioning System) receiver and an accelerometer for identifying individual transportation modes including whether an individual is stationary, walking, running, biking, or in motorized transport [ 19 , 20 ]. This was further explored in the EU-FP7 project ENVIROFI, where the Personal Environmental Information System (mobile phone based application) provided accurate air quality data for the user’s current location based on the GPS coordinates supplied by the user’s phone [ 21 ]. The CitiSense project [ 22 ] had a mobile air quality system that enabled users to track their personal air quality exposure [ 23 , 24 ]. The above studies did not target population level exposure. Studies using mobile air pollution sensors are only active on a relatively small number of vehicles for which the estimated pollution levels will be derived. Through this brief review of the health effects of traffic, we identified: (i) exposure to traffic-related emissions may cause major adverse health effects on the population at or near air polluted roadways; (ii) existing exposure models do not accurately characterize the exposure fields; and (iii) these disadvantages in exposure estimation can attenuate health effect estimates. To address these issues, studies are needed to characterize the personal exposure to traffic-related air pollution, and to better understand the linkages between traffic-related air pollution and public health effects.
In this study, we propose a conceptual framework to improve exposure assessment by using existing, low cost mobile phone data to obtain individual trajectories to further estimate the concentration of the traffic emission in the roadway network and its contribution to exposure for the urban population. We analyse each element along the work process (Figure 1 ) from human mobility, through traffic-related air pollution modelling, to exposure and its implications for public health impact assessment. Our proposed methodology is based upon recent advances in social and information networks using Statistical Physics [ 25 ] and the techniques of Traffic Engineering in mobile phone networks [ 26 ]. The advantage of the proposed study is that we target the entire network of mobile phone users to calculate pollution levels, exposure and health effects. We believe that this has the potential to open up new perspectives in public health research.

An overview of work process for modelling traffic-related air pollution contribution to individual exposure by mobile phone tracking. It is composed of six parts: ① Pollution monitoring using stationary sites; ② Trajectory mapping by mobile phone; ③ Automatic vehicle type identification or estimation; ④ Vehicle trajectories and vehicle emissions; ⑤ Pollution field modelling; and ⑥ Pollution exposure along trajectories.
The science of individual human trajectories is a new research field based on the recent availability of empirical data on human mobility. Developments in this field have been influenced by results in the multidisciplinary field of network physics [ 27 , 28 ]. Databases and technologies that capture human mobility by mobile phone traces are now available and many interesting features have been discovered including the characteristics of individual human trajectories [ 29 ], the interaction between individual mobility and social networks [ 30 ], and meso-scopic structure (i.e., the detailed substructures and spatiotemporal flows of mobility) and social aspects of human mobility [ 31 ].
Modern network physics is starting to influence public health research. For instance, Health InfoScape, part of the Massachusetts Institute of Technology (MIT) SENSEable Cities and the General Electric Company (GE), aim to create new ways of understanding human health in the U.S. [ 32 ]. By analysing data from over 7.2 million anonymous electronic medical records taken from across the U.S., they seek to uncover statistical relationships between space, geography and health.
There is a recent development in Location-Based Services (LBS) [ 33 ] through mobile phone technology. Basic mobile phone based positioning systems include: location of nearest base station; trilateration of two or more neighbouring base stations by signal strength or Time Of Arrival (TOA); multilateration (hyperbolic lateration) by Time Difference Of Arrival (TDOA); and network based triangulation by Angle Of Arrival (AOA) using multiple antennas at a base station. More methods are available with third generation mobile systems [ 34 ]. Thiragarajan et al. [ 35 - 37 ] used a Hidden Markov Model (HMM) to map noisy phone location data to road segments using mobile phone data from both the GPS and the Global System for Mobile Communications (GSM) systems. The road segments are the hidden states of the system and the noisy data is the input to the algorithm. The mobile phone data are run through the algorithm twice, first to find the mobile phone area sequence and then to find the road segment sequence [ 38 ]. Processed position data provided by mobile phones are as accurate as GPS reading intervals of 2 minutes and have much lower energy consumption [ 39 ]. According to studies on probabilistic models for mobile phone trajectory estimation from Thiagarajan [ 39 ], the misplaced road segment error is approximately only 45 metres. While, on average, mobile phone tracking can retrieve over 75% of a user’s drive accurately, even from highly inaccurate (175 metres raw position error) GSM data.
There are several companies providing individual trajectory mobile phone based traffic monitoring services including: Cellint [ 40 ], Intelligent Mechatronic Systems Inc (IMS) [ 41 ], ITIS [ 42 ], and Urban Informatics (UI) [ 43 ], etc. The car navigation company TomTom [ 44 ], has one million GPS users and uses about 80 million other data sources such as GSM to monitor real time traffic and give advice on the best route, taking into account traffic congestion. Google Maps [ 45 ] gets live traffic information displaying road way traffic conditions for main roads in many countries updated every five to ten minutes. Close to real-time traffic maps are provided by all larger search companies including Yahoo, Bing, Baidu and Yandex. For example Yandex also provide real time video cameras of selected junctions in Moscow, and traffic map forecast at 15 minutes intervals up to one hour. The Mobile Millennium project at the University of California (UC), Berkeley, in collaboration with Nokia Research Centre, and NAVTEQ Company uses GPS in cellular phones to gather traffic information, process it, and distribute it back to the phones in real time [ 46 ].
Microscopic traffic simulation [ 47 ] is a field closely related to trajectory mapping. In this context 'microscopic’ means modelling and simulation of the movement of individual vehicles. The vehicles are typically modelled taking into account the distance to the vehicles in front; this allows realistic simulation of congestion waves that move upstream with a group velocity of about 15 km / h . Several simulation models combining microscopic traffic modelling with simulation of air pollution concentrations in street canyons [ 48 - 51 ] make it feasible to calculate traffic-related air pollution by mapped personal trajectories.
This paper combines the above presented unrelated technologies to form a new approach in urban traffic-related air pollution and public health impact research. We develop a concept for air pollution research based on trajectory mapping by using an empirical model that articulate the overall work process, system architecture, traffic-related air pollution and exposure modelling. We also discuss the main challenges that might be faced by applying such an approach to study the traffic-related air pollution to individual exposure and its public health impact assessment.
Work processes and system architecture
The work process is composed of six parts. Figure 1 illustrates the key elements of the work process. Following the work process in Figure 1 , one can both construct the pollution field and estimate the pollution exposure by fixed site data from relatively high density of monitoring stations (steps 1, 5 and 6), or alternatively by mobile phone tracking (steps 2–6), or through combining both mobile phone tracking and fixed site monitoring stations (steps 1–6). The work process is described in detail below.
Pollution monitoring using stationary sites
In the suggested approach, the air pollution data can be directly gathered from a static network of pollution monitoring sites across the city and air pollution maps are generated by interpolating between them. People can then check their exposure levels by mapping their individual trajectory into the pollution map. However, there are some limitations of using static monitoring networks, i.e., mainly the total number of air quality monitoring sites within a city is often limited by practical constraints, and possibly other limitations (e.g., reporting hourly averages, possibly not at real time in some regions, etc.). As a possible complementing source of information, high spatial resolution satellite observations can be used. While satellite measurements have many advantages and are developing rapidly, they do have some major limitations, particularly when considering intra-urban resolution. Although most operational satellite products relevant for air quality applications currently do not yet possess the spatial resolution necessary to directly resolve fine spatial detail at the urban scale, their output can nonetheless be very valuable for improving the output of local and regional chemical transport models through data assimilation schemes, and as such indirectly improve urban-scale air quality estimates. Furthermore, research is currently ongoing on producing higher-resolution (300 m) satellite-based PM measurements at the urban scale [ 52 ]. Finally, significant progress with regards to spatial resolution achievable from space-based platforms will further be made in the near future with new instruments such as TROPOMI (The TROPOspheric Monitoring Instrument) on the Sentinel-5 precursor platform, which will open up entirely new applications for space-based air quality monitoring, in particular over megacities and other large urban agglomerations.
Trajectory mapping by mobile phone
Trajectory mapping uses individual human trajectories generated by advanced mobile phone GSM (GPS-free) tracking systems. The tracking system should be capable of accurately locating mobile phones on road segments in a roadway network by using traffic engineering tracking systems. From frequent location updates, it is possible to track the approximate real-time speed of the mobile phones. This technology is partly qualified, but to date has not been implemented for large populations. Computational capacity may also be a limitation as one PC calculates a one hour individual trajectory in about two minutes [ 39 ]. There are ways to use one individual trajectory for calculating other trajectories on the same road segment at approximately the same time to make the computation of a large number of trajectories feasible. Commercial algorithms are likely to be faster on commuter freeway systems where the vehicles, on average, are travelling on a small set of roadways and stay on the same roadway for quite a long time.
Automatic vehicle type identification or estimation
A mobile phone signal does not identify a vehicle. The vehicle type can be estimated by drawing randomly or conditioned to trajectories such as bus stop patterns to identify that it is a bus from a national vehicle type distribution. Modern Automatic Vehicle Identification (AVI) systems cover or will soon cover most vehicles in a country such as Autopass [ 53 ] in Norway, SINIAV in Brazil [ 54 ], and EVR in Mexico [ 55 ]. These systems are based on Radio Frequency Identification (RFID) [ 56 ] and give the exact date and time for passing a certain check point. Cross correlation of AVI and mobile phone data results in one-to-one assignment of a mobile phone to an identified vehicle. The vehicle type, age, etc., will determine its emission class which is a key to building up a picture of emissions.
Vehicle trajectories and vehicle emissions
Individual human trajectories can be further used to generate vehicle trajectories on road segments in a roadway network by using traffic engineering tracking systems. Emissions from each individual vehicle can be calculated along the vehicle trajectories.
Pollution field modelling
Traffic pollution is not just a product of vehicle exhaust, but also tyre wear, brakes wear, oil leaks, as well as erosion of the road surface itself and deposited sand and salts at icy/snowy winter roads. Dispersion of traffic air pollution in urban areas can be modelled numerically by taking into account the recirculation effect of street canyons, meteorological conditions, pollution type, traffic counts and types, and other parameters. In this case, various dispersion models (e.g., CALINE3/4 [ 57 ] and CAL3QHC/R [ 58 ]), CFD (Computational Fluid Dynamics) models [ 58 , 59 ], geo-statistical interpolation models and hybrid models that are specially developed for or simply used in street canyon applications can be applied. To realize this, an increase in computational power is needed, especially if the model is run over long time periods of weeks and even months; and/or to model air pollution level on a street canyon for an entire city could also be computational intensive. Moreover, there is a need for high resolution input data for the models about the meteorology in the city, including temperature and wind speed and directions.
Pollution exposure along trajectories
Exposure data can be acquired by evaluating the pollution field along the trajectories in space and time. In this case, a micro-environment based exposure model can be applied [ 60 ].
Mobile phone-based mobility tracking used to model traffic pollution contributions to both individual chronic and acute exposure can open new fields of research. This approach can be further developed to estimate the accumulated dose of air pollutants in the human body, and to assess the health impact by coupling individual trajectories to individual physiological information (e.g., age, gender, inhalation rate, body length and weight, health records, etc.) [ 61 , 62 ]. Field studies with personal exposure monitors have found that traditional exposures estimated from average pollution levels in a city based upon residence postal codes are inadequate for pollutants which are spatially variable [ 16 ] and tend to underestimate variability in exposure, in particular when a person’s location is highly correlated to narrow peaks in the pollution field. The trajectory based system proposed in this paper will pick up such correlations. Therefore, the individual trajectory approach would be expected to more accurately identify the individuals with the greatest health risk. The most highly exposed groups may share common trajectory patterns, for example those with commuter/work patterns that have the highest health risk (e.g., professional drivers, traffic police and construction workers, etc.). By coupling mobile phones to electronic health records (EHR) and/or personal health records (PHR), one may identify highly vulnerable/pollution-sensitive groups (for example those with respiratory diseases) among the most risky trajectory patterns. Thus, this may open up new perspectives in empirical public health research.
Figure 2 illustrates the potential to characterize traffic-related microenvironments, e.g., in-vehicle exposure in congestion as opposed to non-congestion: (a) mapping a mobile phone trajectory; (b) associating mobile phone with vehicles, and linking vehicles to air pollution; and (c) estimating exposure for people travelling through the pollution fields [ 63 ]. It needs to be noted that the mobile phone is used to identify the person and a person-related vehicle.

The potential to characterize traffic-related microenvironments, e.g., in-vehicle exposure in congestion as opposed to non-congestion. (a) Mapping a mobile phone trajectory; (b) Associate mobile phones with vehicles, and associate vehicles to air pollution which enables construction of a continuous air pollution field in space and time; (c) Estimate exposure for those people who travelling through the pollution field (the equation is from Reference No. 89).
Figure 3 illustrates the system architecture of a complete system following the work process in Figure 1 . On the Information Communication Technology (ICT) infrastructure part, the raw data are the mobile phone base stations in communication with individual mobile phones. These raw data are used to efficiently generate individual mapped trajectories showing the positions of individual mobile phones through time. Mapping algorithms are used to locate the moving positions onto road segments. The mapped trajectories are used as input to algorithms to construct the pollution field and estimate exposure for people moving in the pollution field.

System architecture for obtaining mapped trajectories and public health impact. It consists of a two-way communication: server side (mobile phone base stations – raw data – mapped trajectories) and person side (mobile phone – individual trajectory, emission, exposure - health effect).
At the population-scale one may investigate high risk groups and check if they share common travel patterns. Note that on the lower right graph in Figure 3 the term 'population’ indicates the population density or frequency in a population histogram. This may be used to identify the population fraction (i.e., area below the curve) and the risk of the high risk group.
Existing traffic air pollution exposure models
The goal of this section is to review some modelling approaches that can be used in this proposed approach, to calculate the traffic-related air pollution and its contribution to individual exposure. Each given model is kept simple and current at a conceptual level, so that each model can be replaced by more advanced models in specific studies.
Dispersion models
A simplifying concept of a roadway air pollution model is to treat the emission from many vehicles as one line source. The pollution is dispersed away from the line source to the entire model domain (and not just near roads) by the mechanisms of diffusion and convection (move with the local velocity of the air). However, due to the dispersive spreading the highest pollution levels are found at the line source. For simpler, roadway-only sources, the CALINE3/4 [ 58 ] and CAL3QHC/R [ 58 ] series of dispersion models explicitly address traffic-induced emissions. AERMOD is also broadly used for traffic-related complex source mixtures, e.g., terminals, ports and roadways considered in combination, large highway corridors and urban scale modelling [ 58 ].
Geo-statistical interpolation models
Geo-statistical interpolation models can be implemented to estimate the traffic-related air pollution based on a statistical interpolation of a dense, well-distributed, monitoring work [ 64 ]. These models allow the estimation of pollution concentrations over several time intervals, but this is limited only by the number of available measurement periods [ 64 ].
Hybrid models
The hybrid models are those that combine personal or regional monitoring with other air pollution exposure methods [ 64 ]. Most studies were conducted in European cities, and in San Diego [ 65 ], e.g., by using personal exposure measurements in conjunction with fixed outdoor stations to estimate hourly exposure for each day, to calculate a traffic-density index [ 66 ]; and by using regional monitoring in conjunction with another modelling scheme to assume long-term exposure to NO 2 and Black Smoke (BS) [ 67 ], etc.
Essential factors to estimate traffic air pollution exposure in the mobile phone tracking approach
No matter which of the above given models is applied, in order to calculate real-time varying traffic-related air pollution, the methods for estimating the traffic emission, emission rates, emission factors, traffic flows, and individual exposure to dynamic traffic air pollution need to be addressed explicitly.
Estimation of traffic-related air pollution
The roadway air dispersion models are usually used to estimate traffic-related air pollution. It generally relies on Gaussian plume equation [ 68 ], including wind speed, wind direction, traffic wakes and meteorological air stability.
Many tools have been developed to estimate the vehicle emission factors covering a broad range of pollutants and allow multiple scale analysis, such as the U.S. EPA MOVES (Motor Vehicle Emission Simulator) [ 69 ] for HC, NOx, CO, gaseous SO2, ammonia, direct PM; the UK Defra (Department of environment, food and rural affairs) EFT (Emission factor toolkit) Version 5.2 for NOx, PM10, PM2.5, CO2 and Hydrocarbons [ 70 ]; and the HBEFA (The Handbook Emission Factors for Road Transport) for atmospheric emission factors for regulated and important non-regulated pollutants from road vehicles in Germany, Austria, Switzerland, Sweden, Norway [ 71 , 72 ]; and the EMFAC (EMission FACtors) for HC, CO, NOx, CO2, PM, fuel consumption, oxides of sulphur (SOx), and lead (Pb) developed and used in California [ 73 ], etc.
Estimation of traffic flows
One challenge in roadway air dispersion modelling is the transformation from a Lagrangian formulation [ 74 ] where each vehicle is a particle with a given speed to an Eulerian formulation [ 75 ] where each road segment has a vehicle density with a local flow velocity. This particular challenge has been investigated by the UC Berkeley Mobile Millennium project [ 72 ] and an Eulerian–Lagrangian cell transmission model for air traffic flow has been developed [ 76 ]. In traffic flow conditions, the two most important densities are the critical density ( n c ) and the traffic jam density ( n jam ). The maximum density achievable under free flow is n c , while the minimum density achieved under jamming congestion is n jam . In general, traffic jam density is about seven times the critical density. Traffic is, however, a complex phenomenon that is described by physics concepts such as synchronization and self-organized criticality (SOC). Kerner [ 77 ] divided traffic flow into three phases from low to high density: 1) free flow, 2) synchronized congested flow, and 3) wide moving jam (wide jam that moves upstream).
The traffic density-interaction between the vehicle wakes (i.e., isolated vehicles, interacting vehicle wakes, strong interacting vehicle wakes) have been studied by Gokhale and Khare [ 78 ], and their results showed that in 'unstable’ conditions, the Vehicle Wake Factor (VWF) varies between 1.63 and 0.3 (for wind direction, θ = 90°) and 2.5 and 0.8 (for wind direction, θ = 180°). During 'neutral’ and 'stable’ conditions, it is in the range of 0.84 – 0.4, 1.91 – 0.85 (for wind direction θ = 270°) and 1.7 – 0.7, 3.1 – 0.3 for wind direction θ = 360°, respectively.
Not all citizens carry a mobile phone and this makes the mapped population an incomplete set of mapped persons/vehicles. One may fill in these gaps using statistical distributions. For simplicity, we assume 100% mobile phone coverage, that is, each individual has a mobile phone that is tracked. Linking individual trajectories to vehicles can be done as follows: (i) Identify bus drivers and passengers by their characteristic trajectory pattern. They follow a pre-defined route with a given stopping pattern. It is important to correctly estimate the number of persons travelling by bus in order to avoid over estimating the number of vehicles. (ii) Identify private car commuters by their regular trajectory patterns from the suburb to the city, and vice versa . (iii) Identify other group travellers (e.g., subway commuters, pedestrians, motorcycles, bicycles riders) by having an identical trajectory. This is to avoid overestimating the number of vehicles with passengers. (iv) The rest of the trajectories are individual drivers and their vehicles may be stochastically drawn from the vehicle type (e.g., electric car, truck or lorry) including the vehicle age, probability density function.
The road network is discretized into piecewise linear road segments. The pollution from each segment is modelled by the linear source equation. The pollutant concentration for people on the road segment is given by the characteristic mid-segment pollution. The vehicle densities (number per length) are estimated by the procedure above. The average speed of traffic in the segment is calculated by the trajectory data (∆X,∆Y,∆t) and ∆Z from terrain/map data.
If the road is a freeway with many lanes one may consider splitting the two driving directions into two line sources and sum the pollution from the two directions taking into account the centre-to-centre distance between the lane directions. For near roadway exposure one may select bands parallel to the roadway network and take into account overlapping contributions from the nearest neighbouring road(s). Traffic also contributes to background pollution, and this may be calculated by longer term smoothing of the total city pollution from stationary sites’ data.
To model individual exposure to traffic air pollution
Human exposure refers to an individual’s contact with a pollutant concentration. Given a close to real-time continuous pollution field, it is straightforward to calculate the direct exposure to air pollution in the transport network. In this context, a microenvironment (e.g., the interior of a car/bus, inside a house, or urban, suburban, and rural areas, etc.) based integrated exposure model can be applied and expressed as [ 60 ]:
Where X i is the total exposure for person i over the specified period of time, C j is the pollutant concentration in microenvironment j , t ij is the residence time of the person i in microenvironment j , and J is the total number of microenvironments. The total exposure is, therefore, the sum of exposures during a given time and has thus the same dimension as exposure in general: (mass) × (time) / (volume) or (concentration) × (time), for example, with units μg d m –3 .
In the case where M persons are exposed to the same concentration for the same time, the exposure in this case is M times that for one person and, for example, with the units (concentration) × (time). The resulting population exposure may then be used to relate the number of hospital admissions to exposure.
A constraint for using the microenvironment based exposure models (see equation above) is that the residence time of the person (termed the time–activity pattern) needs to be known together with the pollution concentrations in each of the microenvironments at the time when the person is present. In this article, however, by using mobile phone tracking we could get both individual time-activity pattern and real time continuous pollution concentration along the traffic network.
Two alternatives to an individual trajectory based system are: (1) one may measure real-time air pollution by large-scale implementation of a Wireless Sensor Network (WSN) [ 79 - 82 ], in which hundreds or thousands of small personal environmental sensors carried by the public rely on cell phones to shuttle information to central computers where it will be analysed, made anonymous and reflected back to individuals, authorities and public health agencies [ 83 ]; and (2) one may rely on stationary based, monitored pollution data together with geo-statistical models and use GPS information [ 84 - 86 ] provided by smart phone applications to track air pollution exposure of a sub-population [ 87 - 89 ] as mentioned earlier in this article. Alternative (1) uses individual trajectories. One may expect that an actual measurement of the air pollution exposure by WSN would be somewhat better than a calculated individual trajectory based system as proposed in this article. This is a point in favour of the WSN-based micro sensor approach. However, the cost of equipping a population with environmental micro sensors limits this method to small-scale studies (Table 1 ). Alternative (2) for an entire city would require stationary measurements at nearly all road segments in the city. Although this could provide high quality data, it is certainly extremely costly due to the extremely high density of measurement stations. It scales with the size of the road network as shown in Table 1 . The cost of up-scaling a mobile phone trajectory system is only the cost of incremental computational power. This is very low since the individual data already exists and can be uploaded from the mobile phone or downloaded from the mobile phone service provider. Less computational challenging studies have already included six million phone users of a Call Detailed Records (CDR) data base [ 90 ]. The detailed traffic trajectories need more computational power, but the algorithms are cost-efficient and are likely very well suited for parallel algorithms, since each trajectory is calculated independently of the other trajectories. Trajectory based data have many opportunities for reducing costs by building on synergies with commercial traffic monitoring systems. A commercial traffic monitoring system may add a health impact service that not only optimizes commuting routes based on the time spent from home to work, but may also propose alternative eco-driving routes that have a positive impact on one’s personal health.
Comparison of alternatives to the base case proposed in this article
Base case in this article | Pollution field is calculated by the aggregate of individual GSM or GPS-based trajectories plus emission and dispersion models. | Accumulated along individual GSM or GPS-based trajectories. | Low cost per individual. Cost of computational power. |
Alternative 1 | Measured on each individual trajectory by people carrying environmental micro-sensors. | Accumulated along individual GPS-based trajectories. | High cost per individual. Additional cost per individual is roughly the cost of a new smart phone. |
Alternative 2 | Stationary monitoring network plus geo-statistics modelling. | Accumulated along GSM or GPS-based trajectories. | Very high investment cost. Need very high density of monitoring stations on the road network. |
Traffic monitoring companies, including the largest search engine companies, may want to show their social responsibility by releasing their data for public health research in the same way as mobile phone tracking data are released for human mobility research. In this way the public health research could be obtained at almost no extra cost. More realistic, we believe that traffic monitoring companies will offer new free services to calculate real time pollution exposure and health risk maps in large cities that can be used by traffic map users with GPS smart phones. We have developed a model to estimate traffic emissions from traffic map data. This modelling is planned to be published in another article. Based on this approach a mobile phone tracking system has the potential of being highly competitive economically for population-wide traffic pollution health studies.
Trajectory based individual exposure analysis opens up new perspectives in public health research. Instead of analysing either the average health risk given by average city pollution levels or analysing a limited number of citizens’ mobility, we are now in a position to analyse the probability distribution density of individual risk for relevant diseases. Furthermore, by linking to HER and/or PHR, the proposed approach can help to better understand the impact of environmental exposure on human health at both individual and population levels, and might enhance environmental public health tracking as well [ 91 - 93 ]. Natural questions that arise include: Who is most at health risk? Is it the large majority of the population with moderate pollution exposure or a small group of highly sensitive/vulnerable persons with unusual exposure trajectories? A natural extension of the trajectory based individual exposure analysis is to do research on optimal regulatory, policy change and urban planning to reduce air pollution. What are the most efficient ways to transform an existing urban environment into a city with a low air pollution health risk?
In polluted cities there are many different options for improving air quality. One may develop trajectory based dynamic computer simulation models where urban planning concepts are evaluated based on simulated performance with regard to cost and improved public health. This approach is similar to dynamic petroleum reservoir simulation by streamlines [ 94 ].
A vision for mobile phone tracking technology is to do global studies that incorporate the global gaps in population of mobile phone users. The gaps in the network, that is, those that do not have a mobile phone, may be inferred directly from the trajectories from home to kindergarten, to school, to work, and scale this number of trajectories to estimate the effect from the whole population. An advantage of this approach is that it is effortless for citizens as the global population does not need to adjust their activity pattern or wear any measurement devices to participate in the study.
There are some limitations to our approach that need to be addressed when used in real practice. First, the proposed approach is mainly targeted at the person who has a mobile phone. This is a limitation for those people that do not tend to have mobile phones (e.g., children, elderly, the poor, etc.). The biased samples are not easily worked out with statistical methods if they are not randomly distributed, which is the case here. Second, the risks arise first from the fact that in order to get the individual human trajectory, the data we need is mostly about people, where they have been, at what times, how often. Therefore privacy and security is a major concern, and needs to be addressed before the opportunities of trajectory mapping can be created. Furthermore, there are also huge issues with privacy and access to link to HER and/or PHR. Encryption and anonymization can provide a level of privacy [ 95 ]. In this study, anonymous data are enough to construct individual human trajectories, pertaining to groups of people. However, to guarantee anonymity is hard in the domain to link/compare the spatio-temporal individual trajectory based exposure-dose modelling with real medical patient data. Many studies that address privacy issues in a spatio-temporal data mining context have already been published. In the Geographic Privacy-Aware Knowledge Discovery and Delivery (GeoPKDD) project, Giannotti and Pedreschi [ 96 ] investigated the various scientific and technological issues of mobility data, open problems, roadmap, and concluded that the privacy issues related to the ICTs can only be addressed through an alliance of technology, legal regulations and social norms. In the meantime, increasingly sophisticated privacy-preserving data mining techniques are being studied and further developed [ 97 ]. Their aim is to achieve appropriate levels of anonymity by means of controlled transformation of data and/or patterns – limited distortion that avoids the undesired side effect on privacy while preserving the possibility of discovering useful knowledge. The project Citisense designed a permissioning system that allows users to configure publicly accessible time Windows of their data [ 98 ]. Mun et al. [ 18 ] suggested a selective sharing and hiding mechanism in PEIR that enable users to share their location traces with people they trust, and generates believable proxy traces for times a user does not want their real location revealed. Furthermore, an alternative to deal with privacy issues related to tracking of individual trajectories is to collect a population-wide data in the form of willing participation, as done by PEIR [ 17 , 18 ] mentioned above, where only individuals that are motivated will take part in the data collection. In this approach, the methods to empower citizens at population level to participate in such studies may need to be further developed.
Another limitation of using mobile phone tracking approach to study individual exposure to traffic-related air pollution and its public health impact assessment is that the external data/information and models needed, such as vehicle type identification, emission dispersion models, emission factors and meteorology data needs to be known together with the vehicle trajectories to model pollution fields; individual physiological information (e.g., height, weight, inhalation rate) is needed in each of the microenvironments at the time when the person is present in order to estimate the accumulated dose in the human body, etc. So this approach also suffers from similar data problems to the more conventional dispersion models. On the other hand, such challenges may indicate that the approach of modelling traffic-related air pollution and its implications for public health impact assessment through mobile phone tracking will require broad cooperation within inter- and multidisciplinary disciplines.
We conclude that there is an opportunity to calculate traffic air pollution and its public health impact by mobile phone trajectory mapping. This approach is promising due to the following characteristics: (i) Low cost : There is no cost from the observation part. The cost is mainly in data power to compute mobile phone trajectories and emission dispersion modelling in real time. Combination of low cost and standardized mobile phone data makes it is feasible to do population-wide traffic pollution studies. The system is suited for developing countries that have a low fraction of mobile phones with GPS. (ii) Near real-time : The system is based on fully automatic calculation and real-time data. One may obtain real-time pollution/exposure fields. This allows identification of high vehicle density congestion events that may contribute significantly to health risk (iii) Effortless citizen participation : Each trajectory reflects one individual citizen. Therefore, this system opens up the possibility of providing information to each individual citizen. One may develop applications for automatic web server feedback to citizens who want to get the data of their own trajectories or want to contribute toward a training data set of true ground GPS measurements. We believe that using the trajectories of individual mobile phones opens up new perspectives on urban dynamics and public health research.
Abbreviations
AOA: Angle of arrival; AOD: Aerosol optical depth; AVI: Automatic vehicle identification; CDR: Call detailed records; CitiSense: Adaptive services for community-driven behavioural and environmental monitoring to induce change; CO: Carbon oxides; EFT: Emission factors toolkit; EHR: Electronic health records; EMFAC: EMission FACtors; GASP: GEOS aerosol/smoke product; GE: The general electric company; GeoPKDD: Geographic privacy-aware knowledge discovery and delivery; GOES: Geostationary operational environmental satellite; GPS: The global positioning system; GSM: The global system for mobile communications; HBEFA: The handbook emission factors for road transport; HC: Hydrogen carbon; HMM: Hidden markov model; ICT: Information communication technology; IMS: Intelligent mechatronic systems inc; LUR: Land use regression; LBS: Location-based services; MIT: The massachusetts institute of technology; MODIS: Moderate resolution imaging spectro-radiometer; MOVES: Motor vehicle emission simulator; NO2: Nitrogen dioxides; NOx: Nitrogen oxides; OMI: Ozone monitoring instrument; Pb: Lead; PEIR: The personal environmental impact report; PHR: Personal health records; PM: Particulate matter; RFID: Radio frequency identification; SO2: Sulphur dioxides; SOx: Oxides of sulphur; TDOA: Time difference of arrival; TOA: Time of arrival; TROPOMI: The TROPOspheric Monitoring Instrument; TSP: Total suspended particulates; UC: University of California; UI: Urban informatics; UN: The United Nations; USC: The University of Southern California; U.S. EPA: The United State environmental protection agency; VWF: Vehicle wake factor; WHO: The World Health Organization; WSN: Wireless sensor network.
Competing interests
The authors declare that they have no competing interests.
Authors’ contributions
HYL and ES planed this work and wrote the manuscript. MJK contributed content and provided editorial input. All authors approved the final version.
Acknowledgements
This study was inspired by the EC FP7 Work Program 2012 Cooperation Theme 6 Environment (including climate change), call ENV.2012.6.5-1-Developing community-based environmental monitoring and information systems using innovative and novel earth observation applications. We would like to thank the referees IIan Levy and Michael Jerrett for their very valuable comments. Special thanks to William A. Lahoz, Alice Newton, Sonja Grossberndt at NILU (Norwegian Institute for Air Research) for helping us with the language. Thanks to Philipp Schneider at NILU for his help to revise the paragraph on how remote sensing from satellite improve the spatial resolution of air pollutants in urban areas. The initial proof of this manuscript benefited from the suggestions and comments from Alena Bartonova and Li Liu from NILU. Any remaining errors are the responsibility of the authors.
- UN report. World’s biggest cities merging into 'mega-regions’ [ http://www.guardian.co.uk/world/2010/mar/22/un-cities-mega-regions ]
- HEI Panel on the Health Effects of Traffic-Related Air Pollution. Traffic-related air pollution: a critical review of the literature on emissions, exposure, and health effects. Boston, Massachusetts: Special Report 17; 2010. [ Google Scholar ]
- Padmanabhan N, Glenn B. EPA research focus – health effects of near-roadway air pollution. [ http://pubs.awma.org/gsearch/em/2009/8/padmanabhan.pdf ]
- Fruin S, Westerdahl D, Sax T, Sioutas C, Fine PM. Measurements and predictors of on-road ultrafine particle concentrations and associated pollutants in Los Angeles. Atmos Environ. 2008; 42 :207–219. doi: 10.1016/j.atmosenv.2007.09.057. [ CrossRef ] [ Google Scholar ]
- Rank J, Folke J, Jespersen PH. Differences in cyclists and car drivers’ exposure to air pollution from traffic in the city of Copenhagen. Sci Total Environ. 2001; 279 :131–136. doi: 10.1016/S0048-9697(01)00758-6. [ PubMed ] [ CrossRef ] [ Google Scholar ]
- van Wijnen JH, Verhoeff AP, Jans HW, van Bruggen M. The exposure of cyclists, car drivers and pedestrians to traffic-related air pollutants. Int Arch Occup Environ Health. 1995; 67 :187–93. [ PubMed ] [ Google Scholar ]
- Soll-Johanning H, Bach E, Olsen JH, Tuchsen F. Cancer incidence in urban bus drivers and tramway employees: a retrospective cohort study. Occup Environ Med. 1998; 55 :594–598. doi: 10.1136/oem.55.9.594. [ PMC free article ] [ PubMed ] [ CrossRef ] [ Google Scholar ]
- WHO. More die from car pollution than road accidents. [ http://www.ibike.org/environment/air-pollution.htm ]
- Pope CA, Dockery DW. Health effects of fine particulate air pollution: lines that connect. J Air Waste Manage Assoc. 2006; 56 :709–742. doi: 10.1080/10473289.2006.10464485. [ PubMed ] [ CrossRef ] [ Google Scholar ]
- Halonen JI, Lanki T, Yli-Tuomi T, Kulmala M, Tiittanen P, Pekkanen J. Urban air pollution and asthma and COPD hospital emergency room visits. Thorax. 2008; 63 :574–476. doi: 10.1136/thx.2008.096073. [ PubMed ] [ CrossRef ] [ Google Scholar ]
- Sørensen M, Hoffmann B, Hvidberg M, Ketzel M, Jensen SS, Andersen ZJ, Tjønneland A, Overvad K, Raaschou-Nielsen O. Long-term exposure to traffic-related air pollution associated with blood pressure and self-reported hypertension in a Danish cohort. Environ Health Perspect. 2012; 120 :418–24. doi: 10.1289/ehp.1103631. [ PMC free article ] [ PubMed ] [ CrossRef ] [ Google Scholar ]
- Raducan GM. Pollutant dispersion modelling with OSPM in a street canyon from Bucharest. Romanian Report in Physics. 2008; 60 :1099–1114. [ Google Scholar ]
- Clougherty JE, Wright RJ, Baxter LK, Levy JI. Land use regression modelling of intra-urban residential variability in multiple traffic-related air pollutants. Environ Health. 2008; 7 :17. doi: 10.1186/1476-069X-7-17. [ PMC free article ] [ PubMed ] [ CrossRef ] [ Google Scholar ]
- Henderson SB, Beckerman B, Jerrett M, Brauer M. Application of land use regression to estimate long-term concentrations of traffic-related nitrogen oxides and fine particulate matter. Environ Sci Technol. 2007; 41 :2422–2428. doi: 10.1021/es0606780. [ PubMed ] [ CrossRef ] [ Google Scholar ]
- Marshall JD, Nethery E, Brauer M. Within-urban variability in ambient air pollution: comparison of estimation methods. Atmos Environ. 2008; 42 :1359–1369. doi: 10.1016/j.atmosenv.2007.08.012. [ CrossRef ] [ Google Scholar ]
- Dijkema M, Traffic Related Air Pollution. Spatial Variation, Health Effects and Mitigation Measures. Utrecht University: Institute for Risk Assessment Sciences; 2011. (PhD thesis). [ Google Scholar ]
- UCLA CENS. PEIR: personal environmental impact report. [ http://www.viewingspace.com/peir.html ]
- Mun M, Reddy S, Shilton K, Yau N, Burke J, Estrin D, Hansen M, Howard E, West R, Boda P. In: Proceedings of MobiSys 2009: the 7th international conference on mobile systems, applications and services: 22–25, June, 2009; Wroclaw, Poland. Zielinski K, Wolisz A, Flinn J, LaMarca A, editor. New York, USA: ACM; 2009. PEIR, the personal environmental impact report, as a platform for participatory sensing systems research; pp. 55–68. [ Google Scholar ]
- Reddy S, Mun M, Burke J, Estrin D, Hansen M, Srivastava M. Using mobile phones to determine transportation modes. ACM Transactions on Sensor Networks (TOSN) 2010; 6 (13):1–13. [ Google Scholar ]
- Stenneth L, Wolfson O, Yu PS, Xu B. In: Proceedings of GIS '11: the 19th ACM SIGSPATIAl international conference on advances in geographic information systems: 1–4, November, 2011; Chicago, IL, USA. Agrawal D, Cruz I, Jensen CS, Ofek E, Tanin E, editor. New York, USA: ACM; 2011. Transportation mode detection using mobile phones and GIS information; pp. 54–63. [ Google Scholar ]
- ENVIROFI: The environmental observation web and its service applications within the future internet. [ http://www.envirofi.eu/ ]
- CitiSense. Adaptive services for community-driven behavioural and environmental monitoring to induce change. [ https://sosa.ucsd.edu/confluence/display/CitiSensePublic/CitiSense ]
- Pau G, Tse R. Challenges and opportunities in immersive vehicular sensing: lessons from urban deployments. Signal Process Image Commun. 2012; 27 :900–908. doi: 10.1016/j.image.2012.01.015. [ CrossRef ] [ Google Scholar ]
- Bales E, Nikzad N, Quick N, Ziftci C, Patrick K, Griswold W. In: Proceedings of 6th international conference on pervasive computing technologies for healthcare (Pervasive Health): 21–24, May, 2012; San Diego, California, USA. Chlamtac I, Mayora-Ibarra O, Varshney U, Osmani V, editor. New York: IEEE Xplore Digital Library; 2012. Citisense: mobile air quality sensing for individuals and communities design and deployment of the citisense mobile air-quality system; pp. 155–158. [ Google Scholar ]
- Yeung CH, Saad D. Networking – a statistical physics perspective. J Phys A Math Theor. 2013; 46 :103001. doi: 10.1088/1751-8113/46/10/103001. [ CrossRef ] [ Google Scholar ]
- Paul U, Subramanian AP, Buddhikot MM, Das SR. In: Proceedings of INFOCOM: 10–15 April 2011; Shanghai, P.R. China. Gopalan K, Striegel AD, editor. : IEEE Xplore Digital Librry; 2011. Understanding traffic dynamics in cellular data networks; pp. 882–890. [ Google Scholar ]
- Barabási A-L, Albert R. Emergence of scaling in random networks. Science. 1999; 286 :509–512. doi: 10.1126/science.286.5439.509. [ PubMed ] [ CrossRef ] [ Google Scholar ]
- Barabási A-L, Albert R. Statistical mechanics of complex networks. Rev Mod Phys. 2002; 74 :47–97. doi: 10.1103/RevModPhys.74.47. [ CrossRef ] [ Google Scholar ]
- Song C, Koren T, Wang P, Barabási A-L. Modelling the scaling properties of human mobility. Nat Phys. 2010; 10 :818–823. [ Google Scholar ]
- Wang D, Pedreschi D, Song C, Giannotti F, Barabasi AL. In: Proceedings of KDD 11, the 17th ACM SIGKDD international conference on Knowledge discovery and data mining: 21–24 August 2011; San Diego, California, USA. Chid A, Joydeep G, Padhraic S, editor. : ACM Digital Library; 2011. Human mobility, social ties, and link prediction; pp. 1100–1108. [ Google Scholar ]
- Bagrow JP, Lin Y-R. Mesoscopic structure and social aspects of human mobility. PLoS One. 2012; 7 :1–6. [ PMC free article ] [ PubMed ] [ Google Scholar ]
- Girardin F, Vaccari A, Gerber A, Biderman A, Ratti C. In: Proceedings of the 11th international conference on computers in urban planning and urban management (CUPUM2009): 16–18 June 2009; Hong Kong, P.R. China. Yeh AGO, Zhang F, editor. Hong Kong: University of Hong Kong; 2009. Towards estimating the presence of visitors from the aggregate mobile phone network activity they generate; pp. 1–11. [ Google Scholar ]
- Wang S, Min J, Yi BK. Location based services for mobiles i: technologies and standards. [ http://blue-penguin.org/cache/location-based-services-for-mobiles.pdf ]
- Wang G, van den Bosch FHM, Kuffer M. Modelling urban traffic air pollution dispersion. Int Arch Photogramm Remote Sens Spat Inf Sci. 2008; XXXVII :153–158. [ Google Scholar ]
- Thiragarajan A, Biagioni J, Gerlich T, Eriksson J. In: Proceedings of the 8th ACM conference on embedded networked sensor systems: 3–5 November 2010; Zurich, Switzerland. Beutel J, Ganesan D, Stankovic J, editor. New York: ACM; 2010. Cooperative Transit Tracking Using GPS-enabled Smart-phones; pp. 85–98. [ Google Scholar ]
- Thiragarajan A, Madden S. In: Proceedings of the ACM SIGMOD international conference on management of data, SIGMOD 2008: 10–12 June 2008; Vancouver, BC, Canada. Wang JTL, editor. New York: ACM; 2008. Querying continuous functions in a database system; pp. 791–804. [ Google Scholar ]
- Thiragarajan A, Ravindranath L, LaCurts K, Madden S, Balakrishnan H, Toledo S, Eriksson J. In: NSDI’11Proceedings of 8th USENIX Symposium on Networked Systems Design and Implementation (NSDI) USENIX Association, editor. Boston, MA, USA: Berkeley: USENIX Association; 2011. Accurate, low-energy trajectory mapping for mobile devices. [ Google Scholar ]
- Thiragarajan A, Ravindranath L, LaCurts K, Toledo S, Eriksson J, Madden S, In: Proceedings of the 7th international conference on embedded networked sensor systems, SenSys 2009: 4–6 November 2009; Berkeley, California, USA. David E, Culler , Jie L, Matt W, editor. New York: ACM; 2009. VTrack: accurate, energy-aware road traffic delay estimation using mobile phones; pp. 85–98. [ Google Scholar ]
- Thiragarajan A. Probabilistic models for mobile phone trajectory estimation. : Massachusetts Institute of Technology, Department of Electrical Engineering and Computer Science; 2011. (Dissertation). [ Google Scholar ]
- Cellint. Cellient traffic solutions making it work for you. [ http://www.cellint.com ]
- IMS. Connected car solutions for life. [ http://www.intellimec.com ]
- ITIS. Integrated transport information system. [ http://www.itis.com.my/atis/index.jsf ]
- UI. Urban informatics. [ http://www.uinformatics.com ]
- TOMTOM. [ http://www.tomtom.com/en_gb/localechange.jsp ]
- Google Maps. [ https://maps.google.com/ ]
- Herrera JC, Work D, Ban X, Herring R, Jacobson Q, Bayen A. Evaluation of traffic data obtained via GPS-enabled mobile phones: the mobile century field experiment. Transportation Research C. 2010; 18 :568–583. doi: 10.1016/j.trc.2009.10.006. [ CrossRef ] [ Google Scholar ]
- Treibner M, Kresting A. An open source microscopic traffic simulator. IEEE Intell Transp Syst Mag. 2010; 2 :6–13. [ Google Scholar ]
- Khalesian M, Pahlavani P, Delavar MR. GIS-based multi-agent traffic micro simulation for modelling the local air pollution. Int Arch Photogramm Remote Sens Spat Inf Sci. 2008; XXXVII :491–496. [ Google Scholar ]
- López-Neri E, Ramírez-Treviño A, López-Mellado E. A modelling framework for urban traffic systems microscopic simulation. Simul Model Pract Theory. 2010; 18 :1145–1161. doi: 10.1016/j.simpat.2009.09.007. [ CrossRef ] [ Google Scholar ]
- Nökel K, Schimdt M, van Vuren T. In: Proceedings of 8th world conference on transport research; volume 2: planning, operation, management and control: 12–17 July 1998; Antwerp, Belgium. Meersman H, van de Voorde E, Winkelmans W, editor. Victoria: ARRB Group Limited; 1999. An integrated dynamic traffic simulation and air pollution decision support system; pp. 237–250. [ Google Scholar ]
- Vardoulaki S, Fisher BEA, Pericleous K, Gonzalez-Flesca N. Modelling air quality in street canyons: a review. Atmos Environ. 2003; 37 :155–182. doi: 10.1016/S1352-2310(02)00857-9. [ CrossRef ] [ Google Scholar ]
- Glantz P, Kokhanovsky A, von Hoyningen-Huene W, Johansson C. Estimating PM2.5 over southern Sweden using space-borne optical measurements. Atmos Environ. 2009; 43 (36):5838–5846. doi: 10.1016/j.atmosenv.2009.05.017. [ CrossRef ] [ Google Scholar ]
- AutoPASS. [ http://www.autopass.no ]
- Siniav. [ http://siniav.net ]
- EVR. [ http://www.electronicvehicleregistration.com ]
- RFID. [ http://en.wikipedia.org/wiki/Radio-frequency_identification ]
- Bailey C. Dispersion modelling for mobile source applications. [ http://www.cleanairinfo.com/regionalstatelocalmodelingworkshop/archive/2009/presentations/06%20Thurs%20AM/Bailey_Dispersion%20Modeling%20for%20Mobile%20Source%20Applications.pdf ]
- Wang YJ, Zhang KM. Modelling near-road air quality using a computational fluid dynamics model, CFD-VIT-RIT. Environ Sci Technol. 2009; 43 :7778–7783. doi: 10.1021/es9014844. [ PubMed ] [ CrossRef ] [ Google Scholar ]
- Vardoulakis S, Fisher BEA, Pericleous K, Gonzalez-Flesca N. Modelling air quality in street canyons: a review. Atmos Environ. 2003; 37 :155–182. doi: 10.1016/S1352-2310(02)00857-9. [ CrossRef ] [ Google Scholar ]
- Time micro-environment activity modeling. [ http://www.integrated-assessment.eu/node/204 ]
- U.S. EPA. Exposure factors handbook 2011 edition (Final) Washington, DC: ; 2011. [ Google Scholar ]
- U.S. EPA report. Extrapolation of the benzene inhalation unit risk estimate to the oral route of exposure. http://www.epa.gov/iris/supdocs/benzsup.pdf .
- Belalcazar LC, Clappier A. An alternative technique to estimate road traffic emissions. [ http://www.epa.gov/ttnchie1/conference/ei20/session7/lbelalcazar_pres.pdf ]
- Jerrett M, Arain A, Kanaroglou P, Beckerman B, Potoglou D, Sahsuvaroglu T, Morrison J, Giovis C. A review and evaluation of intraurban air pollution exposure models. J Expo Anal Environ Epidemiol. 2005; 15 :185–204. doi: 10.1038/sj.jea.7500388. [ PubMed ] [ CrossRef ] [ Google Scholar ]
- Liu LJ, Delfino R, Koutrakis P. Ozone exposure assessment in a southern California community. Environ Health Perspect. 1997; 105 :58–65. doi: 10.1289/ehp.9710558. [ PMC free article ] [ PubMed ] [ CrossRef ] [ Google Scholar ]
- Zmirou D, Gauvin S, Pin I. et al. Five epidemiological studies on transport and asthma: objectives, design and descriptive results. J Expos Anal Environ Epidemiol. 2002; 12 :186–196. doi: 10.1038/sj.jea.7500217. [ PubMed ] [ CrossRef ] [ Google Scholar ]
- Hoek G, Fischer P, Van Den BP, Goldbohm S, Brunekreef B. Estimation of long-term average exposure to outdoor air pollution for a cohort study on mortality. J Expos Anal Environ Epidemiol. 2001; 11 :459–469. doi: 10.1038/sj.jea.7500189. [ PubMed ] [ CrossRef ] [ Google Scholar ]
- Bellander T, Berglind N, Gustavsson P, Jonson T, Nyberg F, Pershagen G, Järup L. Using geographic information systems to assess individual historical exposure to air pollution from traffic and house heating in Stockholm. Environmental Health Perspective. 2001; 109 :633–639. [ PMC free article ] [ PubMed ] [ Google Scholar ]
- U.S. EPA. MOVES (Motor Vehicle Emission Simulator) [ http://www.epa.gov/otaq/models/moves/index.htm ] [ PubMed ]
- Defra. Emissions. [ http://laqm.defra.gov.uk/review-and-assessment/tools/emissions.html#eft ]
- IEHIAS. emission factors. [ http://www.integrated-assessment.eu/guidebook/emission_factors ]
- Handbook emission factor for road transport (HEEFA) http://www.hbefa.net/e/index.html .
- California Air Resources Board. EMFAC software. [ http://www.dot.ca.gov/hq/env/air/pages/emfac.htm ]
- Park JY. Microscopic modelling of air pollution from road traffic. Imperial College London: University of London Centre for Transport Studies; 2005. (PhD thesis). [ Google Scholar ]
- Wang Y, Zhang KM. Modelling near-road air quality using computational fluid dynamics (CFD) model. ES&T. 2009; 43 :7778–7783. [ PubMed ] [ Google Scholar ]
- Bartonova A, Clench-Aas J, Gram F, Gronskei KE, Guerreiro C, Larssen S, Tønnesen DA, Walker SE. Air pollution exposure monitoring and estimation: Part V. Traffic exposure in adults. J Environ Monit. 1999; 1 :337–340. doi: 10.1039/a902780g. [ PubMed ] [ CrossRef ] [ Google Scholar ]
- Kerner BS. Introduction to modern traffic flow theory and control. Springer: The Long Road to Three-Phase Traffic Theory; 2009. [ Google Scholar ]
- Gokhale S, Khare M. Vehicle wake factor for heterogeneous traffic in urban environments. Int J Environ Pollut. 2007; 30 :97–105. doi: 10.1504/IJEP.2007.014505. [ CrossRef ] [ Google Scholar ]
- Ma Y, Richards M, Ghanem M, Guo Y, Hassard J. Air pollution monitoring and mining based on sensor grid in London. Sensors. 2008; 8 :3601–3623. doi: 10.3390/s8063601. [ PMC free article ] [ PubMed ] [ CrossRef ] [ Google Scholar ]
- Khedo KK, Perseedoss R, Mungur A. A wireless sensor network air pollution monitoring system. Int J Wireless Mobile Network. 2010; 2 :31–45. [ Google Scholar ]
- Mishra SA, Tijare DS, Asutkar GM. Design of energy aware air pollution monitoring system using WSW. Int J Adv Eng Tech. 2011; 1 :107–116. [ Google Scholar ]
- Demin Wang D, Agrawal DP, Toruksa W, Chaiwatpongsakorn C, Lu M, Keener TC. Monitoring ambient air quality with carbon monoxide sensor-based wireless network. Commun ACM. 2010; 53 :138–141. [ Google Scholar ]
- Tracking Air Pollution by Cell Phone. [ http://pollutionfree.wordpress.com/2009/12/15/tracking-air-pollution-by-cell-phone/ ]
- Schreiner C, Branzila M, Trandabat A, Ciobanu RC. Air quality and pollution mapping system, using remote measurements and GPS technology. Global NEST Journal. 2006; 8 :315–323. [ Google Scholar ]
- Trandabăţ A, Branzila M, Donciu C, Pîslaru M, Ciobanu RC. Using GPS technology and distributed measurement system for air quality mapping of residential area. Environ Eng Manag J. 2007; 6 :545–548. [ Google Scholar ]
- Al-Ali AR. A mobile GPRS-sensors array for air pollution monitoring. Sensors Journal, IEEE. 2010; 10 :1666–1671. [ Google Scholar ]
- Sherwood CH. Using GPS and a smart phone to track air pollution exposure. [ http://www.smartplanet.com/blog/pure-genius/using-gps-and-a-smartphone-to-track-air-pollution-exposure/5578 ]
- Science Daily. Smartphone app measures particulate air pollution. [ http://www.sciencedaily.com/releases/2010/09/100920135137.htm ]
- Bayir MA, Demirbas M, Cosar A. A web-based personalized mobility service for smartphone applications. Comput J. 2011; 54 :800–814. doi: 10.1093/comjnl/bxq027. [ CrossRef ] [ Google Scholar ]
- Wang D, Pedreschi D, Song C, Giannotti F, Barabasi AL. In: Proceedings of KDD '11 the 17th ACM SIGKDD international conference on Knowledge discovery and data mining: 21–24 August 2011; San Diego, California, USA. Apte C, Ghosh J, Smyth P, editor. New York: ACM; 2011. Human mobility, social ties, and link prediction; pp. 1100–1108. [ Google Scholar ]
- Fitzgerald E, Wartenberg D, Thompson WD, Houston A. Birth and fetal death records and environmental exposures: promising data elements for environmental public health tracking of reproductive outcomes. Public Health Rep. 2009; 124 (6):825–830. [ PMC free article ] [ PubMed ] [ Google Scholar ]
- Environmental Exposure Modules for EHR and PHR Systems. [ http://www.hoise.com/vmw/10/articles/vmw/LV-VM-08-10-6.html ]
- Environmental Exposure & EHR Systems. [ http://www.openhealthnews.com/blogs/groenpj/2011-04-11/environmental-exposure-ehr-systems ]
- Fanchi J. Principles of Applied Reservoir Simulation. 3. Burlington: Elsevier GPP; 2006. [ Google Scholar ]
- Nikzad N, Verma N, Ziftci C, In: Proceedings of Wireless Health: 23-25 October 2012; San Diego, Califonia, USA. Raij A, Venkatasubramanian K, editor. New York: ACM; 2012. CitiSense: Improving Geospatial Environmental Assessment of Air Quality Using a Wireless Personal Exposure Monitoring System; pp. 1–8. [ Google Scholar ]
- Giannotti F, Pedreschi D. In: Mobility, data mining and privacy: geographic knowledge discovery. Giannotti F, Pedreschi D, editor. New York: Springer; 2008. Mobility, data mining and privacy: a vision of convergence; pp. 1–14. [ Google Scholar ]
- Bonchi F, Saygin Y, Verykios VS, Atzori M, Gkoulalas-Divanis A, Kaya SV, Savas E. In: Mobility, data mining and privacy: geographic knowledge discovery. Giannotti F, Pedreschi D, editor. New York: Springer; 2010. Privacy in Spatiotemporal Data Mining; pp. 297–334. [ Google Scholar ]
- Ziftci C, Nikzad N, Verma N, In: Proceedings of the third annual conference on systems, programming, and applications: software for humanity (SPLASH’12): 19-26 October 2012;Tucson, Arizona. Leavens GT, editor. New York: ACM Digital Library; 2012. Citisense: mobile air quality sensing for individuals and communities; pp. 23–24. [ Google Scholar ]
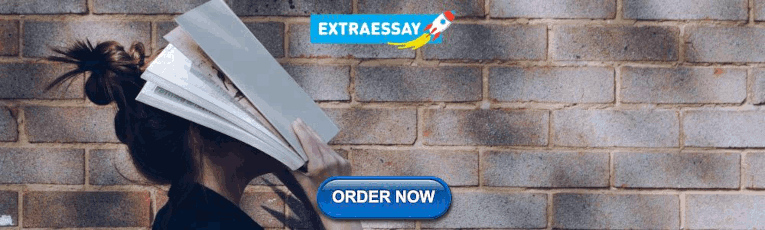
IMAGES
VIDEO
COMMENTS
This study demonstrates a methodology for analyzing the health risks attributable to traffic, specifically using a marginal analysis that shows the effect of incremental increases in traffic volume. To our knowledge, this appears to be the first study examining health risks attributable to congestion-related air pollution using this approach.
When modeling these effects it is important that the models must be epidemiologically meaningful and robust (that is, insensitive to variations in the model parameters). The objective of this paper is to propose a methodology for the assessment of the health impact of air pollution. The proposed methodology involves the construction of models ...
Air quality traffic-related measures have been implemented worldwide to control the pollution levels of urban areas. Although some of those measures are claiming environmental improvements, few studies have checked their real impact. In fact, quantitative estimates are often focused on reducing emis …
Although emerging studies have investigated the effect of air pollution on traffic crashes, it is unclear to scholars whether air pollution affects another road safety problem—traffic violations. To address this gap, the current paper constructs a data set from 1,390,221 traffic violation records of 640,971 drivers from the Wuhan Traffic Management Bureau between January 2018 and December ...
The research on traffic-related air pollution (TRAP) and human health has greatly evolved in the past few decades leading to improved practices and policy decision-making in many regions. Significant advances have been made including advancing the methods to assess traffic activity, vehicle emissions, air pollution, and human exposures.
Multiple studies have concluded that traffic police are highly stressed. A number of occupational factors have been attributed to stress among traffic police. Occupational health studies help us to understand the effects of vehicular pollution and its adverse influence on workers. They also provide opportunity for defined exposures measurements ...
The global concern of escalating ambient air pollution and its profound impact on human health is paramount. While traffic police personnel are critical for maintaining the road safety and transportation system of any city in India, they are susceptible to occupational health risks due to ambient air pollution. This study investigated health challenges faced by traffic police personnel due to ...
DOI: 10.1016/j.envpol.2018.04.061 Corpus ID: 21733957; A proposed methodology for impact assessment of air quality traffic-related measures: The case of PM2.5 in Beijing. @article{Fontes2018APM, title={A proposed methodology for impact assessment of air quality traffic-related measures: The case of PM2.5 in Beijing.}, author={T{\^a}nia Fontes and Peilin Li and Nelson Azevedo Barros and Pengjun ...
Understanding the health implications of air pollution on traffic police personnel is crucial for various reasons. This study investigated the specific health outcomes experi-enced by traffic police personnel in Kolkata by assessing both the short-term and long-term effects of air pollution exposures. 2. Materials and Methods
RFA 23-1 is seeking to fund studies to assess health effects of long-term exposure to TRAP. Studies should propose novel or improved methods and approaches to evaluate exposure to and health effects of traffic-related air pollutants as technologies and fuels change, the fleet turns over, mobility transforms, and electrification makes greater inroads.
1. Introduction. Air pollution has risen to the fourth largest cause of early death, according to the 'State of Global Air (2020) Report' (Institute, 2020).This effect is likely to be observed for the foreseeable future as the majority of Asia, Africa, and the Middle East's middle- and low-income countries continue to have rising levels of air pollution, including increased particulate ...
We propose a new approach to assess the impact of traffic-related air pollution on public health by mapping personal trajectories using mobile phone tracking technology in an urban environment. Although this approach is not based on any empirical studies, we believe that this method has great potential and deserves serious attention. Mobile phone tracking technology makes it feasible to ...
BackgroundDisproportionate life stress and consequent physiologic alteration (i.e., immune dysregulation) has been proposed as a major pathway linking socioeconomic position, environmental exposures, and health disparities. Asthma, for example, disproportionately affects lower-income urban communities, where air pollution and social stressors may be elevated.ObjectivesWe aimed to examine the ...
Abstract. Traffic-related air pollution (TRAP) is ubiquitous in today's cities and a major public health concern resulting in premature mortality and a wide spectrum of global diseases. The importance and relevance of TRAP continue to increase with increasing urbanization and rapid population growth which go hand in hand with increasing ...
Many of us experience some kind of air pollution-related symptoms such as watery eyes, coughing, or wheezing. The actual risk depends on the person's current health status, the pollutant type and concentration, and the length of exposure to the polluted air. Those most vulnerable are - Outdoor workers (Traffic Police, Auto/Bus/Taxi Drivers ...
[email protected]. Tel: (+98 61) 33362536. Fax: (+98 61) 33361544. ABSTRACT: Number of scienti c studies linking possible effects of air pollution on health. are increasing. However, the ...
exposure to environmental air pollution including vehicle exhausts have any adverse effects on the traffic police officers' lung functions. Methods: This cross-sectional study was conducted from July 2020 to July 2022 among 226 traffic police personnel, aged 18 ±55 years,working in Pune city at various traffic junctions. Measurement of ...
Background: The purpose of our study was to evaluate the effects of urban pollution on the lipid balance of members of a municipal police force in a big city. Subjects and methods: Our case-control study comprised 118 male members of the police force performing traffic duties, and 118 blood donors who perform office work, and was paired by age and length of service.
Traffic police officers are at excessive risk for adverse health effects of air pollution compared to general population. Aim: To analyse the Cardiorespiratory Fitness in Traffic Police Officers.Methodology:60 samples in the age group of 18-58 years were selected based on inclusion and exclusion criteria from traffic police department of Pune.
BACKGROUND: The purpose of our study was to evaluate the effects of urban pollution on the lipid balance of members of a municipal police force in a big city. SUBJECTS AND METHODS: Our case-control study comprised 118 male members of the police force ...
Two traffic measures implemented in the city of Beijing are selected to demonstrate its applicability. The next sections present a detailed description of the methodology proposed as well as the data collected to assess it. 2.1. Method. There are several factors playing an important role on atmospheric transport and dispersion of air pollution.
The results suggest that some chemical agents contained in the urban pollution of a big city could cause dyslipidemia among people exposed to such air pollution. BACKGROUND The purpose of our study was to evaluate the effects of urban pollution on the lipid balance of members of a municipal police force in a big city. SUBJECTS AND METHODS Our case-control study comprised 118 male members of ...
An overview of work process for modelling traffic-related air pollution contribution to individual exposure by mobile phone tracking. It is composed of six parts: ① Pollution monitoring using stationary sites; ② Trajectory mapping by mobile phone; ③ Automatic vehicle type identification or estimation; ④ Vehicle trajectories and vehicle emissions; ⑤ Pollution field modelling; and ⑥ ...
traffic signals to reduce accidents, traffic delays, fuel consumption, and air pollution, while improving safety, increasing capacity, and enhancing intersection aesthetics. FHWA research. 17. indicates that emission rates at roundabouts can be lower than at intersections, although reductions are varied depending on specific conditions at each ...