- AI & NLP
- Churn & Loyalty
- Customer Experience
- Customer Journeys
- Customer Metrics
- Feedback Analysis
- Product Experience
- Product Updates
- Sentiment Analysis
- Surveys & Feedback Collection
- Try Thematic
Welcome to the community
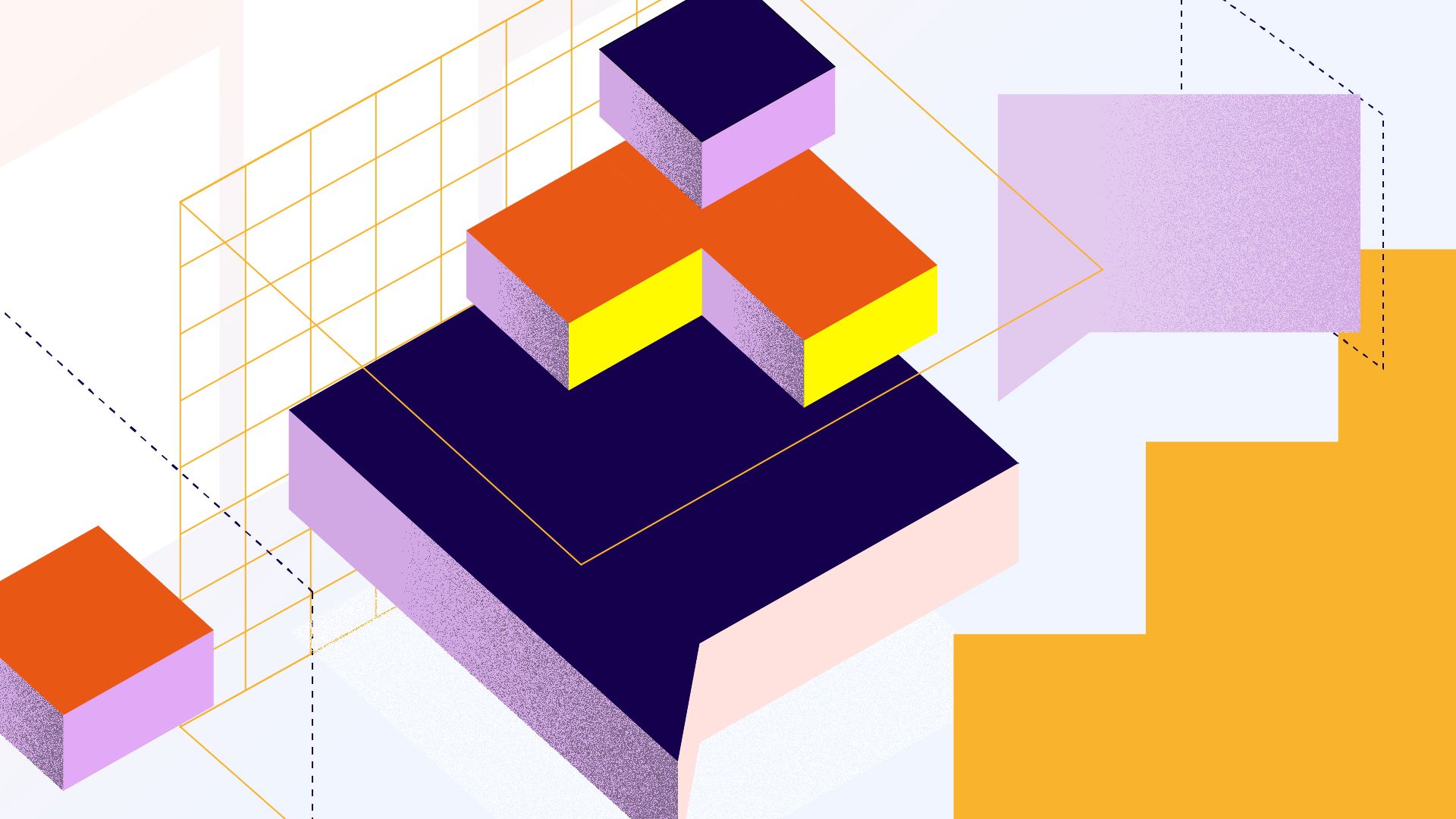
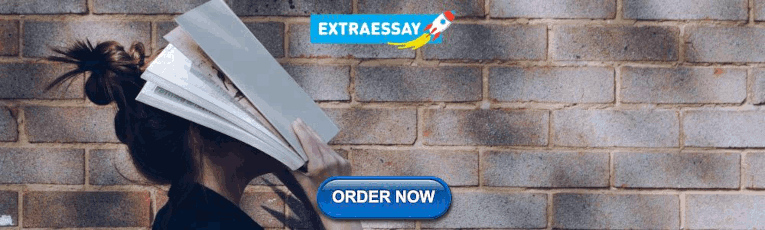
Qualitative Data Analysis: Step-by-Step Guide (Manual vs. Automatic)
When we conduct qualitative methods of research, need to explain changes in metrics or understand people's opinions, we always turn to qualitative data. Qualitative data is typically generated through:
- Interview transcripts
- Surveys with open-ended questions
- Contact center transcripts
- Texts and documents
- Audio and video recordings
- Observational notes
Compared to quantitative data, which captures structured information, qualitative data is unstructured and has more depth. It can answer our questions, can help formulate hypotheses and build understanding.
It's important to understand the differences between quantitative data & qualitative data . But unfortunately, analyzing qualitative data is difficult. While tools like Excel, Tableau and PowerBI crunch and visualize quantitative data with ease, there are a limited number of mainstream tools for analyzing qualitative data . The majority of qualitative data analysis still happens manually.
That said, there are two new trends that are changing this. First, there are advances in natural language processing (NLP) which is focused on understanding human language. Second, there is an explosion of user-friendly software designed for both researchers and businesses. Both help automate the qualitative data analysis process.
In this post we want to teach you how to conduct a successful qualitative data analysis. There are two primary qualitative data analysis methods; manual & automatic. We will teach you how to conduct the analysis manually, and also, automatically using software solutions powered by NLP. We’ll guide you through the steps to conduct a manual analysis, and look at what is involved and the role technology can play in automating this process.
More businesses are switching to fully-automated analysis of qualitative customer data because it is cheaper, faster, and just as accurate. Primarily, businesses purchase subscriptions to feedback analytics platforms so that they can understand customer pain points and sentiment.
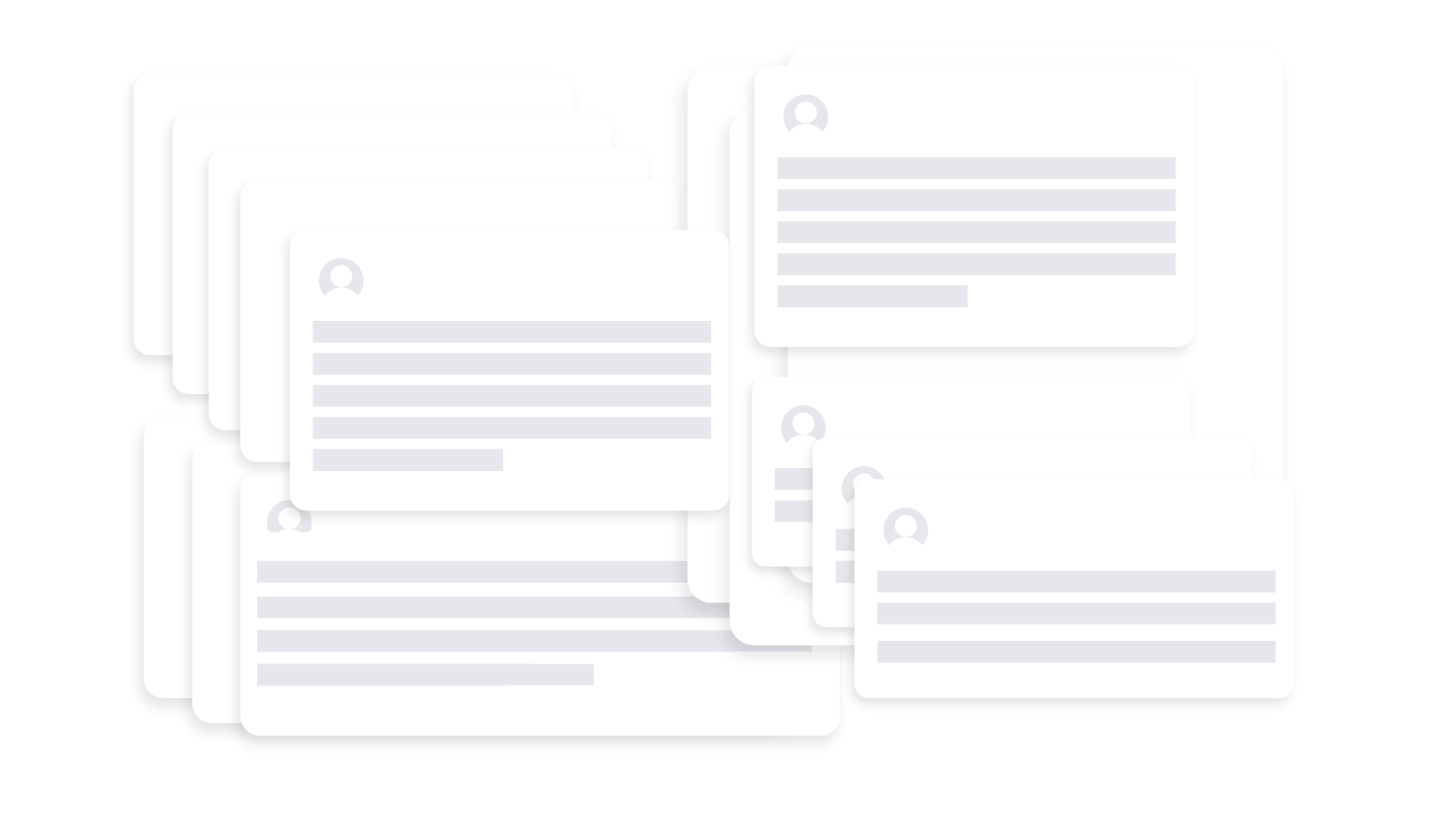
We’ll take you through 5 steps to conduct a successful qualitative data analysis. Within each step we will highlight the key difference between the manual, and automated approach of qualitative researchers. Here's an overview of the steps:
The 5 steps to doing qualitative data analysis
- Gathering and collecting your qualitative data
- Organizing and connecting into your qualitative data
- Coding your qualitative data
- Analyzing the qualitative data for insights
- Reporting on the insights derived from your analysis
What is Qualitative Data Analysis?
Qualitative data analysis is a process of gathering, structuring and interpreting qualitative data to understand what it represents.
Qualitative data is non-numerical and unstructured. Qualitative data generally refers to text, such as open-ended responses to survey questions or user interviews, but also includes audio, photos and video.
Businesses often perform qualitative data analysis on customer feedback. And within this context, qualitative data generally refers to verbatim text data collected from sources such as reviews, complaints, chat messages, support centre interactions, customer interviews, case notes or social media comments.
How is qualitative data analysis different from quantitative data analysis?
Understanding the differences between quantitative & qualitative data is important. When it comes to analyzing data, Qualitative Data Analysis serves a very different role to Quantitative Data Analysis. But what sets them apart?
Qualitative Data Analysis dives into the stories hidden in non-numerical data such as interviews, open-ended survey answers, or notes from observations. It uncovers the ‘whys’ and ‘hows’ giving a deep understanding of people’s experiences and emotions.
Quantitative Data Analysis on the other hand deals with numerical data, using statistics to measure differences, identify preferred options, and pinpoint root causes of issues. It steps back to address questions like "how many" or "what percentage" to offer broad insights we can apply to larger groups.
In short, Qualitative Data Analysis is like a microscope, helping us understand specific detail. Quantitative Data Analysis is like the telescope, giving us a broader perspective. Both are important, working together to decode data for different objectives.
Qualitative Data Analysis methods
Once all the data has been captured, there are a variety of analysis techniques available and the choice is determined by your specific research objectives and the kind of data you’ve gathered. Common qualitative data analysis methods include:
Content Analysis
This is a popular approach to qualitative data analysis. Other qualitative analysis techniques may fit within the broad scope of content analysis. Thematic analysis is a part of the content analysis. Content analysis is used to identify the patterns that emerge from text, by grouping content into words, concepts, and themes. Content analysis is useful to quantify the relationship between all of the grouped content. The Columbia School of Public Health has a detailed breakdown of content analysis .
Narrative Analysis
Narrative analysis focuses on the stories people tell and the language they use to make sense of them. It is particularly useful in qualitative research methods where customer stories are used to get a deep understanding of customers’ perspectives on a specific issue. A narrative analysis might enable us to summarize the outcomes of a focused case study.
Discourse Analysis
Discourse analysis is used to get a thorough understanding of the political, cultural and power dynamics that exist in specific situations. The focus of discourse analysis here is on the way people express themselves in different social contexts. Discourse analysis is commonly used by brand strategists who hope to understand why a group of people feel the way they do about a brand or product.
Thematic Analysis
Thematic analysis is used to deduce the meaning behind the words people use. This is accomplished by discovering repeating themes in text. These meaningful themes reveal key insights into data and can be quantified, particularly when paired with sentiment analysis . Often, the outcome of thematic analysis is a code frame that captures themes in terms of codes, also called categories. So the process of thematic analysis is also referred to as “coding”. A common use-case for thematic analysis in companies is analysis of customer feedback.
Grounded Theory
Grounded theory is a useful approach when little is known about a subject. Grounded theory starts by formulating a theory around a single data case. This means that the theory is “grounded”. Grounded theory analysis is based on actual data, and not entirely speculative. Then additional cases can be examined to see if they are relevant and can add to the original grounded theory.
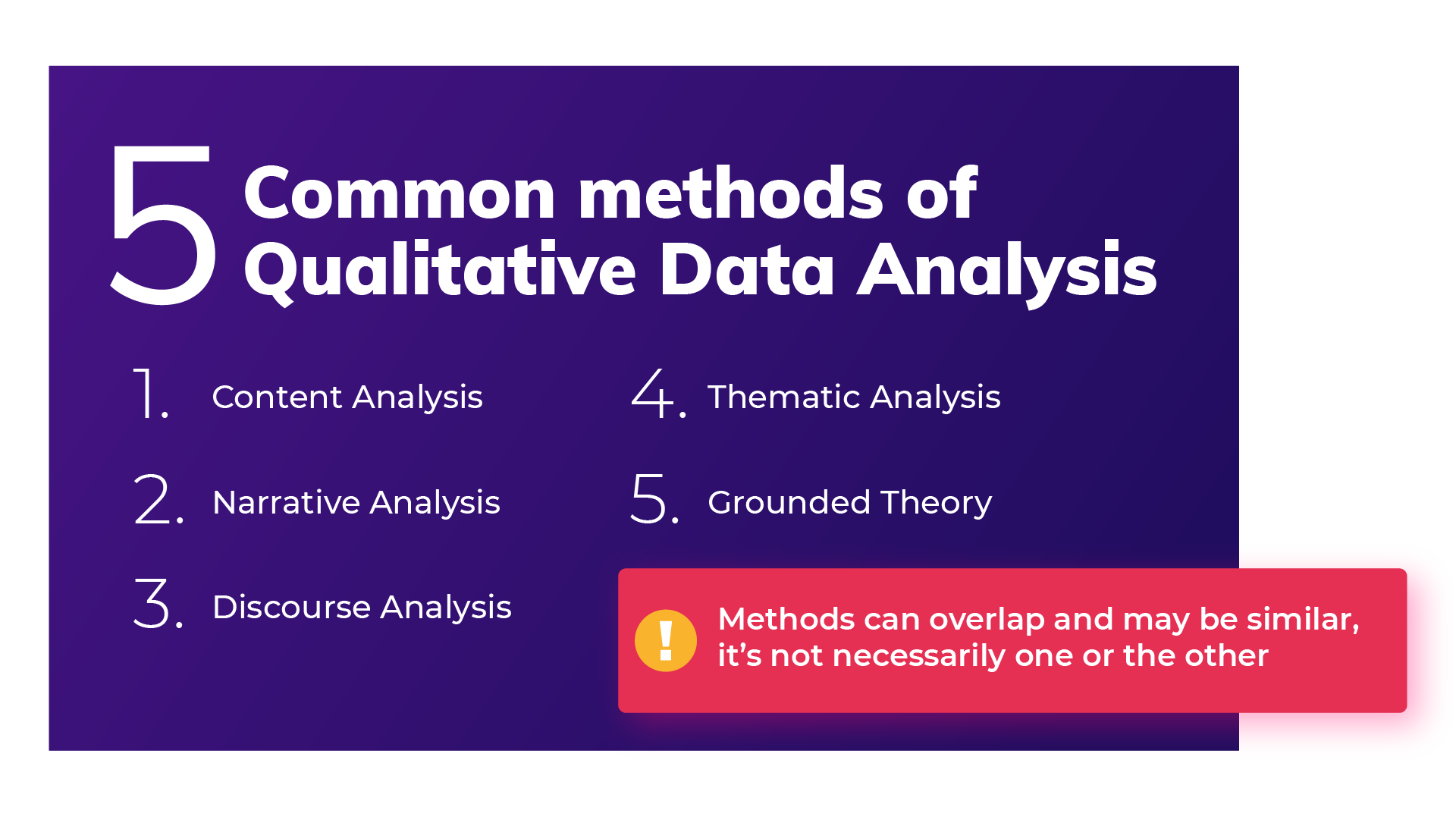
Challenges of Qualitative Data Analysis
While Qualitative Data Analysis offers rich insights, it comes with its challenges. Each unique QDA method has its unique hurdles. Let’s take a look at the challenges researchers and analysts might face, depending on the chosen method.
- Time and Effort (Narrative Analysis): Narrative analysis, which focuses on personal stories, demands patience. Sifting through lengthy narratives to find meaningful insights can be time-consuming, requires dedicated effort.
- Being Objective (Grounded Theory): Grounded theory, building theories from data, faces the challenges of personal biases. Staying objective while interpreting data is crucial, ensuring conclusions are rooted in the data itself.
- Complexity (Thematic Analysis): Thematic analysis involves identifying themes within data, a process that can be intricate. Categorizing and understanding themes can be complex, especially when each piece of data varies in context and structure. Thematic Analysis software can simplify this process.
- Generalizing Findings (Narrative Analysis): Narrative analysis, dealing with individual stories, makes drawing broad challenging. Extending findings from a single narrative to a broader context requires careful consideration.
- Managing Data (Thematic Analysis): Thematic analysis involves organizing and managing vast amounts of unstructured data, like interview transcripts. Managing this can be a hefty task, requiring effective data management strategies.
- Skill Level (Grounded Theory): Grounded theory demands specific skills to build theories from the ground up. Finding or training analysts with these skills poses a challenge, requiring investment in building expertise.
Benefits of qualitative data analysis
Qualitative Data Analysis (QDA) is like a versatile toolkit, offering a tailored approach to understanding your data. The benefits it offers are as diverse as the methods. Let’s explore why choosing the right method matters.
- Tailored Methods for Specific Needs: QDA isn't one-size-fits-all. Depending on your research objectives and the type of data at hand, different methods offer unique benefits. If you want emotive customer stories, narrative analysis paints a strong picture. When you want to explain a score, thematic analysis reveals insightful patterns
- Flexibility with Thematic Analysis: thematic analysis is like a chameleon in the toolkit of QDA. It adapts well to different types of data and research objectives, making it a top choice for any qualitative analysis.
- Deeper Understanding, Better Products: QDA helps you dive into people's thoughts and feelings. This deep understanding helps you build products and services that truly matches what people want, ensuring satisfied customers
- Finding the Unexpected: Qualitative data often reveals surprises that we miss in quantitative data. QDA offers us new ideas and perspectives, for insights we might otherwise miss.
- Building Effective Strategies: Insights from QDA are like strategic guides. They help businesses in crafting plans that match people’s desires.
- Creating Genuine Connections: Understanding people’s experiences lets businesses connect on a real level. This genuine connection helps build trust and loyalty, priceless for any business.
How to do Qualitative Data Analysis: 5 steps
Now we are going to show how you can do your own qualitative data analysis. We will guide you through this process step by step. As mentioned earlier, you will learn how to do qualitative data analysis manually , and also automatically using modern qualitative data and thematic analysis software.
To get best value from the analysis process and research process, it’s important to be super clear about the nature and scope of the question that’s being researched. This will help you select the research collection channels that are most likely to help you answer your question.
Depending on if you are a business looking to understand customer sentiment, or an academic surveying a school, your approach to qualitative data analysis will be unique.
Once you’re clear, there’s a sequence to follow. And, though there are differences in the manual and automatic approaches, the process steps are mostly the same.
The use case for our step-by-step guide is a company looking to collect data (customer feedback data), and analyze the customer feedback - in order to improve customer experience. By analyzing the customer feedback the company derives insights about their business and their customers. You can follow these same steps regardless of the nature of your research. Let’s get started.
Step 1: Gather your qualitative data and conduct research (Conduct qualitative research)
The first step of qualitative research is to do data collection. Put simply, data collection is gathering all of your data for analysis. A common situation is when qualitative data is spread across various sources.
Classic methods of gathering qualitative data
Most companies use traditional methods for gathering qualitative data: conducting interviews with research participants, running surveys, and running focus groups. This data is typically stored in documents, CRMs, databases and knowledge bases. It’s important to examine which data is available and needs to be included in your research project, based on its scope.
Using your existing qualitative feedback
As it becomes easier for customers to engage across a range of different channels, companies are gathering increasingly large amounts of both solicited and unsolicited qualitative feedback.
Most organizations have now invested in Voice of Customer programs , support ticketing systems, chatbot and support conversations, emails and even customer Slack chats.
These new channels provide companies with new ways of getting feedback, and also allow the collection of unstructured feedback data at scale.
The great thing about this data is that it contains a wealth of valubale insights and that it’s already there! When you have a new question about user behavior or your customers, you don’t need to create a new research study or set up a focus group. You can find most answers in the data you already have.
Typically, this data is stored in third-party solutions or a central database, but there are ways to export it or connect to a feedback analysis solution through integrations or an API.
Utilize untapped qualitative data channels
There are many online qualitative data sources you may not have considered. For example, you can find useful qualitative data in social media channels like Twitter or Facebook. Online forums, review sites, and online communities such as Discourse or Reddit also contain valuable data about your customers, or research questions.
If you are considering performing a qualitative benchmark analysis against competitors - the internet is your best friend. Gathering feedback in competitor reviews on sites like Trustpilot, G2, Capterra, Better Business Bureau or on app stores is a great way to perform a competitor benchmark analysis.
Customer feedback analysis software often has integrations into social media and review sites, or you could use a solution like DataMiner to scrape the reviews.
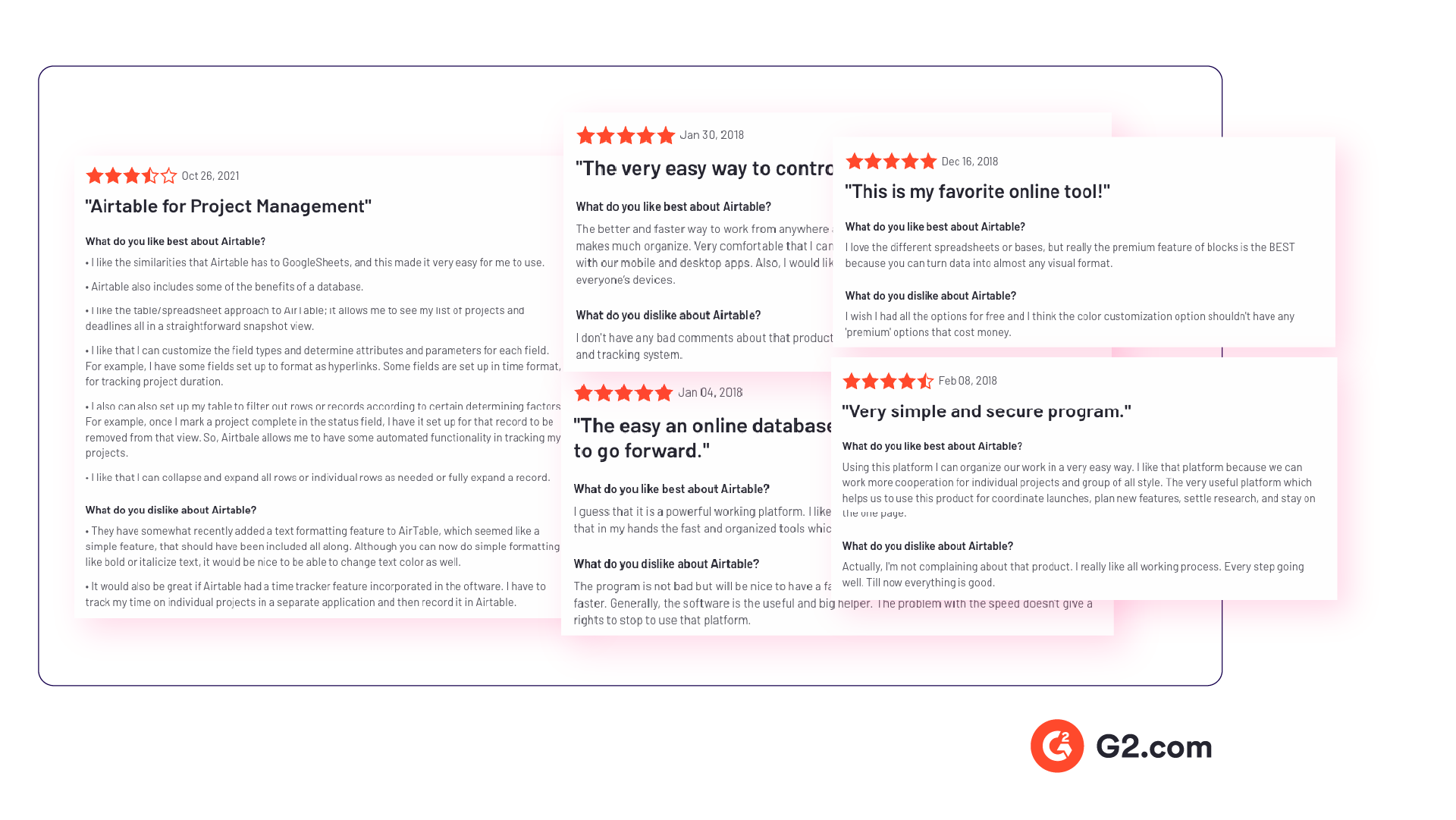
Step 2: Connect & organize all your qualitative data
Now you all have this qualitative data but there’s a problem, the data is unstructured. Before feedback can be analyzed and assigned any value, it needs to be organized in a single place. Why is this important? Consistency!
If all data is easily accessible in one place and analyzed in a consistent manner, you will have an easier time summarizing and making decisions based on this data.
The manual approach to organizing your data
The classic method of structuring qualitative data is to plot all the raw data you’ve gathered into a spreadsheet.
Typically, research and support teams would share large Excel sheets and different business units would make sense of the qualitative feedback data on their own. Each team collects and organizes the data in a way that best suits them, which means the feedback tends to be kept in separate silos.
An alternative and a more robust solution is to store feedback in a central database, like Snowflake or Amazon Redshift .
Keep in mind that when you organize your data in this way, you are often preparing it to be imported into another software. If you go the route of a database, you would need to use an API to push the feedback into a third-party software.
Computer-assisted qualitative data analysis software (CAQDAS)
Traditionally within the manual analysis approach (but not always), qualitative data is imported into CAQDAS software for coding.
In the early 2000s, CAQDAS software was popularised by developers such as ATLAS.ti, NVivo and MAXQDA and eagerly adopted by researchers to assist with the organizing and coding of data.
The benefits of using computer-assisted qualitative data analysis software:
- Assists in the organizing of your data
- Opens you up to exploring different interpretations of your data analysis
- Allows you to share your dataset easier and allows group collaboration (allows for secondary analysis)
However you still need to code the data, uncover the themes and do the analysis yourself. Therefore it is still a manual approach.
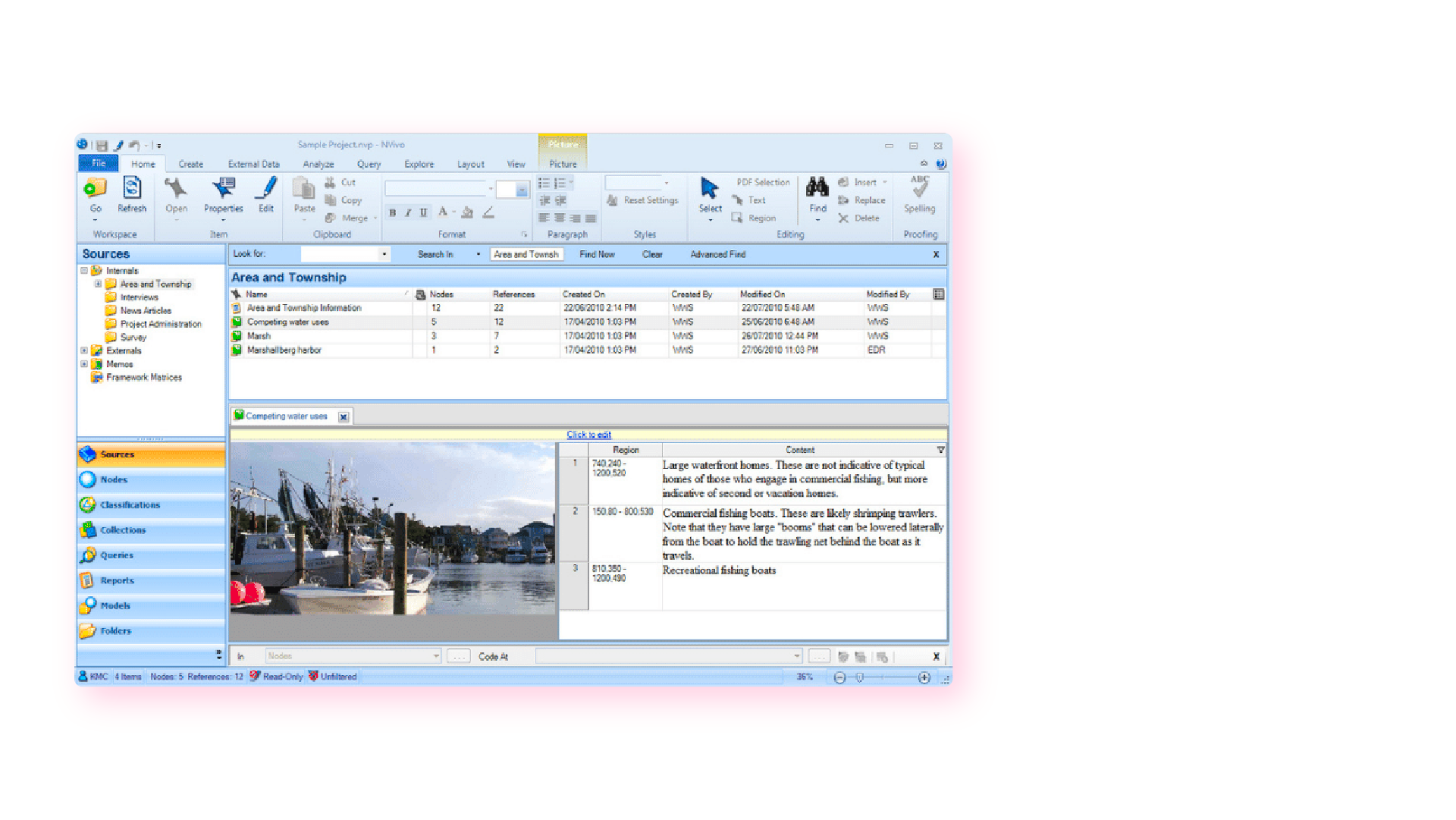
Organizing your qualitative data in a feedback repository
Another solution to organizing your qualitative data is to upload it into a feedback repository where it can be unified with your other data , and easily searchable and taggable. There are a number of software solutions that act as a central repository for your qualitative research data. Here are a couple solutions that you could investigate:
- Dovetail: Dovetail is a research repository with a focus on video and audio transcriptions. You can tag your transcriptions within the platform for theme analysis. You can also upload your other qualitative data such as research reports, survey responses, support conversations, and customer interviews. Dovetail acts as a single, searchable repository. And makes it easier to collaborate with other people around your qualitative research.
- EnjoyHQ: EnjoyHQ is another research repository with similar functionality to Dovetail. It boasts a more sophisticated search engine, but it has a higher starting subscription cost.
Organizing your qualitative data in a feedback analytics platform
If you have a lot of qualitative customer or employee feedback, from the likes of customer surveys or employee surveys, you will benefit from a feedback analytics platform. A feedback analytics platform is a software that automates the process of both sentiment analysis and thematic analysis . Companies use the integrations offered by these platforms to directly tap into their qualitative data sources (review sites, social media, survey responses, etc.). The data collected is then organized and analyzed consistently within the platform.
If you have data prepared in a spreadsheet, it can also be imported into feedback analytics platforms.
Once all this rich data has been organized within the feedback analytics platform, it is ready to be coded and themed, within the same platform. Thematic is a feedback analytics platform that offers one of the largest libraries of integrations with qualitative data sources.
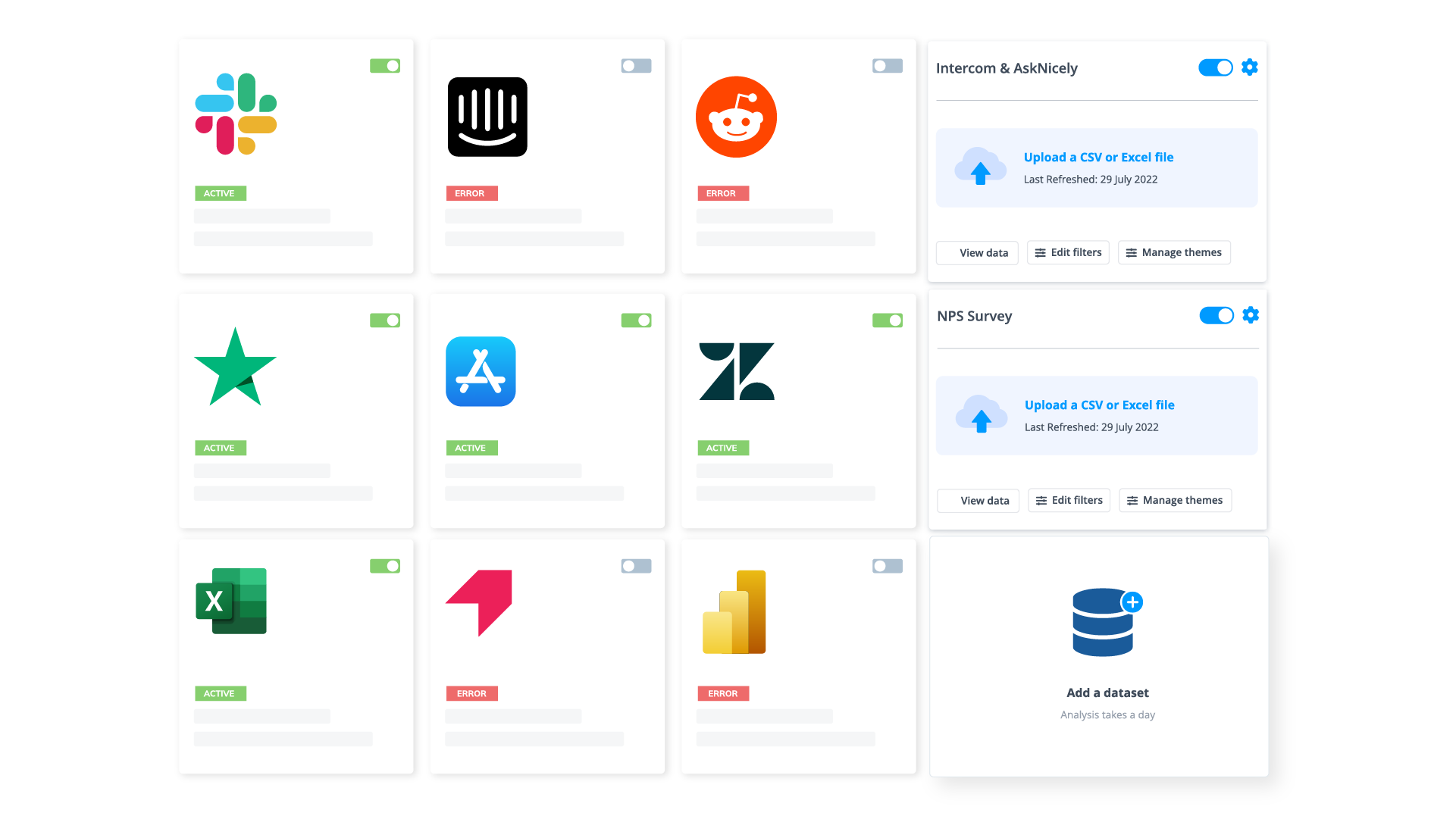
Step 3: Coding your qualitative data
Your feedback data is now organized in one place. Either within your spreadsheet, CAQDAS, feedback repository or within your feedback analytics platform. The next step is to code your feedback data so we can extract meaningful insights in the next step.
Coding is the process of labelling and organizing your data in such a way that you can then identify themes in the data, and the relationships between these themes.
To simplify the coding process, you will take small samples of your customer feedback data, come up with a set of codes, or categories capturing themes, and label each piece of feedback, systematically, for patterns and meaning. Then you will take a larger sample of data, revising and refining the codes for greater accuracy and consistency as you go.
If you choose to use a feedback analytics platform, much of this process will be automated and accomplished for you.
The terms to describe different categories of meaning (‘theme’, ‘code’, ‘tag’, ‘category’ etc) can be confusing as they are often used interchangeably. For clarity, this article will use the term ‘code’.
To code means to identify key words or phrases and assign them to a category of meaning. “I really hate the customer service of this computer software company” would be coded as “poor customer service”.
How to manually code your qualitative data
- Decide whether you will use deductive or inductive coding. Deductive coding is when you create a list of predefined codes, and then assign them to the qualitative data. Inductive coding is the opposite of this, you create codes based on the data itself. Codes arise directly from the data and you label them as you go. You need to weigh up the pros and cons of each coding method and select the most appropriate.
- Read through the feedback data to get a broad sense of what it reveals. Now it’s time to start assigning your first set of codes to statements and sections of text.
- Keep repeating step 2, adding new codes and revising the code description as often as necessary. Once it has all been coded, go through everything again, to be sure there are no inconsistencies and that nothing has been overlooked.
- Create a code frame to group your codes. The coding frame is the organizational structure of all your codes. And there are two commonly used types of coding frames, flat, or hierarchical. A hierarchical code frame will make it easier for you to derive insights from your analysis.
- Based on the number of times a particular code occurs, you can now see the common themes in your feedback data. This is insightful! If ‘bad customer service’ is a common code, it’s time to take action.
We have a detailed guide dedicated to manually coding your qualitative data .
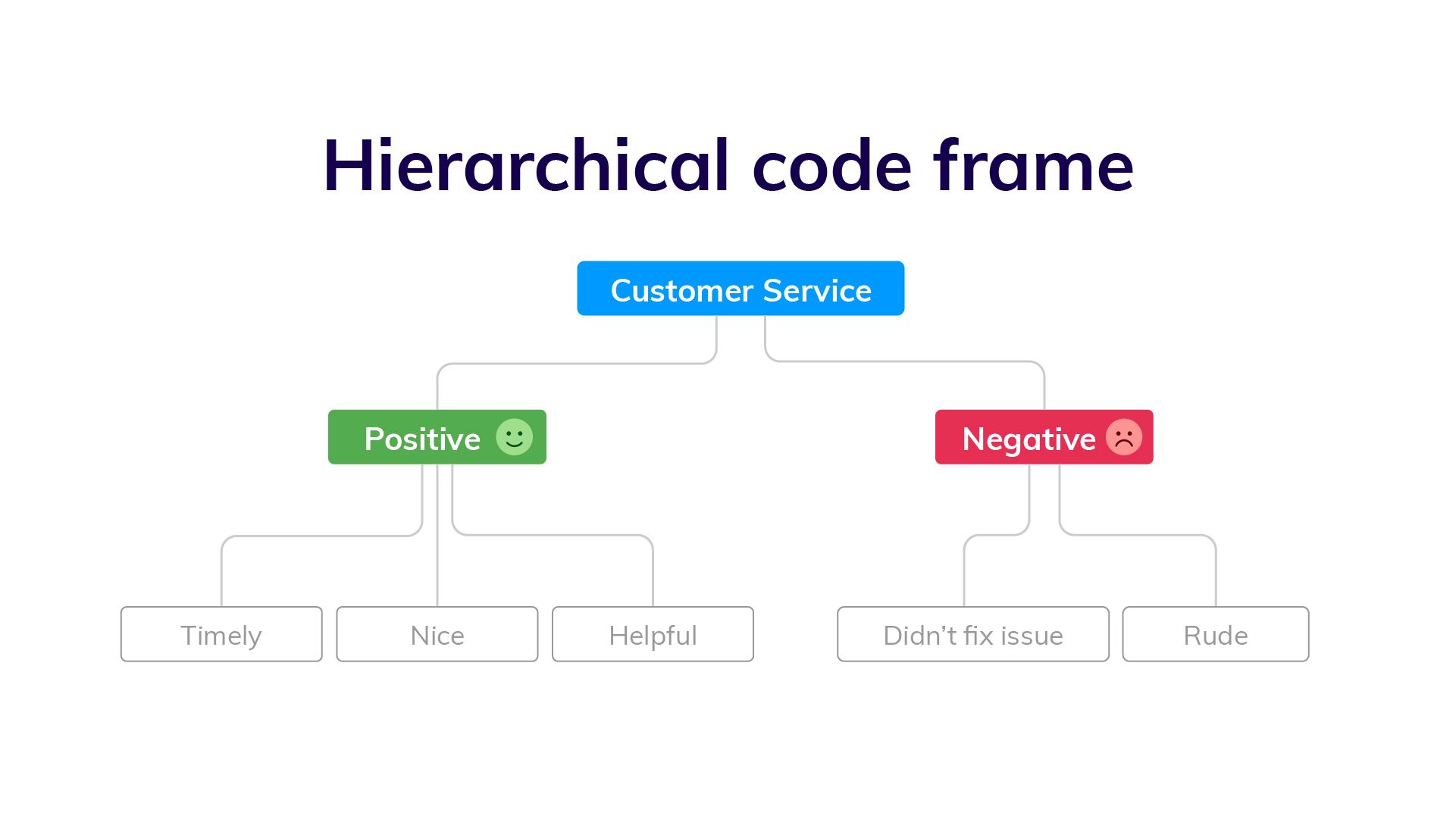
Using software to speed up manual coding of qualitative data
An Excel spreadsheet is still a popular method for coding. But various software solutions can help speed up this process. Here are some examples.
- CAQDAS / NVivo - CAQDAS software has built-in functionality that allows you to code text within their software. You may find the interface the software offers easier for managing codes than a spreadsheet.
- Dovetail/EnjoyHQ - You can tag transcripts and other textual data within these solutions. As they are also repositories you may find it simpler to keep the coding in one platform.
- IBM SPSS - SPSS is a statistical analysis software that may make coding easier than in a spreadsheet.
- Ascribe - Ascribe’s ‘Coder’ is a coding management system. Its user interface will make it easier for you to manage your codes.
Automating the qualitative coding process using thematic analysis software
In solutions which speed up the manual coding process, you still have to come up with valid codes and often apply codes manually to pieces of feedback. But there are also solutions that automate both the discovery and the application of codes.
Advances in machine learning have now made it possible to read, code and structure qualitative data automatically. This type of automated coding is offered by thematic analysis software .
Automation makes it far simpler and faster to code the feedback and group it into themes. By incorporating natural language processing (NLP) into the software, the AI looks across sentences and phrases to identify common themes meaningful statements. Some automated solutions detect repeating patterns and assign codes to them, others make you train the AI by providing examples. You could say that the AI learns the meaning of the feedback on its own.
Thematic automates the coding of qualitative feedback regardless of source. There’s no need to set up themes or categories in advance. Simply upload your data and wait a few minutes. You can also manually edit the codes to further refine their accuracy. Experiments conducted indicate that Thematic’s automated coding is just as accurate as manual coding .
Paired with sentiment analysis and advanced text analytics - these automated solutions become powerful for deriving quality business or research insights.
You could also build your own , if you have the resources!
The key benefits of using an automated coding solution
Automated analysis can often be set up fast and there’s the potential to uncover things that would never have been revealed if you had given the software a prescribed list of themes to look for.
Because the model applies a consistent rule to the data, it captures phrases or statements that a human eye might have missed.
Complete and consistent analysis of customer feedback enables more meaningful findings. Leading us into step 4.
Step 4: Analyze your data: Find meaningful insights
Now we are going to analyze our data to find insights. This is where we start to answer our research questions. Keep in mind that step 4 and step 5 (tell the story) have some overlap . This is because creating visualizations is both part of analysis process and reporting.
The task of uncovering insights is to scour through the codes that emerge from the data and draw meaningful correlations from them. It is also about making sure each insight is distinct and has enough data to support it.
Part of the analysis is to establish how much each code relates to different demographics and customer profiles, and identify whether there’s any relationship between these data points.
Manually create sub-codes to improve the quality of insights
If your code frame only has one level, you may find that your codes are too broad to be able to extract meaningful insights. This is where it is valuable to create sub-codes to your primary codes. This process is sometimes referred to as meta coding.
Note: If you take an inductive coding approach, you can create sub-codes as you are reading through your feedback data and coding it.
While time-consuming, this exercise will improve the quality of your analysis. Here is an example of what sub-codes could look like.
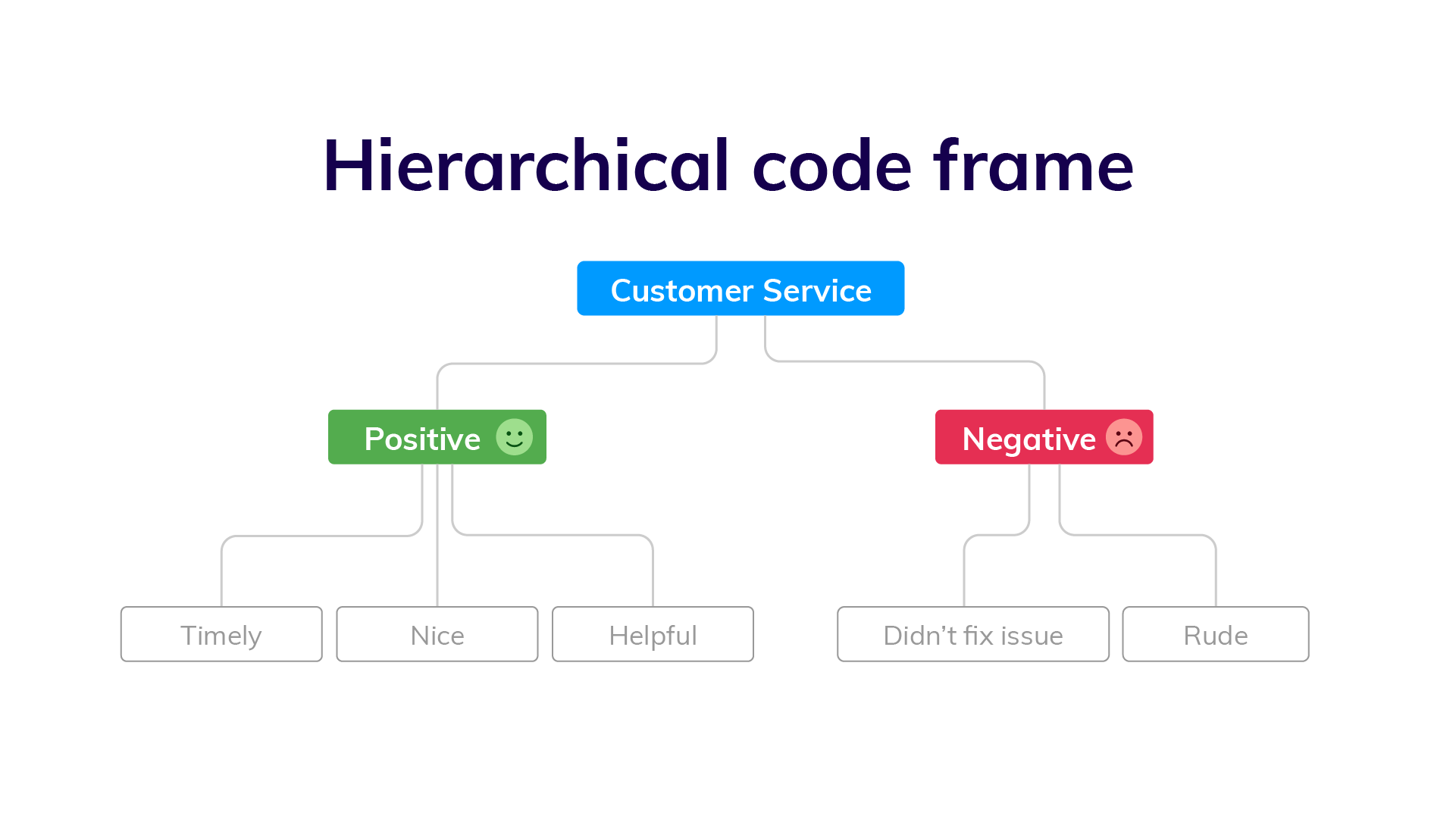
You need to carefully read your qualitative data to create quality sub-codes. But as you can see, the depth of analysis is greatly improved. By calculating the frequency of these sub-codes you can get insight into which customer service problems you can immediately address.
Correlate the frequency of codes to customer segments
Many businesses use customer segmentation . And you may have your own respondent segments that you can apply to your qualitative analysis. Segmentation is the practise of dividing customers or research respondents into subgroups.
Segments can be based on:
- Demographic
- And any other data type that you care to segment by
It is particularly useful to see the occurrence of codes within your segments. If one of your customer segments is considered unimportant to your business, but they are the cause of nearly all customer service complaints, it may be in your best interest to focus attention elsewhere. This is a useful insight!
Manually visualizing coded qualitative data
There are formulas you can use to visualize key insights in your data. The formulas we will suggest are imperative if you are measuring a score alongside your feedback.
If you are collecting a metric alongside your qualitative data this is a key visualization. Impact answers the question: “What’s the impact of a code on my overall score?”. Using Net Promoter Score (NPS) as an example, first you need to:
- Calculate overall NPS
- Calculate NPS in the subset of responses that do not contain that theme
- Subtract B from A
Then you can use this simple formula to calculate code impact on NPS .
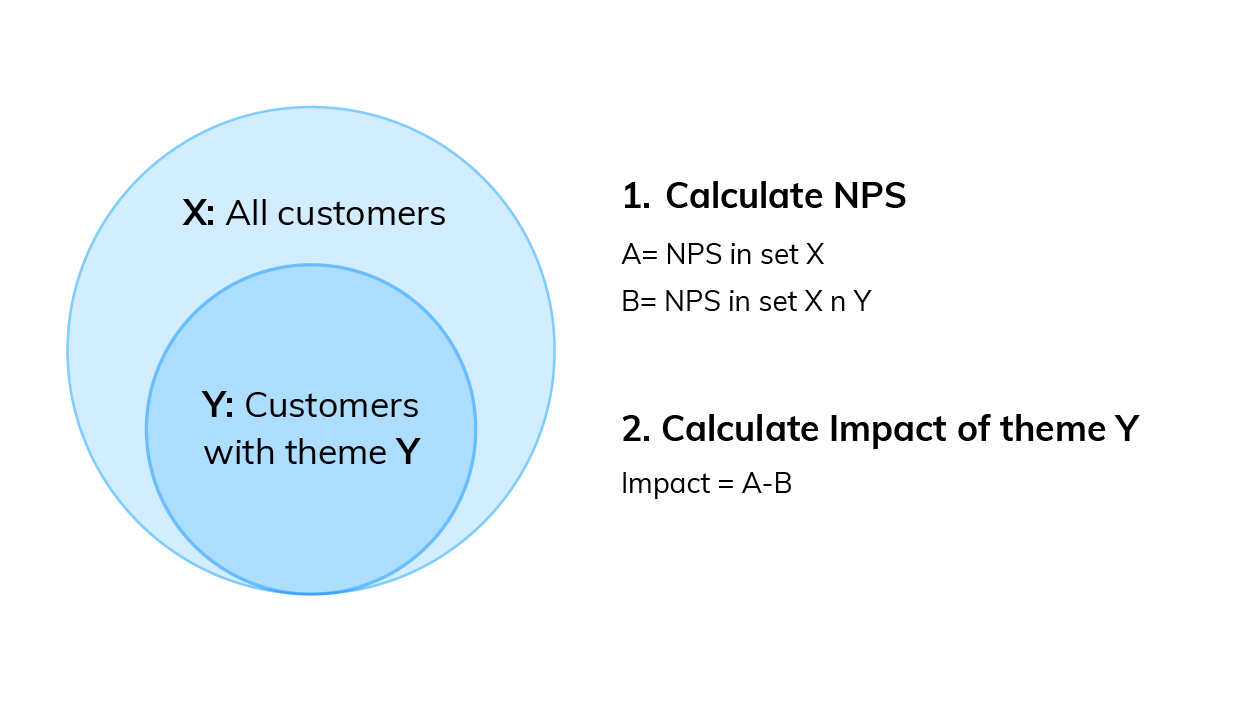
You can then visualize this data using a bar chart.
You can download our CX toolkit - it includes a template to recreate this.
Trends over time
This analysis can help you answer questions like: “Which codes are linked to decreases or increases in my score over time?”
We need to compare two sequences of numbers: NPS over time and code frequency over time . Using Excel, calculate the correlation between the two sequences, which can be either positive (the more codes the higher the NPS, see picture below), or negative (the more codes the lower the NPS).
Now you need to plot code frequency against the absolute value of code correlation with NPS. Here is the formula:
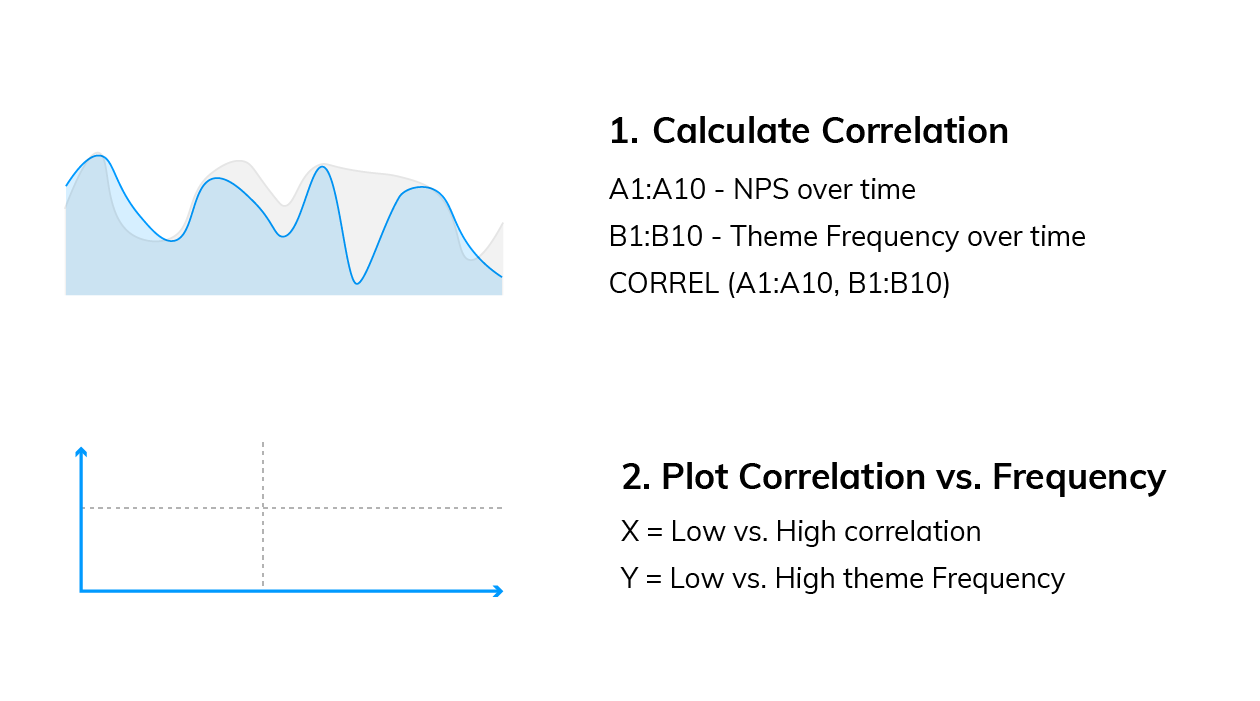
The visualization could look like this:
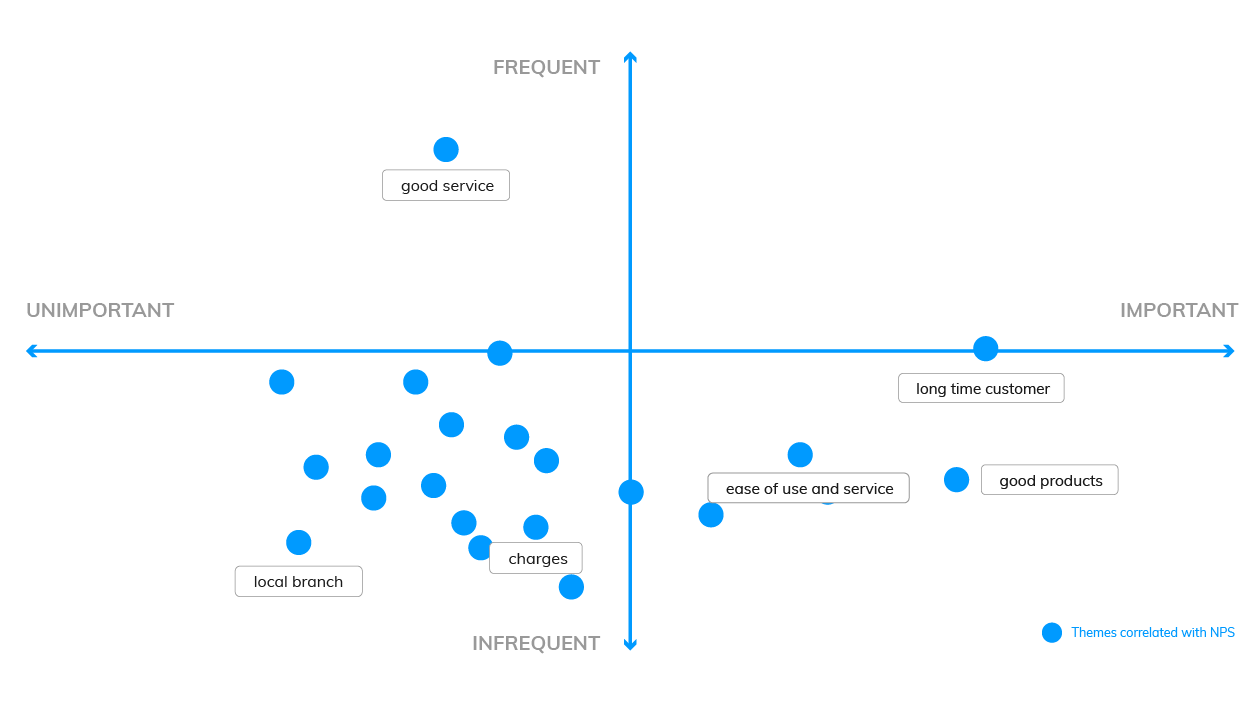
These are two examples, but there are more. For a third manual formula, and to learn why word clouds are not an insightful form of analysis, read our visualizations article .
Using a text analytics solution to automate analysis
Automated text analytics solutions enable codes and sub-codes to be pulled out of the data automatically. This makes it far faster and easier to identify what’s driving negative or positive results. And to pick up emerging trends and find all manner of rich insights in the data.
Another benefit of AI-driven text analytics software is its built-in capability for sentiment analysis, which provides the emotive context behind your feedback and other qualitative textual data therein.
Thematic provides text analytics that goes further by allowing users to apply their expertise on business context to edit or augment the AI-generated outputs.
Since the move away from manual research is generally about reducing the human element, adding human input to the technology might sound counter-intuitive. However, this is mostly to make sure important business nuances in the feedback aren’t missed during coding. The result is a higher accuracy of analysis. This is sometimes referred to as augmented intelligence .
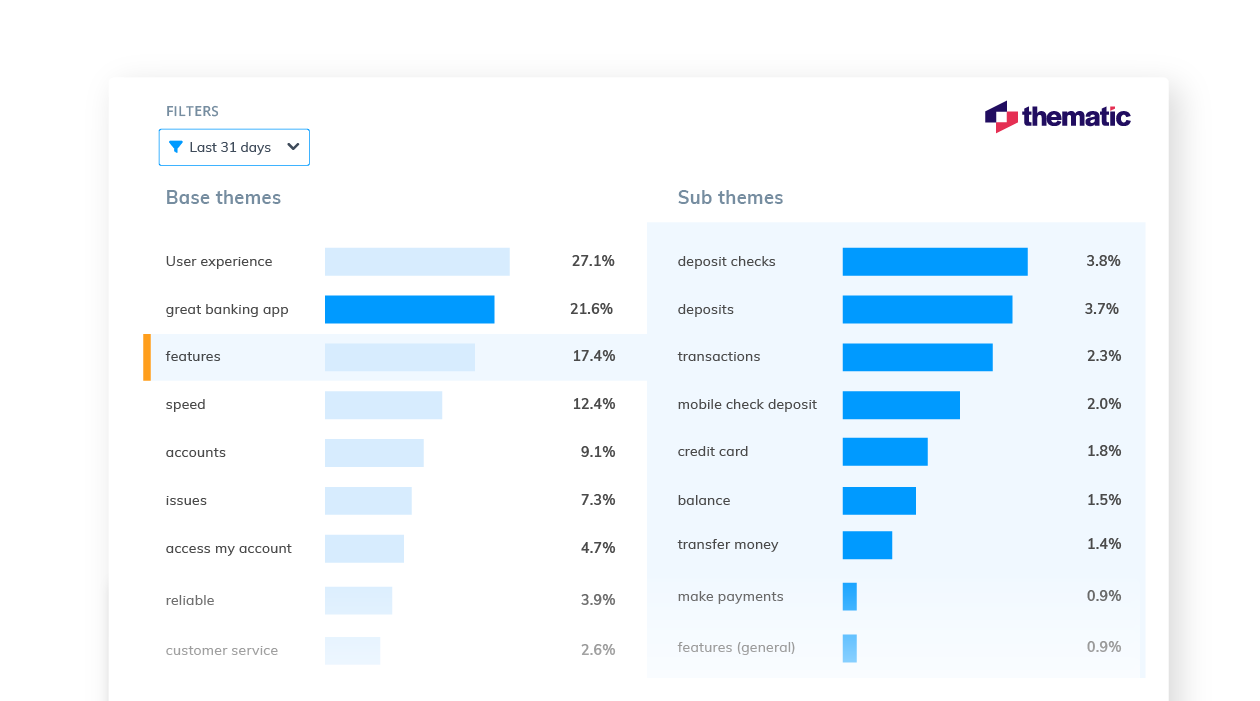
Step 5: Report on your data: Tell the story
The last step of analyzing your qualitative data is to report on it, to tell the story. At this point, the codes are fully developed and the focus is on communicating the narrative to the audience.
A coherent outline of the qualitative research, the findings and the insights is vital for stakeholders to discuss and debate before they can devise a meaningful course of action.
Creating graphs and reporting in Powerpoint
Typically, qualitative researchers take the tried and tested approach of distilling their report into a series of charts, tables and other visuals which are woven into a narrative for presentation in Powerpoint.
Using visualization software for reporting
With data transformation and APIs, the analyzed data can be shared with data visualisation software, such as Power BI or Tableau , Google Studio or Looker. Power BI and Tableau are among the most preferred options.
Visualizing your insights inside a feedback analytics platform
Feedback analytics platforms, like Thematic, incorporate visualisation tools that intuitively turn key data and insights into graphs. This removes the time consuming work of constructing charts to visually identify patterns and creates more time to focus on building a compelling narrative that highlights the insights, in bite-size chunks, for executive teams to review.
Using a feedback analytics platform with visualization tools means you don’t have to use a separate product for visualizations. You can export graphs into Powerpoints straight from the platforms.
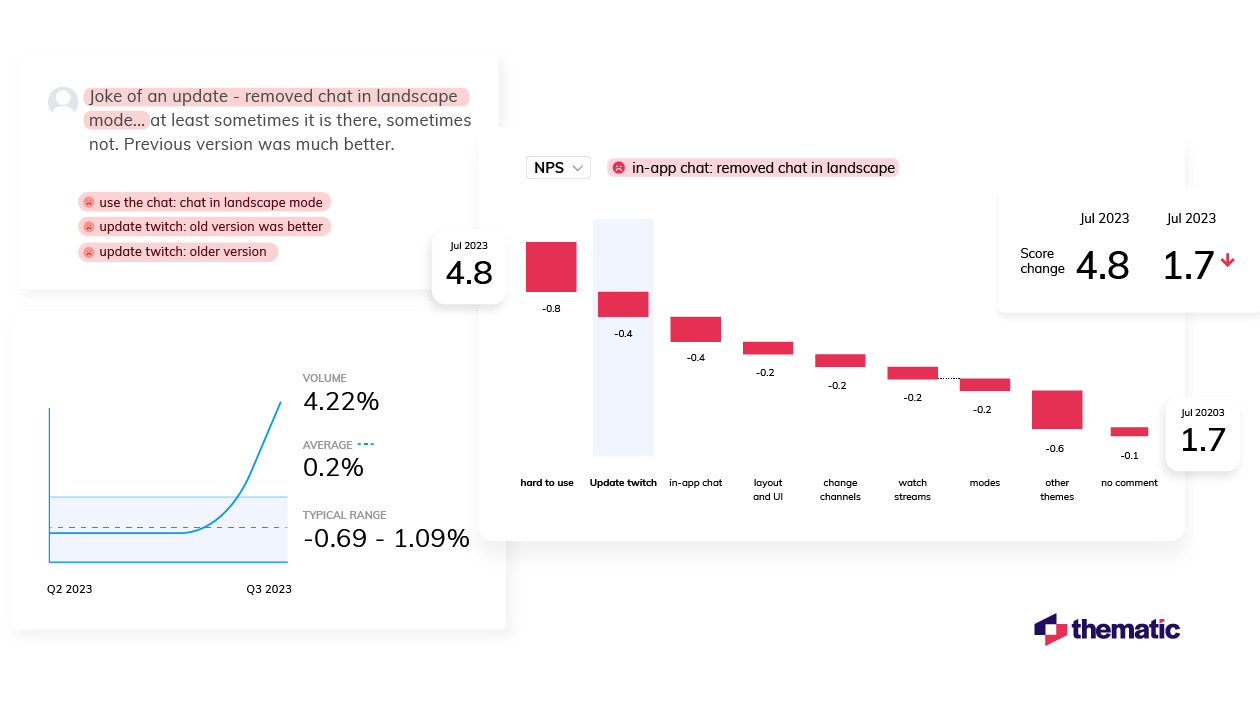
Conclusion - Manual or Automated?
There are those who remain deeply invested in the manual approach - because it’s familiar, because they’re reluctant to spend money and time learning new software, or because they’ve been burned by the overpromises of AI.
For projects that involve small datasets, manual analysis makes sense. For example, if the objective is simply to quantify a simple question like “Do customers prefer X concepts to Y?”. If the findings are being extracted from a small set of focus groups and interviews, sometimes it’s easier to just read them
However, as new generations come into the workplace, it’s technology-driven solutions that feel more comfortable and practical. And the merits are undeniable. Especially if the objective is to go deeper and understand the ‘why’ behind customers’ preference for X or Y. And even more especially if time and money are considerations.
The ability to collect a free flow of qualitative feedback data at the same time as the metric means AI can cost-effectively scan, crunch, score and analyze a ton of feedback from one system in one go. And time-intensive processes like focus groups, or coding, that used to take weeks, can now be completed in a matter of hours or days.
But aside from the ever-present business case to speed things up and keep costs down, there are also powerful research imperatives for automated analysis of qualitative data: namely, accuracy and consistency.
Finding insights hidden in feedback requires consistency, especially in coding. Not to mention catching all the ‘unknown unknowns’ that can skew research findings and steering clear of cognitive bias.
Some say without manual data analysis researchers won’t get an accurate “feel” for the insights. However, the larger data sets are, the harder it is to sort through the feedback and organize feedback that has been pulled from different places. And, the more difficult it is to stay on course, the greater the risk of drawing incorrect, or incomplete, conclusions grows.
Though the process steps for qualitative data analysis have remained pretty much unchanged since psychologist Paul Felix Lazarsfeld paved the path a hundred years ago, the impact digital technology has had on types of qualitative feedback data and the approach to the analysis are profound.
If you want to try an automated feedback analysis solution on your own qualitative data, you can get started with Thematic .
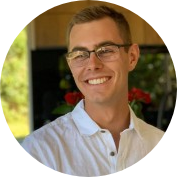
Community & Marketing
Tyler manages our community of CX, insights & analytics professionals. Tyler's goal is to help unite insights professionals around common challenges.
We make it easy to discover the customer and product issues that matter.
Unlock the value of feedback at scale, in one platform. Try it for free now!
- Questions to ask your Feedback Analytics vendor
- How to end customer churn for good
- Scalable analysis of NPS verbatims
- 5 Text analytics approaches
- How to calculate the ROI of CX
Our experts will show you how Thematic works, how to discover pain points and track the ROI of decisions. To access your free trial, book a personal demo today.
Recent posts
Watercare is New Zealand's largest water and wastewater service provider. They are responsible for bringing clean water to 1.7 million people in Tamaki Makaurau (Auckland) and safeguarding the wastewater network to minimize impact on the environment. Water is a sector that often gets taken for granted, with drainage and
Become a qualitative theming pro! Creating a perfect code frame is hard, but thematic analysis software makes the process much easier.
Qualtrics is one of the most well-known and powerful Customer Feedback Management platforms. But even so, it has limitations. We recently hosted a live panel where data analysts from two well-known brands shared their experiences with Qualtrics, and how they extended this platform’s capabilities. Below, we’ll share the
- Skip to main content
- Skip to primary sidebar
- Skip to footer
- QuestionPro

- Solutions Industries Gaming Automotive Sports and events Education Government Travel & Hospitality Financial Services Healthcare Cannabis Technology Use Case NPS+ Communities Audience Contactless surveys Mobile LivePolls Member Experience GDPR Positive People Science 360 Feedback Surveys
- Resources Blog eBooks Survey Templates Case Studies Training Help center

Home Market Research
Qualitative Data Analysis: What is it, Methods + Examples
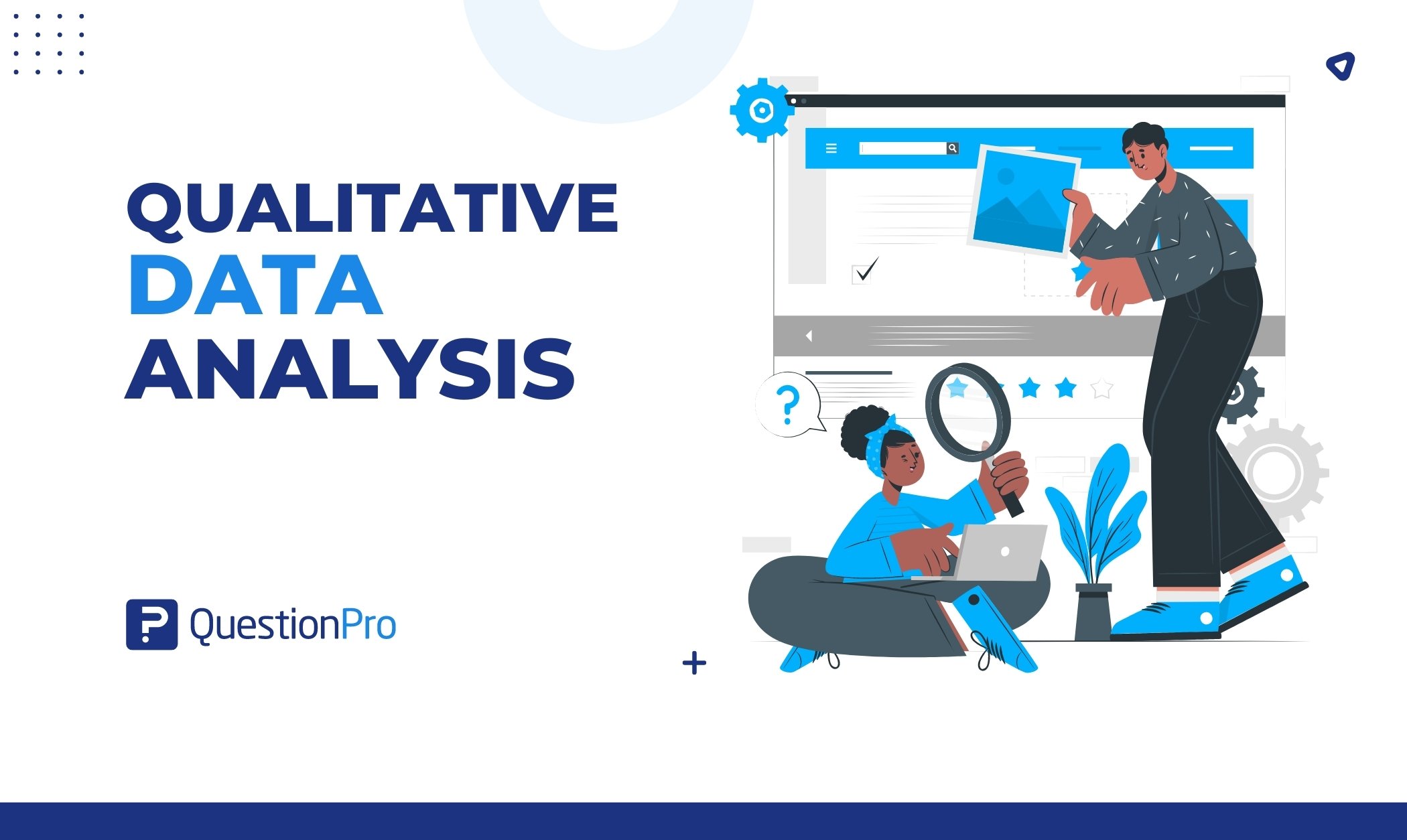
In a world rich with information and narrative, understanding the deeper layers of human experiences requires a unique vision that goes beyond numbers and figures. This is where the power of qualitative data analysis comes to light.
In this blog, we’ll learn about qualitative data analysis, explore its methods, and provide real-life examples showcasing its power in uncovering insights.
What is Qualitative Data Analysis?
Qualitative data analysis is a systematic process of examining non-numerical data to extract meaning, patterns, and insights.
In contrast to quantitative analysis, which focuses on numbers and statistical metrics, the qualitative study focuses on the qualitative aspects of data, such as text, images, audio, and videos. It seeks to understand every aspect of human experiences, perceptions, and behaviors by examining the data’s richness.
Companies frequently conduct this analysis on customer feedback. You can collect qualitative data from reviews, complaints, chat messages, interactions with support centers, customer interviews, case notes, or even social media comments. This kind of data holds the key to understanding customer sentiments and preferences in a way that goes beyond mere numbers.
Importance of Qualitative Data Analysis
Qualitative data analysis plays a crucial role in your research and decision-making process across various disciplines. Let’s explore some key reasons that underline the significance of this analysis:
In-Depth Understanding
It enables you to explore complex and nuanced aspects of a phenomenon, delving into the ‘how’ and ‘why’ questions. This method provides you with a deeper understanding of human behavior, experiences, and contexts that quantitative approaches might not capture fully.
Contextual Insight
You can use this analysis to give context to numerical data. It will help you understand the circumstances and conditions that influence participants’ thoughts, feelings, and actions. This contextual insight becomes essential for generating comprehensive explanations.
Theory Development
You can generate or refine hypotheses via qualitative data analysis. As you analyze the data attentively, you can form hypotheses, concepts, and frameworks that will drive your future research and contribute to theoretical advances.
Participant Perspectives
When performing qualitative research, you can highlight participant voices and opinions. This approach is especially useful for understanding marginalized or underrepresented people, as it allows them to communicate their experiences and points of view.
Exploratory Research
The analysis is frequently used at the exploratory stage of your project. It assists you in identifying important variables, developing research questions, and designing quantitative studies that will follow.
Types of Qualitative Data
When conducting qualitative research, you can use several qualitative data collection methods , and here you will come across many sorts of qualitative data that can provide you with unique insights into your study topic. These data kinds add new views and angles to your understanding and analysis.
Interviews and Focus Groups
Interviews and focus groups will be among your key methods for gathering qualitative data. Interviews are one-on-one talks in which participants can freely share their thoughts, experiences, and opinions.
Focus groups, on the other hand, are discussions in which members interact with one another, resulting in dynamic exchanges of ideas. Both methods provide rich qualitative data and direct access to participant perspectives.
Observations and Field Notes
Observations and field notes are another useful sort of qualitative data. You can immerse yourself in the research environment through direct observation, carefully documenting behaviors, interactions, and contextual factors.
These observations will be recorded in your field notes, providing a complete picture of the environment and the behaviors you’re researching. This data type is especially important for comprehending behavior in their natural setting.
Textual and Visual Data
Textual and visual data include a wide range of resources that can be qualitatively analyzed. Documents, written narratives, and transcripts from various sources, such as interviews or speeches, are examples of textual data.
Photographs, films, and even artwork provide a visual layer to your research. These forms of data allow you to investigate what is spoken and the underlying emotions, details, and symbols expressed by language or pictures.
When to Choose Qualitative Data Analysis over Quantitative Data Analysis
As you begin your research journey, understanding why the analysis of qualitative data is important will guide your approach to understanding complex events. If you analyze qualitative data, it will provide new insights that complement quantitative methodologies, which will give you a broader understanding of your study topic.
It is critical to know when to use qualitative analysis over quantitative procedures. You can prefer qualitative data analysis when:
- Complexity Reigns: When your research questions involve deep human experiences, motivations, or emotions, qualitative research excels at revealing these complexities.
- Exploration is Key: Qualitative analysis is ideal for exploratory research. It will assist you in understanding a new or poorly understood topic before formulating quantitative hypotheses.
- Context Matters: If you want to understand how context affects behaviors or results, qualitative data analysis provides the depth needed to grasp these relationships.
- Unanticipated Findings: When your study provides surprising new viewpoints or ideas, qualitative analysis helps you to delve deeply into these emerging themes.
- Subjective Interpretation is Vital: When it comes to understanding people’s subjective experiences and interpretations, qualitative data analysis is the way to go.
You can make informed decisions regarding the right approach for your research objectives if you understand the importance of qualitative analysis and recognize the situations where it shines.
Qualitative Data Analysis Methods and Examples
Exploring various qualitative data analysis methods will provide you with a wide collection for making sense of your research findings. Once the data has been collected, you can choose from several analysis methods based on your research objectives and the data type you’ve collected.
There are five main methods for analyzing qualitative data. Each method takes a distinct approach to identifying patterns, themes, and insights within your qualitative data. They are:
Method 1: Content Analysis
Content analysis is a methodical technique for analyzing textual or visual data in a structured manner. In this method, you will categorize qualitative data by splitting it into manageable pieces and assigning the manual coding process to these units.
As you go, you’ll notice ongoing codes and designs that will allow you to conclude the content. This method is very beneficial for detecting common ideas, concepts, or themes in your data without losing the context.
Steps to Do Content Analysis
Follow these steps when conducting content analysis:
- Collect and Immerse: Begin by collecting the necessary textual or visual data. Immerse yourself in this data to fully understand its content, context, and complexities.
- Assign Codes and Categories: Assign codes to relevant data sections that systematically represent major ideas or themes. Arrange comparable codes into groups that cover the major themes.
- Analyze and Interpret: Develop a structured framework from the categories and codes. Then, evaluate the data in the context of your research question, investigate relationships between categories, discover patterns, and draw meaning from these connections.
Benefits & Challenges
There are various advantages to using content analysis:
- Structured Approach: It offers a systematic approach to dealing with large data sets and ensures consistency throughout the research.
- Objective Insights: This method promotes objectivity, which helps to reduce potential biases in your study.
- Pattern Discovery: Content analysis can help uncover hidden trends, themes, and patterns that are not always obvious.
- Versatility: You can apply content analysis to various data formats, including text, internet content, images, etc.
However, keep in mind the challenges that arise:
- Subjectivity: Even with the best attempts, a certain bias may remain in coding and interpretation.
- Complexity: Analyzing huge data sets requires time and great attention to detail.
- Contextual Nuances: Content analysis may not capture all of the contextual richness that qualitative data analysis highlights.
Example of Content Analysis
Suppose you’re conducting market research and looking at customer feedback on a product. As you collect relevant data and analyze feedback, you’ll see repeating codes like “price,” “quality,” “customer service,” and “features.” These codes are organized into categories such as “positive reviews,” “negative reviews,” and “suggestions for improvement.”
According to your findings, themes such as “price” and “customer service” stand out and show that pricing and customer service greatly impact customer satisfaction. This example highlights the power of content analysis for obtaining significant insights from large textual data collections.
Method 2: Thematic Analysis
Thematic analysis is a well-structured procedure for identifying and analyzing recurring themes in your data. As you become more engaged in the data, you’ll generate codes or short labels representing key concepts. These codes are then organized into themes, providing a consistent framework for organizing and comprehending the substance of the data.
The analysis allows you to organize complex narratives and perspectives into meaningful categories, which will allow you to identify connections and patterns that may not be visible at first.
Steps to Do Thematic Analysis
Follow these steps when conducting a thematic analysis:
- Code and Group: Start by thoroughly examining the data and giving initial codes that identify the segments. To create initial themes, combine relevant codes.
- Code and Group: Begin by engaging yourself in the data, assigning first codes to notable segments. To construct basic themes, group comparable codes together.
- Analyze and Report: Analyze the data within each theme to derive relevant insights. Organize the topics into a consistent structure and explain your findings, along with data extracts that represent each theme.
Thematic analysis has various benefits:
- Structured Exploration: It is a method for identifying patterns and themes in complex qualitative data.
- Comprehensive knowledge: Thematic analysis promotes an in-depth understanding of the complications and meanings of the data.
- Application Flexibility: This method can be customized to various research situations and data kinds.
However, challenges may arise, such as:
- Interpretive Nature: Interpreting qualitative data in thematic analysis is vital, and it is critical to manage researcher bias.
- Time-consuming: The study can be time-consuming, especially with large data sets.
- Subjectivity: The selection of codes and topics might be subjective.
Example of Thematic Analysis
Assume you’re conducting a thematic analysis on job satisfaction interviews. Following your immersion in the data, you assign initial codes such as “work-life balance,” “career growth,” and “colleague relationships.” As you organize these codes, you’ll notice themes develop, such as “Factors Influencing Job Satisfaction” and “Impact on Work Engagement.”
Further investigation reveals the tales and experiences included within these themes and provides insights into how various elements influence job satisfaction. This example demonstrates how thematic analysis can reveal meaningful patterns and insights in qualitative data.
Method 3: Narrative Analysis
The narrative analysis involves the narratives that people share. You’ll investigate the histories in your data, looking at how stories are created and the meanings they express. This method is excellent for learning how people make sense of their experiences through narrative.
Steps to Do Narrative Analysis
The following steps are involved in narrative analysis:
- Gather and Analyze: Start by collecting narratives, such as first-person tales, interviews, or written accounts. Analyze the stories, focusing on the plot, feelings, and characters.
- Find Themes: Look for recurring themes or patterns in various narratives. Think about the similarities and differences between these topics and personal experiences.
- Interpret and Extract Insights: Contextualize the narratives within their larger context. Accept the subjective nature of each narrative and analyze the narrator’s voice and style. Extract insights from the tales by diving into the emotions, motivations, and implications communicated by the stories.
There are various advantages to narrative analysis:
- Deep Exploration: It lets you look deeply into people’s personal experiences and perspectives.
- Human-Centered: This method prioritizes the human perspective, allowing individuals to express themselves.
However, difficulties may arise, such as:
- Interpretive Complexity: Analyzing narratives requires dealing with the complexities of meaning and interpretation.
- Time-consuming: Because of the richness and complexities of tales, working with them can be time-consuming.
Example of Narrative Analysis
Assume you’re conducting narrative analysis on refugee interviews. As you read the stories, you’ll notice common themes of toughness, loss, and hope. The narratives provide insight into the obstacles that refugees face, their strengths, and the dreams that guide them.
The analysis can provide a deeper insight into the refugees’ experiences and the broader social context they navigate by examining the narratives’ emotional subtleties and underlying meanings. This example highlights how narrative analysis can reveal important insights into human stories.
Method 4: Grounded Theory Analysis
Grounded theory analysis is an iterative and systematic approach that allows you to create theories directly from data without being limited by pre-existing hypotheses. With an open mind, you collect data and generate early codes and labels that capture essential ideas or concepts within the data.
As you progress, you refine these codes and increasingly connect them, eventually developing a theory based on the data. Grounded theory analysis is a dynamic process for developing new insights and hypotheses based on details in your data.
Steps to Do Grounded Theory Analysis
Grounded theory analysis requires the following steps:
- Initial Coding: First, immerse yourself in the data, producing initial codes that represent major concepts or patterns.
- Categorize and Connect: Using axial coding, organize the initial codes, which establish relationships and connections between topics.
- Build the Theory: Focus on creating a core category that connects the codes and themes. Regularly refine the theory by comparing and integrating new data, ensuring that it evolves organically from the data.
Grounded theory analysis has various benefits:
- Theory Generation: It provides a one-of-a-kind opportunity to generate hypotheses straight from data and promotes new insights.
- In-depth Understanding: The analysis allows you to deeply analyze the data and reveal complex relationships and patterns.
- Flexible Process: This method is customizable and ongoing, which allows you to enhance your research as you collect additional data.
However, challenges might arise with:
- Time and Resources: Because grounded theory analysis is a continuous process, it requires a large commitment of time and resources.
- Theoretical Development: Creating a grounded theory involves a thorough understanding of qualitative data analysis software and theoretical concepts.
- Interpretation of Complexity: Interpreting and incorporating a newly developed theory into existing literature can be intellectually hard.
Example of Grounded Theory Analysis
Assume you’re performing a grounded theory analysis on workplace collaboration interviews. As you open code the data, you will discover notions such as “communication barriers,” “team dynamics,” and “leadership roles.” Axial coding demonstrates links between these notions, emphasizing the significance of efficient communication in developing collaboration.
You create the core “Integrated Communication Strategies” category through selective coding, which unifies new topics.
This theory-driven category serves as the framework for understanding how numerous aspects contribute to effective team collaboration. This example shows how grounded theory analysis allows you to generate a theory directly from the inherent nature of the data.
Method 5: Discourse Analysis
Discourse analysis focuses on language and communication. You’ll look at how language produces meaning and how it reflects power relations, identities, and cultural influences. This strategy examines what is said and how it is said; the words, phrasing, and larger context of communication.
The analysis is precious when investigating power dynamics, identities, and cultural influences encoded in language. By evaluating the language used in your data, you can identify underlying assumptions, cultural standards, and how individuals negotiate meaning through communication.
Steps to Do Discourse Analysis
Conducting discourse analysis entails the following steps:
- Select Discourse: For analysis, choose language-based data such as texts, speeches, or media content.
- Analyze Language: Immerse yourself in the conversation, examining language choices, metaphors, and underlying assumptions.
- Discover Patterns: Recognize the dialogue’s reoccurring themes, ideologies, and power dynamics. To fully understand the effects of these patterns, put them in their larger context.
There are various advantages of using discourse analysis:
- Understanding Language: It provides an extensive understanding of how language builds meaning and influences perceptions.
- Uncovering Power Dynamics: The analysis reveals how power dynamics appear via language.
- Cultural Insights: This method identifies cultural norms, beliefs, and ideologies stored in communication.
However, the following challenges may arise:
- Complexity of Interpretation: Language analysis involves navigating multiple levels of nuance and interpretation.
- Subjectivity: Interpretation can be subjective, so controlling researcher bias is important.
- Time-Intensive: Discourse analysis can take a lot of time because careful linguistic study is required in this analysis.
Example of Discourse Analysis
Consider doing discourse analysis on media coverage of a political event. You notice repeating linguistic patterns in news articles that depict the event as a conflict between opposing parties. Through deconstruction, you can expose how this framing supports particular ideologies and power relations.
You can illustrate how language choices influence public perceptions and contribute to building the narrative around the event by analyzing the speech within the broader political and social context. This example shows how discourse analysis can reveal hidden power dynamics and cultural influences on communication.
How to do Qualitative Data Analysis with the QuestionPro Research suite?
QuestionPro is a popular survey and research platform that offers tools for collecting and analyzing qualitative and quantitative data. Follow these general steps for conducting qualitative data analysis using the QuestionPro Research Suite:
- Collect Qualitative Data: Set up your survey to capture qualitative responses. It might involve open-ended questions, text boxes, or comment sections where participants can provide detailed responses.
- Export Qualitative Responses: Export the responses once you’ve collected qualitative data through your survey. QuestionPro typically allows you to export survey data in various formats, such as Excel or CSV.
- Prepare Data for Analysis: Review the exported data and clean it if necessary. Remove irrelevant or duplicate entries to ensure your data is ready for analysis.
- Code and Categorize Responses: Segment and label data, letting new patterns emerge naturally, then develop categories through axial coding to structure the analysis.
- Identify Themes: Analyze the coded responses to identify recurring themes, patterns, and insights. Look for similarities and differences in participants’ responses.
- Generate Reports and Visualizations: Utilize the reporting features of QuestionPro to create visualizations, charts, and graphs that help communicate the themes and findings from your qualitative research.
- Interpret and Draw Conclusions: Interpret the themes and patterns you’ve identified in the qualitative data. Consider how these findings answer your research questions or provide insights into your study topic.
- Integrate with Quantitative Data (if applicable): If you’re also conducting quantitative research using QuestionPro, consider integrating your qualitative findings with quantitative results to provide a more comprehensive understanding.
Qualitative data analysis is vital in uncovering various human experiences, views, and stories. If you’re ready to transform your research journey and apply the power of qualitative analysis, now is the moment to do it. Book a demo with QuestionPro today and begin your journey of exploration.
LEARN MORE FREE TRIAL
MORE LIKE THIS
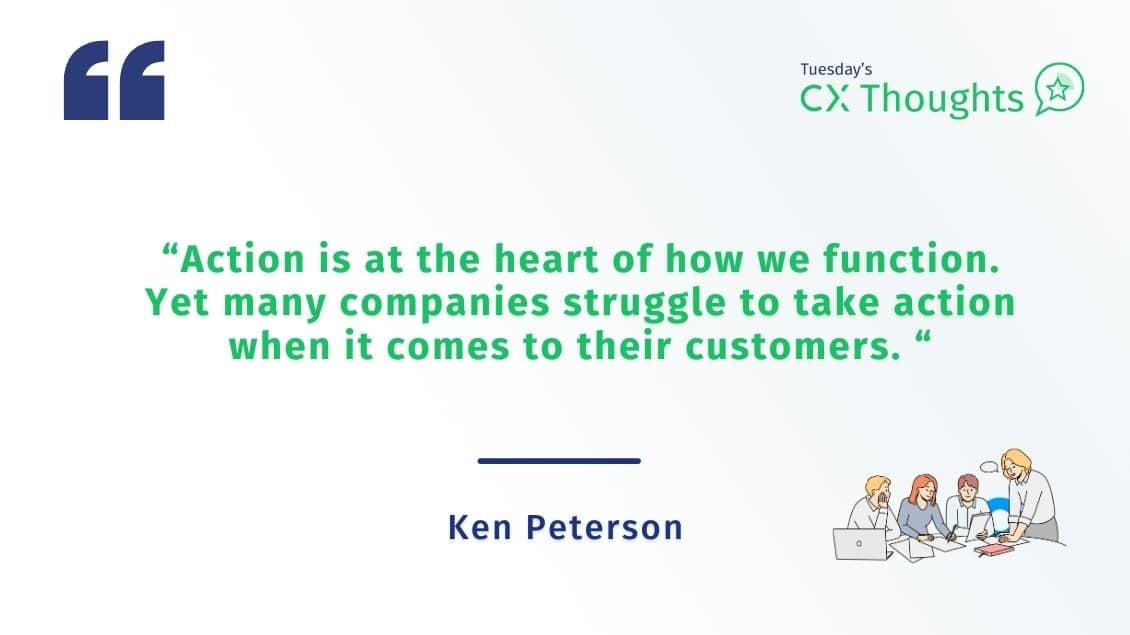
Taking Action in CX – Tuesday CX Thoughts
Apr 30, 2024
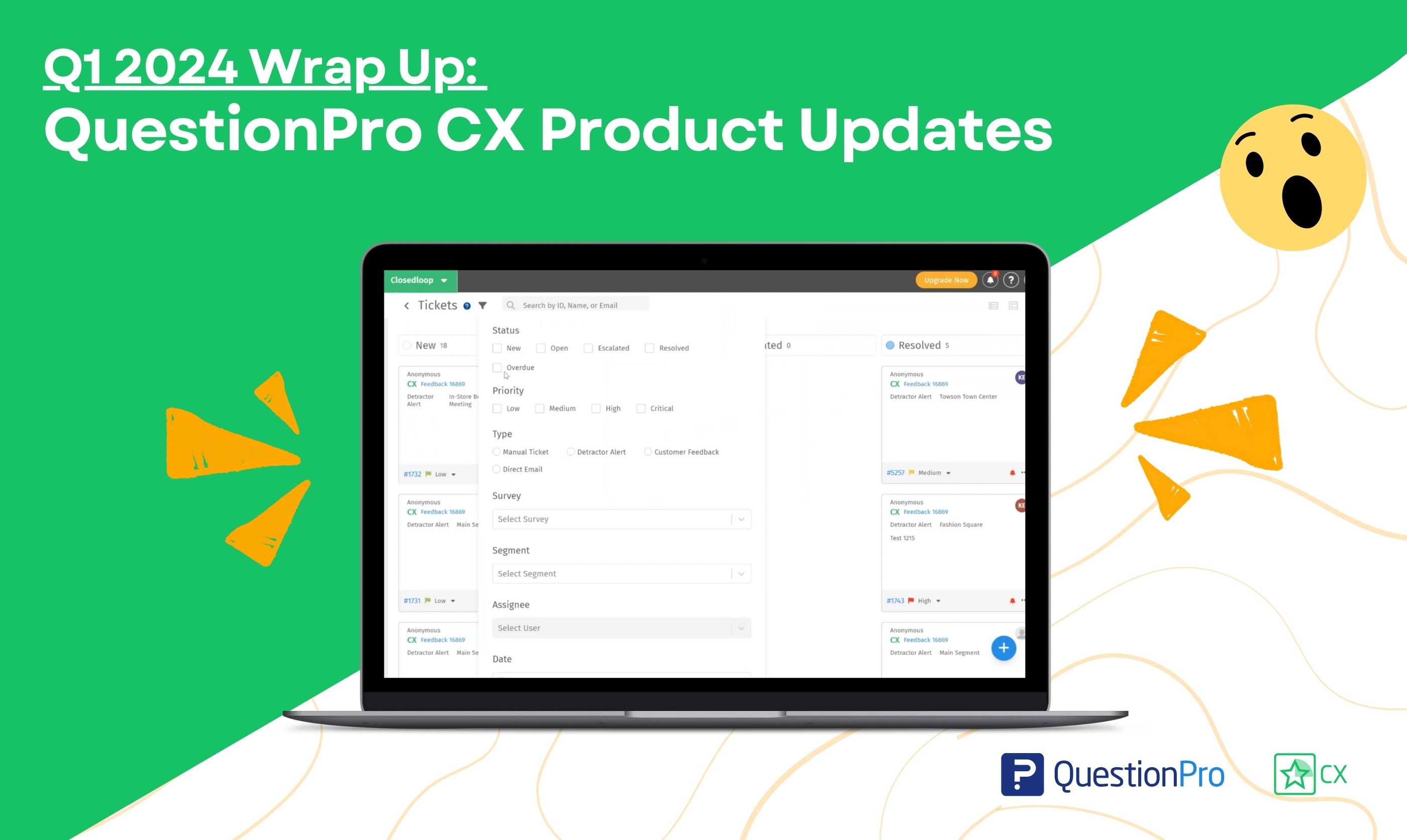
QuestionPro CX Product Updates – Quarter 1, 2024
Apr 29, 2024
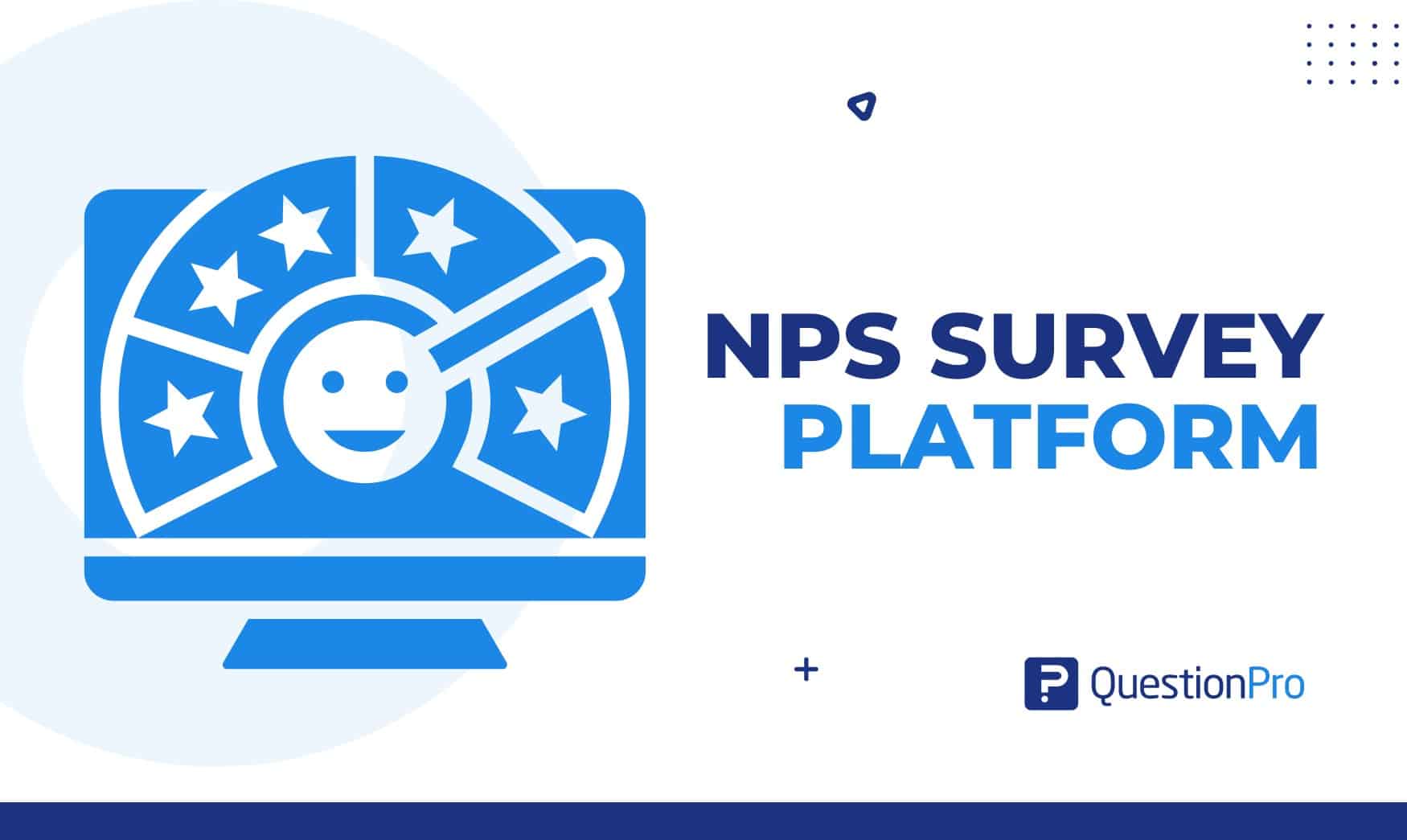
NPS Survey Platform: Types, Tips, 11 Best Platforms & Tools
Apr 26, 2024
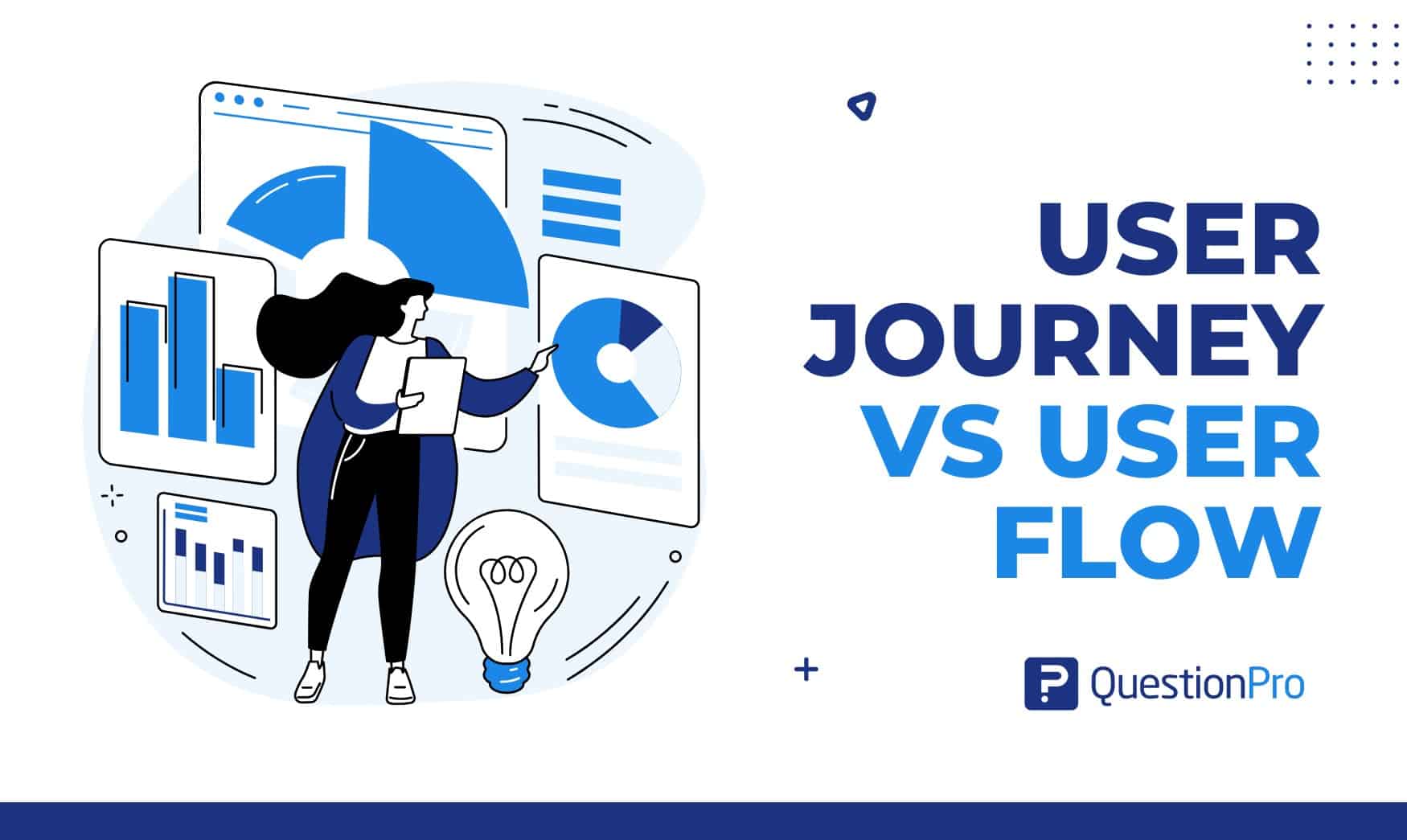
User Journey vs User Flow: Differences and Similarities
Other categories.
- Academic Research
- Artificial Intelligence
- Assessments
- Brand Awareness
- Case Studies
- Communities
- Consumer Insights
- Customer effort score
- Customer Engagement
- Customer Experience
- Customer Loyalty
- Customer Research
- Customer Satisfaction
- Employee Benefits
- Employee Engagement
- Employee Retention
- Friday Five
- General Data Protection Regulation
- Insights Hub
- Life@QuestionPro
- Market Research
- Mobile diaries
- Mobile Surveys
- New Features
- Online Communities
- Question Types
- Questionnaire
- QuestionPro Products
- Release Notes
- Research Tools and Apps
- Revenue at Risk
- Survey Templates
- Training Tips
- Uncategorized
- Video Learning Series
- What’s Coming Up
- Workforce Intelligence
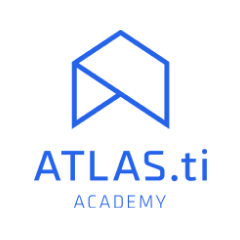
The Ultimate Guide to Qualitative Research - Part 2: Handling Qualitative Data
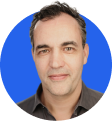
- Handling qualitative data
- Transcripts
- Field notes
- Survey data and responses
- Visual and audio data
- Data organization
- Data coding
- Coding frame
- Auto and smart coding
- Organizing codes
- Introduction
What is qualitative data analysis?
Qualitative data analysis methods, how do you analyze qualitative data, content analysis, thematic analysis.
- Thematic analysis vs. content analysis
- Narrative research
Phenomenological research
Discourse analysis, grounded theory.
- Deductive reasoning
- Inductive reasoning
- Inductive vs. deductive reasoning
- Qualitative data interpretation
- Qualitative analysis software
Qualitative data analysis
Analyzing qualitative data is the next step after you have completed the use of qualitative data collection methods . The qualitative analysis process aims to identify themes and patterns that emerge across the data.
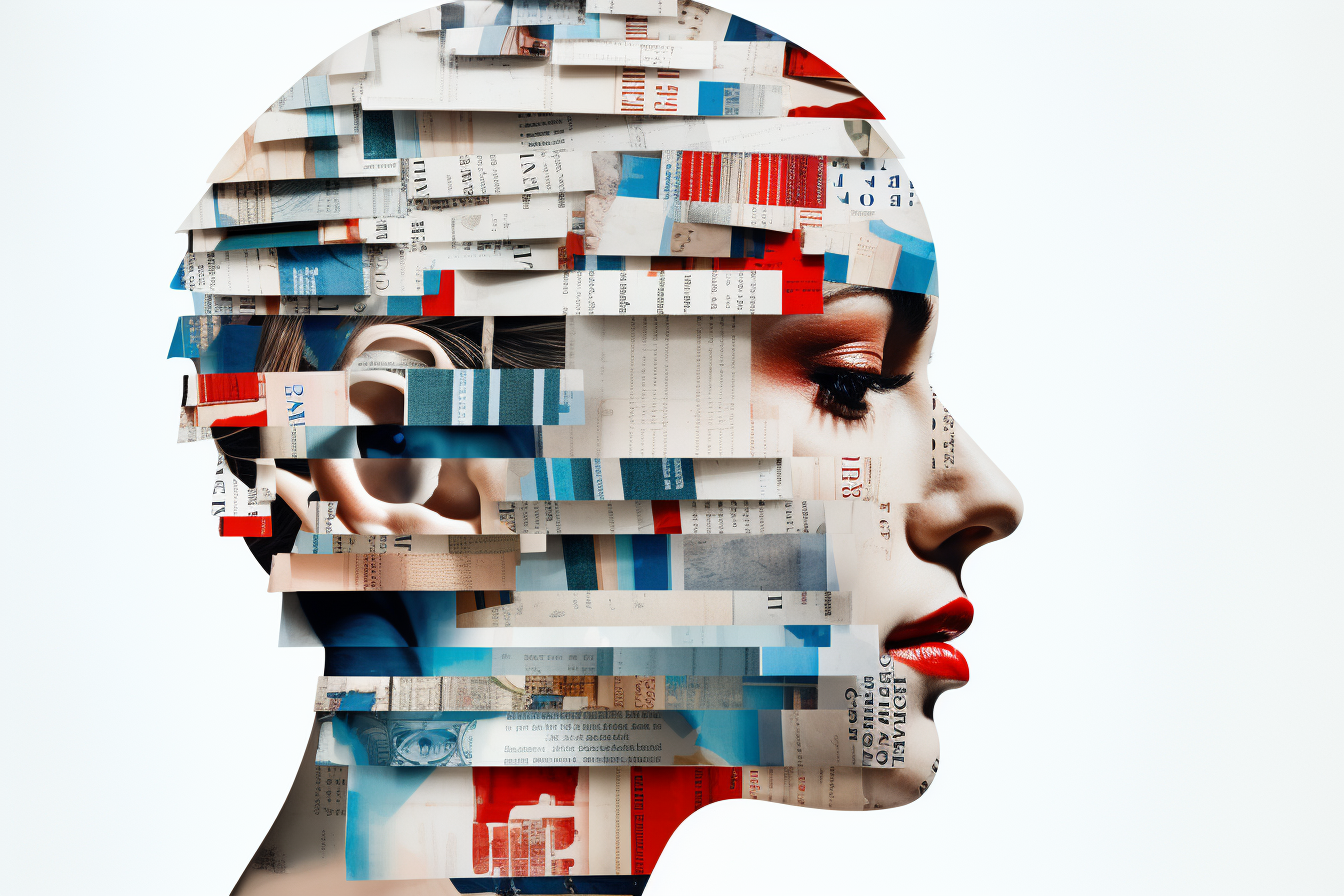
In simplified terms, qualitative research methods involve non-numerical data collection followed by an explanation based on the attributes of the data . For example, if you are asked to explain in qualitative terms a thermal image displayed in multiple colors, then you would explain the color differences rather than the heat's numerical value. If you have a large amount of data (e.g., of group discussions or observations of real-life situations), the next step is to transcribe and prepare the raw data for subsequent analysis.
Researchers can conduct studies fully based on qualitative methodology, or researchers can preface a quantitative research study with a qualitative study to identify issues that were not originally envisioned but are important to the study. Quantitative researchers may also collect and analyze qualitative data following their quantitative analyses to better understand the meanings behind their statistical results.
Conducting qualitative research can especially help build an understanding of how and why certain outcomes were achieved (in addition to what was achieved). For example, qualitative data analysis is often used for policy and program evaluation research since it can answer certain important questions more efficiently and effectively than quantitative approaches.
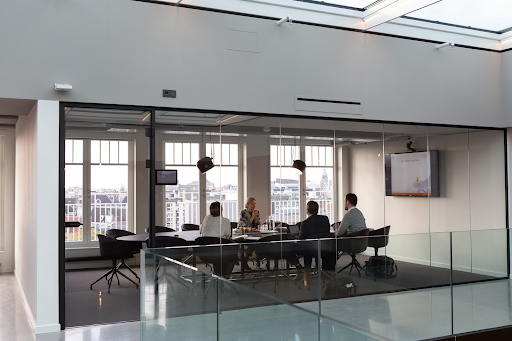
Qualitative data analysis can also answer important questions about the relevance, unintended effects, and impact of programs, such as:
- Were expectations reasonable?
- Did processes operate as expected?
- Were key players able to carry out their duties?
- Were there any unintended effects of the program?
The importance of qualitative data analysis
Qualitative approaches have the advantage of allowing for more diversity in responses and the capacity to adapt to new developments or issues during the research process itself. While qualitative analysis of data can be demanding and time-consuming to conduct, many fields of research utilize qualitative software tools that have been specifically developed to provide more succinct, cost-efficient, and timely results.
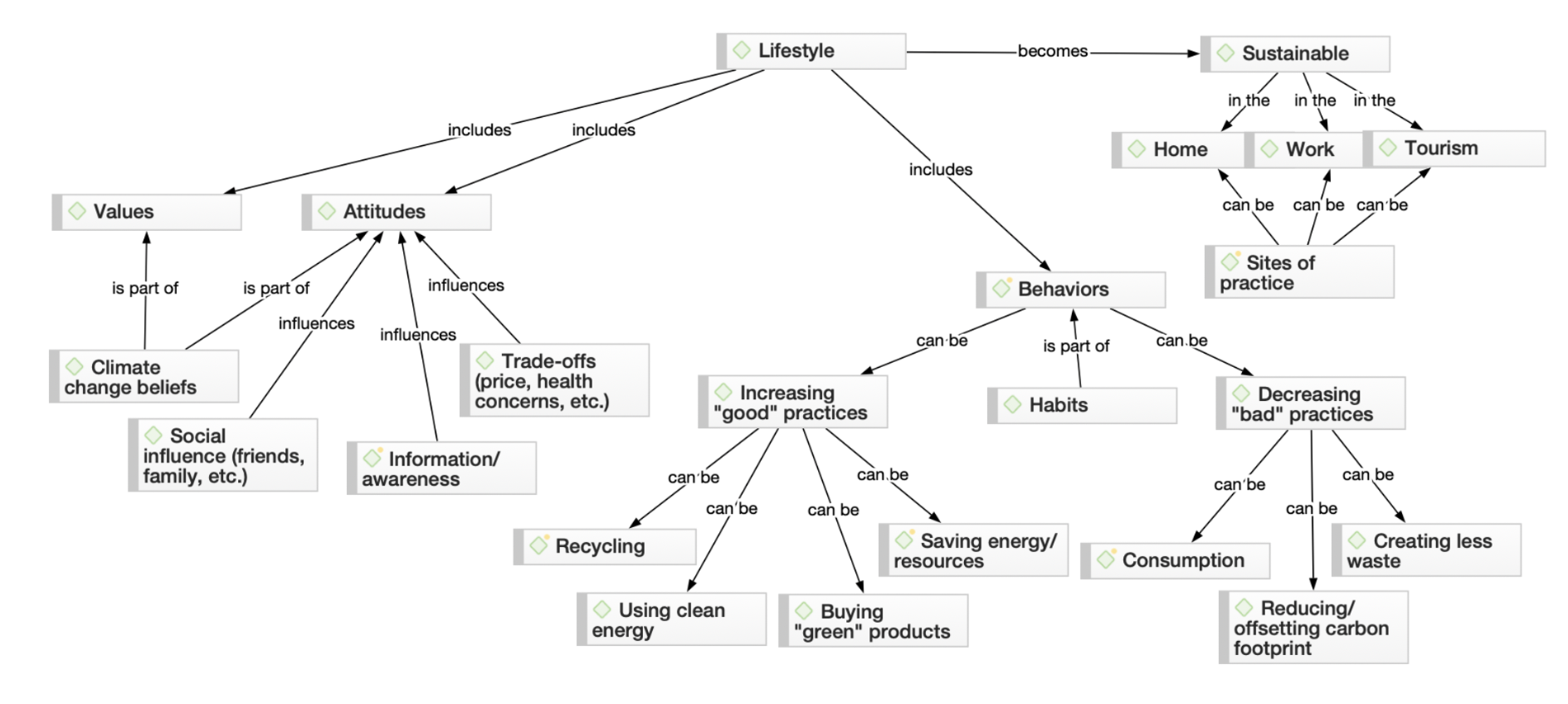
Qualitative data analysis is an important part of research and building greater understanding across fields for a number of reasons. First, cases for qualitative data analysis can be selected purposefully according to whether they typify certain characteristics or contextual locations. In other words, qualitative data permits deep immersion into a topic, phenomenon, or area of interest. Rather than seeking generalizability to the population the sample of participants represent, qualitative research aims to construct an in-depth and nuanced understanding of the research topic.
Secondly, the role or position of the researcher in qualitative analysis of data is given greater critical attention. This is because, in qualitative data analysis, the possibility of the researcher taking a ‘neutral' or transcendent position is seen as more problematic in practical and/or philosophical terms. Hence, qualitative researchers are often exhorted to reflect on their role in the research process and make this clear in the analysis.
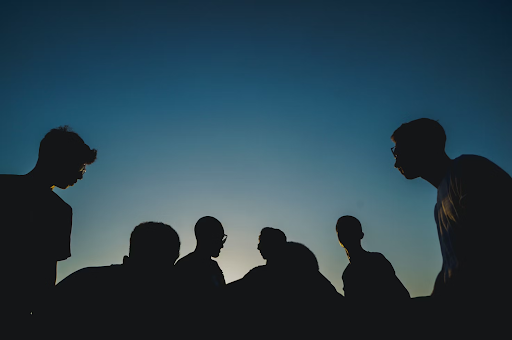
Thirdly, while qualitative data analysis can take a wide variety of forms, it largely differs from quantitative research in the focus on language, signs, experiences, and meaning. In addition, qualitative approaches to analysis are often holistic and contextual rather than analyzing the data in a piecemeal fashion or removing the data from its context. Qualitative approaches thus allow researchers to explore inquiries from directions that could not be accessed with only numerical quantitative data.
Establishing research rigor
Systematic and transparent approaches to the analysis of qualitative data are essential for rigor . For example, many qualitative research methods require researchers to carefully code data and discern and document themes in a consistent and credible way.
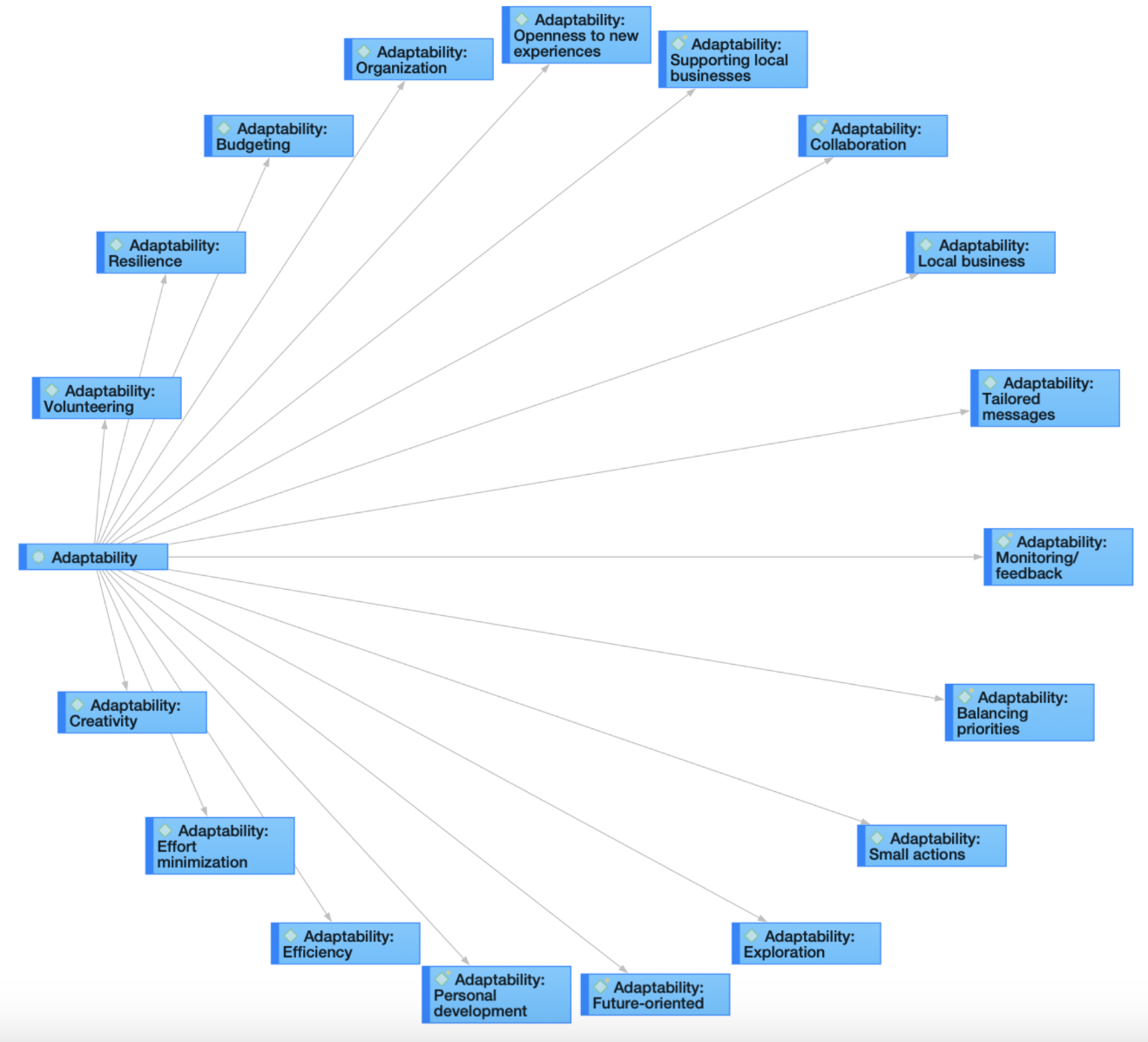
Perhaps the most traditional division in the way qualitative and quantitative research have been used in the social sciences is for qualitative methods to be used for exploratory purposes (e.g., to generate new theory or propositions) or to explain puzzling quantitative results, while quantitative methods are used to test hypotheses .
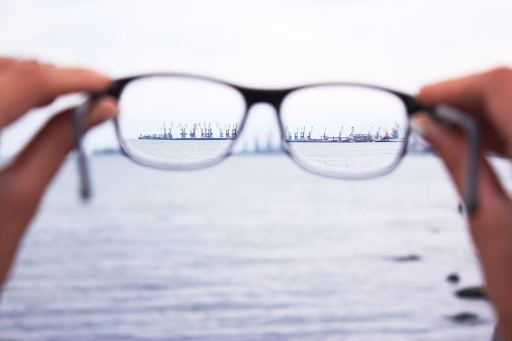
After you’ve collected relevant data , what is the best way to look at your data ? As always, it will depend on your research question . For instance, if you employed an observational research method to learn about a group’s shared practices, an ethnographic approach could be appropriate to explain the various dimensions of culture. If you collected textual data to understand how people talk about something, then a discourse analysis approach might help you generate key insights about language and communication.
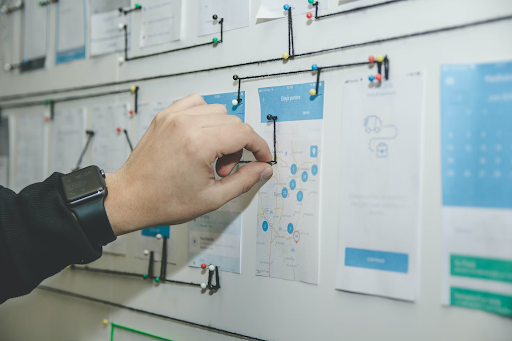
The qualitative data coding process involves iterative categorization and recategorization, ensuring the evolution of the analysis to best represent the data. The procedure typically concludes with the interpretation of patterns and trends identified through the coding process.
To start off, let’s look at two broad approaches to data analysis.
Deductive analysis
Deductive analysis is guided by pre-existing theories or ideas. It starts with a theoretical framework , which is then used to code the data. The researcher can thus use this theoretical framework to interpret their data and answer their research question .
The key steps include coding the data based on the predetermined concepts or categories and using the theory to guide the interpretation of patterns among the codings. Deductive analysis is particularly useful when researchers aim to verify or extend an existing theory within a new context.
Inductive analysis
Inductive analysis involves the generation of new theories or ideas based on the data. The process starts without any preconceived theories or codes, and patterns, themes, and categories emerge out of the data.
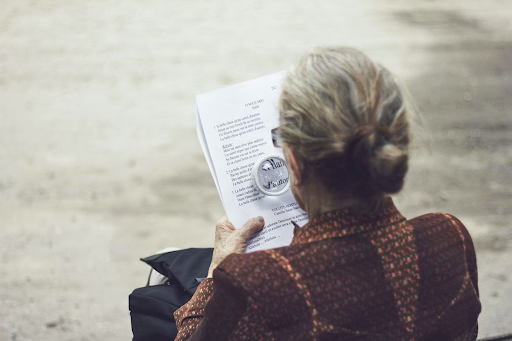
The researcher codes the data to capture any concepts or patterns that seem interesting or important to the research question . These codes are then compared and linked, leading to the formation of broader categories or themes. The main goal of inductive analysis is to allow the data to 'speak for itself' rather than imposing pre-existing expectations or ideas onto the data.
Deductive and inductive approaches can be seen as sitting on opposite poles, and all research falls somewhere within that spectrum. Most often, qualitative analysis approaches blend both deductive and inductive elements to contribute to the existing conversation around a topic while remaining open to potential unexpected findings. To help you make informed decisions about which qualitative data analysis approach fits with your research objectives, let's look at some of the common approaches for qualitative data analysis.
Content analysis is a research method used to identify patterns and themes within qualitative data. This approach involves systematically coding and categorizing specific aspects of the content in the data to uncover trends and patterns. An often important part of content analysis is quantifying frequencies and patterns of words or characteristics present in the data .
It is a highly flexible technique that can be adapted to various data types , including text, images, and audiovisual content . While content analysis can be exploratory in nature, it is also common to use pre-established theories and follow a more deductive approach to categorizing and quantifying the qualitative data.
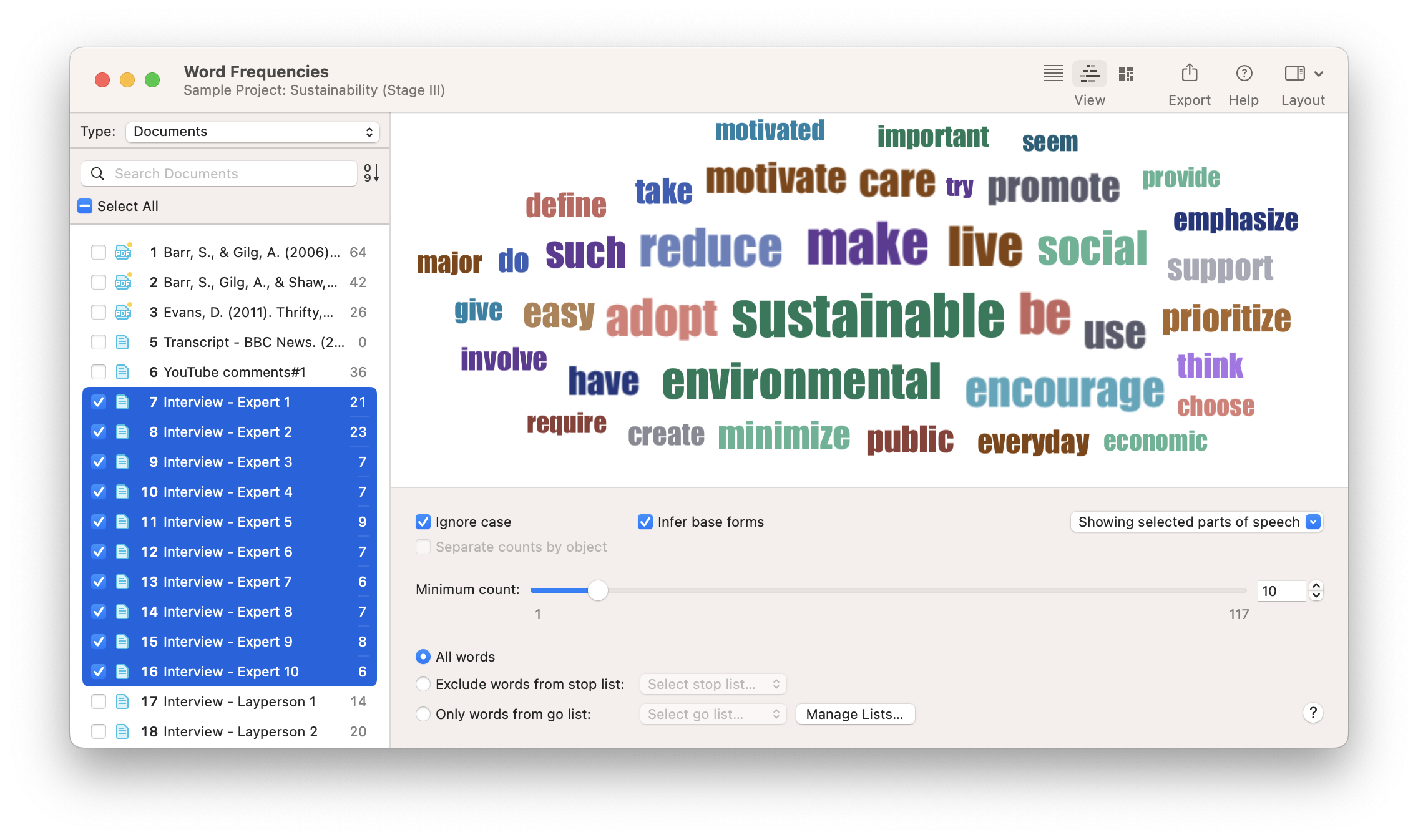
Thematic analysis is a method used to identify, analyze, and report patterns or themes within the data. This approach moves beyond counting explicit words or phrases and focuses on also identifying implicit concepts and themes within the data.
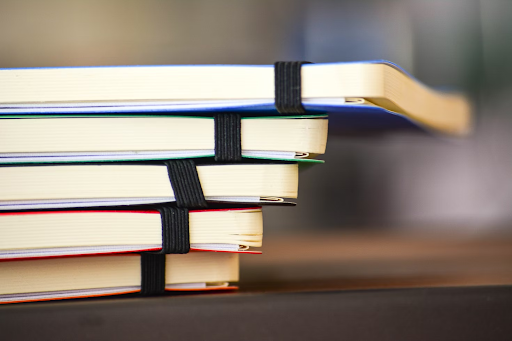
Researchers conduct detailed coding of the data to ascertain repeated themes or patterns of meaning. Codes can be categorized into themes, and the researcher can analyze how the themes relate to one another. Thematic analysis is flexible in terms of the research framework, allowing for both inductive (data-driven) and deductive (theory-driven) approaches. The outcome is a rich, detailed, and complex account of the data.
Grounded theory is a systematic qualitative research methodology that is used to inductively generate theory that is 'grounded' in the data itself. Analysis takes place simultaneously with data collection , and researchers iterate between data collection and analysis until a comprehensive theory is developed.
Grounded theory is characterized by simultaneous data collection and analysis, the development of theoretical codes from the data, purposeful sampling of participants, and the constant comparison of data with emerging categories and concepts. The ultimate goal is to create a theoretical explanation that fits the data and answers the research question .
Discourse analysis is a qualitative research approach that emphasizes the role of language in social contexts. It involves examining communication and language use beyond the level of the sentence, considering larger units of language such as texts or conversations.
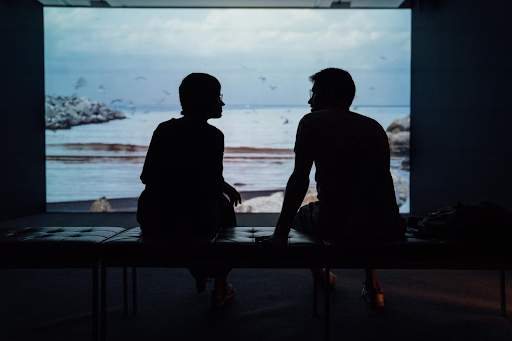
Discourse analysts typically investigate how social meanings and understandings are constructed in different contexts, emphasizing the connection between language and power. It can be applied to texts of all kinds, including interviews , documents, case studies , and social media posts.
Phenomenological research focuses on exploring how human beings make sense of an experience and delves into the essence of this experience. It strives to understand people's perceptions, perspectives, and understandings of a particular situation or phenomenon.
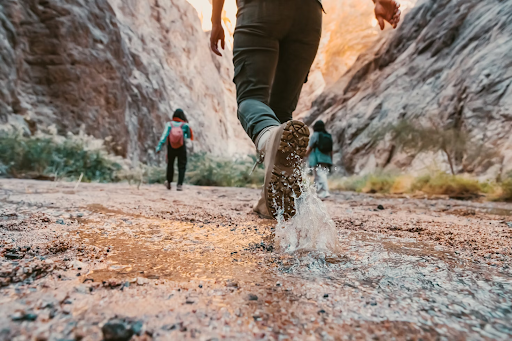
It involves in-depth engagement with participants, often through interviews or conversations, to explore their lived experiences. The goal is to derive detailed descriptions of the essence of the experience and to interpret what insights or implications this may bear on our understanding of this phenomenon.
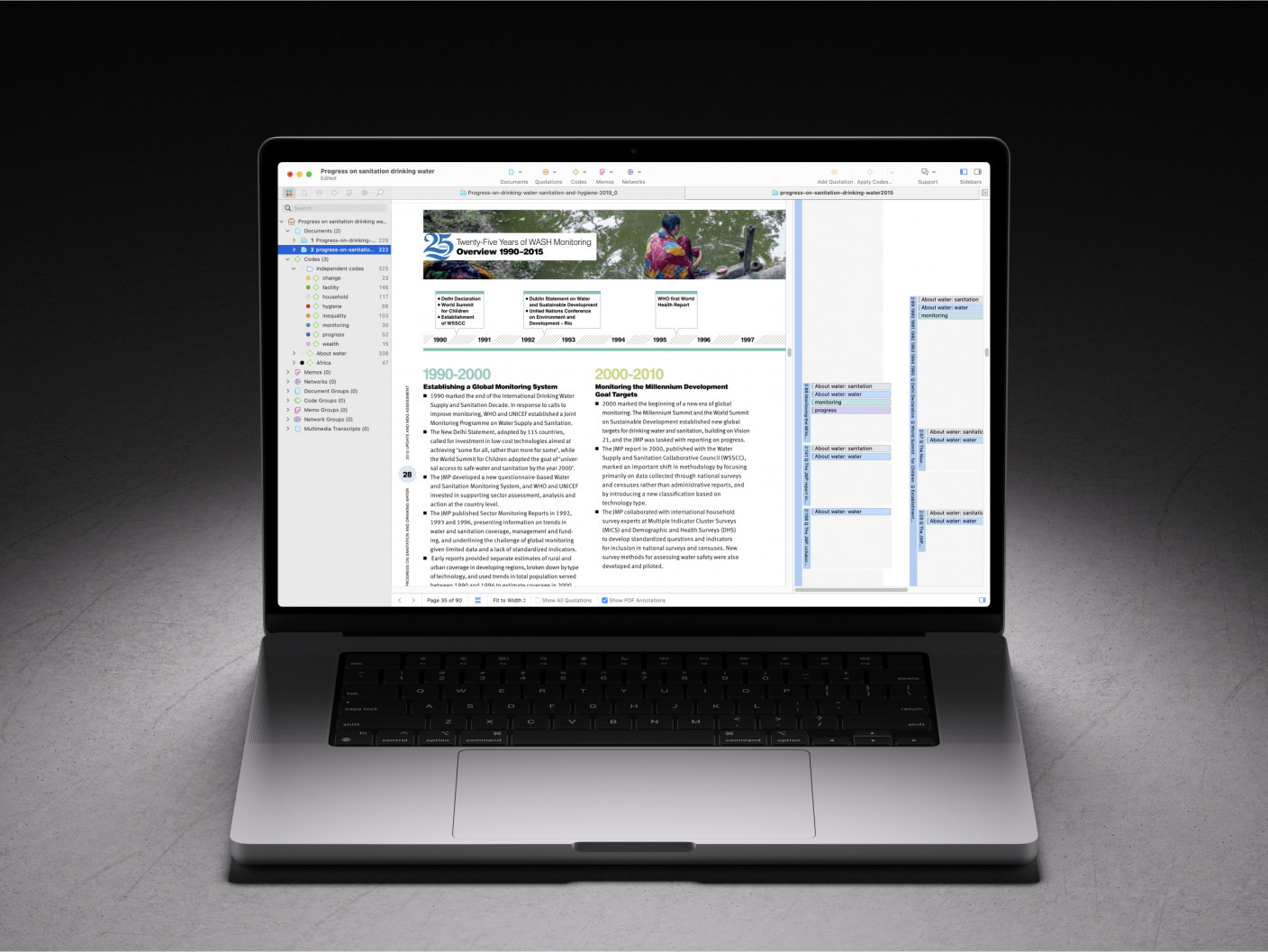
Whatever your data analysis approach, start with ATLAS.ti
Qualitative data analysis done quickly and intuitively with ATLAS.ti. Download a free trial today.
Now that we've summarized the major approaches to data analysis, let's look at the broader process of research and data analysis. Suppose you need to do some research to find answers to any kind of research question, be it an academic inquiry, business problem, or policy decision. In that case, you need to collect some data. There are many methods of collecting data: you can collect primary data yourself by conducting interviews, focus groups , or a survey , for instance. Another option is to use secondary data sources. These are data previously collected for other projects, historical records, reports, statistics – basically everything that exists already and can be relevant to your research.
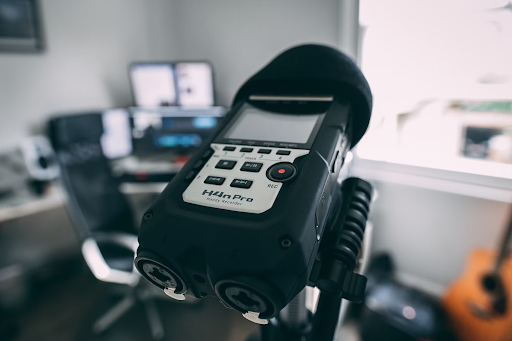
The data you collect should always be a good fit for your research question . For example, if you are interested in how many people in your target population like your brand compared to others, it is no use to conduct interviews or a few focus groups . The sample will be too small to get a representative picture of the population. If your questions are about "how many….", "what is the spread…" etc., you need to conduct quantitative research . If you are interested in why people like different brands, their motives, and their experiences, then conducting qualitative research can provide you with the answers you are looking for.
Let's describe the important steps involved in conducting research.
Step 1: Planning the research
As the saying goes: "Garbage in, garbage out." Suppose you find out after you have collected data that
- you talked to the wrong people
- asked the wrong questions
- a couple of focus groups sessions would have yielded better results because of the group interaction, or
- a survey including a few open-ended questions sent to a larger group of people would have been sufficient and required less effort.
Think thoroughly about sampling, the questions you will be asking, and in which form. If you conduct a focus group or an interview, you are the research instrument, and your data collection will only be as good as you are. If you have never done it before, seek some training and practice. If you have other people do it, make sure they have the skills.

Step 2: Preparing the data
When you conduct focus groups or interviews, think about how to transcribe them. Do you want to run them online or offline? If online, check out which tools can serve your needs, both in terms of functionality and cost. For any audio or video recordings , you can consider using automatic transcription software or services. Automatically generated transcripts can save you time and money, but they still need to be checked. If you don't do this yourself, make sure that you instruct the person doing it on how to prepare the data.
- How should the final transcript be formatted for later analysis?
- Which names and locations should be anonymized?
- What kind of speaker IDs to use?
What about survey data ? Some survey data programs will immediately provide basic descriptive-level analysis of the responses. ATLAS.ti will support you with the analysis of the open-ended questions. For this, you need to export your data as an Excel file. ATLAS.ti's survey import wizard will guide you through the process.
Other kinds of data such as images, videos, audio recordings, text, and more can be imported to ATLAS.ti. You can organize all your data into groups and write comments on each source of data to maintain a systematic organization and documentation of your data.
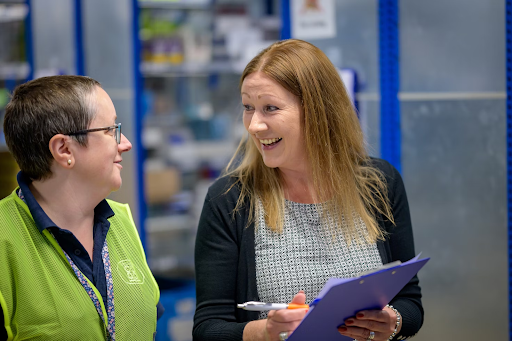
Step 3: Exploratory data analysis
You can run a few simple exploratory analyses to get to know your data. For instance, you can create a word list or word cloud of all your text data or compare and contrast the words in different documents. You can also let ATLAS.ti find relevant concepts for you. There are many tools available that can automatically code your text data, so you can also use these codings to explore your data and refine your coding.
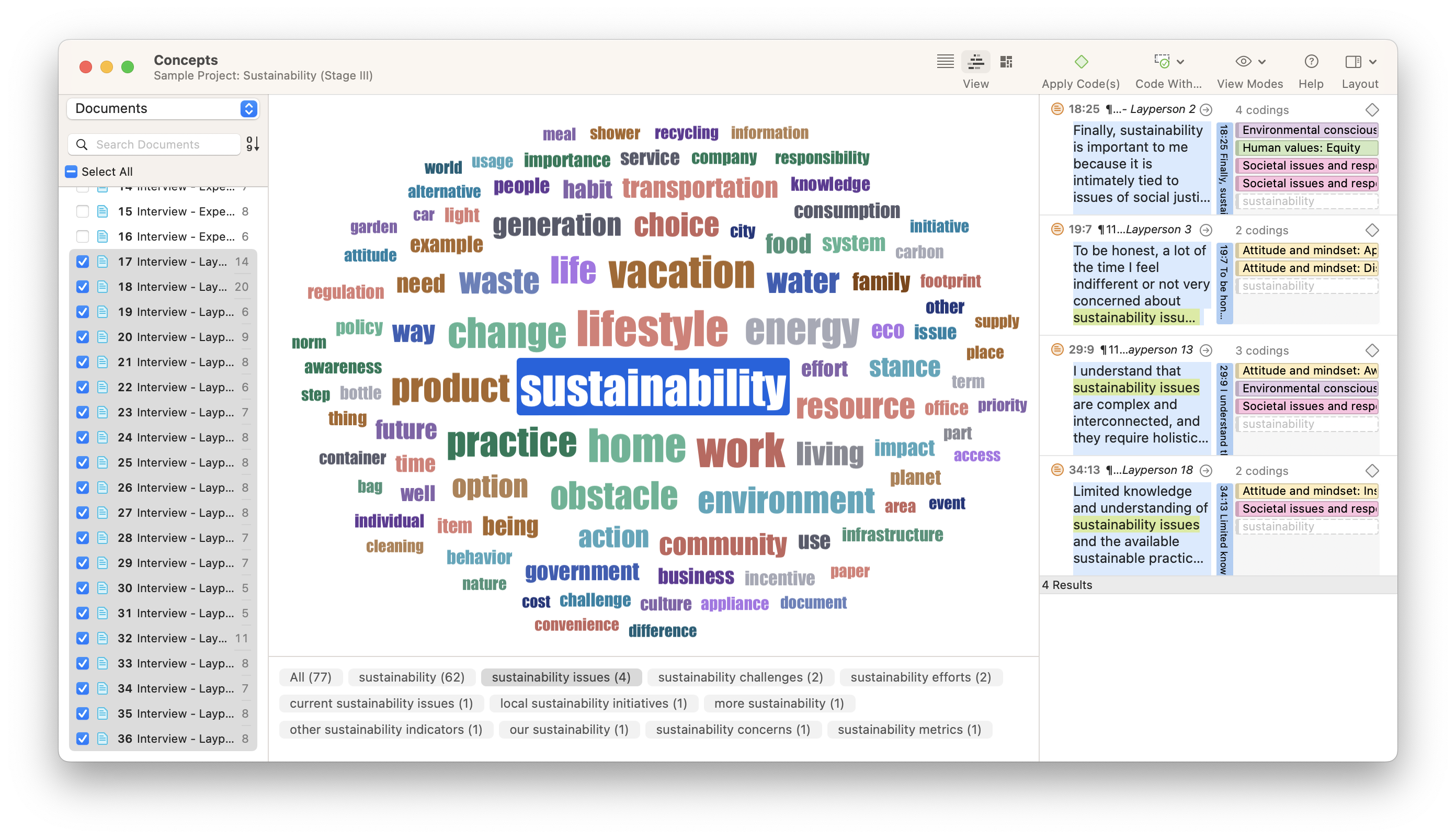
For instance, you can get a feeling for the sentiments expressed in the data. Who is more optimistic, pessimistic, or neutral in their responses? ATLAS.ti can auto-code the positive, negative, and neutral sentiments in your data. Naturally, you can also simply browse through your data and highlight relevant segments that catch your attention or attach codes to begin condensing the data.
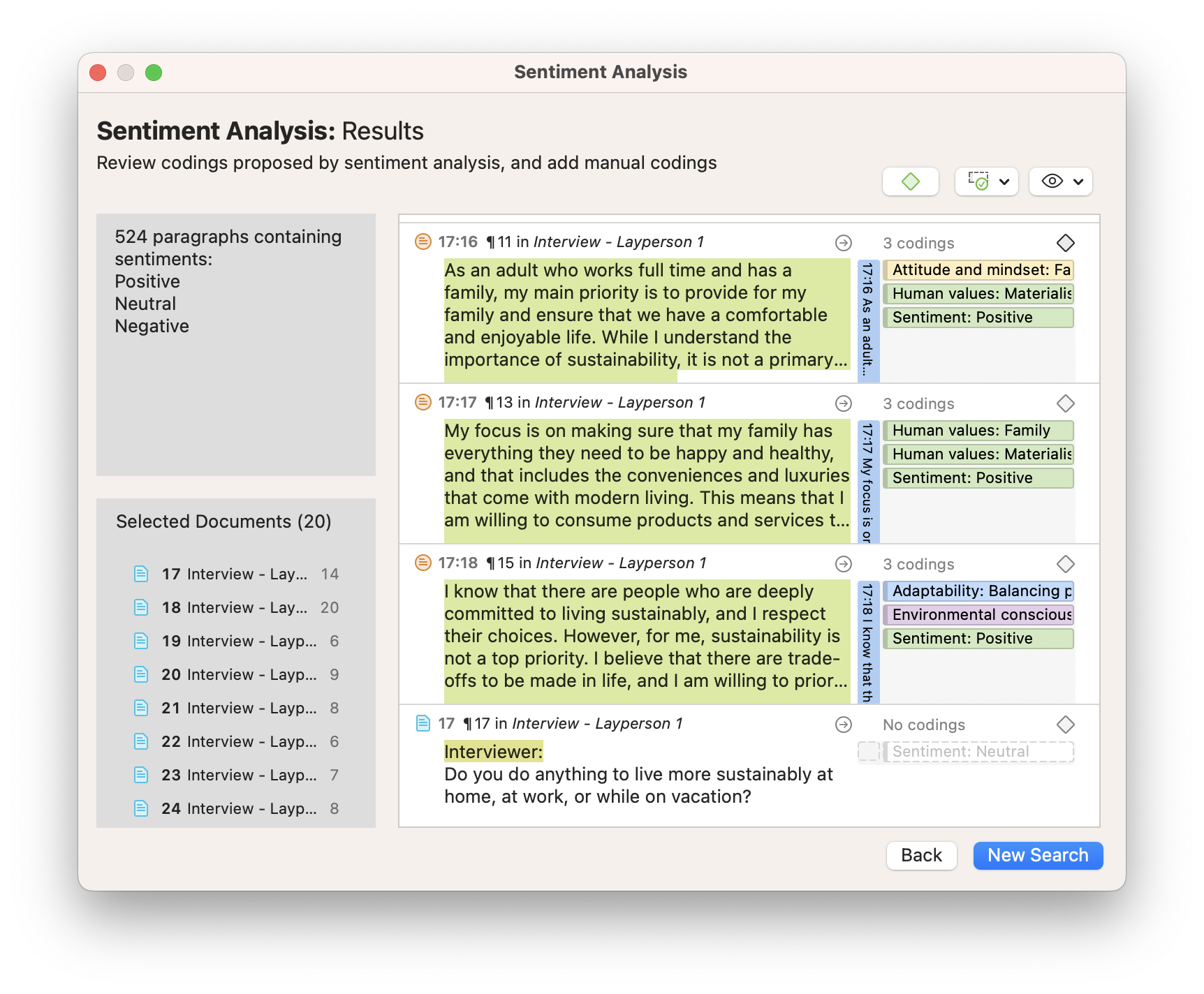
Step 4: Build a code system
Whether you start with auto-coding or manual coding, after having generated some first codes, you need to get some order in your code system to develop a cohesive understanding. You can build your code system by sorting codes into groups and creating categories and subcodes. As this process requires reading and re-reading your data, you will become very familiar with your data. Counting on a tool like ATLAS.ti qualitative data analysis software will support you in the process and make it easier to review your data, modify codings if necessary, change code labels, and write operational definitions to explain what each code means.
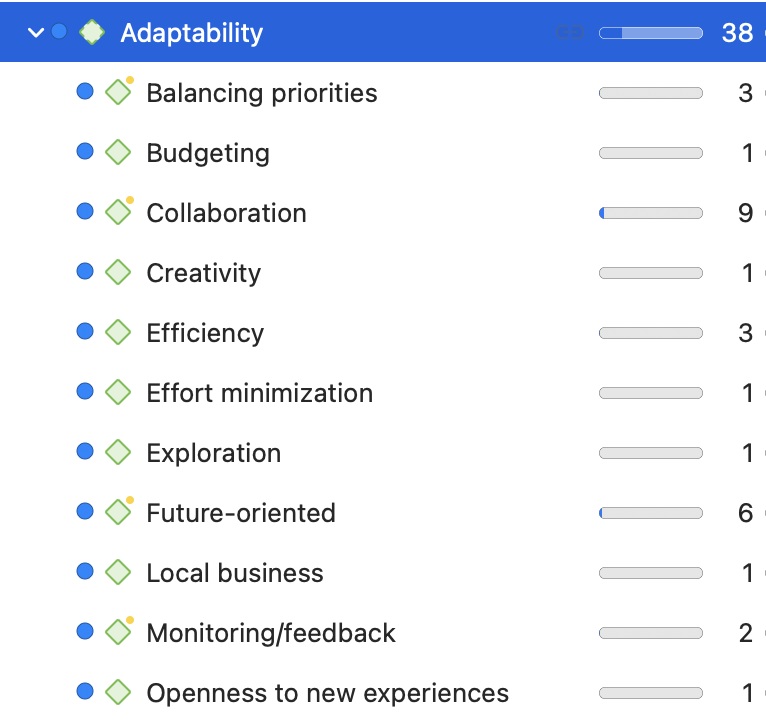
Step 5: Query your coded data and write up the analysis
Once you have coded your data, it is time to take the analysis a step further. When using software for qualitative data analysis , it is easy to compare and contrast subsets in your data, such as groups of participants or sets of themes.

For instance, you can query the various opinions of female vs. male respondents. Is there a difference between consumers from rural or urban areas or among different age groups or educational levels? Which codes occur together throughout the data set? Are there relationships between various concepts, and if so, why?
Step 6: Data visualization
Data visualization brings your data to life. It is a powerful way of seeing patterns and relationships in your data. For instance, diagrams allow you to see how your codes are distributed across documents or specific subpopulations in your data.
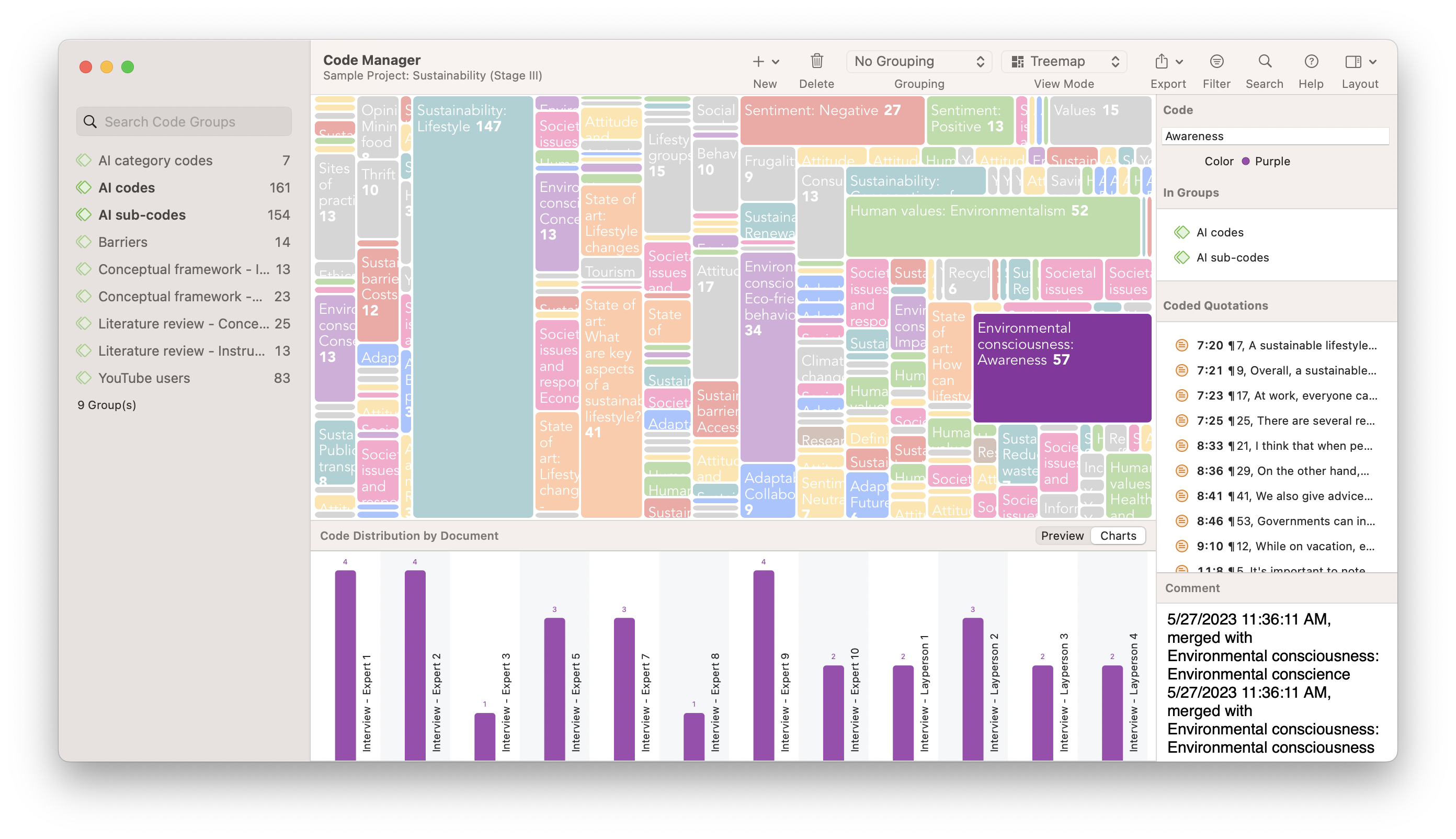
Exploring coded data on a canvas, moving around code labels in a virtual space, linking codes and other elements of your data set, and thinking about how they are related and why – all of these will advance your analysis and spur further insights. Visuals are also great for communicating results to others.
Step 7: Data presentation
The final step is to summarize the analysis in a written report . You can now put together the memos you have written about the various topics, select some salient quotes that illustrate your writing, and add visuals such as tables and diagrams. If you follow the steps above, you will already have all the building blocks, and you just have to put them together in a report or presentation.
When preparing a report or a presentation, keep your audience in mind. Does your audience better understand numbers than long sections of detailed interpretations? If so, add more tables, charts, and short supportive data quotes to your report or presentation. If your audience loves a good interpretation, add your full-length memos and walk your audience through your conceptual networks and illustrative data quotes.
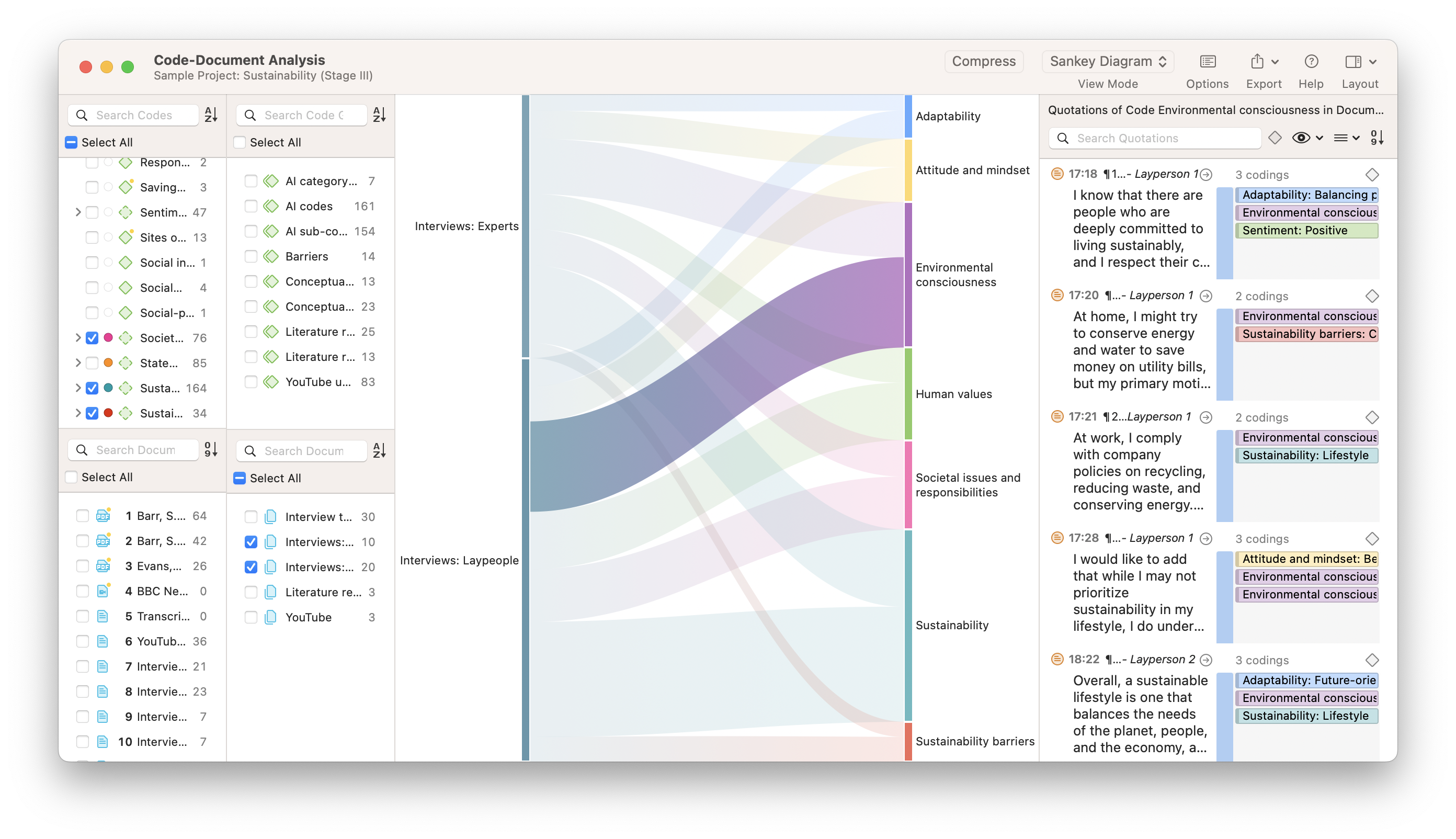
Qualitative data analysis begins with ATLAS.ti
For tools that can make the most out of your data, check out ATLAS.ti with a free trial.
- Privacy Policy

Home » Qualitative Research – Methods, Analysis Types and Guide
Qualitative Research – Methods, Analysis Types and Guide
Table of Contents

Qualitative Research
Qualitative research is a type of research methodology that focuses on exploring and understanding people’s beliefs, attitudes, behaviors, and experiences through the collection and analysis of non-numerical data. It seeks to answer research questions through the examination of subjective data, such as interviews, focus groups, observations, and textual analysis.
Qualitative research aims to uncover the meaning and significance of social phenomena, and it typically involves a more flexible and iterative approach to data collection and analysis compared to quantitative research. Qualitative research is often used in fields such as sociology, anthropology, psychology, and education.
Qualitative Research Methods

Qualitative Research Methods are as follows:
One-to-One Interview
This method involves conducting an interview with a single participant to gain a detailed understanding of their experiences, attitudes, and beliefs. One-to-one interviews can be conducted in-person, over the phone, or through video conferencing. The interviewer typically uses open-ended questions to encourage the participant to share their thoughts and feelings. One-to-one interviews are useful for gaining detailed insights into individual experiences.
Focus Groups
This method involves bringing together a group of people to discuss a specific topic in a structured setting. The focus group is led by a moderator who guides the discussion and encourages participants to share their thoughts and opinions. Focus groups are useful for generating ideas and insights, exploring social norms and attitudes, and understanding group dynamics.
Ethnographic Studies
This method involves immersing oneself in a culture or community to gain a deep understanding of its norms, beliefs, and practices. Ethnographic studies typically involve long-term fieldwork and observation, as well as interviews and document analysis. Ethnographic studies are useful for understanding the cultural context of social phenomena and for gaining a holistic understanding of complex social processes.
Text Analysis
This method involves analyzing written or spoken language to identify patterns and themes. Text analysis can be quantitative or qualitative. Qualitative text analysis involves close reading and interpretation of texts to identify recurring themes, concepts, and patterns. Text analysis is useful for understanding media messages, public discourse, and cultural trends.
This method involves an in-depth examination of a single person, group, or event to gain an understanding of complex phenomena. Case studies typically involve a combination of data collection methods, such as interviews, observations, and document analysis, to provide a comprehensive understanding of the case. Case studies are useful for exploring unique or rare cases, and for generating hypotheses for further research.
Process of Observation
This method involves systematically observing and recording behaviors and interactions in natural settings. The observer may take notes, use audio or video recordings, or use other methods to document what they see. Process of observation is useful for understanding social interactions, cultural practices, and the context in which behaviors occur.
Record Keeping
This method involves keeping detailed records of observations, interviews, and other data collected during the research process. Record keeping is essential for ensuring the accuracy and reliability of the data, and for providing a basis for analysis and interpretation.
This method involves collecting data from a large sample of participants through a structured questionnaire. Surveys can be conducted in person, over the phone, through mail, or online. Surveys are useful for collecting data on attitudes, beliefs, and behaviors, and for identifying patterns and trends in a population.
Qualitative data analysis is a process of turning unstructured data into meaningful insights. It involves extracting and organizing information from sources like interviews, focus groups, and surveys. The goal is to understand people’s attitudes, behaviors, and motivations
Qualitative Research Analysis Methods
Qualitative Research analysis methods involve a systematic approach to interpreting and making sense of the data collected in qualitative research. Here are some common qualitative data analysis methods:
Thematic Analysis
This method involves identifying patterns or themes in the data that are relevant to the research question. The researcher reviews the data, identifies keywords or phrases, and groups them into categories or themes. Thematic analysis is useful for identifying patterns across multiple data sources and for generating new insights into the research topic.
Content Analysis
This method involves analyzing the content of written or spoken language to identify key themes or concepts. Content analysis can be quantitative or qualitative. Qualitative content analysis involves close reading and interpretation of texts to identify recurring themes, concepts, and patterns. Content analysis is useful for identifying patterns in media messages, public discourse, and cultural trends.
Discourse Analysis
This method involves analyzing language to understand how it constructs meaning and shapes social interactions. Discourse analysis can involve a variety of methods, such as conversation analysis, critical discourse analysis, and narrative analysis. Discourse analysis is useful for understanding how language shapes social interactions, cultural norms, and power relationships.
Grounded Theory Analysis
This method involves developing a theory or explanation based on the data collected. Grounded theory analysis starts with the data and uses an iterative process of coding and analysis to identify patterns and themes in the data. The theory or explanation that emerges is grounded in the data, rather than preconceived hypotheses. Grounded theory analysis is useful for understanding complex social phenomena and for generating new theoretical insights.
Narrative Analysis
This method involves analyzing the stories or narratives that participants share to gain insights into their experiences, attitudes, and beliefs. Narrative analysis can involve a variety of methods, such as structural analysis, thematic analysis, and discourse analysis. Narrative analysis is useful for understanding how individuals construct their identities, make sense of their experiences, and communicate their values and beliefs.
Phenomenological Analysis
This method involves analyzing how individuals make sense of their experiences and the meanings they attach to them. Phenomenological analysis typically involves in-depth interviews with participants to explore their experiences in detail. Phenomenological analysis is useful for understanding subjective experiences and for developing a rich understanding of human consciousness.
Comparative Analysis
This method involves comparing and contrasting data across different cases or groups to identify similarities and differences. Comparative analysis can be used to identify patterns or themes that are common across multiple cases, as well as to identify unique or distinctive features of individual cases. Comparative analysis is useful for understanding how social phenomena vary across different contexts and groups.
Applications of Qualitative Research
Qualitative research has many applications across different fields and industries. Here are some examples of how qualitative research is used:
- Market Research: Qualitative research is often used in market research to understand consumer attitudes, behaviors, and preferences. Researchers conduct focus groups and one-on-one interviews with consumers to gather insights into their experiences and perceptions of products and services.
- Health Care: Qualitative research is used in health care to explore patient experiences and perspectives on health and illness. Researchers conduct in-depth interviews with patients and their families to gather information on their experiences with different health care providers and treatments.
- Education: Qualitative research is used in education to understand student experiences and to develop effective teaching strategies. Researchers conduct classroom observations and interviews with students and teachers to gather insights into classroom dynamics and instructional practices.
- Social Work : Qualitative research is used in social work to explore social problems and to develop interventions to address them. Researchers conduct in-depth interviews with individuals and families to understand their experiences with poverty, discrimination, and other social problems.
- Anthropology : Qualitative research is used in anthropology to understand different cultures and societies. Researchers conduct ethnographic studies and observe and interview members of different cultural groups to gain insights into their beliefs, practices, and social structures.
- Psychology : Qualitative research is used in psychology to understand human behavior and mental processes. Researchers conduct in-depth interviews with individuals to explore their thoughts, feelings, and experiences.
- Public Policy : Qualitative research is used in public policy to explore public attitudes and to inform policy decisions. Researchers conduct focus groups and one-on-one interviews with members of the public to gather insights into their perspectives on different policy issues.
How to Conduct Qualitative Research
Here are some general steps for conducting qualitative research:
- Identify your research question: Qualitative research starts with a research question or set of questions that you want to explore. This question should be focused and specific, but also broad enough to allow for exploration and discovery.
- Select your research design: There are different types of qualitative research designs, including ethnography, case study, grounded theory, and phenomenology. You should select a design that aligns with your research question and that will allow you to gather the data you need to answer your research question.
- Recruit participants: Once you have your research question and design, you need to recruit participants. The number of participants you need will depend on your research design and the scope of your research. You can recruit participants through advertisements, social media, or through personal networks.
- Collect data: There are different methods for collecting qualitative data, including interviews, focus groups, observation, and document analysis. You should select the method or methods that align with your research design and that will allow you to gather the data you need to answer your research question.
- Analyze data: Once you have collected your data, you need to analyze it. This involves reviewing your data, identifying patterns and themes, and developing codes to organize your data. You can use different software programs to help you analyze your data, or you can do it manually.
- Interpret data: Once you have analyzed your data, you need to interpret it. This involves making sense of the patterns and themes you have identified, and developing insights and conclusions that answer your research question. You should be guided by your research question and use your data to support your conclusions.
- Communicate results: Once you have interpreted your data, you need to communicate your results. This can be done through academic papers, presentations, or reports. You should be clear and concise in your communication, and use examples and quotes from your data to support your findings.
Examples of Qualitative Research
Here are some real-time examples of qualitative research:
- Customer Feedback: A company may conduct qualitative research to understand the feedback and experiences of its customers. This may involve conducting focus groups or one-on-one interviews with customers to gather insights into their attitudes, behaviors, and preferences.
- Healthcare : A healthcare provider may conduct qualitative research to explore patient experiences and perspectives on health and illness. This may involve conducting in-depth interviews with patients and their families to gather information on their experiences with different health care providers and treatments.
- Education : An educational institution may conduct qualitative research to understand student experiences and to develop effective teaching strategies. This may involve conducting classroom observations and interviews with students and teachers to gather insights into classroom dynamics and instructional practices.
- Social Work: A social worker may conduct qualitative research to explore social problems and to develop interventions to address them. This may involve conducting in-depth interviews with individuals and families to understand their experiences with poverty, discrimination, and other social problems.
- Anthropology : An anthropologist may conduct qualitative research to understand different cultures and societies. This may involve conducting ethnographic studies and observing and interviewing members of different cultural groups to gain insights into their beliefs, practices, and social structures.
- Psychology : A psychologist may conduct qualitative research to understand human behavior and mental processes. This may involve conducting in-depth interviews with individuals to explore their thoughts, feelings, and experiences.
- Public Policy: A government agency or non-profit organization may conduct qualitative research to explore public attitudes and to inform policy decisions. This may involve conducting focus groups and one-on-one interviews with members of the public to gather insights into their perspectives on different policy issues.
Purpose of Qualitative Research
The purpose of qualitative research is to explore and understand the subjective experiences, behaviors, and perspectives of individuals or groups in a particular context. Unlike quantitative research, which focuses on numerical data and statistical analysis, qualitative research aims to provide in-depth, descriptive information that can help researchers develop insights and theories about complex social phenomena.
Qualitative research can serve multiple purposes, including:
- Exploring new or emerging phenomena : Qualitative research can be useful for exploring new or emerging phenomena, such as new technologies or social trends. This type of research can help researchers develop a deeper understanding of these phenomena and identify potential areas for further study.
- Understanding complex social phenomena : Qualitative research can be useful for exploring complex social phenomena, such as cultural beliefs, social norms, or political processes. This type of research can help researchers develop a more nuanced understanding of these phenomena and identify factors that may influence them.
- Generating new theories or hypotheses: Qualitative research can be useful for generating new theories or hypotheses about social phenomena. By gathering rich, detailed data about individuals’ experiences and perspectives, researchers can develop insights that may challenge existing theories or lead to new lines of inquiry.
- Providing context for quantitative data: Qualitative research can be useful for providing context for quantitative data. By gathering qualitative data alongside quantitative data, researchers can develop a more complete understanding of complex social phenomena and identify potential explanations for quantitative findings.
When to use Qualitative Research
Here are some situations where qualitative research may be appropriate:
- Exploring a new area: If little is known about a particular topic, qualitative research can help to identify key issues, generate hypotheses, and develop new theories.
- Understanding complex phenomena: Qualitative research can be used to investigate complex social, cultural, or organizational phenomena that are difficult to measure quantitatively.
- Investigating subjective experiences: Qualitative research is particularly useful for investigating the subjective experiences of individuals or groups, such as their attitudes, beliefs, values, or emotions.
- Conducting formative research: Qualitative research can be used in the early stages of a research project to develop research questions, identify potential research participants, and refine research methods.
- Evaluating interventions or programs: Qualitative research can be used to evaluate the effectiveness of interventions or programs by collecting data on participants’ experiences, attitudes, and behaviors.
Characteristics of Qualitative Research
Qualitative research is characterized by several key features, including:
- Focus on subjective experience: Qualitative research is concerned with understanding the subjective experiences, beliefs, and perspectives of individuals or groups in a particular context. Researchers aim to explore the meanings that people attach to their experiences and to understand the social and cultural factors that shape these meanings.
- Use of open-ended questions: Qualitative research relies on open-ended questions that allow participants to provide detailed, in-depth responses. Researchers seek to elicit rich, descriptive data that can provide insights into participants’ experiences and perspectives.
- Sampling-based on purpose and diversity: Qualitative research often involves purposive sampling, in which participants are selected based on specific criteria related to the research question. Researchers may also seek to include participants with diverse experiences and perspectives to capture a range of viewpoints.
- Data collection through multiple methods: Qualitative research typically involves the use of multiple data collection methods, such as in-depth interviews, focus groups, and observation. This allows researchers to gather rich, detailed data from multiple sources, which can provide a more complete picture of participants’ experiences and perspectives.
- Inductive data analysis: Qualitative research relies on inductive data analysis, in which researchers develop theories and insights based on the data rather than testing pre-existing hypotheses. Researchers use coding and thematic analysis to identify patterns and themes in the data and to develop theories and explanations based on these patterns.
- Emphasis on researcher reflexivity: Qualitative research recognizes the importance of the researcher’s role in shaping the research process and outcomes. Researchers are encouraged to reflect on their own biases and assumptions and to be transparent about their role in the research process.
Advantages of Qualitative Research
Qualitative research offers several advantages over other research methods, including:
- Depth and detail: Qualitative research allows researchers to gather rich, detailed data that provides a deeper understanding of complex social phenomena. Through in-depth interviews, focus groups, and observation, researchers can gather detailed information about participants’ experiences and perspectives that may be missed by other research methods.
- Flexibility : Qualitative research is a flexible approach that allows researchers to adapt their methods to the research question and context. Researchers can adjust their research methods in real-time to gather more information or explore unexpected findings.
- Contextual understanding: Qualitative research is well-suited to exploring the social and cultural context in which individuals or groups are situated. Researchers can gather information about cultural norms, social structures, and historical events that may influence participants’ experiences and perspectives.
- Participant perspective : Qualitative research prioritizes the perspective of participants, allowing researchers to explore subjective experiences and understand the meanings that participants attach to their experiences.
- Theory development: Qualitative research can contribute to the development of new theories and insights about complex social phenomena. By gathering rich, detailed data and using inductive data analysis, researchers can develop new theories and explanations that may challenge existing understandings.
- Validity : Qualitative research can offer high validity by using multiple data collection methods, purposive and diverse sampling, and researcher reflexivity. This can help ensure that findings are credible and trustworthy.
Limitations of Qualitative Research
Qualitative research also has some limitations, including:
- Subjectivity : Qualitative research relies on the subjective interpretation of researchers, which can introduce bias into the research process. The researcher’s perspective, beliefs, and experiences can influence the way data is collected, analyzed, and interpreted.
- Limited generalizability: Qualitative research typically involves small, purposive samples that may not be representative of larger populations. This limits the generalizability of findings to other contexts or populations.
- Time-consuming: Qualitative research can be a time-consuming process, requiring significant resources for data collection, analysis, and interpretation.
- Resource-intensive: Qualitative research may require more resources than other research methods, including specialized training for researchers, specialized software for data analysis, and transcription services.
- Limited reliability: Qualitative research may be less reliable than quantitative research, as it relies on the subjective interpretation of researchers. This can make it difficult to replicate findings or compare results across different studies.
- Ethics and confidentiality: Qualitative research involves collecting sensitive information from participants, which raises ethical concerns about confidentiality and informed consent. Researchers must take care to protect the privacy and confidentiality of participants and obtain informed consent.
Also see Research Methods
About the author
Muhammad Hassan
Researcher, Academic Writer, Web developer
You may also like

Questionnaire – Definition, Types, and Examples

Case Study – Methods, Examples and Guide

Observational Research – Methods and Guide

Quantitative Research – Methods, Types and...

Qualitative Research Methods

Explanatory Research – Types, Methods, Guide
The qualitative research process, end-to-end
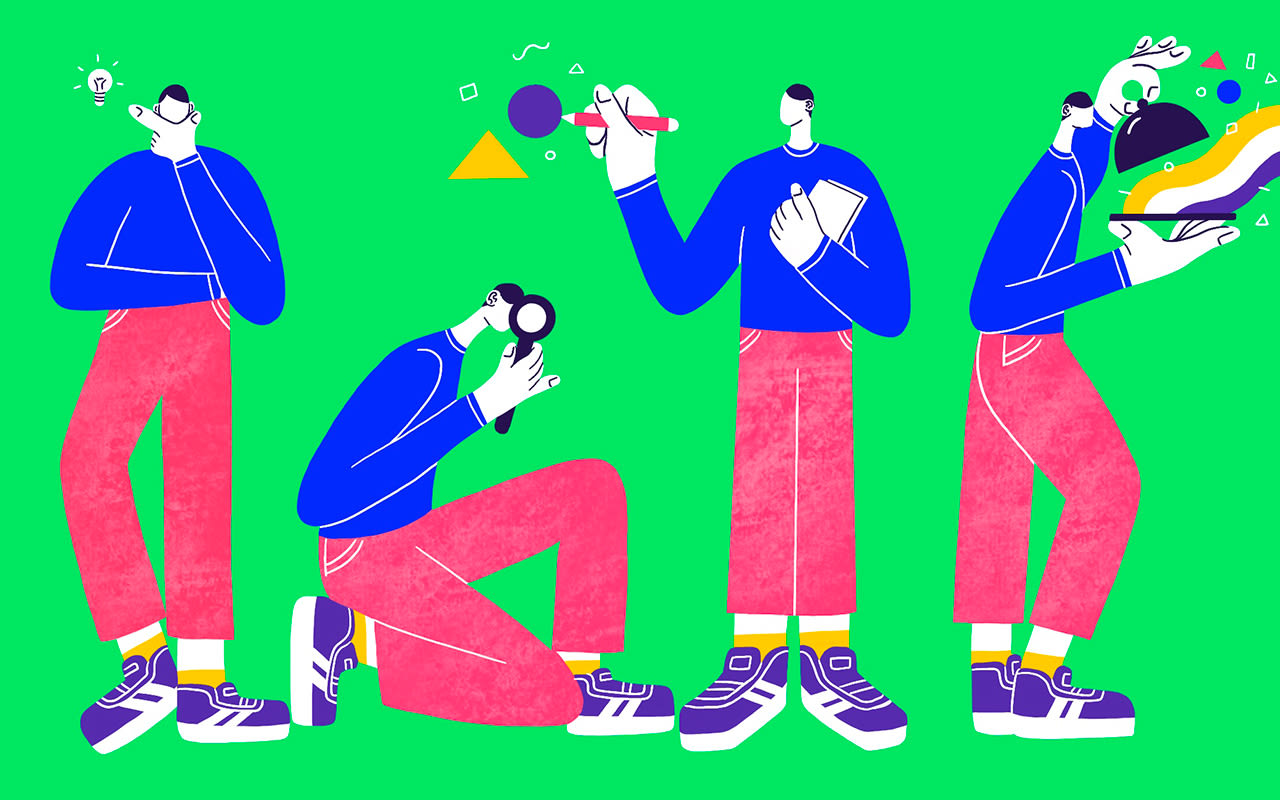
.css-1nrevy2{position:relative;display:inline-block;} The qualitative research process: step by step guide
Although research processes may vary by methodology or project team, some fundamentals exist across research projects. Below outlines the collective experience that qualitative researchers undertake to conduct research.
Step 1: Determine what to research
Once a researcher has determined a list of potential projects to tackle, they will prioritize projects based on the business's impact, available resourcing, timelines & dependencies to create a research roadmap. For each project, they will also identify the key questions they need to answer in the research.
The researcher should identify the participants they plan to research, and any key attributes that are a 'must-have' or 'nice to have' as these can be influential in determining the research approach (e.g. a niche group may require a longer timeline to recruit).
Researchers will generally aim for a mix of project types. Some may be more tactical or requests from stakeholders, and some will be projects that the researcher has proactively identified as opportunities for strategic research.
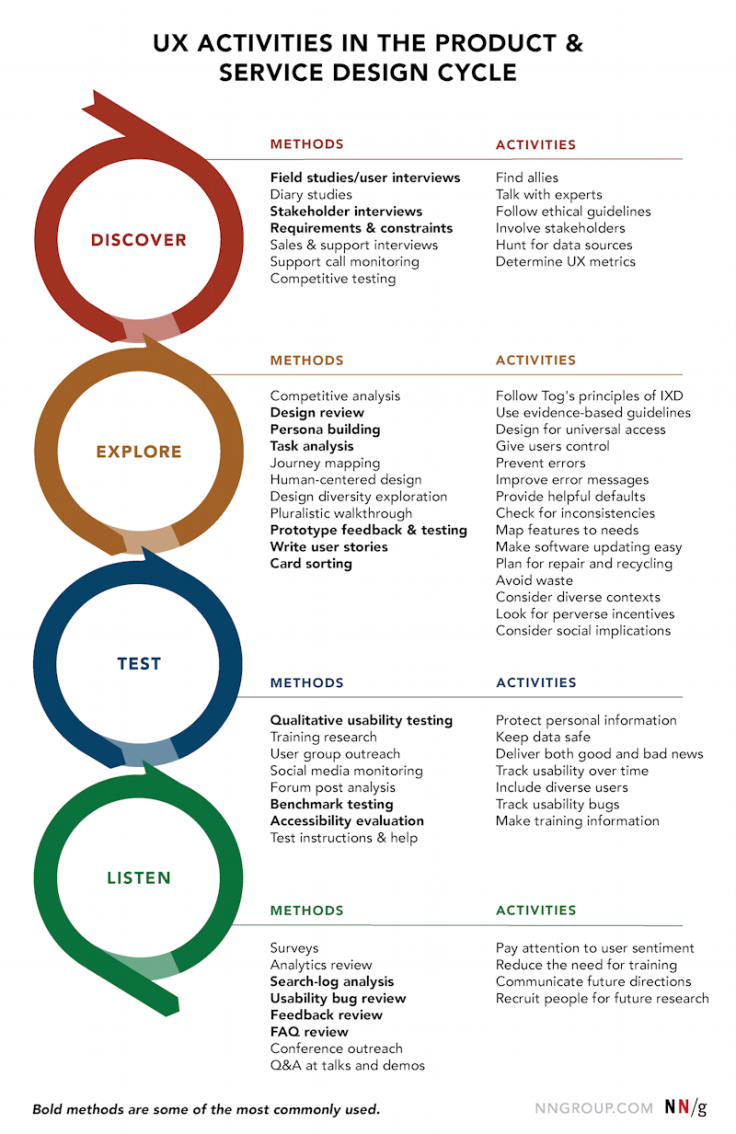
Step 2: Identify how to research it
Once the researcher has finalized the research project, they will need to figure out how they will do the work.
Firstly, the researcher will look through secondary data and research (e.g. analytics, previous research reports). Secondary analysis will help determine if there are existing answers to any of the open questions, ensuring that any net-new study doesn't duplicate current work (unless previous research is out of date).
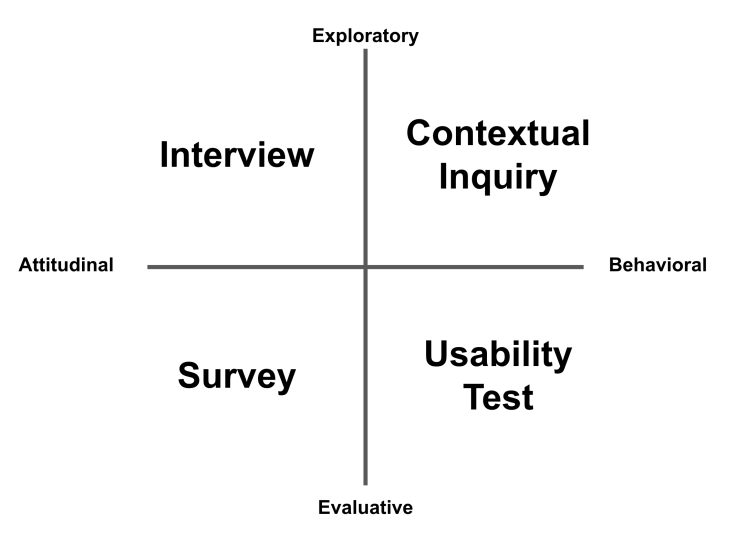
After scoping the research, researchers will determine if the research input needs to be attitudinal (i.e. what someone says) or behavioral (i.e. what someone does); as well as if they need to explore a problem space or evaluate a product – these help determine the methodology to use. There are many methodologies out there, but the main ones you generally will find from a qualitative perspective are:
Interviews [Attitudinal / Exploratory] – semi-structured conversation with a participant focused on a small set of topics. Runs for 30-60 minutes.
Contextual Inquiry [Behavioral / Exploratory] – observation of a participant in their environment. Probing questions may be asked during the observation. Runs for 2-3 hours.
Survey [Attitudinal / Evaluative] – gathering structured information from a sample of people, traditionally to generalize the results to a larger population. Surveys should generally not take participants more than 10 minutes to complete.
Usability Test [Behavioral / Evaluative] – evaluating how representative users are, or are not, able to achieve critical tasks within an experience.
Check out these articles for more information about different methodologies:
When to Use Which User-Experience Research Methods
UX Research Cheat Sheet
Usability.gov
Design Research Kit
Step 3: Get buy-in and alignment from others
Once a researcher has determined what they will be researching and how they will research it, they will generally write up a research plan that includes additional information about the research goals, participant scope, timelines, and dependencies. The plan is typically either a document or presentation shared with stakeholders depending on the company and how they work.
After the research plan is complete, researchers will share the plan for feedback and input from their stakeholders to ensure that the stakeholders have the right expectations going into the research. Stakeholders may ask for additional question topics to be added, ensure that research will be executed against specific timelines, or provide recommendations on how the study will help make product decisions.
At organizations where there is a research team, researchers may also share their plan with other researchers informally or through a 'crit' process. Generally, researchers will provide feedback on the research craft, such as methodologies, participant mixes, and the research goals or questions.
Once the researcher feels confident in their plan, they will either begin to plan the research, or in the case of more junior researchers, get approval from their manager to begin the study.
Step 4: Prepare research
This step is where the researcher will get all of their ducks in a row to execute the research. Preparation activities include:
Equipment: Booking venues, labs, observation rooms, and procuring any appropriate equipment needed to run the study (e.g. cameras, mobile devices).
Participants: Sourcing participants from internal / external databases, reaching out/scheduling participants, managing schedule changes.
Incentives: Find budget, identify incentive type (e.g. Amazon gift card? customer credit? gift baskets), and purchasing.
Assets: Building relevant designs / prototypes (with design or design technologists), creating interview / observation guides and other research tools needed for sessions (e.g. physical cards for in-person card sorts).
Legal & Procurement: Participant waivers or NDAs preparation to ensure they are sent in advance of the research session to participants, vendor procurement, and management.
If Research Operations exists within an organization, they will generally take on most of the load in this area. The researcher will focus on assets required for executing research, such as interview guides.
In some cases, vendors may be engaged for some of these requirements (e.g. labs, participants, and incentive management) if resourcing is not available internally or if a researcher wants a blinded study (i.e. the participant doesn't know what company is running the research). In this case, additional time is incorporated to brief, onboard, and get approvals to work with the vendor.
Step 5: Execute research
Now the researcher gets to research!
Researchers will generally aim to execute research activities for 1–2 weeks, depending on the methodology to ensure they can be efficient in execution. In some more longitudinal methods (e.g., diary studies), or if a participant type is harder to recruit, it may take longer.
In consumer research, there will usually be back up participants available in case of no shows. However, in business or enterprise research, researchers will engage will all recruited participants as participants will generally have relationships with other parts of the company (e.g. sales). It is essential to maintain those relationships post-research.
During sessions, in a perfect world, there is one facilitator (principal researcher). In some cases, a secondary attendee who takes notes – this can be a stakeholder or a more junior researcher who can then learn soft skills from the primary researcher. By delegating note-taking, the principal researcher can focus on driving and managing the participant's conversation.
However, in most cases (especially if there is a "research team of one"), researchers will try to have to do both facilitation and documentation – this can lead to a clunkier conversation as the facilitator attempts to quickly write notes between trying to think of the next question. If a researcher decides to record a session instead, they will have to spend additional time after the research listening to the full recordings and writing notes.
In qualitative research, researchers may begin to see patterns in the findings after five sessions . They may start to tailor the research questions to be more specific to gaps in their understanding.
Researchers may also set up an observation room for stakeholders (or share links to remote sessions) to attend live. Generally, researchers will have a backchannel (e.g., slack, chat, or SMS), so if a stakeholder has a follow-up question to an answer, the researcher can dig deeper. In some cases, researchers will give stakeholders an input form to take their notes that can be shared with the researcher afterward - this can be useful for the researcher to understand how the stakeholder views the research and what the stakeholder perceives as necessary to the research insights.
Step 6: Synthesize and find insights
Once the research capture is complete, the researcher will then aggregate findings to begin to look for common themes (in exploratory) or success rates (in evaluative). Both of these will then lead to insight generation that researchers will then look to tie back to the project's original research goals.
As analysis can be one of the most high-effort tasks in research, researchers will lean towards how to be efficient in their study, generally using digital tools, hacks, or workarounds. Researchers will usually create the analysis process they refine throughout their careers to help them become more efficient.
In cases where researchers are looking to get buy-in for research or capture stakeholder input, they may seem to more visual approaches (e.g. post-it affinity analysis) in war rooms. This process can take longer to process (especially if there is a high volume of data). Still, there can be a higher impact on analyzing research in this way – especially if the researcher is looking to get buy-in for future projects.
Step 7: Create research outputs
After a researcher identifies the key themes and insights, the researcher will reframe these findings to a relevant research output to ensure that stakeholders understand and buy-in to the outcomes. Outputs may include:
Report: Outlines vital findings from research in a document or presentation format. Will most likely include an executive summary, insight themes, and supporting evidence.
Videos: A highlight reel of supporting evidence from crucial findings. Generally seen as more useful and engaging compared to just a report. In most cases, the video will help the research report.
Personas : A written representation of a product's intended users to understand different types of user goals, needs, and behaviors. Also used to help stakeholders build empathy for the end-user of the product.
Journey Map : A visualization of the process that a person goes through to accomplish a goal. Generally created in conjunction with a persona.
Concepts / Wireframes / Designs: If research is evaluative, designs can visualize recommendations.
Storyboarding
Before a researcher makes the output, researchers will spend time planning the structure and storyboarding. Storyboarding is incredibly essential to help researchers define information requirements and ensure they present their findings in the most impactful way to stakeholders.
Having a point of view in outputs
Historically, researchers have tried to stay neutral to the data and not try to have a strong opinion or perspective to let the data speak. However, as researchers become more embedded in the industry, this has shifted to stakeholders wanting a strong point of view or recommendations from researchers that can help other stakeholders (especially product managers and designers) decide the knowledge captured as part of the research.
Having a strong perspective helps researchers have a seat at the table and appear as a trusted advisor/partner in cross-functional settings.
Step 8: Share and follow up on findings
After the research outputs are complete, some researchers will do a "pre-share" or walkthrough with key stakeholders or potential detractors to the research. The purpose of these meetings is to align with stakeholders' expectations and find potential 'watch-outs' (things that may derail a presentation).
Researchers will generally have to share their findings out multiple times to different stakeholder groups and tailor them for each audience. For example, executive meetings will be more higher level than a meeting with a product manager.
After sharing, researchers will follow up with key stakeholders (especially those who provided input to the research) to confirm they understand the findings and identify next steps. Next steps may include incorporating results in product strategy documents, proposals / PRDs, or user stories to ensure that the recommendations or findings have been reflected or sourced.
Keep reading
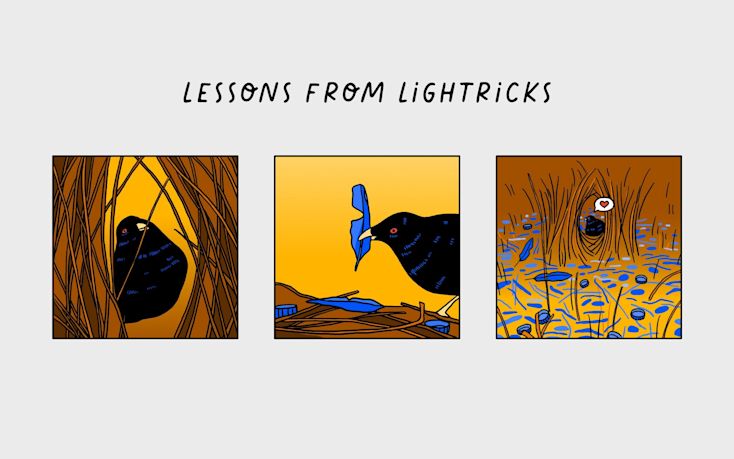
.css-je19u9{-webkit-align-items:flex-end;-webkit-box-align:flex-end;-ms-flex-align:flex-end;align-items:flex-end;display:-webkit-box;display:-webkit-flex;display:-ms-flexbox;display:flex;-webkit-flex-direction:row;-ms-flex-direction:row;flex-direction:row;-webkit-box-flex-wrap:wrap;-webkit-flex-wrap:wrap;-ms-flex-wrap:wrap;flex-wrap:wrap;-webkit-box-pack:center;-ms-flex-pack:center;-webkit-justify-content:center;justify-content:center;row-gap:0;text-align:center;max-width:671px;}@media (max-width: 1079px){.css-je19u9{max-width:400px;}.css-je19u9>span{white-space:pre;}}@media (max-width: 799px){.css-je19u9{max-width:400px;}.css-je19u9>span{white-space:pre;}} Decide what to .css-1kiodld{max-height:56px;display:-webkit-box;display:-webkit-flex;display:-ms-flexbox;display:flex;-webkit-align-items:center;-webkit-box-align:center;-ms-flex-align:center;align-items:center;}@media (max-width: 1079px){.css-1kiodld{display:none;}} build next
Decide what to build next.

Users report unexpectedly high data usage, especially during streaming sessions.

Users find it hard to navigate from the home page to relevant playlists in the app.

It would be great to have a sleep timer feature, especially for bedtime listening.

I need better filters to find the songs or artists I’m looking for.
Log in or sign up
Get started for free

Narrative Analysis 101
Everything you need to know to get started
By: Ethar Al-Saraf (PhD)| Expert Reviewed By: Eunice Rautenbach (DTech) | March 2023
If you’re new to research, the host of qualitative analysis methods available to you can be a little overwhelming. In this post, we’ll unpack the sometimes slippery topic of narrative analysis . We’ll explain what it is, consider its strengths and weaknesses , and look at when and when not to use this analysis method.
Overview: Narrative Analysis
- What is narrative analysis (simple definition)
- The two overarching approaches
- The strengths & weaknesses of narrative analysis
- When (and when not) to use it
- Key takeaways
What Is Narrative Analysis?
Simply put, narrative analysis is a qualitative analysis method focused on interpreting human experiences and motivations by looking closely at the stories (the narratives) people tell in a particular context.
In other words, a narrative analysis interprets long-form participant responses or written stories as data, to uncover themes and meanings . That data could be taken from interviews, monologues, written stories, or even recordings. In other words, narrative analysis can be used on both primary and secondary data to provide evidence from the experiences described.
That’s all quite conceptual, so let’s look at an example of how narrative analysis could be used.
Let’s say you’re interested in researching the beliefs of a particular author on popular culture. In that case, you might identify the characters , plotlines , symbols and motifs used in their stories. You could then use narrative analysis to analyse these in combination and against the backdrop of the relevant context.
This would allow you to interpret the underlying meanings and implications in their writing, and what they reveal about the beliefs of the author. In other words, you’d look to understand the views of the author by analysing the narratives that run through their work.
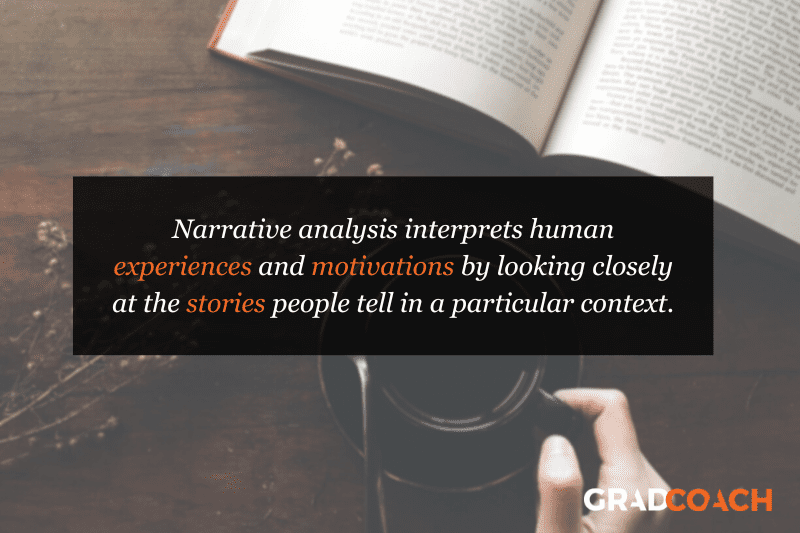
The Two Overarching Approaches
Generally speaking, there are two approaches that one can take to narrative analysis. Specifically, an inductive approach or a deductive approach. Each one will have a meaningful impact on how you interpret your data and the conclusions you can draw, so it’s important that you understand the difference.
First up is the inductive approach to narrative analysis.
The inductive approach takes a bottom-up view , allowing the data to speak for itself, without the influence of any preconceived notions . With this approach, you begin by looking at the data and deriving patterns and themes that can be used to explain the story, as opposed to viewing the data through the lens of pre-existing hypotheses, theories or frameworks. In other words, the analysis is led by the data.
For example, with an inductive approach, you might notice patterns or themes in the way an author presents their characters or develops their plot. You’d then observe these patterns, develop an interpretation of what they might reveal in the context of the story, and draw conclusions relative to the aims of your research.
Contrasted to this is the deductive approach.
With the deductive approach to narrative analysis, you begin by using existing theories that a narrative can be tested against . Here, the analysis adopts particular theoretical assumptions and/or provides hypotheses, and then looks for evidence in a story that will either verify or disprove them.
For example, your analysis might begin with a theory that wealthy authors only tell stories to get the sympathy of their readers. A deductive analysis might then look at the narratives of wealthy authors for evidence that will substantiate (or refute) the theory and then draw conclusions about its accuracy, and suggest explanations for why that might or might not be the case.
Which approach you should take depends on your research aims, objectives and research questions . If these are more exploratory in nature, you’ll likely take an inductive approach. Conversely, if they are more confirmatory in nature, you’ll likely opt for the deductive approach.
Need a helping hand?
Strengths & Weaknesses
Now that we have a clearer view of what narrative analysis is and the two approaches to it, it’s important to understand its strengths and weaknesses , so that you can make the right choices in your research project.
A primary strength of narrative analysis is the rich insight it can generate by uncovering the underlying meanings and interpretations of human experience. The focus on an individual narrative highlights the nuances and complexities of their experience, revealing details that might be missed or considered insignificant by other methods.
Another strength of narrative analysis is the range of topics it can be used for. The focus on human experience means that a narrative analysis can democratise your data analysis, by revealing the value of individuals’ own interpretation of their experience in contrast to broader social, cultural, and political factors.
All that said, just like all analysis methods, narrative analysis has its weaknesses. It’s important to understand these so that you can choose the most appropriate method for your particular research project.
The first drawback of narrative analysis is the problem of subjectivity and interpretation . In other words, a drawback of the focus on stories and their details is that they’re open to being understood differently depending on who’s reading them. This means that a strong understanding of the author’s cultural context is crucial to developing your interpretation of the data. At the same time, it’s important that you remain open-minded in how you interpret your chosen narrative and avoid making any assumptions .
A second weakness of narrative analysis is the issue of reliability and generalisation . Since narrative analysis depends almost entirely on a subjective narrative and your interpretation, the findings and conclusions can’t usually be generalised or empirically verified. Although some conclusions can be drawn about the cultural context, they’re still based on what will almost always be anecdotal data and not suitable for the basis of a theory, for example.
Last but not least, the focus on long-form data expressed as stories means that narrative analysis can be very time-consuming . In addition to the source data itself, you will have to be well informed on the author’s cultural context as well as other interpretations of the narrative, where possible, to ensure you have a holistic view. So, if you’re going to undertake narrative analysis, make sure that you allocate a generous amount of time to work through the data.
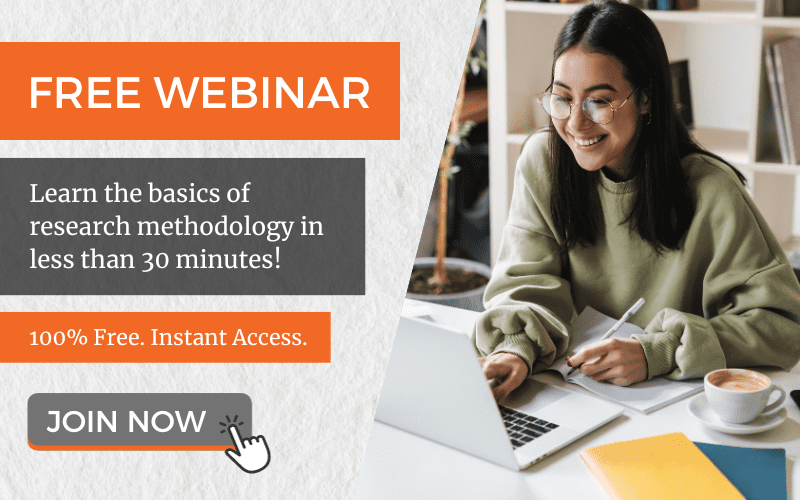
When To Use Narrative Analysis
As a qualitative method focused on analysing and interpreting narratives describing human experiences, narrative analysis is usually most appropriate for research topics focused on social, personal, cultural , or even ideological events or phenomena and how they’re understood at an individual level.
For example, if you were interested in understanding the experiences and beliefs of individuals suffering social marginalisation, you could use narrative analysis to look at the narratives and stories told by people in marginalised groups to identify patterns , symbols , or motifs that shed light on how they rationalise their experiences.
In this example, narrative analysis presents a good natural fit as it’s focused on analysing people’s stories to understand their views and beliefs at an individual level. Conversely, if your research was geared towards understanding broader themes and patterns regarding an event or phenomena, analysis methods such as content analysis or thematic analysis may be better suited, depending on your research aim .
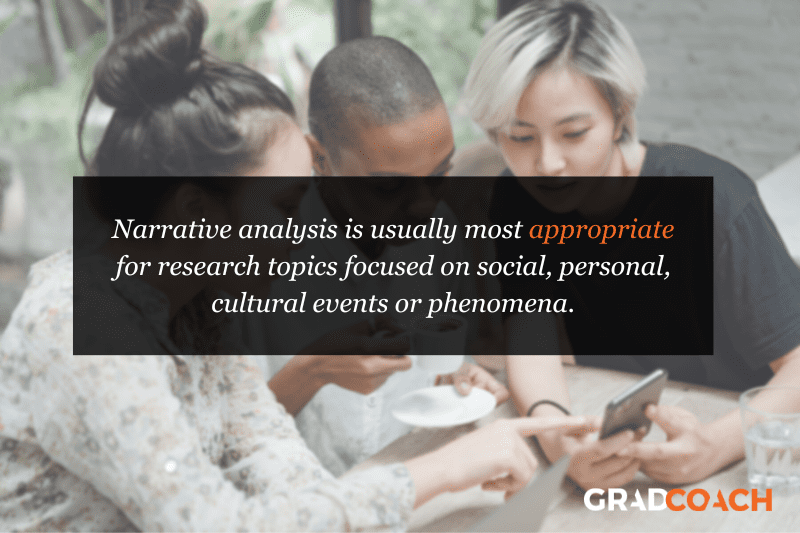
Let’s recap
In this post, we’ve explored the basics of narrative analysis in qualitative research. The key takeaways are:
- Narrative analysis is a qualitative analysis method focused on interpreting human experience in the form of stories or narratives .
- There are two overarching approaches to narrative analysis: the inductive (exploratory) approach and the deductive (confirmatory) approach.
- Like all analysis methods, narrative analysis has a particular set of strengths and weaknesses .
- Narrative analysis is generally most appropriate for research focused on interpreting individual, human experiences as expressed in detailed , long-form accounts.
If you’d like to learn more about narrative analysis and qualitative analysis methods in general, be sure to check out the rest of the Grad Coach blog here . Alternatively, if you’re looking for hands-on help with your project, take a look at our 1-on-1 private coaching service .
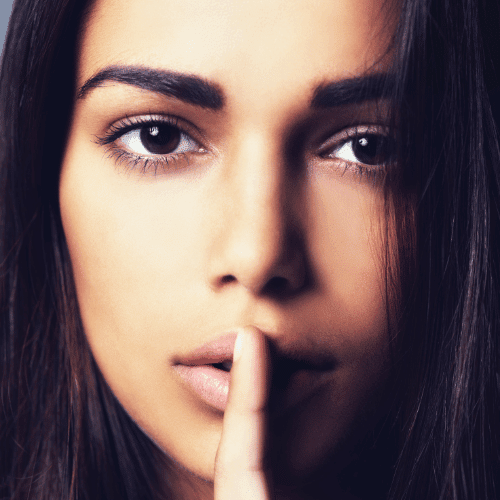
Psst... there’s more!
This post was based on one of our popular Research Bootcamps . If you're working on a research project, you'll definitely want to check this out ...
You Might Also Like:
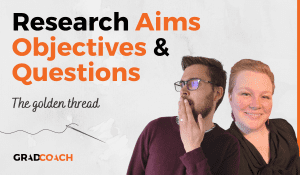
Thanks. I need examples of narrative analysis
Here are some examples of research topics that could utilise narrative analysis:
Personal Narratives of Trauma: Analysing personal stories of individuals who have experienced trauma to understand the impact, coping mechanisms, and healing processes.
Identity Formation in Immigrant Communities: Examining the narratives of immigrants to explore how they construct and negotiate their identities in a new cultural context.
Media Representations of Gender: Analysing narratives in media texts (such as films, television shows, or advertisements) to investigate the portrayal of gender roles, stereotypes, and power dynamics.
Where can I find an example of a narrative analysis table ?
Please i need help with my project,
how can I cite this article in APA 7th style?
please mention the sources as well.
My research is mixed approach. I use interview,key_inforamt interview,FGD and document.so,which qualitative analysis is appropriate to analyze these data.Thanks
Which qualitative analysis methode is appropriate to analyze data obtain from intetview,key informant intetview,Focus group discussion and document.
I’ve finished my PhD. Now I need a “platform” that will help me objectively ascertain the tacit assumptions that are buried within a narrative. Can you help?
Submit a Comment Cancel reply
Your email address will not be published. Required fields are marked *
Save my name, email, and website in this browser for the next time I comment.
- Print Friendly
Root out friction in every digital experience, super-charge conversion rates, and optimize digital self-service
Uncover insights from any interaction, deliver AI-powered agent coaching, and reduce cost to serve
Increase revenue and loyalty with real-time insights and recommendations delivered to teams on the ground
Know how your people feel and empower managers to improve employee engagement, productivity, and retention
Take action in the moments that matter most along the employee journey and drive bottom line growth
Whatever they’re are saying, wherever they’re saying it, know exactly what’s going on with your people
Get faster, richer insights with qual and quant tools that make powerful market research available to everyone
Run concept tests, pricing studies, prototyping + more with fast, powerful studies designed by UX research experts
Track your brand performance 24/7 and act quickly to respond to opportunities and challenges in your market
Explore the platform powering Experience Management
- Free Account
- For Digital
- For Customer Care
- For Human Resources
- For Researchers
- Financial Services
- All Industries
Popular Use Cases
- Customer Experience
- Employee Experience
- Employee Exit Interviews
- Net Promoter Score
- Voice of Customer
- Customer Success Hub
- Product Documentation
- Training & Certification
- XM Institute
- Popular Resources
- Customer Stories
Market Research
- Artificial Intelligence
- Partnerships
- Marketplace
The annual gathering of the experience leaders at the world’s iconic brands building breakthrough business results, live in Salt Lake City.
- English/AU & NZ
- Español/Europa
- Español/América Latina
- Português Brasileiro
- REQUEST DEMO
- Experience Management
- Qualitative Research
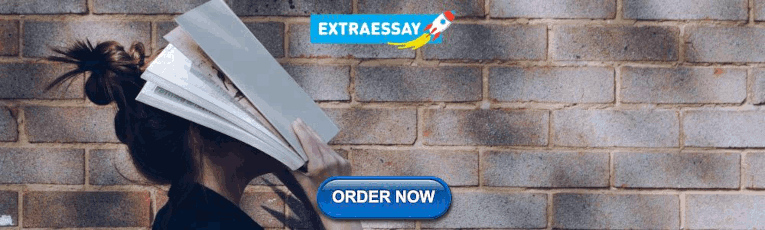
Try Qualtrics for free
Your ultimate guide to qualitative research (with methods and examples).
16 min read You may be already using qualitative research and want to check your understanding, or you may be starting from the beginning. Learn about qualitative research methods and how you can best use them for maximum effect.
What is qualitative research?
Qualitative research is a research method that collects non-numerical data. Typically, it goes beyond the information that quantitative research provides (which we will cover below) because it is used to gain an understanding of underlying reasons, opinions, and motivations.
Qualitative research methods focus on the thoughts, feelings, reasons, motivations, and values of a participant, to understand why people act in the way they do .
In this way, qualitative research can be described as naturalistic research, looking at naturally-occurring social events within natural settings. So, qualitative researchers would describe their part in social research as the ‘vehicle’ for collecting the qualitative research data.
Qualitative researchers discovered this by looking at primary and secondary sources where data is represented in non-numerical form. This can include collecting qualitative research data types like quotes, symbols, images, and written testimonials.
These data types tell qualitative researchers subjective information. While these aren’t facts in themselves, conclusions can be interpreted out of qualitative that can help to provide valuable context.
Because of this, qualitative research is typically viewed as explanatory in nature and is often used in social research, as this gives a window into the behavior and actions of people.
It can be a good research approach for health services research or clinical research projects.
Free eBook: The qualitative research design handbook
Quantitative vs qualitative research
In order to compare qualitative and quantitative research methods, let’s explore what quantitative research is first, before exploring how it differs from qualitative research.
Quantitative research
Quantitative research is the research method of collecting quantitative research data – data that can be converted into numbers or numerical data, which can be easily quantified, compared, and analyzed .
Quantitative research methods deal with primary and secondary sources where data is represented in numerical form. This can include closed-question poll results, statistics, and census information or demographic data.
Quantitative research data tends to be used when researchers are interested in understanding a particular moment in time and examining data sets over time to find trends and patterns.
The difference between quantitative and qualitative research methodology
While qualitative research is defined as data that supplies non-numerical information, quantitative research focuses on numerical data.
In general, if you’re interested in measuring something or testing a hypothesis, use quantitative research methods. If you want to explore ideas, thoughts, and meanings, use qualitative research methods.
While qualitative research helps you to properly define, promote and sell your products, don’t rely on qualitative research methods alone because qualitative findings can’t always be reliably repeated. Qualitative research is directional, not empirical.
The best statistical analysis research uses a combination of empirical data and human experience ( quantitative research and qualitative research ) to tell the story and gain better and deeper insights, quickly.
Where both qualitative and quantitative methods are not used, qualitative researchers will find that using one without the other leaves you with missing answers.
For example, if a retail company wants to understand whether a new product line of shoes will perform well in the target market:
- Qualitative research methods could be used with a sample of target customers, which would provide subjective reasons why they’d be likely to purchase or not purchase the shoes, while
- Quantitative research methods into the historical customer sales information on shoe-related products would provide insights into the sales performance, and likely future performance of the new product range.
Approaches to qualitative research
There are five approaches to qualitative research methods:
- Grounded theory: Grounded theory relates to where qualitative researchers come to a stronger hypothesis through induction, all throughout the process of collecting qualitative research data and forming connections. After an initial question to get started, qualitative researchers delve into information that is grouped into ideas or codes, which grow and develop into larger categories, as the qualitative research goes on. At the end of the qualitative research, the researcher may have a completely different hypothesis, based on evidence and inquiry, as well as the initial question.
- Ethnographic research : Ethnographic research is where researchers embed themselves into the environment of the participant or group in order to understand the culture and context of activities and behavior. This is dependent on the involvement of the researcher, and can be subject to researcher interpretation bias and participant observer bias . However, it remains a great way to allow researchers to experience a different ‘world’.
- Action research: With the action research process, both researchers and participants work together to make a change. This can be through taking action, researching and reflecting on the outcomes. Through collaboration, the collective comes to a result, though the way both groups interact and how they affect each other gives insights into their critical thinking skills.
- Phenomenological research: Researchers seek to understand the meaning of an event or behavior phenomenon by describing and interpreting participant’s life experiences. This qualitative research process understands that people create their own structured reality (‘the social construction of reality’), based on their past experiences. So, by viewing the way people intentionally live their lives, we’re able to see the experiential meaning behind why they live as they do.
- Narrative research: Narrative research, or narrative inquiry, is where researchers examine the way stories are told by participants, and how they explain their experiences, as a way of explaining the meaning behind their life choices and events. This qualitative research can arise from using journals, conversational stories, autobiographies or letters, as a few narrative research examples. The narrative is subjective to the participant, so we’re able to understand their views from what they’ve documented/spoken.
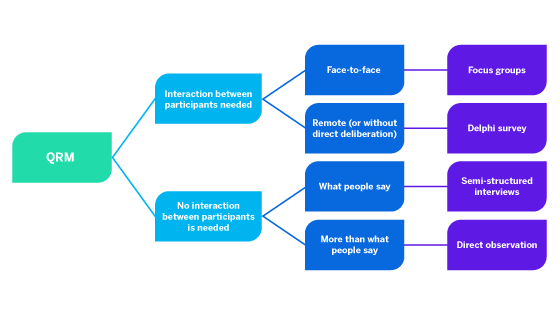
Qualitative research methods can use structured research instruments for data collection, like:
Surveys for individual views
A survey is a simple-to-create and easy-to-distribute qualitative research method, which helps gather information from large groups of participants quickly. Traditionally, paper-based surveys can now be made online, so costs can stay quite low.
Qualitative research questions tend to be open questions that ask for more information and provide a text box to allow for unconstrained comments.
Examples include:
- Asking participants to keep a written or a video diary for a period of time to document their feelings and thoughts
- In-Home-Usage tests: Buyers use your product for a period of time and report their experience
Surveys for group consensus (Delphi survey)
A Delphi survey may be used as a way to bring together participants and gain a consensus view over several rounds of questions. It differs from traditional surveys where results go to the researcher only. Instead, results go to participants as well, so they can reflect and consider all responses before another round of questions are submitted.
This can be useful to do as it can help researchers see what variance is among the group of participants and see the process of how consensus was reached.
- Asking participants to act as a fake jury for a trial and revealing parts of the case over several rounds to see how opinions change. At the end, the fake jury must make a unanimous decision about the defendant on trial.
- Asking participants to comment on the versions of a product being developed , as the changes are made and their feedback is taken onboard. At the end, participants must decide whether the product is ready to launch .
Semi-structured interviews
Interviews are a great way to connect with participants, though they require time from the research team to set up and conduct, especially if they’re done face-to-face.
Researchers may also have issues connecting with participants in different geographical regions. The researcher uses a set of predefined open-ended questions, though more ad-hoc questions can be asked depending on participant answers.
- Conducting a phone interview with participants to run through their feedback on a product . During the conversation, researchers can go ‘off-script’ and ask more probing questions for clarification or build on the insights.
Focus groups
Participants are brought together into a group, where a particular topic is discussed. It is researcher-led and usually occurs in-person in a mutually accessible location, to allow for easy communication between participants in focus groups.
In focus groups , the researcher uses a set of predefined open-ended questions, though more ad-hoc questions can be asked depending on participant answers.
- Asking participants to do UX tests, which are interface usability tests to show how easily users can complete certain tasks
Direct observation
This is a form of ethnographic research where researchers will observe participants’ behavior in a naturalistic environment. This can be great for understanding the actions in the culture and context of a participant’s setting.
This qualitative research method is prone to researcher bias as it is the researcher that must interpret the actions and reactions of participants. Their findings can be impacted by their own beliefs, values, and inferences.
- Embedding yourself in the location of your buyers to understand how a product would perform against the values and norms of that society
Qualitative data types and category types
Qualitative research methods often deliver information in the following qualitative research data types:
- Written testimonials
Through contextual analysis of the information, researchers can assign participants to category types:
- Social class
- Political alignment
- Most likely to purchase a product
- Their preferred training learning style
Advantages of qualitative research
- Useful for complex situations: Qualitative research on its own is great when dealing with complex issues, however, providing background context using quantitative facts can give a richer and wider understanding of a topic. In these cases, quantitative research may not be enough.
- A window into the ‘why’: Qualitative research can give you a window into the deeper meaning behind a participant’s answer. It can help you uncover the larger ‘why’ that can’t always be seen by analyzing numerical data.
- Can help improve customer experiences: In service industries where customers are crucial, like in private health services, gaining information about a customer’s experience through health research studies can indicate areas where services can be improved.
Disadvantages of qualitative research
- You need to ask the right question: Doing qualitative research may require you to consider what the right question is to uncover the underlying thinking behind a behavior. This may need probing questions to go further, which may suit a focus group or face-to-face interview setting better.
- Results are interpreted: As qualitative research data is written, spoken, and often nuanced, interpreting the data results can be difficult as they come in non-numerical formats. This might make it harder to know if you can accept or reject your hypothesis.
- More bias: There are lower levels of control to qualitative research methods, as they can be subject to biases like confirmation bias, researcher bias, and observation bias. This can have a knock-on effect on the validity and truthfulness of the qualitative research data results.
How to use qualitative research to your business’s advantage?
Qualitative methods help improve your products and marketing in many different ways:
- Understand the emotional connections to your brand
- Identify obstacles to purchase
- Uncover doubts and confusion about your messaging
- Find missing product features
- Improve the usability of your website, app, or chatbot experience
- Learn about how consumers talk about your product
- See how buyers compare your brand to others in the competitive set
- Learn how an organization’s employees evaluate and select vendors
6 steps to conducting good qualitative research
Businesses can benefit from qualitative research by using it to understand the meaning behind data types. There are several steps to this:
- Define your problem or interest area: What do you observe is happening and is it frequent? Identify the data type/s you’re observing.
- Create a hypothesis: Ask yourself what could be the causes for the situation with those qualitative research data types.
- Plan your qualitative research: Use structured qualitative research instruments like surveys, focus groups, or interviews to ask questions that test your hypothesis.
- Data Collection: Collect qualitative research data and understand what your data types are telling you. Once data is collected on different types over long time periods, you can analyze it and give insights into changing attitudes and language patterns.
- Data analysis: Does your information support your hypothesis? (You may need to redo the qualitative research with other variables to see if the results improve)
- Effectively present the qualitative research data: Communicate the results in a clear and concise way to help other people understand the findings.
Qualitative data analysis
Evaluating qualitative research can be tough when there are several analytics platforms to manage and lots of subjective data sources to compare.
Qualtrics provides a number of qualitative research analysis tools, like Text iQ , powered by Qualtrics iQ, provides powerful machine learning and native language processing to help you discover patterns and trends in text.
This also provides you with:
- Sentiment analysis — a technique to help identify the underlying sentiment (say positive, neutral, and/or negative) in qualitative research text responses
- Topic detection/categorisation — this technique is the grouping or bucketing of similar themes that can are relevant for the business & the industry (eg. ‘Food quality’, ‘Staff efficiency’ or ‘Product availability’)
How Qualtrics products can enhance & simplify the qualitative research process
Even in today’s data-obsessed marketplace, qualitative data is valuable – maybe even more so because it helps you establish an authentic human connection to your customers. If qualitative research doesn’t play a role to inform your product and marketing strategy, your decisions aren’t as effective as they could be.
The Qualtrics XM system gives you an all-in-one, integrated solution to help you all the way through conducting qualitative research. From survey creation and data collection to textual analysis and data reporting, it can help all your internal teams gain insights from your subjective and categorical data.
Qualitative methods are catered through templates or advanced survey designs. While you can manually collect data and conduct data analysis in a spreadsheet program, this solution helps you automate the process of qualitative research, saving you time and administration work.
Using computational techniques helps you to avoid human errors, and participant results come in are already incorporated into the analysis in real-time.
Our key tools, Text IQ™ and Driver IQ™ make analyzing subjective and categorical data easy and simple. Choose to highlight key findings based on topic, sentiment, or frequency. The choice is yours.
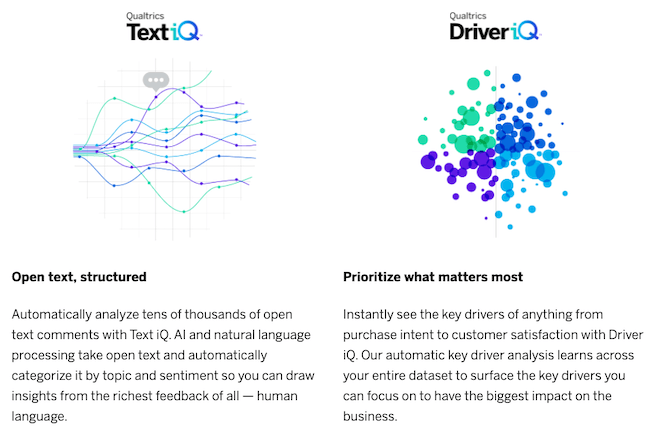
Some examples of your workspace in action, using drag and drop to create fast data visualizations quickly:
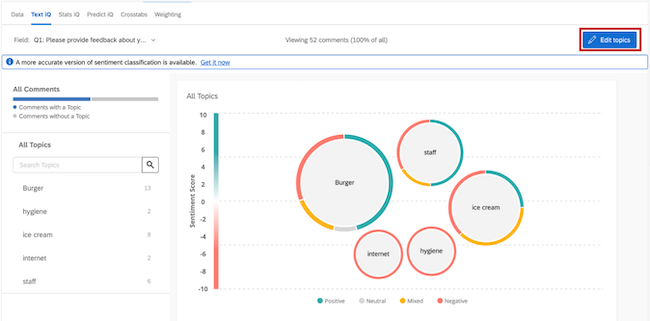
Related resources
Market intelligence 10 min read, marketing insights 11 min read, ethnographic research 11 min read, qualitative vs quantitative research 13 min read, qualitative research questions 11 min read, qualitative research design 12 min read, primary vs secondary research 14 min read, request demo.
Ready to learn more about Qualtrics?
- Open access
- Published: 27 May 2020
How to use and assess qualitative research methods
- Loraine Busetto ORCID: orcid.org/0000-0002-9228-7875 1 ,
- Wolfgang Wick 1 , 2 &
- Christoph Gumbinger 1
Neurological Research and Practice volume 2 , Article number: 14 ( 2020 ) Cite this article
715k Accesses
283 Citations
85 Altmetric
Metrics details
This paper aims to provide an overview of the use and assessment of qualitative research methods in the health sciences. Qualitative research can be defined as the study of the nature of phenomena and is especially appropriate for answering questions of why something is (not) observed, assessing complex multi-component interventions, and focussing on intervention improvement. The most common methods of data collection are document study, (non-) participant observations, semi-structured interviews and focus groups. For data analysis, field-notes and audio-recordings are transcribed into protocols and transcripts, and coded using qualitative data management software. Criteria such as checklists, reflexivity, sampling strategies, piloting, co-coding, member-checking and stakeholder involvement can be used to enhance and assess the quality of the research conducted. Using qualitative in addition to quantitative designs will equip us with better tools to address a greater range of research problems, and to fill in blind spots in current neurological research and practice.
The aim of this paper is to provide an overview of qualitative research methods, including hands-on information on how they can be used, reported and assessed. This article is intended for beginning qualitative researchers in the health sciences as well as experienced quantitative researchers who wish to broaden their understanding of qualitative research.
What is qualitative research?
Qualitative research is defined as “the study of the nature of phenomena”, including “their quality, different manifestations, the context in which they appear or the perspectives from which they can be perceived” , but excluding “their range, frequency and place in an objectively determined chain of cause and effect” [ 1 ]. This formal definition can be complemented with a more pragmatic rule of thumb: qualitative research generally includes data in form of words rather than numbers [ 2 ].
Why conduct qualitative research?
Because some research questions cannot be answered using (only) quantitative methods. For example, one Australian study addressed the issue of why patients from Aboriginal communities often present late or not at all to specialist services offered by tertiary care hospitals. Using qualitative interviews with patients and staff, it found one of the most significant access barriers to be transportation problems, including some towns and communities simply not having a bus service to the hospital [ 3 ]. A quantitative study could have measured the number of patients over time or even looked at possible explanatory factors – but only those previously known or suspected to be of relevance. To discover reasons for observed patterns, especially the invisible or surprising ones, qualitative designs are needed.
While qualitative research is common in other fields, it is still relatively underrepresented in health services research. The latter field is more traditionally rooted in the evidence-based-medicine paradigm, as seen in " research that involves testing the effectiveness of various strategies to achieve changes in clinical practice, preferably applying randomised controlled trial study designs (...) " [ 4 ]. This focus on quantitative research and specifically randomised controlled trials (RCT) is visible in the idea of a hierarchy of research evidence which assumes that some research designs are objectively better than others, and that choosing a "lesser" design is only acceptable when the better ones are not practically or ethically feasible [ 5 , 6 ]. Others, however, argue that an objective hierarchy does not exist, and that, instead, the research design and methods should be chosen to fit the specific research question at hand – "questions before methods" [ 2 , 7 , 8 , 9 ]. This means that even when an RCT is possible, some research problems require a different design that is better suited to addressing them. Arguing in JAMA, Berwick uses the example of rapid response teams in hospitals, which he describes as " a complex, multicomponent intervention – essentially a process of social change" susceptible to a range of different context factors including leadership or organisation history. According to him, "[in] such complex terrain, the RCT is an impoverished way to learn. Critics who use it as a truth standard in this context are incorrect" [ 8 ] . Instead of limiting oneself to RCTs, Berwick recommends embracing a wider range of methods , including qualitative ones, which for "these specific applications, (...) are not compromises in learning how to improve; they are superior" [ 8 ].
Research problems that can be approached particularly well using qualitative methods include assessing complex multi-component interventions or systems (of change), addressing questions beyond “what works”, towards “what works for whom when, how and why”, and focussing on intervention improvement rather than accreditation [ 7 , 9 , 10 , 11 , 12 ]. Using qualitative methods can also help shed light on the “softer” side of medical treatment. For example, while quantitative trials can measure the costs and benefits of neuro-oncological treatment in terms of survival rates or adverse effects, qualitative research can help provide a better understanding of patient or caregiver stress, visibility of illness or out-of-pocket expenses.
How to conduct qualitative research?
Given that qualitative research is characterised by flexibility, openness and responsivity to context, the steps of data collection and analysis are not as separate and consecutive as they tend to be in quantitative research [ 13 , 14 ]. As Fossey puts it : “sampling, data collection, analysis and interpretation are related to each other in a cyclical (iterative) manner, rather than following one after another in a stepwise approach” [ 15 ]. The researcher can make educated decisions with regard to the choice of method, how they are implemented, and to which and how many units they are applied [ 13 ]. As shown in Fig. 1 , this can involve several back-and-forth steps between data collection and analysis where new insights and experiences can lead to adaption and expansion of the original plan. Some insights may also necessitate a revision of the research question and/or the research design as a whole. The process ends when saturation is achieved, i.e. when no relevant new information can be found (see also below: sampling and saturation). For reasons of transparency, it is essential for all decisions as well as the underlying reasoning to be well-documented.
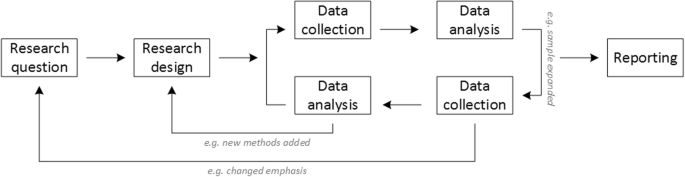
Iterative research process
While it is not always explicitly addressed, qualitative methods reflect a different underlying research paradigm than quantitative research (e.g. constructivism or interpretivism as opposed to positivism). The choice of methods can be based on the respective underlying substantive theory or theoretical framework used by the researcher [ 2 ].
Data collection
The methods of qualitative data collection most commonly used in health research are document study, observations, semi-structured interviews and focus groups [ 1 , 14 , 16 , 17 ].
Document study
Document study (also called document analysis) refers to the review by the researcher of written materials [ 14 ]. These can include personal and non-personal documents such as archives, annual reports, guidelines, policy documents, diaries or letters.
Observations
Observations are particularly useful to gain insights into a certain setting and actual behaviour – as opposed to reported behaviour or opinions [ 13 ]. Qualitative observations can be either participant or non-participant in nature. In participant observations, the observer is part of the observed setting, for example a nurse working in an intensive care unit [ 18 ]. In non-participant observations, the observer is “on the outside looking in”, i.e. present in but not part of the situation, trying not to influence the setting by their presence. Observations can be planned (e.g. for 3 h during the day or night shift) or ad hoc (e.g. as soon as a stroke patient arrives at the emergency room). During the observation, the observer takes notes on everything or certain pre-determined parts of what is happening around them, for example focusing on physician-patient interactions or communication between different professional groups. Written notes can be taken during or after the observations, depending on feasibility (which is usually lower during participant observations) and acceptability (e.g. when the observer is perceived to be judging the observed). Afterwards, these field notes are transcribed into observation protocols. If more than one observer was involved, field notes are taken independently, but notes can be consolidated into one protocol after discussions. Advantages of conducting observations include minimising the distance between the researcher and the researched, the potential discovery of topics that the researcher did not realise were relevant and gaining deeper insights into the real-world dimensions of the research problem at hand [ 18 ].
Semi-structured interviews
Hijmans & Kuyper describe qualitative interviews as “an exchange with an informal character, a conversation with a goal” [ 19 ]. Interviews are used to gain insights into a person’s subjective experiences, opinions and motivations – as opposed to facts or behaviours [ 13 ]. Interviews can be distinguished by the degree to which they are structured (i.e. a questionnaire), open (e.g. free conversation or autobiographical interviews) or semi-structured [ 2 , 13 ]. Semi-structured interviews are characterized by open-ended questions and the use of an interview guide (or topic guide/list) in which the broad areas of interest, sometimes including sub-questions, are defined [ 19 ]. The pre-defined topics in the interview guide can be derived from the literature, previous research or a preliminary method of data collection, e.g. document study or observations. The topic list is usually adapted and improved at the start of the data collection process as the interviewer learns more about the field [ 20 ]. Across interviews the focus on the different (blocks of) questions may differ and some questions may be skipped altogether (e.g. if the interviewee is not able or willing to answer the questions or for concerns about the total length of the interview) [ 20 ]. Qualitative interviews are usually not conducted in written format as it impedes on the interactive component of the method [ 20 ]. In comparison to written surveys, qualitative interviews have the advantage of being interactive and allowing for unexpected topics to emerge and to be taken up by the researcher. This can also help overcome a provider or researcher-centred bias often found in written surveys, which by nature, can only measure what is already known or expected to be of relevance to the researcher. Interviews can be audio- or video-taped; but sometimes it is only feasible or acceptable for the interviewer to take written notes [ 14 , 16 , 20 ].
Focus groups
Focus groups are group interviews to explore participants’ expertise and experiences, including explorations of how and why people behave in certain ways [ 1 ]. Focus groups usually consist of 6–8 people and are led by an experienced moderator following a topic guide or “script” [ 21 ]. They can involve an observer who takes note of the non-verbal aspects of the situation, possibly using an observation guide [ 21 ]. Depending on researchers’ and participants’ preferences, the discussions can be audio- or video-taped and transcribed afterwards [ 21 ]. Focus groups are useful for bringing together homogeneous (to a lesser extent heterogeneous) groups of participants with relevant expertise and experience on a given topic on which they can share detailed information [ 21 ]. Focus groups are a relatively easy, fast and inexpensive method to gain access to information on interactions in a given group, i.e. “the sharing and comparing” among participants [ 21 ]. Disadvantages include less control over the process and a lesser extent to which each individual may participate. Moreover, focus group moderators need experience, as do those tasked with the analysis of the resulting data. Focus groups can be less appropriate for discussing sensitive topics that participants might be reluctant to disclose in a group setting [ 13 ]. Moreover, attention must be paid to the emergence of “groupthink” as well as possible power dynamics within the group, e.g. when patients are awed or intimidated by health professionals.
Choosing the “right” method
As explained above, the school of thought underlying qualitative research assumes no objective hierarchy of evidence and methods. This means that each choice of single or combined methods has to be based on the research question that needs to be answered and a critical assessment with regard to whether or to what extent the chosen method can accomplish this – i.e. the “fit” between question and method [ 14 ]. It is necessary for these decisions to be documented when they are being made, and to be critically discussed when reporting methods and results.
Let us assume that our research aim is to examine the (clinical) processes around acute endovascular treatment (EVT), from the patient’s arrival at the emergency room to recanalization, with the aim to identify possible causes for delay and/or other causes for sub-optimal treatment outcome. As a first step, we could conduct a document study of the relevant standard operating procedures (SOPs) for this phase of care – are they up-to-date and in line with current guidelines? Do they contain any mistakes, irregularities or uncertainties that could cause delays or other problems? Regardless of the answers to these questions, the results have to be interpreted based on what they are: a written outline of what care processes in this hospital should look like. If we want to know what they actually look like in practice, we can conduct observations of the processes described in the SOPs. These results can (and should) be analysed in themselves, but also in comparison to the results of the document analysis, especially as regards relevant discrepancies. Do the SOPs outline specific tests for which no equipment can be observed or tasks to be performed by specialized nurses who are not present during the observation? It might also be possible that the written SOP is outdated, but the actual care provided is in line with current best practice. In order to find out why these discrepancies exist, it can be useful to conduct interviews. Are the physicians simply not aware of the SOPs (because their existence is limited to the hospital’s intranet) or do they actively disagree with them or does the infrastructure make it impossible to provide the care as described? Another rationale for adding interviews is that some situations (or all of their possible variations for different patient groups or the day, night or weekend shift) cannot practically or ethically be observed. In this case, it is possible to ask those involved to report on their actions – being aware that this is not the same as the actual observation. A senior physician’s or hospital manager’s description of certain situations might differ from a nurse’s or junior physician’s one, maybe because they intentionally misrepresent facts or maybe because different aspects of the process are visible or important to them. In some cases, it can also be relevant to consider to whom the interviewee is disclosing this information – someone they trust, someone they are otherwise not connected to, or someone they suspect or are aware of being in a potentially “dangerous” power relationship to them. Lastly, a focus group could be conducted with representatives of the relevant professional groups to explore how and why exactly they provide care around EVT. The discussion might reveal discrepancies (between SOPs and actual care or between different physicians) and motivations to the researchers as well as to the focus group members that they might not have been aware of themselves. For the focus group to deliver relevant information, attention has to be paid to its composition and conduct, for example, to make sure that all participants feel safe to disclose sensitive or potentially problematic information or that the discussion is not dominated by (senior) physicians only. The resulting combination of data collection methods is shown in Fig. 2 .

Possible combination of data collection methods
Attributions for icons: “Book” by Serhii Smirnov, “Interview” by Adrien Coquet, FR, “Magnifying Glass” by anggun, ID, “Business communication” by Vectors Market; all from the Noun Project
The combination of multiple data source as described for this example can be referred to as “triangulation”, in which multiple measurements are carried out from different angles to achieve a more comprehensive understanding of the phenomenon under study [ 22 , 23 ].
Data analysis
To analyse the data collected through observations, interviews and focus groups these need to be transcribed into protocols and transcripts (see Fig. 3 ). Interviews and focus groups can be transcribed verbatim , with or without annotations for behaviour (e.g. laughing, crying, pausing) and with or without phonetic transcription of dialects and filler words, depending on what is expected or known to be relevant for the analysis. In the next step, the protocols and transcripts are coded , that is, marked (or tagged, labelled) with one or more short descriptors of the content of a sentence or paragraph [ 2 , 15 , 23 ]. Jansen describes coding as “connecting the raw data with “theoretical” terms” [ 20 ]. In a more practical sense, coding makes raw data sortable. This makes it possible to extract and examine all segments describing, say, a tele-neurology consultation from multiple data sources (e.g. SOPs, emergency room observations, staff and patient interview). In a process of synthesis and abstraction, the codes are then grouped, summarised and/or categorised [ 15 , 20 ]. The end product of the coding or analysis process is a descriptive theory of the behavioural pattern under investigation [ 20 ]. The coding process is performed using qualitative data management software, the most common ones being InVivo, MaxQDA and Atlas.ti. It should be noted that these are data management tools which support the analysis performed by the researcher(s) [ 14 ].
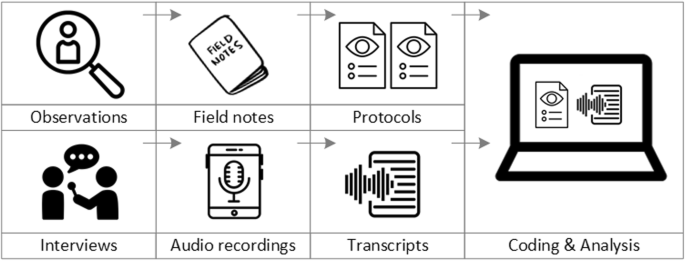
From data collection to data analysis
Attributions for icons: see Fig. 2 , also “Speech to text” by Trevor Dsouza, “Field Notes” by Mike O’Brien, US, “Voice Record” by ProSymbols, US, “Inspection” by Made, AU, and “Cloud” by Graphic Tigers; all from the Noun Project
How to report qualitative research?
Protocols of qualitative research can be published separately and in advance of the study results. However, the aim is not the same as in RCT protocols, i.e. to pre-define and set in stone the research questions and primary or secondary endpoints. Rather, it is a way to describe the research methods in detail, which might not be possible in the results paper given journals’ word limits. Qualitative research papers are usually longer than their quantitative counterparts to allow for deep understanding and so-called “thick description”. In the methods section, the focus is on transparency of the methods used, including why, how and by whom they were implemented in the specific study setting, so as to enable a discussion of whether and how this may have influenced data collection, analysis and interpretation. The results section usually starts with a paragraph outlining the main findings, followed by more detailed descriptions of, for example, the commonalities, discrepancies or exceptions per category [ 20 ]. Here it is important to support main findings by relevant quotations, which may add information, context, emphasis or real-life examples [ 20 , 23 ]. It is subject to debate in the field whether it is relevant to state the exact number or percentage of respondents supporting a certain statement (e.g. “Five interviewees expressed negative feelings towards XYZ”) [ 21 ].
How to combine qualitative with quantitative research?
Qualitative methods can be combined with other methods in multi- or mixed methods designs, which “[employ] two or more different methods [ …] within the same study or research program rather than confining the research to one single method” [ 24 ]. Reasons for combining methods can be diverse, including triangulation for corroboration of findings, complementarity for illustration and clarification of results, expansion to extend the breadth and range of the study, explanation of (unexpected) results generated with one method with the help of another, or offsetting the weakness of one method with the strength of another [ 1 , 17 , 24 , 25 , 26 ]. The resulting designs can be classified according to when, why and how the different quantitative and/or qualitative data strands are combined. The three most common types of mixed method designs are the convergent parallel design , the explanatory sequential design and the exploratory sequential design. The designs with examples are shown in Fig. 4 .

Three common mixed methods designs
In the convergent parallel design, a qualitative study is conducted in parallel to and independently of a quantitative study, and the results of both studies are compared and combined at the stage of interpretation of results. Using the above example of EVT provision, this could entail setting up a quantitative EVT registry to measure process times and patient outcomes in parallel to conducting the qualitative research outlined above, and then comparing results. Amongst other things, this would make it possible to assess whether interview respondents’ subjective impressions of patients receiving good care match modified Rankin Scores at follow-up, or whether observed delays in care provision are exceptions or the rule when compared to door-to-needle times as documented in the registry. In the explanatory sequential design, a quantitative study is carried out first, followed by a qualitative study to help explain the results from the quantitative study. This would be an appropriate design if the registry alone had revealed relevant delays in door-to-needle times and the qualitative study would be used to understand where and why these occurred, and how they could be improved. In the exploratory design, the qualitative study is carried out first and its results help informing and building the quantitative study in the next step [ 26 ]. If the qualitative study around EVT provision had shown a high level of dissatisfaction among the staff members involved, a quantitative questionnaire investigating staff satisfaction could be set up in the next step, informed by the qualitative study on which topics dissatisfaction had been expressed. Amongst other things, the questionnaire design would make it possible to widen the reach of the research to more respondents from different (types of) hospitals, regions, countries or settings, and to conduct sub-group analyses for different professional groups.
How to assess qualitative research?
A variety of assessment criteria and lists have been developed for qualitative research, ranging in their focus and comprehensiveness [ 14 , 17 , 27 ]. However, none of these has been elevated to the “gold standard” in the field. In the following, we therefore focus on a set of commonly used assessment criteria that, from a practical standpoint, a researcher can look for when assessing a qualitative research report or paper.
Assessors should check the authors’ use of and adherence to the relevant reporting checklists (e.g. Standards for Reporting Qualitative Research (SRQR)) to make sure all items that are relevant for this type of research are addressed [ 23 , 28 ]. Discussions of quantitative measures in addition to or instead of these qualitative measures can be a sign of lower quality of the research (paper). Providing and adhering to a checklist for qualitative research contributes to an important quality criterion for qualitative research, namely transparency [ 15 , 17 , 23 ].
Reflexivity
While methodological transparency and complete reporting is relevant for all types of research, some additional criteria must be taken into account for qualitative research. This includes what is called reflexivity, i.e. sensitivity to the relationship between the researcher and the researched, including how contact was established and maintained, or the background and experience of the researcher(s) involved in data collection and analysis. Depending on the research question and population to be researched this can be limited to professional experience, but it may also include gender, age or ethnicity [ 17 , 27 ]. These details are relevant because in qualitative research, as opposed to quantitative research, the researcher as a person cannot be isolated from the research process [ 23 ]. It may influence the conversation when an interviewed patient speaks to an interviewer who is a physician, or when an interviewee is asked to discuss a gynaecological procedure with a male interviewer, and therefore the reader must be made aware of these details [ 19 ].
Sampling and saturation
The aim of qualitative sampling is for all variants of the objects of observation that are deemed relevant for the study to be present in the sample “ to see the issue and its meanings from as many angles as possible” [ 1 , 16 , 19 , 20 , 27 ] , and to ensure “information-richness [ 15 ]. An iterative sampling approach is advised, in which data collection (e.g. five interviews) is followed by data analysis, followed by more data collection to find variants that are lacking in the current sample. This process continues until no new (relevant) information can be found and further sampling becomes redundant – which is called saturation [ 1 , 15 ] . In other words: qualitative data collection finds its end point not a priori , but when the research team determines that saturation has been reached [ 29 , 30 ].
This is also the reason why most qualitative studies use deliberate instead of random sampling strategies. This is generally referred to as “ purposive sampling” , in which researchers pre-define which types of participants or cases they need to include so as to cover all variations that are expected to be of relevance, based on the literature, previous experience or theory (i.e. theoretical sampling) [ 14 , 20 ]. Other types of purposive sampling include (but are not limited to) maximum variation sampling, critical case sampling or extreme or deviant case sampling [ 2 ]. In the above EVT example, a purposive sample could include all relevant professional groups and/or all relevant stakeholders (patients, relatives) and/or all relevant times of observation (day, night and weekend shift).
Assessors of qualitative research should check whether the considerations underlying the sampling strategy were sound and whether or how researchers tried to adapt and improve their strategies in stepwise or cyclical approaches between data collection and analysis to achieve saturation [ 14 ].
Good qualitative research is iterative in nature, i.e. it goes back and forth between data collection and analysis, revising and improving the approach where necessary. One example of this are pilot interviews, where different aspects of the interview (especially the interview guide, but also, for example, the site of the interview or whether the interview can be audio-recorded) are tested with a small number of respondents, evaluated and revised [ 19 ]. In doing so, the interviewer learns which wording or types of questions work best, or which is the best length of an interview with patients who have trouble concentrating for an extended time. Of course, the same reasoning applies to observations or focus groups which can also be piloted.
Ideally, coding should be performed by at least two researchers, especially at the beginning of the coding process when a common approach must be defined, including the establishment of a useful coding list (or tree), and when a common meaning of individual codes must be established [ 23 ]. An initial sub-set or all transcripts can be coded independently by the coders and then compared and consolidated after regular discussions in the research team. This is to make sure that codes are applied consistently to the research data.
Member checking
Member checking, also called respondent validation , refers to the practice of checking back with study respondents to see if the research is in line with their views [ 14 , 27 ]. This can happen after data collection or analysis or when first results are available [ 23 ]. For example, interviewees can be provided with (summaries of) their transcripts and asked whether they believe this to be a complete representation of their views or whether they would like to clarify or elaborate on their responses [ 17 ]. Respondents’ feedback on these issues then becomes part of the data collection and analysis [ 27 ].
Stakeholder involvement
In those niches where qualitative approaches have been able to evolve and grow, a new trend has seen the inclusion of patients and their representatives not only as study participants (i.e. “members”, see above) but as consultants to and active participants in the broader research process [ 31 , 32 , 33 ]. The underlying assumption is that patients and other stakeholders hold unique perspectives and experiences that add value beyond their own single story, making the research more relevant and beneficial to researchers, study participants and (future) patients alike [ 34 , 35 ]. Using the example of patients on or nearing dialysis, a recent scoping review found that 80% of clinical research did not address the top 10 research priorities identified by patients and caregivers [ 32 , 36 ]. In this sense, the involvement of the relevant stakeholders, especially patients and relatives, is increasingly being seen as a quality indicator in and of itself.
How not to assess qualitative research
The above overview does not include certain items that are routine in assessments of quantitative research. What follows is a non-exhaustive, non-representative, experience-based list of the quantitative criteria often applied to the assessment of qualitative research, as well as an explanation of the limited usefulness of these endeavours.
Protocol adherence
Given the openness and flexibility of qualitative research, it should not be assessed by how well it adheres to pre-determined and fixed strategies – in other words: its rigidity. Instead, the assessor should look for signs of adaptation and refinement based on lessons learned from earlier steps in the research process.
Sample size
For the reasons explained above, qualitative research does not require specific sample sizes, nor does it require that the sample size be determined a priori [ 1 , 14 , 27 , 37 , 38 , 39 ]. Sample size can only be a useful quality indicator when related to the research purpose, the chosen methodology and the composition of the sample, i.e. who was included and why.
Randomisation
While some authors argue that randomisation can be used in qualitative research, this is not commonly the case, as neither its feasibility nor its necessity or usefulness has been convincingly established for qualitative research [ 13 , 27 ]. Relevant disadvantages include the negative impact of a too large sample size as well as the possibility (or probability) of selecting “ quiet, uncooperative or inarticulate individuals ” [ 17 ]. Qualitative studies do not use control groups, either.
Interrater reliability, variability and other “objectivity checks”
The concept of “interrater reliability” is sometimes used in qualitative research to assess to which extent the coding approach overlaps between the two co-coders. However, it is not clear what this measure tells us about the quality of the analysis [ 23 ]. This means that these scores can be included in qualitative research reports, preferably with some additional information on what the score means for the analysis, but it is not a requirement. Relatedly, it is not relevant for the quality or “objectivity” of qualitative research to separate those who recruited the study participants and collected and analysed the data. Experiences even show that it might be better to have the same person or team perform all of these tasks [ 20 ]. First, when researchers introduce themselves during recruitment this can enhance trust when the interview takes place days or weeks later with the same researcher. Second, when the audio-recording is transcribed for analysis, the researcher conducting the interviews will usually remember the interviewee and the specific interview situation during data analysis. This might be helpful in providing additional context information for interpretation of data, e.g. on whether something might have been meant as a joke [ 18 ].
Not being quantitative research
Being qualitative research instead of quantitative research should not be used as an assessment criterion if it is used irrespectively of the research problem at hand. Similarly, qualitative research should not be required to be combined with quantitative research per se – unless mixed methods research is judged as inherently better than single-method research. In this case, the same criterion should be applied for quantitative studies without a qualitative component.
The main take-away points of this paper are summarised in Table 1 . We aimed to show that, if conducted well, qualitative research can answer specific research questions that cannot to be adequately answered using (only) quantitative designs. Seeing qualitative and quantitative methods as equal will help us become more aware and critical of the “fit” between the research problem and our chosen methods: I can conduct an RCT to determine the reasons for transportation delays of acute stroke patients – but should I? It also provides us with a greater range of tools to tackle a greater range of research problems more appropriately and successfully, filling in the blind spots on one half of the methodological spectrum to better address the whole complexity of neurological research and practice.
Availability of data and materials
Not applicable.
Abbreviations
Endovascular treatment
Randomised Controlled Trial
Standard Operating Procedure
Standards for Reporting Qualitative Research
Philipsen, H., & Vernooij-Dassen, M. (2007). Kwalitatief onderzoek: nuttig, onmisbaar en uitdagend. In L. PLBJ & H. TCo (Eds.), Kwalitatief onderzoek: Praktische methoden voor de medische praktijk . [Qualitative research: useful, indispensable and challenging. In: Qualitative research: Practical methods for medical practice (pp. 5–12). Houten: Bohn Stafleu van Loghum.
Chapter Google Scholar
Punch, K. F. (2013). Introduction to social research: Quantitative and qualitative approaches . London: Sage.
Kelly, J., Dwyer, J., Willis, E., & Pekarsky, B. (2014). Travelling to the city for hospital care: Access factors in country aboriginal patient journeys. Australian Journal of Rural Health, 22 (3), 109–113.
Article Google Scholar
Nilsen, P., Ståhl, C., Roback, K., & Cairney, P. (2013). Never the twain shall meet? - a comparison of implementation science and policy implementation research. Implementation Science, 8 (1), 1–12.
Howick J, Chalmers I, Glasziou, P., Greenhalgh, T., Heneghan, C., Liberati, A., Moschetti, I., Phillips, B., & Thornton, H. (2011). The 2011 Oxford CEBM evidence levels of evidence (introductory document) . Oxford Center for Evidence Based Medicine. https://www.cebm.net/2011/06/2011-oxford-cebm-levels-evidence-introductory-document/ .
Eakin, J. M. (2016). Educating critical qualitative health researchers in the land of the randomized controlled trial. Qualitative Inquiry, 22 (2), 107–118.
May, A., & Mathijssen, J. (2015). Alternatieven voor RCT bij de evaluatie van effectiviteit van interventies!? Eindrapportage. In Alternatives for RCTs in the evaluation of effectiveness of interventions!? Final report .
Google Scholar
Berwick, D. M. (2008). The science of improvement. Journal of the American Medical Association, 299 (10), 1182–1184.
Article CAS Google Scholar
Christ, T. W. (2014). Scientific-based research and randomized controlled trials, the “gold” standard? Alternative paradigms and mixed methodologies. Qualitative Inquiry, 20 (1), 72–80.
Lamont, T., Barber, N., Jd, P., Fulop, N., Garfield-Birkbeck, S., Lilford, R., Mear, L., Raine, R., & Fitzpatrick, R. (2016). New approaches to evaluating complex health and care systems. BMJ, 352:i154.
Drabble, S. J., & O’Cathain, A. (2015). Moving from Randomized Controlled Trials to Mixed Methods Intervention Evaluation. In S. Hesse-Biber & R. B. Johnson (Eds.), The Oxford Handbook of Multimethod and Mixed Methods Research Inquiry (pp. 406–425). London: Oxford University Press.
Chambers, D. A., Glasgow, R. E., & Stange, K. C. (2013). The dynamic sustainability framework: Addressing the paradox of sustainment amid ongoing change. Implementation Science : IS, 8 , 117.
Hak, T. (2007). Waarnemingsmethoden in kwalitatief onderzoek. In L. PLBJ & H. TCo (Eds.), Kwalitatief onderzoek: Praktische methoden voor de medische praktijk . [Observation methods in qualitative research] (pp. 13–25). Houten: Bohn Stafleu van Loghum.
Russell, C. K., & Gregory, D. M. (2003). Evaluation of qualitative research studies. Evidence Based Nursing, 6 (2), 36–40.
Fossey, E., Harvey, C., McDermott, F., & Davidson, L. (2002). Understanding and evaluating qualitative research. Australian and New Zealand Journal of Psychiatry, 36 , 717–732.
Yanow, D. (2000). Conducting interpretive policy analysis (Vol. 47). Thousand Oaks: Sage University Papers Series on Qualitative Research Methods.
Shenton, A. K. (2004). Strategies for ensuring trustworthiness in qualitative research projects. Education for Information, 22 , 63–75.
van der Geest, S. (2006). Participeren in ziekte en zorg: meer over kwalitatief onderzoek. Huisarts en Wetenschap, 49 (4), 283–287.
Hijmans, E., & Kuyper, M. (2007). Het halfopen interview als onderzoeksmethode. In L. PLBJ & H. TCo (Eds.), Kwalitatief onderzoek: Praktische methoden voor de medische praktijk . [The half-open interview as research method (pp. 43–51). Houten: Bohn Stafleu van Loghum.
Jansen, H. (2007). Systematiek en toepassing van de kwalitatieve survey. In L. PLBJ & H. TCo (Eds.), Kwalitatief onderzoek: Praktische methoden voor de medische praktijk . [Systematics and implementation of the qualitative survey (pp. 27–41). Houten: Bohn Stafleu van Loghum.
Pv, R., & Peremans, L. (2007). Exploreren met focusgroepgesprekken: de ‘stem’ van de groep onder de loep. In L. PLBJ & H. TCo (Eds.), Kwalitatief onderzoek: Praktische methoden voor de medische praktijk . [Exploring with focus group conversations: the “voice” of the group under the magnifying glass (pp. 53–64). Houten: Bohn Stafleu van Loghum.
Carter, N., Bryant-Lukosius, D., DiCenso, A., Blythe, J., & Neville, A. J. (2014). The use of triangulation in qualitative research. Oncology Nursing Forum, 41 (5), 545–547.
Boeije H: Analyseren in kwalitatief onderzoek: Denken en doen, [Analysis in qualitative research: Thinking and doing] vol. Den Haag Boom Lemma uitgevers; 2012.
Hunter, A., & Brewer, J. (2015). Designing Multimethod Research. In S. Hesse-Biber & R. B. Johnson (Eds.), The Oxford Handbook of Multimethod and Mixed Methods Research Inquiry (pp. 185–205). London: Oxford University Press.
Archibald, M. M., Radil, A. I., Zhang, X., & Hanson, W. E. (2015). Current mixed methods practices in qualitative research: A content analysis of leading journals. International Journal of Qualitative Methods, 14 (2), 5–33.
Creswell, J. W., & Plano Clark, V. L. (2011). Choosing a Mixed Methods Design. In Designing and Conducting Mixed Methods Research . Thousand Oaks: SAGE Publications.
Mays, N., & Pope, C. (2000). Assessing quality in qualitative research. BMJ, 320 (7226), 50–52.
O'Brien, B. C., Harris, I. B., Beckman, T. J., Reed, D. A., & Cook, D. A. (2014). Standards for reporting qualitative research: A synthesis of recommendations. Academic Medicine : Journal of the Association of American Medical Colleges, 89 (9), 1245–1251.
Saunders, B., Sim, J., Kingstone, T., Baker, S., Waterfield, J., Bartlam, B., Burroughs, H., & Jinks, C. (2018). Saturation in qualitative research: Exploring its conceptualization and operationalization. Quality and Quantity, 52 (4), 1893–1907.
Moser, A., & Korstjens, I. (2018). Series: Practical guidance to qualitative research. Part 3: Sampling, data collection and analysis. European Journal of General Practice, 24 (1), 9–18.
Marlett, N., Shklarov, S., Marshall, D., Santana, M. J., & Wasylak, T. (2015). Building new roles and relationships in research: A model of patient engagement research. Quality of Life Research : an international journal of quality of life aspects of treatment, care and rehabilitation, 24 (5), 1057–1067.
Demian, M. N., Lam, N. N., Mac-Way, F., Sapir-Pichhadze, R., & Fernandez, N. (2017). Opportunities for engaging patients in kidney research. Canadian Journal of Kidney Health and Disease, 4 , 2054358117703070–2054358117703070.
Noyes, J., McLaughlin, L., Morgan, K., Roberts, A., Stephens, M., Bourne, J., Houlston, M., Houlston, J., Thomas, S., Rhys, R. G., et al. (2019). Designing a co-productive study to overcome known methodological challenges in organ donation research with bereaved family members. Health Expectations . 22(4):824–35.
Piil, K., Jarden, M., & Pii, K. H. (2019). Research agenda for life-threatening cancer. European Journal Cancer Care (Engl), 28 (1), e12935.
Hofmann, D., Ibrahim, F., Rose, D., Scott, D. L., Cope, A., Wykes, T., & Lempp, H. (2015). Expectations of new treatment in rheumatoid arthritis: Developing a patient-generated questionnaire. Health Expectations : an international journal of public participation in health care and health policy, 18 (5), 995–1008.
Jun, M., Manns, B., Laupacis, A., Manns, L., Rehal, B., Crowe, S., & Hemmelgarn, B. R. (2015). Assessing the extent to which current clinical research is consistent with patient priorities: A scoping review using a case study in patients on or nearing dialysis. Canadian Journal of Kidney Health and Disease, 2 , 35.
Elsie Baker, S., & Edwards, R. (2012). How many qualitative interviews is enough? In National Centre for Research Methods Review Paper . National Centre for Research Methods. http://eprints.ncrm.ac.uk/2273/4/how_many_interviews.pdf .
Sandelowski, M. (1995). Sample size in qualitative research. Research in Nursing & Health, 18 (2), 179–183.
Sim, J., Saunders, B., Waterfield, J., & Kingstone, T. (2018). Can sample size in qualitative research be determined a priori? International Journal of Social Research Methodology, 21 (5), 619–634.
Download references
Acknowledgements
no external funding.
Author information
Authors and affiliations.
Department of Neurology, Heidelberg University Hospital, Im Neuenheimer Feld 400, 69120, Heidelberg, Germany
Loraine Busetto, Wolfgang Wick & Christoph Gumbinger
Clinical Cooperation Unit Neuro-Oncology, German Cancer Research Center, Heidelberg, Germany
Wolfgang Wick
You can also search for this author in PubMed Google Scholar
Contributions
LB drafted the manuscript; WW and CG revised the manuscript; all authors approved the final versions.
Corresponding author
Correspondence to Loraine Busetto .
Ethics declarations
Ethics approval and consent to participate, consent for publication, competing interests.
The authors declare no competing interests.
Additional information
Publisher’s note.
Springer Nature remains neutral with regard to jurisdictional claims in published maps and institutional affiliations.
Rights and permissions
Open Access This article is licensed under a Creative Commons Attribution 4.0 International License, which permits use, sharing, adaptation, distribution and reproduction in any medium or format, as long as you give appropriate credit to the original author(s) and the source, provide a link to the Creative Commons licence, and indicate if changes were made. The images or other third party material in this article are included in the article's Creative Commons licence, unless indicated otherwise in a credit line to the material. If material is not included in the article's Creative Commons licence and your intended use is not permitted by statutory regulation or exceeds the permitted use, you will need to obtain permission directly from the copyright holder. To view a copy of this licence, visit http://creativecommons.org/licenses/by/4.0/ .
Reprints and permissions
About this article
Cite this article.
Busetto, L., Wick, W. & Gumbinger, C. How to use and assess qualitative research methods. Neurol. Res. Pract. 2 , 14 (2020). https://doi.org/10.1186/s42466-020-00059-z
Download citation
Received : 30 January 2020
Accepted : 22 April 2020
Published : 27 May 2020
DOI : https://doi.org/10.1186/s42466-020-00059-z
Share this article
Anyone you share the following link with will be able to read this content:
Sorry, a shareable link is not currently available for this article.
Provided by the Springer Nature SharedIt content-sharing initiative
- Qualitative research
- Mixed methods
- Quality assessment
Neurological Research and Practice
ISSN: 2524-3489
- Submission enquiries: Access here and click Contact Us
- General enquiries: [email protected]
- PRO Courses Guides New Tech Help Pro Expert Videos About wikiHow Pro Upgrade Sign In
- EDIT Edit this Article
- EXPLORE Tech Help Pro About Us Random Article Quizzes Request a New Article Community Dashboard This Or That Game Popular Categories Arts and Entertainment Artwork Books Movies Computers and Electronics Computers Phone Skills Technology Hacks Health Men's Health Mental Health Women's Health Relationships Dating Love Relationship Issues Hobbies and Crafts Crafts Drawing Games Education & Communication Communication Skills Personal Development Studying Personal Care and Style Fashion Hair Care Personal Hygiene Youth Personal Care School Stuff Dating All Categories Arts and Entertainment Finance and Business Home and Garden Relationship Quizzes Cars & Other Vehicles Food and Entertaining Personal Care and Style Sports and Fitness Computers and Electronics Health Pets and Animals Travel Education & Communication Hobbies and Crafts Philosophy and Religion Work World Family Life Holidays and Traditions Relationships Youth
- Browse Articles
- Learn Something New
- Quizzes Hot
- This Or That Game
- Train Your Brain
- Explore More
- Support wikiHow
- About wikiHow
- Log in / Sign up
- Education and Communications
How to Do Qualitative Research
Last Updated: October 26, 2022 Fact Checked
This article was co-authored by Jeremiah Kaplan . Jeremiah Kaplan is a Research and Training Specialist at the Center for Applied Behavioral Health Policy at Arizona State University. He has extensive knowledge and experience in motivational interviewing. In addition, Jeremiah has worked in the mental health, youth engagement, and trauma-informed care fields. Using his expertise, Jeremiah supervises Arizona State University’s Motivational Interviewing Coding Lab. Jeremiah has also been internationally selected to participate in the Motivational Interviewing International Network of Trainers sponsored Train the Trainer event. Jeremiah holds a BS in Human Services with a concentration in Family and Children from The University of Phoenix. There are 10 references cited in this article, which can be found at the bottom of the page. This article has been fact-checked, ensuring the accuracy of any cited facts and confirming the authority of its sources. This article has been viewed 747,099 times.
Qualitative research is a broad field of inquiry that uses unstructured data collections methods, such as observations, interviews, surveys and documents, to find themes and meanings to inform our understanding of the world. [1] X Trustworthy Source PubMed Central Journal archive from the U.S. National Institutes of Health Go to source Qualitative research tends to try to cover the reasons for behaviors, attitudes and motivations, instead of just the details of what, where and when. Qualitative research can be done across many disciplines, such as social sciences, healthcare and businesses, and it is a common feature of nearly every single workplace and educational environment.
Preparing Your Research

- The research questions is one of the most important pieces of your research design. It determines what you want to learn or understand and also helps to focus the study, since you can't investigate everything at once. Your research question will also shape how you conduct your study since different questions require different methods of inquiry.
- You should start with a burning question and then narrow it down more to make it manageable enough to be researched effectively. For example, "what is the meaning of teachers' work to teachers" is too broad for a single research endeavor, but if that's what you're interested you could narrow it by limiting the type of teacher or focusing on one level of education. For example, "what is the meaning of teachers' work to second career teachers?" or "what is the meaning of teachers' work to junior high teachers?"
Tip: Find the balance between a burning question and a researchable question. The former is something you really want to know about and is often quite broad. The latter is one that can be directly investigated using available research methods and tools.

- For example, if your research question focuses on how second career teachers attribute meaning to their work, you would want to examine the literature on second career teaching - what motivates people to turn to teaching as a second career? How many teachers are in their second career? Where do most second career teachers work? Doing this reading and review of existing literature and research will help you refine your question and give you the base you need for your own research. It will also give you a sense of the variables that might impact your research (e.g., age, gender, class, etc.) and that you will need to take into consideration in your own study.
- A literature review will also help you to determine whether you are really interested and committed to the topic and research question and that there is a gap in the existing research that you want to fill by conducting your own investigation.

For example, if your research question is "what is the meaning of teachers' work to second career teachers?" , that is not a question that can be answered with a 'yes' or 'no'. Nor is there likely to be a single overarching answer. This means that qualitative research is the best route.

- Consider the possible outcomes. Because qualitative methodologies are generally quite broad, there is almost always the possibility that some useful data will come out of the research. This is different than in a quantitative experiment, where an unproven hypothesis can mean that a lot of time has been wasted.
- Your research budget and available financial resources should also be considered. Qualitative research is often cheaper and easier to plan and execute. For example, it is usually easier and cost-saving to gather a small number of people for interviews than it is to purchase a computer program that can do statistical analysis and hire the appropriate statisticians.

- Action Research – Action research focuses on solving an immediate problem or working with others to solve problem and address particular issues. [7] X Research source
- Ethnography – Ethnography is the study of human interaction in communities through direct participation and observation within the community you wish to study. Ethnographic research comes from the discipline of social and cultural anthropology but is now becoming more widely used. [8] X Research source
- Phenomenology – Phenomenology is the study of the subjective experiences of others. It researches the world through the eyes of another person by discovering how they interpret their experiences. [9] X Research source
- Grounded Theory – The purpose of grounded theory is to develop theory based on the data systematically collected and analyzed. It looks at specific information and derives theories and reasons for the phenomena.
- Case Study Research – This method of qualitative study is an in-depth study of a specific individual or phenomena in its existing context. [10] X Research source
Collecting and Analyzing Your Data

- Direct observation – Direct observation of a situation or your research subjects can occur through video tape playback or through live observation. In direct observation, you are making specific observations of a situation without influencing or participating in any way. [12] X Research source For example, perhaps you want to see how second career teachers go about their routines in and outside the classrooms and so you decide to observe them for a few days, being sure to get the requisite permission from the school, students and the teacher and taking careful notes along the way.
- Participant observation – Participant observation is the immersion of the researcher in the community or situation being studied. This form of data collection tends to be more time consuming, as you need to participate fully in the community in order to know whether your observations are valid. [13] X Research source
- Interviews – Qualitative interviewing is basically the process of gathering data by asking people questions. Interviewing can be very flexible - they can be on-on-one, but can also take place over the phone or Internet or in small groups called "focus groups". There are also different types of interviews. Structured interviews use pre-set questions, whereas unstructured interviews are more free-flowing conversations where the interviewer can probe and explore topics as they come up. Interviews are particularly useful if you want to know how people feel or react to something. For example, it would be very useful to sit down with second career teachers in either a structured or unstructured interview to gain information about how they represent and discuss their teaching careers.
- Surveys – Written questionnaires and open ended surveys about ideas, perceptions, and thoughts are other ways by which you can collect data for your qualitative research. For example, in your study of second career schoolteachers, perhaps you decide to do an anonymous survey of 100 teachers in the area because you're concerned that they may be less forthright in an interview situation than in a survey where their identity was anonymous.
- "Document analysis" – This involves examining written, visual, and audio documents that exist without any involvement of or instigation by the researcher. There are lots of different kinds of documents, including "official" documents produced by institutions and personal documents, like letters, memoirs, diaries and, in the 21st century, social media accounts and online blogs. For example, if studying education, institutions like public schools produce many different kinds of documents, including reports, flyers, handbooks, websites, curricula, etc. Maybe you can also see if any second career teachers have an online meet group or blog. Document analysis can often be useful to use in conjunction with another method, like interviewing.

- Coding – In coding, you assign a word, phrase, or number to each category. Start out with a pre-set list of codes that you derived from your prior knowledge of the subject. For example, "financial issues" or "community involvement" might be two codes you think of after having done your literature review of second career teachers. You then go through all of your data in a systematic way and "code" ideas, concepts and themes as they fit categories. You will also develop another set of codes that emerge from reading and analyzing the data. For example, you may see while coding your interviews, that "divorce" comes up frequently. You can add a code for this. Coding helps you organize your data and identify patterns and commonalities. [15] X Research source tobaccoeval.ucdavis.edu/analysis-reporting/.../CodingQualitativeData.pdf
- Descriptive Statistics – You can analyze your data using statistics. Descriptive statistics help describe, show or summarize the data to highlight patterns. For example, if you had 100 principal evaluations of teachers, you might be interested in the overall performance of those students. Descriptive statistics allow you to do that. Keep in mind, however, that descriptive statistics cannot be used to make conclusions and confirm/disprove hypotheses. [16] X Research source
- Narrative analysis – Narrative analysis focuses on speech and content, such as grammar, word usage, metaphors, story themes, meanings of situations, the social, cultural and political context of the narrative. [17] X Research source
- Hermeneutic Analysis – Hermeneutic analysis focuses on the meaning of a written or oral text. Essentially, you are trying to make sense of the object of study and bring to light some sort of underlying coherence. [18] X Research source
- Content analysis / Semiotic analysis – Content or semiotic analysis looks at texts or series of texts and looks for themes and meanings by looking at frequencies of words. Put differently, you try to identify structures and patterned regularities in the verbal or written text and then make inferences on the basis of these regularities. [19] X Research source For example, maybe you find the same words or phrases, like "second chance" or "make a difference," coming up in different interviews with second career teachers and decide to explore what this frequency might signify.

Community Q&A

- Qualitative research is often regarded as a precursor to quantitative research, which is a more logical and data-led approach which statistical, mathematical and/or computational techniques. Qualitative research is often used to generate possible leads and formulate a workable hypothesis that is then tested with quantitative methods. [20] X Research source Thanks Helpful 0 Not Helpful 0
- Try to remember the difference between qualitative and quantitative as each will give different data. Thanks Helpful 4 Not Helpful 0

You Might Also Like

- ↑ https://www.ncbi.nlm.nih.gov/books/NBK470395/
- ↑ https://owl.purdue.edu/owl/research_and_citation/conducting_research/writing_a_literature_review.html
- ↑ https://academic.oup.com/humrep/article/31/3/498/2384737?login=false
- ↑ https://www.ncbi.nlm.nih.gov/pmc/articles/PMC4275140/
- ↑ http://www.qual.auckland.ac.nz/
- ↑ http://www.socialresearchmethods.net/kb/qualapp.php
- ↑ http://www.socialresearchmethods.net/kb/qualdata.php
- ↑ tobaccoeval.ucdavis.edu/analysis-reporting/.../CodingQualitativeData.pdf
- ↑ https://statistics.laerd.com/statistical-guides/descriptive-inferential-statistics.php
- ↑ https://explorable.com/qualitative-research-design
About This Article

To do qualitative research, start by deciding on a clear, specific question that you want to answer. Then, do a literature review to see what other experts are saying about the topic, and evaluate how you will best be able to answer your question. Choose an appropriate qualitative research method, such as action research, ethnology, phenomenology, grounded theory, or case study research. Collect and analyze data according to your chosen method, determine the answer to your question. For tips on performing a literature review and picking a method for collecting data, read on! Did this summary help you? Yes No
- Send fan mail to authors
Reader Success Stories

Modeste Birindwa
Apr 14, 2020
Did this article help you?

Patricia Eruemu
Apr 13, 2016

Nagalaxmy Vinothe
Sep 21, 2019

Rakel Ngulube
Aug 23, 2017

Mhorshed Alam
Dec 23, 2018

Featured Articles

Trending Articles

Watch Articles

- Terms of Use
- Privacy Policy
- Do Not Sell or Share My Info
- Not Selling Info
wikiHow Tech Help Pro:
Develop the tech skills you need for work and life
- Policy and Social Care Move Fast: How…
Policy and Social Care Move Fast: How Rapid Qualitative Methods Can Help Researchers Match Their Pace
Date posted:.
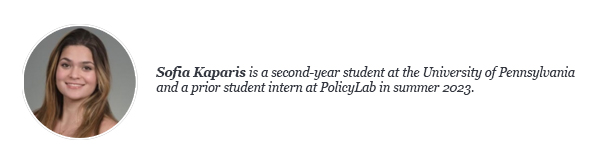
The field of social care integration, which refers to the study and implementation of clinically based programs to address the social needs of patients and families, is advancing at an increasingly rapid pace. This acceleration, driven by heightened need post-pandemic as well as mandates at the state and federal levels for health systems to implement screening and referral programs, has increased the urgency for high-quality evidence to support policy decisions about the delivery of social care—in other words, how health systems identify and address social needs, like access to healthy food and safe housing.
Qualitative research is particularly useful in guiding social care integration as it can shed light on the patient or caregiver experience of participating in social care interventions, barriers to getting help that should be addressed, and appropriate next steps from the perspective of those directly impacted.
However, traditional qualitative data analysis can be time consuming, and evidence-based solutions for addressing families’ social needs from the clinical setting are needed in the short term. In this post, I’ll share how we adapted and applied rapid qualitative methods to a social care-focused study as an example of how this approach can be used to inform social care integration in real time.
Integrating a Rapid Research Approach
The Socially Equitable Care by Understanding Resource Engagement ( SECURE ) study is a mixed method pragmatic trial aimed at understanding how best to increase family-level engagement with social resources from the pediatric health care setting. Caregivers in the study were randomized to complete one of three different social assessments (surveys asking about their social circumstances and/or desire for social resources) before receiving a resource map on their personal smartphone where, if interested, they could search for community resources in their neighborhood. Caregivers also had the option of talking to our study-specific resource navigator to receive additional support finding resources.
The overall goal of the qualitative component of the study is to capture caregivers’ preferences and experiences receiving social care through SECURE. Our traditional qualitative protocol involved transcribing caregiver interviews verbatim, coding transcripts and conducting thematic analysis. Recognizing the need for implementation-oriented results on a fast timeline, our team explored rapid qualitative methodologies to supplement the traditional approach. The rapid methods we chose were derived from existing literature on rapid qualitative approaches, which were then adapted to suit our study’s protocol and the social care field in general.
In our rapid approach, interviewers took notes using a structured template during or immediately after each caregiver interview. The template was designed to capture the data most salient to social care integration efforts such as caregiver’s likes, dislikes and preferences about receiving social care at their child’s doctor’s office. Then, content from the templates was transposed onto an analytic matrix, where we compared data across participants to identify themes. While we explored the full range of themes that emerged from our caregiver interviews in traditional qualitative analysis, we wanted to be sure that rapid analysis focused on findings that would be most applicable to social care integration efforts so the results could inform social care policy at Children’s Hospital of Philadelphia (CHOP) and elsewhere in real time. For example, what parts of participating in SECURE were helpful for caregivers? Did anything make them uncomfortable?
To ensure that our rapid approach produced results in line with those generated through traditional methods, we analyzed ten of our interviews using both traditional and rapid methods and compared the results. This analysis yielded a 92.8% theme match—meaning the two qualitative methods yielded largely the same themes. This builds upon previous literature, indicating that rapid analysis can be an effective tool in capturing implementation-oriented themes from qualitative data.
How the SECURE Study Can Inform Future Research Efforts
Our rapid qualitative methods allowed us to effectively adapt and respond to the quickly evolving landscape of social care integration, even before we had the full study results. I personally saw this first-hand while working with the SECURE team in 2023 conducting caregiver interviews. For example, we were able to inform hospital efforts in response to a recent insurance requirement of health systems to share caregivers' responses to social screening questions. We successfully gathered patients’ feedback on this new requirement and shared this information and suggestions for what CHOP could do to make caregivers feel more comfortable answering social assessment questions.
While not intended to replace traditional qualitative analysis, being able to produce actionable qualitative findings in a timely manner through rapid methods has allowed SECURE findings to help shape social care interventions at CHOP and beyond in real time.
Our hope is that other researchers in social care who face time pressures may find similar rapid qualitative methods as a useful and effective approach to adapt to the dynamic nature of the field and generate family-centered solutions faster than would otherwise be possible.
Latest Blog Posts
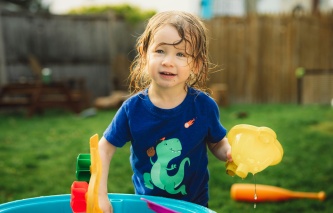
The Heat Is Turned Up: Focus on Climate Solutions for Kids
2023 was the hottest year on record and 2024 is predicted to rank among the top five warmest years. Extreme heat at this scale can be a danger to not…
A Sense of Purpose Can Support Teen Mental Health
Adolescence–the years between about 10 to about 25–is an essential time to help young people proactively build positive mental health and well-being…
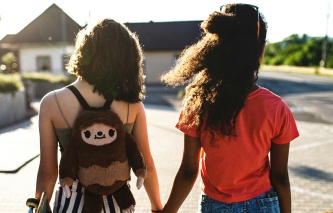
Q&A: Engaging Communities to Alleviate Period Poverty with Lynette Medley
There are 16.9 million women, girls and all other people who experience a menstrual cycle in the United States living in poverty, many of whom are…
Check out our new Publications View Publications
Adolescent Health & Well-Being
Behavioral health, population health sciences, health equity, family & community health.
What is a Thematic Analysis and How to Conduct One
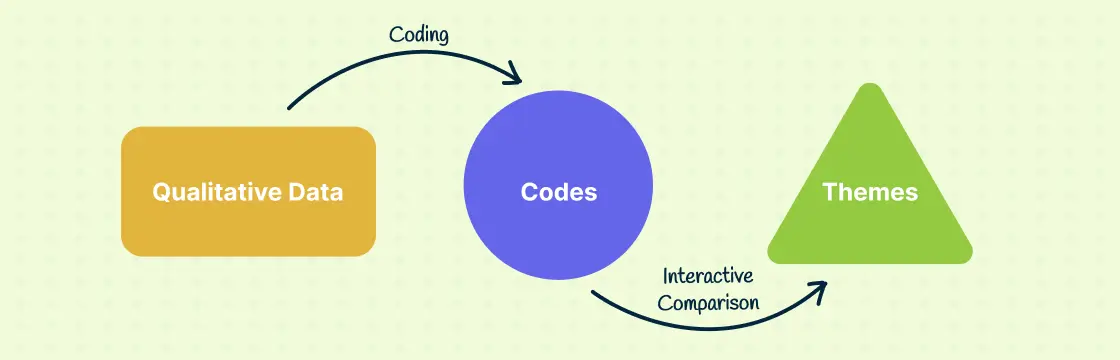
Have you ever wondered how researchers dive into the depths of words to extract common themes and ideas? Well, thematic analysis is their trusty tool for the job! In this guide, we’ll walk you through the basics of thematic analysis, breaking down its six-step process into simple, digestible chunks. By the end, you’ll not only understand how this method works but also gain insights into how it can illuminate the hidden gems within textual data.
What is a Thematic Analysis
Thematic analysis is a qualitative research method used to identify, analyze, and interpret patterns, themes, or meanings within a dataset. It involves examining patterns to reveal significance and scrutinizing the patterns and themes present in your dataset to unveil the implicit meaning. Importantly, this process is driven by your research aims and questions, so it’s not necessary to identify every possible theme in the data, but rather to focus on the key aspects that relate to your research questions
Unlike more prescriptive methods, thematic analysis does not subscribe to a rigid set of rules, making it particularly suitable for beginner researchers unfamiliar with more complicated qualitative research . It supports the inductive development of codes and themes, allowing for a rich interpretation of the data.
Thematic Analysis Versus Other Methods
When compared to other qualitative methods like grounded theory or discourse analysis, thematic analysis is less constrained by pre-existing theoretical frameworks, offering researchers the freedom to shape their analysis based on the data itself. This versatility enables a rich, intricate, and sophisticated description of data, which is invaluable in fields where understanding the nuances of participant experiences is key.
- Ready to use
- Fully customizable template
- Get Started in seconds

For those embarking on the journey of qualitative thematic analysis, tools like Creately’s visual collaboration platform can enhance the process by providing a visual canvas to organize and structure research information, fostering a collaborative workspace, and offering multiple visual frameworks to explore and uncover insights.
Thematic analysis stands out as a flexible and dynamic method within qualitative research, offering a range of advantages for researchers. However, like any method, it also presents certain challenges that must be navigated carefully.
The Advantages of Thematic Analysis
- Flexibility in Data Analysis: Thematic analysis is not tied to a specific theory or structure, allowing researchers to adapt the method to suit their unique data sets and research questions.
- Inductive Approach: This method supports the inductive development of codes and themes, meaning that patterns and meanings can emerge directly from the data without being constrained by preconceived categories.
- Potential for Rich Descriptions: Thematic analysis enables a detailed exploration of data, which can result in a rich and nuanced understanding of the research topic.
Challenges in Thematic Analysis
- Maintaining Consistency and Rigor: The flexibility of thematic analysis can make it challenging to apply a consistent and rigorous approach across different data sets or studies.
- Validity and Reliability: Researchers must be vigilant in their methods to ensure the validity and reliability of their findings, which can be more subjective in thematic analysis compared to quantitative methods.
Despite these challenges, thematic analysis remains a valuable tool for qualitative research, particularly for those new to the field. It’s a method that allows for a deep dive into complex data sets, providing a comprehensive understanding that is both flexible and insightful.
Exploring Different Approaches to Thematic Analysis
- Inductive vs. Deductive Approaches: An inductive approach to thematic analysis allows themes to emerge organically from the data, without preconceived categories influencing the analysis. This bottom-up method is particularly useful when the researcher is exploring new areas where little is known. Conversely, a deductive approach starts with predefined codes or themes, often based on existing theory, and involves assessing the data against these standards. This top-down method can provide a more structured analysis but may limit the discovery of unexpected themes.
- Choosing a Theoretical Framework: The choice of theoretical framework in thematic analysis is crucial as it guides the entire process, from coding to theme development. Whether you lean towards a constructivist paradigm, which acknowledges the subjective interplay between researcher and research subject, or a more positivist approach, the framework shapes the interpretation of data.
Additionally, thematic analysis can be conducted at different levels. A semantic level analysis stays close to the explicit content of the data, while a latent level analysis delves deeper into the underlying ideas and assumptions. The researcher’s subjectivity plays a significant role in both approaches, influencing the analysis through their insights and biases. Ultimately, the choice of approach should align with the research objectives and the nature of the data, ensuring a rich and meaningful interpretation.
Step-by-Step Guide to Conducting a Thematic Analysis
Here’s a step-by-step guide to conducting a thematic analysis, ensuring you capture the richness of your data and present it effectively.
Phase 1: Familiarization with Data
Begin by immersing yourself in the data. Read through your data set multiple times to gain a deep understanding of the content. Take notes of initial ideas, patterns, and elements that stand out. This phase is crucial as it lays the groundwork for the entire analysis.
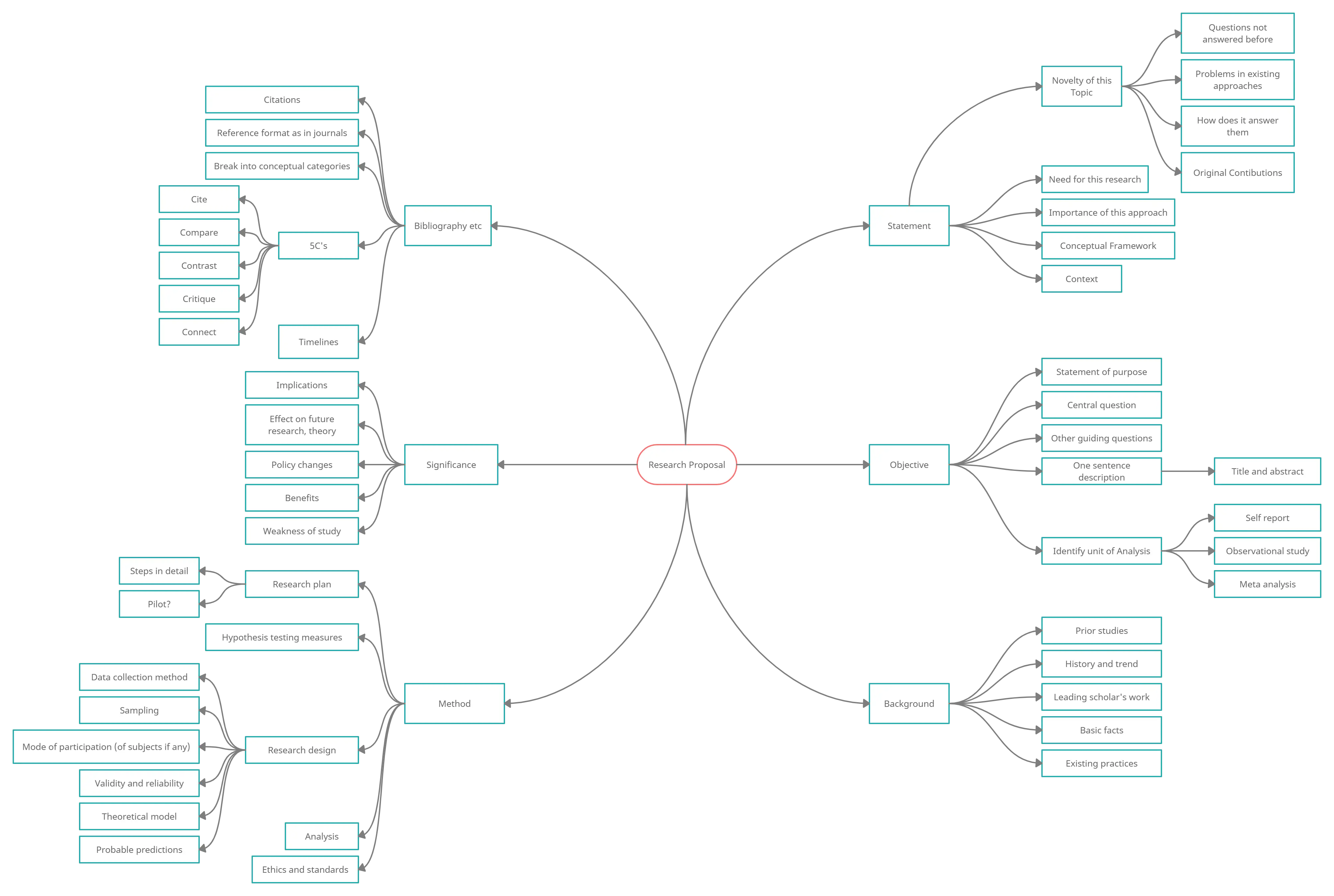
Phase 2: Coding
Next, systematically code your data. Coding involves highlighting segments of the data that are of interest and assigning a label to them. These codes can be words or short phrases that capture the essence of a piece of data. Creately’s visual tools can be particularly useful here, allowing you to organize codes and identify patterns across your data set.
Phase 3: Generating Themes
From the codes, you’ll start to identify broader patterns that will form your themes. Themes are the central concepts you believe are important in the data. They should be linked to your research question and tell a story about your data. At this stage, Creately’s visual canvases can help you to cluster similar codes and visualize the emerging themes.
Phase 4: Reviewing Themes
Once you have a set of candidate themes, review them. Check if they work in relation to the coded extracts and the entire data set. This may involve merging, splitting, or discarding themes. The goal is to ensure that your themes accurately reflect the data and form a coherent pattern.
Phase 5: Defining and Naming Themes
Now, define what each theme is about and what aspect of the data each theme captures. This involves a detailed analysis of each theme and determining the ‘story’ that each one tells. Naming your themes is also crucial; the name should be concise and immediately give an idea of what the theme is about.
Phase 6: Writing Up
The final phase is writing up your findings. This involves weaving together the analytic narrative and data extracts, and contextualizing the analysis in relation to existing literature.
Throughout these phases, remember that thematic analysis is a flexible method that can be tailored to your specific research needs. It allows for an inductive approach, where themes emerge from the data without preconceived notions. Moreover, it supports a rich and detailed description of the data, which can be particularly beneficial for beginner researchers. However, maintaining consistency and rigor is essential to ensure the validity and reliability of your analysis.
By following these steps and utilizing tools like Creately, you can master the art of thematic analysis, turning complex data sets into meaningful insights.
Using Creately’s Visual Canvas to Conduct a Thematic Analysis
Conducting a thorough thematic analysis in qualitative research can be a complex task, but with the right tools, it becomes significantly more manageable. Creately’s visual collaboration platform is designed to enhance the efficiency of this process, offering a suite of features that align perfectly with the needs of researchers conducting thematic analysis.
Organizing Data with Visual Canvases
At the heart of thematic analysis is the organization of data. Creately’s visual canvas provides an expansive workspace where researchers can lay out their data visually. This can be particularly useful when dealing with large and complex data sets, as it allows for the creation of a structured overview. Researchers can use tools like the Mind Map for Research Proposal to brainstorm and connect ideas, ensuring that no data point is overlooked.
Collaborative Research with Creately
Thematic analysis often involves multiple stakeholders, and Creately’s collaborative workspace is ideal for engaging everyone involved. Team members can contribute simultaneously, offering their insights and annotations in real time. This collaborative environment ensures that diverse perspectives are considered, enriching the analysis and leading to more nuanced themes.
Visual Frameworks for Deeper Insights
Uncovering deeper insights is crucial in thematic analysis, and Creately’s multiple visual frameworks support this exploration. Researchers can utilize frameworks such as the Affinity Diagram Tool to sort and prioritize data, which can reveal patterns and connections that might otherwise remain hidden. These visual tools are not only practical but also encourage a more dynamic and engaging research process.
Centralizing Research Information
Having a centralized repository for all research information is invaluable, and Creately excels in this area. By centralizing all research data on a single canvas, researchers have an easy reference point that streamlines the analysis process. This centralization is particularly beneficial during the later stages of thematic analysis, such as reviewing themes and writing up the findings, as it provides a clear and comprehensive view of all the work done.
In conclusion, Creately’s features are not just about visual aesthetics; they are powerful tools that can significantly enhance the thematic analysis process. From organizing complex data sets to fostering collaboration and providing a central point of reference, Creately is an invaluable asset for researchers aiming to conduct a rigorous and insightful thematic analysis.
Join over thousands of organizations that use Creately to brainstorm, plan, analyze, and execute their projects successfully.
More Related Articles
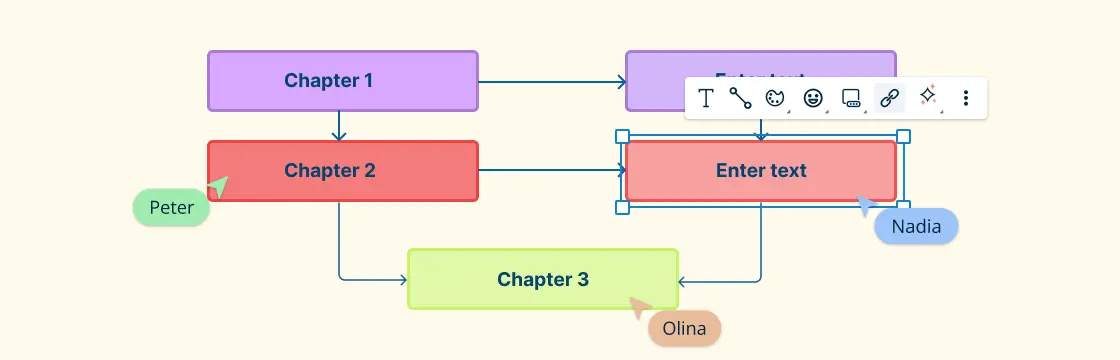
Chiraag George is a communication specialist here at Creately. He is a marketing junkie that is fascinated by how brands occupy consumer mind space. A lover of all things tech, he writes a lot about the intersection of technology, branding and culture at large.
Numbers, Facts and Trends Shaping Your World
Read our research on:
Full Topic List
Regions & Countries
- Publications
- Our Methods
- Short Reads
- Tools & Resources
Read Our Research On:
Writing Survey Questions
Perhaps the most important part of the survey process is the creation of questions that accurately measure the opinions, experiences and behaviors of the public. Accurate random sampling will be wasted if the information gathered is built on a shaky foundation of ambiguous or biased questions. Creating good measures involves both writing good questions and organizing them to form the questionnaire.
Questionnaire design is a multistage process that requires attention to many details at once. Designing the questionnaire is complicated because surveys can ask about topics in varying degrees of detail, questions can be asked in different ways, and questions asked earlier in a survey may influence how people respond to later questions. Researchers are also often interested in measuring change over time and therefore must be attentive to how opinions or behaviors have been measured in prior surveys.
Surveyors may conduct pilot tests or focus groups in the early stages of questionnaire development in order to better understand how people think about an issue or comprehend a question. Pretesting a survey is an essential step in the questionnaire design process to evaluate how people respond to the overall questionnaire and specific questions, especially when questions are being introduced for the first time.
For many years, surveyors approached questionnaire design as an art, but substantial research over the past forty years has demonstrated that there is a lot of science involved in crafting a good survey questionnaire. Here, we discuss the pitfalls and best practices of designing questionnaires.
Question development
There are several steps involved in developing a survey questionnaire. The first is identifying what topics will be covered in the survey. For Pew Research Center surveys, this involves thinking about what is happening in our nation and the world and what will be relevant to the public, policymakers and the media. We also track opinion on a variety of issues over time so we often ensure that we update these trends on a regular basis to better understand whether people’s opinions are changing.
At Pew Research Center, questionnaire development is a collaborative and iterative process where staff meet to discuss drafts of the questionnaire several times over the course of its development. We frequently test new survey questions ahead of time through qualitative research methods such as focus groups , cognitive interviews, pretesting (often using an online, opt-in sample ), or a combination of these approaches. Researchers use insights from this testing to refine questions before they are asked in a production survey, such as on the ATP.
Measuring change over time
Many surveyors want to track changes over time in people’s attitudes, opinions and behaviors. To measure change, questions are asked at two or more points in time. A cross-sectional design surveys different people in the same population at multiple points in time. A panel, such as the ATP, surveys the same people over time. However, it is common for the set of people in survey panels to change over time as new panelists are added and some prior panelists drop out. Many of the questions in Pew Research Center surveys have been asked in prior polls. Asking the same questions at different points in time allows us to report on changes in the overall views of the general public (or a subset of the public, such as registered voters, men or Black Americans), or what we call “trending the data”.
When measuring change over time, it is important to use the same question wording and to be sensitive to where the question is asked in the questionnaire to maintain a similar context as when the question was asked previously (see question wording and question order for further information). All of our survey reports include a topline questionnaire that provides the exact question wording and sequencing, along with results from the current survey and previous surveys in which we asked the question.
The Center’s transition from conducting U.S. surveys by live telephone interviewing to an online panel (around 2014 to 2020) complicated some opinion trends, but not others. Opinion trends that ask about sensitive topics (e.g., personal finances or attending religious services ) or that elicited volunteered answers (e.g., “neither” or “don’t know”) over the phone tended to show larger differences than other trends when shifting from phone polls to the online ATP. The Center adopted several strategies for coping with changes to data trends that may be related to this change in methodology. If there is evidence suggesting that a change in a trend stems from switching from phone to online measurement, Center reports flag that possibility for readers to try to head off confusion or erroneous conclusions.
Open- and closed-ended questions
One of the most significant decisions that can affect how people answer questions is whether the question is posed as an open-ended question, where respondents provide a response in their own words, or a closed-ended question, where they are asked to choose from a list of answer choices.
For example, in a poll conducted after the 2008 presidential election, people responded very differently to two versions of the question: “What one issue mattered most to you in deciding how you voted for president?” One was closed-ended and the other open-ended. In the closed-ended version, respondents were provided five options and could volunteer an option not on the list.
When explicitly offered the economy as a response, more than half of respondents (58%) chose this answer; only 35% of those who responded to the open-ended version volunteered the economy. Moreover, among those asked the closed-ended version, fewer than one-in-ten (8%) provided a response other than the five they were read. By contrast, fully 43% of those asked the open-ended version provided a response not listed in the closed-ended version of the question. All of the other issues were chosen at least slightly more often when explicitly offered in the closed-ended version than in the open-ended version. (Also see “High Marks for the Campaign, a High Bar for Obama” for more information.)

Researchers will sometimes conduct a pilot study using open-ended questions to discover which answers are most common. They will then develop closed-ended questions based off that pilot study that include the most common responses as answer choices. In this way, the questions may better reflect what the public is thinking, how they view a particular issue, or bring certain issues to light that the researchers may not have been aware of.
When asking closed-ended questions, the choice of options provided, how each option is described, the number of response options offered, and the order in which options are read can all influence how people respond. One example of the impact of how categories are defined can be found in a Pew Research Center poll conducted in January 2002. When half of the sample was asked whether it was “more important for President Bush to focus on domestic policy or foreign policy,” 52% chose domestic policy while only 34% said foreign policy. When the category “foreign policy” was narrowed to a specific aspect – “the war on terrorism” – far more people chose it; only 33% chose domestic policy while 52% chose the war on terrorism.
In most circumstances, the number of answer choices should be kept to a relatively small number – just four or perhaps five at most – especially in telephone surveys. Psychological research indicates that people have a hard time keeping more than this number of choices in mind at one time. When the question is asking about an objective fact and/or demographics, such as the religious affiliation of the respondent, more categories can be used. In fact, they are encouraged to ensure inclusivity. For example, Pew Research Center’s standard religion questions include more than 12 different categories, beginning with the most common affiliations (Protestant and Catholic). Most respondents have no trouble with this question because they can expect to see their religious group within that list in a self-administered survey.
In addition to the number and choice of response options offered, the order of answer categories can influence how people respond to closed-ended questions. Research suggests that in telephone surveys respondents more frequently choose items heard later in a list (a “recency effect”), and in self-administered surveys, they tend to choose items at the top of the list (a “primacy” effect).
Because of concerns about the effects of category order on responses to closed-ended questions, many sets of response options in Pew Research Center’s surveys are programmed to be randomized to ensure that the options are not asked in the same order for each respondent. Rotating or randomizing means that questions or items in a list are not asked in the same order to each respondent. Answers to questions are sometimes affected by questions that precede them. By presenting questions in a different order to each respondent, we ensure that each question gets asked in the same context as every other question the same number of times (e.g., first, last or any position in between). This does not eliminate the potential impact of previous questions on the current question, but it does ensure that this bias is spread randomly across all of the questions or items in the list. For instance, in the example discussed above about what issue mattered most in people’s vote, the order of the five issues in the closed-ended version of the question was randomized so that no one issue appeared early or late in the list for all respondents. Randomization of response items does not eliminate order effects, but it does ensure that this type of bias is spread randomly.
Questions with ordinal response categories – those with an underlying order (e.g., excellent, good, only fair, poor OR very favorable, mostly favorable, mostly unfavorable, very unfavorable) – are generally not randomized because the order of the categories conveys important information to help respondents answer the question. Generally, these types of scales should be presented in order so respondents can easily place their responses along the continuum, but the order can be reversed for some respondents. For example, in one of Pew Research Center’s questions about abortion, half of the sample is asked whether abortion should be “legal in all cases, legal in most cases, illegal in most cases, illegal in all cases,” while the other half of the sample is asked the same question with the response categories read in reverse order, starting with “illegal in all cases.” Again, reversing the order does not eliminate the recency effect but distributes it randomly across the population.
Question wording
The choice of words and phrases in a question is critical in expressing the meaning and intent of the question to the respondent and ensuring that all respondents interpret the question the same way. Even small wording differences can substantially affect the answers people provide.
[View more Methods 101 Videos ]
An example of a wording difference that had a significant impact on responses comes from a January 2003 Pew Research Center survey. When people were asked whether they would “favor or oppose taking military action in Iraq to end Saddam Hussein’s rule,” 68% said they favored military action while 25% said they opposed military action. However, when asked whether they would “favor or oppose taking military action in Iraq to end Saddam Hussein’s rule even if it meant that U.S. forces might suffer thousands of casualties, ” responses were dramatically different; only 43% said they favored military action, while 48% said they opposed it. The introduction of U.S. casualties altered the context of the question and influenced whether people favored or opposed military action in Iraq.
There has been a substantial amount of research to gauge the impact of different ways of asking questions and how to minimize differences in the way respondents interpret what is being asked. The issues related to question wording are more numerous than can be treated adequately in this short space, but below are a few of the important things to consider:
First, it is important to ask questions that are clear and specific and that each respondent will be able to answer. If a question is open-ended, it should be evident to respondents that they can answer in their own words and what type of response they should provide (an issue or problem, a month, number of days, etc.). Closed-ended questions should include all reasonable responses (i.e., the list of options is exhaustive) and the response categories should not overlap (i.e., response options should be mutually exclusive). Further, it is important to discern when it is best to use forced-choice close-ended questions (often denoted with a radio button in online surveys) versus “select-all-that-apply” lists (or check-all boxes). A 2019 Center study found that forced-choice questions tend to yield more accurate responses, especially for sensitive questions. Based on that research, the Center generally avoids using select-all-that-apply questions.
It is also important to ask only one question at a time. Questions that ask respondents to evaluate more than one concept (known as double-barreled questions) – such as “How much confidence do you have in President Obama to handle domestic and foreign policy?” – are difficult for respondents to answer and often lead to responses that are difficult to interpret. In this example, it would be more effective to ask two separate questions, one about domestic policy and another about foreign policy.
In general, questions that use simple and concrete language are more easily understood by respondents. It is especially important to consider the education level of the survey population when thinking about how easy it will be for respondents to interpret and answer a question. Double negatives (e.g., do you favor or oppose not allowing gays and lesbians to legally marry) or unfamiliar abbreviations or jargon (e.g., ANWR instead of Arctic National Wildlife Refuge) can result in respondent confusion and should be avoided.
Similarly, it is important to consider whether certain words may be viewed as biased or potentially offensive to some respondents, as well as the emotional reaction that some words may provoke. For example, in a 2005 Pew Research Center survey, 51% of respondents said they favored “making it legal for doctors to give terminally ill patients the means to end their lives,” but only 44% said they favored “making it legal for doctors to assist terminally ill patients in committing suicide.” Although both versions of the question are asking about the same thing, the reaction of respondents was different. In another example, respondents have reacted differently to questions using the word “welfare” as opposed to the more generic “assistance to the poor.” Several experiments have shown that there is much greater public support for expanding “assistance to the poor” than for expanding “welfare.”
We often write two versions of a question and ask half of the survey sample one version of the question and the other half the second version. Thus, we say we have two forms of the questionnaire. Respondents are assigned randomly to receive either form, so we can assume that the two groups of respondents are essentially identical. On questions where two versions are used, significant differences in the answers between the two forms tell us that the difference is a result of the way we worded the two versions.
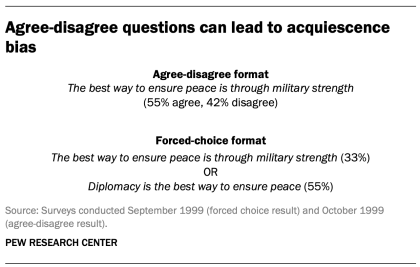
One of the most common formats used in survey questions is the “agree-disagree” format. In this type of question, respondents are asked whether they agree or disagree with a particular statement. Research has shown that, compared with the better educated and better informed, less educated and less informed respondents have a greater tendency to agree with such statements. This is sometimes called an “acquiescence bias” (since some kinds of respondents are more likely to acquiesce to the assertion than are others). This behavior is even more pronounced when there’s an interviewer present, rather than when the survey is self-administered. A better practice is to offer respondents a choice between alternative statements. A Pew Research Center experiment with one of its routinely asked values questions illustrates the difference that question format can make. Not only does the forced choice format yield a very different result overall from the agree-disagree format, but the pattern of answers between respondents with more or less formal education also tends to be very different.
One other challenge in developing questionnaires is what is called “social desirability bias.” People have a natural tendency to want to be accepted and liked, and this may lead people to provide inaccurate answers to questions that deal with sensitive subjects. Research has shown that respondents understate alcohol and drug use, tax evasion and racial bias. They also may overstate church attendance, charitable contributions and the likelihood that they will vote in an election. Researchers attempt to account for this potential bias in crafting questions about these topics. For instance, when Pew Research Center surveys ask about past voting behavior, it is important to note that circumstances may have prevented the respondent from voting: “In the 2012 presidential election between Barack Obama and Mitt Romney, did things come up that kept you from voting, or did you happen to vote?” The choice of response options can also make it easier for people to be honest. For example, a question about church attendance might include three of six response options that indicate infrequent attendance. Research has also shown that social desirability bias can be greater when an interviewer is present (e.g., telephone and face-to-face surveys) than when respondents complete the survey themselves (e.g., paper and web surveys).
Lastly, because slight modifications in question wording can affect responses, identical question wording should be used when the intention is to compare results to those from earlier surveys. Similarly, because question wording and responses can vary based on the mode used to survey respondents, researchers should carefully evaluate the likely effects on trend measurements if a different survey mode will be used to assess change in opinion over time.
Question order
Once the survey questions are developed, particular attention should be paid to how they are ordered in the questionnaire. Surveyors must be attentive to how questions early in a questionnaire may have unintended effects on how respondents answer subsequent questions. Researchers have demonstrated that the order in which questions are asked can influence how people respond; earlier questions can unintentionally provide context for the questions that follow (these effects are called “order effects”).
One kind of order effect can be seen in responses to open-ended questions. Pew Research Center surveys generally ask open-ended questions about national problems, opinions about leaders and similar topics near the beginning of the questionnaire. If closed-ended questions that relate to the topic are placed before the open-ended question, respondents are much more likely to mention concepts or considerations raised in those earlier questions when responding to the open-ended question.
For closed-ended opinion questions, there are two main types of order effects: contrast effects ( where the order results in greater differences in responses), and assimilation effects (where responses are more similar as a result of their order).
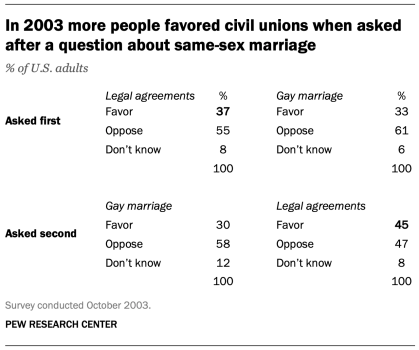
An example of a contrast effect can be seen in a Pew Research Center poll conducted in October 2003, a dozen years before same-sex marriage was legalized in the U.S. That poll found that people were more likely to favor allowing gays and lesbians to enter into legal agreements that give them the same rights as married couples when this question was asked after one about whether they favored or opposed allowing gays and lesbians to marry (45% favored legal agreements when asked after the marriage question, but 37% favored legal agreements without the immediate preceding context of a question about same-sex marriage). Responses to the question about same-sex marriage, meanwhile, were not significantly affected by its placement before or after the legal agreements question.
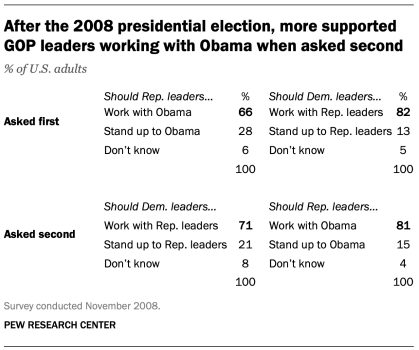
Another experiment embedded in a December 2008 Pew Research Center poll also resulted in a contrast effect. When people were asked “All in all, are you satisfied or dissatisfied with the way things are going in this country today?” immediately after having been asked “Do you approve or disapprove of the way George W. Bush is handling his job as president?”; 88% said they were dissatisfied, compared with only 78% without the context of the prior question.
Responses to presidential approval remained relatively unchanged whether national satisfaction was asked before or after it. A similar finding occurred in December 2004 when both satisfaction and presidential approval were much higher (57% were dissatisfied when Bush approval was asked first vs. 51% when general satisfaction was asked first).
Several studies also have shown that asking a more specific question before a more general question (e.g., asking about happiness with one’s marriage before asking about one’s overall happiness) can result in a contrast effect. Although some exceptions have been found, people tend to avoid redundancy by excluding the more specific question from the general rating.
Assimilation effects occur when responses to two questions are more consistent or closer together because of their placement in the questionnaire. We found an example of an assimilation effect in a Pew Research Center poll conducted in November 2008 when we asked whether Republican leaders should work with Obama or stand up to him on important issues and whether Democratic leaders should work with Republican leaders or stand up to them on important issues. People were more likely to say that Republican leaders should work with Obama when the question was preceded by the one asking what Democratic leaders should do in working with Republican leaders (81% vs. 66%). However, when people were first asked about Republican leaders working with Obama, fewer said that Democratic leaders should work with Republican leaders (71% vs. 82%).
The order questions are asked is of particular importance when tracking trends over time. As a result, care should be taken to ensure that the context is similar each time a question is asked. Modifying the context of the question could call into question any observed changes over time (see measuring change over time for more information).
A questionnaire, like a conversation, should be grouped by topic and unfold in a logical order. It is often helpful to begin the survey with simple questions that respondents will find interesting and engaging. Throughout the survey, an effort should be made to keep the survey interesting and not overburden respondents with several difficult questions right after one another. Demographic questions such as income, education or age should not be asked near the beginning of a survey unless they are needed to determine eligibility for the survey or for routing respondents through particular sections of the questionnaire. Even then, it is best to precede such items with more interesting and engaging questions. One virtue of survey panels like the ATP is that demographic questions usually only need to be asked once a year, not in each survey.
U.S. Surveys
Other research methods, sign up for our weekly newsletter.
Fresh data delivered Saturday mornings
1615 L St. NW, Suite 800 Washington, DC 20036 USA (+1) 202-419-4300 | Main (+1) 202-857-8562 | Fax (+1) 202-419-4372 | Media Inquiries
Research Topics
- Age & Generations
- Coronavirus (COVID-19)
- Economy & Work
- Family & Relationships
- Gender & LGBTQ
- Immigration & Migration
- International Affairs
- Internet & Technology
- Methodological Research
- News Habits & Media
- Non-U.S. Governments
- Other Topics
- Politics & Policy
- Race & Ethnicity
- Email Newsletters
ABOUT PEW RESEARCH CENTER Pew Research Center is a nonpartisan fact tank that informs the public about the issues, attitudes and trends shaping the world. It conducts public opinion polling, demographic research, media content analysis and other empirical social science research. Pew Research Center does not take policy positions. It is a subsidiary of The Pew Charitable Trusts .
Copyright 2024 Pew Research Center
Terms & Conditions
Privacy Policy
Cookie Settings
Reprints, Permissions & Use Policy

The experience of citizenship in a segregated school system: Curricular experiences in citizenship of young people who studied in Chilean schools of different dependencies and modalities
This paper presents the results of a qualitative research with a biographical approach that delved into the curricular experiences in citizenship of young people who completed their secondary education in Chile, within schools of different dependencies and modalities, in different socioeconomic contexts, and during a period of important student mobilizations (2015-2020). To document these experiences, five workshops were held to exchange curricular experiences in citizenship, one for each dependency (public, private-subsidized, and private schools) and modality (oriented to work and higher education). A total of 56 young people between the ages of 20 and 25 participated in these workshops. The information produced was analyzed through a collaborative analysis of qualitative data to describe and compare the curricular experiences in citizenship of those who graduated from these different types of schools. The results show that different types of establishments promote different types of citizenship with different conceptions of citizenship and its exercise. These differences are related to socioeconomic and ideological elements.
Author Biographies
Renato gazmuri-stein, universidad diego portales.
Licenciado en Historia, Universidad Católica de Chile. Máster y Doctor en Didáctica de la Historia y las Ciencias Sociales por la Universidad Autónoma de Barcelona. Profesor asociado Facultad de Educación, Universidad Diego Portales.
María Toledo-Jofré, Universidad Diego Portales
Antropóloga y Licenciada en Antropología, Universidad de Chile, Chile; Doctora en Ciencias de la Educación por la Universidad Católica de Lovaina, Bélgica. Profesora titular de la Escuela de Psicología, Coordinadora Programa Estudios Psicosociales en Contextos Educativos de la Facultad de Psicología, Universidad Diego Portales.
Gabriel Villalón-Gálvez, Universidad de Chile
Profesor de Historia, Geografía y Educación Cívica, Universidad Metropolitana de Ciencias de la Educación y Doctor en Didáctica de la Historia y las Ciencias Sociales por la Universidad Autónoma de Barcelona. Profesor Asistente Departamento de Estudios Pedagógicos de la Facultad de Filosofía y Humanidades, Universidad de Chile.
Eliana Moraleda-Albornoz, Universidad Alberto Hurtado
Profesor de Historia y Geografía, Universidad de Tarapacá. Magister en Currículum, Pontificia Universidad Católica de Chile. Profesional, Universidad Alberto Hurtado.
- PDF (Español)
How to Cite
- Endnote/Zotero/Mendeley (RIS)
Copyright (c) 2024 Renato Gazmuri-Stein, María Toledo-Jofré, Gabriel Villalón-Gálvez, Eliana Moraleda-Albornoz

This work is licensed under a Creative Commons Attribution-ShareAlike 4.0 International License .
Education Policy Analysis Archives/Archivos Analíticos de Políticas Educativas/Arquivos Analíticos de Políticas Educativas (EPAA/AAPE; ISSN 1068-2341 ) is a peer-reviewed, open-access, international, multilingual, and multidisciplinary journal designed for researchers, practitioners, policy makers, and development analysts concerned with education policies.
- Português (Brasil)
- Español (España)
Make a Gift
Submit a manuscript or revision, recent special issues.
Youth and Adult Education, Literacies and Decoloniality
Global Policy Mobilities in Federal Education Systems
Teacher Subjectivities in Latin America
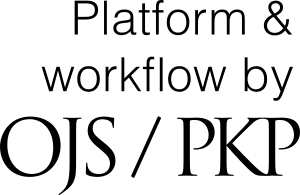
This paper is in the following e-collection/theme issue:
Published on 26.4.2024 in Vol 26 (2024)
Patients’ Representations of Perceived Distance and Proximity to Telehealth in France: Qualitative Study
Authors of this article:

Original Paper
- Amélie Loriot 1 * , MSc ;
- Fabrice Larceneux 1 * , PhD ;
- Valérie Guillard 1 * , PhD ;
- Jean-Philippe Bertocchio 2, 3 * , MD, PhD
1 Paris Dauphine–PSL (Paris Sciences & Lettres) University, Paris, France
2 Service Thyroïde – Tumeurs Endocrines, Hôpital de la Pitié-Salpêtrière, Assistance Publique – Hôpitaux de Paris, Paris, France
3 SKEZI, Annecy, France
*all authors contributed equally
Corresponding Author:
Amélie Loriot, MSc
Paris Dauphine–PSL (Paris Sciences & Lettres) University
Place du Maréchal de Lattre de Tassigny
Paris, 75116
Phone: 33 144054405
Email: [email protected]
Background: In the last 2 decades, new technologies have emerged in health care. The COVID-19 pandemic further accelerated the adoption of technology by both health care professionals and patients. These technologies create remote care practices that bring several benefits to the health care system: easier access to care, improved communication with physicians, and greater continuity of care. However, disparities in the acceptance and use of telehealth tools still exist among patients. These tools also disrupt conventional medical practices and prompt a new reassessment of the perceptions of distance and proximity as physical (ie, time and space dimensions) and nonphysical (ie, behavioral dimensions) concepts. The reasons why patients do or do not adopt telehealth tools for their care and therefore their perspectives on telehealth remain unanswered questions.
Objective: We explored the barriers as well as the motivations for patients to adopt telehealth tools. We specifically focused on the social representations of telehealth to establish a comprehensive conceptual framework to get a better understanding of how telehealth is perceived by patients.
Methods: This study uses a qualitative design through in-depth individual interviews. Participants were recruited using a convenience sampling method with balanced consideration of gender, age, location (urban/rural), and socioeconomic background. After collecting informed consent, interviews were transcribed and analyzed using the thematic analysis methodology.
Results: We conducted 14 interviews, with which data saturation was reached. The 2 main opposed dimensions, perceived proximity and distance, emerged as an essential structure for understanding the social representations of telehealth. A logic of engagement versus hostility emerged as the main tension in adopting telehealth, almost ideological. Interestingly, practical issues emerged regarding the adoption of telehealth: A logic of integration was opposed to a logic of constraints. Altogether, those dimensions enabled us to conceptualize a semiotic square, providing 4 categories with a coherent body of social representations. Due to the dynamic nature of these representations, we proposed 2 “paths” through which adherence to telehealth may improve.
Conclusions: Our semiotic square illustrating patients’ adherence to telehealth differentiates socially beneficial versus socially dangerous considerations and pragmatic from ideological postures. It shows how crucial it is to consider perceived distance and proximity to better understand barriers and motivations to adopting telehealth. These representations can also be considered as leverage that could be modified to encourage the step-by-step adhesion process. Even if reducing the perceived temporal distance to in-person meeting and enhancing the perceived proximity of access to care may be seen as efficient ways to adopt telehealth tools, telehealth can also be perceived as a care practice that threatens the patient-physician relationship. The patient-oriented perceived value turns out to be critical in the future development of and adherence to telehealth tools.
Introduction
Telehealth, a subset of ehealth still ongoing.
Many technologies have been developed in eHealth in recent years. Defined as the “use of information and communication technologies in support of health and health-related fields, including health care services, health monitoring, health literature, and health education, knowledge and research” [ 1 ], eHealth covers a wide range of practices. First, mobile apps and connected devices are referred to as mobile health (mHealth). Second, telehealth (ie, the practice of medicine using information and communication technologies) covers 5 practices: teleconsultation, teleexpertise, medical regulation, remote medical monitoring, and remote medical assistance.
Recent research focusing on remote care has indicated some confusion regarding the wording used to refer to health-related technologies [ 2 ]. For instance, the terms “telemedicine” and “telehealth” are often used interchangeably [ 3 ]. However, some researchers highlight a difference between these 2 concepts. Whereas telemedicine is limited to remote clinical services, telehealth is broader and refers to remote clinical services as well as remote nonclinical services, like administrative meetings [ 4 ]. Thus, telehealth has been defined as “the use of electronic information and telecommunication technologies to support and promote long-distance clinical health care, patient and professional health-related education, public health and health administration” [ 5 ].
eHealth is expected to lead to significant changes in the delivery of care and medical practices [ 6 ]. Because of (1) disparities in access to health care, (2) the aging population, and (3) budget constraints limiting public policies, the development of eHealth devices can be seen as a solution to the future challenges faced by the health system in many high-income countries [ 7 ]. Before the COVID-19 crisis, there were significant disparities in the use of eHealth tools between European countries [ 8 ]. A global shift occurred during the pandemic: The use of many eHealth tools became necessary, democratizing their use in terms of communication, monitoring, or care delivery, and the use of technology to provide health services has accelerated [ 9 , 10 ]. Telehealth may now concern everyone.
Benefits and Barriers of Telehealth
The practice of telehealth presents many benefits for patients, including (1) better access to health care services, in particular in isolated regions like rural areas; (2) improved continuity of care; (3) increased availability of health information [ 11 ]; and (4) empowerment of patients [ 12 ]. As such, telehealth is supposed to increase efficiency and quality of care [ 10 ] and favors patient-centered care by enabling better communication between patients and health care professionals [ 13 ].
However, researchers have pointed out that many barriers exist that limit health equity for all patients. Significant disparities remain regarding the access to, adherence to, and use of telehealth tools [ 14 , 15 ]. In particular, little is known about the role of digital health literacy [ 13 , 15 , 16 ] (ie, “the ability to search for, find, understand and evaluate health information from electronic sources and to apply knowledge acquired to solve a health problem” [ 17 ]). Among individuals in rural areas, low levels of education are associated with lower use of digital health tools [ 18 ]. Some scholars argue that online interactions are impersonal and dangerous because of the lack of a physical examination [ 10 ] and that telehealth may threaten the quality of the relationship between physicians and patients [ 19 ].
Studies among health care professionals have also shown a reluctance to adopt these technologies because of a fear of “dehumanization” by virtualizing patients and care [ 20 ]. This feeling of dehumanization of care could explain negative attitudes toward telehealth [ 21 ].
Patients tend to attribute significant importance to health care professionals’ physical and emotional presence [ 22 ] and direct interactions with them [ 7 ]. However, the digitalization of health is transforming these relationships [ 23 ]: Telehealth disrupts medical practices and reduces physical interactions between patients and physicians. However, it leads to reconsidering notions of distance and proximity [ 23 ], including physical and nonphysical dimensions (ie, cognitive or relational aspects that are perceived by individuals) [ 24 - 26 ]. Physical proximity and perceived proximity are not necessarily aligned. Indeed, individuals can feel themselves close to an element that is physically far but also to perceive it far when it is physically close [ 26 ]. Perceived proximity has a cognitive dimension that refers to “a mental assessment of how distant someone else seems” and an affective component, since these representations are subject to emotions rather than rational thought [ 26 , 27 ]. In health care, Talbot et al [ 28 ] investigated the perceptions French physicians may have about telehealth using the conceptual framework of proximity of Boschma [ 25 ] that includes the following 5 dimensions of proximity: cognitive, organizational, social, institutional, and geographical. However, how patients react to these changes in care delivery and the representations of these practices remain unanswered questions. Therefore, exploring patient’s representations of telehealth is important to better understand psychological mechanisms underlying the adherence to telehealth. The theory of social representations is fruitful in overcoming this limitation.
The Social Representations Theory
The theoretical background of social representations provides a framework for understanding how new concepts become common knowledge. Defined as a collective elaboration “of a social object by the community for the purpose of behaving and communicating” [ 29 ], social representations consist of a system of values, ideas, and practices that enable individuals to orient themselves in their material and social world as well as to master it and provide a code for social exchange [ 30 ]. Therefore, social representations provide people with a common frame of communication that is built in everyday interactions. More precisely, a social representation corresponds to thoughts and feelings being expressed in verbal and overt behavior of actors that constitutes an object for a social group [ 31 ].
Although social representations are commonly shared, some may be more polemical, reflecting oppositions between social groups in society [ 32 ]. In addition, social representations have a dynamic nature across and within social groups of people, and societal practices, communication, and the process of knowledge are strongly connected, particularly in the health field, which has been one of the leading research areas for this theory [ 33 - 35 ].
Interestingly, social representations constitute a structure explaining behaviors that result not only from an individual cognitive process but also from social and cultural representations and that are shared collectively [ 36 , 37 ]. Social representations have been shown to be a significant indicator of attitudes [ 38 , 39 ]. However, social representations of patients have never been studied in the context of telehealth specifically. A qualitative study is well suited to understand these representations. The objective of our qualitative research was to establish a comprehensive conceptual framework to gain a better understanding of how telehealth influences perceived proximity or distance for patients and therefore, to better apprehend their barriers as well as their motivations to adopting telehealth tools.
Study Design
A qualitative study was conducted with an interpretative approach to explore patients’ representations of telehealth and their perception of proximity toward it. We adopted an inductive, constructivist perspective, assuming that people construct their life-worlds through their representations and interpretations of telehealth as a social fact to which they attribute specific terms and meanings.
Setting and Sample
Qualitative in-depth individual interviews were set up using a semistructured thematic interview guide. Convenience sampling was used to recruit participants. Variation sampling was sought [ 40 ] with consideration of gender, age, location (urban/rural), and socioeconomic background ( Multimedia Appendix 1 ). We used the saturation criterion to stop recruitment. This criterion is the point at which gathering more data about a theoretical construct reveals no new properties nor yields any further theoretical insights [ 41 ]. This saturation point is usually reached with 9 to 17 interviews [ 42 ].
Data Collection
After obtaining informed consent, patients were contacted, and an appointment for an interview was set. Interviews lasted from 45 minutes to 75 minutes and were performed directly inside the family home or conducted through the digital platform Microsoft Teams because of the geographical distance between the researcher and the participant. The study took place in May 2022. A total of 14 interviews were gathered: 8 participants were female, 6 were male, and their mean age was 52 (range 23-83) years. Of the interviews, 11 interviews were run face to face, and 4 were online.
The interview guide explored various aspects of how health and telehealth are perceived; including defining what constitutes perceived good health; understanding respondents’ relationship with their own health; examining how they seek health-related information; discussing challenges in accessing care as related to geographical, temporal, and perceived distances; and evaluating respondents’ overall and specific relationships with technology within the context of health care. This comprehensive approach aimed to gain insights into how individuals perceive telehealth and their level of engagement with it.
During each interview, we wrote down our impressions that could possibly impact the interpretation of results. Interviews were digitally audio-recorded with permission, and verbatims were transcribed.
Ethical Considerations
At the beginning of each interview, potential participants were given comprehensive information about the context, objectives, and methods of the study. The interviewees were informed that they could withdraw from this study at any time. After allowing enough time for any questions or clarification they may have required, all the participants gave their informed consent. The study design was reviewed and approved by the Research Ethics Committee of Paris Dauphine–PSL (Paris Sciences & Lettres) University (20231128/01). Additionally, following national legislation, data were pseudonymized during the transcription process in a way that no participant could be directly identified: A number was assigned to each participant with no record of any directly identifying data. Participants received no compensation for participating in this research.
Data Analysis
First, we conducted a vertical analysis and read the transcripts to get an impression of the whole data set. Second, transcribed data were analyzed using a horizontal thematic analysis to develop a narrative of the findings through a categorical approach using qualitative software (NVivo Version 12). We followed the grounded theory approach to code verbatim [ 43 ]: Each transcript was coded inductively by manually marking central key words that could represent a code. The codes were then grouped under themes that emerged through the analysis process. Finally, we categorized the data by collapsing codes that conveyed similar meanings. Multimedia Appendix 2 presents an example of our analysis process.
After the first step of the analysis of social representations, which was to record all the dimensions that emerged from the participants, we used the semiotic square method to map semantic categories highlighting opposing and complementary concepts [ 44 ]. This structure enables the understanding of the tension among symbolic meanings and the elements by which meaning is being expressed [ 45 ]. The semiotic square has been often applied in consumer research [ 46 ] and specifically to explore consumers’ relationships with technology ideology [ 47 ].
First, a specific definition of telehealth emerged from the patient perspective. If researchers define telehealth broadly, the interview analysis revealed that telehealth is associated with teleconsultation for a large majority of patients and rarely with other practices. It concerns mainly remote care and is associated with questions about the quality of interactions with the physician.
Second, the content analysis revealed 4 main types of social representations of telehealth: the expected opposition between engagement and hostility and a more subtle distinction between integration and constraint.
Representations of Proximity: the Logic of Engagement
Our analysis of interviews revealed the first category of very positive social representations related to telehealth that led to a logic of engagement and adherence to this practice. This commitment is based on the idea of optimization of health services. The strong proximity with its practice is explained by a feeling of comfort and a perception of convenience. Telehealth is considered an easy, practical tool. Participant 4 (P4) mentioned:
I found it practical and comfortable.
Perceived practicality and convenience underline the actual benefits of adopting telehealth. Indeed, this practice enables a reduction of the perceived temporal distance to the consultation, leading to representations of efficiency and effectiveness (P13) on one hand and allowing reinforcement of access to care, which creates a feeling of personal usefulness (P8), on the other hand. Participant 13 (P13) mentioned:
Now that everything is overbooked in their appointments, (...), we are at about 15 days/3 weeks for getting any new appointment, both by phone or by Doctolib, in video, it is a little faster.
In addition, participant 8 (P8) said:
It is so quick, it makes everyday life easier!
From this perspective, the main issue behind social representations of proximity is related to an improvement of the functional proximity to telehealth.
Representations of Distance: the Logic of Hostility
At the opposite end to that of the first category, the second category of social representations follows a logic of hostility toward telehealth. It reveals a strong rejection of its development. Although adherence follows a view of functional proximity, rejection is explained by a lack of perceived relational proximity caused by telehealth. These representations of perceived distance reveal a profound fear of the dehumanization of medicine. Telehealth is seen as a dehumanizing practice that is destructive of human interaction by virtualizing both patients and care, as Kaplan [ 20 ] mentioned. This was confirmed by participant 6 (P6), who stated:
It kills the human contact, which is really important to me. I definitely prefer having the secretary over the phone to tell me there is an appointment in three weeks.
The major component of this category is the perceived deterioration of the relationship with the physician. Great importance is given to the human dimension in care. However, the interviews revealed these representations are based on a feeling of detachment from the caregiver caused by telehealth. This emphasizes the impersonal nature of the relationship. Participant 2 (P2) stated:
We dematerialize everything. It brings detachment from the caregiver.
From this perspective, the development of a relationship with perceived proximity and trust seems incompatible with distant and remote care. The virtual nature of this link is intrinsically considered as the opposite of human interaction. Participant 1 (P1) stated:
I do not like it. I like to see the person right in front of me.
Here, social representations of telehealth found an increase of perceived distance between the patient and physician. The perception of actual proximity to the physician tends to disappear with telehealth, which reinforces emotional and affective distance [ 48 ]. These representations finally highlight the fact that telehealth cannot replace an in-person consultation. For instance, participant 5 (P5) stated:
I would not make [a remote physician] my referring physician. There need to be a close relationship with him. I must be able to give him my trust. I am not sure that I will always have the same doctor when using teleconsultation.
Altogether, these depictions of distance nurture the perception that telehealth has a detrimental or potentially harmful impact on society, as it undermines the interpersonal nature of care.
In addition to these 2 opposite categories of representations, proximity versus distance, more nuanced types of social representations also emerged within the verbatims. We labelled them “nondistance” and “nonproximity” representations.
Representations of Nondistance: the Logic of Integration
The third category of social representations reflects “nondistance” to telehealth, as these representations are related neither to total adherence nor to rejection but rather follow a logic of integration: Participants highlighted the actual possibility to choose to use (or not) telehealth tools. Representations do not reflect a full engagement with this practice but rather a nonrejection of telehealth.
First, these representations of nondistance highlight the functional aspects of this practice. In this context, developing a relational proximity with the physician was not judged as necessary. For instance, participant 4 (P4) stated:
I felt more like I was with a teleoperator than a physician. It felt like there was a script behind it, but why not, that is not necessarily a bad thing.
This situation is not seen as a problem; the efficient and nonrelational aspect of the consultation is valued here. Thus, this representation shows a greater emphasis on the functional proximity rather than on the relational proximity [ 24 ].
The importance given to the functional aspects of telehealth was also revealed through the way specific health practices are elicited. For instance, telehealth was mainly seen as a backup or emergency solution, leading to occasional use according to the situation. Participant 5 (P5) stated:
It can be a first step to detect an emergency. For example, if you cannot get a doctor during the weekend, we have remote visits (...) So, to me, it is an emergency solution.
Because it is convenient, patients do not reject telehealth, especially when there is no need to be seen in person, for example for a prescription renewal, as suggested by participant 4 (P4):
It depends on what you are looking for in the consultation. If it is for a medication renewal, yes, I would recommend it.
Thus, these representations of nondistance do not refer to hostility nor engagement toward telehealth but rather to tolerance. The practice is adopted but not entirely accepted. Indeed, the use of telehealth should remain occasional. Participant 11 (P11) said:
If I were starting using a teleconsultation system, I would say to myself ‘no more than three times in a row.’ The fourth time, you still have to go, once every two years for a check-up, I would tend to say that.
Tolerance comes also with some reluctance about the reliability of this practice. Telehealth was perceived as less reliable than a physical consultation because there is no physical contact and no auscultation, which seems to lead to mistrust, as suggested by participants 10 (P10) and 14 (P14).
Auscultation is one of the first things you learn in medicine, like touching the patient. Try to get an auscultation from a machine, to put its hands on the belly. [P10]
When I had my operation, I had a consultation with the anesthesiologist by teleconsultation. It was silly, he told me to pull my tongue out (...) No, for me this is ridiculous! [P14]
Overall, social representations related to nondistance reveal nonrejection of telehealth under conditions of efficiency and reliability. The choice of using telehealth tools is made under specific circumstances and leads to occasional use, based on high value placed on simplicity and functional aspects.
Representations of Nonproximity: the Logic of Constraint
Within the fourth category, social representations are related to “nonproximity,” a label that reflects a logic of constraint. Whereas representations of proximity highlight engagement and active behavior toward telehealth, representations of nonproximity depict situations of the use of telehealth when there is no other choice, as participant 10 (P10) mentioned:
Is telehealth a good thing? Like everyone else, I use it because I am left with no alternative option.
Patients come to telehealth whenever they have no or few alternatives, considering telehealth as a last option, such as during a lockdown for example, as explained by participant 3 (P3):
If I had to use it, it would really be out of obligation, like during a lockdown, and because I do not have the possibility to move around.
In this perspective, telehealth tools are not really accepted and should remain a second option to physical in-person consultations, mainly because telehealth requires digital literacy. Participant 7 (P7) explained:
For the elderly, it is a problem! I have to schedule their appointments from my own mobile phone because they do not have access to the internet.
Thus, like the representations of perceived distance, the representations of nonproximity are also mostly negative. However, they do not reflect a total rejection of the practice of telehealth but rather a nonadherence as patients come to it when they have no other option.
Finally, our qualitative analysis allowed us to structure a semiotic square ( Figure 1 ) with 2 main categories of social representations of telehealth (ie, perceived proximity and perceived distance) as well as subsequent tensions in the discourse. The negation of these 2 terms forms 2 other categories illustrating 4 distinctive classes of meanings highlighting nuanced representations of perceived proximity and distance to telehealth and the opposite and complementary relationships [ 49 ]. The main components of the 4 categories are summarized in Figure 1 . Interestingly, 2 additional analyses of the semiotic square improve our vision of social representations of telehealth, one based on a vertical reading and the other based on a horizontal reading.

Telehealth: Socially Beneficial Versus Socially Dangerous
From a vertical reading of the relationships between the categories, there are 2 structuring representations of telehealth. In the left part of the semiotic square ( Figure 1 ), the complementary relationship, linking proximity and nondistance, refers to favorable representations as well as to discourse encouraging the development of telehealth. These tools are perceived as socially beneficial for all stakeholders, but there is room for improvement in generalizing their use.
Within these favorable representations of telehealth, adherence and nonrejection are based on 2 main drivers. First, trust in the physician is crucial as he or she is considered a legitimate expert, as suggested by participant 4 (P4):
I feel that doctors are experts (...), I trust them entirely because to me they seem to be experts.
Consequently, positive representations of telehealth seem to be linked to the perceived relational proximity with the health care professional. Second, these representations stem from familiarity with the tool. Being familiar with the term “telehealth” and knowing what it means generate a feeling of closeness toward it. Participant 5 (P5) stated:
I heard [about telehealth] because in my profession—I work with pharmacies—we talk about it.
In the right part of the square is the complementary relationship combining perceived distance and nonproximity. This underlines representations of hostility and skepticism toward telehealth, which are considered socially harmful or even dangerous for society. Rejection and nonadherence seem to be explained mainly by insufficient digital literacy as well as difficulties accessing the Internet and telehealth tools, as suggested by participant 9 (P9):
No, I do not use the Internet at all! (...) There are surely many things to do but I do not know how to do them...
This revealed a substantial cognitive distance to telehealth and ultimately making care practices feel more complex. The ancestral role of auscultation in medical consultation and the importance given to touching patients are noted, showing that the lack of perceived physical proximity between the patient and physician tends to reinforce the psychological distance toward telehealth and ultimately the rejection of its practice. Participant 4 (P4) said:
The ability itself of performing an actual auscultation by touching people and listening to them using a stethoscope is being lost at the expense of the care to improve the development of technology.
Telehealth: Ideological Versus Pragmatic Postures
A horizontal reading highlights the similarity of the logics of engagement and hostility, both based on ideological postures: pros and cons of the practice of telehealth depending on whether it seems to belong to the “good” versus “bad” for the society. More efficiencies appear to be pros, and less of a human relationship appears to be a con. Conversely, the logics of integration and constrain reflect pragmatic postures: how to deal with the tool and on what occasion. Sometimes, it appears to be accepted because it is convenient and adapted to specific situations, sometimes because there is no other choice. Interestingly, ideological postures tend to separate opposite groups, while the pragmatic views tend to rebuild a link between the nondistance and nonproximity groups. These nuanced, more balanced perceptions invite us to think about the practical implications, elaborating “paths” of social representations to drive patients toward less rejection of and more adherence to telehealth.
Main Findings
Using qualitative methods, our findings suggest a new conceptual framework to apprehend telehealth from patients’ perspectives based on 4 categories of social representations. First, perceived proximity was associated with social representations reflecting the idea that telehealth is intrinsically an efficient, practical, and effective solution. This logic of engagement is in line with a strong belief in progress and technological tools to face the challenges of the health care system, namely the issue of access to care. On the opposite side, social representations were more related to a feeling of distance from telehealth, enforcing an unfavorable attitude and leading to a rejection of these tools. This logic of hostility is mainly anchored in a fear of dehumanization of society. Telehealth is blamed for compromising the quality of the relations and for accelerating the loss of human contact between patients and physicians. This perceived distance from telehealth highlights a situation of exclusion, especially for patients who do not have access to digital technology or who do not have sufficient digital literacy. Aside from these 2 categories, 2 more nuanced types of representations emerged. First, from a logic of integration, social representations revealed that telehealth is appealing but showed worries and fears about its reliability. This practice can be conditionally accepted according to a situational approach. Second, a logic of constraint reflected social representations based on skepticism but leading to acceptance when there are no alternatives.
From a theoretical point of view, our results, based on a semiotic square, bring new elements to the literature of perceived proximity. We have shown that telehealth leads to reconsidering proximity through several dimensions. Although not diminishing the geographical or physical gap between the patient and the health care provider, technological tools, such as a teleconsultation from home, can enhance accessibility to health care. The relational dimension of proximity, already identified by Boschma [ 25 ], seems to also be impacted by telehealth. Indeed, many social representations have shown that this perception of proximity with the caregiver is reduced by telehealth and revealed a fear of dehumanization in the relationship. In addition, we showed that perceived functional proximity to telehealth leads to increased adherence and a favorable attitude to its development, which should encourage policymakers to strengthen this aspect in communication strategies for telehealth. These findings also constitute a societal contribution. In addition, this research has revealed 2 major oppositions embedded in the social representations. The first one consists of “good,” or a socially beneficial position, versus “bad,” or a socially dangerous position. The second one highlights the posture, rather “ideological” or “pragmatic,” leading to contributions to public policy aiming to foster adherence to eHealth tools.
Building a semiotic square also revealed potential changes in people’s representations of telehealth and thus the potential to contribute to change attitudes toward these tools. They may be adapted to patients’ concerns and aspects that patients value in the practice of consultation. Our qualitative material brings insight to how these representations can be obstacles to the adoption of telehealth, as well as elements that can foster adherence. We propose considering paths through which patients’ representations could evolve. Mobilizing the social representations along these paths could first alleviate the perception of distance to the health care professional then enable the perceived proximity to telehealth. Our analysis emphasized some risks in how telehealth is implemented. If telehealth is developed without considering representations expressing reluctancy, individuals who are subjected to the use of telehealth may remain hostile to its development, may gradually feel a distance to it, and may finally totally reject this practice (coming from nonproximity to distance). To avoid such a vicious circle, 2 paths ( Figure 2 ) may create an increased feeling of proximity to telehealth.

The first path consists of transforming representations related to a perceived nondistance into a perceived proximity to telehealth. This pathway adopts a functional approach to consultation. The challenge is to dispel fears about the technological feasibility of using digital health tools to eliminate skepticism and reinforce favorable representations. It would then be necessary to reassure patients about the importance of any human contact during medical consultations. Highlighting the regular and immediate exchanges with physicians that telehealth allows would be perceived as helpful. Developing remote auscultation solutions and increasing communication about them by highlighting the accuracy and reliability of these technologies would help to alleviate these concerns and encourage adherence to these tools. Finally, reinforcing the benefits in terms of efficiency, time optimization, and practicality would contribute to (1) reducing the perceived temporal distance of access to care and (2) increasing the perceived functional proximity to telehealth.
The second path consists of (1) transforming representations related to a perceived distance into a feeling of nonproximity and subsequently (2) fostering the perceived proximity to telehealth. This path is mainly aimed at individuals who attach great importance to the relational and human dimension of care. The first challenge would be to strengthen trust in the health care system because representations and attitudes toward telehealth are intrinsically linked to the relationship patients develop with the health care system and physicians. It is also necessary to improve access to digital technology to reduce the cognitive distance and to increase their perception of proximity. Finally, highlighting and communicating about the strengthening of relational and affective proximity, allowed by telehealth when facilitating contact between patients and physicians, could lead to favorable representations and attitudes. Therefore, conceiving a system of medical support with a health care professional in telehealth booths could be an effective solution.
Limitations and Research Avenues
This study has some limitations. First, our sample did not include patients with a broad range of diseases: Very few of them had chronic diseases. Due to the sample size, we could not cover all medical specialties: For instance, ophthalmology and the need for emergency surgery may bring specific representations of telehealth for patients. It could also be interesting to interview people from other rural areas known as “medical deserts” (ie, regions with inadequate access to health care). In addition, we interviewed patients who do not practice as health care professionals. To broaden our research findings, we could incorporate additional insights by examining the perceptions of telehealth among other groups, particularly caregivers.
The development of telehealth tools leads to new challenges in medical practice. The social representations telehealth brings go beyond the perception of proximity and distance, are multifaceted, and include postures and attitudes. The social representations revealed by the semiotic square on perceived proximity to telehealth underscore the importance of designing health care strategies based on a patient-centric approach in the implementation of digital health tools.
Acknowledgments
The authors would like to thank all the participants in this study.
Data Availability
The data sets generated and analyzed during this study are available upon reasonable request from the corresponding author.
Authors' Contributions
This study was carried out by all authors working collaboratively. AL initiated the proposal. AL and FL conceived the study, collected the data, and performed the qualitative analysis. AL wrote the first draft of the manuscript. FL, VG, and JPB participated in data interpretation and revised the manuscript. All authors approved the final version of the manuscript.
Conflicts of Interest
JPB is a physician and works at SKEZI, a company that develops digital tools in health. The remaining authors have no conflicts of interest to declare.
Characteristics of patients.
Example of the qualitative analysis process used in this research.
- 58th World Health assembly report. World Health Organization. 2005. URL: https://apps.who.int/gb/ebwha/pdf_files/WHA58-REC1/english/A58_2005_REC1-en.pdf [accessed 2024-04-12]
- Queirós A, Alvarelhão J, Cerqueira M, Silva A, Santos M, Pacheco Rocha N. Remote care technology: a systematic review of reviews and meta-analyses. Technologies. Feb 10, 2018;6(1):22. [ CrossRef ]
- Fatehi F, Wootton R. Telemedicine, telehealth or e-health? A bibliometric analysis of the trends in the use of these terms. J Telemed Telecare. Dec 01, 2012;18(8):460-464. [ CrossRef ] [ Medline ]
- Bitar H, Alismail S. The role of eHealth, telehealth, and telemedicine for chronic disease patients during COVID-19 pandemic: A rapid systematic review. Digit Health. Apr 19, 2021;7:20552076211009396. [ FREE Full text ] [ CrossRef ] [ Medline ]
- Telemedicine and Telehealth. The Office of the National Coordinator for Health Information Technology (ONC). URL: https://www.healthit.gov/topic/health-it-health-care-settings/telemedicine-and-telehealth [accessed 2024-04-12]
- Meskó B, Drobni Z, Bényei É, Gergely B, Győrffy Z. Digital health is a cultural transformation of traditional healthcare. Mhealth. Sep 2017;3:38-38. [ FREE Full text ] [ CrossRef ] [ Medline ]
- Lindberg J, Bhatt R, Ferm A. Older people and rural eHealth: perceptions of caring relations and their effects on engagement in digital primary health care. Scand J Caring Sci. Dec 14, 2021;35(4):1322-1331. [ FREE Full text ] [ CrossRef ] [ Medline ]
- From innovation to implementation: eHealth in the WHO European Region. World Health Organization. 2016. URL: https://apps.who.int/iris/handle/10665/326317 [accessed 2024-04-12]
- Wang W, Sun L, Liu T, Lai T. The use of E-health during the COVID-19 pandemic: a case study in China's Hubei province. Health Sociol Rev. Nov 23, 2022;31(3):215-231. [ FREE Full text ] [ CrossRef ] [ Medline ]
- Gajarawala SN, Pelkowski JN. Telehealth benefits and barriers. J Nurse Pract. Feb 2021;17(2):218-221. [ FREE Full text ] [ CrossRef ] [ Medline ]
- Gagnon M, Duplantie J, Fortin J, Landry R. Implementing telehealth to support medical practice in rural/remote regions: what are the conditions for success? Implement Sci. Aug 24, 2006;1(1):18. [ FREE Full text ] [ CrossRef ] [ Medline ]
- Clemensen J. Patient empowerment and new citizen roles through telehealth technologies - The early stage. Proceedings of The Third International Conference on eHealth, Telemedicine, and Social Medicine. 2011.:114-119. [ FREE Full text ]
- Goodridge D, Marciniuk D. Rural and remote care: Overcoming the challenges of distance. Chron Respir Dis. May 21, 2016;13(2):192-203. [ FREE Full text ] [ CrossRef ] [ Medline ]
- Smith B, Magnani JW. New technologies, new disparities: The intersection of electronic health and digital health literacy. Int J Cardiol. Oct 01, 2019;292:280-282. [ FREE Full text ] [ CrossRef ] [ Medline ]
- Reiners F, Sturm J, Bouw LJ, Wouters EJ. Sociodemographic factors influencing the use of eHealth in people with chronic diseases. Int J Environ Res Public Health. Feb 21, 2019;16(4):645. [ FREE Full text ] [ CrossRef ] [ Medline ]
- Dunn P, Hazzard E. Technology approaches to digital health literacy. Int J Cardiol. Oct 15, 2019;293:294-296. [ CrossRef ] [ Medline ]
- First meeting of WHO GCM/NCD Working Group on health education and health literacy for noncommunicable diseases. Geneva Global Health Hub. Feb 17, 2017. URL: https://g2h2.org/posts/event/first-meeting-of-who-gcmncd-working-group-on-health-education-and-health-literacy-for-noncommunicable-diseases/ [accessed 2024-04-12]
- Salemink K, Strijker D, Bosworth G. Rural development in the digital age: A systematic literature review on unequal ICT availability, adoption, and use in rural areas. Journal of Rural Studies. Aug 2017;54:360-371. [ CrossRef ]
- Hjelm NM. Benefits and drawbacks of telemedicine. J Telemed Telecare. Jun 24, 2005;11(2):60-70. [ CrossRef ] [ Medline ]
- Kaplan B. Revisiting health information technology ethical, legal, and social issues and evaluation: telehealth/telemedicine and COVID-19. Int J Med Inform. Nov 2020;143:104239. [ FREE Full text ] [ CrossRef ] [ Medline ]
- Huryk L. Factors influencing nurses' attitudes towards healthcare information technology. J Nurs Manag. Jul 2010;18(5):606-612. [ CrossRef ] [ Medline ]
- Rørtveit K, Sætre Hansen B, Leiknes I, Joa I, Testad I, Severinsson E. Patients’ experiences of trust in the patient-nurse relationship—a systematic review of qualitative studies. Open Journal of Nursing. 2015;05(03):195-209. [ FREE Full text ] [ CrossRef ]
- Oudshoorn N. Physical and digital proximity: emerging ways of health care in face-to-face and telemonitoring of heart-failure patients. Sociol Health Illn. Apr 2009;31(3):390-405. [ FREE Full text ] [ CrossRef ] [ Medline ]
- Torre A, Rallet A. Proximity and localization. Regional Studies. Feb 2005;39(1):47-59. [ CrossRef ]
- Boschma R. Proximity and innovation: a critical assessment. Regional Studies. Feb 2005;39(1):61-74. [ CrossRef ]
- Wilson JM, Boyer O'Leary M, Metiu A, Jett QR. Perceived proximity in virtual work: explaining the paradox of far-but-close. Organization Studies. May 20, 2008;29(7):979-1002. [ CrossRef ]
- van Zoonen W, Sivunen A, Rice RE, Treem JW. Organizational information and communication technologies and their influence on communication visibility and perceived proximity. International Journal of Business Communication. Oct 06, 2021;60(4):1267-1289. [ CrossRef ]
- Talbot D, Charreire Petit S, Pokrovsky A. La proximité comme perception de la distance. Le cas de la télémédecine. Rev. Fr. Gest. Nov 11, 2020;46(289):51-74. [ CrossRef ]
- Moscovici S. Attitudes and opinions. Annu Rev Psychol. Jan 1963;14(1):231-260. [ CrossRef ] [ Medline ]
- Moscovici S. Foreword. In: Herzlich C, editor. Health and illness A social psychological analysis. London, England. Academic Press; 1973;IX-XIV.
- Wagner W, Duveen G, Farr R, Jovchelovitch S, Lorenzi‐Cioldi F, Marková I, et al. Theory and method of social representations. Asian J of Social Psycho. Dec 18, 2002;2(1):95-125. [ CrossRef ]
- Manuti A, Mininni G. Social representations of medically assisted fecundation a study on the discursive construction of “media texts”. Psychology. 2010;01(05):337-348. [ FREE Full text ] [ CrossRef ]
- de Rosa AS, Wagner W. The social representations of mental illness in children and adults. In: Doise W, Moscovici S, editors. Current issues in European Social Psychology. Cambridge, United Kingdom. Cambridge University Press; 1987;47-138.
- de Rosa AS, Bocci E. Resisting cognitive polyphasia in social representations of madness. In: de Rosa AS, editor. Social Representations in the 'Social Arena'. Abingdon, United Kingdom. Routledge; 2012;310.
- de Rosa AS, Mannarini T. The “Invisible Other”: social representations of COVID-19 pandemic in media and institutional discourse. Papers on Social Representations. 2020;29(2):1-35. [ FREE Full text ]
- Moliner P, Tafani E. Attitudes and social representations: a theoretical and experimental approach. European Journal of Social Psychology. Dec 04, 1998;27(6):687-702. [ CrossRef ]
- Bidjari AF. Attitude and social representation. Procedia - Social and Behavioral Sciences. 2011;30:1593-1597. [ CrossRef ]
- Monterrubio JC, Andriotis K. Social representations and community attitudes towards spring breakers. Tourism Geographies. Mar 03, 2014;16(2):288-302. [ CrossRef ]
- Schultes M, Kollmayer M, Mejeh M, Spiel C. Attitudes toward evaluation: An exploratory study of students' and stakeholders' social representations. Eval Program Plann. Oct 2018;70:44-50. [ CrossRef ] [ Medline ]
- Patton MQ. Qualitative Research & Evaluation Methods. Thousand Oaks, CA. SAGE Publications, Inc; 2002.
- Bryant A, Charmaz K. The SAGE Handbook of Grounded Theory. Thousand Oaks, CA. SAGE Publications Ltd; 2007.
- Hennink M, Kaiser BN. Sample sizes for saturation in qualitative research: A systematic review of empirical tests. Soc Sci Med. Jan 2022;292:114523. [ FREE Full text ] [ CrossRef ] [ Medline ]
- Strauss A, Corbin JM. Grounded Theory in Practice. Thousand Oaks, CA. SAGE Publications, Inc; 1997.
- Greimas AJ. Structural semantics: An attempt at a method. Lincoln, NE. University of Nebraska Press; 1983.
- Signori P, Flint DJ. Revealing the unique blend of meanings in corporate identity: An application of the semiotic square. Journal of Marketing Theory and Practice. Nov 20, 2019;28(1):26-42. [ CrossRef ]
- Floch J. The contribution of structural semiotics to the design of a hypermarket. International Journal of Research in Marketing. Jan 1988;4(3):233-252. [ CrossRef ]
- Kozinets RV. Technology/ideology: how ideological fields influence consumers' technology narratives. Journal of Consumer Research. 2008;34(6):865-881. [ CrossRef ]
- Fiedler K. Construal level theory as an integrative framework for behavioral decision‐making research and consumer psychology. J Consum Psychol. Jan 22, 2008;17(2):101-106. [ CrossRef ]
- Greimas AJ, Rastier F. The interaction of semiotic constraints. Yale French Studies. 1968;(41):86. [ CrossRef ]
Abbreviations
Edited by A Mavragani; submitted 13.01.23; peer-reviewed by S Tubeuf, H Yu; comments to author 13.03.23; revised version received 08.05.23; accepted 19.12.23; published 26.04.24.
©Amélie Loriot, Fabrice Larceneux, Valérie Guillard, Jean-Philippe Bertocchio. Originally published in the Journal of Medical Internet Research (https://www.jmir.org), 26.04.2024.
This is an open-access article distributed under the terms of the Creative Commons Attribution License (https://creativecommons.org/licenses/by/4.0/), which permits unrestricted use, distribution, and reproduction in any medium, provided the original work, first published in the Journal of Medical Internet Research, is properly cited. The complete bibliographic information, a link to the original publication on https://www.jmir.org/, as well as this copyright and license information must be included.
How to Perform User Needs Analysis For SaaS
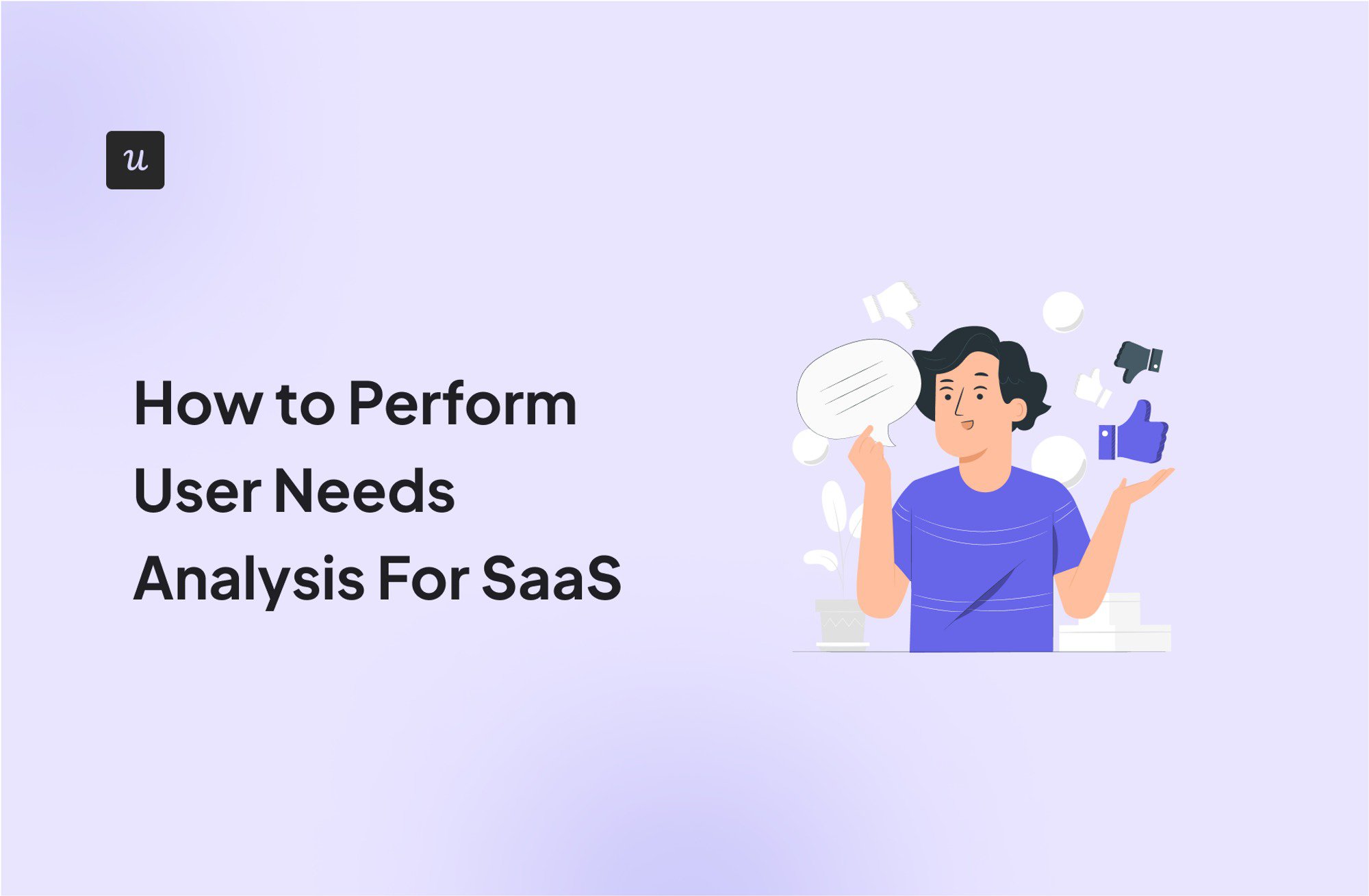
What’s the best way to ensure your product hits the right chord with users? A user needs analysis conducted with product analytics data lets you build products that address user pain points and exceed their expectations.
In this article, we dig deeper into what user needs analysis is and how you can get started with it.
- User needs analysis refers to using various techniques to understand users’ aspirations, goals, and pain points.
- It helps you make data-driven decisions to enhance your product and keep up with changing user needs to improve user satisfaction .
- You can perform a needs analysis using various methods, such as user feedback surveys, focus groups, and interviews. Usability testing can also come in handy.
- The first step to conducting this analysis is to set a SMART goal for your analysis.
- Then, select a user persona to drill into a particular segment’s preferences and problems.
- Analyze user behavior data collected to identify patterns and trends.
- Also, collect qualitative data through open and close-ended surveys from various user segments.
- Guide your product development process with the insights you’ve gathered.
- Userpilot can help you monitor user behavior and collect qualitative feedback with in-app surveys. Book a demo now.

Try Userpilot and Take Your Customer Satisfaction to the Next Level
- 14 Day Trial
- No Credit Card Required
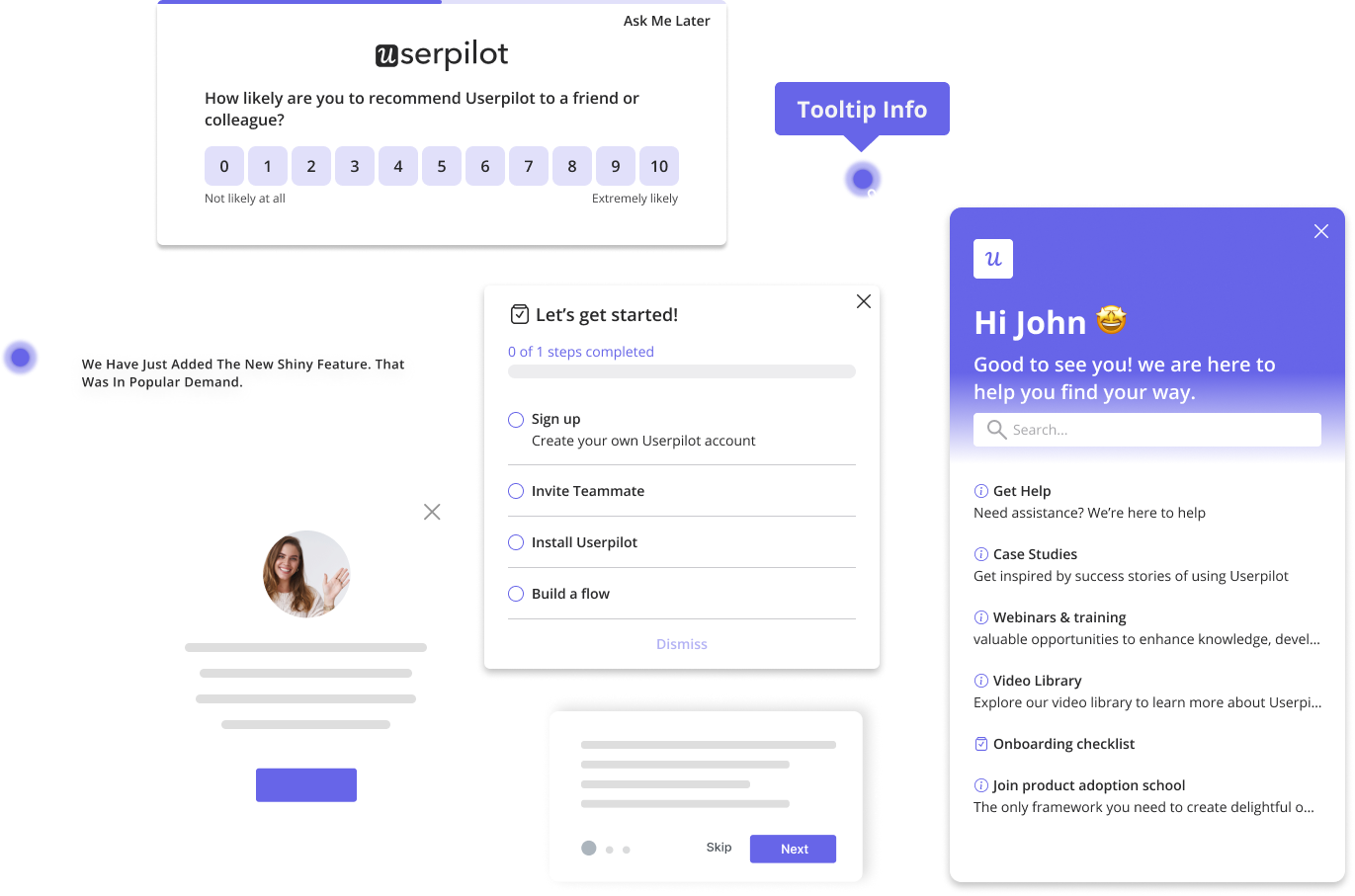
What is a user needs analysis?
User needs analysis employs various techniques to help you gain deeper insights into your user base’s aspirations , goals, and motivations. It also enables you to understand user pain points and preferences.
A needs analysis validates your assumptions regarding your users so you can better guide product development, marketing, and launches .
Why do you need to analyze user needs?
If you want to build a product that attracts, engages, and retains the right users, performing user needs analysis is non-negotiable. Let’s take a look at its key benefits.
- Understanding user pain points – Analyzing user needs helps you build a solid understanding of user challenges. It gives you an idea of what will motivate them to use your product, allowing you to build features shaped around their needs.
- Keeping up with changes in how users behave – With this analysis, you can stay on top of changing target audience preferences and behavior patterns . It ensures that your product adapts as your users’ needs change.
- Creating data-driven product strategies – It takes the guesswork out of product development. Instead, you can use concrete data to determine what features, functionalities, and upgrades will align with your audience’s requirements.
- Increasing user satisfaction – When you build a product tailored to your target user’s expectations and needs, it improves the overall user experience (UX). That, in turn, skyrockets user satisfaction levels and even helps you engage and retain more users.
- Identifying and removing friction – With user needs analysis, you can dig deep into their journey and identify the challenges your target audience faces when navigating your product. It comes in handy for pinpointing and eliminating areas of friction , resulting in enhanced UX.
User research methods to identify user requirements
Analyzing user needs is an integral part of building a product that stands out from the crowd. But what’s the right way to do it?
Here are a few user research techniques that’ll come in handy:
1. User feedback surveys
How do you know what users want? One of the most effective methods is to collect first-hand feedback using in-app surveys.
You can set up and trigger in-app surveys at specific points in a user’s journey to learn more about user requirements, needs, pain points, and preferences. For instance, when a new user logs in, you can use a welcome survey to ask about the tasks they want to accomplish.
On the other hand, if a user has been using your product for some time, you can use in-app surveys to dig deeper into their experience.

A tool like Userpilot comes in handy for creating and implementing feedback surveys. You can choose from several survey templates , such as NPS surveys and CES surveys, and include a mix of open-ended and close-ended questions. You can even use branching logic to modify the flow of questions based on previous responses.

In-app user surveys are a good way to collect feedback from active users. In order to collect feedback from inactive or potential users, you can deploy email surveys.
If you’re already using a CRM platform like HubSpot, you can integrate it with Userpilot . That way, you can leverage existing data from Userpilot to send relevant email surveys via HubSpot.

2. Focus groups
A focus group is an effective user research technique for collecting in-depth qualitative feedback , much like usability testing. It involves gathering a small group of users (usually 6-12 participants) and encouraging them to engage in discussions and debates.
Participants are free to share their experiences, ideas, and opinions about your product, and you can record their discussions. A moderator is present to steer conversations in the desired direction while the users interact.
Focus groups are a valuable resource for informing key product development decisions, such as the design process and UI layout. Also, you can use them for market research and validation.
3. User interviews
Interviews help you engage in one-on-one conversations with existing, new, and potential users. It makes them an excellent way to dig deeper into their minds and learn more about why they behave the way they do .
You can collect in-depth qualitative insights about a user’s journey and understand what works for them. Additionally, you can personalize your follow-up questions based on how the conversation is going.
But how do you encourage more people to participate in user research? A clever tactic is to offer an incentive, such as a discount or gift, in exchange.

4. Usability testing
Usability testing is a technique that helps measure how easy it is for people to use your product. It can help identify friction points users encounter when navigating your product. It also helps understand whether users can successfully realize their goals with your product.
If you want to assess product usability for user needs analysis, you can choose from the following types of tests:
- Guerilla testing – You go to a public location, ask people to try your product (or a part of it), and share their feedback.
- Remote usability testing – You use screen-sharing tools or video conferencing apps to observe how people use your product and collect feedback from them.
- 5-second test – You ask users to experience a part of your product for five seconds and share their feedback afterward.
- First-click testing – You focus on determining how easily users can identify the happy path to complete a given task.
How to perform a needs analysis to extract valuable insights
User needs analysis takes more than launching an in-app survey or conducting user interviews . You need a concrete plan to retrieve the right insights from the analysis, customer surveys, and more.
Here’s a step-by-step guide to help you get started:
1. Define your objectives for user needs analysis
First things first—you must understand why you want to perform a user needs analysis.
Are you looking to validate the idea for a new product? Do you want to identify ways to make users stick around for longer when trying your product? Or do you want to expand user accounts by tempting them with advanced features and add-ons?
Knowing the answers to these questions will give you a clear idea of your objectives . It’ll help you focus on the right user personas and research methods.
It’s a good idea to use the SMART (Specific, Measurable, Achievable, Realistic, Time-bound) goal-setting framework to define goals and KPIs .

2. Choose your user personas
Your customer base comprises different user segments, each with unique aspirations, motivations, pain points , and preferences. Selecting a specific user persona for your analysis will help you drill down on that particular segment’s needs.
The key is to outline detailed user personas , including their professional and socio-economic backgrounds, interests, and struggles.

3. Analyze user behavior data to identify patterns
Now that you’ve selected a user persona, it’s time to monitor and analyze their in-app behavior . A product analytics tool like Userpilot is handy for collecting user behavior data and identifying patterns .
You can leverage techniques, such as funnel and trend analysis to extract more in-depth insights . For instance, with funnel analysis , you can understand how users move from one step to another when completing a task and what causes them to drop off.

Similarly, trend analysis can help you identify and harness recurring patterns in user behavior.
4. Collect qualitative data with surveys to understand user expectations
Next, it’s time to dig deeper into user needs with in-app surveys. These surveys provide an effective way of collecting qualitative feedback from users about their experiences and expectations.
You can ask questions about the tasks they want to accomplish with your product and the challenges they face when using it. With Userpilot, you can choose from a variety of templates to implement different types of in-app surveys.
5. Guide the product development process
Comprehensive user needs analysis takes the guesswork out of product development . With all the qualitative and quantitative data collected in the previous steps, you’ll be better equipped to determine what users want. You can use these findings to guide design and product teams as they develop new features and product enhancements.
Create your product roadmap and add features and enhancements based on their priority. A plan allows multiple teams to collaborate in product development and ensure timely completion.

As you implement these changes, make sure you continue monitoring user activity and behavior. It’ll help you understand whether the modifications are meeting user needs and identify further areas of improvement .
Analyzing user needs offers several benefits, including improved user engagement and satisfaction levels. You can also leverage insights from user needs analysis to inform product design and development decisions.
A product analytics platform such as Userpilot helps SaaS companies collect feedback, monitor user activity, and analyze their behavior. These insights make data-driven product decisions possible. To see how Userpilot can help you with user needs analysis, book your demo today.
Leave a comment Cancel reply
Save my name, email, and website in this browser for the next time I comment.
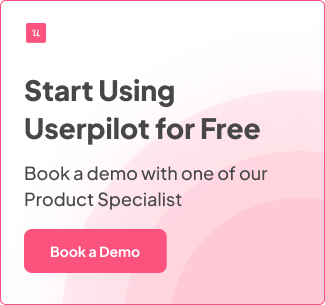
Get The Insights!
The fastest way to learn about Product Growth,Management & Trends.
The coolest way to learn about Product Growth, Management & Trends. Delivered fresh to your inbox, weekly.

The fastest way to learn about Product Growth, Management & Trends.
You might also be interested in ...
[email protected]
10 Customer Metrics to Measure in SaaS
Customer progress tracking: how to do it for saas [+ tools].
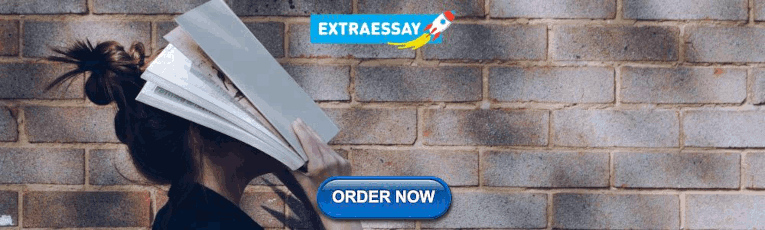
IMAGES
VIDEO
COMMENTS
Step 1: Gather your qualitative data and conduct research (Conduct qualitative research) The first step of qualitative research is to do data collection. Put simply, data collection is gathering all of your data for analysis. A common situation is when qualitative data is spread across various sources.
Qualitative data analysis is vital in uncovering various human experiences, views, and stories. If you're ready to transform your research journey and apply the power of qualitative analysis, now is the moment to do it. Book a demo with QuestionPro today and begin your journey of exploration. LEARN MORE FREE TRIAL
For many researchers unfamiliar with qualitative research, determining how to conduct qualitative analyses is often quite challenging. Part of this challenge is due to the seemingly limitless approaches that a qualitative researcher might leverage, as well as simply learning to think like a qualitative researcher when analyzing data. From framework analysis (Ritchie & Spencer, 1994) to content ...
Qualitative research methods. Each of the research approaches involve using one or more data collection methods.These are some of the most common qualitative methods: Observations: recording what you have seen, heard, or encountered in detailed field notes. Interviews: personally asking people questions in one-on-one conversations. Focus groups: asking questions and generating discussion among ...
Step 5: Query your coded data and write up the analysis. Once you have coded your data, it is time to take the analysis a step further. When using software for qualitative data analysis, it is easy to compare and contrast subsets in your data, such as groups of participants or sets of themes.
Abstract. This paper aims to provide an overview of the use and assessment of qualitative research methods in the health sciences. Qualitative research can be defined as the study of the nature of phenomena and is especially appropriate for answering questions of why something is (not) observed, assessing complex multi-component interventions ...
While many books and articles guide various qualitative research methods and analyses, there is currently no concise resource that explains and differentiates among the most common qualitative approaches. We believe novice qualitative researchers, students planning the design of a qualitative study or taking an introductory qualitative research course, and faculty teaching such courses can ...
of the analysis in qualitative research, in general, a kind of stocktaking of the various approaches to qualitative analysis and of the challenges it faces seems necessary. Anyone interested in the current state and develop-ment of qualitative data analysis will find a field which is constantly growing and becom - ing less structured.
Abstract. Qualitative data is often subjective, rich, and consists of in-depth information normally presented in the form of words. Analysing qualitative data entails reading a large amount of transcripts looking for similarities or differences, and subsequently finding themes and developing categories. Traditionally, researchers 'cut and ...
practice of qualitative research and instructors and mentors who regularly introduce students to qualitative research practices. In this article, we seek to offer what we view as a useful starting point for learning how to do qualitative analysis. We begin by discussing briefly the general landscape of qualitative research methodologies and ...
When to use thematic analysis. Thematic analysis is a good approach to research where you're trying to find out something about people's views, opinions, knowledge, experiences or values from a set of qualitative data - for example, interview transcripts, social media profiles, or survey responses. Some types of research questions you might use thematic analysis to answer:
Qualitative Research. Qualitative research is a type of research methodology that focuses on exploring and understanding people's beliefs, attitudes, behaviors, and experiences through the collection and analysis of non-numerical data. It seeks to answer research questions through the examination of subjective data, such as interviews, focus groups, observations, and textual analysis.
INTRODUCTION. In an earlier paper, 1 we presented an introduction to using qualitative research methods in pharmacy practice. In this article, we review some principles of the collection, analysis, and management of qualitative data to help pharmacists interested in doing research in their practice to continue their learning in this area.
Narrative analysis is a method with a particular history and epistemology, and it is designed to answer certain types of research questions. As part of the growing recognition of the value and legitimacy of qualitative inquiry in psychology, narrative analysis is becoming increasingly articulated and refined.
Qualitative data analysis is. concerned with transforming raw data by searching, evaluating, recogni sing, cod ing, mapping, exploring and describing patterns, trends, themes an d categories in ...
The qualitative research process in just 8 Steps. Learn techniques for collecting and analyzing qualitative data to uncover themes that drive insights. ... Firstly, the researcher will look through secondary data and research (e.g. analytics, previous research reports). Secondary analysis will help determine if there are existing answers to any ...
Let's recap. In this post, we've explored the basics of narrative analysis in qualitative research. The key takeaways are: Narrative analysis is a qualitative analysis method focused on interpreting human experience in the form of stories or narratives.; There are two overarching approaches to narrative analysis: the inductive (exploratory) approach and the deductive (confirmatory) approach.
Effectively present the qualitative research data: Communicate the results in a clear and concise way to help other people understand the findings. Qualitative data analysis. Evaluating qualitative research can be tough when there are several analytics platforms to manage and lots of subjective data sources to compare.
Quality assessment. This paper aims to provide an overview of the use and assessment of qualitative research methods in the health sciences. Qualitative research can be defined as the study of the nature of phenomena and is especially appropriate for answering questions of why something is (not) observed, assessing complex multi-component ...
Content analysis is a research method used to identify patterns in recorded communication. To conduct content analysis, you systematically collect data from a set of texts, which can be written, oral, or visual: Books, newspapers and magazines. Speeches and interviews. Web content and social media posts. Photographs and films.
1. Collect your data. Each of the research methodologies has uses one or more techniques to collect empirical data, including interviews, participant observation, fieldwork, archival research, documentary materials, etc. The form of data collection will depend on the research methodology.
This builds upon previous literature, indicating that rapid analysis can be an effective tool in capturing implementation-oriented themes from qualitative data.How the SECURE Study Can Inform Future Research EffortsOur rapid qualitative methods allowed us to effectively adapt and respond to the quickly evolving landscape of social care ...
Qualitative research is a type of research that explores and provides deeper insights into real-world problems.[1] Instead of collecting numerical data points or intervene or introduce treatments just like in quantitative research, qualitative research helps generate hypotheses as well as further investigate and understand quantitative data. Qualitative research gathers participants ...
Uncover hidden insights in qualitative research with thematic analysis. Learn its flexible process step by step and collaborate effectively using Creately's visual platform. Discover the advantages, challenges, and approaches to thematic analysis. Master the art of qualitative research and unlock the potential of your data with this comprehensive guide.
We frequently test new survey questions ahead of time through qualitative research methods such as focus groups, cognitive interviews, pretesting (often using an online, opt-in sample), or a combination of these approaches. Researchers use insights from this testing to refine questions before they are asked in a production survey, such as on ...
Researchers that endorse this research paradigm privilege intensive, over extensive, research designs, focussing, hence, in ethnographic methods and qualitative interviewing. However, and despite some recent academic proposals, there is little guidance in the literature on how to analyse qualitative data using the lenses of CR to produce causal ...
This paper presents the results of a qualitative research with a biographical approach that delved into the curricular experiences in citizenship of young people who completed their secondary education in Chile, within schools of different dependencies and modalities, in different socioeconomic contexts, and during a period of important student mobilizations (2015-2020).
Having access to qualitative analysis software can be greatly helpful in organising and retrieving analysed data. Just remember, a computer does not analyse the data. As Jennings has stated, "… it is 'peopleware,' not software, that analyses." A major drawback is that qualitative analysis software can be prohibitively expensive.
This paper is in the following e-collection/theme issue: Telehealth and Telemonitoring (1503) E-Health / Health Services Research and New Models of Care (412) Clinical Communication, Electronic Consultation and Telehealth (518) Focus Groups and Qualitative Research for Human Factors Research (724) Theoretical Frameworks and Concepts (218) Theories, Models, and Frameworks in Human Factors (55 ...
The first step to conducting this analysis is to set a SMART goal for your analysis. Then, select a user persona to drill into a particular segment's preferences and problems. Analyze user behavior data collected to identify patterns and trends. Also, collect qualitative data through open and close-ended surveys from various user segments.