Advertisement
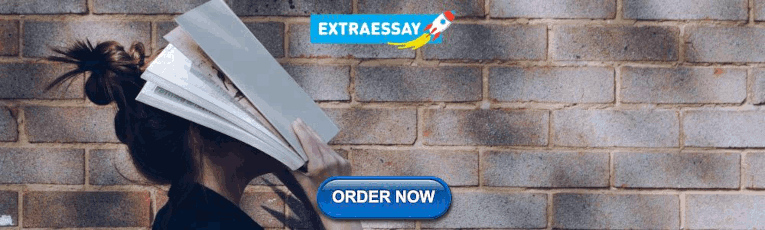
Genetics and Epigenetics in Obesity: What Do We Know so Far?
- Open access
- Published: 11 October 2023
- Volume 12 , pages 482–501, ( 2023 )
Cite this article
You have full access to this open access article
- Maria Keller 1 , 2 na1 ,
- Stina Ingrid Alice Svensson 3 na1 ,
- Kerstin Rohde-Zimmermann 1 , 2 ,
- Peter Kovacs 1 &
- Yvonne Böttcher 3 , 4
2128 Accesses
Explore all metrics
Purpose of Review
Enormous progress has been made in understanding the genetic architecture of obesity and the correlation of epigenetic marks with obesity and related traits. This review highlights current research and its challenges in genetics and epigenetics of obesity.
Recent Findings
Recent progress in genetics of polygenic traits, particularly represented by genome-wide association studies, led to the discovery of hundreds of genetic variants associated with obesity, which allows constructing polygenic risk scores (PGS). In addition, epigenome-wide association studies helped identifying novel targets and methylation sites being important in the pathophysiology of obesity and which are essential for the generation of methylation risk scores (MRS). Despite their great potential for predicting the individual risk for obesity, the use of PGS and MRS remains challenging.
Future research will likely discover more loci being involved in obesity, which will contribute to better understanding of the complex etiology of human obesity. The ultimate goal from a clinical perspective will be generating highly robust and accurate prediction scores allowing clinicians to predict obesity as well as individual responses to body weight loss-specific life-style interventions.
Similar content being viewed by others
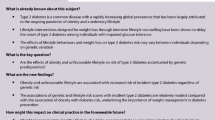
Obesity, unfavourable lifestyle and genetic risk of type 2 diabetes: a case-cohort study
Theresia M. Schnurr, Hermina Jakupović, … Tuomas O. Kilpeläinen
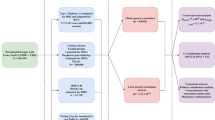
Shared genetic architecture between periodontal disease and type 2 diabetes: a large scale genome-wide cross-trait analysis
Kevin Chun Hei Wu, Lin Liu, … Bernard Man Yung Cheung
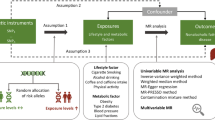
Lifestyle and metabolic factors for nonalcoholic fatty liver disease: Mendelian randomization study
Shuai Yuan, Jie Chen, … Susanna C. Larsson
Avoid common mistakes on your manuscript.
Introduction
Obesity rates are steadily increasing [ 1 ] and represent a major public health thread worldwide. Being an important cause for concomitant metabolic co-morbidities such as type 2 diabetes, dyslipidemia, cardiometabolic diseases including coronary artery diseases, stroke and hypertension as well as for some types of cancers [ 2 ], obesity substantially reduces life expectancy [ 3 ]. As summarised by the World Obesity Atlas 2023 [ 4 ], about 988 million people (aged > 5 years) worldwide were affected with obesity (BMI ≥ 30 kg/m 2 ) in 2020, which is estimated to dramatically increase by 2035 to 1.914 billion. This corresponds to a proportional increase of the population with obesity from 14% in 2020 to 24% in 2035, clearly illustrating the need to prevent and treat obesity.
Obesity is a multifactorial disease being governed by both genetics and environmental factors originating from a rather “obesogenic environment” such as sedentary lifestyle with reduced energy expenditure and high calorie diet intake. The existence of a genetic background in obesity is undisputable and first evidence was provided by family [ 5 , 6 , 7 , 8 ], twin [ 9 , 10 , 11 ] and adoption [ 12 ] studies that have clearly estimated heritability rates for BMI between 40 and 70%. Genome-wide association studies (GWAS) have to a large extent contributed to an improved understanding of the genetic architecture of common obesity and have provided hundreds of novel risk variants [ 13 , 14 , 15 ]. However, although significant advances have been made in describing the mechanistic circuitry for a least some of these genetic variants [ 16 , 17 ], identifying novel risk variants in general precedes the biological and functional understanding of how these variants act in a certain target tissue in order to increase body weight. Furthermore, the variability of BMI attributed to genetic variation is still poorly explained [ 15 ]. The major challenge here is a combination of genetics with environmental factors such as energy intake, physical activity, smoking, but also gene–gene interactions. These interactions may introduce additional inter-individual variability, illustrating the highly dynamic and complex etiology underlying the pathophysiology of obesity.
Epigenetic analyses have therefore been largely accelerated during the last years with epigenome-wide association studies (EWAS) dominating the field. Numerous genes and novel CpG sites were identified conferring changes in methylation profiles in obesity [ 18 ]. However, causal interferences in obesity are still under debate, yet a few studies implicate a causal role of obesity in inducing changes in methylation levels [ 19 •, 20 ].
To translate the bench-side generated knowledge into a clinical day life and to generate a useful tool helping to predict obesity (e.g. based on BMI changes), significant effort was put in designing polygenic risk scores and more recently, also methylation risk scores. These scores represent a weighted combination of several genetic variants or methylated CpG sites at many different positions across the human genome. However, so far, the use of such scores is rather limited as reliable prediction is not yet possible or to a substantial part inaccurate. Taken together, enormous progress has been made in understanding the genetic architecture of obesity and the correlation of epigenetic marks with obesity and related traits. This review aims at highlighting current research and its challenges in genetics and epigenetics of obesity.
Genetic Background of Common Polygenic Obesity
Lessons from monogenic obesity have significantly contributed to our general knowledge on genetics and physiology of body weight regulation. However, non-syndromic monogenic obesity affects only about 5% of the population with obesity [ 21 ]. About 95% of the individuals with obesity develop common polygenic obesity, which is multifactorial and assessing the heritability of polygenic obesity is still one of the major challenges, despite recent advances in genetics of obesity. Genome-wide strategies including linkage and genome-wide association studies (GWAS), which are hypothesis-free per se have been of paramount importance in discovering novel genes involved in the complex etiology of human obesity.
Identifying Novel Genetic Markers by Using Genome Wide Approaches—Linkage Analyses
Genome-wide linkage analyses allow testing for co-segregation of polymorphic genetic markers with phenotypic traits/disease in families, trios or sibling studies. The approach proved to be enormously efficient in discovering genetic variants in monogenic forms of obesity. However, when employed to discovery efforts for underlying genetic markers in polygenic forms of obesity, linkage analyses had only a marginal impact, as most of the identified susceptibility loci for obesity could not be replicated and confirmed in subsequent studies or fine mapped to identify the causal variants affecting the disease. This is most likely to be attributed to small sample sizes in the performed linkage analyses as well as to the poor coverage of genetic variation in tested genomes. One of the very few promising genes discovered in a linkage study was the ectonucleotide pyrophosphatase/phosphodiesterase 1 gene ( ENPP1 ), located on chromosome 6q. The gene was initially discovered to be related to childhood obesity and associated traits by genome-wide linkage analyses [ 22 ] and one of its haplotypes further replicated in independent childhood cohorts as well as adults [ 23 , 24 ]. It is of note however, that despite some inconsistencies in replication efforts, a large meta-analysis including 24,324 individuals clearly supported the potential role of the ENPP1 Q121 variant in the pathophysiology of obesity [ 25 ].
Identifying Novel Genetic Markers by Using Genome Wide Approaches—GWA Studies
Whilst the above-described approaches like candidate gene and genome-wide linkage studies showed only marginal success in discoveries of susceptibility genes for common polygenic obesity, prominent advances in molecular biology, including high-throughput genotyping techniques, have enabled researchers to use GWAS to identify novel genetic loci associated with human obesity. This has indeed led to a dramatic increase of until then unknown genetic variants associated with obesity. Started with the discovery of genetic variants in the fat mass and obesity-associated gene ( FTO ) reported in 2007 [ 26 , 27 ], so far, more than 1000 loci carrying variants including single nucleotide polymorphisms (SNPs) significantly associated with measures of obesity like BMI have been identified in meta-analyses of large-scale GWAS. These efforts were mostly coordinated within international consortia such as GIANT (the Genetic Investigation of ANthropometric Traits) [ 13 , 15 ], which predominantly included populations of European ancestry. However, a number of well-powered studies including populations of Asian [ 28 , 29 ], Hispanic [ 30 ] and African [ 31 ] ancestries contributed to new discoveries or replication of already reported obesity susceptibility loci. These populations helped to increase the size of available cohorts and so the statistical power of the GWAS. Moreover, based on their specific demographic and evolutionary characteristics, they were particularly valuable in identifying genetic variants with larger effect sizes specific for the respective population. One of these ethnic groups is the Greenlandic population, which played a crucial role in identification of obesity-associated polymorphisms in ADCY3 [ 32 , 33 ], a gene which may play a role in the regulation of human body weight [ 34 ].
The GWAS findings indicate that even with hundreds of obesity-associated loci identified to date, they only explain about 6% of the variation of BMI [ 15 ]. Although the remaining variability of BMI remains one of the major challenges of the future research efforts, genome-wide strategies have clearly demonstrated their enormous potential in discovering novel disease susceptibility loci (Fig. 1 ). In the context of obesity, they showed that most of the identified loci harbour genes involved in pathways affecting neuro-circuits of appetite and satiety regulation ( BDNF , MC4R and NEGR ) [ 35 , 36 , 37 ], energy and lipid metabolism ( FTO , RPTOR and MAP2K5 [ 13 , 27 , 38 ], insulin secretion and action ( TCF7L2 , IRS1 ) [ 13 , 38 ] as well as adipogenesis [ 14 ]. Furthermore, GWAS also suggested that many of the identified obesity-associated genes are common also for other metabolic diseases such as diabetes, hypertension, and coronary artery disease, which has been supported in gene ontology analyses (GO) highlighting gene clusters with common shared metabolic pathways for these diseases [ 39 ]. Another important takeaway from GWAS is the fact that numerous common polymorphisms associated with polygenic obesity in ethnically diverse population have been found in genes like PCSK1 [ 40 , 41 , 42 ], MC4R [ 43 ••] and POMC , known to carry rare loss of function variants leading to non-syndromic monogenic obesity. Although the GWAS are an excellent tool to uncover variants associated with complex non-Mendelian traits and diseases, understanding the underlying mechanisms behind these associations remains challenging. The majority of genetic variants associated with obesity map within non-coding regions without any obvious biological function, may however carry regulatory elements essential in molecular processes such as gene regulation. Finding the respective target gene of the associated variants appears often difficult since they may be located in distant chromosomal regions, which need to be assessed in subsequent follow up studies. For instance, despite the relatively large effect of the FTO SNPs on BMI with 0.35 kg/m 2 per allele or 1 kg for a person who is 1.7 m tall reported in 2007 [ 27 ], it took until 2014 to explain the regulatory circuitry and mechanistic chains behind the associations between FTO variants and obesity. Claussnitzer et al. not only showed that the intronic BMI-associated FTO SNP maps within an enhancer element for ARID5B, but could also demonstrate that ARID5B regulates the expression of IRX3 and IRX5 , which finally affect adipogenesis, lipid accumulation and thermogenesis [ 16 ].This study impressively demonstrated that comprehensive and well-designed functional studies are essential to elucidate molecular pathways underlying the observed associations of genetic loci with obesity.
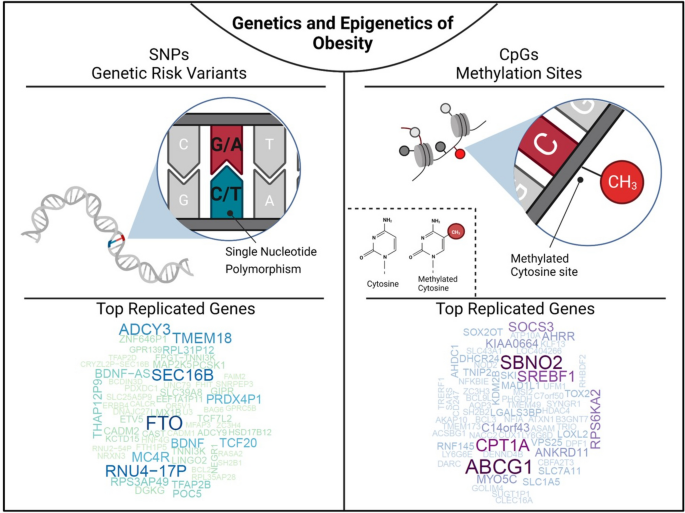
Genetics and epigenetics of obesity. The figure illustrates that single nucleotide polymorphisms are genetic risk variants identified by GWAS and CpG sites being differentially methylated in obesity. To screen for the most frequently replicated genes close to identified SNPs and CpGs for associations with BMI, we accessed the GWAS (BMI in adults and children) and EWAS catalogue (BMI in adults), respectively. SNP and CpG associations with more than one annotation were handled as individual gene count. Only hits with a P < 1 × 10 −8 were included. Associations were analysed for replication frequencies and blotted using the wordcloud package in R (version 4.2.0, https://blog.fellstat.com/?cat=11 ). Most replicated GWAS hits for BMI: Word cloud presenting the most often replicated gene hits for genome-wide association of SNPs with BMI in adults and children (GWAS catalogue accessed 20.03.2023 [ 44 ]). All genes are replicated at least fifteen times. Gene name size and colour intensity (light green to dark blue) are indicating the replication strength (from least [15 times] to most [56 times]). Long-non-coding RNAs were excluded. Most replicated EWAS hits for BMI: Word cloud presenting the most often replicated gene hits for epigenome-wide association studies with site-specific DNA methylation marks for BMI in adults (EWAS catalogue accessed (08.03.2023 [ 18 ]). All genes are replicated at least three times. Gene name size and colour intensity (light blue to dark purple) are indicating the replication strength (from least [three times] to most [nine times]). The upper panel of the figure was generated by using BioRender.com
Genome-Wide Association Studies and Polygenic Risk Scores in Children
The major part of research efforts in polygenic obesity has been focused on adult cohorts [ 45 ], whereas similar studies in childhood obesity are rather sparse [ 46 ] and are mainly concentrating on replication of findings achieved in adults. It is of note however, that most of the loci identified in adults also associate with obesity in children suggesting the impact of genetic variants across the entire lifespan [ 47 , 48 ]. Exemplarily, polymorphisms in FTO and MC4R have been shown to be significantly associated with childhood and adolescent obesity in populations from diverse ethnic backgrounds [ 49 , 50 , 51 , 52 , 53 , 54 , 55 , 56 ]. Nevertheless, effects of some SNPs appear to be more pronounced in children and diminish later in life as has been shown for the associations of variants in TMEM18 , GNDPA2 , MC4R , NEGR1 , BDNF and KTCD15 with early-onset obesity [ 57 ], and particularly for INSIG2 variants [ 58 , 59 , 60 ]. Interestingly, some studies reported that diabetes susceptibility alleles in the HHEX-IDE locus were associated with increased BMI in children, which may underpin the well-acknowledged association between childhood obesity and T2D later in adults [ 61 ].
In the context of childhood obesity, polygenic risk scores (PGS), which represent a simple model to determine genetic risk based on multiple genetic variants at different positions in the genome, may render an important tool in translation towards precision medicine. PGSs calculated in early life would enable detection and stratification of individuals with different degrees of obesity risk, and thus, the specific time windows for targeted individualised therapies could be developed [ 62 ]. Unfortunately, to date, PGSs are mainly calculated from GWAS performed in adults, which might cast doubts on their informative value for paediatrics. However, as shown by Khera et al. (2019), these doubts do not seem to be justified by the recently generated data [ 63 ••]. Here, the authors successfully demonstrated that a polygenic predictor based on 2.1 million known obesity variants is not only associated with a 13 kg increase in body weight in adulthood, but also at birth (+0.06 kg) and at 8 years of age (+3.5 kg) [ 63 ••]. Moreover, this study indicated that PGSs derived from adult data may have a comparable strong association with BMI in children. The predictive potential of PGSs in discriminating weight differences in this study was promising and could even be further refined by considering other non-genetic factors such as maternal BMI [ 64 ]. However, using this PGS in order to predict future obesity in the UK Biobank has been rather disappointing, showing a high proportion of unreliability making it less useful in clinical utility regarding disease prediction [ 45 ]. This clearly sheds light on the difficulties in using genetic information for common polygenic obesity and to translate it into a clinical prediction tool that can be a game changer in clinical day life and decision making. This is currently unrealistic although, it seems conceivable that the performance of PGSs can be increased by combining it with other factors such as environmental or epigenetic indicators to function more accurately [ 45 ]. Indeed, it is well-acknowledged that genetic profiling is gaining general popularity in extensive research endeavours, such as within large-scale biobanks linked to healthcare and clinical trials. As a result, it is more and more common for patients and their doctors to encounter PRS during clinical interactions, such as those related, e.g. to cardiovascular conditions [ 65 ], severe liver disease [ 66 ] and other human pathologies. Thus, refined and robust scores providing more accurate prediction of obesity in the future will undoubtedly become important measures in clinical settings potentially conferring also a predictive value for risk of developing obesity-related co-morbidities such as cardiovascular diseases, liver disease and several types of cancers.
The Importance of Epigenetic Mechanisms
In the context of the complex etiology of human obesity, epigenetic mechanisms based on, e.g. DNA methylation or histone modifications and gene-environment interactions are important to be considered in order to better understand the role of genetics in the development of this multifactorial disease. Despite large-scale GWAS and a simultaneously rising number of studies addressing gene-environment interactions, these studies remain challenging and their findings are often population-specific and not ubiquitously applicable and straightforward. Environmental factors such as physical activity, smoking and dietary components are acting as modifiers of the genetic predisposition to obesity manifestation. This clearly highlights obesity as a preventable disease and further indicates a highly beneficial potential of treatment strategies based on lifestyle interventions. A recent review reported the majority of SNP-environment interactions in association with alcohol consumption, smoking and physical activity [ 67 ]. However, among them were also robustly replicated associations as reported for the FTO locus which effects could be attenuated by increased physical activity, but exaggerated by non-healthy fried food consumption [ 68 , 69 , 70 ]. There is no doubt that the steadily increasing number of large-scale studies including cohorts such as the UK Biobank and similar large-scale efforts will lead to the discovery of new and more robust gene-environment interactions in the future, usable for more precise treatment opportunities. Nevertheless, the underlying causative mechanisms behind the observed associations remain unknown for most of the genes.
Epigenome-Wide Association Studies in Obesity
Epigenetic mechanisms such as DNA methylation or modification of histone core proteins are suggested to mediate gene-environment interactions and therefore may play a substantial role in susceptibility for obesity. DNA methylation is the most stable, easy to measure and best studied epigenetic mark and has been extensively studied over the last years in relation to obesity.
Genome wide DNA methylation patterns are widely used for EWAS aiming to uncover DNA methylation marks correlating with clinical variables of obesity or fat distribution. Thus, a rapid rise of well-powered EWAS (including multi-omics approaches) and partly large case–control studies in twins, family settings or independent subjects started almost one decade ago and discovered novel targets being involved in epigenetic dysregulation in obesity. In the present review, we summarised 45 genome-wide methylation studies including work mostly conducted in Caucasian subjects and performed in DNA samples originating from whole blood, isolated blood cells or adipose tissue, (Table 1 ). Importantly, although DNA methylation analyses truly identify novel candidate CpG sites and genes, additional information such as on genetic variation, gene expression, proteome/metabolome is warranted to understand the causative mechanistic circuitry underlying the correlation with disease relevant clinical traits. For instance, genetic variants may modulate the methylation at specific CpG sites potentially inducing co-methylation patterns at nearby sites, eventually translating into changes in clinical traits and suggesting a genotype–phenotype correlation. Therefore, a rising number of studies focus on multi-omics epigenetic associations with obesity or related traits, mainly promoted by the latest advances in high-throughput technologies and analytical approaches promoting (Table 1 ).
Although the most powerful EWAS reported recognisable sample sizes with more than 5000 subjects in the discovery cohort [ 20 ], the effect sizes are highly variable ranging from 6 to 40 kg/m 2 change in BMI per unit increase in blood DNA methylation. In general, sample sizes in EWAS studies are often much smaller than in GWAS analyses (Table 1 ), with the smaller cohorts mainly estimating methylation differences between individuals with and without obesity/metabolic syndrome. Studies with lower sample sizes also report smaller effect sizes such as 0.8–3.6% BMI increase per 0.1 increase in methylation ß-values [ 73 ]. Interestingly, Vehmeijer et al. demonstrated an increasing effect size with age by meta-analysing 187 methylation loci, previously reported to show cross-sectional association to BMI in adults, in children with an age between 2 and 18 years [ 114 ]. However, most studies are still of explorative nature focussing on the identification of novel candidate sites and genes rather than evaluating whether methylation changes are cause or consequence of obesity (Table 1 ).
To generate an overview about genes reported to show associations between methylation level of specific CpG sites and BMI, we used data from the EWAS catalogue (all P < 1 × 10 −8 ; EWAS catalogue accessed 08.03.2023, [ 18 ]) and performed a word-cloud analysis using gene IDs in R ( wordcloud package, R version 4.2.0). Based on this analysis, we estimated the most replicated genes originating from EWAS for BMI (Fig. 1 ). All genes included were replicated at least in three studies with ABCG1 ( ATP-binding Cassette Sub-family G Member 1 ) being the mostly replicated gene locus followed by CPT1A ( Carnitin Palmitoyltransferase 1 ), SREBF1 ( Sterol Regulatory Element Binding Transcription Factor 1 ), SBNO2 ( Strawberry Notch Homolog 2 ) and SOCS3 ( Suppressor of Cytokine Signaling 3 ). Among them ABCG1 [ 78 , 91 , 96 , 98 ] and CPT1A [ 76 , 78 , 87 , 90 , 99 ] were described across different ethnicities such as Caucasian, African American, Africans and Asians (Table 1 ). Of note, some larger cohorts such as LOLIPOP (London Life Sciences Prospective Population [ 115 ]) or KORA (Cooperative Health Research in the Region of Augsburg [ 116 ]) are more frequently used as replication cohorts.
Several studies support the functional role of, e.g. ABCG1 and CPT1A in obesity. Wahl et al. [ 20 ] for instance reported an association of the BMI genetic risk score with the ABCG1 methylation being consistent with other studies reporting effects of overweight and weight-loss on methylation, expression or protein activity [ 20 , 117 , 118 ]. In general, ABCG1 is involved in mitochondrial cholesterol efflux, thus promoting cellular efflux to HDL. Its silencing leads to massive lipid accumulation in tissues of high fat and high cholesterol fed mice and in 3T3L1 adipocytes [ 119 , 120 , 121 ]. In line with this, ABCG1 and, e.g. SREBF1 methylation levels are also known to correlate with T2D [ 115 , 122 , 123 , 124 ], postulating direct or indirect effects on metabolic consequences of obesity. Similar to ABCG1, CPT1A is involved in mitochondrial fatty acid oxidation and ROS production by regulating the entry of long-chain fatty acids into the mitochondrial matrix [ 125 ] and thereby also contributing to the activation of inflammasomes [ 126 ]. Furthermore, high-fat diet (+/− fructose) fed mice revealed a decreased CTP1a activity and thus a decreased fat metabolism, whereas knockdown of the fructose metabolism enhanced CPT1a activity [ 127 ]. Taken together, EWAS studies have extensively helped to discover CpG sites whose differential methylation levels correlate with important clinical traits of obesity and fat distribution, thus clearly illustrating the importance of epigenetic marks in obesity and its potential dysregulation in disease. However, despite these efforts and multiple novel candidate genes identified during the last years, the precise mechanistic circuitry of those genes in the human pathophysiology of obesity and relevant metabolic traits is still not well understood. Furthermore, although recent studies support the role of methylation changes in obesity, to what extent whole blood methylation profiles can mirror their patterns in target tissues remains under discussion. In addition, the majority of studies included in this review used array based approaches for genome-wide association studies, providing a limited overview of 1.7–3% of all CpG positions in the genome, illustrating that a large part of the remaining sites is undiscovered among these studies [ 128 ].
Ethnicity Specific Findings and Meta-analyses
Although most genome wide DNA methylation analyses were conducted in cohorts with Caucasian ancestry (Table 1 ), recent studies focussed more on the homo- or heterogeneity between the ethnic groups. For instance, a EWAS performed in an Arab population confirmed seven previously identified BMI loci but reported higher effect sizes compared to their replication cohort from the UK [ 87 ]. However, it has to be acknowledged that the reported association of these loci did not reach genome-wide significance level in the Arab discovery cohort. In line with this, previously reported associations between, e.g. CPT1A methylation and BMI have been confirmed across multiple ethnicities such as Caucasian, African American, African, Asian and Arab [ 76 , 78 , 87 , 90 , 99 ]. Moreover, a recently published multi-ethnic study in Asians was able to replicate 110 BMI-associated loci, which were previously reported for Europeans, South Asians and African Americans with a high consistency of the effect directions. Although they reported a great homogeneity across the different Asian ethnicities, they also demonstrated heterogeneity across several loci, where for instance the effects are mainly driven by the Chinese subjects [ 108 •]. Another study, taking into account a longitudinal setting, discovered a total of 287 novel CpG sites correlating with BMI (266 in white participants, 21 in black individuals). Importantly, a major take home message from this report is that, based on the longitudinal design, the authors concluded that obesity seems to precede changes in methylation, underlining, in line with Wahl et al. [ 20 ] that obesity may rather be cause than consequence of epigenetic changes [ 19 •].
In a very recent study, representing the largest meta-analysis so far, Do et al. [ 113 •] performed an EWAS in more than 17,000 individuals to detect CpG sites associated with BMI of European (Caucasian), African and Asian subjects. Following this approach, the study confirmed 553 previously reported loci but also identified 685 novel sites, which were successfully replicated. Interestingly, only five CpG sites were reported showing an interaction with BMI by race/ethnicity among individuals with a European or African ancestry. Importantly, in an attempt to assess the value of such CpG sites in predicting BMI, the study demonstrated that 397 of those identified CpG positions explained 32% of BMI variability illustrating that a methylome-based prediction of BMI in this study performed relatively good [ 113 •].
The Utility of Methylation Risk Scores in Predicting Disease Risk
Similar to genetic analyses, the concept of polygenic risks scores can be transferred to CpG methylation data and can be used to construct methylation risk scores (MRS). Such MRSs may prove useful tools in predicting disease risk or assessing exposure to specific environmental factors in the future. It is noteworthy, however, that in addition to methodological challenges in constructing weighted MRSs, all EWAS findings, and thereby MRSs are highly sensitive to potential confounders such as age, gender, ethnicity and also technological differences in assessing DNA methylation [ 129 ]. In line with Do et al. [ 113 •], who reported 32% of the variability in BMI accounted for by an MRS, also others, such as Hamilton and colleagues [ 130 ], observed that an MRS correlates with adverse health outcomes and accounts for 10% of the variance in BMI. This is similar to observations in adult women where DNA methylation scores roughly explained 10% BMI variance in the population, whilst much less variance was explained in children (1–2%) and young adolescents (3%) [ 131 ]. Furthermore, the same study concluded that MRS is a poor marker for future BMI prediction, illustrating the challenges in using MRSs as a meaningful prediction tool in clinical day life so far. However, it has been shown that epigenetic predictors based on DNA methylation at CpG sites are valuable tools in predicting mortality and exposure to certain environmental factors such as to smoking [ 132 ]. This is largely corroborated by a recent study showing that MRSs performed better in adult individuals than polygenic risk scores in explaining variance in smoking and BMI [ 133 ]. Taken together, there is a potential that MRSs can evolve into useful tools for clinical decision making in the future, although until now there are still conflicting results published. Of note, by increasing the sample sizes, taking into account potential confounders and combining MRSs with polygenic risk scores in the future might help to overcome current obstacles.
Enormous advances have been made during the last years in identifying genetic and epigenetic loci being involved in the pathophysiology of obesity and related clinical traits. GWAS and EWAS approaches are both by nature hypothesis-free strategies that have proven excellent tools in discovering such novel susceptibility loci and sites. Although for most of the genetic risk variants still the mechanistic circuitry needs to be investigated, important progress has been made for a number of important players.. The use of polygenic risk scores in predicting future BMI or obesity is still in its infancy as a relatively frequent mis-prediction is complicating effective use in clinical settings. Likewise, numerous epigenetic studies have identified novel candidate CpGs and genes conferring changes in DNA methylation. Multiple genes also provide a plausible functional implication in related clinical traits. Construction of methylation risk scores has proven successful for predicting exposure to specific environmental factors such as smoking, but again, its utility in clinical day life is limited for prediction of disease risk. At this stage of research, it seems unlikely so far to use either polygenic or methylation risk scores as a valid clinical prediction tool in the near future. However, by further refining the scores, increasing sample sizes and improving weighting statistic, taking into account typical confounders and combining potentially polygenic risk scores with methylation risk scores may prove successful instruments useful in clinical settings such as predicting future BMI and obesity or predicting successful weight loss in the future.
Papers of particular interest, published recently, have been highlighted as: • Of importance •• Of major importance
Ng M, Fleming T, Robinson M, Thomson B, Graetz N, Margono C, Mullany EC, Biryukov S, Abbafati C, Abera SF, et al. Global, regional, and national prevalence of overweight and obesity in children and adults during 1980–2013: a systematic analysis for the Global Burden of Disease Study 2013. Lancet. 2014;384(9945):766–81.
Article PubMed PubMed Central Google Scholar
Bluher M. Adipose tissue inflammation: a cause or consequence of obesity-related insulin resistance. Clin Sci (London, England : 1979). 2016;130(18):1603–14.
Article Google Scholar
Fontaine KR, Redden DT, Wang C, Westfall AO, Allison DB. Years of life lost due to obesity. JAMA. 2003;289(2):187–93.
Article PubMed Google Scholar
World Obesity Atlas 2023. https://www.worldobesityday.org/resources/entry/world-obesity-atlas-2023 .
Katzmarzyk PT, Perusse L, Rao DC, Bouchard C. Familial risk of overweight and obesity in the Canadian population using the WHO/NIH criteria. Obes Res. 2000;8(2):194–7.
Article CAS PubMed Google Scholar
Koeppen-Schomerus G, Wardle J, Plomin R. A genetic analysis of weight and overweight in 4-year-old twin pairs. International journal of obesity and related metabolic disorders : journal of the International Association for the Study of Obesity. 2001;25(6):838–44.
Pietilainen KH, Kaprio J, Rissanen A, Winter T, Rimpela A, Viken RJ, Rose RJ. Distribution and heritability of BMI in Finnish adolescents aged 16y and 17y: a study of 4884 twins and 2509 singletons. International journal of obesity and related metabolic disorders : journal of the International Association for the Study of Obesity. 1999;23(2):107–15.
Allison DB, Kaprio J, Korkeila M, Koskenvuo M, Neale MC, Hayakawa K. The heritability of body mass index among an international sample of monozygotic twins reared apart. International journal of obesity and related metabolic disorders. J Int Assoc Study Obes. 1996;20(6):501–6.
CAS PubMed Google Scholar
Feinleib M, Garrison RJ, Fabsitz R, Christian JC, Hrubec Z, Borhani NO, Kannel WB, Rosenman R, Schwartz JT, Wagner JO. The NHLBI twin study of cardiovascular disease risk factors: methodology and summary of results. Am J Epidemiol. 1977;106(4):284–5.
Stunkard AJ, Foch TT, Hrubec Z. A twin study of human obesity. JAMA. 1986;256(1):51–4.
Stunkard AJ, Harris JR, Pedersen NL, McClearn GE. The body-mass index of twins who have been reared apart. N Engl J Med. 1990;322(21):1483–7.
Stunkard AJ, Sorensen TI, Hanis C, Teasdale TW, Chakraborty R, Schull WJ, Schulsinger F. An adoption study of human obesity. N Engl J Med. 1986;314(4):193–8.
Locke AE, Kahali B, Berndt SI, Justice AE, Pers TH, Day FR, Powell C, Vedantam S, Buchkovich ML, Yang J, et al. Genetic studies of body mass index yield new insights for obesity biology. Nature. 2015;518(7538):197–206.
Article CAS PubMed PubMed Central Google Scholar
Shungin D, Winkler TW, Croteau-Chonka DC, Ferreira T, Locke AE, Mägi R, Strawbridge RJ, Pers TH, Fischer K, Justice AE, et al. New genetic loci link adipose and insulin biology to body fat distribution. Nature. 2015;518(7538):187–96.
Yengo L, Sidorenko J, Kemper KE, Zheng Z, Wood AR, Weedon MN, Frayling TM, Hirschhorn J, Yang J, Visscher PM. Meta-analysis of genome-wide association studies for height and body mass index in ∼700000 individuals of European ancestry. Hum Mol Genet. 2018;27(20):3641–9.
Claussnitzer M, Dankel SN, Kim KH, Quon G, Meuleman W, Haugen C, Glunk V, Sousa IS, Beaudry JL, Puviindran V, et al. FTO obesity variant circuitry and adipocyte browning in humans. N Engl J Med. 2015;373(10):895–907.
Glunk V, Laber S, Sinnott-Armstrong N, Sobreira DR, Strobel SM, Batista TM, Kubitz P, Moud BN, Ebert H, Huang Y, et al. A non-coding variant linked to metabolic obesity with normal weight affects actin remodelling in subcutaneous adipocytes. Nat Metab. 2023;5(5):861–79.
Battram T, Yousefi P, Crawford G, Prince C, Sheikhali Babaei M, Sharp G, Hatcher C, Vega-Salas MJ, Khodabakhsh S, Whitehurst O, et al. The EWAS Catalog: a database of epigenome-wide association studies. Wellcome Open Res. 2022;7:41.
• Sun D, Zhang T, Su S, Hao G, Chen T, Li QZ, Bazzano L, He J, Wang X, Li S, et al. Body mass index drives changes in DNA methylation: a longitudinal study. Circ Res. 2019;125(9):824–33. The study perform ethnicity-specific EWASs and suggests that change in DNA methylation follow rather than precede obesity.
Wahl S, Drong A, Lehne B, Loh M, Scott WR, Kunze S, Tsai PC, Ried JS, Zhang W, Yang Y, et al. Epigenome-wide association study of body mass index, and the adverse outcomes of adiposity. Nature. 2017;541(7635):81–6.
Salum KCR, Rolando JM, Zembrzuski VM, Carneiro JRI, Mello CB, Maya-Monteiro CM, Bozza PT, Kohlrausch FB, da Fonseca ACP. When leptin is not there: a review of what nonsyndromic monogenic obesity cases tell us and the benefits of exogenous leptin. Front Endocrinol (Lausanne). 2021;12:722441.
Meyre D, Lecoeur C, Delplanque J, Francke S, Vatin V, Durand E, Weill J, Dina C, Froguel P. A genome-wide scan for childhood obesity-associated traits in French families shows significant linkage on chromosome 6q22.31-q23.2. Diabetes. 2004;53(3):803–11.
Böttcher Y, Körner A, Reinehr T, Enigk B, Kiess W, Stumvoll M, Kovacs P. ENPP1 variants and haplotypes predispose to early onset obesity and impaired glucose and insulin metabolism in German obese children. J Clin Endocrinol Metab. 2006;91(12):4948–52.
Meyre D, Bouatia-Naji N, Tounian A, Samson C, Lecoeur C, Vatin V, Ghoussaini M, Wachter C, Hercberg S, Charpentier G, et al. Variants of ENPP1 are associated with childhood and adult obesity and increase the risk of glucose intolerance and type 2 diabetes. Nat Genet. 2005;37(8):863–7.
Wang R, Zhou D, Xi B, Ge X, Zhu P, Wang B, Zhou M, Huang Y, Liu J, Yu Y, et al. ENPP1/PC-1 gene K121Q polymorphism is associated with obesity in European adult populations: evidence from a meta-analysis involving 24,324 subjects. Biomed Environ Sci. 2011;24(2):200–6.
PubMed Google Scholar
Dina C, Meyre D, Gallina S, Durand E, Körner A, Jacobson P, Carlsson LM, Kiess W, Vatin V, Lecoeur C, et al. Variation in FTO contributes to childhood obesity and severe adult obesity. Nat Genet. 2007;39(6):724–6.
Frayling TM, Timpson NJ, Weedon MN, Zeggini E, Freathy RM, Lindgren CM, Perry JR, Elliott KS, Lango H, Rayner NW, et al. A common variant in the FTO gene is associated with body mass index and predisposes to childhood and adult obesity. Science. 2007;316(5826):889–94.
Akiyama M, Okada Y, Kanai M, Takahashi A, Momozawa Y, Ikeda M, Iwata N, Ikegawa S, Hirata M, Matsuda K, et al. Genome-wide association study identifies 112 new loci for body mass index in the Japanese population. Nat Genet. 2017;49(10):1458–67.
Buniello A, MacArthur JAL, Cerezo M, Harris LW, Hayhurst J, Malangone C, McMahon A, Morales J, Mountjoy E, Sollis E, et al. The NHGRI-EBI GWAS Catalog of published genome-wide association studies, targeted arrays and summary statistics 2019. Nucleic Acids Res. 2019;47(D1):D1005-d1012.
Wojcik GL, Graff M, Nishimura KK, Tao R, Haessler J, Gignoux CR, Highland HM, Patel YM, Sorokin EP, Avery CL, et al. Genetic analyses of diverse populations improves discovery for complex traits. Nature. 2019;570(7762):514–8.
Ng MCY, Graff M, Lu Y, Justice AE, Mudgal P, Liu CT, Young K, Yanek LR, Feitosa MF, Wojczynski MK, et al. Discovery and fine-mapping of adiposity loci using high density imputation of genome-wide association studies in individuals of African ancestry: African Ancestry Anthropometry Genetics Consortium. PLoS Genet. 2017;13(4): e1006719.
Grarup N, Moltke I, Andersen MK, Dalby M, Vitting-Seerup K, Kern T, Mahendran Y, Jørsboe E, Larsen CVL, Dahl-Petersen IK, et al. Loss-of-function variants in ADCY3 increase risk of obesity and type 2 diabetes. Nat Genet. 2018;50(2):172–4.
Saeed S, Bonnefond A, Tamanini F, Mirza MU, Manzoor J, Janjua QM, Din SM, Gaitan J, Milochau A, Durand E, et al. Loss-of-function mutations in ADCY3 cause monogenic severe obesity. Nat Genet. 2018;50(2):175–9.
Siljee JE, Wang Y, Bernard AA, Ersoy BA, Zhang S, Marley A, Von Zastrow M, Reiter JF, Vaisse C. Subcellular localization of MC4R with ADCY3 at neuronal primary cilia underlies a common pathway for genetic predisposition to obesity. Nat Genet. 2018;50(2):180–5.
Boender AJ, van Rozen AJ, Adan RA. Nutritional state affects the expression of the obesity-associated genes Etv5, Faim2, Fto, and Negr1. Obesity (Silver Spring). 2012;20(12):2420–5.
Ho EV, Klenotich SJ, McMurray MS, Dulawa SC. Activity-based anorexia alters the expression of BDNF transcripts in the mesocorticolimbic reward circuit. PLoS ONE. 2016;11(11): e0166756.
Horstmann A, Kovacs P, Kabisch S, Boettcher Y, Schloegl H, Tönjes A, Stumvoll M, Pleger B, Villringer A. Common genetic variation near MC4R has a sex-specific impact on human brain structure and eating behavior. PLoS ONE. 2013;8(9): e74362.
Kilpeläinen TO, Zillikens MC, Stančákova A, Finucane FM, Ried JS, Langenberg C, Zhang W, Beckmann JS, Luan J, Vandenput L, et al. Genetic variation near IRS1 associates with reduced adiposity and an impaired metabolic profile. Nat Genet. 2011;43(8):753–60.
Su LN, Wang YB, Wnag CG, Wei HP. Network analysis identifies common genes associated with obesity in six obesity-related diseases. J Zhejiang Univ Sci B. 2017;18(8):727–32.
Benzinou M, Creemers JW, Choquet H, Lobbens S, Dina C, Durand E, Guerardel A, Boutin P, Jouret B, Heude B, et al. Common nonsynonymous variants in PCSK1 confer risk of obesity. Nat Genet. 2008;40(8):943–5.
Choquet H, Kasberger J, Hamidovic A, Jorgenson E. Contribution of common PCSK1 genetic variants to obesity in 8,359 subjects from multi-ethnic American population. PLoS ONE. 2013;8(2): e57857.
Rouskas K, Kouvatsi A, Paletas K, Papazoglou D, Tsapas A, Lobbens S, Vatin V, Durand E, Labrune Y, Delplanque J, et al. Common variants in FTO, MC4R, TMEM18, PRL, AIF1, and PCSK1 show evidence of association with adult obesity in the Greek population. Obesity (Silver Spring). 2012;20(2):389–95.
•• Loos RJ, Lindgren CM, Li S, Wheeler E, Zhao JH, Prokopenko I, Inouye M, Freathy RM, Attwood AP, Beckmann JS, et al. Common variants near MC4R are associated with fat mass, weight and risk of obesity. Nat Genet. 2008;40(6):768–75. In addition to being an excellent review summarize the authors the predictive value of polygenic risk scores in predicting future BMI and obesity.
Sollis E, Mosaku A, Abid A, Buniello A, Cerezo M, Gil L, Groza T, Güneş O, Hall P, Hayhurst J, et al. The NHGRI-EBI GWAS Catalog: knowledgebase and deposition resource. Nucleic Acids Res. 2023;51(D1):D977-d985.
Loos RJF, Yeo GSH. The genetics of obesity: from discovery to biology. Nat Rev Genet. 2022;23(2):120–33.
Warner ET, Jiang L, Adjei DN, Turman C, Gordon W, Wang L, Tamimi R, Kraft P, Lindström S. A genome-wide association study of childhood body fatness. Obesity (Silver Spring). 2021;29(2):446–53.
Bradfield JP, Taal HR, Timpson NJ, Scherag A, Lecoeur C, Warrington NM, Hypponen E, Holst C, Valcarcel B, Thiering E, et al. A genome-wide association meta-analysis identifies new childhood obesity loci. Nat Genet. 2012;44(5):526–31.
Scherag A, Dina C, Hinney A, Vatin V, Scherag S, Vogel CI, Müller TD, Grallert H, Wichmann HE, Balkau B, et al. Two new loci for body-weight regulation identified in a joint analysis of genome-wide association studies for early-onset extreme obesity in French and german study groups. PLoS Genet. 2010;6(4): e1000916.
Costa-Urrutia P, Colistro V, Jiménez-Osorio AS, Cárdenas-Hernández H, Solares-Tlapechco J, Ramirez-Alcántara M, Granados J, Ascencio-Montiel IJ, Rodríguez-Arellano ME. Genome-wide association study of body mass index and body fat in Mexican-Mestizo Children. Genes (Basel). 2019;10(11):945.
Couto Alves A, De Silva NMG, Karhunen V, Sovio U, Das S, Taal HR, Warrington NM, Lewin AM, Kaakinen M, Cousminer DL, et al. GWAS on longitudinal growth traits reveals different genetic factors influencing infant, child, and adult BMI. Sci Adv. 2019;5(9):eaaw3095.
den Hoed M, Ekelund U, Brage S, Grontved A, Zhao JH, Sharp SJ, Ong KK, Wareham NJ, Loos RJ. Genetic susceptibility to obesity and related traits in childhood and adolescence: influence of loci identified by genome-wide association studies. Diabetes. 2010;59(11):2980–8.
Liu G, Zhu H, Lagou V, Gutin B, Stallmann-Jorgensen IS, Treiber FA, Dong Y, Snieder H. FTO variant rs9939609 is associated with body mass index and waist circumference, but not with energy intake or physical activity in European- and African-American youth. BMC Med Genet. 2010;11:57.
Liu HY, Alyass A, Abadi A, Peralta-Romero J, Suarez F, Gomez-Zamudio J, Audirac A, Parra EJ, Cruz M, Meyre D. Fine-mapping of 98 obesity loci in Mexican children. Int J Obes (Lond). 2019;43(1):23–32.
Wheeler E, Huang N, Bochukova EG, Keogh JM, Lindsay S, Garg S, Henning E, Blackburn H, Loos RJ, Wareham NJ, et al. Genome-wide SNP and CNV analysis identifies common and low-frequency variants associated with severe early-onset obesity. Nat Genet. 2013;45(5):513–7.
Xi B, Shen Y, Zhang M, Liu X, Zhao X, Wu L, Cheng H, Hou D, Lindpaintner K, Liu L, et al. The common rs9939609 variant of the fat mass and obesity-associated gene is associated with obesity risk in children and adolescents of Beijing. China BMC Med Genet. 2010;11:107.
Yao S, Wu H, Ding JM, Wang ZX, Ullah T, Dong SS, Chen H, Guo Y. Transcriptome-wide association study identifies multiple genes associated with childhood body mass index. Int J Obes (Lond). 2021;45(5):1105–13.
Zhao J, Bradfield JP, Li M, Wang K, Zhang H, Kim CE, Annaiah K, Glessner JT, Thomas K, Garris M, et al. The role of obesity-associated loci identified in genome-wide association studies in the determination of pediatric BMI. Obesity (Silver Spring). 2009;17(12):2254–7.
Bressler J, Fornage M, Hanis CL, Kao WH, Lewis CE, McPherson R, Dent R, Mosley TH, Pennacchio LA, Boerwinkle E. The INSIG2 rs7566605 genetic variant does not play a major role in obesity in a sample of 24,722 individuals from four cohorts. BMC Med Genet. 2009;10:56.
Campa D, Hüsing A, McKay JD, Sinilnikova O, Vogel U, Tjønneland A, Overvad K, Stegger J, Clavel-Chapelon F, Chabbert-Buffet N, et al. The INSIG2 rs7566605 polymorphism is not associated with body mass index and breast cancer risk. BMC Cancer. 2010;10:563.
Herbert A, Gerry NP, McQueen MB, Heid IM, Pfeufer A, Illig T, Wichmann HE, Meitinger T, Hunter D, Hu FB, et al. A common genetic variant is associated with adult and childhood obesity. Science. 2006;312(5771):279–83.
Zhao J, Bradfield JP, Zhang H, Annaiah K, Wang K, Kim CE, Glessner JT, Frackelton EC, Otieno FG, Doran J, et al. Examination of all type 2 diabetes GWAS loci reveals HHEX-IDE as a locus influencing pediatric BMI. Diabetes. 2010;59(3):751–5.
Maher BS. Polygenic scores in epidemiology: risk prediction, etiology, and clinical utility. Curr Epidemiol Rep. 2015;2(4):239–44.
•• Khera AV, Chaffin M, Wade KH, Zahid S, Brancale J, Xia R, Distefano M, Senol-Cosar O, Haas ME, Bick A, et al. Polygenic prediction of weight and obesity trajectories from birth to adulthood. Cell. 2019;177(3):587–96.e589. The study describes a new polygenic risk score that has ability to discriminate differences in weight, obesity, cardiometabolic disease and mortality in adults.
Lange K, Kerr JA, Mansell T, O’Sullivan JM, Burgner DP, Clifford SA, Olds T, Dwyer T, Wake M, Saffery R. Can adult polygenic scores improve prediction of body mass index in childhood? Int J Obes (Lond). 2022;46(7):1375–83.
O’Sullivan JW, Raghavan S, Marquez-Luna C, Luzum JA, Damrauer SM, Ashley EA, O’Donnell CJ, Willer CJ, Natarajan P. Polygenic risk scores for cardiovascular disease: a scientific statement from the American Heart Association. Circulation. 2022;146(8):e93–118.
PubMed PubMed Central Google Scholar
De Vincentis A, Tavaglione F, Jamialahmadi O, Picardi A, Antonelli Incalzi R, Valenti L, Romeo S, Vespasiani-Gentilucci U. A polygenic risk score to refine risk stratification and prediction for severe liver disease by clinical fibrosis scores. Clin Gastroenterol Hepatol. 2022;20(3):658–73.
San-Cristobal R, de Toro-Martín J, Vohl MC. Appraisal of gene-environment interactions in GWAS for evidence-based precision nutrition implementation. Curr Nutr Rep. 2022;11(4):563–73.
Feitosa MF, Kraja AT, Chasman DI, Sung YJ, Winkler TW, Ntalla I, Guo X, Franceschini N, Cheng CY, Sim X, et al. Novel genetic associations for blood pressure identified via gene-alcohol interaction in up to 570K individuals across multiple ancestries. PLoS ONE. 2018;13(6): e0198166.
Graff M, Scott RA, Justice AE, Young KL, Feitosa MF, Barata L, Winkler TW, Chu AY, Mahajan A, Hadley D, et al. Genome-wide physical activity interactions in adiposity - A meta-analysis of 200,452 adults. PLoS Genet. 2017;13(4): e1006528.
Kilpeläinen TO, Qi L, Brage S, Sharp SJ, Sonestedt E, Demerath E, Ahmad T, Mora S, Kaakinen M, Sandholt CH, et al. Physical activity attenuates the influence of FTO variants on obesity risk: a meta-analysis of 218,166 adults and 19,268 children. PLoS Med. 2011;8(11): e1001116.
Carless MA, Kulkarni H, Kos MZ, Charlesworth J, Peralta JM, Göring HH, Blangero J. Genetic effects on DNA methylation and its potential relevance for obesity in Mexican Americans. PloS one. 2013;8(9):e73950. https://doi.org/10.1371/journal.pone.0073950 .
Xu X, Su S, Barnes VA, De Miguel C, Pollock J, Ownby D, Wang X. A genome-wide methylation study on obesity: differential variability and differential methylation. Epigenetics. 2013;8(5):522–33. https://doi.org/10.4161/epi.24506 .
Dick KJ, Nelson CP, Tsaprouni L, Sandling JK, Aïssi D, Wahl S, Meduri E, Morange PE, Gagnon F, Grallert H, et al. DNA methylation and body-mass index: a genome-wide analysis. Lancet. 2014;383(9933):1990–8.
Almén MS, Nilsson EK, Jacobsson JA, Kalnina I, Klovins J, Fredriksson R, Schiöth HB. Genome-wide analysis reveals DNA methylation markers that vary with both age and obesity. Gene. 2014;548(1):61–7. https://doi.org/10.1016/j.gene.2014.07.009 .
Guénard F, Tchernof A, Deshaies Y, Pérusse L, Biron S, Lescelleur O, Vohl MC. Differential methylation in visceral adipose tissue of obese men discordant for metabolic disturbances. Physiol Genomics. 2014;46(6):216–22. https://doi.org/10.1152/physiolgenomics.00160.2013 .
Aslibekyan S, Demerath EW, Mendelson M, Zhi D, Guan W, Liang L, Sha J, Pankow JS, Liu C, Irvin MR, et al. Epigenome-wide study identifies novel methylation loci associated with body mass index and waist circumference. Obesity (Silver Spring). 2015;23(7):1493–501.
Ollikainen M, Ismail K, Gervin K, Kyllönen A, Hakkarainen A, Lundbom J, Kaprio J. Genome-wide blood DNA methylation alterations at regulatory elements and heterochromatic regions in monozygotic twins discordant for obesity and liver fat. Clin Epigenetics. 2015;7(1):39. https://doi.org/10.1186/s13148-015-0073-5 .
Demerath EW, Guan W, Grove ML, Aslibekyan S, Mendelson M, Zhou YH, Hedman ÅK, Sandling JK, Li LA, Irvin MR, et al. Epigenome-wide association study (EWAS) of BMI, BMI change and waist circumference in African American adults identifies multiple replicated loci. Hum Mol Genet. 2015;24(15):4464–79.
Voisin S, Almén MS, Zheleznyakova GY, Lundberg L, Zarei S, Castillo S, Schiöth HB. Many obesity-associated SNPs strongly associate with DNA methylation changes at proximal promoters and enhancers. Genome Med. 2015;7:103. https://doi.org/10.1186/s13073-015-0225-4 .
Arner P, Sinha I, Thorell A, Rydén M, Dahlman-Wright K, Dahlman I. The epigenetic signature of subcutaneous fat cells is linked to altered expression of genes implicated in lipid metabolism in obese women. Clin Epigenetics. 2015;7(1):93. https://doi.org/10.1186/s13148-015-0126-9 .
Rönn T, Volkov P, Gillberg L, Kokosar M, Perfilyev A, Jacobsen AL, Ling C. Impact of age, BMI and HbA1c levels on the genome-wide DNA methylation and mRNA expression patterns in human adipose tissue and identification of epigenetic biomarkers in blood. Hum Mol Genet. 2015;24(13):3792–813. https://doi.org/10.1093/hmg/ddv124 .
Kirchner H, Sinha I, Gao H, Ruby MA, Schönke M, Lindvall JM, Zierath JR. Altered DNA methylation of glycolytic and lipogenic genes in liver from obese and type 2 diabetic patients. Mol Metab. 2016;5(3):171–83. https://doi.org/10.1016/j.molmet.2015.12.004 .
Ali O, Cerjak D, Kent JW Jr, James R, Blangero J, Carless MA, Zhang Y. Methylation of SOCS3 is inversely associated with metabolic syndrome in an epigenome-wide association study of obesity. Epigenetics. 2016;11(9):699–707. https://doi.org/10.1080/15592294.2016.1216284 .
Keller M, Hopp L, Liu X, Wohland T, Rohde K, Cancello R, Böttcher Y. Genome-wide DNA promoter methylation and transcriptome analysis in human adipose tissue unravels novel candidate genes for obesity. Mol Metab. 2017;6(1):86–100. https://doi.org/10.1016/j.molmet.2016.11.003 .
Pietiläinen KH, Ismail K, Järvinen E, Heinonen S, Tummers M, Bollepalli S, Ollikainen M. DNA methylation and gene expression patterns in adipose tissue differ significantly within young adult monozygotic BMI-discordant twin pairs. Int J Obes. 2016;40(4):654–61. https://doi.org/10.1038/ijo.2015.221 .
Article CAS Google Scholar
Volkov P, Olsson AH, Gillberg L, Jørgensen SW, Brøns C, Eriksson KF, Ling C. A genome-wide mQTL analysis in human adipose tissue identifies genetic variants associated with DNA methylation, gene expression and metabolic traits. PloS One. 2016;11(6):e0157776. https://doi.org/10.1371/journal.pone.0157776 .
Al Muftah WA, Al-Shafai M, Zaghlool SB, Visconti A, Tsai PC, Kumar P, Spector T, Bell J, Falchi M, Suhre K. Epigenetic associations of type 2 diabetes and BMI in an Arab population. Clin Epigenetics. 2016;8:13.
Sayols-Baixeras S, Subirana I, Fernández-Sanlés A, Sentí M, Lluís-Ganella C, Marrugat J, Elosua R. DNA methylation and obesity traits: An epigenome-wide association study. The REGICOR study. Epigenetics. 2017;12(10):909–16. https://doi.org/10.1080/15592294.2017.1363951 .
Crujeiras AB, Diaz-Lagares A, Sandoval J, Milagro FI, Navas-Carretero S, Carreira MC, Martinez JA. DNA methylation map in circulating leukocytes mirrors subcutaneous adipose tissue methylation pattern: a genome-wide analysis from non-obese and obese patients. Sci Rep. 2017;7(1):41903. https://doi.org/10.1038/srep41903 .
Meeks KAC, Henneman P, Venema A, Burr T, Galbete C, Danquah I, Schulze MB, Mockenhaupt FP, Owusu-Dabo E, Rotimi CN, et al. An epigenome-wide association study in whole blood of measures of adiposity among Ghanaians: the RODAM study. Clin Epigenetics. 2017;9:103.
Wilson LE, Harlid S, Xu Z, Sandler DP, Taylor JA. An epigenome-wide study of body mass index and DNA methylation in blood using participants from the Sister Study cohort. Int J Obes (Lond). 2017;41(1):194–9.
Guénard F, Tchernof A, Deshaies Y, Biron S, Lescelleur O, Biertho L, Vohl MC. Genetic regulation of differentially methylated genes in visceral adipose tissue of severely obese men discordant for the metabolic syndrome. Transl Res. 2017;184:1–11. https://doi.org/10.1016/j.trsl.2017.01.002 .
Mendelson MM, Marioni RE, Joehanes R, Liu C, Hedman ÅK, Aslibekyan S, Deary IJ. Association of body mass index with DNA methylation and gene expression in blood cells and relations to cardiometabolic disease: a Mendelian randomization approach. PLoS Med. 2017;14(1):e1002215. https://doi.org/10.1371/journal.pmed.1002215 .
Kvaløy K, Page CM, Holmen TL. Epigenome-wide methylation differences in a group of lean and obese women–A HUNT Study. Sci Rep. 2018;8(1):16330. https://doi.org/10.1038/s41598-018-34003-8 .
Dhana K, Braun KV, Nano J, Voortman T, Demerath EW, Guan W, Dehghan A. An epigenome-wide association study of obesity-related traits. Am J Epidemiol. 2018;187(8):1662–9. https://doi.org/10.1093/aje/kwy025 .
Campanella G, Gunter MJ, Polidoro S, Krogh V, Palli D, Panico S, Sacerdote C, Tumino R, Fiorito G, Guarrera S, et al. Epigenome-wide association study of adiposity and future risk of obesity-related diseases. Int J Obes (Lond). 2018;42(12):2022–35.
Orozco LD, Farrell C, Hale C, Rubbi L, Rinaldi A, Civelek M, Pellegrini M. Epigenome-wide association in adipose tissue from the METSIM cohort. Hum Mol Genet. 2018;27(10):1830–46. https://doi.org/10.1093/hmg/ddy093 .
Akinyemiju T, Do AN, Patki A, Aslibekyan S, Zhi D, Hidalgo B, Irvin MR. Epigenome-wide association study of metabolic syndrome in African-American adults. Clin Epigenetics. 2018;10(1):49. https://doi.org/10.1186/s13148-018-0483-2 .
Zaghlool SB, Mook-Kanamori DO, Kader S, Stephan N, Halama A, Engelke R, Sarwath H, Al-Dous EK, Mohamoud YA, Roemisch-Margl W, et al. Deep molecular phenotypes link complex disorders and physiological insult to CpG methylation. Hum Mol Genet. 2018;27(6):1106–21.
Guo Q, Zheng R, Huang J, He M, Wang Y, Guo Z, Chen P. Using integrative analysis of DNA methylation and gene expression data in multiple tissue types to prioritize candidate genes for drug development in obesity. Front Genet. 2018;9:663. https://doi.org/10.3389/fgene.2018.00663 .
Li W, Zhang D, Wang W, Wu Y, Mohammadnejad A, Lund J, Tan Q. DNA methylome profiling in identical twin pairs discordant for body mass index. Int J Obes. 2019;43(12):2491–9. https://doi.org/10.1038/s41366-019-0382-4 .
Li C, Wang Z, Hardy T, Huang Y, Hui Q, Crusto CA, Sun YV. Association of obesity with DNA methylation age acceleration in African American mothers from the InterGEN study. Int J Mol Sci. 2019;20(17):4273. https://doi.org/10.3390/ijms20174273 .
Pan Y, Choi JH, Shi H, Zhang L, Su S, Wang X. Discovery and validation of a novel neutrophil activation marker associated with obesity. Sci Rep. 2019;9(1):3433. https://doi.org/10.1038/s41598-019-39764-4 .
Koh IU, Choi NH, Lee K, Yu HY, Yun JH, Kong JH, Moon S. Obesity susceptible novel DNA methylation marker on regulatory region of inflammation gene: results from the Korea Epigenome Study (KES). BMJ Open Diabetes Res Care. 2020;8(1):e001338. https://doi.org/10.1136/bmjdrc-2020-001338 .
Giri AK, Prasad G, Bandesh K, Parekatt V, Mahajan A, Banerjee P, Bharadwaj D. Multifaceted genome-wide study identifies novel regulatory loci in SLC22A11 and ZNF45 for body mass index in Indians. Mol Genet Genom. 2020;295:1013–26. https://doi.org/10.1007/s00438-020-01678-6 .
Justice AE, Chittoor G, Gondalia R, Melton PE, Lim E, Grove ML, North KE. Methylome-wide association study of central adiposity implicates genes involved in immune and endocrine systems. Epigenomics. 2020;12(17):1483–99. https://doi.org/10.2217/epi-2019-0276 .
Xie T, Gorenjak V, Stathopoulou MG, Dadé S, Marouli E, Masson C, Visvikis-Siest S. Epigenome-wide association study detects a novel loci associated with central obesity in healthy subjects. BMC Med Genom. 2021;14(1):233. https://doi.org/10.1186/s12920-021-01077-9 .
• Chen Y, Kassam I, Lau SH, Kooner JS, Wilson R, Peters A, Sim X. Impact of BMI and waist circumference on epigenome-wide DNA methylation and identification of epigenetic biomarkers in blood: an EWAS in multi-ethnic Asian individuals. Clin Epigenetics. 2021;13(1):195. https://doi.org/10.1186/s13148-021-01162-x . The study suggest that obesity is preceding methylation changes being likely cause rather than consequence.
Cao VT, Lea RA, Sutherland HG, Benton MC, Pishva RS, Haupt LM, Griffiths LR. A genome-wide methylation study of body fat traits in the Norfolk Island isolate. Nutr Metab Cardiovasc Dis. 2021;31(5):1556–63. https://doi.org/10.1016/j.numecd.2021.01.027 .
Do WL, Gohar J, McCullough LE, Galaviz KI, Conneely KN, Narayan KV. Examining the association between adiposity and DNA methylation: a systematic review and meta-analysis. Obes Rev. 2021;22(10):e13319. https://doi.org/10.1111/obr.13319 .
Wu Y, Tian H, Wang W, Li W, Duan H, Zhang D. DNA methylation and waist-to-hip ratio: an epigenome-wide association study in Chinese monozygotic twins. J Endocrinol Invest. 2022;45(12):2365–76. https://doi.org/10.1007/s40618-022-01878-4 .
Taylor JY, Huang Y, Zhao W, Wright ML, Wang Z, Hui Q, Sun YV. Epigenome-wide association study of BMI in Black populations from InterGEN and GENOA. Obesity. 2023;31(1):243–55. https://doi.org/10.1002/oby.23589 .
• Do WL, Sun D, Meeks K, Dugué PA, Demerath E, Guan W, Li S, Chen W, Milne R, Adeyemo A, et al. Epigenome-wide meta-analysis of BMI in nine cohorts: examining the utility of epigenetically predicted BMI. Am J Hum Genet. 2023;110(2):273–83. This work suggests that ~400 CpG sites can be attributed to 32% BMI variance in predicting BMI in a test set.
Vehmeijer FOL, Küpers LK, Sharp GC, Salas LA, Lent S, Jima DD, Tindula G, Reese S, Qi C, Gruzieva O, et al. DNA methylation and body mass index from birth to adolescence: meta-analyses of epigenome-wide association studies. Genome Med. 2020;12(1):105.
Chambers JC, Loh M, Lehne B, Drong A, Kriebel J, Motta V, Wahl S, Elliott HR, Rota F, Scott WR, et al. Epigenome-wide association of DNA methylation markers in peripheral blood from Indian Asians and Europeans with incident type 2 diabetes: a nested case-control study. Lancet Diabetes Endocrinol. 2015;3(7):526–34.
Holle R, Happich M, Löwel H, Wichmann HE. KORA–a research platform for population based health research. Gesundheitswesen. 2005;67(Suppl 1):S19-25.
Aron-Wisnewsky J, Julia Z, Poitou C, Bouillot JL, Basdevant A, Chapman MJ, Clement K, Guerin M. Effect of bariatric surgery-induced weight loss on SR-BI-, ABCG1-, and ABCA1-mediated cellular cholesterol efflux in obese women. J Clin Endocrinol Metab. 2011;96(4):1151–9.
Edgel KA, McMillen TS, Wei H, Pamir N, Houston BA, Caldwell MT, Mai PO, Oram JF, Tang C, Leboeuf RC. Obesity and weight loss result in increased adipose tissue ABCG1 expression in db/db mice. Biochim Biophys Acta. 2012;1821(3):425–34.
Frisdal E, Le Lay S, Hooton H, Poupel L, Olivier M, Alili R, Plengpanich W, Villard EF, Gilibert S, Lhomme M, et al. Adipocyte ATP-binding cassette G1 promotes triglyceride storage, fat mass growth, and human obesity. Diabetes. 2015;64(3):840–55.
Kennedy MA, Barrera GC, Nakamura K, Baldán A, Tarr P, Fishbein MC, Frank J, Francone OL, Edwards PA. ABCG1 has a critical role in mediating cholesterol efflux to HDL and preventing cellular lipid accumulation. Cell Metab. 2005;1(2):121–31.
Wang N, Lan D, Chen W, Matsuura F, Tall AR. ATP-binding cassette transporters G1 and G4 mediate cellular cholesterol efflux to high-density lipoproteins. Proc Natl Acad Sci U S A. 2004;101(26):9774–9.
Dayeh T, Tuomi T, Almgren P, Perfilyev A, Jansson PA, de Mello VD, Pihlajamäki J, Vaag A, Groop L, Nilsson E, et al. DNA methylation of loci within ABCG1 and PHOSPHO1 in blood DNA is associated with future type 2 diabetes risk. Epigenetics. 2016;11(7):482–8.
Hidalgo B, Irvin MR, Sha J, Zhi D, Aslibekyan S, Absher D, Tiwari HK, Kabagambe EK, Ordovas JM, Arnett DK. Epigenome-wide association study of fasting measures of glucose, insulin, and HOMA-IR in the Genetics of Lipid Lowering Drugs and Diet Network study. Diabetes. 2014;63(2):801–7.
Kulkarni H, Kos MZ, Neary J, Dyer TD, Kent JW Jr, Göring HH, Cole SA, Comuzzie AG, Almasy L, Mahaney MC, et al. Novel epigenetic determinants of type 2 diabetes in Mexican-American families. Hum Mol Genet. 2015;24(18):5330–44.
Moon JS, Nakahira K, Chung KP, DeNicola GM, Koo MJ, Pabón MA, Rooney KT, Yoon JH, Ryter SW, Stout-Delgado H, et al. NOX4-dependent fatty acid oxidation promotes NLRP3 inflammasome activation in macrophages. Nat Med. 2016;22(9):1002–12.
Hall CJ, Sanderson LE, Lawrence LM, Pool B, van der Kroef M, Ashimbayeva E, Britto D, Harper JL, Lieschke GJ, Astin JW, et al. Blocking fatty acid-fueled mROS production within macrophages alleviates acute gouty inflammation. J Clin Invest. 2018;128(5):1752–71.
Softic S, Meyer JG, Wang GX, Gupta MK, Batista TM, Lauritzen H, Fujisaka S, Serra D, Herrero L, Willoughby J, et al. Dietary sugars alter hepatic fatty acid oxidation via transcriptional and post-translational modifications of mitochondrial proteins. Cell Metab. 2019;30(4):735-753.e734.
Villicaña S, Bell JT. Genetic impacts on DNA methylation: research findings and future perspectives. Genome Biol. 2021;22(1):127.
Hüls A, Czamara D. Methodological challenges in constructing DNA methylation risk scores. Epigenetics. 2020;15(1–2):1–11.
Hamilton OKL, Zhang Q, McRae AF, Walker RM, Morris SW, Redmond P, Campbell A, Murray AD, Porteous DJ, Evans KL, et al. An epigenetic score for BMI based on DNA methylation correlates with poor physical health and major disease in the Lothian Birth Cohort. Int J Obes (Lond). 2019;43(9):1795–802.
Reed ZE, Suderman MJ, Relton CL, Davis OSP, Hemani G. The association of DNA methylation with body mass index: distinguishing between predictors and biomarkers. Clin Epigenetics. 2020;12(1):50.
McCartney DL, Hillary RF, Stevenson AJ, Ritchie SJ, Walker RM, Zhang Q, Morris SW, Bermingham ML, Campbell A, Murray AD, et al. Epigenetic prediction of complex traits and death. Genome Biol. 2018;19(1):136.
Odintsova VV, Rebattu V, Hagenbeek FA, Pool R, Beck JJ, Ehli EA, van Beijsterveldt CEM, Ligthart L, Willemsen G, de Geus EJC, et al. Predicting complex traits and exposures from polygenic scores and blood and buccal DNA methylation profiles. Front Psychiatry. 2021;12:688464.
Download references
Open access funding provided by University of Oslo (incl Oslo University Hospital)
Author information
Maria Keller and Stina Ingrid Alice Svensson contributed equally to this work.
Authors and Affiliations
Medical Department III–Endocrinology, Nephrology, Rheumatology, Medical Center, University of Leipzig, 04103, Leipzig, Germany
Maria Keller, Kerstin Rohde-Zimmermann & Peter Kovacs
Helmholtz Institute for Metabolic, Obesity and Vascular Research (HI-MAG) of the Helmholtz Center Munich at the University of Leipzig, University Hospital Leipzig, 04103, Leipzig, Germany
Maria Keller & Kerstin Rohde-Zimmermann
EpiGen, Department of Clinical Molecular Biology, Institute of Clinical Medicine, University of Oslo, 0316, Oslo, Norway
Stina Ingrid Alice Svensson & Yvonne Böttcher
EpiGen, Medical Division, Akershus University Hospital, 1478, Lørenskog, Norway
Yvonne Böttcher
You can also search for this author in PubMed Google Scholar
Contributions
All authors were responsible for the conception and design of the manuscript, drafting the manuscript, revising it critically for intellectual content and approving the final version.
Corresponding author
Correspondence to Yvonne Böttcher .
Ethics declarations
Competing interests.
The authors have no competing interests to declare that are relevant to the content of this article.
Human and Animal Rights and Informed Consent
This article does not contain any studies with human or animal subjects performed by any of the authors.
Additional information
Publisher's note.
Springer Nature remains neutral with regard to jurisdictional claims in published maps and institutional affiliations.
Supplementary Information
Below is the link to the electronic supplementary material.
Supplementary file1 (XLSX 2529 KB)
Rights and permissions.
Open Access This article is licensed under a Creative Commons Attribution 4.0 International License, which permits use, sharing, adaptation, distribution and reproduction in any medium or format, as long as you give appropriate credit to the original author(s) and the source, provide a link to the Creative Commons licence, and indicate if changes were made. The images or other third party material in this article are included in the article's Creative Commons licence, unless indicated otherwise in a credit line to the material. If material is not included in the article's Creative Commons licence and your intended use is not permitted by statutory regulation or exceeds the permitted use, you will need to obtain permission directly from the copyright holder. To view a copy of this licence, visit http://creativecommons.org/licenses/by/4.0/ .
Reprints and permissions
About this article
Keller, M., Svensson, S.I.A., Rohde-Zimmermann, K. et al. Genetics and Epigenetics in Obesity: What Do We Know so Far?. Curr Obes Rep 12 , 482–501 (2023). https://doi.org/10.1007/s13679-023-00526-z
Download citation
Accepted : 07 September 2023
Published : 11 October 2023
Issue Date : December 2023
DOI : https://doi.org/10.1007/s13679-023-00526-z
Share this article
Anyone you share the following link with will be able to read this content:
Sorry, a shareable link is not currently available for this article.
Provided by the Springer Nature SharedIt content-sharing initiative
- Genetic variants
- Epigenetic marks
- Polygenic risk scores
- Methylation risk scores
- Find a journal
- Publish with us
- Track your research
- Introduction
- Conclusions
- Article Information
Hazard ratio for obesity was modeled according to mean daily step counts and 25th, 50th, and 75th percentile PRS for body mass index. Shaded regions represent 95% CIs. Model is adjusted for age, sex, mean baseline step counts, cancer status, coronary artery disease status, systolic blood pressure, alcohol use, educational level, and a PRS × mean steps interaction term.
Mean daily steps and polygenic risk score (PRS) for higher body mass index are independently associated with hazard for obesity. Hazard ratios model the difference between the 75th and 25th percentiles for continuous variables. CAD indicate coronary artery disease; and SBP, systolic blood pressure.
Each point estimate is indexed to a hazard ratio for obesity of 1.00 (BMI [calculated as weight in kilograms divided by height in meters squared] ≥30). Error bars represent 95% CIs.
eTable. Cumulative Incidence Estimates of Obesity Based on Polygenic Risk Score for Body Mass Index and Mean Daily Steps at 1, 3, and 5 Years
eFigure 1. CONSORT Diagram
eFigure 2. Risk of Incident Obesity Modeled by Mean Daily Step Count and Polygenic Risk Scores Adjusted for Baseline Body Mass Index
Data Sharing Statement
See More About
Sign up for emails based on your interests, select your interests.
Customize your JAMA Network experience by selecting one or more topics from the list below.
- Academic Medicine
- Acid Base, Electrolytes, Fluids
- Allergy and Clinical Immunology
- American Indian or Alaska Natives
- Anesthesiology
- Anticoagulation
- Art and Images in Psychiatry
- Artificial Intelligence
- Assisted Reproduction
- Bleeding and Transfusion
- Caring for the Critically Ill Patient
- Challenges in Clinical Electrocardiography
- Climate and Health
- Climate Change
- Clinical Challenge
- Clinical Decision Support
- Clinical Implications of Basic Neuroscience
- Clinical Pharmacy and Pharmacology
- Complementary and Alternative Medicine
- Consensus Statements
- Coronavirus (COVID-19)
- Critical Care Medicine
- Cultural Competency
- Dental Medicine
- Dermatology
- Diabetes and Endocrinology
- Diagnostic Test Interpretation
- Drug Development
- Electronic Health Records
- Emergency Medicine
- End of Life, Hospice, Palliative Care
- Environmental Health
- Equity, Diversity, and Inclusion
- Facial Plastic Surgery
- Gastroenterology and Hepatology
- Genetics and Genomics
- Genomics and Precision Health
- Global Health
- Guide to Statistics and Methods
- Hair Disorders
- Health Care Delivery Models
- Health Care Economics, Insurance, Payment
- Health Care Quality
- Health Care Reform
- Health Care Safety
- Health Care Workforce
- Health Disparities
- Health Inequities
- Health Policy
- Health Systems Science
- History of Medicine
- Hypertension
- Images in Neurology
- Implementation Science
- Infectious Diseases
- Innovations in Health Care Delivery
- JAMA Infographic
- Law and Medicine
- Leading Change
- Less is More
- LGBTQIA Medicine
- Lifestyle Behaviors
- Medical Coding
- Medical Devices and Equipment
- Medical Education
- Medical Education and Training
- Medical Journals and Publishing
- Mobile Health and Telemedicine
- Narrative Medicine
- Neuroscience and Psychiatry
- Notable Notes
- Nutrition, Obesity, Exercise
- Obstetrics and Gynecology
- Occupational Health
- Ophthalmology
- Orthopedics
- Otolaryngology
- Pain Medicine
- Palliative Care
- Pathology and Laboratory Medicine
- Patient Care
- Patient Information
- Performance Improvement
- Performance Measures
- Perioperative Care and Consultation
- Pharmacoeconomics
- Pharmacoepidemiology
- Pharmacogenetics
- Pharmacy and Clinical Pharmacology
- Physical Medicine and Rehabilitation
- Physical Therapy
- Physician Leadership
- Population Health
- Primary Care
- Professional Well-being
- Professionalism
- Psychiatry and Behavioral Health
- Public Health
- Pulmonary Medicine
- Regulatory Agencies
- Reproductive Health
- Research, Methods, Statistics
- Resuscitation
- Rheumatology
- Risk Management
- Scientific Discovery and the Future of Medicine
- Shared Decision Making and Communication
- Sleep Medicine
- Sports Medicine
- Stem Cell Transplantation
- Substance Use and Addiction Medicine
- Surgical Innovation
- Surgical Pearls
- Teachable Moment
- Technology and Finance
- The Art of JAMA
- The Arts and Medicine
- The Rational Clinical Examination
- Tobacco and e-Cigarettes
- Translational Medicine
- Trauma and Injury
- Treatment Adherence
- Ultrasonography
- Users' Guide to the Medical Literature
- Vaccination
- Venous Thromboembolism
- Veterans Health
- Women's Health
- Workflow and Process
- Wound Care, Infection, Healing
Get the latest research based on your areas of interest.
Others also liked.
- Download PDF
- X Facebook More LinkedIn
Brittain EL , Han L , Annis J, et al. Physical Activity and Incident Obesity Across the Spectrum of Genetic Risk for Obesity. JAMA Netw Open. 2024;7(3):e243821. doi:10.1001/jamanetworkopen.2024.3821
Manage citations:
© 2024
- Permissions
Physical Activity and Incident Obesity Across the Spectrum of Genetic Risk for Obesity
- 1 Division of Cardiovascular Medicine, Vanderbilt University Medical Center, Nashville, Tennessee
- 2 Center for Digital Genomic Medicine, Department of Medicine, Vanderbilt University Medical Center, Nashville, Tennessee
- 3 Division of Genetic Medicine, Vanderbilt Genetics Institute, Vanderbilt University Medical Center, Nashville, Tennessee
- 4 Vanderbilt Institute of Clinical and Translational Research, Vanderbilt University Medical Center, Nashville, Tennessee
- 5 Department of Medicine, Vanderbilt University Medical Center, Nashville, Tennessee
- 6 Department of Pharmacology, Vanderbilt University Medical Center, Nashville, Tennessee
- 7 Department of Biomedical Informatics, Vanderbilt University Medical Center, Nashville, Tennessee
- 8 Department of Biomedical Engineering, Vanderbilt University Medical Center, Nashville, Tennessee
- 9 Department of Biostatistics, Vanderbilt University Medical Center, Nashville, Tennessee
- 10 Department of Psychiatry and Behavioral Sciences, Vanderbilt University Medical Center, Nashville, Tennessee
Question Does the degree of physical activity associated with incident obesity vary by genetic risk?
Findings In this cohort study of 3124 adults, individuals at high genetic risk of obesity needed higher daily step counts to reduce the risk of obesity than those at moderate or low genetic risk.
Meaning These findings suggest that individualized physical activity recommendations that incorporate genetic background may reduce obesity risk.
Importance Despite consistent public health recommendations, obesity rates in the US continue to increase. Physical activity recommendations do not account for individual genetic variability, increasing risk of obesity.
Objective To use activity, clinical, and genetic data from the All of Us Research Program (AoURP) to explore the association of genetic risk of higher body mass index (BMI) with the level of physical activity needed to reduce incident obesity.
Design, Setting, and Participants In this US population–based retrospective cohort study, participants were enrolled in the AoURP between May 1, 2018, and July 1, 2022. Enrollees in the AoURP who were of European ancestry, owned a personal activity tracking device, and did not have obesity up to 6 months into activity tracking were included in the analysis.
Exposure Physical activity expressed as daily step counts and a polygenic risk score (PRS) for BMI, calculated as weight in kilograms divided by height in meters squared.
Main Outcome and Measures Incident obesity (BMI ≥30).
Results A total of 3124 participants met inclusion criteria. Among 3051 participants with available data, 2216 (73%) were women, and the median age was 52.7 (IQR, 36.4-62.8) years. The total cohort of 3124 participants walked a median of 8326 (IQR, 6499-10 389) steps/d over a median of 5.4 (IQR, 3.4-7.0) years of personal activity tracking. The incidence of obesity over the study period increased from 13% (101 of 781) to 43% (335 of 781) in the lowest and highest PRS quartiles, respectively ( P = 1.0 × 10 −20 ). The BMI PRS demonstrated an 81% increase in obesity risk ( P = 3.57 × 10 −20 ) while mean step count demonstrated a 43% reduction ( P = 5.30 × 10 −12 ) when comparing the 75th and 25th percentiles, respectively. Individuals with a PRS in the 75th percentile would need to walk a mean of 2280 (95% CI, 1680-3310) more steps per day (11 020 total) than those at the 50th percentile to have a comparable risk of obesity. To have a comparable risk of obesity to individuals at the 25th percentile of PRS, those at the 75th percentile with a baseline BMI of 22 would need to walk an additional 3460 steps/d; with a baseline BMI of 24, an additional 4430 steps/d; with a baseline BMI of 26, an additional 5380 steps/d; and with a baseline BMI of 28, an additional 6350 steps/d.
Conclusions and Relevance In this cohort study, the association between daily step count and obesity risk across genetic background and baseline BMI were quantified. Population-based recommendations may underestimate physical activity needed to prevent obesity among those at high genetic risk.
In 2000, the World Health Organization declared obesity the greatest threat to the health of Westernized nations. 1 In the US, obesity accounts for over 400 000 deaths per year and affects nearly 40% of the adult population. Despite the modifiable nature of obesity through diet, exercise, and pharmacotherapy, rates have continued to increase.
Physical activity recommendations are a crucial component of public health guidelines for maintaining a healthy weight, with increased physical activity being associated with a reduced risk of obesity. 2 - 4 Fitness trackers and wearable devices have provided an objective means to capture physical activity, and their use may be associated with weight loss. 5 Prior work leveraging these devices has suggested that taking around 8000 steps/d substantially mitigates risk of obesity. 3 , 4 However, current recommendations around physical activity do not take into account other contributors such as caloric intake, energy expenditure, or genetic background, likely leading to less effective prevention of obesity for many people. 6
Obesity has a substantial genetic contribution, with heritability estimates ranging from 40% to 70%. 7 , 8 Prior studies 9 - 11 have shown an inverse association between genetic risk and physical activity with obesity, whereby increasing physical activity can help mitigate higher genetic risk for obesity. These results have implications for physical activity recommendations on an individual level. Most of the prior work 9 - 11 focused on a narrow set of obesity-associated variants or genes and relied on self-reported physical activity, and more recent work using wearable devices has been limited to 7 days of physical activity measurements. 12 Longer-term capture in large populations will be required to accurately estimate differences in physical activity needed to prevent incident obesity.
We used longitudinal activity monitoring and genome sequencing data from the All of Us Research Program (AoURP) to quantify the combined association of genetic risk for body mass index (BMI; calculated as weight in kilograms divided by height in meters squared) and physical activity with the risk of incident obesity. Activity monitoring was quantified as daily step counts obtained from fitness tracking devices. Genetic risk was quantified by using a polygenic risk score (PRS) from a large-scale genomewide association study (GWAS) of BMI. 13 We quantified the mean daily step count needed to overcome genetic risk for increased BMI. These findings represent an initial step toward personalized exercise recommendations that integrate genetic information.
Details on the design and execution of the AoURP have been published previously. 14 The present study used AoURP Controlled Tier dataset, version 7 (C2022Q4R9), with data from participants enrolled between May 1, 2018, and July 1, 2022. Participants who provided informed consent could share data from their own activity tracking devices from the time their accounts were first created, which may precede the enrollment date in AoURP. We followed the Strengthening the Reporting of Observational Studies in Epidemiology ( STROBE ) reporting guideline. In this study, only the authorized authors who completed All of Us Responsible Conduct of Research training accessed the deidentified data from the Researcher Workbench (a secured cloud-based platform). Since the authors were not directly involved with the participants, institutional review board review was exempted in compliance with AoURP policy.
Activity tracking data for this study came from the Bring Your Own Device program that allowed individuals who already owned a tracking device (Fitbit, Inc) to consent to link their activity data with other data in the AoURP. By registering their personal device on the AoURP patient portal, patients could share all activity data collected since the creation of their personal device account. For many participants, this allowed us to examine fitness activity data collected prior to enrollment in the AoURP. Activity data in AoURP are reported as daily step counts. We excluded days with fewer than 10 hours of wear time to enrich our cohort for individuals with consistently high wear time. The initial personal activity device cohort consisted of 12 766 individuals. Consistent with our prior data curation approach, days with less than 10 hours of wear time, less than 100 steps, or greater than 45 000 steps or for which the participant was younger than 18 years were removed. For time-varying analyses, mean daily steps were calculated on a monthly basis for each participant. Months with fewer than 15 valid days of monitoring were removed.
The analytic cohort included only individuals with a BMI of less than 30 at the time activity monitoring began. The primary outcome was incident obesity, defined as a BMI of 30 or greater documented in the medical record at least 6 months after initiation of activity monitoring. The latter stipulation reduced the likelihood that having obesity predated the beginning of monitoring but had not yet been clinically documented. We extracted BMI values and clinical characteristics from longitudinal electronic health records (EHRs) for the consenting participants who were associated with a health care provider organization funded by the AoURP. The EHR data have been standardized using the Observational Medical Outcomes Partnership Common Data Model. 15 In the AoURP, upon consent, participants are asked to complete the Basics survey, in which they may self-report demographic characteristics such as race, ethnicity, and sex at birth.
We filtered the data to include only biallelic, autosomal single-nucleotide variants (SNVs) that had passed AoURP initial quality control. 16 We then removed duplicate-position SNVs and kept only individual genotypes with a genotype quality greater than 20. We further filtered the SNVs based on their Hardy-Weinberg equilibrium P value (>1.0 × 10 −15 ) and missing rate (<5%) across all samples. Next, we divided the samples into 6 groups (Admixed American, African, East Asian, European, Middle Eastern, and South Asian) based on their estimated ancestral populations 16 , 17 and further filtered the SNVs within each population based on minor allele frequency (MAF) (>0.01), missing rate (<0.02), and Hardy-Weinberg equilibrium P value (>1.0 × 10 −6 ). The SNVs were mapped from Genome Reference Consortium Human Build 38 with coordinates to Build 37. Because the existing PRS models have limited transferability across ancestry groups and to ensure appropriate power of the subsequent PRS analysis, we limited our analysis to the populations who had a sample size of greater than 500, resulting in 5964 participants of European ancestry with 5 515 802 common SNVs for analysis.
To generate principal components, we excluded the regions with high linkage disequilibrium, including chr5:44-51.5 megabase (Mb), chr6:25-33.5 Mb, chr8:8-12 Mb, and chr11:45-57 Mb. We then pruned the remaining SNVs using PLINK, version 1.9 (Harvard University), pairwise independence function with 1-kilobase window shifted by 50 base pairs and requiring r 2 < 0.05 between any pair, resulting in 100 983 SNPs for further analysis. 18 Principal component analysis was run using PLINK, version 1.9. The European ancestry linkage disequilibrium reference panel from the 1000 Genomes Project phase 3 was downloaded, and nonambiguous SNPs with MAF greater than 0.01 were kept in the largest European ancestry GWAS summary statistics of BMI. 13 We manually harmonized the strand-flipping SNPs among the SNP information file, GWAS summary statistics files, and the European ancestry PLINK extended map files (.bim).
We used PRS–continuous shrinkage to infer posterior SNP effect sizes under continuous shrinkage priors with a scaling parameter set to 0.01, reflecting the polygenic architecture of BMI. GWAS summary statistics of BMI measured in 681 275 individuals of European ancestry was used to estimate the SNP weights. 19 The scoring command in PLINK, version 1.9, was used to produce the genomewide scores of the AoURP European individuals with their quality-controlled SNP genotype data and these derived SNP weights. 20 Finally, by using the genomewide scores as the dependent variable and the 10 principal components as the independent variable, we performed linear regression, and the obtained residuals were kept for the subsequent analysis. To check the performance of the PRS estimate, we first fit a generalized regression model with obesity status as the dependent variable and the PRS as the independent variable with age, sex, and the top 10 principal components of genetic ancestry as covariates. We then built a subset logistic regression model, which only uses the same set of covariates. By comparing the full model with the subset model, we measured the incremental Nagelkerke R 2 value to quantify how much variance in obesity status was explained by the PRS.
Differences in clinical characteristics across PRS quartiles were assessed using the Wilcoxon rank sum or Kruskal-Wallis test for continuous variables and the Pearson χ 2 test for categorical variables. Cox proportional hazards regression models were used to examine the association among daily step count (considered as a time-varying variable), PRS, and the time to event for obesity, adjusting for age, sex, mean baseline step counts, cancer status, coronary artery disease status, systolic blood pressure, alcohol use, educational level, and interaction term of PRS × mean steps. We presented these results stratified by baseline BMI and provided a model including baseline BMI in eFigure 2 in Supplement 1 as a secondary analysis due to collinearity between BMI and PRS.
Cox proportional hazards regression models were fit on a multiply imputed dataset. Multiple imputation was performed for baseline BMI, alcohol use, educational status, systolic blood pressure, and smoking status using bootstrap and predictive mean matching with the aregImpute function in the Hmisc package of R, version 4.2.2 (R Project for Statistical Computing). Continuous variables were modeled as restricted cubic splines with 3 knots, unless the nonlinear term was not significant, in which case it was modeled as a linear term. Fits and predictions of the Cox proportional hazards regression models were obtained using the rms package in R, version 4.2.2. The Cox proportional hazards regression assumptions were checked using the cox.zph function from the survival package in R, version 4.2.2.
To identify the combinations of PRS and mean daily step counts associated with a hazard ratio (HR) of 1.00, we used a 100-knot spline function to fit the Cox proportional hazards regression ratio model estimations across a range of mean daily step counts for each PRS percentile. We then computed the inverse of the fitted spline function to determine the mean daily step count where the HR equals 1.00 for each PRS percentile. We repeated this process for multiple PRS percentiles to generate a plot of mean daily step counts as a function of PRS percentiles where the HR was 1.00. To estimate the uncertainty around these estimations, we applied a similar spline function to the upper and lower estimated 95% CIs of the Cox proportional hazards regression model to find the 95% CIs for the estimated mean daily step counts at each PRS percentile. Two-sided P < .05 indicated statistical significance.
We identified 3124 participants of European ancestry without obesity at baseline who agreed to link their personal activity data and EHR data and had available genome sequencing. Among those with available data, 2216 of 3051 (73%) were women and 835 of 3051 (27%) were men, and the median age was 52.7 (IQR, 36.4-62.8) years. In terms of race and ethnicity, 2958 participants (95%) were White compared with 141 participants (5%) who were of other race or ethnicity (which may include Asian, Black or African American, Middle Eastern or North African, Native Hawaiian or Other Pacific Islander, multiple races or ethnicities, and unknown race or ethnicity) ( Table ). The analytic sample was restricted to individuals assigned European ancestry based on the All of Us Genomic Research Data Quality Report. 16 A study flowchart detailing the creation of the analytic dataset is provided in eFigure 1 in Supplement 1 . The BMI-based PRS explained 8.3% of the phenotypic variation in obesity (β = 1.76; P = 2 × 10 −16 ). The median follow-up time was 5.4 (IQR, 3.4-7.0) years and participants walked a median of 8326 (IQR, 6499-10 389) steps/d. The incidence of obesity over the study period was 13% (101 of 781 participants) in the lowest PRS quartile and 43% (335 of 781 participants) in the highest PRS quartile ( P = 1.0 × 10 −20 ). We observed a decrease in median daily steps when moving from lowest (8599 [IQR, 6751-10 768]) to highest (8115 [IQR, 6340-10 187]) PRS quartile ( P = .01).
We next modeled obesity risk stratified by PRS percentile with the 50th percentile indexed to an HR for obesity of 1.00 ( Figure 1 ). The association between PRS and incident obesity was direct ( P = .001) and linear (chunk test for nonlinearity was nonsignificant [ P = .07]). The PRS and mean daily step count were both independently associated with obesity risk ( Figure 2 ). The 75th percentile BMI PRS demonstrated an 81% increase in obesity risk (HR, 1.81 [95% CI, 1.59-2.05]; P = 3.57 × 10 −20 ) when compared with the 25th percentile BMI PRS, whereas the 75th percentile median step count demonstrated a 43% reduction in obesity risk (HR, 0.57 [95% CI, 0.49-0.67]; P = 5.30 × 10 −12 ) when compared with the 25th percentile step count. The PRS × mean steps interaction term was not significant (χ 2 = 1.98; P = .37).
Individuals with a PRS at the 75th percentile would need to walk a mean of 2280 (95% CI, 1680-3310) more steps per day (11 020 total) than those at the 50th percentile to reduce the HR for obesity to 1.00 ( Figure 1 ). Conversely, those in the 25th percentile PRS could reach an HR of 1.00 by walking a mean of 3660 (95% CI, 2180-8740) fewer steps than those at the 50th percentile PRS. When assuming a median daily step count of 8740 (cohort median), those in the 75th percentile PRS had an HR for obesity of 1.33 (95% CI, 1.25-1.41), whereas those at the 25th percentile PRS had an obesity HR of 0.74 (95% CI, 0.69-0.79).
The mean daily step count required to achieve an HR for obesity of 1.00 across the full PRS spectrum and stratified by baseline BMI is shown in Figure 3 . To reach an HR of 1.00 for obesity, when stratified by baseline BMI of 22, individuals at the 50th percentile PRS would need to achieve a mean daily step count of 3290 (additional 3460 steps/d); for a baseline BMI of 24, a mean daily step count of 7590 (additional 4430 steps/d); for a baseline BMI of 26, a mean daily step count of 11 890 (additional 5380 steps/d); and for a baseline BMI of 28, a mean daily step count of 16 190 (additional 6350 steps/d).
When adding baseline BMI to the full Cox proportional hazards regression model, daily step count and BMI PRS both remain associated with obesity risk. When comparing individuals at the 75th percentile with those at the 25th percentile, the BMI PRS is associated with a 61% increased risk of obesity (HR, 1.61 [95% CI, 1.45-1.78]). Similarly, when comparing the 75th with the 25th percentiles, daily step count was associated with a 38% lower risk of obesity (HR, 0.62 [95% CI, 0.53-0.72]) (eFigure 2 in Supplement 1 ).
The cumulative incidence of obesity increases over time and with fewer daily steps and higher PRS. The cumulative incidence of obesity would be 2.9% at the 25th percentile, 3.9% at the 50th percentile, and 5.2% at the 75th percentile for PRS in year 1; 10.5% at the 25th percentile, 14.0% at the 50th percentile, and 18.2% at the 75th percentile for PRS in year 3; and 18.5% at the 25th percentile, 24.3% at the 50th percentile, and 30.9% at the 75th percentile for PRS in year 5 ( Figure 4 ). The eTable in Supplement 1 models the expected cumulative incidence of obesity at 1, 3, and 5 years based on PRS and assumed mean daily steps of 7500, 10 000, and 12 500.
We examined the combined association of daily step counts and genetic risk for increased BMI with the incidence of obesity in a large national sample with genome sequencing and long-term activity monitoring data. Lower daily step counts and higher BMI PRS were both independently associated with increased risk of obesity. As the PRS increased, the number of daily steps associated with lower risk of obesity also increased. By combining these data sources, we derived an estimate of the daily step count needed to reduce the risk of obesity based on an individual’s genetic background. Importantly, our findings suggest that genetic risk for obesity is not deterministic but can be overcome by increasing physical activity.
Our findings align with those of prior literature 9 indicating that engaging in physical activity can mitigate genetic obesity risk and highlight the importance of genetic background for individual health and wellness. Using the data from a large population-based sample, Li et al 9 characterized obesity risk by genotyping 12 susceptibility loci and found that higher self-reported physical activity was associated with a 40% reduction in genetic predisposition to obesity. Our study extends these results in 2 important ways. First, we leveraged objectively measured longitudinal activity data from commercial devices to focus on physical activity prior to and leading up to a diagnosis of obesity. Second, we used a more comprehensive genomewide risk assessment in the form of a PRS. Our results indicate that daily step count recommendations to reduce obesity risk may be personalized based on an individual’s genetic background. For instance, individuals with higher genetic risk (ie, 75th percentile PRS) would need to walk a mean of 2280 more steps per day than those at the 50th percentile of genetic risk to have a comparable risk of obesity.
These results suggest that population-based recommendations that do not account for genetic background may not accurately represent the amount of physical activity needed to reduce the risk of obesity. Population-based exercise recommendations may overestimate or underestimate physical activity needs, depending on one’s genetic background. Underestimation of physical activity required to reduce obesity risk has the potential to be particularly detrimental to public health efforts to reduce weight-related morbidity. As such, integration of activity and genetic data could facilitate personalized activity recommendations that account for an individual’s genetic profile. The widespread use of wearable devices and the increasing demand for genetic information from both clinical and direct-to-consumer sources may soon permit testing the value of personalized activity recommendations. Efforts to integrate wearable devices and genomic data into the EHR further support the potential future clinical utility of merging these data sources to personalize lifestyle recommendations. Thus, our findings support the need for a prospective trial investigating the impact of tailoring step counts by genetic risk on chronic disease outcomes.
The most important limitation of this work is the lack of diversity and inclusion only of individuals with European ancestry. These findings will need validation in a more diverse population. Our cohort only included individuals who already owned a fitness tracking device and agreed to link their activity data to the AoURP dataset, which may not be generalizable to other populations. We cannot account for unmeasured confounding, and the potential for reverse causation still exists. We attempted to diminish the latter concern by excluding prevalent obesity and incident cases within the first 6 months of monitoring. Genetic risk was simplified to be specific to increased BMI; however, genetic risk for other cardiometabolic conditions could also inform obesity risk. Nongenetic factors that contribute to obesity risk such as dietary patterns were not available, reducing the explanatory power of the model. It is unlikely that the widespread use of drug classes targeting weight loss affects the generalizability of our results, because such drugs are rarely prescribed for obesity prevention, and our study focused on individuals who were not obese at baseline. Indeed, less than 0.5% of our cohort was exposed to a medication class targeting weight loss (phentermine, orlistat, or glucagonlike peptide-1 receptor agonists) prior to incident obesity or censoring. Finally, some fitness activity tracking devices may not capture nonambulatory activity as well as triaxial accelerometers.
This cohort study used longitudinal activity data from commercial wearable devices, genome sequencing, and clinical data to support the notion that higher daily step counts can mitigate genetic risk for obesity. These results have important clinical and public health implications and may offer a novel strategy for addressing the obesity epidemic by informing activity recommendations that incorporate genetic information.
Accepted for Publication: January 30, 2024.
Published: March 27, 2024. doi:10.1001/jamanetworkopen.2024.3821
Open Access: This is an open access article distributed under the terms of the CC-BY License . © 2024 Brittain EL et al. JAMA Network Open .
Corresponding Author: Evan L. Brittain, MD, MSc ( [email protected] ) and Douglas M. Ruderfer, PhD ( [email protected] ), Vanderbilt University Medical Center, 2525 West End Ave, Suite 300A, Nashville, TN 37203.
Author Contributions: Drs Brittain and Ruderfer had full access to all of the data in the study and take responsibility for the integrity of the data and the accuracy of the data analysis.
Concept and design: Brittain, Annis, Master, Roden, Ruderfer.
Acquisition, analysis, or interpretation of data: Brittain, Han, Annis, Master, Hughes, Harris, Ruderfer.
Drafting of the manuscript: Brittain, Han, Annis, Master, Ruderfer.
Critical review of the manuscript for important intellectual content: All authors.
Statistical analysis: Brittain, Han, Annis, Master.
Obtained funding: Brittain, Harris.
Administrative, technical, or material support: Brittain, Annis, Master, Roden.
Supervision: Brittain, Ruderfer.
Conflict of Interest Disclosures: Dr Brittain reported receiving a gift from Google LLC during the conduct of the study. Dr Ruderfer reported serving on the advisory board of Illumina Inc and Alkermes PLC and receiving grant funding from PTC Therapeutics outside the submitted work. No other disclosures were reported.
Funding/Support: The All of Us Research Program is supported by grants 1 OT2 OD026549, 1 OT2 OD026554, 1 OT2 OD026557, 1 OT2 OD026556, 1 OT2 OD026550, 1 OT2 OD 026552, 1 OT2 OD026553, 1 OT2 OD026548, 1 OT2 OD026551, 1 OT2 OD026555, IAA AOD21037, AOD22003, AOD16037, and AOD21041 (regional medical centers); grant HHSN 263201600085U (federally qualified health centers); grant U2C OD023196 (data and research center); 1 U24 OD023121 (Biobank); U24 OD023176 (participant center); U24 OD023163 (participant technology systems center); grants 3 OT2 OD023205 and 3 OT2 OD023206 (communications and engagement); and grants 1 OT2 OD025277, 3 OT2 OD025315, 1 OT2 OD025337, and 1 OT2 OD025276 (community partners) from the National Institutes of Health (NIH). This study is also supported by grants R01 HL146588 (Dr Brittain), R61 HL158941 (Dr Brittain), and R21 HL172038 (Drs Brittain and Ruderfer) from the NIH.
Role of the Funder/Sponsor: The NIH had no role in the design and conduct of the study; collection, management, analysis, and interpretation of the data; preparation, review, or approval of the manuscript; and decision to submit the manuscript for publication.
Data Sharing Statement: See Supplement 2 .
Additional Contributions: The All of Us Research Program would not be possible without the partnership of its participants.
- Register for email alerts with links to free full-text articles
- Access PDFs of free articles
- Manage your interests
- Save searches and receive search alerts
Open Access is an initiative that aims to make scientific research freely available to all. To date our community has made over 100 million downloads. It’s based on principles of collaboration, unobstructed discovery, and, most importantly, scientific progression. As PhD students, we found it difficult to access the research we needed, so we decided to create a new Open Access publisher that levels the playing field for scientists across the world. How? By making research easy to access, and puts the academic needs of the researchers before the business interests of publishers.
We are a community of more than 103,000 authors and editors from 3,291 institutions spanning 160 countries, including Nobel Prize winners and some of the world’s most-cited researchers. Publishing on IntechOpen allows authors to earn citations and find new collaborators, meaning more people see your work not only from your own field of study, but from other related fields too.
Brief introduction to this section that descibes Open Access especially from an IntechOpen perspective
Want to get in touch? Contact our London head office or media team here
Our team is growing all the time, so we’re always on the lookout for smart people who want to help us reshape the world of scientific publishing.
Home > Books > Genetic Disorders
Genetics and Obesity
Submitted: 25 April 2012 Published: 09 January 2013
DOI: 10.5772/52403
Cite this chapter
There are two ways to cite this chapter:
From the Edited Volume
Genetic Disorders
Edited by Maria Puiu
To purchase hard copies of this book, please contact the representative in India: CBS Publishers & Distributors Pvt. Ltd. www.cbspd.com | [email protected]
Chapter metrics overview
4,666 Chapter Downloads
Impact of this chapter
Total Chapter Downloads on intechopen.com

Total Chapter Views on intechopen.com
Overall attention for this chapters
Author Information
Maria puiu *.
- University of Medicine and Pharmacy "Victor Babes" Timisoara - Genetics Department; Emergency Hospital for Children "Louis Turcanu" Timisoara - Genetics Department, Romania
Adela Chirita Emandi
- University of Medicine and Pharmacy "Victor Babes" Timisoara - Pediatrics Department; Emergency Hospital for Children "Louis Turcanu" Timisoara - Pediatrics Department, Romania
Smaranda Arghirescu
*Address all correspondence to: [email protected]
1. Introduction
Obesity, in both children and adults, has reached epidemic proportions in multiple countries worldwide, with serious health problems and imposing a substantial economic burden on societies [ 1 – 4 ]. The increase in obesity prevalence among children is particularly alarming because obesity-related complications, including obesity-associated sleep apnea,[ 5 ] non-alcoholic fatty liver disease [ 6 ], and type 2 diabetes,[ 7 ] are increasingly diagnosed in pediatric patients. Excess weight in children may increase the likelihood of heart disease in adulthood as a result of the early establishment of risk factors [ 8 ]. Pediatric obesity has been shown to have a tremendous impact on later health [ 8 ], even independent of adult weight [ 9 ]. Additionally, childhood obesity is linked with important psychosocial consequences and poor general quality of life [ 10 ].
In order to create the best management programs and determine novel therapeutic targets, it becomes essential to understand the factors causing today’s rising epidemic of childhood obesity.
Obesity develops as a result of dietary and lifestyle factors, but studies also suggest a genetic influence on obesity [ 11 ]. Obesity is highly influenced by genetics; available data suggest that 40% to 77% of the observed variance in human body weight can be accounted for, by inherited factors [ 12 – 14 ]. Obesity, also just as clearly has environmental causes; our genetic endowments have changed minimally during the last 40 years, yet the prevalence of childhood obesity has tripled in US [ 15 ] and significantly increased worldwide [ 16 ], an observation that can only be explained by changes in external factors affecting children’s energetic balance.
This chapter provides an overview of the current knowledge on genetic factors implicated in the obesity epidemic.
Methodology
This review chapter has been developed using an evidence-based approach. Data from clinical and observational studies, review articles and twin studies were all considered when shaping this review. Literature searches for topics relating to genetic obesity were carried out in PubMed and EMBASE between 10 June and 10 August 2012, using Medical Subject Heading Terms and relevant keywords. To ensure relevance to the modern day clinical setting, literature searches were limited to articles published since 1 January 2000. Older, historically significant, articles identified by the authors were also included. Only articles from the peer-reviewed literature were included in the literature search. Articles in a non-English language were not included. Abstracts from industry-sponsored meetings were not included.
2. Hypothesis for etiology of the obesity epidemic
Currently, there are quite a few theories that intend to explain the etiology of human obesity: the thrifty gene hypothesis, the fetal programming hypothesis, the predation release hypothesis, the sedentary lifestyle hypothesis, the ethnic shift hypothesis, the increased reproductive fitness hypothesis, the assortative mating hypothesis, and the complex hypothesis [ 17 ]. However, an acceptable consensus in the field is still lacking, probably due to the fact that the development of obesity comes from highly complex interactions. The vast majority of genetic factors are presumed to affect body weight enough to cause obesity, only when specific environmental conditions pertain.
The thrifty gene hypothesis was proposed by Neel in 1962 [ 18 ] and suggests we evolved genes for efficient food collection and fat deposition, in order to survive periods of famine. Now, that food is continuously available, these genes are disadvantageous because they make us obese in preparation for a famine that never comes. In respect to this hypothesis, natural selection favored individuals carrying thrifty alleles that promote the storage of fat and energy. Polynesians likely experienced long periods of cold stress and starvation during their settlement of the Pacific and today have high rates of obesity and type 2 diabetes, possibly due to past positive selection for thrifty alleles [ 19 ].
A growing body of empirical evidence suggests the concept of fetal programming of health and disease risk. The origins of obesity and metabolic dysfunction can be traced back to the intrauterine period of life, at which time the developing fetus is influenced by suboptimal conditions during critical periods of cellular proliferation, differentiation, and maturation by producing structural and functional changes in cells, tissues and organ systems. These changes, in turn, may have long-term consequences to increase the individual's risk for developing obesity and metabolic dysfunction [ 20 – 23 ]. The major nutrition-related pathways discussed in the current literature relate to the effects of maternal nutritional insults on maternal-placental-fetal glucose/insulin physiology and their downstream effects on the developing brain and peripheral systems in the fetal compartment. Also, the potential role of intrauterine stress and stress biology is brought up for discussion in developmental programming of health and disease susceptibility [ 23 ]. In the early 1990s, Hales and Barker et al. [ 24 ], published a paper in Diabetologia postulating that not only type 2 diabetes, but also the key components of the metabolic syndrome, seemed to have at least parts of their origin in early life. Authors proved, in an unselected population sample (407 men) from Hertfordshire, UK, a direct link between low weight at birth and increased risks of developing type 2 diabetes, hypertension, elevated triglycerides and insulin resistance later in life. Thus, showing the role of fetal programming in itself and equally as important, the notion that fetal programming could represent a significant player in the origin of type 2 diabetes, the metabolic syndrome and cardiovascular disease. However, the extent to which the global diabetes epidemic may be driven by a mismatch between being born with a low birth weight and the fast propagation of overnutrition and physical inactivity, seen over recent years in developing countries, needs to be further determined [ 25 ].
The predation release hypothesis , also called the “drifty gene” hypothesis, is opposing the thrifty gene hypothesis, because famines are relatively infrequent modern phenomena that involve insufficient mortality for thrifty genes to propagate. Speakman suggests that early hominids would have been subjected to stabilizing selection for body fatness, with obesity selected against the risk of predation. Around two million years ago predation was removed as a significant factor by the development of social behavior, weapons, and fire. The absence of predation led to a change in the population distribution of body fatness due to random mutations and drift. This novel hypothesis involves random drift, rather than directed selection, thus, explaining why, even in Western society, most people are not obese [ 26 , 27 ].
The sedentary lifestyle together with excessive energy intake is the most popular etiologic hypothesis for the worldwide increasing prevalence of overweight and obesity [ 28 – 30 ].
The ethnic shift hypothesis , claims the mounting obesity rates are due to disproportionate prevalence in the fastest growing ethnicities (namely Hispanic Americans) [ 31 , 32 ].
The increased reproductive fitness hypothesis is also debated as a possible cause for obesity epidemic. Reproductive fitness can be defined as the capacity to pass on one's DNA. It is postulated that body mass index-associated reproductive fitness (natural selection) increases obesity prevalence, due to the fact that BMI has a genetic component and because individuals genetically predisposed toward higher BMIs, reproduce at a higher rate, than do individuals genetically predisposed toward lower BMIs [ 33 – 35 ].
The assortative mating hypothesis proposed by Hebebrand et al. [ 36 ] emphasizes the fact that the current obesity epidemic has a genetic component mediated by increased rates of assortative mating for body fatness. Theoretically, the genetic consequences of assortative mating for complex traits, such as obesity, are expected to become more significant as the correlation between genotype and phenotype (penetrance) increases, even if rates of assortative mating remain constant across generations [ 37 , 38 ]. Spouse concordance for obesity was associated with a 20-fold higher obesity risk for biologic offspring compared with children of concordantly non-obese parents [ 37 ].
The complex hypothesis focuses on the shared nature of common alleles in related common disorders, including obesity. This model, the common variants/multiple disease hypothesis, emphasizes that many disease genes may not be disease specific. Common deleterious alleles, found at a relatively high frequency in the population may play a cumulative role in related clinical phenotypes in the context of different genetic backgrounds and under different environmental conditions [ 39 ].
Genes can favor fat accumulation in a given environment by increased desire to overeat; the tendency to be sedentary; a diminished ability to utilize dietary fats as fuel; an enlarged, easily stimulated capacity to store body fat. The variation in how people respond to the same environmental conditions is an additional indication that genes play an important role in the development of obesity [ 40 ]. This is also consistent with the notion that obesity results from genetic variation interacting with shifting environmental conditions. The influence of genes ranges from polygenic genetic predisposition with impact on appetite, metabolism, and the deposition of fat, to rare monogenic disorders where obesity is the primary feature. Arduous efforts have been made by the scientific society to better understand the physiological basis of obesity. Crucial to this research is the inquiry of how does our body control ingestion, digestion, absorption, and metabolism and how nutrients are distributed among various tissues, organs, and systems [ 41 ]. Simultaneously, there is a growing interest regarding the role of genetics in further explaining feeding regulatory systems [ 14 ].
3. Genetic research methods used for identification of obesity susceptibility genes
The genetic contribution to common obesity has been established initially through family, twin, and adoption studies. Twin studies have shown a relatively high heritability ranging from 40%-77% [ 12 – 14 ]. However, the search for obesity susceptibility genes has been an arduous task. Gene identification for the last 15 years has been based on two broad genetic epidemiological approaches (candidate gene and genome-wide linkage methods). Recently, genome-wide association studies have brought great information on obesity related genes.
Candidate gene methods
The candidacy of a gene for obesity is based on the following resources: animal models using gene knockout and transgenic approaches; cellular model systems showing their role in metabolic pathways involved in glucose metabolism; linkage and positional cloning studies using extreme cases. This approach emphasizes on an association between a variant or mutation within or near the candidate gene and a trait of interest (such as obesity). Candidate gene approach needs to be on a large scale and well powered, in order to detect the expected small effects of genetic variants involved in common traits and disease [ 42 ].
The latest update of the Human Obesity Gene Map reported 127 candidate genes for obesity-related traits. Results of large-scale studies suggest that obesity is strongly associated with genetic variants in the melanocortin-4 receptor (MC4R) gene, leptin gene, adrenergic β 3 receptor (ADRB3) gene, prohormone convertase 1 (PCSK1) gene, brain-derived neurotrophic factor (BDNF) gene, and endocannabinoid receptor 1 (CNR1) gene [ 43 , 44 ].
Genome-wide linkage studies
Genome-wide linkage studies, through surveying the whole genome, aim to identify new, unanticipated genetic variants associated with a disease or trait of interest. Genome-wide linkage studies rely on the relatedness of study participants and test whether certain chromosomal regions cosegregate with a disease or trait across generations [ 42 ]. The latest Human Obesity Gene Map update reported 253 loci from 61 genome-wide linkage scans, of which 15 loci have been replicated in at least three studies [ 45 ]. Yet, none of these replicated loci could be narrowed down sufficiently to pinpoint the genes or variants that underline the linkage signal.
Genome-wide association studies
Genome-wide association studies are used in genetics research to look for associations between many (typically hundreds of thousands) of specific genetic variations (most commonly, single nucleotide polymorphisms -SNP) and particular diseases or traits. Similar to genome-wide linkage, the genome-wide association approach sweeps the entire genome, unrestricted by prior assumptions. Genome-wide association studies screen the whole genome at higher resolution levels than genome-wide linkage studies and are capable to narrow down the associated locus more accurately. The genome-wide association approach has effectively replaced genome-wide linkage approach for common disease [ 42 ].
Recent success of genome-wide association studies has drawn a lot of attention. High-density multistage genome-wide association analyses have so far discovered ~30 loci consistently associated with BMI and obesity-related traits. The strongest signal remains the association with variants within FTO (the fat-mass and obesity-related gene). Other signals near BDNF, SH2B1, and NEGR1 (all implicated in aspects of neuronal function),further support the idea that obesity is a disorder of hypothalamic function [ 42 ].
4. The influential role of genes in obesity
The strongest risk factor for childhood and adolescent obesity is parental obesity [ 46 ]. The risk becomes especially elevated if both parents are obese [ 47 ]. However, obesity inheritance does not usually follow classic Mendelian patterns. A combination of gene mutations, deletions and single nucleotide polymorphisms are all known to contribute to obesity. Most cases are polygenic, the result of multiple genes interacting with a shifting environment. Each “obesity gene” only makes a small contribution to phenotype, but collectively, inherited genetic variations play a major role in determining body mass and how the body maintains a balance between physical activity and nutrition. While obesity is most commonly associated with polygenic inheritance, there are other instances in which the cause is monogenic or syndromic. Monogenic obesity typically is caused by a single gene mutation with severe obesity as the main symptom. Syndromic obesity, on the other hand, has many characteristics, of which obesity is one symptom [ 48 ].
A. Monogenic obesity , that is the obesity associated with a single gene mutation. In these cases single gene variants are sufficient by themselves to cause obesity in food abundant societies. Patients with monogenic obesity usually show extremely severe phenotypes characterized by early childhood obesity onset, often associated with additional behavioral, developmental or endocrine disorders, like hyperphagia and hypogonadism. Significant developmental delays, however, are not commonly seen.
B. Syndromic obesity includes some disorders with Mendelian inheritance, in which patients are clinically obese and are additionally characterized by mental retardation, dysmorfic features, and organ-specific developmental abnormalities.
C. Polygenic obesity , the very common kind of obesity, which concerns the great majority of obese children, arises when an individual’s genetic make-up is susceptible to an environment that promotes energy consumption over energy expenditure [ 49 ].
A. Monogenic obesity
A “monogene” is by textbook definition, a gene with a strong effect on the phenotype (Mendelian traits or Mendelian - single gene conditions), giving rise to a one-on-one relationship between genotype and phenotype. A “major gene” is defined as a gene harboring, a variant which is associated with a high lifetime risk for a disease. Modifier genes and environmental factors additionally play a role in the etiology of the respective diseases [ 50 ].
The genetic causes of monogenic obesity tend to be related to the leptin-melanocortin pathway. This pathway is critical for energy balance and food intake; a disruption in this pathway will lead to severe obesity. Energy homeostasis involves the integration of afferent signals from fat (leptin) and pancreatic beta cells (insulin) and meal-related afferent signals from the gut. These inputs are integrated within the brain and regulate food intake, energy expenditure, energy partitioning and neuroendocrine status. Table 1 . summarizes the peptides proposed to affect appetite regulation (adapted from Burrage and McCandless 2007) [ 51 ].
Leptin is an adipocyte-derived hormone that is secreted proportionally to body fat content, it crosses the blood–brain barrier, and stimulates a subset of neurons in the hypothalamus to produce peptides that reduce feeding and promote increased energy expenditure (leptin–melanocortin pathway). Additionally, leptin inhibits hypothalamic neurons that produce peptides promoting feeding and decreased energy expenditure.
Attention has focused on identifying the molecular events that lie downstream of the leptin receptor in hypothalamic target neurons. In particular, neurons within the hypothalamus act as primary sensors of alterations in energy stores to control appetite and energy homeostasis. Pro-opiomelanocortin (POMC) neurons produce the anorectic peptide a-MSH (a-melanocyte stimulating hormone) together with CART (cocaine and amphetamine-related transcript), whilst a separate group expresses the orexigens: neuropeptide Y (NPY) and agouti-related protein (AGRP). AGRP is a hypothalamic neuropeptide that is a potent melanocortin-3 receptor (MC3R) and melanocortin-4 receptor (MC4R) antagonist. Activation of the NPY/AGRP neurons increases food intake and decreases energy expenditure, whereas activation of the POMC neurons decreases food intake and increases energy expenditure [ 52 ].
Peptides proposed to affect appetite regulation. adapted from Burrage and McCandless, 2007
The cumulative prevalence of monogenic obesity among children with severe obesity is about 5% [ 44 ]. Several monogenic disorders resulting from disruption of the leptin–melanocortin pathway have been identified. In these disorders, severe obesity of early onset is itself the predominant presenting feature, although often accompanied by characteristic patterns of neuroendocrine dysfunction. Mutations in the melanocortin-4 receptor gene ( MC4R ) and the leptin receptor gene ( LEPR ) have been reported in about 2.5 % and 1.5 % of children with severe obesity. An additional 0.5 % of cases can be attributed to a chromosome 16p11.2 deletion where a gene known as SH2B1 is deleted [ 48 ].
Congenital leptin deficiency
In 1997 two severely obese cousins were reported from a highly consanguineous family of Pakistani origin [ 53 ]. Despite their severe obesity, both children had undetectable levels of serum leptin and a mutation in the gene encoding leptin. Leptin deficiency is associated with hyperphagia and increased energy intake. Other phenotypic features include hypogonadotropic hypogonadism, elevated plasma insulin, T-cell abnormalities, and advanced bone age [ 54 ].
The role of leptin in some monogenic forms of obesity was further supported by the striking effect of leptin replacement in an extremely obese child with congenital leptin deficiency. In a 9-year-old boy with congenital leptin deficiency, daily subcutaneous injection of recombinant human leptin for a year, led to a complete reversal of obesity, with sustained fat-mass loss. Moreover, partial leptin deficiency in 13 Pakistani subjects, due to a heterozygous frame shift mutation in the leptin gene, was found to be associated with increased body fat [ 55 , 56 ]. However, only a handful of families with extreme forms of obesity in early infancy have mutations in these genes [ 57 ].
Mutation in the leptin receptor
Shortly after leptin deficiency was discovered, a similar phenotype, but with elevated plasma leptin levels, was identified [ 58 ]. The cause was a homozygous mutation in the leptin receptor. A later study suggested that approximately 3% of severe morbid obesity in a population including both non-consanguineous and consanguineous families could be explained by mutations in the leptin receptor [ 59 ].
Melanocortin-4 receptor deficiency (MC4R)
Mutations in another component of the leptin–melanocortin pathway melanocortin-4 receptor have also been associated with obesity. MC4R deficiency represents the most common monogenic obesity disorder that has been identified so far. It is present in about 5-6% of obese individuals from different ethnic groups, with a higher prevalence in cases with increased severity and earlier age of onset [ 60 , 61 ]. Affected subjects exhibit hyperphagia, but this is not as severe as that seen in leptin deficiency, although it often starts in the first year of life. Alongside the increase in fat mass, MC4R-deficient subjects also have an increase in lean mass, that is not seen in leptin deficiency and a marked increase in bone mineral density. The accelerated linear growth is apparently not related to a dysfunction of the GH axis and may be a consequence of the disproportionate early hyperinsulinaemia. Interestingly, both heterozygous and homozygous mutations in MC4R have been implicated in obesity, but extreme obesity is incompletely penetrant in heterozygous patients. In other words, some individuals with a single copy of the mutation are obese, whereas others are not obese [ 51 ].
Currently, there is no specific therapy for MC4R deficiency, however, it is highly likely that these subjects would respond well to pharmacotherapy that overcame the reduction in the hypothalamic melanocortinergic tone that exists in these patients [ 12 ].
Pro-opiomelanocortin (POMC) deficiency
Small numbers of patients have been described with mutations in the gene encoding pro-opiomelanocortin, which is involved in the leptin-melanocortin pathway [ 62 , 63 ]. In neonatal life, these patients present with adrenal crisis due to ACTH deficiency (POMC is a precursor of ACTH in the pituitary), also, the children have pale skin and red hair due to the lack of MSH action at melanocortin-1 receptors in the skin and hair follicles. POMC deficiency results in hyperphagia and early-onset obesity due to loss of melanocortin signaling at the melanocortin-4 receptor (MC4R) [ 12 ].
Prohormone convertase-1 (PC1) deficiency
Jackson et al described a woman with severe early-onset obesity, hypogonadotropic hypogonadism, postprandial hypoglycaemia, hypocortisolemia, and evidence of impaired processing of POMC and proinsulin who was a compound heterozygote for prohormone convertase-1 mutations [ 63 ].
Although great hope was invested in the studies of patients with early-onset severe obesity, they have revealed the identity of very few genes associated with obesity. Interestingly, the few gene mutations associated with morbid obesity appear to influence body weight primarily by altering appetite. Some of the molecules may also impact activity, but this has not yet been shown to be a significant contributor to obesity. A significant limitation of the strategy of focusing on morbid obesity is that mutations or genetic variants in these genes may not be associated with more common forms of the condition [ 51 ].
B. Syndromic obesity
Syndromic obesity is represented by at least 20 rare syndromes (shown in Table 2) , that are caused by discrete genetic defects or chromosomal abnormalities, both autosomal and X-linked, that are characterized by obesity. Most of these obesity syndromes associate mental retardation.
It was expected that the syndromic forms of obesity could help unravel novel genes relevant for idiopathic obesity. However, although the genes for several of the syndromic forms have been detected, the relevance of these genes for general obesity is still unclear [ 45 , 57 ].
Syndromes characterized by obesity.
Prader–Willi syndrome
Prader–Willi syndrome (PWS) is the most frequent of these syndromes (1 in 25,000 births). It is an autosomal-dominant disorder, characterized by obesity, hyperphagia, muscular hypotonia, mental retardation, short stature and hypogonadotropic hypogonadism. It is usually caused by a paternally inherited deletion at the chromosomal region 15q11.2–q12, and less frequently by maternal uniparental disomy (Orphanet). The cause of hyperphagia in PWS is not proven, although PWS phenotypes are consistent with a combined hypothalamic impairment, causing several endocrine abnormalities. Also, it was suggested that the elevated production of the stomach secreted peptide ghrelin seen in PWS might increase appetite by interacting with the POMC/CART and NPY hypothalamic neurons [ 57 ].
Single Minded Homologue 1 (SIM-1)
The loss of the single minded homologue 1 (SIM1) gene has also been associated with hyperphagia in syndromic obesity. This gene encodes a transcription factor that has a pivotal role in neurogenesis. In humans, deletion or disruption of the SIM1 region results in either a “Prader–Willi-like” phenotype or a form of early-onset obesity, associated with excessive food intake [ 64 ].
WAGR Syndrome
The WAGR syndrome (Wilms tumor, aniridia, genitourinary anomalies and mental retardation syndrome and obesity) is caused by heterozygous contiguous gene deletions that involve at least two genes, WT1 and PAX6 , which are present in the 11p13 region. Although persons with the WAGR syndrome typically have low-normal birth weight, marked obesity subsequently develops in a substantial subgroup [ 65 ].
Pseudohypoparathyroidism type 1A (PHP1A)
PHP1A syndrome is due to a maternally transmitted mutation in GNAS1, which encodes the α-subunit of the Gs protein. Food-intake abnormalities in patients with this syndrome might be due to the expression of the resulting variant Gs protein in the hypothalamic circuitry that controls energy balance, which involves many G-protein coupled receptors [ 66 ].
Bardet–Biedl syndrome (BBS)
Bardet–Biedl syndrome is characterized by six main features: Rod-Cone Dystrophy (the most frequent phenotype), polydactyly, learning disabilities, hypogonadism in males, renal abnormalities and obesity. In BBS patients, obesity has early onset, usually arising within the first few years of life. However, one study of post-pubertal BBS patients found that only 52% were clinically obese; therefore, this syndrome can present with a heterogeneous phenotype [ 67 ].
Albright’s hereditary osteodystrophy
Albright’s hereditary osteodystrophy describes a constellation of physical features, including short adult stature, obesity, brachydactyly, and ectopic ossifications. It is an autosomal dominant disorder due to germline mutations in GNAS1, which encodes for a-subunit of the stimulatory G protein (Gsa) [ 68 ].
Fragile X syndrome
Fragile X syndrome is characterized by moderate to severe mental retardation, macroorchidism, large ears, macrocephaly, prominent jaw (mandibular prognathism), high-pitched jocular speech and obesity. Fragile X syndrome is an X-linked, single gene disorder caused by dysfunction in the transcription of the FMR1 gene that codes for fragile X mental retardation protein (FMRP) [ 69 ].
Borjeson–Forssman–Lehmann syndrome
Borjeson, Forssman and Lehmann described a syndrome characterized by moderate to severe mental retardation, epilepsy, hypogonadism, and obesity with marked gynecomastia [ 70 ]. Mutations in a novel, widely expressed zinc-finger gene plant homeodomain (PHD)-like finger (PHF6) have been identified in affected families, although the functional properties of this protein remain unclear [ 71 ].
Alstrom syndrome
Alstrom syndrome is a homogeneous autosomal recessive disorder that is characterized by childhood obesity associated with hyperinsulinaemia, chronic hyperglycemia and neurosensory deficits. Subsets of affected individuals present with additional features such as dilated cardiomyopathy, hepatic dysfunction, hypothyroidism, male hypogonadism, short stature and mild to moderate developmental delay. Symptoms first appear in infancy and progressive development of multi-organ pathology leads to a reduced life expectancy. Variability in age of onset and severity of clinical symptoms, even within families, is likely due to genetic background. Mutations in a single gene, ALMS1, have been found to be responsible for all cases of Alstrom syndrome [ 72 ].
C. Complex polygenic obesity
Complex polygenic obesity represents the end result of behavioral, environmental, and genetic factors that may influence individual responses to diet and physical activity. Changes in our environment over the last decades, in particular the unlimited supply of cheap, highly palatable, energy-dense foods; plus a sedentary lifestyle, the so called “obesogenic” environment together with a genetic susceptibility are the culprits for today’s obesity epidemic [ 73 ].
Compared with obesity syndromes or single-gene obesity, the recent rapid increase in prevalence of childhood obesity suggests that environmental factors most likely have a larger impact on body weight in common obesity patients, although individual responses to these environmental factors are influenced by genetic factors -“susceptibility genes”.
Some traits can be due to simultaneous presence of DNA variation in multiple genes. Any of a group of alleles, at distinct gene loci that collectively control the inheritance of a quantitative phenotype or modify the expression of a qualitative character, are termed “polygenic” variants. It is generally assumed that for quantitative traits, each allele has a small effect, but the allelic effects can be additive or non-additive. Potentially, many such polygenic variants play a role in body weight regulation. It is estimated that the total number of genes with a small effect most likely exceeds 100 [ 50 ].
a Either in the GWAS or the initial sample
b Reported in the population-based cohorts EPIC, FINRISK97, BPPP and METSIM (N = 18,812)
c Reported for the Islandic sample (N = 25,344)
d Reported for children from the Northern Finland Birth Cohort (N = 5,291)
NEGR1: neuronal growth factor regulator 1; SEC16B; cerevisiae, homolog of, B; RASAL2: RAS protein activator like 2; TMEM18: transmembrane protein 18, INSIG2: insulin induced gene 2, SFRS10: splicing factor, arginine/serine-rich, 10; ETV5:etsvariant 5; DGKG diacylglycerol kinase, gamma, 90kD, GNPDA2: glucosamine-6-phosphate deaminase 2; PRL: prolactin; PTER: phosphotriesterase related; BDNF: brain derived neurotrophic factor; MTCH2: mitochondrial carrier homolog 2 (C. elegans); BCDIN3D: BCDIN3 domain containing; FAIM2: Fas apoptotic inhibitory molecule 2; SH2B1: SH2B adaptor protein 1; ATP2A1: ATPase, Ca++ transporting, cardiac muscle, fast twitch 1; FTO: fat mass and obesity associated; MAF: v-maf musculoaponeurotic fibrosarcoma oncogene homolog (avian); NPC1: Niemann-Pick disease, type C1; MC4R: melanocortin 4 receptor; CHST8: carbohydrate (N-acetylgalactosamine 4-0) sulfotransferase 8; KCTD15: potassium channel tetramerisation domain containing 15
Genetic variants with a polygenic effect on body weight in humans [ 50 ]
A large number of candidate gene association studies, of variable power, have been searched in obesity and related phenotypes. By far the most strongly replicated candidate gene from these analyses is melanocortin 4 receptor, but other replicated associations include those with adipokine and adipokine receptor genes. Further confirming the central role of behavioral stimuli in obesity, alleles of genes encoding dopamine, serotonin, and cannabinoid receptors (DRD2, HTR2C, and CBI) [ 74 – 76 ] are also reported to be associated with feeding behavior and related traits.
Genome-wide association studies have led to the identification of new candidate genes in obesity, most notably the “fat mass and obesity associated” gene (FTO). Rodent studies indicate that FTO mRNA is highly expressed in brain areas important for regulation of energy- and reward driven consumption. Food deprivation alters FTO expression in the hypothalamus in rats and mice. The contribution of the FTO variant is fairly modest, with adult homozygotes for the risk allele having only a 2- to 3-kg increase in weight [ 77 ], but the obesity high-risk allele is common in Caucasian populations and its effects begin early in life. Higher fat mass is observable from the age of 2 weeks, and carriage of the allele is associated with higher BMI and reduced satiety in children [ 11 , 50 ].
Other loci detected in genome wide association studies were identified in large study groups and via meta-analyses. Genome wide association studies (GWAS) reported novel obesity genes with small effects on human body weight. A total of more than 150,000 individuals was analyzed. Hinney et al. made a comprehensive review of these new loci in their paper published in 2010 in European Child Adolescent Psychiatry, see Table 3 -adapted from Hinney et al [ 50 ].
Alternatively, the missing heritability may be accounted for by other genetic factors like genomic copy number variation and epigenetic modifications.
5. Epigenetics
Some people have a different response of to environmental conditions and this may be the result of genetic variation alone, but there is increasing recognition that genetic expression related to disease risk may be modified by the environment during development. The “epigenetic changes” include methylation and alterations to histone proteins that alter the likelihood that specific genes are transcribed. Epigenetic changes usually occur during prenatal development or the early postnatal period. Maternal nutrition is a major factor leading to epigenetic changes. Thus, the levels of vitamins consumed in pregnancy, such as folate, methionine, and vitamin B12, which affect methylation become very important [ 78 ]. Undernutrition during prenatal development has been suggested to lead to postnatal consumption of a fatty diet. On the other hand, overnutrition of the mother is just as influential. The most convincingly shown factor is glycemic status during pregnancy. Hyperglycemia clearly affects infants’ birth weight but, beyond its effects on body weight, may increase the risk for subsequent development of insulin resistance and obesity. Nutritional signals reaching the developing hypothalamus during pregnancy may influence the sensitivity of these neurons to respond to similar signals postnatally. Infant nutrition in the neonatal period may also potentially affect future risk for obesity and its complications [ 79 ].
6. Assessment of the obese child
In order to establish the diagnosis of overweight or obese in a child, the clinician must evaluate the BMI and compare it to the standardized reference chart, appropriate for the age and sex of the child. According to World Health Organization the definition of overweight and obesity in children is established at the following cut-offs [ 80 ]:
Overweight: >+1Standard Deviations (equivalent to BMI 25 kg/m 2 at 19 years)
Obesity: >+2 Standard Deviations (equivalent to BMI 30 kg/m 2 at 19 years).
The cumulative prevalence of monogenic obesity among children with severe obesity is about 5%. There are a lot of genes implicated in obesity and too many obese patients in the world to perform molecular study for everyone. Most genetic and hormonal causes of obesity are rare. The decision to test for these abnormalities should depend upon the presence of particular phenotypes and clinical features suggesting the possibility of a diagnosable disorder [ table 4) . The presence of severe obesity in a young child (<5 yr old) associated to extreme hyperphagia, severe insulin resistance disproportionate for the degree of obesity and a positive family history of early-onset obesity may support a genetic analysis.
Assessment of the obese child.
7. Conclusions
Obesity is caused by complex interactions between environment, behavior and genetic predisposition. The increased heath risk that obesity brings is well established by now. There is growing evidence that genetic predisposition presents a cornerstone role in the development of obesity. Nevertheless, despite the enormous success of genetic studies, there are still important gaps in knowledge. Obesity-specific gene expression pattern may help in understanding the pathogenic mechanisms of obesity and its associated metabolic diseases. Recent advances in identifying genetic risk factors for obesity have contributed to understanding disease pathology, which, in term, may lead to development of new therapeutic strategies, including personalized medicine. In the everyday practice of a clinician, when facing a patient with obesity, it is important to identify particular phenotypes and clinical features that can help to recognize the children who need genetic screening.
© 2013 The Author(s). Licensee IntechOpen. This chapter is distributed under the terms of the Creative Commons Attribution 3.0 License , which permits unrestricted use, distribution, and reproduction in any medium, provided the original work is properly cited.
Continue reading from the same book
Published: 09 January 2013
By Malgorzata Mrugacz
3109 downloads
By Wen Xing, Mingjiang Xu and Feng-Chun Yang
2249 downloads
By Douglas B. Luckie and Mauri E. Krouse
2741 downloads
Thank you for visiting nature.com. You are using a browser version with limited support for CSS. To obtain the best experience, we recommend you use a more up to date browser (or turn off compatibility mode in Internet Explorer). In the meantime, to ensure continued support, we are displaying the site without styles and JavaScript.
- View all journals
- Explore content
- About the journal
- Publish with us
- Sign up for alerts
- Review Article
- Published: 27 February 2019
Obesity: global epidemiology and pathogenesis
- Matthias Blüher 1
Nature Reviews Endocrinology volume 15 , pages 288–298 ( 2019 ) Cite this article
54k Accesses
2422 Citations
989 Altmetric
Metrics details
- Epidemiology
- Health policy
- Pathogenesis
The prevalence of obesity has increased worldwide in the past ~50 years, reaching pandemic levels. Obesity represents a major health challenge because it substantially increases the risk of diseases such as type 2 diabetes mellitus, fatty liver disease, hypertension, myocardial infarction, stroke, dementia, osteoarthritis, obstructive sleep apnoea and several cancers, thereby contributing to a decline in both quality of life and life expectancy. Obesity is also associated with unemployment, social disadvantages and reduced socio-economic productivity, thus increasingly creating an economic burden. Thus far, obesity prevention and treatment strategies — both at the individual and population level — have not been successful in the long term. Lifestyle and behavioural interventions aimed at reducing calorie intake and increasing energy expenditure have limited effectiveness because complex and persistent hormonal, metabolic and neurochemical adaptations defend against weight loss and promote weight regain. Reducing the obesity burden requires approaches that combine individual interventions with changes in the environment and society. Therefore, a better understanding of the remarkable regional differences in obesity prevalence and trends might help to identify societal causes of obesity and provide guidance on which are the most promising intervention strategies.
Obesity prevalence has increased in pandemic dimensions over the past 50 years.
Obesity is a disease that can cause premature disability and death by increasing the risk of cardiometabolic diseases, osteoarthritis, dementia, depression and some types of cancers.
Obesity prevention and treatments frequently fail in the long term (for example, behavioural interventions aiming at reducing energy intake and increasing energy expenditure) or are not available or suitable (bariatric surgery) for the majority of people affected.
Although obesity prevalence increased in every single country in the world, regional differences exist in both obesity prevalence and trends; understanding the drivers of these regional differences might help to provide guidance for the most promising intervention strategies.
Changes in the global food system together with increased sedentary behaviour seem to be the main drivers of the obesity pandemic.
The major challenge is to translate our knowledge of the main causes of increased obesity prevalence into effective actions; such actions might include policy changes that facilitate individual choices for foods that have reduced fat, sugar and salt content.
This is a preview of subscription content, access via your institution
Access options
Access Nature and 54 other Nature Portfolio journals
Get Nature+, our best-value online-access subscription
24,99 € / 30 days
cancel any time
Subscribe to this journal
Receive 12 print issues and online access
195,33 € per year
only 16,28 € per issue
Buy this article
Purchase on Springer Link
Instant access to full article PDF
Prices may be subject to local taxes which are calculated during checkout
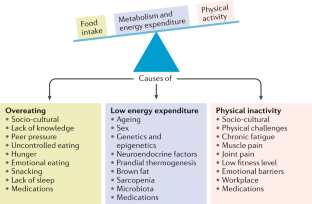
Similar content being viewed by others
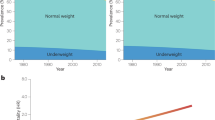
Obesity and the risk of cardiometabolic diseases
Pedro L. Valenzuela, Pedro Carrera-Bastos, … Alejandro Lucia
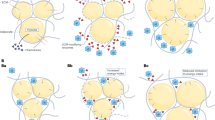
Obesity-induced and weight-loss-induced physiological factors affecting weight regain
Marleen A. van Baak & Edwin C. M. Mariman
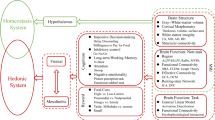
Brain functional and structural magnetic resonance imaging of obesity and weight loss interventions
Guanya Li, Yang Hu, … Gene-Jack Wang
World Health Organization. Noncommunicable diseases progress monitor, 2017. WHO https://www.who.int/nmh/publications/ncd-progress-monitor-2017/en/ (2017).
Fontaine, K. R., Redden, D. T., Wang, C., Westfall, A. O. & Allison, D. B. Years of life lost due to obesity. JAMA 289 , 187–193 (2003).
PubMed Google Scholar
Berrington de Gonzalez, A. et al. Body-mass index and mortality among 1.46 million white adults. N. Engl. J. Med. 363 , 2211–2219 (2010).
CAS PubMed Google Scholar
Prospective Studies Collaboration. Body-mass index and cause-specific mortality in 900000 adults: collaborative analyses of 57 prospective studies. Lancet 373 , 1083–1096 (2009).
PubMed Central Google Scholar
Woolf, A. D. & Pfleger, B. Burden of major musculoskeletal conditions. Bull. World Health Organ. 81 , 646–656 (2003).
PubMed PubMed Central Google Scholar
Bray, G. A. et al. Obesity: a chronic relapsing progressive disease process. A position statement of the World Obesity Federation. Obes. Rev. 18 , 715–723 (2017).
World Health Organization. Obesity and overweight. WHO https://www.who.int/mediacentre/factsheets/fs311/en/ (2016).
World Health Organization. Political declaration of the high-level meeting of the general assembly on the prevention and control of non-communicable diseases. WHO https://www.who.int/nmh/events/un_ncd_summit2011/political_declaration_en.pdf (2012).
Franco, M. et al. Population-wide weight loss and regain in relation to diabetes burden and cardiovascular mortality in Cuba 1980-2010: repeated cross sectional surveys and ecological comparison of secular trends. BMJ 346 , f1515 (2013).
Swinburn, B. A. et al. The global obesity pandemic: shaped by global drivers and local environments. Lancet 378 , 804–814 (2011).
Yanovski, J. A. Obesity: Trends in underweight and obesity — scale of the problem. Nat. Rev. Endocrinol. 14 , 5–6 (2018).
Heymsfield, S. B. & Wadden, T. A. Mechanisms, pathophysiology, and management of obesity. N. Engl. J. Med. 376 , 254–266 (2017).
Murray, S., Tulloch, A., Gold, M. S. & Avena, N. M. Hormonal and neural mechanisms of food reward, eating behaviour and obesity. Nat. Rev. Endocrinol. 10 , 540–552 (2014).
Farooqi, I. S. Defining the neural basis of appetite and obesity: from genes to behaviour. Clin. Med. 14 , 286–289 (2014).
Google Scholar
Anand, B. K. & Brobeck, J. R. Hypothalamic control of food intake in rats and cats. Yale J. Biol. Med. 24 , 123–140 (1951).
CAS PubMed PubMed Central Google Scholar
Zhang, Y. et al. Positional cloning of the mouse obese gene and its human homologue. Nature 372 , 425–432 (1994).
Coleman, D. L. & Hummel, K. P. Effects of parabiosis of normal with genetically diabetic mice. Am. J. Physiol. 217 , 1298–1304 (1969).
Farooqi, I. S. & O’Rahilly, S. 20 years of leptin: human disorders of leptin action. J. Endocrinol. 223 , T63–T70 (2014).
Börjeson, M. The aetiology of obesity in children. A study of 101 twin pairs. Acta Paediatr. Scand. 65 , 279–287 (1976).
Stunkard, A. J., Harris, J. R., Pedersen, N. L. & McClearn, G. E. The body-mass index of twins who have been reared apart. N. Engl. J. Med. 322 , 1483–1487 (1990).
Montague, C. T. et al. Congenital leptin deficiency is associated with severe early-onset obesity in humans. Nature 387 , 903–908 (1997).
Farooqi, I. S. et al. Effects of recombinant leptin therapy in a child with congenital leptin deficiency. N. Engl. J. Med. 341 , 879–884 (1999).
Clément, K. et al. A mutation in the human leptin receptor gene causes obesity and pituitary dysfunction. Nature 392 , 398–401 (1998).
Farooqi, I. S. et al. Dominant and recessive inheritance of morbid obesity associated with melanocortin 4 receptor deficiency. J. Clin. Invest. 106 , 271–279 (2000).
Krude, H. et al. Severe early-onset obesity, adrenal insufficiency and red hair pigmentation caused by POMC mutations in humans. Nat. Genet. 19 , 155–157 (1998).
Hebebrand, J., Volckmar, A. L., Knoll, N. & Hinney, A. Chipping away the ‘missing heritability’: GIANT steps forward in the molecular elucidation of obesity - but still lots to go. Obes. Facts 3 , 294–303 (2010).
Speliotes, E. K. et al. Association analyses of 249,796 individuals reveal 18 new loci associated with body mass index. Nat. Genet. 42 , 937–948 (2010).
Sharma, A. M. & Padwal, R. Obesity is a sign - over-eating is a symptom: an aetiological framework for the assessment and management of obesity. Obes. Rev. 11 , 362–370 (2010).
Berthoud, H. R., Münzberg, H. & Morrison, C. D. Blaming the brain for obesity: integration of hedonic and homeostatic mechanisms. Gastroenterology 152 , 1728–1738 (2017).
Government Office for Science. Foresight. Tackling obesities: future choices – project report. GOV.UK https://assets.publishing.service.gov.uk/government/uploads/system/uploads/attachment_data/file/287937/07-1184x-tackling-obesities-future-choices-report.pdf (2007).
World Health Organization. International Statistical Classification of Diseases and Related Health Problems 10th revision. WHO http://apps.who.int/classifications/icd10/browse/2010/en (2010).
Hebebrand, J. et al. A proposal of the European Association for the Study of Obesity to improve the ICD-11 diagnostic criteria for obesity based on the three dimensions. Obes. Facts 10 , 284–307 (2017).
Ramos Salas, X. et al. Addressing weight bias and discrimination: moving beyond raising awareness to creating change. Obes. Rev. 18 , 1323–1335 (2017).
Sharma, A. M. et al. Conceptualizing obesity as a chronic disease: an interview with Dr. Arya Sharma. Adapt. Phys. Activ Q. 35 , 285–292 (2018).
Hebebrand, J. et al. “Eating addiction”, rather than “food addiction”, better captures addictive-like eating behavior. Neurosci. Biobehav. Rev. 47 , 295–306 (2014).
Phelan, S. M. et al. Impact of weight bias and stigma on quality of care and outcomes for patients with obesity. Obes. Rev. 16 , 319–326 (2015).
Kushner, R. F. et al. Obesity coverage on medical licensing examinations in the United States. What is being tested? Teach Learn. Med. 29 , 123–128 (2017).
NCD Risk Factor Collaboration (NCD-RisC). Worldwide trends in body-mass index, underweight, overweight, and obesity from 1975 to 2016: a pooled analysis of 2416 population-based measurement studies in 128.9 million children, adolescents, and adults. Lancet 390 , 2627–2642 (2017).
NCD Risk Factor Collaboration (NCD-RisC). Trends in adult body-mass index in 200 countries from 1975 to 2014: a pooled analysis of 1698 population-based measurement studies with 19.2 million participants. Lancet 387 , 1377–1396 (2016).
Organisation for Economic Co-operation and Development. Obesity update 2017. OECD https://www.oecd.org/els/health-systems/Obesity-Update-2017.pdf (2017).
Geserick, M. et al. BMI acceleration in early childhood and risk of sustained obesity. N. Engl. J. Med. 379 , 1303–1312 (2018).
Ezzati, M. & Riboli, E. Behavioral and dietary risk factors for noncommunicable diseases. N. Engl. J. Med. 369 , 954–964 (2013).
Kleinert, S. & Horton, R. Rethinking and reframing obesity. Lancet 385 , 2326–2328 (2015).
Roberto, C. A. et al. Patchy progress on obesity prevention: emerging examples, entrenched barriers, and new thinking. Lancet 385 , 2400–2409 (2015).
Lundborg, P., Nystedt, P. & Lindgren, B. Getting ready for the marriage market? The association between divorce risks and investments in attractive body mass among married Europeans. J. Biosoc. Sci. 39 , 531–544 (2007).
McCabe, M. P. et al. Socio-cultural agents and their impact on body image and body change strategies among adolescents in Fiji, Tonga, Tongans in New Zealand and Australia. Obes. Rev. 12 , 61–67 (2011).
Hayashi, F., Takimoto, H., Yoshita, K. & Yoshiike, N. Perceived body size and desire for thinness of young Japanese women: a population-based survey. Br. J. Nutr. 96 , 1154–1162 (2006).
Hardin, J., McLennan, A. K. & Brewis, A. Body size, body norms and some unintended consequences of obesity intervention in the Pacific islands. Ann. Hum. Biol. 45 , 285–294 (2018).
Monteiro, C. A., Conde, W. L. & Popkin, B. M. Income-specific trends in obesity in Brazil: 1975–2003. Am. J. Public Health 97 , 1808–1812 (2007).
Mariapun, J., Ng, C. W. & Hairi, N. N. The gradual shift of overweight, obesity, and abdominal obesity towards the poor in a multi-ethnic developing country: findings from the Malaysian National Health and Morbidity Surveys. J. Epidemiol. 28 , 279–286 (2018).
Gebrie, A., Alebel, A., Zegeye, A., Tesfaye, B. & Ferede, A. Prevalence and associated factors of overweight/ obesity among children and adolescents in Ethiopia: a systematic review and meta-analysis. BMC Obes. 5 , 19 (2018).
Rokholm, B., Baker, J. L. & Sørensen, T. I. The levelling off of the obesity epidemic since the year 1999 — a review of evidence and perspectives. Obes. Rev. 11 , 835–846 (2010).
Hauner, H. et al. Overweight, obesity and high waist circumference: regional differences in prevalence in primary medical care. Dtsch. Arztebl. Int. 105 , 827–833 (2008).
Myers, C. A. et al. Regional disparities in obesity prevalence in the United States: a spatial regime analysis. Obesity 23 , 481–487 (2015).
Wilkinson, R. G. & Pickett, K. The Spirit Level: Why More Equal Societies Almost Always Do Better 89–102 (Bloomsbury Press London, 2009).
Sarget, M. Why inequality is fatal. Nature 458 , 1109–1110 (2009).
Plachta-Danielzik, S. et al. Determinants of the prevalence and incidence of overweight in children and adolescents. Public Health Nutr. 13 , 1870–1881 (2010).
Bell, A. C., Ge, K. & Popkin, B. M. The road to obesity or the path to prevention: motorized transportation and obesity in China. Obes. Res. 10 , 277–283 (2002).
Ludwig, J. et al. Neighborhoods, obesity, and diabetes — a randomized social experiment. N. Engl. J. Med. 365 , 1509–1519 (2011).
Beyerlein, A., Kusian, D., Ziegler, A. G., Schaffrath-Rosario, A. & von Kries, R. Classification tree analyses reveal limited potential for early targeted prevention against childhood overweight. Obesity 22 , 512–517 (2014).
Reilly, J. J. et al. Early life risk factors for obesity in childhood: cohort study. BMJ 330 , 1357 (2005).
Kopelman, P. G. Obesity as a medical problem. Nature 404 , 635–643 (2000).
CAS Google Scholar
Bouchard, C. et al. The response to long-term overfeeding in identical twins. N. Engl. J. Med. 322 , 1477–1482 (1990).
Sadeghirad, B., Duhaney, T., Motaghipisheh, S., Campbell, N. R. & Johnston, B. C. Influence of unhealthy food and beverage marketing on children’s dietary intake and preference: a systematic review and meta-analysis of randomized trials. Obes. Rev. 17 , 945–959 (2016).
Gilbert-Diamond, D. et al. Television food advertisement exposure and FTO rs9939609 genotype in relation to excess consumption in children. Int. J. Obes. 41 , 23–29 (2017).
Frayling, T. M. et al. A common variant in the FTO gene is associated with body mass index and predisposes to childhood and adult obesity. Science 316 , 889–894 (2007).
Loos, R. J. F. & Yeo, G. S. H. The bigger picture of FTO-the first GWAS-identified obesity gene. Nat. Rev. Endocrinol. 10 , 51–61 (2014).
Wardle, J. et al. Obesity associated genetic variation in FTO is associated with diminished satiety. J. Clin. Endocrinol. Metab. 93 , 3640–3643 (2008).
Tanofsky-Kraff, M. et al. The FTO gene rs9939609 obesity-risk allele and loss of control over eating. Am. J. Clin. Nutr. 90 , 1483–1488 (2009).
Hess, M. E. et al. The fat mass and obesity associated gene (Fto) regulates activity of the dopaminergic midbrain circuitry. Nat. Neurosci. 16 , 1042–1048 (2013).
Fredriksson, R. et al. The obesity gene, FTO, is of ancient origin, up-regulated during food deprivation and expressed in neurons of feeding-related nuclei of the brain. Endocrinology 149 , 2062–2071 (2008).
Cohen, D. A. Neurophysiological pathways to obesity: below awareness and beyond individual control. Diabetes 57 , 1768–1773 (2008).
Richard, D. Cognitive and autonomic determinants of energy homeostasis in obesity. Nat. Rev. Endocrinol. 11 , 489–501 (2015).
Clemmensen, C. et al. Gut-brain cross-talk in metabolic control. Cell 168 , 758–774 (2017).
Timper, K. & Brüning, J. C. Hypothalamic circuits regulating appetite and energy homeostasis: pathways to obesity. Dis. Model. Mech. 10 , 679–689 (2017).
Kim, K. S., Seeley, R. J. & Sandoval, D. A. Signalling from the periphery to the brain that regulates energy homeostasis. Nat. Rev. Neurosci. 19 , 185–196 (2018).
Cutler, D. M., Glaeser, E. L. & Shapiro, J. M. Why have Americans become more obese? J. Econ. Perspect. 17 , 93–118 (2003).
Löffler, A. et al. Effects of psychological eating behaviour domains on the association between socio-economic status and BMI. Public Health Nutr. 20 , 2706–2712 (2017).
Chan, R. S. & Woo, J. Prevention of overweight and obesity: how effective is the current public health approach. Int. J. Environ. Res. Public Health 7 , 765–783 (2010).
Hsueh, W. C. et al. Analysis of type 2 diabetes and obesity genetic variants in Mexican Pima Indians: marked allelic differentiation among Amerindians at HLA. Ann. Hum. Genet. 82 , 287–299 (2018).
Schulz, L. O. et al. Effects of traditional and western environments on prevalence of type 2 diabetes in Pima Indians in Mexico and the US. Diabetes Care 29 , 1866–1871 (2006).
Rotimi, C. N. et al. Distribution of anthropometric variables and the prevalence of obesity in populations of west African origin: the International Collaborative Study on Hypertension in Blacks (ICSHIB). Obes. Res. 3 , 95–105 (1995).
Durazo-Arvizu, R. A. et al. Rapid increases in obesity in Jamaica, compared to Nigeria and the United States. BMC Public Health 8 , 133 (2008).
Hu, F. B., Li, T. Y., Colditz, G. A., Willett, W. C. & Manson, J. E. Television watching and other sedentary behaviors in relation to risk of obesity and type 2 diabetes mellitus in women. JAMA 289 , 1785–1791 (2003).
Rissanen, A. M., Heliövaara, M., Knekt, P., Reunanen, A. & Aromaa, A. Determinants of weight gain and overweight in adult Finns. Eur. J. Clin. Nutr. 45 , 419–430 (1991).
Zimmet, P. Z., Arblaster, M. & Thoma, K. The effect of westernization on native populations. Studies on a Micronesian community with a high diabetes prevalence. Aust. NZ J. Med. 8 , 141–146 (1978).
Ulijaszek, S. J. Increasing body size among adult Cook Islanders between 1966 and 1996. Ann. Hum. Biol. 28 , 363–373 (2001).
Snowdon, W. & Thow, A. M. Trade policy and obesity prevention: challenges and innovation in the Pacific Islands. Obes. Rev. 14 , 150–158 (2013).
McLennan, A. K. & Ulijaszek, S. J. Obesity emergence in the Pacific islands: why understanding colonial history and social change is important. Public Health Nutr. 18 , 1499–1505 (2015).
Becker, A. E., Gilman, S. E. & Burwell, R. A. Changes in prevalence of overweight and in body image among Fijian women between 1989 and 1998. Obes. Res. 13 , 110–117 (2005).
Swinburn, B., Sacks, G. & Ravussin, E. Increased food energy supply is more than sufficient to explain the US epidemic of obesity. Am. J. Clin. Nutr. 90 , 1453–1456 (2009).
Swinburn, B. A. et al. Estimating the changes in energy flux that characterize the rise in obesity prevalence. Am. J. Clin. Nutr. 89 , 1723–1728 (2009).
US Department of Agriculture. Food availability (per capita) data system. USDA https://www.ers.usda.gov/data-products/food-availability-per-capita-data-system/ (updated 29 Oct 2018).
Carden, T. J. & Carr, T. P. Food availability of glucose and fat, but not fructose, increased in the U.S. between 1970 and 2009: analysis of the USDA food availability data system. Nutr. J. 12 , 130 (2013).
Hall, K. D., Guo, J., Dore, M. & Chow, C. C. The progressive increase of food waste in America and its environmental impact. PLOS ONE 4 , e7940 (2009).
Scarborough, P. et al. Increased energy intake entirely accounts for increase in body weight in women but not in men in the UK between 1986 and 2000. Br. J. Nutr. 105 , 1399–1404 (2011).
McGinnis, J. M. & Nestle, M. The Surgeon General’s report on nutrition and health: policy implications and implementation strategies. Am. J. Clin. Nutr. 49 , 23–28 (1989).
Krebs-Smith, S. M., Reedy, J. & Bosire, C. Healthfulness of the U.S. food supply: little improvement despite decades of dietary guidance. Am. J. Prev. Med. 38 , 472–477 (2010).
Malik, V. S., Popkin, B. M., Bray, G. A., Després, J. P. & Hu, F. B. Sugar-sweetened beverages, obesity, type 2 diabetes mellitus, and cardiovascular disease risk. Circulation 121 , 1356–1364 (2010).
Schulze, M. B. et al. Sugar-sweetened beverages, weight gain, and incidence of type 2 diabetes in young and middle-aged women. JAMA 292 , 927–934 (2004).
Mozaffarian, D., Hao, T., Rimm, E. B., Willett, W. C. & Hu, F. B. Changes in diet and lifestyle and long-term weight gain in women and men. N. Engl. J. Med. 364 , 2392–2404 (2011).
Malik, V. S. & Hu, F. B. Sugar-sweetened beverages and health: where does the evidence stand? Am. J. Clin. Nutr. 94 , 1161–1162 (2011).
Qi, Q. et al. Sugar-sweetened beverages and genetic risk of obesity. N. Engl. J. Med. 367 , 1387–1396 (2012).
Heiker, J. T. et al. Identification of genetic loci associated with different responses to high-fat diet-induced obesity in C57BL/6N and C57BL/6J substrains. Physiol. Genomics 46 , 377–384 (2014).
Wahlqvist, M. L. et al. Early-life influences on obesity: from preconception to adolescence. Ann. NY Acad. Sci. 1347 , 1–28 (2015).
Rohde, K. et al. Genetics and epigenetics in obesity. Metabolism . https://doi.org/10.1016/j.metabol.2018.10.007 (2018).
Article PubMed Google Scholar
Panzeri, I. & Pospisilik, J. A. Epigenetic control of variation and stochasticity in metabolic disease. Mol. Metab. 14 , 26–38 (2018).
Ruiz-Hernandez, A. et al. Environmental chemicals and DNA methylation in adults: a systematic review of the epidemiologic evidence. Clin. Epigenet. 7 , 55 (2015).
Quarta, C., Schneider, R. & Tschöp, M. H. Epigenetic ON/OFF switches for obesity. Cell 164 , 341–342 (2016).
Dalgaard, K. et al. Trim28 haploinsufficiency triggers bi-stable epigenetic obesity. Cell 164 , 353–364 (2015).
Michaelides, M. et al. Striatal Rgs4 regulates feeding and susceptibility to diet-induced obesity. Mol. Psychiatry . https://doi.org/10.1038/s41380-018-0120-7 (2018).
Article PubMed PubMed Central Google Scholar
Weihrauch-Blüher, S. et al. Current guidelines for obesity prevention in childhood and adolescence. Obes. Facts 11 , 263–276 (2018).
Nakamura, R. et al. Evaluating the 2014 sugar-sweetened beverage tax in Chile: An observational study in urban areas. PLOS Med. 15 , e1002596 (2018).
Colchero, M. A., Molina, M. & Guerrero-López, C. M. After Mexico implemented a tax, purchases of sugar-sweetened beverages decreased and water increased: difference by place of residence, household composition, and income level. J. Nutr. 147 , 1552–1557 (2017).
Brownell, K. D. & Warner, K. E. The perils of ignoring history: Big Tobacco played dirty and millions died. How similar is Big Food? Milbank Q. 87 , 259–294 (2009).
Mialon, M., Swinburn, B., Allender, S. & Sacks, G. ‘Maximising shareholder value’: a detailed insight into the corporate political activity of the Australian food industry. Aust. NZ J. Public Health 41 , 165–171 (2017).
Peeters, A. Obesity and the future of food policies that promote healthy diets. Nat. Rev. Endocrinol. 14 , 430–437 (2018).
Hawkes, C., Jewell, J. & Allen, K. A food policy package for healthy diets and the prevention of obesity and diet-related non-communicable diseases: the NOURISHING framework. Obes. Rev. 14 (Suppl. 2), 159–168 (2013).
World Health Organisation. Global database on the Implementation of Nutrition Action (GINA). WHO https://www.who.int/nutrition/gina/en/ (2012).
Popkin, B., Monteiro, C. & Swinburn, B. Overview: Bellagio Conference on program and policy options for preventing obesity in the low- and middle-income countries. Obes. Rev. 14 (Suppl. 2), 1–8 (2013).
Download references
Reviewer information
Nature Reviews Endocrinology thanks G. Bray, A. Sharma and H. Toplak for their contribution to the peer review of this work.
Author information
Authors and affiliations.
Department of Medicine, University of Leipzig, Leipzig, Germany
Matthias Blüher
You can also search for this author in PubMed Google Scholar
Corresponding author
Correspondence to Matthias Blüher .
Ethics declarations
Competing interests.
The author declares no competing interests.
Additional information
Publisher’s note.
Springer Nature remains neutral with regard to jurisdictional claims in published maps and institutional affiliations.
Rights and permissions
Reprints and permissions
About this article
Cite this article.
Blüher, M. Obesity: global epidemiology and pathogenesis. Nat Rev Endocrinol 15 , 288–298 (2019). https://doi.org/10.1038/s41574-019-0176-8
Download citation
Published : 27 February 2019
Issue Date : May 2019
DOI : https://doi.org/10.1038/s41574-019-0176-8
Share this article
Anyone you share the following link with will be able to read this content:
Sorry, a shareable link is not currently available for this article.
Provided by the Springer Nature SharedIt content-sharing initiative
This article is cited by
Body composition, lifestyle, and depression: a prospective study in the uk biobank.
BMC Public Health (2024)
Physical activity, gestational weight gain in obese patients with early gestational diabetes and the perinatal outcome – a randomised–controlled trial
- Lukasz Adamczak
- Urszula Mantaj
- Ewa Wender-Ozegowska
BMC Pregnancy and Childbirth (2024)
What could be the reasons for not losing weight even after following a weight loss program?
- Jyoti Dabas
- S. Shunmukha Priya
- Praveen Budhrani
Journal of Health, Population and Nutrition (2024)
Effect of COVID-19 Pandemic Lockdowns on Body Mass Index of Primary School Children from Different Socioeconomic Backgrounds
- Ludwig Piesch
- Robert Stojan
- Till Utesch
Sports Medicine - Open (2024)
Prevalence and risk factors of obesity among undergraduate student population in Ghana: an evaluation study of body composition indices
- Christian Obirikorang
- Evans Asamoah Adu
- Lois Balmer
Quick links
- Explore articles by subject
- Guide to authors
- Editorial policies
Sign up for the Nature Briefing newsletter — what matters in science, free to your inbox daily.

- Alzheimer's disease & dementia
- Arthritis & Rheumatism
- Attention deficit disorders
- Autism spectrum disorders
- Biomedical technology
- Diseases, Conditions, Syndromes
- Endocrinology & Metabolism
- Gastroenterology
- Gerontology & Geriatrics
- Health informatics
- Inflammatory disorders
- Medical economics
- Medical research
- Medications
- Neuroscience
- Obstetrics & gynaecology
- Oncology & Cancer
- Ophthalmology
- Overweight & Obesity
- Parkinson's & Movement disorders
- Psychology & Psychiatry
- Radiology & Imaging
- Sleep disorders
- Sports medicine & Kinesiology
- Vaccination
- Breast cancer
- Cardiovascular disease
- Chronic obstructive pulmonary disease
- Colon cancer
- Coronary artery disease
- Heart attack
- Heart disease
- High blood pressure
- Kidney disease
- Lung cancer
- Multiple sclerosis
- Myocardial infarction
- Ovarian cancer
- Post traumatic stress disorder
- Rheumatoid arthritis
- Schizophrenia
- Skin cancer
- Type 2 diabetes
- Full List »
share this!
March 27, 2024
This article has been reviewed according to Science X's editorial process and policies . Editors have highlighted the following attributes while ensuring the content's credibility:
fact-checked
peer-reviewed publication
trusted source
Higher genetic risk of obesity means working out harder for same results, study shows
by Vanderbilt University Medical Center
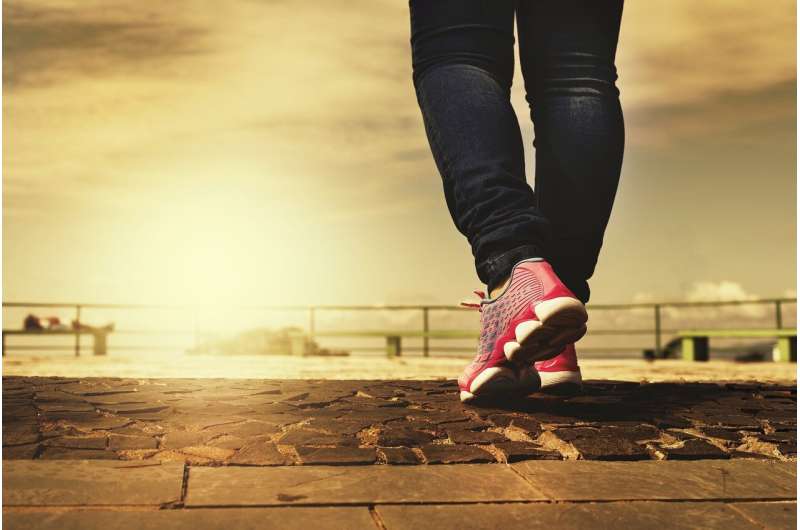
Persons with a higher genetic risk of obesity need to work out harder than those of moderate or low genetic risk to avoid becoming obese, according to a Vanderbilt University Medical Center (VUMC) paper published in JAMA Network Open.
Study authors used activity, clinical and genetic data from the National Institutes of Health's "All of Us" Research Program to explore the association of genetic risk of higher body mass index and the level of physical activity needed to reduce incident obesity .
"Physical activity guidelines do not account for individual differences ," said senior author Douglas Ruderfer, Ph.D., associate professor of Medicine, Division of Genetic Medicine, and director of the Center for Digital Genomic Medicine at VUMC. "Genetic background contributes to the amount of physical activity needed to mitigate obesity. The higher the genetic risk, the more steps needed per day."
"I think an important component to this result is that individuals can be active enough to account for their genetic background, or their genetic risk for obesity, regardless of how high that risk might be," he added. "And there are many other contributors that play a role including diet and environmental factors."
Included in the study were 3,124 middle-aged participants without obesity who owned a Fitbit device and walked an average of 8,326 steps per day for a median of more than five years. The incidence of obesity over the study period increased from 13% to 43% in the lowest and highest polygenic risk score groups.
Individuals with a polygenic risk score in the 75th percentile would need to walk an average of 2,280 more steps per day (a total of 11,020 steps per day) than those in the 50th percentile to have a comparable risk of obesity, according to the study.
Persons with a baseline BMI of 22, 24, 26 and 28 who were in the 75th percentile of polygenic risk score would need to walk an additional 3,460, 4,430, 5,380 and 6,350 steps per day, respectively, to have a comparable risk of obesity to persons in the 25th percentile.
"I think it is intuitive that individuals who have a higher genetic risk of obesity might need to have more physical activity to reduce that risk, but what is new and important from this study is that we were able to put a number on the amount of activity needed to reduce the risk," said lead author Evan Brittain, MD, associate professor of Medicine in the Division of Cardiovascular Medicine at VUMC and lead investigator in Digital Health for the All of Us Research Program Data and Research Center.
"It is becoming more commonplace to know you have a genetic risk for obesity in the genomic era when genetic results are being returned directly to patients. And you can imagine a future in which that data could be integrated with someone's electronic health record and could form the basis of an individual's physical activity recommendation from their doctor."
Study authors said they now want to see if the findings generalize to more representative and diverse populations in order to determine if providing information for individual activity recommendations results in improved health and a lower likelihood of obesity.
"We would like to test whether knowledge of one's genetic risk for obesity actually has an impact on their behavior," Brittain said. "I think these findings could be empowering for patients because the current physical activity guidelines take a one-size-fits-all approach, and what we learned is that depending on your genetic risk, the guidelines may underestimate the amount of activity needed to reduce your risk of obesity.
"Most importantly, I would like for patients to know that your genetic risk doesn't determine your overall risk of obesity, and you can actually overcome that risk by being more active," he added.
Explore further
Feedback to editors

New report presents a global plan to combat prostate cancer
24 minutes ago

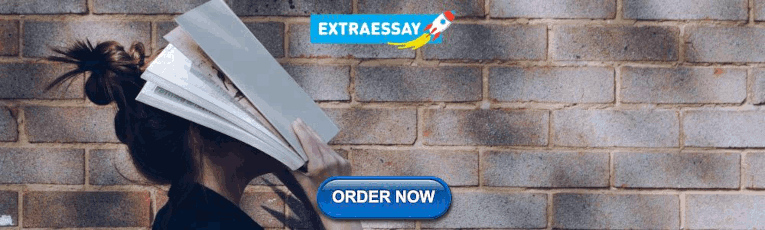
Dogs may provide new insights into human aging and cognition

AI's ability to detect tumor cells could be key to more accurate bone cancer prognoses

Novel pre-clinical models help advance therapeutic development for antibiotic-resistant bacterial infections
2 hours ago

Far-UVC light can virtually eliminate airborne virus in an occupied room, study shows

Las Vegas mass shooting survivors continue to struggle with major depression, PTSD
3 hours ago

Combining food taxes and subsidies can lead to healthier grocery purchases for low-income households

New insights into muscle health: How innervation influences the recovery process

Simulations reveal mechanism behind protein buildup in Parkinson's disease

Increasing positive affect in adolescence could lead to improved health and well-being in adulthood
4 hours ago
Related Stories

People whose genotype supports physical activity found to have lower risk of developing cardiovascular disease
Nov 6, 2023

People with genetic predisposition to obesity may have lower risk of cardiovascular disease
Apr 6, 2023

Genetic information can improve colorectal cancer screening, shows study
Jan 17, 2024

Obesity is a critical risk factor for type 2 diabetes, regardless of genetics
Apr 15, 2020

Investigating the impact of replacing sedentary time with physical activity on genetic risk of coronary heart disease
Oct 23, 2023
Physical inactivity and restless sleep exacerbate genetic risk of obesity
Oct 20, 2017
Recommended for you

New insights into aggressive breast cancer and potential treatment options
6 hours ago

New study maps a group of rare genetic diseases for the first time
7 hours ago

New study seeks hereditary causes of childhood cancer

Gene analysis generates spatial map of intestinal cells and traces their trajectories during gut inflammation

YKT6 gene variants cause a new neurological disorder, finds study
8 hours ago

International study uses AI to show how personality influences the expression of our genes
Apr 1, 2024
Let us know if there is a problem with our content
Use this form if you have come across a typo, inaccuracy or would like to send an edit request for the content on this page. For general inquiries, please use our contact form . For general feedback, use the public comments section below (please adhere to guidelines ).
Please select the most appropriate category to facilitate processing of your request
Thank you for taking time to provide your feedback to the editors.
Your feedback is important to us. However, we do not guarantee individual replies due to the high volume of messages.
E-mail the story
Your email address is used only to let the recipient know who sent the email. Neither your address nor the recipient's address will be used for any other purpose. The information you enter will appear in your e-mail message and is not retained by Medical Xpress in any form.
Newsletter sign up
Get weekly and/or daily updates delivered to your inbox. You can unsubscribe at any time and we'll never share your details to third parties.
More information Privacy policy
Donate and enjoy an ad-free experience
We keep our content available to everyone. Consider supporting Science X's mission by getting a premium account.
E-mail newsletter

- Get Press Releases

- Media Contacts
- News Releases
- Photos & B-Roll Downloads
- VUMC Facts and Figures
Explore by Highlight
- Education & Training
- Growth & Finance
- Leadership Perspectives
- VUMC People
Explore by Topic
- Emergency & Trauma
- Genetics & Genomics
- Health Equity
- Health Policy
- Patient Spotlight
- Tech & Health
Explore by Location
- Monroe Carell Jr. Children’s Hospital at Vanderbilt
- Vanderbilt Bedford County Hospital
- Vanderbilt Health One Hundred Oaks
- Vanderbilt Health Affiliated Network
- Vanderbilt-Ingram Cancer Center
- Vanderbilt Psychiatric Hospital
- Vanderbilt Wilson County Hospital
- Vanderbilt Stallworth Rehabilitation Hospital
- Vanderbilt Tullahoma Harton Hospital
- Vanderbilt University Hospital

- Photos & B-Roll Downloads
Featured Story
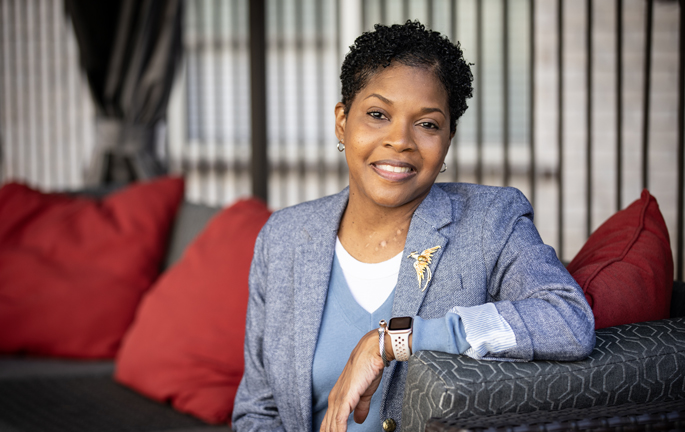
VUMC performs its first combined lung and liver transplant
March 27, 2024, higher genetic risk of obesity means working out harder for same results .
Study authors used activity, clinical and genetic data from the National Institutes of Health’s All of Us Research Program to explore the association of genetic risk of higher body mass index and the level of physical activity needed to reduce incident obesity.
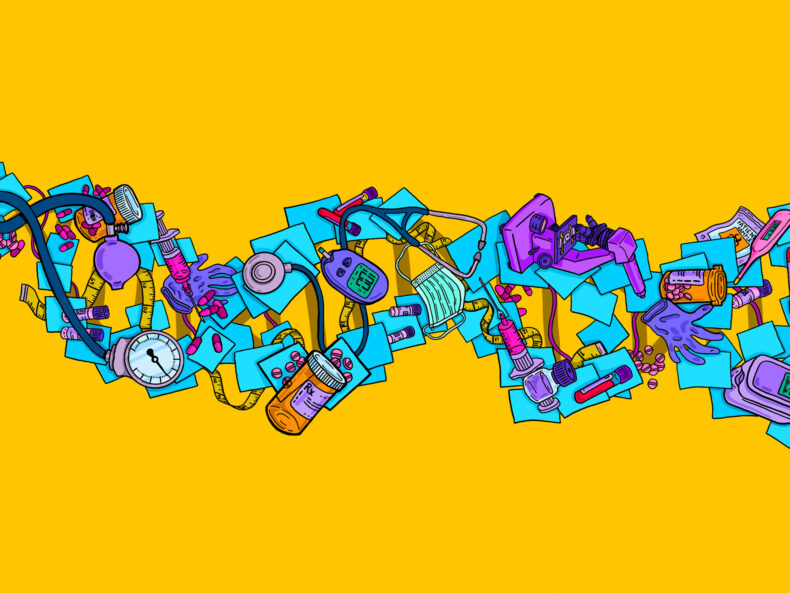
Persons with a higher genetic risk of obesity need to work out harder than those of moderate or low genetic risk to avoid becoming obese, according to a Vanderbilt University Medical Center (VUMC) paper published in JAMA Network Open .
Study authors used activity, clinical and genetic data from the National Institutes of Health’s All of Us Research Program to explore the association of genetic risk of higher body mass index and the level of physical activity needed to reduce incident obesity.
“Physical activity guidelines do not account for individual differences,” said senior author Douglas Ruderfer , PhD, associate professor of Medicine, Division of Genetic Medicine, and director of the Center for Digital Genomic Medicine at VUMC. “Genetic background contributes to the amount of physical activity needed to mitigate obesity. The higher the genetic risk, the more steps needed per day.
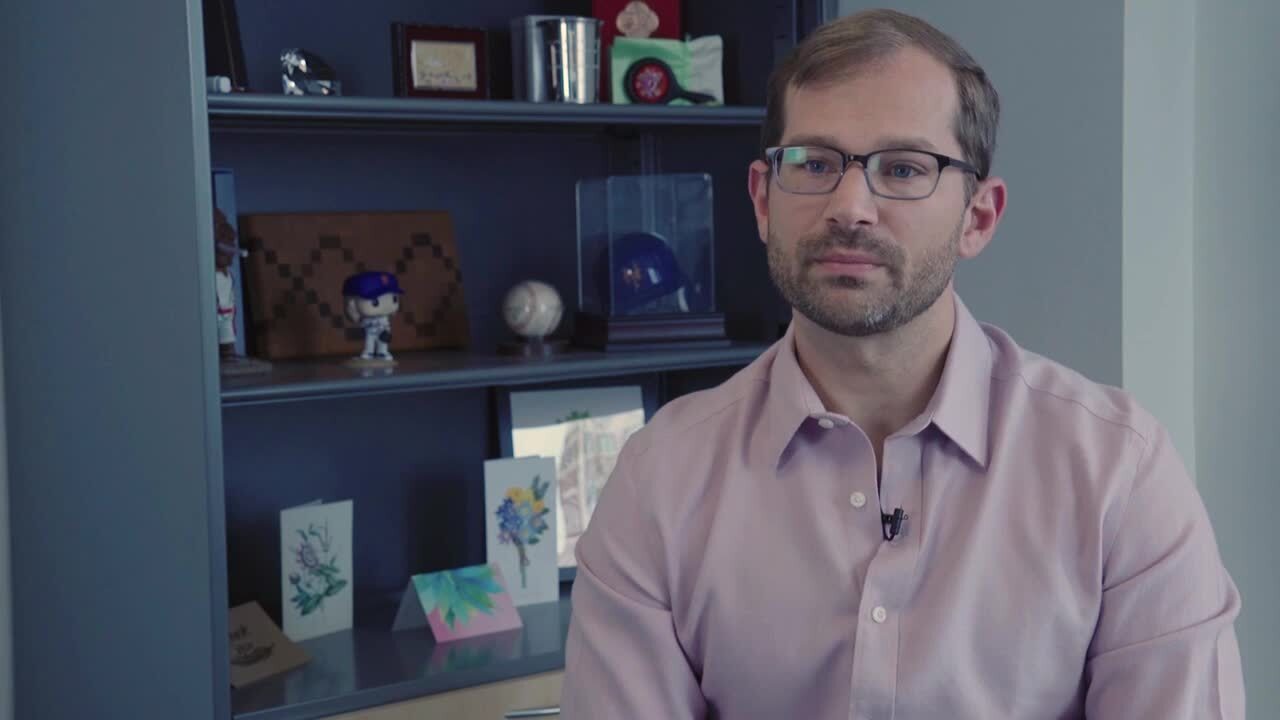
“I think an important component to this result is that individuals can be active enough to account for their genetic background, or their genetic risk for obesity, regardless of how high that risk might be,” he added. “And there are many other contributors that play a role including diet and environmental factors.”
Included in the study were 3,124 middle-aged participants without obesity who owned a Fitbit device and walked an average of 8,326 steps per day for a median of more than 5 years. The incidence of obesity over the study period increased from 13% to 43% in the lowest and highest polygenic risk score groups.
Most importantly, I would like for patients to know that your genetic risk doesn’t determine your overall risk of obesity, and you can actually overcome that risk by being more active Lead author Evan Brittain , MD
Individuals with a polygenic risk score in the 75 th percentile would need to walk an average of 2,280 more steps per day (a total of 11,020 steps per day) than those in the 50 th percentile to have a comparable risk of obesity, according to the study.
Persons with a baseline BMI of 22, 24, 26 and 28 who were in the 75 th percentile of polygenic risk score would need to walk an additional 3,460, 4,430, 5,380 and 6,350 steps per day, respectively, to have a comparable risk of obesity to persons in the 25 th percentile.
“I think it is intuitive that individuals who have a higher genetic risk of obesity might need to have more physical activity to reduce that risk, but what is new and important from this study is that we were able to put a number on the amount of activity needed to reduce the risk,” said lead author Evan Brittain , MD, associate professor of Medicine in the Division of Cardiovascular Medicine at VUMC and lead investigator in Digital Health for the All of Us Research Program Data and Research Center.
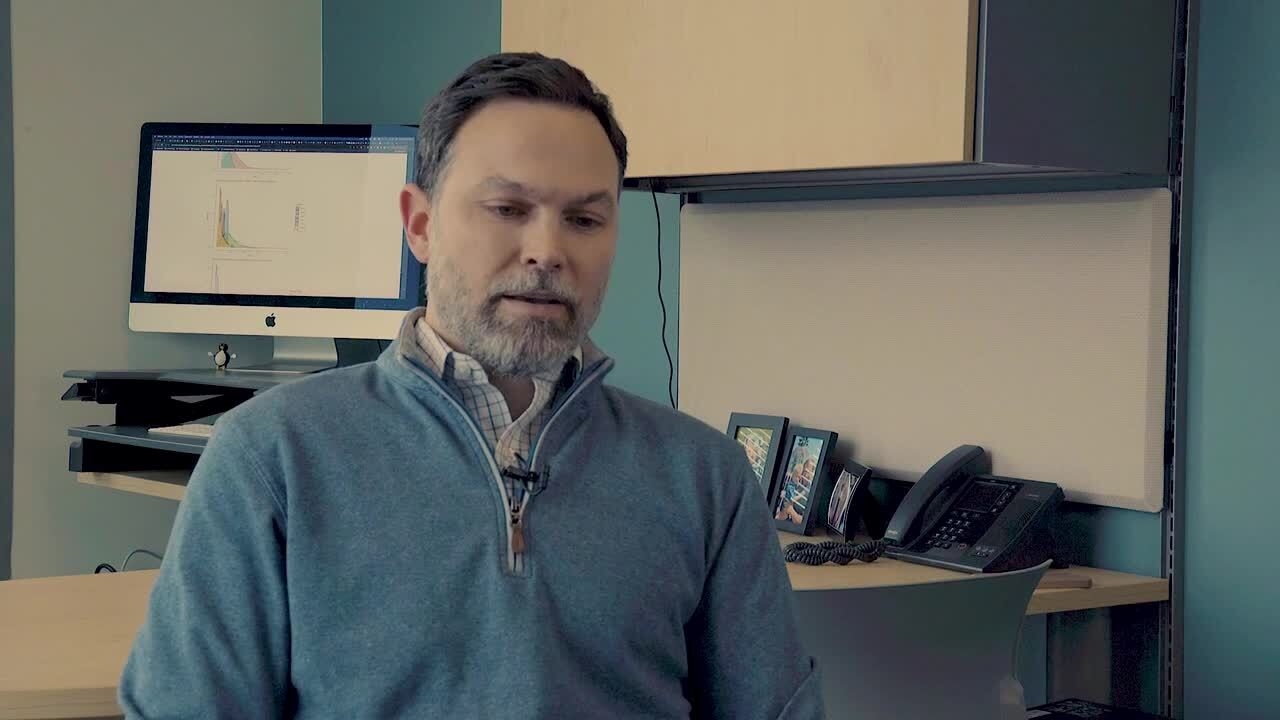
“It is becoming more commonplace to know you have a genetic risk for obesity in the genomic era when genetic results are being returned directly to patients. And you can imagine a future in which that data could be integrated with someone’s electronic health record and could form the basis of an individual’s physical activity recommendation from their doctor.”
Study authors said they now want to see if the findings generalize to more representative and diverse populations in order to determine if providing information for individual activity recommendations results in improved health and a lower likelihood of obesity.
“We would like to test whether knowledge of one’s genetic risk for obesity actually has an impact on their behavior,” Brittain said. “I think these findings could be empowering for patients because the current physical activity guidelines take a one-size-fits-all approach, and what we learned is that depending on your genetic risk, the guidelines may underestimate the amount of activity needed to reduce your risk of obesity.
“Most importantly, I would like for patients to know that your genetic risk doesn’t determine your overall risk of obesity, and you can actually overcome that risk by being more active,” he added.
To access broadcast quality video for download, fill out the submission form.
Related Articles
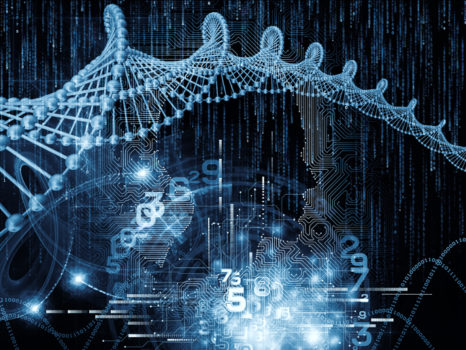
November 19, 2019
Getting the goods on obesity.
Obesity and two post-operative complications linked with it have associated genetic variants in common, suggesting that obesity may be the culprit.
By Paul Govern
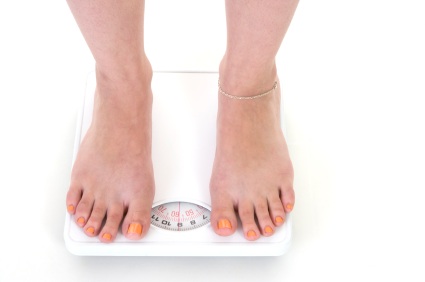
January 20, 2012
Obesity genes linked to uterine cancer.
In addition to body mass index, genetic markers of obesity may provide value in predicting endometrial cancer risk.
By Vumc News And Communications
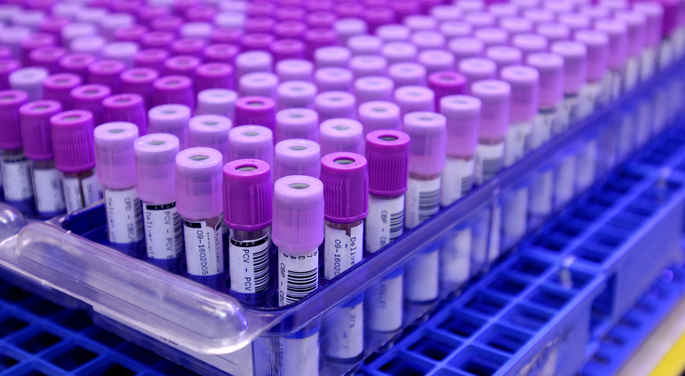
February 1, 2024
A biomarker for early type 2 diabetes.
Genetic analyses suggest that branched chain amino acids may be a sensitive biomarker of early or subclinical Type 2 diabetes and could be used to identify risk and implement preventive measures.
By Bill Snyder
The future of nutrition: Nutrigenomics and nutrigenetics in obesity and cardiovascular diseases
Affiliations.
- 1 a Department of Biochemistry and Molecular Biology-A , University of Murcia , Murcia , Spain.
- 2 b Department of Surgery, Hospital de la Vega Lorenzo Guirao , University of Murcia , Murcia , Spain.
- 3 c Department of Cardiology, Hospital Clínico Universitario Virgen de la Arrixaca (IMIB-Arrixaca) , Universidad de Murcia , Murcia , Spain.
- PMID: 28678615
- DOI: 10.1080/10408398.2017.1349731
Over time, the relationship between diet and health has aroused great interest, since nutrition can prevent and treat several diseases. It has been demonstrated that general recommendations on macronutrients and micronutrients do not affect to every individual in the same way because diet is an important environmental factor that interacts with genes. Thus, there is a growing necessity of improving a personalized nutrition to treat obesity and associated medical conditions, taking into account the interactions between diet, genes and health. Therefore, the knowledge of the interactions between the genome and nutrients at the molecular level, has led to the advent of nutritional genomics, which involves the sciences of nutrigenomics and nutrigenetics. In this review, we will comprehensively analyze the role of the most important genes associated with two interrelated chronic medical conditions, such as obesity and cardiovascular diseases.
Keywords: Gene; cardiovascular diseases; nutrigenetics; nutrigenomics; obesity; polimorphism.
Publication types
- Cardiovascular Diseases / genetics*
- Genetic Predisposition to Disease*
- Nutrigenomics*
- Nutritional Status
- Obesity / genetics*
- See us on facebook
- See us on twitter
- See us on youtube
- See us on linkedin
- See us on instagram
Pilot study shows ketogenic diet improves severe mental illness
A small clinical trial led by Stanford Medicine found that the metabolic effects of a ketogenic diet may help stabilize the brain.
April 1, 2024 - By Nina Bai

A study led by researchers at Stanford Medicine showed that diet can help those with serious mental illness. nishihata
For people living with serious mental illness like schizophrenia or bipolar disorder, standard treatment with antipsychotic medications can be a double-edged sword. While these drugs help regulate brain chemistry, they often cause metabolic side effects such as insulin resistance and obesity, which are distressing enough that many patients stop taking the medications.
Now, a pilot study led by Stanford Medicine researchers has found that a ketogenic diet not only restores metabolic health in these patients as they continue their medications, but it further improves their psychiatric conditions. The results, published March 27 in Psychiatry Research , suggest that a dietary intervention can be a powerful aid in treating mental illness.
“It’s very promising and very encouraging that you can take back control of your illness in some way, aside from the usual standard of care,” said Shebani Sethi , MD, associate professor of psychiatry and behavioral sciences and the first author of the new paper.
Making the connection
Sethi, who is board certified in obesity and psychiatry, remembers when she first noticed the connection. As a medical student working in an obesity clinic, she saw a patient with treatment-resistant schizophrenia whose auditory hallucinations quieted on a ketogenic diet.
That prompted her to dig into the medical literature. There were only a few, decades-old case reports on using the ketogenic diet to treat schizophrenia, but there was a long track record of success in using ketogenic diets to treat epileptic seizures.
“The ketogenic diet has been proven to be effective for treatment-resistant epileptic seizures by reducing the excitability of neurons in the brain,” Sethi said. “We thought it would be worth exploring this treatment in psychiatric conditions.”
A few years later, Sethi coined the term metabolic psychiatry, a new field that approaches mental health from an energy conversion perspective.
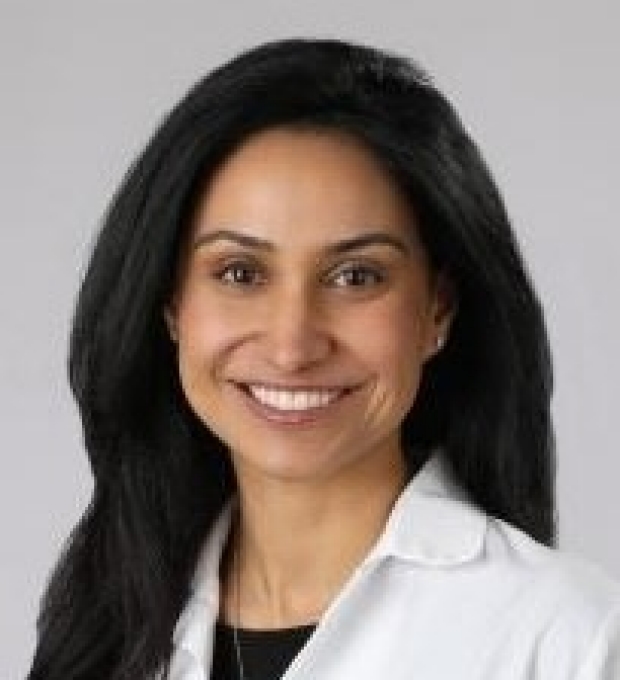
Shebani Sethi
In the four-month pilot trial, Sethi’s team followed 21 adult participants who were diagnosed with schizophrenia or bipolar disorder, taking antipsychotic medications, and had a metabolic abnormality — such as weight gain, insulin resistance, hypertriglyceridemia, dyslipidemia or impaired glucose tolerance. The participants were instructed to follow a ketogenic diet, with approximately 10% of the calories from carbohydrates, 30% from protein and 60% from fat. They were not told to count calories.
“The focus of eating is on whole non-processed foods including protein and non-starchy vegetables, and not restricting fats,” said Sethi, who shared keto-friendly meal ideas with the participants. They were also given keto cookbooks and access to a health coach.
The research team tracked how well the participants followed the diet through weekly measures of blood ketone levels. (Ketones are acids produced when the body breaks down fat — instead of glucose — for energy.) By the end of the trial, 14 patients had been fully adherent, six were semi-adherent and only one was non-adherent.
The participants underwent a variety of psychiatric and metabolic assessments throughout the trial.
Before the trial, 29% of the participants met the criteria for metabolic syndrome, defined as having at least three of five conditions: abdominal obesity, elevated triglycerides, low HDL cholesterol, elevated blood pressure and elevated fasting glucose levels. After four months on a ketogenic diet, none of the participants had metabolic syndrome.
On average, the participants lost 10% of their body weight; reduced their waist circumference by 11% percent; and had lower blood pressure, body mass index, triglycerides, blood sugar levels and insulin resistance.
“We’re seeing huge changes,” Sethi said. “Even if you’re on antipsychotic drugs, we can still reverse the obesity, the metabolic syndrome, the insulin resistance. I think that’s very encouraging for patients.”
The participants reported improvements in their energy, sleep, mood and quality of life.
The psychiatric benefits were also striking. On average, the participants improved 31% on a psychiatrist rating of mental illness known as the clinical global impressions scale, with three-quarters of the group showing clinically meaningful improvement. Overall, the participants also reported better sleep and greater life satisfaction.
“The participants reported improvements in their energy, sleep, mood and quality of life,” Sethi said. “They feel healthier and more hopeful.”
The researchers were impressed that most of the participants stuck with the diet. “We saw more benefit with the adherent group compared with the semi-adherent group, indicating a potential dose-response relationship,” Sethi said.
Alternative fuel for the brain
There is increasing evidence that psychiatric diseases such as schizophrenia and bipolar disorder stem from metabolic deficits in the brain, which affect the excitability of neurons, Sethi said.
The researchers hypothesize that just as a ketogenic diet improves the rest of the body’s metabolism, it also improves the brain’s metabolism.
“Anything that improves metabolic health in general is probably going to improve brain health anyway,” Sethi said. “But the ketogenic diet can provide ketones as an alternative fuel to glucose for a brain with energy dysfunction.”
Likely there are multiple mechanisms at work, she added, and the main purpose of the small pilot trial is to help researchers detect signals that will guide the design of larger, more robust studies.
As a physician, Sethi cares for many patients with both serious mental illness and obesity or metabolic syndrome, but few studies have focused on this undertreated population.
She is the founder and director of the metabolic psychiatry clinic at Stanford Medicine.
“Many of my patients suffer from both illnesses, so my desire was to see if metabolic interventions could help them,” she said. “They are seeking more help. They are looking to just feel better.”
Researchers from the University of Michigan; the University of California, San Francisco; and Duke University contributed to the study.
The study was supported by Baszucki Group Research Fund, Keun Lau Fund and the Obesity Treatment Foundation.
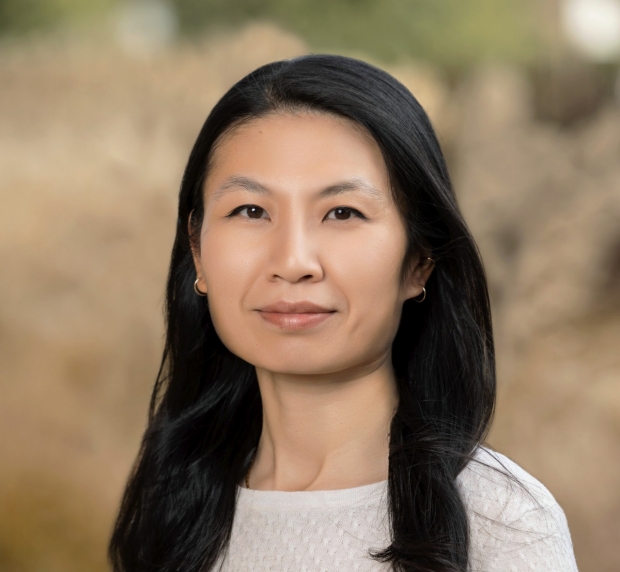
About Stanford Medicine
Stanford Medicine is an integrated academic health system comprising the Stanford School of Medicine and adult and pediatric health care delivery systems. Together, they harness the full potential of biomedicine through collaborative research, education and clinical care for patients. For more information, please visit med.stanford.edu .
Artificial intelligence
Exploring ways AI is applied to health care
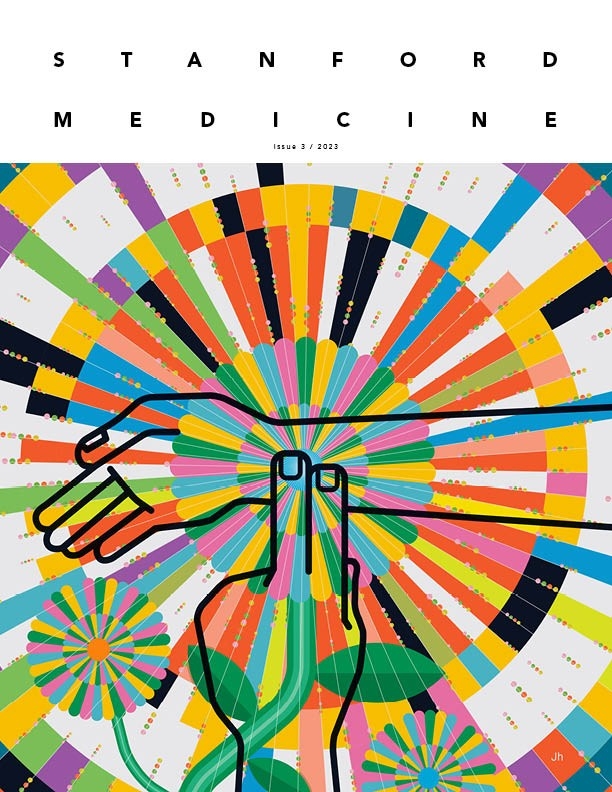
- Share full article
For more audio journalism and storytelling, download New York Times Audio , a new iOS app available for news subscribers.
Ronna McDaniel, TV News and the Trump Problem
The former republican national committee chairwoman was hired by nbc and then let go after an outcry..
This transcript was created using speech recognition software. While it has been reviewed by human transcribers, it may contain errors. Please review the episode audio before quoting from this transcript and email [email protected] with any questions.
From “The New York Times,” I’m Michael Barbaro. This is “The Daily.”
[MUSIC PLAYING]
Today, the saga of Ronna McDaniel and NBC and what it reveals about the state of television news headed into the 2024 presidential race. Jim Rutenberg, a “Times” writer at large, is our guest.
It’s Monday, April 1.
Jim, NBC News just went through a very public, a very searing drama over the past week, that we wanted you to make sense of in your unique capacity as a longtime media and political reporter at “The Times.” This is your sweet spot. You were, I believe, born to dissect this story for us.
Oh, brother.
Well, on the one hand, this is a very small moment for a major network like NBC. They hire, as a contributor, not an anchor, not a correspondent, as a contributor, Ronna McDaniel, the former RNC chairwoman. It blows up in a mini scandal at the network.
But to me, it represents a much larger issue that’s been there since that moment Donald J. Trump took his shiny gold escalator down to announce his presidential run in 2015. This struggle by the news media to figure out, especially on television, how do we capture him, cover him for all of his lies, all the challenges he poses to Democratic norms, yet not alienate some 74, 75 million American voters who still follow him, still believe in him, and still want to hear his reality reflected in the news that they’re listening to?
Right. Which is about as gnarly a conundrum as anyone has ever dealt with in the news media.
Well, it’s proven so far unsolvable.
Well, let’s use the story of what actually happened with Ronna McDaniel and NBC to illustrate your point. And I think that means describing precisely what happened in this situation.
The story starts out so simply. It’s such a basic thing that television networks do. As elections get underway, they want people who will reflect the two parties.
They want talking heads. They want insiders. They want them on their payroll so they can rely on them whenever they need them. And they want them to be high level so they can speak with great knowledge about the two major candidates.
Right. And rather than needing to beg these people to come on their show at 6 o’clock, when they might be busy and it’s not their full-time job, they go off and they basically put them on retainer for a bunch of money.
Yeah. And in this case, here’s this perfect scenario because quite recently, Ronna McDaniel, the chairwoman of the Republican National Committee through the Trump era, most of it, is now out on the market. She’s actually recently been forced out of the party. And all the networks are interested because here’s the consummate insider from Trump world ready to get snatched up under contract for the next election and can really represent this movement that they’ve been trying to capture.
So NBC’S key news executives move pretty aggressively, pretty swiftly, and they sign her up for a $300,000 a year contributor’s contract.
Nice money if you can get it.
Not at millions of dollars that they pay their anchors, but a very nice contract. I’ll take it. You’ll take it. In the eyes of NBC execs she was perfect because she can be on “Meet the Press” as a panelist. She can help as they figure out some of their coverage. They have 24 hours a day to fill and here’s an official from the RNC. You can almost imagine the question that would be asked to her. It’s 10:00 PM on election night. Ronna, what are the Trump people thinking right now? They’re looking at the same numbers you are.
That was good, but that’s exactly it. And we all know it, right? This is television in our current era.
So last Friday, NBC makes what should be a routine announcement, but one they’re very proud of, that they’ve hired Ronna McDaniel. And in a statement, they say it couldn’t be a more important moment to have a voice like Ronna’s on the team. So all’s good, right? Except for there’s a fly in the ointment.
Because it turns out that Ronna McDaniel has been slated to appear on “Meet the Press,” not as a paid NBC contributor, but as a former recently ousted RNC chair with the “Meet The Press” host, Kristen Welker, who’s preparing to have a real tough interview with Ronna McDaniel. Because of course, Ronna McDaniel was chair of the party and at Trump’s side as he tried to refuse his election loss. So this was supposed to be a showdown interview.
From NBC News in Washington, the longest-running show in television history. This is “Meet The Press” with Kristen Welker.
And here, all of a sudden, Kristin Welker is thrown for a loop.
In full disclosure to our viewers, this interview was scheduled weeks before it was announced that McDaniel would become a paid NBC News contributor.
Because now, she’s actually interviewing a member of the family who’s on the same payroll.
Right. Suddenly, she’s interviewing a colleague.
This will be a news interview, and I was not involved in her hiring.
So what happens during the interview?
So Welker is prepared for a tough interview, and that’s exactly what she does.
Can you say, as you sit here today, did Joe Biden win the election fair and square?
He won. He’s the legitimate president.
Did he win fair and square?
Fair and square, he won. It’s certified. It’s done.
She presses her on the key question that a lot of Republicans get asked these days — do you accept Joe Biden was the winner of the election?
But, I do think, Kristen —
Ronna, why has it taken you until now to say that? Why has it taken you until now to be able to say that?
I’m going to push back a little.
McDaniel gets defensive at times.
Because I do think it’s fair to say there were problems in 2020. And to say that does not mean he’s not the legitimate president.
But, Ronna, when you say that, it suggests that there was something wrong with the election. And you know that the election was the most heavily scrutinized. Chris Krebs —
It’s a really combative interview.
I want to turn now to your actions in the aftermath of the 2020 election.
And Welker actually really does go deeply into McDaniel’s record in those weeks before January 6.
On November 17, you and Donald Trump were recorded pushing two Republican Michigan election officials not to certify the results of the election. And on the call —
For instance, she presses McDaniel on McDaniel’s role in an attempt to convince a couple county commissioner level canvassers in Michigan to not certify Biden’s victory.
Our call that night was to say, are you OK? Vote your conscience. Not pushing them to do anything.
McDaniel says, look, I was just telling them to vote their conscience. They should do whatever they think is right.
But you said, do not sign it. If you can go home tonight, do not sign it. How can people read that as anything other than a pressure campaign?
And Welker’s not going to just let her off the hook. Welker presses her on Trump’s own comments about January 6 and Trump’s efforts recently to gloss over some of the violence, and to say that those who have been arrested, he’ll free them.
Do you support that?
I want to be very clear. The violence that happened on January 6 is unacceptable.
And this is a frankly fascinating moment because you can hear McDaniel starting to, if not quite reverse some of her positions, though in some cases she does that, at least really soften her language. It’s almost as if she’s switching uniforms from the RNC one to an NBC one or almost like breaking from a role she was playing.
Ronna, why not speak out earlier? Why just speak out about that now?
When you’re the RNC chair, you kind of take one for the whole team, right? Now, I get to be a little bit more myself.
She says, hey, you know what? Sometimes as RNC chair, you just have to take it for the team sometimes.
Right. What she’s really saying is I did things as chairwoman of the Republican National committee that now that I no longer have that job, I can candidly say, I wished I hadn’t done, which is very honest. But it’s also another way of saying I’m two faced, or I was playing a part.
Ronna McDaniel, thank you very much for being here this morning.
Then something extraordinary happens. And I have to say, I’ve never seen a moment like this in decades of watching television news and covering television news.
Welcome back. The panel is here. Chuck Todd, NBC News chief political analyst.
Welker brings her regular panel on, including Chuck Todd, now the senior NBC political analyst.
Chuck, let’s dive right in. What were your takeaways?
And he launches right into what he calls —
Look, let me deal with the elephant in the room.
The elephant being this hiring of McDaniel.
I think our bosses owe you an apology for putting you in this situation.
And he proceeds, on NBC’S air, to lace into management for, as he describes it, putting Welker in this crazy awkward position.
Because I don’t know what to believe. She is now a paid contributor by NBC News. I have no idea whether any answer she gave to you was because she didn’t want to mess up her contract.
And Todd is very hung up on this idea that when she was speaking for the party, she would say one thing. And now that she’s on the payroll at NBC, she’s saying another thing.
She has credibility issues that she still has to deal with. Is she speaking for herself, or is she speaking on behalf of who’s paying her?
Todd is basically saying, how are we supposed to know which one to believe.
What can we believe?
It is important for this network and for always to have a wide aperture. Having ideological diversity on this panel is something I prided myself on.
And what he’s effectively saying is that his bosses should have never hired her in this capacity.
I understand the motivation, but this execution, I think, was poor.
Someone said to me last night we live in complicated times. Thank you guys for being here. I really appreciate it.
Now, let’s just note here, this isn’t just any player at NBC. Chuck Todd is obviously a major news name at the network. And him doing this appears to just open the floodgates across the entire NBC News brand, especially on its sister cable network, MSNBC.
And where I said I’d never seen anything like what I saw on “Meet the Press” that morning, I’d never seen anything like this either. Because now, the entire MSNBC lineup is in open rebellion. I mean, from the minute that the sun comes up. There is Joe Scarborough and Mika Brzezinski.
We weren’t asked our opinion of the hiring. But if we were, we would have strongly objected to it.
They’re on fire over this.
believe NBC News should seek out conservative Republican voices, but it should be conservative Republicans, not a person who used her position of power to be an anti-democracy election denier.
But it rolls out across the entire schedule.
Because Ronna McDaniel has been a major peddler of the big lie.
The fact that Ms. McDaniel is on the payroll at NBC News, to me that is inexplicable. I mean, you wouldn’t hire a mobster to work at a DA’s office.
Rachel Maddow devotes an entire half hour.
It’s not about just being associated with Donald Trump and his time in the Republican Party. It’s not even about lying or not lying. It’s about our system of government.
Thumbing their noses at our bosses and basically accusing them of abetting a traitorous figure in American history. I mean, just extraordinary stuff. It’s television history.
And let’s face it, we journalists, our bosses, we can be seen as crybabies, and we’re paid complaining. Yeah, that’s what we’re paid to do. But in this case, the NBC executives cannot ignore this, because in the outcry, there’s a very clear point that they’re all making. Ronna McDaniel is not just a voice from the other side. She was a fundamental part of Trump’s efforts to deny his election loss.
This is not inviting the other side. This is someone who’s on the wrong side —
Of history.
Of history, of these moments that we’ve covered and are still covering.
And I think it’s fair to say that at this point, everyone understands that Ronna McDaniel’s time at NBC News is going to be very short lived. Yeah, basically, after all this, the executives at NBC have to face facts it’s over. And on Tuesday night, they release a statement to the staff saying as much.
They don’t cite the questions about red lines or what Ronna McDaniel represented or didn’t represent. They just say we need to have a unified newsroom. We want cohesion. This isn’t working.
I think in the end, she was a paid contributor for four days.
Yeah, one of the shortest tenures in television news history. And look, in one respect, by their standards, this is kind of a pretty small contract, a few hundred thousand dollars they may have to pay out. But it was way more costly because they hired her. They brought her on board because they wanted to appeal to these tens of millions of Americans who still love Donald J. Trump.
And what happens now is that this entire thing is blown up in their face, and those very same people now see a network that, in their view, in the view of Republicans across the country, this network will not accept any Republicans. So it becomes more about that. And Fox News, NBC’S longtime rival, goes wall to wall with this.
Now, NBC News just caved to the breathless demands from their far left, frankly, emotionally unhinged host.
I mean, I had it on my desk all day. And every minute I looked at that screen, it was pounding on these liberals at NBC News driving this Republican out.
It’s the shortest tenure in TV history, I think. But why? Well, because she supports Donald Trump, period.
So in a way, this leaves NBC worse off with that Trump Republican audience they had wanted to court than maybe even they were before. It’s like a boomerang with a grenade on it.
Yeah, it completely explodes in their face. And that’s why to me, the whole episode is so representative of this eight-year conundrum for the news media, especially on television. They still haven’t been able to crack the code for how to handle the Trump movement, the Trump candidacy, and what it has wrought on the American political system and American journalism.
We’ll be right back.
Jim, put into context this painful episode of NBC into that larger conundrum you just diagnosed that the media has faced when it comes to Trump.
Well, Michael, it’s been there from the very beginning, from the very beginning of his political rise. The media was on this kind of seesaw. They go back and forth over how to cover him. Sometimes they want to cover him quite aggressively because he’s such a challenging candidate. He was bursting so many norms.
But at other times, there was this instinct to understand his appeal, for the same reason. He’s such an unusual candidate. So there was a great desire to really understand his voters. And frankly, to speak to his voters, because they’re part of the audience. And we all lived it, right?
But just let me take you back anyway because everything’s fresh again with perspective. And so if you go back, let’s look at when he first ran. The networks, if you recall, saw him as almost like a novelty candidate.
He was going to spice up what was expected to be a boring campaign between the usual suspects. And he was a ratings magnet. And the networks, they just couldn’t get enough of it. And they allowed him, at times, to really shatter their own norms.
Welcome back to “Meet the Press,” sir.
Good morning, Chuck.
Good morning. Let me start —
He was able to just call into the studio and riff with the likes of George Stephanopoulos and Chuck Todd.
What does it have to do with Hillary?
She can’t talk about me because nobody respects women more than Donald Trump.
And CNN gave him a lot of unmitigated airtime, if you recall during the campaign. They would run the press conferences.
It’s the largest winery on the East Coast. I own it 100 percent.
And let him promote his Trump steaks and his Trump wine.
Trump steaks. Where are the steaks? Do we have steaks?
I mean, it got that crazy. But again, the ratings were huge. And then he wins. And because they had previously given him all that airtime, they’ve, in retrospect, sort of given him a political gift, and more than that now have a journalistic imperative to really address him in a different way, to cover him as they would have covered any other candidate, which, let’s face it, they weren’t doing initially. So there’s this extra motivation to make up for lost ground and maybe for some journalistic omissions.
Right. Kind of correct for the lack of a rigorous journalistic filter in the campaign.
Exactly. And the big thing that this will be remembered for is we’re going to call a lie a lie.
I don’t want to sugarcoat this because facts matter, and the fact is President Trump lies.
Trump lies. We’re going to say it’s a lie.
And I think we can’t just mince around it because they are lies. And so we need to call them what they are.
We’re no longer going to use euphemisms or looser language we’re. Going to call it for what it is.
Trump lies in tweets. He spreads false information at rallies. He lies when he doesn’t need to. He lies when the truth is more than enough for him.
CNN was running chyrons. They would fact check Trump and call lies lies on the screen while Trump is talking. They were challenging Trump to his face —
One of the statements that you made in the tail end of the campaign in the midterms that —
Here we go.
That — well, if you don’t mind, Mr. President, that this caravan was an invasion.
— in these crazy press conferences —
They’re are hundreds of miles away, though. They’re hundreds and hundreds of miles away. That’s not an invasion.
Honestly, I think you should let me run the country. You run CNN. And if you did it well, your ratings —
Well, let me ask — if I may ask one other question. Mr. President, if I may ask another question. Are you worried —
That’s enough. That’s enough.
And Trump is giving it right back.
I tell you what, CNN should be ashamed of itself having you working for them. You are a rude, terrible person. You shouldn’t be working for CNN.
Very combative.
So this was this incredibly fraught moment for the American press. You’ve got tens of millions of Trump supporters seeing what’s really basic fact checking. These look like attacks to Trump supporters. Trump, in turn, is calling the press, the reporters are enemies of the people. So it’s a terrible dynamic.
And when January 6 happens, it’s so obviously out of control. And what the traditional press that follows, traditional journalistic rules has to do is make it clear that the claims that Trump is making about a stolen election are just so abjectly false that they don’t warrant a single minute of real consideration once the reporting has been done to show how false they are. And I think that American journalism really emerged from that feeling strongly about its own values and its own place in society.
But then there’s still tens of millions of Trump voters, and they don’t feel so good about the coverage. And they don’t agree that January 6 was an insurrection. And so we enter yet another period, where the press is going to have to now maybe rethink some things.
In what way?
Well, there’s a kind of quiet period after January 6. Trump is off of social media. The smoke is literally dissipating from the air in Washington. And news executives are kind of standing there on the proverbial battlefield, taking a new look at their situation.
And they’re seeing that in this clearer light, they’ve got some new problems, perhaps none more important for their entire business models than that their ratings are quickly crashing. And part of that diminishment is that a huge part of the country, that Trump-loving part of the audience, is really now severed from him from their coverage.
They see the press as actually, in some cases, being complicit in stealing an election. And so these news executives, again, especially on television, which is so ratings dependent, they’ve got a problem. So after presumably learning all these lessons about journalism and how to confront power, there’s a first subtle and then much less subtle rethinking.
Maybe we need to pull back from that approach. And maybe we need to take some new lessons and switch it up a little bit and reverse some of what we did. And one of the best examples of this is none other than CNN.
It had come under new management, was being led by a guy named Chris Licht, a veteran of cable news, but also Stephen Colbert’s late night show in his last job. And his new job under this new management is we’re going to recalibrate a little bit. So Chris Licht proceeds to try to bring the network back to the center.
And how does he do that?
Well, we see some key personalities who represented the Trump combat era start losing air time and some of them lose their jobs. There’s talk of, we want more Republicans on the air. There was a famous magazine article about Chris Licht’s balancing act here.
And Chris Licht says to a reporter, Tim Alberta of the “Atlantic” magazine, look, a lot in the media, including at his own network, quote unquote, “put on a jersey, took a side.” They took a side. And he says, I think we understand that jersey cannot go back on him. Because he says in the end of the day, by the way, it didn’t even work. We didn’t change anyone’s mind.
He’s saying that confrontational approach that defined the four years Trump was in office, that was a reaction to the feeling that TV news had failed to properly treat Trump with sufficient skepticism, that that actually was a failure both of journalism and of the TV news business. Is that what he’s saying?
Yeah. On the business side, it’s easier call, right? You want a bigger audience, and you’re not getting the bigger audience. But he’s making a journalistic argument as well that if the job is to convey the truth and take it to the people, and they take that into account as they make their own voting decisions and formulate their own opinions about American politics, if tens of millions of people who do believe that election was stolen are completely tuning you out because now they see you as a political combatant, you’re not achieving your ultimate goal as a journalist.
And what does Licht’s “don’t put a jersey back on” approach look like on CNN for its viewers?
Well, It didn’t look good. People might remember this, but the most glaring example —
Please welcome, the front runner for the Republican nomination for president, Donald Trump.
— was when he held a town hall meeting featuring Donald J. Trump, now candidate Trump, before an audience packed with Trump’s fans.
You look at what happened during that election. Unless you’re a very stupid person, you see what happens. A lot of the people —
Trump let loose a string of falsehoods.
Most people understand what happened. It was a rigged election.
The audience is pro-Trump audience, was cheering him on.
Are you ready? Are you ready? Can I talk?
Yeah, what’s your answer?
Can I? Do you mind?
I would like for you to answer the question.
OK. It’s very simple to answer.
That’s why I asked it.
It’s very simple. You’re a nasty person, I’ll tell you that.
And during, the CNN anchor hosting this, Kaitlan Collins, on CNN’s own air, it was a disaster.
It felt like a callback to the unlearned lessons of 2016.
Yeah. And in this case, CNN’s staff was up in arms.
Big shakeup in the cable news industry as CNN makes another change at the top.
Chris Licht is officially out at CNN after a chaotic run as chairman and CEO.
And Chris Licht didn’t survive it.
The chief executive’s departure comes as he faced criticism in recent weeks after the network hosted a town hall with Donald Trump and the network’s ratings started to drop.
But I want to say that the CNN leadership still, even after that, as they brought new leadership in, said, this is still the path we’re going to go on. Maybe that didn’t work out, but we’re still here. This is still what we have to do.
Right. And this idea is very much in the water of TV news, that this is the right overall direction.
Yeah. This is, by no means, isolated to CNN. This is throughout the traditional news business. These conversations are happening everywhere. But CNN was living it at that point.
And this, of course, is how we get to NBC deciding to hire Ronna McDaniel.
Right. Because they’re picking up — right where that conversation leaves off, they’re having the same conversation. But for NBC, you could argue this tension between journalistic values and audience. It’s even more pressing. Because even though MSNBC is a niche cable network, NBC News is part of an old-fashioned broadcast network. It’s on television stations throughout the country.
And in fact, those networks, they still have 6:30 newscasts. And believe it or not, millions of people still watch those every night. Maybe not as many as they used to, but there’s still some six or seven million people tuning in to nightly news. That’s important.
Right. We should say that kind of number is sometimes double or triple that of the cable news prime time shows that get all the attention.
On their best nights. So this is big business still. And that business is based on broad — it’s called broadcast for a reason. That’s based on broad audiences. So NBC had a business imperative, and they argue they had a journalistic imperative.
So given all of that, Jim, I think the big messy question here is, when it comes to NBC, did they make a tactical error around hiring the wrong Republican which blew up? Or did they make an even larger error in thinking that the way you handle Trump and his supporters is to work this hard to reach them, when they might not even be reachable?
The best way to answer that question is to tell you what they’re saying right now, NBC management. What the management saying is, yes, this was a tactical error. This was clearly the wrong Republican. We get it.
But they’re saying, we are going to — and they said this in their statement, announcing that they were severing ties with McDaniel. They said, we’re going to redouble our efforts to represent a broad spectrum of the American votership. And that’s what they meant was that we’re going to still try to reach these Trump voters with people who can relate to them and they can relate to.
But the question is, how do you even do that when so many of his supporters believe a lie? How is NBC, how is CNN, how are any of these TV networks, if they have decided that this is their mission, how are they supposed to speak to people who believe something fundamentally untrue as a core part of their political identity?
That’s the catch-22. How do you get that Trump movement person who’s also an insider, when the litmus test to be an insider in the Trump movement is to believe in the denialism or at least say you do? So that’s a real journalistic problem. And the thing that we haven’t really touched here is, what are these networks doing day in and day out?
They’re not producing reported pieces, which I think it’s a little easier. You just report the news. You go out into the world. You talk to people, and then you present it to the world as a nuanced portrait of the country. This thing is true. This thing is false. Again, in many cases, pretty straightforward. But their bread and butter is talking heads. It’s live. It’s not edited. It’s not that much reported.
So their whole business model especially, again, on cable, which has 24 hours to fill, is talking heads. And if you want the perspective from the Trump movement, journalistically, especially when it comes to denialism, but when it comes to some other major subjects in American life, you’re walking into a place where they’re going to say things that aren’t true, that don’t pass your journalistic standards, the most basic standards of journalism.
Right. So you’re saying if TV sticks with this model, the kind of low cost, lots of talk approach to news, then they are going to have to solve the riddle of who to bring on, who represents Trump’s America if they want that audience. And now they’ve got this red line that they’ve established, that that person can’t be someone who denies the 2020 election reality. But like you just said, that’s the litmus test for being in Trump’s orbit.
So this doesn’t really look like a conundrum. This looks like a bit of a crisis for TV news because it may end up meaning that they can’t hire that person that they need for this model, which means that perhaps a network like NBC does need to wave goodbye to a big segment of these viewers and these eyeballs who support Trump.
I mean, on the one hand, they are not ready to do that, and they would never concede that that’s something they’re ready to do. The problem is barring some kind of change in their news model, there’s no solution to this.
But why bar changes to their news model, I guess, is the question. Because over the years, it’s gotten more and more expensive to produce news, the news that I’m talking about, like recorded packages and what we refer to as reporting. Just go out and report the news.
Don’t gab about it. Just what’s going on, what’s true, what’s false. That’s actually very expensive in television. And they don’t have the kind of money they used to have. So the talking heads is their way to do programming at a level where they can afford it.
They do some packages. “60 Minutes” still does incredible work. NBC does packages, but the lion’s share of what they do is what we’re talking about. And that’s not going to change because the economics aren’t there.
So then a final option, of course, to borrow something Chris Licht said, is that a network like NBC perhaps doesn’t put a jersey on, but accepts the reality that a lot of the world sees them wearing a jersey.
Yeah. I mean, nobody wants to be seen as wearing a jersey in our business. No one wants to be wearing a jersey on our business. But maybe what they really have to accept is that we’re just sticking to the true facts, and that may look like we’re wearing a jersey, but we’re not. And that may, at times, look like it’s lining up more with the Democrats, but we’re not.
If Trump is lying about a stolen election, that’s not siding against him. That’s siding for the truth, and that’s what we’re doing. Easier said than done. And I don’t think any of these concepts are new.
I think there have been attempts to do that, but it’s the world they’re in. And it’s the only option they really have. We’re going to tell you the truth, even if it means that we’re going to lose a big part of the country.
Well, Jim, thank you very much.
Thank you, Michael.
Here’s what else you need to know today.
[PROTESTERS CHANTING]
Over the weekend, thousands of protesters took to the streets of Tel Aviv and Jerusalem in some of the largest domestic demonstrations against the government of Prime Minister Benjamin Netanyahu since Israel invaded Gaza in the fall.
[NON-ENGLISH SPEECH]
Some of the protesters called on Netanyahu to reach a cease fire deal that would free the hostages taken by Hamas on October 7. Others called for early elections that would remove Netanyahu from office.
During a news conference on Sunday, Netanyahu rejected calls for early elections, saying they would paralyze his government at a crucial moment in the war.
Today’s episode was produced by Rob Szypko, Rikki Novetsky, and Alex Stern, with help from Stella Tan.
It was edited by Brendan Klinkenberg with help from Rachel Quester and Paige Cowett. Contains original music by Marion Lozano, Dan Powell, and Rowan Niemisto and was engineered by Chris Wood. Our theme music is by Jim Brunberg and Ben Landsverk of Wonderly.
That’s it for “The Daily.” I’m Michael Barbaro. See you tomorrow.

- April 2, 2024 • 29:32 Kids Are Missing School at an Alarming Rate
- April 1, 2024 • 36:14 Ronna McDaniel, TV News and the Trump Problem
- March 29, 2024 • 48:42 Hamas Took Her, and Still Has Her Husband
- March 28, 2024 • 33:40 The Newest Tech Start-Up Billionaire? Donald Trump.
- March 27, 2024 • 28:06 Democrats’ Plan to Save the Republican House Speaker
- March 26, 2024 • 29:13 The United States vs. the iPhone
- March 25, 2024 • 25:59 A Terrorist Attack in Russia
- March 24, 2024 • 21:39 The Sunday Read: ‘My Goldendoodle Spent a Week at Some Luxury Dog ‘Hotels.’ I Tagged Along.’
- March 22, 2024 • 35:30 Chuck Schumer on His Campaign to Oust Israel’s Leader
- March 21, 2024 • 27:18 The Caitlin Clark Phenomenon
- March 20, 2024 • 25:58 The Bombshell Case That Will Transform the Housing Market
- March 19, 2024 • 27:29 Trump’s Plan to Take Away Biden’s Biggest Advantage
Hosted by Michael Barbaro
Featuring Jim Rutenberg
Produced by Rob Szypko , Rikki Novetsky and Alex Stern
With Stella Tan
Edited by Brendan Klinkenberg , Rachel Quester and Paige Cowett
Original music by Marion Lozano , Dan Powell and Rowan Niemisto
Engineered by Chris Wood
Listen and follow The Daily Apple Podcasts | Spotify | Amazon Music
Ronna McDaniel’s time at NBC was short. The former Republican National Committee chairwoman was hired as an on-air political commentator but released just days later after an on-air revolt by the network’s leading stars.
Jim Rutenberg, a writer at large for The Times, discusses the saga and what it might reveal about the state of television news heading into the 2024 presidential race.
On today’s episode

Jim Rutenberg , a writer at large for The New York Times.

Background reading
Ms. McDaniel’s appointment had been immediately criticized by reporters at the network and by viewers on social media.
The former Republican Party leader tried to downplay her role in efforts to overturn the 2020 election. A review of the record shows she was involved in some key episodes .
There are a lot of ways to listen to The Daily. Here’s how.
We aim to make transcripts available the next workday after an episode’s publication. You can find them at the top of the page.
The Daily is made by Rachel Quester, Lynsea Garrison, Clare Toeniskoetter, Paige Cowett, Michael Simon Johnson, Brad Fisher, Chris Wood, Jessica Cheung, Stella Tan, Alexandra Leigh Young, Lisa Chow, Eric Krupke, Marc Georges, Luke Vander Ploeg, M.J. Davis Lin, Dan Powell, Sydney Harper, Mike Benoist, Liz O. Baylen, Asthaa Chaturvedi, Rachelle Bonja, Diana Nguyen, Marion Lozano, Corey Schreppel, Rob Szypko, Elisheba Ittoop, Mooj Zadie, Patricia Willens, Rowan Niemisto, Jody Becker, Rikki Novetsky, John Ketchum, Nina Feldman, Will Reid, Carlos Prieto, Ben Calhoun, Susan Lee, Lexie Diao, Mary Wilson, Alex Stern, Dan Farrell, Sophia Lanman, Shannon Lin, Diane Wong, Devon Taylor, Alyssa Moxley, Summer Thomad, Olivia Natt, Daniel Ramirez and Brendan Klinkenberg.
Our theme music is by Jim Brunberg and Ben Landsverk of Wonderly. Special thanks to Sam Dolnick, Paula Szuchman, Lisa Tobin, Larissa Anderson, Julia Simon, Sofia Milan, Mahima Chablani, Elizabeth Davis-Moorer, Jeffrey Miranda, Renan Borelli, Maddy Masiello, Isabella Anderson and Nina Lassam.
Jim Rutenberg is a writer at large for The Times and The New York Times Magazine and writes most often about media and politics. More about Jim Rutenberg
Advertisement

An official website of the United States government
The .gov means it’s official. Federal government websites often end in .gov or .mil. Before sharing sensitive information, make sure you’re on a federal government site.
The site is secure. The https:// ensures that you are connecting to the official website and that any information you provide is encrypted and transmitted securely.
- Publications
- Account settings
Preview improvements coming to the PMC website in October 2024. Learn More or Try it out now .
- Advanced Search
- Journal List
- Curr Genomics
- v.12(3); 2011 May
Genetics of Obesity: What have we Learned?
Hélène choquet.
1 Ernest Gallo Clinic and Research Center, Department of Neurology, University of California, San Francisco, Emeryville, California 94608, USA
David Meyre
2 Departments of Clinical Epidemiology and Biostatistics, McMaster University, Hamilton, Ontario L8N 3Z5, Canada
Candidate gene and genome-wide association studies have led to the discovery of nine loci involved in Mendelian forms of obesity and 58 loci contributing to polygenic obesity. These loci explain a small fraction of the heritability for obesity and many genes remain to be discovered. However, efforts in obesity gene identification greatly modified our understanding of this disorder. In this review, we propose an overlook of major lessons learned from 15 years of research in the field of genetics and obesity. We comment on the existence of the genetic continuum between monogenic and polygenic forms of obesity that pinpoints the role of genes involved in the central regulation of food intake and genetic predisposition to obesity. We explain how the identification of novel obesity predisposing genes has clarified unsuspected biological pathways involved in the control of energy balance that have helped to understand past human history and to explore causality in epidemiology. We provide evidence that obesity predisposing genes interact with the environment and influence the response to treatment relevant to disease prediction.
INTRODUCTION
In 2001, six genes were linked to monogenic human obesity and no common variants were reproducibly associated with polygenic obesity. By 2008, progress in the field led to the discovery of eight monogenic genes and four polygenic genes ( FTO, PCSK1, MC4R, CTNNBL1 ) from associated studies at the genome-wide level of significance. The recent emergence of the genome-wide association studies (GWAS) has led to further breakthroughs in gene identification and now nine loci are recognized to be involved in Mendelian forms of obesity along with 58 loci contributing to polygenic obesity. In this review, we will discuss what we have learned from this recent progress in elucidating the molecular basis of obesity. We propose an overlook of major lessons learned from 15 years of research in the field of the genetics and obesity.
GENETICS OF OBESITY: LESSONS LEARNED
A continuum between monogenic and polygenic obesity.
A striking observation is the existence of a partially overlapping continuum between monogenic and polygenic forms of obesity. Currently, four genes ( MC4R, PCSK1, POMC and BDNF ) have been involved in the two conditions, and this list is likely to grow in the upcoming years. This continuum is not specific to obesity, since one-fifth of the loci that were found to be associated with complex disease traits include a gene that is mutated in a corresponding single-gene disorder [ 1 ]. The case of MC4R is illustrative of this point of view. Whereas more than 150 loss-of-function coding mutations have been associated with monogenic obesity [ 2 ], two infrequent gain-of-function coding polymorphisms (V103I and I251L) have been associated with the protection from obesity [ 3 , 4 ]. Furthermore, a SNP located 188 kb downstream of the MC4R coding sequence has been associated with a modest increase in the risk for obesity [ 5 ].
Dickson et al . [ 6 ] have recently proposed the synthetic association hypothesis: GWAS signals of common non-functional SNPs outside of coding regions may be the result of a combination of rare/coding functional variants with stronger effects given that these rare variants arose on a haplotype which is tagged by the common SNP. This hypothesis, if true, may explain why some genes are associated with monogenic and polygenic forms of the same disease. A careful investigation of MC4R has therefore invalidated the synthetic association hypothesis at this locus, and supports the concept of an independent contribution of both rare and common variants at the same locus for obesity risk [ 7 ].
Another lesson of the observed continuum between monogenic and polygenic forms of obesity is that GWAS-derived novel loci should be considered as highly relevant candidate genes for monogenic obesity, especially if additional arguments in humans or animal models strengthen the candidacy of the gene. The SH2B1 gene is for instance an interesting candidate as SNPs at the SH2B1 locus are associated with BMI by GWAS [ 8 ], rare deletions including SH2B1 are associated with a Mendelian form of obesity [ 9 , 10 ] and inactivation of SH2B1 in mice leads to hyperphagia, leptin resistance and obesity [ 11 ]. However, notable exceptions have been reported for other promising candidate genes. For example, FTO is the major contributor to polygenic obesity [ 12 ] and mice down or over-expressing FTO are resistant or prone to develop obesity [ 13 , 14 ]. However, heterozygous loss-of-function mutations in FTO are found in both lean and obese subjects and do not contribute to monogenic obesity [ 15 ].
Obesity is an Inherited Disorder of Central Regulation of Food Intake
Defects in eight genes involved in the neuronal differentiation of the paraventricular nucleus and in the leptin/melanocortin pathway, have been shown to lead to human monogenic obesity with hyperphagia as a common feature [ 16 ]. Recent progress in the elucidation of polygenic predisposition to obesity also points to a key role of the central nervous system in body weight regulation [ 17 ].
The association of the two major contributors to polygenic obesity (SNP rs17782313 near MC4R and SNP rs1421085 / rs9939609 in FTO ) [ 5 , 18 , 19 ] with food intake / food behavior-related endophenotypes has been well documented in the literature. The obesity predisposing FTO variant was associated with increased total and fat dietary intake in children [ 20 , 21 ] as well as in adults [ 22 ]. The obesity risk variant was also associated with diminished satiety and / or increased feeling of hunger in children [ 23 ] and in adults [ 24 ]. The obesity predisposing SNP variant near MC4R was associated with increased feeling of hunger [ 25 , 26 ], increased snacking [ 25 ], decreased satiety [ 26 ], and increased total, fat and protein energy intake [ 25 , 27 ], the effects of the variant on food-related parameters being observed both in children and adults. Bauer et al . [ 28 ] recently reported evidence for an association of additional obesity genes recently identified by GWAS ( SH2B1, KCTD15, MTCH2, NEGR1, BDNF ) with dietary intake and nutrient-specific food preference.
The genetic dissection of monogenic and polygenic forms of obesity delineate it as an inherited disorder of central regulation of food intake [ 16 ]. This is in line with the fact that food intake-related parameters are heritable [ 29 ] and are strongly correlated to body mass index [ 30 ].
Gene Identification Illuminates New Pathways Involved in Energy Balance
A primary goal of human genetic agnostic approaches such as GWAS is to improve our understanding of the biologic pathways underlying polygenic diseases and traits [ 1 ]. A majority of obesity loci identified by GWAS studies do not harbor genes with clear connections to the biology of body weight regulation [ 12 ], reflecting our limited understanding of the biology of obesity in contrast with other complex traits (such as autoimmune diseases or lipid levels). Recent progress in genetic dissection of obesity predisposition provides the opportunity to explore novel and sometimes unsuspected pathways related to this condition.
The FTO story constitutes a textbook case: the FTO gene has been cloned in mice in 1999 [ 31 ] but the “buzz” around this gene started after the publication of two seminal genetic studies demonstrating a link between FTO common gene variation and human obesity in 2007 (at this time, FTO was a gene of unknown function in an unknown pathway) [ 18 , 19 ]. More than 400 articles have been published since increasing our understanding of the mechanisms linking this gene to the pathophysiology of obesity. Follow-up studies have confirmed the association between FTO and obesity-related phenotypes not only in populations of European ancestry but also in African, Asian, South Asian, South American and Pima Indian populations [ 32 - 36 ].
Complete FTO deficiency in humans is associated with an autosomal-recessive lethal syndrome including growth retardation, multiple malformations and premature death, indicating that FTO is essential for normal development of the central nervous and cardiovascular systems in human [ 37 ]. Loss of one functional copy of FTO in humans was not associated with a specific phenotype, and heterozygous loss-of-function mutations are found both in lean and in obese subjects [ 15 ]. Complete or partial inactivation of the Fto gene in mice protects from obesity [ 13 , 38 ] whereas over-expression of Fto in mice increases food intake and results in obesity [ 14 ]. These data have provided direct functional evidence that FTO is a causal gene underlying obesity, and suggest the intronic variant in FTO may increase obesity risk in humans through FTO gain of expression. Expression studies in wild-type rodents have shown that FTO is highly expressed in the hypothalamus and is regulated by feeding and fasting [ 39 , 40 ]. Over- or down-expression of Fto in the hypothalamus modulates food intake in mice possibly through the leptin / STAT3 signalling pathway [ 40 , 41 ].
Bioinformatics, in vitro and crystallography studies have shown that FTO is a single-stranded DNA demethylase and is involved in nucleic acid repair or modification processes [ 39 , 42 ]. The link between this genes’ ability to modify nucleic acids and body weight regulation may be puzzling, but may relate to epigenetic processes. FTO has been proposed as a transcriptional coactivator that enhances the transactivation potential of the CCAAT / enhancer binding proteins (C/EBPs) from unmethylated as well as methylation-inhibited promoters, suggesting a role in the epigenetic regulation of the development and maintenance of fat tissue [ 43 ]. In line with this hypothesis the fact that the FTO intronic SNP is associated with a distinct methylation pattern of a 7.7 kb region at the FTO locus, that includes a highly conserved non-coding element validated as a long-range enhancer [ 44 ].
The study of FTO illustrates how human genetic “hypothesis free” approaches can be a catalyst to approaches in functional genomics and the same integrative approach can be applied to other obesity-associated genes markedly increasing our understanding of the physiology of obesity in the upcoming years.
Obesity Genes to Explore Causality in Epidemiology: The Mendelian Randomization Approach
Spurious associations in observational epidemiological studies are commonly caused by confounders due to social, behavioral, or environmental factors and can therefore be difficult to control. They may also be due to reverse causation, in which the phenotypic outcome subsequently influences an environmental exposure such that it is wrongly implicated in the pathogenesis. Genetic epidemiology can be used to uncover more thoroughly and more accurately causal factors underlying common diseases or complex traits. The epidemiologic approach with the most promise is often referred to as Mendelian randomization [ 45 ]. The principle is to use genetic variation as a randomly redistributed variable among populations to control for unobserved confounding variables in an observational setting [ 45 ].
This approach has been successfully used in the obesity arena following the identification of FTO as a major contributor to polygenic variation [ 18 ]. The Frayling et al. [ 18 ] seminal study represents in fact a good example of Mendelian randomization since a BMI-dependent association between FTO and type 2 diabetes mellitus (T2D) has been observed, suggestive of a causative relationship between weight gain and subsequent T2D development. The same approach, when applied to 12 obesity gene variants, confirmed that the genetic predisposition to obesity leads to an increased risk of developing type 2 diabetes, which is completely mediated by its effect on BMI [ 46 ]. The FTO genotype has also been used to confirm the findings of observational epidemiology and a causal relationship between BMI increase and altered glucose [ 47 ], insulin resistance [ 48 ], lipid [ 47 ] and blood pressure values [ 49 ]. The causal association between increased BMI and increased level of inflammation [ 50 ] or increased bone mass [ 51 ] has also been confirmed, and the use of FTO SNP as a randomly redistributed variable strengthened the evidence of a causal link between a BMI increase and an increased risk of atherosclerosis [ 52 ], cardiovascular diseases [ 53 ], endometrial or kidney cancers [ 54 , 55 ] and a decreased risk of lung or prostate cancers [ 54 , 56 ].
Genes are Useful to Understand Past Human History
The human genome contains hundreds of regions whose pattern of genetic diversity indicate recent positive natural selection (positive natural selection is the force that drives the increase in prevalence of advantageous traits like de novo mutations) [ 57 ]. Adaptation to new environments, infectious diseases and changes in diet may explain why certain mutations have been positively selected in human populations [ 58 ]. The transition to agriculture has introduced new adaptive pressures that shaped our genome to an increased fat storage efficiency including exposure to regular famine, adaptation to a variety of local niches favoring population-specific adaptations and the development of social hierarchies which predispose to differential exposure to environmental pressures [ 59 ]. The “thrifty genotype” hypothesis, proposed by Neel in 1962 [ 60 ], was recently confirmed for several obesity genes that show evidence of positive selection across human history. For example, the rs4988235 functional variant in the lactase ( LCT ) gene confers lactase persistence and carriers of at least one T allele, are able to digest the milk sugar lactose across their life span (the activity of the lactase enzyme in intestinal cells declines during childhood in non-carriers) [ 61 , 62 ]. The selective advantage of lactase persistence in milk-producing dairy farming populations has induced positive selection signatures regionally for the LCT rs4988235 T variant strongly related to events of cattle domestication [ 63 , 64 ]. A North to South gradient has been observed for the LCT rs4988235 SNP in Europe [ 63 ] as well as local geographic population substructures among provinces in the United Kingdom [ 65 ]. In line with its proposed selective advantage, the LCT rs4988235 T variant has been consistently associated with higher milk consumption [ 66 ] and with higher body mass index [ 67 ] in European populations.
Interestingly, three functional coding non-synonymous variants in the LEPR (Lys109Arg), ADRB3 (Trp64Arg) and BDNF (Val66Met) genes previously associated with BMI [ 8 , 68 , 69 ], harbor patterns of strong positive selection in population genetic studies [ 70 - 72 ]. Recently, analysis of GWAS-derived obesity gene variants provided evidence of positive natural selection at the FTO , NEGR1 , SH2B1 and FAIM2 loci [ 73 , 74 ].
The evolutionary history of the MC4R obesity locus has been well documented in the literature. The melanocortin 4 receptor coding sequence has been remarkably conserved in structure and pharmacology for more than 400 million years, implying that this receptor participated in vital physiological functions early in vertebrate evolution [ 75 ]. There is a significant paucity of diversity at the MC4R gene in humans in comparison with primates [ 76 ]. The coding region of MC4R has been subject to high levels of continuous purifying selection that increased threefold during primate evolution [ 76 ]. Finally, there is a tendency for non-synonymous mutations that impact MC4R function to be located at amino acid positions that are highly conserved during the 450 million years of MC4R evolution in vertebrates and subject to very strong purifying selection [ 76 ].
Genes Interacting with Environments
As trends over the past several decades suggest an environmental influence on BMI, many researchers have focused on the identification of specific environmental factors that interact with genetic predisposition to obesity. They based their investigations on epidemiological data showing that physical activity, diet, educational status, age, gender and ethnicity among others modulate the risk for obesity [ 77 ].
Recent literature provides firm evidence that genetic susceptibility to obesity can be blunted in part through physical activity. Thirteen independent studies reported an interaction between the FTO obesity risk genotype and physical activity on BMI variation or obesity risk including adults as well as adolescents [ 78 - 81 ]. Similar results were obtained for a genetic predisposition score combining the information of 12 obesity-associated SNPs, and a high level of physical activity associated with a 40% reduction in the genetic predisposition to common obesity [ 82 ].
There is also growing evidence that dietary habits interact with genes to modulate predisposition to obesity. Three studies suggest that a high fat diet can amplify the effect of the FTO genotype on obesity risk [ 79 - 81 ]. An interaction between the Apolipoprotein A-II ( APOA2 ) -265T>C SNP and high-saturated fat in relation to BMI and obesity has been reported in five independent populations [ 83 , 84 ]. Interestingly, this SNP was not identified by recent GWAS approaches, suggesting that some associations restricted to specific environments may be missed in global analyses.
Epidemiological studies have shown that people with a low level of education are more likely to develop obesity [ 85 ]. However, very few studies have investigated the impact of genes on the association between education and obesity-related variables. This well-established negative association between BMI and educational status was not found in MC4R loss-of function mutation carriers, although a significant relationship was seen in MC4R non-mutation carriers of the corresponding pedigrees [ 2 ]. These results suggest that a high level of education has no protective effect on obesity risk in presence of MC4R pathogenic mutations. On the contrary, a significant gene x education interaction has been found for the intron 1 variant in FTO, the significant effect of the SNP on BMI and obesity risk being restricted to subjects with no university education [ 86 ].
Age-dependent genetic associations have been described both in the context of monogenic and polygenic obesity. An age-dependent penetrance of MC4R pathogenic and monogenic mutations on obesity has been found in multigenerational pedigrees, the effect of mutations on the obesity phenotype being amplified by the development of an "obesogenic" environment [ 2 ]. The longitudinal study of adult MC4R mutation carriers showed an increasing age-dependent penetrance (37% at 20 years versus 60% at >40 years) [ 2 ]. The life-course analysis of the intronic FTO gene variant and body mass index in independent longitudinal studies indicates that most of the effect of the SNP on BMI gain occurs during childhood, adolescence and young adulthood [ 87 - 89 ].
Gender can be assimilated as a specific environmental condition. Females are at higher risk of developing morbid obesity than males [ 90 ]. These discrepancies could be explained in part by female-specific genetic associations or by stronger effect sizes of genetic variants in females. This was observed for the carriers of MC4R pathogenic monogenic mutations since BMI was about twice as strong in females than in males [ 2 , 91 ]. The effect of the functional polymorphism R125W polymorphism in TBC1 domain family member 1 ( TBC1D1 ) gene on severe obesity risk was restricted to females in French and US populations [ 92 , 93 ]. Seven out of 14 loci convincingly associated with waist to hip ratio exhibited marked sexual dimorphism, all with a stronger effect on the phenotype in women than men [ 94 , 95 ].
Ethnicity can be considered as an environmental factor that affects the genetic susceptibility to obesity. A convincing example of ethnic-specific association with obesity has been reported for the SIM1 gene. Variants in intronic regions of SIM1 were strongly associated with BMI and obesity risk (P = 4 x 10 -7 ) in Pima Indians contrarily to French Europeans for which a major contribution of SIM1 common variants in polygenic obesity susceptibility was excluded [ 96 , 97 ]. A functional coding variant (W64R) [ 98 ] in the ADRB3 gene has also been convincingly associated with BMI in East Asian but not in European subjects in a large meta-analysis of 44,833 subjects [ 69 ], the effect of the R64 allele on BMI increase being four-fold higher in Asian than in European subjects. More recently, a SNP (rs2074356) in the 24 th intron of the C12orf51 transcript has been strongly associated with waist to hip ratio (P = 7.8 x 10 -12 ) in the Korean population [ 99 ]. This variant has not been identified in a large GWAS meta-analysis for waist to hip ratio conducted in 77,167 individuals of European ancestry [ 95 ], suggesting an ethnic-specific association at this locus. These studies highlight the complex interplay between genetic susceptibility to obesity and environment.
Genes Influencing Response to Treatment
To date, three main therapeutic options are proposed to treat obesity: lifestyle intervention, pharmacotherapy and bariatric surgery. The aim of these therapeutics for obesity are to lose weight and maintain this weight-loss on the long term and attenuate co-morbidities related to obesity. There is growing evidence that genetic factors not only predispose to weight gain and development of obesity, but also modulate the response to therapeutic intervention in terms of weight loss.
Lifestyle Modifications
Individuals with MC4R or POMC monogenic conditions respond well to hypocaloric dietary or multidisciplinary (exercise, behavior, nutrition therapy) interventions as do non-monogenic obese subjects [ 100 , 101 ] but MC4R individuals fail to maintain weight loss after intervention [ 101 ]. The major gene variant contributing to polygenic obesity FTO does not modify the response to lifestyle intervention in terms of weight loss [ 102 ], but may interact with specific components of the lifestyle intervention program like the type of diet proposed during the caloric restriction program (high-fat, low-fat, Mediterranean diets) [ 103 , 104 ] or with physical activity [ 105 ] to modulate weight loss.
Pharmacotherapy
To date, only one successful personalized medicine approach which is based on a genetic diagnosis has been reported in the literature in the context of obesity: individuals with congenital leptin deficiency can be treated with daily injections of recombinant human leptin, which reverses the obesity and associated phenotypic abnormalities [ 106 ]. Leptin administration dramatically reduces food intake, fat mass, hyperinsulinemia, and hyperlipidemia, restores normal pubertal development, endocrine and immune function and increases performances in many neurocognitive domains [ 107 ]. Individuals with complete leptin deficiency are extremely rare (14 are reported so far worldwide) but peripheral leptin supplementation may also be extended to a numerically significant group of obese subjects with partial leptin deficiency, on the observation that peripheral leptin supplementation induces significant weight loss in those with low levels of leptin [ 108 ]. In addition, chemical chaperones and pharmacological agonists efficiently restore cell surface expression and endogenous agonist response of mutated melanocortin 4 receptors [ 109 , 110 ], but in vivo beneficial effects in MC4R deficient monogenic patients remain to be demonstrated.
To date, the two main anti-obesity drugs used are orlistat and sibutramine (a saturated derivative of lipstatin and a serotonin-norepinephrine reuptake inhibitor, respectively). The guanine nucleotide binding protein beta polypeptide 3 ( GNB3 ) gene C825T polymorphism is highly predictive for the identification of obese individuals who will benefit from sibutramine treatment. Thus, three independent pharmacogenetic studies have shown an association between the GNB3 C825T polymorphism and weight loss induced by sibutramine [ 111 - 113 ]. Interestingly, this locus has not been identified by recent GWAS on obesity-related traits, suggesting that the genes associated with BMI variation and obesity risk may be at least partly different from genes involved in therapeutic response in terms of weight loss.
Bariatric Surgery
Bariatric surgery is the most effective long-term treatment for severe obesity, reducing obesity-associated co-morbidities but the mechanisms of weight loss after bariatric surgery and the role of central energy homeostatic pathways in this weight loss process are not well understood. Two recent studies assessed the response to bariatric surgery of MC4R monogenic mutation carriers [ 114 , 115 ]. The Rouxen-Y gastric bypass surgery was associated with a similar percentage of excess weight loss in four heterozygous MC4R mutation carriers and in matched MC4R mutation non-carrier obese controls [ 115 ]. On the contrary, an adolescent with complete MC4R deficiency underwent laparoscopic adjustable gastric banding at 18 years of age which resulted in an initial, but not long-term weight loss [ 114 ]. These preliminary results need to be confirmed in larger studies, but logically suggest that diversionary operations, which are more invasive, efficiently improve the neuro-hormonal control of satiety better than gastric banding procedures, and are therefore indicated in monogenic hyperphagic subjects.
In the Swedish obesity intervention study, the FTO obesity predisposing allele carriers lost 3kg less than common allele homozygotes after obesity surgery, and this association was restricted to those undergoing banding surgery but was not significant in the gastric bypass operated subjects [ 116 ]. In a cohort of 1,001 severely obese subjects who underwent gastric bypass surgery, an allelic risk score combining the genetic information of four obesity-associated SNPs was significantly associated with postoperative weight loss trajectories [ 117 ]. Thus, obesity predisposing genes modulate the response to therapeutic options in terms of weight loss suggesting that genetic diagnosis combined with a genomic personalized medicine approach is a plausible strategy in order to design and implement the most suitable treatment and to achieve higher rates of therapeutic success.
Obesity Genes and Disease Prediction
Traditional approaches for the management of overweight and obesity have proven poor long term efficacy and obesity surgery is an efficient but invasive procedure. Prevention may therefore be considered as a promising strategy to face the obesity epidemic. In that context, the use of genetic information in clinical practice to predict individuals at high risk early in life and before the development of the disease remains the 'Holy Grail' for many geneticists [ 118 ]. Is the current knowledge about obesity genetics, sufficient to envisage such translational medicine applications?
Common Genetic Variants: Still Few Informative
GWAS allowed the identification of 36 polymorphisms robustly associated with BMI. However, identified variants have small effect sizes and collectively explain 1.45% of the variance in BMI (0.34% explained by the SNP in intron 1 of FTO alone) [ 12 ]. Therefore, it is not surprising that the combined information of 12-20 obesity predisposing SNPs provides only a slight increase in the ability to predict obesity in comparison with conventional nongenetic risk factors and has no clinical utility [ 119 ]. Risk prediction using GWAS remain conceivable despite the fact that individual effect sizes of variants associated with the phenotype are mostly small. In fact, it seems that many disease-associated variants are not yet identified prospects for risk prediction, but may improve if more disease predisposing variants are included in the models [ 120 ]. New iterative algorithms have been recently proposed to make better use of the whole-genome SNP information to improve the performance of disease risk assessment by utilizing a larger number of SNPs than those which reach genome-wide significance [ 120 , 121 ].
Monogenic Genes may Explain a Non-Negligible Fraction of Obesity
The cumulative prevalence of monogenic obesity elucidated by the eight currently known genes and the 16p11.2 deletion has not been evaluated in a randomly ascertained cohort of obese subjects to date, but may be estimated between 5 and 10 %. These results re-emphasize the importance of monogenic obesity in elucidating the heritability of obesity, and Mendelian forms of obesity may provide a non-negligible predictive value in classifying young subjects at high risk for the development of childhood obesity, as deleterious coding mutations or chromosomal aberrations in these genes / regions induce highly penetrant forms of obesity. subjects carrying these mutations, present specific features according to the impaired gene (such as a low level of circulating leptin despite severe obesity, a susceptibility to infections, intestinal dysfunction, reactive hypoglycaemia, red hair and pale skin, adrenal insufficiency) that can guide gene sequencing approaches. Early diagnosis is fundamental for personalized prevention and effective therapeutic management, and in young non-obese individuals carrying MC4R monogenic mutations, an appropriate medical follow-up to prevent or at least delay the onset of obesity [ 122 ].
As hyperphagia is a common feature of monogenic obesity, the most effective preventive strategy may be stringent restriction of food access. This will require training and active participation of the parents and care providers and the identification of critical environmental components (physical activity, rural / urban environment, dietary profile, family structure, socioeconomic status, social network, psychosocial stress) that modulate the penetrance of obesity associated with pathogenic mutations in order to avoid unhealthy environments for these subjects.
In conclusion, we have demonstrated that 15 years of gene identification efforts have considerably modified our understanding of the biology of obesity. Promising approaches such as whole-exome and ultimately whole-genome sequencing have the potential to lead to an exhaustive map of obesity predisposing genes in the near future. Recent gene identification efforts have provided a more comprehensive picture of the biological mechanisms involved in the development of obesity and we feel that this information can be meaningful not only for scientists and clinicians but for a more general audience. For instance, the recent discoveries in genetics have found that people differ in their perceptions of hunger and satiety on a genetic basis and that predisposed subgroups of the population may be particularly vulnerable to obesity in “obesogenic” societies with unlimited access to food. This notion must lead to a more open attitude toward obese people and a reduction in discrimination against them [ 123 ], it is clear that obesity cannot be considered as a consequence only of indolence or lack of will, as often thought in our societies. In the long term, we are confident that progress in genetics will help to develop useful diagnostic and predictive tests and design new treatments.
ACKNOWLEDGEMENTS
We thank Yoan Gerrard for the editing of the manuscript, and the reviewers for their helpful comments.
AUTHORS’ CONTRIBUTIONS
Both authors contributed to the conception and production of the manuscript and approved the final version.
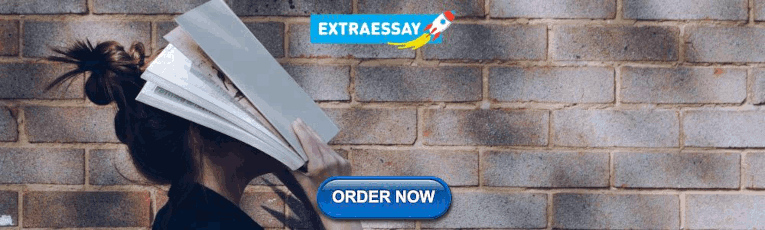
IMAGES
VIDEO
COMMENTS
Crucially, there is a strong genetic component underlying the large interindividual variation in body weight that determines people's response to this 'obesogenic' environment. Twin, family ...
Genetics is now known to play a substantial role in the predisposition to obesity and may contribute up to 70% risk for the disease. Over a hundred genes and gene variants related to excess weight have been discovered. Yet, genetic obesity risk does not always translate into actual obesity development, suggesting complex interactions between ...
According to the Centers for Disease Control and Prevention (CDC), there has been a steady increase in the rates of obesity in the United States over the past two decades, rising from 19.4% in 1997, to 24.5% in 2004, 26.6% in 2007, 33.8% in 2008, and 35.7% of adults in 2010.
2.1. Leptin. Leptin is a protein secreted by white adipose tissue and encoded by a gene on chromosome 7 in humans. Leptin crosses the blood-brain barrier to bind to the presynaptic GABAergic neurons of the hypothalamus and decreases appetite and increases energy expenditure [].In the arcuate nucleus of the hypothalamus, leptin binds to its receptor and inhibits the neuropeptide Y (NPY ...
Obesity-promoting alleles exert minimal effects in normal weight individuals but have larger effects in individuals with a proneness to obesity, suggesting a higher penetrance; however, it is not known whether these larger effect sizes precede obesity or are caused by an obese state.
Introduction. Obesity is associated with premature mortality and is a serious public health threat that accounts for a large proportion of the worldwide non-communicable disease burden, including type 2 diabetes, cardiovascular disease, hypertension and certain cancers 1,2.Mechanical issues resulting from substantially increased weight, such as osteoarthritis and sleep apnoea, also affect ...
Citation 4 Concurrently, research has increasingly recognized that the relationship between dietary intake and obesity is not straightforward, suggesting that genetic factors may modulate the effects of dietary intake on weight status. Genome-wide association studies (GWAS) have identified numerous genetic loci linked to body mass index (BMI).
In the early phase of obesity genetic research, the emphasis was on candidate genes of obesity (See Rankinen et al. for a review) (). A literature search identified 547 candidate genes derived from multiple types of studies, and the contribution of SNPs located in ±10 kb flanking sequences around these genes was investigated (). It was ...
Previous research has investigated the genetic regulation of blood pressure regulatory genes using post-GWAS data , ... demonstrating a strong relationship between the brain and obesity.
Around 250 genes are now associated with obesity. The FTO gene on chromosome 16 is the most important and carries the highest risk of the obesity phenotype. The Genetic Investigation of Anthropomorphic Traits Consortium is the organization involved in furthering research in GWAS. However, genetic mutations alone cannot explain the heritability ...
Obesity is a common, serious, and costly disease. More than 1 billion people worldwide are obese—650 million adults, 340 million adolescents, and 39 million children. The WHO estimates that, by 2025, approximately 167 million people—adults and children—will become less healthy because they are overweight or obese. Obesity-related conditions include heart disease, stroke, type 2 diabetes ...
Some genetic and lifestyle factors affect an individual's likelihood of adult obesity; thus, the significant clusters of obesity observed in specific geographical regions and contexts also signal the impact of socioeconomic and environmental factors in "obesogenic" environments [13].Understanding the causes and determinants of obesity is a critical step toward creating effective policy and ...
Purpose of Review Enormous progress has been made in understanding the genetic architecture of obesity and the correlation of epigenetic marks with obesity and related traits. This review highlights current research and its challenges in genetics and epigenetics of obesity. Recent Findings Recent progress in genetics of polygenic traits, particularly represented by genome-wide association ...
Importance Despite consistent public health recommendations, obesity rates in the US continue to increase. Physical activity recommendations do not account for individual genetic variability, increasing risk of obesity. Objective To use activity, clinical, and genetic data from the All of Us Research Program (AoURP) to explore the association of genetic risk of higher body mass index (BMI ...
The candidate gene approach was first applied in the mid-1990s and aimed to validate genes identified through human and animal models of extreme obesity for a role in common obesity (fig. 3 ...
This paper discusses recent advances in the field of genetics of obesity with an emphasis on several established loci that influence obesity. ... Results suggest a possible relationship between prevalence of obesity and severity of mental ... Genome-wide association study is an approach used in genetics research to look for associations between ...
Obesity develops as a result of dietary and lifestyle factors, but studies also suggest a genetic influence on obesity [ 11 ]. Obesity is highly influenced by genetics; available data suggest that 40% to 77% of the observed variance in human body weight can be accounted for, by inherited factors [ 12 - 14 ].
Obesity prevalence among children is >30% in the Cook Islands, Nauru and Palau, with a notable increase over the past few decades. Worldwide prevalence of obesity increased at an alarming rate in ...
Persons with a higher genetic risk of obesity need to work out harder than those of moderate or low genetic risk to avoid becoming obese, according to a Vanderbilt University Medical Center (VUMC ...
Obesity is a major health problem worldwide. It is more common in established countries but is on the increase in developing countries. The worldwide prevalence of obesity [body mass index (BMI) ≥ 30 kg m 2] has doubled between 1980 and 2008, with a prevalence of 13% in the adult population reported in 2014.In 2013, there were 42 million obese children below the age of five years [].
The obesity epidemic around the world affects not only adults but also children. About 50% of the time, obesity in childhood is carried into adulthood in a phenomenon known as "tracking." Per the latest data from the World Health Organization, the number of overweight and obese children under five years of age is estimated to be close to 39 million. In the United States, 1 in 3 adult Americans ...
Persons with a higher genetic risk of obesity need to work out harder than those of moderate or low genetic risk to avoid becoming obese, according to a Vanderbilt University Medical Center (VUMC) paper published in JAMA Network Open. Study authors used activity, clinical and genetic data from the National Institutes of Health's All of Us Research Program to explore the association of ...
Background: Magnesium (Mg) is an essential element and participates in many metabolic pathways. Many studies have found a certain negative correlation between magnesium and blood glucose parameters, but the dose-response relationship between them is still a relatively narrow research field. We aim to explore the dose-response relationship between plasma and dietary Mg and type 2 diabetes ...
Therefore, the knowledge of the interactions between the genome and nutrients at the molecular level, has led to the advent of nutritional genomics, which involves the sciences of nutrigenomics and nutrigenetics. In this review, we will comprehensively analyze the role of the most important genes associated with two interrelated chronic medical ...
Sethi, who is board certified in obesity and psychiatry, remembers when she first noticed the connection. As a medical student working in an obesity clinic, she saw a patient with treatment-resistant schizophrenia whose auditory hallucinations quieted on a ketogenic diet. That prompted her to dig into the medical literature.
2.0. Syndromic Childhood Obesity. Syndromic childhood obesity is a class of rare forms of the disease, where obesity presents as part of a distinct set of clinical phenotypes, commonly including developmental delay and dysmorphic characteristics [].Approximately 25 different types of syndromic obesity have been described, which are based on a series of genetic abnormalities occurring on the ...
The Sunday Read: 'My Goldendoodle Spent a Week at Some Luxury Dog 'Hotels.' I Tagged Along.'
Obesity is an Inherited Disorder of Central Regulation of Food Intake . Defects in eight genes involved in the neuronal differentiation of the paraventricular nucleus and in the leptin/melanocortin pathway, have been shown to lead to human monogenic obesity with hyperphagia as a common feature [].Recent progress in the elucidation of polygenic predisposition to obesity also points to a key ...