
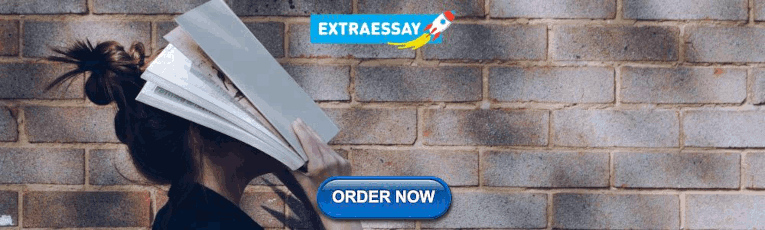
Unraveling Research Population and Sample: Understanding their role in statistical inference
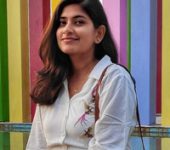
Research population and sample serve as the cornerstones of any scientific inquiry. They hold the power to unlock the mysteries hidden within data. Understanding the dynamics between the research population and sample is crucial for researchers. It ensures the validity, reliability, and generalizability of their findings. In this article, we uncover the profound role of the research population and sample, unveiling their differences and importance that reshapes our understanding of complex phenomena. Ultimately, this empowers researchers to make informed conclusions and drive meaningful advancements in our respective fields.
Table of Contents
What Is Population?
The research population, also known as the target population, refers to the entire group or set of individuals, objects, or events that possess specific characteristics and are of interest to the researcher. It represents the larger population from which a sample is drawn. The research population is defined based on the research objectives and the specific parameters or attributes under investigation. For example, in a study on the effects of a new drug, the research population would encompass all individuals who could potentially benefit from or be affected by the medication.
When Is Data Collection From a Population Preferred?
In certain scenarios where a comprehensive understanding of the entire group is required, it becomes necessary to collect data from a population. Here are a few situations when one prefers to collect data from a population:
1. Small or Accessible Population
When the research population is small or easily accessible, it may be feasible to collect data from the entire population. This is often the case in studies conducted within specific organizations, small communities, or well-defined groups where the population size is manageable.
2. Census or Complete Enumeration
In some cases, such as government surveys or official statistics, a census or complete enumeration of the population is necessary. This approach aims to gather data from every individual or entity within the population. This is typically done to ensure accurate representation and eliminate sampling errors.
3. Unique or Critical Characteristics
If the research focuses on a specific characteristic or trait that is rare and critical to the study, collecting data from the entire population may be necessary. This could be the case in studies related to rare diseases, endangered species, or specific genetic markers.
4. Legal or Regulatory Requirements
Certain legal or regulatory frameworks may require data collection from the entire population. For instance, government agencies might need comprehensive data on income levels, demographic characteristics, or healthcare utilization for policy-making or resource allocation purposes.
5. Precision or Accuracy Requirements
In situations where a high level of precision or accuracy is necessary, researchers may opt for population-level data collection. By doing so, they mitigate the potential for sampling error and obtain more reliable estimates of population parameters.
What Is a Sample?
A sample is a subset of the research population that is carefully selected to represent its characteristics. Researchers study this smaller, manageable group to draw inferences that they can generalize to the larger population. The selection of the sample must be conducted in a manner that ensures it accurately reflects the diversity and pertinent attributes of the research population. By studying a sample, researchers can gather data more efficiently and cost-effectively compared to studying the entire population. The findings from the sample are then extrapolated to make conclusions about the larger research population.
What Is Sampling and Why Is It Important?
Sampling refers to the process of selecting a sample from a larger group or population of interest in order to gather data and make inferences. The goal of sampling is to obtain a sample that is representative of the population, meaning that the sample accurately reflects the key attributes, variations, and proportions present in the population. By studying the sample, researchers can draw conclusions or make predictions about the larger population with a certain level of confidence.
Collecting data from a sample, rather than the entire population, offers several advantages and is often necessary due to practical constraints. Here are some reasons to collect data from a sample:
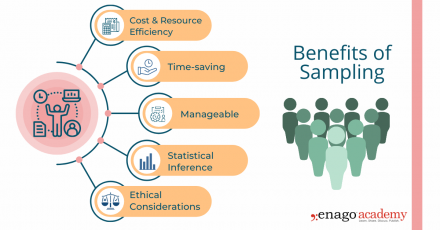
1. Cost and Resource Efficiency
Collecting data from an entire population can be expensive and time-consuming. Sampling allows researchers to gather information from a smaller subset of the population, reducing costs and resource requirements. It is often more practical and feasible to collect data from a sample, especially when the population size is large or geographically dispersed.
2. Time Constraints
Conducting research with a sample allows for quicker data collection and analysis compared to studying the entire population. It saves time by focusing efforts on a smaller group, enabling researchers to obtain results more efficiently. This is particularly beneficial in time-sensitive research projects or situations that necessitate prompt decision-making.
3. Manageable Data Collection
Working with a sample makes data collection more manageable . Researchers can concentrate their efforts on a smaller group, allowing for more detailed and thorough data collection methods. Furthermore, it is more convenient and reliable to store and conduct statistical analyses on smaller datasets. This also facilitates in-depth insights and a more comprehensive understanding of the research topic.
4. Statistical Inference
Collecting data from a well-selected and representative sample enables valid statistical inference. By using appropriate statistical techniques, researchers can generalize the findings from the sample to the larger population. This allows for meaningful inferences, predictions, and estimation of population parameters, thus providing insights beyond the specific individuals or elements in the sample.
5. Ethical Considerations
In certain cases, collecting data from an entire population may pose ethical challenges, such as invasion of privacy or burdening participants. Sampling helps protect the privacy and well-being of individuals by reducing the burden of data collection. It allows researchers to obtain valuable information while ensuring ethical standards are maintained .
Key Steps Involved in the Sampling Process
Sampling is a valuable tool in research; however, it is important to carefully consider the sampling method, sample size, and potential biases to ensure that the findings accurately represent the larger population and are valid for making conclusions and generalizations. While the specific steps may vary depending on the research context, here is a general outline of the sampling process:
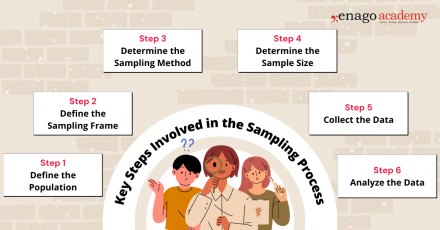
1. Define the Population
Clearly define the target population for your research study. The population should encompass the group of individuals, elements, or units that you want to draw conclusions about.
2. Define the Sampling Frame
Create a sampling frame, which is a list or representation of the individuals or elements in the target population. The sampling frame should be comprehensive and accurately reflect the population you want to study.
3. Determine the Sampling Method
Select an appropriate sampling method based on your research objectives, available resources, and the characteristics of the population. You can perform sampling by either utilizing probability-based or non-probability-based techniques. Common sampling methods include random sampling, stratified sampling, cluster sampling, and convenience sampling.
4. Determine Sample Size
Determine the desired sample size based on statistical considerations, such as the level of precision required, desired confidence level, and expected variability within the population. Larger sample sizes generally reduce sampling error but may be constrained by practical limitations.
5. Collect Data
Once the sample is selected using the appropriate technique, collect the necessary data according to the research design and data collection methods . Ensure that you use standardized and consistent data collection process that is also appropriate for your research objectives.
6. Analyze the Data
Perform the necessary statistical analyses on the collected data to derive meaningful insights. Use appropriate statistical techniques to make inferences, estimate population parameters, test hypotheses, or identify patterns and relationships within the data.
Population vs Sample — Differences and examples
While the population provides a comprehensive overview of the entire group under study, the sample, on the other hand, allows researchers to draw inferences and make generalizations about the population. Researchers should employ careful sampling techniques to ensure that the sample is representative and accurately reflects the characteristics and variability of the population.
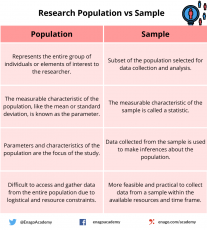
Research Study: Investigating the prevalence of stress among high school students in a specific city and its impact on academic performance.
Population: All high school students in a particular city
Sampling Frame: The sampling frame would involve obtaining a comprehensive list of all high schools in the specific city. A random selection of schools would be made from this list to ensure representation from different areas and demographics of the city.
Sample: Randomly selected 500 high school students from different schools in the city
The sample represents a subset of the entire population of high school students in the city.
Research Study: Assessing the effectiveness of a new medication in managing symptoms and improving quality of life in patients with the specific medical condition.
Population: Patients diagnosed with a specific medical condition
Sampling Frame: The sampling frame for this study would involve accessing medical records or databases that include information on patients diagnosed with the specific medical condition. Researchers would select a convenient sample of patients who meet the inclusion criteria from the sampling frame.
Sample: Convenient sample of 100 patients from a local clinic who meet the inclusion criteria for the study
The sample consists of patients from the larger population of individuals diagnosed with the medical condition.
Research Study: Investigating community perceptions of safety and satisfaction with local amenities in the neighborhood.
Population: Residents of a specific neighborhood
Sampling Frame: The sampling frame for this study would involve obtaining a list of residential addresses within the specific neighborhood. Various sources such as census data, voter registration records, or community databases offer the means to obtain this information. From the sampling frame, researchers would randomly select a cluster sample of households to ensure representation from different areas within the neighborhood.
Sample: Cluster sample of 50 households randomly selected from different blocks within the neighborhood
The sample represents a subset of the entire population of residents living in the neighborhood.
To summarize, sampling allows for cost-effective data collection, easier statistical analysis, and increased practicality compared to studying the entire population. However, despite these advantages, sampling is subject to various challenges. These challenges include sampling bias, non-response bias, and the potential for sampling errors.
To minimize bias and enhance the validity of research findings , researchers should employ appropriate sampling techniques, clearly define the population, establish a comprehensive sampling frame, and monitor the sampling process for potential biases. Validating findings by comparing them to known population characteristics can also help evaluate the generalizability of the results. Properly understanding and implementing sampling techniques ensure that research findings are accurate, reliable, and representative of the larger population. By carefully considering the choice of population and sample, researchers can draw meaningful conclusions and, consequently, make valuable contributions to their respective fields of study.
Now, it’s your turn! Take a moment to think about a research question that interests you. Consider the population that would be relevant to your inquiry. Who would you include in your sample? How would you go about selecting them? Reflecting on these aspects will help you appreciate the intricacies involved in designing a research study. Let us know about it in the comment section below or reach out to us using #AskEnago and tag @EnagoAcademy on Twitter , Facebook , and Quora .

Thank you very much, this is helpful
Very impressive and helpful and also easy to understand….. Thanks to the Author and Publisher….
Rate this article Cancel Reply
Your email address will not be published.
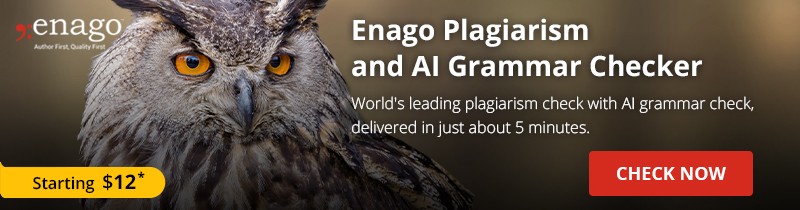
Enago Academy's Most Popular Articles
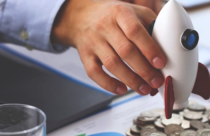
- Diversity and Inclusion
- Trending Now
The Silent Struggle: Confronting gender bias in science funding
In the 1990s, Dr. Katalin Kariko’s pioneering mRNA research seemed destined for obscurity, doomed by…
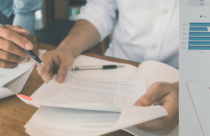
- Reporting Research
Choosing the Right Analytical Approach: Thematic analysis vs. content analysis for data interpretation
In research, choosing the right approach to understand data is crucial for deriving meaningful insights.…
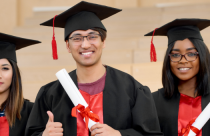
Addressing Barriers in Academia: Navigating unconscious biases in the Ph.D. journey
In the journey of academia, a Ph.D. marks a transitional phase, like that of a…
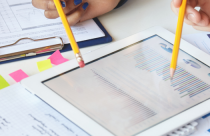
Comparing Cross Sectional and Longitudinal Studies: 5 steps for choosing the right approach
The process of choosing the right research design can put ourselves at the crossroads of…
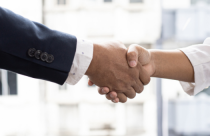
- Career Corner
Unlocking the Power of Networking in Academic Conferences
Embarking on your first academic conference experience? Fear not, we got you covered! Academic conferences…
Choosing the Right Analytical Approach: Thematic analysis vs. content analysis for…
Comparing Cross Sectional and Longitudinal Studies: 5 steps for choosing the right…
Research Recommendations – Guiding policy-makers for evidence-based decision making

Sign-up to read more
Subscribe for free to get unrestricted access to all our resources on research writing and academic publishing including:
- 2000+ blog articles
- 50+ Webinars
- 10+ Expert podcasts
- 50+ Infographics
- 10+ Checklists
- Research Guides
We hate spam too. We promise to protect your privacy and never spam you.
I am looking for Editing/ Proofreading services for my manuscript Tentative date of next journal submission:
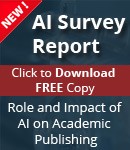
As a researcher, what do you consider most when choosing an image manipulation detector?
- Skip to main content
- Skip to primary sidebar
- Skip to footer
- QuestionPro

- Solutions Industries Gaming Automotive Sports and events Education Government Travel & Hospitality Financial Services Healthcare Cannabis Technology Use Case NPS+ Communities Audience Contactless surveys Mobile LivePolls Member Experience GDPR Positive People Science 360 Feedback Surveys
- Resources Blog eBooks Survey Templates Case Studies Training Help center

Home Market Research Research Tools and Apps
Study Population: Characteristics & Sampling Techniques
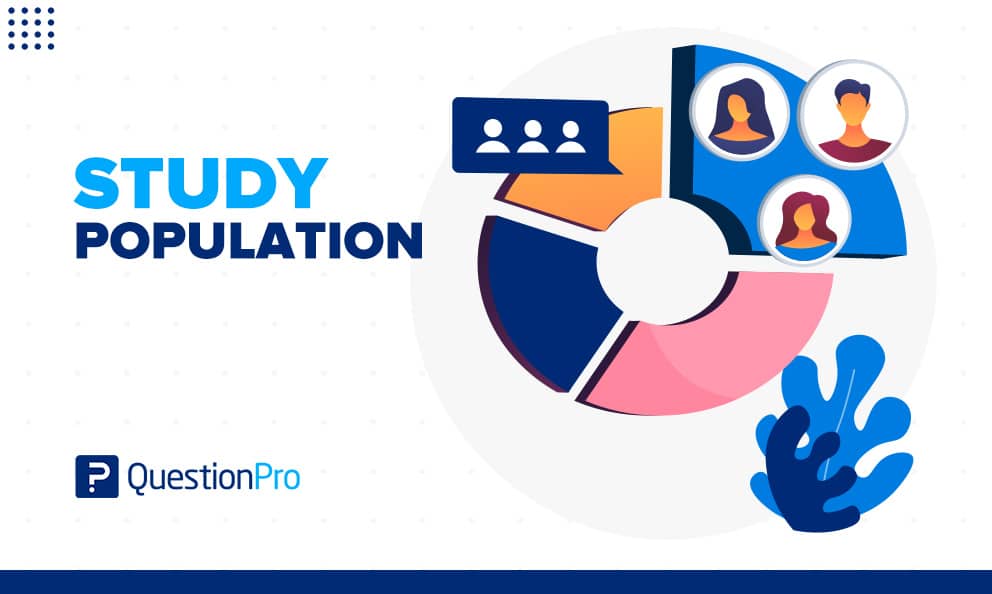
How do you define a study population? Research studies require specific groups to draw conclusions and make decisions based on their results. This group of interest is known as a sample. The method used to select respondents is known as sampling.
What is a Study Population?
A study population is a group considered for a study or statistical reasoning. The study population is not limited to the human population only. It is a set of aspects that have something in common. They can be objects, animals, measurements, etc., with many characteristics within a group.
For example, suppose you are interested in the average time a person between the ages of 30 and 35 takes to recover from a particular condition after consuming a specific type of medication. In that case, the study population will be all people between the ages of 30 and 35.
A medical study examines the spread of a specific disease in stray dogs in a city. Here, the stray dogs belonging to that city are the study population. This population or sample represents the entire population you want to conclude about.
How to establish a study population?
Sampling is a powerful technique for collecting opinions from a wide range of people, chosen from a particular group, to learn more about the whole group in general.
For any research study to be effective, it is necessary to select the study population that truly represents the entire population. Before starting your study, the target population must be identified and agreed upon. By appointing and knowing your sample well in advance, any feedback deemed useless to the study will be largely eliminated.
If your survey aims to understand a product’s or service’s effectiveness, then the study population should be the customers who have used it or are best suited to their needs and who will use the product/service.
It would be costly and time-consuming to collect data from the entire population of your target market. By accurately sampling your study population, it is possible to build a true picture of the target market using the trends in the results.
LEARN ABOUT: Survey Sampling
Choosing an accurate sample from the study population
The decision on an appropriate sample depends on several key factors.
- First, you decide which population parameters you want to estimate.
- Don’t expect estimates from a sample to be exact. Always expect a margin of error when making assumptions based on the results of a sample.
- Understanding the cost of sampling helps us determine how precise our estimates need to be.
- Know how variable the population you want to measure is. It is not necessary to assume that a large sample is required if the study population is large.
- Take into account the response rate of your population. A 20% response rate is considered “good” for an online research study.
Sampling characteristics in the study population
- Sampling is a mechanism to collect data without surveying the entire target population.
- The study population is the entire unit of people you consider for your research. A sample is a subset of this group that represents the population.
- Sampling reduces survey fatigue as it is used to prevent pollsters from conducting too many surveys, thereby increasing response rates.
- Also, it is much cheaper and saves more time than measuring the entire group.
- Tracking the response rate patterns of different groups will help determine how many respondents to select.
- The study is not only limited to the selected part, but is applied to the entire target population.
Sampling techniques for your study population
Now that you understand that you cannot survey the entire study population due to various factors, you should adopt one of the sample selection methodologies that best suits your research study.
In general terms, two methodologies can be applied: probability sampling and non-probability sampling .
Sampling Techniques: Probability Sampling
This method is used to select sample objects from a population based on probability theory. Everyone is included in the sample and has an equal chance of being selected. There is no bias in this type of sample. Every person in the population has the opportunity to be part of the research.
Probability sampling can be categorized into four types:
- Simple Random Sampling : Simple random sampling is the easiest way to select a sample. Here, each member has an equal chance of being part of the sample. The objects in this sample are chosen at random, and each member has exactly the same probability of being selected.
- Cluster sampling : Cluster sampling is a method in which respondents are grouped into clusters. These groups can be defined based on age, gender, location, and demographic parameters.
- Systematic Sampling : In systematic sampling, individuals are chosen at equal intervals from the population. A starting point is selected, and then respondents are chosen at predefined sample intervals.
- Stratified Sampling: S tratified random sampling is a process of dividing respondents into distinct but predefined parameters. In this method, respondents do not overlap but collectively represent the entire population.
Sampling techniques: Non-probabilistic sampling
The non-probability sampling method uses the researcher’s preference regarding sample selection bias . This sampling method derives primarily from the researcher’s ability to access this sample. Here the population members do not have the same opportunities to be part of the sample.
Non-probability sampling can be further classified into four distinct types:
- Convenience Sampling: As the name implies, convenience sampling represents the convenience with which the researcher can reach the respondent. The researchers do not have the authority to select the samples and they are done solely for reasons of proximity and not representativeness.
- Deliberate, critical, or judgmental sampling: In this type of sampling the researcher judges and develops his sample on the nature of the study and the understanding of his target audience. Only people who meet the research criteria and the final objective are selected.
- Snowball Sampling: As a snowball speeds up, it accumulates more snow around itself. Similarly, with snowball sampling, respondents are tasked with providing references or recruiting samples for the study once their participation ends.
- Quota Sampling: Quota sampling is a method where the researcher has the privilege to select a sample based on its strata. In this method, two people cannot exist under two different conditions.
LEARN ABOUT: Theoretical Research
Advantages and disadvantages of sampling in a study population
In most cases, of the total study population, perceptions can only be obtained from predefined samples. This comes with its own advantages and disadvantages. Some of them are listed below.
- Highly accurate – low probability of sampling errors (if sampled well)
- Economically feasible by nature, highly reliable
- High fitness ratio to different surveys Takes less time compared to surveying the entire population Reduced resource deployment
- Data-intensive and comprehensive Properties are applied to a larger population wideIdeal when the study population is vast.
Disadvantages
- Insufficient samples
- Possibility of bias
- Precision problems (if sampling is poor)
- Difficulty obtaining the typical sample
- Lack of quality sources
- Possibility of making mistakes.
At QuestionPro we can help you carry out your study with your study population. Learn about all the features of our online survey software and start conducting your research today!
LEARN MORE FREE TRIAL
MORE LIKE THIS
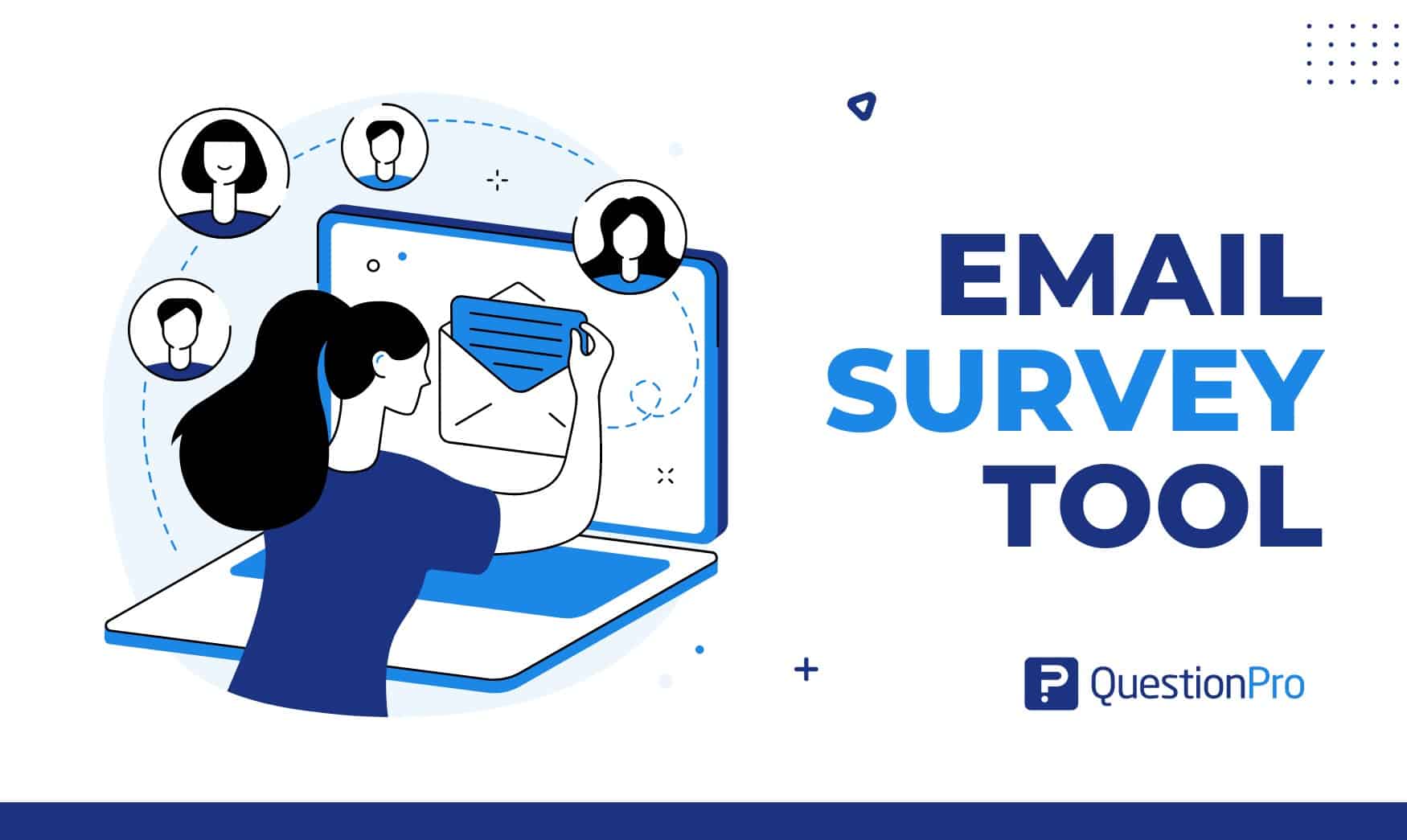
The Best Email Survey Tool to Boost Your Feedback Game
May 7, 2024
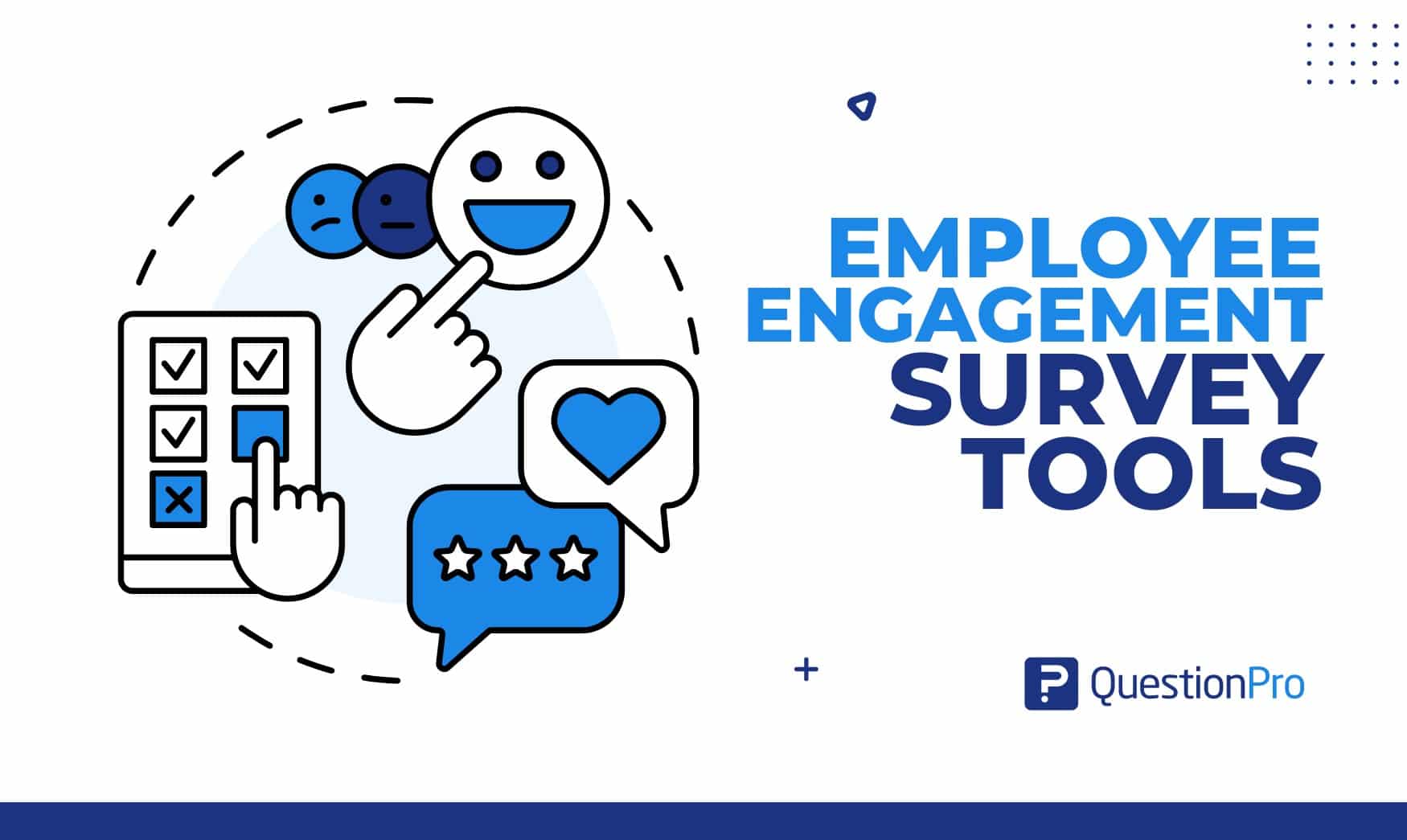
Top 10 Employee Engagement Survey Tools
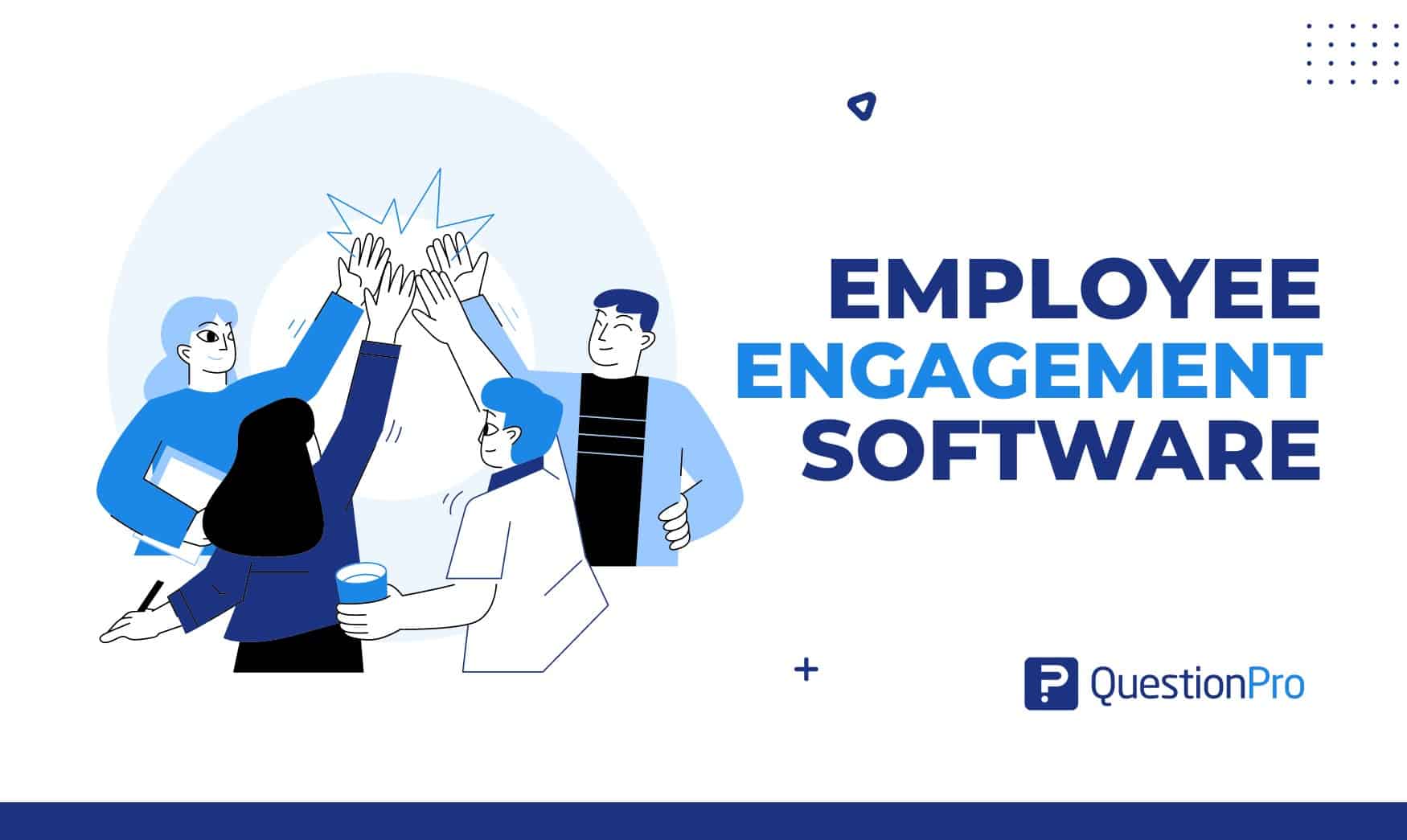
Top 20 Employee Engagement Software Solutions
May 3, 2024
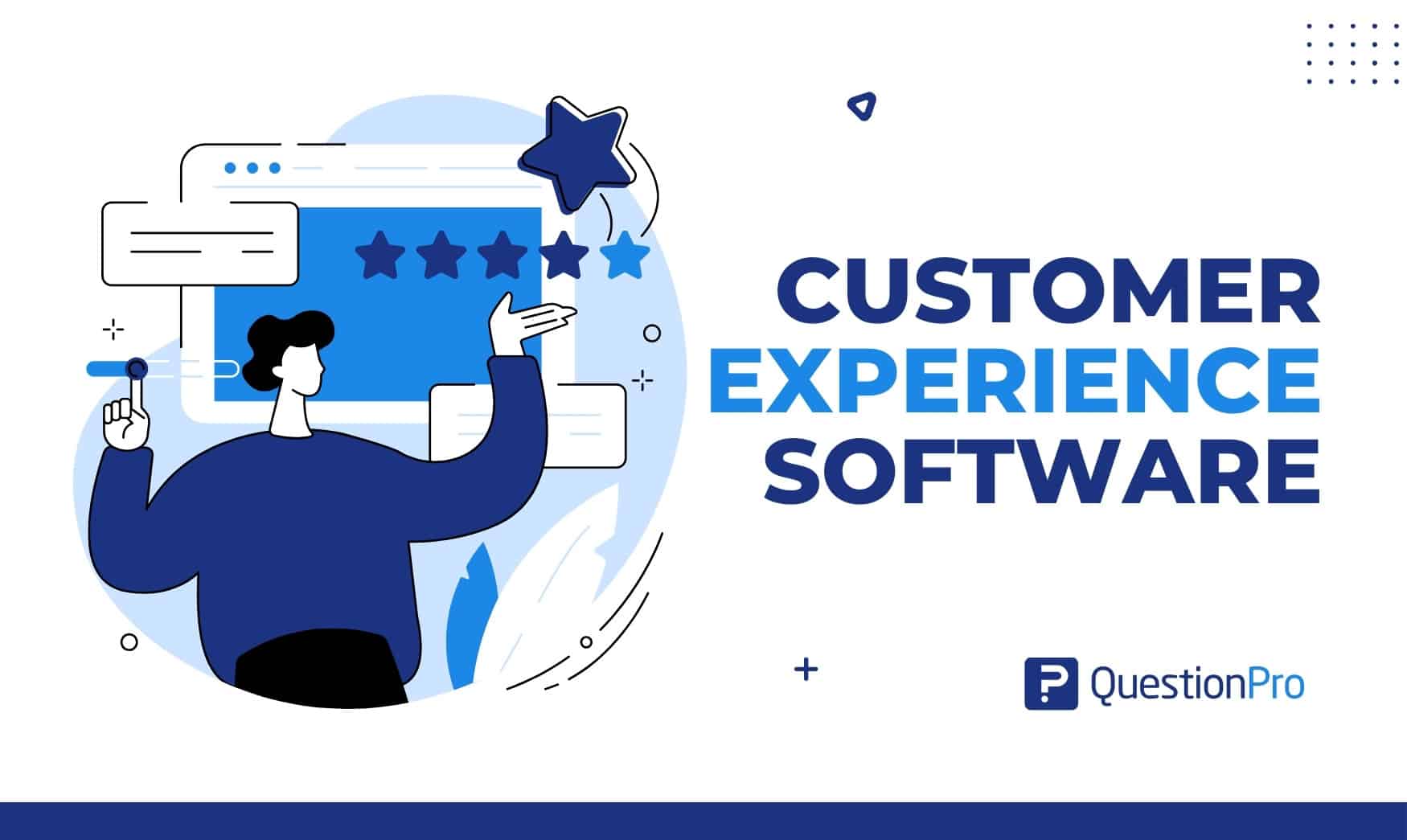
15 Best Customer Experience Software of 2024
May 2, 2024
Other categories
- Academic Research
- Artificial Intelligence
- Assessments
- Brand Awareness
- Case Studies
- Communities
- Consumer Insights
- Customer effort score
- Customer Engagement
- Customer Experience
- Customer Loyalty
- Customer Research
- Customer Satisfaction
- Employee Benefits
- Employee Engagement
- Employee Retention
- Friday Five
- General Data Protection Regulation
- Insights Hub
- Life@QuestionPro
- Market Research
- Mobile diaries
- Mobile Surveys
- New Features
- Online Communities
- Question Types
- Questionnaire
- QuestionPro Products
- Release Notes
- Research Tools and Apps
- Revenue at Risk
- Survey Templates
- Training Tips
- Uncategorized
- Video Learning Series
- What’s Coming Up
- Workforce Intelligence
Have a language expert improve your writing
Run a free plagiarism check in 10 minutes, automatically generate references for free.
- Knowledge Base
- Methodology
- Population vs Sample | Definitions, Differences & Examples
Population vs Sample | Definitions, Differences & Examples
Published on 3 May 2022 by Pritha Bhandari . Revised on 5 December 2022.
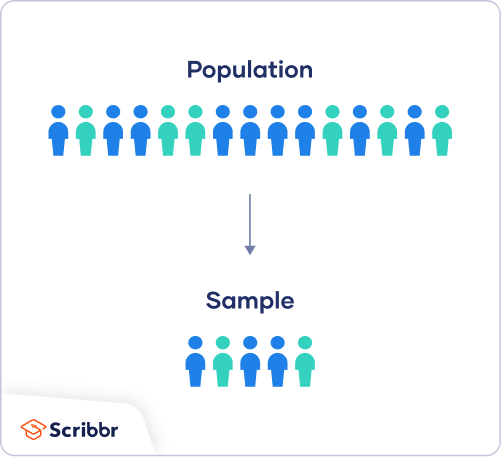
A population is the entire group that you want to draw conclusions about.
A sample is the specific group that you will collect data from. The size of the sample is always less than the total size of the population.
In research, a population doesn’t always refer to people. It can mean a group containing elements of anything you want to study, such as objects, events, organisations, countries, species, or organisms.
Table of contents
Collecting data from a population, collecting data from a sample, population parameter vs sample statistic, practice questions: populations vs samples, frequently asked questions about samples and populations.
Populations are used when your research question requires, or when you have access to, data from every member of the population.
Usually, it is only straightforward to collect data from a whole population when it is small, accessible and cooperative.
For larger and more dispersed populations, it is often difficult or impossible to collect data from every individual. For example, every 10 years, the federal US government aims to count every person living in the country using the US Census. This data is used to distribute funding across the nation.
However, historically, marginalised and low-income groups have been difficult to contact, locate, and encourage participation from. Because of non-responses, the population count is incomplete and biased towards some groups, which results in disproportionate funding across the country.
In cases like this, sampling can be used to make more precise inferences about the population.
Prevent plagiarism, run a free check.
When your population is large in size, geographically dispersed, or difficult to contact, it’s necessary to use a sample. With statistical analysis , you can use sample data to make estimates or test hypotheses about population data.
Ideally, a sample should be randomly selected and representative of the population. Using probability sampling methods (such as simple random sampling or stratified sampling ) reduces the risk of sampling bias and enhances both internal and external validity .
For practical reasons, researchers often use non-probability sampling methods . Non-probability samples are chosen for specific criteria; they may be more convenient or cheaper to access. Because of non-random selection methods, any statistical inferences about the broader population will be weaker than with a probability sample.
Reasons for sampling
- Necessity : Sometimes it’s simply not possible to study the whole population due to its size or inaccessibility.
- Practicality : It’s easier and more efficient to collect data from a sample.
- Cost-effectiveness : There are fewer participant, laboratory, equipment, and researcher costs involved.
- Manageability : Storing and running statistical analyses on smaller datasets is easier and reliable.
When you collect data from a population or a sample, there are various measurements and numbers you can calculate from the data. A parameter is a measure that describes the whole population. A statistic is a measure that describes the sample.
You can use estimation or hypothesis testing to estimate how likely it is that a sample statistic differs from the population parameter.
Sampling error
A sampling error is the difference between a population parameter and a sample statistic. In your study, the sampling error is the difference between the mean political attitude rating of your sample and the true mean political attitude rating of all undergraduate students in the Netherlands.
Sampling errors happen even when you use a randomly selected sample. This is because random samples are not identical to the population in terms of numerical measures like means and standard deviations .
Because the aim of scientific research is to generalise findings from the sample to the population, you want the sampling error to be low. You can reduce sampling error by increasing the sample size.
Samples are used to make inferences about populations . Samples are easier to collect data from because they are practical, cost-effective, convenient, and manageable.
Populations are used when a research question requires data from every member of the population. This is usually only feasible when the population is small and easily accessible.
A statistic refers to measures about the sample , while a parameter refers to measures about the population .
A sampling error is the difference between a population parameter and a sample statistic .
Cite this Scribbr article
If you want to cite this source, you can copy and paste the citation or click the ‘Cite this Scribbr article’ button to automatically add the citation to our free Reference Generator.
Bhandari, P. (2022, December 05). Population vs Sample | Definitions, Differences & Examples. Scribbr. Retrieved 6 May 2024, from https://www.scribbr.co.uk/research-methods/population-versus-sample/
Is this article helpful?
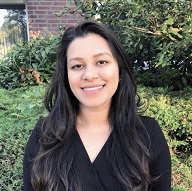
Pritha Bhandari
Other students also liked, sampling methods | types, techniques, & examples, a quick guide to experimental design | 5 steps & examples, what is quantitative research | definition & methods.
Study Population
- Reference work entry
- pp 6412–6414
- Cite this reference work entry
3228 Accesses
Study population is a subset of the target population from which the sample is actually selected. It is broader than the concept sample frame . It may be appropriate to say that sample frame is an operationalized form of study population. For example, suppose that a study is going to conduct a survey of high school students on their social well-being . High school students all over the world might be considered as the target population. Because of practicalities, researchers decide to only recruit high school students studying in China who are the study population in this example. Suppose there is a list of high school students of China, this list is used as the sample frame .
Description
Study population is the operational definition of target population (Henry, 1990 ; Bickman & Rog, 1998 ). Researchers are seldom in a position to study the entire target population, which is not always readily accessible. Instead, only part of it—respondents who are both eligible for the study...
This is a preview of subscription content, log in via an institution to check access.
Access this chapter
- Available as PDF
- Read on any device
- Instant download
- Own it forever
- Available as EPUB and PDF
- Durable hardcover edition
- Dispatched in 3 to 5 business days
- Free shipping worldwide - see info
Tax calculation will be finalised at checkout
Purchases are for personal use only
Institutional subscriptions
Babbie, E. R. (2010). The practice of social research . Belmont, CA: Wadsworth Publishing Company.
Google Scholar
Bickman, L., & Rog, D. J. (1998). Handbook of applied social research methods . Thousand Oaks, CA: Sage Publications.
Friedman, L. M., Furberg, C. D., & DeMets, D. L. (2010). Fundamentals of clinical trials . New York: Springer.
Gerrish, K., & Lacey, A. (2010). The research process in nursing . West Sussex: Wiley-Blackwell.
Henry, G. T. (1990). Practical sampling . Newbury Park, CA: Sage Publications.
Kumar, R. (2011). Research methodology: A step-by-step guide for beginners . London: Sage Publications Limited.
Riegelman, R. K. (2005). Studying a study and testing a test: How to read the medical evidence . Philadelphia: Lippincott Williams & Wilkins.
Download references
Author information
Authors and affiliations.
Sociology Department, National University of Singapore, 11 Arts Link, 117570, Singapore, Singapore
You can also search for this author in PubMed Google Scholar
Corresponding author
Correspondence to Shu Hu .
Editor information
Editors and affiliations.
University of Northern British Columbia, Prince George, BC, Canada
Alex C. Michalos
(residence), Brandon, MB, Canada
Rights and permissions
Reprints and permissions
Copyright information
© 2014 Springer Science+Business Media Dordrecht
About this entry
Cite this entry.
Hu, S. (2014). Study Population. In: Michalos, A.C. (eds) Encyclopedia of Quality of Life and Well-Being Research. Springer, Dordrecht. https://doi.org/10.1007/978-94-007-0753-5_2893
Download citation
DOI : https://doi.org/10.1007/978-94-007-0753-5_2893
Publisher Name : Springer, Dordrecht
Print ISBN : 978-94-007-0752-8
Online ISBN : 978-94-007-0753-5
eBook Packages : Humanities, Social Sciences and Law
Share this entry
Anyone you share the following link with will be able to read this content:
Sorry, a shareable link is not currently available for this article.
Provided by the Springer Nature SharedIt content-sharing initiative
- Publish with us
Policies and ethics
- Find a journal
- Track your research
3. Populations and samples
Populations, unbiasedness and precision, randomisation, variation between samples, standard error of the mean.

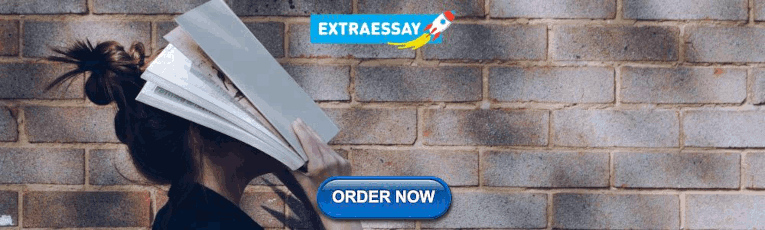
Standard error of a proportion or a percentage
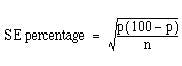
- Foundations
- Write Paper
Search form
- Experiments
- Anthropology
- Self-Esteem
- Social Anxiety

Research Population
All research questions address issues that are of great relevance to important groups of individuals known as a research population.
This article is a part of the guide:
- Non-Probability Sampling
- Convenience Sampling
- Random Sampling
- Stratified Sampling
- Systematic Sampling
Browse Full Outline
- 1 What is Sampling?
- 2.1 Sample Group
- 2.2 Research Population
- 2.3 Sample Size
- 2.4 Randomization
- 3.1 Statistical Sampling
- 3.2 Sampling Distribution
- 3.3.1 Random Sampling Error
- 4.1 Random Sampling
- 4.2 Stratified Sampling
- 4.3 Systematic Sampling
- 4.4 Cluster Sampling
- 4.5 Disproportional Sampling
- 5.1 Convenience Sampling
- 5.2 Sequential Sampling
- 5.3 Quota Sampling
- 5.4 Judgmental Sampling
- 5.5 Snowball Sampling
A research population is generally a large collection of individuals or objects that is the main focus of a scientific query. It is for the benefit of the population that researches are done. However, due to the large sizes of populations, researchers often cannot test every individual in the population because it is too expensive and time-consuming. This is the reason why researchers rely on sampling techniques .
A research population is also known as a well-defined collection of individuals or objects known to have similar characteristics. All individuals or objects within a certain population usually have a common, binding characteristic or trait.
Usually, the description of the population and the common binding characteristic of its members are the same. "Government officials" is a well-defined group of individuals which can be considered as a population and all the members of this population are indeed officials of the government.
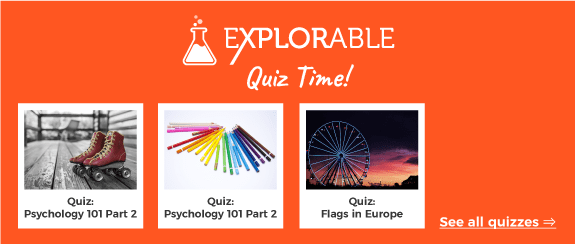
Relationship of Sample and Population in Research
A sample is simply a subset of the population. The concept of sample arises from the inability of the researchers to test all the individuals in a given population. The sample must be representative of the population from which it was drawn and it must have good size to warrant statistical analysis.
The main function of the sample is to allow the researchers to conduct the study to individuals from the population so that the results of their study can be used to derive conclusions that will apply to the entire population. It is much like a give-and-take process. The population “gives” the sample, and then it “takes” conclusions from the results obtained from the sample.
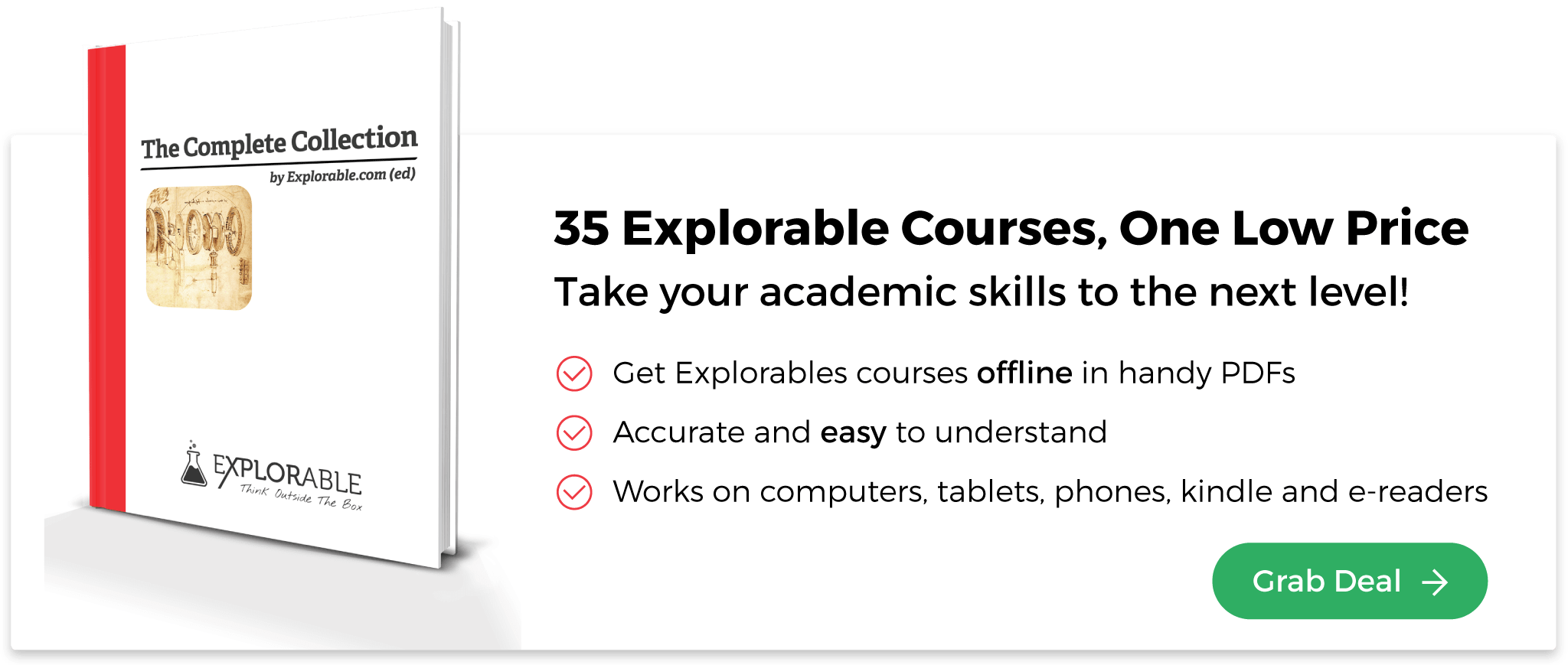
Two Types of Population in Research
Target population.
Target population refers to the ENTIRE group of individuals or objects to which researchers are interested in generalizing the conclusions. The target population usually has varying characteristics and it is also known as the theoretical population.
Accessible Population
The accessible population is the population in research to which the researchers can apply their conclusions. This population is a subset of the target population and is also known as the study population. It is from the accessible population that researchers draw their samples.
- Psychology 101
- Flags and Countries
- Capitals and Countries
Explorable.com (Nov 15, 2009). Research Population. Retrieved May 11, 2024 from Explorable.com: https://explorable.com/research-population
You Are Allowed To Copy The Text
The text in this article is licensed under the Creative Commons-License Attribution 4.0 International (CC BY 4.0) .
This means you're free to copy, share and adapt any parts (or all) of the text in the article, as long as you give appropriate credit and provide a link/reference to this page.
That is it. You don't need our permission to copy the article; just include a link/reference back to this page. You can use it freely (with some kind of link), and we're also okay with people reprinting in publications like books, blogs, newsletters, course-material, papers, wikipedia and presentations (with clear attribution).
Want to stay up to date? Follow us!
Save this course for later.
Don't have time for it all now? No problem, save it as a course and come back to it later.
Footer bottom
- Privacy Policy
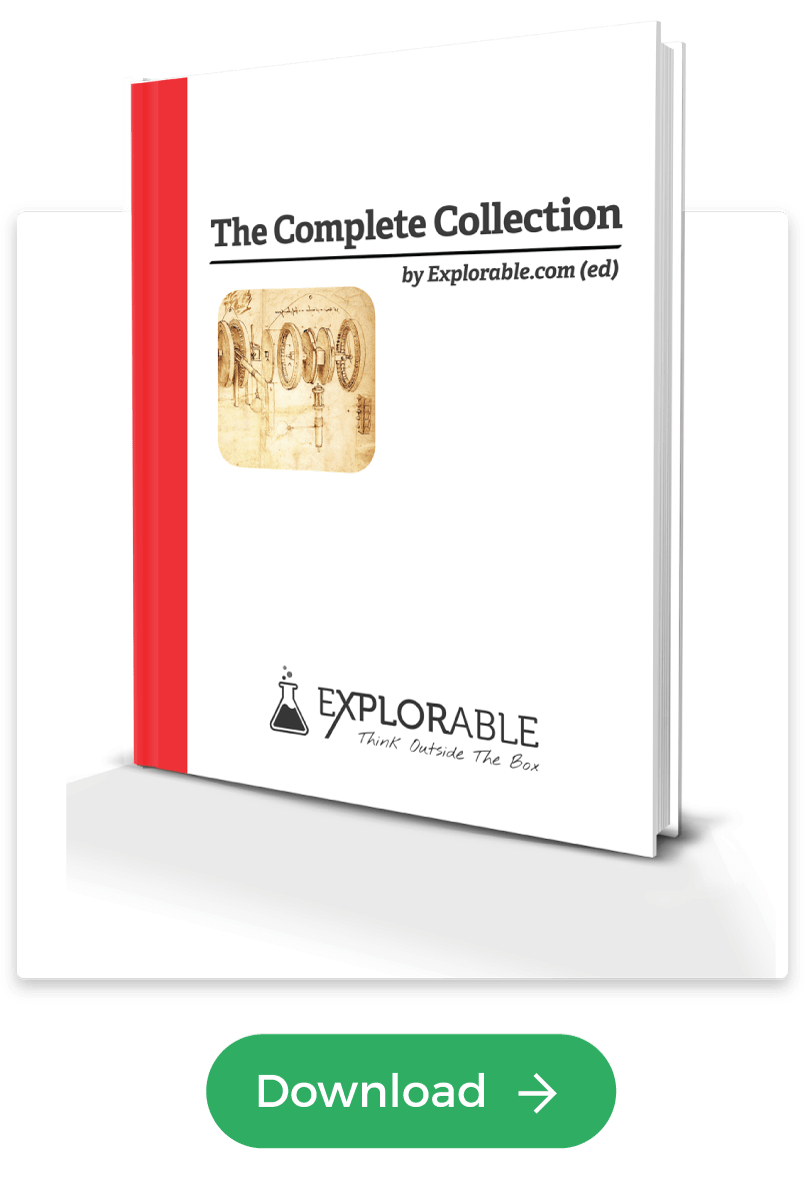
- Subscribe to our RSS Feed
- Like us on Facebook
- Follow us on Twitter
Improving Care for Older Adults
The Population Health Calamity Evidenced in U.S. Life Expectancy Statistics
And what it portends for aging adult health in the coming decades.
- Hoag Levins
- Share this page on Twitter
- Share this page on Facebook
- Share this page on LinkedIn
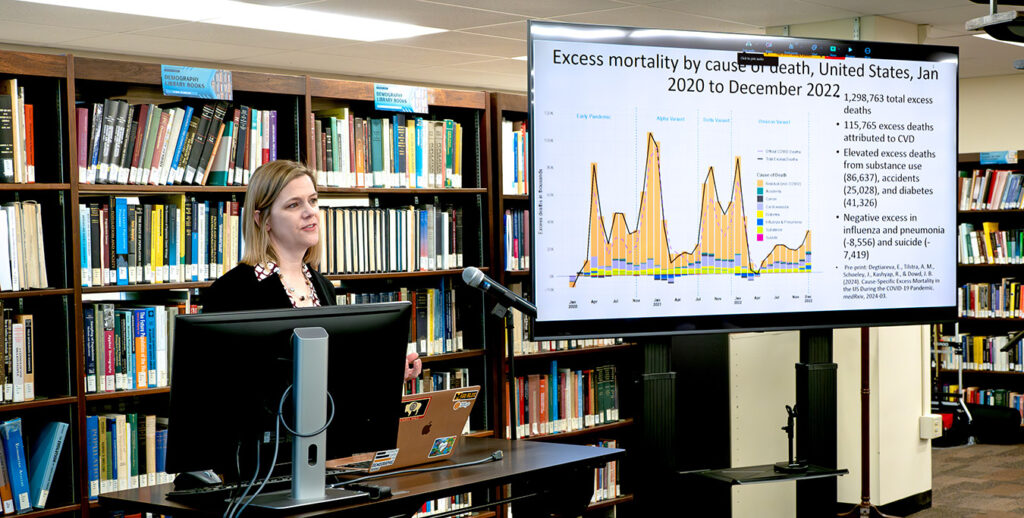
ALSO SEE: Companion photo story from the event
In a keynote address at one of the country’s foremost research centers for aging and health, demographer Jennifer Beam Dowd, PhD , emphasized that most people don’t fully appreciate how seemingly dry life expectancy statistics say a lot about a nation’s health and healthcare-related policies and practices. And, she noted, as world rankings of industrialized countries go, the United States is doing worse in that measure than nearly everybody else.
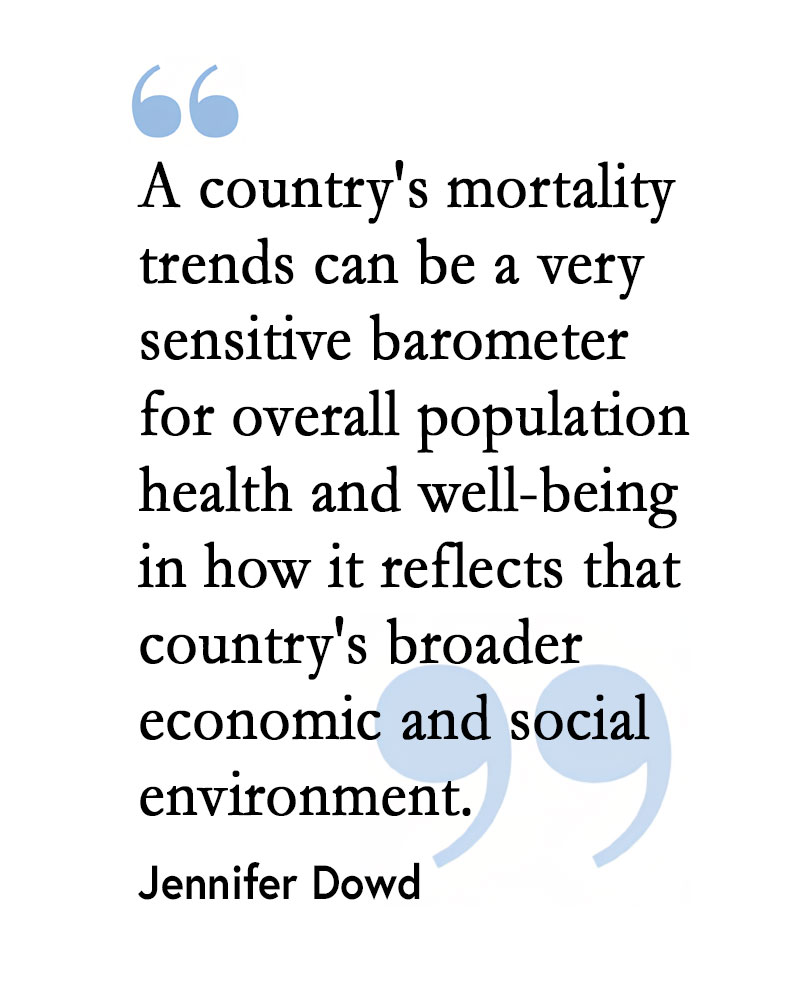
Dowd is Professor of Demography and Population Health and Deputy Director of the Leverhulme Centre for Demographic Science at the University of Oxford. In her address, she spoke about the data and insights from her five-year project funded by the European Research Council (ERC) studying the forces stalling life expectancy gains in the U.S. and UK. She was speaking at the May 3 annual Retreat of the University of Pennsylvania’s Population Aging Research Center (PARC).
“A country’s mortality trends can be a very sensitive barometer for overall population health and well-being in how they reflect that country’s broader economic and social environment,” said Dowd. “When we start to see things go wrong in mortality trends, it probably says a lot about what’s happening earlier in the life course.”
As background, Dowd reminded the audience that the U.S. has witnessed a remarkable increase in life expectancy over the last century, advancing from 47 years in 1900 to 78.9 years in 2014.
The latest data from the Centers for Disease Control and Prevention (CDC) show that life expectancy just before the outbreak of COVID-19 was 78.8 years and dropped to 76.4 years in the second year of the pandemic. It has since risen to 77.5 years. By comparison, life expectancy in the U.K. is 79.0 years, Spain is 83.3 years, Sweden is 83.1 years, and Italy is 82.7 years.
Falling Behind
“U.S. life expectancy has been slowing and falling behind its peers’ for quite some time,” Dowd said, noting that this issue is not so much about numbers as it is about the individuals whose early deaths it documents. She pointed to a 2023 “ Missing Americans ” study co-authored by LDI Senior Fellow Atheendar Venkataramani, MD, PhD , that found that from 1980 to 2019 11 million excess deaths would not have occurred if the U.S. life expectancy rate (or age-standardized mortality rate (ASMR)) had been the same as other wealthy countries. In 1980 the U.S. life expectancy rate began to diverge from that of other peer nations.
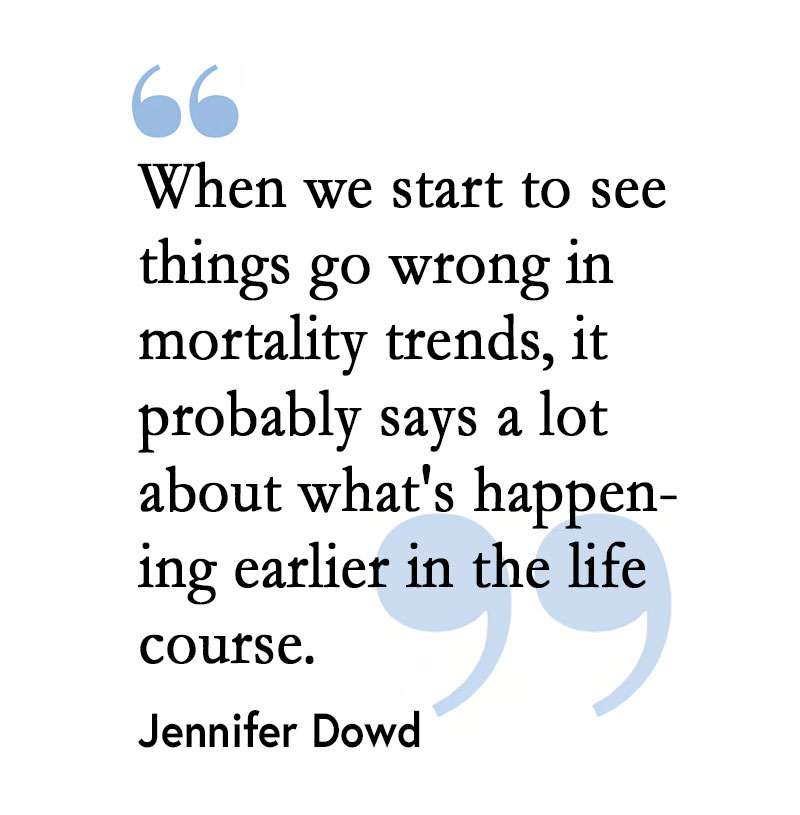
A watershed event in this trend occurred in 2015 when U.S. life expectancy declined for the first time in decades. Princeton economists Anne Case and Angus Deaton attributed this to “ deaths of despair ,” a term they coined to describe the rising mortality rates among middle-aged white Americans due to suicide, drug overdose, and alcohol-related diseases driven by economic hardship, social isolation, and lack of accessible health care.
“Missing Americans”
“The ‘Missing Americans’ idea is just a good reminder that we are really falling short of what we actually know is attainable, because we see it being achieved in other countries,” said Dowd.
In terms of the future of the U.S. population mortality rate, Dowd noted it’s difficult to make predictions, but several continuing population health threats seem to be obvious concerns. These include:
- COVID-19 and its continued persistence . While its much less in the news, COVID deaths have remained relatively high during this past winter and have shown no signs of declining to flu-like levels. It’s possible that instead of returning to a pre-pandemic baseline, COVID could be a new, additional health risk for older and immunocompromised adults in a way that could impact mortality rates for decades to come.
- “ Generational health drift .” The term refers to increases in chronic disease and disability such that younger generations whose members are not as healthy as their parents were at the same age. Midlife health declines in the U.S. presage large numbers of people who will experience earlier deaths, more debilitating chronic diseases, and more functional limitations as they age.
- Anti-vaccine politics . The U.S. was unique in the level and ferocity of its politicization of COVID and other vaccines resulting in a drop-off of vaccinations of all kinds. This has resulted in increasing numbers of communicable diseases like measles and pertussis outbreaks among the unvaccinated, and could put us in a dangerous place with new pandemic threats like H5N1 bird flu.
- A big cohort change in obesity . The U.S. is the leading edge of a worldwide obesity epidemic. A diminishing proportion of its population has a normal weight and body mass index (BMI) of 25 or less as a rapidly increasing proportion is severely obese with a BMI above 40 and greatly increased serious health risks. None of the world’s societies have yet been able to halt or reverse the rise in obesity levels.
- The drug overdose epidemic . The levels of drug use and overdose deaths continue at an unabated high level, further exacerbated by the introduction of deadly new substances like fentanyl and other synthetic opioids that drive overdose mortality.
- The mental health crisis . A large and growing mental health crisis among both adults and children puts them at risk for poorer health and earlier mortality.
- Inequity in U.S. health and health care . Affluent and marginalized populations in the U.S. continue to experience dramatically different levels of health and quality of health care that impact their mortality rates.
Reason for Optimism
“At the same time as we discuss these pessimistic things, I also want to talk about some reasons for optimism as we look forward,” said Dowd. “In the past new and unexpected breakthroughs in technology have suddenly impacted population health in very positive ways. Right now, we may be looking at one of those with the new anti-obesity medications like Ozempic. It is early days, and we still have a lot to learn about these drugs but the results being seen in clinical trials with weight loss, reductions in cardiovascular deaths, kidney failure, and heart failure seem very promising.”
“But perhaps the best reason to be optimistic is that we have examples of other countries that are doing much better in population health with lower mortality rates,” said Dowd. “We can actually see what is feasible in other human populations and find inspiration in that. That’s why us social scientists need to continue to study these cross-country differences so we can better understand what factors and policies lead to better or worse health outcomes.”
“I like to think about how Eastern Europe suffered a very bad mortality crisis after the fall of the Soviet Union but if you look at their mortality rates now, they’re actually starting to overtake the U.S. in some age groups and performing quite well,” said Dowd. “They are a sign that these mortality crises don’t necessarily last forever, even ones that emerge from dramatic social and economic upheavals. There is always hope that things can get better.”
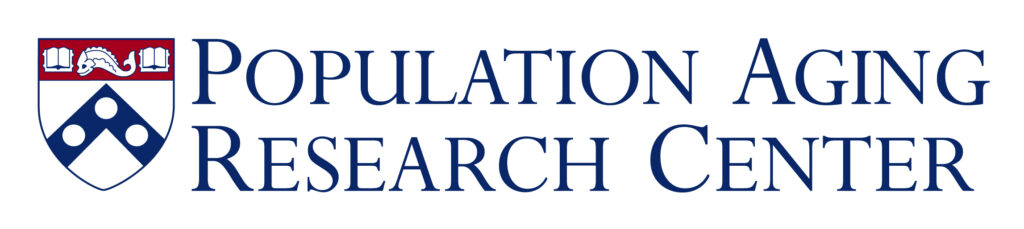
Editor, Digital Publications
More ldi news.
In Their Own Words
Health Care Access & Coverage
What Nurses Really Need is Better Staffing
The Common Strategies to Raise Nurse Morale Need an Update
- Karen Lasater, PhD, RN
- Jane Muir, PhD, APRN
Bipartisan Support Grows To Solve One of Health Care’s Biggest Problems
Dually Eligible Americans on Medicare and Medicaid Are Getting the Attention They Deserve
- Rachel M. Werner, MD, PhD
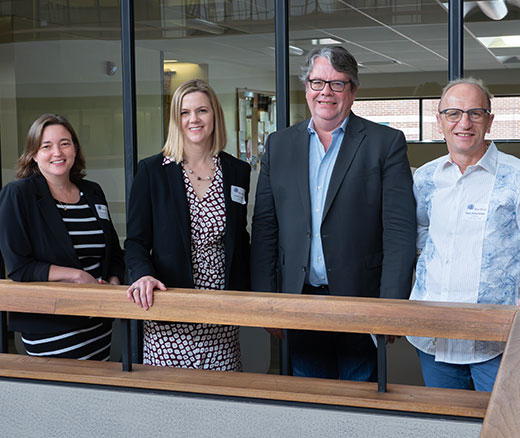
Photo Report from the Population Aging Research Center 2024 Retreat
Defining the Health and Health Care Problems and Prospects of Aging Americans
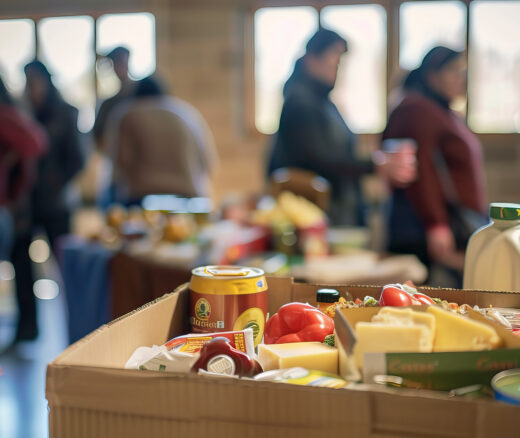
Health Equity
Parents Want Providers to Log Their Children’s Social Needs but with Transparency and Respect
Parents Also Fear Disclosing Social Needs Will Lead to Child Welfare Referrals, a Study by LDI Fellows Finds
- Miles Meline, MBE

News | Video
Penn Study in Navajo Nation Boosts Guideline Heart Failure Drug Uptake by 53%
LDI Senior Fellow Lauren Eberly Leads Team in New Mexico
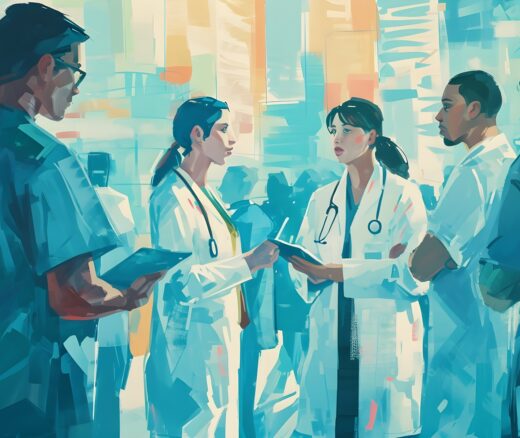
Growing Health Worker Migration to the U.S. and U.K. Raises Fairness and Training Issues
The U.S. Needs to Help Fix Migration’s Root Causes and Build Better Training Systems
- Farouk Dako, MD, MPH
Thank you for visiting nature.com. You are using a browser version with limited support for CSS. To obtain the best experience, we recommend you use a more up to date browser (or turn off compatibility mode in Internet Explorer). In the meantime, to ensure continued support, we are displaying the site without styles and JavaScript.
- View all journals
- My Account Login
- Explore content
- About the journal
- Publish with us
- Sign up for alerts
- Open access
- Published: 09 May 2024
Population health implications of exposure to pervasive military aircraft noise pollution
- Giordano Jacuzzi ORCID: orcid.org/0009-0005-4351-5682 1 ,
- Lauren M. Kuehne ORCID: orcid.org/0000-0002-0054-613X 2 ,
- Anne Harvey 3 ,
- Christine Hurley 3 ,
- Robert Wilbur 4 ,
- Edmund Seto ORCID: orcid.org/0000-0003-4058-0313 5 &
- Julian D. Olden ORCID: orcid.org/0000-0003-2143-1187 1
Journal of Exposure Science & Environmental Epidemiology ( 2024 ) Cite this article
27 Altmetric
Metrics details
While the adverse health effects of civil aircraft noise are relatively well studied, impacts associated with more intense and intermittent noise from military aviation have been rarely assessed. In recent years, increased training at Naval Air Station Whidbey Island, USA has raised concerns regarding the public health and well-being implications of noise from military aviation.
This study assessed the public health risks of military aircraft noise by developing a systematic workflow that uses acoustic and aircraft operations data to map noise exposure and predict health outcomes at the population scale.
Acoustic data encompassing seven years of monitoring efforts were integrated with flight operations data for 2020–2021 and a Department of Defense noise simulation model to characterize the noise regime. The model produced contours for day-night, nighttime, and 24-h average levels, which were validated by field monitoring and mapped to yield the estimated noise burden. Established thresholds and exposure-response relationships were used to predict the population subject to potential noise-related health effects, including annoyance, sleep disturbance, hearing impairment, and delays in childhood learning.
Over 74,000 people within the area of aircraft noise exposure were at risk of adverse health effects. Of those exposed, substantial numbers were estimated to be highly annoyed and highly sleep disturbed, and several schools were exposed to levels that place them at risk of delay in childhood learning. Noise in some areas exceeded thresholds established by federal regulations for public health, residential land use and noise mitigation action, as well as the ranges of established exposure-response relationships.
Impact statement
This study quantified the extensive spatial scale and population health burden of noise from military aviation. We employed a novel GIS-based workflow for relating mapped distributions of aircraft noise exposure to a suite of public health outcomes by integrating acoustic monitoring and simulation data with a dasymetric population density map. This approach enables the evaluation of population health impacts due to past, current, and future proposed military operations. Moreover, it can be modified for application to other environmental noise sources and offers an improved open-source tool to assess the population health implications of environmental noise exposure, inform at-risk communities, and guide efforts in noise mitigation and policy governing noise legislation, urban planning, and land use.
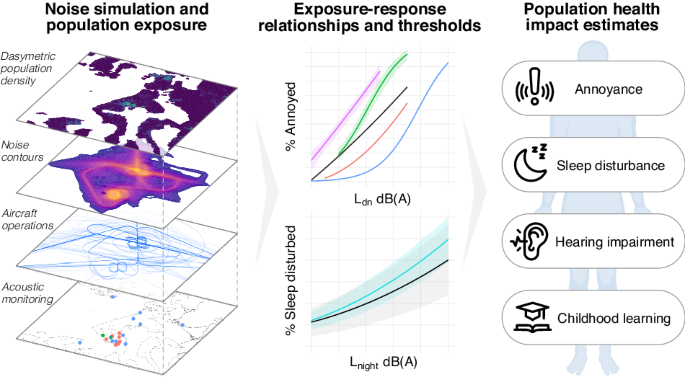
Similar content being viewed by others
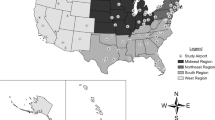
Characterizing temporal trends in populations exposed to aircraft noise around U.S. airports: 1995–2015
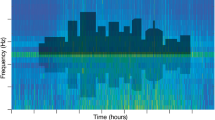
Long-term measurement study of urban environmental low frequency noise
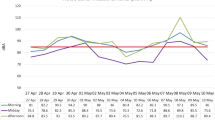
Quantifying traffic noise pollution levels: a cross-sectional survey in South Africa
Introduction.
The adverse effects of noise pollution, or unwanted or disturbing sound, on human physical and psychological health are widely recognized. Documented impacts range from annoyance and stress to elevated risk of sleep disturbance, cardiovascular disease, hearing impairment, and compromised childhood learning [ 1 , 2 , 3 , 4 , 5 ]. Recognition of these impacts and their associated costs to public health have highlighted the problem of increasing environmental noise originating from diverse human activities [ 6 , 7 ]. Of these, noise from pervasive aviation is a primary and growing cause of concern internationally and has been reported to elicit more severe health impacts than other sources of transportation noise [ 8 , 9 , 10 ].
The ability to assess the health consequences of aircraft noise is fundamental to inform affected communities about risks, devise strategies to mitigate impacts, and guide policy to protect or restore public health. In recent decades, growing scientific inquiry has led to the development of quantitative exposure-response relationships that directly link aircraft noise exposure with estimated population health outcomes [ 9 , 11 , 12 ]. However, while policies enacted in a handful of regions (e.g., European Noise Directive, Environmental Quality Standards for Noise) have improved the assessment of environmental noise exposure and health impacts, regulatory actions to reduce noise pollution and/or mitigate exposures have largely been slow and irregular [ 13 , 14 , 15 , 16 ]. Furthermore, compared to research in civil aviation noise (i.e., commercial and private aircraft), studies concerned with the health implications of noise from military aviation specifically have faced unique challenges (e.g., intermittent or unpublished operations, a lack of data to inform exposure-response relationships) that have limited the scale and scope of data available to assess population health risks [ 17 , 18 , 19 ].
Noise regimes of military aviation differ dramatically in their level, spectra, repetition, and character [ 17 , 18 , 20 , 21 , 22 , 23 ]. Unlike commercial aircraft noise, which is consistent and largely predictable in the frequency of events and duration of exposure, noise from military activity can vary widely over time. Military air bases are ubiquitous around the world, and a small but growing body of research has begun to investigate the unique human health implications of military aircraft noise exposure. For example, noise from military aviation can elicit different, and often greater, impacts on human disturbance and health compared to civil aviation [ 19 , 24 , 25 ]. These studies remain in the minority, leading to insufficient understanding to inform impacted communities and guide specialized policy [ 7 ]. Furthermore, military airfields and air spaces may often be exempted from or subject to differing regulations for noise assessment and mitigation [ 26 , 27 ], leading to a policy or regulatory vacuum whereby communities must petition for such actions. For example, although federal policy allows compensation for sound insulation in high exposure areas around civilian airports in the US, this policy applies only to “public use airports” [ 27 ]. While the spatiotemporal extent of operations and noise exposure from American civilian transportation is readily available (e.g., US National Transportation Noise Map [ 28 ]), corresponding data on how military aircraft operations are the source of noise permeating across space and time is reported much less often to the public.
In recent years, public concern has grown regarding noise experienced by communities in Washington State, USA, from military training activities at Naval Air Station Whidbey Island (NASWI) [ 29 ]. Since the US Navy consolidated its fleet of EA-18G Growler aircraft at NASWI and expanded flight operations in 2013, noise exposures have grown dramatically for residents of multiple counties [ 17 , 18 ]. An environmental impact statement was conducted in accordance with the National Environmental Protection Act, which provided evidence for community annoyance, speech and classroom interference, as well as an increase in the probability of awakening and the population vulnerable to potential hearing loss due to military aircraft noise events [ 30 ]. However, the assessment of population health impacts has consistently come under scrutiny, and as early as 2017 the Washington State Board of Health concluded that available data was insufficient to assess the impacts of a proposed operational increase, and a full public health risk assessment was needed [ 31 ]. This was supported by a subsequent review that found that operations around NASWI largely exceeded those of all health-related studies of military low-elevation flights worldwide [ 17 ]. A lawsuit jointly filed by a citizens group and Washington State in 2019 ultimately resulted in a 2022 ruling that the Navy did not adequately consider ramifications for childhood learning, and a subsequent order to reconduct the environmental impact statement [ 32 ].
The present study sought to address this knowledge gap by conducting a transparent and reproducible quantitative assessment of military aviation noise and its implications for public health and well-being at a regional scale. Employing a novel workflow for evaluating the human health impacts of noise pollution, we quantify the sonic character and spatiotemporal distribution of aircraft noise exposure by integrating acoustic monitoring and simulations of aircraft activity with a population density map to ultimately derive a suite of population health outcomes, including estimates of annoyance, sleep disturbance, hearing impairment, and compromised childhood learning throughout the study region. This research was guided by input from community partners, and public webinars reported routinely on progress and outcomes throughout the entire study period. Results from this study provide evidence for the pervasive noise pollution, and resulting public health implications, stemming from military aviation at NASWI. More broadly, it provides a workflow to systematically assess the population health risks of noise pollution from sources other than military aircraft, which could be used as a basis for future environmental and public health impact assessments.
Materials and methods
Study region.
Military training operations at NASWI originate from two primary airfields on Whidbey Island, Washington State, USA (Fig. 1 ). Ault Field is located approximately 5 km from the city of Oak Harbor, the largest community in Island County, while Outlying Landing Field (OLF) Coupeville is located 4 km from the town of Coupeville. Aircraft operations conducted at NASWI range from sessions of repeated closed-pattern routines (including “touch-and-go” field carrier landing practice, FCLP), to interfacility transfers and arrivals from and departures to off-station areas, including the Olympic Military Operations Area (MOA) on the Olympic Peninsula (the primary location of electronic warfare and air-to-air combat training). The flight paths for these operations extend across northwestern Washington, from the Pacific coast to the Cascade Mountains, encompassing the counties of Clallam, Jefferson, Island, San Juan, Skagit, and Snohomish, in Washington State, USA.
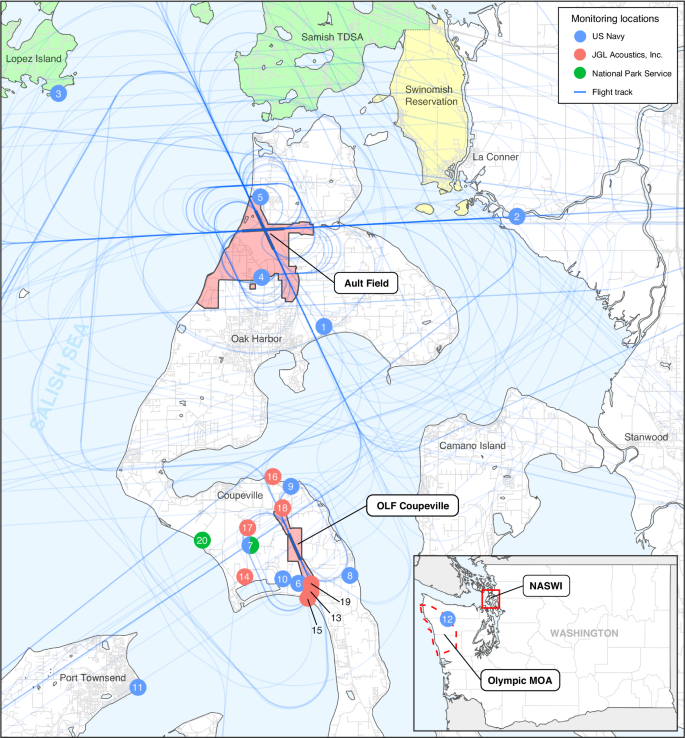
Monitoring locations are shown from the US Navy, JGL Acoustics Inc., and the National Park Service. The Swinomish Reservation and Samish Tribal Designated Statistical Area are indicated in yellow and green, respectively.
Analysis workflow
Acoustic metrics characterizing individual aircraft noise events and cumulative exposure levels were derived from acoustic data recorded at monitoring locations and used to validate a model simulating noise exposure across the entire study region. Modeled spatial predictions, expressed as noise contours, were overlaid with a dasymetric population density map to estimate population noise exposures at a fine spatial scale. Established thresholds and exposure-response functions were used to estimate the effect of the noise regime on multiple population health outcomes. This analysis workflow is detailed in Fig. 2 .
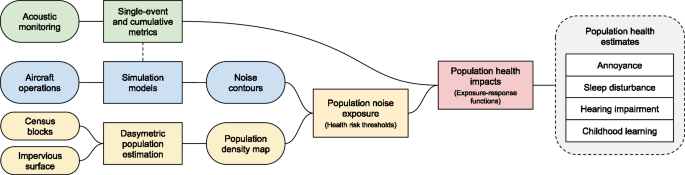
Data are shown as oblongs, while models and processing steps are shown as rectangles.
Acoustic monitoring data and metrics
Acoustic monitoring data consisted of sound pressure level (SPL) measurements collected during previous investigations into military aircraft noise, primarily from locations near the Ault and Coupeville airfields or their associated flight paths, both on Whidbey Island and throughout the surrounding region. Congress passed unique legislation in 2019 requiring the Navy to conduct acoustic monitoring around NASWI during four discrete weeks in 2020–2021, and at one location within the Olympic MOA for 365 days [ 33 ]. These data were obtained from the Naval Facilities Engineering Systems Command [ 34 ]. Additional monitoring data from 2015 to 2019 were obtained from JGL Acoustics, Inc. and the National Park Service Night Skies and Natural Sounds Division [ 21 , 35 , 36 ]. In total, 20 unique locations were examined (Supplementary Table S 1 ). SPL measurements were conducted with a class 1 sound level meter at a 1 Hz sampling rate and included A frequency-weighted equivalent continuous SPL L Aeq and, where available, A-weighted fast time-weighted maximum SPL L AFmax and peak C-weighted L Cpeak . Frequency spectrum measurements consisted of Z-weighted L Zeq in one-third octave bands and were only available for a subset of locations. Further details regarding data collection can be found in the relevant references [ 21 , 35 , 36 , 37 ].
We calculated a suite of acoustic metrics to characterize noise from single overflight events and cumulative noise levels associated with aircraft operations. Metrics were selected for their ubiquity in domestic and international standards and policy for land use compatibility, and because they provide the basis for exposure-response relationships concerning human health impacts [ 7 , 9 , 11 , 12 , 38 , 39 , 40 ]. All metrics throughout this study use A frequency weighting unless otherwise specified.
Single event metrics included the sound exposure level L E (also referred to as SEL), the 1-second average event maximum L max , the fast time-weighted maximum L Fmax , and (when available) the instantaneous C-weighted peak sound pressure level L Cpeak . All metrics were calculated in accordance with standards established by the International Organization for Standardization (ISO) and the Navy [ 11 , 37 ]. The spectral content of noise events was measured in one-third octave frequency bands for a subset of monitoring locations near Coupeville airfield (locations 6-10) having a high prevalence of FCLP aircraft events. Spectrums were energy-averaged for individual events, then energy-averaged within sites to yield a representative FCLP for each location.
Overflight events were detected from continuous SPL time-series data according to guidelines established in ISO 20906 and the SAE Aerospace Recommended Practice [ 38 , 41 ], and following the approach used by the Navy for noise monitoring [ 37 ]. A 10 second moving average was applied to each SPL time-series, smoothing the signal and reducing small variations that might otherwise be incorrectly labeled as events. An individual event was detected when this level exceeded a threshold varying with ambient conditions; ISO procedures recommend estimating background sound by the 95% exceedance level of total sound L 95 , and aircraft maxima should measure at least 15 dB above residual sound [ 38 ]. We note that some time-series data were collected only during periods of active aircraft operations (Supplementary Table S 1 ) and lacked a representative reference background. The threshold for event detection for these time-series was the maximum value between the L 95 of the hour (+/−30 min) and a baseline 35 dB + 15 = 50 dB ambient value for each second. An event was determined to terminate when the level fell and remained below the threshold for 5 s. Detected events containing multiple peaks above a local exceedance threshold (e.g., due to rapid flybys or multiple aircraft operating simultaneously) were subdivided into individual events corresponding with each peak. Detected acoustic events at locations 1–12 were cross-referenced against reported events from the Navy [ 34 ] and verified as military aircraft events accordingly. Detected events at locations 13–20 were manually verified by a trained observer [ 21 , 35 , 36 ].
Cumulative metrics quantify noise exposure over periods of time and form the basis of most community or public health impact assessments. Calculated cumulative metrics included: L dn , the day-night average sound level (also referred to as DNL), with a +10 dB penalty applied to nighttime periods (22:00-07:00); L den , the day-evening-night average sound level, with a penalty of +5 and +10 dB applied to evening (19:00-22:00) and nighttime (22:00-07:00) periods, respectively; L night , the equivalent continuous sound pressure level during nighttime hours; and L eqH , the equivalent continuous sound pressure level over a specified time period H , such as 24 h. Cumulative noise exposure within the Olympic MOA was quantified only with L dnmr , the onset-rate adjusted monthly day-night average sound level, as it is conventionally used to account for the sporadic nature and potentially high onset rates of noise within special-use airspace [ 37 ].
Cumulative acoustic metrics were calculated for every monitoring location and date, including L dn , L den , L night , L eq24h , and hourly L eq . These metrics were computed directly from continuous time-series measurements L eq,1s , rather than an aggregation of individual noise events L E , in accordance with ISO standards [ 11 ] and to enable direct comparisons of ambient noise levels on days with and without flight operations.
Aircraft operations data and simulation models
Detailed flight operations records were obtained from the Naval Facilities Engineering Systems Command for the four weeklong monitoring periods in 2020 and 2021, which were designed to capture “a range of flight operations across a range of seasonal weather conditions… during periods of high, medium, and low flight activity” [ 34 , 37 ]. These records documented flight profile and track activity from Ault Field and OLF Coupeville, as well as maintenance and engine run-up operations. Records for training routes and airspace profiles within the Olympic MOA were also obtained for a 365-day period within 2020 and 2021. These data were originally collected for the Navy Real-Time Aircraft Sound Monitoring Study [ 33 ] and presented a unique opportunity to investigate direct links between military aircraft operations and the noise regime.
We used the Noisemap software suite to simulate and spatially map noise exposure across the study region [ 42 ]. Noisemap is a noise modeling tool approved by the United States Department of Defense and used by the Navy to predict noise from flight operations. It integrates airfield operational data, flight profile specifications (including track, altitude, and speed), and a library of reference noise measurements with environmental terrain data to simulate the acoustic propagation of generated noise and resulting exposure at a grid of points on the ground level. The number of operations used by Noisemap is based on the average annual day, or the average number of airfield operations that would occur during a single day assuming 365 days of flying per year [ 37 ]. The average number of total operations during the four discrete monitoring periods was approximately 83% of the projected total operations for an “average year” at NASWI for 2021 [ 30 ], thus underestimating true flight activity at the annual scale.
Operations data were summarized as the total number of operations per flight profile for each period, and the mean number of operations per flight profile was calculated across all monitoring periods. This yielded a final model representing average flight activity across all periods throughout the year. Noisemap then simulated this activity, including additional noise due to maintenance and preflight ground run-up operations, such that the total predicted aircraft noise exposure was the accumulated noise exposure generated from all active operations of aircraft on all flight profiles [ 42 ].
The Noisemap model produced noise exposure contours in 1 dB increments for L dn , L night , and L eq24h from a grid of points spaced evenly at a standard distance of 914 m, or 3000 ft. The model also calculated noise exposure at specific locations corresponding to monitoring locations 1–11 to enable comparison of simulated noise metrics with those empirically measured by acoustic monitoring in the field. A second simulation was created to estimate noise exposure within the Olympic MOA using operations data averaged across the year.
Lastly, we applied the models to simulate the health impacts of alternative noise regimes by scaling the relative quantity of total flight operations across the range of 50–150%, ultimately projecting the response of population health outcomes to decreases or increases in aircraft activity. While this included estimates for the total number of operations projected for 2021 from the Navy environmental impact statement, it should be noted that this simple scaling of operations quantities from the four discrete monitoring periods does not accurately reflect the true operations and fleet composition active throughout 2021, and the projected population impact estimates are not representative, but rather demonstrative.
Population noise exposure
US population distributions are often derived from census units, which vary in geographic size based on population density. Units in urban areas are typically small with evenly distributed populations, while units in rural areas are larger with irregularly distributed populations. Using census units as a basis for population assessment can substantially limit the resolution of any spatial analysis of rural communities, and can reduce the accuracy of estimated impacts from socio-environmental problems [ 43 , 44 ].
To overcome this limitation, we implemented a workflow established by Swanwick et al. to create a 30-m resolution population density estimate for the study area [ 45 ]. This approach dasymetrically distributed block-level population estimates across all non-transportation impervious surfaces for each census block in the study area. We used the same approach to estimate population density for federally- and state-recognized tribal reservations and tribal-designated statistical areas (TDSA). Population data were obtained from the US Census Bureau’s 2021 American Community Survey, and impervious surface area data from the most recently available 2019 National Land Cover Database [ 46 ]. Simulated noise contours produced from Noisemap were rasterized to the same 30-m resolution as the population density map and intersected to yield an estimate of the number of people exposed to noise levels at or above thresholds established by domestic policy and international guidelines and associated with a substantial risk of impact on human health.
The World Health Organization (WHO) strongly recommends reducing aircraft noise levels below 45 dB L den , as aircraft noise above this level is associated with adverse health effects [ 9 ]. As such, we considered the 45 dB L dn contour to represent the spatial extent of adverse cumulative noise exposure, and the population residing within this area was therefore exposed to quantities of noise known to be harmful to human health. Additional thresholds used to estimate the at-risk population included aircraft noise levels associated with annoyance (45 dB L den ) [ 9 ], adverse effects on sleep (40 dB L night ) [ 9 ], a risk of hearing impairment over time (70 dBA L eq24 ) [ 3 , 39 ], and land use incompatibility according to regulations set by the Navy, Federal Aviation Administration (FAA), and US Department of Housing and Urban Development (65 dB L dn ) [ 27 , 47 , 48 ]. The number of individuals predicted to be impacted by these health risks vary according to the relationships described in the following section.
Population health impacts
Population health impacts, evaluated according to the number of individuals estimated to experience an adverse health outcome due to noise exposure, were calculated using established exposure-response relationships for annoyance, sleep disturbance, and compromised childhood learning (Fig. 3 ). These health outcomes were selected because they serve as critical indicators of community health [ 2 , 3 , 4 ], they are ubiquitous in noise law (e.g. environmental assessment [ 30 ], land-use [ 27 , 47 , 48 ]), and they have published exposure-response relationships that are commonly implemented in domestic and international policy and standards to assess health outcomes from noise [ 9 , 11 , 16 , 49 ]. In particular, WHO guidelines identify these outcomes as having sufficiently robust exposure-response relationships to support quantitative health assessment [ 9 ]. These outcomes are also the first responses in a stress-mediated chain of physiological effects that can lead to more severe health consequences. Noise exerts effects either directly though objective sound exposure (hearing impairment or sleep disturbance) or indirectly through the subjective emotional and cognitive perception of sound (annoyance) [ 1 , 4 , 50 ]. Both of these pathways elicit neurobiological stress responses that in turn promote cardiovascular risk factors (blood pressure, glucose levels) that can manifest in disease (hypertension, ischemic heart disease) [ 1 , 4 , 50 , 51 ] or induce psychological effects that jeopardize mental health (anxiety, depression) [ 4 , 50 , 52 ].
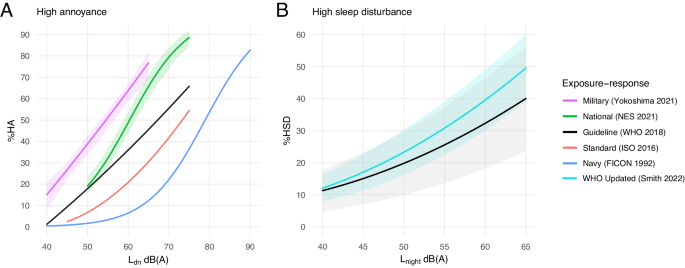
Functions relating L dn to high annoyance ( A ) and L night to high sleep disturbance ( B ) are illustrated with shaded 95% confidence intervals, where available.
These downstream health outcomes, namely cardiometabolic and psychological effects, were excluded from consideration in the present study because they currently lack generalized exposure-response relationships for public health assessment and are not widely used in domestic and international noise policy and guidelines. While relationships have been quantified for cardiometabolic and psychological effects [ 52 , 53 , 54 ], inconclusive empirical support and methodological differences between studies has precluded the development of robust generalized exposure-response relationships [ 53 ] and led to the exclusion of these health outcome assessments from WHO guidelines [ 9 , 55 , 56 ]. The chosen outcomes of annoyance, sleep disturbance, and childhood learning serve as proven indicators of community health that can be used to inform policy and prioritize future primary assessments of additional health outcomes from members of the population directly.
While most international noise policies and guidelines rely on L den as the primary cumulative noise metric [ 9 , 11 , 49 ], a majority of US states (including Washington) do not apply a penalty to the evening time period, and instead rely on L dn . As such, operational flight profile data from the simulation models were only available in day-night periods, and the following health analyses use L dn in lieu of L den . This is expected to result in slightly more conservative estimates than would be expected if L den were available, given that aircraft flight operations were not uncommon during evening hours.
To predict prevalence of high annoyance and high sleep disturbance throughout the population, associated exposure-response functions were used to obtain an estimated percentage of the population impacted from the noise exposure level at the 30 m 2 spatial grain (raster). Levels exceeding the defined range of a function were capped at the maximum predicted response value, while levels below were assigned a value of zero. The estimated population of each raster was multiplied by this percentage and summed across all units within the study area to estimate the total population subject to each health outcome.
Exposure-response curves quantifying the relationship between aircraft noise exposure and human annoyance can differ dramatically by region, community, and type of aircraft and activity. Similarly, curves used in public health policy vary widely between nations. For example, the dose-response curve endorsed by the Federal Interagency Committee on Noise (FICON) [ 40 ] remains the current US standard for estimating community response to noise exposure, and is employed by the FAA and Navy. However, the recent comprehensive Neighborhood Environmental Survey (NES) conducted by the FAA found that this standard does not reflect the current US public perception of aviation noise and provided an updated and nationally representative exposure-response curve [ 12 ]. Exposure-response curves developed and recommended by the ISO and WHO represent intermediate responses for a given noise exposure level [ 9 , 11 ].
Although these relationships are commonly applied in the implementation of health risk assessment and noise policy related to commercial and civil aircraft noise, there is evidence that they may underestimate impacts of noise from military aircraft due to the dramatic differences in the frequency and intensity of military aircraft events [ 12 , 19 , 20 , 22 , 57 ]. For these reasons, we include a unique exposure-response relationship developed by Yokoshima et al., based on a synthesis of individual studies on aircraft noise from US military and Japan Self-Defense Forces [ 19 ]. Collectively, these five exposure-response curves were used to assess the range of predicted impacts by relating aircraft noise L dn to the probability of a population being highly annoyed (Fig. 3A ).
- Sleep disturbance
Substantial evidence supports the considerable and consistent effects of aircraft noise on sleep disturbance [ 9 , 58 ]. These exposure-response relationships are based on survey and experimental assessments that identify aircraft noise as the cause of awakenings from sleep, the process of falling asleep, and/or sleep disturbance. Nighttime noise exposure near military airfields has been found to substantially reduce sleep quality [ 20 , 59 ]. However, because these studies are highly limited in number, exposure-response curves relating sleep disturbance to military aircraft noise exposure are not available. As such, we employed two published exposure-response curves that relate nighttime aircraft noise L night to the probability of being highly sleep disturbed (Fig. 3B ), namely, the guideline curve presented by the WHO and an updated version of this curve by Smith et al. that includes more recent survey data [ 9 , 58 ]. As previously discussed, these curves are expected to result in conservative estimates of impacted populations.
Childhood learning
We investigated the noise exposure levels at geographic centers of public, private, and postsecondary schools within the study area, obtained from the National Center for Education Statistics [ 60 ]. Systematic reviews conducted by the WHO and National Academies of Sciences, Engineering, and Medicine found evidence for a negative effect of aircraft noise exposure on reading and oral comprehension, standardized assessment performance, and long-term and short-term memory in children at school [ 5 , 61 ]. Specifically, WHO guidelines identify an increased risk of impaired reading and oral comprehension at 55 dB L den , equating to a 1 month delay in reading age, and an additional 1–2 month delay for each 5 dB increase beyond 55 dB L den [ 9 ]. As simulations produced estimates of L dn for the average annual day, assuming 365 days of exposure, we derived this noise level specific risk for each school according to its level of equivalent continuous exposure over a school year duration of 180 days.
Hearing impairment
Environmental noise pollution associated with military airfields and military operating areas can occur at levels that can result in both short- and long-term hearing impairment [ 62 , 63 , 64 ]. An exposure-response curve directly relating cumulative noise exposure to hearing impairment has not been developed at the population scale. Instead, acute noise exposures that could impact hearing were calculated and compared against action levels for occupational noise according to protocols established by the Occupational Safety and Health Administration and the National Institute for Occupational Safety and Health [ 65 , 66 ]. Because this analysis requires measurement of continuous sound levels over time as opposed to cumulative metrics, daily noise exposure doses using a 24-h reference duration (representing potential exposure experienced by residents) were calculated for monitoring locations only. Single-event noise levels were also compared against established thresholds for direct physiological impairment [ 24 , 67 ].
Military aircraft noise regime
Noise events from military aircraft operations often exhibited a characteristic contour, with a fast onset rising to a maximum peak, followed by a gradual decay (Fig. 4 ). The magnitude, onset rate, and duration of events varied by operation and monitoring location across the study region.
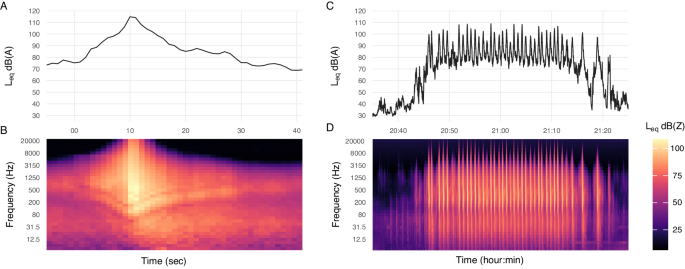
A single FCLP noise event L eq ( A ) and spectrogram ( B ); an FCLP training session ( C , D ). Data recorded at monitoring location 7 on August 10, 2021.
The loudest aircraft noise event measured at each monitoring location ranged from 85.4–119.8 dB L max and 94.3–122.7 dB L E (Supplementary Table S 2 ). At all monitoring locations on Whidbey Island, as well as off-island locations in Port Townsend (location 11) and the Skagit River (location 2), events were measured in excess of 100 dB L max . Noise events during active operations at the MOA location were recorded up to 90 dB L max , 95.4 dB L E . The single loudest event occurred during a touch-and-go operation near OLF Coupeville (location 15) measuring 119.8 dBA L max , 121.1 dBA L Fmax , 136.2 dBC L Cpeak , 122.7 dBA L E , at the time of approximately 23:57.
Spectral energy of aircraft noise occupied an expansive range of the frequency spectrum extending beyond the limits of human audibility. The amount of high frequency noise increased with proximity to aircraft, though substantial amounts of low-frequency energy were present in all noise events, regardless of distance. Energy-averaged Z-weighted levels of one-third octave band spectra from locations within approximately 3 km of an active FCLP session exhibited a broad peak around roughly 300 Hz of 65 to 85 dBZ, and up to 69 dBZ at infrasonic frequencies and 51 dBZ at 20 kHz.
During the four 2020–2021 monitoring periods, Ault Field conducted a wide range of operations, including Olympic MOA departure, arrival, and pattern operations, while the vast majority of operations at OLF Coupeville were FCLPs. Inter-field transit operations were common between both airfields. Ault Field conducted an average of approximately 1134 operations, including 145 FCLPs, per weeklong monitoring period, while OLF Coupeville conducted an average of 760 operations, including 690 FCLPs [ 33 ]. A single FCLP counts as two operations, one for takeoff and one for landing. The average weekly number of combat training operations conducted within the Olympic MOA was 66.
Flight operations were concentrated from Monday through Thursday (91.4%), with less activity on Friday (7.1%) and minimal activity on weekends (1.4%). On Friday, Saturday, and Sunday, operations were only conducted from Ault Field. Roughly 70% of operations occurred during daytime hours, 20% during the evening, and 10% at night. Operations were recorded at all hours except 2:00 and 4:00 AM. FCLP sessions at OLF Coupeville took place during the hours of 11:30 to 23:30, ranging in duration from 30 min to 3 h (lasting approximately 1 h on average), with multiple sessions typically occurring on a single day. Sessions have continued past midnight during other recorded monitoring periods [ 35 ]. The vast majority of operations within the Olympic MOA occurred during weekdays, with roughly 97% occurring during the day, and 3% at night.
L den increased dramatically on days with substantial flight activity. Compared to weekends (little operation at Ault Field, none at OLF Coupeville), weekdays at monitoring locations within and immediately surrounding Whidbey Island exhibited energy-averaged increases ranging from approximately 8–28 dB L den , except for those at Lopez Island (location 3) and Port Townsend (location 11) where differences were negligible. Similarly, L night increased during weekdays, with differences ranging from approximately 3–29 dB. Average hourly L eq increased by up to roughly 35 dB during flight operations, compared to hours when no operations were occurring. As an increase of 10 dB is typically perceived by the human ear as a doubling in loudness, this equates to a roughly 11-fold increase in loudness during hours of operation.
Simulation model validation
The Navy determined that Noisemap modeling “operates as intended and provides an accurate prediction of noise exposure levels from aircraft operations for use in impact assessments and long-term land use planning” [ 37 ]. For each location within the 2020–2021 monitoring period (excluding the Olympic MOA), we compared the estimated L dn from our simulation against results from the Navy’s monitoring study, namely a) the modeled DNL, simulated by Noisemap per-period and then energy-averaged across periods, and b) the real-time measured DNL, calculated from discrete event SEL metrics computed directly from acoustic monitoring data. We found that our simulated results were highly correlated with both the modeled and measured DNL, with Pearson correlation coefficients of 0.99 ( P < 0.001) and 0.97 ( P < 0.001), respectively (Supplementary Fig. S 1 ). The mean difference between our simulated L dn and the modeled DNL was 0.72 dB (min 0, max 3), while the mean difference with the measured DNL was 4.25 dB (min 0.9, max 8.2), falling within the accepted range expected by the Navy’s monitoring programs [ 33 ]. Simulation of airspace activity within the Olympic MOA yielded a cumulative noise exposure of approximately 35 dB L dnmr throughout the area, approximately 4.8 dB less than the measured energy-average of 39.8 dB DNL during periods of operations across the entire 365-day period.
The total area of noise exposure associated with adverse health effects was 1278 km 2 (427.5 km 2 not including water) (Fig. 5 ), with an estimated exposed population of 74,316 people (Fig. 6 ). Exposure was most severe along flight tracks for airbase arrival, departure, and closed-pattern routines, with L dn reaching beyond 90 dB near landing strips at both Ault Field and OLF Coupeville. Interfacility transit operations also substantially contributed to the spatial extent of noise exposure, exhibiting L dn contours of up to 65 dB.
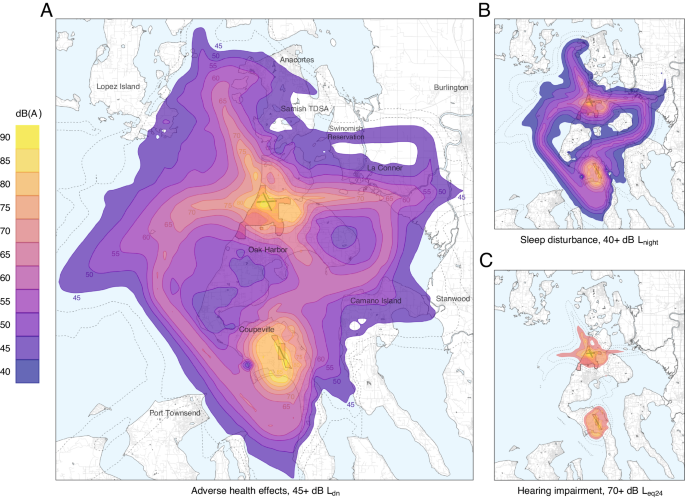
L dn , day-night average sound level, from 45 dB ( A ); L night , night average sound level, from 40 dB ( B ); L eq24 , 24-h equivalent continuous sound level, from 70 dB ( C ). Additional contours below risk thresholds are shown as dotted lines for context.
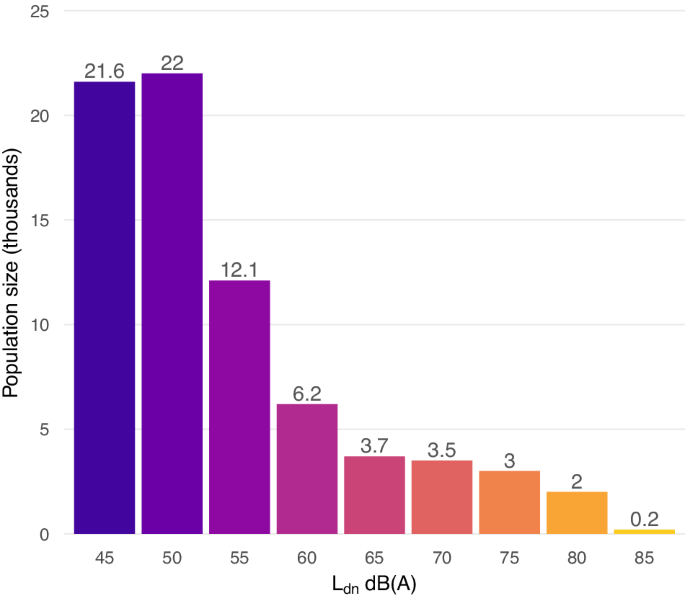
Population size, in thousands, is illustrated per 5 dB L dn of military aircraft noise exposure (lower bound inclusive).
Simulated operations within the Olympic MOA resulted in a cumulative noise exposure of approximately 35 dB L dnmr throughout the area, with measured individual monthly L dn ranging from approximately 34–47 dB L dn . Because the annual average was below the 45 dB L dn threshold, we determined that no health impacts could be estimated for the MOA.
Estimated populations at risk of adverse health outcomes were substantial (Table 1 ). Island County was the most severely impacted region in terms of total number of exposed people (56,813), amounting to approximately two thirds of the total county population. This included all residents of the cities of Oak Harbor and Coupeville. The Swinomish Reservation was the most severely impacted region in terms of the percentage of community exposure, at roughly 85% of the local reservation population. A total of 74,316 people were exposed to day-night average levels of at least 45 dB L den , posing a risk of annoyance [ 9 ], while 41,089 were exposed to nighttime average levels of at least 40 dB L night , posing a risk of adverse effects on sleep [ 9 ]. An estimated 8059 people, all residing within fairly close proximity to Ault Field or OLF Coupeville, were exposed to average noise levels exceeding 70 dBA L eq24 that can induce hearing impairment over time [ 3 , 39 ]. All monitoring locations on Whidbey Island during the same monitoring periods exceeded this level on at least one day, including those lying outside of 70 dBA L eq24 contours. A total of 12,449 people were exposed to 65 dB L dn or greater, noise levels incompatible with residential land use according to regulations set by the Navy, FAA, and US Department of Housing and Urban Development, and eligible for community noise mitigation funds [ 27 , 33 , 47 , 48 , 68 ].
The estimated population health impacts vary according to different published benchmarks (Table 2 ). According to WHO guidelines, 20,840 people were estimated to be highly annoyed. This estimate ranges from 5873 to 36,916 depending on the exposure-response function used (FICON or Yokoshima, respectively). A total of 5265 people were estimated to be exposed to levels at or beyond the defined range of all annoyance exposure-response functions except FICON (75 dB L dn ). Between 8315 to 9770 people were estimated to be highly sleep disturbed (WHO versus Smith). Importantly, 4967 people were estimated to be exposed to levels at or beyond the defined range of sleep disturbance exposure-response functions (65 dB L night ).
Several monitoring locations within roughly 3 km of OLF Coupeville surpassed the recommended exposure limit of the National Institute for Occupational Safety and Health (≥85 dB time-weighted average). Days with multiple FCLP sessions occasionally surpassed exposure thresholds for the FAA Hearing Conservation Program, which would require providing hearing protection and testing for employees [ 69 ]. Multiple noise events at a residence near OLF Coupeville (location 15) reached short-term intensities that may cause direct, acute mechanical damage to the inner ear [ 24 , 67 ].
Six schools were exposed to noise levels associated with increased risk of reduced reading and oral comprehension (≥55 dB L dn , Supplementary Table S 3 ) [ 9 ], with the quantitative risk of a 1–2 month delay in learning per 5 dB increase. The most severely impacted schools (Coupeville Middle School, Coupeville High School, and Crescent Harbor Elementary) had an estimated exposure of 60–63 dB L dn , suggesting the risk of a 2–3 month delay in learning for students. An additional 12 schools were exposed to noise levels within 5 dB of the 55 dB L dn threshold. Columbia College, a postsecondary school near Ault Field, experienced exposure of 70 L dn ; impacts were not able to be estimated, however, given that the average student is older than the ages for which this relationship is defined.
Particularly loud individual aircraft noise events occurred during school hours at schools in proximity of flight tracks. At the field monitoring location nearest Crescent Harbor Elementary (location 1, distance of approximately 1 km), events surpassed 103 dB L max and 113 dB L E . Considering a standard 15–25 dB reduction to approximate indoor levels, noise events of such magnitude are known to interfere with student and teacher conversation and comprehension [ 70 , 71 , 72 ].
Simulation of alternative noise regimes
Simulation of alternative noise regimes revealed a positive relationship between aircraft operations and the estimated population impacted by all health outcomes across a wide range of total operations (Fig. 7 ). This relationship is nonlinear, as it is dependent on both the spatial distribution of the population and the range of levels for which thresholds and exposure-response relationships are defined. A 50% increase in annual operations yielded a 6.8% increase in the total exposed population, while a 50% decrease in operations yielded a 37% decrease in the exposed population. A 50% increase in operations increased cumulative noise levels within the Olympic MOA to no more than 36.4 dB L dnmr .
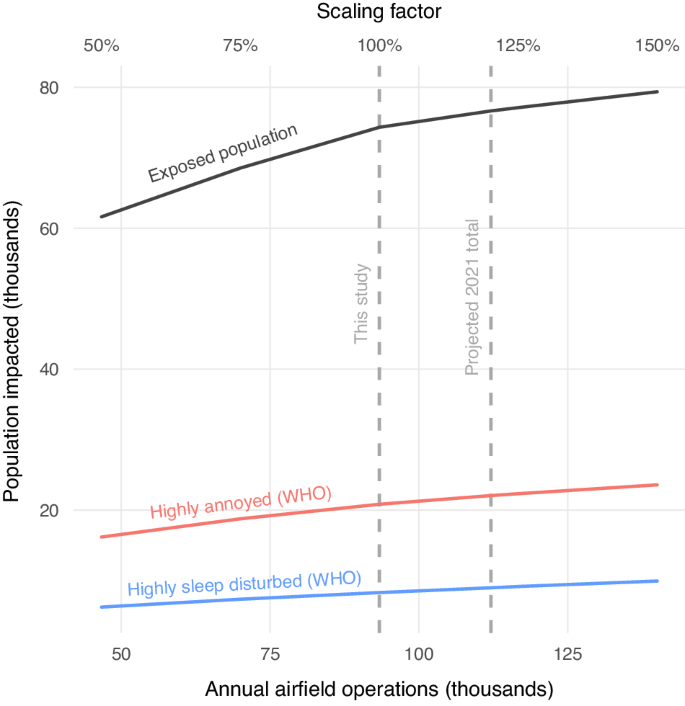
The equivalent annual number of operations for the four monitoring periods and the total number of operations projected for 2021 from the Navy environmental impact statement are both shown as vertical dashed lines.
Pervasive noise pollution from military aviation in western Washington exposes communities to an array of risks and impacts to human health and well-being. A majority of countries, including the United States, lack regulations that limit environmental noise pollution. This supports the needs for approaches, such as the one presented here, that allow for quantifying population health risks and impacts relative to established exposure-response relationships and regulatory guidelines from other noise-focused policies (e.g., occupational noise, land-use compatibility for civilian airports). Through the estimation of potential health burdens and projected increases and decreases in aircraft operations, this approach can inform impacted communities, highlight points of interest and areas that should be prioritized for alleviation and noise mitigation strategies, and better inform aircraft operational design, urban planning, and the development of improved policies to protect the public from the adverse human burden of noise exposure.
Our results suggest that noise exposure due to military aircraft activities within the study region poses a substantial risk and impact to public health. A majority of the resident population of Island County (66%) were exposed to noise levels associated with adverse health effects. Using WHO guidelines, 21% were estimated to be highly annoyed and 9% to be highly sleep disturbed. The Swinomish Indian Tribal Community of the Swinomish Reservation was extremely vulnerable to health risks, with nearly 85% of residents being exposed. The greatest impacts were predicted for populations residing near airfields and flight tracks, though the effects permeate broadly across the landscape. Our predictive modeling of health outcomes focuses on annoyance and sleep disturbance as critical indicators of community health for which sufficient research exists to employ exposure-response relationships. Ultimately, these outcomes are the first responses in a chain of physiological effects that can result in more severe health impacts. Stress responses can be triggered as a downstream consequence of sleep disturbance or the emotional and cognitive perception of sound (annoyance), promoting cardiovascular and psychological risk factors [ 4 , 52 ]. Mounting evidence from recent laboratory and longitudinal studies point to these underlying physiological and neurobiological mechanisms as pathways through which noise increases the risk of, or leads to, the onset of disease [ 4 , 50 , 51 , 52 ]. This strongly suggests that noise exposure should be considered as an environmental risk factor for the development of cardiometabolic and psychological disease and disorder [ 50 ]. While our study did not include a primary assessment of health outcomes from members of the population directly, the scale and severity of the indicators presented here imply considerable and diverse impacts to public health that warrant further investigation.
The estimated extent of population impacts varies widely depending on the standard or exposure-response relationship that is employed. For example, use of the FAA’s newly revised NES exposure-response curve for high annoyance, as opposed to the current FICON standard, increased population health impacts by almost 4-fold. Health impacts can also vary across individuals, and exposure-response relationships are influenced by the life experience and culture of the populations from which they are derived [ 19 ]. This wide range in estimated impacts suggests that there is uncertainty associated with current methods for health assessment, and conventional thresholds governing permissible community exposure, such as the 65 dB L dn contour used by the Navy, FAA, and US Department of Housing and Urban Development for land-use compatibility, may fail to protect populations from the adverse effects of noise exposure they were designed to. Despite this, the exposure-response relationships used in this study are currently employed in health assessments at an international scale, meaning that estimated public health risks and associated costs to society will vary widely. We suggest that variation in population health impact estimates starkly illustrates the need to reduce uncertainties in our understanding of how noise exposure results in human health outcomes and identify levels of permissible exposure informed by the best available science.
Critical gaps remain in our ability to assess health outcomes from military aviation noise specifically, and environmental noise pollution in general. First, an important finding of this study was that a substantial portion of the population was exposed to noise levels at or beyond the defined range of exposure-response relationships. This indicates that these levels of exposure are unprecedented in community noise analyses. Although there are few circumstances outside of close proximity to military airfields where such exposure is likely to be routine, it remains that the expected community response and health impacts from aircraft noise exposure at such extreme levels is unknown. Second, our simulation also produced contours for noise levels below those commonly presented in conventional assessments, but still proven to elicit adverse health effects. For example, domestic assessments often limit contours to 65 dB L dn and above [ 33 ], while the Environmental Noise Directive requires that assessments report population estimates exposed to 55 dB L den and above [ 49 ], despite evidence that adverse human health effects can be experienced at 45 dB L dn [ 9 ]. When considering an entire population, even comparatively low levels of exposure can yield substantial societal effects. As such, we urge future health assessments to consider the entire range of noise exposure known to be harmful to human health.
Perhaps the most significant knowledge gap involves the difference in estimated impacts between the exposure-response curves for noise from military versus civil aircraft [ 19 , 25 ]. This difference is dramatic and warrants further study to understand the role of acoustic (both single-event and cumulative metrics) and non-acoustic indicators in future community health assessments of noise from military aviation. For example, military aircraft noise within the study region exhibited substantial low-frequency energy. The conventional use of A frequency weighting underestimates the contribution of this energy to noise measurements and has been criticized as inadequate to quantify such low-frequency noise events [ 73 , 74 ]. Low-frequency noise propagates farther than higher frequency noise, and therefore A-weighted contours may underestimate the true spatial extent of noise exposure. Additionally, low-frequency noise is not easily attenuated by physical barriers such as walls and windows and can resonate with building structures and the human body, creating secondary effects of rattling and vibration [ 73 , 75 , 76 ]. This minimizes the efficacy of sound insulation and can promote feelings of helplessness in residents [ 73 ]. While the specific health impacts of low-frequency noise exposure remain understudied, evidence suggests that it can elicit a substantially more rapid increase in perceived loudness, annoyance, and sleep disturbance, even at low sound pressure levels [ 3 , 73 , 75 , 76 ]. The WHO and ISO suggest that guidelines for permissible exposure to low-frequency noise should be lowered and rating procedures for the assessment of such noise should be modified [ 11 ]. However, there remains little consensus on what modifications are appropriate due to a dearth of research relating low-frequency noise exposure to health impacts. Future improvements to noise simulation tools such as Noisemap should include the ability to calculate C-weighted levels to better quantify exposure to low-frequency noise.
Military aircraft activity within the study region also exhibited a more sporadic noise regime than that associated with common commercial or civil aviation, which may contribute to more severe health impacts than are predicted by cumulative acoustic metrics such as L dn [ 22 ]. Individual events can have significant implications for health outcomes that cumulative metrics fail to account for. This suggests that communities outside of the 45 dB L dn contour may still be subject to noise exposure that is associated with adverse health effects. For example, although most residents of Port Townsend, Camano Island, Lopez Island, and Forks (a city within the Olympic MOA) reside outside the area that poses a relevant risk of annoyance according to the WHO, these communities have reported high numbers of noise complaints [ 29 ]. This may be exacerbated in areas within the Olympic MOA in particular, such as Forks, due to the intermittent nature of noise events and comparatively reduced presence of other environmental noise [ 18 ]. However, current research linking single-event indicators to long-term population health impacts remains tentative, and as such these metrics are not widely employed in assessment guidelines [ 9 ].
Simulations of alternative noise regimes suggest that population health impacts can be slightly reduced, but definitely not avoided, by decreasing the volume of aircraft operations. While increased operations are associated with more severe health impacts, the spatial extent of noise exposure (and the mere presence of health risks) is driven in particular by the flight pattern of active operations. Therefore, reducing population exposure and subsequent health impacts may best be achieved by strategically discontinuing or altering the flight paths of operations that result in the most egregious impacts, such as FCLP operations, as well as reducing the volume. Changing the timing of operations to avoid sensitive periods, such as school and nighttime hours, may also substantially reduce the risk of associated health impacts. Further opportunities may exist in the creation of subsidy programs for sound insulation or even the purchase of homes in high exposure areas, such as those for commercial airports in the US [ 27 , 71 ] or military airfields abroad [ 20 , 25 ]. However, it should be noted that although sound insulation has been shown to reduce indoor exposure to traffic and commercial aircraft noise [ 77 , 78 ], prior research suggests that it may be ineffective in reducing the high intensity and low-frequency noise associated with military aircraft [ 20 ].
Finally, the spatial extent of noise exposure presented in this study, and thus the magnitude of predicted health risks and impacts, is likely underestimated due to limitations of available data. The scaling of operations occurring within the four weeklong monitoring periods to the entire year amounted to 83% of the volume of total operations suggested by the Navy for all of 2021 [ 30 ]. Compared to our simulation, modeled yearly average L dn values presented in the Navy environmental impact statement are louder at most monitoring locations, some by up to 10.5 dB [ 37 ], and the area of the 65 dB L dn contour is approximately 1.23 times as large (not including water or the NASWI complex) [ 30 ]. This suggests that human health impacts from the true annual noise regime are likely even more severe than those shown here.
The approach presented here improves on existing methods of assessing the population health risk of noise by increasing transparency and reproducibility. Although acute and chronic noise pollution is an issue for many communities worldwide, noise and operations from NASWI have been closely scrutinized for many years. This has led to unique monitoring datasets and a rare legal action that critically examined, and ultimately rejected, the environmental impact statement process and procedures as implemented by the Department of Defense [ 79 ]. Other recent successful lawsuits also underscore the inadequacy of current environmental assessment practices and policies related to noise [ 80 ], and the burden on communities to organize and self-advocate for change [ 81 ]. Our approach offers several benefits and improvements for noise impact assessment. The first is that we rely on published exposure-response relationships to transparently and systematically estimate impacts for multiple health outcomes. These relationships can be expanded or updated as new information becomes available (e.g., exposure-response curves for cardiovascular or psychological impacts). A second improvement lies in the integration of dasymetric population density maps which provide a more accurate estimation of exposed populations, particularly across different types of non-urban areas such as those prevalent in the study region. This is important as noise pollution is not an exclusively urban issue, extending well outside city centers. Finally, this workflow can be used to project the expected magnitude and geography of population health risk resulting from proposed changes in activity, including both increases and decreases in flight operations related to new policy and mitigation strategies (e.g., land use changes, sound insulation programs). We believe that this approach can be employed by both noise producers and affected communities as a basis for common dialogs that extend beyond noise exposure to discuss human health impacts and potential solutions.
Military aviation operations data are typically not available to the public, and noise monitoring around airfields is rare. This study purposefully leveraged datasets that arose from unique policy instruments [ 33 ] and robust monitoring funded by federal agencies [ 21 ] and community organizations [ 35 , 36 ]. We believe this approach offers a roadmap that communities elsewhere can use to effectively plan and implement rigorous noise monitoring and systematically collect operational data. For example, the datasets that facilitated this assessment have common features, including the use of class 1 sound level meters to collect an array of SPL measurements and a robust means to identify individual events automatically or through simultaneous observation for comparison with operations data. The workflow presented makes more accessible the conversion of these raw acoustic data into meaningful metrics for communication. Using this example, community-led initiatives could advocate for government funding to support noise monitoring studies or conduct their own investigations with volunteers. The ongoing evolution of inexpensive monitoring equipment and advances in acoustic analysis increase this likelihood, with strong potential for application of AI-based automated detection of noise events from long-term passive monitoring [ 82 ]. Such datasets collected through community science could provide useful validation of noise modeling and exposure [ 83 , 84 ], although overcoming sporadicity must be considered [ 85 ]. This may offer a much-needed mechanism to organize and advocate for mitigation and could go a long way to alleviating the powerlessness that communities commonly experience related to noise pollution [ 86 ].
By using a modular analytical framework, this approach can be extended to diverse noise sources well beyond the purview of military aircraft, including any context where the spatial extent of noise exposure can be modeled. This offers a promising avenue to bridge monitoring gaps for other environmental noise sources that are poorly documented and monitored yet still affect communities worldwide. The framework can also be readily updated or customized with other health thresholds and exposure-response relationships to serve future assessments in other contexts. The insights obtained through this approach may help better inform efforts in mitigating community noise exposure and developing policy governing noise legislation and land use. We strongly encourage future public health assessments of environmental noise pollution to leverage such a workflow in an effort to obtain a comprehensive understanding of the magnitude of health implications associated with noise exposure at the population scale with the best available science.
Data availability
Code for the methods and workflow routines can be found at the open-source repository github.com/giojacuzzi/noise-pollution-pop-health-naswi [ 87 ]. Noisemap software is available from the US Department of Defense Community and Environmental Noise Primer resources at dodnoise.org/primer_resources. BaseOps version 7.368 was used as the graphical user interface for Noisemap simulation data entry and management in concert with a) Omega10 and Omega11 to calculate sound over distance for aircraft flight operations, ground maintenance, and run-up operations; b) NMap 7.3 and MRNMap to calculate noise exposure values on the ground; c) NMPlot 4.974 to convert calculated noise exposure values to noise contour plots. Data from the 2020–2021 Navy monitoring periods are available from the Naval Facilities Engineering Systems Command Aircraft Sound Monitoring database [ 34 ], including acoustic monitoring data, flight operations data, and noise modeling data. Acoustic monitoring data from the 2015 National Park Service Night Skies and Sounds Division report and 2016 and 2019 JGL Acoustics, Inc. reports are available upon reasonable request. Impervious surface data used in the dasymetric population density mapping are available from the National Land Cover Database [ 46 ]. School geographic locations are available from the National Center for Education Statistics [ 60 ].
Basner M, Babisch W, Davis A, Brink M, Clark C, Janssen S, et al. Auditory and non-auditory effects of noise on health. Lancet. 2014;383:1325–32.
Article PubMed Google Scholar
Stansfeld SA, Matheson MP. Noise pollution: Non-auditory effects on health. Br Med Bull. 2003;68:243–57.
Berglund B, Lindvall T, Schwela DH. Guidelines for community noise. Geneva, Switzerland: World Health Organization; 1999.
Sørensen M, Pershagen G, Thacher JD, Lanki T, Wicki B, Röösli M, et al. Health position paper and redox perspectives - Disease burden by transportation noise. Redox Biol. 2024;69:102995.
Sharp BH, Connor TL, McLaughlin D, Clark C, Stansfeld SA, Hervey J. Assessing Aircraft Noise Conditions Affecting Student Learning, Volume 1: Final Report. Washington, D.C.: Transportation Research Board; 2014.
Swinburn TK, Hammer MS, Neitzel RL. Valuing quiet: An economic assessment of US environmental noise as a cardiovascular health hazard. Am J Preventive Med. 2015;49:345–53.
Article Google Scholar
Hammer MS, Swinburn TK, Neitzel RL. Environmental noise pollution in the United States: Developing an effective public health response. Environ Health Perspect. 2014;122:115–9.
Astley RJ. Can technology deliver acceptable levels of aircraft noise? Inter Noise Noise Con Congr Conf Proc. 2014;249:2622–33.
Google Scholar
World Health Organization. Environmental noise guidelines for the European Region. Geneva, Switzerland: World Health Organization; 2018.
European Environment Agency. Environmental noise in Europe—2020. Luxembourg, Luxembourg: European Environment Agency; 2020.
International Organization for Standardization. Acoustics – Description, measurement and assessment of environmental noise – Part 1: Basic quantities and assessment procedures. ISO 1996-1 Third edition. Geneva, Switzerland: International Organization for Standardization; 2016.
US Department of Transportation, Federal Aviation Administration. Analysis of the Neighborhood Environmental Survey. Washington, DC: US Department of Transportation; 2021.
D'Alessandro F, Schiavoni S. A review and comparative analysis of European priority indices for noise action plans. Sci Total Environ. 2015;518:290–301.
Hammer MS, Fan Y, Hammer SS, Swinburn TK, Weber M, Weinhold D, et al. Applying a novel environmental health framework theory (I-ACT) to noise pollution policies in the United States, United Kingdom, and the Netherlands. J Environ Plan Manag. 2018;61:2111–32.
Perna M, Padois T, Trudeau C, Bild E, Laplace J, Dupont T, et al. Comparison of road noise policies across Australia, Europe, and North America. Int J Environ Res Public Health. 2021;19:173.
Article PubMed PubMed Central Google Scholar
Fidell S. A review of US aircraft noise regulatory policy. Acoust Today. 2015;11:25–34.
Kuehne LM, Erbe C, Ashe E, Bogaard LT, Salerno Collins M, Williams R. Above and below: Military aircraft noise in air and under water at Whidbey Island. Wash J Mar Sci Eng. 2020;8:923.
Kuehne LM, Olden JD. Military flights threaten the wilderness soundscapes of the olympic peninsula, Washington. Northwest Sci. 2020;94:188–202.
Yokoshima S, Morinaga M, Tsujimura S, Shimoyama K, Morihara T. Representative exposure-annoyance relationships due to transportation noises in Japan. Int J Environ Res Public Health. 2021;18:10935.
Matsui T. A Report on the Aircraft Noise as a Public Health Problem in Okinawa. Okinawa, Japan: Department of Culture and Environmental Affairs Okinawa Prefectural Government; 1999.
Pipkin A. Ebey’s Landing National Historical Reserve: Acoustical monitoring report. Natural resource report. NPS/ELBA/NRR—2016/1299. Fort Collins, CO: National Park Service; 2016.
Gelderblom FB, Gjestland TT, Granøien ILN, Taraldsen G. The impact of civil versus military aircraft noise on noise annoyance. Inter Noise Noise Con Congr Conf Proc. 2014;249:786–95.
Waitz IA, Lukachko SP, Lee JJ. Military aviation and the environment: Historical trends and comparison to civil aviation. J Aircr. 2005;42:329–39.
Ising H, Rebentisch E, Poustka F, Curio I. Annoyance and health risk caused by military low-altitude flight noise. Int Arch Occup Environ Health. 1990;62:357–63.
Article CAS PubMed Google Scholar
Morinaga M, Kawai K, Makino K. Aircraft noise annoyance around military airfields in Japan. Inter Noise Noise Con Congr Conf Proc. 2016;253:854–63.
US Department of Defense. DoD Instruction 4715.13 DoD operational noise program. Washington, DC: US Department of Defense; 2020.
Code of Federal Regulations. 14 CFR part 150–Airport noise compatibility planning. Washington, DC: Federal Aviation Administration.
US Department of Transportation. National transportation noise map documentation. Washington, DC: US Department of Transportation; 2020.
Quiet Skies Over San Juan County. Jet noise density maps. 2022. Available from: https://www.quietskies.info/basic-01 .
US Department of the Navy. Final environmental impact statement for EA-18G growler airfield operations at Naval Air Station Whidbey Island Complex. Washington, DC: US Department of the Navy; 2018.
Fox J, Morris L. A summary of the association between noise and health. Olympia, WA: Washington State Department of Health; 2017.
US District Court Western District of Washington at Seattle. Case No. 2:19-cv-01059-RAJ-JRC Document 119. Seattle, WA: US District Court Western District of Washington at Seattle; 2022.
US Department of the Navy. Real-time aircraft sound monitoring final report. Washington, DC: US Department of the Navy; 2021.
Naval Facilities Engineering Systems Command. Aircraft sound monitoring. 2018. Available from: https://www.navfac.navy.mil/Directorates/Public-Works/Products-and-Services/Aircraft-Sound-Monitoring/ .
Lilly J. Military jet noise measurements OLF Coupeville Whidbey Island, WA. Issaquah, WA: JGL Acoustics, Inc.; 2020.
Lilly J. Whidbey Island military jet noise measurements. Issaquah, WA: JGL Acoustics, Inc.; 2016.
Downing M, Gillis J, Manning B, Mellon J, Calton M. Navy real-time aircraft sound monitoring study: Technical report. Asheville, NC: Blue Ridge Research and Consulting, LLC; 2022.
International Organization for Standardization. Acoustics – Unattended monitoring of aircraft sound in the vicinity of airports. ISO 20906, 1st ed. Geneva, Switzerland: International Organization for Standardization; 2009.
US Environmental Protection Agency. Information on levels of environmental noise requisite to protect public health and welfare with an adequate margin of safety. Washington, DC: US Environmental Protection Agency; 1974.
Federal Interagency Committee on Noise. Federal agency review of selected airport noise analysis issues. Washington, DC: Federal Interagency Committee on Noise; 1992.
SAE International. ARP4721. Monitoring aircraft noise and operations in the vicinity of airports: System description, acquisition, and operation. Warrendale, PA: SAE International; 2021.
US Air Force Civil Engineer Center. BaseOps 7.368 User’s Guide. 2023. Available from: http://dodnoise.org/resources/pdfs/BaseOps_User_Guide.pdf .
Jelinski DE, Wu J. The modifiable areal unit problem and implications for landscape ecology. Landsc Ecol. 1996;11:129–40.
Tiecke TG, Liu X, Zhang A, Gros A, Li N, Yetman G, et al. Mapping the world population one building at a time. arXiv. 2017;1712:05839.
Swanwick RH, Read QD, Guinn SM, Williamson MA, Hondula KL, Elmore AJ. Dasymetric population mapping based on US census data and 30-m gridded estimates of impervious surface. Sci Data. 2022;9:523.
Yang L, Jin S, Danielson P, Homer C, Gass L, Bender SM, et al. A new generation of the United States National Land Cover Database: Requirements, research priorities, design, and implementation strategies. ISPRS J Photogramm Remote Sens. 2018;146:108–23.
US Department of Defense. Air installations compatible use zones program. Washington, DC: US Department of Defense; 2022.
Code of Federal Regulations. 24 CFR B–Noise abatement and control. Washington, DC: US Department of Housing and Urban Development; 2013.
European Parliament and the Council of the European Union. Directive 2002/49/EC. Strasbourg, France: European Parliament and the Council of the European Union; 2002.
Münzel T, Sørensen M, Daiber A. Transportation noise pollution and cardiovascular disease. Nat Rev Cardiol. 2021;18:619–36.
Osborne MT, Radfar A, Hassan MZO, Abohashem S, Oberfeld B, Patrich T, et al. A neurobiological mechanism linking transportation noise to cardiovascular disease in humans. Eur Heart J. 2020;41:772–82.
Hahad O, Kuntic M, Al-Kindi S, Kuntic I, Gilan D, Petrowski K, et al. Noise and mental health: evidence, mechanisms, and consequences. J Exp Sci Environ Epidemiol. 2024;26;1–8.
Babisch W, Kamp I. Exposure-response relationship of the association between aircraft noise and the risk of hypertension. Noise Health. 2009;11:161.
Vienneau D, Schindler C, Perez L, Probst-Hensch N, Röösli M. The relationship between transportation noise exposure and ischemic heart disease: a meta-analysis. Environ Res. 2015;138:372–80.
Van Kempen E, Casas M, Pershagen G, Foraster M. WHO Environmental Noise Guidelines for the European Region: A Systematic Review on Environmental Noise and Cardiovascular and Metabolic Effects: A Summary. Int J Environ Res Public Health. 2018;15:379.
Clark C, Paunovic K. WHO Environmental Noise Guidelines for the European Region: A Systematic Review on Environmental Noise and Quality of Life. Wellbeing Ment Health Int J Environ Res Public Health. 2018;15:2400.
European Commission. Position paper on dose response relationships between transportation noise and annoyance. Luxembourg, Luxembourg: European Commission; 2002.
Smith MG, Cordoza M, Basner M. Environmental noise and effects on sleep: An update to the WHO systematic review and meta-analysis. Environ Health Perspect. 2022;130:076001.
Kim SJ, Chai SK, Lee KW, Park JB, Min KB, Kil HG, et al. Exposure–response relationship between aircraft noise and sleep quality: A community-based cross-sectional study. Osong Public Health Res Perspect. 2014;5:108–14.
National Center for Education Statistics. School locations & geoassignments. 2019. Available from: https://nces.ed.gov/programs/edge/geographic/schoollocations .
Clark C, Paunovic K. WHO environmental noise guidelines for the European region: A systematic review on environmental noise and cognition. Int J Environ Res Public Health. 2018;15:285.
Ising H, Joachims Z, Babisch W, Rebentisch E. Effects of military low-altitude flight noise part I: Temporary threshold shift in humans. Z fur Audiologie. 1999;38:118–27.
Kuronen P, Pääkkönen R, Savolainen S. Low-altitude overflights of fighters and the risk of hearing loss. Aviat Space Environ Med. 1999;70:650–5.
CAS PubMed Google Scholar
Hiramatsu K, Matsui T, Ito A, Miyakita T, Osad Y, Yamamoto T. The Okinawa study: An estimation of noise-induced hearing loss on the basis of the records of aircraft noise exposure around Kadena Air Base. J Sound Vib. 2004;277:617–25.
Occupational Safety and Health Administration. 1910.95 Appendix A - Noise Exposure Computation. Washington, DC: Occupational Safety and Health Administration; 2021.
US Department of Health and Human Services National Institute for Occupational Safety and Health. Occupational noise exposure; criteria for a recommended standard. Washington, DC: US Department of Health and Human Services National Institute for Occupational Safety and Health; 1998.
Babisch W. Noise and Health. Environ Health Perspect. 2005;113:A14–5.
US Department of Defense. Community noise mitigation. 2021. Available from: https://oldcc.gov/our-programs/community-noise-mitigation .
US Department of Transportation, Federal Aviation Administration. Flight standards service hearing conservation program. Order 3900.66A. Washington, DC: US Department of Transportation; 2021.
US Department of Defense. Improving aviation noise planning, analysis and public communication with supplemental metrics. Washington, DC: US Department of Defense; 2009.
Lind SJ, Pearsons K, Fidell S. Sound insulation requirements for mitigation of aircraft noise impact on Highline school district facilities volume I. BBN Systems and Technologies, BBN Report No. 8240. Cambridge, MA: BBN Systems and Technologies; 1998.
Sharp BH, Plotkin KJ. Selection of noise criteria for school classrooms. Arlington, VA: Wyle Research; 1984.
Berglund B, Hassmén P, Job RFS. Sources and effects of low‐frequency noise. J Acoust Soc Am. 1996; 99:2985–3002.
Committee on Technology for a Quieter America. Technology for a Quieter America. Washington, DC: National Academies Press; 2010.
Vos J, Houben MMJ. Annoyance caused by the low-frequency sound produced by aircraft during takeoff. J Acoust Soc Am. 2022;152:3706.
Persson K, Björkman M. Rylander R. Loudness, annoyance and dBA in evaluating low frequency sounds. J Low Frequency Noise, Vib Act Control. 1990;9:32–45.
Ehrlich GE, Gurovich Y. A Typical Case Study of School Sound Insulation. Arlington, VA: Sound and Vibration; 2004.
Xie J, Zhu L, Lee HM. Aircraft Noise Reduction Strategies and Analysis of the Effects. Int J Environ Res Public Health. 2023;20:1352.
Kelly JK. The sound of freedom at Naval Air Station Whidbey: Environmental impact review under the National Historic Preservation Act and National Environmental Policy Act. Villanova Environ Law J. 2020;31:3.
Mitsuzumi S. Okinawans file mass lawsuit over U.S. base noise pollution. The Asahi Shimbun. 2022. Available from: https://www.asahi.com/ajw/articles/14535305 .
Lorenzana R, Cherrier M, Seto E, Curle D, Albert J, Wong L, et al. Beacon Hill Seattle noise measurement project. OSF. 2019. Available from: http://osf.io/fn5z6 .
Morinaga M, Mori J, Yamamoto I. Aircraft model identification using convolutional neural network trained by those noises in a wide area around an airfield. Acoustical Sci Technol. 2023;44:131–6.
Noise Project. Homepage. 2023. Available from: https://noiseproject.org/ .
Challéat S, Farrugia N, Gasc A, Froidevaux J, Hatlauf J, Dziock F, et al. Silent Cities. 2023. Available from: https://osf.io/h285u/ .
Aceves-Bueno E, Adeleye AS, Feraud M, Huang Y, Tao M, Yang Y, et al. The accuracy of citizen science data: A quantitative review. Bull Ecol Soc Am. 2017;98:278–90.
Dreger S, Schüle SA, Hilz LK, Bolte G. Social inequalities in environmental noise exposure: A review of evidence in the WHO European region. Int J Environ Res Public Health. 2019;16:1011.
Jacuzzi G. giojacuzzi/noise-pollution-pop-health-naswi: 1.0. Zenodo. Available from: https://doi.org/10.5281/zenodo.10652377 (2024).
Download references
Acknowledgements
We are grateful to individuals and organizations that shared and facilitated access to noise monitoring data: Jerry Lilly of JGL Acoustics, Inc., and Ashley Pipkin of the National Park Service Night Skies and Natural Sounds Division. We appreciate the contributions and feedback of many community members and stakeholders that provided input on the project design and interim results via three community webinars. We also thank three anonymous reviewers for their helpful suggestions in improving the manuscript.
Funding for this study was provided by a Tier 2 research grant from the University of Washington’s Population Health Initiative.
Author information
Authors and affiliations.
School of Aquatic and Fishery Sciences, College of the Environment, University of Washington, Seattle, WA, 98195, USA
Giordano Jacuzzi & Julian D. Olden
Omfishient Consulting, Bremerton, WA, 98310, USA
Lauren M. Kuehne
Sound Defense Alliance, PO Box 373, Coupeville, WA, 98239, USA
Anne Harvey & Christine Hurley
Citizens of Ebey’s Reserve, PO Box 202, Coupeville, WA, 98239, USA
Robert Wilbur
School of Public Health, University of Washington, Seattle, WA, 98195, USA
Edmund Seto
You can also search for this author in PubMed Google Scholar
Contributions
GJ: Methodology, software, validation, formal analysis, data curation, writing (original draft), writing (review and editing), visualization. LMK: Conceptualization, methodology, validation, investigation, data curation, writing (review and editing), funding acquisition. AH: Conceptualization, writing (review and editing), funding acquisition. CH: Conceptualization, data curation, writing (review and editing), funding acquisition. RW: Conceptualization, data curation, writing (review and editing), funding acquisition. ES: Conceptualization, methodology, writing (review and editing), funding acquisition. JDO: Conceptualization, methodology, formal analysis, writing (review and editing), supervision, funding acquisition.
Corresponding author
Correspondence to Giordano Jacuzzi .
Ethics declarations
Competing interests.
The authors declare no competing interests.
Ethical approval
Ethical approval was not required for the purposes of this study as no research was conducted with personal human data. Data from the US Census Bureau and American Community Survey is anonymized.
Additional information
Publisher’s note Springer Nature remains neutral with regard to jurisdictional claims in published maps and institutional affiliations.
Supplementary information
Supplementary information, rights and permissions.
Open Access This article is licensed under a Creative Commons Attribution 4.0 International License, which permits use, sharing, adaptation, distribution and reproduction in any medium or format, as long as you give appropriate credit to the original author(s) and the source, provide a link to the Creative Commons licence, and indicate if changes were made. The images or other third party material in this article are included in the article’s Creative Commons licence, unless indicated otherwise in a credit line to the material. If material is not included in the article’s Creative Commons licence and your intended use is not permitted by statutory regulation or exceeds the permitted use, you will need to obtain permission directly from the copyright holder. To view a copy of this licence, visit http://creativecommons.org/licenses/by/4.0/ .
Reprints and permissions
About this article
Cite this article.
Jacuzzi, G., Kuehne, L.M., Harvey, A. et al. Population health implications of exposure to pervasive military aircraft noise pollution. J Expo Sci Environ Epidemiol (2024). https://doi.org/10.1038/s41370-024-00670-1
Download citation
Received : 17 October 2023
Revised : 22 March 2024
Accepted : 27 March 2024
Published : 09 May 2024
DOI : https://doi.org/10.1038/s41370-024-00670-1
Share this article
Anyone you share the following link with will be able to read this content:
Sorry, a shareable link is not currently available for this article.
Provided by the Springer Nature SharedIt content-sharing initiative
- Noise assessment
- Environmental noise
- Exposure-response
Quick links
- Explore articles by subject
- Guide to authors
- Editorial policies

ORIGINAL RESEARCH article
Rheumatoid arthritis increases the risk of heart failure: results from the cross-sectional study in the us population and mendelian randomization analysis in the european population.
- 1 Department of Cardiology, First Affiliated Hospital of Xinjiang Medical University, Urumqi, China
- 2 Center for Reproductive Medicine, Chongqing Health Center for Women and Children, Women and Children's Hospital of Chongqing Medical University, Chongqing, Chongqing Municipality, China
- 3 ChongQing College of Electronic Engineering, Chongqing, China
- 4 Wuchang Hospital, Wuhan, Hubei Province, China
- 5 Second Affiliated Hospital, Chongqing Medical University, Chongqing, China
- 6 Xinjiang Medical University, Ürümqi, Xinjiang Uyghur Region, China
- 7 Suqian Hospital , Affiliated to Nanjing University of Chinese Medicine, Suqian, Jiangsu Province, China
- 8 Third Affiliated Hospital of Wenzhou Medical University, Wenzhou, Zhejiang Province, China
- 9 Beijing Shijitan Hospital, Capital Medical University, Beijing, Beijing Municipality, China
The final, formatted version of the article will be published soon.
Select one of your emails
You have multiple emails registered with Frontiers:
Notify me on publication
Please enter your email address:
If you already have an account, please login
You don't have a Frontiers account ? You can register here
Objective: Rheumatoid arthritis (RA) is a chronic systemic autoimmune disease. Among its various complications, heart failure (HF) has been recognized as the second leading cause of cardiovascular death in RA patients. The objective of this study was to investigate the relationship between RA and HF using epidemiological and genetic approaches.The study included 37,736 participants from the 1999-2020 National Health and Nutrition Examination Survey. Associations between RA and HF in the US population were assessed with weighted multivariate logistic regression analysis. A two-sample Mendelian randomization (MR) analysis was employed to establish the causal relationship between the two variables. The primary analysis method utilized was inverse variance weighting (IVW). Additionally, horizontal pleiotropy and heterogeneity were assessed to account for potential confounding factors. In cases where multiple independent datasets were accessible during MR analysis, we combined the findings through a meta-analytical approach.In observational studies, the prevalence of HF in combination with RA reached 7.11% (95%CI 5.83 to 8.39). RA was positively associated with an increased prevalence of HF in the US population [odds ratio (OR):1.93, 95% confidence interval (CI):1.47-2.54, P<0.001]. In a MR analysis utilizing a meta-analytical approach to amalgamate the results of the IVW method, we identified a significant causal link between genetically predicted RA and a heightened risk of HF (OR = 1.083, 95% CI: 1.028-1.141; P = 0.003). However, this association was not deemed significant for seronegative RA (SRA) (OR = 1.028, 95% CI: 0.992-1.065; P = 0.126). These findings were consistent across sensitivity analyses and did not indicate any horizontal pleiotropy.Conclusions: RA correlates with an elevated prevalence of HF within the US population. Furthermore, genetic evidence derived from European populations underscores a causal link between RA and the risk of HF. However this association was not significant in SRA.
Keywords: Rheumatoid arthritis, seronegative rheumatoid arthritis, Heart Failure, National Health and Nutrition Examination Survey, Mendelian randomization
Received: 27 Jan 2024; Accepted: 08 May 2024.
Copyright: © 2024 Kadier, Zhang, Li, Kuang, Huang, Cai, Ling, Kong and Liu. This is an open-access article distributed under the terms of the Creative Commons Attribution License (CC BY) . The use, distribution or reproduction in other forums is permitted, provided the original author(s) or licensor are credited and that the original publication in this journal is cited, in accordance with accepted academic practice. No use, distribution or reproduction is permitted which does not comply with these terms.
* Correspondence: Xiaozhu Liu, Beijing Shijitan Hospital, Capital Medical University, Beijing, Beijing Municipality, China
Disclaimer: All claims expressed in this article are solely those of the authors and do not necessarily represent those of their affiliated organizations, or those of the publisher, the editors and the reviewers. Any product that may be evaluated in this article or claim that may be made by its manufacturer is not guaranteed or endorsed by the publisher.

Decoding the three ancestral components of the Japanese people
A research group led by scientists from the RIKEN Center for Integrative Medical Sciences in Japan have used whole-genome sequencing to unravel the complex ancestry of the Japanese population. The analysis supports an earlier study that proposed that the Japanese population originated from admixtures of three ancestral groups, challenging the long-held dual-structure model, and also provides new evidence of Neanderthal and Denisovan influences in the population.
The study, published in Science Advances , started with the group performing whole-genome sequencing on 3,256 samples from the Biobank Japan -- a large collection of samples collected from hospitals in Japan. The samples were chosen to reflect the population distribution of Japanese throughout the archipelago, from Hokkaido in the northeast to Okinawa and the other Ryukyu islands in the south.
The first finding was that the Japanese population can be optimally divided into three clusters, with different concentrations in Okinawa, Northeastern Japan, and Western Japan. While it was previously believed that the current Japanese people are mainly descended either from the indigenous Jomon population or other East Asians, principally Han Chinese, the research group found that there is also a Northeast Asian ancestry, which is likely composed of people from the Korean peninsula or from early non-Jomon Japanese populations. They found that Jomon ancestry was most dominant in Okinawa, where it made up 28.5 percent of the sampled people's DNA, though it also made up roughly 19 percent in Northeastern Japan and 12 percent in Western Japan, and was slightly higher in the south of Japan, closest to Okinawa. The East Asian ancestry, generally understood to be from China, was most common in Western Japan, gradually decreasing to the east, while the Northeast Asian lineage was most common in Northeastern Japan, decreasing to the west.
To see whether these findings could provide insights into clinically relevant genetic variants, they examined two well-known pathogenic variants associated with hereditary breast cancer. The first, a variant of the BRCA1 gene, is only found in Japanese populations, and is more common in the east than the west of the country. This implied that it arose in Japanese people with Northeast Asian ancestry and then spread westward. The other, a variant of the BRCA2 gene, is more common in the west, and is also found in China and Korea, leading to the conclusion that this variant was likely brought into Japan from continental Asia.
The study also offered insights into gene sequences derived from Neanderthals and Denisovans -- extinct human lineages that existed together with modern humans and left us genes through a process known as introgression, a gradual movement of genes from one lineage into another through hybridization. Specifically, they found gene sequences associated with type 2 diabetes and height derived from Denisovans, and while they mostly confirmed previous studies regarding gene introgressions from Neanderthals, they also found an interesting link to another gene associated with type 2 diabetes, which might affect sensitivity to semaglutide, an oral agent used to treat the disease.
According to Chikashi Terao, leader of the research group, "Though our findings were interesting because of the new insights they have given us into the origins of the Japanese people, we also believe that our work will serve as a reference for future genetic research beyond the Japanese population, as it could be used in areas such as personalized medicine."
- Personalized Medicine
- Gene Therapy
- Human Biology
- Social Issues
- Racial Disparity
- STEM Education
- Public Health
- Ethnic group
- Population dynamics of fisheries
- Acupuncture
- Bioinformatics
- Public health
- Artificial neural network
Story Source:
Materials provided by RIKEN . Note: Content may be edited for style and length.
Journal Reference :
- Xiaoxi Liu, Satoshi Koyama, Kohei Tomizuka, Sadaaki Takata, Yuki Ishikawa, Shuji Ito, Shunichi Kosugi, Kunihiko Suzuki, Keiko Hikino, Masaru Koido, Yoshinao Koike, Momoko Horikoshi, Takashi Gakuhari, Shiro Ikegawa, Kochi Matsuda, Yukihide Momozawa, Kaoru Ito, Yoichiro Kamatani, Chikashi Terao. Decoding triancestral origins, archaic introgression, and natural selection in the Japanese population by whole-genome sequencing . Science Advances , 2024; 10 (16) DOI: 10.1126/sciadv.adi8419
Cite This Page :
Explore More
- Controlling Shape-Shifting Soft Robots
- Brain Flexibility for a Complex World
- ONe Nova to Rule Them All
- AI Systems Are Skilled at Manipulating Humans
- Planet Glows With Molten Lava
- A Fragment of Human Brain, Mapped
- Symbiosis Solves Long-Standing Marine Mystery
- Surprising Common Ideas in Environmental ...
- 2D All-Organic Perovskites: 2D Electronics
- Generative AI That Imitates Human Motion
Trending Topics
Strange & offbeat.
Colon-cancer cases have more than doubled in young people since 1999, new research finds
- Cancer rates in Americans under 45 have surged over the past two decades, researchers found.
- Diagnoses are increasing most rapidly in teens and people in their early 20s.
- Factors such as genetics, alcohol use, diet, and physical activity may affect cancer risk.

There's new evidence that colon-cancer rates have skyrocketed in people younger than 45.
A team of researchers led by Dr. Islam Mohamed, a physician at the University of Missouri-Kansas City, analyzed CDC data on colon-cancer cases in Americans aged 10 to 44 over two decades since 1999.
The team found that cases have more than tripled in teenagers and more than doubled for people in their early 20s.
While the biggest jump in cancer diagnoses was in younger people, the surge in cancer rates was significant across all age groups under 45:
- Late 20s to early 30s: The rate of Americans diagnosed with colon cancer in this age range increased about 70% from 1999 to 2020.
- Late 30s: For this age group, the rate increased 58% over the same time period.
- Early 40s: This group had the highest overall rate. Cancer cases increased by 45% for Americans in their early 40s between 1999 and 2020.
The researchers plan to present the study at Digestive Disease Week, a meeting for professionals in gastroenterology and related fields, in Washington, DC, later this month.
They say young people need to be better educated about the disease and how to prevent and treat it.
Related stories
"Colorectal cancer is no longer considered just a disease of the elderly population," Mohamed said in a press release. "It's important that the public is aware of signs and symptoms of colorectal cancer."
Colon-cancer signs to look out for
Regular testing can be particularly important for colon cancer because its symptoms are harder to recognize until the disease reaches more advanced stages.
Mohamed said the most common early signs of colon cancer include abdominal pain, rectal bleeding, iron deficiency, and changes to bathroom habits like diarrhea or constipation.
Though the increase is striking, the overall number of colon-cancer diagnoses in young Americans is still low. In 2020, there were 1.3 cases per 100,000 teenagers and two cases per 100,000 people in their early 20s.
The CDC says colonoscopy screenings are only recommended for people 45 and older.
Strategies to prevent colon cancer
Data suggests that people who consume a lot of processed foods and added sugar have a higher risk of developing colon cancer. Cutting back on those foods could help people avoid the disease.
To reduce your chances of developing colon cancer , doctors recommend eating a high-fiber diet and avoiding tobacco and alcohol. Regular exercise has also been linked to a lower risk of multiple types of cancer.
However, colon-cancer risk can also be genetic. And there's ongoing research is investigating whether the gut microbiome , or bacteria in the digestive system, plays a role in colon cancer and if potential risk factors include food additives or some antibiotics that disrupt microbes in the gut.
Watch: Why more young people are getting cancer
- Main content
Maternal treatment with selective serotonin reuptake inhibitors during pregnancy and delayed neonatal adaptation: a population-based cohort study
Selective serotonin reuptake inhibitor (SSRI) use is common in pregnancy. It is associated with delayed neonatal adaptation. Most previous studies have not adjusted for the severity of maternal mental health disorders or examined the impact of SSRI type and dosage. We examined whether treatment with SSRIs in late pregnancy (after 20 weeks) is associated with delayed neonatal adaptation independent of maternal depression and anxiety. Retrospective population-based birth cohort of 280 090 term infants born at 15 Kaiser Permanente Northern California hospitals, 2011-2019. Individual-level pharmacy, maternal, pregnancy and neonatal data were obtained from electronic medical records. Dispensed maternal SSRI prescription after 20 weeks of pregnancy. Delayed neonatal adaptation defined as a 5 min Apgar score ≤5, resuscitation at birth or admission to a neonatal intensive care unit for respiratory support. Secondary outcomes included each individual component of the primary outcome and more severe neonatal outcomes (pulmonary hypertension, hypoxic-ischaemic encephalopathy and seizures). 7573 (2.7%) infants were exposed to SSRIs in late pregnancy. Delayed neonatal adaptation occurred in 11.2% of exposed vs 4.4% of unexposed infants (relative risk 2.52 (95% CI 2.36 to 2.70)). After multivariable adjustment, there was an association between SSRI exposure and delayed neonatal adaptation (adjusted OR 2.14 (95% CI 1.96 to 2.32)). This association was dose dependent. Escitalopram and fluoxetine were associated with the highest risk of delayed neonatal adaptation. Infants exposed to SSRIs have increased risks of delayed adaptation in a type and dose-dependent relationship, pointing toward a causal relationship.
Authors: Cornet, Marie-Coralie;Wu, Yvonne W;Forquer, Heather;Avalos, Lyndsay A;Sriram, Achyuth;Scheffler, Aaron W;Newman, Thomas B;Kuzniewicz, Michael W
Arch Dis Child Fetal Neonatal Ed. 2024 Apr 18;109(3):294-300. Epub 2024-04-18.
PubMed abstract
Explore all studies and publications
Division of Research 4480 Hacienda Drive Pleasanton, CA 94588
Privacy Policy | Terms and Conditions
Follow us on these external social media sites that will open in a new browser window.
© 2024 Kaiser Permanente Division of Research
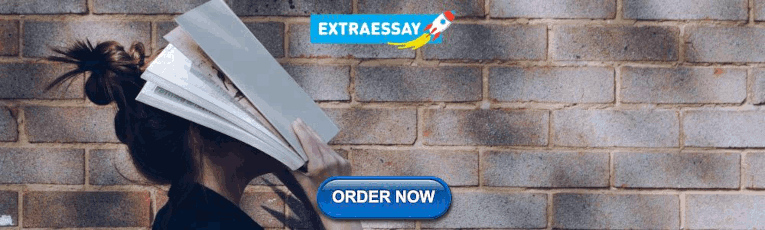
IMAGES
VIDEO
COMMENTS
A population is the entire group that you want to draw conclusions about.. A sample is the specific group that you will collect data from. The size of the sample is always less than the total size of the population. In research, a population doesn't always refer to people. It can mean a group containing elements of anything you want to study, such as objects, events, organizations, countries ...
The research population is defined based on the research objectives and the specific parameters or attributes under investigation. For example, in a study on the effects of a new drug, the research population would encompass all individuals who could potentially benefit from or be affected by the medication.
A population is a complete set of people with specified characteristics, while a sample is a subset of the population. 1 In general, most people think of the defining characteristic of a population in terms of geographic location. However, in research, other characteristics will define a population.
A study population is a group considered for a study or statistical reasoning. The study population is not limited to the human population only. It is a set of aspects that have something in common. They can be objects, animals, measurements, etc., with many characteristics within a group. For example, suppose you are interested in the average ...
A population is the entire group that you want to draw conclusions about. A sample is the specific group that you will collect data from. The size of the sample is always less than the total size of the population. In research, a population doesn't always refer to people. It can mean a group containing elements of anything you want to study ...
In trying to answer the research question posed by a study, determining the appropriate study population is essential to determining the sample size and whether the research question can be answered. For example, in a randomized trial, if a study population has a low event rate, then a much larger sample size may be needed, which may impact the ...
Research studies are usually carried out on sample of subjects rather than whole populations. The most challenging aspect of fieldwork is drawing a random sample from the target population to which the results of the study would be generalized. ... A population for a research study may comprise groups of people defined in many different ways ...
Study population is a subset of the target population from which the sample is actually selected. It is broader than the concept sample frame.It may be appropriate to say that sample frame is an operationalized form of study population. For example, suppose that a study is going to conduct a survey of high school students on their social well-being. ...
A population commonly contains too many individuals to study conveniently, so an investigation is often restricted to one or more samples drawn from it. A well chosen sample will contain most of the information about a particular population parameter but the relation between the sample and the population must be such as to allow true inferences ...
Defining a study population early in the design stages of a research project will help to facilitate a smooth implementation phase. Clear definitions inform the value of applying research results to relevant populations for real world purposes. Importantly, a carefully and accurately defined study population enhances the completed study's ...
A research population is generally a large collection of individuals or objects that is the main focus of a scientific query. It is for the benefit of the population that researches are done. However, due to the large sizes of populations, researchers often cannot test every individual in the population because it is too expensive and time ...
The sampling frame intersects the target population. The sam-ple and sampling frame described extends outside of the target population and population of interest as occa-sionally the sampling frame may include individuals not qualified for the study. Figure 1. The relationship between populations within research.
Tell your students that you will read a scenario and they must decide on whether the research scenario relates to a population or a sample. If it is a sample, they must identify the type of sample ...
which a study population can be constrained by the criteria for inclusion of the study subjects. ... research question being asked. If a group is excluded, you will have to consider the characteristics of that group and consider the effect of their exclusion on your results. For example, the age distribution of students is concentrated
Population study is an interdisciplinary field of scientific study that uses various statistical methods and models to analyse, determine, address, and predict population challenges and trends from data collected through various data collection methods such as population census, registration method, sampling, and some other systems of data ...
Findings. Four propositions emerge: (1) the meaningfulness of means depends on how meaningfully the populations are defined in relation to the inherent intrinsic and extrinsic dynamic generative relationships by which they are constituted; (2) structured chance drives population distributions of health and entails conceptualizing health and disease, including biomarkers, as embodied phenotype ...
design, population of interest, study setting, recruit ment, and sampling. Study Design. The study design is the use of e vidence-based. procedures, protocols, and guidelines that provide the ...
The importance of differentiating between the population and. target population in research cannot be overemphasised as. these provide a backbone of any research study. Research. methods of any ...
researcher aims to study and draw conclusions about (Jilcha Sileyew, 2020; Garg, 2016). Defining the population is a critical step in research design as it sets the boundaries and scope of the study's findings. In teaching and learning research, the population could be any specific group of interest, such as
This study is a correlational study and the sample of this study is 160 students in the second year of the English Department at Tikrit University in the academic year 2021/2022, and the data is ...
The aim of the research is to generalize the findings to the target population via your sample. In research, there are 2 kinds of populations: the target pop- ulation and the accessible population. The accessible popula- tion is exactly what it sounds like, the subset of the target population that we can easily get our hands on to conduct our ...
Dowd is Professor of Demography and Population Health and Deputy Director of the Leverhulme Centre for Demographic Science at the University of Oxford. In her address, she spoke about the data and insights from her five-year project funded by the European Research Council (ERC) studying the forces stalling life expectancy gains in the U.S. and UK.
Studies are conducted on samples because it is usually impossible to study the entire population. Conclusions drawn from samples are intended to be generalized to the population, and sometimes to the future as well. The sample must therefore be representative of the population. This is best ensured by the use of proper methods of sampling.
Study region. Military training operations at NASWI originate from two primary airfields on Whidbey Island, Washington State, USA (Fig. 1).Ault Field is located approximately 5 km from the city of ...
This article is part of the Research Topic Immune system disorders: from molecular mechanisms to clinical implications View all 21 articles. Rheumatoid arthritis increases the risk of heart failure: results from the cross-sectional study in the US population and mendelian randomization analysis in the European population
A research group have used whole-genome sequencing to unravel the complex ancestry of the Japanese population. The analysis supports an earlier study that proposed that the Japanese population ...
New research says there's been a sharp rise in cases of colon cancer in Americans under 45. ... The researchers plan to present the study at Digestive Disease Week, a meeting for professionals in ...
Selective serotonin reuptake inhibitor (SSRI) use is common in pregnancy. It is associated with delayed neonatal adaptation. Most previous studies have not adjusted for the severity of maternal mental health disorders or examined the impact of SSRI type and dosage. We examined whether treatment with SSRIs in late pregnancy (after 20 weeks) is associated with…
It is the ability of the test to detect a difference in the sample, when it exists in the target population. Calculated as 1-Beta. The greater the power, the larger the required sample size will be. A value between 80%-90% is usually used. Relationship between non-exposed/exposed groups in the sample.
contact us / faq. contact us; advertising; career opportunities; top