High Impact Tutoring Built By Math Experts
Personalized standards-aligned one-on-one math tutoring for schools and districts
Free ready-to-use math resources
Hundreds of free math resources created by experienced math teachers to save time, build engagement and accelerate growth
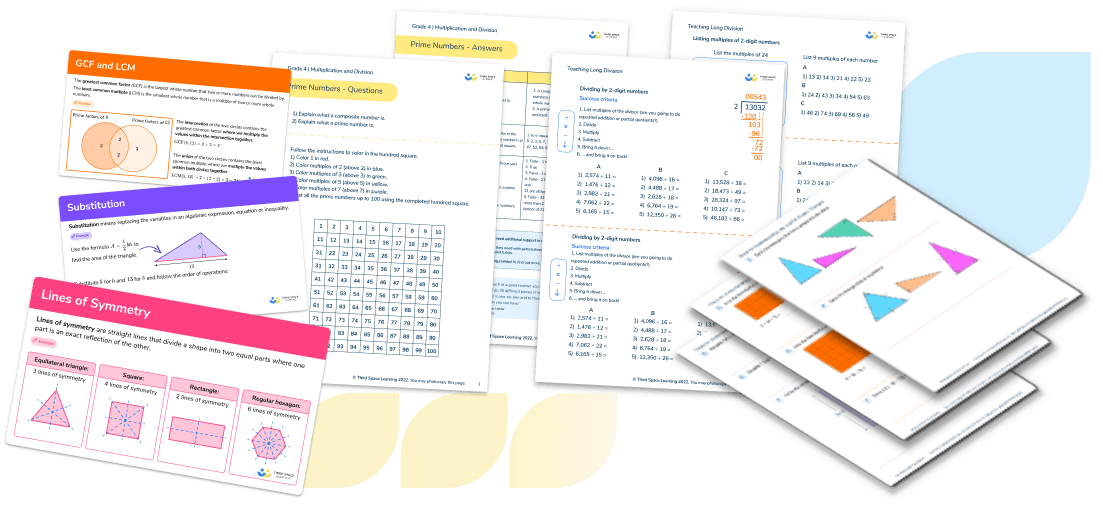
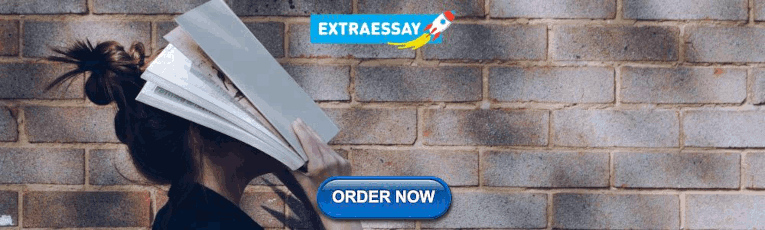
Fluency, Reasoning and Problem Solving: What This Looks Like In Every Math Lesson
Neil Almond
Fluency, reasoning and problem solving are central strands of mathematical competency, as recognized by the National Council of Teachers of Mathematics (NCTM) and the National Research Council’s report ‘Adding It Up’.
They are key components to the Standards of Mathematical Practice, standards that are interwoven into every mathematics lesson. Here we look at how these three approaches or elements of math can be interwoven in a child’s math education through elementary and middle school.
We look at what fluency, reasoning and problem solving are, how to teach them, and how to know how a child is progressing in each – as well as what to do when they’re not, and what to avoid.
The hope is that this blog will help elementary and middle school teachers think carefully about their practice and the pedagogical choices they make around the teaching of what the common core refers to as ‘mathematical practices’, and reasoning and problem solving in particular.
Before we can think about what this would look like in Common Core math examples and other state-specific math frameworks, we need to understand the background to these terms.
What is fluency in math?
What is reasoning in math, what is problem solving in math, mathematical problem solving is a learned skill, performance vs learning: what to avoid when teaching fluency, reasoning, and problem solving.
- What IS ‘performance vs learning’?
- Teaching to “cover the curriculum” hinders development of strong problem solving skills.
- Fluency and reasoning – Best practice in a lesson, a unit, and a semester
Best practice for problem solving in a lesson, a unit, and a semester
Fluency, reasoning and problem solving should not be taught by rote .
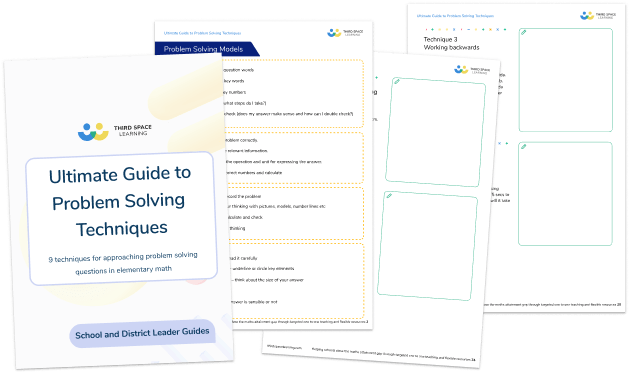
The Ultimate Guide to Problem Solving Techniques
Develop problem solving skills in the classroom with this free, downloadable worksheet
Fluency in math is a fairly broad concept. The basics of mathematical fluency – as defined by the Common Core State Standards for math – involve knowing key mathematical skills and being able to carry them out flexibly, accurately and efficiently.
But true fluency in math (at least up to middle school) means being able to apply the same skill to multiple contexts, and being able to choose the most appropriate method for a particular task.
Fluency in math lessons means we teach the content using a range of representations, to ensure that all students understand and have sufficient time to practice what is taught.
Read more: How the best schools develop math fluency
Reasoning in math is the process of applying logical thinking to a situation to derive the correct problem solving strategy for a given question, and using this method to develop and describe a solution.
Put more simply, mathematical reasoning is the bridge between fluency and problem solving. It allows students to use the former to accurately carry out the latter.
Read more: Developing math reasoning: the mathematical skills required and how to teach them .
It’s sometimes easier to start off with what problem solving is not. Problem solving is not necessarily just about answering word problems in math. If a child already has a readily available method to solve this sort of problem, problem solving has not occurred. Problem solving in math is finding a way to apply knowledge and skills you have to answer unfamiliar types of problems.
Read more: Math problem solving: strategies and resources for primary school teachers .
We are all problem solvers
First off, problem solving should not be seen as something that some students can do and some cannot. Every single person is born with an innate level of problem-solving ability.
Early on as a species on this planet, we solved problems like recognizing faces we know, protecting ourselves against other species, and as babies the problem of getting food (by crying relentlessly until we were fed).
All these scenarios are a form of what the evolutionary psychologist David Geary (1995) calls biologically primary knowledge. We have been solving these problems for millennia and they are so ingrained in our DNA that we learn them without any specific instruction.
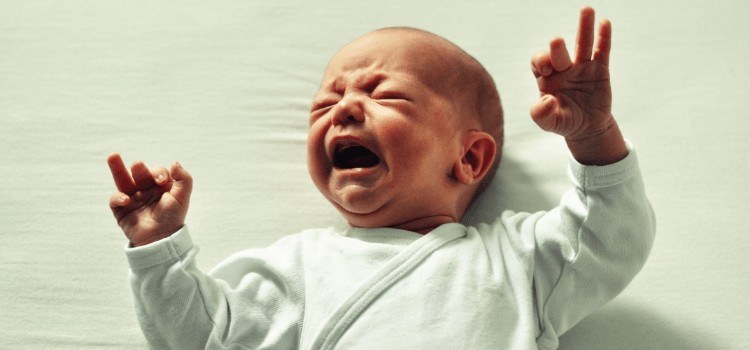
Why then, if we have this innate ability, does actually teaching problem solving seem so hard?
As you might have guessed, the domain of mathematics is far from innate. Math doesn’t just happen to us; we need to learn it. It needs to be passed down from experts that have the knowledge to novices who do not.
This is what Geary calls biologically secondary knowledge. Solving problems (within the domain of math) is a mixture of both primary and secondary knowledge.
The issue is that problem solving in domains that are classified as biologically secondary knowledge (like math) can only be improved by practicing elements of that domain.
So there is no generic problem-solving skill that can be taught in isolation and transferred to other areas.
This will have important ramifications for pedagogical choices, which I will go into more detail about later on in this blog.
The educationalist Dylan Wiliam had this to say on the matter: ‘for…problem solving, the idea that students can learn these skills in one context and apply them in another is essentially wrong.’ (Wiliam, 2018) So what is the best method of teaching problem solving to elementary and middle school math students?
The answer is that we teach them plenty of domain specific biological secondary knowledge – in this case, math. Our ability to successfully problem solve requires us to have a deep understanding of content and fluency of facts and mathematical procedures.
Here is what cognitive psychologist Daniel Willingham (2010) has to say:
‘Data from the last thirty years leads to a conclusion that is not scientifically challengeable: thinking well requires knowing facts, and that’s true not simply because you need something to think about.
The very processes that teachers care about most—critical thinking processes such as reasoning and problem solving—are intimately intertwined with factual knowledge that is stored in long-term memory (not just found in the environment).’
Colin Foster (2019), a reader in Mathematics Education in the Mathematics Education Center at Loughborough University, UK, says, ‘I think of fluency and mathematical reasoning, not as ends in themselves, but as means to support students in the most important goal of all: solving problems.’
In that paper he produces this pyramid:
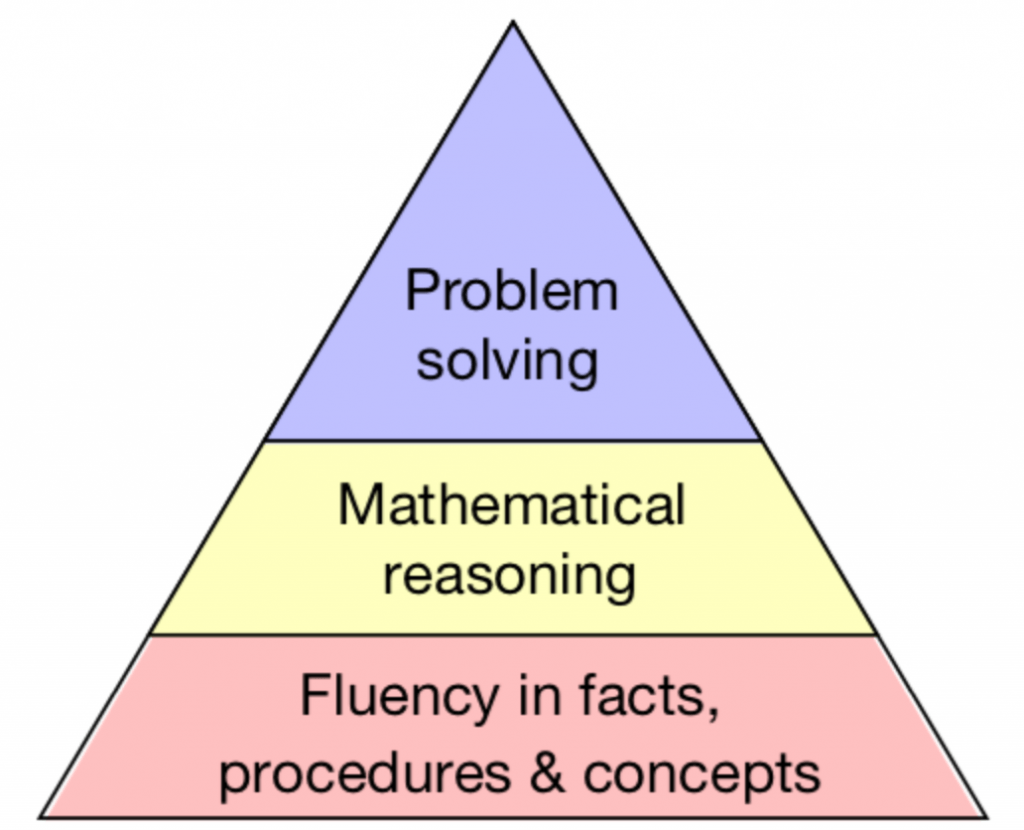
This is important for two reasons:
1) It splits up reasoning skills and problem solving into two different entities
2) It demonstrates that fluency is not something to be rushed through to get to the ‘problem solving’ stage but is rather the foundation of problem solving.
In my own work I adapt this model and turn it into a cone shape, as education seems to have a problem with pyramids and gross misinterpretation of them (think Bloom’s taxonomy).
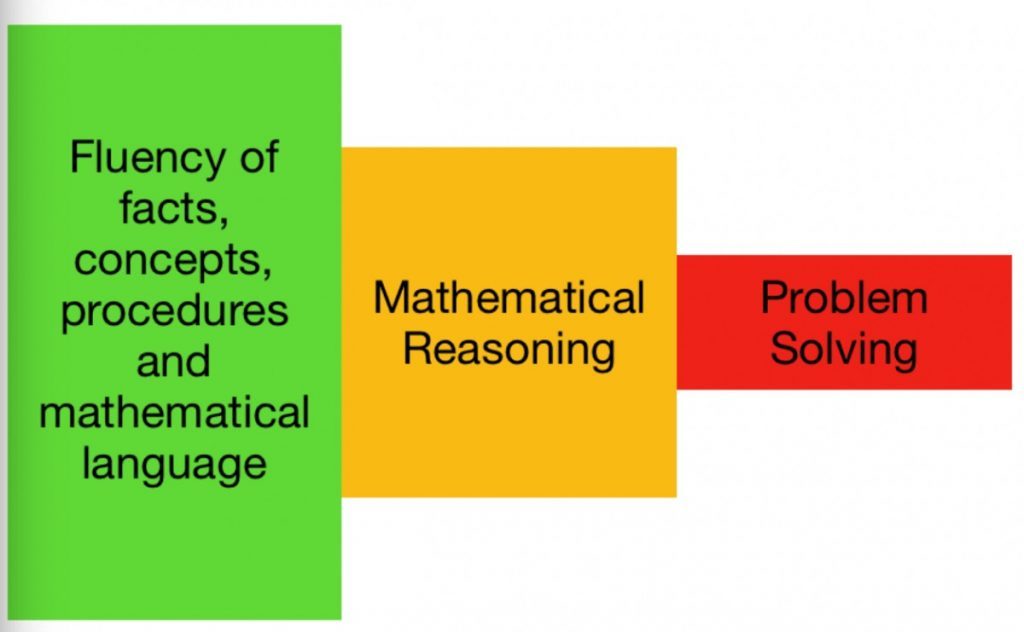
Notice how we need plenty of fluency of facts, concepts, procedures and mathematical language.
Having this fluency will help with improving logical reasoning skills, which will then lend themselves to solving mathematical problems – but only if it is truly learnt and there is systematic retrieval of this information carefully planned across the curriculum.
I mean to make no sweeping generalization here; this was my experience both at university when training and from working in schools.
At some point, schools become obsessed with the ridiculous notion of moving students through content at an accelerated rate. I have heard it used in all manner of educational contexts while training and being a teacher. ‘You will need to show ‘accelerated progress in math’ in this lesson,’ ‘School officials will be looking for ‘accelerated progress’ etc.
I have no doubt that all of this came from a good place and from those wanting the best possible outcomes – but it is misguided.
I remember being told that we needed to get students onto the problem solving questions as soon as possible to demonstrate this mystical ‘accelerated progress’.
This makes sense; you have a group of students and you have taken them from not knowing something to working out pretty sophisticated 2-step or multi-step word problems within an hour. How is that not ‘accelerated progress?’
This was a frequent feature of my lessons up until last academic year: teach a mathematical procedure; get the students to do about 10 of them in their books; mark these and if the majority were correct, model some reasoning/problem solving questions from the same content as the fluency content; give the students some reasoning and word problem questions and that was it.
I wondered if I was the only one who had been taught this while at university so I did a quick poll on Twitter and found that was not the case.
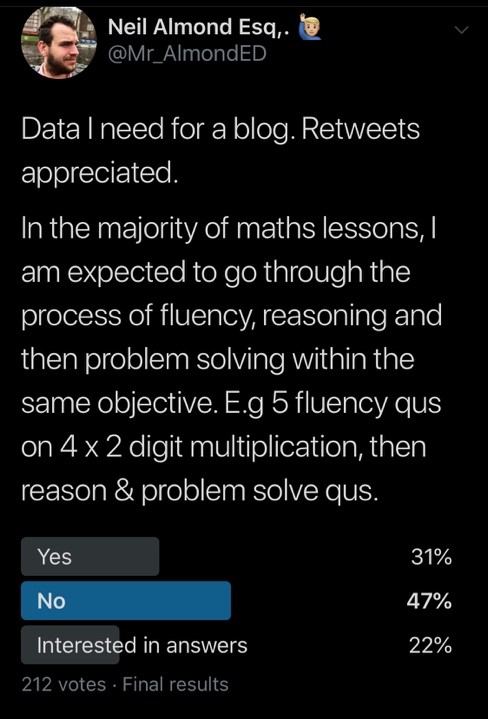
I know these numbers won’t be big enough for a representative sample but it still shows that others are familiar with this approach.
The issue with the lesson framework I mentioned above is that it does not take into account ‘performance vs learning.’
What IS ‘performance vs learning’?
The premise is that performance in a lesson is not a good proxy for learning.
Yes, those students were performing well after I had modeled a mathematical procedure for them, and managed to get questions correct.
But if problem solving depends on a deep knowledge of mathematics, this approach to lesson structure is going to be very ineffective.
As mentioned earlier, the reasoning and problem solving questions were based on the same math content as the fluency exercises, making it more likely that students would solve problems correctly whether they fully understood them or not.
Chances are that all they’d need to do is find the numbers in the questions and use the same method they used in the fluency section to get their answers (a process referred to as “number plucking”) – not exactly high level problem solving skills.
Teaching to “cover the curriculum” hinders development of strong problem solving skills.
This is one of my worries with ‘math mastery schemes’ that block content so that, in some circumstances, it is not looked at again until the following year (and with new objectives).
The pressure for teachers to ‘get through the curriculum’ results in many opportunities to revisit content being missed in the classroom.
Students are unintentionally forced to skip ahead in the fluency, reasoning, problem solving chain without proper consolidation of the earlier processes.
As David Didau (2019) puts it, ‘When novices face a problem for which they do not have a conveniently stored solution, they have to rely on the costlier means-end analysis.
This is likely to lead to cognitive overload because it involves trying to work through and hold in mind multiple possible solutions.
It’s a bit like trying to juggle five objects at once without previous practice. Solving problems is an inefficient way to get better at problem solving.’
Fluency and reasoning – Best practice in a lesson, a unit, and a semester
By now I hope you have realized that when it comes to problem solving, fluency is king. As such we should look to mastery math based teaching to ensure that the fluency that students need is there.
The answer to what fluency looks like will obviously depend on many factors, including the content being taught and the grade you find yourself teaching.
But we should not consider rushing them on to problem solving or logical reasoning in the early stages of this new content as it has not been learnt, only performed.
I would say that in the early stages of learning, content that requires the end goal of being fluent should take up the majority of lesson time – approximately 60%. The rest of the time should be spent rehearsing and retrieving other knowledge that is at risk of being forgotten about.
This blog on mental math strategies students should learn at each grade level is a good place to start when thinking about the core aspects of fluency that students should achieve.
Little and often is a good mantra when we think about fluency, particularly when revisiting the key mathematical skills of number bond fluency or multiplication fluency. So when it comes to what fluency could look like throughout the day, consider all the opportunities to get students practicing.
They could chant multiplication facts when transitioning. If a lesson in another subject has finished earlier than expected, use that time to quiz students on number bonds. Have fluency exercises as part of the morning work.
Read more: How to teach multiplication for instant recall
What about best practice over a longer period?
Thinking about what fluency could look like across a unit of work would again depend on the unit itself.
Look at this unit below from a popular scheme of work.
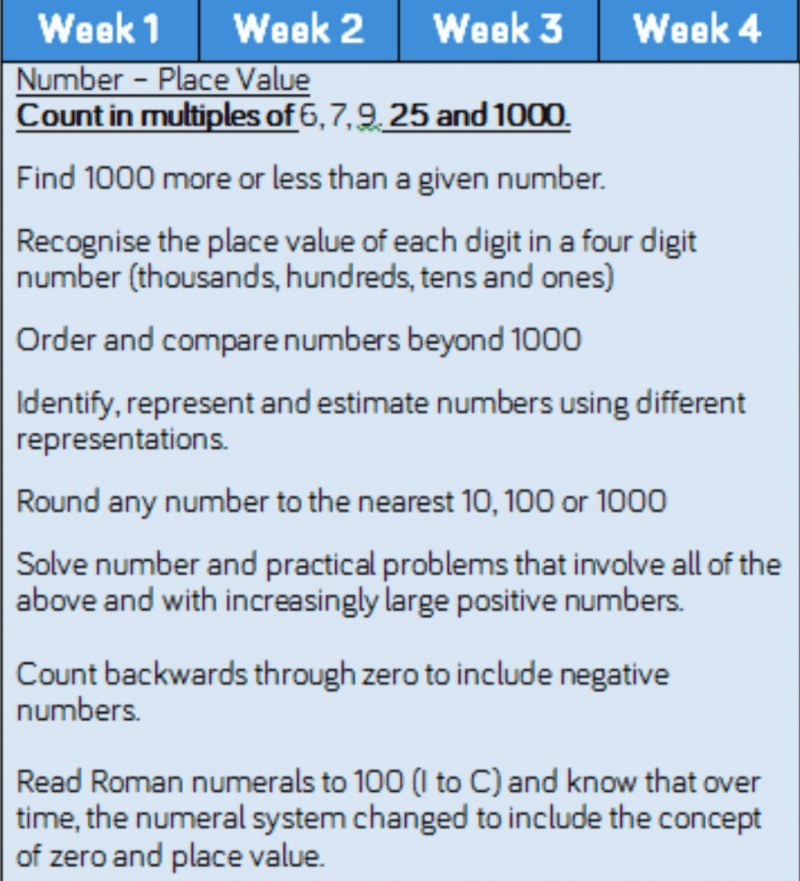
They recommend 20 days to cover 9 objectives. One of these specifically mentions problem solving so I will forget about that one at the moment – so that gives 8 objectives.
I would recommend that the fluency of this unit look something like this:
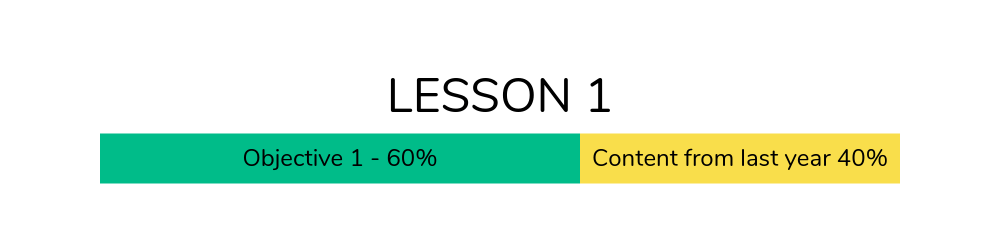
This type of structure is heavily borrowed from Mark McCourt’s phased learning idea from his book ‘Teaching for Mastery.’
This should not be seen as something set in stone; it would greatly depend on the needs of the class in front of you. But it gives an idea of what fluency could look like across a unit of lessons – though not necessarily all math lessons.
When we think about a semester, we can draw on similar ideas to the one above except that your lessons could also pull on content from previous units from that semester.
So lesson one may focus 60% on the new unit and 40% on what was learnt in the previous unit.
The structure could then follow a similar pattern to the one above.
When an adult first learns something new, we cannot solve a problem with it straight away. We need to become familiar with the idea and practice before we can make connections, reason and problem solve with it.
The same is true for students. Indeed, it could take up to two years ‘between the mathematics a student can use in imitative exercises and that they have sufficiently absorbed and connected to use autonomously in non-routine problem solving.’ (Burkhardt, 2017).
Practice with facts that are secure
So when we plan for reasoning and problem solving, we need to be looking at content from 2 years ago to base these questions on.
You could get students in 3rd grade to solve complicated place value problems with the numbers they should know from 1st or 2nd grade. This would lessen the cognitive load , freeing up valuable working memory so they can actually focus on solving the problems using content they are familiar with.
Increase complexity gradually
Once they practice solving these types of problems, they can draw on this knowledge later when solving problems with more difficult numbers.
This is what Mark McCourt calls the ‘Behave’ phase. In his book he writes:
‘Many teachers find it an uncomfortable – perhaps even illogical – process to plan the ‘Behave’ phase as one that relates to much earlier learning rather than the new idea, but it is crucial to do so if we want to bring about optimal gains in learning, understanding and long term recall.’ (Mark McCourt, 2019)
This just shows the fallacy of ‘accelerated progress’; in the space of 20 minutes some teachers are taught to move students from fluency through to non-routine problem solving, or we are somehow not catering to the needs of the child.
When considering what problem solving lessons could look like, here’s an example structure based on the objectives above.
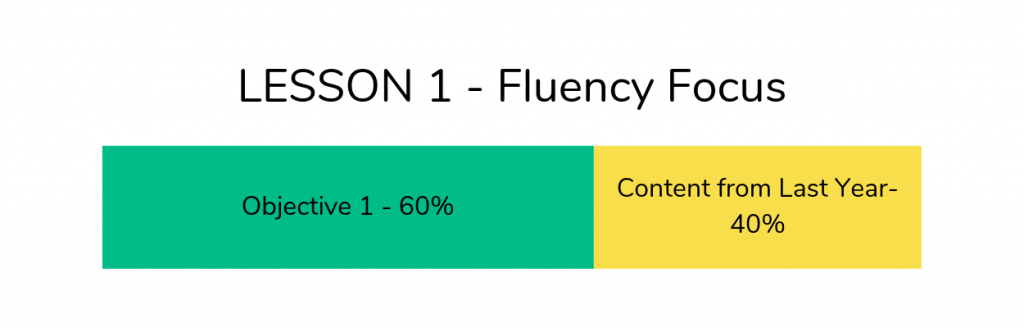
It is important to reiterate that this is not something that should be set in stone. Key to getting the most out of this teaching for mastery approach is ensuring your students (across abilities) are interested and engaged in their work.
Depending on the previous attainment and abilities of the children in your class, you may find that a few have come across some of the mathematical ideas you have been teaching, and so they are able to problem solve effectively with these ideas.
Equally likely is encountering students on the opposite side of the spectrum, who may not have fully grasped the concept of place value and will need to go further back than 2 years and solve even simpler problems.
In order to have the greatest impact on class performance, you will have to account for these varying experiences in your lessons.
Read more:
- Math Mastery Toolkit : A Practical Guide To Mastery Teaching And Learning
- Problem Solving and Reasoning Questions and Answers
- Get to Grips with Math Problem Solving For Elementary Students
- Mixed Ability Teaching for Mastery: Classroom How To
- 21 Math Challenges To Really Stretch Your More Able Students
- Why You Should Be Incorporating Stem Sentences Into Your Elementary Math Teaching
Do you have students who need extra support in math? Give your students more opportunities to consolidate learning and practice skills through personalized math tutoring with their own dedicated online math tutor. Each student receives differentiated instruction designed to close their individual learning gaps, and scaffolded learning ensures every student learns at the right pace. Lessons are aligned with your state’s standards and assessments, plus you’ll receive regular reports every step of the way. Personalized one-on-one math tutoring programs are available for: – 2nd grade tutoring – 3rd grade tutoring – 4th grade tutoring – 5th grade tutoring – 6th grade tutoring – 7th grade tutoring – 8th grade tutoring Why not learn more about how it works ?
The content in this article was originally written by primary school lead teacher Neil Almond and has since been revised and adapted for US schools by elementary math teacher Jaclyn Wassell.
Related articles
Ultimate guide to metacognition [free].
Looking for a summary on metacognition in relation to math teaching and learning?
Check out this guide featuring practical examples, tips and strategies to successfully embed metacognition across your school to accelerate math growth.
Privacy Overview
- Big Ideas Login
- Request a Sample
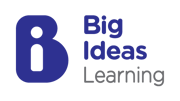
Mathematical Fluency: What Is It and Why Does It Matter?
- Judy Hickman
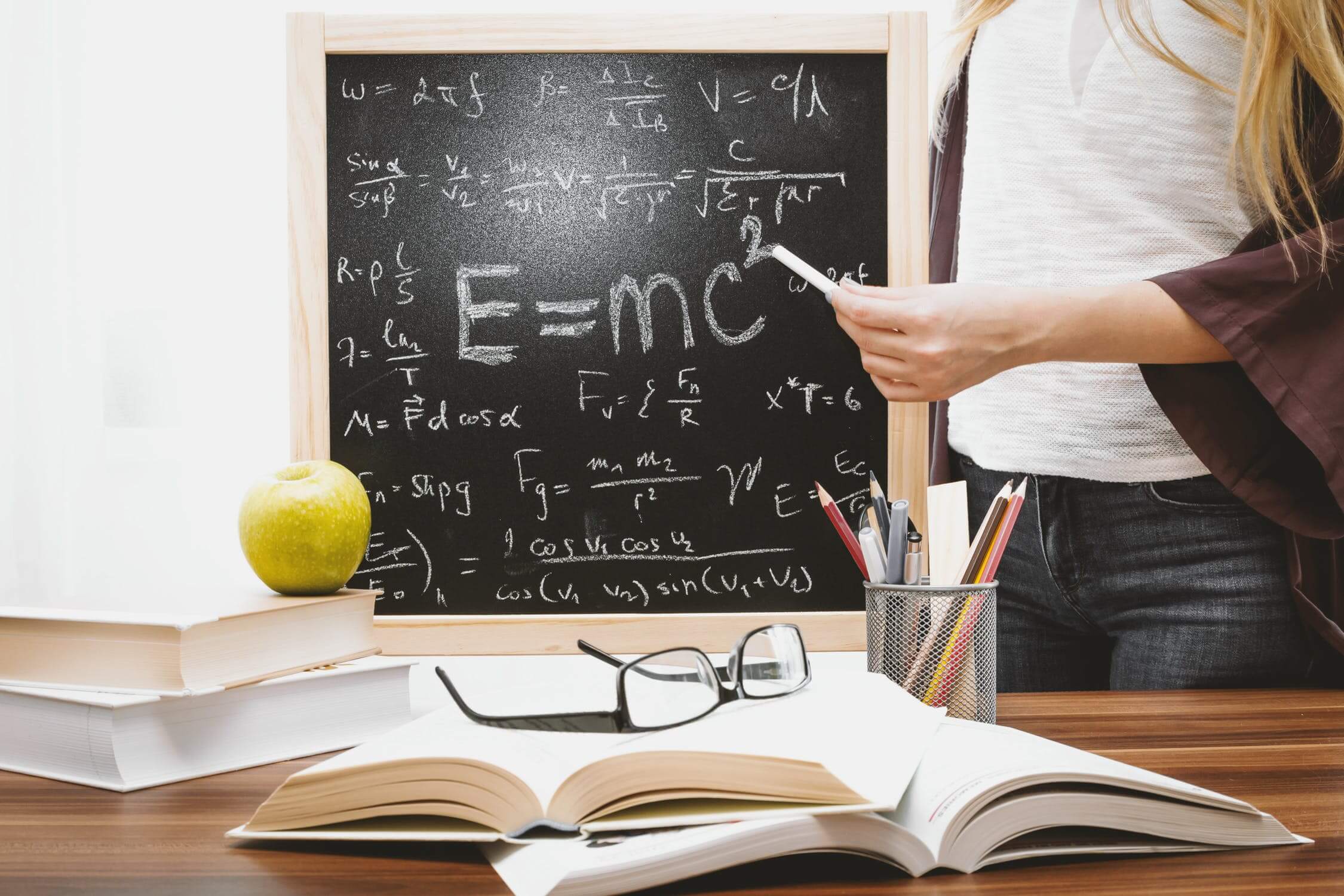
Let’s address fluency in math by defining what fluency is, why it matters, and how the three stages of fluency are defined by Florida in the B.E.S.T. Standards for Mathematics.
What is mathematical fluency.
“When we are fluent in a language, we can respond and converse without having to think too hard. The language comes naturally, and we do not use up space in our brain thinking about what word to use. Fluency comes from using the language in multiple settings, from trying things out, and failing and trying again.” – Dr. Nic, Creative Maths
This approach to fluency in any language applies to the language of mathematics, too.
In mathematics, fluency builds on a foundation of conceptual understanding, strategic reasoning, and problem-solving to achieve automaticity. Students connect conceptual understanding (Stage 1) with strategies and methods (Stage 2) and use the methods in a way that makes sense to them (Stage 3) .
When students go through these stages to build fluency, they gain an understanding of the operations and the strategies and methods in their toolbox for solving them, and they become strategic thinkers who can efficiently compute arithmetic.
Fluency is often misunderstood as being able to quickly compute basic math facts, regardless of conceptual understanding, otherwise known as memorization. But being fluent in mathematics is more than memorization, accuracy, and speed.
Accuracy goes beyond memorizing a procedure to get the right answer; it involves understanding the meaning of the procedure, applying it carefully, and checking to see if the answer makes sense. Emphasizing speed can discourage flexible thinking. True fluency is built when students are permitted to stop, think, and use strategies that make sense to efficiently solve a problem.
Why is mathematical fluency important?
By building fluency in math, students can efficiently use foundational skills to solve deeper, more meaningful problems that they encounter in the world around them. Fluency contributes to success in the math classroom and in everyday life.
For example, math fluency is useful for:
- adding scores while playing a game
- using mental math to decide the best buy while shopping at a grocery store
- estimating a percent when determining a tip for a delivery driver
- and so much more!
Throughout everyday life, fluent math thinkers use strategies and methods that they understand to efficiently compute operations and check that their answers are reasonable.
“While being fluent with math facts doesn’t make word problems easy, it does reduce the number of cognitive resources needed to tackle the computation portion of the process, allowing those resources to be allocated to other components of the process.” – Differentiated Teaching
3 Stages of Fluency Defined by Florida’s B.E.S.T. Standards for Mathematics
Let’s examine the three stages of fluency as defined by Florida’s B.E.S.T Standards for Mathematics .
Stage 1: Exploration
- Students investigate arithmetic operations to increase understanding by using manipulatives, visual models, and engaging in rich discussion.
- Models help build on prior learning and make connections between concepts.
- Exercises classified as Stage 1 will prompt students to use a model to solve.
Stage 2: Procedural Reliability
- Students utilize skills from the exploration stage to develop an accurate, reliable method that aligns with the student’s understanding and learning style.
- Students may need the teacher’s help to choose a method, and they are learning how to use a method without help.
- Students choose any method to solve problems independently. Then students are asked to describe their method to ensure that they understand the method and why it works.
Stage 3: Procedural Fluency
- Students build on their conceptual understanding from Stages 1 and 2 and use an efficient and accurate procedure to compute an operation, including the standard algorithms.
- Students are no longer asked to describe their method because they are proving that they can solve accurately and without assistance.
Note: E mbedded within Stages 1-3 is Automaticity . Automaticity is the ability to act according to an automatic response which is easily retrieved from long-term memory. It usually results from repetition and practice.
How do math programs and curriculum incorporate fluency?
When looking for a new math curriculum, districts should consider math programs that use a variety of models (Stage 1) and strategies (Stage 2) as well as standard algorithms (Stage 3) to teach math.
Practice problems should encourage the use of various methods to solve problems as well as student explanations of the methods they choose to use (Stage 2). Student exploration, collaboration, and peer discussion will also aid students in the development of their mathematical thinking.
Programs that integrate foundational mathematical thinking and reasoning skills will help students become mathematical thinkers who can strategically choose efficient methods to solve problems.
By acquiring mathematical fluency, students will have a greater cognitive capacity to solve more complex problems in the real world.
Related Articles
Understanding florida's mathematical thinking and reasoning (mtr) standards.
Topics: Florida , MTR
Oxford Education Blog
The latest news and views on education from oxford university press., the role of reasoning in supporting problem solving and fluency.
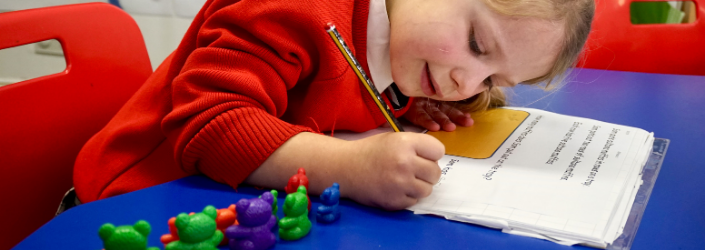
A recent webinar with Mike Askew explored the connection between reasoning, problem solving and fluency. This blog post summaries the key takeaways from this webinar.
Using reasoning to support fluency and problem solving
You’ll probably be very familiar with the aims of the National Curriculum for mathematics in England: fluency, problem-solving and reasoning. An accepted logic of progression for these is for children to become fluent in the basics, apply this to problem-solving, and then reason about what they have done. However, this sequence tends towards treating reasoning as the icing on the cake, suggesting that it might be a final step that not all children in the class will reach. So let’s turn this logic on its head and consider the possibility that much mathematical reasoning is in actual fact independent of arithmetical fluency.
What does progress in mathematical reasoning look like?
Since we cannot actually ‘see’ children’s progression in learning, in the way we can see a journey’s progression on a SatNav, we often use metaphors to talk about progression in learning. One popular metaphor is to liken learning to ‘being on track’, with the implication that we can check if children going in the right direction, reaching ‘stations’ of fluency along the way. Or we talk about progression in learning as though it were similar to building up blocks, where some ideas provide the ‘foundations’ that can be ‘built upon’.
Instead of thinking about reasoning as a series of stations along a train track or a pile of building blocks, we can instead take a gardening metaphor, and think about reasoning as an ‘unfolding’ of things. With this metaphor, just as the sunflower ‘emerges’ from the seed, so our mathematical reasoning is contained within our early experiences. A five-year-old may not be able to solve 3 divided by 4, but they will be able to share three chocolate bars between four friends – that early experience of ‘sharing chocolate’ contains the seeds of formal division leading to fractions. 1
Of course, the five-year-old is not interested in how much chocolate each friend gets, but whether everyone gets the same amount – it’s the child’s interest in relationships between quantities, rather than the actual quantities that holds the seeds of thinking mathematically.
The role of relationships in thinking mathematically
Quantitative relationships.
Quantitative relationships refer to how quantities relate to each other. Consider this example:
I have some friends round on Saturday for a tea party and buy a packet of biscuits, which we share equally. On Sunday, I have another tea party, we share a second, equivalent packet of the biscuits. We share out the same number of biscuits as yesterday, but there are more people at the table. Does each person get more or less biscuits? 2
Once people are reassured that this is not a trick question 3 then it is clear that if there are more people and the same quantity of biscuits, everyone must get a smaller amount to eat on Sunday than the Saturday crowd did. Note, importantly, we can reason this conclusion without knowing exact quantities, either of people or biscuits.
This example had the change from Saturday to Sunday being that the number of biscuits stayed the same, while the number of people went up. As each of these quantities can do three things between Saturday and Sunday – go down, stay the same, go up – there are nine variations to the problem, summarised in this table, with the solution shown to the particular version above.

Before reading on, you might like to take a moment to think about which of the other cells in the table can be filled in. (The solution is at the end of this blog).
It turns out that in 7 out of 9 cases, we can reason what will happen without doing any arithmetic. 4 We can then use this reasoning to help us understand what happens when we do put numbers in. For example, what we essentially have here is a division – quantity of biscuits divided between number of friends – and we can record the changes in the quantities of biscuits and/or people as fractions:

So, the two fractions represent 5 biscuits shared between 6 friends (5/6) and 5 biscuits shared between 8 (5/8). To reason through which of these fractions is bigger we can apply our quantitative reasoning here to see that everyone must get fewer biscuits on Sunday – there are more friends, but the same quantity of biscuits to go around. We do not need to generate images of each fraction to ‘see’ which is larger, and we certainly do not need to put them both over a common denominator of 48. We can reason about these fractions, not as being static parts of an object, but as a result of a familiar action on the world and in doing so developing our understanding of fractions. This is exactly what MathsBeat does, using this idea of reasoning in context to help children understand what the abstract mathematics might look like.
Structural relationships :
By structural relationships, I mean how we can break up and deal with a quantity in structural ways. Try this:
Jot down a two-digit number (say, 32) Add the two digits (3 + 2 = 5) Subtract that sum from your original number (32 – 5 = 27) Do at least three more Do you notice anything about your answers?
If you’ve done this, then you’ll probably notice that all of your answers are multiples of nine (and, if like most folks, you just read on, then do check this is the case with a couple of numbers now).
This result might look like a bit of mathematical magic, but there must be a reason.
We might model this using three base tens, and two units, decomposing one of our tens into units in order to take away five units. But this probably gives us no sense of the underlying structure or any physical sensation of why we always end up with a multiple of nine.

If we approach this differently, thinking about where our five came from –three tens and two units – rather than decomposing one of the tens into units, we could start by taking away two, which cancels out.
And then rather than subtracting three from one of our tens, we could take away one from each ten, leaving us with three nines. And a moment’s reflection may reveal that this will work for any starting number: 45 – (4 + 5), well the, five within the nine being subtracted clears the five ones in 45, and the 4 matches the number of tens, and that will always be the case. Through the concrete, we begin to get the sense that this will always be true.

If we take this into more formal recording, we are ensuring that children have a real sense of what the structure is: a structural sense , which complements their number sense.
Decomposing and recomposing is one way of doing subtraction, but we’re going beyond this by really unpacking and laying bare the underlying structure: a really powerful way of helping children understand what’s going on.
So in summary, much mathematical reasoning is independent of arithmetical fluency.
This is a bold statement, but as you can see from the examples above, our reasoning doesn’t necessarily depend upon or change with different numbers. In fact, it stays exactly the same. We can even say something is true and have absolutely no idea how to do the calculation. (Is it true that 37.5 x 13.57 = 40 x 13.57 – 2.5 x 13.37?)
Maybe it’s time to reverse the logic and start to think about mathematics emerging from reasoning to problem-solving to fluency.

Mike Askew: Before moving into teacher education, Professor Mike Askew began his career as a primary school teacher. He now researches, speaks and writes on teaching and learning mathematics. Mike believes that all children can find mathematical activity engaging and enjoyable, and therefore develop the confidence in their ability to do maths.
Mike is also the Series Editor of MathsBeat , a new digitally-led maths mastery programme that has been designed and written to bring a consistent and coherent approach to the National Curriculum, covering all of the aims – fluency, problem solving and reasoning – thoroughly and comprehensively. MathsBeat’s clear progression and easy-to-follow sequence of tasks develops children’s knowledge, fluency and understanding with suggested prompts, actions and questions to give all children opportunities for deep learning. Find out more here .
You can watch Mike’s full webinar, The role of reasoning in supporting problem solving and fluency , here . (Note: you will be taken to a sign-up page and asked to enter your details; this is so that we can email you a CPD certificate on competition of the webinar).
Solution to Changes from Saturday to Sunday and the result

1 If you would like to read more about this, I recommend Lakoff, G., & Núñez, R. E. (2000). Where mathematics comes from: How the embodied mind brings mathematics into being. Basic Books.
2 Adapted from a problem in: Lamon, S. (2005). Teaching Fractions and Ratios for Understanding. Essential Content Knowledge and Instructional Strategies for Teachers, 2nd Edition. Routledge.
3 Because, of course in this mathematical world of friends, no one is on a diet or gluten intolerant!
4 The more/more and less/less solutions are determined by the actual quantities: biscuits going up by, say, 20 , but only one more friend turning up on Sunday is going to be very different by only having 1 more biscuit on Sunday but 20 more friends arriving.
Share this:
One thought on “ the role of reasoning in supporting problem solving and fluency ”.
Hi Mike, I enjoyed reading your post, it has definitely given me a lot of insight into teaching and learning about mathematics, as I have struggled to understand generalisations and concepts when dealing solely with numbers, as a mathematics learner. I agree with you in that students’ ability to reason and develop an understanding of mathematical concepts, and retain a focus on mathematical ideas and why these ideas are important, especially when real-world connections are made, because this is relevant to students’ daily lives and it is something they are able to better understand rather than being presented with solely arithmetic problems and not being exposed to understanding the mathematics behind it. Henceforth, the ideas you have presented are ones I will take on when teaching: ensuring that students understand the importance of understanding mathematical ideas and use this to justify their responses, which I believe will help students develop confidence and strengthen their skills and ability to extend their thinking when learning about mathematics.
Comments are closed.
- International
- Topical and themed
- Early years
- Special needs
- Schools directory
- Resources Jobs Schools directory News Search
Fluency, reasoning and problem solving in primary maths
Primary maths, australia and new zealand, tes resources team.
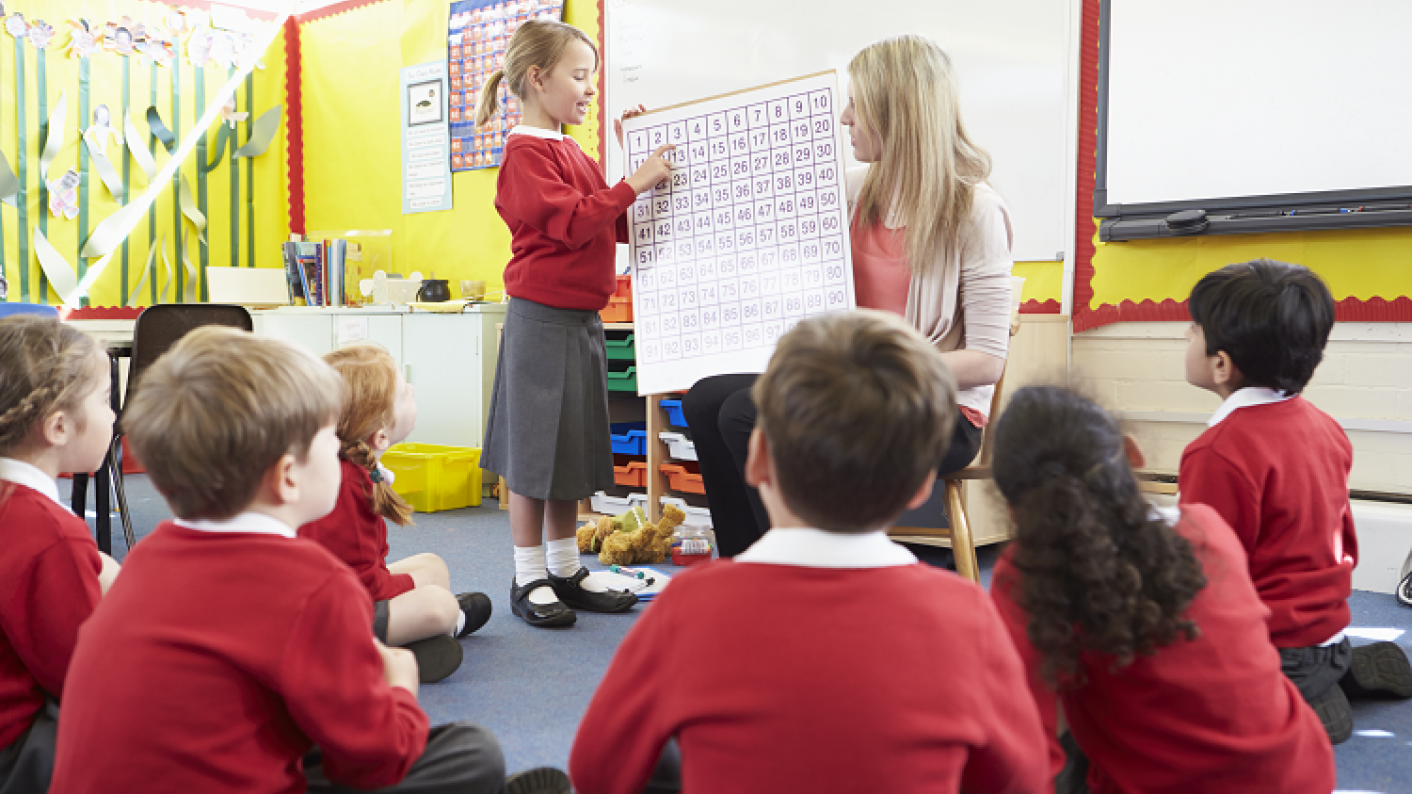
Develop fluency, reasoning and problem solving within any topic as part of a mastery approach
The skills of fluency, reasoning and problem solving are well-known to all primary maths teachers. In mastery teaching , they play an essential role in helping pupils to gain a deeper understanding of a topic. But what does this look like in practice?
For more information on mastery, check out this handy introduction .
Firstly, problem solving is at the heart of mastering maths. While there is nothing new about using problem-solving questions to consolidate understanding, mastery gets teachers to rethink the traditional lengthy word-problem format. Instead, problem-solving questions are often open-ended, with more than one right answer.
Problem solving is an important skill for all ages and abilities and, as such, needs to be taught explicitly. It is therefore useful to have challenges like these at the end of every lesson.
Secondly, verbal reasoning demonstrates that pupils understand the maths. Talk is an integral part of mastery as it encourages students to reason, justify and explain their thinking. This is tricky for many teachers who are not used to focusing on verbal reasoning in their maths lessons. You might, for example, get young learners to voice their thought processes. Older students could take part in class debates, giving them the space to challenge their peers using logical reasoning.
Introducing scaffolded sentence structures when talking about maths gives pupils the confidence to communicate their ideas clearly, before writing them down. A mastery classroom should never be a quiet classroom.
Finally, fluency, reasoning and problem solving underpins the deepening of understanding. Fluency alone doesn’t give students the chance to delve deeper into the mathematics. They may well be able to answer the questions, but can they also justify their answer or explore other possibilities?
Typically, teachers start new topics by developing fluency in order to give learners confidence with the skill. However, sometimes starting with a problem-solving question – eg, Prove that 4+3=7 – deepens understanding sooner. How? Pupils have to be reliant on resources they’ve used elsewhere, such as concrete manipulatives and pictorial representations, to help them explain the maths.
When planning, try not to get hung up on whether an activity focuses on either reasoning or problem solving as often it is a combination. Instead, turn your attention to using these types of questions to secure fluency and ensure that all children move beyond it into a world of deeper understanding.
Fluency, reasoning and problem solving in your classroom
Embedding these concepts into your everyday teaching can take time so patience is key! Mastery specialists recommend being more fluid with your planning and investing more time in making resources that will allow you to be reactionary to progress made in the lessons.
We’ve hand-picked these useful ideas to get you started:
This blog post was written with grateful thanks to Jenny Lewis, Primary Maths Specialist at the White Rose Maths Hub, and Helen Williams, Director of Primary at Mathematics Mastery, for their insights.
Want to know more about primary maths mastery? Check out our collection of free resources, quality assured by mastery experts and helpfully mapped by topic to year groups and learning objectives.
Building fluency through problem solving
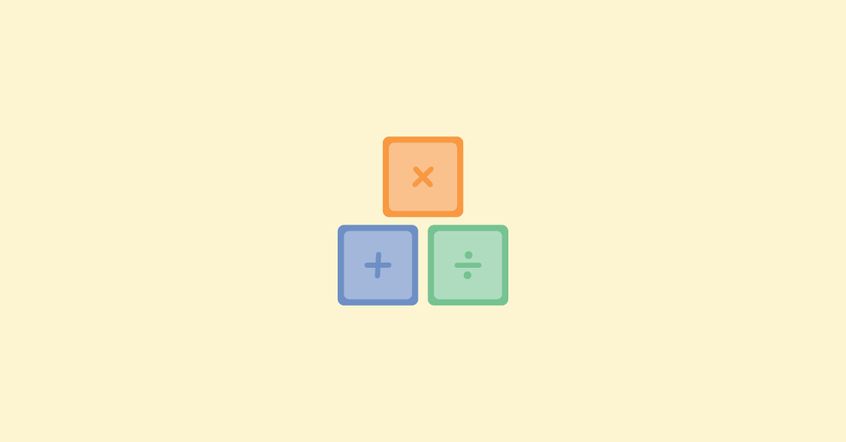
Editor’s Note:
This is an updated version of a blog post published on January 13, 2020.
Problem solving builds fluency and fluency builds problem solving. How can you help learners make the most of this virtuous cycle and achieve mastery?
Fluency. It’s so important that I have written not one , not two , but three blog posts on the subject. It’s also one of the three key aims for the national curriculum.
It’s a common dilemma. Learners need opportunities to apply their knowledge in solving problems and reasoning (the other two NC aims), but can’t reason or solve problems until they’ve achieved a certain level of fluency.
Instead of seeing this as a catch-22, think of fluency and problem solving as a virtuous cycle — working together to help learners achieve true mastery.
Supporting fluency when solving problems
Fluency helps children spot patterns, make conjectures, test them out, create generalisations, and make connections between different areas of their learning — the true skills of working mathematically. When learners can work mathematically, they’re better equipped to solve problems.
But what if learners are not totally fluent? Can they still solve problems? With the right support, problem solving helps learners develop their fluency, which makes them better at problem solving, which develops fluency…
Here are ways you can support your learners’ fluency journey.
Don’t worry about rapid recall
What does it mean to be fluent? Fluency means that learners are able to recall and use facts in a way that is accurate, efficient, reliable, flexible and fluid. But that doesn’t mean that good mathematicians need to have super-speedy recall of facts either.
Putting pressure on learners to recall facts in timed tests can negatively affect their ability to solve problems. Research shows that for about one-third of students, the onset of timed testing is the beginning of maths anxiety . Not only is maths anxiety upsetting for learners, it robs them of working memory and makes maths even harder.
Just because it takes a learner a little longer to recall or work out a fact, doesn’t mean the way they’re working isn’t becoming accurate, efficient, reliable, flexible and fluid. Fluent doesn’t always mean fast, and every time a learner gets to the answer (even if it takes a while), they embed the learning a little more.
Give learners time to think and reason
Psychologist Daniel Willingham describes memory as “the residue of thought”. If you want your learners to become fluent, you need to give them opportunities to think and reason. You can do this by looking for ways to extend problems so that learners have more to think about.
Here’s an example: what is 6 × 7 ? You could ask your learners for the answer and move on, but why stop there? If learners know that 6 × 7 = 42 , how many other related facts can they work out from this? Or if they don’t know 6 × 7 , ask them to work it out using facts they do know, like (5 × 7) + (1 × 7) , or (6 × 6) + (1 × 6) ?
Spending time exploring problems helps learners to build fluency in number sense, recognise patterns and see connections, and visualise — the three key components of problem solving.
Developing problem solving when building fluency
Learners with strong problem-solving skills can move flexibly between different representations, recognising and showing the links between them. They identify the merits of different strategies, and choose from a range of different approaches to find the one most appropriate for the maths problem at hand.
So, what type of problems should you give learners when they are still building their fluency? The best problem-solving questions exist in a Goldilocks Zone; the problems are hard enough to make learners think, but not so hard that they fail to learn anything.
Here’s how to give them opportunities to develop problem solving.
Centre problems around familiar topics
Learners can develop their problem-solving skills if they’re actively taught them and are given opportunities to put them into practice. When our aim is to develop problem-solving skills, it’s important that the mathematical content isn’t too challenging.
Asking learners to activate their problem-solving skills while applying new learning makes the level of difficulty too high. Keep problems centred around familiar topics (this can even be content taught as long ago as two years previously).
Not only does choosing familiar topics help learners practice their problem-solving skills, revisiting topics will also improve their fluency.
Keep the focus on problem solving, not calculation
What do you want learners to notice when solving a problem? If the focus is developing problem-solving skills, then the takeaway should be the method used to answer the question.
If the numbers involved in a problem are ‘nasty’, learners might spend their limited working memory on calculating and lose sight of the problem. Chances are they’ll have issues recalling the way they solved the problem. On top of that, they’ll learn nothing about problem-solving strategies.
It’s important to make sure that learners have a fluent recall of the facts needed to solve the problem. This way, they can focus on actually solving it rather than struggling to recall facts. To understand the underlying problem-solving strategies, learners need to have the processing capacity to spot patterns and make connections.
The ultimate goal of teaching mathematics is to create thinkers. Making the most of the fluency virtuous cycle helps learners to do so much more than just recall facts and memorise procedures. In time, your learners will be able to work fluently, make connections, solve problems, and become true mathematical thinkers.
Jo Boaler (2014). Research Suggests that Timed Tests Cause Math Anxiety. Teaching Children Mathematics , 20(8), p.469.
Willingham, D. (2009). Why don’t students like school?: A Cognitive Scientist Answers Questions About How the Mind Works and What It Means for Your Classroom. San Francisco: Jossey-Bass.
Gill Knight
Browse by Topic
Your teaching practice.
Boost your teaching confidence with the latest musings on pedagogy, classroom management, and teacher mental health.
Maths Mastery Stories
You’re part of a growing community. Get smart implementation advice and hear inspiring maths mastery stories from teachers just like you.
Teaching Tips
Learn practical maths teaching tips and strategies you can use in your classroom right away — from teachers who’ve been there.
Classroom Assessment
Identify where your learners are at and where to take them next with expert assessment advice from seasoned educators.
Your Learners
Help every learner succeed with strategies for managing behaviour, supporting mental health, and differentiating instruction for all attainment levels.
Teaching Maths for Mastery
Interested in Singapore maths, the CPA approach, bar modelling, or number bonds? Learn essential maths mastery theory and techniques here.
Deepen your mastery knowledge with our biweekly newsletter

By clicking “Accept All” , you agree to the storing of cookies on your device to enhance site navigation, analyze site usage and assist in our marketing efforts.
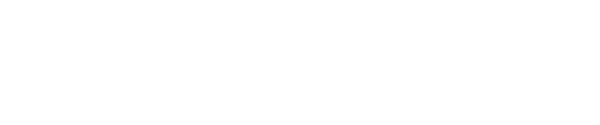
Or search by topic
Number and algebra
- The Number System and Place Value
- Calculations and Numerical Methods
- Fractions, Decimals, Percentages, Ratio and Proportion
- Properties of Numbers
- Patterns, Sequences and Structure
- Algebraic expressions, equations and formulae
- Coordinates, Functions and Graphs
Geometry and measure
- Angles, Polygons, and Geometrical Proof
- 3D Geometry, Shape and Space
- Measuring and calculating with units
- Transformations and constructions
- Pythagoras and Trigonometry
- Vectors and Matrices
Probability and statistics
- Handling, Processing and Representing Data
- Probability
Working mathematically
- Thinking mathematically
- Mathematical mindsets
- Cross-curricular contexts
- Physical and digital manipulatives
For younger learners
- Early Years Foundation Stage
Advanced mathematics
- Decision Mathematics and Combinatorics
- Advanced Probability and Statistics
Going Deeper: Achieving Greater Depth in the Primary Classroom
As a mastery approach becomes increasingly common in many schools, we have been working with teachers on a project to uncover effective ways to support their learners working 'at greater depth'. In this article, we will share our key findings and some of our favourite whole-class teaching resources which offer opportunities for teaching 'at greater depth'. (Several of these are linked from the NCETM's resources which exemplify their 'ready to progress criteria'.)
Teaching at 'greater depth'
One of the challenges facing many teachers is engaging all learners throughout the lesson when adopting a whole-class teaching approach. As guidance issued to schools indicates, "It is inevitable that some pupils will grasp concepts more rapidly than others and will need to be stimulated and challenged to ensure continued progression” (Askew et al., 2015, p.6).
Aware of these concerns, the guidance adopted the phrase ‘mastery at greater depth’ and suggested that pupils working at this level should be able to:
• solve problems of greater complexity (i.e. where the approach is not immediately obvious), demonstrating creativity and imagination;
• independently explore and investigate mathematical contexts and structures, communicate results clearly and systematically explain and generalise the mathematics.
(Askew et al. 2015, p.7)
The requirement for learners working 'at greater depth' to investigate more complex problems, work independently and communicate their ideas to others are the very foundations of our Low Threshold High Ceiling (LTHC) approach at NRICH: a low threshold ensures that every learner can get started on a problem and the high ceiling ensures that there is a suitable level of challenege, often unseen, for the learner to consider if and when they are ready to do so.
We teamed up with a group of schools based in Tower Hamlets, who were participating in a joint professional development programme with NRICH and the Tower Hamlets Education Partnership, to invesigate ways to maximise the potential of our curriculum-mapped LTHC resources for teaching 'at greater depth'. In this article we share some of their top tips.
- Be prepared to be surprised
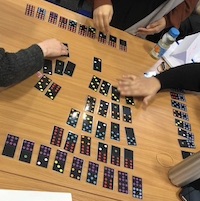
During a visit, we joined a very engaged class who quickly got started on the challenge. Working in pairs, with their teacher taking reguar opportunities to pause the groups and allow time to share their ideas with the wider class, the learners began to organise their dominoes so that they could more easily check which, if any, were missing. Some learners who had completed the initial task were further challenged to consider how many dominoes they might have if the maximum number of dots on each side was increased to seven:
Teacher: We’re doing the seven row. Prove to me that there’s eight in the seven row.
Learner: It will only be like this, seven zero add one, seven one, seven two, seven three, seven four, seven five, seven six, seven seven but you can’t go over that.
Teacher: So when you get to the seven seven you can’t go any further. So you proved it. There’s no doubles and you can’t go any further than seven seven, can you?
The learning did not stop there, the lesson went much deeper as the learners were encouraged by their teacher to set themselves the challenge of thinking about sets of dominoes which go up to 'double 10', or 'double 20' or even 'double 100'. Working with these much larger domino sets encouraged those working 'at greater depth' to generalise their ideas, and their enthusiasm led them to continue working through their break time!
Reflecting on the lesson, the teacher noted that one member of that day's 'at greater depth' group had previously struggled to engage with their mathematics:
What’s great about NRICH activities is that it just shows children can shine even if we’ve got them in a box under 'expected'. That’s what I love about it... You know, he completely steps out of the box and he’s shining.
Clearly, one of the many benefits of working with LTHC resources is their potential for challenging all learners and enabling them to reveal their potential. Be prepared to be surprised.
Encourage a growth mindset
No-one enjoys trying to do too much at once, any activity can become frustrating if it is too far beyond our comfort zone. This is true in our classrooms too. In our mathematics lessons, our curriculum challenges teachers to develop fluency, reasoning and problem-solving skills, and trying to find a balance within a particular lesson can be a challenge (this is an aspect we explore more fully in this article by Clare Lee and Sue Johnston-Wilder). By choosing LTHC activities, learners can easily get started on a task, building their confidence and willingness to engage with further challenges later on.
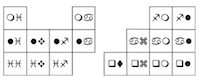
One of the strategies we used to solve the coded 100 chart was to look for patterns. We did this by looking in each column and finding that the symbols end with the same shape. For example, the first column of numbers always end in a rhombus shape. The same thing with the rows. Each row, we noticed while putting the symbols together, begins with the same shape. To solve this problem, we think it's best to work with the shape you first put in the 100 chart, and keep building off of that one. The first piece you out in the 100 chart works best if it fits into a corner of the chart.
In contrast, Nathan adoted a very different approach:
Start by placing any one piece and think which one will fit. Before putting it there, think if any other shape could fit. Then, if not try another shape that could fit. After the one you choose is put on, repeat until the coded hundred chart is filled. If you mess up, click on pieces to show the full piece and see if you can change a piece for another piece. Remember the first strategy to check if another one can fit after that one and then repeat again and again until you're done. After I fit the pieces together, I looked down each column. I checked that all the symbols in the ones placed matched. I looked across each row and checked that the symbols in the ones places were in order (1,2,3,4...).
- Celebrate mathematical thinking
For learners to be working 'at greater depth', they need to be communicating their ideas clearly to others. In our project schools, the teachers often encouraged their learners to reflect on their problem-solving by recording their ideas in a class book. These books were proudly shared with visitors on arrival to their classes, including the NRICH team. Although we cannot visit every classroom, we do enjoy reading solutions to our problems. For example, Jordan, Juoiana and Nathan all submitted their ideas about the Coded Hundred Square to the team and their ideas were published on NRICH. Your classes are very welcome to share their ideas about our problem-solving tasks too, simply visit our Live Problems page for our very latest opportunities to communicate mathematically with the team. We publish a selection of the submissions we receive on our website.
There are 10 leaves per twig There are 10 twigs per branch 10 leaves x 10 twigs = 100 leaves per branch There are 10 branches per trunk 100 leaves x 10 branches = 1000 leaves per trunk There are 10 trunks per tree 1000 leaves x 10 trunks = 10 000 leaves on the tree
Cut off one trunk: 10 000 - 1000 = 9000 leaves left Cut off one branch: 9000 - 100 = 8900 leaves left Cut off one twig: 8900 - 10 = 8890 leaves left Pull off one leaf: 8890 - 1 = 8889 leaves left
There are 8889 leaves left on the tree.
Another learner called Rachel seemed to approach the problem in the same way as Kirsty, but she found the total number of leaves which had been pulled off the tree before finding the total number of leaves on the tree initially.
Submitting solutions often leads to published solutions, and the teachers in our project schools also reported on the usefulness of the solutions accompanying our resources. Some teachers set aside time the following day after introducing an NRICH problem to their classes to enable their learners to compare their solutions with those from other classes:
I really like the solution thing now that I know how to use it and things. Getting the language out of it and stuff and using it after they’ve done it. Maybe tomorrow... we might start by looking at the solution that was there... Everyone will understand it tomorrow and then we can really, you know, look at it and decide whether we like it and pull it apart.
Allow time for you to think mathematically too
Another key finding from our project teachers was the importance of setting aside time to explore each LTHC activity for themselves before the lesson. This approach enabled the teachers to consider the possibilities for extending the learners where needed, to enable teaching 'at greater depth', but it was often seen as a thoroughly enjoyable experience too:
I mean, I consider myself greater depth, okay? ... I do have, you know, quite a high level of maths. So, basically, if I have a greater depth and I’m enjoying it and taking it on and forward progressing, clearly those children can do the same, can’t they?
Our school-based research revealed these four 'tips' for schools to support their learners working 'at greater depth' using NRICH tasks:
- Encourage a growth mindset
- Allow time for you to think mathematically too.
We hope that reading this article will inspire readers to explore the potential of using NRICH tasks to support their own learners working 'at greater depth'.
This project would not have beeen possible without the generous support of the team at the Tower Hamlets Education Partnership and the teachers who welcomed the NRICH team into their classrooms. We were delighted to share our findings at the British Society for Research into Learning Mathematics Day (BSRLM) Conference in November 2020 - you can access a copy of the accompanying research paper which was published in the BSRLM's Proceedings here .
Askew, A., Bishop, S., Christie, C., Eaton, S., Griffin, P. and Morgan, D. (2015). Teaching for Mastery: Questions, tasks and activities to support assessment . Oxford University Press.
Lee, C. and Johnson-Wilder, S. (2018). Getting into and staing in the Growth Zone. Retrieved from https://nrich.maths.org/13491
You are using an outdated browser. Upgrade your browser today or install Google Chrome Frame to better experience this site.
- Professional learning
Teach. Learn. Grow.
Teach. learn. grow. the education blog.
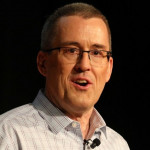
Why it’s important to support fluency in mathematics
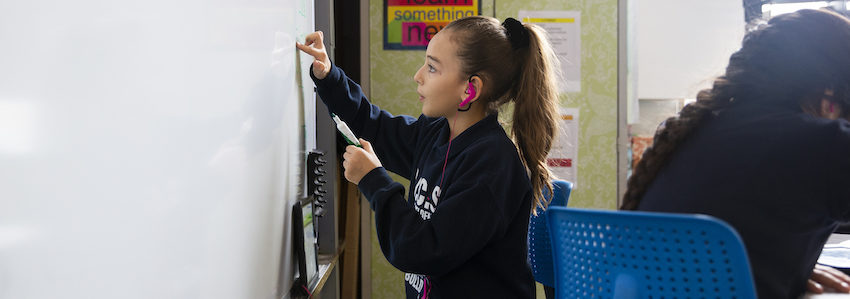
The idea of fluency is big in K–12 mathematics education. However, there is not a clear, shared meaning of the word. In fact, if you were to ask educators to define “fluency” in the context of learning mathematics, you would probably get a dozen different answers.
I encourage you to stop reading this post and think about fluency. Go ahead and pause for a moment. This post can wait. What do you believe fluency in math education is?
What fluency is when we’re talking about math?
Sometimes educators think about fluency as getting the right answer quickly. Sometimes, it’s about accurately performing a standard algorithm efficiently or remembering facts. But there is much more to it.
Fluency demands thinking about mathematical procedures with efficiency, accuracy, flexibility, and appropriateness. Being fluent means that students can choose among methods and strategies to solve contextual and mathematical problems, that they understand and are able to explain their approaches, and that they are able to produce accurate answers efficiently.
It helps to also define what I mean by “efficiency,” “accuracy,” “flexibility,” and “appropriateness”:
- “Efficiency” is the ways in which students carry out strategic procedural plans easily, keep track of sub-problems, and make use of intermediate results to solve a problem
- “Accuracy” means students reliably produce the correct answer
- “Flexibility” refers to students knowing more than one approach, being able to choose a viable strategy, and using one method to solve and another method to double-check their work
- “Appropriateness” means students know when to apply a particular procedure
An example: The role of fluency in understanding fractions
Simply memorizing facts and procedures does not a fluent student make. According to research done by the Rational Number Project , students who only memorize will experience challenges in making sense of math. This is especially true when students begin to learn about fractions and operations with fractions. And, even more specifically, difficulty with operations with fractions often stems from a lack of a robust understanding of the idea of a fraction.
For example, children have difficulty internalizing that the symbol for a fraction represents a single entity. When asked if 2/3 is one or two numbers, many children will say that the symbol represents two numbers. When students consider 2/3 as two numbers, then it makes sense that they would try to treat them like whole numbers. For example, when students add two fractions by adding the numerators and then denominators, they are interpreting the symbols as four numbers, not two. Many errors with fractions can be traced to students’ lack of mental images for the quantity a symbol represents.
Students who have not fully developed fluency with fractions often have difficulty ordering fractions, since ordering fractions is more complex than ordering whole numbers. Comparing 1/4 and 1/6, for example, conflicts with children’s whole number ideas. Six is greater than four, but 1/4 is greater than 1/6. With fractions, more can mean less. In contrast, 3/5 is greater than 2/5 because three of the same-size parts are greater than two of the same-size parts. In this case, more implies more.
Being able to order plays an important part in estimating fraction addition and subtraction. Ideally when a student adds 1/4 and 1/3, they should be able to reason, from their mental images of the symbols, that a) the answer is greater than 1/2, but less than one, and b) 2/7 is an unreasonable answer because it is less than 1/2.
Understanding fraction equivalence is not as simple as it may seem. Some children have difficulty noting equivalence from pictures.
Imagine a circle partitioned into fourths with one of those fourths partitioned into three equal parts. Some children may be unable to agree that 3/12 equals 1/4, even though they can see that the two sections of the circle are the same size. Children can struggle to remove the extra lines drawn in the fourth that’s partitioned into three equal parts. But that is just what must be done to understand fraction equivalence from a picture.
All of these challenges related to a foundational understanding of the idea of a fraction can lead to the difficulties children have with fraction addition and subtraction. These difficulties come from asking students to operate on fractions before they have a strong conceptual understanding of these new numbers.
Fluency involves getting answers as well as thinking about reasons, providing explanations, and communicating thinking clearly.
How asking—and answering—“why” can improve mathematical fluency
To help students gain fluency in math, it’s important to help them explore and understand why.
Consider the following problem: 2/3 x 3/4. Most readers will likely be able to compute the correct answer mentally (the answer is 1/2). It is important to be able to get this answer but also to explain how it makes sense. When working with students on a problem like this one, consider asking them questions like these:
- Why does 2/3 x 3/4 equal 1/2?
- Both 2/3 and 3/4 are bigger than 1/2. Why does multiplying produce a number smaller than the two numbers being multiplied?
- Why do we multiply the numerators and the denominators?
Again, both the answer and the explanation of how the answer makes sense are important.
Why does 2/3 x 3/4 equal 1/2? Because when we multiply fractions, we multiply the numerators and the denominators:
- 2/3 x 3/4 = (2 x 3)/(3 x 4)
- 2 x 3 = 6 and 3 x 4 = 12, so (2 x 3)/(3 x 4) = 6/12
Why does multiplying produce a number smaller than the two numbers multiplied? It helps to begin by thinking about the second part of our equation, 3/4, since that’s the larger number.
What does 3/4 look like? Picture a rectangle.
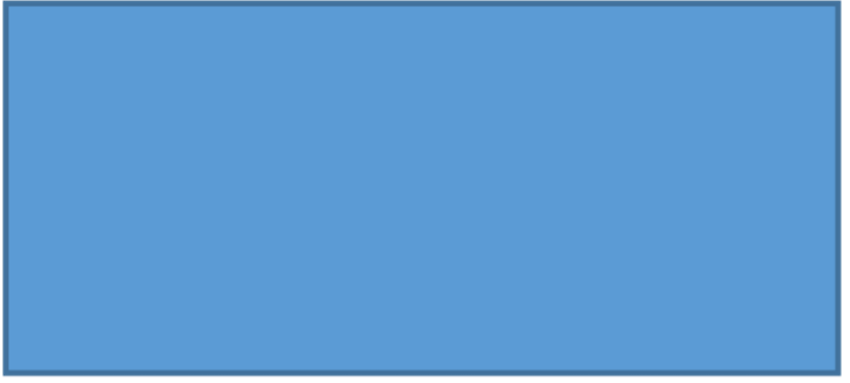
3 non-negotiables for differentiation in the math classroom
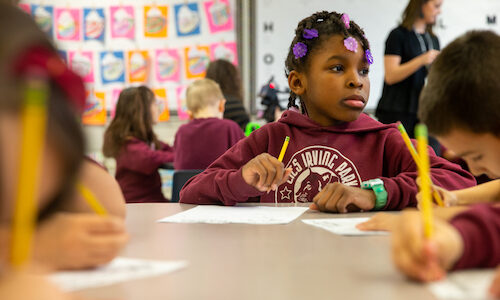
4 ways to engage students with writing in math class
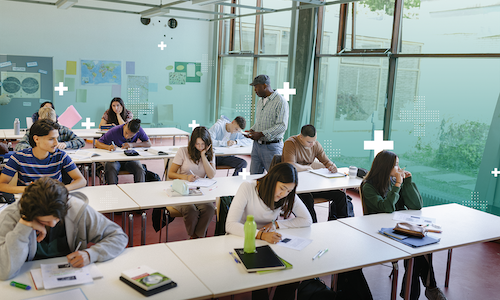
That’s not how I learned it! 4 ways to help your child with “new math”
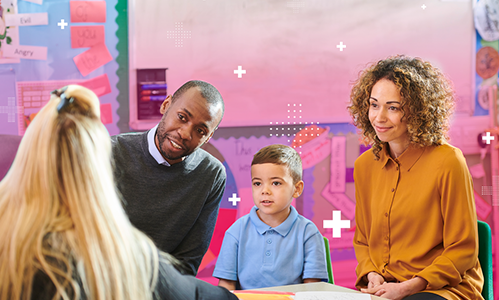
Helping students grow
Students continue to rebound from pandemic school closures. NWEA® and Learning Heroes experts talk about how best to support them here on our blog, Teach. Learn. Grow.
See the post
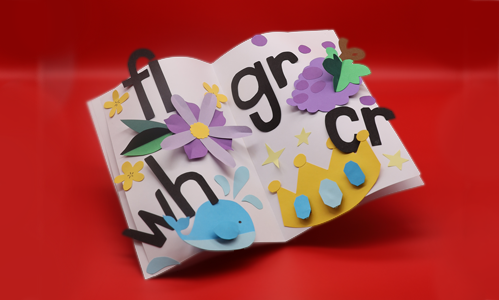
Put the science of reading into action
The science of reading is not a buzzword. It’s the converging evidence of what matters and what works in literacy instruction. We can help you make it part of your practice.
Get the guide
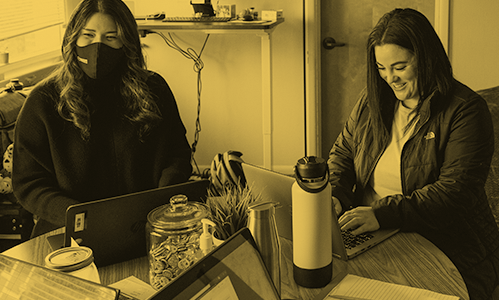
Support teachers with PL
High-quality professional learning can help teachers feel invested—and supported—in their work.
Read the article
STAY CURRENT by subscribing to our newsletter
You are now signed up to receive our newsletter containing the latest news, blogs, and resources from nwea..
- Mathematics proficiencies
Introduction
The Australian Curriculum: Mathematics aims to be relevant and applicable to the 21st century. The inclusion of the proficiencies of understanding, fluency, problem-solving and reasoning in the curriculum is to ensure that student learning and student independence are at the centre of the curriculum. The curriculum focuses on developing increasingly sophisticated and refined mathematical understanding, fluency, reasoning, and problem-solving skills. These proficiencies enable students to respond to familiar and unfamiliar situations by employing mathematical strategies to make informed decisions and solve problems efficiently.
The proficiency strands describe the actions in which students can engage when learning and using the content of the Australian Curriculum: Mathematics.
Understanding
Students build a robust knowledge of adaptable and transferable mathematical concepts. They make connections between related concepts and progressively apply the familiar to develop new ideas. They develop an understanding of the relationship between the ‘why’ and the ‘how’ of mathematics. Students build understanding when they connect related ideas, when they represent concepts in different ways, when they identify commonalities and differences between aspects of content, when they describe their thinking mathematically and when they interpret mathematical information
Students develop skills in choosing appropriate procedures; carrying out procedures flexibly, accurately, efficiently and appropriately; and recalling factual knowledge and concepts readily. Students are fluent when they calculate answers efficiently, when they recognise robust ways of answering questions, when they choose appropriate methods and approximations, when they recall definitions and regularly use facts, and when they can manipulate expressions and equations to find solutions.
Problem-Solving
Students develop the ability to make choices, interpret, formulate, model and investigate problem situations, and communicate solutions effectively. Students formulate and solve problems when they use mathematics to represent unfamiliar or meaningful situations, when they design investigations and plan their approaches, when they apply their existing strategies to seek solutions, and when they verify that their answers are reasonable.
Students develop an increasingly sophisticated capacity for logical thought and actions, such as analysing, proving, evaluating, explaining, inferring, justifying and generalising. Students are reasoning mathematically when they explain their thinking, when they deduce and justify strategies used and conclusions reached, when they adapt the known to the unknown, when they transfer learning from one context to another, when they prove that something is true or false, and when they compare and contrast related ideas and explain their choices.
Useful Links
- Australian Curriculum: Mathematics F–10
- Review by Kaye Stacey of 'Adding it up: helping children learn mathematics' report
- Peter Sullivan presentation: Designing learning experiences to exemplify the proficiencies
- Peter Sullivan presentation: Create your own lessons
- Peter Sullivan paper: Using the proficiencies to enrich mathematics teaching and assessment
Explore Mathematics proficiencies portfolios and illustrations
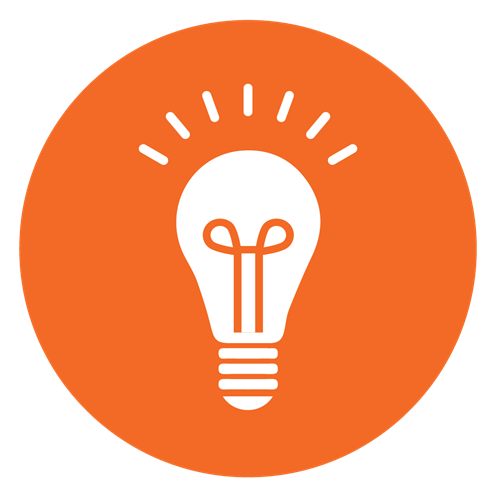

Adding It Up: Helping Children Learn Mathematics (2001)
Chapter: 4 the strands of mathematical proficiency, 4 the strands of mathematical proficiency.
During the twentieth century, the meaning of successful mathematics learning underwent several shifts in response to changes in both society and schooling. For roughly the first half of the century, success in learning the mathematics of pre-kindergarten to eighth grade usually meant facility in using the computational procedures of arithmetic, with many educators emphasizing the need for skilled performance and others emphasizing the need for students to learn procedures with understanding. 1 In the 1950s and 1960s, the new math movement defined successful mathematics learning primarily in terms of understanding the structure of mathematics together with its unifying ideas, and not just as computational skill. This emphasis was followed by a “back to basics” movement that proposed returning to the view that success in mathematics meant being able to compute accurately and quickly. The reform movement of the 1980s and 1990s pushed the emphasis toward what was called the development of “mathematical power,” which involved reasoning, solving problems, connecting mathematical ideas, and communicating mathematics to others. Reactions to reform proposals stressed such features of mathematics learning as the importance of memorization, of facility in computation, and of being able to prove mathematical assertions. These various emphases have reflected different goals for school mathematics held by different groups of people at different times.
Our analyses of the mathematics to be learned, our reading of the research in cognitive psychology and mathematics education, our experience as learners and teachers of mathematics, and our judgment as to the mathematical knowledge, understanding, and skill people need today have led us to adopt a
composite, comprehensive view of successful mathematics learning. This view, admittedly, represents no more than a single committee’s consensus. Yet our various backgrounds have led us to formulate, in a way that we hope others can and will accept, the goals toward which mathematics learning should be aimed. In this chapter, we describe the kinds of cognitive changes that we want to promote in children so that they can be successful in learning mathematics.
Recognizing that no term captures completely all aspects of expertise, competence, knowledge, and facility in mathematics, we have chosen mathematical proficiency to capture what we believe is necessary for anyone to learn mathematics successfully. Mathematical proficiency, as we see it, has five components, or strands:
conceptual understanding —comprehension of mathematical concepts, operations, and relations
procedural fluency —skill in carrying out procedures flexibly, accurately, efficiently, and appropriately
strategic competence —ability to formulate, represent, and solve mathematical problems
adaptive reasoning —capacity for logical thought, reflection, explanation, and justification
productive disposition —habitual inclination to see mathematics as sensible, useful, and worthwhile, coupled with a belief in diligence and one’s own efficacy.
These strands are not independent; they represent different aspects of a complex whole. Each is discussed in more detail below. The most important observation we make here, one stressed throughout this report, is that the five strands are interwoven and interdependent in the development of profi ciency in mathematics (see Box 4–1 ). Mathematical proficiency is not a one-dimensional trait, and it cannot be achieved by focusing on just one or two of these strands. In later chapters, we argue that helping children acquire mathematical proficiency calls for instructional programs that address all its strands. As they go from pre-kindergarten to eighth grade, all students should become increasingly proficient in mathematics. That proficiency should enable them to cope with the mathematical challenges of daily life and enable them to continue their study of mathematics in high school and beyond.
The five strands are interwoven and interdependent in the development of proficiency in mathematics.
The five strands provide a framework for discussing the knowledge, skills, abilities, and beliefs that constitute mathematical proficiency. This frame-
work has some similarities with the one used in recent mathematics assessments by the National Assessment of Educational Progress (NAEP), which features three mathematical abilities (conceptual understanding, procedural knowledge, and problem solving) and includes additional specifications for reasoning, connections, and communication. 2 The strands also echo components of mathematics learning that have been identified in materials for teachers. At the same time, research and theory in cognitive science provide general support for the ideas contributing to these five strands. Fundamental in that work has been the central role of mental representations. How learners represent and connect pieces of knowledge is a key factor in whether they will understand it deeply and can use it in problem solving. Cognitive
scientists have concluded that competence in an area of inquiry depends upon knowledge that is not merely stored but represented mentally and organized (connected and structured) in ways that facilitate appropriate retrieval and application. Thus, learning with understanding is more powerful than simply memorizing because the organization improves retention, promotes fluency, and facilitates learning related material. The central notion that strands of competence must be interwoven to be useful reflects the finding that having a deep understanding requires that learners connect pieces of knowledge, and that connection in turn is a key factor in whether they can use what they know productively in solving problems. Furthermore, cognitive science studies of problem solving have documented the importance of adaptive expertise and of what is called metacognition: knowledge about one’s own thinking and ability to monitor one’s own understanding and problem-solving activity. These ideas contribute to what we call strategic competence and adaptive reasoning. Finally, learning is also influenced by motivation, a component of productive disposition. 3
Although there is not a perfect fit between the strands of mathematical proficiency and the kinds of knowledge and processes identified by cognitive scientists, mathematics educators, and others investigating learning, we see the strands as reflecting a firm, sizable body of scholarly literature both in and outside mathematics education.
Conceptual Understanding
Conceptual understanding refers to an integrated and functional grasp of mathematical ideas. Students with conceptual understanding know more than isolated facts and methods. They understand why a mathematical idea is important and the kinds of contexts in which is it useful. They have organized their knowledge into a coherent whole, which enables them to learn new ideas by connecting those ideas to what they already know. 4 Conceptual understanding also supports retention. Because facts and methods learned with understanding are connected, they are easier to remember and use, and they can be reconstructed when forgotten. 5 If students understand a method, they are unlikely to remember it incorrectly. They monitor what they remember and try to figure out whether it makes sense. They may attempt to explain the method to themselves and correct it if necessary. Although teachers often look for evidence of conceptual understanding in students’ ability to verbalize connections among concepts and representations, conceptual understanding need not be explicit. Students often understand before they can verbalize that understanding. 6
Conceptual understanding refers to an integrated and functional grasp of mathematical ideas.
A significant indicator of conceptual understanding is being able to represent mathematical situations in different ways and knowing how different representations can be useful for different purposes. To find one’s way around the mathematical terrain, it is important to see how the various representations connect with each other, how they are similar, and how they are different. The degree of students’ conceptual understanding is related to the richness and extent of the connections they have made.

Connections are most useful when they link related concepts and methods in appropriate ways. Mnemonic techniques learned by rote may provide connections among ideas that make it easier to perform mathematical operations, but they also may not lead to understanding. 7 These are not the kinds of connections that best promote the acquisition of mathematical proficiency.
Knowledge that has been learned with understanding provides the basis for generating new knowledge and for solving new and unfamiliar problems. 8 When students have acquired conceptual understanding in an area of mathematics, they see the connections among concepts and procedures and can give arguments to explain why some facts are consequences of others. They gain confidence, which then provides a base from which they can move to another level of understanding.
With respect to the learning of number, when students thoroughly understand concepts and procedures such as place value and operations with single-digit numbers, they can extend these concepts and procedures to new areas. For example, students who understand place value and other multidigit number concepts are more likely than students without such understanding to invent their own procedures for multicolumn addition and to adopt correct procedures for multicolumn subtraction that others have presented to them. 9
Thus, learning how to add and subtract multidigit numbers does not have to involve entirely new and unrelated ideas. The same observation can be made for multiplication and division.
Conceptual understanding helps students avoid many critical errors in solving problems, particularly errors of magnitude. For example, if they are multiplying 9.83 and 7.65 and get 7519.95 for the answer, they can immediately decide that it cannot be right. They know that 10×8 is only 80, so multiplying two numbers less than 10 and 8 must give a product less than 80. They might then suspect that the decimal point is incorrectly placed and check that possibility.
Conceptual understanding frequently results in students having less to learn because they can see the deeper similarities between superficially unrelated situations. Their understanding has been encapsulated into compact clusters of interrelated facts and principles. The contents of a given cluster may be summarized by a short sentence or phrase like “properties of multiplication,” which is sufficient for use in many situations. If necessary, however, the cluster can be unpacked if the student needs to explain a principle, wants to reflect on a concept, or is learning new ideas. Often, the structure of students’ understanding is hierarchical, with simpler clusters of ideas packed into larger, more complex ones. A good example of a knowledge cluster for mathematically proficient older students is the number line. In one easily visualized picture, the student can grasp relations between all the number systems described in chapter 3 , along with geometric interpretations for the operations of arithmetic. It connects arithmetic to geometry and later in schooling serves as a link to more advanced mathematics.
As an example of how a knowledge cluster can make learning easier, consider the cluster students might develop for adding whole numbers. If students understand that addition is commutative (e.g., 3+5=5+3), their learning of basic addition combinations is reduced by almost half. By exploiting their knowledge of other relationships such as that between the doubles (e.g., 5+5 and 6+6) and other sums, they can reduce still further the number of addition combinations they need to learn. Because young children tend to learn the doubles fairly early, they can use them to produce closely related sums. 10 For example, they may see that 6+7 is just one more than 6+6. These relations make it easier for students to learn the new addition combinations because they are generating new knowledge rather than relying on rote memorization. Conceptual understanding, therefore, is a wise investment that pays off for students in many ways.
Procedural Fluency
Procedural fluency refers to knowledge of procedures, knowledge of when and how to use them appropriately, and skill in performing them flexibly, accurately, and efficiently. In the domain of number, procedural fluency is especially needed to support conceptual understanding of place value and the meanings of rational numbers. It also supports the analysis of similarities and differences between methods of calculating. These methods include, in addition to written procedures, mental methods for finding certain sums, differences, products, or quotients, as well as methods that use calculators, computers, or manipulative materials such as blocks, counters, or beads.
Procedural fluency refers to knowledge of procedures, knowledge of when and how to use them appropriately, and skill in performing them flexibly, accurately, and efficiently.
Students need to be efficient and accurate in performing basic computations with whole numbers (6+7, 17–9, 8×4, and so on) without always having to refer to tables or other aids. They also need to know reasonably efficient and accurate ways to add, subtract, multiply, and divide multidigit numbers, both mentally and with pencil and paper. A good conceptual understanding of place value in the base-10 system supports the development of fluency in multidigit computation. 11 Such understanding also supports simplified but accurate mental arithmetic and more flexible ways of dealing with numbers than many students ultimately achieve.
Connected with procedural fluency is knowledge of ways to estimate the result of a procedure. It is not as critical as it once was, for example, that students develop speed or efficiency in calculating with large numbers by hand, and there appears to be little value in drilling students to achieve such a goal. But many tasks involving mathematics in everyday life require facility with algorithms for performing computations either mentally or in writing.
In addition to providing tools for computing, some algorithms are important as concepts in their own right, which again illustrates the link between conceptual understanding and procedural fluency. Students need to see that procedures can be developed that will solve entire classes of problems, not just individual problems. By studying algorithms as “general procedures,” students can gain insight into the fact that mathematics is well structured (highly organized, filled with patterns, predictable) and that a carefully developed procedure can be a powerful tool for completing routine tasks.
It is important for computational procedures to be efficient, to be used accurately, and to result in correct answers. Both accuracy and efficiency can be improved with practice, which can also help students maintain fluency. Students also need to be able to apply procedures flexibly. Not all computational situations are alike. For example, applying a standard pencil-and-paper algorithm to find the result of every multiplication problem is neither neces-
sary nor efficient. Students should be able to use a variety of mental strategies to multiply by 10, 20, or 300 (or any power of 10 or multiple of 10). Also, students should be able to perform such operations as finding the sum of 199 and 67 or the product of 4 and 26 by using quick mental strategies rather than relying on paper and pencil. Further, situations vary in their need for exact answers. Sometimes an estimate is good enough, as in calculating a tip on a bill at a restaurant. Sometimes using a calculator or computer is more appropriate than using paper and pencil, as in completing a complicated tax form. Hence, students need facility with a variety of computational tools, and they need to know how to select the appropriate tool for a given situation.
Procedural fluency and conceptual understanding are often seen as competing for attention in school mathematics. But pitting skill against understanding creates a false dichotomy. 12 As we noted earlier, the two are interwoven. Understanding makes learning skills easier, less susceptible to common errors, and less prone to forgetting. By the same token, a certain level of skill is required to learn many mathematical concepts with understanding, and using procedures can help strengthen and develop that understanding. For example, it is difficult for students to understand multidigit calculations if they have not attained some reasonable level of skill in single-digit calculations. On the other hand, once students have learned procedures without understanding, it can be difficult to get them to engage in activities to help them understand the reasons underlying the procedure. 13 In an experimental study, fifth-grade students who first received instruction on procedures for calculating area and perimeter followed by instruction on understanding those procedures did not perform as well as students who received instruction focused only on understanding. 14
Without sufficient procedural fluency, students have trouble deepening their understanding of mathematical ideas or solving mathematics problems. The attention they devote to working out results they should recall or compute easily prevents them from seeing important relationships. Students need well-timed practice of the skills they are learning so that they are not handicapped in developing the other strands of proficiency.
When students practice procedures they do not understand, there is a danger they will practice incorrect procedures, thereby making it more difficult to learn correct ones. For example, on one standardized test, the grade 2 national norms for two-digit subtraction problems requiring borrowing, such as 62–48=?, are 38% correct. Many children subtract the smaller from the larger digit in each column to get 26 as the difference between 62 and 48 (see Box 4–2 ). If students learn to subtract with understanding, they rarely make
this error. 15 Further, when students learn a procedure without understanding, they need extensive practice so as not to forget the steps. If students do understand, they are less likely to forget critical steps and are more likely to be able to reconstruct them when they do. Shifting the emphasis to learning with understanding, therefore, can in the long run lead to higher levels of skill than can be attained by practice alone.
If students have been using incorrect procedures for several years, then instruction emphasizing understanding may be less effective. 16 When children learn a new, correct procedure, they do not always drop the old one. Rather, they use either the old procedure or the new one depending on the situation. Only with time and practice do they stop using incorrect or inefficient methods. 17 Hence initial learning with understanding can make learning more efficient.
When skills are learned without understanding, they are learned as isolated bits of knowledge. 18 Learning new topics then becomes harder since there is no network of previously learned concepts and skills to link a new topic to. This practice leads to a compartmentalization of procedures that can become quite extreme, so that students believe that even slightly different problems require different procedures. That belief can arise among children in the early grades when, for example, they learn one procedure for subtraction problems without regrouping and another for subtraction problems with regrouping. Another consequence when children learn without understanding is that they separate what happens in school from what happens outside. 19 They have one set of procedures for solving problems outside of school and another they learned and use in school—without seeing the relation between the two. This separation limits children’s ability to apply what they learn in school to solve real problems.
Also, students who learn procedures without understanding can typically do no more than apply the learned procedures, whereas students who learn
with understanding can modify or adapt procedures to make them easier to use. For example, students with limited understanding of addition would ordinarily need paper and pencil to add 598 and 647. Students with more understanding would recognize that 598 is only 2 less than 600, so they might add 600 and 647 and then subtract 2 from that sum. 20
Strategic Competence
Strategic competence refers to the ability to formulate mathematical problems, represent them, and solve them. This strand is similar to what has been called problem solving and problem formulation in the literature of mathematics education and cognitive science, and mathematical problem solving, in particular, has been studied extensively. 21
Strategic competence refers to the ability to formulate mathematical problems, represent them, and solve them.
Although in school, students are often presented with clearly specified problems to solve, outside of school they encounter situations in which part of the difficulty is to figure out exactly what the problem is. Then they need to formulate the problem so that they can use mathematics to solve it. Consequently, they are likely to need experience and practice in problem formulating as well as in problem solving. They should know a variety of solution strategies as well as which strategies might be useful for solving a specific problem. For example, sixth graders might be asked to pose a problem on the topic of the school cafeteria. 22 Some might ask whether the lunches are too expensive or what the most and least favorite lunches are. Others might ask how many trays are used or how many cartons of milk are sold. Still others might ask how the layout of the cafeteria might be improved.
With a formulated problem in hand, the student’s first step in solving it is to represent it mathematically in some fashion, whether numerically, symbolically, verbally, or graphically. Fifth graders solving problems about getting from home to school might describe verbally the route they take or draw a scale map of the neighborhood. Representing a problem situation requires, first, that the student build a mental image of its essential components. Becoming strategically competent involves an avoidance of “number grabbing” methods (in which the student selects numbers and prepares to perform arithmetic operations on them) 23 in favor of methods that generate problem models (in which the student constructs a mental model of the variables and relations described in the problem). To represent a problem accurately, students must first understand the situation, including its key features. They then need to generate a mathematical representation of the problem that captures the core mathematical elements and ignores the irrelevant features. This
step may be facilitated by making a drawing, writing an equation, or creating some other tangible representation. Consider the following two-step problem:
At ARCO, gas sells for $1.13 per gallon.
This is 5 cents less per gallon than gas at Chevron.
How much does 5 gallons of gas cost at Chevron?
In a common superficial method for representing this problem, students focus on the numbers in the problem and use so-called keywords to cue appropriate arithmetic operations. 24 For example, the quantities $1.83 and 5 cents are followed by the keyword less, suggesting that the student should subtract 5 cents from $1.13 to get $1.08. Then the keywords how much and 5 gallons suggest that 5 should be multiplied by the result, yielding $5.40.
In contrast, a more proficient approach is to construct a problem model— that is, a mental model of the situation described in the problem. A problem model is not a visual picture per se; rather, it is any form of mental representation that maintains the structural relations among the variables in the problem. One way to understand the first two sentences, for example, might be for a student to envision a number line and locate each cost per gallon on it to solve the problem.
In building a problem model, students need to be alert to the quantities in the problem. It is particularly important that students represent the quantities mentally, distinguishing what is known from what is to be found. Analyses of students’ eye fixations reveal that successful solvers of the two-step problem above are likely to focus on terms such as ARCO, Chevron, and this, the principal known and unknown quantities in the problem. Less successful problem solvers tend to focus on specific numbers and keywords such as $1.13, 5 cents, less, and 5 gallons rather than the relationships among the quantities. 25
Not only do students need to be able to build representations of individual situations, but they also need to see that some representations share common mathematical structures. Novice problem solvers are inclined to notice similarities in surface features of problems, such as the characters or scenarios described in the problem. More expert problem solvers focus more on the structural relationships within problems, relationships that provide the clues for how problems might be solved. 26 For example, one problem might ask students to determine how many different stacks of five blocks can be made using red and green blocks, and another might ask how many different ways hamburgers can be ordered with or without each of the following:
catsup, onions, pickles, lettuce, and tomato. Novices would see these problems as unrelated; experts would see both as involving five choices between two things: red and green, or with and without. 27
In becoming proficient problem solvers, students learn how to form mental representations of problems, detect mathematical relationships, and devise novel solution methods when needed. A fundamental characteristic needed throughout the problem-solving process is flexibility. Flexibility develops through the broadening of knowledge required for solving nonroutine problems rather than just routine problems.
Routine problems are problems that the learner knows how to solve based on past experience. 28 When confronted with a routine problem, the learner knows a correct solution method and is able to apply it. Routine problems require reproductive thinking; the learner needs only to reproduce and apply a known solution procedure. For example, finding the product of 567 and 46 is a routine problem for most adults because they know what to do and how to do it.
In contrast, nonroutine problems are problems for which the learner does not immediately know a usable solution method. Nonroutine problems require productive thinking because the learner needs to invent a way to understand and solve the problem. For example, for most adults a nonroutine problem of the sort often found in newspaper or magazine puzzle columns is the following:
A cycle shop has a total of 36 bicycles and tricycles in stock.
Collectively there are 80 wheels.
How many bikes and how many tricycles are there?
One solution approach is to reason that all 36 have at least two wheels for a total of 36×2=72 wheels. Since there are 80 wheels in all, the eight additional wheels (80–72) must belong to 8 tricycles. So there are 36–8=28 bikes.
A less sophisticated approach would be to “guess and check”: If there were 20 bikes and 16 tricycles, that would give (20×2)+(16×3)=88 wheels, which is too many. Reducing the number of tricycles, a guess of 24 bikes and 12 tricycles gives (24×2)+(12×3)=84 wheels—still too many. Another reduction of the number of tricycles by 4 gives 28 bikes, 8 tricycles, and the 80 wheels needed.
A more sophisticated, algebraic approach would be to let b be the number of bikes and t the number of tricycles. Then b + t =36 and 2 b +3 t =80. The solution to this system of equations also yields 28 bikes and 8 tricycles.
A student with strategic competence could not only come up with several approaches to a nonroutine problem such as this one but could also choose flexibly among reasoning, guess-and-check, algebraic, or other methods to suit the demands presented by the problem and the situation in which it was posed.
Flexibility of approach is the major cognitive requirement for solving nonroutine problems. It can be seen when a method is created or adjusted to fit the requirements of a novel situation, such as being able to use general principles about proportions to determine the best buy. For example, when the choice is between a 4-ounce can of peanuts for 45 cents and a 10-ounce can for 90 cents, most people use a ratio strategy: the larger can costs twice as much as the smaller can but contains more than twice as many ounces, so it is a better buy. When the choice is between a 14-ounce jar of sauce for 79 cents and an 18-ounce jar for 81 cents, most people use a difference strategy: the larger jar costs just 2 cents more but gets you 4 more ounces, so it is the better buy. When the choice is between a 3-ounce bag of sunflower seeds for 30 cents and a 4-ounce bag for 44 cents, the most common strategy is unit-cost: The smaller bag costs 10 cents per ounce, whereas the larger costs 11 cents per ounce, so the smaller one is the better buy.
There are mutually supportive relations between strategic competence and both conceptual understanding and procedural fluency, as the various approaches to the cycle shop problem illustrate. The development of strategies for solving nonroutine problems depends on understanding the quantities involved in the problems and their relationships as well as on fluency in solving routine problems. Similarly, developing competence in solving nonroutine problems provides a context and motivation for learning to solve routine problems and for understanding concepts such as given, unknown, condition, and solution .
There are mutually supportive relations between strategic competence and both conceptual understanding and procedural fluency,
Strategic competence comes into play at every step in developing procedural fluency in computation. As students learn how to carry out an operation such as two-digit subtraction (for example, 86–59), they typically progress from conceptually transparent and effortful procedures to compact and more efficient ones (as discussed in detail in chapter 6 ). For example, an initial procedure for 86–59 might be to use bundles of sticks (see Box 4–3 ). A compact procedure involves applying a written numerical algorithm that carries out the same steps without the bundles of sticks. Part of developing strategic competence involves learning to replace by more concise and efficient procedures those cumbersome procedures that might at first have been helpful in understanding the operation.
Students develop procedural fluency as they use their strategic competence to choose among effective procedures. They also learn that solving challenging mathematics problems depends on the ability to carry out procedures readily and, conversely, that problem-solving experience helps them acquire new concepts and skills. Interestingly, very young children use a variety of strategies to solve problems and will tend to select strategies that are well suited to particular problems. 29 They thereby show the rudiments of adaptive reasoning, the next strand to be discussed.
Adaptive Reasoning
Adaptive reasoning refers to the capacity to think logically about the relationships among concepts and situations. Such reasoning is correct and valid, stems from careful consideration of alternatives, and includes knowledge of how to justify the conclusions. In mathematics, adaptive reasoning is the glue that holds everything together, the lodestar that guides learning. One uses it to navigate through the many facts, procedures, concepts, and solution methods and to see that they all fit together in some way, that they make sense. In mathematics, deductive reasoning is used to settle disputes and disagreements. Answers are right because they follow from some agreed-upon assumptions through series of logical steps. Students who disagree about a mathematical answer need not rely on checking with the teacher, collecting opinions from their classmates, or gathering data from outside the classroom. In principle, they need only check that their reasoning is valid.
Adaptive reasoning refers to the capacity to think logically about the relationships among concepts and situations.
Many conceptions of mathematical reasoning have been confined to formal proof and other forms of deductive reasoning. Our notion of adaptive reasoning is much broader, including not only informal explanation and justification but also intuitive and inductive reasoning based on pattern, analogy, and metaphor. As one researcher put it, “The human ability to find analogical correspondences is a powerful reasoning mechanism.” 30 Analogical reasoning, metaphors, and mental and physical representations are “tools to think with,” often serving as sources of hypotheses, sources of problem-solving operations and techniques, and aids to learning and transfer. 31
Some researchers have concluded that children’s reasoning ability is quite limited until they are about 12 years old. 32 Yet when asked to talk about how they arrived at their solutions to problems, children as young as 4 and 5 display evidence of encoding and inference and are resistant to counter suggestion. 33 With the help of representation-building experiences, children can demonstrate sophisticated reasoning abilities. After working in pairs and

One manifestation of adaptive reasoning is the ability to justify one’s work. We use justify in the sense of “provide sufficient reason for.” Proof is a form of justification, but not all justifications are proofs. Proofs (both formal and informal) must be logically complete, but a justification may be more telegraphic, merely suggesting the source of the reasoning. Justification and proof are a hallmark of formal mathematics, often seen as the province of older students. However, as pointed out above, students can start learning to justify their mathematical ideas in the earliest grades in elementary school. 38 Kindergarten and first-grade students can be given regular opportunities to talk about the concepts and procedures they are using and to provide good reasons for what they are doing. Classroom norms can be established in which students are expected to justify their mathematical claims and make them clear to others. Students need to be able to justify and explain ideas in order to make their reasoning clear, hone their reasoning skills, and improve their conceptual understanding. 39
It is not sufficient to justify a procedure just once. As we discuss below, the development of proficiency occurs over an extended period of time. Students need to use new concepts and procedures for some time and to explain and justify them by relating them to concepts and procedures that they already understand. For example, it is not sufficient for students to do only practice problems on adding fractions after the procedure has been developed. If students are to understand the algorithm, they also need experience in explaining and justifying it themselves with many different problems.
Adaptive reasoning interacts with the other strands of proficiency, particularly during problem solving. Learners draw on their strategic competence to formulate and represent a problem, using heuristic approaches that may provide a solution strategy, but adaptive reasoning must take over when
they are determining the legitimacy of a proposed strategy. Conceptual understanding provides metaphors and representations that can serve as a source of adaptive reasoning, which, taking into account the limitations of the representations, learners use to determine whether a solution is justifiable and then to justify it. Often a solution strategy will require fluent use of procedures for calculation, measurement, or display, but adaptive reasoning should be used to determine whether the procedure is appropriate. And while carrying out a solution plan, learners use their strategic competence to monitor their progress toward a solution and to generate alternative plans if the current plan seems ineffective. This approach both depends upon productive disposition and supports it.
Productive Disposition
Productive disposition refers to the tendency to see sense in mathematics, to perceive it as both useful and worthwhile, to believe that steady effort in learning mathematics pays off, and to see oneself as an effective learner and doer of mathematics. 40 If students are to develop conceptual understanding, procedural fluency, strategic competence, and adaptive reasoning abilities, they must believe that mathematics is understandable, not arbitrary; that, with diligent effort, it can be learned and used; and that they are capable of figuring it out. Developing a productive disposition requires frequent opportunities to make sense of mathematics, to recognize the benefits of perseverance, and to experience the rewards of sense making in mathematics.
Productive disposition refers to the tendency to see sense in mathematics, to perceive it as both useful and worthwhile, to believe that steady effort in learning mathematics pays off, and to see oneself as an effective learner and doer of mathematics.
A productive disposition develops when the other strands do and helps each of them develop. For example, as students build strategic competence in solving nonroutine problems, their attitudes and beliefs about themselves as mathematics learners become more positive. The more mathematical concepts they understand, the more sensible mathematics becomes. In contrast, when students are seldom given challenging mathematical problems to solve, they come to expect that memorizing rather than sense making paves the road to learning mathematics, 41 and they begin to lose confidence in themselves as learners. Similarly, when students see themselves as capable of learning mathematics and using it to solve problems, they become able to develop further their procedural fluency or their adaptive reasoning abilities. Students’ disposition toward mathematics is a major factor in determining their educational success. Students who view their mathematical ability as fixed and test questions as measuring their ability rather than providing opportunities to learn are likely to avoid challenging problems and be easily dis-
couraged by failure. 42 Students who view ability as expandable in response to experience and training are more likely to seek out challenging situations and learn from them. Cross-cultural research studies have found that U.S. children are more likely to attribute success in school to ability rather than effort when compared with students in East Asian countries. 43
Most U.S. children enter school eager to learn and with positive attitudes toward mathematics. It is critical that they encounter good mathematics teaching in the early grades. Otherwise, those positive attitudes may turn sour as they come to see themselves as poor learners and mathematics as nonsensical, arbitrary, and impossible to learn except by rote memorization. 44 Such views, once adopted, can be extremely difficult to change. 45
The teacher of mathematics plays a critical role in encouraging students to maintain positive attitudes toward mathematics. How a teacher views mathematics and its learning affects that teacher’s teaching practice, 46 which ultimately affects not only what the students learn but how they view themselves as mathematics learners. Teachers and students inevitably negotiate among themselves the norms of conduct in the class, and when those norms allow students to be comfortable in doing mathematics and sharing their ideas with others, they see themselves as capable of understanding. 47 In chapter 9 we discuss some of the ways in which teachers’ expectations and the teaching strategies they use can help students maintain a positive attitude toward mathematics, and in chapter 10 we discuss some programs of teacher development that may help teachers in that endeavor.
An earlier report from the National Research Council identified the cause of much poor performance in school mathematics in the United States:
The unrestricted power of peer pressure often makes good performance in mathematics socially unacceptable. This environment of negative expectation is strongest among minorities and women— those most at risk—during the high school years when students first exercise choice in curricular goals. 48
Some of the most important consequences of students’ failure to develop a productive disposition toward mathematics occur in high school, when they have the opportunity to avoid challenging mathematics courses. Avoiding such courses may eliminate the need to face up to peer pressure and other sources of discouragement, but it does so at the expense of precluding careers in science, technology, medicine, and other fields that require a high level of mathematical proficiency.
Research with older students and adults suggests that a phenomenon termed stereotype threat might account for much of the observed differences in mathematics performance between ethnic groups and between male and female students. 49 In this phenomenon, good students who care about their performance in mathematics and who belong to groups stereotyped as being poor at mathematics perform poorly on difficult mathematics problems under conditions in which they feel pressure to conform to the stereotype. So-called wise educational environments 50 can reduce the harmful effects of stereotype threat. These environments emphasize optimistic teacher-student relationships, give challenging work to all students, and stress the expandability of ability, among other factors.
Students who have developed a productive disposition are confident in their knowledge and ability. They see that mathematics is both reasonable and intelligible and believe that, with appropriate effort and experience, they can learn. It is counterproductive for students to believe that there is some mysterious “math gene” that determines their success in mathematics.
Hence, our view of mathematical proficiency goes beyond being able to understand, compute, solve, and reason. It includes a disposition toward mathematics that is personal. Mathematically proficient people believe that mathematics should make sense, that they can figure it out, that they can solve mathematical problems by working hard on them, and that becoming mathematically proficient is worth the effort.
Properties of Mathematical Proficiency
Now that we have looked at each strand separately, let us consider mathematical proficiency as a whole. As we indicated earlier and as the preceding discussion illustrates, the five strands are interconnected and must work together if students are to learn successfully. Learning is not an all-or-none phenomenon, and as it proceeds, each strand of mathematical proficiency should be developed in synchrony with the others. That development takes time. One of the most challenging tasks faced by teachers in pre-kindergarten to grade 8 is to see that children are making progress along every strand and not just one or two.
Learning is not an all-or-none phenomenon, and as it proceeds, each strand of mathematical proficiency should be developed in synchrony with the others.
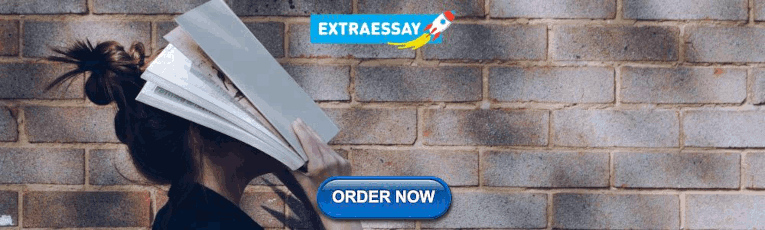
The Strands of Proficiency Are interwoven
How the strands of mathematical proficiency interweave and support one another can be seen in the case of conceptual understanding and procedural fluency. Current research indicates that these two strands of proficiency con-
tinually interact. 51 As a child gains conceptual understanding, computational procedures are remembered better and used more flexibly to solve new problems. In turn, as a procedure becomes more automatic, the child is enabled to think about other aspects of a problem and to tackle new kinds of problems, which leads to new understanding. When using a procedure, a child may reflect on why the procedure works, which may in turn strengthen existing conceptual understanding. 52 Indeed, it is not always necessary, useful, or even possible to distinguish concepts from procedures because understanding and doing are interconnected in such complex ways.
Consider, for instance, the multiplication of multidigit whole numbers. Many algorithms for computing 47×268 use one basic meaning of multiplication as 47 groups of 268, together with place-value knowledge of 47 as 40+7, to break the problem into two simpler ones: 40×268 and 7×268. For example, a common algorithm for computing 47×268 is written the following way, with the two so-called partial products, 10720 and 1876, coming from the two simpler problems:
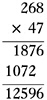
Familiarity with this algorithm may make it hard for adults to see how much knowledge is needed for it. It requires knowing that 40×268 is 4×10×268; knowing that in the product of 268 and 10, each digit of 268 is one place to the left; having enough fluency with basic multiplication combinations to find 7×8, 7×60, 7×200, and 4×8, 4×60, 4×200; and having enough fluency with multidigit addition to add the partial products. As students learn to execute a multidigit multiplication procedure such as this one, they should develop a deeper understanding of multiplication and its properties. On the other hand, as they deepen their conceptual understanding, they should become more fluent in computation. A beginner who happens to forget the algorithm but who understands the role of the distributive law can reconstruct the process by writing 268×47=268×(40+7)=(268×40)+(268×7) and working from there. A beginner who has simply memorized the algorithm without understanding much about how it works can be lost later when memory fails.
Proficiency is Not All or Nothing
Mathematical proficiency cannot be characterized as simply present or absent. Every important mathematical idea can be understood at many levels and in many ways. For example, even seemingly simple concepts such as even and odd require an integration of several ways of thinking: choosing alternate points on the number line, grouping items by twos, grouping items into two groups, and looking at only the last digit of the number. When children are first learning about even and odd, they may know one or two of these interpretations. 53 But at an older age, a deep understanding of even and odd means all four interpretations are connected and can be justified one based on the others.
The research cited in chapter 5 shows that schoolchildren are never complete mathematical novices. They bring important mathematical concepts and skills with them to school as well as misconceptions that must be taken into account in planning instruction. Obviously, a first grader’s understanding of addition is not the same as that of a mathematician or even a lay adult. It is still reasonable, however, to talk about a first grader as being proficient with single-digit addition, as long as the student’s thinking in that realm incorporates all five strands of proficiency. Students should not be thought of as having proficiency when one or more strands are undeveloped.
Proficiency Develops Over Time
Proficiency in mathematics is acquired over time. Each year they are in school, students ought to become increasingly proficient. For example, third graders should be more proficient with the addition of whole numbers than they were in the first grade.
Acquiring proficiency takes time in another sense. Students need enough time to engage in activities around a specific mathematical topic if they are to become proficient with it. When they are provided with only one or two examples to illustrate why a procedure works or what a concept means and then move on to practice in carrying out the procedure or identifying the concept, they may easily fail to learn. To become proficient, they need to spend sustained periods of time doing mathematics—solving problems, reasoning, developing understanding, practicing skills—and building connections between their previous knowledge and new knowledge.
How Mathematically Proficient Are U.S. Students Today?
One question that warrants an immediate answer is whether students in U.S. elementary and middle schools today are becoming mathematically proficient. The answer is important because it influences what might be recommended for the future. If students are failing to develop proficiency, the question of how to improve school mathematics takes on a different cast than if students are already developing high levels of proficiency.
The best source of information about student performance in the United States is, as we noted in chapter 2 , the National Assessment of Educational Progress (NAEP), a regular assessment of students’ knowledge and skills in the school subjects. NAEP includes a large and representative sample of U.S. students at about ages 9, 13, and 17, so the results provide a good picture of students’ mathematical performance. We sketched some of that performance in chapter 2 , but now we look at it through the frame of mathematical proficiency.
Although the items in the NAEP assessments were not constructed to measure directly the five strands of mathematical proficiency, they provide some useful information about these strands. As in chapter 2 , the data reported here are from the 1996 main NAEP assessment except when we refer explicitly to the long-term trend assessment. In general, the performance of 13-year-olds over the past 25 years tells the following story: Given traditional curricula and methods of instruction, students develop proficiency among the five strands in a very uneven way. They are most proficient in aspects of procedural fluency and less proficient in conceptual understanding, strategic competence, adaptive reasoning, and productive disposition. Many students show few connections among these strands. Examples from each strand illustrate the current situation. 54
Students’ conceptual understanding of number can be assessed in part by asking them about properties of the number systems. Although about 90% of U.S. 13-year-olds could add and subtract multidigit numbers, only 60% of them could construct a number given its digits and their place values (e.g., in the number 57, the digit 5 should represent five tens). 55 That is a common finding: More students can calculate successfully with numbers than can work with the properties of the same numbers.
The same is true for rational numbers. Only 35% of 13-year-olds correctly ordered three fractions, all in reduced form, 56 and only 35%, asked for a number between .03 and .04, chose the correct response. 57 These findings suggest that students may be calculating with numbers that they do not really understand.
An overall picture of procedural fluency is provided by the NAEP long-term trend mathematics assessment, 58 which indicates that U.S. students’ performance has remained quite steady over the past 25 years (see Box 4–4 ). A closer look reveals that the picture of procedural fluency is one of high levels of proficiency in the easiest contexts. Questions in which students are asked to add or subtract two- and three-digit whole numbers presented numerically in the standard format are answered correctly by about 90% of 13-year-olds, with almost as good performance among 9-year-olds. 59 Performance is slightly lower among 13-year-olds for division. 60
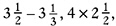
Results from NAEP dating back over 25 years have continually documented the fact that one of the greatest deficits in U.S. students’ learning of mathematics is in their ability to solve problems. In the 1996 NAEP, students in the fourth, eighth, and twelfth grades did well on questions about basic whole number operations and concepts in numerical and simple applied contexts. However, students, especially those in the fourth and eighth grades, had difficulty with more complex problem-solving situations. For example, asked to add or subtract two- and three-digit numbers, 73% of fourth graders and 86% of eighth graders gave correct answers. But on a multistep addition and subtraction word problem involving similar numbers, only 33% of fourth graders gave a correct answer (although 76% of eighth graders did). On the 23 problem-solving tasks given as part of the 1996 NAEP in which students had to construct an extended response, the incidence of satisfactory or better responses was less than 10% on about half of the tasks. The incidence of satisfactory responses was greater than 25% on only two tasks. 61
Performance on word problems declines dramatically when additional features are included, such as more than one step or extraneous information. Small changes in problem wording, context, or presentation can yield dramatic changes in students’ success, 62 perhaps indicating how fragile students’ problem-solving abilities typically are.
Several kinds of items measure students’ proficiency in adaptive reasoning, though often in conjunction with other strands. One kind of item asks students to reason about numbers and their properties and also assesses their conceptual understanding. For example,
If 49+83=132 is true, which of the following is true?
132–49=83
83–132=49
Only 61% of 13-year-olds chose the right answer, which again is considerably lower than the percentage of students who can actually compute the result.

A second kind of item that measures adaptive reasoning is one that asks students to justify and explain their solutions. One such item ( Box 4–5 ) required that students use subtraction and division to justify claims about the population growth in two towns. Only 1% of eighth graders in 1996 provided a satisfactory response for both claims, and only another 21% provided a partially correct response. The results were only slightly better at grade 12. In this item, Darlene’s claim is stated somewhat cryptically, and students may not have understood that they needed to think about population growth not additively—as in the case of Brian’s claim—but multiplicatively so as to conclude that Town A actually had the larger rate of growth. But given the low levels of performance on the item, we conclude that Darlene’s enigmatic claim was not the only source of difficulty. Students apparently have trouble justifying their answers even in relatively simple cases.
Research related to productive disposition has not examined many aspects of the strand as we have defined it. Such research has focused on attitudes
SOURCE: 1996 NAEP assessment. Cited in Wearne and Kouba, 2000, p. 186. Used by permission of National Council of Teachers of Mathematics.
toward mathematics, beliefs about one’s own ability, and beliefs about the nature of mathematics. In general, U.S. boys have more positive attitudes toward mathematics than U.S. girls do, even though differences in achievement between boys and girls are, in general, not as pronounced today as they were some decades ago. 64 Girls’ attitudes toward mathematics also decline more sharply through the grades than those of boys. 65 Differences in mathematics achievement remain larger across groups that differ in such factors as race, ethnicity, and social class, but differences in attitudes toward mathematics across these groups are not clearly associated with achievement differences. 66
The complex relationship between attitudes and achievement is well illustrated in recent international studies. Although within most countries, positive attitudes toward mathematics are associated with high achievement, eighth graders in some East Asian countries, whose average achievement in mathematics is among the highest in the world, have tended to have, on average, among the most negative attitudes toward mathematics. U.S. eighth
graders, whose achievement is around the international average, have tended to be about average in their attitudes. 67 Similarly, within a country, students who perceive themselves as good at mathematics tend to have high levels of achievement, but that relationship does not hold across countries. In Asian countries, perhaps because of cultural traditions encouraging humility or because of the challenging curriculum they face, eighth graders tend to perceive themselves as not very good at mathematics. In the United States, in contrast, eighth graders tend to believe that mathematics is not especially difficult for them and that they are good at it. 68
Data from the NAEP student questionnaire show that many U.S. students develop a variety of counterproductive beliefs about mathematics and about themselves as learners of mathematics. For example, 54% of the fourth graders and 40% of the eighth graders in the 1996 NAEP assessment thought that mathematics is mostly a set of rules and that learning mathematics means memorizing the rules. On the other hand, approximately 75% of the fourth graders and 75% of the eighth graders sampled reported that they understand most of what goes on in mathematics class. The data do not indicate, however, whether the students thought they could make sense out of the mathematics themselves or depended on others for explanations.
Despite the finding that many students associate mathematics with memorization, students at all grade levels appear to view mathematics as useful. The 1996 NAEP revealed that 69% of the fourth graders and 70% of the eighth graders agreed that mathematics is useful for solving everyday problems. Although students appear to think mathematics is useful for everyday problems or important to society in general, it is not clear that they think it is important for them as individuals to know a lot of mathematics. 69
Proficiency in Other Domains of Mathematics
Although our discussion of mathematical proficiency in this report is focused on the domain of number, the five strands apply equally well to other domains of mathematics such as geometry, measurement, probability, and statistics. Regardless of the domain of mathematics, conceptual understanding refers to an integrated and functional grasp of the mathematical ideas. These may be ideas about shape and space, measure, pattern, function, uncertainty, or change. When applied to other domains of mathematics, procedural fluency refers to skill in performing flexibly, accurately, and efficiently such procedures as constructing shapes, measuring space, computing probabilities, and describing data. It also refers to knowing when and how to use
The five strands apply equally well to other domains of mathematics such as geometry, measurement, probability, and statistics.
those procedures. Strategic competence refers to the ability to formulate mathematical problems, represent them, and solve them whether the problems arise in the context of number, algebra, geometry, measurement, probability, or statistics. Similarly, the capacity to think logically about the relationships among concepts and situations and to reason adaptively applies to every domain of mathematics, not just number, as does the notion of a productive disposition. The tendency to see sense in mathematics, to perceive it as both useful and worthwhile, to believe that steady effort in learning mathematics pays off, and to see oneself as an effective learner and doer of mathematics applies equally to all domains of mathematics. We believe that proficiency in any domain of mathematics means the development of the five strands, that the strands of proficiency are interwoven, and that they develop over time. Further, the strands are interwoven across domains of mathematics in such a way that conceptual understanding in one domain, say geometry, supports conceptual understanding in another, say number.
All Students Should Be Mathematically Proficient
Becoming mathematically proficient is necessary and appropriate for all students. People sometimes assume that only the brightest students who are the most attuned to school can achieve mathematical proficiency. Those students are the ones who have traditionally tended to achieve no matter what kind of instruction they have encountered. But perhaps surprisingly, it is students who have historically been less successful in school who have the most potential to benefit from instruction designed to achieve proficiency. 70 All will benefit from a program in which mathematical proficiency is the goal.
Historically, the prevailing ethos in mathematics and mathematics education in the United States has been that mathematics is a discipline for a select group of learners. The continuing failure of some groups to master mathematics—including disproportionate numbers of minorities and poor students—has served to confirm that assumption. More recently, mathematics educators have highlighted the universal aspects of mathematics and have insisted on mathematics for all students, but with little attention to the differential access that some students have to high-quality mathematics teaching. 71
One concern has been that too few girls, relative to boys, are developing mathematical proficiency and continuing their study of mathematics. That situation appears to be improving, although perhaps not uniformly across
grades. The 1990 and 1992 NAEP assessments indicated that the few gender differences in mathematics performance that did appear favored male students at grade 12 but not before. These differences were only partly explained by the historical tendency of male students to take more high school mathematics courses than female students do, since that gap had largely closed by 1992. In the 1996 NAEP mathematics assessment, the average scores for male and female students were not significantly different at either grade 8 or grade 12, but the average score for fourth-grade boys was 2% higher than the score for fourth-grade girls. 72
With regard to differences among racial and ethnic groups, the situation is rather different. The racial/ethnic diversity of the United States is much greater now than at any previous period in history and promises to become progressively more so for some time to come. The strong connection between economic advantage, school funding, and achievement in the United States has meant that groups of students whose mathematics achievement is low have tended to be disproportionately African American, Hispanic, Native American, students acquiring English, or students located in urban or rural school districts. 73 In the NAEP assessments from 1990 to 1996, white students recorded increases in their average mathematics scores at all grades. Over the same period, African American and Hispanic students recorded increases at grades 4 and 12, but not at grade 8. 74 Scores for African American, Hispanic, and American Indian students remained below scale scores for white students. The mathematics achievement gaps between average scores for these subgroups did not decrease in 1996. 75 The gap appears to be widening for African American students, particularly among students of the best-educated parents, which suggests that the problem is not one solely of poverty and disadvantage. 76
Students identified as being of middle and high socioeconomic status (SES) enter school with higher achievement levels in mathematics than low-SES students, and students reporting higher levels of parental education tend to have higher average scores on NAEP assessments. At all three grades, in contrast, students eligible for free or reduced-price lunch programs score lower than those not eligible. 77 Such SES-based differences in mathematics achievement are greater among whites than among other racial or ethnic groups. 78 Some studies have suggested that the basis for the differences resides in the opportunities available to students, including opportunities to attend effective schools, 79 opportunities afforded by social and economic factors of the home and school community, 80 and opportunities to get encouragement to continue the study of mathematics. 81
Goals for mathematics instruction like those outlined in our discussion of mathematical proficiency need to be set in full recognition of the differential access students have to high-quality mathematics teaching and the differential performance they show. Those goals should never be set low, however, in the mistaken belief that some students do not need or cannot achieve proficiency. In this day of rapidly changing technologies, no one can anticipate all the skills that students will need over their lifetimes or the problems they will encounter. Proficiency in mathematics is therefore an important foundation for further instruction in mathematics as well as for further education in fields that require mathematical competence. Schools need to prepare students to acquire new skills and knowledge and to adapt their knowledge to solve new problems.
The currency of value in the job market today is more than computational competence. It is the ability to apply knowledge to solve problems. 82 For students to be able to compete in today’s and tomorrow’s economy, they need to be able to adapt the knowledge they are acquiring. They need to be able to learn new concepts and skills. They need to be able to apply mathematical reasoning to problems. They need to view mathematics as a useful tool that must constantly be sharpened. In short, they need to be mathematically proficient.
Students who have learned only procedural skills and have little understanding of mathematics will have limited access to advanced schooling, better jobs, and other opportunities. If any group of students is deprived of the opportunity to learn with understanding, they are condemned to second-class status in society, or worse.
A Broader, Deeper View
Many people in the United States consider procedural fluency to be the heart of the elementary school mathematics curriculum. They remember school mathematics as being devoted primarily to learning and practicing computational procedures. In this report, we present a much broader view of elementary and middle school mathematics. We also raise the standard for success in learning mathematics and being able to use it. In a significant and fortuitous twist, raising the standard by requiring development across all five strands of mathematical proficiency makes the development of any one strand more feasible. Because the strands interact and boost each other, students who have opportunities to develop all strands of proficiency are more likely to become truly competent with each.
We conclude that during the past 25 years mathematics instruction in U.S. schools has not sufficiently developed mathematical proficiency in the sense we have defined it. It has developed some procedural fluency, but it clearly has not helped students develop the other strands very far, nor has it helped them connect the strands. Consequently, all strands have suffered. In the next four chapters, we look again at students’ learning. We consider not just performance levels but also the nature of the learning process itself. We describe what students are capable of, what the big obstacles are for them, and what knowledge and intuition they have that might be helpful in designing effective learning experiences. This information, we believe, reveals how to improve current efforts to help students become mathematically proficient.
Alexander, P.A., White, C.S., & Daugherty, M. (1997). Analogical reasoning and early mathematics learning. In L.D.English (Ed.), Mathematical reasoning: Analogies, metaphors, and images (pp. 117–147). Mahwah, NJ: Erlbaum.
Alibali, M.W. (1999). How children change their minds: Strategy change can be gradual or abrupt. Developmental Psychology , 35 , 127–145.
Ansell, E., & Doerr, H.M. (2000). NAEP findings regarding gender: Achievement, affect, and instructional experiences. In E.A.Silver & P.A.Kenney (Eds.), Results from the seventh mathematics assessment of the National Assessment of Educational Progress (pp. 73– 106). Reston, VA, National Council of Teachers of Mathematics.
Backer, A., & Akin, S. (Eds.). (1993). Every child can succeed: Reading for school improvement . Bloomington, IN: Agency for Instructional Television.
Baddeley, A.D. (1976). The psychology of memory . New York: Basic Books.
Ball, D.L., & Bass, H. (2000). Making believe: The collective construction of public mathematical knowledge in the elementary classroom. In D.Phillips (Ed.), Constructivism in education: Opinions and second opinions on controversial issues (Ninety-ninth Yearbook of the National Society for the Study of Education, Part 1, pp. 193– 224). Chicago: University of Chicago Press.
Beaton, A.E., Mullis, I.V.S., Martin, M.O., Gonzalez, E.J., Kelly, D.L., & Smith, T.A. (1996). Mathematics achievement in the middle school years: IEA’s Third International Mathematics and Science Study . Chestnut Hill, MA: Boston College, Center for the Study of Testing, Evaluation, and Educational Policy. Available: http://www.timss.org/timss1995i/MathB.html .
Bempechat, J. & Drago-Severson, E. (1999). Cross-national differences in academic achievement: Beyond etic conceptions of children’s understanding. Review of Educational Research , 69 (3), 287–314.
Bowman, B.T., Donovan, M.S., Burns, M.S. (Eds.). (2001). Eager to learn: Educating our preschoolers . Washington, DC: National Academy Press. Available: http://books.nap.edu/catalog/9745.html . [July 10, 2001].
Bransford, J.D., Brown, A.L., & Cocking, R.R. (Eds.). (1999). How people learn: Brain, mind, experience, and school . Washington, DC: National Academy Press. Available: http://books.nap.edu/catalog/6160.html . [July 10, 2001].
Brownell, W.A. (1935). Psychological considerations in the learning and the teaching of arithmetic. In W.D.Reeve (Ed.), The teaching of arithmetic (Tenth Yearbook of the National Council of Teachers of Mathematics, pp. 1–31). New York: Columbia University, Teachers College, Bureau of Publications.
Brownell, W.A. (1987). AT classic: Meaning and skill—maintaining the balance. Arithmetic Teacher , 34 (8), 18–25. (Original work published 1956).
Bruner, J.S. (1960). The process of education . New York: Vintage Books.
Campbell, J.R., Hombo, C.M., & Mazzeo, J. (2000). NAEP 1999 trends in academic progress: Three decades of student performance , NCES 2000–469. Washington, DC: National Center for Education Statistics. Available: http://nces.ed.gov/spider/webspider/2000469.shtml . [July 10, 2001].
Campbell, J.R., Voelkl, K.E., & Donahue, P.L. (2000). NAEP 1996 trends in academic progress (NCES 97–985r). Washington, DC: National Center for Education Statistics. Available: http://nces.ed.gov/spider/webspider/97985r.shtml . [July 10, 2001].
Carpenter, T.P., Corbitt, M.K., Kepner, H.S., Jr., Lindquist, M.M., & Reys, R.E. (1981). Results from the second mathematics assessment of the National Assessment of Educational Progress . Reston, VA: National Council of Teachers of Mathematics.
Carpenter, T.P., Franke, M.L., Jacobs, V.R., Fennema, E., & Empson, S.B. (1998). A longitudinal study of invention and understanding in children’s multidigit addition and subtraction. Journal for Research in Mathematics Education 29 , 3–20.
Carpenter, T.P., & Lehrer, R. (1999). Teaching and learning mathematics with understanding. In E.Fennema & T.A.Romberg (Eds.), Mathematics classrooms that promote understanding (pp. 19–32). Mahway, NJ: Erlbaum.
Carpenter, T.P., & Levi, L. (1999, April). Developing conceptions of algebraic reasoning in the primary grades. Paper presented at the annual meeting of the American Educational Research Association, Montreal.
Cobb, P., & Bauersfeld, H. (Eds.). (1995). The emergence of mathematical meaning: Interaction in classroom cultures . Hillsdale, NJ: Erlbaum.
Cobb, P., Yackel, E., & Wood, T. (1989). Young children’s emotional acts during mathematical problem solving. In D.B.McLeod & V.M.Adams (Eds.), Affect and mathematical problem solving: A new perspective (pp. 117–148). New York: Springer-Verlag.
Cobb, P., Yackel, E., & Wood, T. (1995). The teaching experiment classroom. In P.Cobb & H.Bauersfeld (Eds.), The emergence of mathematical meaning: Interaction in classroom cultures (pp. 17–24). Hillsdale, NJ: Erlbaum.
Committee for Economic Development, Research and Policy Committee. (1995). Connecting students to a changing world: A technology strategy for improving mathematics and science education: A statement . New York: Author.
Davis, R.B., & Maher, C.A. (1997). How students think: The role of representations. In L.D.English (Ed.), Mathematical reasoning: Analogies, metaphors, and images (pp. 93– 115). Mahwah, NJ: Erlbaum.
Donovan, M.S., Bransford, J.D., & Pellegrino, J.W. (Eds.). (1999). How people learn: Bridging research and practice . Washington, DC: National Academy Press. Available: http://books.nap.edu/catalog/9457.html . [July 10, 2001].
Druckman, D., & Bjork, R.A. (Eds.). (1991). In the mind’s eye: Enhancing human performance . Washington, DC: National Academy Press. Available: http://books.nap.edu/catalog/1580.html . [July 10, 2001].
Dweck, C. (1986). Motivational processes affecting learning. American Psychologist , 41 , 1040–1048.
English, L.D. (1997a). Analogies, metaphors, and images: Vehicles for mathematical reasoning. In L.D.English (Ed.), Mathematical reasoning: Analogies, metaphors, and images (pp. 3–18). Mahwah, NJ: Erlbaum.
English, L.D. (Ed.). (1997b). Mathematical reasoning: Analogies, metaphors, and images . Mahwah, NJ: Erlbaum.
Fennema, E. (1995). Mathematics, gender and research. In B.Grevholm & G.Hanna (Eds.), Gender and mathematics education (pp. 21–38). Lund, Sweden: Lund University Press.
Fennema, E., & Romberg, T.A. (Eds.). (1999). Mathematics classroom that promote understanding . Mahwah, NJ: Erlbaum.
Fuson, K.C. (1990). Conceptual structures for multiunit numbers: Implications for learning and teaching multidigit addition, subtraction, and place value. Cognition and Instruction 7 , 343–403.
Fuson, K.C. (1992a). Research on learning and teaching addition and subtraction of whole numbers. In G.Leinhardt, R.T.Putnam, & R.A.Hattrup (Eds.), The analysis of arithmetic for mathematics teaching (pp. 53–187). Hillsdale, NJ: Erlbaum.
Fuson, K.C. (1992b). Research on whole number addition and subtraction. In D.Grouws (Ed.), Handbook of research on mathematics teaching and learning (pp. 243–275). New York: Macmillan.
Fuson, K.C., & Briars, D.J. (1990). Using a base-ten blocks learning/teaching approach for first- and second-grade place-value and multidigit addition and subtraction. Journal for Research in Mathematics Education 21 , 180–206.
Fuson, K.C., & Burghardt, B.H. (1993). Group case studies of second graders inventing multidigit addition procedures for base-ten blocks and written marks. In J.R.Becker & B.J.Pence (Eds.), Proceedings of the fifteenth annual meeting of the North American Chapter of the International Group for the Psychology of Mathematics Education (pp. 240– 246). San Jose, CA: San Jose State University. (ERIC Document Reproduction Service No. ED 372 917).
Fuson, K.C., Carroll, W.M., & Landis, J. (1996). Levels in conceptualization and solving addition and subtraction compare word problems. Cognition and Instruction , 14 , 345– 371.
Geary, D.C. (1995). Reflections of evolution and culture in children’s cognition. American Psychologist , 50 (1), 24–37.
Greeno, J.G., Pearson, P.D., & Schoenfeld, A.H. (1997). Implications for the NAEP of research on learning and cognition. In R.Linn, R.Glaser, & G.Bohrnstedt (Eds.), Assessment in transition: Monitoring the nation’s educational progress (Background Studies, pp. 151–215). Stanford, CA: National Academy of Education.
Hagarty, M., Mayer, R.E., & Monk, C.A. (1995). Comprehension of arithmetic word problems: A comparison of successful and unsuccessful problem solvers. Journal of Educational Psychology , 87 , 18–32.
Hatano, G. (1988, Fall). Social and motivational bases for mathematical understanding. New Directions for Child Development , 41 , 55–70.
Hiebert, J. (Ed.). (1986). Conceptual and procedural knowledge: The case of mathematics . Hillsdale, NJ: Erlbaum.
Hiebert, J., & Carpenter, T.P. (1992). Learning and teaching with understanding. In D. A.Grouws (Ed.), Handbook of research on mathematics teaching and learning (pp. 65–97). New York: Macmillan.
Hiebert, J., Carpenter, T.P., Fennema, E., Fuson, K.C., Wearne, D., Murray, H., Olivier, A., & Human, P. (1997). Making sense: Teaching and learning mathematics with understanding . Portsmouth, NH: Heinemann.
Hiebert, J., & Wearne, D. (1986). Procedures over concepts: The acquisition of decimal number knowledge. In J.Hiebert (Ed.), Conceptual and procedural knowledge: The case of mathematics (pp. 199–223). Hillsdale, NJ: Erlbaum.
Hiebert, J., & Wearne, D. (1996). Instruction, understanding, and skill in multidigit addition and subtraction. Cognition and Instruction , 14 , 251–283.
Hilgard, E.R. (1957). Introduction to psychology (2nd ed.). New York: Harcourt Brace.
Inhelder, B., & Piaget, J. (1958). The growth of logical thinking from childhood to adolescence . New York: Basic Books.
Katona, G. (1940). Organizing and memorizing . New York: Columbia University Press.
Kilpatrick, J. (1985). Doing mathematics without understanding it: A commentary on Higbee and Kunihira. Educational Psychologist , 20 (2), 65–68.
Knapp, M.S., Shields, P.M., & Turnbull, B.J. (1995). Academic challenge in high-poverty classrooms. Phi Delta Kappan , 76 , 770–776.
Kouba, V.L., Carpenter, T.P., & Swafford, J.O. (1989). Number and operations. In M. M.Lindquist (Ed.), Results from the fourth mathematics assessment of the National Assessment of Educational Progress (pp. 64–93). Reston, VA: National Council of Teachers of Mathematics.
Kouba, V.L., & Wearne, D. (2000). Whole number properties and operations. In E.A. Silver & P.A.Kenney (Eds.), Results from the seventh mathematics assessment of the National Assessment of Educational Progress (pp. 141–161). Reston, VA: National Council of Teachers of Mathematics.
Krutetskii, V.A. (1976). The psychology of mathematical abilities in schoolchildren (J.Kilpatrick & I.Wirszup, Eds.; J.Teller, Trans.). Chicago: University of Chicago Press. (Original work published 1968).
Ladson-Billings, G. (1999). Mathematics for all? Perspectives on the mathematics achievement gap. Paper prepared for the Mathematics Learning Study Committee, National Research Council, Washington, DC.
Leder, G.C. (1992). Mathematics and gender: Changing perspectives. In D.Grouws (Ed.), Handbook of research on mathematics teaching and learning (pp. 597–622). New York: Macmillan.
Lemaire, P., & Siegler, R.S. (1995). Four aspects of strategic change: Contributions to children’s learning of multiplication. Journal of Experimental Psychology: General , 124 , 83–97.
Mack, N.K. (1995). Confounding whole-number and fraction concepts when building on informal knowledge. Journal for Research in Mathematics Education , 26 , 422–441.
Maher, C.A., & Martino, A.M. (1996). The development of the idea of mathematical proof: A 5-year case study. Journal for Research in Mathematics Education , 27 , 194–214.
Mason, D., Schroeter, D., Combs, R., & Washington, K. (1992). Assigning average achieving eighth graders to advanced mathematics classes in an urban junior high. Elementary School Journal , 92 , 587–599.
Mayer, R.E. (1999). The promise of educational psychology . Upper Saddle River, NJ: Prentice Hall .
Mayer, R.E., & Hegarty, M. (1996). The process of understanding mathematical problems. In R.J.Sternberg & T.Ben-Zee (Eds.), The nature of mathematical thinking (Studies in Mathematical Thinking and Learning Series, pp. 29–53). Mahwah, NJ: Erlbaum.
Mayer, R.E., & Wittrock, M.C. (1996). Problem-solving transfer. In D.C.Berliner & R. C.Calfee (Eds.), Handbook of educational psychology (pp. 47–62). New York: Macmillan.
McLeod, D.B. (1992). Research on affect in mathematics education: A reconceptualization. In D.A.Grouws (Ed.), Handbook of research on mathematics teaching and learning (pp. 575–596). New York: Macmillan.
Mullis, I.V.S., Jenkins, F., & Johnson, E.G. (1994). Effective schools in mathematics . Washington, DC: National Center for Education Statistics.
Mullis, I.V.S., Martin, M.O., Gonzalez, E.J., Gregory, K.D., Garden, R.A., O’Connor, K. M., Chrostowski, S.J., & Smith, T.A. (2000). TIMSS 1999 international mathematics report: Findings from IEA’s repeat of the Third International Mathematics and Science Study at the eighth grade . Chestnut Hill, MA: Boston College, Lynch School of Education, International Study Center. Available: http://www.timss.org/timss1999i/math_achievement_report.html . [July 10, 2001].
National Assessment Governing Board. (2000). Mathematics framework for the 1996 and 2000 National Assessment of Educational Progress . Washington, DC: Author. Available: http://www.nagb.org/pubs/96–2000math/toc.html . [July 10, 2001].
National Research Council. (1989). Everybody counts: A report to the nation on the future of mathematics education . Washington, DC: National Academy Press. Available: http://books.nap.edu/catalog/1199.html . [July 10, 2001].
National Research Council. (1998). High school mathematics at work: Essays and Examples for the education of all students . Washington, DC: National Academy Press. Available: http://books.nap.edu/catalog/5777.html . [July 10, 2001].
Nunes, T. (1992a). Cognitive invariants and cultural variation in mathematical concepts. International Journal of Behavioral Development , 15 , 433–453.
Nunes, T. (1992b). Ethnomathematics and everyday cognition. In D.A.Grouws (Ed.), Handbook of research on mathematics teaching and learning (pp. 557–574). New York: Macmillan.
Oakes, J. (1990). Multiplying inequalities: The effects of race, social class, and tracking on opportunities to learn mathematics and science . Santa Monica: CA: RAND Corporation.
Pólya, G. (1945). How to solve it: A new aspect of mathematical method . Princeton, NJ: Princeton University Press.
Pesek, D.D., & Kirshner, D. (2000). Interference of instrumental instruction in subsequent relational learning. Journal for Research in Mathematics Education , 31 , 524–540.
Reese, C.M., Miller, K.E., Mazzeo, J., & Dossey, J.A. (1997). NAEP 1996 mathematics report card for the nation and the states . Washington, DC: National Center for Education Statistics. Available: http://nces.ed.gov/spider/webspider/97488.shtml . [July 10, 2001].
Resnick, L.B. (1987). Education and learning to think . Washington, DC: National Academy Press. Available: http://books.nap.edu/catalog/1032.html . [July 10, 2001].
Resnick, L.B., & Omanson, S.F. (1987). Learning to understand arithmetic. In R. Glaser (Ed.), Advances in instructional psychology (vol. 3, pp. 41–95). Hillsdale, NJ: Erlbaum.
Rittle-Johnson, B., & Alibali, M.W. (1999). Conceptual and procedural knowledge of mathematics: Does one lead to the other? Journal of Educational Psychology , 91 , 175– 189.
Rittle-Johnson, B., & Siegler, R.S. (1998). The relation between conceptual and procedural knowledge in learning mathematics: A review. In C.Donlan (Ed.), The development of mathematical skills (pp. 75–110). East Sussex, UK: Psychology Press.
Saxe, G. (1990). Culture and cognitive development . Hillsdale, NJ: Erlbaum.
Schifter, D. (1999). Reasoning about operations: Early algebraic thinking in grades K-6. In L.V.Stiff (Ed.), Developing mathematical reasoning in grades K-12 (1999 Yearbook of the National Council of Teachers of Mathematics, pp. 62–81). Reston, VA: NCTM.
Schoenfeld, A.H. (1988). Problem solving in context(s). In R.I.Charles & E.A.Silver (Eds.), The teaching and assessing of mathematical problem solving (Research Agenda for Mathematics Education, vol. 3, pp. 82–92). Reston, VA: National Council of Teachers of Mathematics.
Schoenfeld, A.H. (1989). Explorations of students’ mathematical beliefs and behavior. Journal for Research in Mathematics Education , 20 , 338–355.
Schoenfeld, A.H. (1992). Learning to think mathematically: Problem solving, metacognition, and sense making in mathematics. In D.Grouws (Ed.), Handbook of research on mathematics teaching and learning (pp. 334–370). New York: Macmillan.
Secada, W.G. (1992). Race, ethnicity, social class, language, and achievement in mathematics. In D.Grouws (Ed.), Handbook of research on mathematics teaching and learning (pp. 623–660). New York: Macmillan.
Shannon, A. (1999). Keeping score . Washington, DC: National Academy Press. Available: http://books.nap.edu/catalog/9635.html . [July 10, 2001].
Siegler, R.S., & Jenkins, E.A. (1989). How children discover new strategies . Hillsdale, NJ: Erlbaum.
Silver, E.A., Alacaci, C., & Stylianou, D.A. (2000). Students’ performance on extended constructed-response tasks. In E.A.Silver & P.A.Kenney (Eds.), Results from the seventh mathematics assessment of the National Assessment of Educational Progress (pp. 301– 341). Reston, VA: National Council of Teachers of Mathematics.
Silver, E.A., & Kenney, P.A. (2000). Results from the seventh mathematics assessment of the National Assessment of Educational Progress . Reston, VA: National Council of Teachers of Mathematics.
Silver, E.A., Strutchens, M.E., & Zawojewski, J.S. (1997). NAEP findings regarding race/ethnicity and gender: Affective issues, mathematics performance, and instructional context. In E.A.Silver & P.A.Kenney (Eds.), Results from the sixth mathematics assessment of the National Assessment of Educational Progress (pp. 33–59). Reston, VA: National Council of Teachers of Mathematics.
Steele, C.M. (1997). A threat in the air: How stereotypes shape intellectual identity and performance. American Psychologist , 52 , 613–629.
Steele, C.M., & Aronson, J. (1995). Stereotype threat and the intellectual test performance of African-Americans. Journal of Personality and Social Psychology , 69 , 797–811.
Steinberg, R.M. (1985). Instruction on derived facts strategies in addition and subtraction. Journal for Research in Mathematics Education , 16 , 337–355.
Sternberg, R.J., & Rifkin, B. (1979). The development of analogical reasoning processes. Journal of Experimental Child Psychology , 27 , 195–232.
Stevenson, H.W., & Stigler, J.W. (1992). The learning gap: Why our schools are failing and what we can learn from Japanese and Chinese education . New York: Simon & Schuster.
Strutchens, M.E., & Silver, E.A. (2000). NAEP findings regarding race/ethnicity: Students’ performance, school experiences, and attitudes and beliefs. In E.A.Silver & P.A. Kenney (Eds.), Results from the seventh mathematics assessment of the National Assessment of Educational Progress (pp. 45–72). Reston, VA: National Council of Teachers of Mathematics .
Swafford, J.O., & Brown, C.A. (1989). Attitudes. In M.M.Lindquist (Ed.), Results from the fourth mathematics assessment of the National Assessment of Educational Progress (pp. 106–116). Reston, VA: National Council of Teachers of Mathematics.
Tate, W.F. (1997). Race, ethnicity, SES, gender, and language proficiency trends in mathematics achievement: An update. Journal for Research in Mathematics Education , 28 , 652–679.
Thompson, A.G. (1992). Teachers’ beliefs and conceptions: A synthesis of the research. In D.Grouws (Ed.), Handbook of research on mathematics teaching and learning (pp. 127– 146). New York: Macmillan.
Thornton, C.A., & Toohey, M.A. (1985). Basic math facts: Guidelines for teaching and learning. Learning Disabilities Focus , 1 (1), 44–57.
U.S. Department of Labor, Secretary’s Commission on Achieving Necessary Skills. (1991). What work requires of schools: A SCANS report for America 2000 . Washington, DC: Author. (ERIC Document Reproduction Service No. ED 332 054).
Wearne, D., & Hiebert, J. (1988). A cognitive approach to meaningful mathematics instruction: Testing a local theory using decimal numbers. Journal for Research in Mathematics Education , 19 , 371–384.
Wearne, D., & Kouba, V.L. (2000). Rational numbers. In E.A.Silver & P.A.Kenney (Eds.), Results from the seventh mathematics assessment of the National Assessment of Educational Progress (pp. 163–191). Reston, VA: National Council of Teachers of Mathematics.
Wertheimer, M. (1959). Productive thinking . New York: Harper & Row.
Wiest, L.R. (2000). Mathematics that whets the appetite: Student-posed projects problems. Mathematics Teaching in the Middle School , 5 , 286–291.
Wu, H. (1999, Fall). Basic skills versus conceptual understanding: A bogus dichotomy in mathematics education. American Educator , 14–19, 50–52.
Yaffee, L. (1999). Highlights of related research. In D.Schifter, V.Bastable, & S.J. Russell with L.Yaffee, J.B.Lester, & S.Cohen, Number and operations: Making meaning for operations. Casebook (pp. 127–149). Parsippany, NJ: Dale Seymour.
Zernike, K. (2000, August 25). Gap widens again on tests given to blacks and whites: Disparity widest among the best educated. The New York Times , p. A14.
Welcome to OpenBook!
You're looking at OpenBook, NAP.edu's online reading room since 1999. Based on feedback from you, our users, we've made some improvements that make it easier than ever to read thousands of publications on our website.
Do you want to take a quick tour of the OpenBook's features?
Show this book's table of contents , where you can jump to any chapter by name.
...or use these buttons to go back to the previous chapter or skip to the next one.
Jump up to the previous page or down to the next one. Also, you can type in a page number and press Enter to go directly to that page in the book.
Switch between the Original Pages , where you can read the report as it appeared in print, and Text Pages for the web version, where you can highlight and search the text.
To search the entire text of this book, type in your search term here and press Enter .
Share a link to this book page on your preferred social network or via email.
View our suggested citation for this chapter.
Ready to take your reading offline? Click here to buy this book in print or download it as a free PDF, if available.
Get Email Updates
Do you enjoy reading reports from the Academies online for free ? Sign up for email notifications and we'll let you know about new publications in your areas of interest when they're released.
Developing mathematical fluency: comparing exercises and rich tasks
- Open access
- Published: 26 September 2017
- Volume 97 , pages 121–141, ( 2018 )
Cite this article
You have full access to this open access article
- Colin Foster ORCID: orcid.org/0000-0003-1648-7485 1
22k Accesses
26 Citations
59 Altmetric
Explore all metrics
Achieving fluency in important mathematical procedures is fundamental to students’ mathematical development. The usual way to develop procedural fluency is to practise repetitive exercises, but is this the only effective way? This paper reports three quasi-experimental studies carried out in a total of 11 secondary schools involving altogether 528 students aged 12–15. In each study, parallel classes were taught the same mathematical procedure before one class undertook traditional exercises while the other worked on a “mathematical etude” (Foster International Journal of Mathematical Education in Science and Technology , 44 (5), 765–774, 2013b ), designed to be a richer task involving extensive opportunities for practice of the relevant procedure. Bayesian t tests on the gain scores between pre- and post-tests in each study provided evidence of no difference between the two conditions. A Bayesian meta-analysis of the three studies gave a combined Bayes factor of 5.83, constituting “substantial” evidence (Jeffreys, 1961 ) in favour of the null hypothesis that etudes and exercises were equally effective, relative to the alternative hypothesis that they were not. These data support the conclusion that the mathematical etudes trialled are comparable to traditional exercises in their effects on procedural fluency. This could make etudes a viable alternative to exercises, since they offer the possibility of richer, more creative problem-solving activity, with comparable effectiveness in developing procedural fluency.
Similar content being viewed by others
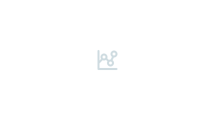
Word problems in mathematics education: a survey
Lieven Verschaffel, Stanislaw Schukajlow, … Wim Van Dooren
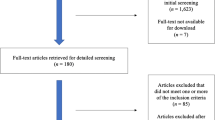
Retrieval Practice Consistently Benefits Student Learning: a Systematic Review of Applied Research in Schools and Classrooms
Pooja K. Agarwal, Ludmila D. Nunes & Janell R. Blunt
Research on early childhood mathematics teaching and learning
Camilla Björklund, Marja van den Heuvel-Panhuizen & Angelika Kullberg
Avoid common mistakes on your manuscript.
1 Introduction
Attaining fluency in key mathematical procedures is essential to students’ mathematical development (Department for Education [DfE], 2013 ; National Council of Teachers of Mathematics [NCTM], 2014 ; Truss, 2013 ). Being secure with important mathematical procedures gives students increased power to tackle more complicated mathematics at a more conceptual level (Codding, Burns, & Lukito, 2011 ; Foster, 2013b , 2016 ), since automating skills frees up mental capacity for being creative (Lemov, Woolway, & Yezzi, 2012 , p. 36). Devising ways to support the development of robust fluency with mathematical procedures is currently a focus of attention. For example, in England the national curriculum for mathematics emphasises procedural fluency as the first stated aim (DfE, 2013 ), and the current “mastery” agenda stresses “intelligent practice” as a route to simultaneously developing procedural fluency and conceptual understanding (Hodgen, 2015 ; National Association of Mathematics Advisors [NAMA], 2016 ; National Centre for Excellence in the Teaching of Mathematics [NCETM], 2016 ).
However, a focus on procedural fluency is sometimes seen as a threat to reform approaches to the learning of mathematics, which emphasise sense making through engagement with rich problem-solving tasks (Advisory Committee on Mathematics Education [ACME], 2012 ; Office for Standards in Education [Ofsted], 2012 ). In a technological age, in which calculators and computers can perform mathematical procedures quickly and accurately, it may be argued that teaching problem solving should be prioritised over practising procedures. It may also be that an excessive focus on basic procedures fails to kindle students’ interest in mathematics and could be linked to students, especially girls, not choosing to pursue mathematics beyond a compulsory phase (Boaler, 2002 ). Nevertheless, in a high-stakes assessment culture, where procedural skills are perceived to be the most straightforward ones to assess, the backwash effect of examinations is likely to lead to schools and teachers feeling constrained to prioritise the development of procedural fluency over these other aspects of learning mathematics (Foster, 2013c ; Ofsted, 2012 ; Taleporos, 2005 ).
In this context, it has been suggested that a mathematics task genre of etudes might be capable of addressing procedural fluency at the same time as offering a richer experience of learning mathematics (Foster, 2013b , 2014 ). Etudes are mathematics tasks in which extensive practice of a well-defined mathematical procedure is embedded within a rich mathematical problem (Foster, 2013b ). Such tasks aim to generate plentiful practice incidentally as learners tackle a rich, open-ended problem. East Asian countries which perform well in large-scale international assessments such as the Programme for International Student Assessment (PISA) and the Trends in International Mathematics and Science Study (TIMSS) are thought to succeed in emphasising mathematical fluency without resorting to low-level rote learning of procedures (Askew et al., 2010 ; Fan & Bokhove, 2014 ; Leung, 2014 ).
There have been many attempts to design tasks that incorporate meaningful practice (Kling & Bay-Williams, 2015 ) or exploit systematic variation (NAMA, 2016 ) to address fluency goals within deeper and more thought-provoking contexts. Not only might this lead to greater interest and motivation for students (Li, 1999 ), it is conceivable that it could assist in the development of procedural fluency by to some extent shifting students’ focus away from the details of the procedure, perhaps thereby aiding automation. From the point of view of being economical with students’ learning time, Hewitt ( 1996 ) described the generation of purposeful practice by subordinating the role of practice to a component of a larger mathematical problem. In this way, attention is placed not on the procedure being performed but instead on the effect of its use on a desired goal (Hewitt, 2015 ).
Mathematical etudes draw on these intentions to situate procedural practice within rich, problem-solving tasks. Although anecdotally etudes have been very favourably received by mathematics teachers, and appear to be popular with students, it is not known whether or not they are as effective as traditional exercises at developing procedural fluency. While etudes might be expected to offer other advantages, such as greater engagement and opportunities for creative problem solving and exploration, it is not known whether this comes at a cost of effectiveness in narrow terms of developing procedural fluency. It seems possible that diverting students’ attention away from the details of carrying out a procedure and onto some wider mathematics problem could hinder their immediate progress in procedural fluency. However, on the contrary, problem-solving aspects of an etude could potentially focus students on the details of a procedure in a way that supports development of fluency. So this paper investigates whether or not etudes are as effective as exercises for developing students’ procedural fluency.
2 Mathematical etudes
2.1 background.
Procedural fluency involves knowing when and how to apply a procedure and being able to perform it “accurately, efficiently, and flexibly” (NCTM, 2014 , p. 1). The Mathematical Etudes Project Footnote 1 aims to devise creative ways to help learners of mathematics develop their fluency in important mathematical procedures. It might be supposed that any varied diet of rich problem-solving tasks would automatically generate plentiful opportunities for students to gain practice in a multitude of important mathematical procedures, and that this would be a natural way for procedural fluency to be addressed in the curriculum. However, a rich, open-ended task may be approached in a variety of ways (Yeo, 2017 ), and, where a choice of approaches is possible, students may be drawn to those which utilise skills with which they are already familiar and comfortable. In this way, areas of weakness may remain unaddressed. For example, a student lacking confidence with algebra may be able to solve a mathematical problem successfully using numerical trial and improvement approaches, or perhaps by drawing an accurate graph. From the point of view of problem solving, selecting to use tools with which one is already competent is an entirely appropriate strategy, but if algebraic objectives were central to why the teacher selected the task, then the task has failed pedagogically. In this way, an open-ended task cannot necessarily be relied on to focus students’ attention onto a specific mathematical procedure. Even if it does succeed in doing this, it may not generate sufficient practice of the particular technique to develop the desired fluency, since a broader problem is likely to contain other aspects which also demand the student’s time and attention.
For this reason, an etude cannot simply be a problem which provides an opportunity for students to use the desired procedure; it must place that procedure at the centre of the students’ activity and force its repeated use. Success with the task must be contingent on repeated accurate application of the desired procedure. The Mathematical Etudes Project has developed numerous practical classroom tasks which embed extensive practice of single specified mathematical procedures within rich problem-solving contexts (Foster, 2011 , 2012a , b , 2013a , b , d , 2014 , 2015a ). It is whether such tasks are as effective as traditional exercises in developing fluency or not that is the subject of this research.
The term “etude” is borrowed from music, where an etude is “originally a study or technical exercise, later a complete and musically intelligible composition exploring a particular technical problem in an esthetically satisfying manner” (Encyclopaedia Britannica, 2007 ). Originally, etudes were intended for private practice, rather than performance, but later ones sought to achieve the twin objectives of satisfying an audience in concert as well as working as an effective tool for the development of the performer’s fluency. This latter sense inspires the idea of a mathematical etude, which is defined as a mathematics task that embeds “extensive practice of a well-defined mathematical technique within a richer, more aesthetically pleasing mathematical context” (Foster, 2013b , p. 766). In musical etudes, such as those by Chopin, the self-imposed constraint of focusing on (normally) a single specific technique may contribute to the beauty of the music.
The idea of practising a basic skill in the context of more advanced skills is common in areas such as sport (Willingham, 2009 , p. 125), and has been used within mathematics education. For example, Andrews ( 2002 ) outlined “a means by which practice could be embedded within a more meaningful and mathematically coherent activity” (p. 16). Boaler advised that it is best to “learn number facts and number sense through engaging activities that focus on mathematical understanding rather than rote memorization” (Boaler, 2015 , p. 6), and many have argued that algorithms do not necessarily have to be learned in a rote fashion (Fan & Bokhove, 2014 ). Watson and De Geest ( 2014 ) described systematic variation of tasks for the development of fluency, and it is known that, to be effective, practice must be purposeful, and systematically focused on small elements, and that feedback is essential (Ericsson & Pool, 2016 ). The challenge is to devise mathematics tasks which do this within a rich context.
The three etudes trialled in the studies described in this paper will now be discussed. Two of these etudes address solving linear equations in which the unknown quantity appears on both sides (studies 1 and 2), and the third etude concerns performing an enlargement of a given shape on a squared grid with a specified positive integer scale factor (study 3).
2.2 Linear equations etudes
The first two etudes described focus on solving linear equations. Both are intended to generate practice at solving linear equations in which the unknown quantity appears on both sides.
2.2.1 Expression polygons etude
In this etude, students are presented with the diagram shown in Fig. 1 , called an expression polygon (Foster, 2012a , 2013a , 2014 , 2015a ). Each line joining two expressions indicates that they are equated, and the initial task for students is to solve the six equations produced, writing each solution next to the appropriate line. For example, the top horizontal line joining x + 5 to 2 x + 2 generates the equation x + 5 = 2 x + 2, the solution to which is x = 3, so students write 3 next to this line. In addition to recording their solutions on the expression polygon, a student could write out their step-by-step methods on a separate piece of paper.
Expression polygons etude. (Taken from Foster, 2015a )
Having completed this, the students will obtain the solutions 1, 2, 3, 4, 5 and 6. The pattern is provocative, and students typically comment on it (Foster, 2012a , 2015a ). This leads naturally to a challenge: “Can you make up an expression polygon of your own that has a nice, neat set of solutions?” Students make choices over what they regard as “nice” and “neat”. They might choose to aim for the first six even numbers, first six prime numbers, first six squares or some other significant set of six numbers. Regardless of the specific target numbers chosen, the experimentation involved in producing their expression polygon is intended to generate extensive practice in solving linear equations. Working backwards from the desired solution to a possible equation, and modifying the numbers to make it work, necessitates unpicking the equation-solving process, which could contribute to understanding of and facility with the procedure. Students are expected to attend more to the solutions obtained than they would when working through traditional exercises, where the answers typically form no pattern and are of no wider significance than that individual question. As students gain facility in solving equations, they focus their attention increasingly on strategic decisions about which expressions to choose. They might even go on to explore what sets of six numbers may be the solutions of an expression polygon, or experiment with having five expressions rather than four, for instance. In this way, the task is intended to self-differentiate through being naturally extendable (Foster, 2015a , b ).
2.2.2 Devising equations etude
2.3 enlargements etude.
In this third etude, which addresses the topic of performing an enlargement of a given shape, students are presented with the diagram shown in Fig. 2 , containing a right-angled isosceles triangle on a squared grid. The task is to find the locus of all possible positions for a centre of enlargement such that, for a scale factor of 3, the image produced lies completely on the grid. Students can generally find, without too much difficulty, one centre of enlargement that will work, but finding all possible points is demanding and may entail reverse reasoning from the possible image vertex positions to those of the original triangle. Further extensions are possible by considering different starting shapes, different positions of the starting shape on the grid and different scale factors. In all of this work, the enlargement procedure is being practised extensively within a wider investigative context.
Enlargement etude grid. (Taken from Foster, 2013d )
2.4 Summary
Each of the three etudes described above is intended to generate extensive opportunities for practising a single specified procedure within a rich problem-solving context. However, although etudes might be anticipated to have benefits for students in terms of greater engagement and creative problem solving, it is not known how effective they are in comparison with the standard approach of traditional exercises in the narrow objective of developing students’ procedural fluency. It might be thought that incorporating other aspects beyond repetition of the desired procedure might to some extent diminish the effectiveness of a task for developing students’ procedural fluency. However, the opposite could be the case if the problem-solving context to some extent directs students’ attention away from the performance of the procedure and onto conceptual aspects, leading to greater automation. Consequently, the research question for these studies is: Are etudes as effective as traditional exercises at developing students’ procedural fluency or not?
In these exploratory studies, it is important to emphasise that a choice was made to compare etudes and exercises only in very narrow terms of procedural fluency. While it is likely that etudes offer other, harder-to-measure benefits for students, such as providing opportunities for creative, open-ended, inquiry-based exploration and problem solving, unless they are at least about as good as traditional exercises at developing students’ procedural fluency, it is unlikely, in a high-stakes assessment culture, that schools and teachers will feel able to use them regularly as an alternative. Traditional exercises are widely used by teachers not because they are perceived to be imaginative and creative sets of tasks but because they are believed to work in the narrow sense of developing fluency at necessary procedures. If there were some other way to achieve this, that did not entail the tedium of repetitive drill, it would presumably be preferred—provided that it were equally effective at the main job. For this reason, in these studies the focus was entirely on the effect of etudes on procedural fluency. Rather than trying to measure the plausible but more nebulous ways in which etudes might be superior, in this first exploratory set of studies it was decided to focus solely on the question of the effectiveness of etudes for the purpose of developing procedural fluency.
3 Study 1: Expression polygons
The aim of this study was to investigate whether a particular etude (“Expression polygons”, see Section 2.2.1 ) is as effective as traditional exercises at developing students’ procedural fluency in solving linear equations, relative to the alternative hypothesis that the etude and the exercises are not equally effective.
A quasi-experimental design was used, with pairs of classes at the same school assigned to either the intervention (the etude) or control (traditional exercises) condition. Data was collected across one or two lessons, in which students in the intervention group tackled an etude while those in the control group worked through as many traditional exercises as possible in the same amount of time. Pre- and post-tests were administered at the beginning and end of the lesson(s).
A classical t test on the gain scores (post-test − pre-test) would be suitable for detecting a statistically significant difference between the two groups; however, within the paradigm of null hypothesis significance testing, failure to find such a difference would not constitute evidence for the null hypothesis of no difference—it would simply be inconclusive (Dienes, 2014 ). No evidence of a difference is not evidence of no difference. This is because failure to detect a difference might be a consequence of an underpowered study, which might have been able to detect a difference had a larger sample size (or more sensitive test) been used. For this reason, it is necessary to use a Bayesian approach for these studies, in order to establish how likely is the hypothesis of no difference between the two treatments (etude and traditional exercises) in terms of gain in procedural fluency, relative to the alternative hypothesis that there is a difference. Thus, Bayesian t tests were carried out on the gain scores obtained in each study, as described below.
3.1.1 Instrument
The “Expression polygons” etude (Foster, 2012a , 2013a , 2015a ) discussed in Section 2.2.1 was used for the intervention groups, and the control groups were provided with traditional exercises and asked to complete as many as possible in the same amount of time (see Fig. 3 for both), normally around 20 min. The exercises consisted of linear equations in which the unknown appears on both sides, leading to small integer solutions. Pre- and post-tests were designed (Fig. 4 ), each consisting of four equations of the same kind as those used in the exercises. In this way, it was hoped that any bias in the focus of the tests would be toward the control group (exercises), since the matching between the exercises and the post-test was intended to be as close as possible. Each test was scored out of 4, with one mark given for the correct solution to each equation. The post-test included a space at the end for open comments, asking students to write down “what you think about the work you have done on solving equations”. This question was intended to capture students’ perceptions of the two different tasks.
Study 1 materials: expression polygons etude (intervention) and traditional exercises (control)
Study 1 pre-test and post-test
3.1.2 Participants
Schools were recruited through a Twitter request for help, and schools A, B and C (Table 1 ) took part in this study. These schools were a convenience sample, spanning a range of sizes and composition. In most schools in England, mathematics classes are set by attainment, and this was the case for schools A and B, while school C used mixed attainment classes. In all of the schools, teachers were asked to:
choose two similar classes (e.g. Year 8 or 9 parallel sets) who you are teaching to solve linear equations with the unknown on both sides (e.g. equations like 7 x − 1 = 5 x + 3). In these materials, all the solutions are whole numbers, but some may be negative.
A total of 241 mathematics students from Years 8 and 9 (age 12–14) participated. Forty-eight students’ pre- and post-tests could not be matched, either because they were not present for one of them or (for the vast majority) because they did not put their name clearly on the test. These students’ tests were excluded from the analysis, leaving N = 193. The large number of tests which could not be matched here was mainly due to the fact that in one particular class (20 students) none of the students wrote their names on either of their tests, and so none of the data from this class could be used.
3.1.3 Administration
Teachers were asked to use the materials with a pair of “parallel” classes across one or preferably two of the students’ normal mathematics lessons. Allocation was at class level, and schools were responsible for choosing pairs of classes that they regarded as similar, which were normally a pair from the same Year group which were setted classes at the same level (e.g., both set 3 out of 6). In most cases, the same teacher taught both classes, so as to minimise teacher effects.
Pre- and post-tests were administered individually in the same amount of time and until most students had finished (normally about 10 min. for each). Both classes were then taught by the teacher how to solve linear equations with the unknown on both sides. Teachers were asked to teach both classes “as you would normally, in the same way, and for approximately the same amount of time”. Following this, the control class received traditional exercises (Fig. 3 ), with the expectation that the number of questions would be more than enough for the time available (normally about 20 min.) and that students would not complete all of them, which generally proved to be the case. The intervention group received the “Expression polygons” etude (Fig. 3 ). Teachers were advised that “It is important that [the students] go beyond solving the six equations and spend some time generating their own expression polygons (or trying to).” Teachers were asked to allow the two classes the same amount of time to work on these tasks: “however much time you have available and feel is appropriate; ideally at least a whole lesson and perhaps more”. It is estimated that this was generally about 20–30 min. During this phase, teachers were asked to help both classes as they would normally, using their professional judgement as to what was appropriate, so that the students would benefit from the time that they spent on these tasks. Then the post-test was administered in the same way as the pre-test.
3.2 Results
The mean and standard deviation of the scores for both conditions at pre- and post-test, along with mean gain scores calculated as the mean of (post-test − pre-test) for each student, are shown in Table 2 and Fig. 5 . The similarity of the mean scores on the pre-test is reassuring regarding the matching of the parallel classes. A Bayesian t test was carried out on the gain scores, using the BayesFactor Footnote 2 package in R , comparing the fit of the data under the null hypothesis (the etude is as effective as the traditional exercises) and the alternative hypothesis (the etude and the exercises are not equally effective). A Bayes factor B indicates the relative strength of evidence for two hypotheses (Dienes, 2014 ; Rouder, Speckman, Sun, Morey, & Iverson, 2009 ), and means that the data are B times as likely under the null hypothesis as under the alternative. With a Cauchy prior width of .707, an estimated Bayes factor (null/alternative) of 1.03 was obtained, indicating no reason to conclude in favour of either hypothesis. (Conventionally, a Bayes factor between 3 and 10 represents “substantial” evidence [Jeffreys, 1961 ].) Prior robustness graphs for all of the Bayesian analyses described in this paper are included in the Appendix . In this case, calculation indicates that an exceptionally wide Cauchy prior width of more than 2.39 would be needed in order to obtain a “substantial” (Jeffreys, 1961 ) Bayes factor. The 95% credible interval Footnote 3 for the standardised effect size was [− .545, .005].
Study 1 results. (Error bars indicate ± 1 standard error)
Students’ comments on the study were few and generally related to the teaching episode rather than the etude or exercises. Insufficient responses meant that analysis of students’ perceptions of the two tasks was not possible.
3.3 Discussion
Such an inconclusive result does not allow us to say that either the exercises or the etude is superior in terms of developing procedural fluency, and neither does it allow us to say that there is evidence of no difference. Scrutiny of the students’ work suggested that in the time available many had engaged only superficially with the etude, whereas students in the control group had generally completed many exercises. It is possible that the style of the etude task was unfamiliar and/or that students were unclear regarding what they were supposed to do. For this reason, it was decided to devise a new etude to address the same topic of linear equations, one that it was hoped would be easier for students to understand and more similar in style to tasks that they might be familiar with. This etude formed the basis of study 2.
4 Study 2: Devising equations
The aim of this study was to investigate whether a different etude (“Devising equations”, see Section 2.2.2 ) is as effective as traditional exercises at developing students’ procedural fluency in solving linear equations, relative to the alternative hypothesis that the etude and the exercises are not equally effective.
The same quasi-experimental design was used as in study 1, with pairs of classes at the same school assigned to either the intervention (the “Devising equations” etude, see Section 2.2.2 ) or control (traditional exercises).
4.1.1 Instrument and administration
This time the intervention group received the “Devising equations” etude, as described in Section 2.2.2 (see Fig. 6 ). The control group received the same set of traditional exercises as used in study 1 (see Fig. 3 ) and were asked to complete as many as possible in the same amount of time as given to the etudes group. The same pre- and post-tests were used as in study 1 (see Fig. 4 ). Administration was exactly as for study 1, except that this time the only advice given to teachers regarding the etude was that students should “generate and solve their own equations”.
Study 2 “Devising equations” task
4.1.2 Participants
Schools were again recruited through a Twitter request. Schools D, E, F, G and H (Table 1 ) took part, all of which used attainment setting for mathematics. Teachers were again asked to choose parallel classes, and a total of 213 mathematics students from Years 8 and 9 (age 12–14) participated. This time, 19 students’ pre- and post-tests could not be matched, because students did not always put their names on their tests, leaving N = 194.
4.2 Results
Results are shown in Table 3 and Fig. 7 . As in study 1, a Bayesian t test was carried out on the gain scores (Dienes, 2014 ; Rouder et al., 2009 ), with a Cauchy prior width of .707, this time giving a Bayes factor (null/alternative) of 5.92. This means that the data are nearly six times as likely under the null hypothesis (the etude is as effective as the traditional exercises) as under the alternative hypothesis (the etude and the exercises are not equally effective). Conventionally, a Bayes factor between 3 and 10 represents “substantial” evidence (Jeffreys, 1961 ). The prior robustness graph (see Appendix ) indicates that any Cauchy prior width of more than .317 would have led to a Bayes factor of at least 3, which suggests that this finding is robust. The 95% credible interval for the standardised effect size was [−.326, .233].
Study 2 results. (Error bars indicate ± 1 standard error)
Again, students’ comments were insufficiently plentiful or focused on the task to enable an analysis.
4.3 Discussion
Study 2 provides substantial evidence that there is little difference across one or two lessons between the effect on students’ procedural fluency of using traditional exercises or the “Devising equations” etude. Examination of students’ work showed a much greater engagement with this etude than with the “Expression polygons” one used in study 1, as evidenced by far more written work, so it is plausible that the effect of this etude might consequently have been stronger and, in this case, was closely matched to that of the exercises.
In an attempt to extend the bounds of generalisability of this finding, a third study was conducted, using the enlargements etude discussed in Section 2.3 , in order to see whether a similar result would be obtained in a different topic area.
5 Study 3: Enlargements
The aim of this study was to investigate whether a third etude (“Enlargements”, see Section 2.3 ) is as effective as traditional exercises at developing students’ procedural fluency in a different (geometric) topic area: performing an enlargement of a given shape on a squared grid with a specified positive integer scale factor. As before, the alternative hypothesis was that the etude and the exercises are not equally effective.
The same quasi-experimental design was used as in studies 1 and 2, with pairs of parallel classes in each school assigned to either the intervention (this time the “Enlargements” etude) or control (traditional exercises) condition.
5.1.1 Instrument and administration
The “Enlargements” etude (Foster, 2013d ) discussed in Section 2.3 was used for the intervention groups, and the control groups were provided with traditional exercises and asked to complete as many as possible in the same amount of time (see Fig. 8 for both). The exercises consisted of a squared grid containing five right-angled triangles and four given points. Each question asked students to enlarge one of the given shapes by a scale factor of 2, 3 or 5, using as centre of enlargement one of the given points. Pre- and post-tests were administered (Fig. 9 ), in which students were asked to enlarge a given triangle with a scale factor of 4 on a squared grid about a centre of enlargement marked with a dot. The pre- and post-tests were intended to be as similar as possible in presentation to the traditional exercises, again in the hope that any bias in the focus of the post-test would be in favour of the control group. As before, the post-test included a space at the end for open comments, asking students to write down “what you think about the work you have done on enlargements”. Each test was scored out of 4, with one mark for each correctly positioned vertex and one for an enlarged triangle of the correct shape, size and orientation (not necessarily position). Administration was exactly as for studies 1 and 2, except that this time teachers were asked to ensure
that the students understand that they are meant to try to find as many possible positions for the centre of enlargement as they can—perhaps even the whole region where these centres can be. Students could also go on to explore what happens if the starting triangle is in a different position, or is a different shape, or if a different scale factor is used (original emphasis).
The purpose of this was to try to ensure that the students would engage extensively with the etude and not assume that finding one viable centre of enlargement was all that was required.
Study 3 materials: enlargement etude (intervention) and traditional exercises (control)
Study 3 pre-test and post-test
5.1.2 Participants
As before, schools were recruited through a Twitter request. Schools I, J and K in Table 1 took part, all of which used attainment setting for mathematics lessons. Teachers were again asked to choose parallel classes, and a total of 151 mathematics students from Years 9 and 10 (age 13–15) participated. Year 9–10 classes were used this time, rather than Year 8–9 classes, due to teachers’ choices about suitability for this different topic. This time, only 10 students’ pre- and post-tests could not be matched, again because of missing names on some of the tests, leaving N = 141.
5.2 Results
Analysis proceeded as before, and the results are shown in Table 4 and Fig. 10 . Again, a Bayesian t test was carried out on the gain scores (Dienes, 2014 ; Rouder et al., 2009 ), with a Cauchy prior width of .707, this time giving a Bayes factor (null/alternative) of 5.20, meaning that the data are about five times as likely under the null hypothesis (the etude is as effective as the traditional exercises) as under the alternative hypothesis (the etude and the exercises are not equally effective). Conventionally, a Bayes factor between 3 and 10 represents “substantial” evidence (Jeffreys, 1961 ). The prior robustness graph (see Appendix ) indicates that any Cauchy prior width of more than .365 would have led to a Bayes factor of at least 3, which suggests that the finding is robust. The 95% credible interval for the standardised effect size was [−.384, .257].
Study 3 results. (Error bars indicate ± 1 standard error)
Once again, student comments were too few to allow a reasonable analysis.
5.3 Discussion
Study 3 provides substantial evidence that there is no difference across one or two lessons between the effect on students’ procedural fluency of using traditional exercises or this enlargement etude. Examination of students’ work showed a lot of drawing on the sheets, with many students correctly finding the locus of all possible positions for the centre of enlargement. It may be that this greater degree of engagement (relative to study 1) could account for this etude being of comparable benefit to the exercises, as was the case in study 2.
6 General discussion
The Bayes factors obtained in these three studies were combined using the BayesFactor package in R and the meta.ttestBF Bayesian meta-analysis function. Again using a Cauchy prior width of .707, this time an estimated combined Bayes factor (null/alternative) of 5.83 was obtained (Table 5 ). This again falls within the conventionally accepted range of 3 to 10 for “substantial” evidence (Jeffreys, 1961 ). This means that, taken together, the three studies reported support the conclusion in favour of the null hypothesis that the etudes are as effective as the traditional exercises in developing students’ procedural fluency, relative to the alternative hypothesis that the etudes and the exercises are not equally effective.
The smaller Bayes factor for study 1 may have resulted from a less clearly articulated etude that was unfamiliar in style to the students, requiring a greater degree of initiative in constructing expressions than is normally expected in mathematics classrooms. If it is the case that students were less sure what was expected of them, this could explain why the etudes group carried out less equation solving here than the exercises group did. As reported above, in studies 2 and 3, a greater effort was made in the teacher instructions to explain the intentions of the task, and a greater engagement with the etudes was inferred from the quantity of written work produced.
7 Conclusion
These three exploratory studies suggest that the etudes trialled here are as effective as the traditional exercises in developing students’ procedural fluency. Consequently, for a hypothetical teacher whose sole objective was to develop students’ procedural fluency, it should be a matter of indifference whether to do this by means of exercises or etudes. Given the plausible benefits of etudes in terms of richness of experience and opportunity for open-ended problem solving and creative thinking, it may be that etudes might on balance be preferred (Foster, 2013b ).
It should be stressed that only three etudes were tested in these studies across only two mathematics topics and with students aged 12–15. Further studies using other etudes in other topic areas and with students outside this age range would be necessary to extend the generalisability of this finding. In addition, studies including delayed post-tests would be highly desirable, but were not practicable for this initial exploratory study. It would also be important to examine evidence for the hypothesised benefits of etudes beyond the narrow focus of these studies on procedural fluency. For example, it is plausible that etudes are more engaging for students, provide opportunities for students to operate more autonomously and solve problems, promote discussion and reasoning and support conceptual understanding of the mathematics. Classroom observation data, other kinds of assessments, as well as canvassing teacher and student perspectives, would be necessary to explore the extent to which this might be the case.
Caution must be exercised in interpreting these findings, since the constraints of the participating schools did not allow random allocation of students to condition (etude or exercises). Instead, schools selected pairs of “parallel” classes, generally based on level of class within the Year group (e.g., set 3 out of 6). It is reassuring that pre-test scores were generally close across the two conditions, but there remains the possibility that the parallel classes differed on some relevant factor. It should also be noted that some pre- and post-tests could not be matched, as students did not write their names on their tests, meaning that these tests had to be excluded from the data. In studies 2 and 3, the percentages of tests excluded were 9% and 7%, respectively, but in study 1 the percentage was much higher (20%). However, this was largely the result of one particular class, in which none of the students wrote their names on either test; ignoring this class, the percentage of missing data was a less severe 12%. However, these higher than desirable percentages of missing data are a reason to be cautious in interpreting these findings.
The extent of the guidance given to teachers about how to use the etudes was necessarily highly limited by the constraints of these studies. For practical reasons, the entire instructions on conducting the trials were restricted to one side of A4 paper. No professional development was involved, as these trials were carried out at a distance, and in most cases the participating schools and teachers were recruited via Twitter and contacted solely by email, and were not known personally to the researcher. It may be supposed that students would derive far greater benefit from etudes if they were deployed by teachers who had received professional development which involved prior opportunities to think about and discuss ways of working with these sorts of tasks. It remains for future work to explore this possibility.
www.mathematicaletudes.com
http://bayesfactorpcl.r-forge.r-project.org/
A credible interval is the Bayesian analogue of a frequentist confidence interval. A credible interval has the much simpler interpretation that the probability of the parameter lying in the 95% credible interval is .95. The 95% highest-density intervals were also calculated for the standardised effect size for each study, and in each case were extremely close to the 95% credible intervals, and so are not reported.
ACME. (2012). Raising the bar: Developing able young mathematicians . London: Advisory Committee on Mathematics Education. Retrieved from http://www.acme-uk.org/media/10498/raisingthebar.pdf . Accessed 23 Sep 2017.
Andrews, P. (2002). Angle measurement: An opportunity for equity. Mathematics in School , 31 (5), 16–18.
Google Scholar
Askew, M., Hodgen, J., Hossain, S., & Bretscher, N. (2010). Values and variables: Mathematics education in high-performing countries . London: Nuffield Foundation.
Boaler, J. (2002). Experiencing school mathematics: Traditional and reform approaches to teaching and their impact on student learning . London: Routledge.
Boaler, J. (2015). Fluency without fear: Research evidence on the best ways to learn math facts . Stanford, CA: youcubed. Retrieved from https://www.youcubed.org/fluency-without-fear/ . Accessed 23 Sep 2017.
Codding, R. S., Burns, M. K., & Lukito, G. (2011). Meta-analysis of mathematic basic-fact fluency interventions: A component analysis. Learning Disabilities Research & Practice , 26 (1), 36–47.
Article Google Scholar
Department for Education (DfE). (2013). Mathematics programmes of study: Key stage 3: National curriculum in England . London: DfE.
Dienes, Z. (2014). Using Bayes to get the most out of non-significant results. Frontiers in Psychology , 5 , 781.
Encyclopaedia Britannica. (2007). Étude . Retrieved from https://www.britannica.com/art/etude-music . Accessed 11 Feb 2017.
Ericsson, A., & Pool, R. (2016). Peak: Secrets from the new science of expertise . New York: Houghton Mifflin Harcourt.
Fan, L., & Bokhove, C. (2014). Rethinking the role of algorithms in school mathematics: A conceptual model with focus on cognitive development. ZDM Mathematics Education , 46 (3), 481–492.
Foster, C. (2011). A picture is worth a thousand exercises. Mathematics Teaching , 224 , 10–11.
Foster, C. (2012a). Connected expressions. Mathematics in School , 41 (5), 32–33.
Foster, C. (2012b). Working without a safety net. The Australian Mathematics Teacher , 68 (2), 25–29.
Foster, C. (2013a). Connected quadratics. Teach Secondary , 2 (1), 46–48.
Foster, C. (2013b). Mathematical études: Embedding opportunities for developing procedural fluency within rich mathematical contexts. International Journal of Mathematical Education in Science and Technology , 44 (5), 765–774.
Foster, C. (2013c). Resisting reductionism in mathematics pedagogy. Curriculum Journal , 24 (4), 563–585.
Foster, C. (2013d). Staying on the page. Teach Secondary , 3 (1), 57–59.
Foster, C. (2014). Mathematical fluency without drill and practice. Mathematics Teaching , 240 , 5–7.
Foster, C. (2015a). Expression polygons. Mathematics Teacher , 109 (1), 62–65.
Foster, C. (2015b). The convergent–divergent model: An opportunity for teacher–learner development through principled task design. Educational Designer , 2 (8).
Foster, C. (2016). Confidence and competence with mathematical procedures. Educational Studies in Mathematics , 91 (2), 271–288.
Hewitt, D. (1996). Mathematical fluency: The nature of practice and the role of subordination. For the Learning of Mathematics , 16 (2), 28–35.
Hewitt, D. (2015). The economic use of time and effort in the teaching and learning of mathematics. In S. Oesterle & D. Allan (Eds.), Proceedings of the 2014 Annual Meeting of the Canadian Mathematics Education Study Group (pp. 3–23). Edmonton, Canada: University of Alberta.
Hodgen, J. (2015). Unlocking potential in our curriculum: Mastering subjects. In A. Bingham (Ed.), Lessons learned: Putting experience to work (pp. 35–44). London: Ark, King’s College London.
Jeffreys, H. (1961). The theory of probability (3rd ed.). Oxford: Oxford University Press.
Kling, G., & Bay-Williams, J. M. (2015). Three steps to mastering multiplication facts. Teaching Children Mathematics , 21 (9), 548–559.
Lemov, D., Woolway, E., & Yezzi, K. (2012). Practice perfect: 42 rules for getting better at getting better . San Francisco, CA: Jossey-Bass.
Leung, F. K. (2014). What can and should we learn from international studies of mathematics achievement? Mathematics Education Research Journal, 26 (3), 579–605.
Li, S. (1999). Does practice make perfect? For the Learning of Mathematics , 19 (3), 33–35.
National Association of Mathematics Advisors [NAMA]. (2016). Five myths of mastery in mathematics. Mathematics Teaching , 251 , 20–24.
National Centre for Excellence in the Teaching of Mathematics [NCETM] (2016). The essence of maths teaching for mastery . Retrieved from https://www.ncetm.org.uk/files/37086535/The+Essence+of+Maths+Teaching+for+Mastery+june+2016.pdf . Accessed 23 Sep 2017.
National Council of Teachers of Mathematics [NCTM]. (2014). Procedural fluency in mathematics: A position of the National Council of Teachers of Mathematics . Reston, VA: NCTM.
Office for Standards in Education [Ofsted]. (2012). Mathematics: Made to measure . London: Ofsted. Retrieved from https://www.gov.uk/government/uploads/system/uploads/attachment_data/file/417446/Mathematics_made_to_measure.pdf . Accessed 23 Sep 2017.
Rouder, J. N., Speckman, P. L., Sun, D., Morey, R. D., & Iverson, G. (2009). Bayesian t tests for accepting and rejecting the null hypothesis. Psychonomic Bulletin & Review , 16 (2), 225–237.
Taleporos, E. (2005). Consequential validity: A practitioner’s perspective. Educational Measurement: Issues and Practice , 17 (2), 20–23.
Truss, E. (2013, January 17). Education minister Elizabeth Truss speaks at the North of England Education Conference . Retrieved from https://www.gov.uk/government/speeches/education-minister-elizabeth-truss-speaks-at-the-north-of-england-education-conference . Accessed 11 Feb 2017.
Watson, A., & De Geest, E. (2014). Department-initiated change. Educational Studies in Mathematics , 87 (3), 351–368.
Willingham, D. T. (2009). Why don’t students like school: A cognitive scientist answers questions about how the mind works and what it means for the classroom . San Francisco, CA: Jossey-Bass.
Yeo, J. B. (2017). Development of a framework to characterise the openness of mathematical tasks. International Journal of Science and Mathematics Education, 15 (1), 175–191.
Download references
Author information
Authors and affiliations.
Centre for Research in Mathematics Education, School of Education, University of Nottingham, Nottingham, NG8 1BB, UK
Colin Foster
You can also search for this author in PubMed Google Scholar
Corresponding author
Correspondence to Colin Foster .
Appendix: Prior robustness graphs for the three Bayesian analyses
Horizontal dashed lines show the conventional cut-off Bayes Factor of 3 for “substantial” evidence (Jeffreys, 1961 ).
Rights and permissions
Open Access This article is distributed under the terms of the Creative Commons Attribution 4.0 International License (http://creativecommons.org/licenses/by/4.0/), which permits unrestricted use, distribution, and reproduction in any medium, provided you give appropriate credit to the original author(s) and the source, provide a link to the Creative Commons license, and indicate if changes were made.
Reprints and permissions
About this article
Foster, C. Developing mathematical fluency: comparing exercises and rich tasks. Educ Stud Math 97 , 121–141 (2018). https://doi.org/10.1007/s10649-017-9788-x
Download citation
Published : 26 September 2017
Issue Date : February 2018
DOI : https://doi.org/10.1007/s10649-017-9788-x
Share this article
Anyone you share the following link with will be able to read this content:
Sorry, a shareable link is not currently available for this article.
Provided by the Springer Nature SharedIt content-sharing initiative
- Bayesian hypothesis testing
- Enlargements
- Linear equations
- Mathematics education
- Find a journal
- Publish with us
- Track your research

An official website of the United States government
The .gov means it’s official. Federal government websites often end in .gov or .mil. Before sharing sensitive information, make sure you’re on a federal government site.
The site is secure. The https:// ensures that you are connecting to the official website and that any information you provide is encrypted and transmitted securely.
- Publications
- Account settings
Preview improvements coming to the PMC website in October 2024. Learn More or Try it out now .
- Advanced Search
- Journal List
- HHS Author Manuscripts

The Role of Cognitive Processes, Foundational Math Skill, and Calculation Accuracy and Fluency in Word-Problem Solving versus Pre-Algebraic Knowledge
Lynn s. fuchs.
1 Vanderbilt University
Jennifer K. Gilbert
Sarah r. powell.
2 University of Texas at Austin
Paul T. Cirino
3 University of Houston
Douglas Fuchs
Carol l. hamlett, pamela m. seethaler, tammy d. tolar.
The purpose of this study was to examine child-level pathways in development of pre-algebraic knowledge versus word-problem solving, while evaluating the contribution of calculation accuracy and fluency as mediators of foundational skills/processes. Children ( n = 962; mean 7.60 years) were assessed on general cognitive processes and early calculation, word-problem, and number knowledge at start of grade 2; calculation accuracy and calculation fluency at end of grade 2; and pre-algebraic knowledge and word-problem solving at end of grade 4. Important similarities in pathways were identified, but path analysis also indicated that language comprehension is more critical for later word-problem solving than pre-algebraic knowledge. We conclude that pathways in development of these forms of 4 th -grade mathematics performance are more alike than different, but demonstrate the need to fine-tune instruction for strands of the mathematics curriculum in ways that address individual students’ foundational mathematics skills or cognitive processes.
Little is understood about how aspects of mathematical cognition relate to each other: which are shared or distinct or how difficulty in one domain corresponds to difficulty in another. Such understanding would provide theoretical insight into the nature of mathematics competence and practical guidance about how to organize curriculum and design instruction. In the present longitudinal study, we focused on two forms of higher-order mathematics among 7- to 10-year-olds: pre-algebraic knowledge and word-problem solving.
Algebra is a high priority area. It represents a gateway to higher forms of learning in mathematics, science, technology, and engineering and is required for successful participation in the workforce ( National Mathematics Advisory Panel [NMAP], 2008 ; RAND Mathematics Study Panel, 2003 ). Yet, a substantial portion of students fail to complete an algebra course, and 93% of 17-year-olds cannot solve multistep algebra problems ( U.S. Department of Education, 2008 ). The NMAP emphasized the need for “longitudinal research … to identify early predictors of success or failure with algebra” (p.33). The hope is that “the identification of these predictors will help guide the design of interventions that will build the foundational skills needed for success with algebra” (p. 33). However, we identified only several studies that have examined predictors of algebra performance.
At the same time, performance on word problems is the best school-age predictor of employment and wages in adulthood ( Bynner, 1997 ; Every Child a Chance Trust, 2009 ), and word problems represent a major emphasis in almost every strand of the math curriculum from kindergarten through high school. As with algebra, however, word problems are a stumbling block for many students ( Fuchs, Fuchs, Stuebing, et al., 2008 ); they can be a persistent deficit even when calculation skill is adequate ( Swanson et al., 2008 ); and the cognitive processes involved in word problems differ from and are more numerous than those underlying calculation skill (e.g., Fuchs et al., 2010 ; Fuchs et al., 2006 ; Fuchs, Fuchs, Stuebing et al., 2008 ). Thus, as with algebra, word-problem difficulty may be multiply determined and difficult to prevent.
Given the importance of algebra and word problems, along with the cognitive complexity of both domains, the purpose of the present study was to contrast sources of individual differences in the development of end-of-fourth-grade pre-algebraic knowledge versus word-problem competence. We considered cognitive processes (reasoning, language comprehension, working memory, attentive behavior, processing speed) and foundational mathematics competencies (calculation skill, understanding about number) measured at the start of second grade. This permitted us to assess whether development in these two challenging domains is more alike or different.
Additionally, although calculation skill is transparently required for success with both outcomes, disagreement among mathematics educators focuses on whether calculation accuracy is sufficient or whether calculation fluency provides added value (e.g., Greene, 2010 ; Warner, 2006 ). Consensus, as reflected in state standards, presently sides on the need for fluency (e.g., National Governors Association for Best Practices, 2001). Yet, we identified only one study ( Carr & Alexeev, 2011 ) that examined whether calculation fluency provides added value over accuracy. This was also a major purpose of the present study.
Algebra involves symbolizing and operating on numerical relationships and mathematical structures. Algebraic expressions can be treated procedurally, by substituting numerical values to yield numerical results ( Kieran, 1990 ). This suggests that understanding of arithmetic principles involves generalizations that are algebraic in nature. Pillay, Wilss, and Boulton-Lewis’s (1998) model of learning poses three stages in the development of algebraic competence. The first involves arithmetic skill: operating numerically and understanding of operational laws and relational meaning of the equal sign (i.e., both sides are the same value) in standard equations. This provides the foundation for a pre-algebraic stage, characterized by understanding of relational meaning of the equal sign in nonstandard equations, the concept of unknowns in equations, and the concept of a variable. This stage supports development of the final stage, formal algebraic competence. Pillay et al.’s model (1998) is consistent with a connection between arithmetic and algebra, as conceptualized by Blanton and Kaput (2001) , Carraher and Schliemann (2002) , and the NMAP (2008) . Moreover, the model’s second stage, with its focus on the equal sign’s relational meaning in nonstandard equations, the concept of unknowns in equations, and the concept of a variable, clarifies the potential connection between arithmetic and algebra, and it captures essential foci for measuring the progression from arithmetic to algebra.
Research illustrates how conventional instruction ( Powell, 2012 ) fails to support understanding of the equal sign as relational ( Baroody & Ginsberg, 1983 ; McNeil & Alibali, 2005 ). In answering 5 + 4 = __ + 7, common errors are 9 (i.e., students ignore the operation to the right side of the equal sign) and 16 (i.e., they add all knowns; Falkner, Levi, & Carpenter, 1999 ). Both errors reflect misunderstanding about the equal sign as operational (signaling the problem solver to apply an operation). Such confusion, which often persists into high school ( NMAP, 2008 ), is associated with difficulty in using algebraic notation to represent word problems ( Powell & Fuchs, 2010 ) and solve linear equations ( Alibali, Knuth, Hattikudur, McNeil, & Stephens, 2007 ; Knuth, Stephens, McNeil, & Alibali, 2006 ). This literature illustrates how understanding of the equal sign as a relational symbol represents an important form of pre-algebraic knowledge.
A growing body of research operationalizes pre-algebraic knowledge in line with Pillay et al. (1998) , in terms of understanding of the equal sign, variables, and unknowns in nonstandard equations. In the closest prior study, Fuchs et al. (2012) found that second-grade arithmetic calculations and word problems are foundational to such pre-algebraic knowledge at third grade. Results also revealed direct contributions of reasoning and language comprehension, measured at the start of second grade, to pre-algebraic knowledge, as well as indirect effects that occurred via third-grade arithmetic calculations and word problems. By contrast, attentive behavior and processing speed contributed to pre-algebraic knowledge only indirectly, but working memory’s paths (direct and indirect) were not significant.
In terms of the contributions of calculation accuracy and fluency, a large literature demonstrates a role for one or the other in a variety of mathematics outcomes. In the only study we identified that included both variables in the same models to estimate the contribution of one while controlling for the effects of the other, Carr and Alexeev (2011) indexed fluency and accuracy at the start of second grade as students solved 1-digit problems. Growth mixture modeling was used to estimate the effect of fluency and accuracy on latent classes that described the mix of strategies children used over grades 2 through 4 to solve more complex addition and subtraction problems. Fluency predicted growth in strategies, whereas accuracy was associated with strategies used at the start of second grade. Latent class membership in turn predicted whether students exceeded, passed, or failed the state’s fourth-grade mathematics test indexing a variety of skills. The authors concluded that both dimensions of calculation skill contributed to later math achievement. Yet, as they noted, the study did not control for conceptual knowledge about number or general cognitive processes. Also, because the outcome was a general mathematics achievement test, it was not possible to identify whether fluency and accuracy affect different types of higher-order mathematics outcomes in varying ways.
Extensions to the Literature and Hypotheses
We extended Carr and Alexeev (2011) , Fuchs et al. (2012) , and other studies (e.g., Lee, Ng, Bull, Pe, & Ho, 2011 ; Tolar, Lederberg, & Fletcher, 2009 ) in six ways. First, we examined direct effects of calculation accuracy and fluency on two specific, highly valued outcomes, while evaluating accuracy and fluency as potential mediators of earlier foundational skills/processes. Second, we created a more stringent test of the predictors of pre-algebra knowledge by contrasting them to the predictors involved in later word problems. This allowed us to assess which predictors of pre-algebra are unique to or similar across the two higher-order forms of mathematical competence. Third, in examining sources of individual differences in the development of pre-algebra, we considered a longer timeframe, from start of second through end of fourth grade, when pre-algebraic thinking is gaining sophistication. Fifth, our large sample provided power to detect more subtle effects. Sixth, as outlined by Duncan et al. (2014) , we conducted within-sample robustness checks on our key findings. Our hypotheses were as follows.
Direct effects of intermediary calculation and fluency
We expected a direct, unique contribution of intermediary calculation accuracy and fluency on each fourth-grade outcome. We based this on Pillay et al.’s (1998) model and Carr and Alexeev’s (2011) findings. Moreover, calculation accuracy is a transparent component of pre-algebra tasks and word-problem solving, and a longstanding assumption in the mathematics and reading literatures poses that fluency on lower-level skill frees attentional and working memory resources to permit students to focus on the cognitive complexities of higher-order performance ( Geary et al., 2008 ; Kim, Wagner, & Foster, 2011 ). In mathematics, calculation fluency may also reflect understanding and generalization of arithmetic operational laws, which may directly support pre-algebraic thinking and generation of word-problem models.
Relation between fourth-grade pre-algebra knowledge and word-problem solving
We hypothesized a strong relation between fourth-grade pre-algebra and word problems for three reasons. First, in a randomized control trial, Fuchs et al. (2014) found that word-problem instruction (that did not include instruction on calculations) improved pre-algebra performance. Second, relational understanding of the equal sign, reflected in measures of pre-algebraic knowledge, is associated with the ability to use algebraic notation to represent word problems ( Powell & Fuchs, 2010 ). Third, word-problem solving reflects understanding of relationships between known and unknown quantities and may therefore be supported by pre-algebraic thinking. A key source of error in word problems involves transforming problem narratives into algebraic equations ( Geary et al., 2008 ). For example, competent problem solvers translate “Sue had 3 more than Bill” to S = 3 + B, by recognizing the smaller quantity must increase to equal the larger quantity. Yet, a common error is S + 3 = B.
Direct and indirect effects of foundational (start-of-second-grade) processes
We anticipated that foundational calculation skill and understanding about number would directly affect both outcomes, while expecting indirect effects for these foundational math competencies via intermediary calculation accuracy and fluency. In terms of general cognitive processes, we anticipated a combination of direct and indirect effects for reasoning and language comprehension on both outcomes (e.g., Fuchs et al., 2012 for pre-algebra; e.g., Fuchs et al., 2010 for word problems), but expected lower-level processes (attentive behavior, processing speed) to contribute only indirectly via intermediary math skills. This was the case in Fuchs et al. (2012) , the closest prior study on pre-algebra, and a connection for calculation skill with attentive behavior and processing speed has been widely established. Finally, we hypothesized direct and indirect effects for working memory. We did so because, even though its effects were not significant in Fuchs et al. (2012) , a longstanding assumption holds that working memory supports cognitively complex, multi-step problem solving, as required for both outcomes, and individual differences in working memory may emerge as a predictor of the more complex pre-algebra tasks expected of fourth graders (compared to third grade, as in Fuchs et al., 2012 ).
Participants
At start of second grade, we identified 1062 second-grade children for participation to represent low-, average-, and high-performing students. By spring of fourth grade (three school years later), 135 of these students had moved to schools beyond our reach. After dropping those cases, 962 students with complete data remained, providing a representative sample as reflected on the Wide Range Achievement Test-3 (WRAT; Wilkinson, 1993 ) Arithmetic subtest ( M = 100.61; SD = 10.87) and Reading subtest ( M = 100.92; SD = 15.42). The 962 children were in 95 second-grade classrooms in 17 schools. They dispersed to 238 third-grade classrooms in 80 schools and then to 295 fourth-grade classrooms across 91 schools (creating too many unique classroom and school sequences to make clustering relevant in our statistical models). There were no significant differences on any demographic or performance variable at the start of grade 2 between students who remained in the study and those who moved. Of the 962 students, 52% were male; 83.25% received subsidized lunch; and 42% were African American, 27% Caucasian, and 25% White Hispanic. As start of second grade, 5.20% had been retained 1 school year; 5% received special education services; and 12% received English language services. Mean age was 7.60 ( SD = 0.35).
Control Variable
Word problems.
Following Jordan and Hanich (2000) , Word Problems comprises 14 brief word problems involving change, combine, compare, and equalize relationships and requiring single-digit addition or subtraction for solution (i.e., sums of 7, 8, or 9 or subtrahends of 6, 7, 8, or 9; there are no addends or minuends of zero or one; answers to the subtraction problems are from 2 to 6). The tester reads each item aloud; students have 30 sec to respond and can ask for re-reading(s) as needed. The score is the number of correct answers. On this sample, α was .87.
Foundational (Start-of-Second-Grade) Mathematics and Cognitive Measures
Calculations.
WRAT-3-Arithmetic ( Wilkinson, 1993 ) comprises an oral and a written component. The 15-item oral portion focuses on early numerical competencies (e.g., counting objects, identifying Arabic numerals, holding up a specified number of fingers). Because we wanted to estimate start-of-second-grade calculation skill, we relied on the written component, which provides students 10 min to answer calculation problems of increasing difficulty. All students finished working in < 10 min. In this sample, the mean score on the written portion at start of second grade was 4.98 ( SD = 1.91). The first seven items on the written portion are 1- and 2-digit adding or subtracting problems involving basic facts without regrouping. With a SD of 1.91, 68% of participants were within a score of zero and 7. On this sample, α was .89.
Number understanding
With Number Line Estimation ( Siegler & Booth, 2004 ), children locate Arabic numerals on a number line marked with 0 and 100 as endpoints. A number line is presented with a target number (3, 4, 6, 8, 12, 17, 21, 23, 25, 29, 33, 39, 43, 48, 52, 57, 61, 64, 72, 79, 81, 84, 90, 96) shown above the line. Children place the target number on the line, without a time limit. The score is the absolute difference between the student’s placement and the actual placement, averaged over trials. Lower scores indicate stronger performance, but we multiplied scores by −1 before running correlational analyses. Test-retest reliability on 87 children was .85.
WASI Matrix Reasoning ( Wechsler, 1999 ) includes pattern completion, classification, analogy, and serial reasoning tasks. Children complete matrices. From each, a section is missing, and the child restores the matrix by selecting from 5 options. For example, an item might show a 2 by 2 grid with the same picture of a box, half of one color and half another color, in three cells and a question mark in the fourth cell. The bottom of the page shows a row of 5 boxes, each with the same colors. The tester instructs the child to say which of the five boxes goes where the question mark is. On this sample, α was .90.
Language comprehension
Woodcock Diagnostic Reading Battery ( WDRB) - Listening Comprehension ( Woodcock, 1997 ) measures the ability to understand sentences or passages. With 38 items, students supply the word missing at the end of sentences or passages that progress from simple verbal analogies and associations to discerning implications. The test manual provides examples of correct responses to guide the tester’s scoring. On this sample, α was .81.
Working memory
We used the dual-task central executive Listening Recall subtest from the Working Memory Test Battery for Children (WMTB-C; Pickering & Gathercole, 2001 ), with which the child determines if each sentence in a series is true and then recalls the last word in each sentence. It has six items at span levels from 1-6 to 1-9. Passing four items at a level moves the child to the next level. At each span level, the number of items to be remembered increases by one. Failing three items at a given span terminates the subtest. We used the trials correct score. Test-retest reliability on 82 students was .82.
Attentive behavior
SWAN is an 18-item teacher rating scale ( Swanson et al., 2012 ) that samples items from the Diagnostic and Statistical Manual of Mental Disorders-IV (APA, l994) criteria for Attention-Deficit/Hyperactivity Disorder for inattention (items 1-9) and hyperactivity/impulsivity (items 10-18). Validity is supported in the literature ( Arnett et al., 2013 ; Lakes, Swanson, & Riggs, 2012 ; Swanson et al., 2012 ). Items are rated as 1 = Far Below, 2 = Below, 3 = Slightly Below; 4 = Average, 5 = Slightly Above, 6 = Above, 7 = Far Above. We report data for the attentive behavior subscale as the average rating across the nine relevant items. We selected this subscale to index attentive behavior, or the ability to maintain focus of attention. SWAN correlates well with other dimensional assessments of behavior related to attention. On this sample, α was .99.
Processing speed
With WJ-III Visual Matching ( Woodcock, McGrew, & Mather, 2001 ), children locate and circle two identical numbers in each row of six numbers. They have 3 min to complete 60 rows. On this sample, reliability was .86.
Intermediary (End-of-Second-Grade) Mathematics Measures
Calculation accuracy.
With Addition Strategy Assessment ( Geary, Hoard, Byrd-Craven, & DeSoto, 2004 ), 14 simple addition problems are presented horizontally, one at a time at the center of a computer screen. Addends are 2 - 9; doubles problems are not used. The child solves each problem without paper and is directed to use whatever strategy is easiest to obtain the answer. There is no time limit. The score is the number of items answered correctly. On this sample, α was .93.
Calculation fluency
From the Second-Grade Calculations Battery ( Fuchs, Hamlett, & Powell, 2003 ), we administered two subtests of single-digit addition: Sums to 12 and Sums to 18. For each, students have 1 min to complete 25 problems. We combined the subtests to create one score. On a sample of 79 students, test-retest reliability was .87.
Mathematics Outcome (End-of-Fourth-Grade) Measures
With the Iowa Test of Basic Skills -Level 10 (ITBS; Hoover, Hieronymous, Dunbar, & Frisbie, 1993 ), students respond in multi-choice format to 24 word problems that require a single-step calculation (3 items); multiple-step calculations (7 items); identify insufficient information (2 items); choose solution methods (2 items); read amounts on bar graphs (2 items); locate information in specific cell in table (1 item); and compare quantities and interpret relationships and trends to determine rank (2 items), determine a sum (1 item), determine difference (1 item), find ratio (1 item); understand underlying relationship (1 item); and generalize (1 item). On this sample, α was .83.
Pre-algebraic knowledge
The Test of Pre-Algebraic Knowledge ( Fuchs, Seethaler, & Powell, 2009 ) comprises two types of problems. The first (20 items) involves mathematical equivalence items with letters standing for missing quantities: 18 in nonstandard format (e.g., y + 4 = 9; 1 + 5 = 4 + x ; 8 − 4 = 6 − y ; 8 − x = 3 + 3); two in standard format (i.e., 1 + 5 = x ; 8 − 3 = y ). The second problem type (4 items) involves function tables, each of which shows a 2-column table. The first column shows a variable, and the second column shows a function involving that variable; each row shows a value for the variable and the resulting value for the function. In one row, the value of the function is empty; the task is to complete that row. The functions are x + 3, y − 6, 2 x + 1, and 3 y . The tester demonstrates how to complete a problem for each problem type (i.e., to ensure students understand the task demands). Testers provide 8 min for students to complete the first problem type; as much time as needed (until all but two students are finished) to complete the other problem type. The correlation between the two problem types at fourth grade was .61. The pattern of results was the same for the two problem types; so we used the total score. On this sample, α was .86.
Testers were trained to criterion at each testing occasion and used standard directions for administration. In September of second grade, they administered calculation and word-problem tests in large groups. In September-October of second grade, they administered the number line and general cognitive measures in individual sessions. In spring of second grade, they administered the calculation accuracy measure individually and the fluency measure in large groups. In spring of fourth grade, they administered word-problem solving and pre-algebraic knowledge measures in small groups. All individual sessions were audiotaped; 15% of tapes were selected randomly, stratifying by tester, for accuracy checks by an independent scorer. Agreement exceeded 99%.
Data Analysis and Results
To investigate the relations of foundational skills and processes, intermediary calculation competence, and later higher-level mathematics outcomes, we estimated a minimally restricted path model that included direct as well as indirect paths of seven foundational processes to both end-of-fourth-grade math outcomes, using the post-hoc MODEL INDIRECT command in Mplus 7.31 ( Muthén & Muthén, 1998-2012 ). All variables submitted to the model were continuous, and we applied alpha of .05 to evaluate significance. Because the eighth foundational skill, word-problem solving, represented an autoregressive effect on the word-problem outcome (and because we did not have a beginning-of-second-grade index of pre-algebra due to expected floor effects), we only modeled beginning-of-second-grade word-problem’s direct effect on the outcomes with the goal of adjusting results for prior skill. In the rest of the paper, we use the term foundational processes to refer to the seven foundational variables of interest (excluding beginning-of-grade-2 word problems). Global fit statistics indicated this model fit the data well (see Kline, 2011 ), χ 2 (2) = 3.641, p = .162; RMSEA = .029 (90% CI = [.000, .076]), p = .706; CFI = .998; TLI = .959; and variance/covariance residuals all below < .10. We also tested the difference in model fit between this model and one in which the direct paths from each variable to the two outcomes were simultaneously constrained to equality. This constrained model fit the data significantly worse than our model in which all direct effects were estimated freely, χ 2 (10) = 24.58, p = .006.
Equality of direct effects, indirect effects, and total effects on the later outcomes were calculated on standardized estimates using the MODEL CONSTRAINT command. Note that because the two fourth-grade outcomes were measured on approximately the same scale (min, max, mean, standard deviation [ SD ]), a change in 1 SD is substantively comparable between the outcomes. We conducted tests of the equality of paths that were significant for one but not the other outcome to determine whether effects were significantly different across the two outcomes. We opted for this criterion, despite its arbitrariness (i.e., two significant paths could significantly differ from one another), because null-hypothesis significance testing forces a dichotomous decision about whether an effect is different from 0 and because we wanted to test actual differences in cases where one might be tempted to conclude that an effect is important for one outcome but not for another. 1
Table 1 shows zero-order correlations among the variables of interest. All were positive, but none showed evidence of extreme collinearity (tolerances > .50) when predictors were entered into simple regression models for the two outcomes. Because skew, kurtosis, and extreme values were detected in our data and because ceiling effects were detected for the intermediary accuracy variable, we used the CENSORED option for accuracy and requested 95% bias-corrected bootstrap confidence intervals (BCBS CI) based on 5,000 replications to account for non-normality violations. The default estimator for models including censored variables, WLSMV, was retained.
Means, Standard Deviations, and Correlations (N = 962)
Note. Word-problem solving skill is Iowa Test of Basic Skill-Level 10-Math. Pre-algebraic knowledge is Test of Pre-Algebraic Knowledge. Calculation accuracy is Addition Strategy Assessment. Calculation fluency is Second-Grade Calculations Battery. Calculation skill is Wide Range Achievement Test-Arithmetic. Word-problem solving skill is Word Problems. Number understanding is Number Line Estimation (reverse scored). Reasoning is Wechsler Abbreviated Scale of Intelligence-Matrix Reasoning. Language comprehension is Woodcock Diagnostic Reading Battery-Listening Comprehension. Working memory is Working Memory Test Battery for Children-Listening Recall. Attentive behavior is Strengths and Weaknesses of ADHD Symptoms and Normal Behavior Rating Scales. Processing speed is Woodcock-Johnson Tests of Achievement III-Visual Matching.
Direct effects are reported in Table 2 , indirect effects in Table 3 , and total effects (sum of direct and indirect effects) in Table 4 . Each table shows unstandardized results with the corresponding 95% BCBS CI as well as the standardized path/effect. Figure 1 depicts the significant effects (bolded lines) and nonsignificant effects (dashed lines). Residual correlations were .22 between the intermediary variables and .40 between the higher-order mathematics outcomes. Statistical significance of each path/effect was judged by the unstandardized 95% BCBS CI, but standardized paths/effects are presented throughout the remainder of the Results section to give readers a sense of path/effect magnitude.

Note. Path analysis diagram for Model 1 showing hypothesized direct and indirect paths from beginning of grade 2 foundational skills and processes to end of grade 4 higher-level mathematics outcomes through end of grade 2 intermediary calculation skills. Solid lines represent significant direct paths. Dashed lines represent nonsignificant direct paths. Tested indirect paths are listed to the left of each foundational skill/process; significant indirect paths have been bolded. Word-problem solving skill is Word Problems; shading indicates this variable had only direct and not indirect paths to the outcomes. Calculation skill is Wide Range Achievement Test-Arithmetic. Number understanding is Number Line Estimation (reverse scored). Reasoning is Wechsler Abbreviated Scale of Intelligence-Matrix Reasoning. Language comprehension is Woodcock Diagnostic Reading Battery-Listening Comprehension. Working memory is Working Memory Test Battery for Children-Listening Comprehension. Working memory is Working Memory Test Battery for Children Listening Recall. Attentive behavior is Strengths and Weaknesses of ADHD Symptoms and Normal Behavior Rating Scales. Processing speed is Woodcock-Johnson tests of Achievement III-Visual Matching. Intermediary calculation accuracy (ACC) is Addition Strategy Assessment. Intermediary calculation fluency (FLU) is Second-Grade Calculations Battery. Later word-problem solving skill (WP) is Iowa Test of Basic Skill-Level 10-Math. Later pre-algebraic knowledge (ALG) is Test of Pre-Algebraic Knowledge.
Direct Effects of Foundational Skills and Processes to Intermediary Skills and Later Higher-Level Mathematics Outcomes (N = 962)
Note. BCBS CI is bias-corrected bootstrap confidence interval; intervals not including 0 signify the path is significantly different from 0 at α = .05. β is standardized path. Underlined estimates indicate that paths were tested for equality between the outcomes; different letters within a row within an intermediary skill/outcome indicate significant differences between direct effects. Intermediary calculation accuracy is Addition Strategy Assessment. Intermediary calculation fluency is Second-Grade Calculations Battery. Later word-problem solving skill is Iowa Test of Basic Skill-Level 10-Math. Later pre-algebraic knowledge is Test of Pre-Algebraic Knowledge. Calculation skill is Wide Range Achievement Test-Arithmetic. Number understanding is Number Line Estimation (reverse scored). Reasoning is Wechsler Abbreviated Scale of Intelligence-Matrix Reasoning. Language comprehension is Woodcock Diagnostic Reading Battery-Listening Comprehension. Working memory is Working Memory Test Battery for Children-Listening Recall. Attentive behavior is Strengths and Weaknesses of ADHD Symptoms and Normal Behavior Rating Scales. Processing speed is Woodcock-Johnson Tests of Achievement III-Visual Matching.
Specific Indirect Effects of Foundational Skills through Intermediary Calculation Skills on Later Higher-Level Math Outcomes (N = 962)
Note. Three decimal places are reported for indirect effects to help readers distinguish among such small values. BCBS CI is bias-corrected bootstrap confidence interval; intervals not including 0 signify the path is significantly different from 0 at α = .05. β is standardized path. Intermediary Calculation accuracy is Addition Strategy Assessment. Later word-problem solving skill is Iowa Test of Basic Skill-Level 10-Math. Later pre-algebraic knowledge is Test of Pre-Algebraic Knowledge. Calculation skill is Wide Range Achievement Test-Arithmetic. Number understanding is Number Line Estimation (reverse scored). Reasoning is Wechsler Abbreviated Scale of Intelligence-Matrix Reasoning. Language comprehension is Woodcock Diagnostic Reading Battery-Listening Comprehension. Working memory is Working Memory Test Battery for Children-Listening Recall. Attentive behavior is Strengths and Weaknesses of ADHD Symptoms and Normal Behavior Rating Scales. Processing speed is Woodcock-Johnson Tests of Achievement III-Visual Matching. Intermediary calculation fluency is end of grade 2 Second-Grade Calculations Battery.
Total Effects of Foundational Skills and Processes on Later Higher-Level Math Outcomes (N = 962)
Note. BCBS CI is bias-corrected bootstrap confidence interval; intervals not including 0 signify the effect is significantly different from 0 at α = .05. β is standardized effect. Underlined estimates indicate that effects were tested for equality between the outcomes; different letters within a row within an intermediary skill/outcome indicate significant differences between total effects. Later word-problem solving skill is Iowa Test of Basic Skill-Level 10-Math. Later pre-algebraic knowledge is Test of Pre-Algebraic Knowledge. Calculation skill is Wide Range Achievement Test-Arithmetic. Number understanding is Number Line Estimation (reverse scored). Reasoning is Wechsler Abbreviated Scale of Intelligence-Matrix Reasoning. Language comprehension is Woodcock Diagnostic Reading Battery-Listening Comprehension. Working memory is Working Memory Test Battery for Children-Listening Recall. Attentive behavior is Strengths and Weaknesses of ADHD Symptoms and Normal Behavior Rating Scales. Processing speed is Woodcock-Johnson Tests of Achievement III-Visual Matching.
To assist readers in interpreting the magnitude of path values, we explain the direct and one indirect effect of foundational number understanding on the word-problem outcome. The standardized path value (β) for the direct effect indicates that, while controlling for other important mathematical skills and cognitive processes, every increase in foundational number understanding of one standard deviation ( SD ) is associated with a .15 SD higher performance in later word-problem solving skill. The significant indirect effect for number understanding on the word-problem outcome via intermediary calculation fluency is the product of paths a and b , in which every SD increase in number understanding is associated with a .07 SD higher performance in intermediary calculation fluency (path a ) and every SD increase in intermediary calculation fluency is associated with a .12 SD (path b) higher performance in fourth-grade word-problem solving, controlling for the other variables in the model. The product of the a and b paths produces an indirect effect of .01 (.07*.12), which means that end-of-fourth-grade word-problem solving is expected to change by .01 SD indirectly, through fluency, per one SD change in number understanding.
Together, this direct and indirect effect suggests that (a) stronger number understanding is directly associated with performance on intermediary calculation fluency and later word-problem skill and (b) early number understanding’s effect on later word-problem is further enhanced via stronger intermediary calculation fluency. Across the direct and two indirect effects, the total unique effect for number understanding on the word-problem solving outcome is .16 (.15 for the direct effect + .01 for the indirect effect via intermediary calculation accuracy + .01 for the indirect effect via intermediary calculation fluency; the apparent discrepancy in total and summed effects are due to rounding).
Direct Effects of Foundational Skills/Processes on Intermediary Skills ( a Paths) and Direct Effects of Intermediary Skills on the Outcomes ( b Paths)
We describe direct paths from foundational processes to the two forms of intermediary calculation competence and direct paths from the two forms of intermediary calculation competence on the two fourth-grade outcomes, because these effects respectively represent the a and b paths for this study’s indirect effects. The direct paths from foundational processes to intermediary calculation accuracy were all significant. For foundational calculation skill, the β of .14 indicates that every increase in foundational calculation skill of one SD , is associated with a .14 SD higher performance in intermediary calculation accuracy, when controlling for other foundational processes. For both working memory and attentive behavior, a one SD increase is associated with a .12 SD higher score in accuracy; for every SD increase in each of the following, number understanding, reasoning, and processing speed, the corresponding performance in accuracy is .08 SD higher. The direct effect of language comprehension on intermediary calculation accuracy was negative. This was unexpected theoretically and statistically, given positive zero-order correlations, and likely represents a suppressor effect. Both direct paths from intermediary calculation accuracy ( b paths) to outcomes were significant, with a standardized effect on word-problem solving of .07 and an effect on pre-algebraic knowledge of .10, controlling for calculation fluency, all other foundational processes, and initial word-problem skill.
Direct paths to intermediary calculation fluency were significant for each foundational process except language comprehension and working memory. Every increase in foundational calculation skill of one SD is associated with a .32 SD higher score in intermediary calculation fluency. For attentive behavior, a one SD increase is associated with a .15 SD higher score in fluency; for processing speed, a .09 SD higher score; and for number understanding and reasoning, a .07 SD higher score. Both direct paths from intermediary calculation fluency ( b paths) to outcomes were significant, with an effect on word-problem solving of .12, and an effect on pre-algebraic knowledge of .07.
Pathways to Word-Problem Solving
Five foundational processes (number understanding, reasoning, language comprehension, working memory, attentive behavior) had significant direct paths to the word-problem outcome, with respective standardized path values of .15, .09, .15, .06, and .15. (The autoregressive effect of foundational word-problem solving skill, not shown in Table 2 , also accounted for unique variance with a standardized path value of .31.) Direct effects for foundational calculation skill and processing speed were not significant. Indirect effects via intermediary calculation accuracy were significant for all, each with 95% BCBS CIs not including 0. However, the language comprehension indirect effect, with its negative value, likely represents a suppressor effect. Indirect effects via intermediary calculation fluency were significant for foundational calculation skill, number understanding, reasoning, attentive behavior, and processing speed but not for language comprehension or working memory. Total effects were significant for calculations (.09), number understanding (.16), reasoning (.10), language comprehension (.14), working memory (.07), attentive behavior (.17), and processing speed (.05).
Pathways to Pre-Algebraic Knowledge
Six foundational processes had significant direct paths to the pre-algebraic knowledge outcome: calculations (.10), number understanding (.13), reasoning (.09), working memory (.07), attentive behavior (.17), and processing speed (.08). (The autoregressive effect of foundational word-problem solving skill, not shown in Table 2 , also accounted for unique variance with a standardized path value of .24.) The direct effect of language comprehension was not significant. Indirect effects via intermediary calculation accuracy were significant for all, each with a path coefficient of .01 except for language comprehension, with a −.01 value likely representing a suppressor effect. Indirect effects via intermediary calculation fluency were significant for foundational calculations (.02), number understanding (.01), reasoning (.01), attentive behavior (.01), and processing speed (.01), not for language comprehension or working memory. Total effects were significant for calculation skill (.14), number understanding (.14), reasoning (.10), working memory (.08), attentive behavior (.19), and processing speed (.10), but not language comprehension.
Tests of Equality Constraints
Underlined estimates in Table 2 indicate that direct paths were tested for equality between the two outcomes for calculation skill, language comprehension, and processing speed. No estimates in Table 3 are underlined because none of the indirect paths through accuracy or through fluency differed in statistical significance status between the two outcomes. Underlined estimates in Table 4 indicate that the total effect for language comprehension was tested for equality between the two outcomes. The 95% BCBS CI for the difference between standardized direct effects from language comprehension to the two outcomes revealed that the two were significantly different, CI [.040, .156], as were the total effects for language comprehension, CI [.041, .158]. By contrast, neither the direct effects from calculation to the two outcomes nor processing speed to the two outcomes were significantly different, [−.120, .003] and [−.100, .011], respectively, controlling for the other foundational and intermediary variables in the model.
Within-Sample Robustness Checks
As outlined by Duncan et al. (2014) , we also conducted within-sample robustness checks on our key findings: Pathways to later word-problem and pre-algebra competence differ in terms of language comprehension direct effects and language comprehension total effects. One robustness check examined the reliability of results; the other addressed the generalizability of results. To examine reliability, we created two subsamples consisting of odd-numbered observations and even-numbered observations. In separate odd-even models, 95% BCBS CIs for the difference in standardized effects corroborated both findings in both subsamples. Language comprehension direct effects differed between the two outcomes, with respective CIs of [.022, .182] and [.026, .193]. Language comprehension total effects differed between the two outcomes, with respective CIs of [.022, .181] and [.026, .193].
To examine generalizability, we analyzed two subgroups in our sample, children with economic disadvantage and economic advantage, operationalized with a dichotomous variable distinguishing between students who qualified for federal lunch subsidy ( n = 800 for the subsample qualifying for subsidized lunch; n = 161 for the subsample that did not qualify; this variable was missing for one student). In the economic disadvantage sample, the difference in the language comprehension direct effect and the language comprehension total effect for algebra versus word problems was corroborated. Respective CIs were [.032, .161] and [.033, .161]. Direct effect path coefficients were .15 for the word-problem outcome versus .06 for the pre-algebra outcome; total effect path coefficients were .15 for the word-problem outcome versus .05 for the pre-algebra outcome. (Mean performance on the language comprehension predictor, the word-problem outcome, and the pre-algebra outcome, respectively, were 16.11 [ SD = 4.54; range: 1-32], 15.54 [ SD = 5.17; range: 3-29], and 18.87 [ SD = 6.62; range 2-31].)
By contrast, in the economic advantage sample, the direct and total effects for language comprehension were not significantly different for pre-algebra versus word problems. Respective CIs were [−.128, .171] and [−.114, .1810]. Direct effect path coefficients were .18 for the word-problem outcome and .17 for the pre-algebra outcome; total effect path coefficients were .17 for the word-problem outcome and .14 for the pre-algebra outcome. (Mean performance on the language comprehension predictor, the word-problem outcome, and the pre-algebra outcome, respectively, were 20.32 [ SD = 3.84; range = 5-27], 18.63 [ SD = 5.45; range = 6-29], and 21.78 [ SD = 7.05; range = 5-31].)
The purpose of this study was to examine child-level pathways in the development of pre-algebraic knowledge versus word-problem solving, while evaluating intermediary calculation accuracy and fluency as potential mediators of foundational mathematics skills and cognitive processes. We identified important similarities along with a major distinction in the role of foundational mathematics and cognitive processes on these forms of higher-level mathematics. In this discussion, we address similarities before the distinction. Then we draw overall conclusions and note study limitations.
Similarities in Pathways to the Two Outcomes
Results support two broad conclusions about similarities in the pathways. First, both types of fourth-grade outcomes depend on a combination of general cognitive processes and early domain-specific skill, as has been documented in the individual differences literature for mathematics ( Baily, Watts, Littlefield, & Geary, 2014 ; DeStephano & LeFevre, 2004 ; Fuchs et al., 2012 ; Fuchs et al., 2010 ; Geary, 2011 ). Second, for both outcomes, the effect of early mathematics competencies on later achievement is substantial. Every increase in initial math competence of one unit (across foundational calculations and understanding about number) was associated with an increase of .25 SD unit in later word-problem solving and an increase of .28 SD unit in later pre-algebraic knowledge, even when controlling for the other foundational processes and intermediary calculation variables in the model. (These estimates do not take into account the contribution of foundational word-problem solving skill. Also note that we use standardized path values to interpret the magnitude of effects.)
This corroborates previous work showing that mathematics achievement trajectories are established early ( Baily et al., 2014 ; Duncan et al., 2007; Fuchs, Geary, Fuchs, Compton, & Hamlett, 2016 ). It extends the literature by contrasting two specific forms of higher-order mathematics while controlling for a variety of cognitive processes, thereby demonstrating the robust importance of foundational mathematics performance for later development. Moreover, we documented that the effects of intermediary calculation skill (accuracy and fluency) are substantial for both outcomes even after accounting for prior cognitive processes and foundational calculation skill and understanding about number: .19 in predicting the word-problem solving outcome and .17 in predicting the pre-algebra outcome.
Findings also support conclusions about five more specific similarities across the two fourth-grade outcomes. First, the correlation between fourth-grade pre-algebraic thinking and word-problem solving was .71. We expected a significant relation based on prior work showing connections between the domains. In a randomized control trial ( Fuchs et al., 2014 ), word-problem instruction (that did not include instruction on calculations) improved pre-algebra performance. Also, relational understanding of the equal sign, reflected in measures of pre-algebraic knowledge, has been associated with the ability to use algebraic notation to represent word problems ( Powell & Fuchs, 2010 ). And word-problem solving, which reflects understanding of relationships between known and unknown quantities, may be supported by pre-algebraic thinking. Despite these earlier findings suggesting a connection, the correlation of .71 is noteworthy.
The second more specific similarity in the child-level variables that support development of competence in the two domains concerns the roles of intermediary calculation accuracy versus fluency. This issue is important for practice because disagreement among mathematics educators focuses on whether calculation accuracy is sufficient or whether calculation fluency provides added value (e.g., Greene, 2010 ; Warner, 2006 ). A large literature demonstrates a role for one or the other, but we identified only one prior study that included both variables in the same models to estimate the contribution of one while controlling for the effects of the other. Carr and Alexeev (2011) concluded that both dimensions of second-grade calculation skill contribute to fourth-grade performance on a general mathematics achievement test. Our findings provide corroborating data.
At the same time, the present study extends Carr and Alexeev (2011) by demonstrating that fluency and accuracy affect two specific types higher-order mathematics performance in comparable ways (direct paths of .17 and .19), by controlling for early number knowledge and general cognitive processes, and by indexing calculation fluency later when it may provide finer discriminations among children. Finding that both dimensions of calculation competence, accuracy and fluency, independently contribute to success with two higher-order outcomes, word problems and pre-algebra, indicates that mathematics instruction in schools should be designed to ensure accurate calculation performance as well as fluent execution of procedures. This makes sense given that fluency on lower-level skill frees attentional and working memory resources to permit students to focus on the cognitive complexities of higher-order performance.
The remaining salient similarities in pathways to the two forms of fourth-grade higher-order mathematics performance involve four cognitive processes. Reasoning, working memory, attentive behavior, and processing speed each exerted a comparable direct effect on both outcomes (.07 and .09 for reasoning; .06 and .07 for working memory; .15 and .17 for attentive behavior; .04 and .09 for processing speed), and indirect effects also accrued for reasoning, attentive behavior, and processing speed via both dimensions of intermediary calculation skill on both outcomes.
A shared role for reasoning reflects the challenging cognitive demands associated with fourth-grade pre-algebra ( Fuchs et al., 2012 ) and word-problem solving (Fuchs, Malone, et al., in press). Individual differences in reasoning ability may be further heightened by the difficulty many elementary-grade teachers have in formulating conceptually rich instruction in these domains. Given the number of unique school and classroom sequences students experienced as they progressed through grades 2-4, it was not possible in the present study to capture individual student instruction or control for this source of variance. However, prior work (e.g., Baroody & Ginsberg, 1983 ; Fuchs, Malone, et al., in press ; McNeil & Alibali, 2005 ; Powell, 2012 ) suggests that teachers experience difficulty in providing the kinds of instructional support needed to reduce the role of reasoning ability in these higher-order forms of mathematics.
Attentive behavior, working memory, and processing speed as sources of individual differences in both outcomes also make sense, even though Fuchs et al. (2012) found only indirect effects for attentive behavior and processing speed on pre-algebraic knowledge at third grade and no significant effects for working memory. Identifying and testing number replacements for variables, handling operations on both sides of the equal sign, and building problem models while deciphering text for word-problem solving are transparently demanding of working memory and attentional resources, while processing speed may support working memory and attention resources. So we note that a key distinction between the present study and Fuchs et al. (2012) is that we assessed outcomes later, at end of fourth instead of third grade.
Major Distinctions in Pathways to the Two Outcomes
Despite these important similarities, we observed major distinctions in the child-level variables that support development of competence in the two domains. That is, the direct and total effects of language comprehension on fourth-grade word problems were, respectively, .15 and .14 (both significant). By contrast, respective coefficients in predicting fourth-grade pre-algebra were .05 and .04 (each nonsignificant), and the test of equality between the direct paths and between the totals paths indicated a stronger role for language comprehension on word-problem solving than on pre-algebra knowledge. Further, our robustness reliability check, conducted on odd versus even cases, provided cross-validation for a distinctive role for language comprehension on word-problems versus pre-algebra.
These finding suggest that word-problem performance with its symbolic complexity (involving language and the representation of quantity) taps a greater variety of cognitive systems than the pre-algebra performance expected at end of fourth grade. This makes sense given the transparent need for language comprehension in word-problem solving. Even so, language comprehension is also required for processing school instruction. This finding, in which language comprehension exerted an effect on word-problems but not pre-algebra, suggests that it operates primarily via the former, not the latter process, at least at fourth grade in these two specific mathematics domains for an urban population of dominantly economic disadvantage.
Finding that language comprehension affects later word-problem solving more than later pre-algebraic knowledge in this population also has instructional implications. On the one hand, results suggest the importance of infusing arithmetic instruction with an emphasis on unknowns, variables, and relational understanding of the equal sign. This is in line with Pillay et al.’s (1998) model that poses a connection between arithmetic and algebra via pre-algebraic understanding of the relational meaning of the equal sign and understanding about variables. On the other hand, findings suggest the potential value of ensuring that word-problem instruction addresses the needs of students who experience limitations in language comprehension.
Even so, despite that the within-sample robustness reliability check bolsters confidence in the conclusion that foundational language comprehension plays a greater role in later word-problem solving that in later pre-algebraic knowledge, caution is in order. This is because the robustness generalizability check indicates a different pattern of effects for children of economic advantage versus disadvantage. With economic disadvantage, analyses corroborated a greater role for language comprehension in later word-problem solving than in later pre-algebra. However, with economic advantage, language comprehension was comparably active in supporting later performance in both higher-order mathematics domains.
Readers might wonder if the lack of statistically significant differences for language comprehension on word-problem solving versus pre-algebra in the subsample of 161 students of economic advantage is due to inadequate statistical power (the economic disadvantage subsample numbered 800). Yet, path coefficients associated with the economic advantage subsample indicate otherwise: The direct effect of language comprehension on later word problem solving was .18, while the direct effect of language comprehension on pre-algebra was .17; respective total effects of language comprehension on pre-algebra were .17 and .14. (In the economic disadvantage subsample, the direct effect of language comprehension on later word problem solving was .15, while the direct effect of language comprehension on pre-algebra was .06; respective total effects of language comprehension on pre-algebra were .15 and .05.)
A more plausible explanation for the differential pattern of effects for language comprehension as a function of fourth-grade mathematics outcome for these two populations resides with the stronger language comprehension performance of the economic advantage group. The effect size distinguishing the groups at start of second grade approached 1 SD unit. The association between economic status and language development is well documented (e.g., Betancourt, Brodsky, & Hurt, 2015 ; Fernald, Marchman, & Weisleder, 2013 ; Walker, Greenwood, Hart, & Carta, 1994 ). The present study extends this literature by demonstrating that the increased language capacity economic advantage affords may alter its foundational relation with later, higher-order mathematics performance. Here, second-grade language comprehension appears to play a stronger supporting role in later pre-algebra for children with versus without economic advantage.
We already discussed two ways in which language comprehension may support later mathematics performance: its transparent requirement for solving word problems, and teachers’ heavily reliance on language-mediated explanations of math ideas and procedures. A third way is via the inner speech ( Zakin, 2007 ) and self-explaining ( Chi & Van Lehn, 1991 ; Siegler, 2002 ) children may use to decipher and navigate complex problem-solving processes. This includes identifying and executing strategies for solving nonstandard equations that are rarely addressed in school curriculum. Such reliance, however, requires a level of verbal facility ( Rittle-Johnson, 2006 ). So the economic advantage group, with a higher level of overall performance across this group’s span of individual differences, may have provided the basis for a stronger connection between foundational language comprehension and later pre-algebra, via reliance on inner speech and self-explaining. Research on this potential explanation is warranted. More generally, the failure of effects to generalize to the smaller subsample of students who did not qualify for the subsidy indicates the need for additional research examining how and why the role of language comprehension on pre-algebra differs for children of economic advantage and disadvantage.
Conclusions and Limitations
As reflected in the influence of developmental studies that focus on general mathematics achievement tests ( Duncan, Dowsett, Classens, et al., 2008 ; Halberda, Mazzzocco, & Feigenson, 2008 ; Krajewski & Schneider, 2008), a frequent assumption is that the pathways to higher-order mathematics competence are shared across the various strands of performance. This would make sense for the development of word-problem solving and pre-algebraic knowledge, given the potential connections and the empirical findings already discussed for these two domains. Moreover, we found more sources of similarity than difference in the pathways, which supports an overall conclusion that the pathways are more alike than different.
Even so, our results suggest the need for more refined analysis. Despite many important similarities, we did identify a major distinction between the pathways to fourth-grade pre-algebraic versus word-problem performance. That is, language comprehension (at the start of second grade) is more critical to the development of later word-problem solving than for later pre-algebraic knowledge. This finding suggests the need to adjust instruction on word problems and pre-algebra in ways that address individual student profiles of cognitive resources. It also suggests the importance of additional research to explore the similarities and distinctions in pathways for other specific mathematics domains at other grades.
These conclusions need to be understood within the constraints of four study limitations. First, although our predictors captured a substantial portion of the variance in the two outcomes, figures that are similar to those reported in previous individual difference studies in mathematics, 52% of the variance remained unexplained for the pre-algebra outcome and 43% remained unexplained for the word-problem outcome. Considering additional cognitive processes, such as other forms of working memory (inhibition and updating; Miyake & Shah, 1999 ), 3-D spatial visualization ( Tolar et al., 2009 ), and analogical or inferential reasoning (e.g., Holyoak & Thagard, 1997 ), may produce insights into additional processes that support the transition from early to higher-order forms of mathematical competence. Second, our measure of pre-algebraic knowledge assessed solving unknowns in nonstandard equations and identifying unknowns in function tables. Other methods for operationalizing pre-algebra may produce different results. Future work should contrast strategies. Third, we measured each construct with a particular measure. Including multiple measures to permit use of latent constructs is preferable, and this should be pursued. Fourth, conclusions about causality should be avoided because, although predictive relations were examined, our methods were correlational.
Finally, we note again that the main distinctions in the language comprehension pathways to pre-algebra versus word-problem solving, although internally reliable, appear to generalize only to children of economic disadvantage. This underscores the need to conduct generalizability checks, as suggested by Duncan et al. (2008) , when examining the effects of foundational skill and cognitive processes on later academic outcomes.
Acknowledgments
This research was supported by Award Number R01 HD053714, R24 HD075443, and Core Grant #HD15052 from the Eunice Kennedy Shriver National Institute of Child Health & Human Development to Vanderbilt University and from Grant R305A110067 from the Institute of Education Sciences in the U.S. Department of Education to the University of Houston. The content is solely the responsibility of the authors and does not necessarily represent the official views of the Eunice Kennedy Shriver National Institute of Child Health & Human Development, the National Institutes of Health, the Institute of Education Sciences, or the U.S. Department of Education.
1 As a function of the review process, we tested separately the equality of all effects of interest: 7 direct effects from foundational skills to higher-level outcomes, 14 indirect effects from foundational skills through intermediary skills to higher-level outcomes, and 7 total effects from foundational skills to higher-level outcomes. The pattern of results was the same. Direct effects of language comprehension as well as the total effect of language comprehension distinguished between the two outcomes.
- Alibali MW, Knuth EJ, Hattikudur S, McNeil NM, Stephens AC. A longitudinal examination of middle school students’ understanding of the equal sign and equivalent equations. Mathematical Thinking and Learning. 2007; 9 :221–246. doi:10.1080/10986060701360902. [ Google Scholar ]
- Arnett AB, Pennington BF, Friend A, Willcutt EG, Byrne B, Samuelsson S, Olson RK. The SWAN captures variance at the negative and positive ends of the ADHD symptom dimension. Journal of Attention Disorders. 2013; 17 :152–62. [ PMC free article ] [ PubMed ] [ Google Scholar ]
- Bailey DH, Watts, Littlefield AK, Geary DC. State and trait effects on individual differences in children’s mathematical development. Psychological Science. 2014; 25 :2017–2026. [ PMC free article ] [ PubMed ] [ Google Scholar ]
- Baroody AJ, Ginsburg HP. The effects of instruction on children’s understanding of the “equals” sign. Elementary School Journal. 1983; 84 :199–212. doi:10.1086/46135. [ Google Scholar ]
- Betancourt LM, Brodsky NL, Hurt H. Socioeconomic (SES) differences in language are evident in female infants at 7%months of age. Early Human Development. 2015; 91 :719–724. http://dx.doi.org/10.1016/j.earlhumdev.2015.08.002 . [ PubMed ] [ Google Scholar ]
- Blanton M, Kaput J. In: Chick H, Stacey K, Vincent J, Vincent J, editors. Algebraifying the elementary mathematics experience. Part II: Transforming practice on a district wide scale; The future of the teaching and learning of algebra Proceedings of the 12th ICMI Study Conference; University of Melbourne, Australia. 2001. pp. 87–95. [ Google Scholar ]
- Bynner J. Basic skills in adolescents’ occupational preparation. Career Development Quarterly. 1997; 45 :305–321. [ Google Scholar ]
- Carr M, Aleexev N. Fluency, accuracy, and gender predict developmental trajectories of arithmetic strategies. Journal of Educational Psychology. 2011; 103 :617–631. doi: 10.1037/a00238604. [ Google Scholar ]
- Carraher DW, Schliemann AD. Moshkovich J, Brenner M, editors. Is everyday mathematics truly relevant to mathematics education? Everyday mathematics. Monographs of the Journal for Research in Mathematics Education. 2002; 11 :131–153. doi:10.2307/749968. [ Google Scholar ]
- Chi MTH, Van Lehn KA. The content of physics self-explanations. Journal of the Learning Sciences. 1991; 1 :69–105. doi:10.1207/s15327809jls0101_4. [ Google Scholar ]
- DeStephano D, LeFevre J-A. The role of working memory in mental arithmetic. European Journal of Cognitive Psychology. 2004; 16 :353–386. [ Google Scholar ]
- Duncan GJ, Dowsett CJ, Classens A, et al. School readiness and later achievement. Developmental Psychology. 2008; 43 :1428–1446. [ PubMed ] [ Google Scholar ]
- Duncan GJ, Engel M, Claessens A, Dowsett CJ. Replication and robustness in developmental research. Developmental Psychology. 2014; 50 :2417–2425. doi: 10.1037/a0037996. [ PubMed ] [ Google Scholar ]
- Every Child a Chance Trust The long-term costs of numeracy difficulties. 2009 Retrieved. August 14, 2009, http://www.everychildachancetrust.org/counts/index.cfm .
- Falkner KP, Levi L, Carpenter TP. Children’s understanding of equality: A foundation for algebra. Teaching Children Mathematics. 1999; 6 :232–236. [ Google Scholar ]
- Fernald A, Marchman A, Weisleder A. SES differences in language processing skill and vocabulary are evident at 18 months. Developmental Science. 2103; 16 :234–248. doi: 10.1111/desc.12019. [ PMC free article ] [ PubMed ] [ Google Scholar ]
- Fuchs LS, Compton DL, Fuchs D, Powell SR, Schumacher RF, Hamlett CL, Namkung JM, Vukovic RK. Contributions of domain-general cognitive resources and different forms of arithmetic development to pre-algebraic knowledge. Developmental Psychology. 2012; 48 :1315–1326. doi: 10.1037/a0027475. [ PMC free article ] [ PubMed ] [ Google Scholar ]
- Fuchs LS, Fuchs D, Compton DL, Powell SR, Seethaler PM, Capizzi AM, Schatschneider C, Fletcher JM. The cognitive correlates of third-grade skill in arithmetic, algorithmic computation, and arithmetic word problems. Journal of Educational Psychology. 2006; 98 :29–43. doi:10.1037/0022-0663.98.1.29. [ Google Scholar ]
- Fuchs LS, Fuchs D, Stuebing K, Fletcher JM, Hamlett CL, Lambert WE. Problem-solving and computation skills: Are they shared or distinct aspects of mathematical cognition? Journal of Educational Psychology. 2008; 100 :30–47. doi:10.1037/0022-0663.100.1.30. [ PMC free article ] [ PubMed ] [ Google Scholar ]
- Fuchs LS, Geary DC, Compton DL, Fuchs D, Hamlett CL, Seethaler PM, Bryant JV, Schatschneider C. Do different types of school mathematics development depend on different constellations of numerical and general cognitive abilities? Developmental Psychology. 2010; 46 :1731–1746. doi:10.1037/a0020662. [ PMC free article ] [ PubMed ] [ Google Scholar ]
- Fuchs LS, Geary DL, Fuchs D, Compton DL, Hamlett CL. Pathways to third-grade calculation versus word-reading competence: Are they more alike or different? Child Development. 2016; 87 :558–567. DOI: 10.1111/cdev.12474. [ PMC free article ] [ PubMed ] [ Google Scholar ]
- Fuchs LS, Hamlett CL, Powell SR. In: Computational Battery. Fuchs LS, editor. 228 Peabody, Vanderbilt University, Nashville, TN 37203: 2003. [ Google Scholar ]
- Fuchs LS, Malone A, Schumacher RF, Namkung J, Hamlett CL, Jordan NC, Siegler RS, Gersten R, Changas P. Supported self-explaining during fraction intervention. Journal of Educational Psychology. in press. [ Google Scholar ]
- Fuchs LS, Powell SR, Cirino PT, Schumacher RF, Marrin S, Hamlett CL, Fuchs D, Compton DL, Changas PC. Does calculation or word-problem instruction provide a stronger route to pre-algebraic knowledge? Journal of Educational Psychology. 2014; 106 :990–1006. [ PMC free article ] [ PubMed ] [ Google Scholar ]
- Fuchs LS, Seethaler PM, Powell SR. In: Test of Emerging Algebraic Cognition. Fuchs LS, editor. 228 Peabody, Vanderbilt University, Nashville, TN 37203: 2009. [ Google Scholar ]
- Geary DC. Cognitive predictors of achievement growth in mathematics: A 5-year longitudinal study. Developmental Psychology. 2011; 47 :1539–1552. doi:10.137/a0025510. [ PMC free article ] [ PubMed ] [ Google Scholar ]
- Geary DC, Boykin AW, Embretson S, Reyna V, Siegler R, Berch DB, Graban J. The Final Report of the National Mathematics Advisory Panel. U.S. Department of Education; Washington, DC: 2008. Report of the task group on learning processes. [ Google Scholar ]
- Geary DC, Hoard MK, Byrd-Craven J, DeSoto CM. Strategy choices in simple and complex addition: Contributions of working memory and counting knowledge for children with mathematical disability. Journal of Experimental Child Psychology. 2004; 88 :121–151. [ PubMed ] [ Google Scholar ]
- Greene JP. Drill and kill kerfuffle. 2010 Education Next ( http://educationnext.org/drill-and-kill-kerfuffle/ )
- Halberda J, Mazzocco MMM, Feigenson L. Individual differences in non-verbal number acuity correlate with maths achievement. Nature. 2008; 445 :665–668. [ PubMed ] [ Google Scholar ]
- Holyoak KJ, Thagard P. The analogical mind. American Psychologist. 1997; 52 :35–44. doi:10.1037/0003-066X.52.1.35. [ PubMed ] [ Google Scholar ]
- Hoover HD, Hieronymous AN, Dunbar SB, Frisbie DA. Iowa Test of Basic Skills, Form K. Riverside; Itasca, IL: 1993. [ Google Scholar ]
- Jordan NC, Hanich L. Mathematical thinking in second-grade children with different forms of LD. Journal of Learning Disabilities. 2000; 33 :567–578. doi:10.1177/002221940003300605. [ PubMed ] [ Google Scholar ]
- Kieran C. Cognitive processes involved in learning school algebra. In: Nesher P, Kilpatrick J, editors. Mathematics and cognition: a research synthesis by the International Group for the Psychology of Mathematics Education. Cambridge University Press; New York: 1990. pp. 96–112. doi:10.1017/CBO9781139013499.007. [ Google Scholar ]
- Kim YS, Wagner RK, Foster E. Relations among oral reading fluency, silent reading fluency, and reading comprehension: A latent variable study of first-grade readers. Scientific Studies of Reading. 2011; 15 :338–352. doi: 10.1080/10888438.2010.493964. [ PMC free article ] [ PubMed ] [ Google Scholar ]
- Kline RB. Principles and practice of structural equation modeling. 3rd Guilford; New York: 2011. [ Google Scholar ]
- Knuth EJ, Stephens AC, McNeil NM, Alibali MW. Does understanding the equal sign matter? Evidence from solving equations. Journal for Research in Mathematics Education. 2006; 37 :297–312. [ Google Scholar ]
- Krajewski K, Schneider W. Early development of quantity to number-word linkage as a precursor of mathematical school achievement and mathematical difficulties: Findings from a four-year longitudinal study. Learning and Instruction. 2009; 19 :513–526. [ Google Scholar ]
- Lakes KD, Swanson JM, Riggs M. The reliability and validity of the English and Spanish Strengths and Weaknesses of ADHD and Normal behavior rating scales in a preschool sample: continuum measures of hyperactivity and inattention. Journal of Attention Disorders. 2012; 16 :510–516. [ PMC free article ] [ PubMed ] [ Google Scholar ]
- Lee K, Ng SF, Bull R, Pe ML, Ho RHM. Are patterns important? An investigation of the relationships between proficiency in patterns, computation, executive functioning, and algebraic word problems. Journal of Educational Psychology. 2011; 103 :269–281. doi:10.1037/a0023068. [ Google Scholar ]
- McNeil NM, Alibali MW. Why won’t you change your mind? Knowledge of operational patterns hinders learning and performance on equations. Child Development. 2005; 76 :883–899. doi:10.1111/j.1467-8624.2005.00884.x. [ PubMed ] [ Google Scholar ]
- Miyake A, Shah P. Models of working memory: Mechanisms of active maintenance and executive control. Cambridge University Press; Cambridge, UK: 1999. [ Google Scholar ]
- National Mathematics Advisory Panel . Final report. U.S. Department of Education; Washington, DC: 2008. [ Google Scholar ]
- Muthén LK, Muthén BO. Mplus User’s Guide. Seventh Muthén & Muthén; Los Angeles, CA: 1998-2012. [ Google Scholar ]
- Pickering S, Gathercole S. Working Memory Test Battery for Children. The Psychological Corporation; London: 2001. [ Google Scholar ]
- Pillay H, Wilss L, Boulton-Lewis G. Sequential development of algebra knowledge: A cognitive analysis. Mathematics Education Research Journal. 1998; 10 (2):87–102. doi:10.1007/BF03217344. [ Google Scholar ]
- Powell SR. Equations and the equal sign in elementary mathematics textbooks. Elementary School Journal. 2012; 112 :627–648. doi:10.1086/665009. [ PMC free article ] [ PubMed ] [ Google Scholar ]
- Powell SR, Fuchs LS. Contribution of equal-sign instruction beyond word-problem tutoring for third-grade students with mathematics difficulty. Journal of Educational Psychology. 2010; 102 :381–394. doi:10.1037/a0018447. [ PMC free article ] [ PubMed ] [ Google Scholar ]
- RAND Mathematics Study Panel . Mathematical proficiency for all students: Toward a strategic research and development program in mathematics education (No. 08330331X) RAND; Santa Monica, CA: 2003. [ Google Scholar ]
- Rittle-Johnson B. Promoting transfer: Effects of self-explanation and direct instruction. Child Development. 2006; 77 :1–15. doi: 10.1111/j.1467-8624.2006.00852.x. [ PubMed ] [ Google Scholar ]
- Satorra A, Bentler PM. A scaled difference chi-square test statistic for moment structure analysis. Psychometrika. 2001; 66 :507–514. [ PMC free article ] [ PubMed ] [ Google Scholar ]
- Siegler RS. Microgenetic studies of self-explanation. In: Garnott N, Parziale J, editors. Microdevelopment: A process-oriented perspective for studying development and learning. Cambridge University Press; Cambridge: 2002. pp. 31–58. [ Google Scholar ]
- Siegler RS, Booth JL. Development of numerical estimation in young children. Child Development. 2004; 75 :428–444. [ PubMed ] [ Google Scholar ]
- Swanson HL, Jerman O, Zheng X. Growth in working memory and mathematical problem solving in children at risk and not at risk for serious math difficulties. Journal of Educational Psychology. 2008; 100 :343–379. [ Google Scholar ]
- Swanson JM, Schuck S, Porter MM, Carlson C, Hartman CA, Sergeant JA, Clevenger W, Wasdell M, McCleary R, Lakes K, Wigal T. Categorical and dimensional definitions and evaluations of symptoms of ADHD: History of the SNAP and the SWAN rating scales. The International Journal of Educational and Psychological Assessment. 2012; 10 :51–69. [ PMC free article ] [ PubMed ] [ Google Scholar ]
- Tolar TD, Lederberg AR, Fletcher JM. A structural model of algebra achievement: Computational fluency and spatial visualization as mediators of the effect of working memory on algebra achievement. Educational Psychology. 2009; 29 :239–266. doi:10.1080/01443410802708903. [ Google Scholar ]
- U.S. Department of Education Digest of Education Statistics. 2008 Retrieved from http://nces.ed.gov/pubs2009/2009020.pdf .
- Walker D, Greenwood C, Hart B, Carta J. Prediction of school outcomes based on early language production and socioeconomic factors. Child Development. 1994; 65 :606–21. [ PubMed ] [ Google Scholar ]
- Warner J. The fog of ‘math wars.” The New York Times Opinionator. 2006 ( http//opinionator.blogs.nytimes.com/2006/06/01/the-fog-of-math-wars/ )
- Wechsler D. Wechsler Abbreviated Scale of Intelligence. Psychological Corporation; San Antonio, TX: 1999. [ Google Scholar ]
- Wilkinson GS. Wide Range Achievement Test 3. Wide Range; Wilmington: 1993. [ Google Scholar ]
- Woodcock RW. Woodcock Diagnostic Reading Battery. Riverside; Itasca, IL: 1997. [ Google Scholar ]
- Woodcock RW, McGrew KS, Mather N. Woodcock-Johnson III. Riverside; Itasca, IL: 2001. [ Google Scholar ]
- Zakin A. Metacognition and the use of inner speech in children’s thinking: A tool teachers can use. Journal of Education and Human Development. 2007; 1 :1–14. [ Google Scholar ]
- Progression Maps
Reasoning Skills
Developing opportunities and ensuring progression in the development of reasoning skills
Achieving the aims of the new National Curriculum:
Developing opportunities and ensuring progression in the development of reasoning skills.
The aims of the National Curriculum are to develop fluency and the ability to reason mathematically and solve problems. Reasoning is not only important in its own right but impacts on the other two aims. Reasoning about what is already known in order to work out what is unknown will improve fluency; for example if I know what 12 × 12 is, I can apply reasoning to work out 12 × 13. The ability to reason also supports the application of mathematics and an ability to solve problems set in unfamiliar contexts.
Research by Nunes (2009) identified the ability to reason mathematically as the most important factor in a pupil’s success in mathematics. It is therefore crucial that opportunities to develop mathematical reasoning skills are integrated fully into the curriculum. Such skills support deep and sustainable learning and enable pupils to make connections in mathematics.
This resource is designed to highlight opportunities and strategies that develop aspects of reasoning throughout the National Curriculum programmes of study. The intention is to offer suggestions of how to enable pupils to become more proficient at reasoning throughout all of their mathematics learning rather than just at the end of a particular unit or topic.
We take the Progression Map for each of the National Curriculum topics, and augment it with a variety of reasoning activities (shaded sections) underneath the relevant programme of study statements for each year group. The overall aim is to support progression in reasoning skills. The activities also offer the opportunity for children to demonstrate depth of understanding, and you might choose to use them for assessment purposes as well as regular classroom activities.
Place Value Reasoning
Addition and subtraction reasoning, multiplication and division reasoning, fractions reasoning, ratio and proportion reasoning, measurement reasoning, geometry - properties of shapes reasoning, geometry - position direction and movement reasoning, statistics reasoning, algebra reasoning.
The strategies embedded in the activities are easily adaptable and can be integrated into your classroom routines. They have been gathered from a range of sources including real lessons, past questions, children’s work and other classroom practice.
Strategies include:
- Spot the mistake / Which is correct?
- True or false?
- What comes next?
- Do, then explain
- Make up an example / Write more statements / Create a question / Another and another
- Possible answers / Other possibilities
- What do you notice?
- Continue the pattern
- Missing numbers / Missing symbols / Missing information/Connected calculations
- Working backwards / Use the inverse / Undoing / Unpicking
- Hard and easy questions
- What else do you know? / Use a fact
- Fact families
- Convince me / Prove it / Generalising / Explain thinking
- Make an estimate / Size of an answer
- Always, sometimes, never
- Making links / Application
- Can you find?
- What’s the same, what’s different?
- Odd one out
- Complete the pattern / Continue the pattern
- Another and another
- Testing conditions
- The answer is…
- Visualising
These strategies are a very powerful way of developing pupils’ reasoning skills and can be used flexibly. Many are transferable to different areas of mathematics and can be differentiated through the choice of different numbers and examples.
Nunes, T. (2009) Development of maths capabilities and confidence in primary school, Research Report DCSF-RR118 (PDF)
Is there anything wrong with this page?
Subscribe to our newsletter
The NCETM is led and delivered by Tribal Education Services, with MEI as a key partner. Learn more about Tribal Education Services and what they do via the link to their website in 'About the NCETM'.
About this website
Stay connected.
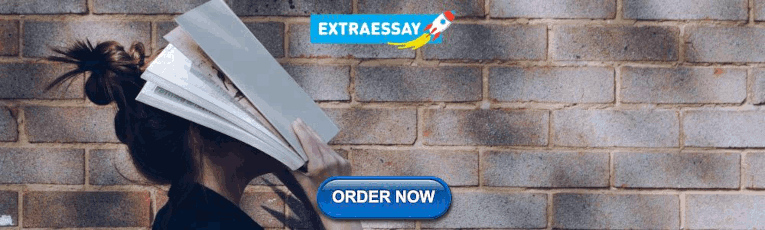
IMAGES
VIDEO
COMMENTS
In that paper he produces this pyramid: This is important for two reasons: 1) It splits up reasoning skills and problem solving into two different entities. 2) It demonstrates that fluency is not something to be rushed through to get to the 'problem solving' stage but is rather the foundation of problem solving.
Fluency, reasoning and problem solving are central strands of mathematical competency, as recognized by the National Council of Teachers of Mathematics (NCTM) and the National Research Council's report 'Adding It Up'. They are key components to the Standards of Mathematical Practice, standards that are interwoven into every mathematics ...
In summary: Mathematical fluency skills help students think faster and more clearly, giving them the energy, attention and focus to tackle complex problem-solving and reasoning questions. The future needs problem-solvers with reasoning skills. But as education shifts its focus to the critical and creative angle of mathematics problems, we can't lose sight of the abilities […]
In mathematics, fluency builds on a foundation of conceptual understanding, strategic reasoning, and problem-solving to achieve automaticity. Students connect conceptual understanding (Stage 1) with strategies and methods (Stage 2) and use the methods in a way that makes sense to them (Stage 3). When students go through these stages to build ...
Dowker (1992) The phrase 'number sense' is often used to mean conceptual fluency - understanding place value and the relationships between operations. Children need to be both procedurally and conceptually fluent - they need to know both how and why. Children who engage in a lot of practice without understanding what they are doing often forget ...
Using reasoning to support fluency and problem solving You'll probably be very familiar with the aims of the National Curriculum for mathematics in England: fluency, problem-solving and reasoning. An accepted logic of progression for these is for children to become fluent in the basics, apply this to problem-solving, and then reason about ...
Develop fluency, reasoning and problem solving within any topic as part of a mastery approach The skills of fluency, reasoning and problem solving are well-known to all primary maths teachers. In mastery teaching, they play an essential role in helping pupils to gain a deeper understanding of a topic. But what does this look like in practice?
understand this one problem. A student with procedural knowledge and fluency could generalize their conceptual knowledge to the procedure of finding the product of 0.25 x 84 = 21 to solve the problem. This procedure could be practiced to improve the efficiency of solving this common type of problem. Example 3: Understanding Area
The ultimate goal of teaching mathematics is to create thinkers. Making the most of the fluency virtuous cycle helps learners to do so much more than just recall facts and memorise procedures. In time, your learners will be able to work fluently, make connections, solve problems, and become true mathematical thinkers. References. Jo Boaler (2014).
Developing fluency, reasoning and problem solving are the three key aims of the primary maths curriculum in England (DfE, 2013). However, arithmetic fluency tends to dominate in many classrooms. This is despite research indicating that reasoning skills having a greater impact than arithmetic fluency on mathematical attainment among 7-11 year ...
In our mathematics lessons, our curriculum challenges teachers to develop fluency, reasoning and problem-solving skills, and trying to find a balance within a particular lesson can be a challenge (this is an aspect we explore more fully in this article by Clare Lee and Sue Johnston-Wilder). By choosing LTHC activities, learners can easily get ...
Developing Fluency with Addition Facts. Computational fluency, according to NCTM's Principles and Standards for School Mathematics, means more than quickly producing correct answers. It requires conceptual understanding and is exhibited through: Eficiency. Accuracy. Flexibility.
flexibility is a major goal of fluency, because a good strategy for one problem may or may not be as effective for another problem. Declarations The following declarations describe necessary actions to ensure that every student has access to and develops procedural fluency. These declarations apply to computational fluency across the K-12
How asking—and answering—"why" can improve mathematical fluency. To help students gain fluency in math, it's important to help them explore and understand why. Consider the following problem: 2/3 x 3/4. Most readers will likely be able to compute the correct answer mentally (the answer is 1/2). It is important to be able to get this ...
The Australian Curriculum: Mathematics aims to be relevant and applicable to the 21st century. The inclusion of the proficiencies of understanding, fluency, problem-solving and reasoning in the curriculum is to ensure that student learning and student independence are at the centre of the curriculum. The curriculum focuses on developing ...
work has some similarities with the one used in recent mathematics assessments by the National Assessment of Educational Progress (NAEP), which features three mathematical abilities (conceptual understanding, procedural knowledge, and problem solving) and includes additional specifications for reasoning, connections, and communication. 2 The strands also echo components of mathematics learning ...
Students' oral responses explain actions, concepts and reasons to show mathematical fluency. •. Students use high modality words as evidence of well-developed levels of fluency. •. Everyday verbs and connectives are important for observing reasoning as a fluency characteristic. •.
Build Procedural Fluency from Conceptual Understanding Teacher Actions: •Provide students with opportunities to use their own reasoning strategies and methods for solving problems. •Ask students to discuss and explain why the procedures that they are using work to solve particular problems. •Connect student-generated strategies and methods to
The aim of this study was to investigate whether a particular etude ("Expression polygons", see Section 2.2.1) is as effective as traditional exercises at developing students' procedural fluency in solving linear equations, relative to the alternative hypothesis that the etude and the exercises are not equally effective.. 3.1 Method. A quasi-experimental design was used, with pairs of ...
Children (n = 962; mean 7.60 years) were assessed on general cognitive processes and early calculation, word-problem, and number knowledge at start of grade 2; calculation accuracy and calculation fluency at end of grade 2; and pre-algebraic knowledge and word-problem solving at end of grade 4. Important similarities in pathways were identified ...
The aims of the National Curriculum are to develop fluency and the ability to reason mathematically and solve problems. Reasoning is not only important in its own right but impacts on the other two aims. Reasoning about what is already known in order to work out what is unknown will improve fluency; for example if I know what 12 × 12 is, I can ...
Standards for School Mathematics (National Council of Teachers of Mathematics, 2000). Problem solving involves students applying four processes: reasoning, communication, connections, and representation. Problem solving can also provide opportunities for students to apply content knowledge in all five mathematic domains. Problem solving
Proficiency in mathematical problem-solving is an essential talent that students must attain. The primary objective of learning mathematics is to acquire problem-solving skills, as difficulties ...
procedural fluency, AND mathematical reasoning or problem-solving skills? The Guiding Question . The Guiding Question addresses how a candidate's plans build a learning segment of three to five lessons around a central focus. Candidates will explain how they plan to organize tasks, activities, and/or materials to align with the central focus ...