Get science-backed answers as you write with Paperpal's Research feature
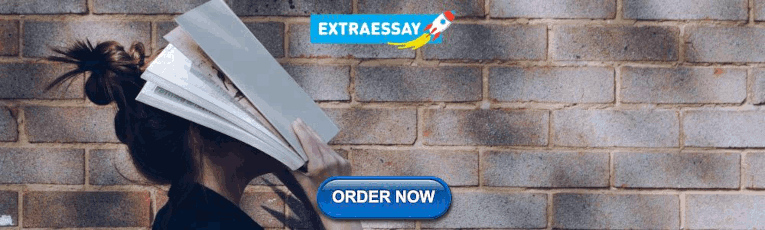
How to Write a Conclusion for Research Papers (with Examples)
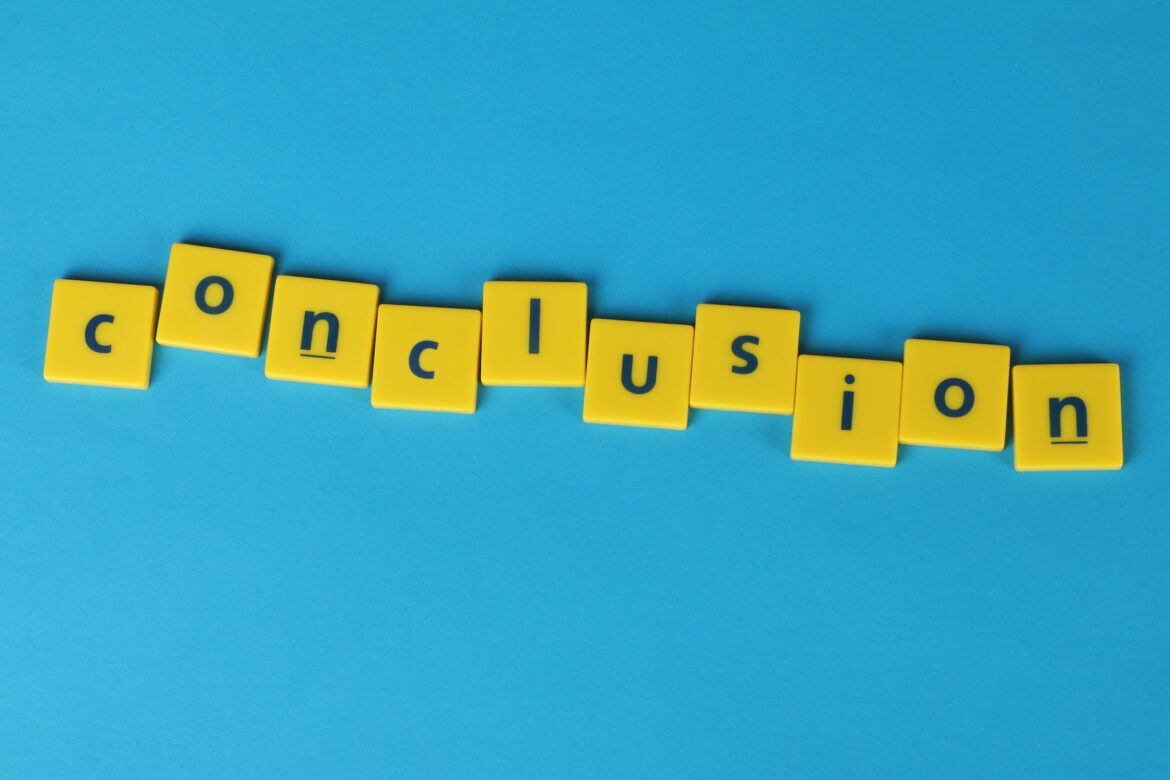
The conclusion of a research paper is a crucial section that plays a significant role in the overall impact and effectiveness of your research paper. However, this is also the section that typically receives less attention compared to the introduction and the body of the paper. The conclusion serves to provide a concise summary of the key findings, their significance, their implications, and a sense of closure to the study. Discussing how can the findings be applied in real-world scenarios or inform policy, practice, or decision-making is especially valuable to practitioners and policymakers. The research paper conclusion also provides researchers with clear insights and valuable information for their own work, which they can then build on and contribute to the advancement of knowledge in the field.
The research paper conclusion should explain the significance of your findings within the broader context of your field. It restates how your results contribute to the existing body of knowledge and whether they confirm or challenge existing theories or hypotheses. Also, by identifying unanswered questions or areas requiring further investigation, your awareness of the broader research landscape can be demonstrated.
Remember to tailor the research paper conclusion to the specific needs and interests of your intended audience, which may include researchers, practitioners, policymakers, or a combination of these.
Table of Contents
What is a conclusion in a research paper, summarizing conclusion, editorial conclusion, externalizing conclusion, importance of a good research paper conclusion, how to write a conclusion for your research paper, research paper conclusion examples.
- How to write a research paper conclusion with Paperpal?
Frequently Asked Questions
A conclusion in a research paper is the final section where you summarize and wrap up your research, presenting the key findings and insights derived from your study. The research paper conclusion is not the place to introduce new information or data that was not discussed in the main body of the paper. When working on how to conclude a research paper, remember to stick to summarizing and interpreting existing content. The research paper conclusion serves the following purposes: 1
- Warn readers of the possible consequences of not attending to the problem.
- Recommend specific course(s) of action.
- Restate key ideas to drive home the ultimate point of your research paper.
- Provide a “take-home” message that you want the readers to remember about your study.
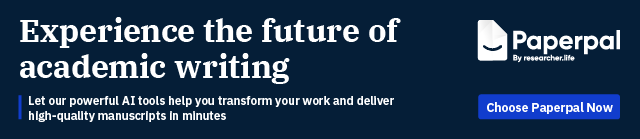
Types of conclusions for research papers
In research papers, the conclusion provides closure to the reader. The type of research paper conclusion you choose depends on the nature of your study, your goals, and your target audience. I provide you with three common types of conclusions:
A summarizing conclusion is the most common type of conclusion in research papers. It involves summarizing the main points, reiterating the research question, and restating the significance of the findings. This common type of research paper conclusion is used across different disciplines.
An editorial conclusion is less common but can be used in research papers that are focused on proposing or advocating for a particular viewpoint or policy. It involves presenting a strong editorial or opinion based on the research findings and offering recommendations or calls to action.
An externalizing conclusion is a type of conclusion that extends the research beyond the scope of the paper by suggesting potential future research directions or discussing the broader implications of the findings. This type of conclusion is often used in more theoretical or exploratory research papers.
Align your conclusion’s tone with the rest of your research paper. Start Writing with Paperpal Now!
The conclusion in a research paper serves several important purposes:
- Offers Implications and Recommendations : Your research paper conclusion is an excellent place to discuss the broader implications of your research and suggest potential areas for further study. It’s also an opportunity to offer practical recommendations based on your findings.
- Provides Closure : A good research paper conclusion provides a sense of closure to your paper. It should leave the reader with a feeling that they have reached the end of a well-structured and thought-provoking research project.
- Leaves a Lasting Impression : Writing a well-crafted research paper conclusion leaves a lasting impression on your readers. It’s your final opportunity to leave them with a new idea, a call to action, or a memorable quote.
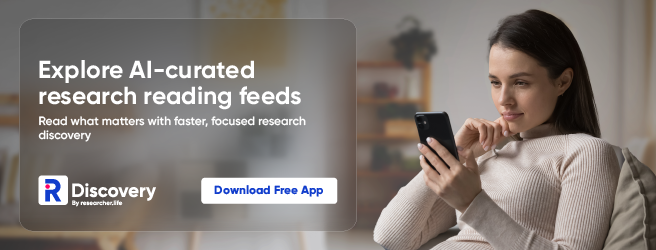
Writing a strong conclusion for your research paper is essential to leave a lasting impression on your readers. Here’s a step-by-step process to help you create and know what to put in the conclusion of a research paper: 2
- Research Statement : Begin your research paper conclusion by restating your research statement. This reminds the reader of the main point you’ve been trying to prove throughout your paper. Keep it concise and clear.
- Key Points : Summarize the main arguments and key points you’ve made in your paper. Avoid introducing new information in the research paper conclusion. Instead, provide a concise overview of what you’ve discussed in the body of your paper.
- Address the Research Questions : If your research paper is based on specific research questions or hypotheses, briefly address whether you’ve answered them or achieved your research goals. Discuss the significance of your findings in this context.
- Significance : Highlight the importance of your research and its relevance in the broader context. Explain why your findings matter and how they contribute to the existing knowledge in your field.
- Implications : Explore the practical or theoretical implications of your research. How might your findings impact future research, policy, or real-world applications? Consider the “so what?” question.
- Future Research : Offer suggestions for future research in your area. What questions or aspects remain unanswered or warrant further investigation? This shows that your work opens the door for future exploration.
- Closing Thought : Conclude your research paper conclusion with a thought-provoking or memorable statement. This can leave a lasting impression on your readers and wrap up your paper effectively. Avoid introducing new information or arguments here.
- Proofread and Revise : Carefully proofread your conclusion for grammar, spelling, and clarity. Ensure that your ideas flow smoothly and that your conclusion is coherent and well-structured.
Write your research paper conclusion 2x faster with Paperpal. Try it now!
Remember that a well-crafted research paper conclusion is a reflection of the strength of your research and your ability to communicate its significance effectively. It should leave a lasting impression on your readers and tie together all the threads of your paper. Now you know how to start the conclusion of a research paper and what elements to include to make it impactful, let’s look at a research paper conclusion sample.
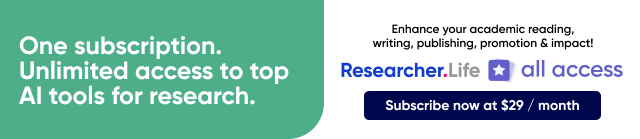
How to write a research paper conclusion with Paperpal?
A research paper conclusion is not just a summary of your study, but a synthesis of the key findings that ties the research together and places it in a broader context. A research paper conclusion should be concise, typically around one paragraph in length. However, some complex topics may require a longer conclusion to ensure the reader is left with a clear understanding of the study’s significance. Paperpal, an AI writing assistant trusted by over 800,000 academics globally, can help you write a well-structured conclusion for your research paper.
- Sign Up or Log In: Create a new Paperpal account or login with your details.
- Navigate to Features : Once logged in, head over to the features’ side navigation pane. Click on Templates and you’ll find a suite of generative AI features to help you write better, faster.
- Generate an outline: Under Templates, select ‘Outlines’. Choose ‘Research article’ as your document type.
- Select your section: Since you’re focusing on the conclusion, select this section when prompted.
- Choose your field of study: Identifying your field of study allows Paperpal to provide more targeted suggestions, ensuring the relevance of your conclusion to your specific area of research.
- Provide a brief description of your study: Enter details about your research topic and findings. This information helps Paperpal generate a tailored outline that aligns with your paper’s content.
- Generate the conclusion outline: After entering all necessary details, click on ‘generate’. Paperpal will then create a structured outline for your conclusion, to help you start writing and build upon the outline.
- Write your conclusion: Use the generated outline to build your conclusion. The outline serves as a guide, ensuring you cover all critical aspects of a strong conclusion, from summarizing key findings to highlighting the research’s implications.
- Refine and enhance: Paperpal’s ‘Make Academic’ feature can be particularly useful in the final stages. Select any paragraph of your conclusion and use this feature to elevate the academic tone, ensuring your writing is aligned to the academic journal standards.
By following these steps, Paperpal not only simplifies the process of writing a research paper conclusion but also ensures it is impactful, concise, and aligned with academic standards. Sign up with Paperpal today and write your research paper conclusion 2x faster .
The research paper conclusion is a crucial part of your paper as it provides the final opportunity to leave a strong impression on your readers. In the research paper conclusion, summarize the main points of your research paper by restating your research statement, highlighting the most important findings, addressing the research questions or objectives, explaining the broader context of the study, discussing the significance of your findings, providing recommendations if applicable, and emphasizing the takeaway message. The main purpose of the conclusion is to remind the reader of the main point or argument of your paper and to provide a clear and concise summary of the key findings and their implications. All these elements should feature on your list of what to put in the conclusion of a research paper to create a strong final statement for your work.
A strong conclusion is a critical component of a research paper, as it provides an opportunity to wrap up your arguments, reiterate your main points, and leave a lasting impression on your readers. Here are the key elements of a strong research paper conclusion: 1. Conciseness : A research paper conclusion should be concise and to the point. It should not introduce new information or ideas that were not discussed in the body of the paper. 2. Summarization : The research paper conclusion should be comprehensive enough to give the reader a clear understanding of the research’s main contributions. 3 . Relevance : Ensure that the information included in the research paper conclusion is directly relevant to the research paper’s main topic and objectives; avoid unnecessary details. 4 . Connection to the Introduction : A well-structured research paper conclusion often revisits the key points made in the introduction and shows how the research has addressed the initial questions or objectives. 5. Emphasis : Highlight the significance and implications of your research. Why is your study important? What are the broader implications or applications of your findings? 6 . Call to Action : Include a call to action or a recommendation for future research or action based on your findings.
The length of a research paper conclusion can vary depending on several factors, including the overall length of the paper, the complexity of the research, and the specific journal requirements. While there is no strict rule for the length of a conclusion, but it’s generally advisable to keep it relatively short. A typical research paper conclusion might be around 5-10% of the paper’s total length. For example, if your paper is 10 pages long, the conclusion might be roughly half a page to one page in length.
In general, you do not need to include citations in the research paper conclusion. Citations are typically reserved for the body of the paper to support your arguments and provide evidence for your claims. However, there may be some exceptions to this rule: 1. If you are drawing a direct quote or paraphrasing a specific source in your research paper conclusion, you should include a citation to give proper credit to the original author. 2. If your conclusion refers to or discusses specific research, data, or sources that are crucial to the overall argument, citations can be included to reinforce your conclusion’s validity.
The conclusion of a research paper serves several important purposes: 1. Summarize the Key Points 2. Reinforce the Main Argument 3. Provide Closure 4. Offer Insights or Implications 5. Engage the Reader. 6. Reflect on Limitations
Remember that the primary purpose of the research paper conclusion is to leave a lasting impression on the reader, reinforcing the key points and providing closure to your research. It’s often the last part of the paper that the reader will see, so it should be strong and well-crafted.
- Makar, G., Foltz, C., Lendner, M., & Vaccaro, A. R. (2018). How to write effective discussion and conclusion sections. Clinical spine surgery, 31(8), 345-346.
- Bunton, D. (2005). The structure of PhD conclusion chapters. Journal of English for academic purposes , 4 (3), 207-224.
Paperpal is a comprehensive AI writing toolkit that helps students and researchers achieve 2x the writing in half the time. It leverages 21+ years of STM experience and insights from millions of research articles to provide in-depth academic writing, language editing, and submission readiness support to help you write better, faster.
Get accurate academic translations, rewriting support, grammar checks, vocabulary suggestions, and generative AI assistance that delivers human precision at machine speed. Try for free or upgrade to Paperpal Prime starting at US$19 a month to access premium features, including consistency, plagiarism, and 30+ submission readiness checks to help you succeed.
Experience the future of academic writing – Sign up to Paperpal and start writing for free!
Related Reads:
- 5 Reasons for Rejection After Peer Review
- Ethical Research Practices For Research with Human Subjects
7 Ways to Improve Your Academic Writing Process
- Paraphrasing in Academic Writing: Answering Top Author Queries
Preflight For Editorial Desk: The Perfect Hybrid (AI + Human) Assistance Against Compromised Manuscripts
You may also like, measuring academic success: definition & strategies for excellence, phd qualifying exam: tips for success , ai in education: it’s time to change the..., is it ethical to use ai-generated abstracts without..., what are journal guidelines on using generative ai..., quillbot review: features, pricing, and free alternatives, what is an academic paper types and elements , should you use ai tools like chatgpt for..., 9 steps to publish a research paper, what are the different types of research papers.
- USC Libraries
- Research Guides
Organizing Your Social Sciences Research Paper
- 9. The Conclusion
- Purpose of Guide
- Design Flaws to Avoid
- Independent and Dependent Variables
- Glossary of Research Terms
- Reading Research Effectively
- Narrowing a Topic Idea
- Broadening a Topic Idea
- Extending the Timeliness of a Topic Idea
- Academic Writing Style
- Applying Critical Thinking
- Choosing a Title
- Making an Outline
- Paragraph Development
- Research Process Video Series
- Executive Summary
- The C.A.R.S. Model
- Background Information
- The Research Problem/Question
- Theoretical Framework
- Citation Tracking
- Content Alert Services
- Evaluating Sources
- Primary Sources
- Secondary Sources
- Tiertiary Sources
- Scholarly vs. Popular Publications
- Qualitative Methods
- Quantitative Methods
- Insiderness
- Using Non-Textual Elements
- Limitations of the Study
- Common Grammar Mistakes
- Writing Concisely
- Avoiding Plagiarism
- Footnotes or Endnotes?
- Further Readings
- Generative AI and Writing
- USC Libraries Tutorials and Other Guides
- Bibliography
The conclusion is intended to help the reader understand why your research should matter to them after they have finished reading the paper. A conclusion is not merely a summary of the main topics covered or a re-statement of your research problem, but a synthesis of key points derived from the findings of your study and, if applicable, where you recommend new areas for future research. For most college-level research papers, two or three well-developed paragraphs is sufficient for a conclusion, although in some cases, more paragraphs may be required in describing the key findings and their significance.
Conclusions. The Writing Center. University of North Carolina; Conclusions. The Writing Lab and The OWL. Purdue University.
Importance of a Good Conclusion
A well-written conclusion provides you with important opportunities to demonstrate to the reader your understanding of the research problem. These include:
- Presenting the last word on the issues you raised in your paper . Just as the introduction gives a first impression to your reader, the conclusion offers a chance to leave a lasting impression. Do this, for example, by highlighting key findings in your analysis that advance new understanding about the research problem, that are unusual or unexpected, or that have important implications applied to practice.
- Summarizing your thoughts and conveying the larger significance of your study . The conclusion is an opportunity to succinctly re-emphasize your answer to the "So What?" question by placing the study within the context of how your research advances past research about the topic.
- Identifying how a gap in the literature has been addressed . The conclusion can be where you describe how a previously identified gap in the literature [first identified in your literature review section] has been addressed by your research and why this contribution is significant.
- Demonstrating the importance of your ideas . Don't be shy. The conclusion offers an opportunity to elaborate on the impact and significance of your findings. This is particularly important if your study approached examining the research problem from an unusual or innovative perspective.
- Introducing possible new or expanded ways of thinking about the research problem . This does not refer to introducing new information [which should be avoided], but to offer new insight and creative approaches for framing or contextualizing the research problem based on the results of your study.
Bunton, David. “The Structure of PhD Conclusion Chapters.” Journal of English for Academic Purposes 4 (July 2005): 207–224; Conclusions. The Writing Center. University of North Carolina; Kretchmer, Paul. Twelve Steps to Writing an Effective Conclusion. San Francisco Edit, 2003-2008; Conclusions. The Writing Lab and The OWL. Purdue University; Assan, Joseph. "Writing the Conclusion Chapter: The Good, the Bad and the Missing." Liverpool: Development Studies Association (2009): 1-8.
Structure and Writing Style
I. General Rules
The general function of your paper's conclusion is to restate the main argument . It reminds the reader of the strengths of your main argument(s) and reiterates the most important evidence supporting those argument(s). Do this by clearly summarizing the context, background, and necessity of pursuing the research problem you investigated in relation to an issue, controversy, or a gap found in the literature. However, make sure that your conclusion is not simply a repetitive summary of the findings. This reduces the impact of the argument(s) you have developed in your paper.
When writing the conclusion to your paper, follow these general rules:
- Present your conclusions in clear, concise language. Re-state the purpose of your study, then describe how your findings differ or support those of other studies and why [i.e., what were the unique, new, or crucial contributions your study made to the overall research about your topic?].
- Do not simply reiterate your findings or the discussion of your results. Provide a synthesis of arguments presented in the paper to show how these converge to address the research problem and the overall objectives of your study.
- Indicate opportunities for future research if you haven't already done so in the discussion section of your paper. Highlighting the need for further research provides the reader with evidence that you have an in-depth awareness of the research problem but that further investigations should take place beyond the scope of your investigation.
Consider the following points to help ensure your conclusion is presented well:
- If the argument or purpose of your paper is complex, you may need to summarize the argument for your reader.
- If, prior to your conclusion, you have not yet explained the significance of your findings or if you are proceeding inductively, use the end of your paper to describe your main points and explain their significance.
- Move from a detailed to a general level of consideration that returns the topic to the context provided by the introduction or within a new context that emerges from the data [this is opposite of the introduction, which begins with general discussion of the context and ends with a detailed description of the research problem].
The conclusion also provides a place for you to persuasively and succinctly restate the research problem, given that the reader has now been presented with all the information about the topic . Depending on the discipline you are writing in, the concluding paragraph may contain your reflections on the evidence presented. However, the nature of being introspective about the research you have conducted will depend on the topic and whether your professor wants you to express your observations in this way. If asked to think introspectively about the topics, do not delve into idle speculation. Being introspective means looking within yourself as an author to try and understand an issue more deeply, not to guess at possible outcomes or make up scenarios not supported by the evidence.
II. Developing a Compelling Conclusion
Although an effective conclusion needs to be clear and succinct, it does not need to be written passively or lack a compelling narrative. Strategies to help you move beyond merely summarizing the key points of your research paper may include any of the following:
- If your essay deals with a critical, contemporary problem, warn readers of the possible consequences of not attending to the problem proactively.
- Recommend a specific course or courses of action that, if adopted, could address a specific problem in practice or in the development of new knowledge leading to positive change.
- Cite a relevant quotation or expert opinion already noted in your paper in order to lend authority and support to the conclusion(s) you have reached [a good source would be from your literature review].
- Explain the consequences of your research in a way that elicits action or demonstrates urgency in seeking change.
- Restate a key statistic, fact, or visual image to emphasize the most important finding of your paper.
- If your discipline encourages personal reflection, illustrate your concluding point by drawing from your own life experiences.
- Return to an anecdote, an example, or a quotation that you presented in your introduction, but add further insight derived from the findings of your study; use your interpretation of results from your study to recast it in new or important ways.
- Provide a "take-home" message in the form of a succinct, declarative statement that you want the reader to remember about your study.
III. Problems to Avoid
Failure to be concise Your conclusion section should be concise and to the point. Conclusions that are too lengthy often have unnecessary information in them. The conclusion is not the place for details about your methodology or results. Although you should give a summary of what was learned from your research, this summary should be relatively brief, since the emphasis in the conclusion is on the implications, evaluations, insights, and other forms of analysis that you make. Strategies for writing concisely can be found here .
Failure to comment on larger, more significant issues In the introduction, your task was to move from the general [the field of study] to the specific [the research problem]. However, in the conclusion, your task is to move from a specific discussion [your research problem] back to a general discussion framed around the implications and significance of your findings [i.e., how your research contributes new understanding or fills an important gap in the literature]. In short, the conclusion is where you should place your research within a larger context [visualize your paper as an hourglass--start with a broad introduction and review of the literature, move to the specific analysis and discussion, conclude with a broad summary of the study's implications and significance].
Failure to reveal problems and negative results Negative aspects of the research process should never be ignored. These are problems, deficiencies, or challenges encountered during your study. They should be summarized as a way of qualifying your overall conclusions. If you encountered negative or unintended results [i.e., findings that are validated outside the research context in which they were generated], you must report them in the results section and discuss their implications in the discussion section of your paper. In the conclusion, use negative results as an opportunity to explain their possible significance and/or how they may form the basis for future research.
Failure to provide a clear summary of what was learned In order to be able to discuss how your research fits within your field of study [and possibly the world at large], you need to summarize briefly and succinctly how it contributes to new knowledge or a new understanding about the research problem. This element of your conclusion may be only a few sentences long.
Failure to match the objectives of your research Often research objectives in the social and behavioral sciences change while the research is being carried out. This is not a problem unless you forget to go back and refine the original objectives in your introduction. As these changes emerge they must be documented so that they accurately reflect what you were trying to accomplish in your research [not what you thought you might accomplish when you began].
Resist the urge to apologize If you've immersed yourself in studying the research problem, you presumably should know a good deal about it [perhaps even more than your professor!]. Nevertheless, by the time you have finished writing, you may be having some doubts about what you have produced. Repress those doubts! Don't undermine your authority as a researcher by saying something like, "This is just one approach to examining this problem; there may be other, much better approaches that...." The overall tone of your conclusion should convey confidence to the reader about the study's validity and realiability.
Assan, Joseph. "Writing the Conclusion Chapter: The Good, the Bad and the Missing." Liverpool: Development Studies Association (2009): 1-8; Concluding Paragraphs. College Writing Center at Meramec. St. Louis Community College; Conclusions. The Writing Center. University of North Carolina; Conclusions. The Writing Lab and The OWL. Purdue University; Freedman, Leora and Jerry Plotnick. Introductions and Conclusions. The Lab Report. University College Writing Centre. University of Toronto; Leibensperger, Summer. Draft Your Conclusion. Academic Center, the University of Houston-Victoria, 2003; Make Your Last Words Count. The Writer’s Handbook. Writing Center. University of Wisconsin Madison; Miquel, Fuster-Marquez and Carmen Gregori-Signes. “Chapter Six: ‘Last but Not Least:’ Writing the Conclusion of Your Paper.” In Writing an Applied Linguistics Thesis or Dissertation: A Guide to Presenting Empirical Research . John Bitchener, editor. (Basingstoke,UK: Palgrave Macmillan, 2010), pp. 93-105; Tips for Writing a Good Conclusion. Writing@CSU. Colorado State University; Kretchmer, Paul. Twelve Steps to Writing an Effective Conclusion. San Francisco Edit, 2003-2008; Writing Conclusions. Writing Tutorial Services, Center for Innovative Teaching and Learning. Indiana University; Writing: Considering Structure and Organization. Institute for Writing Rhetoric. Dartmouth College.
Writing Tip
Don't Belabor the Obvious!
Avoid phrases like "in conclusion...," "in summary...," or "in closing...." These phrases can be useful, even welcome, in oral presentations. But readers can see by the tell-tale section heading and number of pages remaining that they are reaching the end of your paper. You'll irritate your readers if you belabor the obvious.
Assan, Joseph. "Writing the Conclusion Chapter: The Good, the Bad and the Missing." Liverpool: Development Studies Association (2009): 1-8.
Another Writing Tip
New Insight, Not New Information!
Don't surprise the reader with new information in your conclusion that was never referenced anywhere else in the paper. This why the conclusion rarely has citations to sources. If you have new information to present, add it to the discussion or other appropriate section of the paper. Note that, although no new information is introduced, the conclusion, along with the discussion section, is where you offer your most "original" contributions in the paper; the conclusion is where you describe the value of your research, demonstrate that you understand the material that you’ve presented, and position your findings within the larger context of scholarship on the topic, including describing how your research contributes new insights to that scholarship.
Assan, Joseph. "Writing the Conclusion Chapter: The Good, the Bad and the Missing." Liverpool: Development Studies Association (2009): 1-8; Conclusions. The Writing Center. University of North Carolina.
- << Previous: Limitations of the Study
- Next: Appendices >>
- Last Updated: May 1, 2024 9:19 AM
- URL: https://libguides.usc.edu/writingguide
- Privacy Policy

Home » Research Paper Conclusion – Writing Guide and Examples
Research Paper Conclusion – Writing Guide and Examples
Table of Contents

Research Paper Conclusion
Definition:
A research paper conclusion is the final section of a research paper that summarizes the key findings, significance, and implications of the research. It is the writer’s opportunity to synthesize the information presented in the paper, draw conclusions, and make recommendations for future research or actions.
The conclusion should provide a clear and concise summary of the research paper, reiterating the research question or problem, the main results, and the significance of the findings. It should also discuss the limitations of the study and suggest areas for further research.
Parts of Research Paper Conclusion
The parts of a research paper conclusion typically include:
Restatement of the Thesis
The conclusion should begin by restating the thesis statement from the introduction in a different way. This helps to remind the reader of the main argument or purpose of the research.
Summary of Key Findings
The conclusion should summarize the main findings of the research, highlighting the most important results and conclusions. This section should be brief and to the point.
Implications and Significance
In this section, the researcher should explain the implications and significance of the research findings. This may include discussing the potential impact on the field or industry, highlighting new insights or knowledge gained, or pointing out areas for future research.
Limitations and Recommendations
It is important to acknowledge any limitations or weaknesses of the research and to make recommendations for how these could be addressed in future studies. This shows that the researcher is aware of the potential limitations of their work and is committed to improving the quality of research in their field.
Concluding Statement
The conclusion should end with a strong concluding statement that leaves a lasting impression on the reader. This could be a call to action, a recommendation for further research, or a final thought on the topic.
How to Write Research Paper Conclusion
Here are some steps you can follow to write an effective research paper conclusion:
- Restate the research problem or question: Begin by restating the research problem or question that you aimed to answer in your research. This will remind the reader of the purpose of your study.
- Summarize the main points: Summarize the key findings and results of your research. This can be done by highlighting the most important aspects of your research and the evidence that supports them.
- Discuss the implications: Discuss the implications of your findings for the research area and any potential applications of your research. You should also mention any limitations of your research that may affect the interpretation of your findings.
- Provide a conclusion : Provide a concise conclusion that summarizes the main points of your paper and emphasizes the significance of your research. This should be a strong and clear statement that leaves a lasting impression on the reader.
- Offer suggestions for future research: Lastly, offer suggestions for future research that could build on your findings and contribute to further advancements in the field.
Remember that the conclusion should be brief and to the point, while still effectively summarizing the key findings and implications of your research.
Example of Research Paper Conclusion
Here’s an example of a research paper conclusion:
Conclusion :
In conclusion, our study aimed to investigate the relationship between social media use and mental health among college students. Our findings suggest that there is a significant association between social media use and increased levels of anxiety and depression among college students. This highlights the need for increased awareness and education about the potential negative effects of social media use on mental health, particularly among college students.
Despite the limitations of our study, such as the small sample size and self-reported data, our findings have important implications for future research and practice. Future studies should aim to replicate our findings in larger, more diverse samples, and investigate the potential mechanisms underlying the association between social media use and mental health. In addition, interventions should be developed to promote healthy social media use among college students, such as mindfulness-based approaches and social media detox programs.
Overall, our study contributes to the growing body of research on the impact of social media on mental health, and highlights the importance of addressing this issue in the context of higher education. By raising awareness and promoting healthy social media use among college students, we can help to reduce the negative impact of social media on mental health and improve the well-being of young adults.
Purpose of Research Paper Conclusion
The purpose of a research paper conclusion is to provide a summary and synthesis of the key findings, significance, and implications of the research presented in the paper. The conclusion serves as the final opportunity for the writer to convey their message and leave a lasting impression on the reader.
The conclusion should restate the research problem or question, summarize the main results of the research, and explain their significance. It should also acknowledge the limitations of the study and suggest areas for future research or action.
Overall, the purpose of the conclusion is to provide a sense of closure to the research paper and to emphasize the importance of the research and its potential impact. It should leave the reader with a clear understanding of the main findings and why they matter. The conclusion serves as the writer’s opportunity to showcase their contribution to the field and to inspire further research and action.
When to Write Research Paper Conclusion
The conclusion of a research paper should be written after the body of the paper has been completed. It should not be written until the writer has thoroughly analyzed and interpreted their findings and has written a complete and cohesive discussion of the research.
Before writing the conclusion, the writer should review their research paper and consider the key points that they want to convey to the reader. They should also review the research question, hypotheses, and methodology to ensure that they have addressed all of the necessary components of the research.
Once the writer has a clear understanding of the main findings and their significance, they can begin writing the conclusion. The conclusion should be written in a clear and concise manner, and should reiterate the main points of the research while also providing insights and recommendations for future research or action.
Characteristics of Research Paper Conclusion
The characteristics of a research paper conclusion include:
- Clear and concise: The conclusion should be written in a clear and concise manner, summarizing the key findings and their significance.
- Comprehensive: The conclusion should address all of the main points of the research paper, including the research question or problem, the methodology, the main results, and their implications.
- Future-oriented : The conclusion should provide insights and recommendations for future research or action, based on the findings of the research.
- Impressive : The conclusion should leave a lasting impression on the reader, emphasizing the importance of the research and its potential impact.
- Objective : The conclusion should be based on the evidence presented in the research paper, and should avoid personal biases or opinions.
- Unique : The conclusion should be unique to the research paper and should not simply repeat information from the introduction or body of the paper.
Advantages of Research Paper Conclusion
The advantages of a research paper conclusion include:
- Summarizing the key findings : The conclusion provides a summary of the main findings of the research, making it easier for the reader to understand the key points of the study.
- Emphasizing the significance of the research: The conclusion emphasizes the importance of the research and its potential impact, making it more likely that readers will take the research seriously and consider its implications.
- Providing recommendations for future research or action : The conclusion suggests practical recommendations for future research or action, based on the findings of the study.
- Providing closure to the research paper : The conclusion provides a sense of closure to the research paper, tying together the different sections of the paper and leaving a lasting impression on the reader.
- Demonstrating the writer’s contribution to the field : The conclusion provides the writer with an opportunity to showcase their contribution to the field and to inspire further research and action.
Limitations of Research Paper Conclusion
While the conclusion of a research paper has many advantages, it also has some limitations that should be considered, including:
- I nability to address all aspects of the research: Due to the limited space available in the conclusion, it may not be possible to address all aspects of the research in detail.
- Subjectivity : While the conclusion should be objective, it may be influenced by the writer’s personal biases or opinions.
- Lack of new information: The conclusion should not introduce new information that has not been discussed in the body of the research paper.
- Lack of generalizability: The conclusions drawn from the research may not be applicable to other contexts or populations, limiting the generalizability of the study.
- Misinterpretation by the reader: The reader may misinterpret the conclusions drawn from the research, leading to a misunderstanding of the findings.
About the author
Muhammad Hassan
Researcher, Academic Writer, Web developer
You may also like

How to Cite Research Paper – All Formats and...

Data Collection – Methods Types and Examples

Delimitations in Research – Types, Examples and...

Research Paper Format – Types, Examples and...

Research Process – Steps, Examples and Tips

Research Design – Types, Methods and Examples

- Walden University
- Faculty Portal
Writing a Paper: Conclusions
Writing a conclusion.
A conclusion is an important part of the paper; it provides closure for the reader while reminding the reader of the contents and importance of the paper. It accomplishes this by stepping back from the specifics in order to view the bigger picture of the document. In other words, it is reminding the reader of the main argument. For most course papers, it is usually one paragraph that simply and succinctly restates the main ideas and arguments, pulling everything together to help clarify the thesis of the paper. A conclusion does not introduce new ideas; instead, it should clarify the intent and importance of the paper. It can also suggest possible future research on the topic.
An Easy Checklist for Writing a Conclusion
It is important to remind the reader of the thesis of the paper so he is reminded of the argument and solutions you proposed.
Think of the main points as puzzle pieces, and the conclusion is where they all fit together to create a bigger picture. The reader should walk away with the bigger picture in mind.
Make sure that the paper places its findings in the context of real social change.
Make sure the reader has a distinct sense that the paper has come to an end. It is important to not leave the reader hanging. (You don’t want her to have flip-the-page syndrome, where the reader turns the page, expecting the paper to continue. The paper should naturally come to an end.)
No new ideas should be introduced in the conclusion. It is simply a review of the material that is already present in the paper. The only new idea would be the suggesting of a direction for future research.
Conclusion Example
As addressed in my analysis of recent research, the advantages of a later starting time for high school students significantly outweigh the disadvantages. A later starting time would allow teens more time to sleep--something that is important for their physical and mental health--and ultimately improve their academic performance and behavior. The added transportation costs that result from this change can be absorbed through energy savings. The beneficial effects on the students’ academic performance and behavior validate this decision, but its effect on student motivation is still unknown. I would encourage an in-depth look at the reactions of students to such a change. This sort of study would help determine the actual effects of a later start time on the time management and sleep habits of students.
Related Webinar

Didn't find what you need? Email us at [email protected] .
- Previous Page: Thesis Statements
- Next Page: Writer's Block
- Office of Student Disability Services
Walden Resources
Departments.
- Academic Residencies
- Academic Skills
- Career Planning and Development
- Customer Care Team
- Field Experience
- Military Services
- Student Success Advising
- Writing Skills
Centers and Offices
- Center for Social Change
- Office of Academic Support and Instructional Services
- Office of Degree Acceleration
- Office of Research and Doctoral Services
- Office of Student Affairs
Student Resources
- Doctoral Writing Assessment
- Form & Style Review
- Quick Answers
- ScholarWorks
- SKIL Courses and Workshops
- Walden Bookstore
- Walden Catalog & Student Handbook
- Student Safety/Title IX
- Legal & Consumer Information
- Website Terms and Conditions
- Cookie Policy
- Accessibility
- Accreditation
- State Authorization
- Net Price Calculator
- Contact Walden
Walden University is a member of Adtalem Global Education, Inc. www.adtalem.com Walden University is certified to operate by SCHEV © 2024 Walden University LLC. All rights reserved.

Conclusions
What this handout is about.
This handout will explain the functions of conclusions, offer strategies for writing effective ones, help you evaluate conclusions you’ve drafted, and suggest approaches to avoid.
About conclusions
Introductions and conclusions can be difficult to write, but they’re worth investing time in. They can have a significant influence on a reader’s experience of your paper.
Just as your introduction acts as a bridge that transports your readers from their own lives into the “place” of your analysis, your conclusion can provide a bridge to help your readers make the transition back to their daily lives. Such a conclusion will help them see why all your analysis and information should matter to them after they put the paper down.
Your conclusion is your chance to have the last word on the subject. The conclusion allows you to have the final say on the issues you have raised in your paper, to synthesize your thoughts, to demonstrate the importance of your ideas, and to propel your reader to a new view of the subject. It is also your opportunity to make a good final impression and to end on a positive note.
Your conclusion can go beyond the confines of the assignment. The conclusion pushes beyond the boundaries of the prompt and allows you to consider broader issues, make new connections, and elaborate on the significance of your findings.
Your conclusion should make your readers glad they read your paper. Your conclusion gives your reader something to take away that will help them see things differently or appreciate your topic in personally relevant ways. It can suggest broader implications that will not only interest your reader, but also enrich your reader’s life in some way. It is your gift to the reader.
Strategies for writing an effective conclusion
One or more of the following strategies may help you write an effective conclusion:
- Play the “So What” Game. If you’re stuck and feel like your conclusion isn’t saying anything new or interesting, ask a friend to read it with you. Whenever you make a statement from your conclusion, ask the friend to say, “So what?” or “Why should anybody care?” Then ponder that question and answer it. Here’s how it might go: You: Basically, I’m just saying that education was important to Douglass. Friend: So what? You: Well, it was important because it was a key to him feeling like a free and equal citizen. Friend: Why should anybody care? You: That’s important because plantation owners tried to keep slaves from being educated so that they could maintain control. When Douglass obtained an education, he undermined that control personally. You can also use this strategy on your own, asking yourself “So What?” as you develop your ideas or your draft.
- Return to the theme or themes in the introduction. This strategy brings the reader full circle. For example, if you begin by describing a scenario, you can end with the same scenario as proof that your essay is helpful in creating a new understanding. You may also refer to the introductory paragraph by using key words or parallel concepts and images that you also used in the introduction.
- Synthesize, don’t summarize. Include a brief summary of the paper’s main points, but don’t simply repeat things that were in your paper. Instead, show your reader how the points you made and the support and examples you used fit together. Pull it all together.
- Include a provocative insight or quotation from the research or reading you did for your paper.
- Propose a course of action, a solution to an issue, or questions for further study. This can redirect your reader’s thought process and help them to apply your info and ideas to their own life or to see the broader implications.
- Point to broader implications. For example, if your paper examines the Greensboro sit-ins or another event in the Civil Rights Movement, you could point out its impact on the Civil Rights Movement as a whole. A paper about the style of writer Virginia Woolf could point to her influence on other writers or on later feminists.
Strategies to avoid
- Beginning with an unnecessary, overused phrase such as “in conclusion,” “in summary,” or “in closing.” Although these phrases can work in speeches, they come across as wooden and trite in writing.
- Stating the thesis for the very first time in the conclusion.
- Introducing a new idea or subtopic in your conclusion.
- Ending with a rephrased thesis statement without any substantive changes.
- Making sentimental, emotional appeals that are out of character with the rest of an analytical paper.
- Including evidence (quotations, statistics, etc.) that should be in the body of the paper.
Four kinds of ineffective conclusions
- The “That’s My Story and I’m Sticking to It” Conclusion. This conclusion just restates the thesis and is usually painfully short. It does not push the ideas forward. People write this kind of conclusion when they can’t think of anything else to say. Example: In conclusion, Frederick Douglass was, as we have seen, a pioneer in American education, proving that education was a major force for social change with regard to slavery.
- The “Sherlock Holmes” Conclusion. Sometimes writers will state the thesis for the very first time in the conclusion. You might be tempted to use this strategy if you don’t want to give everything away too early in your paper. You may think it would be more dramatic to keep the reader in the dark until the end and then “wow” them with your main idea, as in a Sherlock Holmes mystery. The reader, however, does not expect a mystery, but an analytical discussion of your topic in an academic style, with the main argument (thesis) stated up front. Example: (After a paper that lists numerous incidents from the book but never says what these incidents reveal about Douglass and his views on education): So, as the evidence above demonstrates, Douglass saw education as a way to undermine the slaveholders’ power and also an important step toward freedom.
- The “America the Beautiful”/”I Am Woman”/”We Shall Overcome” Conclusion. This kind of conclusion usually draws on emotion to make its appeal, but while this emotion and even sentimentality may be very heartfelt, it is usually out of character with the rest of an analytical paper. A more sophisticated commentary, rather than emotional praise, would be a more fitting tribute to the topic. Example: Because of the efforts of fine Americans like Frederick Douglass, countless others have seen the shining beacon of light that is education. His example was a torch that lit the way for others. Frederick Douglass was truly an American hero.
- The “Grab Bag” Conclusion. This kind of conclusion includes extra information that the writer found or thought of but couldn’t integrate into the main paper. You may find it hard to leave out details that you discovered after hours of research and thought, but adding random facts and bits of evidence at the end of an otherwise-well-organized essay can just create confusion. Example: In addition to being an educational pioneer, Frederick Douglass provides an interesting case study for masculinity in the American South. He also offers historians an interesting glimpse into slave resistance when he confronts Covey, the overseer. His relationships with female relatives reveal the importance of family in the slave community.
Works consulted
We consulted these works while writing this handout. This is not a comprehensive list of resources on the handout’s topic, and we encourage you to do your own research to find additional publications. Please do not use this list as a model for the format of your own reference list, as it may not match the citation style you are using. For guidance on formatting citations, please see the UNC Libraries citation tutorial . We revise these tips periodically and welcome feedback.
Douglass, Frederick. 1995. Narrative of the Life of Frederick Douglass, an American Slave, Written by Himself. New York: Dover.
Hamilton College. n.d. “Conclusions.” Writing Center. Accessed June 14, 2019. https://www.hamilton.edu//academics/centers/writing/writing-resources/conclusions .
Holewa, Randa. 2004. “Strategies for Writing a Conclusion.” LEO: Literacy Education Online. Last updated February 19, 2004. https://leo.stcloudstate.edu/acadwrite/conclude.html.
You may reproduce it for non-commercial use if you use the entire handout and attribute the source: The Writing Center, University of North Carolina at Chapel Hill
Make a Gift
In a short paper—even a research paper—you don’t need to provide an exhaustive summary as part of your conclusion. But you do need to make some kind of transition between your final body paragraph and your concluding paragraph. This may come in the form of a few sentences of summary. Or it may come in the form of a sentence that brings your readers back to your thesis or main idea and reminds your readers where you began and how far you have traveled.
So, for example, in a paper about the relationship between ADHD and rejection sensitivity, Vanessa Roser begins by introducing readers to the fact that researchers have studied the relationship between the two conditions and then provides her explanation of that relationship. Here’s her thesis: “While socialization may indeed be an important factor in RS, I argue that individuals with ADHD may also possess a neurological predisposition to RS that is exacerbated by the differing executive and emotional regulation characteristic of ADHD.”
In her final paragraph, Roser reminds us of where she started by echoing her thesis: “This literature demonstrates that, as with many other conditions, ADHD and RS share a delicately intertwined pattern of neurological similarities that is rooted in the innate biology of an individual’s mind, a connection that cannot be explained in full by the behavioral mediation hypothesis.”
Highlight the “so what”
At the beginning of your paper, you explain to your readers what’s at stake—why they should care about the argument you’re making. In your conclusion, you can bring readers back to those stakes by reminding them why your argument is important in the first place. You can also draft a few sentences that put those stakes into a new or broader context.
In the conclusion to her paper about ADHD and RS, Roser echoes the stakes she established in her introduction—that research into connections between ADHD and RS has led to contradictory results, raising questions about the “behavioral mediation hypothesis.”
She writes, “as with many other conditions, ADHD and RS share a delicately intertwined pattern of neurological similarities that is rooted in the innate biology of an individual’s mind, a connection that cannot be explained in full by the behavioral mediation hypothesis.”
Leave your readers with the “now what”
After the “what” and the “so what,” you should leave your reader with some final thoughts. If you have written a strong introduction, your readers will know why you have been arguing what you have been arguing—and why they should care. And if you’ve made a good case for your thesis, then your readers should be in a position to see things in a new way, understand new questions, or be ready for something that they weren’t ready for before they read your paper.
In her conclusion, Roser offers two “now what” statements. First, she explains that it is important to recognize that the flawed behavioral mediation hypothesis “seems to place a degree of fault on the individual. It implies that individuals with ADHD must have elicited such frequent or intense rejection by virtue of their inadequate social skills, erasing the possibility that they may simply possess a natural sensitivity to emotion.” She then highlights the broader implications for treatment of people with ADHD, noting that recognizing the actual connection between rejection sensitivity and ADHD “has profound implications for understanding how individuals with ADHD might best be treated in educational settings, by counselors, family, peers, or even society as a whole.”
To find your own “now what” for your essay’s conclusion, try asking yourself these questions:
- What can my readers now understand, see in a new light, or grapple with that they would not have understood in the same way before reading my paper? Are we a step closer to understanding a larger phenomenon or to understanding why what was at stake is so important?
- What questions can I now raise that would not have made sense at the beginning of my paper? Questions for further research? Other ways that this topic could be approached?
- Are there other applications for my research? Could my questions be asked about different data in a different context? Could I use my methods to answer a different question?
- What action should be taken in light of this argument? What action do I predict will be taken or could lead to a solution?
- What larger context might my argument be a part of?
What to avoid in your conclusion
- a complete restatement of all that you have said in your paper.
- a substantial counterargument that you do not have space to refute; you should introduce counterarguments before your conclusion.
- an apology for what you have not said. If you need to explain the scope of your paper, you should do this sooner—but don’t apologize for what you have not discussed in your paper.
- fake transitions like “in conclusion” that are followed by sentences that aren’t actually conclusions. (“In conclusion, I have now demonstrated that my thesis is correct.”)
- picture_as_pdf Conclusions
- Link to facebook
- Link to linkedin
- Link to twitter
- Link to youtube
- Writing Tips
How to Write a Conclusion for a Research Paper
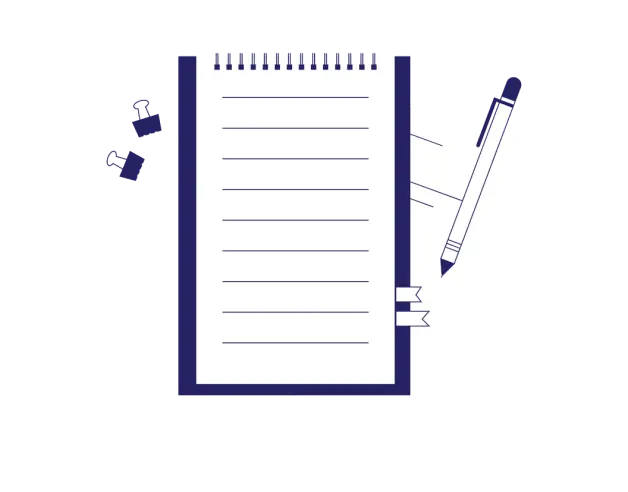
3-minute read
- 29th August 2023
If you’re writing a research paper, the conclusion is your opportunity to summarize your findings and leave a lasting impression on your readers. In this post, we’ll take you through how to write an effective conclusion for a research paper and how you can:
· Reword your thesis statement
· Highlight the significance of your research
· Discuss limitations
· Connect to the introduction
· End with a thought-provoking statement
Rewording Your Thesis Statement
Begin your conclusion by restating your thesis statement in a way that is slightly different from the wording used in the introduction. Avoid presenting new information or evidence in your conclusion. Just summarize the main points and arguments of your essay and keep this part as concise as possible. Remember that you’ve already covered the in-depth analyses and investigations in the main body paragraphs of your essay, so it’s not necessary to restate these details in the conclusion.
Find this useful?
Subscribe to our newsletter and get writing tips from our editors straight to your inbox.
Highlighting the Significance of Your Research
The conclusion is a good place to emphasize the implications of your research . Avoid ambiguous or vague language such as “I think” or “maybe,” which could weaken your position. Clearly explain why your research is significant and how it contributes to the broader field of study.
Here’s an example from a (fictional) study on the impact of social media on mental health:
Discussing Limitations
Although it’s important to emphasize the significance of your study, you can also use the conclusion to briefly address any limitations you discovered while conducting your research, such as time constraints or a shortage of resources. Doing this demonstrates a balanced and honest approach to your research.
Connecting to the Introduction
In your conclusion, you can circle back to your introduction , perhaps by referring to a quote or anecdote you discussed earlier. If you end your paper on a similar note to how you began it, you will create a sense of cohesion for the reader and remind them of the meaning and significance of your research.
Ending With a Thought-Provoking Statement
Consider ending your paper with a thought-provoking and memorable statement that relates to the impact of your research questions or hypothesis. This statement can be a call to action, a philosophical question, or a prediction for the future (positive or negative). Here’s an example that uses the same topic as above (social media and mental health):
Expert Proofreading Services
Ensure that your essay ends on a high note by having our experts proofread your research paper. Our team has experience with a wide variety of academic fields and subjects and can help make your paper stand out from the crowd – get started today and see the difference it can make in your work.
Share this article:
Post A New Comment
Got content that needs a quick turnaround? Let us polish your work. Explore our editorial business services.
How to insert a text box in a google doc.
Google Docs is a powerful collaborative tool, and mastering its features can significantly enhance your...
2-minute read
How to Cite the CDC in APA
If you’re writing about health issues, you might need to reference the Centers for Disease...
5-minute read
Six Product Description Generator Tools for Your Product Copy
Introduction If you’re involved with ecommerce, you’re likely familiar with the often painstaking process of...
What Is a Content Editor?
Are you interested in learning more about the role of a content editor and the...
4-minute read
The Benefits of Using an Online Proofreading Service
Proofreading is important to ensure your writing is clear and concise for your readers. Whether...
6 Online AI Presentation Maker Tools
Creating presentations can be time-consuming and frustrating. Trying to construct a visually appealing and informative...
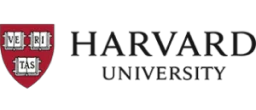
Make sure your writing is the best it can be with our expert English proofreading and editing.
When you choose to publish with PLOS, your research makes an impact. Make your work accessible to all, without restrictions, and accelerate scientific discovery with options like preprints and published peer review that make your work more Open.
- PLOS Biology
- PLOS Climate
- PLOS Complex Systems
- PLOS Computational Biology
- PLOS Digital Health
- PLOS Genetics
- PLOS Global Public Health
- PLOS Medicine
- PLOS Mental Health
- PLOS Neglected Tropical Diseases
- PLOS Pathogens
- PLOS Sustainability and Transformation
- PLOS Collections
- How to Write Discussions and Conclusions

The discussion section contains the results and outcomes of a study. An effective discussion informs readers what can be learned from your experiment and provides context for the results.
What makes an effective discussion?
When you’re ready to write your discussion, you’ve already introduced the purpose of your study and provided an in-depth description of the methodology. The discussion informs readers about the larger implications of your study based on the results. Highlighting these implications while not overstating the findings can be challenging, especially when you’re submitting to a journal that selects articles based on novelty or potential impact. Regardless of what journal you are submitting to, the discussion section always serves the same purpose: concluding what your study results actually mean.
A successful discussion section puts your findings in context. It should include:
- the results of your research,
- a discussion of related research, and
- a comparison between your results and initial hypothesis.
Tip: Not all journals share the same naming conventions.
You can apply the advice in this article to the conclusion, results or discussion sections of your manuscript.
Our Early Career Researcher community tells us that the conclusion is often considered the most difficult aspect of a manuscript to write. To help, this guide provides questions to ask yourself, a basic structure to model your discussion off of and examples from published manuscripts.

Questions to ask yourself:
- Was my hypothesis correct?
- If my hypothesis is partially correct or entirely different, what can be learned from the results?
- How do the conclusions reshape or add onto the existing knowledge in the field? What does previous research say about the topic?
- Why are the results important or relevant to your audience? Do they add further evidence to a scientific consensus or disprove prior studies?
- How can future research build on these observations? What are the key experiments that must be done?
- What is the “take-home” message you want your reader to leave with?
How to structure a discussion
Trying to fit a complete discussion into a single paragraph can add unnecessary stress to the writing process. If possible, you’ll want to give yourself two or three paragraphs to give the reader a comprehensive understanding of your study as a whole. Here’s one way to structure an effective discussion:
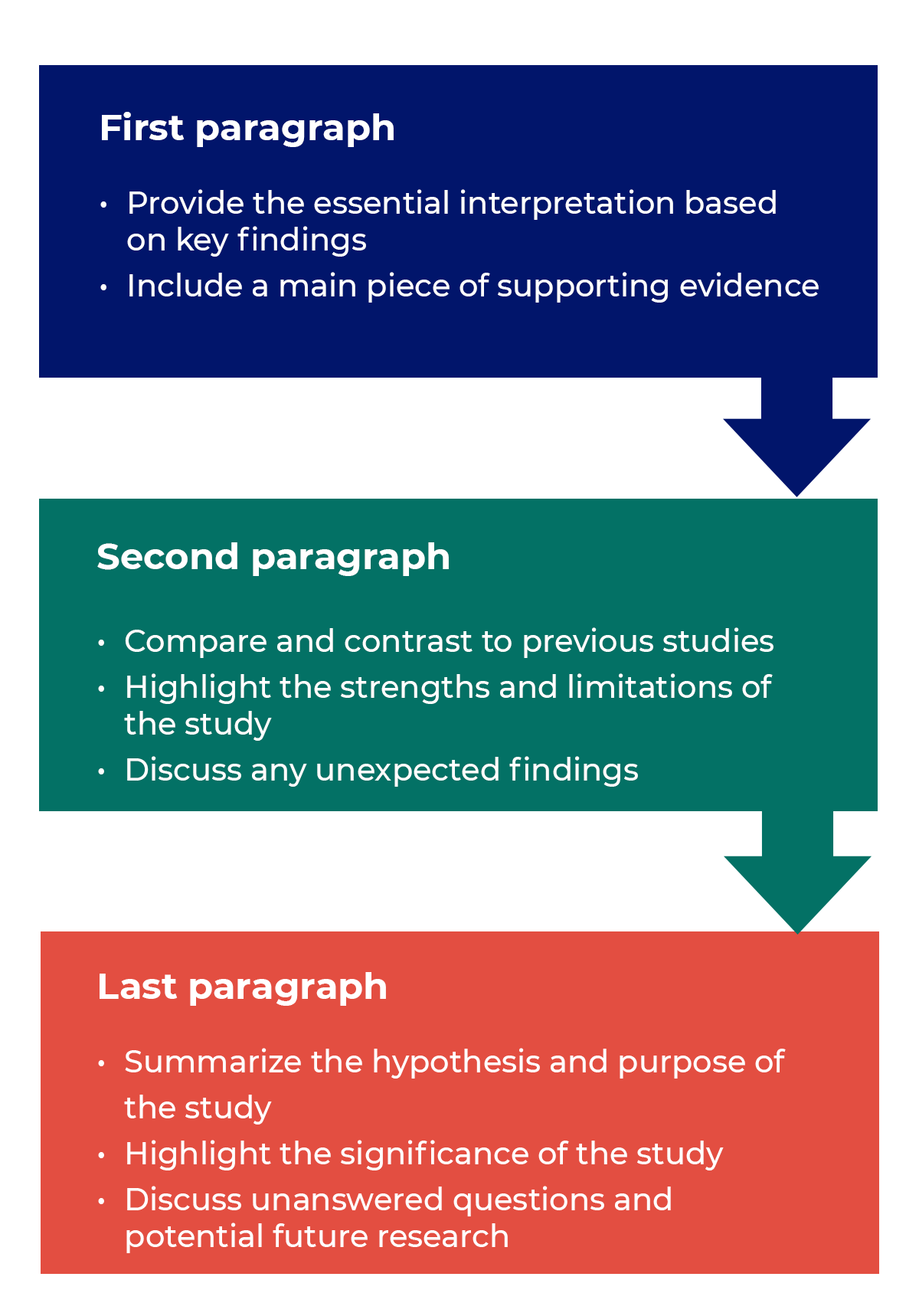
Writing Tips
While the above sections can help you brainstorm and structure your discussion, there are many common mistakes that writers revert to when having difficulties with their paper. Writing a discussion can be a delicate balance between summarizing your results, providing proper context for your research and avoiding introducing new information. Remember that your paper should be both confident and honest about the results!

- Read the journal’s guidelines on the discussion and conclusion sections. If possible, learn about the guidelines before writing the discussion to ensure you’re writing to meet their expectations.
- Begin with a clear statement of the principal findings. This will reinforce the main take-away for the reader and set up the rest of the discussion.
- Explain why the outcomes of your study are important to the reader. Discuss the implications of your findings realistically based on previous literature, highlighting both the strengths and limitations of the research.
- State whether the results prove or disprove your hypothesis. If your hypothesis was disproved, what might be the reasons?
- Introduce new or expanded ways to think about the research question. Indicate what next steps can be taken to further pursue any unresolved questions.
- If dealing with a contemporary or ongoing problem, such as climate change, discuss possible consequences if the problem is avoided.
- Be concise. Adding unnecessary detail can distract from the main findings.
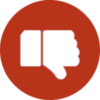
Don’t
- Rewrite your abstract. Statements with “we investigated” or “we studied” generally do not belong in the discussion.
- Include new arguments or evidence not previously discussed. Necessary information and evidence should be introduced in the main body of the paper.
- Apologize. Even if your research contains significant limitations, don’t undermine your authority by including statements that doubt your methodology or execution.
- Shy away from speaking on limitations or negative results. Including limitations and negative results will give readers a complete understanding of the presented research. Potential limitations include sources of potential bias, threats to internal or external validity, barriers to implementing an intervention and other issues inherent to the study design.
- Overstate the importance of your findings. Making grand statements about how a study will fully resolve large questions can lead readers to doubt the success of the research.
Snippets of Effective Discussions:
Consumer-based actions to reduce plastic pollution in rivers: A multi-criteria decision analysis approach
Identifying reliable indicators of fitness in polar bears
- How to Write a Great Title
- How to Write an Abstract
- How to Write Your Methods
- How to Report Statistics
- How to Edit Your Work
The contents of the Peer Review Center are also available as a live, interactive training session, complete with slides, talking points, and activities. …
The contents of the Writing Center are also available as a live, interactive training session, complete with slides, talking points, and activities. …
There’s a lot to consider when deciding where to submit your work. Learn how to choose a journal that will help your study reach its audience, while reflecting your values as a researcher…
How to write a strong conclusion for your research paper
Last updated
17 February 2024
Reviewed by
Writing a research paper is a chance to share your knowledge and hypothesis. It's an opportunity to demonstrate your many hours of research and prove your ability to write convincingly.
Ideally, by the end of your research paper, you'll have brought your readers on a journey to reach the conclusions you've pre-determined. However, if you don't stick the landing with a good conclusion, you'll risk losing your reader’s trust.
Writing a strong conclusion for your research paper involves a few important steps, including restating the thesis and summing up everything properly.
Find out what to include and what to avoid, so you can effectively demonstrate your understanding of the topic and prove your expertise.
- Why is a good conclusion important?
A good conclusion can cement your paper in the reader’s mind. Making a strong impression in your introduction can draw your readers in, but it's the conclusion that will inspire them.
- What to include in a research paper conclusion
There are a few specifics you should include in your research paper conclusion. Offer your readers some sense of urgency or consequence by pointing out why they should care about the topic you have covered. Discuss any common problems associated with your topic and provide suggestions as to how these problems can be solved or addressed.
The conclusion should include a restatement of your initial thesis. Thesis statements are strengthened after you’ve presented supporting evidence (as you will have done in the paper), so make a point to reintroduce it at the end.
Finally, recap the main points of your research paper, highlighting the key takeaways you want readers to remember. If you've made multiple points throughout the paper, refer to the ones with the strongest supporting evidence.
- Steps for writing a research paper conclusion
Many writers find the conclusion the most challenging part of any research project . By following these three steps, you'll be prepared to write a conclusion that is effective and concise.
- Step 1: Restate the problem
Always begin by restating the research problem in the conclusion of a research paper. This serves to remind the reader of your hypothesis and refresh them on the main point of the paper.
When restating the problem, take care to avoid using exactly the same words you employed earlier in the paper.
- Step 2: Sum up the paper
After you've restated the problem, sum up the paper by revealing your overall findings. The method for this differs slightly, depending on whether you're crafting an argumentative paper or an empirical paper.
Argumentative paper: Restate your thesis and arguments
Argumentative papers involve introducing a thesis statement early on. In crafting the conclusion for an argumentative paper, always restate the thesis, outlining the way you've developed it throughout the entire paper.
It might be appropriate to mention any counterarguments in the conclusion, so you can demonstrate how your thesis is correct or how the data best supports your main points.
Empirical paper: Summarize research findings
Empirical papers break down a series of research questions. In your conclusion, discuss the findings your research revealed, including any information that surprised you.
Be clear about the conclusions you reached, and explain whether or not you expected to arrive at these particular ones.
- Step 3: Discuss the implications of your research
Argumentative papers and empirical papers also differ in this part of a research paper conclusion. Here are some tips on crafting conclusions for argumentative and empirical papers.
Argumentative paper: Powerful closing statement
In an argumentative paper, you'll have spent a great deal of time expressing the opinions you formed after doing a significant amount of research. Make a strong closing statement in your argumentative paper's conclusion to share the significance of your work.
You can outline the next steps through a bold call to action, or restate how powerful your ideas turned out to be.
Empirical paper: Directions for future research
Empirical papers are broader in scope. They usually cover a variety of aspects and can include several points of view.
To write a good conclusion for an empirical paper, suggest the type of research that could be done in the future, including methods for further investigation or outlining ways other researchers might proceed.
If you feel your research had any limitations, even if they were outside your control, you could mention these in your conclusion.
After you finish outlining your conclusion, ask someone to read it and offer feedback. In any research project you're especially close to, it can be hard to identify problem areas. Having a close friend or someone whose opinion you value read the research paper and provide honest feedback can be invaluable. Take note of any suggested edits and consider incorporating them into your paper if they make sense.
- Things to avoid in a research paper conclusion
Keep these aspects to avoid in mind as you're writing your conclusion and refer to them after you've created an outline.
Dry summary
Writing a memorable, succinct conclusion is arguably more important than a strong introduction. Take care to avoid just rephrasing your main points, and don't fall into the trap of repeating dry facts or citations.
You can provide a new perspective for your readers to think about or contextualize your research. Either way, make the conclusion vibrant and interesting, rather than a rote recitation of your research paper’s highlights.
Clichéd or generic phrasing
Your research paper conclusion should feel fresh and inspiring. Avoid generic phrases like "to sum up" or "in conclusion." These phrases tend to be overused, especially in an academic context and might turn your readers off.
The conclusion also isn't the time to introduce colloquial phrases or informal language. Retain a professional, confident tone consistent throughout your paper’s conclusion so it feels exciting and bold.
New data or evidence
While you should present strong data throughout your paper, the conclusion isn't the place to introduce new evidence. This is because readers are engaged in actively learning as they read through the body of your paper.
By the time they reach the conclusion, they will have formed an opinion one way or the other (hopefully in your favor!). Introducing new evidence in the conclusion will only serve to surprise or frustrate your reader.
Ignoring contradictory evidence
If your research reveals contradictory evidence, don't ignore it in the conclusion. This will damage your credibility as an expert and might even serve to highlight the contradictions.
Be as transparent as possible and admit to any shortcomings in your research, but don't dwell on them for too long.
Ambiguous or unclear resolutions
The point of a research paper conclusion is to provide closure and bring all your ideas together. You should wrap up any arguments you introduced in the paper and tie up any loose ends, while demonstrating why your research and data are strong.
Use direct language in your conclusion and avoid ambiguity. Even if some of the data and sources you cite are inconclusive or contradictory, note this in your conclusion to come across as confident and trustworthy.
- Examples of research paper conclusions
Your research paper should provide a compelling close to the paper as a whole, highlighting your research and hard work. While the conclusion should represent your unique style, these examples offer a starting point:
Ultimately, the data we examined all point to the same conclusion: Encouraging a good work-life balance improves employee productivity and benefits the company overall. The research suggests that when employees feel their personal lives are valued and respected by their employers, they are more likely to be productive when at work. In addition, company turnover tends to be reduced when employees have a balance between their personal and professional lives. While additional research is required to establish ways companies can support employees in creating a stronger work-life balance, it's clear the need is there.
Social media is a primary method of communication among young people. As we've seen in the data presented, most young people in high school use a variety of social media applications at least every hour, including Instagram and Facebook. While social media is an avenue for connection with peers, research increasingly suggests that social media use correlates with body image issues. Young girls with lower self-esteem tend to use social media more often than those who don't log onto social media apps every day. As new applications continue to gain popularity, and as more high school students are given smartphones, more research will be required to measure the effects of prolonged social media use.
What are the different kinds of research paper conclusions?
There are no formal types of research paper conclusions. Ultimately, the conclusion depends on the outline of your paper and the type of research you’re presenting. While some experts note that research papers can end with a new perspective or commentary, most papers should conclude with a combination of both. The most important aspect of a good research paper conclusion is that it accurately represents the body of the paper.
Can I present new arguments in my research paper conclusion?
Research paper conclusions are not the place to introduce new data or arguments. The body of your paper is where you should share research and insights, where the reader is actively absorbing the content. By the time a reader reaches the conclusion of the research paper, they should have formed their opinion. Introducing new arguments in the conclusion can take a reader by surprise, and not in a positive way. It might also serve to frustrate readers.
How long should a research paper conclusion be?
There's no set length for a research paper conclusion. However, it's a good idea not to run on too long, since conclusions are supposed to be succinct. A good rule of thumb is to keep your conclusion around 5 to 10 percent of the paper's total length. If your paper is 10 pages, try to keep your conclusion under one page.
What should I include in a research paper conclusion?
A good research paper conclusion should always include a sense of urgency, so the reader can see how and why the topic should matter to them. You can also note some recommended actions to help fix the problem and some obstacles they might encounter. A conclusion should also remind the reader of the thesis statement, along with the main points you covered in the paper. At the end of the conclusion, add a powerful closing statement that helps cement the paper in the mind of the reader.
Get started today
Go from raw data to valuable insights with a flexible research platform
Editor’s picks
Last updated: 21 December 2023
Last updated: 16 December 2023
Last updated: 6 October 2023
Last updated: 25 November 2023
Last updated: 12 May 2023
Last updated: 15 February 2024
Last updated: 11 March 2024
Last updated: 12 December 2023
Last updated: 18 May 2023
Last updated: 6 March 2024
Last updated: 10 April 2023
Last updated: 20 December 2023
Latest articles
Related topics, log in or sign up.
Get started for free
- PRO Courses Guides New Tech Help Pro Expert Videos About wikiHow Pro Upgrade Sign In
- EDIT Edit this Article
- EXPLORE Tech Help Pro About Us Random Article Quizzes Request a New Article Community Dashboard This Or That Game Popular Categories Arts and Entertainment Artwork Books Movies Computers and Electronics Computers Phone Skills Technology Hacks Health Men's Health Mental Health Women's Health Relationships Dating Love Relationship Issues Hobbies and Crafts Crafts Drawing Games Education & Communication Communication Skills Personal Development Studying Personal Care and Style Fashion Hair Care Personal Hygiene Youth Personal Care School Stuff Dating All Categories Arts and Entertainment Finance and Business Home and Garden Relationship Quizzes Cars & Other Vehicles Food and Entertaining Personal Care and Style Sports and Fitness Computers and Electronics Health Pets and Animals Travel Education & Communication Hobbies and Crafts Philosophy and Religion Work World Family Life Holidays and Traditions Relationships Youth
- Browse Articles
- Learn Something New
- Quizzes Hot
- This Or That Game
- Train Your Brain
- Explore More
- Support wikiHow
- About wikiHow
- Log in / Sign up
- Education and Communications
- College University and Postgraduate
- Academic Writing
- Research Papers
How to Write a Conclusion for a Research Paper
Last Updated: June 29, 2023 Approved
This article was co-authored by Christopher Taylor, PhD . Christopher Taylor is an Adjunct Assistant Professor of English at Austin Community College in Texas. He received his PhD in English Literature and Medieval Studies from the University of Texas at Austin in 2014. wikiHow marks an article as reader-approved once it receives enough positive feedback. This article received 42 testimonials and 82% of readers who voted found it helpful, earning it our reader-approved status. This article has been viewed 2,258,016 times.
The conclusion of a research paper needs to summarize the content and purpose of the paper without seeming too wooden or dry. Every basic conclusion must share several key elements, but there are also several tactics you can play around with to craft a more effective conclusion and several you should avoid to prevent yourself from weakening your paper's conclusion. Here are some writing tips to keep in mind when creating a conclusion for your next research paper.
Sample Conclusions
Writing a basic conclusion.

- Do not spend a great amount of time or space restating your topic.
- A good research paper will make the importance of your topic apparent, so you do not need to write an elaborate defense of your topic in the conclusion.
- Usually a single sentence is all you need to restate your topic.
- An example would be if you were writing a paper on the epidemiology of infectious disease, you might say something like "Tuberculosis is a widespread infectious disease that affects millions of people worldwide every year."
- Yet another example from the humanities would be a paper about the Italian Renaissance: "The Italian Renaissance was an explosion of art and ideas centered around artists, writers, and thinkers in Florence."

- A thesis is a narrowed, focused view on the topic at hand.
- This statement should be rephrased from the thesis you included in your introduction. It should not be identical or too similar to the sentence you originally used.
- Try re-wording your thesis statement in a way that complements your summary of the topic of your paper in your first sentence of your conclusion.
- An example of a good thesis statement, going back to the paper on tuberculosis, would be "Tuberculosis is a widespread disease that affects millions of people worldwide every year. Due to the alarming rate of the spread of tuberculosis, particularly in poor countries, medical professionals are implementing new strategies for the diagnosis, treatment, and containment of this disease ."

- A good way to go about this is to re-read the topic sentence of each major paragraph or section in the body of your paper.
- Find a way to briefly restate each point mentioned in each topic sentence in your conclusion. Do not repeat any of the supporting details used within your body paragraphs.
- Under most circumstances, you should avoid writing new information in your conclusion. This is especially true if the information is vital to the argument or research presented in your paper.
- For example, in the TB paper you could summarize the information. "Tuberculosis is a widespread disease that affects millions of people worldwide. Due to the alarming rate of the spread of tuberculosis, particularly in poor countries, medical professionals are implementing new strategies for the diagnosis, treatment, and containment of this disease. In developing countries, such as those in Africa and Southeast Asia, the rate of TB infections is soaring. Crowded conditions, poor sanitation, and lack of access to medical care are all compounding factors in the spread of the disease. Medical experts, such as those from the World Health Organization are now starting campaigns to go into communities in developing countries and provide diagnostic testing and treatments. However, the treatments for TB are very harsh and have many side effects. This leads to patient non-compliance and spread of multi-drug resistant strains of the disease."

- Note that this is not needed for all research papers.
- If you already fully explained what the points in your paper mean or why they are significant, you do not need to go into them in much detail in your conclusion. Simply restating your thesis or the significance of your topic should suffice.
- It is always best practice to address important issues and fully explain your points in the body of your paper. The point of a conclusion to a research paper is to summarize your argument for the reader and, perhaps, to call the reader to action if needed.

- Note that a call for action is not essential to all conclusions. A research paper on literary criticism, for instance, is less likely to need a call for action than a paper on the effect that television has on toddlers and young children.
- A paper that is more likely to call readers to action is one that addresses a public or scientific need. Let's go back to our example of tuberculosis. This is a very serious disease that is spreading quickly and with antibiotic-resistant forms.
- A call to action in this research paper would be a follow-up statement that might be along the lines of "Despite new efforts to diagnose and contain the disease, more research is needed to develop new antibiotics that will treat the most resistant strains of tuberculosis and ease the side effects of current treatments."

- For example, if you are writing a history paper, then you might discuss how the historical topic you discussed matters today. If you are writing about a foreign country, then you might use the conclusion to discuss how the information you shared may help readers understand their own country.
Making Your Conclusion as Effective as Possible

- Since this sort of conclusion is so basic, you must aim to synthesize the information rather than merely summarizing it.
- Instead of merely repeating things you already said, rephrase your thesis and supporting points in a way that ties them all together.
- By doing so, you make your research paper seem like a "complete thought" rather than a collection of random and vaguely related ideas.

- Ask a question in your introduction. In your conclusion, restate the question and provide a direct answer.
- Write an anecdote or story in your introduction but do not share the ending. Instead, write the conclusion to the anecdote in the conclusion of your paper.
- For example, if you wanted to get more creative and put a more humanistic spin on a paper on tuberculosis, you might start your introduction with a story about a person with the disease, and refer to that story in your conclusion. For example, you could say something like this before you re-state your thesis in your conclusion: "Patient X was unable to complete the treatment for tuberculosis due to severe side effects and unfortunately succumbed to the disease."
- Use the same concepts and images introduced in your introduction in your conclusion. The images may or may not appear at other points throughout the research paper.

- Include enough information about your topic to back the statement up but do not get too carried away with excess detail.
- If your research did not provide you with a clear-cut answer to a question posed in your thesis, do not be afraid to indicate as much.
- Restate your initial hypothesis and indicate whether you still believe it or if the research you performed has begun swaying your opinion.
- Indicate that an answer may still exist and that further research could shed more light on the topic at hand.

- This may not be appropriate for all types of research papers. Most research papers, such as one on effective treatment for diseases, will have the information to make the case for a particular argument already in the paper.
- A good example of a paper that might ask a question of the reader in the ending is one about a social issue, such as poverty or government policy.
- Ask a question that will directly get at the heart or purpose of the paper. This question is often the same question, or some version of it, that you may have started with when you began your research.
- Make sure that the question can be answered by the evidence presented in your paper.
- If desired you can briefly summarize the answer after stating the question. You could also leave the question hanging for the reader to answer, though.

- Even without a call to action, you can still make a recommendation to your reader.
- For instance, if you are writing about a topic like third-world poverty, you can various ways for the reader to assist in the problem without necessarily calling for more research.
- Another example would be, in a paper about treatment for drug-resistant tuberculosis, you could suggest donating to the World Health Organization or research foundations that are developing new treatments for the disease.
Avoiding Common Pitfalls

- These sayings usually sound stiff, unnatural, or trite when used in writing.
- Moreover, using a phrase like "in conclusion" to begin your conclusion is a little too straightforward and tends to lead to a weak conclusion. A strong conclusion can stand on its own without being labeled as such.

- Always state the main argument or thesis in the introduction. A research paper is an analytical discussion of an academic topic, not a mystery novel.
- A good, effective research paper will allow your reader to follow your main argument from start to finish.
- This is why it is best practice to start your paper with an introduction that states your main argument and to end the paper with a conclusion that re-states your thesis for re-iteration.

- All significant information should be introduced in the body of the paper.
- Supporting evidence expands the topic of your paper by making it appear more detailed. A conclusion should narrow the topic to a more general point.
- A conclusion should only summarize what you have already stated in the body of your paper.
- You may suggest further research or a call to action, but you should not bring in any new evidence or facts in the conclusion.

- Most often, a shift in tone occurs when a research paper with an academic tone gives an emotional or sentimental conclusion.
- Even if the topic of the paper is of personal significance for you, you should not indicate as much in your paper.
- If you want to give your paper a more humanistic slant, you could start and end your paper with a story or anecdote that would give your topic more personal meaning to the reader.
- This tone should be consistent throughout the paper, however.

- Apologetic statements include phrases like "I may not be an expert" or "This is only my opinion."
- Statements like this can usually be avoided by refraining from writing in the first-person.
- Avoid any statements in the first-person. First-person is generally considered to be informal and does not fit with the formal tone of a research paper.
Community Q&A

You Might Also Like

- ↑ http://owl.english.purdue.edu/owl/resource/724/04/
- ↑ http://www.crlsresearchguide.org/18_Writing_Conclusion.asp
- ↑ http://writing.wisc.edu/Handbook/PlanResearchPaper.html#conclusion
- ↑ http://writingcenter.unc.edu/handouts/conclusions/
- ↑ http://writing2.richmond.edu/writing/wweb/conclude.html
About This Article

To write a conclusion for a research paper, start by restating your thesis statement to remind your readers what your main topic is and bring everything full circle. Then, briefly summarize all of the main points you made throughout your paper, which will help remind your readers of everything they learned. You might also want to include a call to action if you think more research or work needs to be done on your topic by writing something like, "Despite efforts to contain the disease, more research is needed to develop antibiotics." Finally, end your conclusion by explaining the broader context of your topic and why your readers should care about it, which will help them understand why your topic is relevant and important. For tips from our Academic co-author, like how to avoid common pitfalls when writing your conclusion, scroll down! Did this summary help you? Yes No
- Send fan mail to authors
Reader Success Stories

Ummay Aimen
Sep 30, 2016
Did this article help you?

Oct 22, 2017

Sally Larrin
Mar 17, 2018

Maya Loeven
Jun 4, 2017

Sep 26, 2016

Featured Articles

Trending Articles

Watch Articles

- Terms of Use
- Privacy Policy
- Do Not Sell or Share My Info
- Not Selling Info
Get all the best how-tos!
Sign up for wikiHow's weekly email newsletter
- Affiliate Program

- UNITED STATES
- 台灣 (TAIWAN)
- TÜRKIYE (TURKEY)
- Academic Editing Services
- - Research Paper
- - Journal Manuscript
- - Dissertation
- - College & University Assignments
- Admissions Editing Services
- - Application Essay
- - Personal Statement
- - Recommendation Letter
- - Cover Letter
- - CV/Resume
- Business Editing Services
- - Business Documents
- - Report & Brochure
- - Website & Blog
- Writer Editing Services
- - Script & Screenplay
- Our Editors
- Client Reviews
- Editing & Proofreading Prices
- Wordvice Points
- Partner Discount
- Plagiarism Checker
- APA Citation Generator
- MLA Citation Generator
- Chicago Citation Generator
- Vancouver Citation Generator
- - APA Style
- - MLA Style
- - Chicago Style
- - Vancouver Style
- Writing & Editing Guide
- Academic Resources
- Admissions Resources
How to Write a Research Paper Conclusion Section
What is a conclusion in a research paper?
The conclusion in a research paper is the final paragraph or two in a research paper. In scientific papers, the conclusion usually follows the Discussion section , summarizing the importance of the findings and reminding the reader why the work presented in the paper is relevant.
However, it can be a bit confusing to distinguish the conclusion section/paragraph from a summary or a repetition of your findings, your own opinion, or the statement of the implications of your work. In fact, the conclusion should contain a bit of all of these other parts but go beyond it—but not too far beyond!
The structure and content of the conclusion section can also vary depending on whether you are writing a research manuscript or an essay. This article will explain how to write a good conclusion section, what exactly it should (and should not) contain, how it should be structured, and what you should avoid when writing it.
Table of Contents:
What does a good conclusion section do, what to include in a research paper conclusion.
- Conclusion in an Essay
- Research Paper Conclusion
- Conclusion Paragraph Outline and Example
- What Not to Do When Writing a Conclusion
The conclusion of a research paper has several key objectives. It should:
- Restate your research problem addressed in the introduction section
- Summarize your main arguments, important findings, and broader implications
- Synthesize key takeaways from your study
The specific content in the conclusion depends on whether your paper presents the results of original scientific research or constructs an argument through engagement with previously published sources.
You presented your general field of study to the reader in the introduction section, by moving from general information (the background of your work, often combined with a literature review ) to the rationale of your study and then to the specific problem or topic you addressed, formulated in the form of the statement of the problem in research or the thesis statement in an essay.
In the conclusion section, in contrast, your task is to move from your specific findings or arguments back to a more general depiction of how your research contributes to the readers’ understanding of a certain concept or helps solve a practical problem, or fills an important gap in the literature. The content of your conclusion section depends on the type of research you are doing and what type of paper you are writing. But whatever the outcome of your work is, the conclusion is where you briefly summarize it and place it within a larger context. It could be called the “take-home message” of the entire paper.
What to summarize in the conclusion
Your conclusion section needs to contain a very brief summary of your work , a very brief summary of the main findings of your work, and a mention of anything else that seems relevant when you now look at your work from a bigger perspective, even if it was not initially listed as one of your main research questions. This could be a limitation, for example, a problem with the design of your experiment that either needs to be considered when drawing any conclusions or that led you to ask a different question and therefore draw different conclusions at the end of your study (compared to when you started out).
Once you have reminded the reader of what you did and what you found, you need to go beyond that and also provide either your own opinion on why your work is relevant (and for whom, and how) or theoretical or practical implications of the study , or make a specific call for action if there is one to be made.
How to Write an Essay Conclusion
Academic essays follow quite different structures than their counterparts in STEM and the natural sciences. Humanities papers often have conclusion sections that are much longer and contain more detail than scientific papers. There are three main types of academic essay conclusions.
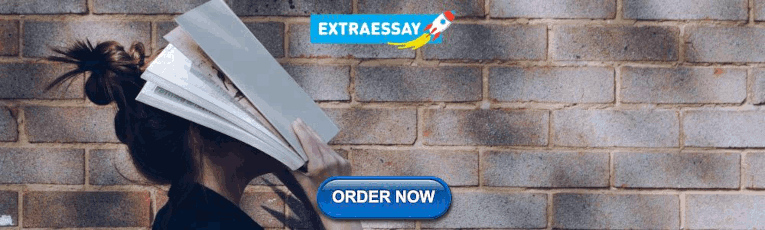
Summarizing conclusion
The most typical conclusion at the end of an analytical/explanatory/argumentative essay is a summarizing conclusion . This is, as the name suggests, a clear summary of the main points of your topic and thesis. Since you might have gone through a number of different arguments or subtopics in the main part of your essay, you need to remind the reader again what those were, how they fit into each other, and how they helped you develop or corroborate your hypothesis.
For an essay that analyzes how recruiters can hire the best candidates in the shortest time or on “how starving yourself will increase your lifespan, according to science”, a summary of all the points you discussed might be all you need. Note that you should not exactly repeat what you said earlier, but rather highlight the essential details and present those to your reader in a different way.
Externalizing conclusion
If you think that just reminding the reader of your main points is not enough, you can opt for an externalizing conclusion instead, that presents new points that were not presented in the paper so far. These new points can be additional facts and information or they can be ideas that are relevant to the topic and have not been mentioned before.
Such a conclusion can stimulate your readers to think about your topic or the implications of your analysis in a whole new way. For example, at the end of a historical analysis of a specific event or development, you could direct your reader’s attention to some current events that were not the topic of your essay but that provide a different context for your findings.
Editorial conclusion
In an editorial conclusion , another common type of conclusion that you will find at the end of papers and essays, you do not add new information but instead present your own experiences or opinions on the topic to round everything up. What makes this type of conclusion interesting is that you can choose to agree or disagree with the information you presented in your paper so far. For example, if you have collected and analyzed information on how a specific diet helps people lose weight, you can nevertheless have your doubts on the sustainability of that diet or its practicability in real life—if such arguments were not included in your original thesis and have therefore not been covered in the main part of your paper, the conclusion section is the place where you can get your opinion across.
How to Conclude an Empirical Research Paper
An empirical research paper is usually more concise and succinct than an essay, because, if it is written well, it focuses on one specific question, describes the method that was used to answer that one question, describes and explains the results, and guides the reader in a logical way from the introduction to the discussion without going on tangents or digging into not absolutely relevant topics.
Summarize the findings
In a scientific paper, you should include a summary of the findings. Don’t go into great detail here (you will have presented your in-depth results and discussion already), but do clearly express the answers to the research questions you investigated.
Describe your main findings, even if they weren’t necessarily the ones anticipated, and explain the conclusion they led you to. Explain these findings in as few words as possible.
Instead of beginning with “ In conclusion, in this study, we investigated the effect of stress on the brain using fMRI …”, you should try to find a way to incorporate the repetition of the essential (and only the essential) details into the summary of the key points. “ The findings of this fMRI study on the effect of stress on the brain suggest that …” or “ While it has been known for a long time that stress has an effect on the brain, the findings of this fMRI study show that, surprisingly… ” would be better ways to start a conclusion.
You should also not bring up new ideas or present new facts in the conclusion of a research paper, but stick to the background information you have presented earlier, to the findings you have already discussed, and the limitations and implications you have already described. The one thing you can add here is a practical recommendation that you haven’t clearly stated before—but even that one needs to follow logically from everything you have already discussed in the discussion section.
Discuss the implications
After summing up your key arguments or findings, conclude the paper by stating the broader implications of the research , whether in methods , approach, or findings. Express practical or theoretical takeaways from your paper. This often looks like a “call to action” or a final “sales pitch” that puts an exclamation point on your paper.
If your research topic is more theoretical in nature, your closing statement should express the significance of your argument—for example, in proposing a new understanding of a topic or laying the groundwork for future research.
Future research example
Future research into education standards should focus on establishing a more detailed picture of how novel pedagogical approaches impact young people’s ability to absorb new and difficult concepts. Moreover, observational studies are needed to gain more insight into how specific teaching models affect the retention of relationships and facts—for instance, how inquiry-based learning and its emphasis on lateral thinking can be used as a jumping-off point for more holistic classroom approaches.
Research Conclusion Example and Outline
Let’s revisit the study on the effect of stress on the brain we mentioned before and see what the common structure for a conclusion paragraph looks like, in three steps. Following these simple steps will make it easy for you to wrap everything up in one short paragraph that contains all the essential information:
One: Short summary of what you did, but integrated into the summary of your findings:
While it has been known for a long time that stress has an effect on the brain, the findings of this fMRI study in 25 university students going through mid-term exams show that, surprisingly, one’s attitude to the experienced stress significantly modulates the brain’s response to it.
Note that you don’t need to repeat any methodological or technical details here—the reader has been presented with all of these before, they have read your results section and the discussion of your results, and even (hopefully!) a discussion of the limitations and strengths of your paper. The only thing you need to remind them of here is the essential outcome of your work.
Two: Add implications, and don’t forget to specify who this might be relevant for:
Students could be considered a specific subsample of the general population, but earlier research shows that the effect that exam stress has on their physical and mental health is comparable to the effects of other types of stress on individuals of other ages and occupations. Further research into practical ways of modulating not only one’s mental stress response but potentially also one’s brain activity (e.g., via neurofeedback training) are warranted.
This is a “research implication”, and it is nicely combined with a mention of a potential limitation of the study (the student sample) that turns out not to be a limitation after all (because earlier research suggests we can generalize to other populations). If there already is a lot of research on neurofeedback for stress control, by the way, then this should have been discussed in your discussion section earlier and you wouldn’t say such studies are “warranted” here but rather specify how your findings could inspire specific future experiments or how they should be implemented in existing applications.
Three: The most important thing is that your conclusion paragraph accurately reflects the content of your paper. Compare it to your research paper title , your research paper abstract , and to your journal submission cover letter , in case you already have one—if these do not all tell the same story, then you need to go back to your paper, start again from the introduction section, and find out where you lost the logical thread. As always, consistency is key.
Problems to Avoid When Writing a Conclusion
- Do not suddenly introduce new information that has never been mentioned before (unless you are writing an essay and opting for an externalizing conclusion, see above). The conclusion section is not where you want to surprise your readers, but the take-home message of what you have already presented.
- Do not simply copy your abstract, the conclusion section of your abstract, or the first sentence of your introduction, and put it at the end of the discussion section. Even if these parts of your paper cover the same points, they should not be identical.
- Do not start the conclusion with “In conclusion”. If it has its own section heading, that is redundant, and if it is the last paragraph of the discussion section, it is inelegant and also not really necessary. The reader expects you to wrap your work up in the last paragraph, so you don’t have to announce that. Just look at the above example to see how to start a conclusion in a natural way.
- Do not forget what your research objectives were and how you initially formulated the statement of the problem in your introduction section. If your story/approach/conclusions changed because of methodological issues or information you were not aware of when you started, then make sure you go back to the beginning and adapt your entire story (not just the ending).
Consider Receiving Academic Editing Services
When you have arrived at the conclusion of your paper, you might want to head over to Wordvice AI’s AI Writing Assistant to receive a free grammar check for any academic content.
After drafting, you can also receive English editing and proofreading services , including paper editing services for your journal manuscript. If you need advice on how to write the other parts of your research paper , or on how to make a research paper outline if you are struggling with putting everything you did together, then head over to the Wordvice academic resources pages , where we have a lot more articles and videos for you.
Purdue Online Writing Lab Purdue OWL® College of Liberal Arts
Conclusions
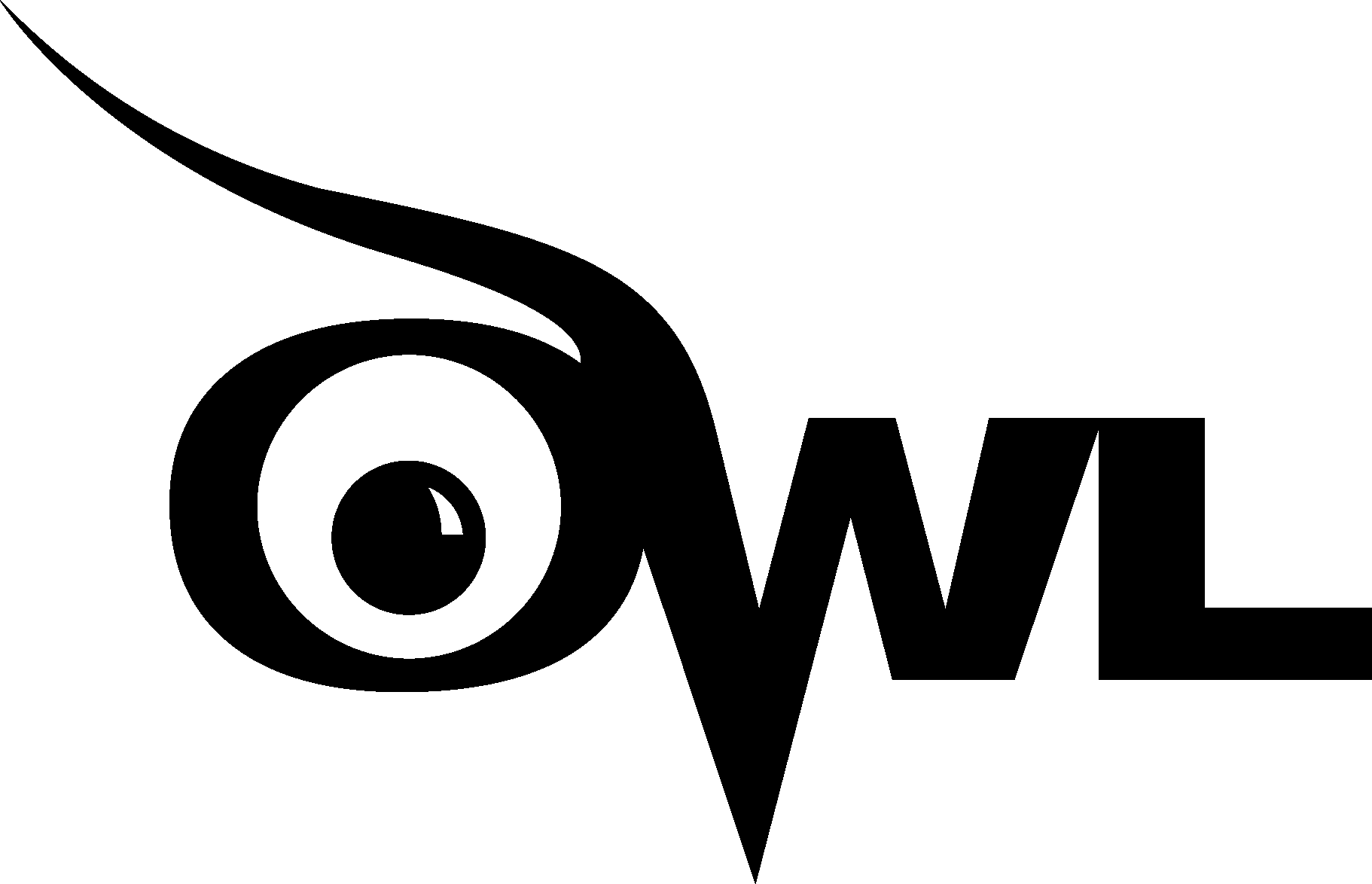
Welcome to the Purdue OWL
This page is brought to you by the OWL at Purdue University. When printing this page, you must include the entire legal notice.
Copyright ©1995-2018 by The Writing Lab & The OWL at Purdue and Purdue University. All rights reserved. This material may not be published, reproduced, broadcast, rewritten, or redistributed without permission. Use of this site constitutes acceptance of our terms and conditions of fair use.
Conclusions wrap up what you have been discussing in your paper. After moving from general to specific information in the introduction and body paragraphs, your conclusion should begin pulling back into more general information that restates the main points of your argument. Conclusions may also call for action or overview future possible research. The following outline may help you conclude your paper:
In a general way,
- Restate your topic and why it is important,
- Restate your thesis/claim,
- Address opposing viewpoints and explain why readers should align with your position,
- Call for action or overview future research possibilities.
Remember that once you accomplish these tasks, unless otherwise directed by your instructor, you are finished. Done. Complete. Don't try to bring in new points or end with a whiz bang(!) conclusion or try to solve world hunger in the final sentence of your conclusion. Simplicity is best for a clear, convincing message.
The preacher's maxim is one of the most effective formulas to follow for argument papers:
Tell what you're going to tell them (introduction).
Tell them (body).
Tell them what you told them (conclusion).

Organizing Academic Research Papers: 9. The Conclusion
- Purpose of Guide
- Design Flaws to Avoid
- Glossary of Research Terms
- Narrowing a Topic Idea
- Broadening a Topic Idea
- Extending the Timeliness of a Topic Idea
- Academic Writing Style
- Choosing a Title
- Making an Outline
- Paragraph Development
- Executive Summary
- Background Information
- The Research Problem/Question
- Theoretical Framework
- Citation Tracking
- Content Alert Services
- Evaluating Sources
- Primary Sources
- Secondary Sources
- Tertiary Sources
- What Is Scholarly vs. Popular?
- Qualitative Methods
- Quantitative Methods
- Using Non-Textual Elements
- Limitations of the Study
- Common Grammar Mistakes
- Avoiding Plagiarism
- Footnotes or Endnotes?
- Further Readings
- Annotated Bibliography
- Dealing with Nervousness
- Using Visual Aids
- Grading Someone Else's Paper
- How to Manage Group Projects
- Multiple Book Review Essay
- Reviewing Collected Essays
- About Informed Consent
- Writing Field Notes
- Writing a Policy Memo
- Writing a Research Proposal
- Acknowledgements
The conclusion is intended to help the reader understand why your research should matter to them after they have finished reading the paper. A conclusion is not merely a summary of your points or a re-statement of your research problem but a synthesis of key points. For most essays, one well-developed paragraph is sufficient for a conclusion, although in some cases, a two-or-three paragraph conclusion may be required.
Importance of a Good Conclusion
A well-written conclusion provides you with several important opportunities to demonstrate your overall understanding of the research problem to the reader. These include:
- Presenting the last word on the issues you raised in your paper . Just as the introduction gives a first impression to your reader, the conclusion offers a chance to leave a lasting impression. Do this, for example, by highlighting key points in your analysis or findings.
- Summarizing your thoughts and conveying the larger implications of your study . The conclusion is an opportunity to succinctly answer the "so what?" question by placing the study within the context of past research about the topic you've investigated.
- Demonstrating the importance of your ideas . Don't be shy. The conclusion offers you a chance to elaborate on the significance of your findings.
- Introducing possible new or expanded ways of thinking about the research problem . This does not refer to introducing new information [which should be avoided], but to offer new insight and creative approaches for framing/contextualizing the research problem based on the results of your study.
Conclusions . The Writing Center. University of North Carolina; Kretchmer, Paul. Twelve Steps to Writing an Effective Conclusion . San Francisco Edit, 2003-2008.
Structure and Writing Style
https://writing.wisc.edu/wp-content/uploads/sites/535/2018/07/conclusions_uwmadison_writingcenter_aug2012.pdf I. General Rules
When writing the conclusion to your paper, follow these general rules:
- State your conclusions in clear, simple language.
- Do not simply reiterate your results or the discussion.
- Indicate opportunities for future research, as long as you haven't already done so in the discussion section of your paper.
The function of your paper's conclusion is to restate the main argument . It reminds the reader of the strengths of your main argument(s) and reiterates the most important evidence supporting those argument(s). Make sure, however, that your conclusion is not simply a repetitive summary of the findings because this reduces the impact of the argument(s) you have developed in your essay.
Consider the following points to help ensure your conclusion is appropriate:
- If the argument or point of your paper is complex, you may need to summarize the argument for your reader.
- If, prior to your conclusion, you have not yet explained the significance of your findings or if you are proceeding inductively, use the end of your paper to describe your main points and explain their significance.
- Move from a detailed to a general level of consideration that returns the topic to the context provided by the introduction or within a new context that emerges from the data.
The conclusion also provides a place for you to persuasively and succinctly restate your research problem, given that the reader has now been presented with all the information about the topic . Depending on the discipline you are writing in, the concluding paragraph may contain your reflections on the evidence presented, or on the essay's central research problem. However, the nature of being introspective about the research you have done will depend on the topic and whether your professor wants you to express your observations in this way.
NOTE : Don't delve into idle speculation. Being introspective means looking within yourself as an author to try and understand an issue more deeply not to guess at possible outcomes.
II. Developing a Compelling Conclusion
Strategies to help you move beyond merely summarizing the key points of your research paper may include any of the following.
- If your essay deals with a contemporary problem, warn readers of the possible consequences of not attending to the problem.
- Recommend a specific course or courses of action.
- Cite a relevant quotation or expert opinion to lend authority to the conclusion you have reached [a good place to look is research from your literature review].
- Restate a key statistic, fact, or visual image to drive home the ultimate point of your paper.
- If your discipline encourages personal reflection, illustrate your concluding point with a relevant narrative drawn from your own life experiences.
- Return to an anecdote, an example, or a quotation that you introduced in your introduction, but add further insight that is derived from the findings of your study; use your interpretation of results to reframe it in new ways.
- Provide a "take-home" message in the form of a strong, succient statement that you want the reader to remember about your study.
III. Problems to Avoid Failure to be concise The conclusion section should be concise and to the point. Conclusions that are too long often have unnecessary detail. The conclusion section is not the place for details about your methodology or results. Although you should give a summary of what was learned from your research, this summary should be relatively brief, since the emphasis in the conclusion is on the implications, evaluations, insights, etc. that you make. Failure to comment on larger, more significant issues In the introduction, your task was to move from general [the field of study] to specific [your research problem]. However, in the conclusion, your task is to move from specific [your research problem] back to general [your field, i.e., how your research contributes new understanding or fills an important gap in the literature]. In other words, the conclusion is where you place your research within a larger context. Failure to reveal problems and negative results Negative aspects of the research process should never be ignored. Problems, drawbacks, and challenges encountered during your study should be included as a way of qualifying your overall conclusions. If you encountered negative results [findings that are validated outside the research context in which they were generated], you must report them in the results section of your paper. In the conclusion, use the negative results as an opportunity to explain how they provide information on which future research can be based. Failure to provide a clear summary of what was learned In order to be able to discuss how your research fits back into your field of study [and possibly the world at large], you need to summarize it briefly and directly. Often this element of your conclusion is only a few sentences long. Failure to match the objectives of your research Often research objectives change while the research is being carried out. This is not a problem unless you forget to go back and refine your original objectives in your introduction, as these changes emerge they must be documented so that they accurately reflect what you were trying to accomplish in your research [not what you thought you might accomplish when you began].
Resist the urge to apologize If you've immersed yourself in studying the research problem, you now know a good deal about it, perhaps even more than your professor! Nevertheless, by the time you have finished writing, you may be having some doubts about what you have produced. Repress those doubts! Don't undermine your authority by saying something like, "This is just one approach to examining this problem; there may be other, much better approaches...."
Concluding Paragraphs. College Writing Center at Meramec. St. Louis Community College; Conclusions . The Writing Center. University of North Carolina; Conclusions . The Writing Lab and The OWL. Purdue University; Freedman, Leora and Jerry Plotnick. Introductions and Conclusions . The Lab Report. University College Writing Centre. University of Toronto; Leibensperger, Summer. Draft Your Conclusion. Academic Center, the University of Houston-Victoria, 2003; Make Your Last Words Count . The Writer’s Handbook. Writing Center. University of Wisconsin, Madison; Tips for Writing a Good Conclusion . Writing@CSU. Colorado State University; Kretchmer, Paul. Twelve Steps to Writing an Effective Conclusion . San Francisco Edit, 2003-2008; Writing Conclusions . Writing Tutorial Services, Center for Innovative Teaching and Learning. Indiana University; Writing: Considering Structure and Organization . Institute for Writing Rhetoric. Dartmouth College.
Writing Tip
Don't Belabor the Obvious!
Avoid phrases like "in conclusion...," "in summary...," or "in closing...." These phrases can be useful, even welcome, in oral presentations. But readers can see by the tell-tale section heading and number of pages remaining to read, when an essay is about to end. You'll irritate your readers if you belabor the obvious.
Another Writing Tip
New Insight, Not New Information!
Don't surprise the reader with new information in your Conclusion that was never referenced anywhere else in the paper. If you have new information to present, add it to the Discussion or other appropriate section of the paper. Note that, although no actual new information is introduced, the conclusion is where you offer your most "original" contributions in the paper; it's where you describe the value of your research, demonstrate your understanding of the material that you’ve presented, and locate your findings within the larger context of scholarship on the topic.
- << Previous: Limitations of the Study
- Next: Appendices >>
- Last Updated: Jul 18, 2023 11:58 AM
- URL: https://library.sacredheart.edu/c.php?g=29803
- QuickSearch
- Library Catalog
- Databases A-Z
- Publication Finder
- Course Reserves
- Citation Linker
- Digital Commons
- Our Website
Research Support
- Ask a Librarian
- Appointments
- Interlibrary Loan (ILL)
- Research Guides
- Databases by Subject
- Citation Help
Using the Library
- Reserve a Group Study Room
- Renew Books
- Honors Study Rooms
- Off-Campus Access
- Library Policies
- Library Technology
User Information
- Grad Students
- Online Students
- COVID-19 Updates
- Staff Directory
- News & Announcements
- Library Newsletter
My Accounts
- Interlibrary Loan
- Staff Site Login

FIND US ON
Research Paper Guide
Research Paper Discussion Section

How To Write A Discussion For A Research Paper | Examples & Tips
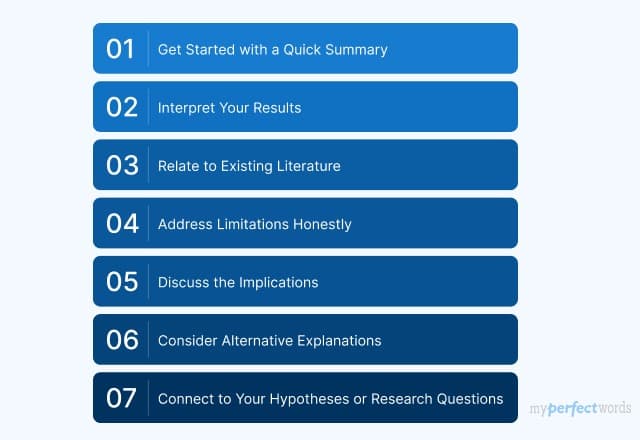
People also read
Research Paper Writing - A Step by Step Guide
Research Paper Examples - Free Sample Papers for Different Formats!
Guide to Creating Effective Research Paper Outline
Interesting Research Paper Topics for 2024
Research Proposal Writing - A Step-by-Step Guide
How to Start a Research Paper - 7 Easy Steps
How to Write an Abstract for a Research Paper - A Step by Step Guide
Writing a Literature Review For a Research Paper - A Comprehensive Guide
Qualitative Research - Methods, Types, and Examples
8 Types of Qualitative Research - Overview & Examples
Qualitative vs Quantitative Research - Learning the Basics
200+ Engaging Psychology Research Paper Topics for Students in 2024
Learn How to Write a Hypothesis in a Research Paper: Examples and Tips!
20+ Types of Research With Examples - A Detailed Guide
Understanding Quantitative Research - Types & Data Collection Techniques
230+ Sociology Research Topics & Ideas for Students
How to Cite a Research Paper - A Complete Guide
Excellent History Research Paper Topics- 300+ Ideas
A Guide on Writing the Method Section of a Research Paper - Examples & Tips
How To Write an Introduction Paragraph For a Research Paper: Learn with Examples
Crafting a Winning Research Paper Title: A Complete Guide
Writing a Research Paper Conclusion - Step-by-Step Guide
Writing a Thesis For a Research Paper - A Comprehensive Guide
How To Write The Results Section of A Research Paper | Steps & Examples
Writing a Problem Statement for a Research Paper - A Comprehensive Guide
Finding Sources For a Research Paper: A Complete Guide
A Guide on How to Edit a Research Paper
200+ Ethical Research Paper Topics to Begin With (2024)
300+ Controversial Research Paper Topics & Ideas - 2024 Edition
150+ Argumentative Research Paper Topics For You - 2024
How to Write a Research Methodology for a Research Paper
Ever find yourself stuck when trying to write the discussion part of your research paper? Don't worry, it happens to a lot of people.
The discussion section is super important in your research paper . It's where you explain what your results mean. But turning all that data into a clear and meaningful story? That's not easy.
Guess what? MyPerfectWords.com has come up with a solution.
This blog is your guide to writing an outstanding discussion section. We'll guide you step by step with useful tips to make sure your research stands out.
So, let’s get started!

- 1. What Exactly is a Discussion Section in the Research Paper?
- 2. How to Write the Discussion Section of a Research Paper?
- 3. Examples of Good Discussion for a Research Paper
- 4. Mistakes to Avoid in Your Research Paper's Discussion
What Exactly is a Discussion Section in the Research Paper?
In a research paper, the discussion section is where you explain what your results really mean. It's like answering the questions, "So what?" and "What's the big picture?"
The discussion section is your chance to help your readers understand why your findings are important and how they fit into the larger context. It's more than just summarizing; it's about making your research understandable and meaningful to others.
Importance of the Discussion Section
The discussion section isn't just a formality; it's the heart of your research paper. This is where your findings transform from data into knowledge.
Let's break down why it's so crucial:
- Interpretation of Results : The discussion is where you get to tell readers what your results really mean. You go into the details, helping them understand the story behind the numbers or findings.
- Connecting the Dots : You connect different parts of your research, showing how they relate. This helps your readers see the bigger picture.
- Relevance to the Big Picture : You get to highlight why your research matters. How does it contribute to the broader understanding of the topic? This is your time to make your research significant.
- Addressing Limitations : In the discussion, you can acknowledge any limitations in your study and discuss how they might impact your results.
- Suggestions for Further Research : The discussion is where you suggest areas for future exploration. It's like passing the baton to the next researcher, indicating where more work could be done.
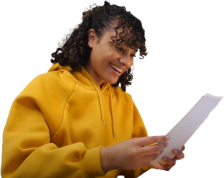
Tough Essay Due? Hire Tough Writers!
How to Write the Discussion Section of a Research Paper?
The Discussion section in a research paper plays a vital role in interpreting findings and formulating a conclusion . Given below are the main components of the discussion section:
- Quick Summary: A brief recap of your main findings.
- Interpretation: Significance and meaning of your results in relation to your research question.
- Literature Review : Connecting your findings with previous research or similar studies.
- Limitations: Discussing any study limitations, addressing potential concerns.
- Implications: Broader implications of your findings, considering practical and theoretical aspects.
- Alternative Explanations: Evaluating alternative interpretations, demonstrating a comprehensive analysis.
- Connecting to Hypotheses : Summarizing how your result section aligns or diverges from your initial hypotheses.
Now let’s explore the steps to write an effective discussion section that will effectively communicate the significance of your research:
Step 1: Get Started with a Quick Summary
Start by quickly telling your readers the main things you found in your research. Don't explain them in detail just yet; just give a simple overview.
This helps your readers get the big picture before diving into the details.
Step 2: Interpret Your Results
In the next step, talk about what your findings really mean. Share why the information you gathered is important. Connect each result to the questions you were trying to answer and the goals you set for your research.
Step 3: Relate to Existing Literature
In this step, link up your discoveries with what other researchers have already figured out.
Share if your results are similar to or different from what's been found before. This helps give more background to your study and shows you know what other scientists have been up to.
Step 4: Address Limitations Honestly
Every study has its limitations. Acknowledge them openly in your discussion. This not only shows transparency but also helps readers interpret your results more accurately.
Step 5: Discuss the Implications
Explore the implications of your findings. How do they contribute to the field? What real-world applications or changes might they suggest?
Dig into why your discoveries are important. How do they help the subject you studied?
This step is like looking at the bigger picture and asking, "So, what can we do with this information?"
Paper Due? Why Suffer? That's our Job!
Step 6: Consider Alternative Explanations
After discussing the implications, challenge yourself by exploring alternative explanations for your results.
Discuss different perspectives and show that you've considered multiple angles.
Step 7: Connect to Your Hypotheses or Research Questions
For the last step, revisit your initial hypotheses or research questions. Explain whether your results support what you thought might happen or if they surprised you.
Examples of Good Discussion for a Research Paper
Learning from well-crafted discussions can significantly enhance your own writing. Given below are some examples to help you understand how to write your own.
Discussion for a Research Paper Example Pdf
Discussion for a Medical Research Paper
Discussion Section for a Qualitative Research Paper
Mistakes to Avoid in Your Research Paper's Discussion
Writing the discussion section of your research paper can be tricky. To make sure you're on the right track, be mindful of these common mistakes:
- Overstating or Overinterpreting Results
Avoid making your findings sound more groundbreaking than they are. Stick to what your data actually shows, and don't exaggerate.
- Neglecting Alternative Explanations
Failing to consider other possible explanations for your results can weaken your discussion. Always explore alternative perspectives to present a well-rounded view.
- Ignoring Limitations
Don't sweep limitations under the rug. Acknowledge them openly and discuss how they might affect the validity or generalizability of your results.
- Being Overly Technical or Jargon-laden
Remember that your audience may not be experts in your specific field. Avoid using overly technical language or excessive jargon that could alienate your readers.
- Disregarding the 'So What' Factor
Always explain the significance of your findings. Don't leave your readers wondering why your research matters or how it contributes to the broader understanding of the subject.
- Rushing the Conclusion
The conclusion section of your discussion is critical. Don't rush it. Summarize the key points and leave your readers with a strong understanding of the significance of your research.
So, there you have it —writing a discussion and conclusion section isn't easy, but avoiding some common mistakes can make it much smoother.
Remember to keep it real with your results, think about what else could explain things, and don't forget about any limits in your study.
But if you're feeling stuck, MyPerfectWords.com is here for you.
Our team of experts knows their way around discussions. Whether you need some guidance or want someone to handle the writing for you, we've got your back.
Don't let discussion writing stress you out. Let our essay writing service for college make your academic life easier.

Write Essay Within 60 Seconds!

Dr. Barbara is a highly experienced writer and author who holds a Ph.D. degree in public health from an Ivy League school. She has worked in the medical field for many years, conducting extensive research on various health topics. Her writing has been featured in several top-tier publications.

Paper Due? Why Suffer? That’s our Job!
Keep reading
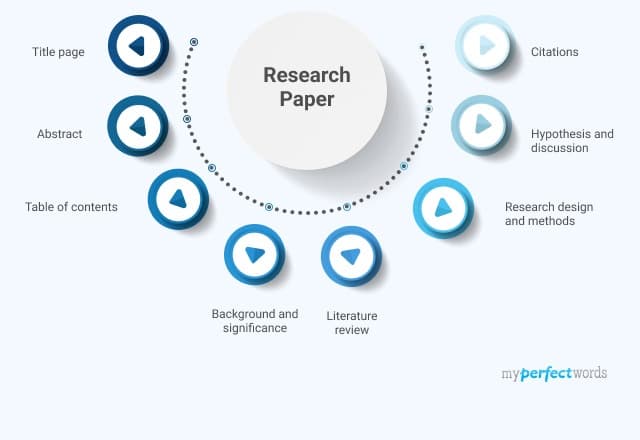
- Open access
- Published: 17 April 2024
A data-driven combined prediction method for the demand for intensive care unit healthcare resources in public health emergencies
- Weiwei Zhang 1 &
- Xinchun Li 1
BMC Health Services Research volume 24 , Article number: 477 ( 2024 ) Cite this article
246 Accesses
1 Altmetric
Metrics details
Public health emergencies are characterized by uncertainty, rapid transmission, a large number of cases, a high rate of critical illness, and a high case fatality rate. The intensive care unit (ICU) is the “last line of defense” for saving lives. And ICU resources play a critical role in the treatment of critical illness and combating public health emergencies.
This study estimates the demand for ICU healthcare resources based on an accurate prediction of the surge in the number of critically ill patients in the short term. The aim is to provide hospitals with a basis for scientific decision-making, to improve rescue efficiency, and to avoid excessive costs due to overly large resource reserves.
A demand forecasting method for ICU healthcare resources is proposed based on the number of current confirmed cases. The number of current confirmed cases is estimated using a bilateral long-short-term memory and genetic algorithm support vector regression (BILSTM-GASVR) combined prediction model. Based on this, this paper constructs demand forecasting models for ICU healthcare workers and healthcare material resources to more accurately understand the patterns of changes in the demand for ICU healthcare resources and more precisely meet the treatment needs of critically ill patients.
Data on the number of COVID-19-infected cases in Shanghai between January 20, 2020, and September 24, 2022, is used to perform a numerical example analysis. Compared to individual prediction models (GASVR, LSTM, BILSTM and Informer), the combined prediction model BILSTM-GASVR produced results that are closer to the real values. The demand forecasting results for ICU healthcare resources showed that the first (ICU human resources) and third (medical equipment resources) categories did not require replenishment during the early stages but experienced a lag in replenishment when shortages occurred during the peak period. The second category (drug resources) is consumed rapidly in the early stages and required earlier replenishment, but replenishment is timelier compared to the first and third categories. However, replenishment is needed throughout the course of the epidemic.
The first category of resources (human resources) requires long-term planning and the deployment of emergency expansion measures. The second category of resources (drugs) is suitable for the combination of dynamic physical reserves in healthcare institutions with the production capacity reserves of corporations. The third category of resources (medical equipment) is more dependent on the physical reserves in healthcare institutions, but care must be taken to strike a balance between normalcy and emergencies.
Peer Review reports
Introduction
The outbreak of severe acute respiratory syndrome (SARS) in 2003 was the first global public health emergency of the 21st century. From SARS to the coronavirus disease (COVID-19) pandemic at the end of 2019, followed shortly by the monkeypox epidemic of 2022, the global community has witnessed eight major public health events within the span of only 20 years [ 1 ]. These events are all characterized by high infection and fatality rates. For example, the number of confirmed COVID-19 cases worldwide is over 700 million, and the number of deaths has exceeded 7 million [ 2 ]. Every major public health emergency typically consists of four stages: incubation, outbreak, peak, and decline. During the outbreak and transmission, surges in the number of infected individuals and the number of critically ill patients led to a corresponding increase in the urgent demand for intensive care unit (ICU) medical resources. ICU healthcare resources provide material security for rescue work during major public health events as they allow critically ill patients to be treated, which decreases the case fatality rate and facilitates the prevention and control of epidemics. Nevertheless, in actual cases of prevention and control, the surge in patients has often led to shortages of ICU healthcare resources and a short-term mismatch of supply and demand, which are problems that have occurred several times in different regions. These issues can drastically impact anti-epidemic frontline healthcare workers and the treatment outcomes of infected patients. According to COVID-19 data from recent years, many infected individuals take about two weeks to progress from mild to severe disease. As the peak of severe cases tends to lag behind that of infected cases, predicting the changes in the number of new infections can serve as a valuable reference for healthcare institutions in forecasting the demand for ICU healthcare resources. The accurate forecasting of the demand for ICU healthcare resources can facilitate the rational resource allocation of hospitals under changes in demand patterns, which is crucial for improving the provision of critical care and rescue efficiency. Therefore, in this study, we combined a support vector regression (SVR) prediction model optimized by a genetic algorithm (GA) with bidirectional long-short-term memory (BILSTM), with the aim of enhancing the dynamic and accurate prediction of the number of current confirmed cases. Based on this, we forecasted the demand for ICU healthcare resources, which in turn may enable more efficient resource deployment during severe epidemic outbreaks and improve the precise supply of ICU healthcare resources.
Research on the demand forecasting of emergency materials generally employs quantitative methods, and traditional approaches mainly include linear regression and GM (1,1). Linear regression involves the use of regression equations to make predictions based on data. Sui et al. proposed a method based on multiple regression that aimed to predict the demand for emergency supplies in the power grid system following natural disasters [ 3 ]. Historical data was used to obtain the impact coefficient of each factor on emergency resource forecasting, enabling the quick calculation of the demand for each emergency resource during a given type of disaster. However, to ensure prediction accuracy, regression analysis needs to be supported by data from a large sample size. Other researchers have carried out demand forecasting for emergency supplies from the perspective of grey prediction models. Li et al. calculated the development coefficient and grey action of the grey GM (1,1) model using the particle swarm optimization algorithm to minimize the relative errors between the real and predicted values [ 4 ]. Although these studies have improved the prediction accuracy of grey models, they mainly involve pre-processing the initial data series without considering the issue of the excessively fast increase in predicted values by traditional grey GM (1,1) models. In emergency situations, the excessively fast increase in predicted values compared to real values will result in the consumption of a large number of unnecessary resources, thereby decreasing efficiency and increasing costs. As traditional demand forecasting models for emergency supplies have relatively poor perfect order rates in demand analysis, which result in low prediction accuracy, they are not mainstream.
At present, dynamic models of infectious diseases and demand forecasting models based on machine learning are at the cutting edge of research. With regard to the dynamic models of infectious diseases, susceptible infected recovered model (SIR) is a classic mathematical model employed by researchers [ 5 , 6 , 7 ]. After many years of development, the SIR model has been expanded into various forms within the field of disease transmission, including susceptible exposed infected recovered model (SEIR) and susceptible exposed infected recovered dead model (SEIRD) [ 8 , 9 ]. Nevertheless, with the outbreak of COVID-19, dynamic models of infectious diseases have once again come under the spotlight, with researchers combining individual and group variables and accounting for different factors to improve the initial models and reflect the state of COVID-19 [ 10 , 11 , 12 , 13 ]. Based on the first round of epidemic data from Wuhan, Li et al. predicted the time-delay distributions, epidemic doubling time, and basic reproductive number [ 14 ]. Upon discovering the presence of asymptomatic COVID-19 infections, researchers began constructing different SEIR models that considered the infectivity of various viral incubation periods, yielding their respective predictions of the inflection point. Based on this, Anggriani et al. further considered the impact of the status of infected individuals and established a transmission model with seven compartments [ 15 ]. Efimov et al. set the model parameters for separating the recovered and the dead as uncertain and applied the improved SEIR model to analyze the transmission trend of the pandemic [ 16 ]. In addition to analyzing the transmission characteristics of normal COVID-19 infection to predict the status of the epidemic, many researchers have also used infectious disease models to evaluate the effects of various epidemic preventive measures. Lin et al. applied an SEIR model that considered individual behavioral responses, government restrictions on public gatherings, pet-related transmission, and short-term population movements [ 17 ]. Cao et al. considered the containment effect of isolation measures on the pandemic and solved the model using Euler’s numerical method [ 18 ]. Reiner et al. employed an improved SEIR model to study the impact of non-pharmaceutical interventions implemented by the government (e.g., restricting population movement, enhancing disease testing, and increasing mask use) on disease transmission and evaluated the effectiveness of social distancing and the closure of public spaces [ 19 ]. These studies have mainly focused on modeling the COVID-19 pandemic to perform dynamic forecasting and analyze the effectiveness of control measures during the epidemic. Infectious disease dynamics offer good predictions for the early transmission trends of epidemics. However, this approach is unable to accurately estimate the spread of the virus in open-flow environments. Furthermore, it is also impossible to set hypothetical parameters, such as disease transmissibility and the recovery probability constant, that are consistent with the conditions in reality. Hence, with the increase in COVID-19 data, this approach has become inadequate for the accurate long-term analysis of epidemic trends.
Machine learning has shown significant advantages in this regard [ 20 , 21 ]. Some researchers have adopted the classic case-based reasoning approach in machine learning to make predictions. However, it is not feasible to find historical cases that fully match the current emergency event, so this approach has limited operability. Other researchers have also employed neural network training in machine learning to make predictions. For example, Hamou et al. predicted the number of injuries and deaths, which in turn were used to forecast the demand for emergency supplies [ 22 ]. However, this approach requires a large initial dataset and a high number of training epochs, while uncertainty due to large changes in intelligence information can lead to significant errors in data prediction [ 23 , 24 , 25 ]. To address these problems, researchers have conducted investigations that account (to varying degrees) for data characterized by time-series and non-linearity and have employed time-series models with good non-linear fitting [ 26 , 27 , 28 ]. The use of LSTM to explore relationships within the data can improve the accuracy of predicting COVID-19 to some extent. However, there are two problems with this approach. First, LSTM neural networks require extremely large datasets, and each wave of the epidemic development cycle would be insufficient to support a dataset suitable for LSTM. Second, neural networks involve a large number of parameters and highly complex models and, hence, are susceptible to overfitting, which can prevent them from achieving their true and expected advantages in prediction.
Overall, Our study differs from other papers in the following three ways. First, the research object of this paper focuses on the specific point of ICU healthcare resource demand prediction, aiming to improve the rate of critical care patient treatment. However, past research on public health emergencies has focused more on resource prediction , such as N95 masks, vaccines, and generalized medical supplies during the epidemic , to mitigate the impact of rapid transmission and high morbidity rates. This has led to less attention being paid to the reality of the surge in critically ill patients due to their high rates of severe illness and mortality.
Second, the idea of this paper is to further forecast resource needs based on the projected number of people with confirmed diagnoses, which is more applicable to healthcare organizations than most other papers that only predict the number of people involved. However, in terms of the methodology for projecting the number of people, this paper adopts a combined prediction method that combines regression algorithms and recurrent neural networks to propose a BILSTM-GASVR prediction model for the number of confirmed diagnoses. It capitalizes on both the suitability of SVR for small samples and non-linear prediction as well as the learning and memory abilities of BILSTM in processing time-series data. On the basis of the prediction model for the number of infected cases, by considering the characteristics of ICU healthcare resources, we constructed a demand forecasting model of emergency healthcare supplies. Past public health emergencies are more likely to use infectious disease models or a single prediction model in deep learning. some of the articles, although using a combination of prediction, but also more for the same method domain combination, such as CNN-LSTM, GRU-LSTM, etc., which are all recurrent neural networks.
Third, in terms of specific categorization of resources to be forecasted, considering the specificity of ICU medical resources, we introduce human resource prediction on the basis of previous studies focusing on material security, and classified ICU medical resources into three categories: ICU human resources, drugs and medical equipment. The purpose of this classification is to match the real-life prediction scenarios of public health emergencies and improve the demand forecasting performance for local ICU healthcare resources. Thus, it is easy for healthcare institutions to grasp the overall development of events, optimizing decision-making, and reducing the risk of healthcare systems collapsing during the outbreak stage.
In this section, we accomplish the following two tasks. Firstly, we introduce the idea of predicting the number of infected cases and show the principle of the relevant models. Secondly, based on the number of infected cases, ICU healthcare resources are divided into two categories (healthcare workers and healthcare supplies), and their respective demand forecasting models are constructed.
Prediction model for the number of infected cases
Gasvr model.
Support vector machine (SVM) is a machine-learning language for classification developed by Vapnik [ 29 ]. Suppose there are two categories of samples: H1 and H2. If hyperplane H is able to correctly classify the samples into these two categories and maximize the margin between the two categories, it is known as the optimal separating hyperplane (OSH). The sample vectors closest to the OSH in H1 and H2 are known as the support vectors. To apply SVM to prediction, it is essential to perform regression fitting. By introducing the \(\varepsilon\) -insensitive loss function, SVM can be converted to a support vector regression machine, where the role of the OSH is to minimize the error of all samples from this plane. SVR has a theoretical basis in statistical learning and relatively high learning performance, making it suitable for performing predictions in small-sample, non-linear, and multi-dimensional fields [ 30 , 31 ].
Assume the training sample set containing \(l\) training samples is given by \(\{({x}_{i},{y}_{i}),i=\mathrm{1,2},...,l\}\) , where \({x}_{i}=[{x}_{i}^{1},{x}_{i}^{2},...,{x}_{i}^{d}{]}^{\rm T}\) and \({y}_{i}\in R\) are the corresponding output values.
Let the regression function be \(f(x)=w\Phi (x)+b\) , where \(\phi (x)\) is the non-linear mapping function. The linear \(\varepsilon\) -insensitive loss function is defined as shown in formula ( 1 ).
Among the rest, \(f(x)\) is the predicted value returned by the regression function, and \(y\) is the corresponding real value. If the error between \(f(x)\) and \(y\) is ≤ \(\varepsilon\) , the loss is 0; otherwise, the loss is \(\left|y-f(x)\right|-\varepsilon\) .
The slack variables \({\xi }_{i}\) and \({\xi }_{i}^{*}\) are introduced, and \(w\) , \(b\) are solved using the following equation as shown in formula ( 2 ).
Among the rest, \(C\) is the penalty factor, with larger values indicating a greater penalty for errors > \(\varepsilon\) ; \(\varepsilon\) is defined as the error requirement, with smaller values indicating a smaller error of the regression function.
The Lagrange function is introduced to solve the above function and transformed into the dual form to give the formula ( 3 ).
Among the rest, \(K({x}_{i},{x}_{j})=\Phi ({x}_{i})\Phi ({x}_{j})\) is the kernel function. The kernel function determines the structure of high-dimensional feature space and the complexity of the final solution. The Gaussian kernel is selected for this study with the function \(K({x}_{i},{x}_{j})=\mathit{exp}(-\frac{\Vert {x}_{i}-{x}_{j}\Vert }{2{\sigma }^{2}})\) .
Let the optimal solution be \(a=[{a}_{1},{a}_{2},...,{a}_{l}]\) and \({a}^{*}=[{a}_{1}^{*},{a}_{2}^{*},...,{a}_{l}]\) to give the formula ( 4 ) and formula ( 5 ).
Among the rest, \({N}_{nsv}\) is the number of support vectors.
In sum, the regression function is as shown in formula ( 6 ).
when some of the parameters are not 0, the corresponding samples are the support vectors in the problem. This is the principle of SVR. The values of the three unknown parameters (penalty factor C, ε -insensitive loss function, and kernel function coefficient \(\sigma )\) , can directly impact the model effect. The penalty factor C affects the degree of function fitting through the selection of outliers in the sample by the function. Thus, excessively large values lead to better fit but poorer generalization, and vice versa. The ε value in the ε-insensitive loss function determines the accuracy of the model by affecting the width of support vector selection. Thus, excessively large values lead to lower accuracy that does not meet the requirements and excessively small values are overly complex and increase the difficulty. The kernel function coefficient \(\sigma\) determines the distribution and range of the training sample by controlling the size of inner product scaling in high-dimensional space, which can affect overfitting.
Therefore, we introduce other algorithms for optimization of the three parameters in SVR. Currently the commonly used algorithms are 32and some heuristic algorithms. Although the grid search method is able to find the highest classification accuracy, which is the global optimal solution. However, sometimes it can be time-consuming to find the optimal parameters for larger scales. If a heuristic algorithm is used, we could find the global optimal solution without having to trace over all the parameter points in the grid. And GA is one of the most commonly used heuristic algorithms, compared to other heuristic algorithms, it has the advantages of strong global search, generalizability, and broader blending with other algorithms.
Given these factors, we employ a GA to encode and optimize the relevant parameters of the model. The inputs are the experimental training dataset, the Gaussian kernel function expression, the maximum number of generations taken by the GA, the accuracy range of the optimized parameters, the GA population size, the fitness function, the probability of crossover, and the probability of mutation. The outputs are the optimal penalty factor C, ε-insensitive loss function parameter \(\varepsilon ,\) and optimal Gaussian kernel parameter \(\sigma\) of SVR, thus achieving the optimization of SVR. The basic steps involved in GA optimization are described in detail below, and the model prediction process is shown in Fig. 1 .
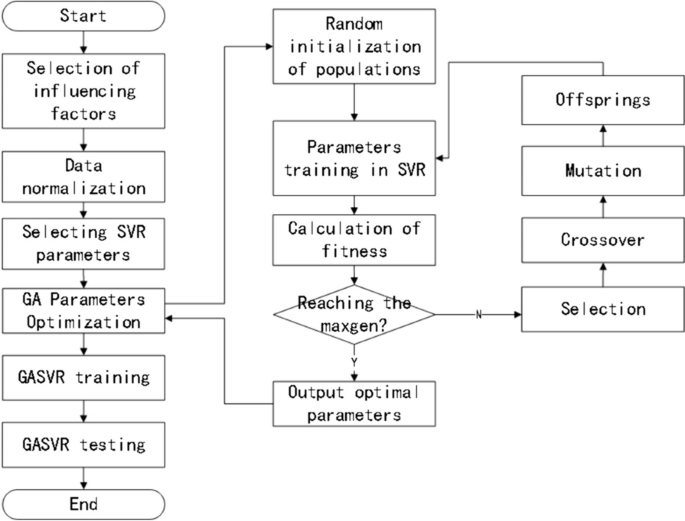
Prediction process of the GASVR model
Population initialization
The three parameters are encoded using binary arrays composed of 0–1 bit-strings. Each parameter consisted of six bits, and the initial population is randomly generated. The population size is set at 60, and the number of iterations is 200.
Fitness calculation
In the same dataset, the K-fold cross-validation technique is used to test each individual in the population, with K = 5. K-fold cross validation effectively avoids the occurrence of model over-learning and under-learning. For the judgment of the individual, this paper evaluates it in terms of fitness calculations. Therefore, combining the two enables the effective optimization of the model’s selected parameters and improves the accuracy of regression prediction.
Fitness is calculated using the mean error method, with smaller mean errors indicating better fitness. The fitness function is shown in formula ( 7 ) [ 32 ].
The individual’s genotype is decoded and mapped to the corresponding parameter value, which is substituted into the SVR model for training. The parameter optimization range is 0.01 ≤ C ≤ 100, 0.1 ≤ \(\sigma\) ≤ 20, and 0.001 ≤ ε ≤ 1.
Selection: The selection operator is performed using the roulette wheel method.
Crossover: The multi-point crossover operator, in which two chromosomes are selected and multiple crossover points are randomly chosen for swapping, is employed. The crossover probability is set at 0.9.
Mutation: The inversion mutation operator, in which two points are randomly selected and the gene values between them are reinserted to the original position in reverse order, is employed. The mutation probability is set at 0.09.
Decoding: The bit strings are converted to parameter sets.
The parameter settings of the GASVR model built in this paper are shown in Table 1 .
BILSTM model
The LSTM model is a special recurrent neural network algorithm that can remember the long-term dependencies of data series and has an excellent capacity for self-learning and non-linear fitting. LSTM automatically connects hidden layers across time points, such that the output of one time point can arbitrarily enter the output terminal or the hidden layer of the next time point. Therefore, it is suitable for the sample prediction of time-series data and can predict future data based on stored data. Details of the model are shown in Fig. 2 .
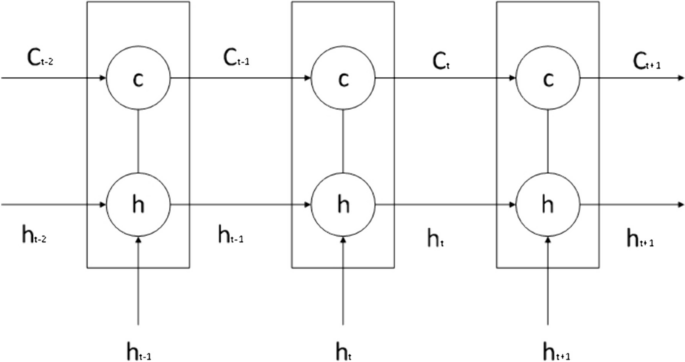
Schematic diagram of the LSTM model
LSTM consists of a forget gate, an input gate, and an output gate.
The forget gate combines the previous and current time steps to give the output of the sigmoid activation function. Its role is to screen the information from the previous state and identify useful information that truly impacts the subsequent time step. The equation for the forget gate is shown in formula ( 8 ).
Among the number, \(W_{f}\) is the weight of the forget gate, \({b}_{f}\) is the bias, \(\sigma\) is the sigmoid activation function, \({f}_{t}\) is the output of the sigmoid activation function, \(t-1\) is the previous time step, \(t\) is the current time step, and \({x}_{t}\) is the input time-series data at time step \(t\) .
The input gate is composed of the output of the sigmoid and tanh activation functions, and its role is to control the ratio of input information entering the information of a given time step. The equation for the input gate is shown in formula ( 9 ).
Among the number, \({W}_{i}\) is the output weight of the input gate, \({i}_{t}\) is the output of the sigmoid activation function, \({b}_{i}\) and \({b}_{C}\) are the biases of the input gate, and \({W}_{C}\) is the output of the tanh activation function.
The role of the output gate is to control the amount of information output at the current state, and its equation is shown in formula ( 10 ).
Among the number, \({W}_{o}\) is the weight of \({o}_{t}\) , and \({b}_{o}\) is the bias of the output gate.
The values of the above activation functions \(\sigma\) and tanh are generally shown in formulas ( 11 ) and ( 12 ).
\({C}_{t}\) is the data state of the current time step, and its value is determined by the input information of the current state and the information of the previous state. It is shown in formula ( 13 ).
Among the number, \(\widetilde{{C}_{t}}=\mathit{tan}h({W}_{c}[{h}_{t-1},{x}_{t}]+{b}_{c})\) .
\({h}_{t}\) is the state information of the hidden layer at the current time step, \({h}_{t}={o}_{t}\times \mathit{tan}h({c}_{t})\) .Each time step \({T}_{n}\) has a corresponding state \({C}_{t}\) . By undergoing the training process, the model can learn how to modify state \({C}_{t}\) through the forget, output, and input gates. Therefore, this state is consistently passed on, implying that important distant information will neither be forgotten nor significantly affected by unimportant information.
The above describes the principle of LSTM, which involves forward processing when applied. BILSTM consists of two LSTM networks, one of which processes the input sequence in the forward direction (i.e., the original order), while the other inputs the time series in the backward direction into the LSTM model. After processing both LSTM networks, the outputs are combined, which eventually gives the output results of the BILSTM model. Details of the model are presented in Fig. 3 .
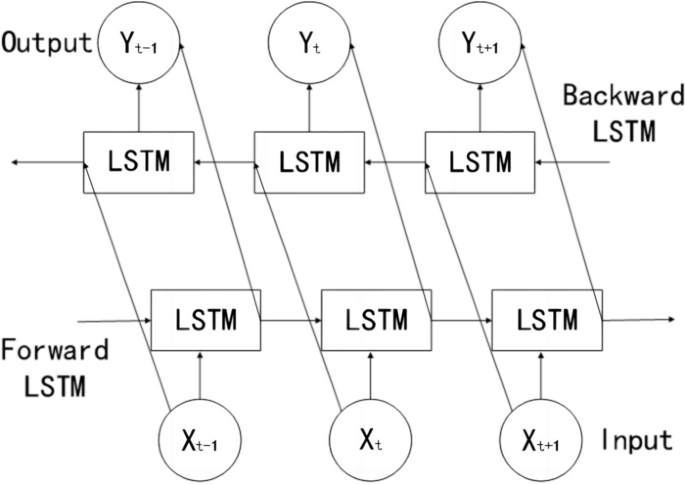
Schematic diagram of the BILSTM model
Compared to LSTM, BILSTM can achieve bidirectional information extraction of the time-series and connect the two LSTM layers onto the same output layer. Therefore, in theory, its predictive performance should be superior to that of LSTM. In BILSTM, the equations of the forward hidden layer( \(\overrightarrow{{h}_{t}}\) ) , backward hidden layer( \(\overleftarrow{{h}_{t}}\) ) , and output layer( \({o}_{t}\) ) are shown in formulas ( 14 ) , ( 15 ) and ( 16 ).
The parameter settings of the BILSTM model built in this paper are shown in Table 2 .
Informer model
The Informer model follows the compiler-interpreter architecture in the Transformer model, and based on this, structural optimizations have been made to reduce the computational time complexity of the algorithm and to optimize the output form of the interpreter. The two optimization methods are described in detail next.
With large amounts of input data, neural network models can have difficulty capturing long-term interdependencies in sequences, which can produce gradient explosions or gradient vanishing and affect the model's prediction accuracy. Informer model solves the existential gradient problem by using a ProbSparse Self-attention mechanism to make more efficient than conventional self-attention.
The value of Transformer self-attention is shown in formula ( 17 ).
Among them, \(Q\in {R}^{{L}_{Q}\times d}\) is the query matrix, \(K\in {R}^{{L}_{K}\times d}\) is the key matrix, and \(V\in {R}^{{L}_{V}\times d}\) is the value matrix, which are obtained by multiplying the input matrix X with the corresponding weight matrices \({W}^{Q}\) , \({W}^{K}\) , \({W}^{V}\) respectively, and d is the dimensionality of Q, K, and V. Let \({q}_{i}\) , \({k}_{i}\) , \(v_{i}\) represent the ith row in the Q, K, V matrices respectively, then the ith attention coefficient is shown in formula ( 18 ) as follows.
Therein, \(p({k}_{j}|{q}_{i})\) denotes the traditional Transformer's probability distribution formula, and \(k({q}_{i},{K}_{l})\) denotes the asymmetric exponential sum function. Firstly, q=1 is assumed, which implies that the value of each moment is equally important; secondly, the difference between the observed distribution and the assumed one is evaluated by the KL scatter, if the value of KL is bigger, the bigger the difference with the assumed distribution, which represents the more important this moment is. Then through inequality \(ln{L}_{k}\le M({q}_{i},K)\le {\mathit{max}}_{j}\left\{\frac{{q}_{i}{k}_{j}^{\rm T}}{\sqrt{d}}\right\}-\frac{1}{{L}_{k}}{\sum }_{j=1}^{{L}_{k}}\left\{\frac{{q}_{i}{k}_{j}^{\rm T}}{\sqrt{d}}\right\}+ln{L}_{k}\) , \(M({q}_{i},K)\) is transformed into \(\overline{M}({q}_{i},K)\) . According to the above steps, the ith sparsity evaluation formula is obtained as shown in formula ( 19 ) [ 33 ].
One of them, \(M({q}_{i},K)\) denotes the ith sparsity measure; \(\overline{M}({q}_{i},K)\) denotes the ith approximate sparsity measure; \({L}_{k}\) is the length of query vector. \(TOP-u\) quantities of \(\overline{M}\) are selected to form \(\overline{Q}\) , \(\overline{Q}\) is the first u sparse matrices, and the final sparse self-attention is shown in Formula ( 20 ). At this point, the time complexity is still \(O({n}^{2})\) , and to solve this problem, only l moments of M2 are computed to reduce the time complexity to \(O(L\cdot \mathit{ln}(L))\) .
Informer uses a generative decoder to obtain long sequence outputs.Informer uses the standard decoder architecture shown in Fig. 4 , in long time prediction, the input given to the decoder is shown in formula ( 21 ).
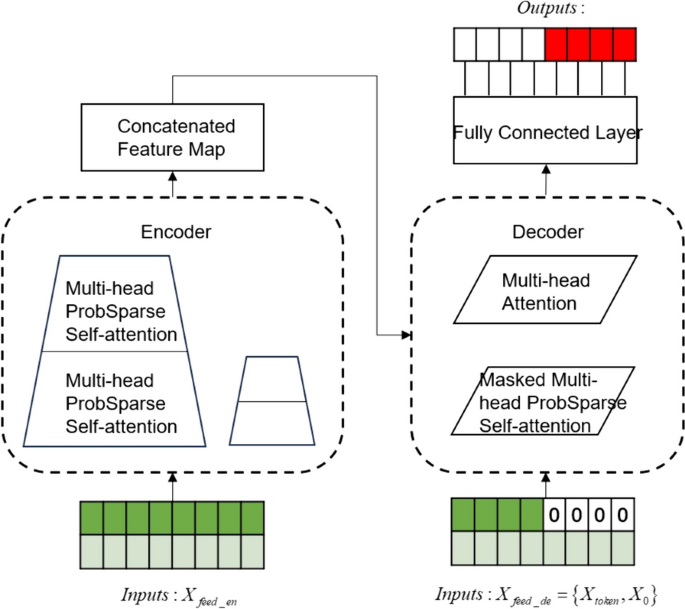
Informer uses a generative decoder to obtain long sequence outputs
Therein, \({X}_{de}^{t}\) denotes the input to the decoder; \({X}_{token}^{t}\in {R}^{({L}_{token}+{L}_{y})\times {d}_{\mathit{mod}el}}\) is the dimension of the encoder output, which is the starting token without using all the output dimensions; \({X}_{0}^{t}\in {R}^{({L}_{token}+{L}_{y})\times {d}_{\mathit{mod}el}}\) is the dimension of the target sequence, which is uniformly set to 0; and finally the splicing input is performed to the encoder for prediction.
The parameter settings of Informer model created in this paper are shown in Table 3 .
BILSTM-GASVR combined prediction model
SVR has demonstrated good performance in solving problems like finite samples and non-linearity. Compared to deep learning methods, it offers faster predictions and smaller empirical risks. BILSTM has the capacity for long-term memory, can effectively identify data periodicity and trends, and is suitable for the processing of time-series data. Hence, it can be used to identify the effect of time-series on the number of confirmed cases. Given the advantages of these two methods in different scenarios, we combined them to perform predictions using GASVR, followed by error repair using BILSTM. The basic steps for prediction based on the BILSTM-GASVR model are as follows:
Normalization is performed on the initial data.
The GASVR model is applied to perform training and parameter optimization of the data to obtain the predicted value \(\widehat{{y}_{i}}\) .
After outputting the predicted value of GASVR, the residual sequence between the predicted value and real data is extracted to obtain the error \({\gamma }_{i}\) (i.e., \({\gamma }_{i}={y}_{i}-\widehat{{y}_{i}}\) ).
The BILSTM model is applied to perform training of the error to improve prediction accuracy. The BILSTM model in this paper is a multiple input single output model. Its inputs are the true and predicted error values \({\gamma }_{i}\) and its output is the new error value \(\widehat{{\gamma }_{i}}\) predicted by BILSTM.
The final predicted value is the sum of the GASVR predicted value and the BILSTM residual predicted value (i.e., \({Y}_{i}=\widehat{{y}_{i}}+\widehat{{\gamma }_{i}}\) ).
The parameter settings of the BILSTM-GASVR model built in this paper are shown in Table 4 .
Model testing criteria
To test the effect of the model, the prediction results of the BILSTM-GASVR model are compared to those of GASVR, LSTM, BILSTM and Informer. The prediction error is mainly quantified using three indicators: mean squared error (MSE), root mean squared error (RMSE), and correlation coefficient ( \(R^{2}\) ). Their respective equations are shown in formulas ( 22 ), ( 23 ) and ( 24 ).
Demand forecasting model of ICU healthcare resources
ICU healthcare resources can be divided into human and material resources. Human resources refer specifically to the professional healthcare workers in the ICU. Material resources, which are combined with the actual consumption of medical supplies, can be divided into consumables and non-consumables. Consumables refer to the commonly used drugs in the ICU, which include drugs for treating cardiac insufficiency, vasodilators, anti-shock vasoactive drugs, analgesics, sedatives, muscle relaxants, anti-asthmatic drugs, and anticholinergics. Given that public health emergencies have a relatively high probability of affecting the respiratory system, we compiled a list of commonly used drugs for respiratory diseases in the ICU (Table 5 ).
Non-consumables refer to therapeutic medical equipment, including electrocardiogram machines, blood gas analyzers, electrolyte analyzers, bedside diagnostic ultrasound machines, central infusion workstations, non-invasive ventilators, invasive ventilators, airway clearance devices, defibrillators, monitoring devices, cardiopulmonary resuscitation devices, and bedside hemofiltration devices.
The demand forecasting model of ICU healthcare resources constructed in this study, as well as its relevant parameters and definitions, are described below. \({R}_{ij}^{n}\) is the forecasted demand for the \(i\) th category of resources on the \(n\) th day in region \(j\) . \({Y}_{j}^{n}\) is the predicted number of current confirmed cases on the \(n\) th day in region \(j\) . \({M}_{j}^{n}\) is the number of ICU healthcare workers on the \(n\) th day in region \(j\) , which is given by the following formula: number of healthcare workers the previous day + number of new recruits − reduction in number the previous day, where the reduction in number refers to the number of healthcare workers who are unable to work due to infection or overwork. In general, the number of ICU healthcare workers should not exceed 5% of the number of current confirmed cases (i.e., it takes the value range [0, \(Y_{j}^{n}\) ×5%]). \(U_{i}\) is the maximum working hours or duration of action of the \(i\) th resource category within one day. \({A}_{j}\) is the number of resources in the \(i\) th category allocated to patients (i.e., how many units of resources in the \(i\) th category is needed for a patient who need the \(i\) th unit of the given resource). \({\varphi }_{i}\) is the demand conversion coefficient (i.e., the proportion of the current number of confirmed cases who need to use the \(i\) th resource category). \({C}_{ij}^{n}\) is the available quantity of material resources of the \(i\) th category on the \(n\) th day in region \(j\) . At the start, this quantity is the initial reserve, and once the initial reserve is exhausted, it is the surplus from the previous day. The formula for this parameter is given as follows: available quantity from the previous day + replenishment on the previous day − quantity consumed on the previous day, where if \({C}_{ij}^{n}\) is a negative number, it indicates the amount of shortage for the given category of resources on the previous day.
In summary, the demand forecast for emergency medical supplies constructed in this study is shown in formula ( 25 ).
The number of confirmed cases based on data-driven prediction is introduced into the demand forecasting model for ICU resources to forecast the demand for the various categories of resources. In addition to the number of current confirmed cases, the main variables of the first demand forecasting model for human resources are the available quantity and maximum working hours. The main variable of the second demand forecasting model for consumable resources is the number of units consumed by the available quantity. The main variable of the third model for non-consumable resources is the allocated quantity. These three resource types can be predicted using the demand forecasting model constructed in this study.
Prediction of the number of current infected cases
The COVID-19 situation in Shanghai is selected for our experiment. A total of 978 entries of epidemic-related data in Shanghai between January 20, 2020, and September 24, 2022, are collected from the epidemic reporting platform. This dataset is distributed over a large range and belongs to a right-skewed leptokurtic distribution. The specific statistical description of data is shown in Table 6 . Part of the data is shown in Table 7 .
And we divided the data training set and test set in an approximate 8:2 ratio, namely, 798 days for training (January 20, 2020 to March 27, 2022) and 180 days for prediction (March 28, 2022 to September 24, 2022).
Due to the large difference in order of magnitude between the various input features, directly implementing training and model construction would lead to suboptimal model performance. Such effects are usually eliminated through normalization. In terms of interval selection, [0, 1] reflects the probability distribution of the sample, whereas [-1, 1] mostly reflects the state distribution or coordinate distribution of the sample. Therefore, [-1, 1] is selected for the normalization interval in this study, and the processing method is shown in formula ( 26 ).
Among the rest, \(X\) is the input sample, \({X}_{min}\) and \({X}_{max}\) are the minimum and maximum values of the input sample, and \({X}_{new}\) is the input feature after normalization.
In addition, we divide the data normalization into two parts, considering that the amount of data in the training set is much more than the test set in the real operating environment. In the first step, we normalize the training set data directly according to the above formula; in the second step, we normalize the test data set using the maximum and minimum values of the training data set.
The values of the preprocessed data are inserted into the GASVR, LSTM, Informer, BILSTM models and the BILSTM-GASVR model is constructed. Figures 5 , 6 , 7 , 8 and 9 show the prediction results. From Figs. 5 , 6 , and 7 , it can be seen that in terms of data accuracy, GASVR more closely matches the real number of infected people relative to BILSTM and LSTM. Especially in the most serious period of the epidemic in Shanghai (April 17, 2022 to April 30, 2022), the advantage of the accuracy of the predicted data of GASVR is even more obvious, which is due to the characteristics of GASVR for small samples and nonlinear prediction. However, in the overall trend of the epidemic, BILSTM and LSTM, which have the ability to learn and memorize to process time series data, are superior. It is clearly seen that in April 1, 2022-April 7, 2022 and May 10, 2022-May 15, 2022, there is a sudden and substantial increase in GASVR in these two time phases, and a sudden and substantial decrease in April 10, 2022-April 14, 2022. These errors also emphasize the stability of BILSTM and LSTM, which are more closely matched to the real epidemic development situation in the whole process of prediction, and the difference between BILSTM and LSTM prediction is that the former predicts data more accurately than the latter, which is focused on the early stage of prediction as well as the peak period of the epidemic. Informer is currently an advanced time series forecasting method. From Fig. 8 , it can be seen that the prediction data accuracy and the overall trend of the epidemic are better than the single prediction models of GASVR, LSTM and BILSTM. However, Informer is more suitable for long time series and more complex and large prediction problems, so the total sample size of less than one thousand cases is not in the comfort zone of Informer model. Figure 9 shows that the BILSTM-GASVR model constructed in this paper is more suitable for this smaller scale prediction problem, with the best prediction results, closest to the actual parameter (number of current confirmed cases), demonstrating small sample and time series advantages. In Short, the prediction effect of models is ranked as follows: BILSTM-GASVR> Informer> GASVR> BILSTM> LSTM.
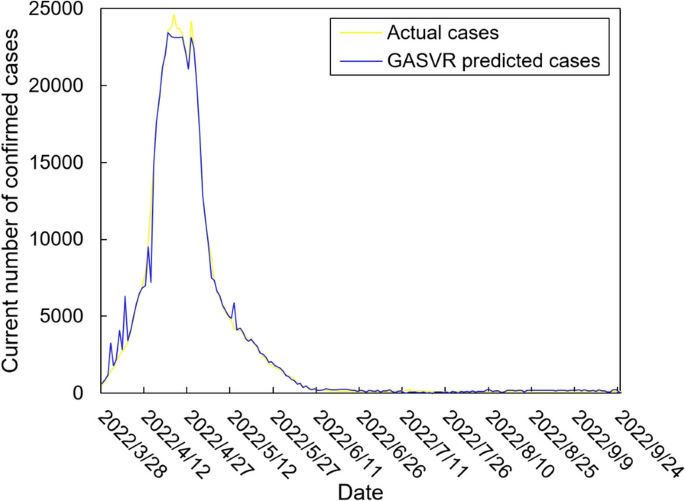
The prediction result of the GASVR model
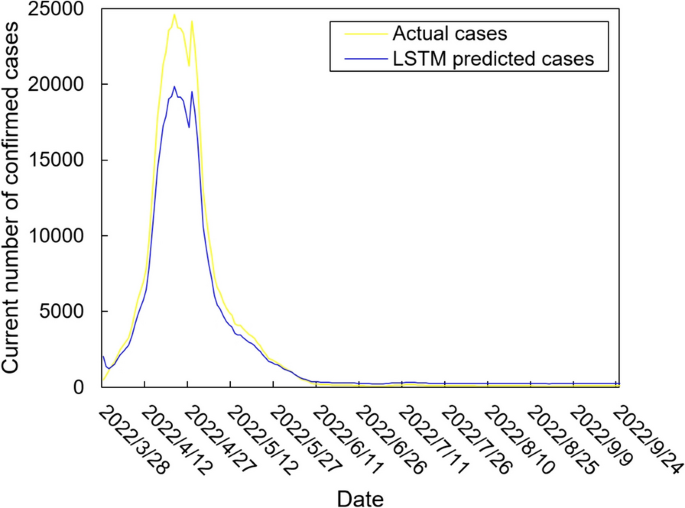
The prediction result of the LSTM model
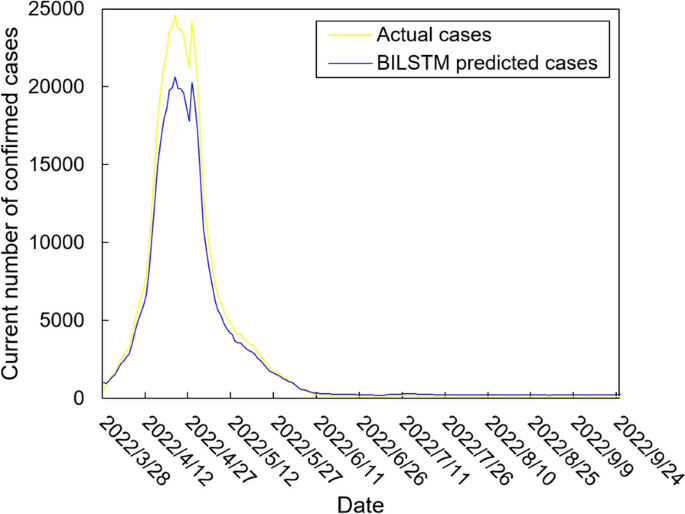
The prediction result of the BILSTM model
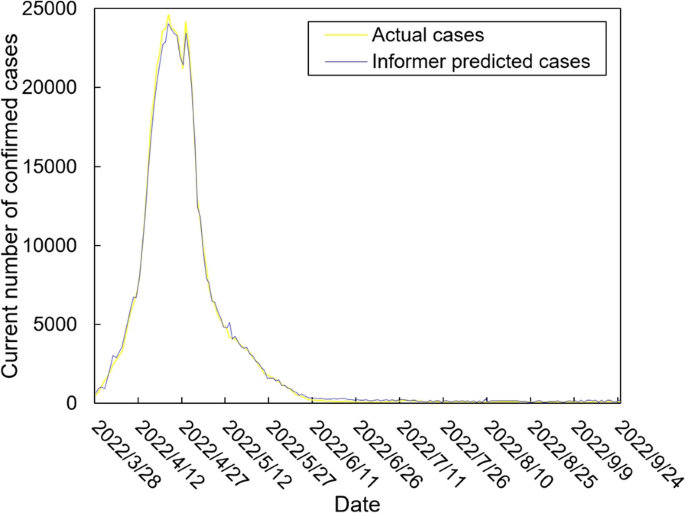
The prediction result of the Informer model
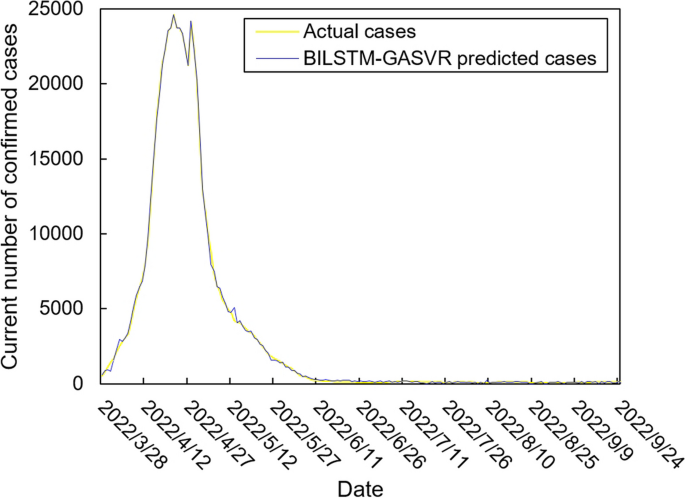
The prediction result of the BILSTM-GASVR model
The values of the three indicators (MSE, RMSE, and correlation coefficient \({R}^{2}\) ) for the five models are shown in Table 8 . MSE squares the error so that the larger the model error, the larger the value, which help capture the model's prediction error more sensitively. RMSE is MSE with a root sign added to it, which allows for a more intuitive representation of the order of magnitude difference from the true value. \({R}^{2}\) is a statistical indicator used to assess the overall goodness of fit of the model, which reflects the overall consistency of the predicted trend and does not specifically reflect the degree of data. The results in the Table 8 are consistent with the prediction results in the figure above, while the ranking of MSE, RMSE, and \({R}^{2}\) are also the same (i.e., BILSTM-GASVR> Informer> GASVR> BILSTM> LSTM).
In addition, we analyze the five model prediction data using significance tests as a way of demonstrating whether the model used is truly superior to the other baseline models. The test dataset with kurtosis higher than 4 does not belong to the approximate normal distribution, so parametric tests are not used in this paper. Given that the datasets predicted by each of the five models are continuous and independent datasets, this paper uses the Kruskal-Wallis test, which is a nonparametric test. The test steps are as follows.
Determine hypotheses (H0, H1) and significance level ( \(\alpha\) ).
For each data set, all its sample data are combined and ranked from smallest to largest. Then find the number of data items ( \({n}_{i}\) ), rank sum ( \({R}_{i}\) ) and mean rank of each group of data respectively.
Based on the rank sum, the test statistic (H) is calculated for each data set in the Kruskal-Wallis test. The specific calculation is shown in formula ( 27 ).
According to the test statistic and degrees of freedom, find the corresponding p-value in the Kruskal-Wallis distribution table. Based on the P-value, determine whether the original hypothesis is valid.
In the significance test, we set the significance setting original hypothesis (H0) as there is no significant difference between the five data sets obtained from the five predictive models. We set the alternative hypothesis (H1) as there is a significant difference between the five data sets obtained from the five predictive models. At the same time, we choose the most commonly used significance level taken in the significance test, namely 0.05. In this paper, multiple comparisons and two-by-two comparisons of the five data sets obtained from the five predictive models are performed through the SPSS software. The results of the test show that in the multiple comparison session, P=0.001<0.05, so H0 is rejected, which means that the difference between the five groups of data is significant. In the two-by-two comparison session, BILSTM-GASVR is less than 0.05 from the other four prediction models. The specific order of differences is Informer < GASVR < BILSTM < LSTM, which means that the BILSTM-GASVR prediction model does get a statistically significant difference between the dataset and the other models.
In summary, combined prediction using the BILSTM-GASVR model is superior to the other four single models in various aspects in the case study analysis of Shanghai epidemic with a sample size of 978.
Demand forecasting of ICU healthcare resources
Combined with the predicted number of current infected cases, representatives are selected from the three categories of resources for forecasting. The demand for nurses is selected as the representative for the first category of resources.
In view of the fact that there are currently no specific medications that are especially effective for this public health emergency, many ICU treatment measures involved helping patients survive as their own immune systems eliminated the virus. This involved, for example, administering antibiotics when patients developed a secondary bacterial infection. glucocorticoids are used to temporarily suppress the immune system when their immune system attacked and damaged lung tissues causing patients to have difficulty breathing. extracorporeal membrane oxygenation (ECMO) is used for performing cardiopulmonary resuscitation when patients are suffering from cardiac arrest. In this study, we take dexamethasone injection (5 mg), a typical glucocorticoid drug, as the second category of ICU resources (i.e., drugs); and invasive ventilators as the third category of ICU resources (i.e., medical equipment).
During the actual epidemic in Shanghai, the municipal government organized nine critical care teams, which are stationed in eight municipally designated hospitals and are dedicated to the treatment of critically ill patients. In this study, the ICU nurses, dexamethasone injections, and invasive ventilators in Shanghai are selected as the prediction targets and introduced into their respective demand forecasting models. Forecasting of ICU healthcare resources is then performed for the period from March 28, 2022, to April 28, 2022, as an example. Part of the parameter settings for the three types of resources are shown in Tables 9 , 10 , and 11 , respectively.
Table 12 shows the forecasting results of the demand for ICU nurses, dexamethasone injections, and invasive ventilators during the epidemic wave in Shanghai between March 28, 2022, and April 28, 2022.
For the first category (i.e., ICU nurses), human resource support is only needed near the peak period, but the supply could not be replenished immediately. In the early stages, Shanghai could only rely on the nurses’ perseverance, alleviating the shortage of human resources by reducing the number of shifts and increasing working hours. This situation persisted until about April 10 and is only resolved when nurses from other provinces and regions successively arrived in Shanghai.
The second category of ICU resources is drugs, which are rapidly consumed. The pre-event reserve of 30,000 dexamethasone injections could only be maintained for a short period and is fully consumed during the outbreak. Furthermore, daily replenishment is still needed, even when the epidemic has passed its peak and begun its decline.
The third category is invasive ventilators, which are non-consumables. Thus, the reserve lasted for a relatively long period of time in the early stages and did not require replenishment after its maximum usage during the peak period.
Demand forecasting models are constructed based on the classification of healthcare resources according to their respective features. We choose ICU nurses, dexamethasone injections, and invasive ventilators as examples, and then forecast demand for the epidemic wave in Shanghai between March 28, 2022, and April 28, 2022. The main conclusions are as follows:
A long period of time is needed to train ICU healthcare workers who can independently be on duty, taking at least one year from graduation to entering the hospital, in addition to their requiring continuous learning, regular theoretical training, and the accumulation of clinical experience during this process. Therefore, for the first category of ICU healthcare resources, in the long term, healthcare institutions should place a greater emphasis on their talent reserves. Using China as an example, according to the third ICU census, the ratio of the number of ICU physicians to the number of beds is 0.62:1 and the ratio of the number of nurses to the number of beds is 1.96:1, which are far lower than those stipulated by China itself and those of developed countries. Therefore, a fundamental solution is to undertake proactive and systematic planning and construction to ensure the more effective deployment of human resources in the event of a severe outbreak. In the short term, healthcare institutions should focus on the emergency expansion capacity of their human resources. In case there are healthcare worker shortages during emergencies, the situation can be alleviated by summoning retired workers back to work and asking senior medical students from various universities to help in the hospitals to prevent the passive scenario of severely compressing the rest time of existing staff or waiting for external aid. However, it is worth noting that to ensure the effectiveness of such a strategy of using retired healthcare workers or senior students of university medical faculties, it is necessary for healthcare organizations to provide them with regular training in the norm, such as organizing 2-3 drills a year, to ensure the professionalism and proficiency of healthcare workers who are temporarily and suddenly put on the job. At the same time, it is also necessary to fully mobilize the will of individuals. Medical institutions can provide certain subsidies to retired health-care workers and award them with honorable titles. For senior university medical students, volunteer certificates are issued and priority is given to their internships, so that health-care workers can be motivated to self-realization through spiritual and material rewards.
Regarding the second category of ICU resources (i.e., drugs), healthcare institutions perform the subdivision of drug types and carry out dynamic physical preparations based on 15–20% of the service recipient population for clinically essential drugs. This will enable a combination of good preparedness during normal times and emergency situations. In addition, in-depth collaboration with corporations is needed to fully capitalize on their production capacity reserves. This helps medical institutions to be able to scientifically and rationally optimize the structure and quantity of their drug stockpiles to prevent themselves from being over-stressed. Yet the lower demand for medicines at the end of the epidemic led to the problem of excess inventory of enterprises at a certain point in time must be taken into account. So, the medical institutions should sign a strategic agreement on stockpiling with enterprises, take the initiative to bear the guaranteed acquisition measures, and consider the production costs of the cooperative enterprises. These measures are used to truly safeguard the enthusiasm of the cooperative enterprises to invest in the production capacity.
Regarding the third category of ICU resources (i.e., medical equipment), large-scale medical equipment cannot be rapidly mass-produced due to limitations in the capacity for emergency production and conversion of materials. In addition, the bulk procurement of high-end medical equipment is also relatively difficult in the short term. Therefore, it is more feasible for healthcare institutions to have physical reserves of medical equipment, such as invasive ventilators. However, the investment costs of medical equipment are relatively high. Ventilators, for example, cost up to USD $50,000, and subsequent maintenance costs are also relatively high. After all, according to the depreciable life of specialized hospital equipment, the ventilator, as a surgical emergency equipment, is depreciated over five years. And its depreciation rate is calculated at 20% annually for the first five years, which means a monthly depreciation of $835. Thus, the excessively low utilization rate of such equipment will also impact the hospital. Healthcare institutions should, therefore, conduct further investigations on the number of beds and the reserves of ancillary large-scale medical equipment to find a balance between capital investment and patient needs.
The limitations of this paper are reflected in the following three points. Firstly, in the prediction of the number of infections, the specific research object in this paper is COVID-19, and other public health events such as SARS, H1N1, and Ebola are not comparatively analyzed. The main reason for this is the issue of data accessibility, and it is easier for us to analyze events that have occurred in recent years. In addition, using the Shanghai epidemic as a specific case may be more representative of the epidemic situation in an international metropolis with high population density and mobility. Hence, it has certain regional limitations, and subsequent studies should expand the scope of the case study to reflect the characteristics of epidemic transmission in different types of urban areas and enhance the generalizability.
Secondly, the main emphasis of this study is on forecasting the demand for ICU healthcare resources across the entire region of the epidemic, with a greater focus on patient demand during public emergencies. Our aims are to help all local healthcare institutions more accurately identify changes in ICU healthcare resource demand during this local epidemic wave, gain a more accurate understanding of the treatment demands of critically ill patients, and carry out comprehensive, scientifically based decision-making. Therefore, future studies can examine individual healthcare institutions instead and incorporate the actual conditions of individual units to construct multi-objective models. In this way, medical institutions can further grasp the relationship between different resource inputs and the recovery rate of critically ill patients, and achieve the balance between economic and social benefits.
Finally, for the BILSTM-GASVR prediction method, in addition to the number of confirmed diagnoses predicted for an outbreak in a given region, other potential applications beyond this type of medium-sized dataset still require further experimentation. For example, whether the method is suitable for procurement planning of a certain supply in production management, forecasting of goods sales volume in marketing management, and other long-period, large-scale and other situations.
Within the context of major public health events, the fluctuations and uncertainties in the demand for ICU resources can lead to large errors between the healthcare supply and actual demand. Therefore, this study focuses on the question of forecasting the demand for ICU healthcare resources. Based on the number of current confirmed cases, we construct the BILSTM-GASVR model for predicting the number of patients. By comparing the three indicators (MSE, MAPE, and correlation coefficient \(R^{2}\) ) and the results of the BILSTM, LSTM, and GASVR models, we demonstrate that our model have a higher accuracy. Our findings can improve the timeliness and accuracy of predicting ICU healthcare resources and enhance the dynamics of demand forecasting. Hence, this study may serve as a reference for the scientific deployment of ICU resources in healthcare institutions during major public events.
Given the difficulty in data acquisition, only the Shanghai epidemic dataset is selected in this paper, which is one of the limitations mentioned in Part 4. Although the current experimental cases of papers in the same field do not fully conform to this paper, the results of the study cannot be directly compared. However, after studying the relevant reviews and the results of the latest papers, we realize that there is consistency in the prediction ideas and prediction methods [ 34 , 35 ]. Therefore, we summarize the similarities and differences between the results of the study and other research papers in epidemic forecasting as shown below.
Similarities: on the one hand, we all characterize trends in the spread of the epidemic and predict the number of infections over 14 days. On the other hand, we all select the current mainstream predictive models as the basis and combine or improve them. Moreover, we all use the same evaluation method (comparison of metrics such as MSE and realistic values) to evaluate the improvements against other popular predictive models.
Differences: on the one hand, other papers focus more on predictions at the point of the number of patients, such as hospitalization rate, number of infections, etc. This paper extends the prediction from the number of patients to the specific healthcare resources. This paper extends the prediction from the number of patients to specific healthcare resources. We have divided the medical resources and summarized the demand regularities of the three types of information in the epidemic, which provides the basis for decision-making on epidemic prevention to the government or medical institutions. On the other hand, in addition to the two assessment methods mentioned in the same point, this paper assesses the performance of the prediction methods with the help of significance tests, which is a statistical approach to data. This can make the practicality of the forecasting methodology more convincing.
Availability of data and materials
The datasets used and/or analysed during the current study available from the corresponding author on reasonable request.
Yuan, R., Yang, Y., Wang, X., Duo, J. & Li, J. Study on the forecasting and allocation of emergency medical material needs in the event of a major infectious disease outbreak. J Saf Environ. https://doi.org/10.13637/j.issn.1009-6094.2023.2448 .
Total epidemic data (global). 2024. Retrieved March 30, 2024, from: https://www.sy72.com/world/ .
Sui, K., Wang, Y., Wang, S., Chen, C., & Sun, X. A multiple regression analysis-based method for forecasting the demand of emergency materials for power grids. Electron Technol Softw Eng. 2016; (23), 195-197.
Li K, Liu L, Zhai J, Khoshgoftaar TM, Li T. The improved grey model based on particle swarm optimization algorithm for time series prediction. Eng Appl Artif Intell. 2016;55:285–91. https://doi.org/10.1016/j.engappai.2016.07.005 .
Article Google Scholar
Fan R, Wang Y, Luo M. SEIR-based COVID-19 transmission model and inflection point prediction analysis. J Univ Electron Sci Technol China. 2020;49(3):369–74.
Google Scholar
Neves AGM, Guerrero G. Predicting the evolution of the COVID-19 epidemic with the A-SIR model: Lombardy, Italy and São Paulo state Brazil. Physica D. 2020;413:132693. https://doi.org/10.1016/j.physd.2020.132693 .
Article PubMed PubMed Central Google Scholar
Li R, Pei S, Chen B, Song Y, Zhang T, Wan Y, Shaman J. Substantial undocumented infection facilitates the rapid dissemination of novel coronavirus (SARS-CoV-2). Science. 2020;368(6490):489–93. https://doi.org/10.1126/science.abb3221 .
Article CAS PubMed PubMed Central Google Scholar
Kermack WO, McKendrick AG. A contribution to the mathematical theory of epidemics. Proc R Soc Lond. 1927;115(772):700–21. https://doi.org/10.1098/rspa.1927.0118 .
Hethcote HW. The mathematics of infectious diseases. Siam Rev. 2000;42(4):599–653. https://doi.org/10.1137/SIREAD000042000004000655000001 .
Estrada E. COVID-19 and SARS-CoV-2. Modeling the present, looking at the future. Phys Rep. 2020;869:1–51. https://doi.org/10.1016/j.physrep.2020.07.005 .
Mizumoto K, Chowell G. Transmission potential of the novel coronavirus (COVID-19) onboard the diamond Princess Cruises Ship, 2020. Infect Dis Model. 2020;5:264–70. https://doi.org/10.1016/j.idm.2020.02.003 .
Delamater PL, Street EJ, Leslie TF, Yang YT, Jacobsen KH. Complexity of the Basic Reproduction Number. Emerg Infect Dis. 2019;25(1):1–4. https://doi.org/10.3201/eid2501.171901 .
Annas S, Isbar Pratama M, Rifandi M, Sanusi W, Side S. Stability analysis and numerical simulation of SEIR model for pandemic COVID-19 spread in Indonesia. Chaos Solit Fract. 2020;139:110072. https://doi.org/10.1016/j.chaos.2020.110072 .
Li Q, Guan X, Wu P, Wang X, Zhou L, Tong Y, Ren R, Leung KSM. Early Transmission Dynamics in Wuhan, China, of Novel Coronavirus-Infected Pneumonia. N Engl J Med. 2020;382(13):1199–209. https://doi.org/10.1056/NEJMoa2001316 .
Anggriani N, Ndii MZ, Amelia R, Suryaningrat W, Pratama MAA. A mathematical COVID-19 model considering asymptomatic and symptomatic classes with waning immunity. Alexandria Eng J. 2022;61(1):113–24. https://doi.org/10.1016/j.aej.2021.04.104 .
Efimov D, Ushirobira R. On an interval prediction of COVID-19 development based on a SEIR epidemic model. Ann Rev Control. 2021;51:477–87. https://doi.org/10.1016/j.arcontrol.2021.01.006 .
Lin Q, Zhao S, Gao D, Lou Y, Yang S, Musa SS, Wang MH. A conceptual model for the coronavirus disease 2019 (COVID-19) outbreak in Wuhan, China with individual reaction and governmental action. Int J Infect Dis. 2020;93:211–16.
Chao L, Feng P, Shi P. Study on the epidemic development of COVID-19 in Hubei. J Zhejiang Univ (Med Sci). 2020;49(2):178–84.
Reiner RC, Barber RM, Collins JK, et al. Modeling COVID-19 scenarios for the United States. Nat Med. 2021;27(1):94–105. https://doi.org/10.1038/s41591-020-1132-9 .
Article CAS Google Scholar
Shahid F, Zameer A, Muneeb M. Predictions for COVID-19 with deep learning models of LSTM GRU and Bi-LSTM. Chaos Solit Fract. 2020;140:110212. https://doi.org/10.1016/j.chaos.2020.110212 .
Chimmula VKR, Zhang L. Time series forecasting of COVID-19 transmission in Canada using LSTM networks. Chaos Solit Fract. 2020;135:109864. https://doi.org/10.1016/j.chaos.2020.109864 .
Hamou AA, Azroul E, Hammouch Z, Alaoui AAL. A fractional multi-order model to predict the COVID-19 outbreak in Morocco. Appl Comput Math. 2021;20(1):177–203.
Zhou, L., Zhao, C., Liu, N., Yao, X., & Cheng, Z. Improved LSTM-based deep learning model for COVID-19 prediction using optimized approach. Eng Appl Artif Intell. 2023; 122. https://doi.org/10.1016/j.engappai.2023.106157 .
Huang C, Chen Y, Ma Y, Kuo P. Multiple-Input Deep Convolutional Neural Network Model for COVID-19 Forecasting in China. medRxiv. 2020;74822–34.
Gautam Y. Transfer Learning for COVID-19 cases and deaths forecast using LSTM network. Isa Trans. 2022;124:41–56. https://doi.org/10.1016/j.isatra.2020.12.057 .
Article PubMed Google Scholar
Ghany KKA, Zawbaa HM, Sabri HM. COVID-19 prediction using LSTM algorithm: GCC case study. Inform Med Unlock. 2021;23:100566. https://doi.org/10.1016/j.imu.2021.100566 .
Devaraj J, Madurai Elavarasan R, Pugazhendhi R, Shafiullah GM, Ganesan S, Jeysree AK, Khan IA, Hossain E. Forecasting of COVID-19 cases using deep learning models: Is it reliable and practically significant? Results Phys. 2021;21:103817. https://doi.org/10.1016/j.rinp.2021.103817 .
Arora P, Kumar H, Panigrahi BK. Prediction and analysis of COVID-19 positive cases using deep learning models: a descriptive case study of India. Chaos Solit Fract. 2020;139:110017. https://doi.org/10.1016/j.chaos.2020.110017 .
Liu Q, Fung DLX, Lac L, Hu P. A Novel Matrix Profile-Guided Attention LSTM Model for Forecasting COVID-19 Cases in USA. Front Public Health. 2021;9:741030. https://doi.org/10.3389/fpubh.2021.741030 .
Ribeiro MHDM, Da Silva RG, Mariani VC, Coelho LDS. Short-term forecasting COVID-19 cumulative confirmed cases: perspectives for Brazil. Chaos Solit Fract. 2020;135:109853. https://doi.org/10.1016/j.chaos.2020.109853 .
Shoko C, Sigauke C. Short-term forecasting of COVID-19 using support vector regression: an application using Zimbabwean data. Am J Infect Control. 2023. https://doi.org/10.1016/j.ajic.2023.03.010 .
Feng T, Peng Y, Wang J. ISGS: A Combinatorial Model for Hysteresis Effects. Acta Electronica Sinica. 2023;09:2504–9.
Zhou H, Zhang S, Peng J, Zhang S, Li J, Xiong H, Zhang W. Informer: Beyond Efficient Transformer for Long Sequence Time-Series Forecasting. AAAI Conference Artif Intell. 2020;35(12):11106–15.
Rahimi I, Chen F, Gandomi AH. A review on COVID-19 forecasting models. Neural Comput Applic. 2023;35:23671–81. https://doi.org/10.1007/s00521-020-05626-8 .
Chen J, Creamer GG, Ning Y, Ben-Zvi T. Healthcare Sustainability: Hospitalization Rate Forecasting with Transfer Learning and Location-Aware News Analysis. Sustainability. 2023;15(22):15840. https://doi.org/10.3390/su152215840 .
Download references
Acknowledgements
We would like to acknowledge the hard and dedicated work of all the staff that implemented the intervention and evaluation components of the study.
No external funding received to conduct this study.
Author information
Authors and affiliations.
School of Logistics, Beijing Wuzi University, No.321, Fuhe Street, Tongzhou District, Beijing, 101149, China
Weiwei Zhang & Xinchun Li
You can also search for this author in PubMed Google Scholar
Contributions
WWZ and XCL conceived the idea and conceptualised the study. XCL collected the data. WWZ analysed the data. WWZ and XCL drafted the manuscript, then WWZ and XCLreviewed the manuscript. WWZ and XCL read and approved the final draft.
Corresponding author
Correspondence to Xinchun Li .
Ethics declarations
Ethics approval and consent to participate.
Not applicable.
Consent for publication
Competing interests.
The authors declare no competing interests.
Additional information
Publisher’s note.
Springer Nature remains neutral with regard to jurisdictional claims in published maps and institutional affiliations.
Rights and permissions
Open Access This article is licensed under a Creative Commons Attribution 4.0 International License, which permits use, sharing, adaptation, distribution and reproduction in any medium or format, as long as you give appropriate credit to the original author(s) and the source, provide a link to the Creative Commons licence, and indicate if changes were made. The images or other third party material in this article are included in the article's Creative Commons licence, unless indicated otherwise in a credit line to the material. If material is not included in the article's Creative Commons licence and your intended use is not permitted by statutory regulation or exceeds the permitted use, you will need to obtain permission directly from the copyright holder. To view a copy of this licence, visit http://creativecommons.org/licenses/by/4.0/ . The Creative Commons Public Domain Dedication waiver ( http://creativecommons.org/publicdomain/zero/1.0/ ) applies to the data made available in this article, unless otherwise stated in a credit line to the data.
Reprints and permissions
About this article
Cite this article.
Zhang, W., Li, X. A data-driven combined prediction method for the demand for intensive care unit healthcare resources in public health emergencies. BMC Health Serv Res 24 , 477 (2024). https://doi.org/10.1186/s12913-024-10955-8
Download citation
Received : 21 September 2023
Accepted : 05 April 2024
Published : 17 April 2024
DOI : https://doi.org/10.1186/s12913-024-10955-8
Share this article
Anyone you share the following link with will be able to read this content:
Sorry, a shareable link is not currently available for this article.
Provided by the Springer Nature SharedIt content-sharing initiative
- Public health emergency
- ICU healthcare resource demand
- Machine learning
- Combined prediction
BMC Health Services Research
ISSN: 1472-6963
- General enquiries: [email protected]
Thank you for visiting nature.com. You are using a browser version with limited support for CSS. To obtain the best experience, we recommend you use a more up to date browser (or turn off compatibility mode in Internet Explorer). In the meantime, to ensure continued support, we are displaying the site without styles and JavaScript.
- View all journals
- My Account Login
- Explore content
- About the journal
- Publish with us
- Sign up for alerts
- Open access
- Published: 30 April 2024
Incremental high average-utility itemset mining: survey and challenges
- Jing Chen 1 , 3 na1 ,
- Shengyi Yang 2 na1 ,
- Weiping Ding 4 ,
- Peng Li 5 ,
- Aijun Liu 3 ,
- Hongjun Zhang 1 &
- Tian Li 6
Scientific Reports volume 14 , Article number: 9924 ( 2024 ) Cite this article
Metrics details
- Computer science
- Information technology
The High Average Utility Itemset Mining (HAUIM) technique, a variation of High Utility Itemset Mining (HUIM), uses the average utility of the itemsets. Historically, most HAUIM algorithms were designed for static databases. However, practical applications like market basket analysis and business decision-making necessitate regular updates of the database with new transactions. As a result, researchers have developed incremental HAUIM (iHAUIM) algorithms to identify HAUIs in a dynamically updated database. Contrary to conventional methods that begin from scratch, the iHAUIM algorithm facilitates incremental changes and outputs, thereby reducing the cost of discovery. This paper provides a comprehensive review of the state-of-the-art iHAUIM algorithms, analyzing their unique characteristics and advantages. First, we explain the concept of iHAUIM, providing formulas and real-world examples for a more in-depth understanding. Subsequently, we categorize and discuss the key technologies used by varying types of iHAUIM algorithms, encompassing Apriori-based, Tree-based, and Utility-list-based techniques. Moreover, we conduct a critical analysis of each mining method's advantages and disadvantages. In conclusion, we explore potential future directions, research opportunities, and various extensions of the iHAUIM algorithm.
Introduction
Data Mining (DM) refers to a technique for discovering interesting and meaningful data patterns in large databases. This discipline effectively integrates machine learning, statistics, and database systems 1 , 2 to analyze datasets and discover hidden relationships. ARM 3 , 4 , 5 , 6 is a data mining method that is well-known for discovering significant relationships between database items 7 , 8 , 9 . Frequent Pattern Mining (FPM) 10 , 11 , 12 , 13 , an approach for detecting recurrent patterns in binary datasets 14 , 15 , is widely used in ARM 16 , 17 , 18 . The approach can effectively find the relationships between patterns 16 , 17 , 18 and has been implemented in various real-world problems 19 , 20 , 21 , 22 .
Two commonly used algorithms for mining patterns in binary databases are Apriori 23 and FP-Growth 24 . Apriori uses a breadth-first search (BFS) algorithm and requires multiple scans of the database. FP-Growth, on the other hand, uses a DepthFirst Search (DFS) algorithm with an FP-tree structure, requiring only two scans of the database. Frequent Itemset Mining (FIM) 25 , 26 , 27 , 28 is a well-known research topic aiming to discover frequent itemsets (FI) 24 from a database. However, frequency alone is not always accurate or meaningful in real-world mining scenarios. In a retail market, for example, frequent items may indicate low-profit products, as lower-priced products tend to sell better. Conversely, infrequent itemsets have the potential to generate high profits.
Addressing the limitations inherent in conventional techniques, comprehensive research and successive studies 29 , 30 , 31 have led to proposition and development 32 , 33 , 34 , 35 of the HUIM algorithm. Unlike conventional methods that concentrate only on the frequency of itemsets, each item’s internal and external utility were considered in HUIM. Utilizing the High-Utility Itemset Mining approach, the utility value of an itemset elevates in correlation with its size, underlined by the quantity of elements within the itemset. Consequently, itemsets of greater length typically yield higher profits, representing a crucial metric for determining high-utility itemsets.
Hong et al. 36 proposed the HAUIM approach, which measures the average utility of itemsets based on their length to provide a fair evaluation of itemsets. If the average utility of an itemset meets or exceeds a predetermined minimum threshold, it is identified as a High Average Utility Itemset (HAUI). Consequently, as compared to traditional HUIM, presents a distinct set of challenges that necessitate the development of novel techniques, including downward-closure properties, upper-bound models, pruning strategies, and mining procedures. In their work, Lin et al. introduced a tree-based algorithm called HAUP tree 37 for mining HAUI sets. They leveraged this efficient tree structure to enhance mining performance. Furthermore, to enhance the speed of the mining process, they introduced a projection-based algorithm called PAI 38 . In a subsequent study, Lin et al. 39 devised an innovative data structure known as the Average Utility (AU) list, which efficiently mines HAUI from static databases. This AU list-based approach represents the current state-of-the-art algorithm for mining HAUI.
The efficiency of existing techniques used to detect HAUI in a static database can be compromised when the size of the database undergoes changes. Specifically, when new transactions are introduced, it necessitates reprocessing the entire database in order to update the results. To address this issue, Cheung et al. 40 introduced the concept of FUP (Fast Update) to preserve the discovered frequent itemsets through incremental updates. As the database changes, their framework considers four scenarios in which the updates are handled differently depending on the prescribed methods. The FUP concept has already been applied to ARM 40 , 41 , HUIM 42 , 43 , and HAUIM 44 , 45 . However, these methods still have the disadvantage of rescanning certain itemsets and requiring additional database scans to obtain these itemsets. To address this challenge, Wu et al. 46 introduced a hierarchical approach that incorporates the pre-large concept in HAUIM for incremental mining. Nevertheless, an important limitation of their model lies in the absence of theoretical evidence supporting the ability of the pre-large concept to effectively preserve the correctness and completeness of the maintained HAUI.
In the past 10 years, researchers have developed over ten algorithms specifically designed for handling dynamic databases in the context of transaction insertion for iHAUIM. The objective of iHAUIM is to identify patterns that meet the minimum utility constraints while continuously inserting new records into the original database. This problem can be considered as a constraint-based mining problem. The development of efficient iHAUIM algorithms is a new research problem because it makes iHAUIM tasks more scalable with respect to database updates.
Real-time processing of data streams has become essential due to the increasing number of applications, including auditors, online clickstreams, and power throughput, that generate data streams that require immediate processing. These data streams are generated rapidly and accumulate in real time, demanding efficient processing methods. To address this, a single scan of the data stream is typically employed to build a data structure, which is then maintained throughout the execution. This approach ensures that newly generated data influences the resulting patterns. When new data is inserted, the data structure is updated and reconstructed to enable efficient mining. Traditional methods used for processing static data, which involve multiple scans of the database and deletion of unwanted items, are not suitable for handling data streams. Instead, techniques like sliding windows 47 , 48 , 49 , damping windows 50 , 51 , 52 , and landmark windows 53 are employed to effectively handle stream data.
Moreover, in 54 , a sliding window model is utilized, alongside a decay factor. MPM 55 and DMAUP 52 are both mining methods aiming to identify high average utility patterns, by employing a damping window concept. Essentially, they analyze recent transactions more heavily than prior ones, but they struggle to function effectively with large databases, especially patterns that frequently occur in recently made transactions. This is due to their tendency to process the entire database each time they encounter a new data stream. In addition to this, each computation of the decay factor is considerably computation-heavy. MPM, being a tree-based method, is unable to store the actual utility of the respective items. This results in consuming a lot of runtime and memory for generating candidate patterns. Plus, verifying candidate patterns for accuracy demands extra database scans, and therefore, it is unsuitable for data stream analysis.
Although the iHAUIM algorithms have been developed, there has been no comprehensive exploration or empirical study to compare their performance. The primary objective of this paper is to provide a comprehensive and in-depth analysis of the notable progress in iHAUIM. The methodologies discussed in this study can serve as valuable insights not only for iHAUIM but also for other data mining tasks, including incremental data mining 56 , 57 and dynamic data mining 58 . In 57 , a dynamic and incremental profit environment is explored, and a unique approach named IncDEFIM is introduced. This method employs strategies like merging transactions, projecting databases, and setting strict upper bounds to minimize the expenses associated with database scans while efficiently removing unproductive item sets. By examining these advancements, this research aims to contribute to the broader field of data mining and inspire further developments in related domains.
This article made three distinct contributions. Firstly, it provided a comprehensive overview of the essential technologies employed in iHAUIM algorithms. Secondly, it conducted a comprehensive review of the latest advancements in this field. Lastly, it identified and emphasized potential areas for future research in data mining. Moreover, this research paper presents a new classification system that integrates contemporary methods for extracting HAUIs from dynamic datasets. As a result, it offers a valuable framework for advanced iHAUIM algorithms, eliminating redundancies in the existing literature. The main contributions of this work are as follows:
The paper proposes a classification approach for the most advanced iHAUIM algorithms that includes the most up-to-date information on methodologies for extracting HAUIs from dynamic datasets.
Based on the dynamic datasets, we categorize HAUIM algorithms into three types: Apriori-based, Tree-based, and Utility-list-based.
The article provides a thorough comparison of the benefits and drawbacks of the most sophisticated iHAUIM algorithms, including metrics such as running time, memory usage, scalability, data structures, and pruning techniques.
Furthermore, this paper offers a comprehensive summary and discussion of current iHAUIM techniques. Lastly, it outlines potential research possibilities and key areas for future iHAUIM research.
The structure of this article is as follows: “ Preliminaries and problem statement of iHAUIM ” section provides an overview of the fundamental concepts and definitions related to iHAUIM. “ State-of-the-art algorithms for iHUIM ” section classifies and explains iHAUIM approaches based on dynamic datasets, evaluating their advantages and disadvantages. “ Summary and discussion” section presents a thorough overview and evaluation of the latest iHAUIM techniques, which highlights potential research directions and opportunities for future advancements in iHAUIM. Lastly, “ Conclusion ” section concludes the survey, summarizing the key findings and contributions of the article.
Preliminaries and problem statement of iHAUIM
In this section, we lay the foundation by providing essential preparations and presenting a formal definition of the iHAUIM problem. We will also introduce the symbols that will be used throughout the rest of this paper, as shown in Table 1 , and these symbols will be explained in subsequent sections. Below are examples of the original database and item utility table, presented as Table 2 and Table 3 , respectively. The original database comprises five transactions, each identified by a transaction identifier (TID) and containing non-redundant items. The internal utility of each item is specified after a colon. Table 3 displays seven items that are present in the original database, represented as I = {a, b, c, d, e, f, g}. The external utility of each item is shown in Table 3 . The minimum high average utility upper-bound threshold δ and the lower-bound threshold δ L are set based on the user’s preference (positive integers). Below are commonly used definitions for incremental high average utility pattern mining 44 , 59 , 60 , sliding window 47 , 49 , 61 , and dampened window models 52 , 55 , derived from the provided original database and item utility table.
Definition 1
Item utility 62 . The utility of an item i j in a transaction T p is represented as u(i j , T p ) and is computed as the product of its internal utility in transaction T p , denoted as iu(i j , T p ) 62 , and its external utility eu(i j ).
For instance, in Table 2 , the item utility of ’a’ in T 1 is calculated as u(a, T 1 ) = 3 × 4 = 12.
Definition 2
Transaction utility 63 . The transaction utility of 63 T p is indicated and computed as follows 52 .
For instance, in Table 2 , the transaction utility of T 1 is calculated as u(T 1 ) = u(a, T 1 ) + u(b, T 1 ) + u(c, T 1 ) + u(d, T 1 ) = 12 + 5 + 8 + 8 = 33.
Definition 3
Total utility 64 . The total utility (tu DB ) of a database DB is defined as follows:
As an example, the total utility in the illustrated case of Table 2 is computed as tu DB = 33 + 69 + 40 + 41 + 49 (= 232).
Definition 4
Average utility 62 .The average utility of item X in transaction T p , denoted as au(X, T p ), is calculated by dividing the sum of item utilities in X by the length of X 61 , |X|.
Definition 5
Itemset Average utility. The average utility of X in the database (au(X)) is determined by summing up the average utilities of X in all transactions present in the database DB 61 .
For instance, in Table 2 , the average utility of ’ac’ in the database is calculated as au(ac) = au(ac, T 1 ) + au(ac,T 3 ) = 10 + 9.5 = 19.5.
Definition 6
HAUI. An itemset is categorized as a HAUI if its au satisfies 65 :
For example, if δ is 8%, then itemset a, c is a HAUI since au(a, c) = 19.5 ≥ 2320.08 = 18.56.
Definition 7
Maximum utility 66 . The maximum utility of 66 transaction T p is notated as follows:
For instance, in Table 2 , the maximum utility of T 1 is calculated as mu(T 1 ) = 12.
Definition 8
AUUB 55 . For an item i j , the AUUB of i j is as follows:
For instance, in Table 2 , the AUUB of a is auub(a) = mu(T 1 ) + mu(T 3 ) + mu(T 5 ) = 12 + 21 + 35 = 68.
DC, Downward closure property of AUUB 46 .
According to the downward closure property of AUUB 46 , if an itemset Y is a superset of itemset X 46 , denoted as Y ⊇ X, the following formula ( 9 ) can be obtained.
Hence, if auub(X) DB ≥ tu DB × δ then auub(Y) DB ≤ auub(X) DB ≤ tu DB × δ is satified for any superset of X 46 .
Definition 10
HAUUBI 46 . For the dataset DB, if an itemset X is a HAUUBI DB , it should satisfy the following condition as 46 :
Definition 11
PAUUBI. Itemset X is a PAUUBIDB in the initial database 62 :
For instance 62 , suppose δ the and δ L are respectively set as 13% and 8%. The itemset (ce) is PAUUBI with an auub of 26, which lies between δ L (= 232 × 8%)(= 18.56) and δ(= 232 × 13%) (= 30.16).
Definition 12
The condition of HAUI UDB .In the updated (DB + DBn) database, in Table 4 , an itemset X qualifies as a HAUI UDB if it meets the following conditions 46 :
where au(X) UDB indicates the new average-utility of X,TU DB and TU DBn+ are respectively the transaction utility in DB and DBn + , and δ is the upper bound of utility threshold 46 .
State-of-the-art algorithms for iHUIM
In recent years, a considerable number of iHAUIM (Insert-based High Average Utility Itemset Mining) techniques have been developed to handle dynamic databases involving transaction insertions. So far,a total of 19 iHAUIM algorithms have been proposed, as shown in Fig. 1 , which can be classified into three main categories: apriori-based, tree-based, and utility-list-based methodologies. In the upcoming sections, we will evaluate the strengths and weaknesses of each algorithm, as indicated in Table 5 , with the primary aim of mining itemsets that exhibit high average utility during transaction updates.
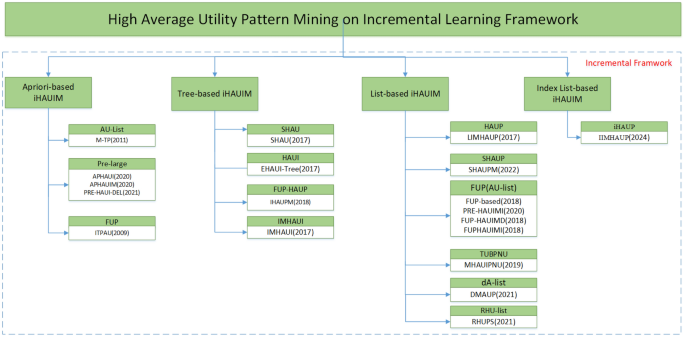
Classification of iHAUIM Algorithms.
The traditional HAUIM algorithm is only applicable to static datasets. However, if the dataset undergoes record updates, the static techniques necessitate processing all the data from the start to extract HAUI. Consequently, this results in high time and memory consumption.
Apriori-based iHAUIM
Based on the Fast Update (FUP) concept 40 , the TPAU 67 algorithm discovered HAUI from dynamic datasets that change with the insertion of new records. FUP records the previously frequent large itemsets and their counts for use in the maintenance process. when a new transactions are added, the FUP(Frequent Utility Pattern) algorithm generates candidate 1-itemsets. Subsequently, the candidate itemsets are compared with the previous itemsets in order to classify them into the following four cases:
The itemset is large in both the original database and the newly added transactions, resulting in its categorization as large in both domains.
The itemset is large in the original database but not in the newly inserted transactions.
Although not considered large in the original database, the itemset demonstrates significance in the newly inserted transactions.
The itemset does not meet the threshold for being deemed large in either the original database or the newly inserted transactions.
The suggested algorithm adopts an approach similar to Apriori to systematically explore the layers of HAUI. To optimize the search process, it employs early pruning techniques to discard low-utility itemsets. The algorithm leverages the downward closure property in a two-stage process, enabling it to generate a reduced set of candidate items at each level. In the first stage, an overestimated itemset is obtained using an average utility upper bound. In the second stage, an actual average utility value is computed, considering a high upper bound. Through these steps, the algorithm efficiently extracts HAUIs from incremental transaction datasets, enhancing its mining capabilities. Afterwards, the modified itemsets are categorized into four groups based on their characteristics, and whether their count difference in the modified records is positive, negative, or zero. Each group is then subjected to its specific processing approach.
The M-TP 59 proposes a two-stage record modification maintenance method, aimed at mining HAUI from updated datasets. To begin, this approach calculates the count difference by comparing the AUUB (Average Utility Upper Bound) of each modified itemset before and after modification. Then, the modified itemsets are divided into four parts based on their characteristics. This classification is determined by whether they are HAUUBI (High Average Utility Upper Bound Itemsets) in the original dataset and whether their count difference in the modified records is positive or negative (including zero). Each part is then subjected to its specific processing approach. The M-TP algorithm reduces the time required to reprocess the entire updated dataset. In the original dataset, the itemsets are larger in the first two cases, and smaller in the last two cases. Conversely, the first and third cases exhibit a positive count difference, while in the modified records, the count difference turns negative or remains zero in the second and fourth cases. Lan et al. 59 proposed four cases of modifying records from existing datasets in Fig. 2 .
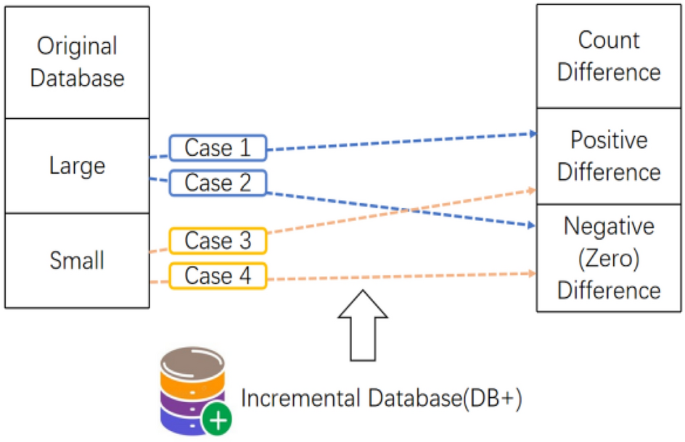
Four cases when records are modified from an existing dataset.
In contrast to conventional approaches, the algorithm 59 reduced the time required for the entire dataset updating time. In terms of runtime, the M-TP algorithm demonstrates superior performance to the Batch TP algorithm across different minimum average utility thresholds 68 .
The algorithm 69 is proposed to handle transaction deletions in dynamic databases using the pre-large concept on HAUIM, called PRE-HAUI-DEL. The pre-large concept is used as a buffer on HAUIM to reduce the number of database scans, particularly during transaction deletions, and its overview is illustrated in Fig. 3 . Additionally, two upper bounds are established in the algorithm to early prune unpromising candidates, which can accelerate computation costs. Compared to Apriori-like models, PRE-HAUI-DEL excels in efficiently mining high-average utility items in updated databases. In addition, the developed algorithm also uses the LPUB upper bound model, which can significantly reduce the number of candidate items that need to be checked in the search space. Compared to the general model that updates discovered knowledge Using batch processing mode, our designed PRE-HAUI-DEL can effectively maintain the discovered HAUI without the need for multiple database scans, as illustrated in Figs. 4 and 5 . This not only reduces computational costs but also correctly and completely maintains knowledge about HAUI.
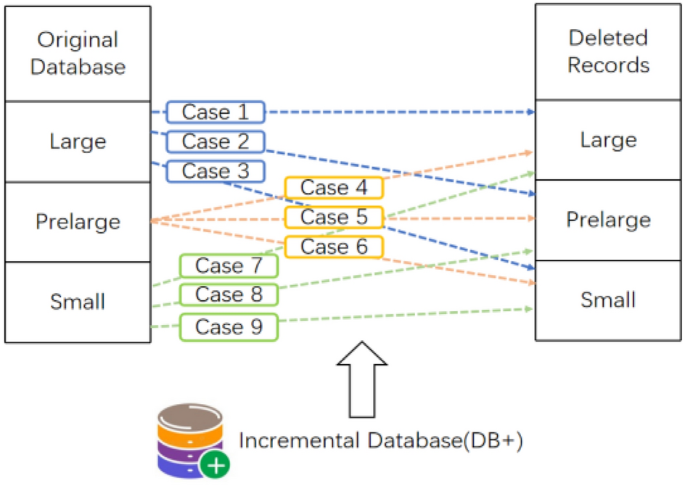
Nine cases of the pre-large concept.
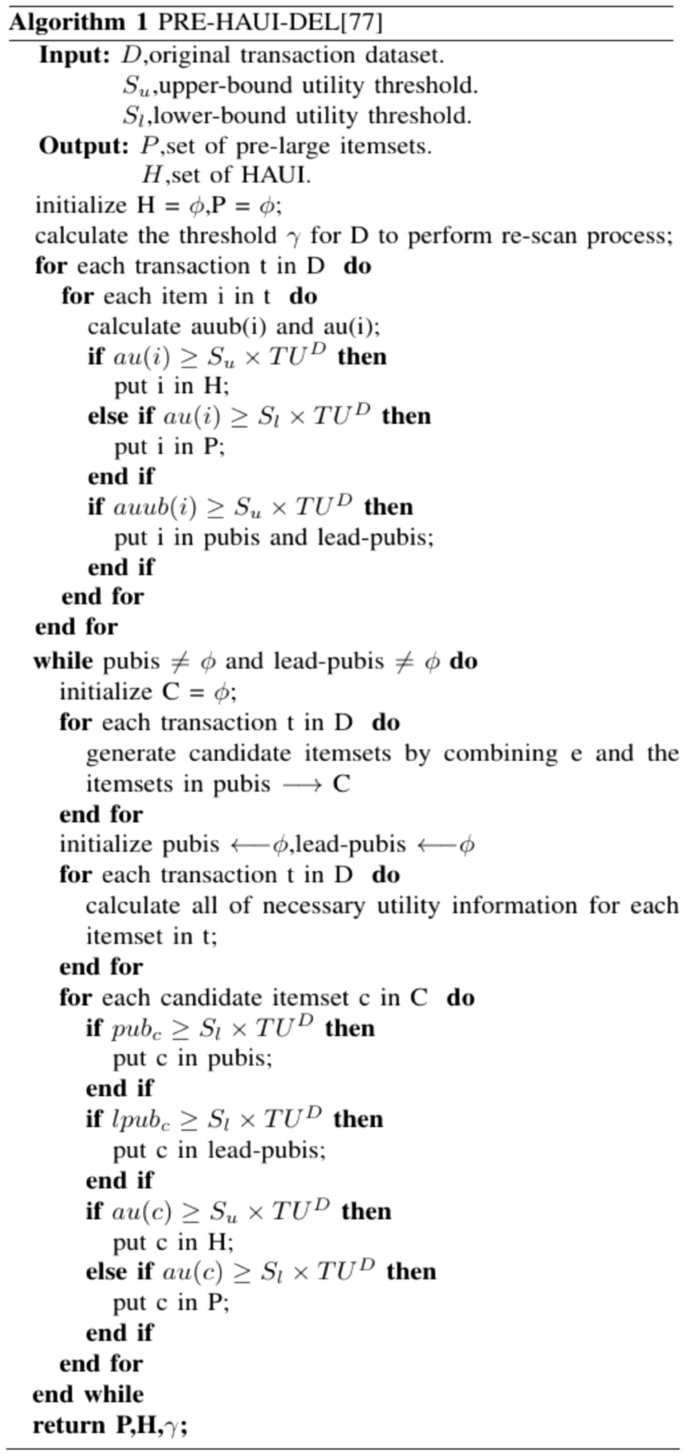
PRE-HAUI-DEL.
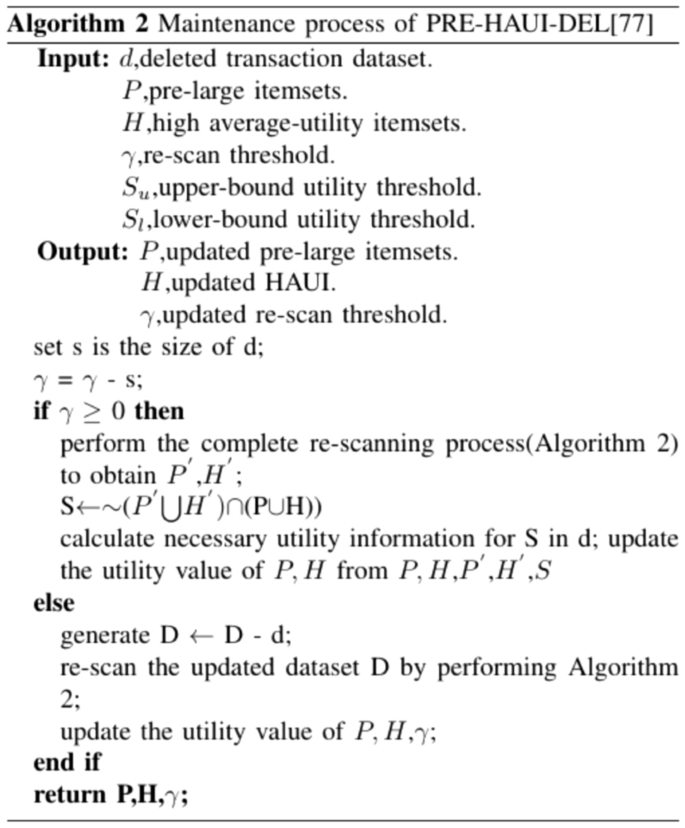
Maintenance process of PRE-HAUI-DEL.
In 65 , this article introduces the APHAUI algorithm, a HAUP (High-Utility Association Pattern) algorithm based on Apriori, capable of effectively mining HAUI from dynamic datasets. This algorithm follows an Apriori-like approach 23 and employs the pre-large concept 56 to reduce the search space and proactively prune less promising candidate items, revealing promising itemsets during maintenance. The final results of cases 1, 5, 6, 8, and 9 remain unaffected. Moreover, the amount of information discovered in cases 2 and 3 can be reduced, while some new information might emerge in cases 4 and 7. As shown in Fig. 6 ,the pre-large concept can easily handle itemsets in cases 2, 3, and 4. The authors devised two upper bounds, namely Partial Upper Bound (pub) and Lower pub (lpub), to enhance the efficiency of the mining process. The pub serves as astringent upper limit that reduces the size and upper utility bound of promising itemsets. A High pub itemset (pubi) with greater utility than pub was developed.
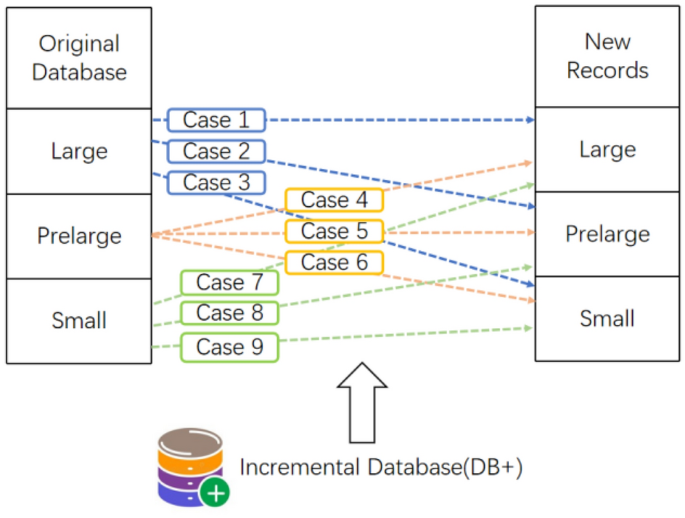
Furthermore, the algorithm introduces a subset named lpubi (Lead-pubi) as a part of pubi, capable of further reducing the candidate itemset for subsequent mining processes. Despite the algorithm generating both pubi and lpubi itemsets, the applicability of lpubi is constrained compared to pubi. Lead-pubi contributes to reducing the count of candidate items. Additionally, a formula is employed to curtail unnecessary dataset scans. Lastly, the introduction of a linked list ensures that each transaction is scanned at most once, thereby minimizing the frequency of dataset scans during the update process.
The algorithm begins by scanning the input dataset, followed by the dynamic processing flow of the APHAUI method. By employing a designed re-scanning threshold, it can automatically determine the update pace of the incremental dataset, enhancing mining efficiency. During the algorithm’s execution, two upper bounds, pub, and lead-pub, along with two itemsets, pubi and lead-pubi, are used to reveal the complete set of HAUIs within the transaction dataset. This algorithm not only demonstrates strong performance but also holds significant potential in real-time scenarios.
Previous HAUIM algorithms processed dynamic datasets using batch processing. As a result, the APHAUIM 46 incurred costs in terms of past computations and the discovery of pattern information. To address this issue, the concept of FUP (Frequent Update Pattern) was introduced 40 for real-time pattern discovery and storage of pattern information. However, this requires rescanning the dataset to acquire the latest information. In 70 , a new model called Apriori-based Potential High Utility Itemset Mining (APHAUIM) is proposed, which effectively reveals potential high utility patterns from uncertain databases in industrial IoT by maintaining two item sets (phps and plhps) using two tight upper-bound values (pub and lead-pub), while ensuring the completeness and correctness of the mining results.
Based on the concepts of pre-large 56 , 58 and the Apriori method 23 , a new algorithm called APHAUIM is introduced to mine HAUI from incremental transaction datasets. PAUBI is introduced to retain promising HAUBI. PAUBI acts as a buffer to minimize the rescans needed for checking whether a small itemset evolves into a large itemset. An overview of the pre-large concept is depicted in Fig. 6 .
Compared with the benchmark FUP-based HAUIM algorithm 67 , the designed algorithm is better suited for streaming environments in dynamic datasets. However, a limitation lies in the fact that, similar to the benchmark method, the proposed algorithm also incurs a considerable amount of rescanning time. This is because locating itemsets in the buffer to update the insertion process requires additional time. Therefore, selecting appropriate thresholds is a topic of significant importance.
Tree-based iHAUIM
The SHAU 61 introduces an effective algorithm named SHAU for analyzing time-sensitive data in terms of significance. The algorithm employs the HAUPM algorithm based on sliding windows to process data streams. The HAUPM algorithm considers only new data during the pattern mining process for discovering data streams. As the algorithm is based on the concept of sliding windows 71 , 72 , 73 , 74 , it divides the data stream into multiple blocks or batches. The concept of sliding windows for data streams was initially proposed by Yun et al. 61 .
The SHAU algorithm employs a novel SHAU tree structure. In this tree, each node consists of three elements: the first element stores the tid that includes the item, the second element is used to store the recent auub data information of the data stream batch by batch, and the third element is a link pointing to another node with the same tid. The auub of different items in the data stream is stored in the header table of the SHAU tree. Additionally, the efficiency of SHAU is enhanced by utilizing a new strategy called RUG.
The EHAUI-tree algorithm 75 is proposed as an improved iteration of the HAUI-tree algorithm 76 . The primary objective is to enhance mining efficiency and reduce memory consumption. The algorithm aims to mine by adding new transactions instead of restarting the dataset. It utilizes the downward closure property and employs an index table structure. This innovative approach enhances computational efficiency while simultaneously reducing memory requirements. In addition, the algorithm introuces a bit-array structure to compute utility values more efficiently. However, the algorithm performs poorly on large datasets or small thresholds.
In 45 , a new approach called IHAUPM is proposed for handling frequent transaction insertions in updated datasets. The algorithm leverages an adapted FUP concept to efficiently integrate prior information and update the results when new information is discovered during updates. The newly inserted transactions are categorized into four distinct cases, considering the occurrence frequency of the original dataset and the newly inserted transactions. This categorization ensures effective handling of different scenarios and minimizes repetition during the updating process. In cases where the itemset is the original dataset or the HAUUBI in the new insert, it remains a HAUUBI, while in cases where it is not, it remains non-HAUUBI.
For cases where it is necessary to determine whether the itemset is actually a HAUUBI based on existing information or to rescan the original dataset, the algorithm employs a compressed HAUP tree data structure to store and utilize the required information. This approach requires minimal scanning of the original dataset and is highly efficient while preserving the count of prefix items processed in each node of the tree.
This article 60 proposes an algorithm called IMHAUI, which is based on the IHAUI-tree and uses node sharing to preserve the information of the incremental dataset, thereby addressing the problem of adding new data to the dataset which may cause the number of items to exceed or fall below the minimum support threshold. Each time new data is added, node sharing undergoes reconstruction. To achieve this, transactions within the dataset are sorted in descending order based on their AUUB values. During the reconstruction process, each path is rearranged in decreasing order of the optimal AUUB value. To maintain compactness, a path adjustment technique is utilized 77 . Additionally, the algorithm preserves the AUUB value of each itemset by maintaining a header table. Subsequently, the mined tree is examined to access candidate itemsets, and their actual average utility is computed during the candidate validation phase.
FIMHAUI based on mIHAUI-tree, to address the problems of time-consuming candidate itemset generation and expanding search space while determining the upper limit value caused by IMHAUI 60 . The algorithm performs a singlescan of the dataset to extract information from HAUI. It stores transaction information in each node of IHAUI-Tree, which completely overlaps with the path from the root to that node, and thus only saves the necessary information in the leaf nodes of mIHAUI-Tree. Initially, all transactions are inserted into an empty mIHAUI-tree in a sequential order based on alphabetical order. Subsequently, the path adjustment method proposed in 60 is to adjust the paths in order to enhance the sharing efficiency of nodes within the mIHAUI-tree. The algorithm uses data set projection and merge techniques to efficiently find itemsets. mIHAUI-tree introduces a novel approach by directly obtaining the projected data set for candidate itemsets, eliminating the need for generating conditional patterns and local trees. Additionally, a transaction merge technique identifies identical transactions in dictionary order within one scan. In contrast to the IHAUI-tree, the proposed algorithm offers not only time savings but also a reduction in repetition. However, the performance of the algorithm is unsatisfactory when applied to large datasets or small thresholds.
In 55 the MAMs algorithm was designed to effectively analyze time-sensitive data which was applicable to data streams and employs an exponential damping window model and pattern growth methods. Furthermore, the algorithm considers the temporal aspect of the provided data to acquire pertinent and current pattern knowledge. The algorithm employs DAT structure and TUL to handle dynamic data streams. As new data is inserted into a transaction, the algorithm constructs a DAT data structure and incorporates average utility information. This procedure persists until a user-initiated mining request is encountered. Upon receiving such a request, the MPM algorithm follows the pattern growth approach on the dataset.
The common goal of these algorithms is to enhance the efficiency of data mining, reduce memory consumption, and adapt to the dynamic nature of data. The SHAU algorithm utilizes the HAUPM algorithm based on sliding windows to process data streams, employing the SHAU tree structure to store itemset information from the data stream and enhancing efficiency through the RUG strategy. The EHAUI-tree algorithm, as an improved version of the HAUI-tree algorithm, and the IHAUPM algorithm, introduce new methods for handling frequent transaction insertions in updated datasets. The FIMHAUI algorithm, based on the mIHAUI-tree, addresses the time-consuming generation of candidate itemsets and the expansion of search space in IMHAUI. These algorithms share a common challenge in that they attempt to optimize the mining process through various data structures and strategies to accommodate the dynamic changes and time sensitivity of data. However, they may encounter performance issues when dealing with large datasets or small thresholds, indicating that further optimization and improvement may be necessary in practical applications.
List-based iHAUIM
To address the issue of inadequate performance in mining advanced association rules in dynamic environments, Wu et al. proposed an update algorithm 44 to update the obtained advanced association rules using transaction insertion. The proposed algorithm builds upon the AU list 39 and incorporates the concept of FUP (Frequency Upper Bound) 40 to enhance its performance. To adapt and update advanced association rules with transaction insertion, the proposed algorithm employs a two-stage approach. In the initial stage, the 1-HAUUBI set is derived from the original dataset. Subsequently, an AU list is constructed from the 1-HAUUBI set, facilitating subsequent processing. In the second stage, the algorithm efficiently handles transaction insertion by dividing the HAUUBI set into four partitions based on the FUP (Frequency Upper Bound) criterion. This partitioning strategy minimizes repetition and enhances efficiency during the updating process. The proposed algorithm, as described in 44 , presents four distinct cases for handling transaction insertion, as illustrated in Fig. 7 .
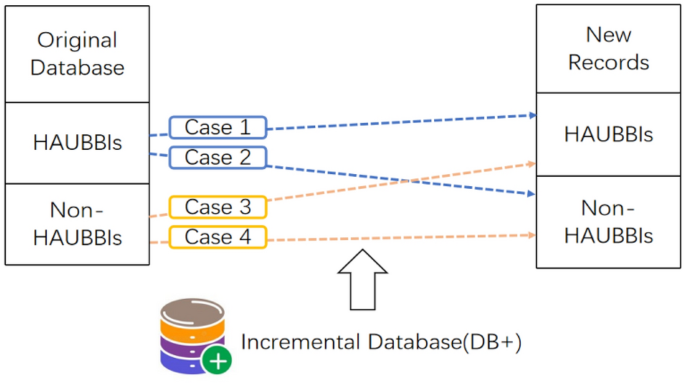
Four cases of the proposed algorithm with transaction insertion.
In each case, the algorithm preserves the HAUUBI set for each partition, with the exception of non-advanced association rules in case 4. These non-advanced items are excluded from the HAUUBI set during dataset updates, as they do not qualify as advanced association rules. This approach effectively reduces redundancy in the algorithm, as illustrated in Fig. 8 . The updateADD and updateDEL methods are used for adding and deleting items in the AU-list structure, respectively. The updateADD function can easily update the auub value of the itemsets based on the AU-list structure. As for the updateDEL function, it can directly remove the unpromising itemsets based on the AU-list structure after the database has been updated.
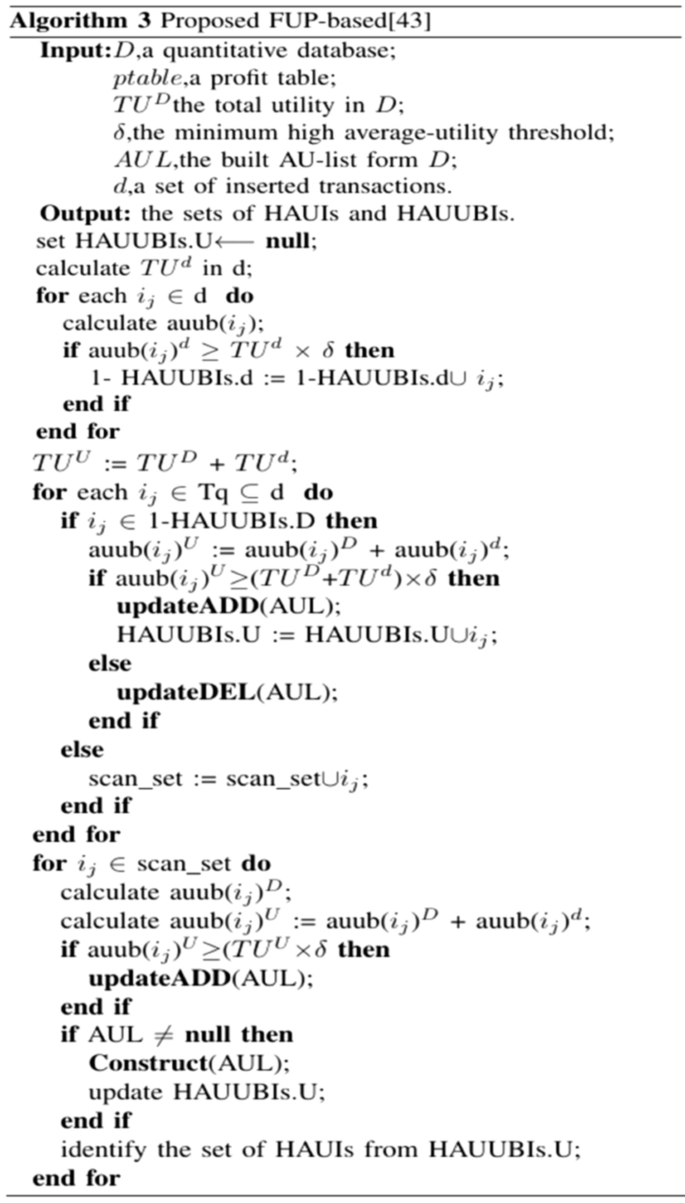
Proposed FUP-based.
The AU list reduces the number of scans on the dataset and the generation of candidate itemsets. After updating the dataset, HAUUBI is added to the AU list, while non-HAUUBI is removed from the AU list. The proposed algorithm effectively updates HAUUBI to identify the actual HAUI in the updated dataset. Subsequently, the remaining itemsets in the AU list are compared against the minimum high average utility threshold, resulting in the identification of the true HAUI within the updated dataset. The proposed algorithm efficiently updates the HAUUBI to discover the actual HAUI. However, sometimes more candidate items need to be evaluated.
The FUP-HAUIMI 78 algorithm is a modified version based on the FUP concept 40 , for discovering HAUI from updated datasets. The algorithm consistently preserves and updates the uncovered information, eliminating the requirement to create data for transaction deletion. Furthermore, it improves the updating process by avoiding the need for multiple scans of the dataset.
The algorithm first constructs the AU-list 39 data structure by scanning the original dataset effectively storing information for mining patterns (candidates) and gradually updating results. All items inserted in transactions are kept in the initial AU-list, and then 1-HAUUBI is classified into four categories based on the FUP concept, as described in 45 , with these four categories illustrated in Fig. 9 . Finally, the algorithm is able to efficiently discover updated HAUUBI and HAUI without generating candidates, as illustrated in Figs. 10 , 16 and 17 .
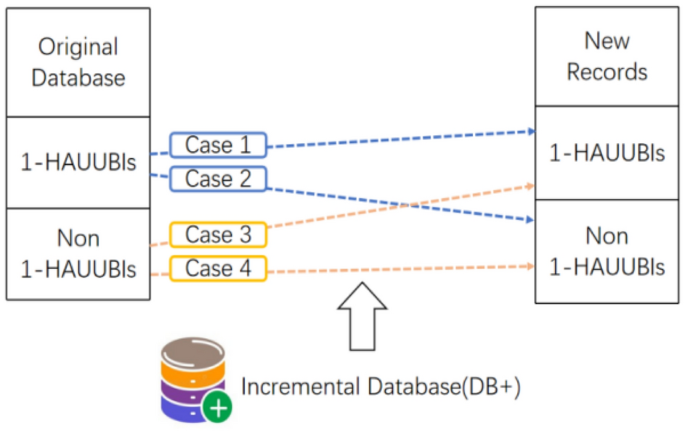
Four cases of the adapted FUP concept.
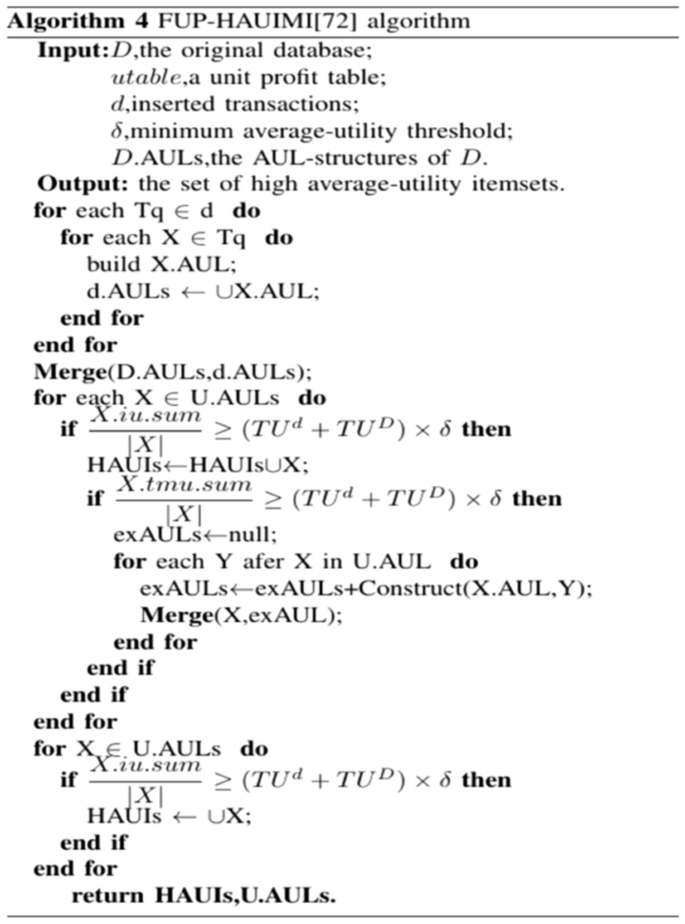
FUP-HAUIMI algorithm.
After the dataset is updated, the concept of FUP is applied to handle transaction insertions 42 . Moreover, a depth-first search approach is employed to generate candidate itemsets.
A data mining method called the FUP-HAUIMD algorithm 79 , which is based on the removal of transactions from the original dataset and utilizes the MFUP (modified FUP) 40 extension from 80 . In this algorithm, deleted transactions can be categorized into four types, each with distinct implications for identifying HAUUBI (Highly Associated Unordered Unique Binary Itemsets), as illustrated in Fig. 11 . In the first category, existing information can be used to determine whether the itemset remains a HAUUBI. For the second category, the item continues to be a HAUUBI. The third category can be safely discarded as it only contains non-HAUUBI. For the fourth category, a complete rescan of the original dataset is necessary. The auub value of each HAUUBI is stored in an AU list 39 , and the AU list is updated every time data is removed. Mining the enumeration tree allows for the evaluation of its true HAUI without requiring multiple scans of the dataset, as illustrated in Figs. 12 and 13 .
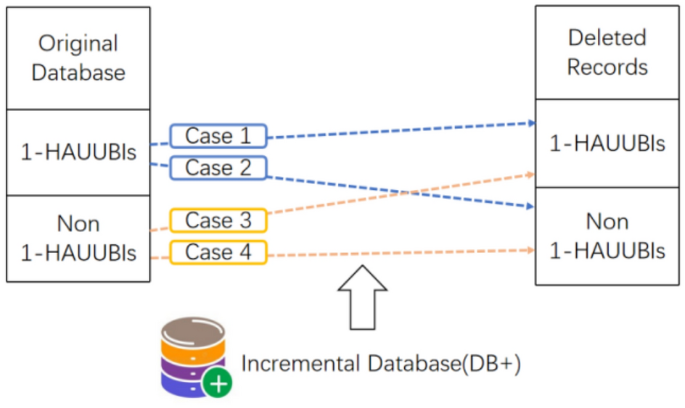
Four cases of the designed FUP-HAUIMD algorithm.
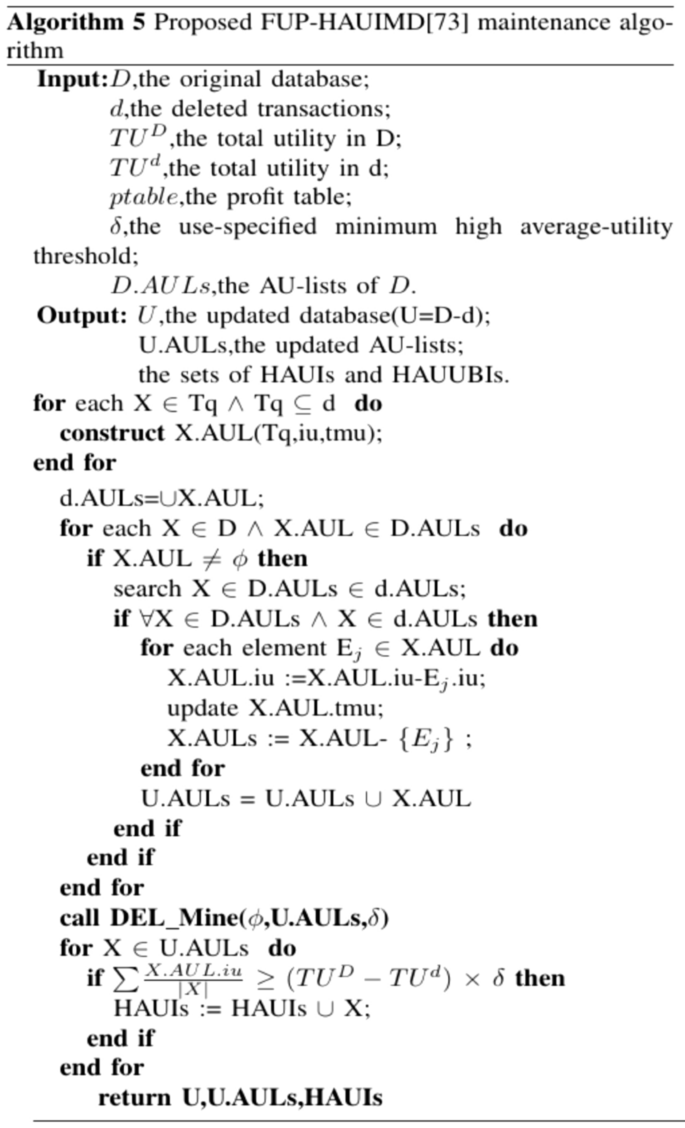
Proposed FUP-HAUIMD maintenance algorithm.
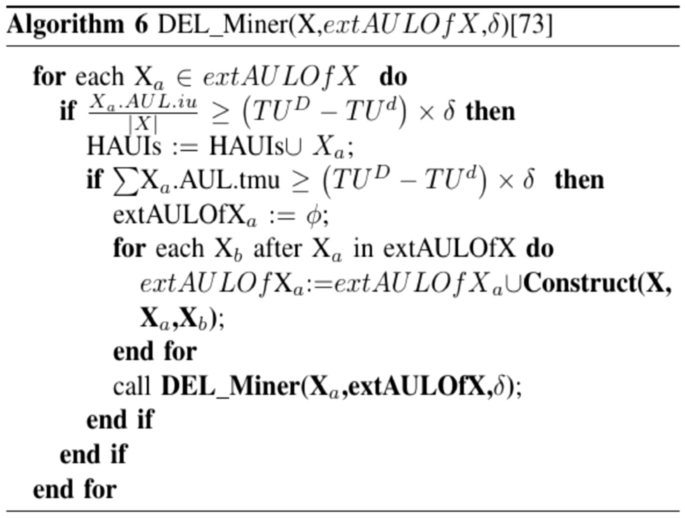
DEL_Miner algorithmin FUP-HAUIMD.
Initially, Algorithm 4 scans the database to identify items from the recently added transactions, creating their AUL structures. Subsequently, the AUL structures originating from the initial database and the added transactions are combined. Upon merging the AUL structures, if the mean utility of an itemset surpasses the revised minimum average utility count, it qualifies as a high average utility itemset. Following this, its supersets are explored through a depth-first search approach based on the enumeration tree. This iterative process continues recursively until no additional tree nodes are generated. The average utility of the chosen itemsets is then computed, culminating in the algorithm's conclusion. The revised patterns are then successfully derived.
The process of Algorithm 5 commences by examining the removed transactions in order to form the AU-lists for 1-itemsets. Subsequently, utilizing these eliminated transactions, the AU-lists within the original database are modified, resulting in the acquisition of the revised AU-lists. Following this, Algorithm 6 is iterated recursively, merging the AU-lists of k-itemsets through a depth-first search strategy based on the enumeration tree structure. Should an itemset satisfy specific criteria, it is designated as an HAUI. In instances where these conditions are not met, the auub value of the itemset is compared to the updated minimum high utility count to ascertain its superset. Additional details regarding the construction function are provided in reference 39 . Subsequent to the retrieval of the revised AU-lists, if the average utility of an itemset equals or exceeds the minimum high utility count, it is identified as an HAUI. Ultimately, the algorithm yields the updated outcomes and concludes its operation.
By default, Algorithm 7 initializes the buffer (buf) to 0 in the first iteration. Next, it computes the safety boundary (f) and the total utility d. Following this, AUL structures for all 1-item sets in d are generated to guarantee the accuracy and entirety of the resulting HAUIs. This approach is logical as, in practice, the number of transactions in d is typically small compared to the original database D. The AUL structures from D and d are then merged through a sub-routine, and the total utility of the combined databases is calculated. The updated AUL structures are managed, and if the auub value of an itemset X does not exceed the upper utility, a HAUI is detected. Subsequently, the supersets of X are evaluated for potential scanning using the recursive PRE-HAUIMI method. The list of HAUIs is updated, with PHAUIs serving as the buffer, while the AUL structures are refreshed for subsequent maintenance.
Aims to mine Highly Associated Unordered Unique Binary Itemsets (HAUI) while simultaneously reducing their search space and the number of database scans, the MHAUIPNU algorithm 81 employs a database with both positive and negative utilities. It introduces a novel, tighter upper-bound model named TUBPN, alongside a list data structure to store the required information for mining HAUI. Furthermore, three new pruning strategies are proposed to further enhance the algorithm’s performance. The first strategy is based on characteristics derived from the TUBPN model, while the other two leverage attributes are associated with items (or itemsets) having negative utilities.
The paper 65 proposes an algorithm called PRE-HAUMI (High Average Utility Itemset Mining with Pre-large Itemset concept) which efficiently mines HAUI from the updated dataset with transaction insertions. The algorithm utilizes the Pre-large Itemset concept to effectively discover HAUIs and maintains an Average Utility List (AUL) structure, which ensures that each transaction is scanned at most once during the maintenance process, as illustrated in Figs. 14 , 15 , 16 , 17 .
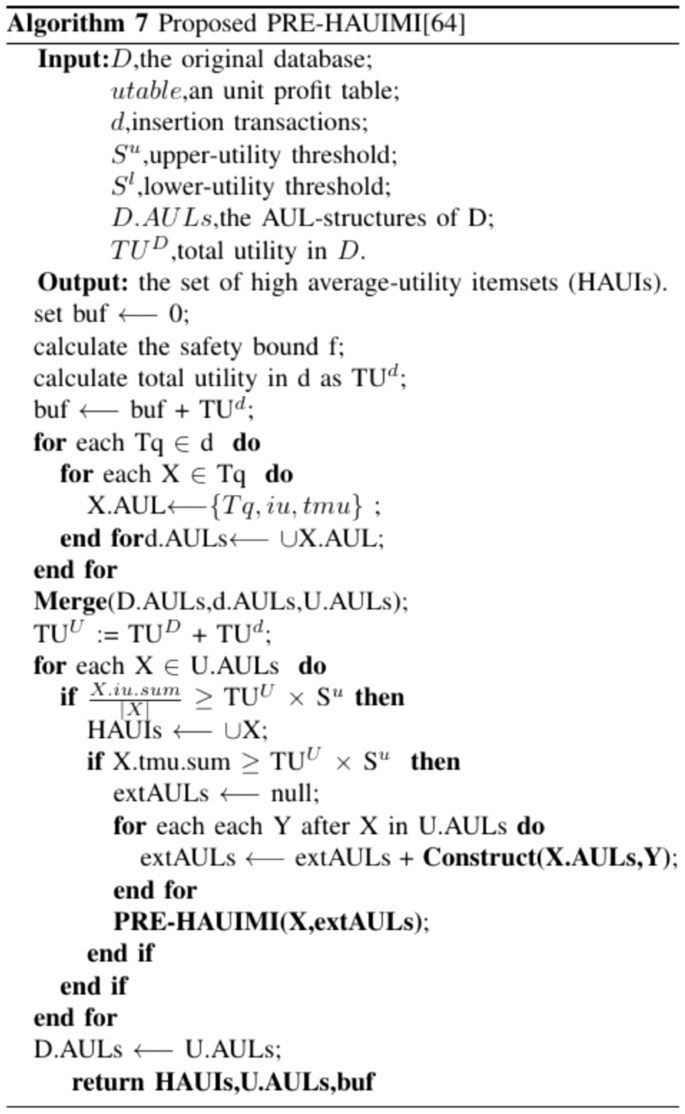
Proposed PRE-HAUIMI.
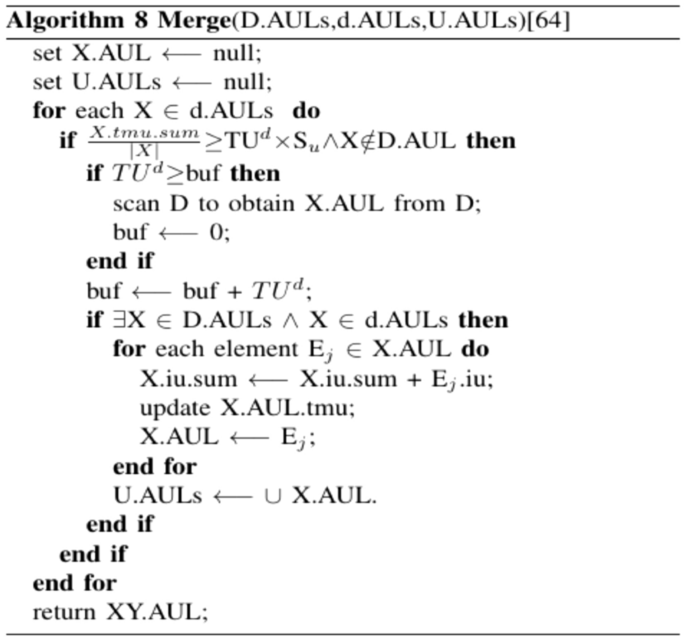
Merge algorithm in PRE-HAUIMI.
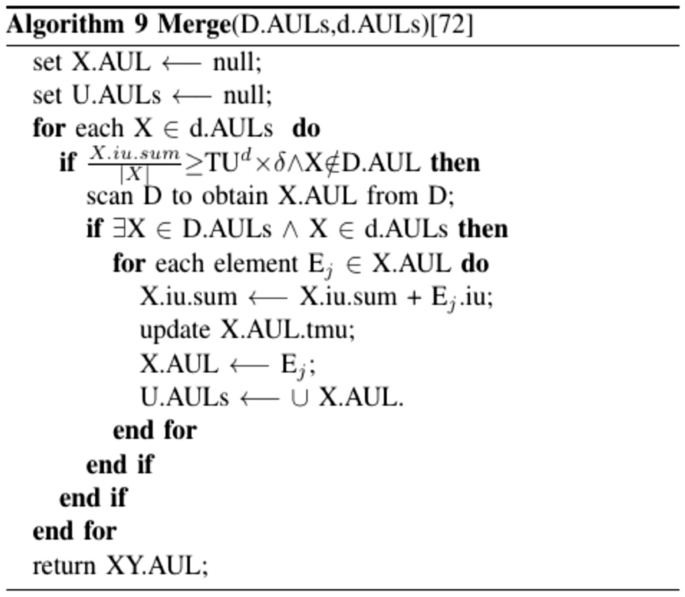
Merge algorithm in FUP-HAUIMI.
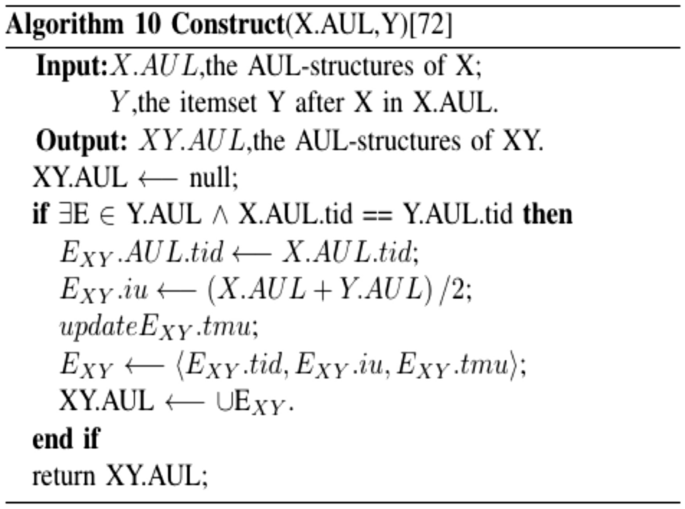
Construct algorithm in FUP-HAUIMI.
In 63 , the paper introduces an efficient algorithm called LIMHAUP, which requires only a single scan of the dataset to extract HAUP from the updated dataset, thereby reducing the cost of performing multiple dataset scans. Additionally, a new structure named HAUP List is introduced, which stores pattern information in a compact manner, eliminating the need for candidate patterns. The algorithm constructs the HAUP List through a single dataset scan and eliminates numerous irrelevant patterns, resulting in reduced execution time and memory consumption during the mining process. Initially, all HAUP Lists are rearranged in real-time order from small to large items,aiming to shrink the search space. Then, organization process is designed to rebuild the HAUP List with an effective sorting order. Ultimately, the algorithm effectively handles new insertions in the incremental dataset.
Unpromising patterns are not removed from the global HAUI list, as they might be HAUPs in a dynamic dataset. This is because the upper-bound pruning strategy can potentially overestimate the average utility. Therefore, an additional pruning strategy called MAU 82 is required to better reduce unpromising patterns. MAU rigorously mines extended patterns. The proposed algorithm demonstrates superior performance in terms of memory consumption, runtime, and scalability compared to the baseline algorithm.
The DMAUP 52 utilizes a damping window framework to extract time-sensitive patterns from incremental databases, aiming to mine high-utility frequent patterns. This method effectively extracts the latest high-utility frequent patterns, thanks to its use of damping factors to adjust item utility values based on their arrival time. Furthermore, to efficiently identify the latest high-utility frequent patterns, the method introduces new data structures known as dA-List, MU, and dUB tables. For incremental data streams, the dA-List undergoes a rebuilding process to incorporate newly added data. Moreover, the mining algorithm employs two pruning techniques, namely damping upper bound and damping maximum average utility, in compliance with the elastic properties of the damping window model. By following these steps, the method can effectively extract the most recent high-utility frequent patterns.
For the purpose of managing a portion of the most recent data using a sliding window model. RHUPS 83 employs an RHU list,a list-based data structure, to swiftly remove the oldest batch data from the global list, thereby displaying real-time updates of the most recent batch data in the global list. Consequently, when encountering dynamic changes in the window, the RHUPS algorithm can promptly mine the most recent efficient utility itemsets from the latest batches within the current window, without generating candidate itemsets. The data structure and mining techniques proposed in this article have the potential to develop into a large-scale machine learning system.
The algorithm 49 utilizes a newly developed list structure, the SHAUP list, to gather information on recent batches. By deleting the oldest batch and introducing a new one after completing the mining process of the current window, the algorithm effectively addresses the most recent stream data. The proposed approach extracts valuable and trustworthy pattern results while considering the length of pat- terns in unlimited data streams. To optimize performance, a new pruning strategy is implemented to reduce the search space, lowering the upper bound by utilizing residual utility. Prior algorithms resulted in numerous candidate patterns and suffered from performance degradation when computing the actual average utility. Conversely, our approach utilizes a list structure to store actual utility information of patterns. Through experimental analysis, results show the SHAUPM algorithm is superior in runtime, memory usage, and scalability on both real-time and synthetic datasets compared to the latest algorithms.
Indexed list based iHAUIM
In the realm of mining high average utility patterns, multiple algorithms have been developed for handling incremental environments. Nevertheless, tree-based algorithms produce potential patterns that necessitate validation through additional database scans. Conversely, list-based algorithms do not generate potential patterns but require numerous comparison operations to identify shared transaction entries with identical identifiers throughout the mining process. These limitations have adverse impacts on algorithms aiming to expediently deliver result patterns. Conversely, indexed list structures 84 , 85 effectively mitigate these shortcomings and have demonstrated superior efficiency compared to tree and list structures in mining high utility patterns.
A novel method for enhancing the efficiency of current average utility driven methods is introduced in the literature as IIMHAUP 86 (Indexed List Based Incremental Mining of High Average Utility Patterns). This approach involves designing a structured list index to facilitate the mining of high average utility patterns in incremental databases. In the IIHAUP algorithm uses three key subroutines to efficiently discover resultant patterns from the initial database ODB.
Summary and discussion
Categories of ihauim.
The previous section provided an overview of three primary categories of iHAUIM algorithms: those utilizing the Apriori algorithm 46 , 59 , 65 , 67 , 69 , those using tree algorithms 45 , 55 , 60 , 75 , and those relying on utility lists 44 , 49 , 52 , 63 , 65 , 78 , 79 , 81 , 83 . These algorithms differ in six key ways:
number of scans of the original database;
strategy for updating and maintaining high average utility itemsets when data changes dynamically;
method for searching for HAUIM;
type of upper bound strategy to reduce candidate itemsets;
type of data structure for maintaining transaction and itemset information (tree-based or utility-list-based);
pruning strategies to reduce search space and speed up mining.
Tables 6 , 7 summarizes these characteristics for the 19 algorithms discussed, noting that not all have been comprehensively studied in the literature. Moving forward, we will delve deeper into these iHUIM algorithms, analyzing and discussing them from the angles of runtime and memory consumption.
Runtime, memory consumption and scalability
The performance of various algorithms for itemset mining has been evaluated, including those proposed by APITPAU Hong et al. 67 and SHAU Yunet al. 61 that utilize tree structures, as well as IHAUPM Lin et al. 45 , FUPHAUIMI Zhang et al. 78 , and LIMHAUP Kim et al. 63 that use utility lists. The results indicate that utility-list-based algorithms exhibit superior performance comparable to Apriori-based methods. Each iHAUIM algorithm has its own limitations, which have been analyzed. Both utility-list-based and tree-structure-based approaches can reduce the number of candidate itemsets generated and the transactions scanned during maintenance. The use of the pre-large concept strategy has been found to be more effective than the FUP concept strategy based on experimental results obtained from FUP-based Wu et al. 44 and PRE-HAUIMI Lin et al. 65 . Lastly, sliding windows and pruning techniques have been shown to enhance the runtime of the algorithm based on the experimental results of LIMHAUP Kim et al. 63 and SHAUPM Lee et al. 49 .
Challenges and future directions
Despite the effectiveness of the existing methods, there are still many future directions that require being explored. Following are some crucial research opportunities associated with the iHAUIM algorithm.
Enhancing the effectiveness of the algorithms
The iHAUIM algorithm can be time-consuming and occupy a large memory while executing, which can raise concerns in real-time dynamic database updates. Even though the current incremental high-utility mining algorithms are faster than their predecessors, there is a scope for improvement. To name a few, compact data structures like trees or lists and more efficient pruning strategies could be developed for mining methods.
Handling the complex dynamic data
Real-life data is highly dynamic, comprising vast and complex datasets used in various fields. Although the principle behind it is straightforward, integrating it into the design of data mining algorithms is complicated. Discovering dynamic data environments is much more difficult and challenging than analyzing static data.
Analyzing the massive amounts of data
Incremental mining of big databases has higher computational costs and memory consumption. Nonetheless, in the era of big data, processing data step-by-step and having a look at earlier analyzed results is indispensable. Research opportunities exist for iHAUIM to process large databases, such as designing parallelized iHUIM algorithms.
Analyzing the runtime
In the experiment, we assessed the runtime of five algorithms across various TH values while maintaining a fixed IR (= 1%), as depicted in Fig. 18 . As depicted in Fig. 18 , it’s clear that the designed PRE-HAUIMI algorithm outperforms the other two algorithms across six datasets.
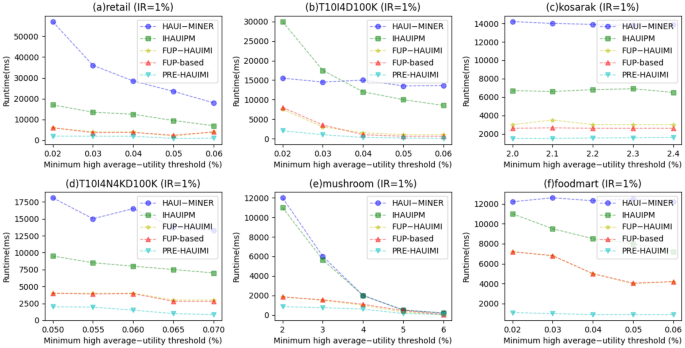
Runtimes for various threshold values.
As the TH value increases, the running time of the five algorithms decreases. This is reasonable because as TH increases, less HAUI is found. Therefore, these five algorithms require less runtime. In addition, it can be seen that for some datasets, such as Fig. 19 a,c,f, the PRE-HAUIMI algorithm designed remains stable for various TH values. HAUI Miner represents the most advanced algorithm for mining HAUI using the auub model, while IHAUIM stands as the most advanced algorithm for incremental HAUIM utilizing tree structures. Consequently, it can be concluded that the designed PRE-HAUUIMI, FUP-HAUIMI, and FUP-based algorithms exhibit strong performance when handling dynamic databases with transaction inserts. The efficiency of the AUL (Average Utility List) structure facilitates streamlined calculations and retrieval of the required HAUI. Experimental evaluations were conducted on six datasets, maintaining fixed TH (Transaction-Utility) values, while varying IR (Item Reduction) values. Figure 19 presents the results derived from these experiments, showcasing the comparative performance of the algorithms.
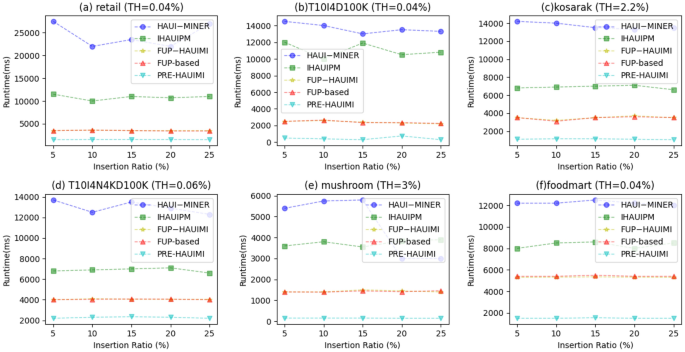
Runtimes for various insertion ratios.
As illustrated in Fig. 19 , the PRE-HAUIMI algorithm demonstrates superior performance compared to both FUP-HAUIMI and FUP-based algorithms. Furthermore, it is observed that the FUP-HAUIMI and FUP-based algorithms still outperform the HAUI Miner and IHAUPM algorithms. The stability of all algorithms, particularly the PRE-HAUIMI algorithm, is evident as the IR (Item Reduction) increases. This indicates that as the IR increases, the performance of all algorithms remains consistent, with the PRE-HAUIMI algorithm consistently displaying the best performance.
Memory usage improvement
We conducted experiments to analyze the memory usage of various algorithms considering fixed IR values and different TH values. The results are depicted in Fig. 20 . Notably, the HAUI Miner algorithm demonstrates superior memory usage performance across datasets (Fig. 20 a,c,e). This can be attributed to the utilization of a utility list structure in HAUI Miner, which efficiently compresses and maintains discovered information. As a result, it usually demands less memory when compared to the IHAUPM algorithm, which utilizes a tree structure for incremental maintenance. Moreover, HAUI Miner doesn’t necessitate holding extra information for maintenance purposes. Instead, when the database size changes, the algorithm rescan the database to acquire updated information, resulting in potential computational costs but lesser memory requirements.
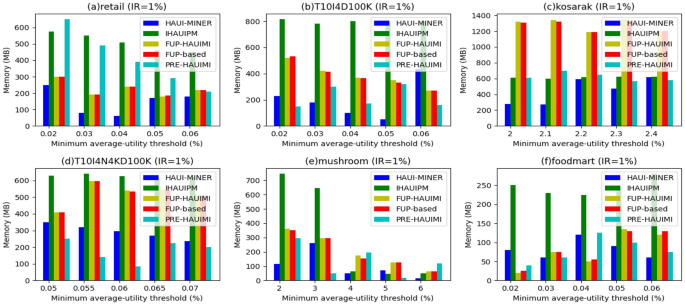
The results of memory usage w.r.t varied thresholds.
Through experiments with fixed IR values and different TH values, we evaluated the memory usage of various algorithms. Figure 21 illustrates the results, showcasing the superior memory usage performance of the HAUI Miner algorithm across datasets 21a, c, and e. This advantage can be attributed to the efficient compression and maintenance of discovered information facilitated by the utility list structure utilized by HAUI Miner. Consequently, it requires less memory compared to the IHAUPM algorithm, which employs a tree structure for incremental maintenance. Additionally, HAUI Miner does not require the retention of additional information for maintenance. Instead, it rescans the database when its size changes, obtaining updated information at the cost of computational overhead but with reduced memory requirements.
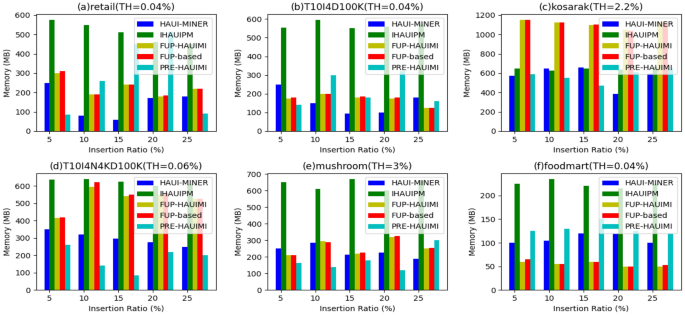
Usage for various insertion ratios.
Number of patterns
The experiment involved evaluating the number of candidate patterns generated during the discovery of actual HAUI. The results, considering different TH values with fixed IR, are presented in Fig. 22 . Observing Fig. 22 , it is evident that, with the exception of Fig. 22 c and d, the proposed PRE-HAUIMI, FUP-HAUIMI, and FUP-based algorithms generate significantly fewer candidate patterns compared to the HAUI Miner and IHAUPM algorithms. Notably, the PRE-HAUIMI algorithm produces the fewest number of candidate patterns.
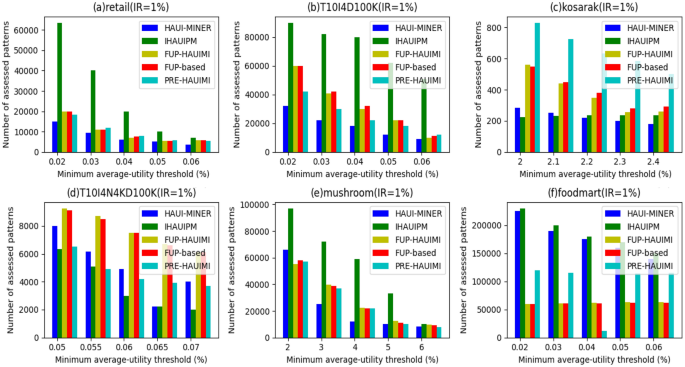
Number of candidate patterns for various threshold values.
This discrepancy can be attributed to the dense nature of the T10I4N4KD100K dataset, where many transactions contain the same maintenance items. As a result, the proposed PRE-HAUIMI, FUP-HAUIMI, and FUP-based algorithms may require additional checks in the enumeration tree to determine if a superset needs to be generated. However, overall, these algorithms still evaluate fewer patterns compared to the other algorithms. This highlights the effectiveness of the AUL structure and adaptive FUP (Frequent Utility Pattern) concept in reducing the incremental mining cost of average utility itemsets. The results, considering different DR (Dependency Ratio) values with fixed TH, are depicted in Fig. 23 .
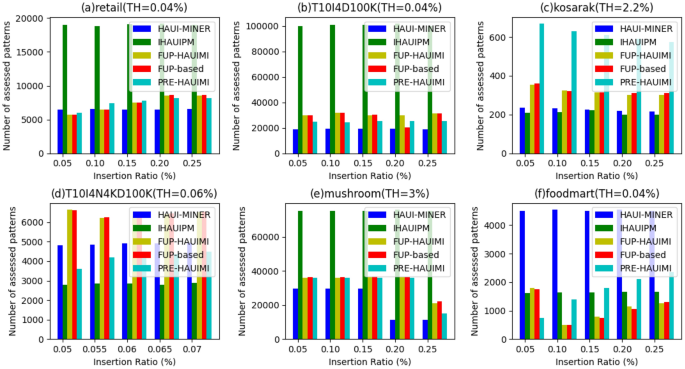
Number of candidate patterns for various insertion ratios.
Similarly, it is observed that in very sparse and dense datasets, such as the ones depicted in Fig. 23 c and d, the PRE-HAUUIMI, FUP-HAUIMI, and FUP-based algorithms may require checking more candidate patterns. However, for other datasets, like those in Fig. 23 a,b,e, these algorithms surpass the performance of the IHAUPM algorithm and even achieve the best outcomes, as demonstrated in Fig. 23 f.
In terms of runtime performance, the proposed PRE-HAUUIMI, FUP-HAUIMI, and FUP-based algorithms outshine the alternative approaches. This can be attributed to the efficiency derived from the FUP concept and the AUL structure, enabling a significant reduction in runtime. Considering the overall results, it can be inferred that while the PRE-HAUUIMI, FUP-HAUIMI, and FUP-based algorithms require additional memory usage and may need to check more candidate patterns in certain scenarios, nevertheless, they consistently achieve higher levels of efficiency and effectiveness in the majority of cases. Among them, the PRE-HAUUIMI algorithm performs the best, with the exception of very sparse datasets with long transactions or extremely dense datasets.
Taking these findings into account, it becomes evident that there are numerous directions that can be explored to further enhance and improve the iHAUIM algorithm, catering to the ever-evolving and dynamic demands of data mining.
A detailed summary of different algorithms for the IHAUIM problem is presented in this paper. We provide an all-inclusive and current analysis of IHAUIM algorithms in dynamic datasets and propose a classification system for the existing IHAUIM techniques. We explore various iHAUIM algorithms for modifying datasets in dynamic data settings, streaming data, and sequential datasets, and evaluate the advantages and drawbacks of the most advanced approaches. Additionally, we identify the significant areas for future research in incremental high-average utility itemset mining.
Data availability
The dataset used in this paper is a publicly available dataset sourced from the internet, and it can be accessed from the following website: https://www.kaggle.com/uciml/pima-indians-diabetes-database .
Han, E.-H., Karypis, G. & Kumar, V. Scalable parallel data mining for association rules. ACM SIGMOD Rec. 26 (2), 277–288 (1997).
Article Google Scholar
Cheung, D. W. & Xiao, Y. Effect of data distribution in parallel mining of associations. Data Min. Knowl. Disc. 3 , 291–314 (1999).
Deng, Z.-H. Mining high occupancy itemsets. Future Gener. Comput. Syst. 102 , 222–229 (2020).
Djenouri, Y., Belhadi, A., Fournier-Viger, P. & Fujita, H. Mining diversified association rules in big datasets: A cluster/gpu/genetic approach. Inf. Sci. 459 , 117–134 (2018).
Article MathSciNet Google Scholar
Fournier-Viger, P., Li, Z., Lin, J.C.-W., Kiran, R. U. & Fujita, H. Efficient algorithms to identify periodic patterns in multiple sequences. Inf. Sci. 489 , 205–226 (2019).
Gan, W., Lin, J.C.-W., Fournier-Viger, P., Chao, H.-C. & Yu, P. S. A survey of parallel sequential pattern mining. ACM Trans. Knowl. Discov. Data 13 (3), 1–34 (2019).
Lee, G. & Yun, U. Performance and characteristic analysis of maximal frequent pattern mining methods using additional factors. Soft Comput. 22 , 4267–4273 (2018).
Lin, J.C.-W., Gan, W., Fournier-Viger, P., Chao, H.-C. & Hong, T.-P. Efficiently mining frequent itemsets with weight and recency constraints. Appl. Intell. 47 , 769–792 (2017).
Lin, J.C.-W., Yang, L., Fournier-Viger, P. & Hong, T.-P. Mining of skyline patterns by considering both frequent and utility constraints. Eng. Appl. Artif. Intell. 77 , 229–238 (2019).
Zou, C., Deng, H., Wan, J., Wang, Z. & Deng, P. Mining and updating association rules based on fuzzy concept lattice. Future Gener. Comput. Syst. 82 , 698–706 (2018).
Cafaro, M., Epicoco, I. & Pulimeno, M. Mining frequent items in unstructured p2p networks. Future Gener. Comput. Syst. 95 , 1–16 (2019).
Han, X. et al. Efficiently mining frequent itemsets on massive data. IEEE Access 7 , 31409–31421 (2019).
Ismail, W. N., Hassan, M. M. & Alsalamah, H. A. Mining of productive periodic frequent patterns for iot data analytics. Future Gener. Comput. Syst. 88 , 512–523 (2018).
Lee, G., Yun, U. & Ryu, K. H. Mining frequent weighted itemsets without storing transaction ids and generating candidates. Int. J. Uncertain. Fuzziness Knowl.-Based Syst. 25 (01), 111–144 (2017).
Lee, G. & Yun, U. An efficient approach for mining frequent sub-graphs with support affinities. In International Conference on Hybrid Information Technology , 525–532 (Springer, 2012).
Abed, S., Abdelaal, A. A., Al-Shayeji, M. H. & Ahmad, I. Sat-based and cp based declarative approaches for top-rank-k closed frequent itemset mining. Int. J. Intell. Syst. 36 (1), 112–151 (2021).
Aggarwal, A. & Toshniwal, D. Frequent pattern mining on time and location aware air quality data. IEEE Access 7 , 98921–98933 (2019).
Song, C., Liu, X., Ge, T. & Ge, Y. Top-k frequent items and item frequency tracking over sliding windows of any size. Inf. Sci. 475 , 100–120 (2019).
Singh, S. & Yassine, A. Mining energy consumption behavior patterns for house-holds in smart grid. IEEE Trans. Emerg. Topics Comput. 7 (3), 404–419 (2017).
Tanbeer, S. K., Hassan, M. M., Almogren, A., Zuair, M. & Jeong, B.-S. Scalable regular pattern mining in evolving body sensor data. Future Gener. Comput. Syst. 75 , 172–186 (2017).
Yun, U., Lee, G. & Yoon, E. Advanced approach of sliding window based erasable pattern mining with list structure of industrial fields. Inf. Sci. 494 , 37–59 (2019).
Yao, H., Xiong, M., Zeng, D. & Gong, J. Mining multiple spatial temporal paths from social media data. Future Gener. Comput. Syst. 87 , 782–791 (2018).
Agrawal, R. et al. Fast algorithms for mining association rules. In Proc. 20th Int. Conf. Very Large Data Bases, VLDB , vol. 1215, 487–499 (1994)
Han, J., Pei, J. & Yin, Y. Mining frequent patterns without candidate generation. ACM Sigmod Rec. 29 (2), 1–12 (2000).
Agrawal, R., Imielínski, T. & Swami, A. Mining association rules between sets of items in large databases. In Proceedings of the 1993 ACM SIGMOD International Conference on Management of Data , 207–216 (1993)
Deng, Z.-H. Diffnodesets: An efficient structure for fast mining frequent itemsets. Appl. Soft Comput. 41 , 214–223 (2016).
Huang, H., Wu, X. & Relue, R. Mining frequent patterns with the pattern tree. New Gener. Comput. 23 , 315–337 (2005).
Lin, C.-W., Hong, T.-P. & Lu, W.-H. Using the structure of pre-large trees to incrementally mine frequent itemsets. New Gener. Comput. 28 , 5–20 (2010).
Krishnamoorthy, S. Pruning strategies for mining high utility itemsets. Expert Syst. Appl. 42 (5), 2371–2381 (2015).
Liu, J., Wang, K. & Fung, B. C. Mining high utility patterns in one phase without generating candidates. IEEE Trans. Knowl. Data Eng. 28 (5), 1245–1257 (2015).
Liu, M. & Qu, J. Mining high utility itemsets without candidate generation. In Proceedings of the 21st ACM International Conference on Information and Knowledge Management , 55–64 (2012)
Liu, Y., Liao, W.-K. & Choudhary, A. A two-phase algorithm for fast discovery of high utility itemsets. In Advances in Knowledge Discovery and Data Mining: 9th Pacific-Asia Conference, PAKDD 2005, Hanoi, Vietnam, May 18–20, 2005. Proceedings vol. 9, 689–695 (Springer, 2005).
Tseng, V. S., Shie, B.-E., Wu, C.-W. & Philip, S. Y. Efficient algorithms for mining high utility itemsets from transactional databases. IEEE Trans. Knowl. Data Eng. 25 (8), 1772–1786 (2012).
Tseng, V.S., Wu, C.-W., Shie, B.-E. & Yu, P.S. Up-growth: an efficient algorithm for high utility itemset mining. In Proceedings of the 16th ACM SIGKDD International Conference on Knowledge Discovery and Data Mining , 253–262 (2010)
Kim, H. et al. Pre-large based high utility pattern mining for transaction insertions in incremental database. Knowl.-Based Syst. 268 , 110478 (2023).
Hong, T.-P., Lee, C.-H. & Wang, S.-L. Effective utility mining with the measure of average utility. Expert Syst. Appl. 38 (7), 8259–8265 (2011).
Lin, C.-W., Hong, T.-P. & Lu, W.-H. An effective tree structure for mining high utility itemsets. Expert Syst. Appl. 38 (6), 7419–7424 (2011).
Lan, G.-C., Hong, T.-P. & Tseng, V. S. Efficiently mining high average-utility itemsets with an improved upper-bound strategy. Int. J. Inf. Technol. Decis. Mak. 11 (05), 1009–1030 (2012).
Lin, J.C.-W. et al. An efficient algorithm to mine high average-utility itemsets. Adv. Eng. Inform. 30 (2), 233–243 (2016).
Cheung, D. W., Han, J., Ng, V. T. & Wong, C. Maintenance of discovered association rules in large databases: An incremental updating technique. In Proceedings of the Twelfth International Conference on Data Engineering , 106–114 (IEEE, 1996).
Hong, T.-P., Lin, C.-W. & Wu, Y.-L. Incrementally fast updated frequent pattern trees. Expert Syst. Appl. 34 (4), 2424–2435 (2008).
Lin, C.-W., Lan, G.-C. & Hong, T.-P. An incremental mining algorithm for high utility itemsets. Expert Syst. Appl. 39 (8), 7173–7180 (2012).
Lin, C.-W., Hong, T.-P. & Lu, W.-H. Maintaining high utility pattern trees in dynamic databases. In 2010 Second International Conference on Computer Engineering and Applications , vol. 1, 304–308 (IEEE, 2010).
Wu, T.-Y., Lin, J.C.-W., Shao, Y., Fournier-Viger, P. & Hong, T.-P. Updating the discovered high average-utility patterns with transaction insertion. In Genetic and Evolutionary Computing: Proceedings of the Eleventh International Conference on Genetic and Evolutionary Computing, November 6–8, 2017, Kaohsiung, Taiwan 11 , 66–73 (Springer, 2018).
Lin, J.C.-W., Ren, S., Fournier-Viger, P., Pan, J.-S. & Hong, T.-P. Efficiently updating the discovered high average-utility itemsets with transaction insertion. Eng. Appl. Artif. Intell. 72 , 136–149 (2018).
Wu, J.M.-T., Teng, Q., Lin, J.C.-W., Yun, U. & Chen, H.-C. Updating high average-utility itemsets with pre-large concept. J. Intell. Fuzzy Syst. 38 (5), 5831–5840 (2020).
Bui, H., Nguyen-Hoang, T.-A., Vo, B., Nguyen, H. & Le, T. A sliding window based approach for mining frequent weighted patterns over data streams. IEEE Access 9 , 56318–56329 (2021).
Cheng, H., Han, M., Zhang, N., Wang, L. & Li, X. Etkds: An efficient algorithm of top-k high utility itemsets mining over data streams under sliding window model. J. Intell. Fuzzy Syst. 41 (2), 3317–3338 (2021).
Lee, C. et al. Efficient approach of sliding window-based high average-utility pattern mining with list structures. Knowl.-Based Syst. 256 , 109702 (2022).
Nam, H., Yun, U., Yoon, E. & Lin, J.C.-W. Efficient approach of recent high utility stream pattern mining with indexed list structure and pruning strategy considering arrival times of transactions. Inf. Sci. 529 , 1–27 (2020).
Nam, H. et al. Efficient approach for damped window-based high utility pattern mining with list structure. IEEE Access 8 , 50958–50968 (2020).
Kim, J. et al. Average utility driven data analytics on damped windows for intelligent systems with data streams. Int. J. Intell. Syst. 36 (10), 5741–5769 (2021).
Li, A., Xu, W., Liu, Z. & Shi, Y. Improved incremental local outlier detection for data streams based on the landmark window model. Knowl. Inf. Syst. 63 (8), 2129–2155 (2021).
Kim, H. et al. Damped sliding based utility oriented pattern mining over stream data. Knowl.-Based Syst. 213 , 106653 (2021).
Yun, U., Kim, D., Yoon, E. & Fujita, H. Damped window based high average utility pattern mining over data streams. Knowl.-Based Syst. 144 , 188–205 (2018).
Hong, T.-P., Wang, C.-Y. & Tao, Y.-H. A new incremental data mining algorithm using pre-large itemsets. Intell. Data Anal. 5 (2), 111–129 (2001).
Kim, S. et al. Efficient approach for mining high-utility patterns on incremental databases with dynamic profits. Knowl.-Based Syst. 282 , 111060 (2023).
Lin, C.-W., Hong, T.-P. & Lu, W.-H. The pre-fufp algorithm for incremental mining. Expert Syst. Appl. 36 (5), 9498–9505 (2009).
Lan, G.-C., Lin, C.-W., Hong, T.-P. & Tseng, V.S. Updating high average-utility itemsets in dynamic databases. In 2011 9th World Congress on Intelligent Control and Automation , 932–936 (IEEE, 2011).
Kim, D. & Yun, U. Efficient algorithm for mining high average-utility itemsets in incremental transaction databases. Appl. Intell. 47 , 114–131 (2017).
Yun, U., Kim, D., Ryang, H., Lee, G. & Lee, K.-M. Mining recent high average utility patterns based on sliding window from stream data. J. Intell. Fuzzy Syst. 30 (6), 3605–3617 (2016).
Singh, K., Kumar, R. & Biswas, B. High average-utility itemsets mining: A survey. Appl. Intell. 52 , 3901–3938 (2022).
Kim, J., Yun, U., Yoon, E., Lin, J.C.-W. & Fournier-Viger, P. One scan based high average-utility pattern mining in static and dynamic databases. Future Gener. Comput. Syst. 111 , 143–158 (2020).
Wu, R. & He, Z. Top-k high average-utility itemsets mining with effective pruning strategies. Appl. Intell. 48 (10), 3429–3445 (2018).
Lin, J.C.-W., Pirouz, M., Djenouri, Y., Cheng, C.-F. & Ahmed, U. Incrementally updating the high average-utility patterns with pre-large concept. Appl. Intell. 50 , 3788–3807 (2020).
Wang, L. & Wang, S. Huil-tn & hui-tn: Mining high utility itemsets based on pattern-growth. Plos one 16 (3), 0248349 (2021).
Google Scholar
Hong, T.-P., Lee, C.-H. & Wang, S.-L. An incremental mining algorithm for high average-utility itemsets. In 2009 10th International Symposium on Pervasive Systems, Algorithms, and Networks 421–425 (IEEE, 2009).
Hong, T.-P., Lee, C.-H. & Wang, S.-L. Mining high average-utility itemsets. In 2009 IEEE International Conference on Systems, Man and Cybernetics , 2526–2530 (IEEE, 2009).
Wu, J.M.-T., Teng, Q., Tayeb, S. & Lin, J.C.-W. Dynamic maintenance model for high average-utility pattern mining with deletion operation. Appl. Intell. 52 (15), 17012–17025 (2022).
Wu, J. M. T. et al. Analytics of high average-utility patterns in the industrial internet of things. Appl. Intell. 52 (6), 6450–6463 (2022).
Ahmed, C. F., Tanbeer, S. K., Jeong, B.-S. & Choi, H.-J. Interactive mining of high utility patterns over data streams. Expert Syst. Appl. 39 (15), 11979–11991 (2012).
Chen, H., Shu, L., Xia, J. & Deng, Q. Mining frequent patterns in a varying-size sliding window of online transactional data streams. Inf. Sci. 215 , 15–36 (2012).
Lee, G., Yun, U. & Ryu, K. H. Sliding window based weighted maximal frequent pattern mining over data streams. Expert Syst. Appl. 41 (2), 694–708 (2014).
Tanbeer, S. K., Ahmed, C. F., Jeong, B.-S. & Lee, Y.-K. Sliding window-based frequent pattern mining over data streams. Inf. Sci. 179 (22), 3843–3865 (2009).
Phuong, N. & Duy, N. D. Constructing a new algorithm for high average utility itemsets mining. In 2017 International Conference on System Science and Engineering (ICSSE) , 273–278 (IEEE, 2017).
Lu, T., Vo, B., Nguyen, H. T. & Hong, T.-P. A new method for mining high average utility itemsets. In Computer Information Systems and Industrial Management: 13th IFIP TC8 International Conference, CISIM 2014, Ho Chi Minh City, Vietnam, November 5–7, 2014. Proceedings 14 , 33–42 (Springer, 2014).
Koh, J.-L. & Shieh, S.-F. An efficient approach for maintaining association rules based on adjusting fp-tree structures. In International Conference on Database Systems for Advanced Applications , 417–424 (Springer, 2004).
Zhang, B., Lin, J.C.-W., Shao, Y., Fournier-Viger, P. & Djenouri, Y. Maintenance of discovered high average-utility itemsets in dynamic databases. Appl. Sci. 8 (5), 769 (2018).
Lin, J.C.-W., Shao, Y., Fournier-Viger, P., Djenouri, Y. & Guo, X. Maintenance algorithm for high average-utility itemsets with transaction deletion. Appl. Intell. 48 , 3691–3706 (2018).
Cheung, D. W., Lee, S. D. & Kao, B. A general incremental technique for maintaining discovered association rules. In Database Systems For Advanced Applications’ 97 , 185–194 (World Scientific, 1997)
Yildirim, I. & Celik, M. Mining high-average utility itemsets with positive and negative external utilities. New Gener. Comput. 38 , 153–186 (2020).
Yun, U. & Kim, D. Mining of high average-utility itemsets using novel list structure and pruning strategy. Future Gener. Comput. Syst. 68 , 346–360 (2017).
Baek, Y. et al. Rhups: Mining recent high utility patterns with sliding window–based arrival time control over data streams. ACM Trans. Intell. Syst. Technol. 12 (2), 1–27 (2021).
Ryang, H. & Yun, U. Indexed list-based high utility pattern mining with utility upper-bound reduction and pattern combination techniques. Knowl. Inf. Syst. 51 , 627–659 (2017).
Yun, U. et al. Efficient approach for incremental high utility pattern mining with indexed list structure. Future Gener. Comput. Syst. 95 , 221–239 (2019).
Kim, H. et al. Efficient approach of high average utility pattern mining with indexed list-based structure in dynamic environments. Inf. Sci. 657 , 119924 (2024).
Lin, J.C.-W., Ren, S., Fournier-Viger, P. & Hong, T.-P. Ehaupm: Efficient high average-utility pattern mining with tighter upper bounds. IEEE Access 5 , 12927–12940 (2017).
Kim, D. & Yun, U. Mining high utility itemsets based on the time decaying model. Intell. Data Anal. 20 (5), 1157–1180 (2016).
Yun, U., Lee, G. & Yoon, E. Efficient high utility pattern mining for establishing manufacturing plans with sliding window control. IEEE Trans. Ind. Electron. 64 (9), 7239–7249 (2017).
Kim, H. et al. Efficient list based mining of high average utility patterns with maximum average pruning strategies. Inf. Sci. 543 , 85–105 (2021).
Download references
Acknowledgements
The subject is sponsored by the National Natural Science Foundation of P. R. China (No.61976120, No. 62102194, No. 62102196). Natural Science Foundation of Inner Mongolia Autonomous Region of China (No. 2022MS06010), Natural Science Research Project of Department of Education of Guizhou Province (No. QJJ2022015). Inner Mongolia Autonomous Region Higher Education Institutions Science and Technology Research Project (NJSY23004). Scientific Research Project of Baotou Teachers' College (BSYKY2021-ZZ01, BSYHY202212, BSYHY202211, BSJG23Z07).
Author information
These authors contributed equally: Jing Chen and Shengyi Yang.
Authors and Affiliations
School of Internet of Things, Nanjing University of Posts and Telecommunications, Nanjing, 210023, Jiangsu, China
Jing Chen & Hongjun Zhang
School of Physics and Mechatronic Engineering, Guizhou Minzu University, Guiyang, 550025, Guizhou, China
Shengyi Yang
Baotou Teachers’ College of Inner Mongolia University of Science and Technology, Baotou, 014030, Inner Mongolia, China
Jing Chen & Aijun Liu
School of Information Science and Technology, Nantong University, Nantong, 226019, Jiangsu, China
Weiping Ding
School of Computer Science, Nanjing University of Posts and Telecommunications, Nanjing, 210023, Jiangsu, China
School of Computer and Software, Nanjing Vocational University of Industry Technology, Nanjing, 210003, China
You can also search for this author in PubMed Google Scholar
Contributions
Chen Jing: writing original draft, review response, commentary, revision. Yang Shengyi: writing original draft, review response, commentary, revision. Weiping Ding and Li Peng: conceptualization, funding acquisition, methodology, supervision, review. Liu Aijun: writing original draft, commentary. Zhang Hongjun: validations. Tian Li: validation, conceptualization.
Corresponding authors
Correspondence to Weiping Ding or Aijun Liu .
Ethics declarations
Competing interests.
The authors declare no competing interests.
Additional information
Publisher's note.
Springer Nature remains neutral with regard to jurisdictional claims in published maps and institutional affiliations.
Rights and permissions
Open Access This article is licensed under a Creative Commons Attribution 4.0 International License, which permits use, sharing, adaptation, distribution and reproduction in any medium or format, as long as you give appropriate credit to the original author(s) and the source, provide a link to the Creative Commons licence, and indicate if changes were made. The images or other third party material in this article are included in the article's Creative Commons licence, unless indicated otherwise in a credit line to the material. If material is not included in the article's Creative Commons licence and your intended use is not permitted by statutory regulation or exceeds the permitted use, you will need to obtain permission directly from the copyright holder. To view a copy of this licence, visit http://creativecommons.org/licenses/by/4.0/ .
Reprints and permissions
About this article
Cite this article.
Chen, J., Yang, S., Ding, W. et al. Incremental high average-utility itemset mining: survey and challenges. Sci Rep 14 , 9924 (2024). https://doi.org/10.1038/s41598-024-60279-0
Download citation
Received : 27 December 2023
Accepted : 21 April 2024
Published : 30 April 2024
DOI : https://doi.org/10.1038/s41598-024-60279-0
Share this article
Anyone you share the following link with will be able to read this content:
Sorry, a shareable link is not currently available for this article.
Provided by the Springer Nature SharedIt content-sharing initiative
- Dynamic data mining
- High Utility Item Mining
- High Average Utility Item Mining
- Pattern mining
By submitting a comment you agree to abide by our Terms and Community Guidelines . If you find something abusive or that does not comply with our terms or guidelines please flag it as inappropriate.
Quick links
- Explore articles by subject
- Guide to authors
- Editorial policies
Sign up for the Nature Briefing: AI and Robotics newsletter — what matters in AI and robotics research, free to your inbox weekly.

Two Decades of Academic Service-Learning in Chinese Higher Education: A Review of Research Literature
- Published: 01 May 2024
Cite this article
- Liu Hong ORCID: orcid.org/0000-0002-2250-773X 1 ,
- Yang-yang Wan 1 ,
- Wan-ting Yang 2 ,
- Zhi-jian Gong 1 ,
- Xiao-yue Hu 1 &
- Gaoming Ma 3
Service-learning is globally recognized as an impactful pedagogical approach in higher education. This study reviews literature from the past two decades on university course-based, credit-bearing academic service-learning in the Chinese mainland. It reveals a steady growth in service-learning courses across all regions of the Chinese mainland since 2006, with fifty-two courses documented in the academic literature by 2023. The courses span various disciplines and service populations and have shown positive impacts on university students’ cognitive skills, peer and community connectedness, development of self, disciplinary, and career identities, as well as social responsibility. The study observes the presence of heterogeneous research designs, incomplete reporting of course information, variability in writing styles, structure, and content across different journals and disciplines, indicating an early stage in the field’s development. This underscores the need for systematic documentation of course designs and processes and outcome evaluation with research rigor. The paper discusses the implications for the local development of service-learning practices and the advancement of research in this domain in light of the growing global interest in this educational approach.
This is a preview of subscription content, log in via an institution to check access.
Access this article
Price includes VAT (Russian Federation)
Instant access to the full article PDF.
Rent this article via DeepDyve
Institutional subscriptions
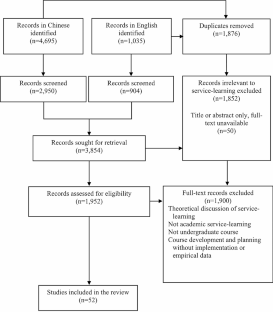
Data availability
The data that support the findings of this study are available from the corresponding author upon reasonable request.
Anorico, H. C. (2019). Service-learning in the Philippines: The university of Santo Thomas’ national service training program. Gateways: International Journal of Community Research and Engagement , 12 (1). https://doi.org/10.5130/ijcre.v12i1.5944 .
Antonak, R. F., & Harth, R. (1994). Psychometric analysis and revision of the mental retardation attitude inventory. Mental Retardation , 32 (4), 272–280. https://doi.org/10.1007/s003300000751 .
Article Google Scholar
Bennett, D., Sunderland, N., Bartleet, B. L., & Power, A. (2016). Implementing and sustaining higher education service-learning initiatives: Revisiting Young et al.’s organizational tactics. Journal of Experiential Education , 39 (2), 145–163. https://doi.org/10.1177/1053825916629987 .
Billig, S. H. (2009). Does quality really matter: Testing the new K–12 service-learning standards for quality practice. In B. E. Moely, S. H. Billig, & B. A. Holland (Eds.), Creating our identities in service-learning and community engagement (pp. 131–158). Information Age.
Braun, V., & Clarke, V. (2012). Thematic analysis. In H. Cooper, P. M. Camic, D. L. Long, A. T. Panter, D. Rindskopf, & K. J. Sher (Eds.), APA handbook of research methods in psychology, vol 2: Research designs: Quantitative, qualitative, neuropsychological, and biological (pp. 57–71). American Psychological Association. https://doi.org/10.1037/13620-004 .
Bringle, R. G., & Hatcher, J. A. (2000). Institutionalization of service learning in higher education. Journal of Higher Education , 71 (3), 273–290.
Butin, D. W. (2010). Service-learning in theory and practice: The future of community engagement in higher education . Palgrave Macmillan US. https://doi.org/10.1057/9780230106154 .
Celio, C. I., Durlak, J., & Dymnicki, A. (2011). A meta-analysis of the impact of service-learning on students. Journal of Experiential Education , 34 (2), 19. https://doi.org/10.1177/105382591103400205 .
Cen, X. Y., Zhang, H. C., Li, D. Q., Li, R. C., & Zheng, Q. R. (2007). Zai Shequ laonian fuwu zhong chengzhang [Growing in community elderly services]. Shehui Gongzuo Xiabanyue (Lilun) , 12 , 43–44.
Google Scholar
Chen, L. G. (2012). Kecheng daoxiang de shuzihua fuwu xuexi sheji yanjiu [Master’s thesis, Jiangsu Normal University].
Chen, H. L., & Zhang, Y. (2021). Xianshang fuwu xuexi shijian: Yiqing zhong de shehui gongzuo shiwu jiaoyu chengxiao yanjiu [Online service learning practice: A study on the effectiveness of practical social work education during the pandemic]. Shehui Jianshe , 8 (4), 24–35.
Choi, Y., Han, J., & Kim, H. (2023). Exploring key service-learning experiences that promote students’ learning in higher education. Asia Pacific Education Review . https://doi.org/10.1007/s12564-023-09833-5 .
Collins, J., Clark, E., Chau, C., & Pignataro, R. (2019). Impact of an international service learning experience in India for DPT students: Short- and long-term benefits. Journal of Allied Health , 48 (1), 22–30.
Compare, C., & Albanesi, C. (2023). Belief, attitude and critical understanding. A systematic review of social justice in service-learning experiences. Journal of Community & Applied Social Psychology , 33 (2), 332–355. https://doi.org/10.1002/casp.2639 .
Conway, J. M., Amel, E. L., & Gerwien, D. P. (2009). Teaching and learning in the social context: A meta-analysis of service learning’s effects on academic, personal, social, and citizenship outcomes. Teaching of Psychology , 36 (4), 233–245. https://doi.org/10.1080/00986280903172969 .
Dai, X. (2022). Fuwu Xuexi Zai shehui gongzuo kecheng shixi zhong de yunyong ——yi Ge’an Shehui Gongzuo kecheng weili [Application of service learning in social work course internship — case Social Work course as an example]. Zhongguo Shehui Gongzuo , (13) , 30–32.
Dai, K. Y., Hong, L., & Xu, K. (2023). Ti Xian Liang xing Yi Du De Jisuanjilei Fuwu Xuexi Kecheng De Sheji Yu shijian [Design and practice of computer service learning courses reflecting two sexes and one degree]. Ruanjian Daokan , 1–6.
Eyler, J., & Giles, D. E. Jr. (1997). The importance of program quality in service-learning. In A. S. Waterman (Ed.), Service-learning: Applications from the research (pp. 57–76). Lawrence Erlbaum Associates.
Eyler, J., Giles Jr, D. E., Stenson, C. M., & Gray, C. J. (2001). At a glance: What we know about the effects of service-learning on college students, faculty, institutions and communities, 1993–2000. Higher Education , 139.
Faulconer, E. (2021). eService-learning: A decade of research in undergraduate online service–learning. American Journal of Distance Education , 35 (2), 100–117. https://doi.org/10.1080/08923647.2020.1849941 .
Fu, J., & Li, Y. L. (2016). Fuwu Xuexi Dui daxuesheng shehui chengshudu de yingxiang [The impact of service learning on the social maturity of college students]. Guangxi Jiaoyu , 7 , 48–50.
Furco, A. (2007). Institutionalising service-learning in higher education. Higher Education and Civic Engagement: International perspectives . Routledge.
Gao, Z. M. (2016). Fuwu—Xuexi Bentuhua De Tansuo Yu sikao [Exploration and thinking on the localization of service-learning]. Gaojiao Xuekan , 17 , 24–25.
Gonzales, A. D., Harmon, K. S., & Fenn, N. E. (2020). Perceptions of service learning in pharmacy education: A systematic review. Currents in Pharmacy Teaching and Learning , 12 (9), 1150–1161. https://doi.org/10.1016/j.cptl.2020.04.005 .
Gordon, C. S., Pink, M. A., Rosing, H., & Mizzi, S. (2022). A systematic meta-analysis and meta-synthesis of the impact of service-learning programs on university students’ empathy. Educational Research Review , 37 , 100490. https://doi.org/10.1016/j.edurev.2022.100490 .
Guo, Y. Y. (2015). Fuwu-Xuexi xing jiaoxue fangfa zai peiyang shehui gongzuo xuesheng zhuanye nengli fangmian de yunyong yanjiu [Master’s thesis, Yunnan University].
Hammond, C. (1994). Integrating service and academic study: Faculty motivation and satisfaction in Michigan higher education. Michigan Journal of Community Service Learning , 1 (1), 21–28.
Han, Y. M. (2018). Oral history of Sian leprosy rehabilitation village: A service-learning project conducted by South China Normal University. In C. M. Hok-ka, A. C. Cheung-ming, A. L. Cheng, & F. M. Mui-fong (Eds.), Service-learning as a new paradigm in higher education of China (pp. 85–92). Michigan State University.
Harte, A., Persyn, A., Louro, J., de Smet, L., Harvey, K., Laabs, K., Minotti, S., Naujanyte, P., Porkola, R., & Semenski, S. (2019). & others. European student reflections and perspectives on service learning. In Embedding Service Learning in European Higher Education (pp. 196–209). Routledge.
Haski-Leventhal, D., GrÖNlund, H., Holmes, K., Meijs, L. C. P. M., Cnaan, R. A., Handy, F., Brudney, J. L., Hustinx, L., Kang, C., Kassam, M., Pessi, A. B., Ranade, B., Smith, K. A., Yamauchi, N., & Zrinscak, S. (2010). Service-learning: Findings from a 14-Nation study. Journal of Nonprofit & Public Sector Marketing , 22 (3), 161–179. https://doi.org/10.1080/10495141003702332 .
Hawes, J. K., Johnson, R., Payne, L., Ley, C., Grady, C. A., Domenech, J., Evich, C. D., Kanach, A., Koeppen, A., Roe, K., Caprio, A., Puente Castro, J., LeMaster, P., & Blatchley, E. R. (2021). Global service-learning: A systematic review of principles and practices. International Journal of Research on Service-Learning and Community Engagement , 9 (1). https://doi.org/10.37333/001c.31383 .
He, X. J. (2012). Fuwu Xuexi: Shehui gongzuo zhuanye nengli peiyang de guocheng—yici shehui xingzheng kecheng jiaoxue de shijian [Service learning: The process of cultivating social work professional ability—a practice of Social Administration course teaching]. Shehui Gongzuo , 1 , 41–43.
He, L., Zhu, X. Y., & Zhao, S. L. (2015). Fuwu Xuexi Linian Xia De Ketang Xuexi Yu Shequ fuwu—Yi Ertong Quanli Yu Baohu Kecheng Weili [Classroom learning and community service under the concept of service learning—taking children’s rights and Protection course as an example]. Zhongguo Qingnian Shehui Kexue , 34 (4), 93–97.
Holland, B. (1997). Analyzing institutional commitment to service: A model of key organizational factors. Michigan Journal of Community Service Learning , 4 , 30–41.
Hong, L. (2019). An alternative blueprint? Institutionalizing service-learning in a comprehensive university in China. Proceedings of the 3rd International Conference on Service-Learning (pp. 193–196). ICSL 2019
Hong, L., Peng, S. Q., Xu, K., & Chen, Y. (2020). Fuwu xuexi kecheng de sheji: Jiyu sheji siwei de shijian kuangjia [Service-Learning curriculum design: A practical framework based on design thinking]. Fudan Education Forum , 18 (5), 63–70
Hong, Q. N., Pluye, P., Bujold, M., & Wassef, M. (2017). Convergent and sequential synthesis designs: Implications for conducting and reporting systematic reviews of qualitative and quantitative evidence. Systematic Reviews , 6 (1), 61. https://doi.org/10.1186/s13643-017-0454-2 .
Honnet, E. P., & Poulsen, S. J. (1989). Wingspread special report: Principles of good practice for combining service and learning . The Johnson Foundation.
Huang, X. R., & Ye, L. H. (2022). Tiyanshi Gongyi De Bentuhua Tansuo Yu Shijian—Yi Huaqiao Daxue Tongshi Kecheng Qiaoai Zhiyuan Fuwu Xiangmu Shequ Fuwu Xuexi Wei Li [Exploration and practice of localizing experiential philanthropy — with the example of Qiao Ai Volunteer Service Community Service Learning of Huaqiao University’s general education course]. Xibu Suzhi Jiaoyu , 8 (4), 4–6.
ICSL 2019 (The 3rd International Conference on Service-Learning). Proceedings of the 3rd International Conference on Service-Learning, Hong Kong Polytechnic University, 10–12 (January, 2019). http://hdl.handle.net/10397/81823 .
Lemieux, C. M., & Allen, P. D. (2007). Service learning in social work education: The state of knowledge, pedagogical practicalities, and practice conundrums. Journal of Social Work Education , 43 (2), 309–326. https://doi.org/10.5175/JSWE.2007.200500548 .
Li, Y. H. (2008). Shehui Gongzuo Shiwu Kecheng Fuwu Xuexi Jiaoxue Moshi tansuo—Yi Laonian Shehui Gongzuo fuwu xuexi xiangmu weili [Exploration of service learning teaching model in social work practical courses: A case study of Elderly Social Work service learning project]. Shehui Gongzuo Xiabanyue (Lilun) , 3 , 18–21.
Li, Z., Wang, Q. Q., & Qi, B. C. (2011). Dangdai daxuesheng zhuanye rentongdu de xianzhuang ji duice yanjiu [Current situation and countermeasures research on contemporary college students’ professional identity]. Gaojiao Tansuo , 2 , 131–136.
Li, Y., Yao, M., Song, F., Fu, J., & Chen, X. (2018). Building a just world: The effects of service-learning on social justice beliefs of Chinese college students. Educational Psychology , 39 (5), 591–616. https://doi.org/10.1080/01443410.2018.1530733 .
Li, H., McDougle, L. M., & Gupta, A. (2019). Experiential philanthropy in China. Journal of Public Affairs Education , 26 (2), 205–227. https://doi.org/10.1080/15236803.2019.1667185 .
Li, L. W., Hou, J. W., & Wei, G. J. (2023). Fuwuxing Xuexi Zai Erke Hulixue kecheng zhong de xingdong yanjiu [Action research on service learning in Pediatric nursing course]. Quanke Huli , 21 (2), 286–288.
Lin, R., & Chen, Y. H. (2022). Jiyu jiji xinlixue de gongyi kecheng shijian de ge’an yanjiu [Case study on the practice of philanthropy courses based on positive psychology]. Yangzhou Daxue Xuebao (Gaojiao Yanjiuban) , 25 (4), 103–110.
Lin, P., & Huang, W. (2013). Fuwu Xuexi Linian Yingyong Yu wuli shifan jiaoyu de shijian [Application of service learning concept in physics normal education practice]. Hezhou Xueyuan Xuebao , 29 (1), 130–132.
Lin, Y., & Jiang, A. L. (2004). Huli zhuanye daxuesheng zizhu xuexi nengli ceping liangbiao de yanzhi [Development of the self-directed learning ability assessment scale for nursing college students]. Jiefangjun huli Zazhi , 6 , 1–4.
Liu, N., Yang, Y. Y., Dong, Z. G., Zhang, Y. Y., & Xu, Y. (2015). Husheng canjia Laonian Hulixue kecheng fuwuxing xuexi de tiyan ji fansi [Nursing students’ experience and reflection on service learning in geriatric nursing course]. Jiefangjun Huli Zazhi , 32 (7), 6–9.
Liu, J. E., Fan, G. H., Xu, L. S., Lin, X. Y., Zhang, A. Q., Huang, W. H., Zheng, S. Y., Zeng, Y., & Yang, M. H. (2021). Shantou Daxue Yixueyuan Yizhe Zhi Xin (HEART) yixue renwen jiaoyu Moshi Yu Shijian—Yi Yixuesheng Gongyi Zhongzi Peiyu Jihua Wei shijiao [Shantou University Medical College’s HEART medical humanities education model and practice — from the perspective of Medical Student Public Welfare seed cultivation plan]. Yixue Jiaoyu Guanli , 7 (4), 366–372.
Lo, K. W. K., Ngai, G., Chan, S. C. F., & Kwan, K. (2022). How students’ motivation and learning experience affect their service-learning outcomes: A structural equation modeling analysis. Frontiers in Psychology , 13 , 825902. https://doi.org/10.3389/fpsyg.2022.825902 .
Long, L., Wan, Y., Zou, K., Shen, Z. Q., Hao, Y., Zhao, X. Y., Feng, W. T., & Li, J. Y. (2022). Yi Fuwu Xuexi Wei Zhidao Sixiang De Kecheng Sheji Ji Fei Biaozhunhua Kaohe Moshi Tansuo—Yi Yufang Yixue Yu Gonggong Weisheng Fuwu Xuexi Shijian Kecheng Wei Li [Exploration of course design and non-standard assessment model guided by service learning — taking the Practice Course of Preventive Medicine and Public Health Service Learning as an example]. Xiandai Yufang Yixue , 49 (22), 4221–4224.
Ma, Y. J. (2020). Fuwu Xuexi Yanjiu: Yi Xinguan feiyan yiqing zhong Tiyanshi Cishan jiaoxue shijian weili [Service learning research: Taking Experiential Philanthropy teaching practice in COVID-19 as an example]. Gaodeng Jiaoyu Yanjiu , 41 (9), 93–102.
Marco-Gardoqui, M., Eizaguirre, A., & García-Feijoo, M. (2020). The impact of service-learning methodology on business schools’ students worldwide: A systematic literature review. Plos One , 15 (12), e0244389. https://doi.org/10.1371/journal.pone.0244389 .
Mashino, T. (2022). Not for democracy? Service-learning in Japanese higher education as compared to the United States. Educational Studies in Japan , 16 (0), 59–70. https://doi.org/10.7571/esjkyoiku.16.59 .
McIlrath, L., Aramburuzabala, P., & Opazo, H. (2019). Developing a culture of civic engagement through service learning within higher education in Europe. In P. Aramburuzabala, L. McIlrath, & H. Opazo (Eds.), Embedding Service Learning in European Higher Education: Developing a culture of Civic Engagement (pp. 69–80). Routledge.
Meijs, L. C., Maas, S. A., & Aramburuzabala, P. (2019). Institutionalisation of service learning in European higher education. In P. Aramburuzabala, L. McIlrath, & H. Opazo (Eds.), Embedding service learning in European higher education (pp. 213–229). Routledge.
Moely, B. E., & Ilustre, V. (2014). The impact of service-learning course characteristics on university students’ learning outcomes. Michigan Journal of Community Service Learning , 21 (1), 5–16. https://doi.org/10.3382/ps.0680287 .
Moely, B. E., Mercer, S. H., Ilustre, V., Miron, D., & McFarland, M. (2002). Psychometric properties and correlates of the Civic attitudes and skills Questionnaire (CASQ): A measure of students’ attitudes related to service-learning. Michigan Journal of Community Service Learning , 8 , 15–26. http://hdl.handle.net/2027/spo.3239521.0008.202 .
Mouton, J., & Wildschut, L. (2005). Service learning in South Africa: Lessons learnt through systematic evaluation. Acta Academica, sup-3 , 116–150.
Natarajarathinam, M., Qiu, S., & Lu, W. (2021). Community engagement in engineering education: A systematic literature review. Journal of Engineering Education , 110 (4), 1049–1077. https://doi.org/10.1002/jee.20424 .
Peng, H. M., Chen, X. F., & Gao, Y. X. (2009). Fuwu Xuexi: Qingnian Zhiyuan Fuwu Yu Daxue Jiaoyu Zhenghe Moshi yanjiu [Service learning: A study on the integration of youth volunteer service and university education]. Zhongguo Qingnian Yanjiu , 4 , 87–91.
Pérez-Ordás, R., Nuviala, A., Grao-Cruces, A., & Fernández-Martínez, A. (2021). Implementing service-learning programs in physical education; teacher education as teaching and learning models for all the agents involved: A systematic review. International Journal of Environmental Research and Public Health , 18 (2), 669. https://doi.org/10.3390/ijerph18020669 .
Preradović, N. M., & Mažeikienė, N. (2019). Service learning in post-communist countries: Lithuania and Croatia. Embedding Service Learning in European Higher Education (pp. 180–195). Routledge.
Qiu, X. (2013). Lun Fuwu Xuexi Moshi Zai Shehui Xingzheng shijian jiaoxue zhong de yunyong [On the application of service learning model in the practical teaching of Social Administration]. Heihe Xuekan , 5 , 125–126.
Queiruga-Dios, M., Santos Sánchez, M. J., Queiruga-Dios, M. Á., Castellanos, A., P. M., & Queiruga-Dios, A. (2021). Assessment methods for service-learning projects in engineering in higher education: A systematic review. Frontiers in Psychology , 12 , 629231. https://doi.org/10.3389/fpsyg.2021.629231 .
Salam, M., Awang Iskandar, D. N., Ibrahim, D. H. A., & Farooq, M. S. (2019). Service learning in higher education: A systematic literature review. Asia Pacific Education Review , 20 (4), 573–593. https://doi.org/10.1007/s12564-019-09580-6 .
Sandelowski, M., Voils, C. I., & Barroso, J. (2006). Defining and designing mixed research synthesis studies. Research in the Schools , 13 (1), 29–40.
Shek, D. T. L., Siu, A. M. H., & Lee, T. Y. (2007). The Chinese positive youth development scale: A validation study. Research on Social Work Practice , 17 (3), 380–391. https://doi.org/10.1177/1049731506296196 .
Shek, D. T. L., Yang, Z., Ma, C. M. S., & Chai, C. W. Y. (2021). Subjective outcome evaluation of service-learning by the service recipients: Scale development, normative profiles and predictors. Child Indicators Research , 14 (1), 411–434. https://doi.org/10.1007/s12187-020-09765-1 .
Sigmon, R. (1979). Service-learning: Three principles. Synergist , 8 (1), 9–11.
Song, F. N., & Chen, X. L. (2015). Fuwu Xuexi Dui daxuesheng zhuanye rentong Yu Zhiye Chengshudu De Yingxiang yanjiu [The influence of service learning on college students’ professional identity and vocational maturity]. Guangxi Daxue Xuebao (Zhexue Shehui Kexueban) , 37 (6), 115–119.
Sotelino-Losada, A., Arbués-Radigales, E., García-Docampo, L., & González-Geraldo, J. L. (2021). Service-learning in Europe. Dimensions and understanding from academic publication. Frontiers in Education , 6 , 604825. https://doi.org/10.3389/feduc.2021.604825 .
Stetten, N. E., Black, E. W., Edwards, M., Schaefer, N., & Blue, A. V. (2019). Interprofessional service learning experiences among health professional students: A systematic search and review of learning outcomes. Journal of Interprofessional Education & Practice , 15 , 60–69. https://doi.org/10.1016/j.xjep.2019.02.002 .
Stewart, T., & Wubbena, Z. C. (2015). A systematic review of service-learning in medical education: 1998–2012. Teaching and Learning in Medicine: An International Journal , 27 (2), 115–122. https://doi.org/10.1080/10401334.2015.1011647 .
Sun, Z. W. (2017). Ziran kexue kecheng zhiru fuwu xuexi de moshi tansuo [Exploring the model of integrating service learning into natural science courses]. Presented at the China Association for Higher Education Quality Education Research Branch 2017 Annual Meeting and the Sixth University Quality Education High-level Forum, Nanjing.
Tan, S. Y., & Soo, S. H. J. (2020). Service-learning and the development of student teachers in Singapore. Asia Pacific Journal of Education , 40 (2), 263–276. https://doi.org/10.1080/02188791.2019.1671809 .
Thomas, J., & Harden, A. (2008). Methods for the thematic synthesis of qualitative research in systematic reviews. BMC Medical Research Methodology , 8 (1), 45. https://doi.org/10.1186/1471-2288-8-45 .
Tijsma, G., Hilverda, F., Scheffelaar, A., Alders, S., Schoonmade, L., Blignaut, N., & Zweekhorst, M. (2020). Becoming productive 21st century citizens: A systematic review uncovering design principles for integrating community service learning into higher education courses. Educational Research , 62 (4), 390–413. https://doi.org/10.1080/00131881.2020.1836987 .
Vergés Bosch, N., Freude, L., & Camps Calvet, C. (2021). Service learning with a gender perspective: Reconnecting service learning with feminist research and pedagogy in sociology. Teaching Sociology , 49 (2), 136–149. https://doi.org/10.1177/0092055X21993465 .
Wang, Y. (2006). Fuwu Xuexi Chutan [Preliminary exploration of service learning]. Shehui Gongzuo , 11 , 51–53.
Wang, J., Zhao, F., & Ge, R. Q. (2011). Fuwu Xuexi Yingyong Yu Gaoxiao Yingyu Jiaoxue Chutan [A preliminary study on the application of service learning in university English teaching]. Sheke Zongheng , 26 (3), 174–175.
Wang, C., Yao, M. L., Li, Y. L., & Guo, F. F. (2014). Fuwu Xuexi Zhong daxuesheng de teshu ertong rongheguan de bianhua: Yingyong butong daoxiang de fansi zhijia [Changes in college students’ inclusion of special children in service learning: Applying different reflection scaffolds]. Zhongguo Teshu Jiaoyu , 8 , 3–8.
Wang, S. S., Liu, Y. L., Lin, C. X., Lu, X., Huang, Z., & Guo, W. (2019a). Fuwu Xuexi Moshi Zai Shequ hulixue jiaoxue zhong de yingyong [Application of service learning model in community nursing teaching]. Hulixue Zazhi , 34 (17), 60–62.
Wang, X. L., Chen, H. Z., & Su, J. (2019b). Fuwuxing Xuexi Zai laonian hulixue shijian jiaoxue Zhong De Tansuo Yu yingyong [Exploration and application of service learning in geriatric nursing practical teaching]. Weisheng Zhiye Jiaoyu , 37 (19), 67–68.
Wang, Y., Hu, Y., Xiang, D., Fu, Y., & Hong, L. (2019). Application of social work supervision model in university service-learning courses in Mainland China: A qualitative study on students’ participation. 7th Asia-pacific regional conference on service-learning . Singapore
Warren, J. L. (2012). Does service-learning increase student learning? A meta-analysis. Michigan Journal of Community Service Learning , 18 (2), 56–61.
Wei, C. (2022). Fuwu Xuexi: Shehui Gongzuo Jiaoxue De Fansi Shijian Yu guanxixing cunzai [Service learning: Reflection on the practice of social work teaching and its relational existence]. Yinshan Xuekan , 35 (1), 95–106.
Weigert, K. M. (1998). Academic service learning: Its meaning and relevance. New Directions for Teaching & Learning , 1998 (73), 3–10. https://doi.org/10.1002/tl.7301 .
Wu, B. (2020). Fuwu Xuexi jiaoxuefa de yici xingdong tansuo [An action exploration of service learning teaching method]. Jiaoyu Xueshu Yuekan , 1 , 95–105.
Wu, L. P., Chen, X. Q., & Xu, J. Z. (2015). Yi Kecheng Wei zaiti de fuwuxing xuexi moshi tansuo [Exploration of service learning model with course as carrier]. Xiandai Yiyao Weisheng , 31 (15), 2378–2380.
Xing, J., & Ma, C. H. K. (2010). Service-learning in Asia: Curricular models and practices . Hong Kong University.
Xu, W. (2015). Fuwu Xuexi Yunyong Yu daxuesheng zhiyuan fuwu Zhong De Chengxiao chutan—Yi Zhuhai Mou gaoxiao zhiyuan huodong kecheng weili [A preliminary exploration of the effectiveness of service learning in college students’ volunteer service—taking the volunteer activity course of a university in Zhuhai as an example]. Qingshaonian Yanjiu Yu Shijian , 30 (3), 77–81.
Xu, L. L. (2022). Jiyu fuwu xuexi de Laonian Shehui Gongzuo kecheng shijian jiaoxue moshi tanxi [Exploration of practical teaching mode of Elderly Social Work course based on service learning]. Sudu , 21 , 175–177.
Yao, D., & Xiao, X. L. (2017). Zai shequ ketang xunlian zizhu xuexi nengli—jianzhuxue benke fuwu xuexi kecheng tansuo [Training self-study ability in community classroom—exploration of undergraduate service learning course in architecture]. Zhongguo Jianzhu Jiaoyu , Z1 , 112–117.
Yao, J., Pan, A. Q., & Yang, Y. (2017). Suzhi Jiaoyu Yu Tongshi Jiaoyu Lilun Dui Zhiyuan Fuwu Xuexi Kecheng De qifa—Yi tianjin mou gaoxiao weili [The enlightenment of quality education and general education theory to volunteer service learning course—taking a university in Tianjin as an example]. Xibu Suzhi Jiaoyu , 3 (6), 83–85.
Yao, Q. L., Hu, H., & Zheng, T. Y. (2018). Husheng Dui Zhongyi huli fuwu xuexi shijian jiaoxue moshi de zhenji tiyan [Nursing students’ true experience of traditional Chinese nursing service learning practical teaching model]. Huli Xuebao , 25 (14), 1–4.
Yao, J., Liu, F., Lu, D. D., Guo, Y., & Li, X. W. (2019). Zhuoyue Yixue Jiaoyu Beijing Xia fuwuxing xuexi zai fuchanke hulixue jiaoxue zhong de yingyong [Application of service learning in obstetrics and gynecology nursing teaching under the background of excellent medical education]. Xiandai Yangsheng , 4 , 188–189.
Yorio, P. L., & Ye, F. (2012). A meta-analysis on the effects of service-learning on the social, personal, and cognitive outcomes of learning. Academy of Management Learning & Education , 11 (1), 9–27. https://doi.org/10.5465/amle.2010.0072 .
Yu, X. L., Zhang, J. H., & Liu, S. J. (2020). Fuwu Xuexi Moshi Zai Shehui Gongzuo Rencai Shiwu Nengli Peiyang Zhong De Yunyong—Yi Shequ Gongzuo kecheng weili [Application of service learning model in cultivating practical abilities of social workers — taking Community Work course as an example]. Jingji Yanjiu Daokan , 21 , 145–146.
Yue, S. T., & Liu, L. M. (2019). Fuwuxing xuexi shijiao xia laonian huli shijian jiaoxue Gaige De Tansuo Yu sikao [Exploration and thinking of geriatric nursing practical teaching reform from the perspective of service learning]. Quanke Huli , 17 (24), 3071–3073.
Zhang, C. (2017). Fuwu Xuexi Quxiang De Shehui Gongzuo Jiaoxue Moshi tansuo—Yi Xuexiao Shehui Gongzuo shiwulei kecheng weili [Exploration of social work teaching model oriented by service learning—taking School Social Work practical course as an example]. Tonghua Shifan Xueyuan Xuebao , 38 (12), 96–98.
Zhang, L. J. (2018). Jiyu fuwu xuexi de Laonian Shehui Gongzuo shijian jiaoxue gaige tansuo [Exploration of the practical teaching reform of Elderly Social Work based on service learning]. Gaojiao Luntan , 5 , 30–32.
Zhang, X., & Fan, Y. M. (2014). Jiyu Fuwu Xuexi Moshi De Shengming Jiaoyu Kecheng Sheji Yu shishi—Yi Shantou daxue shengming jiaoyu kecheng weili [Design and implementation of life education curriculum based on service learning model—taking Shantou University life education curriculum as an example]. Shandong Qingnian , 3 , 9–10.
Zhang, Y., & Li, H. B. (2018). Jiyu fuwu—xuexi de gaoxiao sixiang zhengzhi lilunke shijian jiaoxue moshi yanjiu [Study on the practical teaching model of ideological and political theory courses in colleges and universities based on service—learning]. Dangdai Zhongguo Makesi Zhuyi Pinglun , 2 , 179–185.
Zhang, Z. Y., Rong, Y., & Guan, Y. J. (2006). Zhongguo daxuesheng zhiye chengshudu liangbiao de Xindu Yu xiaodu [Reliability and validity of the measurement scale of vocational maturity for Chinese college students]. Xinan Daxue Xuebao (Renwen Shehui Kexueban) , 5 , 1–6. https://doi.org/10.13718/j.cnki.xdsk.2006.05.002 .
Zhang, R. X., Hao, S. J., Yuan, Y. M., Wang, J. Q., Zhang, H. L., Fang, J. H., Xia, Z. Y., & Wang, J. (2022). Jiyu Xinxihua Huanjing de fuwuxing xuexi zai zhongyi hulixue kecheng shijian jiaoxue zhong de yingyong [Application of service learning in the practical teaching of traditional Chinese medicine nursing course based on information environment]. Anhui Zhongyiyao Daxue Xuebao , 41 (3), 97–99.
Zhao, R., & Lilly, A. G. (2022). The role of state mobilization for volunteerism in China. Nonprofit and Voluntary Sector Quarterly , 51 (6), 1304–1323. https://doi.org/10.1177/08997640211057458 .
Zhou, Z., Mu, L., Qi, S., & Shek, D. T. L. (2022). Service leadership through serving minority adolescents in rural China using a rural version of a positive youth development program. Applied Research in Quality of Life , 1–23. https://doi.org/10.1007/s11482-022-10098-0 .
Zhu, J. G. (2020). Fuwu Xuexi: Shehui gongzuo jiaoyu de tongshihua [Service learning: The generalization of social work education]. Xuehai , 1 , 113–118.
Zhu, Y. Z., Ma, L. Y., & Cao, X. Y. (2020). Nixiang Sheji Kuangjia Xia De Fuwu Xuexi Kecheng Shishi Tansuo—Yi Huiben Yingyu Jiaoxue Shijian kecheng weili [Exploration of service learning course implementation under the framework of reverse design — taking the Picture Book English teaching practice course as an example]. Gaojiao Xuekan , 21 , 106–108.
Zhu, Z., Xing, W., Liang, Y., Hong, L., & Hu, Y. (2022). Nursing students’ experiences with service learning: A qualitative systematic review and meta-synthesis. Nurse Education Today , 108 , 105206. https://doi.org/10.1016/j.nedt.2021.105206 .
Zhuang, J. Y., Hu, R., Chen, Y. M., & Shen, L. (2016). Fuwuxing xuexi zai shequ hulixue shijian jiaoxue zhong de yingyong [The application of service learning in community nursing practice teaching]. Zhonghua Huli Jiaoyu , 13 (4), 287–290.
Download references
This research received no funding.
Author information
Authors and affiliations.
School of Social Development and Public Policy, Fudan University, Shanghai, China
Liu Hong, Yang-yang Wan, Zhi-jian Gong & Xiao-yue Hu
Lexium Centre for Innovation and Public Purpose, Shanghai, China
Wan-ting Yang
Center of Social Welfare and Governance, Department of Social Welfare and Risk Management, School of Public Affairs, Zhejiang University, Zijingang Campus, No. 866 Yuhangtang Road, City of Hangzhou, Zhejiang Province, China
You can also search for this author in PubMed Google Scholar
Corresponding author
Correspondence to Gaoming Ma .
Ethics declarations
Conflict of interest.
All authors declare no conflict of interest.
Additional information
Publisher’s note.
Springer Nature remains neutral with regard to jurisdictional claims in published maps and institutional affiliations.
Rights and permissions
Springer Nature or its licensor (e.g. a society or other partner) holds exclusive rights to this article under a publishing agreement with the author(s) or other rightsholder(s); author self-archiving of the accepted manuscript version of this article is solely governed by the terms of such publishing agreement and applicable law.
Reprints and permissions
About this article
Hong, L., Wan, Yy., Yang, Wt. et al. Two Decades of Academic Service-Learning in Chinese Higher Education: A Review of Research Literature. Applied Research Quality Life (2024). https://doi.org/10.1007/s11482-024-10318-9
Download citation
Received : 31 July 2023
Accepted : 19 April 2024
Published : 01 May 2024
DOI : https://doi.org/10.1007/s11482-024-10318-9
Share this article
Anyone you share the following link with will be able to read this content:
Sorry, a shareable link is not currently available for this article.
Provided by the Springer Nature SharedIt content-sharing initiative
- Academic service-learning
- Literature review
- Higher education
- Positive youth development
- Find a journal
- Publish with us
- Track your research
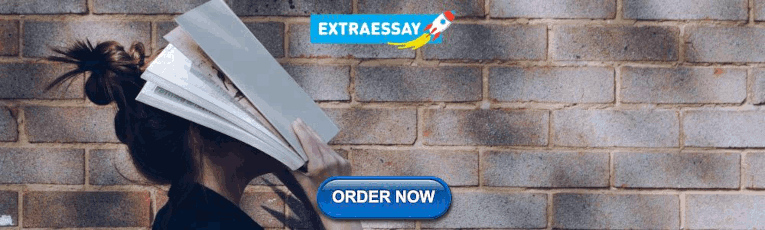
IMAGES
VIDEO
COMMENTS
Table of contents. Step 1: Restate the problem. Step 2: Sum up the paper. Step 3: Discuss the implications. Research paper conclusion examples. Frequently asked questions about research paper conclusions.
The conclusion in a research paper is the final section, where you need to summarize your research, presenting the key findings and insights derived from your study. Check out this article on how to write a conclusion for a research paper, with examples.
The conclusion is intended to help the reader understand why your research should matter to them after they have finished reading the paper. A conclusion is not merely a summary of the main topics covered or a re-statement of your research problem, but a synthesis of key points derived from the findings of your study and, if applicable, where you recommend new areas for future research.
A research paper conclusion is the final section of a research paper that summarizes the key findings, significance, and implications of the research. It is the writer's opportunity to synthesize the information presented in the paper, draw conclusions, and make recommendations for future research or actions. ...
Writing a Conclusion. A conclusion is an important part of the paper; it provides closure for the reader while reminding the reader of the contents and importance of the paper. It accomplishes this by stepping back from the specifics in order to view the bigger picture of the document. In other words, it is reminding the reader of the main ...
The conclusion pushes beyond the boundaries of the prompt and allows you to consider broader issues, make new connections, and elaborate on the significance of your findings. Your conclusion should make your readers glad they read your paper. Your conclusion gives your reader something to take away that will help them see things differently or ...
For example, while the conclusion to a STEM paper could focus on questions for further study, the conclusion of a literature paper could include a quotation from your central text that can now be understood differently in light of what has been discussed in the paper. ... In a short paper—even a research paper—you don't need to provide an ...
Begin your conclusion by restating your thesis statement in a way that is slightly different from the wording used in the introduction. Avoid presenting new information or evidence in your conclusion. Just summarize the main points and arguments of your essay and keep this part as concise as possible. Remember that you've already covered the ...
Step 2: Summarize and reflect on your research. Step 3: Make future recommendations. Step 4: Emphasize your contributions to your field. Step 5: Wrap up your thesis or dissertation. Full conclusion example. Conclusion checklist. Other interesting articles. Frequently asked questions about conclusion sections.
Begin with a clear statement of the principal findings. This will reinforce the main take-away for the reader and set up the rest of the discussion. Explain why the outcomes of your study are important to the reader. Discuss the implications of your findings realistically based on previous literature, highlighting both the strengths and ...
Step 1: Restate the problem. Always begin by restating the research problem in the conclusion of a research paper. This serves to remind the reader of your hypothesis and refresh them on the main point of the paper. When restating the problem, take care to avoid using exactly the same words you employed earlier in the paper.
The point of a conclusion to a research paper is to summarize your argument for the reader and, perhaps, to call the reader to action if needed. 5. Make a call to action when appropriate. If and when needed, you can state to your readers that there is a need for further research on your paper's topic.
The conclusion of a research paper has several key objectives. It should: Restate your research problem addressed in the introduction section. Summarize your main arguments, important findings, and broader implications. Synthesize key takeaways from your study. The specific content in the conclusion depends on whether your paper presents the ...
The conclusion is where you describe the consequences of your arguments by justifying to your readers why your arguments matter (Hamilton College, 2014). Derntl (2014) also describes conclusion as the counterpart of the introduction. Using the Hourglass Model (Swales, 1993) as a visual reference, Derntl describes conclusion as the part of the ...
Step 1: Return to your thesis. To begin your conclusion, signal that the essay is coming to an end by returning to your overall argument. Don't just repeat your thesis statement —instead, try to rephrase your argument in a way that shows how it has been developed since the introduction. Example: Returning to the thesis.
What to include in the conclusion. On the other hand, you may pick up some text from the introduction, especially from its end, namely, the objectives. Here is a made-up example of a research paper conclusion: "The highest yield among the plots that had received different doses of fertilizers was from the one that was supplied 25 kg each of ...
Research paper conclusion examples. Below, we've created basic templates showing the key parts of a research paper conclusion. Keep in mind that the length of your conclusion will depend on the length of your paper. The order of the parts may vary, too; these templates only demonstrate how to tie them together. 1. Empirical research paper ...
Conclusions. Conclusions wrap up what you have been discussing in your paper. After moving from general to specific information in the introduction and body paragraphs, your conclusion should begin pulling back into more general information that restates the main points of your argument. Conclusions may also call for action or overview future ...
A conclusion is the final paragraph of a research paper and serves to help the reader understand why your research should matter to them. The conclusion of a conclusion should: Restate your topic and why it is important. Restate your thesis/claim. Address opposing viewpoints and explain why readers should align with your position.
To set up future work by suggesting ideas for further research or questions to explore. To recast, or further explain, the thesis or purpose statement in a way that benefits from the improved understanding provided in the paper. When you take time to think about and write a strong, purposeful conclusion, you are investing in the overall quality ...
The conclusion is intended to help the reader understand why your research should matter to them after they have finished reading the paper. A conclusion is not merely a summary of your points or a re-statement of your research problem but a synthesis of key points. For most essays, one well-developed paragraph is sufficient for a conclusion ...
How to Write the Discussion Section of a Research Paper? The Discussion section in a research paper plays a vital role in interpreting findings and formulating a conclusion.Given below are the main components of the discussion section:
Conclusion. The first category of resources (human resources) requires long-term planning and the deployment of emergency expansion measures. ... First, the research object of this paper focuses on the specific point of ICU healthcare resource demand prediction, aiming to improve the rate of critical care patient treatment. However, past ...
In conclusion, we explore potential future directions, research opportunities, and various extensions of the iHAUIM algorithm. ... this research paper presents a new classification system that ...
Table of contents. Step 1: Introduce your topic. Step 2: Describe the background. Step 3: Establish your research problem. Step 4: Specify your objective (s) Step 5: Map out your paper. Research paper introduction examples. Frequently asked questions about the research paper introduction.
Given the broad and exploratory nature of the research questions, the diverse ASL practices rooted in different disciplines, the heterogeneity of research methods employed, and the varied outcomes reported in this field, we decided to utilize a narrative review approach rather than a typical systematic review and meta-analytic process for synthesizing the qualitative and quantitative evidence.
4. Conclusion. Understanding the food security status and its determinants is required to improve food security through providing policy makers evidence to design effective food security interventions. The study found that there is a significant difference in household food security among beneficiary, graduated and non-beneficiary both in FIES ...
In conclusion, it can be stated that the use of thermoplastic starch in TPS/PLA blends accelerates the biodegradation of PLA as a slowly biodegradable polymer. While the addition of citric acid offers significant advantages for TPS/PLA blends, further research is needed to optimize the formulation and processing parameters to achieve the ...