Exploratory Data Analysis
- First Online: 15 September 2017
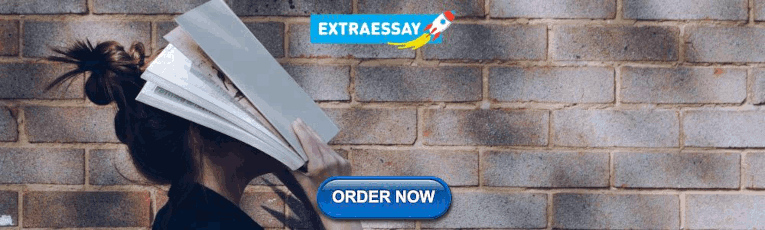
Cite this chapter
- Karen A. Monsen 2
1345 Accesses
This chapter presents Exploratory Data Analysis (EDA) as an approach for gaining understanding and insight about a particular dataset, in order to support and validate statistical findings and also to potentially generate, identify, and create new hypotheses based on patterns in data. Examples of EDA are provided and interpretations are discussed. EDA may be used at any stage in the data analysis process from cleaning through transformation and descriptive analysis, as well as using results from every stage. Discovery of patterns may inspire a new direction in intervention effectiveness research, as well as further supporting or validating existing projects. Data visualization skills are essential for individuals working with large datasets.
This is a preview of subscription content, log in via an institution to check access.
Access this chapter
- Available as PDF
- Read on any device
- Instant download
- Own it forever
- Available as EPUB and PDF
- Compact, lightweight edition
- Dispatched in 3 to 5 business days
- Free shipping worldwide - see info
- Durable hardcover edition
Tax calculation will be finalised at checkout
Purchases are for personal use only
Institutional subscriptions
Tukey JW (1977) Exploratory data analysis. Addison-Wesley, Reading, MA
Google Scholar
Monsen KA, Peterson JJ, Mathiason MA, Kim E, Lee S, Chi CL, Pieczkiewicz DS (2015) Data visualization techniques to showcase nursing care quality. Comput Inform Nurs 33(10):417–426
Article PubMed Google Scholar
Dzemyda G, Kurasova O, Zilinskas J (2013) Strategies for multidimensional data visualization. In Multidimensional data visualization: Methods and applications. Springer, New York, NY, pp. 5–40
Lee S, Kim E, Monsen KA (2015) Public health nurse perceptions of Omaha System data visualization. Int J Med Inform 84(10):826–834
Kirk A (2012) Data visualization: a successful design process. Packt, Birmingham
Gehlenborg N, Wong B (2012) Points of view: heat maps. Nat Methods 9(3):213
Article CAS PubMed Google Scholar
Aigner W, Miksch S, Schumann H, Tominski C (2011) Visualization of time-oriented data. Springer, London
Book Google Scholar
Kim E, Monsen K, Pieczkiewicz D (2013) Visualization of Omaha System data enables data-driven analysis of outcomes. In: American Medical Informatics Association Annual Meeting, Washington, DC
Monsen KA, Peterson JJ, Mathiason MA, Kim E, Votova B, Pieczkiewicz DS (2017) Discovering public health nurse–specific family home visiting intervention patterns using visualization techniques. West J Nurs Res 39(1):127–146
Article Google Scholar
Mendenhall W, Beaver RJ, Beaver BM (2012) Introduction to probability and statistics. Cengage Learning, Boston, MA
What is R? [Internet]. [cited 12 May 2017]. Available from https://www.r-project.org/about.html
What is Tableau? [Internet]. [cited 12 May 2017]. Available from http://www.tableau.com/products/desktop#TGETWeLmfvacBMZt.99
D3 Data Driven Documents [Internet]. [cited 12 May 2017]. Available from https://d3js.org /
Anscombe FJ (1973) Graphs in statistical analysis. Am Stat 27(1):17–21. doi: 10.2307/2682899
Green SB, Salkind NJ (2010) Using SPSS for windows and macintosh: analyzing and understanding data. Prentice Hall, Upper Saddle River, NJ
Radhakrishnan K, Monsen KA, Bae SH, Zhang W (2016) Visual analytics for pattern discovery in home care. Appl Clin Inform 7(3):711–730
Article PubMed PubMed Central Google Scholar
Chi C (2015) Data mining for translation to practice. Presented at 2nd international conference on research methods for standard terminologies, West Paul, MN
Harter JM, Wu X, Alabi OS, Phadke M, Pinto L, Dougherty D, Petersen H, Bass S, Taylor II RM (2012) Increasing the perceptual salience of relationships in parallel coordinate plots. In: IS&T/SPIE Electronic Imaging, 2012 Jan 22. International Society for Optics and Photonics, pp 82940T–82940T
Inselberg A (2009) Parallel coordinates: visual multidimensional geometry and its applications. Springer, New York, pp 1–554
Few S. Multivariate analysis using parallel coordinates. Perceptual Edge web site. [Internet]. [cited 12 May 2017]. Available from http://www.perceptualedge.com/articles/b-eye/parallel_coordinates.pdf . Published 12 Sept 2006. Updated 2015
Download references
Author information
Authors and affiliations.
School of Nursing, University of Minnesota System School of Nursing, Minneapolis, Minnesota, USA
Karen A. Monsen
You can also search for this author in PubMed Google Scholar
Rights and permissions
Reprints and permissions
Copyright information
© 2018 Springer International Publishing AG
About this chapter
Monsen, K.A. (2018). Exploratory Data Analysis. In: Intervention Effectiveness Research: Quality Improvement and Program Evaluation. Springer, Cham. https://doi.org/10.1007/978-3-319-61246-1_7
Download citation
DOI : https://doi.org/10.1007/978-3-319-61246-1_7
Published : 15 September 2017
Publisher Name : Springer, Cham
Print ISBN : 978-3-319-61245-4
Online ISBN : 978-3-319-61246-1
eBook Packages : Medicine Medicine (R0)
Share this chapter
Anyone you share the following link with will be able to read this content:
Sorry, a shareable link is not currently available for this article.
Provided by the Springer Nature SharedIt content-sharing initiative
- Publish with us
Policies and ethics
- Find a journal
- Track your research
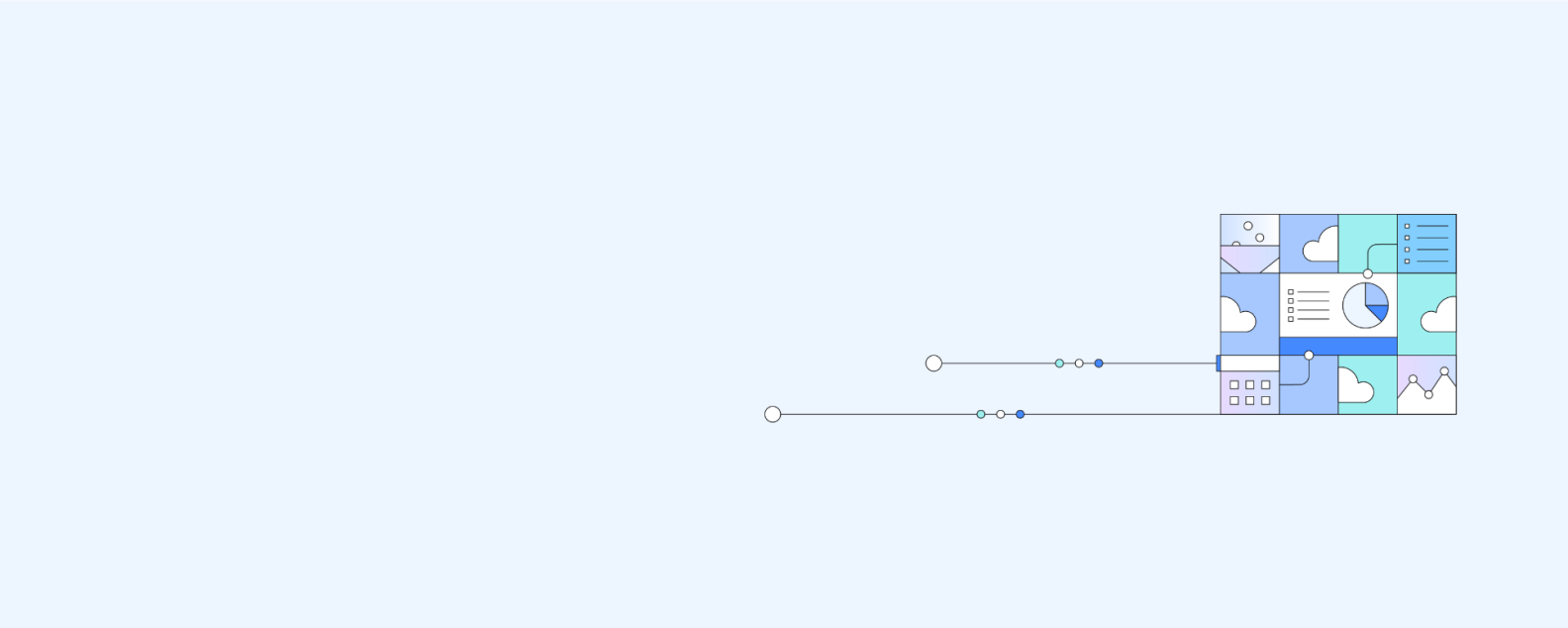
Exploratory data analysis (EDA) is used by data scientists to analyze and investigate data sets and summarize their main characteristics, often employing data visualization methods.
EDA helps determine how best to manipulate data sources to get the answers you need, making it easier for data scientists to discover patterns, spot anomalies, test a hypothesis, or check assumptions.
EDA is primarily used to see what data can reveal beyond the formal modeling or hypothesis testing task and provides a provides a better understanding of data set variables and the relationships between them. It can also help determine if the statistical techniques you are considering for data analysis are appropriate. Originally developed by American mathematician John Tukey in the 1970s, EDA techniques continue to be a widely used method in the data discovery process today.
Learn how to leverage the right databases for applications, analytics and generative AI.
Register for the ebook on generative AI
The main purpose of EDA is to help look at data before making any assumptions. It can help identify obvious errors, as well as better understand patterns within the data, detect outliers or anomalous events, find interesting relations among the variables.
Data scientists can use exploratory analysis to ensure the results they produce are valid and applicable to any desired business outcomes and goals. EDA also helps stakeholders by confirming they are asking the right questions. EDA can help answer questions about standard deviations, categorical variables, and confidence intervals. Once EDA is complete and insights are drawn, its features can then be used for more sophisticated data analysis or modeling, including machine learning .
Specific statistical functions and techniques you can perform with EDA tools include:
- Clustering and dimension reduction techniques, which help create graphical displays of high-dimensional data containing many variables.
- Univariate visualization of each field in the raw dataset, with summary statistics.
- Bivariate visualizations and summary statistics that allow you to assess the relationship between each variable in the dataset and the target variable you’re looking at.
- Multivariate visualizations, for mapping and understanding interactions between different fields in the data.
- K-means Clustering is a clustering method in unsupervised learning where data points are assigned into K groups, i.e. the number of clusters, based on the distance from each group’s centroid. The data points closest to a particular centroid will be clustered under the same category. K-means Clustering is commonly used in market segmentation, pattern recognition, and image compression.
- Predictive models, such as linear regression, use statistics and data to predict outcomes.
There are four primary types of EDA:
- Univariate non-graphical. This is simplest form of data analysis, where the data being analyzed consists of just one variable. Since it’s a single variable, it doesn’t deal with causes or relationships. The main purpose of univariate analysis is to describe the data and find patterns that exist within it.
- Stem-and-leaf plots, which show all data values and the shape of the distribution.
- Histograms, a bar plot in which each bar represents the frequency (count) or proportion (count/total count) of cases for a range of values.
- Box plots, which graphically depict the five-number summary of minimum, first quartile, median, third quartile, and maximum.
- Multivariate nongraphical: Multivariate data arises from more than one variable. Multivariate non-graphical EDA techniques generally show the relationship between two or more variables of the data through cross-tabulation or statistics.
- Multivariate graphical: Multivariate data uses graphics to display relationships between two or more sets of data. The most used graphic is a grouped bar plot or bar chart with each group representing one level of one of the variables and each bar within a group representing the levels of the other variable.
Other common types of multivariate graphics include:
- Scatter plot, which is used to plot data points on a horizontal and a vertical axis to show how much one variable is affected by another.
- Multivariate chart, which is a graphical representation of the relationships between factors and a response.
- Run chart, which is a line graph of data plotted over time.
- Bubble chart, which is a data visualization that displays multiple circles (bubbles) in a two-dimensional plot.
- Heat map, which is a graphical representation of data where values are depicted by color.
Some of the most common data science tools used to create an EDA include:
- Python: An interpreted, object-oriented programming language with dynamic semantics. Its high-level, built-in data structures, combined with dynamic typing and dynamic binding, make it very attractive for rapid application development, as well as for use as a scripting or glue language to connect existing components together. Python and EDA can be used together to identify missing values in a data set, which is important so you can decide how to handle missing values for machine learning.
- R: An open-source programming language and free software environment for statistical computing and graphics supported by the R Foundation for Statistical Computing. The R language is widely used among statisticians in data science in developing statistical observations and data analysis.
For a deep dive into the differences between these approaches, check out " Python vs. R: What's the Difference? "
Use IBM Watson® Studio to determine whether the statistical techniques that you are considering for data analysis are appropriate.
Learn the importance and the role of EDA and data visualization techniques to find data quality issues and for data preparation, relevant to building ML pipelines.
Learn common techniques to retrieve your data, clean it, apply feature engineering, and have it ready for preliminary analysis and hypothesis testing.
Train, validate, tune and deploy generative AI, foundation models and machine learning capabilities with IBM watsonx.ai, a next-generation enterprise studio for AI builders. Build AI applications in a fraction of the time with a fraction of the data.
- Privacy Policy

Home » Exploratory Research – Types, Methods and Examples
Exploratory Research – Types, Methods and Examples
Table of Contents

Exploratory Research
Definition:
Exploratory research is a type of research design that is used to investigate a research question when the researcher has limited knowledge or understanding of the topic or phenomenon under study.
The primary objective of exploratory research is to gain insights and gather preliminary information that can help the researcher better define the research problem and develop hypotheses or research questions for further investigation.
Exploratory Research Methods
There are several types of exploratory research, including:
Literature Review
This involves conducting a comprehensive review of existing published research, scholarly articles, and other relevant literature on the research topic or problem. It helps to identify the gaps in the existing knowledge and to develop new research questions or hypotheses.
Pilot Study
A pilot study is a small-scale preliminary study that helps the researcher to test research procedures, instruments, and data collection methods. This type of research can be useful in identifying any potential problems or issues with the research design and refining the research procedures for a larger-scale study.
This involves an in-depth analysis of a particular case or situation to gain insights into the underlying causes, processes, and dynamics of the issue under investigation. It can be used to develop a more comprehensive understanding of a complex problem, and to identify potential research questions or hypotheses.
Focus Groups
Focus groups involve a group discussion that is conducted to gather opinions, attitudes, and perceptions from a small group of individuals about a particular topic. This type of research can be useful in exploring the range of opinions and attitudes towards a topic, identifying common themes or patterns, and generating ideas for further research.
Expert Opinion
This involves consulting with experts or professionals in the field to gain their insights, expertise, and opinions on the research topic. This type of research can be useful in identifying the key issues and concerns related to the topic, and in generating ideas for further research.
Observational Research
Observational research involves gathering data by observing people, events, or phenomena in their natural settings to gain insights into behavior and interactions. This type of research can be useful in identifying patterns of behavior and interactions, and in generating hypotheses or research questions for further investigation.
Open-ended Surveys
Open-ended surveys allow respondents to provide detailed and unrestricted responses to questions, providing valuable insights into their attitudes, opinions, and perceptions. This type of research can be useful in identifying common themes or patterns, and in generating ideas for further research.
Data Analysis Methods
Exploratory Research Data Analysis Methods are as follows:
Content Analysis
This method involves analyzing text or other forms of data to identify common themes, patterns, and trends. It can be useful in identifying patterns in the data and developing hypotheses or research questions. For example, if the researcher is analyzing social media posts related to a particular topic, content analysis can help identify the most frequently used words, hashtags, and topics.
Thematic Analysis
This method involves identifying and analyzing patterns or themes in qualitative data such as interviews or focus groups. The researcher identifies recurring themes or patterns in the data and then categorizes them into different themes. This can be helpful in identifying common patterns or themes in the data and developing hypotheses or research questions. For example, a thematic analysis of interviews with healthcare professionals about patient care may identify themes related to communication, patient satisfaction, and quality of care.
Cluster Analysis
This method involves grouping data points into clusters based on their similarities or differences. It can be useful in identifying patterns in large datasets and grouping similar data points together. For example, if the researcher is analyzing customer data to identify different customer segments, cluster analysis can be used to group similar customers together based on their demographic, purchasing behavior, or preferences.
Network Analysis
This method involves analyzing the relationships and connections between data points. It can be useful in identifying patterns in complex datasets with many interrelated variables. For example, if the researcher is analyzing social network data, network analysis can help identify the most influential users and their connections to other users.
Grounded Theory
This method involves developing a theory or explanation based on the data collected during the exploratory research process. The researcher develops a theory or explanation that is grounded in the data, rather than relying on pre-existing theories or assumptions. This can be helpful in developing new theories or explanations that are supported by the data.
Applications of Exploratory Research
Exploratory research has many practical applications across various fields. Here are a few examples:
- Marketing Research : In marketing research, exploratory research can be used to identify consumer needs, preferences, and behavior. It can also help businesses understand market trends and identify new market opportunities.
- Product Development: In product development, exploratory research can be used to identify customer needs and preferences, as well as potential design flaws or issues. This can help companies improve their product offerings and develop new products that better meet customer needs.
- Social Science Research: In social science research, exploratory research can be used to identify new areas of study, as well as develop new theories and hypotheses. It can also be used to identify potential research methods and approaches.
- Healthcare Research : In healthcare research, exploratory research can be used to identify new treatments, therapies, and interventions. It can also be used to identify potential risk factors or causes of health problems.
- Education Research: In education research, exploratory research can be used to identify new teaching methods and approaches, as well as identify potential areas of study for further research. It can also be used to identify potential barriers to learning or achievement.
Examples of Exploratory Research
Here are some more examples of exploratory research from different fields:
- Social Science : A researcher wants to study the experience of being a refugee, but there is limited existing research on this topic. The researcher conducts exploratory research by conducting in-depth interviews with refugees to better understand their experiences, challenges, and needs.
- Healthcare : A medical researcher wants to identify potential risk factors for a rare disease but there is limited information available. The researcher conducts exploratory research by reviewing medical records and interviewing patients and their families to identify potential risk factors.
- Education : A teacher wants to develop a new teaching method to improve student engagement, but there is limited information on effective teaching methods. The teacher conducts exploratory research by reviewing existing literature and interviewing other teachers to identify potential approaches.
- Technology : A software developer wants to develop a new app, but is unsure about the features that users would find most useful. The developer conducts exploratory research by conducting surveys and focus groups to identify user preferences and needs.
- Environmental Science : An environmental scientist wants to study the impact of a new industrial plant on the surrounding environment, but there is limited existing research. The scientist conducts exploratory research by collecting and analyzing soil and water samples, and conducting interviews with residents to better understand the impact of the plant on the environment and the community.
How to Conduct Exploratory Research
Here are the general steps to conduct exploratory research:
- Define the research problem: Identify the research problem or question that you want to explore. Be clear about the objective and scope of the research.
- Review existing literature: Conduct a review of existing literature and research on the topic to identify what is already known and where gaps in knowledge exist.
- Determine the research design : Decide on the appropriate research design, which will depend on the nature of the research problem and the available resources. Common exploratory research designs include case studies, focus groups, interviews, and surveys.
- Collect data: Collect data using the chosen research design. This may involve conducting interviews, surveys, or observations, or collecting data from existing sources such as archives or databases.
- Analyze data: Analyze the data collected using appropriate qualitative or quantitative techniques. This may include coding and categorizing qualitative data, or running descriptive statistics on quantitative data.
- I nterpret and report findings: Interpret the findings of the analysis and report them in a way that is clear and understandable. The report should summarize the findings, discuss their implications, and make recommendations for further research or action.
- Iterate : If necessary, refine the research question and repeat the process of data collection and analysis to further explore the topic.
When to use Exploratory Research
Exploratory research is appropriate in situations where there is limited existing knowledge or understanding of a topic, and where the goal is to generate insights and ideas that can guide further research. Here are some specific situations where exploratory research may be particularly useful:
- New product development: When developing a new product, exploratory research can be used to identify consumer needs and preferences, as well as potential design flaws or issues.
- Emerging technologies: When exploring emerging technologies, exploratory research can be used to identify potential uses and applications, as well as potential challenges or limitations.
- Developing research hypotheses: When developing research hypotheses, exploratory research can be used to identify potential relationships or patterns that can be further explored through more rigorous research methods.
- Understanding complex phenomena: When trying to understand complex phenomena, such as human behavior or societal trends, exploratory research can be used to identify underlying patterns or factors that may be influencing the phenomenon.
- Developing research methods : When developing new research methods, exploratory research can be used to identify potential issues or limitations with existing methods, and to develop new methods that better capture the phenomena of interest.
Purpose of Exploratory Research
The purpose of exploratory research is to gain insights and understanding of a research problem or question where there is limited existing knowledge or understanding. The objective is to explore and generate ideas that can guide further research, rather than to test specific hypotheses or make definitive conclusions.
Exploratory research can be used to:
- Identify new research questions: Exploratory research can help to identify new research questions and areas of inquiry, by providing initial insights and understanding of a topic.
- Develop hypotheses: Exploratory research can help to develop hypotheses and testable propositions that can be further explored through more rigorous research methods.
- Identify patterns and trends : Exploratory research can help to identify patterns and trends in data, which can be used to guide further research or decision-making.
- Understand complex phenomena: Exploratory research can help to provide a deeper understanding of complex phenomena, such as human behavior or societal trends, by identifying underlying patterns or factors that may be influencing the phenomena.
- Generate ideas: Exploratory research can help to generate new ideas and insights that can be used to guide further research, innovation, or decision-making.
Characteristics of Exploratory Research
The following are the main characteristics of exploratory research:
- Flexible and open-ended : Exploratory research is characterized by its flexible and open-ended nature, which allows researchers to explore a wide range of ideas and perspectives without being constrained by specific research questions or hypotheses.
- Qualitative in nature : Exploratory research typically relies on qualitative methods, such as in-depth interviews, focus groups, or observation, to gather rich and detailed data on the research problem.
- Limited scope: Exploratory research is generally limited in scope, focusing on a specific research problem or question, rather than attempting to provide a comprehensive analysis of a broader phenomenon.
- Preliminary in nature : Exploratory research is preliminary in nature, providing initial insights and understanding of a research problem, rather than testing specific hypotheses or making definitive conclusions.
- I terative process : Exploratory research is often an iterative process, where the research design and methods may be refined and adjusted as new insights and understanding are gained.
- I nductive approach : Exploratory research typically takes an inductive approach to data analysis, seeking to identify patterns and relationships in the data that can guide further research or hypothesis development.
Advantages of Exploratory Research
The following are some advantages of exploratory research:
- Provides initial insights: Exploratory research is useful for providing initial insights and understanding of a research problem or question where there is limited existing knowledge or understanding. It can help to identify patterns, relationships, and potential hypotheses that can guide further research.
- Flexible and adaptable : Exploratory research is flexible and adaptable, allowing researchers to adjust their methods and approach as they gain new insights and understanding of the research problem.
- Qualitative methods : Exploratory research typically relies on qualitative methods, such as in-depth interviews, focus groups, and observation, which can provide rich and detailed data that is useful for gaining insights into complex phenomena.
- Cost-effective : Exploratory research is often less costly than other research methods, such as large-scale surveys or experiments. It is typically conducted on a smaller scale, using fewer resources and participants.
- Useful for hypothesis generation : Exploratory research can be useful for generating hypotheses and testable propositions that can be further explored through more rigorous research methods.
- Provides a foundation for further research: Exploratory research can provide a foundation for further research by identifying potential research questions and areas of inquiry, as well as providing initial insights and understanding of the research problem.
Limitations of Exploratory Research
The following are some limitations of exploratory research:
- Limited generalizability: Exploratory research is typically conducted on a small scale and uses non-random sampling techniques, which limits the generalizability of the findings to a broader population.
- Subjective nature: Exploratory research relies on qualitative methods and is therefore subject to researcher bias and interpretation. The findings may be influenced by the researcher’s own perceptions, beliefs, and assumptions.
- Lack of rigor: Exploratory research is often less rigorous than other research methods, such as experimental research, which can limit the validity and reliability of the findings.
- Limited ability to test hypotheses: Exploratory research is not designed to test specific hypotheses, but rather to generate initial insights and understanding of a research problem. It may not be suitable for testing well-defined research questions or hypotheses.
- Time-consuming : Exploratory research can be time-consuming and resource-intensive, particularly if the researcher needs to gather data from multiple sources or conduct multiple rounds of data collection.
- Difficulty in interpretation: The open-ended nature of exploratory research can make it difficult to interpret the findings, particularly if the researcher is unable to identify clear patterns or relationships in the data.
About the author
Muhammad Hassan
Researcher, Academic Writer, Web developer
You may also like

Questionnaire – Definition, Types, and Examples

Case Study – Methods, Examples and Guide

Observational Research – Methods and Guide

Quantitative Research – Methods, Types and...

Qualitative Research Methods

Explanatory Research – Types, Methods, Guide
Publications per year
Exploratory Data Analysis. Data is big. We dig it.
How can we discover novel insights from data? How can we learn inherently interpretable models? How can we draw reliable causal conclusions?
That's exactly what we develop theory and algorithms for.
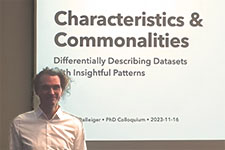
Sebastian Dalleiger is now a Doctor of Natural Sciences
Thursday, November 16th, 2023, Sebastian Dalleiger succesfully defended his Ph.D. thesis titled 'Characteristics and Commonalities – Differentially Describing Datasets with Insightful Patterns'. The promotion committee, consisting of Profs. Thomas Gaertner, Gerhard Weikum, Sven Rahmann, and Jilles Vreeken, were impressed with the thesis, presentation, and discussion and decided that Sebastian passed the requirements for a degree of Doctor of Natural Sciences with the distinction Magna Cum Laude . Congratulations, Dr. rer. nat. Dalleiger!
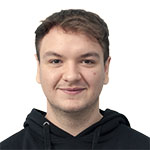
Nils starts as a PhD student
Warm welcome to Nils Walter as a new PhD student in the EDA group! Nils recently finished his MSc thesis at DFKI while working with us as a HiWi. He now joins EDA to pursue his PhD. Nils is very broadly interested in explainable, interpretable, exploratory and predictive methods. Welcome, Nils!
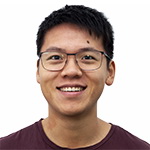
Sascha starts as a PhD student
Warm welcome to Sascha Xu as a new PhD student in the EDA group! Sascha recently finished his MSc thesis at DFKI while working with us as a HiWi. He now joins EDA to pursue his PhD. Last year he already presented a paper at ICML. For his PhD he will probably continue his exploration of the wonderful world of causation and interpretability. Welcome, Sascha!
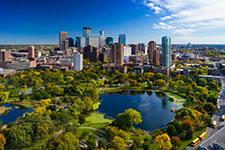
CueMin to be presented at SDM 2023
Boris will present his paper on learning interpretable models for predicting waiting and sojourn times accepted for presentation at the 2023 SIAM International Conference on Data Mining (SDM). In this paper, he proposes the MDL-based Cuemin algorithm that automatically determines the type and parameterization of the queing behaviour in the observed data. Extensive experiments show that Cuemin is not only more generally applicable, but that it performs on par with specialized solutions that require much more knowledge, as well as generalizes and extrapolates better than the state of the art. Congratulations Boris!
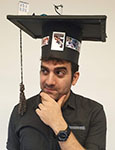
Janis Kalofolias is now a Doctor of Natural Sciences
Thursday, December 8th, 2022, Janis Kalofolias succesfully defended his Ph.D. thesis titled 'Subgroup Discovery for Structured Targets'. The promotion committee, consisting of Profs. Raimund Seidel, Gerhard Weikum, Peter Flach, and Jilles Vreeken, were impressed with the thesis, presentation, and discussion and decided that Janis passed the requirements for a degree of Doctor of Natural Sciences with the distinction Magna Cum Laude . Congratulations, Dr. rer. nat. Kalofolias!
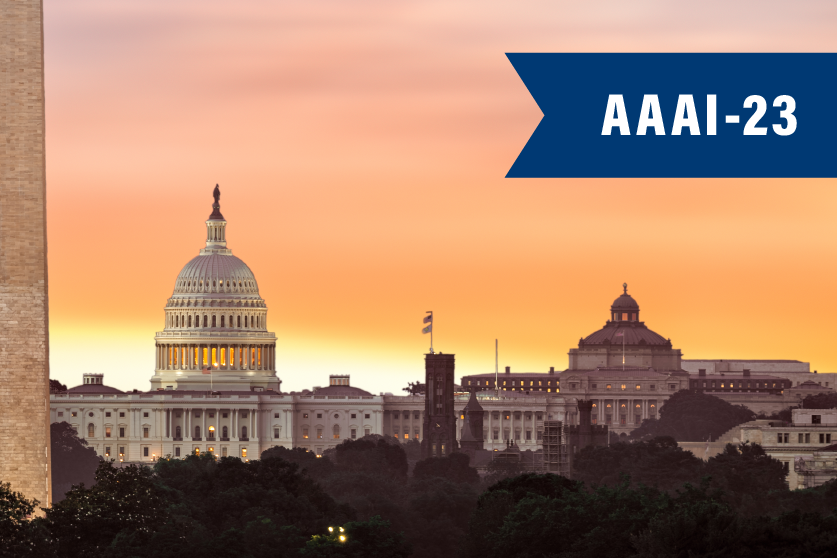
Two papers at AAAI 2023
David and Osman had their papers accepted for presentation at the 2023 AAAI International Conference on Artificial Intelligence (AAAI). David will present theory and methods for identifying whether a dataset has been subject to selection bias that if we disregard this could thwarth our causal analysis. Osman will present Orion for identifying directed causal graphs as well as interventions thereupon from data drawn from multiple enviroments. Congratulations to both!
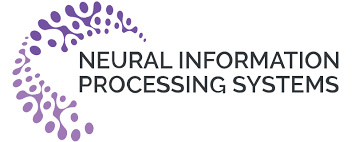
elBMF to be presented at NeurIPS 2022
Sebastian will present elBMF , a novel and highly scalable approach to Boolean matrix factorization at NeurIPS 2022 in New Orleans. The secret ingredient elBMF is that it uses continuous rather than combinatorial optimization. To ensure that the results are Boolean, Sebastian introduces an elastic-net-like regularizer, which has the benefit that no post-processing (Booleanification) of the results are necessar. You can find the paper and implementation here . Congratulations Sebastian!
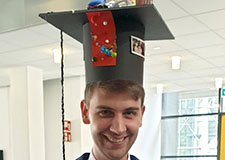
Jonas Fischer is now a Doctor of Natural Sciences
Thursday, July 28th, 2022, Jonas Fischer succesfully defended his Ph.D. thesis titled 'More than the Sum of its Parts — Pattern Mining, Neural Networks, and How They Complement Each Other'. The promotion committee, consisting of Profs. Sven Rahmann, Gerhard Weikum, Srinivasan Parthasararthy, and Jilles Vreeken, were deeply impressed with the thesis, presentation, and discussion and decided that Jonas therewith passed the requirements for a degree of Doctor of Natural Sciences with the distinction Summa Cum Laude . Congratulations, Dr. rer. nat. Fischer!
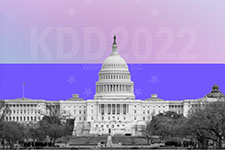
Two papers at KDD 2022
EDA will present two papers at the ACM International Conference on Knowledge Discovery and Data Mining Learning (KDD). Sarah and David will present Vario for discovering which environments share an invariant mechanism, for determining what those mechanisms are, what the causal parents are, and how to use \(\pi\)-invariance for causal discovery over multiple environments. Sebastian will have a lot of fun presenting Spass , which is a novel approach to discovering patterns that are significantly associated with one or multiple class labels, while controlling for multiple-hypothesis testing using either FDR or FWER. Congratulations to all!
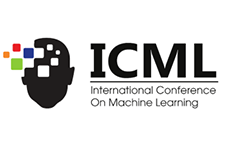
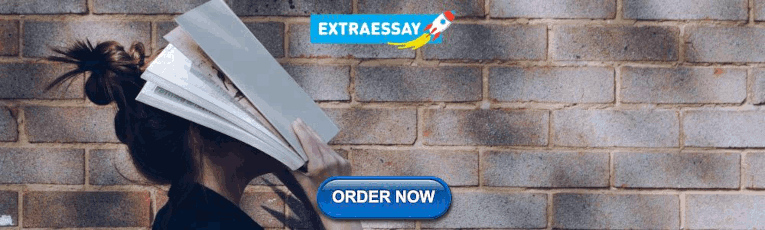
Two papers at ICML 2022
EDA will present two papers at the International Conference on Machine Learning (ICML). Sascha will present Heci which is an effective method for determining cause from effect when noise is heteroscedastic. Together with Osman and Alex he proposed a causal model that permits non-stationary noise, determined the conditions under which it is identifiable, and give an effective algorithm based on dynamic programming. Jonas and Michael will present Premise for characterizing when an arbitrary complex classifier goes wrong in easily interpretable terms. As they show, the patterns they find are not only insightful but also actionable, allowing to improve classifiers by targeted fine-tuning. Congratulations to all!
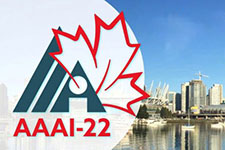
Three papers at AAAI 2022
Great success for the EDA group — three papers accepted for presentation at the 2022 AAAI International Conference on Artificial Intelligence (AAAI). Boris will present Consequence for mining J interpretable data-to-sequence generators. Corinna and Sebastian will present Gragra for describing what is common and what is different between groups of graphs. Janis will present Nuts for kernelized subgroup discovery, or, more technically, naming the most anomalous cluster in Hilbert Space for structures with attribute information. Congratulations to all!
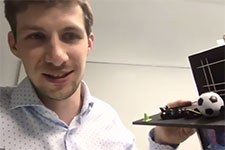
Alexander Marx is now a Doctor of Natural Sciences
Tuesday June 29th 2021, Alexander Marx succesfully defended his Ph.D. thesis titled 'Information-Theoretic Causal Discovery'. The promotion committee, consisting of Profs. Isabel Valera, Gerhard Weikum, Thijs van Ommen, and Jilles Vreeken, were impressed with the thesis, presentation, and discussion and decided he passed the requirements for a degree of Doctor of Natural Sciences with the distinction Magna Cum Laude . Congratulations, Dr. rer. nat. Marx!
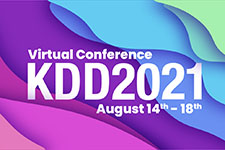
Four papers at KDD, ICML, and UAI 2021
Three conferences, four papers: at ICML, Jonas and Anna will present ExplaiNN for exploring how information is encoded within, and flows through, a deep convolutional neural network. At KDD, Jonas will present BinaPs for mining high-quality pattern sets from high dimensional data using a special binarized auto-encoder. Corinna will present Momo for describing the similarity between two (partially aligned) graphs in easily understandable terms. Alex will present his work with Joris Mooij and Arthur Gretton on the more realistic 2-adjacency faithfulness assumption at UAI. Congratulations to all!
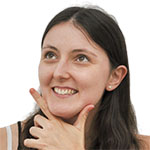
Jana makes Causal Discovery more Realistic
It is impossible to draw causal conclusions from data alone; we also need to make assumptions on the data generating process. Faithfulness is the assumption that if there exists a dependency between two variables in the process, these two variables are also dependent in the data. Jana shows that XOR-like dependencies, which are of great interest in biological applications, are hence not detectable by any algorithm that assumes faithfulness! To save the day, she shows how we can discover Markov blankets and causal networks under the more realistic assumption of 2-adjacency faithfulness, which allow her to discover XOR-like dependencies in biological data that existing algorithms miss. Congratulations, Jana!
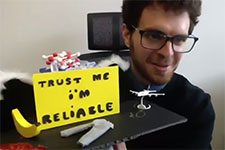
Panagiotis Mandros is now a Doctor of Engineering
On Thursday March 4th, Panagiotis Mandros succesfully defended his Ph.D. thesis titled 'Discovering Robust Dependencies from Data'. The promotion committee consisting of Profs. Dietrich Klakow, Gerhard Weikum, Geoff Webb, and Jilles Vreeken, decided that he not only passed the requirements for a degree of Doctor of Engineering but also awarded his thesis with the distinction Summa Cum Laude . Congratulations, Dr.-Ing. Mandros!
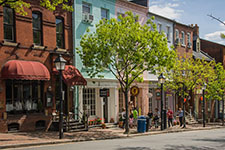
Four papers accepted for presentation at SDM 2021
Great success for the EDA group: we got four papers accepted for presentation at the 2021 SIAM International Conference on Data Mining (SDM). Alex will present his joint work with Lincen Yang on estimating conditional mutual information for discrete-continuous mixtures. Boris will present ProSeqo for mining concise yet powerful models from event sequence data. Janis will present Susan , the structural similarity random walk kernel that he developed together with Pascal Welke. Last, but not least, Kailash will present Dice for mining reliable causal rules. Congratulations to all!
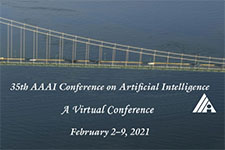
Globe to be presented at AAAI 2021
Osman and Alex will present their work on discovering fully oriented causal networks at next year's AAAI, the International Conference on Artificial Intelligence. In their work, they propose a score-based causal discovery algorithm that builds upon the algorithmic Markov condition to automatically orient all edges in the most likely causal direction. The Globe algorithm is remarkably robust and outperforms state-of-the-art score and constraint based solutions.
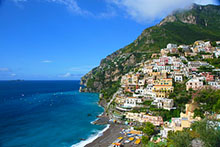
Two papers accepted for presentation at IEEE ICDM 2020
Joscha and Sebastian will present two papers at this year's IEEE International Conference on Data Mining. Joscha's will present Omen for discovering patterns that do not only predict that something of interest will happen, but are also reliable in telling when this will be. Sebastian proposes Reaper , a new relaxed formulation of the Maximum Entropy distribution that, through dynamic factorizations, is as accurrate yet orders of magnitudes faster than traditional approaches – so enabling principled discovery of highly informative patterns from much larger and much more complex data than ever before.
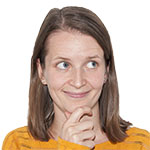
Anna explores the secret life of neural networks
With ExplaiNN , Anna Oláh proposes a highly scalable method that provides deep insight into the black box that neural networks are. In her Master thesis, Anna proposes to mine activation patterns between neurons in different layers in the form of robust rules. Not only does she propose an efficient and highly scalable algorithm, she also shows how we can use these rules to gain insight beyond the state of the art, both in what drives decisions for individual classes, as well as the differences between. Who knew, that in the eye of a neural network, Malamutes are essentially fluffy Husky's, and Husky's are essentially sharply drawn Malamutes! Congratulations, Anna!
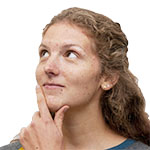
Edith shows us what we didn't know yet
In her Master thesis, Edith Heiter studies the problem of how to factor out prior knowledge from low-dimensional embeddings. In other words, how can we visualise a high dimensional dataset, such that we reveal structure that goes beyond what we already knew? In her thesis, Edith proposes not one, but two methods to factor out arbitrary distance matrices. With Jedi she proposes to adapt the objective function of t-SNE in a well-founded manner, while with Confetti she proposes a method that allows us to factor out knowledge from arbitrary embedding algorithms. Through many experiments, she showed that both work well in practice, earning her the title Master of Science. Congratulations, Edith!
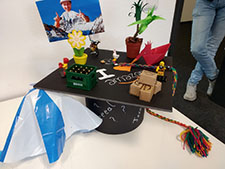
Kailash Budhathoki is now a Doctor of Natural Sciences
On Monday July 3rd, Kailash Budhathoki succesfully defended his Ph.D. thesis titled 'Causal Inference on Discrete Data'. The promotion committee consisting of Profs. Dietrich Klakow, Gerhard Weikum, Tom Heskes, and Jilles Vreeken, decided that he not only passed the requirements for a degree of Doctor of Philosophy of the Natural Sciences (Dr.rer.nat.) but also awarded his thesis with the distinction Summa Cum Laude . Congratulations, Dr. Budhathoki!
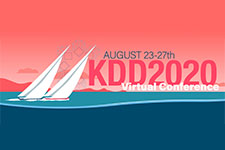
Three papers accepted at ACM SIGKDD 2020
EDA will present three papers at ACM SIGKDD 2020, the flagship conference in data mining. Jonas will present his work on discovering patterns of mutual exclusivity, in which he proposed the Mexican algorithm. Panagiotis will present his work together with Frederic Penerath on how to use smoothing to measure and mine reliable functional dependencies, as well as work together with David on how to discover functional dependencies from mixed-type data.
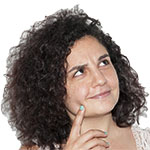
Sandra lays an opinion-spam trap
How can we detect review spam campaigns, the colluding groups of spammers, as well as determine the spamicity of individual reviewers that actively try to hide their spamming behaviour? In her Master thesis, Sandra Sukarieh answers all three questions. The main premise is that a campaign requires multiple users and abnormal scores. Sprap identifies users that surprisingly often review products together with other users that surprisingly often score differently from the norm. Experiments show her method works remarkably well in practice, without even having to consider the content of the reviews. In other words, Sandra's Master thesis campaign was a great success. Congratulations!
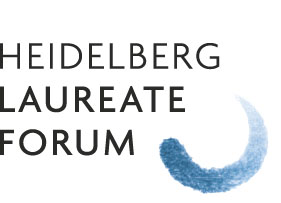
Alex invited to the Heidelberg Laureate Forum
Alexander Marx has been invited to attend the Heidelberg Laureate Forum . While the actual event is postponed to next year due to Corona, he will then get to meet laureates of the most prestiguous awards in Mathematics and Computer Science, such as Turing Award winners Manuel Blum, Vinton Cerf, Richard Karp, and Judea Pearl, as well as 199 other highly talented young scientists.
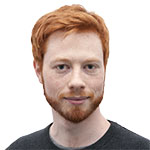
Joscha joins EDA as a PhD student
Warm welcome to Joscha Cueppers as a PhD student in the EDA group! Joscha recently finished his MSc thesis with us, and now joins to pursue his PhD. He'll be working on statistically well-founded pattern discovery from structured data, such as sequences and graphs, to gain insight in the causal processes that generated this data. Welcome, Joscha!
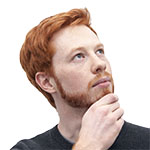
Joscha bakes a Cake
In his Master thesis, Joscha Cueppers considers the problem of discovering patterns that reliably predict future events. That is, he is interested in discovering sequential patterns from an event sequence \(X\) for which with high accuracy how long it will take until we see an interesting event happening in event sequence \(Y\). He modelled the problem using MDL, and proposes the Cake algorithm to discover a small set of non-redundant patterns that together predict \(Y\) as well as possible given \(X\). The experiments show the results are very tasty. Congratulations, Joscha!
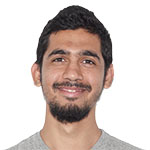
Osman joins EDA as a PhD student
Warm welcome to Osman Ali Mian as a PhD student in the EDA group! Osman recently finished his MSc thesis with us on the topic of discovering fully directed causal networks, and now joins to pursue his PhD. He'll be working on theory and methods for doing causal inference in realistic settings – e.g. methods that scale, can deal with data from multiple sources, can deal with missing data, and so on. Welcome, Osman!
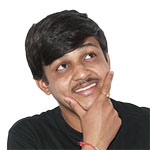
Divyam summarizes temporal graphs with Mango
Suppose we are given multiple snapshots of a graph over time, how can we discover patterns of change and similarity between them? Divyam Saran proposed the MDL-based Mango algorithm to discover succinct and non-redundant summaries that give clear insight in what is happening between the graphs. In a nutshell, he discovers significant structure per graph, and then uses the structures from adjacent graphs to refine the overall temporal summary – identifying growing, shrinking, and changing structures such as cliques, stars, and bi-partite subgraphs. Congratulations, Divyam!
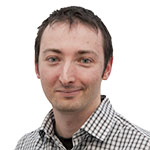
Boris joins EDA as a PhD student
We warmly welcome Boris Wiegand as a PhD student in the EDA group! Boris is employed by the Dillinger steel works, and will work on topics related to extracting high-quality models from production logs – for example, to gain insight in patterns, bottlenecks, as well as to optimize both planning and production. He will be co-supervised by Jilles Vreeken and Dietrich Klakow. Welcome, Boris!
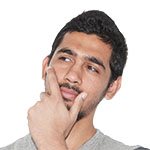
Osman trots the Globe
How can we discover fully oriented causal networks from observational data? In this Master thesis, Osman Ali Mian shows how we can use the Algorithmic Markov Condition to not only discover high quality causal skeletons, but at the same time orient all the edges from cause to effect. To find such networks from data, he proposes Globe , which instantiates the ideal using MDL and non-parametric multivariate regression splines. The experiments show that his proposal outperforms the state of the art constraint-based as well as score-based methods. Congratulations, Osman!
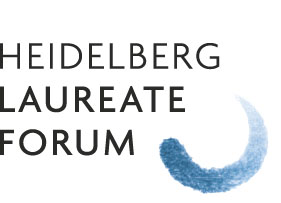
Panagiotis invited to the Heidelberg Laureate Forum
Panagiotis has been invited to attend the Heidelberg Laureate Forum . During the 3rd week of September he will he will then get to meet laureates of the most prestiguous awards in Mathematics and Computer Science, such as Turing Award winners Manuel Blum, Vinton Cerf, Richard Karp, and Yoshua Bengio, as well as 199 other highly talented young scientists.
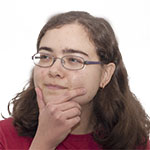
Simina shows there is more to it than a single answer
While almost all data analysis methods produce a single model, reality is more complex than that. How can we discover not one, but multiple high-quality explanations for a dataset, each of which show increasingly yet significantly more detail than the others? This is exactly the answer that Simina Ana Cotop answers in her Master thesis, in which she proposes the Grim algorithm that instantiates Kolmogorov's structure function for pattern-based summarization. Through many experiments she shows that Grim indeed returns insightful high level as well as detailed in-depth summaries. Congratulations, Simina!
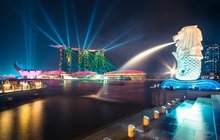
Panos, Mario and Jilles win the IEEE ICDM 2018 Best Paper Award
Out of 948 submissions, the award committee of IEEE ICDM 2018 selected our paper Discovering Reliable Dependencies from Data: Hardness and Improved Algorithms by Panagiotis Mandros , Mario Boley , and Jilles Vreeken for the IEEE ICDM 2018 Best Paper Award! We will receive the award in Singapore on November 19th. Hurray!
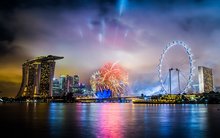
Jilles wins the IEEE ICDM Tao Li Award
The IEEE ICDM Tao Li Award recognizes excellent early career researchers for their impact on research contributions, impact, and services within the first ten years of their obtaining their PhD. This inaugural year, the award committee selected Jilles Vreeken for this honour — who is both deeply honoured, and uncharacteristically speechless.
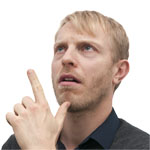
Mario starts Tenure Track at Monash University
While we're very sad that Mario Boley will leave us, we are very happy that on October 1st 2018 he will make the next step in his career and join Monash University in Melbourne, Australia as Tenure Track faculty. We wish Mario all the best, and are looking forward to continue working together on topics such as subgroup and functional dependency discovery. Congratulations, Mario!
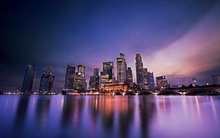
Two papers accepted for presentation at IEEE ICDM 2018
Kailash Budhathoki and Panagiotis Mandros will present two papers at IEEE ICDM 2018 in Singapore. Kailash will present his work on accurate causal inference on discrete data , in which he shows that by simply optimising the residual entropy we can accurately identify the most likely causal direction—with guarantees. Panagiotis will present his work on discovering reliable approximate functional dependencies , in which he shows that although this problem is NP-hard, using his optimistic estimator we can solve it exactly in reasonable time, as well as get extremely good solutions using a greedy strategy too.
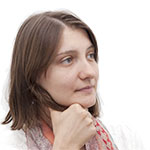
Iva gives guarantees and fast algorithms for mining patterns that overlap
Iva Farag was unhappy with the fact that Slim was restricted to using patterns without overlap, and looked into the theoretical details as well as the practical algorithmics for how to alleviate this. In her Master thesis, she shows that the problem is related to weighted set cover, and based on this proposes three cover algorithms that do allow overlap, two of which give guarantees on the quality of the solution. Experiments show that with GreCo we find more succinct, more insightful patterns that are less prone to fitting noise. Congratulations, Iva!
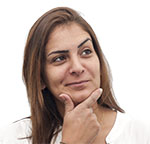
Maha smoothly smooths discrete data with Smoothie
With Smoothie , Maha Aburahma proposes a parameter-free algorithm for smoothing discrete data. In short, given a noisy transaction database, the algorithm makes local adjustments such that the overall MDL-complexity of the data and model is minimised. It does so step by step, providing a continuum of increasingly smoothened data. The MDL-optimum coincides with the optimal denoised data, which lends itself for pattern mining and knowledge discovery. Congratulations, Maha!
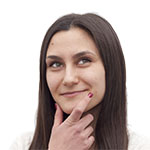
Yuliia proposes Grip for non-parametric dependency network reconstruction
For her Master thesis, Yuliia Brendel studied how we can recover the dependency network over a multivariate continuous-valued data set, without having to assume anything about the data distribution. She did so using the notion of cumulative entropy, and proposes the Grip algorithm to robustly estimate it for multivariate case. Experiments show that Grip performs very well even for highly non-linear, highly noisy, and high dimensional data and dependencies. Congratulations, Yuliia!
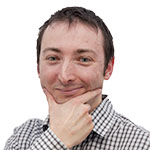
Boris predicts the wear and tear of rolling mills in a steel factory
During his studies, Boris Wiegand worked at the Dillinger steel plant, where among others they use specialized rolling mills to highly precisely turn chunks of red-hot steel into plates of specified thickness. These rolls in these mills undergo incredible temprature and pressure, and hence need to be replaced ever so often. The question is, when? In his Master thesis, Boris proposed a data-driven model that outperforms the industry-standard phsyics-based model, as well as how we can use this to optimize the milling schedule. Congratulations, Boris!
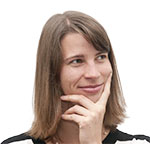
Maike shows how to reverse engineer epidemics in weighted graphs
In her Master thesis, Maike Eissfeller considered the problem of how to identify which nodes were most likely responsible for starting an epidemic in a large, weighted graph. She build upon the NetSleuth algorithm, and showed how to extend the theory to weighted graphs, how to make it more robust against the non-convex score, and how to improve its results by local re-optimization. Congratulations, Maike!
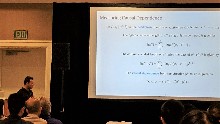
Kailash explains how to be Cute at SDM
Given two discrete valued time series can we tell whether they are causally related? That is, can we tell whether \(x\) causes \(y\), or whether \(y\) causes \(x\)? In the paper he presented on May 3rd at the SIAM Data Mining Conference, Kailash shows we can do so accurately, efficiently, and without having to make assumptions on the distribution of these time series, or about the lag of the causal effect. You can find the paper and implementation here .
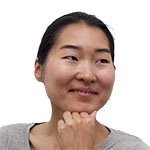
Tatiana shows how to robustly discretizing multivariate data
Tatiana Dembelova received her Master of Science degree for her thesis on how to how to discretize multivariate data such that we maintain the most important interactions between the attributes. In particular, she showed that existing work based on interaction distances performs less well than desired, and proposed a new approach based on footprint interactions that is highly robust against noise and the curse of dimensionality both in theory and in practice. Congratulations, Tatiana!
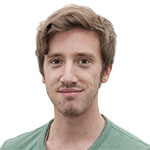
Robin introduces the Fire approach to discover interesting patterns
Robin Burghartz received his Master of Science degree for his thesis on how to identify interesting non-redundant pattern sets through the use of adaptive codes. Loosely speaking, he showed that when describing a row of data, if we adaptively only consider those patterns we know we can possibly use, instead of all, we can identify those patterns that stand out strongly from those already selected are chosen, leading to much smaller and much less redundant pattern sets. Congratulations, Robin!
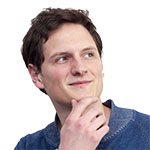
Henrik presents Explore to efficiently discover powerlaw communities
Henrik Jilke presented his Master thesis on the efficient discovery of powerlaw-distributed communities in large graphs. He proposed a lossless score based on the Minimum Descrtipion Length principle to identify whether a subgraph stands out sufficiently to be considered a community, and gave the efficient Explore algorithm to heuristically discover the best set of such communities. Experiments validate his method is able to discover large, powerlaw-distributed communities that other methods miss. Congratulations, Henrik!
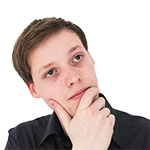
Benjamin proposes to automatically Refine ontologies for a specific corpus
Benjamin Hättasch finished his Master of Science by handing in his thesis on the automatic refinement of ontologies using compression-based learning. In a nutshell, Benjamn shows how we can efficiently describe a given text using an ontology. His main result is the Refine algorithm, that iteratively refines the ontology such that we maximize the compression. The resulting ontologies are a much better representation of the text distribution, as well as allow him to identify the key topics of the text without supervision. Congratulations, Benjamin!
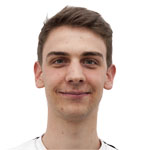
Jonas receives IMPRS-CS PhD Fellowship
We are happy and proud to announce that Jonas Fischer got accepted as a PhD student in the International Max Planck Research School for Computer Science (IMPRS-CS) to pursue a PhD on the topic of algorithmic data analysis. He was already a student in the Saarbrücken Graduate School of Computer Science, and recently finished his Master thesis in Bioinformatics on the topic of highly efficient methylation calling.
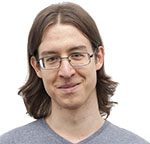
David receives IMPRS-CS PhD Fellowship
We are excited to announce that David Kaltenpoth got accepted as a PhD student in the International Max Planck Research School for Computer Science (IMPRS-CS). He was already a member of the Saarbrücken Graduate School of Computer Science. He will work on the topic of information theoretic causal inference, in particular the theory and practice of determining whether potential causal dependencies are confounded.
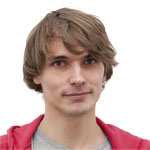
Sebastian joins EDA as a PhD student
We warmly welcome Sebastian Dalleiger as a PhD student in the Exploratory Data Analysis group. Sebastian finished his Master's in Informatics at Saarland University in 2016, and will now join our group to work on information theoretic approaches to mining interpretable and useful structure from data.
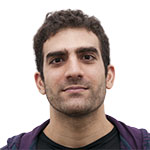
Janis joins EDA as a PhD student
We warmly welcome Janis Kalofolias as a PhD student in the Exploratory Data Analysis group. Janis recently finished his Master's in Informatics at Saarland University, and will now join our group to work on the theoretical foundations of mining interesting patterns from data.
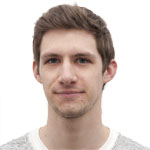
Alex receives IMPRS-CS PhD Fellowship
We are happy to announce that Alexander Marx got accepted as a PhD student in the International Max Planck Research School for Computer Science (IMPRS-CS) and the Saarbrücken Graduate School of Computer Science! He will work on the efficient discovery and interpretable description of interesting sub-populations in data, with the grand goals of discovering causal dependencies that lead to the discovery of novel materials.
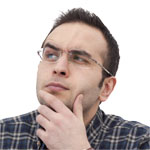
Amir proposes BVCorr to discover non-linearly correlated segments
Amirhossein Baradaranshahroudi finished his Master of Science by handing in his thesis on fast discovery of non-linearly correlated segments in multivariate time series. In his thesis, Amir shows that through fast-fourier transformation, convolution, and pre-computation we can bring down the computational complexity of computing the distance correlation between all pairwise windows in \(O(n^4 \log n)\) instead of \(O(n^5 d)\). For discovery in long time series, he proposes an effective and efficient heuristic that only takes \(O(nwd)\) time. Congratulations, Amir!
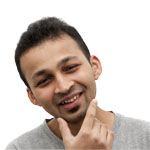
Apratim shows how to Squish event sequences
Apratim Bhattacharyya finished his Master of Science by handing in his thesis 'Squish: Efficiently Summarising Event Sequences with Rich and Interleaving Patterns' . Squish improves over the state of the art by considering a much richer description language, allowing both nesting and interleaving of patterns, as well as both variances and partial occurrences of patterns. Moreover, Squish is not only orders of magnitude faster than the state of the art, experiments show it also discovers much better and more easily interpretable models. Congratulations, Apratim!
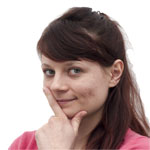
Beata untangles a pile of spaghetti
Beata Wójciak handed in her thesis 'Spaghetti: Finding Storylines in Large Collections of Documents' on the 29th of September, and so fullfilled the requirements to become a Master of Science in Informatics. In her thesis, Beata studied the problem of making sense from large, time-stamped, collections of documents, and proposed the efficient Spaghetti algorithm to discover the pattern storylines in a corpus. This allows us to draw a map showing which documents are connected, as well as easily interpret the storylines. Congratulations, Beatka!
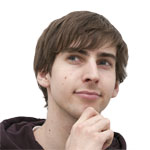
Magnus combines sketching and Slim into Skim
For this Bachelor thesis, Magnus Halbe studied whether sketching can speed up Slim . In particular, he investigated whether DHP and min-hashing can used to reliably and efficiently identify co-occurring patterns. In this thesis, titled ' Skim : Alternative Candidate Selections for Slim through Sketching' , Magnus shows that the answer is 'not really.' . Whereas the sketches ably identify heavy hitters, they are less efficient in identifying more subtle patterns. He therefore proposes the Skim algorithm, that combines the best of both worlds. Congratulations, Magnus!
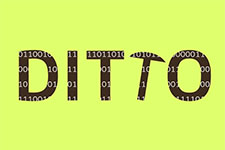
Roel presents Ditto at KDD
During the summer of 2014 Roel Bertens did an internship in our group. He presented the resulting paper, ` Keeping it Short and Simple ' at ACM SIGKDD 2016. Together with Arno Siebes we studied the problem of finding summaries of complex event sequences in terms of patterns that span over multiple attributes and which may include gaps. We propose the Ditto algorithm, to reliably and efficiently discover succinct and non-redundant models from multivariate event sequences. We give a short explanation, without kittens, on YouTube .
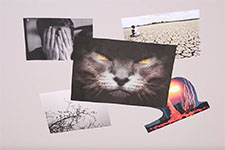
Polina presents CulT at KDD
Last summer, Polina Rozenshtein did an internship in our group. She presented the resulting paper ` Reconstructing an Epidemic over Time ' at ACM SIGKDD 2016. Together with B. Aditya Prakash and Aris Gionis we studied the problem of finding the seed nodes of an epidemic, if we are given an interaction graph, and a sparse and noisy sample of node states over time. We propose the CulT (Culprits in Time) algorithm, that reliably, efficiently, and without making any assumptions on the viral process can recover both the number and location of the original seed nodes. We give a short explanation, with kittens, on YouTube .
Kailash invited to the Heidelberg Laureate Forum
Kailash Budhathoki has been invited to attend the Heidelberg Laureate Forum . During the 18th and 23rd of September 2016, he will get to meet laureates of the most prestiguous awards in Mathematics and Computer Science, such as Turing Award winners Manuel Blum, Vinton Cerf, Richard Karp, and John Hopcroft, as well as 199 other highly talented young scientists.
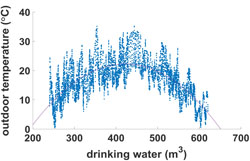
Panos and Jilles present Flexi , Light , and UdS at SIAM SDM
Panagiotis Mandros presented uds , which allows for Universal Dependency Analysis . That is, it is a robust and efficient measure for non-linear and multivariate correlations, which does not require any prior assumptions, yet does allow for meaningful comparison, no matter the cardinality or distribution of the subspace. Jilles Vreeken presented light , a linear-time method for detecting non-linear change points in massively high dimensional time series, and flexi , a highly flexible method for mining high quality subgroups through optimal discretisation, that works with virtually any quality measure.
Exploratory Data Analysis: A Growing Toolbox Illustrated by Long-Term Ecosystem Monitoring Data from the UK Environmental Change Network
Datasets are collected as part of designed experiments, observational studies, automatic recordings, or composed from other datasets. Exploratory data analysis (EDA) is an essential first phase that sets the scene for decided which downstream analyses would best address your research questions.
There is no universally applicable recipe for how EDA should be carried out because this highly depends on the structure of the data, the scientific context, and the research questions, but there is a broad agreement on its goals. In this talk we shed light on the evolving range of available tools considering the origin, structure, size, and quality of the data. The is particularly important prior to the application of machine learning techniques where raw data characteristics can have a large impact, potentially more than intended. We look EDA from a historical perspective focussing on its beginnings und the leadership of the father of data sciences, John Tukey. We also consider it from a modern lens addressing the requirements triggered by the use of data in conjunction with the AI (Artificial Intelligence) development. Furthermore, we discuss how AI could potentially be used to support this work.
Share this on:
Share this page on Facebook
Share this page on Twitter
Share this page on Email
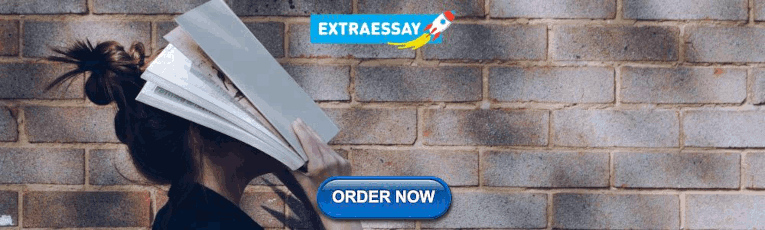
IMAGES
VIDEO
COMMENTS
Exploratory research data collection Collecting information on a previously unexplored topic can be challenging. Exploratory research can help you narrow down your topic and formulate a clear hypothesis and problem statement , as well as giving you the "lay of the land" on your topic.
15.1 Introduction. Exploratory data analysis (EDA) is an essential step in any research analysis. The. primary aim with exploratory analysis is to examine the data for distribution, outliers and ...
ing at numbers to be tedious, boring, and/or overwhelming. Exploratory data analysis techniques have been devised as an aid in this situation. Most of these techniques work in part by hiding certain aspects of the data while making other aspects more clear. Exploratory data analysis is generally cross-classi ed in two ways. First, each
Exploratory data analysis stems from the collection of work by the statistician John Tukey in the 1960s and 1970s [24,39,40,67]. His seminal book [67] compiles a collection of data visualization tech-niques as well as robust and non-parametric statistics for data explo-ration. Many communities including Statistics, Human-Computer In-
the data can inspire you to ask new questions, and it is foolish not to explore your hard-earned data. Exploratory data analysis (EDA; Tukey, 1977; Cleveland, 1993; Hoaglin et al., 2000, 2006) is a set of graphical techniques for finding interesting patterns in data. EDA was developed in the late 1970s when computer graphics first
Such critiques motivate our proposal that research on supporting exploratory visual analysis should embrace theories of graphical inference. In the following section we propose an alternative understanding of exploratory visual analysis as guided by model checks, and describe possible formalizations of this theory. 4.
Introduction. Exploratory Data Analysis (EDA) is the single most important task to conduct at the beginning of every data science project. In essence, it involves thoroughly examining and characterizing your data in order to find its underlying characteristics, possible anomalies, and hidden patterns and relationships.
The goal of this thesis is to apply Exploratory Data Analysis to the qualitative and quantitative dataset, to answer the research questions on the characteristics of the users, patterns of their behavior, and at the same time on the in uence of environmental factors. Multiple data mining models have been applied in the thesis, including
Exploratory Data Analysis (EDA) in the auditing domain. Chapter one introduces the motivation and methodology of this thesis and provides an extended literature review of the concept of EDA and its enabling techniques. The three essays are included in chapter two, three and four, respectively. The last chapter concludes the dissertation by
Exploratory Data Analysis (EDA) is an approach advocated by renowned statistician J. W. Tukey and others. It uses data visualization as applied to raw data or summarized information (Chapter 5) from a dataset to understand relationships within a dataset.It may be used to discover patterns which can then be tested using standard inferential statistics (Chapter 6) [].
In exploratory data analysis, attempts were made to identify the major features of a data set of interest and to generate ideas for further investigations (Cox, & Jones, 1981). For analyzing open ...
My major thesis is that educators do too little data exploration. They tend to dismiss exploratory methods as "merely descriptive." In this article, I hope to present exploratory research to the educa-tion community for a fresh consideration. John Tukey and Frederic Mosteller have recently pub-lished two books- Exploratory Data Analysis and
Research data management (RDM) is defined as, "the organisation of data, from its entry to the research cycle through to the dissemination and archiving of valuable results" (Whyte and Tedds 2011, 1), and borrowing from Tenopir et al (2015), "refers to the broad suite of services
illustrates a large drop-off in the amount of feedback from the first 10 reviews and the next 10. reviews. Both the amount of positive feedback and amount of total feedback decline over time. Furthermore, plotting the average rating by reviewers over time also shows that there exists.
Exploratory data analysis (EDA) is used by data scientists to analyze and investigate data sets and summarize their main characteristics, often employing data visualization methods. EDA helps determine how best to manipulate data sources to get the answers you need, making it easier for data scientists to discover patterns, spot anomalies, test ...
Exploratory factor analysis (EFA) is a multivariate statistical method that has become a fundamental tool in the development and validation of psychological theories and measurements. However, researchers must make several thoughtful and evidence-based methodological decisions while conducting an EFA, and there are a number of options available ...
Also, as it shown at the figure 19 with higher bullets is Segment 2 and Segment 4 with satisfaction level 1.92 and 2.54, respectively. Segment 2 and Segment 4 contain 8.3% and 8.4 % of the data, respectively, where the proportions are small but should be taken into consideration the impact on overall satisfaction.
Exploratory data analysis (EDA) is a well-established statistical tradition that provides conceptual and computational tools for discovering patterns to foster hypothesis development and refinement.
The researcher develops a theory or explanation that is grounded in the data, rather than relying on pre-existing theories or assumptions. This can be helpful in developing new theories or explanations that are supported by the data. Applications of Exploratory Research. Exploratory research has many practical applications across various fields.
The aim of this article was to present a comprehensive Exploratory Data Analysis template for time series forecasting. EDA is a fundamental step in any type of data science study since it allows to understand the nature and the peculiarities of the data and lays the foundation to feature engineering, which in turn can dramatically improve model ...
Exploratory Data Analaysis at CISPA Helmholtz Center for Information Security. Toggle navigation. X. Exploratory Data Analysis EDA. People; Teaching; Research ... Mandros, P Information-Theoretic Supervised Feature Selection for Continuous Data. M.Sc. Thesis, Saarland University, 2015. 2014. Miettinen, P & Vreeken, ...
Table 1.1. This step is necessary in clustering algorithms that work on a matrix of proximity or distance values instead of working on the original pattern set. It is then useful in such situations to precompute the. n(n 1) 2 pairwise distance values for the n patterns and store them in a symmetric matrix.
Statistical Analysis. This helps quantitatively assess the main characteristics of data: Mean: Calculated as the total sum of all numbers in the set divided by their count, reflecting the "average" point.; Median: The middle value of a dataset, or the average of the two middle values if the dataset size is even.; Mode: Indicates the number that appears most frequently in a dataset, serving ...
We warmly welcome Sebastian Dalleiger as a PhD student in the Exploratory Data Analysis group. Sebastian finished his Master's in Informatics at Saarland University in 2016, and will now join our group to work on information theoretic approaches to mining interpretable and useful structure from data. (2 Sep 2017)
The methodological focus of the current article is to demonstrate how an exploratory sequential approach can be used for complex intervention development by presenting and integrating the qualitative and quantitative findings from a series of four studies on the considerations for a tailored self-management program for individuals with spinal cord injury (SCI; Munce et al., 2014a; Munce et al ...
Exploratory data analysis (EDA) is an essential first phase that sets the scene for decided which downstream analyses would best address your research questions. ... The is particularly important prior to the application of machine learning techniques where raw data characteristics can have a large impact, potentially more than intended. ...