
An official website of the United States government
The .gov means it’s official. Federal government websites often end in .gov or .mil. Before sharing sensitive information, make sure you’re on a federal government site.
The site is secure. The https:// ensures that you are connecting to the official website and that any information you provide is encrypted and transmitted securely.
- Publications
- Account settings
Preview improvements coming to the PMC website in October 2024. Learn More or Try it out now .
- Advanced Search
- Journal List
- Int J Environ Res Public Health

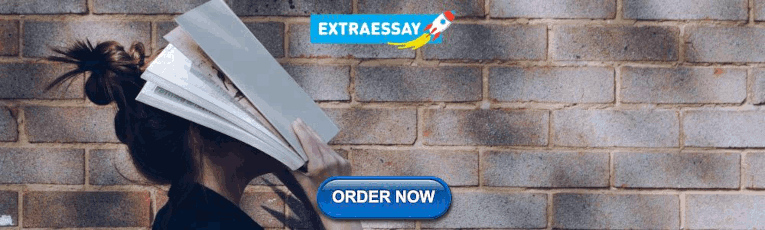
Job Satisfaction: Knowledge, Attitudes, and Practices Analysis in a Well-Educated Population
Paolo montuori.
1 Department of Public Health, University “Federico II”, Via Sergio Pansini N° 5, 80131 Naples, Italy
Michele Sorrentino
Pasquale sarnacchiaro.
2 Department of Law and Economics, University “Federico II”, 80131 Naples, Italy
Fabiana Di Duca
Alfonso nardo, bartolomeo ferrante, daniela d’angelo, salvatore di sarno, francesca pennino, armando masucci, maria triassi, antonio nardone, associated data.
The data that support the findings of this study are available upon reasonable request from the corresponding author. The data are not publicly available due to privacy or ethical restrictions.
Job satisfaction has a huge impact on overall life quality involving social relationships, family connection and perceived health status, affecting job performances, work absenteeism and job turnover. Over the past decades, the attention towards it has grown constantly. The aim of this study is to analyze simultaneously knowledge, attitudes, and practices toward job satisfaction in a general population in a large metropolitan area. The data acquired from 1043 questionnaires—administered to subjects with an average age of 35.24 years—revealed that only 30% is satisfied by his job. Moreover, among all the tested sample, 12% receive, or often receive intimidation by their superior, and 23% wake up unhappy to go to work. Marital status and having children seem to be an important factor that negatively influences job satisfaction through worst behaviours. The multiple linear regression analysis shows how knowledge is negatively correlated to practices; although this correlation is not present in a simple linear regression showing a mediation role of attitudes in forming practices. On the contrary, attitudes, correlated both to knowledge and practices, greatly affect perceived satisfaction, leading us to target our proposed intervention toward mindfulness and to improve welfare regulation towards couples with children.
1. Introduction
Job satisfaction has been defined as a “pleasurable or positive emotional state, resulting from the appraisal of one’s job experiences” [ 1 ]. Job satisfaction reflects on overall life quality involving social relationships, family connection and perceived health status, affecting job performances, work absenteeism and job turnover, leading, in some cases, to serious psychological condition such as burnout [ 2 , 3 , 4 , 5 , 6 ].
The recent Gallup statistics on job satisfaction indicated that a very large portion of the world’s 1 billion full-time workers is disengaged, more precisely, only 15% of workers are happy and production in the workplace, the remaining 47% of workers are “not engaged,” psychologically unattached to their work and company [ 7 ]. In the EU, approximately one in five residents (16.9%) currently in employment expressed low levels of satisfaction with their job, on the other hand approximately one in four (24.6%) expressed high levels of satisfaction, the remaining residents (58.5%) declared medium levels of satisfaction with their job [ 8 ]. Characteristics such as age, sex, education, occupation, commuting time and difficulty as inadequate income, seems to be related to job satisfaction as they tent to influence expectation and preferences of individuals’ reflection on their perceived working condition [ 9 , 10 ]; however, as assessed in Eurofound, European Working Conditions Surveys [ 11 ] the relation between age and job satisfaction is very weak, although a slight increase in low satisfaction prevalence was found in elder population, it does not increase significantly with age even though expectations change during lifetime; educational attainment and income seem to play a significant role in job satisfaction as they grow in parallel, leading to better positions and a higher wages, along with power and more decisional autonomy. Sex is a factor as women seems to be overall more satisfied by their job in despite of the worst general conditions [ 11 , 12 , 13 , 14 ]. Job satisfaction also relates to marital status as single subjects’ results as the most satisfied by their work in some European Countries [ 15 ]. In Italy, the overall perceived job satisfaction seems to be similar to other regions in EU, and social relations as well as family composition appear to play a relevant role [ 16 ].
Job satisfaction has been studied mostly over a specific category of workers [ 17 , 18 ], as some types of works seems to be more related to pathological conditions such as burnout [ 19 , 20 ] and job-related stress [ 21 , 22 , 23 ]; however, as reported by those authors, this kind of selection method could lead to selection biases. According to van Saane [ 24 ], although many studies were carried as since Job Satisfaction broke out in the last 70’s as a central topic of interest, nor a mathematical instrument as reliable as desired nor a comparative method were found, usually those studies were based on single components of job satisfaction, taken out from extra working environment, and without analysing the consequences on behaviours in day life [ 25 , 26 , 27 ]. The literature research demonstrated that practices are the results of knowledge, attitudes, or their interaction. The KAP Survey Questionnaire [ 28 ] can be applied to highlight the main features of knowledge, attitude, and practice of a person, and to assess that person’s views on the matter. The purpose, when using the KAP Survey Model, is to measure a phenomenon through the quantitative collection method of a large amount of data through the administration of questionnaires and then statistically process the information obtained. Through a questionnaire, however, seems to be easier to quantify job satisfaction. In addition to that, studying broader populations’ consent to explore different components, both personal and environmental, which concur to influence it [ 29 , 30 ].
In the recent literature, a KAP model was used only once to analyse behaviours toward job satisfaction. In his work, Alavi [ 31 ] conducted a survey based cross-sectional study on 530 Iranian radiation workers; although it comprehends simultaneously knowledge, attitude, and practices, it was conducted on a specific category of workers and on a narrower population. Therefore, since to the best of our knowledge none of the studies presented in the literature are carried out on a broader population relating both knowledge and attitudes to behaviours on job satisfaction, the aim of this study is to analyse simultaneously knowledge, attitudes, and behaviours toward job satisfaction in a large metropolitan area. It is important to investigate this phenomenon to evaluate the condition and develop health education programs and community-based intervention to increase job satisfaction and knowledge and positively orienting attitudes.
2. Material and Methods
2.1. participants and procedure.
This cross-sectional study was conducted from November 2021 to February 2022 in the large metropolitan area of Naples, southern Italy, among working places, universities, and community centres. No specific category of participants was selected. In the questionnaire, respondents indicated their occupation by choosing from the following options: lawyer, architect, engineer, doctor, accountant, entrepreneur, teacher, law enforcement, trader, student, employee, worker, unemployed, other. Table 1 shows the categories indicated by the participants. The criteria for inclusion in the study required that respondents of a general population were over 18 years old, belonging to one of the categories of employment listed in Table 1 , and resided in the metropolitan area of Naples. Every participant directly received a questionnaire (available upon request from the corresponding author) and at the time of filling out the questionnaire, the aim of the study and the anonymity and privacy of the data collecting method being used was explained, both in written form, as an introduction part of the questionnaire, and verbally to each of the participants. The questionnaire consisted of basic information about participants (age, gender, children, civil state, education level, profession, smoke habits) and three pools of questions divided in knowledge, attitudes and behaviours concerning their job satisfaction for a total number of 37 questions. The construction of the questionnaire was carried out as recommended by the KAP Model [ 28 ], briefly was divided into four phases: (1) Constructing the survey protocol; (2) Preparing the survey; (3) Course of the KAP survey in field; (4) Data analysis and presentation of the survey report. To develop the questionnaire, research questions based on the “Objectives of the study” were first carried out to develop the research questions, according to KAP Survey Model [ 28 ], the knowledge was considered as a set of understandings, knowledge, and “science” while Attitude as a way of being, a position. After, the research questions were reduced in number by removing those questions that require unnecessary information. When the above step is also done, the difficult questions have been changed/removed (closed questions have been used because one of the most important things that will increase the relevance of the questions is that the questions must be closed questions). Knowledge and attitudes were assessed on a three-point Likert scale with options for “agree”, “uncertain”, and “disagree”, while inquiries regarding behaviours were in a four-answer format of “never”, “sometimes”, “often”, and “yes/always”. A pilot study was also carried out to test the questionnaire and to verify the reliability of questions. Finally, all the collected questionnaires were digitalized submitting the codified answers in an Excel worksheet (MS Office).
Study population characteristics.
2.2. Statistical Analysis
Data reported by the study were analysed using IBM SPSS (vers. 27) statistical software program. The analysis was carried out in two stages. In the first stage, a descriptive statistic was used to summarize the basic information of the statistical units. In the second stage, a Multiple Linear Regression Analysis (MLRA) was used to model the linear relationship between the independent variables and dependent variable.
The dependent variables (Knowledge, Attitudes and Behaviours) had been obtained by adding the scores obtained in the corresponding questions (questions with inverse answers have been coded inversely). The independent variables were included in all models: sex (1 = male, 2 = female); age, in years; education level (1 = primary school, 2 = middle school, 3 = high school, 4 = university degree); civil state (1 = Single; 2 = In a relationship; 3 = Married; 4 = Separated/Divorced; 5 = Widowed).
The main results from a MLRA contains the statistical significance of the regression model as well as the estimation and the statistical significance of the beta coefficients ( p -value < 0.05) and the coefficient of determination (R-squared and adjusted R-squared), used to measure how much of the variation in outcome can be explained by the variation in the independent variables. Three MLRA were developed:
- (1) Knowledge about job satisfaction (Model 1);
- (2) Attitudes toward resilience and mindfulness (Model 2);
- (3) Actual behaviours regarding Job and Job-related life (Model 3).
In Model 2, we added Knowledge to the independent variables, and in Model 3, we added Knowledge and Attitudes to the independent variables. In the analysis, we considered Attitudes and Knowledge as indexes rather than a scale, which means that each observed variable (A1, …, A13 and K1, …, K12) is assumed to cause the latent variables associated (Attitude and Knowledge). In other terms, the relationship between observed variables and latent variables is formative. Therefore, inter-observed variables correlations are not required. On the contrary, the relationship between the observed variables (B1, …, B14) and latent variable Behaviour could be considered reflective (Cronbach’s alpha = 0.825). All statistical tests were two-tailed, and the results were statistically significant if the p -values were less than or equal to 0.05.
3. Results and Discussion
Out of the 1057 participants, 1043 anonymous self-report surveys were returned, resulting in a response rate of 98.7%. Table 1 shows the characteristics of the study population: the mean age of the study population is 35.24 years; in 18–70 age range, the main group of distribution was 18–30 representing 44.6% of the sample; sex distribution shows that: 427 are men, 616 are woman. A large majority (73.5%) does not have children, while 26.5% of the sample has them. Most of the participants have a post graduate degree, while 29.1% are high school graduates. Among them, 22.2% are physicians, 15.1% teachers and 14.0% students ( Table 1 ).
Respondent’s knowledge about job satisfaction is presented in Table 2 . While a large majority of the sample population (91.7%) has a well-defined knowledge about job satisfaction main characteristics such as mains definitions, both of work-related stress and mobbing, most of them does not know or are not aware which risks are specifically related as only 31.4% knows that job related stress and mobbing are a threat to their cardiovascular health. Only 28.7% of the population knows that “Only 15% of worker, globally, are satisfied by their work” demonstrating that while knowledge regarding job related stress is well spread, the sample does not know how diffused it is and what kind of risks it involves, and that state provide a compensation for job related stress.
Knowledge of respondents toward job satisfaction.
* INAIL: Istituto Nazionale Assicurazione Infortuni sul Lavoro (National Institute for Occupational Accident Insurance).
In Table 3 are described attitudes toward job satisfaction. Most of the participants think that working out is relaxing and spending time is regenerating, showing a good attitude to copy with work related stress. According to 93.4% of the sample, workload plays a key role in job satisfaction, as well as adequate wages and a clear task schedule. Several studies have enlightened that when workers lack a clear definition of the tasks which are necessary to fulfil a specific role, their levels of job satisfaction are likely to be negatively affected [ 32 , 33 , 34 ]. Interestingly, most of the population sees challenges as a motivation to do better (80.2%) and are motivated by career opportunities (90.7%); however, 50.5% of the population has a negative attitude about changes. In confirmation of that, when asked if “Changes lead to stress”, only a small fraction of the sample (14.6%) disagreed. This allowed us to assume that, although most of the population sees problems as an opportunity to learn, improve and progress in their work, they are aware of the difficulties connected to changing scenarios. About 27.2% of the sample does not have a positive attitude toward sharing their feeling about problems at work talking out loud. Bad interpersonal relationships with co-workers are another reason for job dissatisfaction. Poor or unsupportive relationships and conflicts with colleagues and/or supervisors lead to negative psychological intensions, resulting in job dissatisfaction [ 35 , 36 ].
Attitude of respondents toward job satisfaction.
Behaviours of respondents are listed in Table 4 : A consistent part of the sample responded positively to the group of question toward behaviours regarding their coping level of stressful situation (B2, B4, B8, B9, B10) showing a reported good resilience. Commuting seems to be a problem for at least a third of the sample, also in a metropolitan area served by 2 subways, full bus service, car sharing services and a speedway. Job satisfaction is associated negatively with constraints such as commuting time. This dead time, mostly unpaid, is mandatory for workers to reach workplace. Although this is not considered as working time, and only a specific class is refunded, from the employers’ perspective, it is time dedicated to work and a strong determinant for low satisfaction levels. EU workers were much more likely to be highly (37.9%) or moderately satisfied (41.7%) with their commuting time compared to their job satisfaction. Most of the sample responded to not having experienced mobbing; although even a “low” result, such as a cumulative, summing both “yes/always” and “often”, of 11.8% is alarming and pushes us to study more about this phenomenon. Interestingly, 30.9% of respondents are satisfied about their work, reaching a total of 59.5%. In addition, with a “often” response showing a large appreciation of their jobs, 22.9% of the respondents “wake up unhappy to go to work”, and feel “stuck in a job with no career opportunities” (27.7%). The sample has no problems managing their work and social life (48.3%); however, only a complex of 35% of the sample usually spend their time with colleagues outside the office.
Behaviour of respondents toward job satisfaction.
Table 5 illustrates results of linear multiple regression in three models: in Model I Knowledge, as dependent variable, correlate, with a p -value < 0.001; with “sex”, interestingly, woman seem to have a higher overall score of knowledge in disagreement with Gulavani [ 37 ] whose study was conducted among a sample of nurses and found no significant relation between sex and knowledge on job satisfaction. Al-Haroon [ 38 ] evidenced that among health workers, men had a better overall level of knowledge. These results, however, were collected over specific categories of employees, in a narrower sample; whereas our study was represented by a general population of a metropolitan area. No statistically significant correlation between knowledge and age, civil status, children, and education levels was encountered.
Results of the linear multiple regression.
Previous research asses that attitude plays a key a role in job satisfaction, as some attitudinal characteristics of the subject influence perspective, coping skills and stressful situation management [ 39 , 40 , 41 ]. In Model II ( Table 5 ) we correlated, through MLRA, attitudes with age, sex, civil state, having children, education, and overall knowledge score. With a p -value < 0.001, two correlations were found with education and overall knowledge score, both positively. Those results reflect, in accordance with Alavi [ 31 ], who found that higher level of education was among 3 factors that predicted job satisfaction and attaining a higher university degree compared to lower degrees contributes to a feeling of coherence, success at work, personal growth and self-respect, self-realization and intrinsic motivation, that education level and therefore a higher level of knowledge contributes to generating a sense of job satisfaction. In the questionnaire we tried to collect all those propension and as a result: in agreement with Hermanwan [ 42 ], Andrews [ 43 ] and Choi [ 44 ], subjects with better knowledge and high levels of education tent to have better attitudes.
In Model III, behaviours taken as a dependent variable are correlated to age, sex, civil state, children, education, knowledge, and attitudes. The results of linear multiple regression in this model assess that behaviours are negatively correlated to civil state, sons, and knowledge, and positively correlated to attitudes. Our findings show that there is a positive correlation between behaviours and attitudes, in agreement with previous literature [ 45 , 46 , 47 ], demonstrating that people with better attitudes tent to have a better overall behaviour. Surprisingly, in Model III, knowledge also has a statistically significant correlation to behaviours but in a negative way. This correlation, however, is not present when we correlate those variables alone in a Pearson’s correlation between knowledge, attitudes, and behaviours ( Table 6 ). This evidence, therefore, suggests that attitude mediates the effect of knowledge on behaviours, assessing an important relation between those two determinants. People with a better overall score in behaviours tend to have a higher score in knowledge and attitude. In this sample, those who have a lower score in knowledge also has a higher behaviour score in accordance with a part of the previous literature [ 48 , 49 ]. This enlightens the importance of high levels of knowledge in order to form better attitudes in the pursuit of job satisfaction. Civil state and having children seem to play a key role in performing a better behaviour about job satisfaction; which is also evident in one specific question about behaviour: Question “B14” enlightens the social practices of subjects with colleagues outside the work environment, and the statistical analysis on this topic shows that subject with a more stable sentimental situation or with child tend to hang out with their colleagues less, likely worsening their relationships at work and getting a worse overall behaviour score and worse attitude toward the topic in agreement with Sousa-Poza [ 50 ] and Armstrong [ 51 ]. Job satisfaction has a strong correlation to family characteristics: Subjectst who have families with children have less positive behaviours towards their job satisfaction, directly affecting their overall behaviour score; this evidence is in contrast with Alavi [ 31 ], who states that job satisfaction is positively affected by family, assessing that “married employees have opportunities to receive support or advice from their family to mediate job conflicts,” Although he admits that in the literature, this result is controversial as some authors, such as Clark [ 52 ], found that “married employees experienced a higher level of job satisfaction than their unmarried co-workers”, and Booth and Van Ours’ [ 53 ], study did not find a statistically relevant correlation with the presence of children. Those results, therefore, suggest creating targeted educational programs, community-based intervention, and legal regulation, to improve self-awareness and resilience among workers, and a more practical intervention could be directed to families with child.
Pearson’s correlation between knowledge, attitudes, and behaviours.
4. Conclusions
This study shows that the metropolitan population has general good knowledge about job satisfaction as well as a positive attitude. Job satisfaction, however, is reflected accordingly only with attitudes. While it has a negative relation to civil state and having children, this means that the experimental results of this study may be used to create targeted educational programs, community-based intervention, and legal regulation, to improve self-awareness and resilience among workers. A more direct intervention could be directed to families with children. Social networking with colleagues has an important impact on job satisfaction, as the part of the sample who responded positively to the specific question, had an overall better behaviour. Although, in this case, having children seems to be, as they negative correlate, a huge limitation to this practice. Considering that, as previously stated, the impact of job satisfaction on the population has a strong impact in terms of life balance, health, and economics, and it is well known that only a small fraction of workers are fully satisfied. It might be important to promote welfare regulation to allow a larger part of the population to conciliate work and family. Results of this paper could be an indicator of how to establish an educational program more efficiently. It is mandatory to strengthen specific knowledge about job satisfaction through the general population toward the importance of job satisfaction and the benefits related to a correct approach to work-life. The impact of a public health intervention could be even more effective by integrating another program to orient and define attitudes, which in turn will influence people to practice a mindfulness mental setting toward job satisfaction. In conclusion, a training program based on fundamental practices of job satisfaction should be improved in the young population, in early stage of family life, or before they have children, in order to achieve a double objective: “training family and spreading the practice to a future generation”.
Funding Statement
This research received no external funding.
Author Contributions
Data curation: P.M., M.S., P.S., F.D.D., A.N. (Alfonso Nardo), B.F., D.D., S.D.S., F.P., A.M. and M.T.; Formal analysis: M.S., F.D.D., A.N. (Alfonso Nardo), B.F., D.D., S.D.S. and F.P.; Resources: P.M. and M.T.; Software: P.S.; Supervision, P.M., M.T. and A.N. (Antonio Nardone); Writing—original draft: M.S., F.D.D., A.N. (Alfonso Nardo), B.F., D.D. and S.D.S.; Writing—review and editing: P.M., M.S., P.S., F.P., A.M., M.T. and A.N. (Antonio Nardone). All authors have read and agreed to the published version of the manuscript.
Institutional Review Board Statement
Not applicable.
Informed Consent Statement
Data availability statement, conflicts of interest.
The authors declare no conflict of interest.
Publisher’s Note: MDPI stays neutral with regard to jurisdictional claims in published maps and institutional affiliations.
Job Satisfaction and the ‘Great Resignation’: An Exploratory Machine Learning Analysis
- Original Research
- Open access
- Published: 30 October 2023
- Volume 170 , pages 1097–1118, ( 2023 )
Cite this article
You have full access to this open access article
- Mehmet Güney Celbiş ORCID: orcid.org/0000-0002-2790-6035 1 , 2 ,
- Pui-Hang Wong ORCID: orcid.org/0000-0001-6818-983X 2 , 3 ,
- Karima Kourtit ORCID: orcid.org/0000-0002-7171-994X 4 , 5 &
- Peter Nijkamp ORCID: orcid.org/0000-0002-4068-8132 4 , 5
2249 Accesses
2 Citations
Explore all metrics
Labor market dynamics is shaped by various social, psychological and economic drivers. Studies have suggested that job quit and labor market turnover are associated with job satisfaction. This study examines the determinants of job satisfaction using a large survey dataset, namely the LISS Work and Schooling module on an extensive sample of persons from the Netherlands. To handle these big data, machine learning models based on binary recursive partitioning algorithms are employed. Particularly, sequential and randomized tree-based techniques are used for prediction and clustering purposes. In order to interpret the results, the study calculates the sizes and directions of the effects of model features using computations based on the concept of Shapley value in cooperative game theory. The findings suggest that satisfaction with the social atmosphere among colleagues, wage satisfaction, and feeling of being appreciated are major determinants of job satisfaction.
Similar content being viewed by others
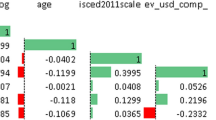
Exploring the Influences of Job Satisfaction for Europeans Aged 50 + from Ex-communist vs. Non-communist Countries
Daniel Homocianu, Octavian Dospinescu & Napoleon-Alexandru Sireteanu
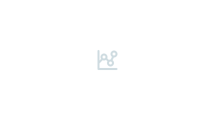
A Longitudinal Analysis of Job Satisfaction During a Recession in the Netherlands
Patrick Pilipiec, Wim Groot & Milena Pavlova
Analysis of Big Five Personality Factors to Determine the Appropriate Type of Career Using the C4.5 Algorithm
Avoid common mistakes on your manuscript.
1 Introduction
Though the global health crisis has ended, its economic impacts have only started to ripple over the global labor market. In the United States, about four million workers voluntarily quit their jobs in April 2021 (Reuters, 2019 ). This so-called Great Resignation is observed in other advanced economies as well. In the Netherlands, it is reported that nearly one out of five people have switched their jobs in 2022 (Algemeen Dagblad, 2023 ). A macroeconomic explanation maintains that labor shortages in some sectors lead workers to believe that they may find a better offer to compensate the drops in real wage under a tight labor market, which may explain the high turnovers (Duval et al., 2022 ). While this explanation has some truth in it, research on organizational behaviors may argue that the phenomenon can be driven by low job satisfaction. Unhappy workers are more likely to quit their jobs (Green, 2010 ), especially during and after the pandemic period. For instance, Martin et al. ( 2022 ) found that remote working has increased job stress and reduced job satisfaction in Luxembourg. Demirkaya et al. ( 2022 ) reported that the feeling of entrapment is significantly correlated with job quits in Turkey.
Departing from the job satisfaction perspective, this article evaluates an array of individual-level factors that influence job satisfaction of Dutch workers using newly available Dutch household survey data. This study does not seek to explain the occurrence of Great Resignation. It highlights that job satisfaction has escaped from the discussion. Based on the multifaceted approach of job attitudes (Judge & Kammeyer-Mueller, 2012 ), an economic explanation would suggest that satisfaction of pay and of work conditions are major drivers of the behaviors of workers. In this study we examine to what extent economic concerns predict overall job satisfaction during 2022 using a novel machine-learning approach. Findings from this study should help us to assess which facets of job satisfaction are associated with the ‘Great Resignation’ in the Dutch labor market.
2 Job Satisfaction and the Utility Approach
Job satisfaction is commonly referred to as employees' affect and attitude toward their job. Emphasized on the affective dimension, Locke ( 1976 ) defined the concept as “a pleasurable or positive emotional state resulting from the appraisal of one's job or job experiences” (p. 1300). Focusing on the cognitive aspect of job satisfaction, Weiss ( 2002 ) defined the concept as “a positive (or negative) evaluative judgment one makes about one's job or job situation” (p. 6). The affective view sees affect at work as an indicator of job satisfaction, while the cognitive, evaluative approach considers affective experiences on the job as a source of job satisfaction.
In economics, job satisfaction is frequently treated as a unidimensional variable and a function of wage (Borjas, 1979 ; Hamermesh, 1977 ). Footnote 1 An implication of this formulation is that, when members of certain social groups (e.g., women) are discriminated in the labor markets, they would experience a lower level of job satisfaction because of a lower wage or other non-wage benefits (Bartel, 1981 ). Interestingly, while a gender wage gap is found to exist (Blau and Kahn, 2017 ), studies have shown that women usually report a higher level of job satisfaction than men, a stylized fact that contradicts what the theory predicts (Blanchflower & Oswald, 1999 ; Clark, 1997 ; Sloane & Ward, 2001 ). However, when factors such as age and job expectations are controlled for, the gap in job satisfaction between the two genders diminishes, suggesting that job satisfaction can depend on some often-overlooked demographic factors.
The utility function approach also implies that satisfaction is, at least partly, driven by extrinsic incentives. This early conceptualization is based on the utility theory that labor supply is a rational choice after a careful deliberation to the trade-off between leisure and consumption backed by the paycheck. Although studies have found that salary is an important determinant of job satisfaction, many studies have documented that workers do value a wide range of nonpecuniary characteristics of a job, which include job security, autonomy, shorter and more flexible work hour (Berger et al., 2019 ; Clark, 2001 ; Lepinteur, 2019 ; Origo & Pagani, 2009 ). A study by Lange ( 2012 ) further showed that these job characteristics could be even more influential than individual-specific factors like personality traits and values.
If people are happy with their jobs, they should be less likely to switch jobs. Some studies use quit data to analyze impacts of pecuniary and non-pecuniary factors on job satisfaction. Clark ( 2001 ) not only showed that job satisfaction is a powerful predictor of job change, it also found that job security and pay are the most important determinants of quit. Although not been emphasized in the literature, analyses of quit data may suggest that factors contributing to satisfaction and dissatisfaction may not be identical and their impacts could also be asymmetric. These asymmetric effects, however, have not been highlighted and emphasized in the existing literature.
One major conclusion from the job satisfaction literature is that happy workers are more productive (Oswald et al., 2015 ). But what beneath is the belief that a well-designed reward system should improve workers productivity. The starting point of personnel economics is the principal-agent conceptualization (Laffont & Martimort, 2002 ) that workers may shirk (i.e., moral hazard) and productive workers are costly to recruit (i.e., adverse selection). Clever economic mechanisms are required to identify these workers ex ante and to induce their efforts ex post. From this perspective, many of the human resource management practices such as performance pay, promotion, and job autonomy can be seen as performance optimizing mechanisms. Although financial and non-pecuniary rewards are parts of the job satisfaction equation (Cassar & Meier, 2018 ; Cornelissen et al., 2011 ; Ellingsen & Johannesson, 2007 ; Gosnell et al., 2020 ; Jones et al., 2009 ), from this cynical view, job satisfaction is nothing more than a happy by-product of human resources policies or a means to productivity and performance.
3 Individual and Social Dimensions of Job Satisfaction
An underlying assumption behind the utility approach is that income induces satisfaction and/or happiness. Although studies have found a significant but weak relationship between the two variables, behavioral studies have challenged this fundamental assumption (Clark et al., 2005 ; Easterlin, 1995 ). While some studies suggest that the relationship may be causal (Powdthavee, 2010 ), three decades of economics of happiness research have contested this finding (Clark et al., 2008 ). One major conclusion from the literature is that subjective well-being does not always increase with income. When examined the dynamic relationship between income and subjective well-being, Easterlin ( 2001 ) argued that income increases happiness initially. But aspirations grow as one climbs the income ladder. Over a life cycle, people's level of happiness remains stable and does not increase along with salary. Increase in income has only a short-term effect on happiness. The same mechanism may explain why job satisfaction may not catch up with income.
Another explanation to the weak statistical correlation between money and satisfaction over time is related to social comparisons. Taking a geographical approach, Luttmer ( 2005 ) found that people who earn an income lower than the local average feel worse off. A large amount of economics research in job satisfaction has been testing this hypothesis. Using data on British workers, Clark and Oswald ( 1996 ) found a similar relationship between job satisfaction and expected income. In an experimental study, Card et al. ( 2012 ) showed that the knowledge about their earnings below the median income of their peers significantly reduces job satisfaction and increases job search intentions. People form expectations as well as inspirations based on their peers, work conditions, and wage history (Diriwaechter & Shvartsman, 2018 ; McBride, 2001 ; Poggi, 2010 ). When the expectation-aspiration spiral is kick-started, an increase in aspirations can negatively affect people's satisfaction levels (Mcbride, 2010 ). What remains unclear is whether job dissatisfaction is due to pure social comparisons or fairness concerns (Card et al., 2012 ; d’Ambrosio et al., 2018 ; Smith, 2015 ). Interestingly, in a study using matched employer–employee panel data in Denmark, Clark et al. ( 2009 ) found that job satisfaction is positively correlated with their co-workers', a finding that counters many of the existing research in this area. They interpret that this relationship can be related to people's expectations about their future earnings. A higher average salary level leads the thinking that their potential wage may increase soon. All in all, while studies have shown that expectations and aspiration matter, it remains unclear how they are formed based on social comparisons and wage profiles.
One major assumption behind the neoclassical formulation of the utility function is that people gain utility mainly from consumption but not from the job per se. While the personnel economics literature covers aspects such as reward systems from a well-grounded, humanized (i.e., incentive-based) perspective, it is not unrealistic to think that under certain conditions, work can lead to a sense of achievement, which in turns shapes aspirations and hence job satisfaction (Genicot & Ray, 2020 ). Another stylized fact in the literature is that entrepreneurs and the self-employed are found to have a higher level of job satisfaction (Lange, 2012 ; Millán et al., 2013 ). The risk-adjusted returns and job security of entrepreneurship is known to be low. The existence of intrinsic motivations, however, offers a plausible explanation to the surprising, stylized fact (Carree & Verheul, 2012 ). In a laboratory setting, Ariely et al. ( 2008 ) and Chandler and Kapelner ( 2013 ) manipulated the perceived meaningfulness of a task and found that meaningfulness influences effort and labor supply behaviors. This lends support to the idea that the meaning of work could be part of the utility function in its own right (Cassar & Meier, 2018 ). Drakopoulos and Theodossiou ( 1997 ) considered a hierarchical utility function, in which increase in earnings, up to a certain point, ceases to induce utility, and the marginal utility of other work-related variables becomes much higher thereafter. Although the proposed modification does not directly speak to the fulfillment mechanism, the modification formally speaks to human's intrinsic motivations and the feeling of satisfaction.
If intrinsic motivations involve meaning making, a job that is connected to a person's educational background, skill sets, and competency should be more fulfilling. In fact, Nikolova and Cnossen ( 2020 ) showed that intrinsic motivations, measured by perceived job autonomy and competence, matter even more than extrinsic rewards. Feeling competent is pleasant and induces satisfaction (Loewenstein, 1999 ). Nevertheless, some studies have shown that the competence concern may backfire. García-Mainar and Montuenga-Gómez ( 2020 ) found that, in terms of education, overqualified workers tend to dissatisfy with their jobs. The same applies to horizontal educational mismatch—when graduates are employed in an occupation unrelated to their fields of study (Levels et al., 2014 )—and skill mismatches (Vieira, 2005 ). However, there is only little evidence on the effects of skill obsolescence and gaps on job satisfaction (McGuinness et al., 2018 ).
One fundamental criticism on the study of job satisfaction is that many findings from these empirical studies may not be easily fed into neoclassical economic theories. To some economists, if job satisfaction is nothing more than yet another term in a utility function, it can only be assumed but not explained. Accordingly, this leads to a proposal that, because job satisfaction is unobserved and is related to some volatile, external factors like economic fluctuations and labor market policies (Pilipiec et al., 2020 , 2021 ; Ravid et al., 2017 ), and is unstable even within-individual (Bryson & MacKerron, 2017 ), instead of fixating on job satisfaction and treating it as a dependent variable, a more fruitful approach is to treat it as an explanatory variable to study worker behaviors such as quits and labor market functioning like employee turnover (Hamermesh, 2004 ).
Although the general working environment and coworker relationship has been an important dimension of job satisfaction in organizational psychology (Jolly et al., 2021 ; Kinicki et al., 2002 ; Smith et al., 1969 ), the variable has received relatively little attention in economics literature, probably due to its difficultly in incorporating into the existing theoretical framework. The same also seems to hold in organizational studies (Judge & Kammeyer-Mueller, 2012 ). Cassar and Meier ( 2018 ) discussed feelings of relatedness in the context of meaning of work and productivity, but they did not articulate how the concept can be related to job satisfaction. They also mentioned social comparison and fairness, which was discussed above, but they clearly did not relate it to coworker relationship. Intuitively it is easy to understand why collegial relationship may influence job satisfaction. Karlsson et al. ( 2004 ) explained that social extensions such as a family and work ameliorate feelings of inconsequentiality, and people could find meaning in one's life. But it is not obvious how it can be related to the meaning of a job (Nikolova & Cnossen, 2020 ). One possibility is about the pursuit of common (organizational) goals in a team setting. On a material level, cooperation makes success more likely and helps to achieve higher output or goals which cannot be completed alone. On the social level, contributing to a common goal can create a warm-glow effect when individuals consider working with or “helping” their colleagues as altruistic acts and gain utility from that (Andreoni, 1990 ). From this perspective, a friendly work environment can be considered as a public good. Even for pure egoists, they can gain utility from contributing to the building of a constructive work environment and creating positive spillovers simultaneously. In this regard, an affable work environment can be considered as a by-product rather than a source of job satisfaction.
In our study, the relative importance of different facets of job satisfaction will be tested using a predictive, machine learning approach. Many psychometric tests have been developed to assist in clinical diagnoses. Thus, a predictive approach is well-established in psychology. In fact, predictive validity is a core property of psychometric measures (Mulder et al., 2014 ). Linearity is commonly assumed in traditional statistical measures and tools such as correlation coefficients and structural equation modeling when linear algebra is used. However, higher dimension interactions and linearity are plagued in many relations. The machine learning approach employed in this study is able to capture nonlinearity which is not easily modeled using traditional regression methods (James et al., 2013 ). Additionally, as argued above, there are two major limitations in traditional studies using regression analysis: (1) a variable that influences job satisfaction necessarily affects dissatisfaction, and (2) the effects of a variable on job satisfaction are symmetric. An analytical advantage of the machine learning approach is its ability to reveal potential asymmetry between variables in a relationship. The notion of nonlinearity would become clearer when it is discussed in the result section.
Data in our analysis were drawn from the Work and Schooling module of the Dutch Longitudinal Internet studies for the Social Sciences (LISS; Streefkerk & Centerdata, 2022 ). The LISS panel is administered by Centerdata research institute based in Tilburg University. Based on the population register of the national statistical office of the Netherlands, a random, nationally representative sample was drawn. In this study the fifteenth wave of the LISS survey was used, which is the most recent one after the pandemic. The online survey was conducted between the 4th of April 2022 and the 31st of May 2022. The cross-sectional dataset that was used consists of 420 variables with 5775 responses.
One advantage of the machine learning approach is its ability to include in an analysis a large number of variables, an approach which is seldom adopted in a typical regression analysis due to the concern of multicollinearity. Instead of pre-selecting variables, which can involve personal bias, the machine learning method takes a data-driven approach and includes as many of context relevant variables as possible. The method, however, involves a trade-off: the inclusion of additional variables usually reduces the sample size because of the missing value problem. Therefore, following Celbiş et al. ( 2023 ), an algorithmic process is implemented in order to optimize the number of observations. In each iteration, a simple regression tree analysis is conducted, and the root mean squared error (RMSE) is recorded. In the next step, the algorithm searches for the variable that, if excluded, would cause the greatest reduction in the number of observations. Then the variable is dropped from the dataset and the process is repeated. The data matrix that keeps most of the observations and has smaller impact on accuracy is used for the final analysis. Figure 6 (in the Appendix ), visualizes the observation-feature trade-off of the procedure. In each iteration, represented by the x-axis, either one or more variables are dropped. Identifying the variable(s) to be dropped in each iteration is done through generating an UpSet plot developed by Lex et al. ( 2014 ). Footnote 2 In Fig. 7 (in the Appendix ) we present a sample UpSet plot built in the 4th iteration as an example. According to this UpSet plot, the intersection of the features cw22o582, cw22o583, cw22o584, cw22o585, and cw22o586 account for the largest loss in observation as shown by the first vertical bar, suggesting that these features may represent connected or follow-up questions in the survey which are usually entered as missing in conjunction. Within the iteration, a regression tree is fitted into the subset of the training data which omits the above specified variables and its RMSE is noted. In the next iteration, the highest horizontal bar will correspond to the variable cw22o510, as the five features with higher bars below it will have already been dropped. In this new iteration, cw22o510 would account for the largest decrease in observations by itself unlike the earlier dropped group of variables. Therefore, the iteration will only drop cw22o510. Footnote 3 A new regression tree then is fit into this new subset of the training data and a new RMSE value will be computed. The recursive steps continue until the features of the dataset are exhausted or until the dataset has no observations left with missing values. In our case, this corresponds to iteration 54 (as shown in Fig. 6 ) where the y-axis represents the percentage of observations (persons) or variables that is left in this iteration. As variables responsible for high missing values are dropped, the percentage of observations retained increases. The purpose of this procedure is to identify the optimum combination of the number of observations and variables that is likely to yield the highest prediction performance in our subsequent main empirical implementations of machine learning models. However, the case at hand suggests that RMSE (not represented by any axis) is not sensitive to this trade-off as suggested by the nearly flat RMSE curve in Fig. 6 . As a result, the choice of the desired observation-variable balance becomes somewhat subjective. We selected the combination which retains a balance in this trade-off such that the difference between the percentage of persons retained and the percentage of variables retained is at a minimum. This corresponds to iteration 30. Prior to implementing the above outlined steps, variables that are completely consisted of missing information, variables with no variation (i.e., same value reported for all persons), administrative variables coded into the questionnaire (e.g., start date of the interview, duration of the interview) were dropped. After extensive data cleaning and validation procedure, which involved the above algorithmic trimming of the dataset, the final dataset consists of 1878 individuals and 89 variables. 30% of this data is randomly selected and set aside as the test dataset. All models are applied on the remaining training data. The results are assessed by evaluating the root mean squared error of the models using the test data. The definitions of the top ten features selected by the model in addition to the dependent variable (job satisfaction) are presented in Table 1 .
5 Empirical Models
The empirical analysis takes on two steps: prediction and interpretation. The prediction step is based on a variation of the Gradient Boosting Machine (GBM) technique by Friedman ( 2001 , 2002 ). GBM is applied using the Extreme Gradient Boosting (XGBoost) algorithm by Chen and Guestrin ( 2016 ). XGBoost extends the usability of GBM by allowing regularization and adding further randomization options. The prediction also relies, to a lesser extent, on the Random Forest (Breiman, 2001 ) technique for clustering. Both XGBoost and Random Forest are collections of weak learners based on the binary recursive partitioning algorithm by Breiman et al. ( 1984 ). Hence, randomized (Random Forest) and sequential and randomized (XGBoost) tree-based ensemble machine learning models are used in this study. The XGBoost algorithm allows for cross validation for regularization and determining the optimum model parameters including the learning rate. We partitioned the training sample into 10 subsamples (i.e., internal validation sets) to decide parameters pertaining to tree complexity (i.e., the maximum tree depth and minimum number of observations in terminal nodes) and the learning rate through cross validation. While regression trees are normally pruned through n-fold cross validation, the random forest model produces unpruned trees. However, while cross validation is absent from the random forest proximity clustering, unbiasedness is achieved through the use of the out-of-bag (OOB) observations. The resulting Shapley Additive Explanations (SHAP) values are derived from the above mentioned cross validated gradient boosting model.
XGBoost and Random Forest present several advantages thanks to their ability to consider all possible interactions and nonlinearities as the algorithms are based on binary recursive partitioning (James et al., 2013 ; Varian, 2014 ). The aggregation of many classification trees with high variance but low bias (due to their unpruned structures), built by taking repeated samples from the training data, can significantly improve prediction accuracy while reducing the variance on the prediction function (Breiman, 1996 , 2001 ; Friedman, 2001 ; Friedman et al., 2001 ; James et al., 2013 ). However, as trees built using the same training dataset are expected to be highly correlated, the benefits of using an ensemble would be limited (Aldrich & Auret, 2013 ; Breiman, 2001 ; Friedman et al., 2001 ). The random forest algorithm aims to cope with this correlation by introducing randomized restrictions on the feature space at each iteration (i.e., it randomly selects input features in each tree) (Breiman, 2001 ; Breiman & Cutler, 2020 ; Friedman et al., 2001 ; James et al., 2013 ). Therefore, in addition to the reduction in variance through aggregation, further reduction is made possible compared to bootstrap aggregation which is an ensemble model with correlated trees (James et al., 2013 ). However, gradient boosting does not decorrelate trees. Instead, each tree is a modified version of the previous one (Friedman, 2001 , 2002 ). Nevertheless, GBM embodies randomization like Random Forest but also introduces regularization which is not present in Random Forest. As a result, a group of weak learners with low variance are chained sequentially and modified with learning steps in between leading to the bias in prediction being lowered gradually in each iteration (Friedman, 2001 , 2002 ; Friedman et al., 2001 ).
A random forest with 500 unregularized regression trees is generated for predicting the individual job satisfaction level for the \(N\) persons in the training data. At each iteration, some individuals are left out of the computation, because the bootstrap aggregation algorithm on which a random forest is based draws random subsamples of 2/3 of the size of the training dataset (Breiman, 2001 ; James et al., 2013 ). Further randomization is applied by selecting a split feature from a random subset of size 1/3 of the feature set at each split (Breiman, 2001 ). A random forest proximity matrix ( \(N\times N\) ) is produced where the proximity score of two persons is increased by 1 each time when they are predicted to fall into the same terminal unpruned regression tree node in a random forest iteration in which they were out-of-bag (i.e., randomly left out). The matrix is divided by 500 (the number of trees) and the additive inverse is computed (Aldrich & Auret, 2013 ; Breiman & Cutler, 2020 ; Friedman, 2001 ).
The exploration of clusters is performed based on the random forest results. Random Forest uses the proximity scores among the observations in the training dataset in order to detect cluster structures (Aldrich & Auret, 2013 ; Cutler et al., 2009 ; Friedman, 2001 ). The distance measures used in conventional clustering techniques such as hierarchical and k -means clustering are prone to be dominated by uninformative features that may cloud the effects of the important model features (Cutler et al., 2012 ). In this regard, the main advantage of the random forest proximity matrix is due to its randomization procedure which aims the aforementioned decorrelation process. In addition, unlike classic clustering approaches, feature selection in random forest proximity plots is based on the underlying model which employs algorithmic selection (Xu & Tian, 2015 ). Furthermore, the random forest proximity plot used for clustering in the present study is generated using OOB observations pair-wise frequencies of sharing a terminal node, which is an internal validation procedure, leading to improvements in out-of-sample performance (Breiman & Cutler, 2020 ; Friedman, 2001 ).
The random forest proximity plots tend to detect and represent one class in one arm of a star shaped visual where pure class regions of out-of-bag observations in the training data are grouped towards the extremities of an arm due to the tree-based structure of the underlying algorithm (Hastie et al., 2009 ; Aldrich & Auret, 2013 ; Cutler et al., 2009 ). In this regard Friedman et al., ( 2001 , p. 595) state that “The idea is that even though the data may be high-dimensional, involving mixed variables, etc., the proximity plot gives an indication of which observations are effectively close together in the eyes of the random forest classifier”.
The interpretation of the machine learning findings is an essential part of any study in social sciences, as predictions alone—regardless of their success—cannot provide clear information and policy implications. The main interpretable machine learning tool employed in this paper is the computation and assessment of Shapley Additive Explanations (SHAP) values (Lundberg & Lee, 2017 ) based on the cooperative game theoretical approach by Shapley ( 1953 ). SHAP values have been introduced recently to the machine learning fields. As opposed to older approaches such as calculating variable importance scores (Lundberg & Lee, 2017 ; Molnar, 2019 ), the SHAP values approach can measure both the sizes and the directions of the relationships. The computation of SHAP values is preformed using the “SHAPforxgboost” module by Liu and Just ( 2020 ). A remarkable advantage of the SHAP value approach is that the calculations of the effect sizes are done by considering many different values and (theoretically all) combinations of model features (Celbiş, 2022 ; Lundberg & Lee, 2017 ; Molnar, 2019 ). Consequently, when computing the effect of a given feature for a given data instance (i.e., individual) all other variables are not held constant as is usually done in traditional econometric approaches. The departure from the ceteris paribus restriction leads to more realistic assessments of effect sizes, as in the real-world other factors can never be held constant in the context of a social science research. Finally, because considering all possible feature combinations and values is computationally not feasible, an approximation formulated by Štrumbelj and Kononenko ( 2013 ) is used in this study.
Among the included variables, both satisfaction with coworker relations and the pay are important features in predicting job satisfaction. The random forest proximity plot shown in Fig. 1 visualizes the clusters based on proximities in prediction between individuals. Footnote 4 The plot suggests the existence of about three clusters based on the roles of model features in explaining job satisfaction.
highly satisfied by colleagues atmosphere (yellow).
moderately satisfied by colleagues atmosphere (green).
poorly satisfied by colleagues atmosphere (dark green).
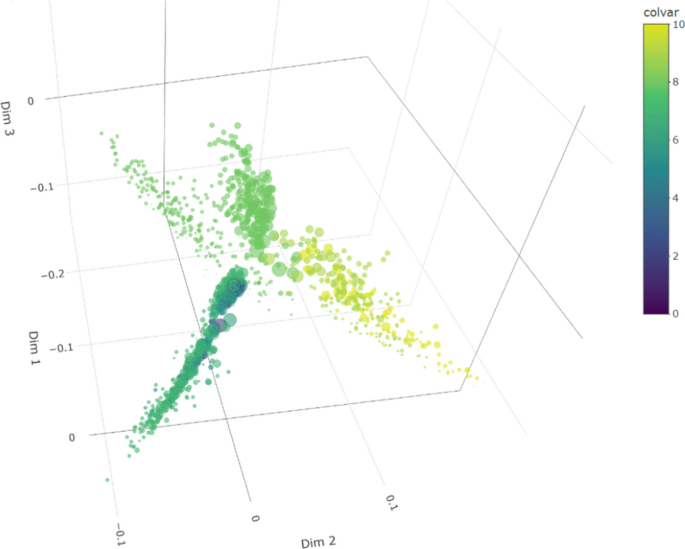
Clusters detected by random forest predictions
In the plot, larger-sized circles indicate individuals with lower wage satisfaction. These individuals are grouped towards the intersection of the “arms”, suggesting that the model has a harder time in distinguishing them (i.e., in iterations in which they were out of sample, people with low wage satisfaction often fell into same terminal nodes when run down the tree). The clear formation of the arms as separate clusters suggests a successful differentiation of the individuals through their inherent similarities. We also observe that people who are poorly satisfied with their jobs are not all part of the same cluster; suggesting that job dissatisfaction may arise from a diverse set of factors.
Regarding the SHAP analysis, a grid search procedure yielded the following optimal parameters for the XGBoost model except for subsample and number of trees, where the former is decided based on the finding by Friedman ( 2002 ) and the latter on computational restrictions:
Learning rate: 0.01
Maximum tree depth: 10
Minimum number of observations in terminal nodes: 1
Subsample ratio in each iteration: 0.5
Feature subsample ratio in each iteration: 0.5
Number of Trees: 10,000
The model is run on the training data, which consists of 70% of the observations randomly sampled from the full dataset. The job satisfaction levels of the individuals in the remaining test data are predicted with a RMSE of 1.14. The SHAP values that are computed based on the XGBoost predictions are visualized in Fig. 2 , where each dot represents an individual. The values show the contribution of each feature value—where features are on the y-axis and higher values are represented with darker colors—on the deviation of a specific individual's predicted value from the mean prediction (the point 0 on the x-axis). The top ten features with the highest SHAP importance values, listed next to the variable name, are presented in the figure. The features that affect job satisfaction the strongest are the first three variables, as the SHAP importance values of the remaining features are all less than 0.1.
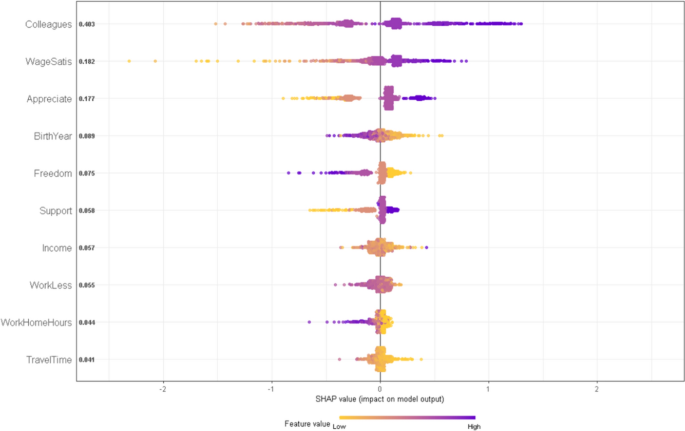
SHAP values
The SHAP analysis summarized in Fig. 2 suggests that a high satisfaction of the atmosphere among colleagues has a positive effect on job satisfaction. Furthermore, this variable has the highest importance in the prediction of job satisfaction. The relationship is shown in more detail in the SHAP dependence plot in Fig. 3 (a slight amount of jitter is used for better representation). A value of 10 for this variable alone can account to up to more than 1 point (in Likert scale) positive deviation from the mean job satisfaction value in the training data.
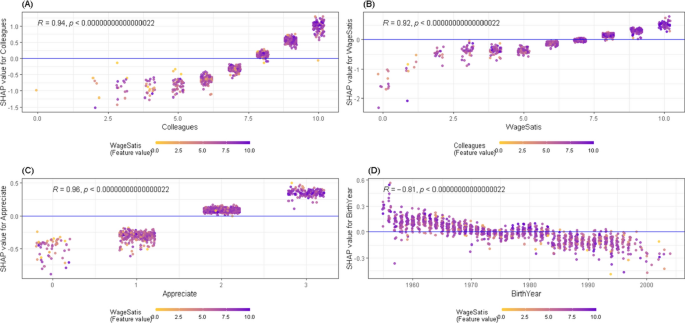
SHAP feature dependence plots
Focusing on wage satisfaction, we observe that high wage satisfaction has a positive effect on job satisfaction. While this is not surprising, it should be highlighted that the importance of wage satisfaction is less than half of that of satisfaction in colleagues. The interaction and dependence between these two top variables are further visualized in the form of a two-way partial dependence plot (PDP) in panel A of Fig. 4 . Unlike the SHAP dependence plots, the PDPs represent joint predictions by holding constant all other features, except one or two features of interest (Friedman, 2001 ). The predictions are recalculated for each value of the features(s) of interest and averaged over the individuals in the training data (Friedman, 2001 ). However, some predictions suggested by the PDPs may be implausible for features that have high correlation with variables that are held constant (Friedman, 2001 ; Molnar, 2019 ). The PDP for Colleagues and Wage Satisfaction suggests that high wage satisfaction, while making a difference, does not predict truly high job satisfaction levels in the absence of high satisfaction in the atmosphere with colleagues. A similar outcome can be deducted from panel B of Fig. 4 which shows that high wage satisfaction without feeling of appreciation does not predict high job satisfaction. We also observe in panel C of Fig. 4 that higher wage satisfaction is associated with high job satisfaction, but the effect is stronger for older individuals. Finally, panel D suggests that high wage satisfaction without work freedom does not predict high job satisfaction.
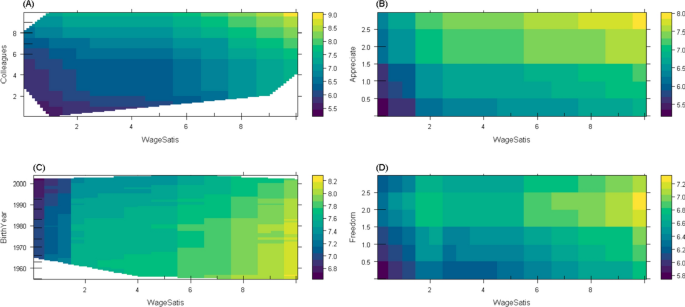
Two-way patrial dependence plots
The feature Appreciate is ranked third in Fig. 2 . This result suggests that the perception that people get the appreciation they deserve for their work has a positive effect on job satisfaction. Feeling unappreciated has a slightly stronger negative effect than the positive effect of feeling appreciated.
The SHAP values of the variable Birth Year are quite symmetrically spread about zero and indicate that younger individuals in the dataset tend to be less satisfied with their jobs. The effect of this variable and the remaining ones are relatively small compared to those of Colleagues , Wage Satisfaction , and Appreciate .
The remaining features in the set of top ten variables with the highest SHAP importance values do not determine job satisfaction to a high extent individually, but collectively they affect the prediction. We briefly summarize the suggestions of their SHAP values. Lack of freedom in organizing one's work has a negative effect on job satisfaction. However, the effect is not symmetric: the positive effect of high freedom is less than the negative effect of lower freedom. Furthermore, the perception of getting enough support in difficult situations has a positive effect. Similar to the freedom variable, the effect of perceived support is also not symmetric.
The income variable is among the top used variables by the algorithm in predicting satisfaction. However, its effect is small and not clear. In elaborating personal economic gain, we instead focus on the wage satisfaction feature.
Preferring to work less hours than current is negatively associated with job satisfaction, but the effect is not very pronounced. Another feature in relation to work hours, Work Home Hours suggest that more hours worked from home has a negative effect on job satisfaction. Again, the effect is not symmetric. Finally, for travel time the effect is weak, but it is evident that long travel duration has a negative impact on job satisfaction.
Further information is given by the SHAP dependence plots. In panels A and B of Fig. 3 we observe that the positive effect of Colleagues and Wage Satisfaction begin after about a score of 7, and that there exist individuals for whom their high satisfaction with their colleagues make a big impact on their job satisfaction despite low wage satisfaction. On the other hand, panel C shows that feeling appreciated has a positive effect that becomes stronger if the person agrees with this opinion stronger. Finally, in panel D we observe that younger persons (born after around 1985) tend to have lower job satisfaction even if they may have high wage satisfaction. The effect is more negative the younger the person is.
Whereas the above discussed partial dependence computations visualize the mean predictions of individual job satisfaction level, individual conditional expectation (ICE) plots (Goldstein et al., 2015 ) visualize the predicted change in everyone’s job satisfaction level by plotting individual curves. Centered ICE plots provide a more explicit presentation by anchoring each individual curve at a given y-intercept value (Goldstein et al., 2015 ; Molnar, 2019 ). The ICE plots shown in Fig. 5 visualize the predicted paths for each individual in the training data where each line represents an individual. The plots for Colleagues , Wage Satisfaction , Appreciate , and Birth Year respectively suggest that the direction of the effects is mostly similar for individuals, although a small amount of heterogeneity in expectations exists.
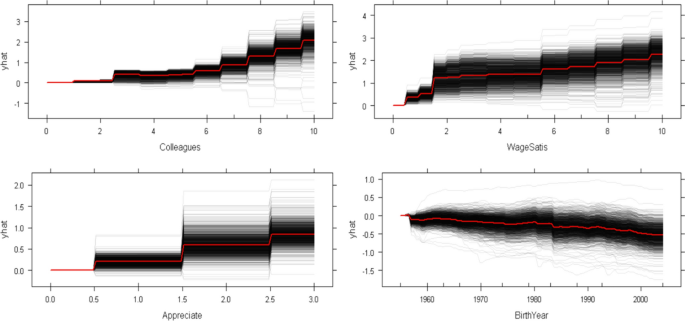
Individual conditional expectation plots
7 Discussion and Conclusion
Welfare maximization is generally, in standard economic textbooks, regarded as a respectable economic objective or driver in any society. But the empirical measurement of welfare (including life satisfaction) is still fraught with many hurdles and uncertainties. In practice, GDP per capita—or, in a labor market context, wage rates—are often regarded as signposts for economic performance. However, this measuring rod has many serious shortcomings, such as the neglect of distributional and equity aspects, the exclusive focus on income to the detriment of essential consumption categories (such as human health, food, safety, education, green environment, quality of life), the bias caused by the presence of negative externalities or social costs (e.g., climate change, social stress, environmental decay), or the omission of the welfare implications of the worker’s balance between leisure time and working time. The welfare of a society depends among other aspects on how satisfied laborers in that society are with their work and work environments. The present study has aimed to shed further light on the individual dimension of social welfare.
The present study used the most recent wave available of the Work and Schooling module of the LISS survey on individuals in the Netherlands. The empirical analysis was mostly founded on tree-based sequential ensemble prediction algorithms. The predictions were elaborated in detail using interpretable machine learning techniques to quantify the strengths and directions of the relationships between the survey features and the level of job satisfaction of an individual.
The main result is that wage satisfaction alone is not sufficient to ensure job satisfaction for the analyzed sample of individuals from the Netherlands. Being satisfied with the atmosphere among one's colleagues and feeling appreciated are also essential for job satisfaction. While low wage satisfaction can have a strong negative effect on job satisfaction, high satisfaction with colleagues has a stronger potential positive effect on job satisfaction compared to the effect of wage satisfaction. Among other results, we also observe that younger people are less satisfied with their jobs.
It is believed that facet-based measures such as the Job Descriptive Index (JDI) predict overall job satisfaction well (Judge & Klinger, 2008 ). These measures cover similar dimensions of job satisfaction (Dunham et al., 1977 ; Kinicki et al., 2002 ). For instance, the JDI examines job satisfaction in five dimensions: work, supervision, coworkers, pay, and promotion. The Minnesota Satisfaction Questionnaire (MSQ; Weiss et al., 1967 ) measures job satisfaction in terms of compensation, advancement, coworkers, and supervisor human relations. The Index of Organizational Reactions (IOR; Smith, 1976 ) looks at supervision, the kind and amount of work, finance, coworkers, physical conditions, career prospects, and company identification. Most if not all related variables are featured in our machine learning analysis. Results from our machine learning approach generally support the construct of these popular job satisfaction measurements. However, based on the SHAP importance values of features, using the value of 0.1 as a cut-off point, it is found that three features predict job satisfaction particularly well: coworker atmosphere, pay satisfaction, and recognition. And among the top three factors, the coworker dimension performs the best. Based on results from our study, simple measures like the JDI and MSQ perform reasonably well. Nevertheless, the lower predictive power of some features suggests that looking only at few features may not be sufficient capturing the overall picture. More is not necessarily better given the lower predictive performance of some other indicators.
Relatedly, features that capture job characteristics do not perform particularly well. This challenges the job characteristics model (Hackman & Oldham, 1976 ), the dominant approach in job satisfaction research (Judge et al., 2017 ). Although the social dimension of job satisfaction is well recognized and is featured in all major measures, coworker relations is understudied when compared with other dimensions such as work conditions and pay satisfaction (Judge & Kammeyer-Mueller, 2012 ). Our findings also suggest that if an objective of the many traditional human resources management policies is to improve job satisfaction, some of the focus on, for example, skills mismatch and training, could be less important than the cultivation of a supportive and collegial working environment. Future research should focus more on the social aspect. There are several prominent theories related to the social environment in workplace (Jolly et al., 2021 ). The conservation of resources theory (Halbesleben et al., 2014 ; Hobfoll, 1989 ) maintains that, as a resource, (perceived) social supports from colleague and job supervisors helps workers to regulate resources in times of difficulty to prevent (mental) resource loss such as burnout. Focusing on job performance and engagement, the job demands–resources theory (Bakker & Demerouti, 2007 ; Gerich & Weber, 2020 ) suggests that social resources in workplace could help workers to improve their performance. Given the general interpretation of the survey question, it remains unclear in which way coworker atmosphere in our analysis entails. Future research is still required to disentangle the mechanism behind the finding. Furthermore, since support has been picked up by a separate top-ranked variable, and work pressure, while included, was not a highly relevant variable in predicting job satisfaction. One interpretation is that work pressure has been captured by other variables, which have lower importance, one may favor the job demands–resources theory which emphasizes the role of support on performance. Another interpretation is that work pressure has a lower importance because part of it has been captured by social support. If this interpretation is correct, the conservation of resources theory might be more important. Which explanation of the finding is correct remains a topic of future research.
What do our findings suggest about the role of job satisfaction in the Great Resignation in the Netherlands? The importance of pay satisfaction is partly consistent with the explanation about the tightness of the labor market in the pandemic era. Under high inflation, workers are predictably unsatisfied with their wages. A tight labor market would favor workers to shift jobs and to ask for a high bid. However, the predictive power of collegial atmosphere out-weights that of wage satisfaction by a lot. It is likely that job satisfaction, mainly through its impact on coworker relations, plausibly related to remote working and home office, has a greater impact on job shifts when compared with the wage factor. As the Netherlands is a developed economy, the hierarchy of needs for individuals are likely to be different from middle and low income countries. The importance of coworker relationship may have relatively less importance compared to wages and other features pertaining to living standards in countries when working individuals are considerably more concerned about their socioeconomic well-being. While our evidence could shed light on the research question at hand with respect to high-income countries, empirical research on data from lower income countries may point towards different results.
A comparative study by Dolbier et al. ( 2005 ) shows the single-item measure of job satisfaction can be a psychometrically sound measurement.
The implementation of the UpSet plots in this study is done through the nainar package in R developed by Tierney and Cook ( 2023 ).
The recursive step of building sequential UpSet plots and selecting the variable or groups of variables to omit is coded by the authors.
The matrix dimensions are reduced using metric multidimensional scaling (Friedman, 2001 ).
Aldrich, C., & Auret, L. (2013). Unsupervised process monitoring and fault diagnosis with machine learning methods . Springer.
Book Google Scholar
Algemeen Dagblad. (2023). Grote ontslaggolf waait over uit amerika, 1 op de 5 werknemers wisselt van baan. Retrieved 23 January 2023 from https://www.ad.nl/economie/grote-ontslaggolf-waait-over-uit-amerika-1 -op-de-5-werknemers-wisselt-van-baan ad658da1/.
Andreoni, J. (1990). Impure altruism and donations to public goods: A theory of warm-glow giving. Economic Journal, 100 (401), 464–477. https://doi.org/10.2307/2234133
Article Google Scholar
Ariely, D., Kamenica, E., & Prelec, D. (2008). Man’s search for meaning: The case of Legos. Journal of Economic Behavior & Organization, 67 (3–4), 671–677. https://doi.org/10.1016/j.jebo.2008.01.004
Bakker, A. B., & Demerouti, E. (2007). The job demands-resources model: State of the art. Journal of Managerial Psychology, 22 (3), 309–328.
Bartel, A. P. (1981). Race differences in job satisfaction: A reappraisal. Journal of Human Resources, 16 (2), 294–303. https://doi.org/10.2307/145514
Berger, T., Frey, C. B., Levin, G., & Danda, S. R. (2019). Uber happy? Work and well-being in the ‘gig economy.’ Economic Policy, 34 (99), 429–477. https://doi.org/10.1093/epolic/eiz007
Blanchflower, D. G., & Oswald, A. J. (1999). Well-being, insecurity and the decline of American job satisfaction. NBER working paper, 7487.
Blau, F. D., & Kahn, L. M. (2017). The gender wage gap: Extent, trends, and explanations. Journal of Economic Literature, 55 (3), 789–865. https://doi.org/10.1257/jel.20160995
Borjas, G. J. (1979). Job satisfaction, wages, and unions. Journal of Human Resources, 14 (1), 21–40. https://doi.org/10.2307/145536
Breiman, L. (1996). Bagging predictors. Machine Learning, 24 (2), 123–140. https://doi.org/10.1007/BF00058655
Breiman, L. (2001). Random forests. Machine Learning, 45 (1), 5–32. https://doi.org/10.1023/A:1010933404324
Breiman, L., & Cutler, A. (accessed February 1, 2020). Random Forests. https://www.stat.berkeley.edu/~breiman/RandomForests/cc_home.htm .
Breiman, L., Friedman, J. H., Olshen, R. A., & Stone, C. J. (1984). Classification and regression trees . Monterey: Wadsworth and Brooks.
Google Scholar
Bryson, A., & MacKerron, G. (2017). Are you happy while you work? Economic Journal, 127 (599), 106–125. https://doi.org/10.1111/ecoj.12269
Card, D., Mas, A., Moretti, E., & Saez, E. (2012). Inequality at work: The effect of peer salaries on job satisfaction. American Economic Review, 102 (6), 2981–3003. https://doi.org/10.1257/aer.102.6.2981
Carree, M. A., & Verheul, I. (2012). What makes entrepreneurs happy? Determinants of satisfaction among founders. Journal of Happiness Studies, 13 , 371–387. https://doi.org/10.1007/s10902-011-9269-3
Cassar, L., & Meier, S. (2018). Nonmonetary incentives and the implications of work as a source of meaning. Journal of Economic Perspectives, 32 (3), 215–238. https://doi.org/10.1257/jep.32.3.215
Celbiş, M. G. (2022). Unemployment in rural Europe: A machine learning perspective. Applied Spatial Analysis and Policy . https://doi.org/10.1007/s12061-022-09464-0
Celbiş, M. G., Wong, P., Kourtit, K., & Nijkamp, P. (2023). Impacts of the COVID-19 outbreak on older-age cohorts in European labor markets: A machine learning exploration of vulnerable groups. Regional Science Policy & Practice, 15 (3), 559–584. https://doi.org/10.1111/rsp3.12520
Chandler, D., & Kapelner, A. (2013). Breaking monotony with meaning: Motivation in crowdsourcing markets. Journal of Economic Behavior & Organization, 90 , 123–133. https://doi.org/10.1016/j.jebo.2013.03.003
Chen, T., & Guestrin, C. (2016). xgboost: A scalable tree boosting system. In Proceedings of the 22nd ACM SIGKDD international conference on knowledge discovery and data mining (pp. 785–794). ACM.
Clark, A. E. (1997). Job satisfaction and gender: Why are women so happy at work? Labour Economics, 4 (4), 341–372. https://doi.org/10.1016/S0927-5371(97)00010-9
Clark, A. E. (2001). What really matters in a job? Hedonic measurement using quit data. Labour Economics, 8 (2), 223–242. https://doi.org/10.1016/S0927-5371(01)00031-8
Clark, A., Etilé, F., Postel-Vinay, F., Senik, C., & Van der Straeten, K. (2005). Heterogeneity in reported well-being: Evidence from twelve European countries. Economic Journal, 115 (502), C118–C132. https://doi.org/10.1111/j.0013-0133.2005.00983.x
Clark, A. E., Frijters, P., & Shields, M. A. (2008). Relative income, happiness, and utility: An explanation for the Easterlin paradox and other puzzles. Journal of Economic Literature, 46 (1), 95–144. https://doi.org/10.1257/jel.46.1.95
Clark, A. E., Kristensen, N., & Westergård-Nielsen, N. (2009). Job satisfaction and co-worker wages: Status or signal? Economic Journal, 119 (536), 430–447. https://doi.org/10.1111/j.1468-0297.2008.02236.x
Clark, A. E., & Oswald, A. J. (1996). Satisfaction and comparison income. Journal of Public Economics, 61 (3), 359–381. https://doi.org/10.1016/0047-2727(95)01564-7
Cornelissen, T., Heywood, J. S., & Jirjahn, U. (2011). Performance pay, risk attitudes and job satisfaction. Labour Economics, 18 (2), 229–239. https://doi.org/10.1016/j.labeco.2010.09.005
Cutler, A., Cutler, D. R., & Stevens, J. R. (2009). Tree-based methods. In X. Li & R. Xu (Eds.), High-dimensional data analysis in cancer research (pp. 83–102). Springer. https://doi.org/10.1007/978-0-387-69765-9_5
Chapter Google Scholar
Cutler, A., Cutler, D. R., & Stevens, J. R. (2012). Random forests. In C. Zhang & Y. Ma (Eds.), Ensemble machine learning: Methods and applications (pp. 157–175). Springer.
D’Ambrosio, C., Clark, A. E., & Barazzetta, M. (2018). Unfairness at work: Well-being and quits. Labour Economics, 51 , 307–316. https://doi.org/10.1016/j.labeco.2018.02.007
Demirkaya, H., Aslan, M., Güngör, H., Durmaz, V., & Şahin, D. R. (2022). Covid-19 and quitting jobs. Frontiers in Psychology, 13 , 916222. https://doi.org/10.3389/fpsyg.2022.916222
Diriwaechter, P., & Shvartsman, E. (2018). The anticipation and adaptation effects of intra-and interpersonal wage changes on job satisfaction. Journal of Economic Behavior & Organization, 146 , 116–140. https://doi.org/10.1016/j.jebo.2017.12.010
Dolbier, C. L., Webster, J. A., McCalister, K. T., Mallon, M. W., & Steinhardt, M. A. (2005). Reliability and validity of a single-item measure of job satisfaction. American Journal of Health Promotion, 19 (3), 194–198. https://doi.org/10.4278/0890-1171-19.3.194
Drakopoulos, S. A., & Theodossiou, I. (1997). Job satisfaction and target earnings. Journal of Economic Psychology, 18 (6), 693–704. https://doi.org/10.1016/S0167-4870(97)00030-5
Dunham, R. B., Smith, F. J., & Blackburn, R. S. (1977). Validation of the index of organizational reactions with the JDI, the MSQ, and faces scales. Academy of Management Journal, 20 (3), 420–432. https://doi.org/10.5465/255415
Duval, R., Oikonomou, M., & Tavares, M. M. (2022). Tight jobs market is a boon for workers but could add to inflation risks. Retrieved 23 January 2023 from https://www.imf.org/en/Blogs/Articles/2022/03/31/tight-jobs-market-is-a-boon-for-workers-but-could -add-to-inflation-risks.
Easterlin, R. A. (1995). Will raising the incomes of all increase the happiness of all? Journal of Economic Behavior & Organization, 27 (1), 35–47. https://doi.org/10.1016/0167-2681(95)00003-B
Easterlin, R. A. (2001). Income and happiness: Towards a unified theory. Economic Journal, 111 (473), 465–484. https://doi.org/10.1111/1468-0297.00646
Ellingsen, T., & Johannesson, M. (2007). Paying respect. Journal of Economic Perspectives, 21 (4), 135–149. https://doi.org/10.1257/jep.21.4.135
Friedman, J. H. (2001). Greedy function approximation: A gradient boosting machine. Annals of Statistics, 5 , 1189–1232.
Friedman, J., Hastie, T., & Tibshirani, R. (2001). The elements of statistical learning . Springer.
Friedman, J. H. (2002). Stochastic gradient boosting. Computational Statistics & Data Analysis, 38 (4), 367–378. https://doi.org/10.1016/S0167-9473(01)00065-2
García-Mainar, I., & Montuenga-Gómez, V. M. (2020). Over-qualification and the dimensions of job satisfaction. Social Indicators Research, 147 , 591–620. https://doi.org/10.1007/s11205-019-02167-z
Genicot, G., & Ray, D. (2020). Aspirations and economic behavior. Annual Review of Economics, 12 , 715–746. https://doi.org/10.1146/annurev-economics-080217-053245
Gerich, J., & Weber, C. (2020). The ambivalent appraisal of job demands and the moderating role of job control and social support for burnout and job satisfaction. Social Indicators Research, 148 , 251–280. https://doi.org/10.1007/s11205-019-02195-9
Goldstein, A., Kapelner, A., Bleich, J., & Pitkin, E. (2015). Peeking inside the black box: Visualizing statistical learning with plots of individual conditional expectation. Journal of Computational and Graphical Statistics, 24 (1), 44–65. https://doi.org/10.1080/10618600.2014.907095
Gosnell, G. K., List, J. A., & Metcalfe, R. D. (2020). The impact of management practices on employee productivity: A field experiment with airline captains. Journal of Political Economy, 128 (4), 1195–1233. https://doi.org/10.1086/705375
Green, F. (2010). Well-being, job satisfaction and labour mobility. Labour Economics, 17 (6), 897–903. https://doi.org/10.1016/j.labeco.2010.04.002
Hackman, J. R., & Oldham, G. R. (1976). Motivation through the design of work: Test of a theory. Organizational Behavior and Human Performance, 16 , 250–279. https://doi.org/10.1016/0030-5073(76)90016-7
Halbesleben, J. R., Neveu, J. P., Paustian-Underdahl, S. C., & Westman, M. (2014). Getting to the “COR” understanding the role of resources in conservation of resources theory. Journal of Management, 40 (5), 1334–1364. https://doi.org/10.1177/0149206314527130
Hamermesh, D. S. (1977). Economic aspects of job satisfaction . Wiley.
Hamermesh, D. S. (2004). Subjective outcomes in economics. Southern Economic Journal, 71 (1), 1–11. https://doi.org/10.1002/j.2325-8012.2004.tb00619.x
Hastie, T., Tibshirani, R., & Friedman, J. (2009). The elements of statistical learning . Springer.
Hobfoll, S. E. (1989). Conservation of resources: A new attempt at conceptualizing stress. American Psychologist, 44 (3), 513–524. https://doi.org/10.1037/0003-066X.44.3.513
James, G., Witten, D., Hastie, T., & Tibshirani, R. (2013). An introduction to statistical learning . Springer.
Jolly, P. M., Kong, D. T., & Kim, K. Y. (2021). Social support at work: An integrative review. Journal of Organizational Behavior, 42 (2), 229–251. https://doi.org/10.1002/job.2485
Jones, M. K., Jones, R. J., Latreille, P. L., & Sloane, P. J. (2009). Training, job satisfaction, and workplace performance in Britain: Evidence from WERS 2004. Labour, 23 , 139–175. https://doi.org/10.1111/j.1467-9914.2008.00434.x
Judge, T. A., & Kammeyer-Mueller, J. D. (2012). Job attitudes. Annual Review of Psychology, 63 , 341–367. https://doi.org/10.1146/annurev-psych-120710-100511
Judge, T. A., & Klinger, R. (2008). Job satisfaction: Subjective well-being at work. In M. Eid & R. J. Larsen (Eds.), The science of subjective well-being (pp. 393–413). Press.
Judge, T. A., Weiss, H. M., Kammeyer-Mueller, J. D., & Hulin, C. L. (2017). Job attitudes, job satisfaction, and job affect: A century of continuity and of change. Journal of Applied Psychology, 102 (3), 356–374. https://doi.org/10.1037/apl0000181
Karlsson, N., Loewenstein, G., & McCafferty, J. (2004). The economics of meaning. Nordic Journal of Political Economy, 30 (1), 61–75.
Kinicki, A. J., McKee-Ryan, F. M., Schriesheim, C. A., & Carson, K. P. (2002). Assessing the construct validity of the job descriptive index: A review and meta-analysis. Journal of Applied Psychology, 87 (1), 14.
Laffont, J., & Martimort, D. (2002). The theory of incentives . Princeton University Press.
Lange, T. (2012). Job satisfaction and self-employment: Autonomy or personality? Small Business Economics, 38 , 165–177. https://doi.org/10.1007/s11187-009-9249-8
Lepinteur, A. (2019). The shorter workweek and worker wellbeing: Evidence from Portugal and France. Labour Economics, 58 , 204–220. https://doi.org/10.1016/j.labeco.2018.05.010
Levels, M., Van der Velden, R., & Allen, J. (2014). Educational mismatches and skills: New empirical tests of old hypotheses. Oxford Economic Papers, 66 (4), 959–982. https://doi.org/10.1093/oep/gpu024
Lex, A., Gehlenborg, N., Strobelt, H., Vuillemot, R., & Pfister, H. (2014). UpSet: Visualization of intersecting sets. IEEE Transactions on Visualization and Computer Graphics, 20 (12), 1983–1992. https://doi.org/10.1109/TVCG.2014.2346248
Liu, Y., & Just, A. (2020). SHAPforxgboost: SHAP Plots for ‘XGBoost’. R package version 0.1.0.
Locke, E. A. (1976). The nature and causes of job satisfaction . Rand McNally College Publishing Company.
Loewenstein, G. (1999). Because it is there: The challenge of mountaineering… for utility theory. Kyklos, 52 (3), 315–343. https://doi.org/10.1111/j.1467-6435.1999.tb00221.x
Lundberg, S. M., & Lee, S.-I. (2017). A unified approach to interpreting model predictions. In Proceedings of the 31st international conference on neural information processing systems (pp. 4768–4777).
Luttmer, E. F. (2005). Neighbors as negatives: Relative earnings and well-being. Quarterly Journal of Economics, 120 (3), 963–1002. https://doi.org/10.1093/qje/120.3.963
Martin, L., Hauret, L., & Fuhrer, C. (2022). Digitally transformed home office impacts on job satisfaction, job stress and job productivity COVID-19 findings. PLoS ONE, 17 (3), e0265131. https://doi.org/10.1371/journal.pone.0265131
McBride, M. (2001). Relative-income effects on subjective well-being in the cross-section. Journal of Economic Behavior & Organization, 45 (3), 251–278. https://doi.org/10.1016/S0167-2681(01)00145-7
McBride, M. (2010). Money, happiness, and aspirations: An experimental study. Journal of Economic Behavior & Organization, 74 (3), 262–276. https://doi.org/10.1016/j.jebo.2010.03.002
McGuinness, S., Pouliakas, K., & Redmond, P. (2018). Skills mismatch: Concepts, measurement and policy approaches. Journal of Economic Surveys, 32 (4), 985–1015. https://doi.org/10.1111/joes.12254
Millán, J. M., Hessels, J., Thurik, R., & Aguado, R. (2013). Determinants of job satisfaction: A European comparison of self-employed and paid employees. Small Business Economics, 40 , 651–670. https://doi.org/10.1007/s11187-011-9380-1
Molnar, C. (2019). Interpretable Machine Learning. https://christophm.github.io/ interpretable-ml-book/.
Mulder, H., Hoofs, H., Verhagen, J., van der Veen, I., & Leseman, P. P. (2014). Psychometric properties and convergent and predictive validity of an executive function test battery for two-year-olds. Frontiers in Psychology, 5 , 733. https://doi.org/10.3389/fpsyg.2014.00733
Nikolova, M., & Cnossen, F. (2020). What makes work meaningful and why economists should care about it. Labour Economics, 65 , 101847. https://doi.org/10.1016/j.labeco.2020.101847
Origo, F., & Pagani, L. (2009). Flexicurity and job satisfaction in Europe: The importance of perceived and actual job stability for well-being at work. Labour Economics, 16 (5), 547–555. https://doi.org/10.1016/j.labeco.2009.02.003
Oswald, A. J., Proto, E., & Sgroi, D. (2015). Happiness and productivity. Journal of Labor Economics, 33 (4), 789–822. https://doi.org/10.1086/681096
Pilipiec, P., Groot, W., & Pavlova, M. (2020). A longitudinal analysis of job satisfaction during a recession in The Netherlands. Social Indicators Research, 149 , 239–269. https://doi.org/10.1007/s11205-019-02233-6
Pilipiec, P., Groot, W., & Pavlova, M. (2021). The causal influence of increasing the statutory retirement age on job satisfaction among older workers in the Netherlands. Applied Economics, 53 (13), 1498–1527. https://doi.org/10.1080/00036846.2020.1827136
Poggi, A. (2010). Job satisfaction, working conditions and aspirations. Journal of Economic Psychology, 31 (6), 936–949. https://doi.org/10.1016/j.joep.2010.08.003
Powdthavee, N. (2010). How much does money really matter? estimating the causal effects of income on happiness. Empirical Economics, 39 , 77–92. https://doi.org/10.1007/s00181-009-0295-5
Ravid, O., Malul, M., & Zultan, R. (2017). The effect of economic cycles on job satisfaction in a two-sector economy. Journal of Economic Behavior & Organization, 138 , 1–9. https://doi.org/10.1016/j.jebo.2017.03.028
Reuters. (2019). U.S. job openings, quits hit record highs in April. Retrieved 23 January 2023 from https://www.reuters.com/business/us-trade-deficit-narrows-april-2021-06-08/ .
Shapley, L. S. (1953). A value for n-person games. In H. Kuhn & A. Tucker (Eds.), Contributions to the theory of games II, annals of mathematics studies (Vol. 28, pp. 307–317). Princeton University Press.
Sloane, P. J., & Ward, M. E. (2001). Cohort effects and job satisfaction of academics. Applied Economics Letters, 8 (12), 787–791. https://doi.org/10.1080/13504850110045733
Smith, F. J. (1976). The Index of Organizational Reactions (IOR). JSAS Catalog of Selected Document in Psychology , Vol. 6 (i976), Ms. No. 1265.
Smith, J. C. (2015). Pay growth, fairness, and job satisfaction: Implications for nominal and real wage rigidity. Scandinavian Journal of Economics, 117 (3), 852–877. https://doi.org/10.1111/sjoe.12091
Smith, P. C., Kendall, L. M., & Hulin, C. L. (1969). The measurement of satisfaction in work and retirement: A strategy for the study of attitudes . Rand Mcnally.
Streefkerk, M., & Centerdata (2022). LISS panel—work and schooling—wave 15.
Štrumbelj, E., & Kononenko, I. (2013). Explaining prediction models and individual predictions with feature contributions. Knowledge and Information Systems, 41 (3), 647–665. https://doi.org/10.1007/s10115-013-0679-x
Tierney, N., & Cook, D. (2023). Expanding tidy data principles to facilitate missing data exploration, visualization and assessment of imputations. Journal of Statistical Software, 105 (7), 1–31. https://doi.org/10.18637/jss.v105.i07
Varian, H. R. (2014). Big data: New tricks for econometrics. Journal of Economic Perspectives, 28 (2), 3–28. https://doi.org/10.1257/jep.28.2.3
Vieira, J. A. C. (2005). Skill mismatches and job satisfaction. Economics Letters, 89 (1), 39–47. https://doi.org/10.1016/j.econlet.2005.05.009
Weiss, D. J., Dawis, R. V., England, G. W., & Lofquist, L. (1967). Minnesota studies in vocational rehabilitation. Manual for the Minnesota Satisfaction Questionnaire . University of Minnesota.
Weiss, H. M. (2002). Deconstructing job satisfaction: Separating evaluations, beliefs and affective experiences. Human Resource Management Review, 12 (2), 173–194. https://doi.org/10.1016/S1053-4822(02)00045-1
Xu, D., & Tian, Y. A. (2015). comprehensive survey of clustering algorithms. Annals of Data Science, 2 , 165–193. https://doi.org/10.1007/s40745-015-0040-1
Download references
The authors acknowledge the grant from the Romanian Ministry of Research, Innovation and Digitisation, CNCS—UEFISCDI, project number PN-III-P4-PCCE-2021-1878, within PNCDI III, project—Institutions, Digitalisation and Regional Development in the EU.
Author information
Authors and affiliations.
LAET, ENTPE, CNRS, University of Lyon, Lyon, France
Mehmet Güney Celbiş
UNU-MERIT, United Nations University, Maastricht, The Netherlands
Mehmet Güney Celbiş & Pui-Hang Wong
Maastricht Graduate School of Governance, Maastricht University, 6211 AX, Maastricht, The Netherlands
Pui-Hang Wong
Faculty of Management, Open University of the Netherlands, Heerlen, The Netherlands
Karima Kourtit & Peter Nijkamp
Centre for European Studies, Alexandru Ioan Cuza University, Iași, Romania
You can also search for this author in PubMed Google Scholar
Corresponding author
Correspondence to Pui-Hang Wong .
Ethics declarations
Conflict of interest.
The authors declare that the research was conducted in the absence of any commercial or financial relationships that could be construed as a potential conflict of interest.
Additional information
Publisher's note.
Springer Nature remains neutral with regard to jurisdictional claims in published maps and institutional affiliations.
See Figs. 6 and 7 .
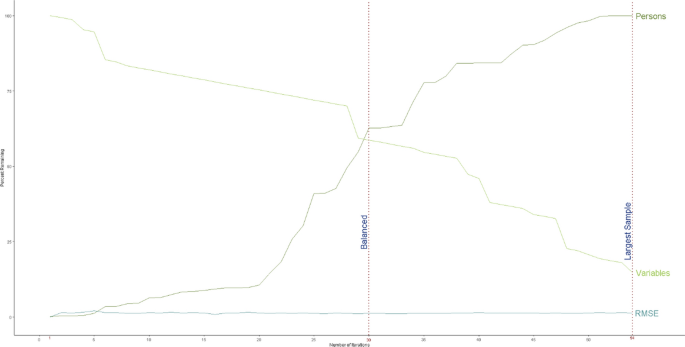
Iterations and the feature-observation trade-off
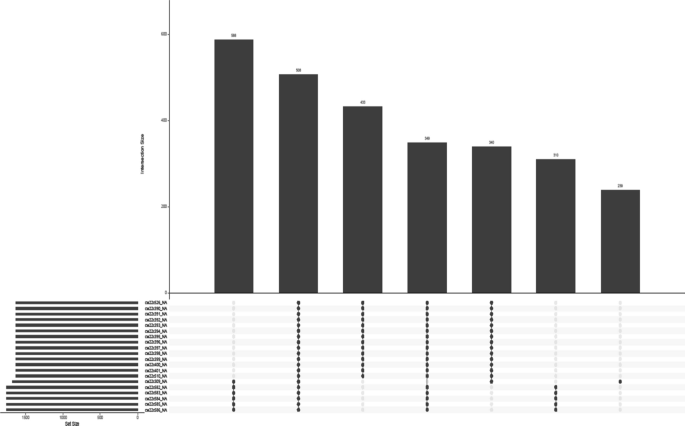
A sample UpSet plot from the fourth iteration
Rights and permissions
Open Access This article is licensed under a Creative Commons Attribution 4.0 International License, which permits use, sharing, adaptation, distribution and reproduction in any medium or format, as long as you give appropriate credit to the original author(s) and the source, provide a link to the Creative Commons licence, and indicate if changes were made. The images or other third party material in this article are included in the article's Creative Commons licence, unless indicated otherwise in a credit line to the material. If material is not included in the article's Creative Commons licence and your intended use is not permitted by statutory regulation or exceeds the permitted use, you will need to obtain permission directly from the copyright holder. To view a copy of this licence, visit http://creativecommons.org/licenses/by/4.0/ .
Reprints and permissions
About this article
Celbiş, M.G., Wong, PH., Kourtit, K. et al. Job Satisfaction and the ‘Great Resignation’: An Exploratory Machine Learning Analysis. Soc Indic Res 170 , 1097–1118 (2023). https://doi.org/10.1007/s11205-023-03233-3
Download citation
Accepted : 02 October 2023
Published : 30 October 2023
Issue Date : December 2023
DOI : https://doi.org/10.1007/s11205-023-03233-3
Share this article
Anyone you share the following link with will be able to read this content:
Sorry, a shareable link is not currently available for this article.
Provided by the Springer Nature SharedIt content-sharing initiative
- Job satisfaction
- Satisfaction with coworker
- Pay satisfaction
- Work conditions
- Job attitudes
- Find a journal
- Publish with us
- Track your research
Thank you for visiting nature.com. You are using a browser version with limited support for CSS. To obtain the best experience, we recommend you use a more up to date browser (or turn off compatibility mode in Internet Explorer). In the meantime, to ensure continued support, we are displaying the site without styles and JavaScript.
- View all journals
- My Account Login
- Explore content
- About the journal
- Publish with us
- Sign up for alerts
- Review Article
- Open access
- Published: 24 April 2023
The factors associated with teachers’ job satisfaction and their impacts on students’ achievement: a review (2010–2021)
- Kazi Enamul Hoque 1 ,
- Xingsu Wang 1 ,
- Yang Qi 1 &
- Normarini Norzan 2
Humanities and Social Sciences Communications volume 10 , Article number: 177 ( 2023 ) Cite this article
13k Accesses
3 Citations
1 Altmetric
Metrics details
The success of any educational organization depends heavily on the effectiveness of its teachers, who are tasked with transferring knowledge, supervising students, and enhancing the standard of instruction. Teachers’ job satisfaction has a significant impact on the lessons they teach since they are directly involved in transferring knowledge to students. In order to determine the effect of teachers’ job satisfaction (TJS) on students’ accomplishments, the researchers sought to analyze the empirical studies conducted over the previous 12 years (SA). To determine the characteristics that link to instructors’ job satisfaction and their effect on students’ achievement, thirty-two empirical studies were examined. The analysis of world-wide empirical research findings shows four types of results: (i) In some countries, teachers’ job satisfaction is low, but students’ achievement is high (Shanghai, China, South Korea, Japan, Singapore) (ii) In some countries, teacher job satisfaction is high, but student achievement is low (Mexico, Malaysia, Chile, Italy). (iii) In some countries, teachers’ job satisfaction is high, and so is student achievement (Finland, Alberta, Canada, Australia). (iv) In some countries, teacher job satisfaction is low, which has a negative impact on student achievement (Bulgaria, Brazil, Russia). In sum, irrespective of countries, highly satisfied teachers give their best to their students’ success, not only by imparting knowledge but also by giving extra attention to ensure the better achievement of each student. The review of this study makes it even more worthwhile to reflect on the need to avoid stereotypical considerations and assessments of any objective presentation of the phenomenon and to reflect more deeply on the need to assess the validity of the relationship study.
Similar content being viewed by others
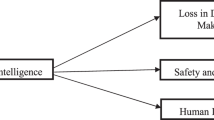
Impact of artificial intelligence on human loss in decision making, laziness and safety in education
Sayed Fayaz Ahmad, Heesup Han, … Antonio Ariza-Montes
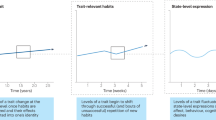
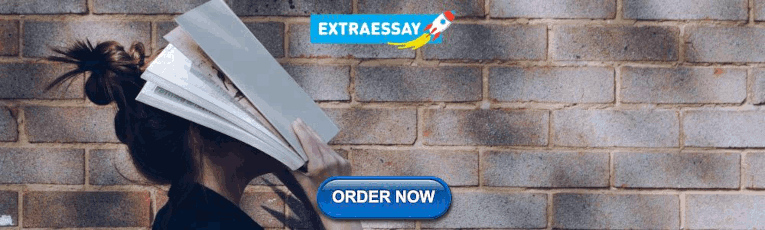
The process and mechanisms of personality change
Joshua J. Jackson & Amanda J. Wright
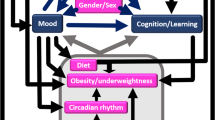
Effect of sleep and mood on academic performance—at interface of physiology, psychology, and education
Kosha J. Mehta
Introduction
Teachers are the main part of the school education system. The factors affecting students’ achievement (SA) should be multifaceted. Among them, teachers are one of the important factors that affect students’ academic performance (Ma, 2012 ). Teacher job satisfaction (TJS) refers to teachers’ satisfaction with their current work, which can be divided into internal satisfaction and external satisfaction (Wang, 2019 ). TJS inquiry and analysis can help managers not only comprehend teachers’ professional attitudes and avoid burnout but also provide some guidance for management decision-making. Improving TJS will assist instructors in maintaining a high level of passion and enthusiasm for their profession for a long time, allowing them to play even better in the lesson and ensuring consistent teaching quality (Zong, 2016 ). Teachers must have proper work satisfaction in order to be fully ready to transmit knowledge and skills important for learners to develop in SA. Teachers have been revered as “nation builders”. More specifically, teachers who teach in colleges and train students into elites and have talents in different disciplines are the key to a nation. Low TJS may lead to lower levels of education (Borah, 2016 ).
Many studies show that TJS has a significant positive correlation with job performance. As an example, Hayati & Caniago ( 2012 ) found that higher job satisfaction is conductive to higher job performance, while Ejimofor’s ( 2015 ) finding shows triadic relationships in which it indicates that TJS improves teaching quality, and teaching quality has the direct effect of improving students’ quality. One of the most important topics in every academic organization is TJS and SA. TJS not only increases productivity but also helps promote a productive teaching and learning environment. Based on this, both school administrators and the government should give more attention to meeting the needs of teachers to improve their motivational level to achieve educational goals so as to improve student academic performance (Ihueze et al., 2018 ). Surprisingly, though some researchers did not find a substantial link between TJS and SA (Ejimofor, 2015 ; Borah, 2016 ), the intuition and popular expectation is that TJS affects SA significantly and directly (Fisher, 2003 ). Moreover, Lopes & Oliveira ( 2020 ), utilizing information from the 2013 Teaching and Learning International Survey, demonstrated that teacher job satisfaction is a vital element of teachers’ and schools’ performance as well as students’ academic and educational attainment (TALIS). They also discovered that aspects of interpersonal relationships are the most effective predictors of job happiness. They advised schools to improve by addressing interpersonal problems, especially in the classroom, where the majority of perceived job satisfaction tends to reside. The findings demonstrated that, (1) among the personal traits of teachers, teacher efficacy had significant effects on job satisfaction (You et al., 2017 ). As the factors affecting teachers’ job satisfaction vary depending on the context of different countries, this review included studies from different regions to give a comprehensive scenario of findings based on different regional factors. The review results have revealed the associated factors of teachers’ job satisfaction and their impacts on student achievement. The findings can be the subject of further exploration. The review study set out to accomplish the following objectives:
Objectives:
to find whether teachers’ job satisfaction (TJS) has an impact on student achievement (SA)
to find the factors that have a positive impact on TJS and SA
to find the factors that influence the effect of TJS on SA and that manifest differently in different countries
Theoretical background
Between 2010 and 2021, the number of studies examining the impact of TJS on SA increased significantly.
Conceptualization of teachers’ job satisfaction
Job satisfaction is one of the important topics in the fields of occupational psychology, organizational behavior, and human resource management to explore employee productivity and organizational effectiveness (Fisher, 2003 ). With the development of humanistic thinking and the concept of lifelong education, this concept has been generally accepted by people, and the academic community has increasingly paid attention to the work-related emotional experiences of different professional or occupational groups such as teachers, nurses, etc.
Generally speaking, TJS refers to a teacher’s overall emotional experience and cognitive expression of their occupation, working conditions, and state. The international community generally believes that, as a variable of emotional attitude, TJS itself not only covers different dimensions but, more importantly, TJS has an important and direct impact on teachers’ enthusiasm and commitment to teaching. Daily work efficiency and effectiveness are also powerful predictors of SA. In addition, from the perspective of organizational commitment, improving TJS is an important way to enhance teachers’ sense of identity and belonging to the school, as well as to improve teachers’ professional attractiveness.
From the perspective of logical inference, according to the important phenomenon of the mentoring effect in the rise of talent chains and talent groups in the history of scientific development, as well as the practical experience that “the greatest happiness of teachers comes from the extraordinary achievements of students,” and even the word “teacher” often used when praising teachers, judging from the phrases such as “famous teachers produce master apprentices” and “peaches and plums fill the world,” in practical work, SA should also be one of the important sources of TJS (Wang & Zhang, 2020 ).
Factors affecting the teachers’ job satisfaction
Different studies have used different elements that have direct, indirect, or even no impacts on the job satisfaction of teachers. In their research on college teachers’ job satisfaction, Shi et al. ( 2011 ) revealed that work treatment, job pressure, leadership behavior, gender, age, etc. have more or less influence on the job satisfaction of college teachers. Existing research usually divides the factors that affect TJS into four levels: individual, school, work, and others. The influencing factors at the individual level can be grouped into objective factors and subjective factors. Among them, objective factors include teachers’ educational background, teaching years (experience), gender, professional title, monthly income and workload, teaching subjects, etc.; subjective factors include occupational preference and work engagement.
The two main influencing variables at the school level are students and management. While the management aspect comprises the institutional culture of the school and student management, the student aspect includes the student’s learning environment. The professional growth environment, work pressure, learning exchange possibilities, etc. are examples of workplace factors. The location of the school (eastern, central, or western) and whether it is located in an urban or rural area are examples of other levels (Beijing Normal University Teachers’ Labor Market Research Group et al., 2021 ).
These elements can be categorized into three groups when considered collectively: the elements of the college professors themselves, the elements of the institutions, and the level of compatibility between individuals and roles. The author’s research focuses on the connection between these three variables and college professors’ job satisfaction (Shi et al., 2011 ).
Professional title, educational background, and job satisfaction are among the factors that teachers can control for themselves, but these variables have very weak correlations and cannot be used as explanatory variables in the regression equation. Age, on the other hand, has a weak correlation with job satisfaction but can be used as a variable to explain job satisfaction. Salary level is the school component that has the greatest impact on TJS. The primary output that teachers receive from the organization is compensation, which is also a key component that teachers demand from the organization. The degree of alignment between instructors’ expectations and their compensation is the most significant element determining TJS in terms of the matching of people to roles.
Student achievement
Defining a student’s grades is not an easy task. The most common metric of achievement is undoubtedly student performance on achievement exams in academic disciplines like reading, language arts, math, science, and history. The quality of schools and teachers, students’ backgrounds and situations, and a host of other variables all have an impact on academic attainment (Cunningham, 2012 ). The researchers looked at academic levels, achievement gaps, graduation and dropout rates, student and school development over time, and student success after high school.
Academic achievement is the ability to complete educational tasks. Such achievements can be general or topic-specific. Academic achievement refers to students’ scores in courses, curriculums, courses, and books that they have studied, expressed in the form of marks, percentages, or any other scale of marks (Borah, 2016 ). It is important to highlight that academic performance encompasses not only students’ achievement in tests and exams, but also their participation in social events, cultural events, entertainment, athletics, and other activities in academic institutions and organizations.
Conceptual framework of the study
How to improve the academic performance of students is a popular topic in the field of education. After all, the purpose of education is to train students to become talents in society. Between 2010 and 2021, scholars from many different countries studied the relationship between TJS and SA. Many scholars’ studies have shown that there is a significant positive correlation between TJS and SA (McWherter, 2012 ; Crawford, 2017 ; Andrew, 2017 ; Iqbal et al., 2016 ). In these studies, the effects of TJS on SA were investigated, suggesting that it is fairly common in the literature to study the relationship between the two as a theme (Ejimofor, 2015 ; Borah, 2016 ).
Specifically, some districts have high SA but lower TJS than average schools. While some districts had high TJS, this did not improve SA. Therefore, it makes sense to understand the findings of these studies as a whole. What’s more, it is necessary to sort out the reasons for this divergence among numerous studies and make comparisons. The following questions serve as a guide for this study’s analysis of the findings in the literature on the effect of TJS on SA.
Does research show that TJS has an impact on SA?
What factors will have positive impact on tjs and sa.
What factors influence the effect of TJS on SA that manifests differently in different countries?
By examining previous research, this work seeks to characterize teacher motivation and assess the evaluation criteria and processes that account for student performance. The research method is systematically summarizing and analyzing based on a literature review, which helps us research and analyze the topic from a dialectical perspective. This study refers to the model of PSALSAR. The process of selecting documents starts with analyzing the topic, searching and classifying relevant documents, screening relevant documents from different sources according to the selection criteria, and finally extracting the most relevant documents for sorting out. Analysis (Bearman et al., 2012 ). The scope of this review also followed four criteria as outlined in the review work of Wayne & Youngs ( 2003 ).
Data sources
The data for this literature review was extracted from three major data sources: Web of Science, Scopus, Google Scholar, and HowNet. The review intended to take into account all recognized and relevant sources reporting on studies that are in English and falls within the duration of the study, which is a 12-year period from 2010 to 2021. The aim of the search is to locate all appropriate literature without expanding the search too much and retrieving a huge number of unrelated results. After applying an analytical inclusion/exclusion criterion to the 721 papers that were found, 32 papers were found to be applicable to the study’s objectives.
Data screening
The databases were searched using the following terms: “career management of teachers,” “teacher job satisfaction,” “student achievement,” and “teacher job satisfaction and student achievement.”
There is much literature on the relationship between TJS and SA, many of which use TJS as a mediator, or TJS is just one of the variables to promote SA. Since the focus of this review was on the impact of TJS on SA, the literature search consisted of two phases to ensure that all relevant literature on the relationship between the two was included. In the first phase, which focused on TJS, the following search terms were used: “teacher job satisfaction”, “teacher work satisfaction” and “teacher satisfaction”, combined with the search term “SA”. In the second stage, the focus is on the effect of TJS on the SA selected from the first stage choices. After screening for keywords and selecting the year interval as 2010–2021, 26 documents were finally extracted for research. The Preferred Reporting Items for Systematic Reviews and Meta-Analyses (PRISMA) is an evidence-based framework that clearly defines the bare minimum of items to be included in systematic reviews and meta-analyses (Moher et al., 2009 ).
Inclusion and exclusion criteria
In order to check on the quality and validity of the data obtained, the most recent journals available on the topic were chosen. Also, a high priority was given to reading the findings and extracts of every journal before it was selected for review. Literature analysis adopts the selected research selected by narrative methods so that the author can understand the literature and find the mode by carefully reading and interpreting the research results (De Rijdt et al., 2013 ).
Next, each article is completely reread to determine the important part. Based on the content analysis method, the paragraphs of important information containing the answer to the hypothesis research question are encoded. In this literature review, as mentioned in an earlier section, the PRISMA is applied to the inclusion and exclusion criteria for the review. The table below depicts the details (Table 1 ).
The review results of 32 empirical studies have revealed the following answers to formulated research questions. The answers are organized according to research questions.
From 2010 to 2021, most studies from different countries have shown that TJS has a positive impact on students SA (McWherter, 2012 ; Crawford, 2017 ; Farooqi & Shabbir et al., 2016 ) The morale of teachers is closely related to the academic achievements of students (Sabin, 2015 ). This is because when the teacher is dissatisfied with their work, they will transfer it to students in many ways, including the absence of classes. When this happens, students will suffer, and their academic performance will inevitably be negatively affected.
The research results also show that the more teachers believe that teaching is a valuable occupation, the more satisfied they are (Armstrong, 2009 ), and the better the students’ outcomes. A study in two sub-Saharan African nations examined the degree of teacher satisfaction in Uganda and Nigeria, its causes, and how it affects the quality of instruction and learning (Nkengne et al., 2021 ). According to research, teachers who are satisfied with their jobs are more likely to teach effectively, which should help their pupils learn more in the classroom. In order to make employees play a greater role, the work itself must have satisfactory characteristics. If it is interesting, it has good income and work safety. A teacher with high work satisfaction usually puts more effort into teaching and learning (Ihueze et al., 2018 ). However, whether it is based on the conclusions of existing mainstream theoretical research or on practical experience, low job satisfaction will not only affect teachers’ teaching enthusiasm but also cause teachers to have a teaching attitude problem of “not happy to teach”, as a result, it may even lead to the problem of “poor teaching” ability and ultimately have a negative impact on students’ academic performance; at the same time, it will also have a negative effect on the organizational commitment of in-service teachers and the professional attractiveness of teachers (Wang and Zhang, 2020 ).
The results of several earlier investigations likewise show the opposite. The intuition and general expectations of the work satisfaction of teachers will affect the students’ grades (Fisher, 2003 ). TJS lacks a significant relationship with student grades, which is in line with the findings of Banerjee et al. ( 2017 ). According to their longitudinal study of young children between kindergarten and fifth grade, student reading growth has no association with teacher job satisfaction, but there is a slight but favorable relationship between the two. Although this seems to be a violation, it is consistent with the previous 41 research (Iaffaldano & Muchinsky, 1985 ; Fisher, 2003 ). Studies lack significant relationships between TJS and children’s academic achievements (Ejimofor, 2015 ). The relationship between TJS and SA can be ignored, which is to say that teachers’ job satisfaction has no significant impact on SA (Borah, 2016 ). Despite such findings, most of the scholars agree with the notion that job satisfaction concerning school teachers reflects their strong motivation towards their dedication to students’ performance (Manandhar et al., 2021 ).
TJS has a positive impact on the quality of education; therefore, affecting teacher job satisfaction can affect the quality of education. However, because multiple factors have a significant effect on TJS and SA, not all things that improve TJS also improve academic performance. In many circumstances, the aims of increasing job happiness and enhancing student accomplishment are antagonistic rather than complimentary (Michaelowa, 2002 ).
System control factors and incentive structures, in particular, due to a complete lack of job protection, have been found to have a significant positive impact on teacher performance, although they tend to be strongly opposed by the instructors involved. According to a study (Tsai & Antoniou, 2021 ) conducted in Taiwan with 113 teachers and 2,334 students to examine the relationships between teacher attitudes toward teaching mathematics, teacher self-efficacy, student achievement, and teacher job satisfaction, teacher attitudes toward teaching mathematics, efficacy in the classroom, and student achievement in mathematics could, to some extent, explain variations in teacher job satisfaction. The majority of the variation in teacher job satisfaction, which may translate into improved teacher efficacy and student achievement, was explained by teacher attitudes about teaching mathematics. It suggests that improving the quality of education for children is a complex process for which variables like instructors’ attitudes, their level of self-efficacy, and their pleasure and satisfaction at work may be responsible (Khalid, 2014 ). According to a study by Rutkowski et al. ( 2013 ) on 81 elementary school teachers from a sizable metropolitan school district in the United States, the PD program improved teachers’ pedagogical topic knowledge and subject-matter expertise. The teachers that participated in the professional development program showed a greater level of topic and instructional strategy understanding.
In fact, only a few variables had a clear positive effect on both goals. One was related to classroom equipment, which had a clear positive effect on teachers’ well-being. By accessing the material support of a supportive and satisfying working environment, teachers are more likely to be more actively involved in their teaching activities, which in turn is an important factor in how this leads to the creation of relevance for students’ teaching practice (Benevene et al., 2020 ). Among the device variables, many variables do not have any significant effect on SA and therefore do not even appear in the regressions. However, the situation is different for students’ teaching materials, which are highly correlated with SA and positively correlated with teacher job satisfaction. Therefore, improving the supply of textbooks is certainly a relevant policy option (Hee et al., 2019 ). In terms of class size, however, it does have a significant impact on teacher work satisfaction (Hee et al., 2019 ). TJS can be improved by reducing class size. Increasing class size is clearly the best answer to high student numbers for both teachers and kids. The disadvantages of double-shifting are so severe that they are applicable to classes of up to 100 pupils. Teacher efficacy has been shown to correlate with the presence of classroom processes and procedures, and the presentation of good classroom processes may contribute to better outcomes for students (Perera et al., 2022 ). At the same time, good teacher efficacy will also enable teachers to provide more effective pedagogical support, resulting in better outcomes for students in teaching and learning.
The commonly held belief that low salaries and large class sizes are the key reasons for low teacher job satisfaction and low SA has no support in this study. This had no discernible impact on SA. As a result of this research, an extraordinarily costly endeavor to enhance teacher compensation does not appear to be a suitable policy option in general.
What factors influence the effect of TJS on SA that manifest differently in different countries?
In their study, Dicke et al. ( 2020 ) found that the working context item was associated with student accomplishment for both teachers and principals; however, only the general and working environment factors of teacher job satisfaction were related to the disciplinary climate observed by students. International Student Evaluation Projects (PISA) and the Teaching and Learning International Survey (TALIS) found that in countries and regions with outstanding academic performance of students, their teachers showed lower work satisfaction (OECD, 2016 ; TALIS, 2013). For example, for two consecutive years in the PISA test, it is hailed by the World Bank as Shanghai, China, which has the highest education system in the world, shows that the teacher’s work satisfaction is significantly lower in the Talis 2013 survey results (Liang et al., 2016 ). In fact, Shanghai is not a special case. PISA (2015) data shows that, as a whole, despite the outstanding performance of students, the work satisfaction and occupational satisfaction of East Asian countries and regions are lower than the international average (Chen, 2017 ). At the same time, in those countries or regions that are less ideal in the PISA test, their teachers’ work satisfaction and occupational satisfaction are often significantly higher than the international average (Wang and Zhang, 2020 ). Data from 1,539 teachers at 306 secondary schools in the two Indian metropolises of New Delhi and Kolkata supported the notion that instructional leadership has indirect effects on teaching and learning and that the social and affective climate of the classroom has direct effects on teacher job satisfaction, which in turn affects student achievement (Dutta & Sahney, 2016 ). De Vries et al. ( 2013 ) conducted a study on teacher professional development (PD) in the context of inquiry-based science education (IBSE). The study aimed to investigate the effects of a long-term PD program on teachers’ knowledge, attitudes, and behavior. A total of 62 primary school teachers from the Netherlands participated in the trial and were randomized to either the PD program or a control group. Surveys, interviews, and classroom observations were used to gauge the teachers’ awareness of, attitudes about, and behavior with regard to IBSE. The outcomes demonstrated that the PD program had a favorable impact on teachers’ attitudes and knowledge to IBSE. IBSE knowledge was higher among the teachers in the PD program group, and they were more enthusiastic about advantages for their pupils.
Australia, Chile, Czech Republic, Germany, Italy, Korea, Portugal, Spain, USA, Brazil, China, Colombia, Dominia, Peru, Arab, Netherlands Combining the survey and test data of TALIS 2013 and PISA 2012, it is found that there are four main correspondences between teachers’ job satisfaction index and students’ test scores in different countries (regions) (OECD, 2016 ; TALIS, 2013). Countries (regions) with better test performance, such as Finland, Canada (Alberta), Australia, the Netherlands, etc. The second is countries (regions) where teachers’ job satisfaction is high but students’ performance is significantly worse, such as Mexico, Malaysia, Chile, Italy, etc.; the third is countries (regions) where teachers’ job satisfaction is significantly lower than the international average level, but students’ test scores are significantly higher than the international average or even among the best, such as Shanghai (China), South Korea, Japan, Singapore, and other East Asian countries and regions; the fourth is countries with low teacher job satisfaction and low student test scores, such as Bulgaria, Brazil, Russia, etc.
There is a clear correlation between cultural differences in different countries (regions) and teachers’ job satisfaction. Firstly, teachers’ job satisfaction in countries (regions) with a high power distance index is generally lower; secondly, individualism is different in different countries (regions), and there is a potential positive correlation between the index and teacher job satisfaction; furthermore, countries with a high long-term orientation index tend to have lower teacher job satisfaction and vice versa; and lastly, countries with a high indulgence index score. In other regions, teachers’ job satisfaction is generally higher (Wang and Zhang, 2020 ).
Combined with the analysis results, it can be determined to a large extent that national (or regional) culture has a potential impact on teachers’ job satisfaction that cannot be ignored, and compared with the usual experience of “good SA, TJS should also be high”. From the perspective of stereotyped thinking, the degree of influence of cultural differences on teachers’ job satisfaction, or at least the degree of correlation between the two, is more obvious and stronger. Therefore, it seems that a more reasonable explanation can be made for the puzzling differences in teacher job satisfaction in different countries (regions) shown by the TALIS 2013 survey data (Sims, 2017 ; Wang and Zhang, 2020 ). In order to highlight areas for development and to wrap up the section, Kravarušić ( 2021 ) can be quoted. He looked at the fundamental components of the structure of factors in the Republic of Serbia. He discovered that the status of society, the immediate social context, the quality of the study program, the professional environment, continuous professional development, pedagogical practice, the personal characteristics of educators, job satisfaction, and private life all influence the level of competence of teachers. As a result, the setting is a key factor in determining how satisfied teachers are with their work, which in turn influences student progress.
Limitations & recommendations
This review summarizes and analyzes the existing literature on teacher job satisfaction and student achievement, which will help improve student performance from the perspective of teachers’ job satisfaction in the future. However, there are still some limitations in the research process, which can be considered in the follow-up research.
First of all, it is about theoretical research. Although the research on teachers’ job satisfaction theory has been refined and divided into three stages for discussion and definition, the influencing factors obtained from the experimental analysis based on this definition have also been proved to be effective. However, this method of definition has not been widely accepted, which does not mean that researchers have not paid enough attention, precisely because job satisfaction theory involves too much content and there is not enough practice to demonstrate that the theory is true and effective. In addition, due to the repetition and contradiction of different types of theories caused by too many related studies, it has seriously affected the research on the classification and influencing factors of teachers’ job satisfaction at different stages. As the working lives of teachers cannot be simply divided into pre-service teachers and in-service teachers, such as teachers before retirement, teachers in private schools, etc., these can be the basis for classification, and the factors that affect teachers’ satisfaction are also different. At this stage, the theoretical knowledge of teachers’ job satisfaction is simply divided into stages, but teaching is a process, and its complexity and variability cannot be explained clearly by existing theories. The research at this stage cannot realize the analysis of teachers in terms of process. Therefore, future research can focus on defining teachers job satisfaction from different aspects through practice and strive to obtain the most accurate factors that affect teacher satisfaction so as to achieve the adjustment of students’ achievement.
Secondly, the articles chosen for this study include a reasonably high proportion of quantitative research, which is the primary method for studying the theory of teacher satisfaction and examining its affecting elements. However, this means that the research method is single, and the research results mainly come from the results of questionnaires and data analysis. The research results on the influencing factors to improve teachers’ job satisfaction promote the development of teachers’ personal professional abilities, and thus students’ achievement have been confirmed. However, some researchers said that relying too much on questionnaires and data made them ignore the complexity of the research content, and the validity of the research results also weakened their in-depth research ideas to a certain extent. In order to gain a deeper understanding of teachers’ job satisfaction, a qualitative investigation should be used to truly understand the source of teachers’ satisfaction and provide more possibilities for research on influencing factors (Fig. 1 ).
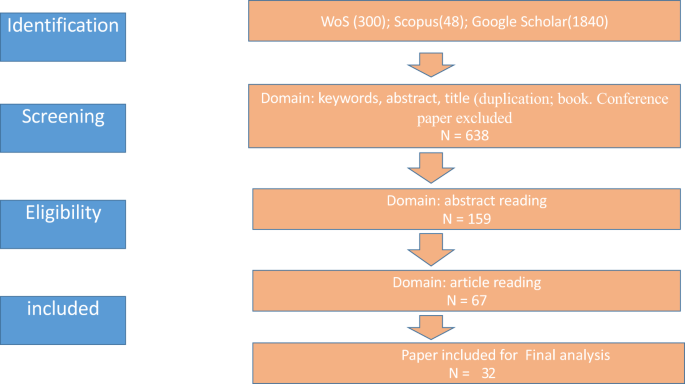
An overview of the overall screening procedures as well as the workflow associated with selecting relevant material. At the beginning of the process, a total of 2188 records were discovered from the databases. After eliminating gray literatures, duplicated papers, book and book chapters and conference papers, the number of articles maintained for further title reading and abstract review was decreased to 632. Following this, only 159 papers met the eligibility requirements for additional abstract reading and main body skimming. Out of that, 67 remained to be read in their entirety. During the main body reading, articles 32 without content pertaining either to teachers’ professional development or student achievement were excluded manually. At last, 32 papers met eligibility requirements for SLR study remained.
Concluding remarks
In the past 12 years, most studies from different countries have paid much attention to the effect of teachers’ job satisfaction on student achievement. Most research shows that TJS will have a positive impact on students achievement (McWherter, 2012 ; Crawford, 2017 ; Andrew, 2017 ; Iqbal et al., 2016 ). Although there is still a small subset of studies showing no significant relationship between TJS and SA, the number of these studies is far lower than the number of studies that believe that TJS has a positive effect on SA (Ejimofor, 2015 ; Borah, 2016 ). There are many factors that affect TJS, but only work treatment, work pressure, co-worker relationships, etc. However, research shows that only classroom equipment and classroom size have a positive effect on both. Research shows that it is largely certain that national (or regional) culture has a non-negligible potential impact on teacher job satisfaction. Moreover, compared with the usual experience and stereotyped thinking that “students have good grades, so teachers’ job satisfaction should also be high”, the degree of influence of cultural differences on teachers’ job satisfaction, or at least the degree of correlation between the two, is more obvious and relevant. The review of this study makes it even more worthwhile to reflect on the need to avoid stereotypical considerations and assessments of any objective presentation of the phenomenon and to reflect more deeply on the need to assess the validity of the relationship study. As in the case of measuring teacher job satisfaction, the extent to which cultural differences affect teacher job satisfaction cannot be ignored. This is why it is important for scholars to develop a framework for measuring teachers’ job satisfaction according to cultural contexts and specific social needs and to give more dimensions to reflection and further measurement. Only on this basis can the overall level of teacher satisfaction be improved, thus increasing the overall level of teacher effectiveness and well-being, and better motivating students to engage in teaching and learning activities that lead to better quality learning outcomes.
Data availability
Data sharing is not applicable to this research as no data were generated or analyzed
Andrew K (2017) Teacher job satisfaction and student academic performance at Uganda Certificate of Education in secondary schools in Uganda: a case study of kamwenge district. Doctoral dissertation, Kabale University
Armstrong M (2009) Armstrong’s handbook of management and leadership a guide to managing for results. Kogan
Banerjee N, Stearns E, Moller S, Mickelson RA (2017) Teacher job satisfaction and student achievement: the roles of teacher professional community and teacher collaboration in schools. Am J Educ 123(2):203–241
Article Google Scholar
Bearman M, Smith CD, Carbone A, Slade S, S, Baik C, Hughes-Warrington M, Neumann DL (2012) Systematic review methodology in higher education. High Educ Res Dev 31(5):625–640
Benevene P, De Stasio S, Fiorilli C (2020) Editorial: well-being of school teachers in their work environment. Front Psychol 11:1239. https://doi.org/10.3389/fpsyg.2020.01239
Article PubMed PubMed Central Google Scholar
Borah A (2016) Impact of teachers’ job satisfaction in academic achievement of the students in higher technical institutions: A study in the Kamrup district of Assam. Clar Int Multidiscip J 8(1):51–55. http://www.journalijdr.com
Chen C (2017) An empirical study on the influencing factors of middle school teachers’ job satisfaction——based on the analysis of the Pisa 2015 Teacher Survey Data [in Chinese]. Teach Educ Res 29(2):9
ADS Google Scholar
Crawford JD (2017) Teacher job satisfaction as related to student performance on state-mandated testing. Doctoral dissertation, Lindenwood University
Cunningham J (2012) Student achievement. In: National Conference of State Legislatures. pp. 1–6
Dicke T, Marsh HW, Parker PD, Guo JS, Riley P, Waldeyer J (2020) Job satisfaction of teachers and their principals in relation to climate and student achievement. J Educ Psychol 112(5):1061–1073
Dutta V, Sahney S (2016) School leadership and its impact on student achievement The mediating role of school climate and teacher job satisfaction. Int J Educ Manag 30(6):941–958
Ejimofor AD (2015) Teachers’ job satisfaction, their professional development and the academic achievement of low-income kindergartners. Doctoral dissertation, University of North Carolina at Greensboro
Farooqi R, Shabbir F (2016) Impact of teacher professional development on the teaching and learning of English as a second language. J Educ Pract 7(17):41–50
Google Scholar
Fisher CD (2003) Why do lay people believe that satisfaction and performance are correlated? possible source of a commonsense theory. J Organ Behav 24:753–777. https://doi.org/10.1002/job.219
Beijing Normal University Teachers’ Labor Market Research Group, Guan CH, Xing CB, Chen CF (2021) Secondary school teachers’ career satisfaction and willingness to move and their influencing factors: experience from China Education Tracking Survey data (ceps) Evidence. Beijing Soc Sci 3:19
Hayati K, Caniago I (2012) Islamic work ethic: the role of intrinsic motivation, job satisfaction, organizational commitment and job performance. Proc Soc Behav Sci 65:272–277. https://doi.org/10.1016/j.sbspro.2012.11.122
Hee OC, Shukor MFA, Ping LL, Kowang TO, Fei GC (2019) Factors influencing teacher job satisfaction in Malaysia. Int J Acad Res Bus Soc Sci 9(1):1166–1174. https://doi.org/10.6007/IJARBSS/v9-i1/5628
Iaffaldano MT, Muchinsky PM (1985) Job satisfaction and job performance: a meta-analysis. Psychol Bull 97(2):251–273. https://doi.org/10.1037/0033-2909.97.2.251
Ihueze S, Unachukwu GO, Onyali LC (2018) Motivation and teacher job satisfaction as correlates of students’ academic performance in secondary schools in Anambra state. UNIZIK J Educ Manag Policy 2(1):59–68. https://journals.unizik.edu.ng/index.php/ujoemp/article/view/569
Iqbal A, Aziz F, Farooqi T, Ali S (2016) Relationship between teachers’ job satisfaction and students’ academic performance. Eurasian J Educ Res 16(65):1–35. https://doi.org/10.14689/ejer.2016.65.19
Kravarušić VB (2021) Factors of professional activity of educators in pedagogical practice, international journal of cognitive research in Science. Eng Educ 9(3):385–398
Liang X, Kidwai H, Zhang M, Zhang Y (2016) How Shanghai does it: Insights and lessons from the highest-ranking education system in the world. World Bank Publications
Lopes J, Oliveira C (2020) Teacher and school determinants of teacher job satisfaction: a multilevel analysis. Sch Eff Sch Improv 31(4):641–659
Ma Y (2012) Talking about the influence of teachers on students’ academic performance [in Chinese]. New Curr Teach Res Edn 12:140–141
Manandhar P, Manandhar N, Joshi SK (2021) Job satisfaction among school teachers in Duwakot, Bhaktapur District, Nepal. Int J Occup Safe Health 11(3):165–169
McWherter S (2012) The effects of teacher and student satisfaction on student achievement. Gardner-Webb University
Michaelowa K (2002) Teacher job satisfaction, student achievement, and the cost of primary education in Francophone Sub-Saharan Africa, 188. HWWA Discussion Paper
Moher D, Liberati A, Tetzlaff J, Altman DG (2009) Preferred reporting items for systematic reviews and meta-analyses: the PRISMA statement. British Medical Association, Ontario, Canada
Nkengne P, Pieume O, Tsimpo C, Ezeugwu G, Wodon Q (2021) Teacher satisfaction and its determinants: analysis based on data from Nigeria and Uganda. Int Stud Cath Educ 13(2):190–208
OECD F (2016) FDI in figures. Organisation for European Economic Cooperation, Paris
Perera H, Maghsoudlou A, Miller C, McIlveen P, Barber D, Part R, Reyes A (2022) Relations of science teaching self-efficacy with instructional practices, student achievement and support, and teacher job satisfaction. Contemp Educ Psychol 69:102041. https://doi.org/10.1016/j.cedpsych.2021.102041
De Rijdt C, Stes A, van der Vleuten C, Dochy F (2013) Influencing variables and moderators of transfer of learning to the workplace within the area of staff development in higher education: a research review. Educ Res Rev 8:48–74. https://doi.org/10.1016/j.edurev.2012.05.007
Rutkowski D, Rutkowski L, Bélanger J, Knoll S, Weatherby K, Prusinski E (2013) Teaching and Learning International Survey TALIS 2013: conceptual framework. Final. OECD Publishing
Sabin JennyT (2015) Teacher morale, student engagement, and student achievement growth in reading: a correlational study. J Organ Educ Leadersh 1:5, http://digitalcommons.gardner-webb.edu/joel/vol1/iss1/5
Shi J, Peng HC, Huang YF (2011) A survey of influencing factors of teachers’ satisfaction in colleges and universities [in Chinese]. J Zhanjiang Norm Coll 32(1):5
Sims S (2017) TALIS 2013: Working conditions, teacher job satisfaction and retention. Statistical working paper. UK Department for Education. Castle View House East Lane, Runcorn, Cheshire, WA7 2GJ, UK
Sun H, Li H, Lin C (2008) Secondary school teachers’ job satisfaction status and its related factors [in Chinese]. Stud Psychol Behav 6(4):260–265
Tayyar KAl (2014) Job satisfaction and motivation amongst secondary school teachers in Saudi Arabia. PhD Thesis, University of York, Department of Education
Tsai P, Antoniou P (2021) Teacher job satisfaction in Taiwan: making the connections with teacher attitudes, teacher self-efficacy and student achievement. Int J Educ Manag 35(5):1016–1029
De Vries S, Jansen EPWA, Van de Grift WJCM (2013) Profiling teachers’ continuing professional development and the relations with their beliefs about learning and teaching. Teach Teach Educ 33:78–89. https://doi.org/10.1016/j.tate.2013.02.006
Wang Y (2019) Research on the relationship between the leadership of primary and secondary school principals and teacher satisfaction [in Chinese]. Shanghai J Educ Eval 8(6):5
MathSciNet Google Scholar
Wang Z, Zhang M (2020) International comparison of teachers’ job satisfaction: differences, reasons and countermeasures: an empirical analysis based on TALIS data [in Chinese]. Prim Second School Abroad 1:19
Wayne AJ, Youngs P (2003) Teacher characteristics and student achievement aains: a review. Rev Educ Res 73(1):89–122
You S, Kim AY, Lim SA (2017) Job satisfaction among secondary teachers in Korea: effects of teachers’ sense of efficacy and school culture. Educ Manag Adm Leadersh 45(2):284–297
Zong QZ (2016) Influencing factors and incentives of teachers’ job satisfaction. Mod Bus Trade Ind 37(26):2. https://doi.org/10.19311/j.cnki.1672-3198.2016.26.060
Download references
Author information
Authors and affiliations.
Faculty of Education, Universiti Malaya, 50603, Kuala Lumpur, Malaysia
Kazi Enamul Hoque, Xingsu Wang & Yang Qi
Universiti Pendidikan Sultan Idris, 35900, Tanjung Malim, Perak, Malaysia
Normarini Norzan
You can also search for this author in PubMed Google Scholar
Corresponding author
Correspondence to Kazi Enamul Hoque .
Ethics declarations
Competing interests.
The authors declare no competing interests.
Ethical approval
This manuscript does not contain any studies with human participants performed by any of the authors
Informed consent
Since there were no human subjects involved in this review study, no consent was required.
Additional information
Publisher’s note Springer Nature remains neutral with regard to jurisdictional claims in published maps and institutional affiliations.
Rights and permissions
Open Access This article is licensed under a Creative Commons Attribution 4.0 International License, which permits use, sharing, adaptation, distribution and reproduction in any medium or format, as long as you give appropriate credit to the original author(s) and the source, provide a link to the Creative Commons license, and indicate if changes were made. The images or other third party material in this article are included in the article’s Creative Commons license, unless indicated otherwise in a credit line to the material. If material is not included in the article’s Creative Commons license and your intended use is not permitted by statutory regulation or exceeds the permitted use, you will need to obtain permission directly from the copyright holder. To view a copy of this license, visit http://creativecommons.org/licenses/by/4.0/ .
Reprints and permissions
About this article
Cite this article.
Hoque, K.E., Wang, X., Qi, Y. et al. The factors associated with teachers’ job satisfaction and their impacts on students’ achievement: a review (2010–2021). Humanit Soc Sci Commun 10 , 177 (2023). https://doi.org/10.1057/s41599-023-01645-7
Download citation
Received : 23 August 2022
Accepted : 27 March 2023
Published : 24 April 2023
DOI : https://doi.org/10.1057/s41599-023-01645-7
Share this article
Anyone you share the following link with will be able to read this content:
Sorry, a shareable link is not currently available for this article.
Provided by the Springer Nature SharedIt content-sharing initiative
This article is cited by
Determinants of job satisfaction among faculty members of a veterinary university in india: an empirical study.
- Rachna Singh
- Gautam Singh
- Anika Malik
Current Psychology (2024)
Quick links
- Explore articles by subject
- Guide to authors
- Editorial policies

Academia.edu no longer supports Internet Explorer.
To browse Academia.edu and the wider internet faster and more securely, please take a few seconds to upgrade your browser .
Enter the email address you signed up with and we'll email you a reset link.
- We're Hiring!
- Help Center
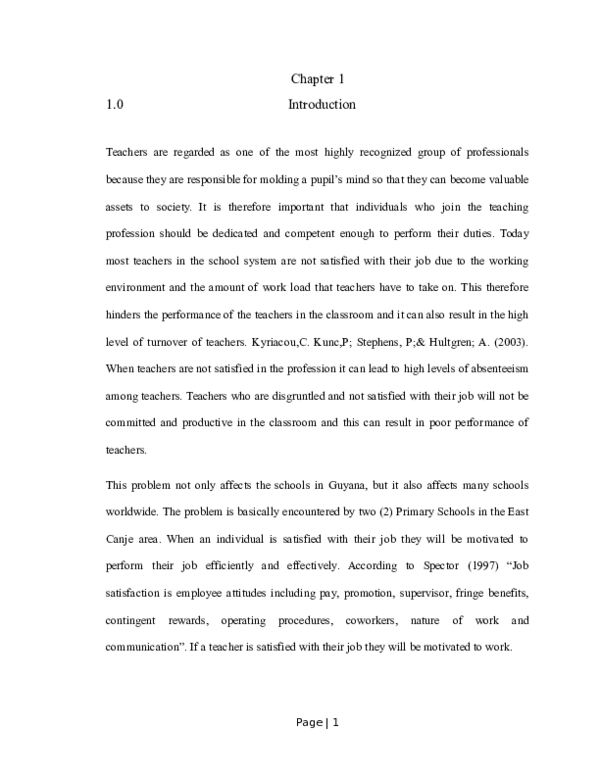
Job Satisfaction Research Proposal

Related Papers
Raphael A Balogun
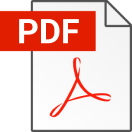
SANJAY JAIN , Dr.Shiladitya Verma
An individual’s general outlook towards his or her work is called as job satisfaction. It is the disparity between the amount of rewards one receives and the amount he or she believes he or she should receive. Job satisfaction has the direct relation with the job performance of an employee. Lower job satisfaction tends to lead to both turnover and absenteeism, whereas high job satisfaction results in increased job performance. Students are one of the valuable assets of any society. Well-being of society depends upon its students because these are the student who will take the accountability of the triumph of the society in future and in achieving this objective, the teacher’s play extremely vital role. They are source of guidance at many crucial steps in academic life. When teachers are satisfied with their job they can perform the responsibilities with more concentration, dedication and proficiency. College Teachers are the most imperative group of professionals for our nation’s future. Therefore, it is astonishing to know that still today many of the college teachers are dissatisfied with their jobs. Job satisfaction among college teachers is good, not only for themselves but for the society as a whole. It increases productivity and classroom performance in the college. Wellbeing of any society depends upon the role played by the teacher. Teachers are the source of guidance in all the essential steps in the scholastic lives of the students. When teachers are satisfied with their job they can execute their responsibilities with more concentration and dedication. Job satisfaction is one of the most widely discussed issues in organizational behavior, personnel and human resource management and organizational management. As teaching does require a great deal of meticulousness and dedication, so in teaching it is more important to have mental commitment and loyalty than physical presence.
IJRDO - Journal of Social Science and Humanities Research ISSN: 2456-2971
Sheena Mae T . Comighud, EdD
This study aimed to determine the motivational factors that influence teachers' job performance. The data pertain to the motivational factors that influence teachers' motivation which in turn affects their performance. These factors are categorized as existence needs, relatedness needs, and growth needs. The respondents of the study are the teachers of Bacong District, Division of Negros Oriental during the school year 2016-2017. The study utilized the descriptive-correlational method of research in the sense that it attempted to determine the motivational factors and their relationship to teachers' job performance. The study made use of a researcher-made questionnaire that determined the extent to which the motivational factors influence teachers' performance. The statistical tools used for the treatment of data are Frequency Distribution and Percentage, weighted mean and Pearson Product-Moment Correlation Coefficient. The following are the salient finding of the study. Majority of the teachers in Bacong District are female, on their prime of life, have advanced professionally, occupy Teacher 2 plantilla position, and with considerable years of teaching experience.Teachers' performance based on the RPMS is "Very Satisfactory". Teachers are "Very Highly Motivated" by the motivational factors relative to existence needs, relatedness needs and growth needs. The extent to which teachers are motivated by the motivational factors is significantly related to their job performance. Teachers' profile in terms of sex, educational qualification and teaching experience is significantly related to their job performance based on the RPMS, however, in relation to the extent to which teachers are motivated by the motivational factors, teachers' profile was found to be insignificant. In general, it was concluded that the higher is teachers' motivation, the better is their job performance.
M.ED SEMINAR PAPER
ABAYOMI I S A A C ARAIYEGBEMI
The main purpose of this study was to examine the factors militating against teacher’s performance in secondary school. Four(4) research questions were formulated to guide this study. The questionnaire was used for data collection from teachers. The analysis was done using frequency and percentage. It was found that: Poor classroom management, poor communication, poor salary and unfavorable condition of service, lack of content knowledge, lack of enthusiasm, lack of effective school administration and conducive work environment, teachers' family problems, and discouraging behaviors by students are all factors that can make a teacher not to perform effectively in secondary schools. It was then recommended that teachers be motivated by their employees through a favorable salary structure, teachers should ensure to be enthusiastic about teaching and they should develop themselves for optimal productivity
AL-ISHLAH: Jurnal Pendidikan
Nisaul Khaira
This research aims to determine the effect of work motivation and principal's performance on teacher job satisfaction together at a senior high school in … sebutkan nama kota/kabupaten/provinsinya. This research uses a quantitative approach with associative methods. The analysis technique data used is simple and multiple linear regression analysis, t-test, and F test. The results showed that: 1) There is a significant influence between the principal's work motivation on teacher job satisfaction by 79.1%. This means that work motivation will affect the job satisfaction of teachers in schools for the achievement of maximum performance; 2) There is a significant influence between the principal's performance on teacher job satisfaction by 49.7%. Therefore, the two variables can go hand in hand, the better the principal's performance, the better the teacher's job satisfaction; 3) There is a significant influence between work motivation and principal performance on tea...
Miguel Soliman
Teaching is a highly noble profession and teachers are always a boon to the society. The ultimate process of education could be simplified as a meaningful interaction between the teacher and the taught. The teacher thus plays a direct and crucial role in moulding a pupil towards education. Since a teacher is a role model for the students, job satisfaction and eventually performance of teachers become very vital in the fields of education. Thus the researcher felt the need to investigate the job satisfaction and performance of teachers in different categories of schools following different systems of education. From the total population, a sample of 196 teachers from state board schools, 198 teachers from matriculation board schools and 194 teachers from central board schools were drawn. The results of the study indicated that teachers in central board schools were significantly better in their job satisfaction and performance compared to their counterparts in matriculation and state board schools. This may be attributed to the fact that central board school teachers enjoy better infrastructure facilities and congenial working environment than the matriculation and state board teachers. It is for the school authorities, policy makers and society at large to ensure factors contributing to job satisfaction of teachers to the maximum possible extent and thereby enhancing their teaching perforrmance to its optimum.
Mark Akoto-senaman
The purpose of this study was to ascertain the extent of job satisfaction of teachers in private and public basic schools in the Ketu South District. The study was guided by four research questions all of which sought to find out teachers’ level of job satisfaction and issues related to their job satisfaction. The literature reviewed acknowledged job satisfaction as one part of the motivational process. While motivation is primarily concerned with goal-directed behaviour, job satisfaction refers to the fulfilment acquired by experiencing various job activities and rewards. Descriptive survey design was the design employed for the study. There was a total population of 98 schools and 2180 teachers, out of which 150 teachers from 10 schools were used as the sample for the study. A questionnaire was used to gather information from respondents. The gathered data were analyzed using the Statistical Package for Social Sciences (SPSS) computer software and organized in frequency distribution tables according to the demands of the research questions. The study showed that most teachers were dissatisfied and would not encourage pupils to become teachers in future. This is due to the fact that they believe that there is no prestige in the job and the teaching profession is not lucrative. There was no vast difference between the level of job satisfaction in the private schools and in the public schools. The study therefore recommends that there should be enhanced salaries to help boost the morale of the teachers.
This research examined student’s appraisal of the conduct of undergraduate examination in Olabisi Onabanjo University, Ago-Iwoye, Ogun State. The instrument used in this research is a Student Questionnaire/Opinionaire on University Examination (SQOUE) designed specifically to investigate the perception of student on the conduct of examination in Olabisi Onabanjo University. The sample size of 100 questionnaire were used. Three hypotheses were tested using the Pearson Chi-Square method. The first hypothesis revealed that there is a significant relationship between student assessment and school examination timetable. The second hypothesis revealed that there is a significant relationship between student opinion and activities of the examination invigilators and the third hypothesis revealed that there is a significant relationship between examination ethics and examination malpractice. Also, at the end of the study, recommendations that were considered valid were suggested.
Indonesia journal of multidisciplinary research
Onikoyi Olawale
The study investigated the teachers job satisfaction and it's perceived influence on secondary School students academic performance using a descriptive survey research approach
Social Sciences
Berita Simonaitienė
RELATED PAPERS
Jurnal Sains Kesehatan
Desi Fitriani
Capital Markets Law Journal
Ross Buckley
PEDRO VICUÑA JURADO
Landslide Science and Practice
Jorge Yepes
Croatian Operational Research Review
Darija Marković
The Proceedings of the National Symposium on Power and Energy Systems
Takemi Chikahisa
Roberto Gerundo
Rizka Annisa
Holz als Roh- und Werkstoff
Επιθεώρηση Κοινωνικών Ερευνών
Dimitris Emmanuel
Case Studies in Thermal Engineering
Mahmoud Khaled
TELKOMNIKA (Telecommunication Computing Electronics and Control)
Iwan Kurnia Wirawan
hoàng nguyễn
Pathophysiology
masoumeh nozari
International Journal of Applied Mathematics, Electronics and Computers
Mohamed El-mahallawy
Raquel França
arXiv (Cornell University)
Mohammad Rasouli Azad
Citizenship teaching and learning
Kristina Llewellyn
Physical Review E
Stanislav Denisov
Adan Pando Moreno
Ichwan Santoso
Suprema - Revista de Estudos Constitucionais
Livia Guimaraes
- We're Hiring!
- Help Center
- Find new research papers in:
- Health Sciences
- Earth Sciences
- Cognitive Science
- Mathematics
- Computer Science
- Academia ©2024
Ask a question from expert
Research Proposal on Job Satisfaction of Employees
Added on 2020-05-16
Added on 2020-05-16
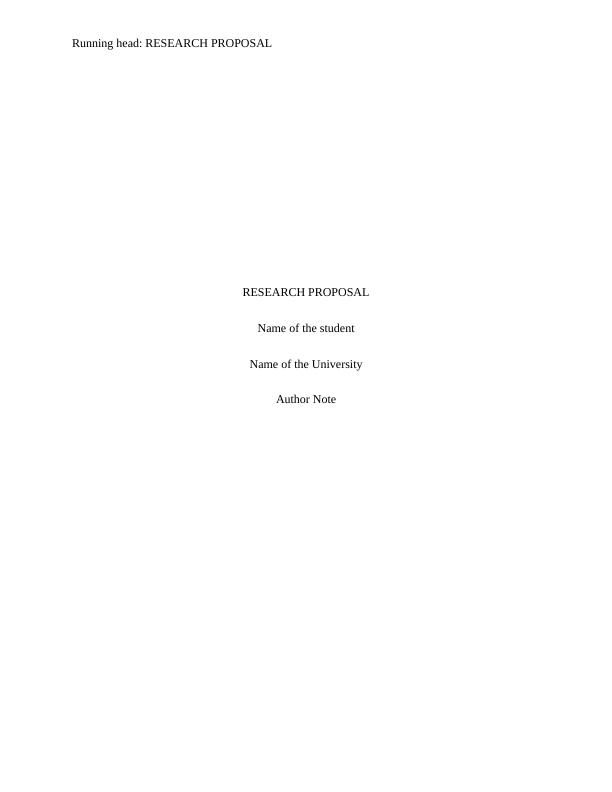
End of preview
Want to access all the pages? Upload your documents or become a member.
Determinants of Nurse Job Satisfaction in China: A Case Study of Guangdong General Hospital lg ...
Employee motivation and employees' job satisfaction lg ..., research proposal on impact of employee motivation lg ..., research proposal on the organizational behavior lg ..., business psychology, coaching and mentoring lg ..., impact of job satisfaction on organizational performance: a case study of shell abuja lg ....
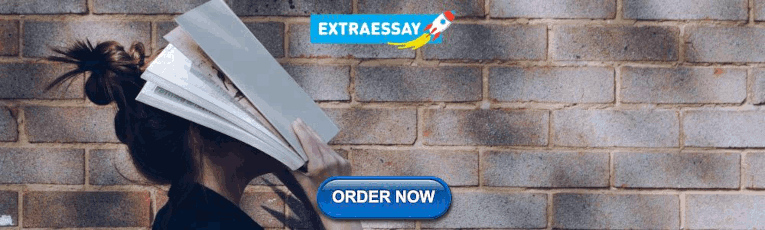
IMAGES
VIDEO
COMMENTS
The research results show that: (1) Knowledge influences performance, (2) Work quality influences performance, (3) Commitment influences performance, (4) Knowledge influences job satisfaction, (5 ...
(Chang et al., 2021). Little is known about how COVID-19 has impacted the job satisfaction of those who left the traditional office space to work remotely. The specific problem is that research regarding employee job satisfaction is lacking among the population of individuals who were asked to work from home due to the global crisis.
The survey data were created using a 5-point Likert scale based on existing research on job satisfaction, advancement opportunities & possibilities, compensation system, work-life balance, company culture, management, and company recommendations. We commissioned an online research company PMI to conduct an online survey of former & current ...
As regards the involvement in the solution of problems and in the proposal of new ideas, 18 interviewees (56%) believe they feel involved and listened to: ... The main purpose of the research was to explore, through qualitative research, job satisfaction, work engagement and work-related stress/burnout of professionals working in a facility ...
The aim of this study is to analyze simultaneously knowledge, attitudes, and practices toward job satisfaction in a general population in a large metropolitan area. The data acquired from 1043 questionnaires—administered to subjects with an average age of 35.24 years—revealed that only 30% is satisfied by his job.
A large amount of economics research in job satisfaction has been testing this hypothesis. Using data on British workers, ... Accordingly, this leads to a proposal that, because job satisfaction is unobserved and is related to some volatile, external factors like economic fluctuations and labor market policies (Pilipiec et al., 2020, 2021 ...
satisfaction could impact employee job performance. Every person is unique and might expect a different outcome from the job; however, there are some job satisfaction factors that psychologists usually agree will improve employee satisfaction (Lane, Alino, & Schneider, 2017). I investigated job satisfaction as a two-way exchange process between
This research proposal has been presented for examination with my approval as the appointed supervisor. Signed: _____ Date: _____ Dr. Paul Katuse ... (2013) ,job satisfaction is generally recognized as the most important and frequently field . 2 studied attitude in organizational behaviour. Luthans (1998) posited that job satisfaction takes ...
The analysis of world-wide empirical research findings shows four types of results: (i) In some countries, teachers' job satisfaction is low, but students' achievement is high (Shanghai, China ...
Since most research on teacher job satisfaction is cross-sectional, the above study makes an important contribution to the field by employing a longitudinal design. School composition. School composition is an important environmental factor for teacher job satisfaction and turnover intentions as, paired with teacher knowledge and skills, it ...
This paper theoretically examined the impact of job satisfaction on organizational performance. It notes that job satisfaction is vital for improved organizational performances. The paper posits that an employee with a high level of job satisfaction holds positive attitudes towards his job. On
RESEARCH PROPOSAL FOR JOB SATISFACTION INTRODUCTION: Employee satisfaction or job satisfaction is, rather simply, how comfortable or employees are satisfied with their jobs. Satisfaction survey is the main measurement process using to find employees satisfaction. . Its all are the important to companies who want to keep their employees happy ...
Ms. Anishma Bajracharya has defended research proposal entitled "Determinants of job satisfaction and their impact on employee commitment: A study of foreign joint venture banks ... (Sempane et al., 2002). Research has indicated that job satisfaction does not come about in isolation, as it is dependent on organizational variables such as ...
An individual's general outlook towards his or her work is called as job satisfaction. It is the disparity between the amount of rewards one receives and the amount he or she believes he or she should receive. Job satisfaction has the direct relation with the job performance of an employee. Lower job satisfaction tends to lead to both ...
Research Proposal: Job Satisfaction of Tenured Employees. One of the important factors that contribute to the success of any institution is its employees' satisfaction. In determining whether employees are contented and happy in fulfilling their work, employee job satisfaction measurement and evaluation must be done on a regular basis.
3 RESEARCH PROPOSAL Introduction The following report contains the research proposal for gauging the job satisfaction of the employees of Sydney based companies.The job satisfaction of the employee is the psychological self satisfaction of the employees while working in an organization due to various organizational and psychological factors. The project objective and research outlines the ...
Overall, there does not seem clear consensus among academics what factor really affect job satisfaction level the most because those varied according to the person and the organization. In association with the literature review the flowing conceptual framework was crate to structure the research. 2.