
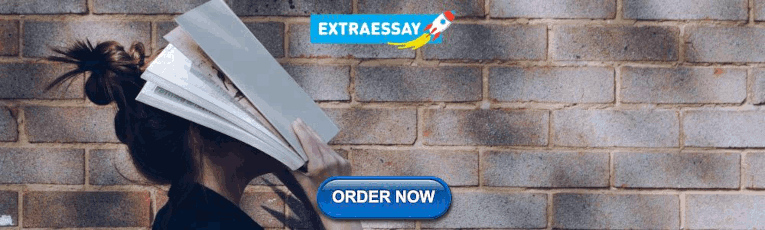
The Impact of COVID-19 on the Careers of Women in Academic Sciences, Engineering, and Medicine (2021)
Chapter: 8 major findings and research questions, 8 major findings and research questions, introduction.
The COVID-19 pandemic, which began in late 2019, created unprecedented global disruption and infused a significant level of uncertainty into the lives of individuals, both personally and professionally, around the world throughout 2020. The significant effect on vulnerable populations, such as essential workers and the elderly, is well documented, as is the devastating effect the COVID-19 pandemic had on the economy, particularly brick-and-mortar retail and hospitality and food services. Concurrently, the deaths of unarmed Black people at the hands of law enforcement officers created a heightened awareness of the persistence of structural injustices in U.S. society.
Against the backdrop of this public health crisis, economic upheaval, and amplified social consciousness, an ad hoc committee was appointed to review the potential effects of the COVID-19 pandemic on women in academic science, technology, engineering, mathematics, and medicine (STEMM) during 2020. The committee’s work built on the National Academies of Sciences, Engineering, and Medicine report Promising Practices for Addressing the Underrepresentation of Women in Science, Engineering, and Medicine: Opening Doors (the Promising Practices report), which presents evidence-based recommendations to address the well-established structural barriers that impede the advancement of women in STEMM. However, the committee recognized that none of the actions identified in the Promising Practices report were conceived within the context of a pandemic, an economic downturn, or the emergence of national protests against structural racism. The representation and vitality of academic women in STEMM had already warranted national attention prior to these events, and the COVID-19
pandemic appeared to represent an additional risk to the fragile progress that women had made in some STEMM disciplines. Furthermore, the future will almost certainly hold additional, unforeseen disruptions, which underscores the importance of the committee’s work.
In times of stress, there is a risk that the divide will deepen between those who already have advantages and those who do not. In academia, senior and tenured academics are more likely to have an established reputation, a stable salary commitment, and power within the academic system. They are more likely, before the COVID-19 pandemic began, to have established professional networks, generated data that can be used to write papers, and achieved financial and job security. While those who have these advantages may benefit from a level of stability relative to others during stressful times, those who were previously systemically disadvantaged are more likely to experience additional strain and instability.
As this report has documented, during 2020 the COVID-19 pandemic had overall negative effects on women in academic STEMM in areas such productivity, boundary setting and boundary control, networking and community building, burnout rates, and mental well-being. The excessive expectations of caregiving that often fall on the shoulders of women cut across career timeline and rank (e.g., graduate student, postdoctoral scholar, non-tenure-track and other contingent faculty, tenure-track faculty), institution type, and scientific discipline. Although there have been opportunities for innovation and some potential shifts in expectations, increased caregiving demands associated with the COVID-19 pandemic in 2020, such as remote working, school closures, and childcare and eldercare, had disproportionately negative outcomes for women.
The effects of the COVID-19 pandemic on women in STEMM during 2020 are understood better through an intentionally intersectional lens. Productivity, career, boundary setting, mental well-being, and health are all influenced by the ways in which social identities are defined and cultivated within social and power structures. Race and ethnicity, sexual orientation, gender identity, academic career stage, appointment type, institution type, age, and disability status, among many other factors, can amplify or diminish the effects of the COVID-19 pandemic for a given person. For example, non-cisgender women may be forced to return to home environments where their gender identity is not accepted, increasing their stress and isolation, and decreasing their well-being. Women of Color had a higher likelihood of facing a COVID-19–related death in their family compared with their white, non-Hispanic colleagues. The full extent of the effects of the COVID-19 pandemic for women of various social identities was not fully understood at the end of 2020.
Considering the relative paucity of women in many STEMM fields prior to the COVID-19 pandemic, women are more likely to experience academic isolation, including limited access to mentors, sponsors, and role models that share gender, racial, or ethnic identities. Combining this reality with the physical isolation stipulated by public health responses to the COVID-19 pandemic,
women in STEMM were subject to increasing isolation within their fields, networks, and communities. Explicit attention to the early indicators of how the COVID-19 pandemic affected women in academic STEMM careers during 2020, as well as attention to crisis responses throughout history, may provide opportunities to mitigate some of the long-term effects and potentially develop a more resilient and equitable academic STEMM system.
MAJOR FINDINGS
Given the ongoing nature of the COVID-19 pandemic, it was not possible to fully understand the entirety of the short- or long-term implications of this global disruption on the careers of women in academic STEMM. Having gathered preliminary data and evidence available in 2020, the committee found that significant changes to women’s work-life boundaries and divisions of labor, careers, productivity, advancement, mentoring and networking relationships, and mental health and well-being have been observed. The following findings represent those aspects that the committee agreed have been substantiated by the preliminary data, evidence, and information gathered by the end of 2020. They are presented either as Established Research and Experiences from Previous Events or Impacts of the COVID-19 Pandemic during 2020 that parallel the topics as presented in the report.
Established Research and Experiences from Previous Events
___________________
1 This finding is primarily based on research on cisgender women and men.
Impacts of the COVID-19 Pandemic during 2020
Research questions.
While this report compiled much of the research, data, and evidence available in 2020 on the effects of the COVID-19 pandemic, future research is still needed to understand all the potential effects, especially any long-term implications. The research questions represent areas the committee identified for future research, rather than specific recommendations. They are presented in six categories that parallel the chapters of the report: Cross-Cutting Themes; Academic Productivity and Institutional Responses; Work-Life Boundaries and Gendered Divisions of Labor; Collaboration, Networking, and Professional Societies; Academic Leadership and Decision-Making; and Mental Health and Well-being. The committee hopes the report will be used as a basis for continued understanding of the impact of the COVID-19 pandemic in its entirety and as a reference for mitigating impacts of future disruptions that affect women in academic STEMM. The committee also hopes that these research questions may enable academic STEMM to emerge from the pandemic era a stronger, more equitable place for women. Therefore, the committee identifies two types of research questions in each category; listed first are those questions aimed at understanding the impacts of the disruptions from the COVID-19 pandemic, followed by those questions exploring the opportunities to help support the full participation of women in the future.
Cross-Cutting Themes
- What are the short- and long-term effects of the COVID-19 pandemic on the career trajectories, job stability, and leadership roles of women, particularly of Black women and other Women of Color? How do these effects vary across institutional characteristics, 2 discipline, and career stage?
2 Institutional characteristics include different institutional types (e.g., research university, liberal arts college, community college), locales (e.g., urban, rural), missions (e.g., Historically Black Colleges and Universities, Hispanic-Serving Institutions, Asian American/Native American/Pacific Islander-Serving Institutions, Tribal Colleges and Universities), and levels of resources.
- How did the confluence of structural racism, economic hardships, and environmental disruptions affect Women of Color during the COVID-19 pandemic? Specifically, how did the murder of George Floyd, Breonna Taylor, and other Black citizens impact Black women academics’ safety, ability to be productive, and mental health?
- How has the inclusion of women in leadership and other roles in the academy influenced the ability of institutions to respond to the confluence of major social crises during the COVID-19 pandemic?
- How can institutions build on the involvement women had across STEMM disciplines during the COVID-19 pandemic to increase the participation of women in STEMM and/or elevate and support women in their current STEMM-related positions?
- How can institutions adapt, leverage, and learn from approaches developed during 2020 to attend to challenges experienced by Women of Color in STEMM in the future?
Academic Productivity and Institutional Responses
- How did the institutional responses (e.g., policies, practices) that were outlined in the Major Findings impact women faculty across institutional characteristics and disciplines?
- What are the short- and long-term effects of faculty evaluation practices and extension policies implemented during the COVID-19 pandemic on the productivity and career trajectories of members of the academic STEMM workforce by gender?
- What adaptations did women use during the transition to online and hybrid teaching modes? How did these techniques and adaptations vary as a function of career stage and institutional characteristics?
- What are examples of institutional changes implemented in response to the COVID-19 pandemic that have the potential to reduce systemic barriers to participation and advancement that have historically been faced by academic women in STEMM, specifically Women of Color and other marginalized women in STEMM? How might positive institutional responses be leveraged to create a more resilient and responsive higher education ecosystem?
- How can or should funding arrangements be altered (e.g., changes in funding for research and/or mentorship programs) to support new ways of interaction for women in STEMM during times of disruption, such as the COVID-19 pandemic?
Work-Life Boundaries and Gendered Divisions of Labor
- How do different social identities (e.g., racial; socioeconomic status; culturally, ethnically, sexually, or gender diverse; immigration status; parents of young children and other caregivers; women without partners) influence the management of work-nonwork boundaries? How did this change during the COVID-19 pandemic?
- How have COVID-19 pandemic-related disruptions affected progress toward reducing the gender gap in academic STEMM labor-force participation? How does this differ for Women of Color or women with caregiving responsibilities?
- How can institutions account for the unique challenges of women faculty with parenthood and caregiving responsibilities when developing effective and equitable policies, practices, or programs?
- How might insights gained about work-life boundaries during the COVID-19 pandemic inform how institutions develop and implement supportive resources (e.g., reductions in workload, on-site childcare, flexible working options)?
Collaboration, Networking, and Professional Societies
- What were the short- and long-term effects of the COVID-19 pandemic-prompted switch from in-person conferences to virtual conferences on conference culture and climate, especially for women in STEMM?
- How will the increase in virtual conferences specifically affect women’s advancement and career trajectories? How will it affect women’s collaborations?
- How has the shift away from attending conferences and in-person networking changed longer-term mentoring and sponsoring relationships, particularly in terms of gender dynamics?
- How can institutions maximize the benefits of digitization and the increased use of technology observed during the COVID-19 pandemic to continue supporting women, especially marginalized women, by increasing accessibility, collaborations, mentorship, and learning?
- How can organizations that support, host, or facilitate online and virtual conferences and networking events (1) ensure open and fair access to participants who face different funding and time constraints; (2) foster virtual connections among peers, mentors, and sponsors; and (3) maintain an inclusive environment to scientists of all backgrounds?
- What policies, practices, or programs can be developed to help women in STEMM maintain a sense of support, structure, and stability during and after periods of disruption?
Academic Leadership and Decision-Making
- What specific interventions did colleges and universities initiate or prioritize to ensure that women were included in decision-making processes during responses to the COVID-19 pandemic?
- How effective were colleges and universities that prioritized equity-minded leadership, shared leadership, and crisis leadership styles at mitigating emerging and potential negative effects of the COVID-19 pandemic on women in their communities?
- What specific aspects of different leadership models translated to more effective strategies to advance women in STEMM, particularly during the COVID-19 pandemic?
- How can examples of intentional inclusion of women in decision-making processes during the COVID-19 pandemic be leveraged to develop the engagement of women as leaders at all levels of academic institutions?
- What are potential “top-down” structural changes in academia that can be implemented to mitigate the adverse effects of the COVID-19 pandemic or other disruptions?
- How can academic leadership, at all levels, more effectively support the mental health needs of women in STEMM?
Mental Health and Well-being
- What is the impact of the COVID-19 pandemic and institutional responses on the mental health and well-being of members of the academic STEMM workforce as a function of gender, race, and career stage?
- How are tools and diagnostic tests to measure aspects of wellbeing, including burnout and insomnia, used in academic settings? How does this change during times of increased stress, such as the COVID-19 pandemic?
- How might insights gained about mental health during the COVID-19 pandemic be used to inform preparedness for future disruptions?
- How can programs that focus on changes in biomarkers of stress and mood dysregulation, such as levels of sleep, activity, and texting patterns, be developed and implemented to better engage women in addressing their mental health?
- What are effective interventions to address the health of women academics in STEMM that specifically account for the effects of stress on women? What are effective interventions to mitigate the excessive levels of stress for Women of Color?
This page intentionally left blank.
The spring of 2020 marked a change in how almost everyone conducted their personal and professional lives, both within science, technology, engineering, mathematics, and medicine (STEMM) and beyond. The COVID-19 pandemic disrupted global scientific conferences and individual laboratories and required people to find space in their homes from which to work. It blurred the boundaries between work and non-work, infusing ambiguity into everyday activities. While adaptations that allowed people to connect became more common, the evidence available at the end of 2020 suggests that the disruptions caused by the COVID-19 pandemic endangered the engagement, experience, and retention of women in academic STEMM, and may roll back some of the achievement gains made by women in the academy to date.
The Impact of COVID-19 on the Careers of Women in Academic Sciences, Engineering, and Medicine identifies, names, and documents how the COVID-19 pandemic disrupted the careers of women in academic STEMM during the initial 9-month period since March 2020 and considers how these disruptions - both positive and negative - might shape future progress for women. This publication builds on the 2020 report Promising Practices for Addressing the Underrepresentation of Women in Science, Engineering, and Medicine to develop a comprehensive understanding of the nuanced ways these disruptions have manifested. The Impact of COVID-19 on the Careers of Women in Academic Sciences, Engineering, and Medicine will inform the academic community as it emerges from the pandemic to mitigate any long-term negative consequences for the continued advancement of women in the academic STEMM workforce and build on the adaptations and opportunities that have emerged.
READ FREE ONLINE
Welcome to OpenBook!
You're looking at OpenBook, NAP.edu's online reading room since 1999. Based on feedback from you, our users, we've made some improvements that make it easier than ever to read thousands of publications on our website.
Do you want to take a quick tour of the OpenBook's features?
Show this book's table of contents , where you can jump to any chapter by name.
...or use these buttons to go back to the previous chapter or skip to the next one.
Jump up to the previous page or down to the next one. Also, you can type in a page number and press Enter to go directly to that page in the book.
Switch between the Original Pages , where you can read the report as it appeared in print, and Text Pages for the web version, where you can highlight and search the text.
To search the entire text of this book, type in your search term here and press Enter .
Share a link to this book page on your preferred social network or via email.
View our suggested citation for this chapter.
Ready to take your reading offline? Click here to buy this book in print or download it as a free PDF, if available.
Get Email Updates
Do you enjoy reading reports from the Academies online for free ? Sign up for email notifications and we'll let you know about new publications in your areas of interest when they're released.
Greater Good Science Center • Magazine • In Action • In Education
Society Articles & More
11 questions to ask about covid-19 research, how can you tell if a scientific study about the pandemic is valid and useful we have some tips..
Debates have raged on social media, around dinner tables, on TV, and in Congress about the science of COVID-19. Is it really worse than the flu? How necessary are lockdowns? Do masks work to prevent infection? What kinds of masks work best? Is the new vaccine safe?
You might see friends, relatives, and coworkers offer competing answers, often brandishing studies or citing individual doctors and scientists to support their positions. With so much disagreement—and with such high stakes—how can we use science to make the best decisions?
Here at Greater Good , we cover research into social and emotional well-being, and we try to help people apply findings to their personal and professional lives. We are well aware that our business is a tricky one.
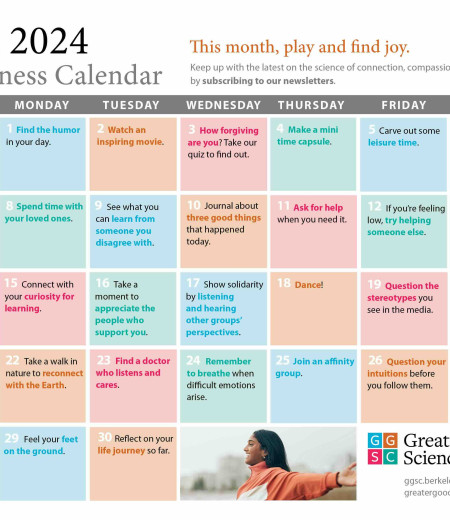
Summarizing scientific studies and distilling the key insights that people can apply to their lives isn’t just difficult for the obvious reasons, like understanding and then explaining formal science terms or rigorous empirical and analytic methods to non-specialists. It’s also the case that context gets lost when we translate findings into stories, tips, and tools, especially when we push it all through the nuance-squashing machine of the Internet. Many people rarely read past the headlines, which intrinsically aim to be relatable and provoke interest in as many people as possible. Because our articles can never be as comprehensive as the original studies, they almost always omit some crucial caveats, such as limitations acknowledged by the researchers. To get those, you need access to the studies themselves.
And it’s very common for findings and scientists to seem to contradict each other. For example, there were many contradictory findings and recommendations about the use of masks, especially at the beginning of the pandemic—though as we’ll discuss, it’s important to understand that a scientific consensus did emerge.
Given the complexities and ambiguities of the scientific endeavor, is it possible for a non-scientist to strike a balance between wholesale dismissal and uncritical belief? Are there red flags to look for when you read about a study on a site like Greater Good or hear about one on a Fox News program? If you do read an original source study, how should you, as a non-scientist, gauge its credibility?
Here are 11 questions you might ask when you read about the latest scientific findings about the pandemic, based on our own work here at Greater Good.
1. Did the study appear in a peer-reviewed journal?
In peer review, submitted articles are sent to other experts for detailed critical input that often must be addressed in a revision prior to being accepted and published. This remains one of the best ways we have for ascertaining the rigor of the study and rationale for its conclusions. Many scientists describe peer review as a truly humbling crucible. If a study didn’t go through this process, for whatever reason, it should be taken with a much bigger grain of salt.
“When thinking about the coronavirus studies, it is important to note that things were happening so fast that in the beginning people were releasing non-peer reviewed, observational studies,” says Dr. Leif Hass, a family medicine doctor and hospitalist at Sutter Health’s Alta Bates Summit Medical Center in Oakland, California. “This is what we typically do as hypothesis-generating but given the crisis, we started acting on them.”
In a confusing, time-pressed, fluid situation like the one COVID-19 presented, people without medical training have often been forced to simply defer to expertise in making individual and collective decisions, turning to culturally vetted institutions like the Centers for Disease Control (CDC). Is that wise? Read on.
2. Who conducted the study, and where did it appear?
“I try to listen to the opinion of people who are deep in the field being addressed and assess their response to the study at hand,” says Hass. “With the MRNA coronavirus vaccines, I heard Paul Offit from UPenn at a UCSF Grand Rounds talk about it. He literally wrote the book on vaccines. He reviewed what we know and gave the vaccine a big thumbs up. I was sold.”
From a scientific perspective, individual expertise and accomplishment matters—but so does institutional affiliation.
Why? Because institutions provide a framework for individual accountability as well as safety guidelines. At UC Berkeley, for example , research involving human subjects during COVID-19 must submit a Human Subjects Proposal Supplement Form , and follow a standard protocol and rigorous guidelines . Is this process perfect? No. It’s run by humans and humans are imperfect. However, the conclusions are far more reliable than opinions offered by someone’s favorite YouTuber .
Recommendations coming from institutions like the CDC should not be accepted uncritically. At the same time, however, all of us—including individuals sporting a “Ph.D.” or “M.D.” after their names—must be humble in the face of them. The CDC represents a formidable concentration of scientific talent and knowledge that dwarfs the perspective of any one individual. In a crisis like COVID-19, we need to defer to that expertise, at least conditionally.
“If we look at social media, things could look frightening,” says Hass. When hundreds of millions of people are vaccinated, millions of them will be afflicted anyway, in the course of life, by conditions like strokes, anaphylaxis, and Bell’s palsy. “We have to have faith that people collecting the data will let us know if we are seeing those things above the baseline rate.”
3. Who was studied, and where?
Animal experiments tell scientists a lot, but their applicability to our daily human lives will be limited. Similarly, if researchers only studied men, the conclusions might not be relevant to women, and vice versa.
Many psychology studies rely on WEIRD (Western, educated, industrialized, rich and democratic) participants, mainly college students, which creates an in-built bias in the discipline’s conclusions. Historically, biomedical studies also bias toward gathering measures from white male study participants, which again, limits generalizability of findings. Does that mean you should dismiss Western science? Of course not. It’s just the equivalent of a “Caution,” “Yield,” or “Roadwork Ahead” sign on the road to understanding.
This applies to the coronavirus vaccines now being distributed and administered around the world. The vaccines will have side effects; all medicines do. Those side effects will be worse for some people than others, depending on their genetic inheritance, medical status, age, upbringing, current living conditions, and other factors.
For Hass, it amounts to this question: Will those side effects be worse, on balance, than COVID-19, for most people?
“When I hear that four in 100,000 [of people in the vaccine trials] had Bell’s palsy, I know that it would have been a heck of a lot worse if 100,000 people had COVID. Three hundred people would have died and many others been stuck with chronic health problems.”
4. How big was the sample?
In general, the more participants in a study, the more valid its results. That said, a large sample is sometimes impossible or even undesirable for certain kinds of studies. During COVID-19, limited time has constrained the sample sizes.
However, that acknowledged, it’s still the case that some studies have been much larger than others—and the sample sizes of the vaccine trials can still provide us with enough information to make informed decisions. Doctors and nurses on the front lines of COVID-19—who are now the very first people being injected with the vaccine—think in terms of “biological plausibility,” as Hass says.
Did the admittedly rushed FDA approval of the Pfizer-BioNTech vaccine make sense, given what we already know? Tens of thousands of doctors who have been grappling with COVID-19 are voting with their arms, in effect volunteering to be a sample for their patients. If they didn’t think the vaccine was safe, you can bet they’d resist it. When the vaccine becomes available to ordinary people, we’ll know a lot more about its effects than we do today, thanks to health care providers paving the way.
5. Did the researchers control for key differences, and do those differences apply to you?
Diversity or gender balance aren’t necessarily virtues in experimental research, though ideally a study sample is as representative of the overall population as possible. However, many studies use intentionally homogenous groups, because this allows the researchers to limit the number of different factors that might affect the result.
While good researchers try to compare apples to apples, and control for as many differences as possible in their analyses, running a study always involves trade-offs between what can be accomplished as a function of study design, and how generalizable the findings can be.

The Science of Happiness
What does it take to live a happier life? Learn research-tested strategies that you can put into practice today. Hosted by award-winning psychologist Dacher Keltner. Co-produced by PRX and UC Berkeley’s Greater Good Science Center.
- Apple Podcasts
- Google Podcasts
You also need to ask if the specific population studied even applies to you. For example, when one study found that cloth masks didn’t work in “high-risk situations,” it was sometimes used as evidence against mask mandates.
However, a look beyond the headlines revealed that the study was of health care workers treating COVID-19 patients, which is a vastly more dangerous situation than, say, going to the grocery store. Doctors who must intubate patients can end up being splattered with saliva. In that circumstance, one cloth mask won’t cut it. They also need an N95, a face shield, two layers of gloves, and two layers of gown. For the rest of us in ordinary life, masks do greatly reduce community spread, if as many people as possible are wearing them.
6. Was there a control group?
One of the first things to look for in methodology is whether the population tested was randomly selected, whether there was a control group, and whether people were randomly assigned to either group without knowing which one they were in. This is especially important if a study aims to suggest that a certain experience or treatment might actually cause a specific outcome, rather than just reporting a correlation between two variables (see next point).
For example, were some people randomly assigned a specific meditation practice while others engaged in a comparable activity or exercise? If the sample is large enough, randomized trials can produce solid conclusions. But, sometimes, a study will not have a control group because it’s ethically impossible. We can’t, for example, let sick people go untreated just to see what would happen. Biomedical research often makes use of standard “treatment as usual” or placebos in control groups. They also follow careful ethical guidelines to protect patients from both maltreatment and being deprived necessary treatment. When you’re reading about studies of masks, social distancing, and treatments during the COVID-19, you can partially gauge the reliability and validity of the study by first checking if it had a control group. If it didn’t, the findings should be taken as preliminary.
7. Did the researchers establish causality, correlation, dependence, or some other kind of relationship?
We often hear “Correlation is not causation” shouted as a kind of battle cry, to try to discredit a study. But correlation—the degree to which two or more measurements seem connected—is important, and can be a step toward eventually finding causation—that is, establishing a change in one variable directly triggers a change in another. Until then, however, there is no way to ascertain the direction of a correlational relationship (does A change B, or does B change A), or to eliminate the possibility that a third, unmeasured factor is behind the pattern of both variables without further analysis.
In the end, the important thing is to accurately identify the relationship. This has been crucial in understanding steps to counter the spread of COVID-19 like shelter-in-place orders. Just showing that greater compliance with shelter-in-place mandates was associated with lower hospitalization rates is not as conclusive as showing that one community that enacted shelter-in-place mandates had lower hospitalization rates than a different community of similar size and population density that elected not to do so.
We are not the first people to face an infection without understanding the relationships between factors that would lead to more of it. During the bubonic plague, cities would order rodents killed to control infection. They were onto something: Fleas that lived on rodents were indeed responsible. But then human cases would skyrocket.
Why? Because the fleas would migrate off the rodent corpses onto humans, which would worsen infection. Rodent control only reduces bubonic plague if it’s done proactively; once the outbreak starts, killing rats can actually make it worse. Similarly, we can’t jump to conclusions during the COVID-19 pandemic when we see correlations.
8. Are journalists and politicians, or even scientists, overstating the result?
Language that suggests a fact is “proven” by one study or which promotes one solution for all people is most likely overstating the case. Sweeping generalizations of any kind often indicate a lack of humility that should be a red flag to readers. A study may very well “suggest” a certain conclusion but it rarely, if ever, “proves” it.
This is why we use a lot of cautious, hedging language in Greater Good , like “might” or “implies.” This applies to COVID-19 as well. In fact, this understanding could save your life.
When President Trump touted the advantages of hydroxychloroquine as a way to prevent and treat COVID-19, he was dramatically overstating the results of one observational study. Later studies with control groups showed that it did not work—and, in fact, it didn’t work as a preventative for President Trump and others in the White House who contracted COVID-19. Most survived that outbreak, but hydroxychloroquine was not one of the treatments that saved their lives. This example demonstrates how misleading and even harmful overstated results can be, in a global pandemic.
9. Is there any conflict of interest suggested by the funding or the researchers’ affiliations?
A 2015 study found that you could drink lots of sugary beverages without fear of getting fat, as long as you exercised. The funder? Coca Cola, which eagerly promoted the results. This doesn’t mean the results are wrong. But it does suggest you should seek a second opinion : Has anyone else studied the effects of sugary drinks on obesity? What did they find?
It’s possible to take this insight too far. Conspiracy theorists have suggested that “Big Pharma” invented COVID-19 for the purpose of selling vaccines. Thus, we should not trust their own trials showing that the vaccine is safe and effective.
But, in addition to the fact that there is no compelling investigative evidence that pharmaceutical companies created the virus, we need to bear in mind that their trials didn’t unfold in a vacuum. Clinical trials were rigorously monitored and independently reviewed by third-party entities like the World Health Organization and government organizations around the world, like the FDA in the United States.
Does that completely eliminate any risk? Absolutely not. It does mean, however, that conflicts of interest are being very closely monitored by many, many expert eyes. This greatly reduces the probability and potential corruptive influence of conflicts of interest.
10. Do the authors reference preceding findings and original sources?
The scientific method is based on iterative progress, and grounded in coordinating discoveries over time. Researchers study what others have done and use prior findings to guide their own study approaches; every study builds on generations of precedent, and every scientist expects their own discoveries to be usurped by more sophisticated future work. In the study you are reading, do the researchers adequately describe and acknowledge earlier findings, or other key contributions from other fields or disciplines that inform aspects of the research, or the way that they interpret their results?
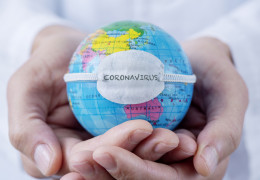
Greater Good’s Guide to Well-Being During Coronavirus
Practices, resources, and articles for individuals, parents, and educators facing COVID-19
This was crucial for the debates that have raged around mask mandates and social distancing. We already knew quite a bit about the efficacy of both in preventing infections, informed by centuries of practical experience and research.
When COVID-19 hit American shores, researchers and doctors did not question the necessity of masks in clinical settings. Here’s what we didn’t know: What kinds of masks would work best for the general public, who should wear them, when should we wear them, were there enough masks to go around, and could we get enough people to adopt best mask practices to make a difference in the specific context of COVID-19 ?
Over time, after a period of confusion and contradictory evidence, those questions have been answered . The very few studies that have suggested masks don’t work in stopping COVID-19 have almost all failed to account for other work on preventing the disease, and had results that simply didn’t hold up. Some were even retracted .
So, when someone shares a coronavirus study with you, it’s important to check the date. The implications of studies published early in the pandemic might be more limited and less conclusive than those published later, because the later studies could lean on and learn from previously published work. Which leads us to the next question you should ask in hearing about coronavirus research…
11. Do researchers, journalists, and politicians acknowledge limitations and entertain alternative explanations?
Is the study focused on only one side of the story or one interpretation of the data? Has it failed to consider or refute alternative explanations? Do they demonstrate awareness of which questions are answered and which aren’t by their methods? Do the journalists and politicians communicating the study know and understand these limitations?
When the Annals of Internal Medicine published a Danish study last month on the efficacy of cloth masks, some suggested that it showed masks “make no difference” against COVID-19.
The study was a good one by the standards spelled out in this article. The researchers and the journal were both credible, the study was randomized and controlled, and the sample size (4,862 people) was fairly large. Even better, the scientists went out of their way to acknowledge the limits of their work: “Inconclusive results, missing data, variable adherence, patient-reported findings on home tests, no blinding, and no assessment of whether masks could decrease disease transmission from mask wearers to others.”
Unfortunately, their scientific integrity was not reflected in the ways the study was used by some journalists, politicians, and people on social media. The study did not show that masks were useless. What it did show—and what it was designed to find out—was how much protection masks offered to the wearer under the conditions at the time in Denmark. In fact, the amount of protection for the wearer was not large, but that’s not the whole picture: We don’t wear masks mainly to protect ourselves, but to protect others from infection. Public-health recommendations have stressed that everyone needs to wear a mask to slow the spread of infection.
“We get vaccinated for the greater good, not just to protect ourselves ”
As the authors write in the paper, we need to look to other research to understand the context for their narrow results. In an editorial accompanying the paper in Annals of Internal Medicine , the editors argue that the results, together with existing data in support of masks, “should motivate widespread mask wearing to protect our communities and thereby ourselves.”
Something similar can be said of the new vaccine. “We get vaccinated for the greater good, not just to protect ourselves,” says Hass. “Being vaccinated prevents other people from getting sick. We get vaccinated for the more vulnerable in our community in addition for ourselves.”
Ultimately, the approach we should take to all new studies is a curious but skeptical one. We should take it all seriously and we should take it all with a grain of salt. You can judge a study against your experience, but you need to remember that your experience creates bias. You should try to cultivate humility, doubt, and patience. You might not always succeed; when you fail, try to admit fault and forgive yourself.
Above all, we need to try to remember that science is a process, and that conclusions always raise more questions for us to answer. That doesn’t mean we never have answers; we do. As the pandemic rages and the scientific process unfolds, we as individuals need to make the best decisions we can, with the information we have.
This article was revised and updated from a piece published by Greater Good in 2015, “ 10 Questions to Ask About Scientific Studies .”
About the Authors

Jeremy Adam Smith
Uc berkeley.
Jeremy Adam Smith edits the GGSC’s online magazine, Greater Good . He is also the author or coeditor of five books, including The Daddy Shift , Are We Born Racist? , and (most recently) The Gratitude Project: How the Science of Thankfulness Can Rewire Our Brains for Resilience, Optimism, and the Greater Good . Before joining the GGSC, Jeremy was a John S. Knight Journalism Fellow at Stanford University.

Emiliana R. Simon-Thomas
Emiliana R. Simon-Thomas, Ph.D. , is the science director of the Greater Good Science Center, where she directs the GGSC’s research fellowship program and serves as a co-instructor of its Science of Happiness and Science of Happiness at Work online courses.
You May Also Enjoy
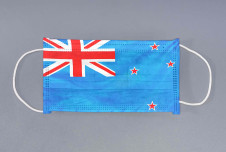
This article — and everything on this site — is funded by readers like you.
Become a subscribing member today. Help us continue to bring “the science of a meaningful life” to you and to millions around the globe.
- Research article
- Open access
- Published: 04 June 2021
Coronavirus disease (COVID-19) pandemic: an overview of systematic reviews
- Israel Júnior Borges do Nascimento 1 , 2 ,
- Dónal P. O’Mathúna 3 , 4 ,
- Thilo Caspar von Groote 5 ,
- Hebatullah Mohamed Abdulazeem 6 ,
- Ishanka Weerasekara 7 , 8 ,
- Ana Marusic 9 ,
- Livia Puljak ORCID: orcid.org/0000-0002-8467-6061 10 ,
- Vinicius Tassoni Civile 11 ,
- Irena Zakarija-Grkovic 9 ,
- Tina Poklepovic Pericic 9 ,
- Alvaro Nagib Atallah 11 ,
- Santino Filoso 12 ,
- Nicola Luigi Bragazzi 13 &
- Milena Soriano Marcolino 1
On behalf of the International Network of Coronavirus Disease 2019 (InterNetCOVID-19)
BMC Infectious Diseases volume 21 , Article number: 525 ( 2021 ) Cite this article
16k Accesses
28 Citations
13 Altmetric
Metrics details
Navigating the rapidly growing body of scientific literature on the SARS-CoV-2 pandemic is challenging, and ongoing critical appraisal of this output is essential. We aimed to summarize and critically appraise systematic reviews of coronavirus disease (COVID-19) in humans that were available at the beginning of the pandemic.
Nine databases (Medline, EMBASE, Cochrane Library, CINAHL, Web of Sciences, PDQ-Evidence, WHO’s Global Research, LILACS, and Epistemonikos) were searched from December 1, 2019, to March 24, 2020. Systematic reviews analyzing primary studies of COVID-19 were included. Two authors independently undertook screening, selection, extraction (data on clinical symptoms, prevalence, pharmacological and non-pharmacological interventions, diagnostic test assessment, laboratory, and radiological findings), and quality assessment (AMSTAR 2). A meta-analysis was performed of the prevalence of clinical outcomes.
Eighteen systematic reviews were included; one was empty (did not identify any relevant study). Using AMSTAR 2, confidence in the results of all 18 reviews was rated as “critically low”. Identified symptoms of COVID-19 were (range values of point estimates): fever (82–95%), cough with or without sputum (58–72%), dyspnea (26–59%), myalgia or muscle fatigue (29–51%), sore throat (10–13%), headache (8–12%) and gastrointestinal complaints (5–9%). Severe symptoms were more common in men. Elevated C-reactive protein and lactate dehydrogenase, and slightly elevated aspartate and alanine aminotransferase, were commonly described. Thrombocytopenia and elevated levels of procalcitonin and cardiac troponin I were associated with severe disease. A frequent finding on chest imaging was uni- or bilateral multilobar ground-glass opacity. A single review investigated the impact of medication (chloroquine) but found no verifiable clinical data. All-cause mortality ranged from 0.3 to 13.9%.
Conclusions
In this overview of systematic reviews, we analyzed evidence from the first 18 systematic reviews that were published after the emergence of COVID-19. However, confidence in the results of all reviews was “critically low”. Thus, systematic reviews that were published early on in the pandemic were of questionable usefulness. Even during public health emergencies, studies and systematic reviews should adhere to established methodological standards.
Peer Review reports
The spread of the “Severe Acute Respiratory Coronavirus 2” (SARS-CoV-2), the causal agent of COVID-19, was characterized as a pandemic by the World Health Organization (WHO) in March 2020 and has triggered an international public health emergency [ 1 ]. The numbers of confirmed cases and deaths due to COVID-19 are rapidly escalating, counting in millions [ 2 ], causing massive economic strain, and escalating healthcare and public health expenses [ 3 , 4 ].
The research community has responded by publishing an impressive number of scientific reports related to COVID-19. The world was alerted to the new disease at the beginning of 2020 [ 1 ], and by mid-March 2020, more than 2000 articles had been published on COVID-19 in scholarly journals, with 25% of them containing original data [ 5 ]. The living map of COVID-19 evidence, curated by the Evidence for Policy and Practice Information and Co-ordinating Centre (EPPI-Centre), contained more than 40,000 records by February 2021 [ 6 ]. More than 100,000 records on PubMed were labeled as “SARS-CoV-2 literature, sequence, and clinical content” by February 2021 [ 7 ].
Due to publication speed, the research community has voiced concerns regarding the quality and reproducibility of evidence produced during the COVID-19 pandemic, warning of the potential damaging approach of “publish first, retract later” [ 8 ]. It appears that these concerns are not unfounded, as it has been reported that COVID-19 articles were overrepresented in the pool of retracted articles in 2020 [ 9 ]. These concerns about inadequate evidence are of major importance because they can lead to poor clinical practice and inappropriate policies [ 10 ].
Systematic reviews are a cornerstone of today’s evidence-informed decision-making. By synthesizing all relevant evidence regarding a particular topic, systematic reviews reflect the current scientific knowledge. Systematic reviews are considered to be at the highest level in the hierarchy of evidence and should be used to make informed decisions. However, with high numbers of systematic reviews of different scope and methodological quality being published, overviews of multiple systematic reviews that assess their methodological quality are essential [ 11 , 12 , 13 ]. An overview of systematic reviews helps identify and organize the literature and highlights areas of priority in decision-making.
In this overview of systematic reviews, we aimed to summarize and critically appraise systematic reviews of coronavirus disease (COVID-19) in humans that were available at the beginning of the pandemic.
Methodology
Research question.
This overview’s primary objective was to summarize and critically appraise systematic reviews that assessed any type of primary clinical data from patients infected with SARS-CoV-2. Our research question was purposefully broad because we wanted to analyze as many systematic reviews as possible that were available early following the COVID-19 outbreak.
Study design
We conducted an overview of systematic reviews. The idea for this overview originated in a protocol for a systematic review submitted to PROSPERO (CRD42020170623), which indicated a plan to conduct an overview.
Overviews of systematic reviews use explicit and systematic methods for searching and identifying multiple systematic reviews addressing related research questions in the same field to extract and analyze evidence across important outcomes. Overviews of systematic reviews are in principle similar to systematic reviews of interventions, but the unit of analysis is a systematic review [ 14 , 15 , 16 ].
We used the overview methodology instead of other evidence synthesis methods to allow us to collate and appraise multiple systematic reviews on this topic, and to extract and analyze their results across relevant topics [ 17 ]. The overview and meta-analysis of systematic reviews allowed us to investigate the methodological quality of included studies, summarize results, and identify specific areas of available or limited evidence, thereby strengthening the current understanding of this novel disease and guiding future research [ 13 ].
A reporting guideline for overviews of reviews is currently under development, i.e., Preferred Reporting Items for Overviews of Reviews (PRIOR) [ 18 ]. As the PRIOR checklist is still not published, this study was reported following the Preferred Reporting Items for Systematic Reviews and Meta-Analyses (PRISMA) 2009 statement [ 19 ]. The methodology used in this review was adapted from the Cochrane Handbook for Systematic Reviews of Interventions and also followed established methodological considerations for analyzing existing systematic reviews [ 14 ].
Approval of a research ethics committee was not necessary as the study analyzed only publicly available articles.
Eligibility criteria
Systematic reviews were included if they analyzed primary data from patients infected with SARS-CoV-2 as confirmed by RT-PCR or another pre-specified diagnostic technique. Eligible reviews covered all topics related to COVID-19 including, but not limited to, those that reported clinical symptoms, diagnostic methods, therapeutic interventions, laboratory findings, or radiological results. Both full manuscripts and abbreviated versions, such as letters, were eligible.
No restrictions were imposed on the design of the primary studies included within the systematic reviews, the last search date, whether the review included meta-analyses or language. Reviews related to SARS-CoV-2 and other coronaviruses were eligible, but from those reviews, we analyzed only data related to SARS-CoV-2.
No consensus definition exists for a systematic review [ 20 ], and debates continue about the defining characteristics of a systematic review [ 21 ]. Cochrane’s guidance for overviews of reviews recommends setting pre-established criteria for making decisions around inclusion [ 14 ]. That is supported by a recent scoping review about guidance for overviews of systematic reviews [ 22 ].
Thus, for this study, we defined a systematic review as a research report which searched for primary research studies on a specific topic using an explicit search strategy, had a detailed description of the methods with explicit inclusion criteria provided, and provided a summary of the included studies either in narrative or quantitative format (such as a meta-analysis). Cochrane and non-Cochrane systematic reviews were considered eligible for inclusion, with or without meta-analysis, and regardless of the study design, language restriction and methodology of the included primary studies. To be eligible for inclusion, reviews had to be clearly analyzing data related to SARS-CoV-2 (associated or not with other viruses). We excluded narrative reviews without those characteristics as these are less likely to be replicable and are more prone to bias.
Scoping reviews and rapid reviews were eligible for inclusion in this overview if they met our pre-defined inclusion criteria noted above. We included reviews that addressed SARS-CoV-2 and other coronaviruses if they reported separate data regarding SARS-CoV-2.
Information sources
Nine databases were searched for eligible records published between December 1, 2019, and March 24, 2020: Cochrane Database of Systematic Reviews via Cochrane Library, PubMed, EMBASE, CINAHL (Cumulative Index to Nursing and Allied Health Literature), Web of Sciences, LILACS (Latin American and Caribbean Health Sciences Literature), PDQ-Evidence, WHO’s Global Research on Coronavirus Disease (COVID-19), and Epistemonikos.
The comprehensive search strategy for each database is provided in Additional file 1 and was designed and conducted in collaboration with an information specialist. All retrieved records were primarily processed in EndNote, where duplicates were removed, and records were then imported into the Covidence platform [ 23 ]. In addition to database searches, we screened reference lists of reviews included after screening records retrieved via databases.
Study selection
All searches, screening of titles and abstracts, and record selection, were performed independently by two investigators using the Covidence platform [ 23 ]. Articles deemed potentially eligible were retrieved for full-text screening carried out independently by two investigators. Discrepancies at all stages were resolved by consensus. During the screening, records published in languages other than English were translated by a native/fluent speaker.
Data collection process
We custom designed a data extraction table for this study, which was piloted by two authors independently. Data extraction was performed independently by two authors. Conflicts were resolved by consensus or by consulting a third researcher.
We extracted the following data: article identification data (authors’ name and journal of publication), search period, number of databases searched, population or settings considered, main results and outcomes observed, and number of participants. From Web of Science (Clarivate Analytics, Philadelphia, PA, USA), we extracted journal rank (quartile) and Journal Impact Factor (JIF).
We categorized the following as primary outcomes: all-cause mortality, need for and length of mechanical ventilation, length of hospitalization (in days), admission to intensive care unit (yes/no), and length of stay in the intensive care unit.
The following outcomes were categorized as exploratory: diagnostic methods used for detection of the virus, male to female ratio, clinical symptoms, pharmacological and non-pharmacological interventions, laboratory findings (full blood count, liver enzymes, C-reactive protein, d-dimer, albumin, lipid profile, serum electrolytes, blood vitamin levels, glucose levels, and any other important biomarkers), and radiological findings (using radiography, computed tomography, magnetic resonance imaging or ultrasound).
We also collected data on reporting guidelines and requirements for the publication of systematic reviews and meta-analyses from journal websites where included reviews were published.
Quality assessment in individual reviews
Two researchers independently assessed the reviews’ quality using the “A MeaSurement Tool to Assess Systematic Reviews 2 (AMSTAR 2)”. We acknowledge that the AMSTAR 2 was created as “a critical appraisal tool for systematic reviews that include randomized or non-randomized studies of healthcare interventions, or both” [ 24 ]. However, since AMSTAR 2 was designed for systematic reviews of intervention trials, and we included additional types of systematic reviews, we adjusted some AMSTAR 2 ratings and reported these in Additional file 2 .
Adherence to each item was rated as follows: yes, partial yes, no, or not applicable (such as when a meta-analysis was not conducted). The overall confidence in the results of the review is rated as “critically low”, “low”, “moderate” or “high”, according to the AMSTAR 2 guidance based on seven critical domains, which are items 2, 4, 7, 9, 11, 13, 15 as defined by AMSTAR 2 authors [ 24 ]. We reported our adherence ratings for transparency of our decision with accompanying explanations, for each item, in each included review.
One of the included systematic reviews was conducted by some members of this author team [ 25 ]. This review was initially assessed independently by two authors who were not co-authors of that review to prevent the risk of bias in assessing this study.
Synthesis of results
For data synthesis, we prepared a table summarizing each systematic review. Graphs illustrating the mortality rate and clinical symptoms were created. We then prepared a narrative summary of the methods, findings, study strengths, and limitations.
For analysis of the prevalence of clinical outcomes, we extracted data on the number of events and the total number of patients to perform proportional meta-analysis using RStudio© software, with the “meta” package (version 4.9–6), using the “metaprop” function for reviews that did not perform a meta-analysis, excluding case studies because of the absence of variance. For reviews that did not perform a meta-analysis, we presented pooled results of proportions with their respective confidence intervals (95%) by the inverse variance method with a random-effects model, using the DerSimonian-Laird estimator for τ 2 . We adjusted data using Freeman-Tukey double arcosen transformation. Confidence intervals were calculated using the Clopper-Pearson method for individual studies. We created forest plots using the RStudio© software, with the “metafor” package (version 2.1–0) and “forest” function.
Managing overlapping systematic reviews
Some of the included systematic reviews that address the same or similar research questions may include the same primary studies in overviews. Including such overlapping reviews may introduce bias when outcome data from the same primary study are included in the analyses of an overview multiple times. Thus, in summaries of evidence, multiple-counting of the same outcome data will give data from some primary studies too much influence [ 14 ]. In this overview, we did not exclude overlapping systematic reviews because, according to Cochrane’s guidance, it may be appropriate to include all relevant reviews’ results if the purpose of the overview is to present and describe the current body of evidence on a topic [ 14 ]. To avoid any bias in summary estimates associated with overlapping reviews, we generated forest plots showing data from individual systematic reviews, but the results were not pooled because some primary studies were included in multiple reviews.
Our search retrieved 1063 publications, of which 175 were duplicates. Most publications were excluded after the title and abstract analysis ( n = 860). Among the 28 studies selected for full-text screening, 10 were excluded for the reasons described in Additional file 3 , and 18 were included in the final analysis (Fig. 1 ) [ 25 , 26 , 27 , 28 , 29 , 30 , 31 , 32 , 33 , 34 , 35 , 36 , 37 , 38 , 39 , 40 , 41 , 42 ]. Reference list screening did not retrieve any additional systematic reviews.
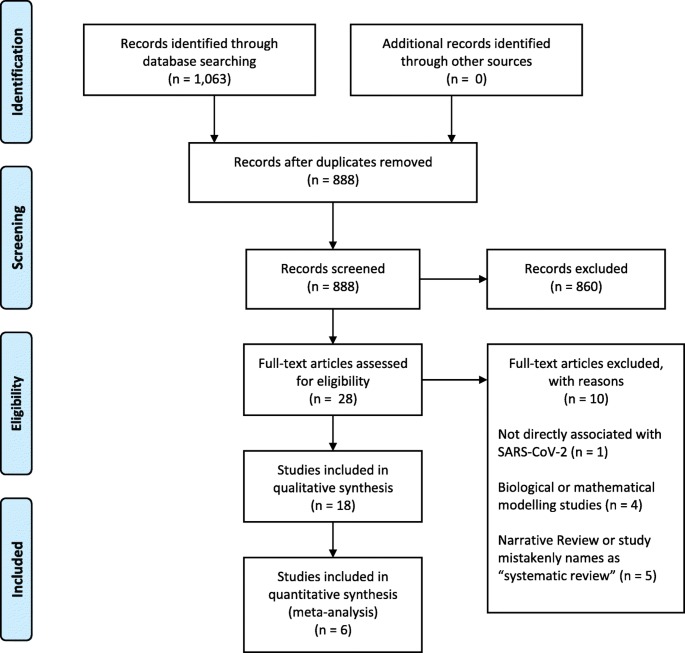
PRISMA flow diagram
Characteristics of included reviews
Summary features of 18 systematic reviews are presented in Table 1 . They were published in 14 different journals. Only four of these journals had specific requirements for systematic reviews (with or without meta-analysis): European Journal of Internal Medicine, Journal of Clinical Medicine, Ultrasound in Obstetrics and Gynecology, and Clinical Research in Cardiology . Two journals reported that they published only invited reviews ( Journal of Medical Virology and Clinica Chimica Acta ). Three systematic reviews in our study were published as letters; one was labeled as a scoping review and another as a rapid review (Table 2 ).
All reviews were published in English, in first quartile (Q1) journals, with JIF ranging from 1.692 to 6.062. One review was empty, meaning that its search did not identify any relevant studies; i.e., no primary studies were included [ 36 ]. The remaining 17 reviews included 269 unique studies; the majority ( N = 211; 78%) were included in only a single review included in our study (range: 1 to 12). Primary studies included in the reviews were published between December 2019 and March 18, 2020, and comprised case reports, case series, cohorts, and other observational studies. We found only one review that included randomized clinical trials [ 38 ]. In the included reviews, systematic literature searches were performed from 2019 (entire year) up to March 9, 2020. Ten systematic reviews included meta-analyses. The list of primary studies found in the included systematic reviews is shown in Additional file 4 , as well as the number of reviews in which each primary study was included.
Population and study designs
Most of the reviews analyzed data from patients with COVID-19 who developed pneumonia, acute respiratory distress syndrome (ARDS), or any other correlated complication. One review aimed to evaluate the effectiveness of using surgical masks on preventing transmission of the virus [ 36 ], one review was focused on pediatric patients [ 34 ], and one review investigated COVID-19 in pregnant women [ 37 ]. Most reviews assessed clinical symptoms, laboratory findings, or radiological results.
Systematic review findings
The summary of findings from individual reviews is shown in Table 2 . Overall, all-cause mortality ranged from 0.3 to 13.9% (Fig. 2 ).
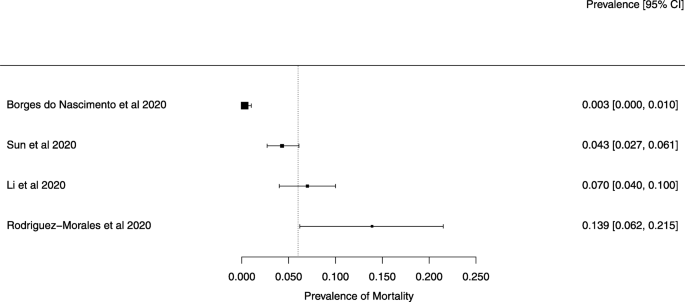
A meta-analysis of the prevalence of mortality
Clinical symptoms
Seven reviews described the main clinical manifestations of COVID-19 [ 26 , 28 , 29 , 34 , 35 , 39 , 41 ]. Three of them provided only a narrative discussion of symptoms [ 26 , 34 , 35 ]. In the reviews that performed a statistical analysis of the incidence of different clinical symptoms, symptoms in patients with COVID-19 were (range values of point estimates): fever (82–95%), cough with or without sputum (58–72%), dyspnea (26–59%), myalgia or muscle fatigue (29–51%), sore throat (10–13%), headache (8–12%), gastrointestinal disorders, such as diarrhea, nausea or vomiting (5.0–9.0%), and others (including, in one study only: dizziness 12.1%) (Figs. 3 , 4 , 5 , 6 , 7 , 8 and 9 ). Three reviews assessed cough with and without sputum together; only one review assessed sputum production itself (28.5%).
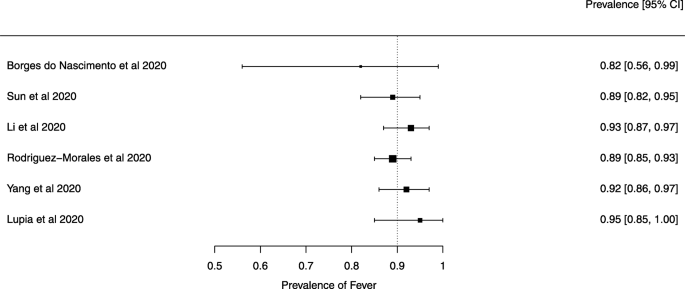
A meta-analysis of the prevalence of fever
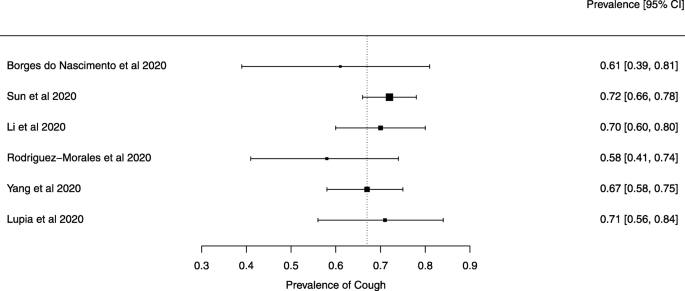
A meta-analysis of the prevalence of cough
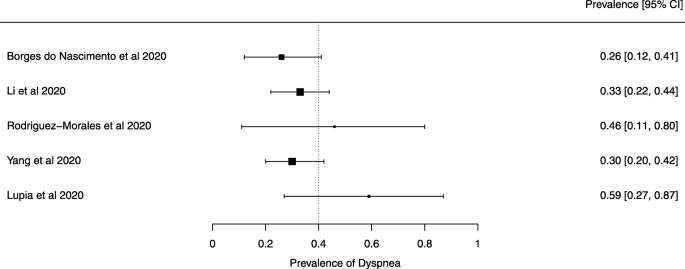
A meta-analysis of the prevalence of dyspnea
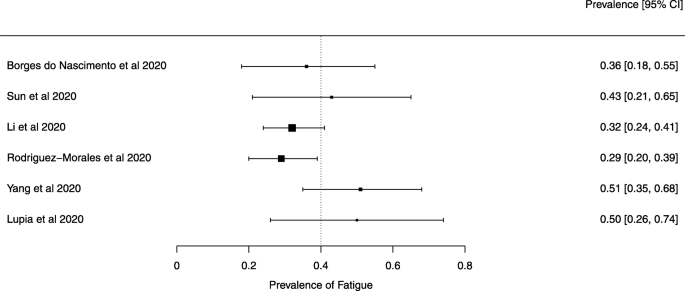
A meta-analysis of the prevalence of fatigue or myalgia
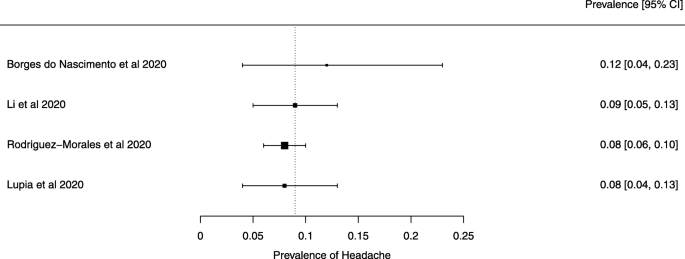
A meta-analysis of the prevalence of headache
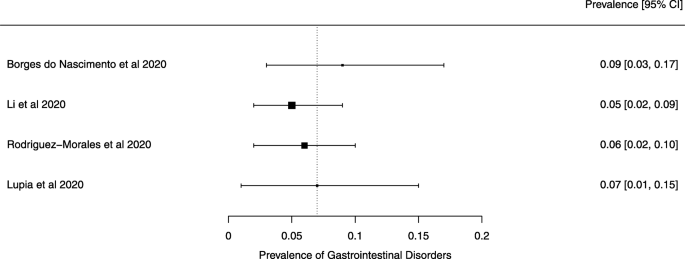
A meta-analysis of the prevalence of gastrointestinal disorders
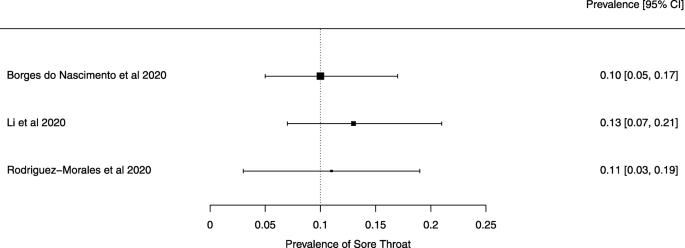
A meta-analysis of the prevalence of sore throat
Diagnostic aspects
Three reviews described methodologies, protocols, and tools used for establishing the diagnosis of COVID-19 [ 26 , 34 , 38 ]. The use of respiratory swabs (nasal or pharyngeal) or blood specimens to assess the presence of SARS-CoV-2 nucleic acid using RT-PCR assays was the most commonly used diagnostic method mentioned in the included studies. These diagnostic tests have been widely used, but their precise sensitivity and specificity remain unknown. One review included a Chinese study with clinical diagnosis with no confirmation of SARS-CoV-2 infection (patients were diagnosed with COVID-19 if they presented with at least two symptoms suggestive of COVID-19, together with laboratory and chest radiography abnormalities) [ 34 ].
Therapeutic possibilities
Pharmacological and non-pharmacological interventions (supportive therapies) used in treating patients with COVID-19 were reported in five reviews [ 25 , 27 , 34 , 35 , 38 ]. Antivirals used empirically for COVID-19 treatment were reported in seven reviews [ 25 , 27 , 34 , 35 , 37 , 38 , 41 ]; most commonly used were protease inhibitors (lopinavir, ritonavir, darunavir), nucleoside reverse transcriptase inhibitor (tenofovir), nucleotide analogs (remdesivir, galidesivir, ganciclovir), and neuraminidase inhibitors (oseltamivir). Umifenovir, a membrane fusion inhibitor, was investigated in two studies [ 25 , 35 ]. Possible supportive interventions analyzed were different types of oxygen supplementation and breathing support (invasive or non-invasive ventilation) [ 25 ]. The use of antibiotics, both empirically and to treat secondary pneumonia, was reported in six studies [ 25 , 26 , 27 , 34 , 35 , 38 ]. One review specifically assessed evidence on the efficacy and safety of the anti-malaria drug chloroquine [ 27 ]. It identified 23 ongoing trials investigating the potential of chloroquine as a therapeutic option for COVID-19, but no verifiable clinical outcomes data. The use of mesenchymal stem cells, antifungals, and glucocorticoids were described in four reviews [ 25 , 34 , 35 , 38 ].
Laboratory and radiological findings
Of the 18 reviews included in this overview, eight analyzed laboratory parameters in patients with COVID-19 [ 25 , 29 , 30 , 32 , 33 , 34 , 35 , 39 ]; elevated C-reactive protein levels, associated with lymphocytopenia, elevated lactate dehydrogenase, as well as slightly elevated aspartate and alanine aminotransferase (AST, ALT) were commonly described in those eight reviews. Lippi et al. assessed cardiac troponin I (cTnI) [ 25 ], procalcitonin [ 32 ], and platelet count [ 33 ] in COVID-19 patients. Elevated levels of procalcitonin [ 32 ] and cTnI [ 30 ] were more likely to be associated with a severe disease course (requiring intensive care unit admission and intubation). Furthermore, thrombocytopenia was frequently observed in patients with complicated COVID-19 infections [ 33 ].
Chest imaging (chest radiography and/or computed tomography) features were assessed in six reviews, all of which described a frequent pattern of local or bilateral multilobar ground-glass opacity [ 25 , 34 , 35 , 39 , 40 , 41 ]. Those six reviews showed that septal thickening, bronchiectasis, pleural and cardiac effusions, halo signs, and pneumothorax were observed in patients suffering from COVID-19.
Quality of evidence in individual systematic reviews
Table 3 shows the detailed results of the quality assessment of 18 systematic reviews, including the assessment of individual items and summary assessment. A detailed explanation for each decision in each review is available in Additional file 5 .
Using AMSTAR 2 criteria, confidence in the results of all 18 reviews was rated as “critically low” (Table 3 ). Common methodological drawbacks were: omission of prospective protocol submission or publication; use of inappropriate search strategy: lack of independent and dual literature screening and data-extraction (or methodology unclear); absence of an explanation for heterogeneity among the studies included; lack of reasons for study exclusion (or rationale unclear).
Risk of bias assessment, based on a reported methodological tool, and quality of evidence appraisal, in line with the Grading of Recommendations Assessment, Development, and Evaluation (GRADE) method, were reported only in one review [ 25 ]. Five reviews presented a table summarizing bias, using various risk of bias tools [ 25 , 29 , 39 , 40 , 41 ]. One review analyzed “study quality” [ 37 ]. One review mentioned the risk of bias assessment in the methodology but did not provide any related analysis [ 28 ].
This overview of systematic reviews analyzed the first 18 systematic reviews published after the onset of the COVID-19 pandemic, up to March 24, 2020, with primary studies involving more than 60,000 patients. Using AMSTAR-2, we judged that our confidence in all those reviews was “critically low”. Ten reviews included meta-analyses. The reviews presented data on clinical manifestations, laboratory and radiological findings, and interventions. We found no systematic reviews on the utility of diagnostic tests.
Symptoms were reported in seven reviews; most of the patients had a fever, cough, dyspnea, myalgia or muscle fatigue, and gastrointestinal disorders such as diarrhea, nausea, or vomiting. Olfactory dysfunction (anosmia or dysosmia) has been described in patients infected with COVID-19 [ 43 ]; however, this was not reported in any of the reviews included in this overview. During the SARS outbreak in 2002, there were reports of impairment of the sense of smell associated with the disease [ 44 , 45 ].
The reported mortality rates ranged from 0.3 to 14% in the included reviews. Mortality estimates are influenced by the transmissibility rate (basic reproduction number), availability of diagnostic tools, notification policies, asymptomatic presentations of the disease, resources for disease prevention and control, and treatment facilities; variability in the mortality rate fits the pattern of emerging infectious diseases [ 46 ]. Furthermore, the reported cases did not consider asymptomatic cases, mild cases where individuals have not sought medical treatment, and the fact that many countries had limited access to diagnostic tests or have implemented testing policies later than the others. Considering the lack of reviews assessing diagnostic testing (sensitivity, specificity, and predictive values of RT-PCT or immunoglobulin tests), and the preponderance of studies that assessed only symptomatic individuals, considerable imprecision around the calculated mortality rates existed in the early stage of the COVID-19 pandemic.
Few reviews included treatment data. Those reviews described studies considered to be at a very low level of evidence: usually small, retrospective studies with very heterogeneous populations. Seven reviews analyzed laboratory parameters; those reviews could have been useful for clinicians who attend patients suspected of COVID-19 in emergency services worldwide, such as assessing which patients need to be reassessed more frequently.
All systematic reviews scored poorly on the AMSTAR 2 critical appraisal tool for systematic reviews. Most of the original studies included in the reviews were case series and case reports, impacting the quality of evidence. Such evidence has major implications for clinical practice and the use of these reviews in evidence-based practice and policy. Clinicians, patients, and policymakers can only have the highest confidence in systematic review findings if high-quality systematic review methodologies are employed. The urgent need for information during a pandemic does not justify poor quality reporting.
We acknowledge that there are numerous challenges associated with analyzing COVID-19 data during a pandemic [ 47 ]. High-quality evidence syntheses are needed for decision-making, but each type of evidence syntheses is associated with its inherent challenges.
The creation of classic systematic reviews requires considerable time and effort; with massive research output, they quickly become outdated, and preparing updated versions also requires considerable time. A recent study showed that updates of non-Cochrane systematic reviews are published a median of 5 years after the publication of the previous version [ 48 ].
Authors may register a review and then abandon it [ 49 ], but the existence of a public record that is not updated may lead other authors to believe that the review is still ongoing. A quarter of Cochrane review protocols remains unpublished as completed systematic reviews 8 years after protocol publication [ 50 ].
Rapid reviews can be used to summarize the evidence, but they involve methodological sacrifices and simplifications to produce information promptly, with inconsistent methodological approaches [ 51 ]. However, rapid reviews are justified in times of public health emergencies, and even Cochrane has resorted to publishing rapid reviews in response to the COVID-19 crisis [ 52 ]. Rapid reviews were eligible for inclusion in this overview, but only one of the 18 reviews included in this study was labeled as a rapid review.
Ideally, COVID-19 evidence would be continually summarized in a series of high-quality living systematic reviews, types of evidence synthesis defined as “ a systematic review which is continually updated, incorporating relevant new evidence as it becomes available ” [ 53 ]. However, conducting living systematic reviews requires considerable resources, calling into question the sustainability of such evidence synthesis over long periods [ 54 ].
Research reports about COVID-19 will contribute to research waste if they are poorly designed, poorly reported, or simply not necessary. In principle, systematic reviews should help reduce research waste as they usually provide recommendations for further research that is needed or may advise that sufficient evidence exists on a particular topic [ 55 ]. However, systematic reviews can also contribute to growing research waste when they are not needed, or poorly conducted and reported. Our present study clearly shows that most of the systematic reviews that were published early on in the COVID-19 pandemic could be categorized as research waste, as our confidence in their results is critically low.
Our study has some limitations. One is that for AMSTAR 2 assessment we relied on information available in publications; we did not attempt to contact study authors for clarifications or additional data. In three reviews, the methodological quality appraisal was challenging because they were published as letters, or labeled as rapid communications. As a result, various details about their review process were not included, leading to AMSTAR 2 questions being answered as “not reported”, resulting in low confidence scores. Full manuscripts might have provided additional information that could have led to higher confidence in the results. In other words, low scores could reflect incomplete reporting, not necessarily low-quality review methods. To make their review available more rapidly and more concisely, the authors may have omitted methodological details. A general issue during a crisis is that speed and completeness must be balanced. However, maintaining high standards requires proper resourcing and commitment to ensure that the users of systematic reviews can have high confidence in the results.
Furthermore, we used adjusted AMSTAR 2 scoring, as the tool was designed for critical appraisal of reviews of interventions. Some reviews may have received lower scores than actually warranted in spite of these adjustments.
Another limitation of our study may be the inclusion of multiple overlapping reviews, as some included reviews included the same primary studies. According to the Cochrane Handbook, including overlapping reviews may be appropriate when the review’s aim is “ to present and describe the current body of systematic review evidence on a topic ” [ 12 ], which was our aim. To avoid bias with summarizing evidence from overlapping reviews, we presented the forest plots without summary estimates. The forest plots serve to inform readers about the effect sizes for outcomes that were reported in each review.
Several authors from this study have contributed to one of the reviews identified [ 25 ]. To reduce the risk of any bias, two authors who did not co-author the review in question initially assessed its quality and limitations.
Finally, we note that the systematic reviews included in our overview may have had issues that our analysis did not identify because we did not analyze their primary studies to verify the accuracy of the data and information they presented. We give two examples to substantiate this possibility. Lovato et al. wrote a commentary on the review of Sun et al. [ 41 ], in which they criticized the authors’ conclusion that sore throat is rare in COVID-19 patients [ 56 ]. Lovato et al. highlighted that multiple studies included in Sun et al. did not accurately describe participants’ clinical presentations, warning that only three studies clearly reported data on sore throat [ 56 ].
In another example, Leung [ 57 ] warned about the review of Li, L.Q. et al. [ 29 ]: “ it is possible that this statistic was computed using overlapped samples, therefore some patients were double counted ”. Li et al. responded to Leung that it is uncertain whether the data overlapped, as they used data from published articles and did not have access to the original data; they also reported that they requested original data and that they plan to re-do their analyses once they receive them; they also urged readers to treat the data with caution [ 58 ]. This points to the evolving nature of evidence during a crisis.
Our study’s strength is that this overview adds to the current knowledge by providing a comprehensive summary of all the evidence synthesis about COVID-19 available early after the onset of the pandemic. This overview followed strict methodological criteria, including a comprehensive and sensitive search strategy and a standard tool for methodological appraisal of systematic reviews.
In conclusion, in this overview of systematic reviews, we analyzed evidence from the first 18 systematic reviews that were published after the emergence of COVID-19. However, confidence in the results of all the reviews was “critically low”. Thus, systematic reviews that were published early on in the pandemic could be categorized as research waste. Even during public health emergencies, studies and systematic reviews should adhere to established methodological standards to provide patients, clinicians, and decision-makers trustworthy evidence.
Availability of data and materials
All data collected and analyzed within this study are available from the corresponding author on reasonable request.
World Health Organization. Timeline - COVID-19: Available at: https://www.who.int/news/item/29-06-2020-covidtimeline . Accessed 1 June 2021.
COVID-19 Dashboard by the Center for Systems Science and Engineering (CSSE) at Johns Hopkins University (JHU). Available at: https://coronavirus.jhu.edu/map.html . Accessed 1 June 2021.
Anzai A, Kobayashi T, Linton NM, Kinoshita R, Hayashi K, Suzuki A, et al. Assessing the Impact of Reduced Travel on Exportation Dynamics of Novel Coronavirus Infection (COVID-19). J Clin Med. 2020;9(2):601.
Chinazzi M, Davis JT, Ajelli M, Gioannini C, Litvinova M, Merler S, et al. The effect of travel restrictions on the spread of the 2019 novel coronavirus (COVID-19) outbreak. Science. 2020;368(6489):395–400. https://doi.org/10.1126/science.aba9757 .
Article CAS PubMed PubMed Central Google Scholar
Fidahic M, Nujic D, Runjic R, Civljak M, Markotic F, Lovric Makaric Z, et al. Research methodology and characteristics of journal articles with original data, preprint articles and registered clinical trial protocols about COVID-19. BMC Med Res Methodol. 2020;20(1):161. https://doi.org/10.1186/s12874-020-01047-2 .
EPPI Centre . COVID-19: a living systematic map of the evidence. Available at: http://eppi.ioe.ac.uk/cms/Projects/DepartmentofHealthandSocialCare/Publishedreviews/COVID-19Livingsystematicmapoftheevidence/tabid/3765/Default.aspx . Accessed 1 June 2021.
NCBI SARS-CoV-2 Resources. Available at: https://www.ncbi.nlm.nih.gov/sars-cov-2/ . Accessed 1 June 2021.
Gustot T. Quality and reproducibility during the COVID-19 pandemic. JHEP Rep. 2020;2(4):100141. https://doi.org/10.1016/j.jhepr.2020.100141 .
Article PubMed PubMed Central Google Scholar
Kodvanj, I., et al., Publishing of COVID-19 Preprints in Peer-reviewed Journals, Preprinting Trends, Public Discussion and Quality Issues. Preprint article. bioRxiv 2020.11.23.394577; doi: https://doi.org/10.1101/2020.11.23.394577 .
Dobler CC. Poor quality research and clinical practice during COVID-19. Breathe (Sheff). 2020;16(2):200112. https://doi.org/10.1183/20734735.0112-2020 .
Article Google Scholar
Bastian H, Glasziou P, Chalmers I. Seventy-five trials and eleven systematic reviews a day: how will we ever keep up? PLoS Med. 2010;7(9):e1000326. https://doi.org/10.1371/journal.pmed.1000326 .
Lunny C, Brennan SE, McDonald S, McKenzie JE. Toward a comprehensive evidence map of overview of systematic review methods: paper 1-purpose, eligibility, search and data extraction. Syst Rev. 2017;6(1):231. https://doi.org/10.1186/s13643-017-0617-1 .
Pollock M, Fernandes RM, Becker LA, Pieper D, Hartling L. Chapter V: Overviews of Reviews. In: Higgins JPT, Thomas J, Chandler J, Cumpston M, Li T, Page MJ, Welch VA (editors). Cochrane Handbook for Systematic Reviews of Interventions version 6.1 (updated September 2020). Cochrane. 2020. Available from www.training.cochrane.org/handbook .
Higgins JPT, Thomas J, Chandler J, Cumpston M, Li T, Page MJ, et al. Cochrane handbook for systematic reviews of interventions version 6.1 (updated September 2020). Cochrane. 2020; Available from www.training.cochrane.org/handbook .
Pollock M, Fernandes RM, Newton AS, Scott SD, Hartling L. The impact of different inclusion decisions on the comprehensiveness and complexity of overviews of reviews of healthcare interventions. Syst Rev. 2019;8(1):18. https://doi.org/10.1186/s13643-018-0914-3 .
Pollock M, Fernandes RM, Newton AS, Scott SD, Hartling L. A decision tool to help researchers make decisions about including systematic reviews in overviews of reviews of healthcare interventions. Syst Rev. 2019;8(1):29. https://doi.org/10.1186/s13643-018-0768-8 .
Hunt H, Pollock A, Campbell P, Estcourt L, Brunton G. An introduction to overviews of reviews: planning a relevant research question and objective for an overview. Syst Rev. 2018;7(1):39. https://doi.org/10.1186/s13643-018-0695-8 .
Pollock M, Fernandes RM, Pieper D, Tricco AC, Gates M, Gates A, et al. Preferred reporting items for overviews of reviews (PRIOR): a protocol for development of a reporting guideline for overviews of reviews of healthcare interventions. Syst Rev. 2019;8(1):335. https://doi.org/10.1186/s13643-019-1252-9 .
Moher D, Liberati A, Tetzlaff J, Altman DG, PRISMA Group. Preferred reporting items for systematic reviews and meta-analyses: the PRISMA statement. Open Med. 2009;3(3):e123–30.
Krnic Martinic M, Pieper D, Glatt A, Puljak L. Definition of a systematic review used in overviews of systematic reviews, meta-epidemiological studies and textbooks. BMC Med Res Methodol. 2019;19(1):203. https://doi.org/10.1186/s12874-019-0855-0 .
Puljak L. If there is only one author or only one database was searched, a study should not be called a systematic review. J Clin Epidemiol. 2017;91:4–5. https://doi.org/10.1016/j.jclinepi.2017.08.002 .
Article PubMed Google Scholar
Gates M, Gates A, Guitard S, Pollock M, Hartling L. Guidance for overviews of reviews continues to accumulate, but important challenges remain: a scoping review. Syst Rev. 2020;9(1):254. https://doi.org/10.1186/s13643-020-01509-0 .
Covidence - systematic review software. Available at: https://www.covidence.org/ . Accessed 1 June 2021.
Shea BJ, Reeves BC, Wells G, Thuku M, Hamel C, Moran J, et al. AMSTAR 2: a critical appraisal tool for systematic reviews that include randomised or non-randomised studies of healthcare interventions, or both. BMJ. 2017;358:j4008.
Borges do Nascimento IJ, et al. Novel Coronavirus Infection (COVID-19) in Humans: A Scoping Review and Meta-Analysis. J Clin Med. 2020;9(4):941.
Article PubMed Central Google Scholar
Adhikari SP, Meng S, Wu YJ, Mao YP, Ye RX, Wang QZ, et al. Epidemiology, causes, clinical manifestation and diagnosis, prevention and control of coronavirus disease (COVID-19) during the early outbreak period: a scoping review. Infect Dis Poverty. 2020;9(1):29. https://doi.org/10.1186/s40249-020-00646-x .
Cortegiani A, Ingoglia G, Ippolito M, Giarratano A, Einav S. A systematic review on the efficacy and safety of chloroquine for the treatment of COVID-19. J Crit Care. 2020;57:279–83. https://doi.org/10.1016/j.jcrc.2020.03.005 .
Li B, Yang J, Zhao F, Zhi L, Wang X, Liu L, et al. Prevalence and impact of cardiovascular metabolic diseases on COVID-19 in China. Clin Res Cardiol. 2020;109(5):531–8. https://doi.org/10.1007/s00392-020-01626-9 .
Article CAS PubMed Google Scholar
Li LQ, Huang T, Wang YQ, Wang ZP, Liang Y, Huang TB, et al. COVID-19 patients’ clinical characteristics, discharge rate, and fatality rate of meta-analysis. J Med Virol. 2020;92(6):577–83. https://doi.org/10.1002/jmv.25757 .
Lippi G, Lavie CJ, Sanchis-Gomar F. Cardiac troponin I in patients with coronavirus disease 2019 (COVID-19): evidence from a meta-analysis. Prog Cardiovasc Dis. 2020;63(3):390–1. https://doi.org/10.1016/j.pcad.2020.03.001 .
Lippi G, Henry BM. Active smoking is not associated with severity of coronavirus disease 2019 (COVID-19). Eur J Intern Med. 2020;75:107–8. https://doi.org/10.1016/j.ejim.2020.03.014 .
Lippi G, Plebani M. Procalcitonin in patients with severe coronavirus disease 2019 (COVID-19): a meta-analysis. Clin Chim Acta. 2020;505:190–1. https://doi.org/10.1016/j.cca.2020.03.004 .
Lippi G, Plebani M, Henry BM. Thrombocytopenia is associated with severe coronavirus disease 2019 (COVID-19) infections: a meta-analysis. Clin Chim Acta. 2020;506:145–8. https://doi.org/10.1016/j.cca.2020.03.022 .
Ludvigsson JF. Systematic review of COVID-19 in children shows milder cases and a better prognosis than adults. Acta Paediatr. 2020;109(6):1088–95. https://doi.org/10.1111/apa.15270 .
Lupia T, Scabini S, Mornese Pinna S, di Perri G, de Rosa FG, Corcione S. 2019 novel coronavirus (2019-nCoV) outbreak: a new challenge. J Glob Antimicrob Resist. 2020;21:22–7. https://doi.org/10.1016/j.jgar.2020.02.021 .
Marasinghe, K.M., A systematic review investigating the effectiveness of face mask use in limiting the spread of COVID-19 among medically not diagnosed individuals: shedding light on current recommendations provided to individuals not medically diagnosed with COVID-19. Research Square. Preprint article. doi : https://doi.org/10.21203/rs.3.rs-16701/v1 . 2020 .
Mullins E, Evans D, Viner RM, O’Brien P, Morris E. Coronavirus in pregnancy and delivery: rapid review. Ultrasound Obstet Gynecol. 2020;55(5):586–92. https://doi.org/10.1002/uog.22014 .
Pang J, Wang MX, Ang IYH, Tan SHX, Lewis RF, Chen JIP, et al. Potential Rapid Diagnostics, Vaccine and Therapeutics for 2019 Novel coronavirus (2019-nCoV): a systematic review. J Clin Med. 2020;9(3):623.
Rodriguez-Morales AJ, Cardona-Ospina JA, Gutiérrez-Ocampo E, Villamizar-Peña R, Holguin-Rivera Y, Escalera-Antezana JP, et al. Clinical, laboratory and imaging features of COVID-19: a systematic review and meta-analysis. Travel Med Infect Dis. 2020;34:101623. https://doi.org/10.1016/j.tmaid.2020.101623 .
Salehi S, Abedi A, Balakrishnan S, Gholamrezanezhad A. Coronavirus disease 2019 (COVID-19): a systematic review of imaging findings in 919 patients. AJR Am J Roentgenol. 2020;215(1):87–93. https://doi.org/10.2214/AJR.20.23034 .
Sun P, Qie S, Liu Z, Ren J, Li K, Xi J. Clinical characteristics of hospitalized patients with SARS-CoV-2 infection: a single arm meta-analysis. J Med Virol. 2020;92(6):612–7. https://doi.org/10.1002/jmv.25735 .
Yang J, Zheng Y, Gou X, Pu K, Chen Z, Guo Q, et al. Prevalence of comorbidities and its effects in patients infected with SARS-CoV-2: a systematic review and meta-analysis. Int J Infect Dis. 2020;94:91–5. https://doi.org/10.1016/j.ijid.2020.03.017 .
Bassetti M, Vena A, Giacobbe DR. The novel Chinese coronavirus (2019-nCoV) infections: challenges for fighting the storm. Eur J Clin Investig. 2020;50(3):e13209. https://doi.org/10.1111/eci.13209 .
Article CAS Google Scholar
Hwang CS. Olfactory neuropathy in severe acute respiratory syndrome: report of a case. Acta Neurol Taiwanica. 2006;15(1):26–8.
Google Scholar
Suzuki M, Saito K, Min WP, Vladau C, Toida K, Itoh H, et al. Identification of viruses in patients with postviral olfactory dysfunction. Laryngoscope. 2007;117(2):272–7. https://doi.org/10.1097/01.mlg.0000249922.37381.1e .
Rajgor DD, Lee MH, Archuleta S, Bagdasarian N, Quek SC. The many estimates of the COVID-19 case fatality rate. Lancet Infect Dis. 2020;20(7):776–7. https://doi.org/10.1016/S1473-3099(20)30244-9 .
Wolkewitz M, Puljak L. Methodological challenges of analysing COVID-19 data during the pandemic. BMC Med Res Methodol. 2020;20(1):81. https://doi.org/10.1186/s12874-020-00972-6 .
Rombey T, Lochner V, Puljak L, Könsgen N, Mathes T, Pieper D. Epidemiology and reporting characteristics of non-Cochrane updates of systematic reviews: a cross-sectional study. Res Synth Methods. 2020;11(3):471–83. https://doi.org/10.1002/jrsm.1409 .
Runjic E, Rombey T, Pieper D, Puljak L. Half of systematic reviews about pain registered in PROSPERO were not published and the majority had inaccurate status. J Clin Epidemiol. 2019;116:114–21. https://doi.org/10.1016/j.jclinepi.2019.08.010 .
Runjic E, Behmen D, Pieper D, Mathes T, Tricco AC, Moher D, et al. Following Cochrane review protocols to completion 10 years later: a retrospective cohort study and author survey. J Clin Epidemiol. 2019;111:41–8. https://doi.org/10.1016/j.jclinepi.2019.03.006 .
Tricco AC, Antony J, Zarin W, Strifler L, Ghassemi M, Ivory J, et al. A scoping review of rapid review methods. BMC Med. 2015;13(1):224. https://doi.org/10.1186/s12916-015-0465-6 .
COVID-19 Rapid Reviews: Cochrane’s response so far. Available at: https://training.cochrane.org/resource/covid-19-rapid-reviews-cochrane-response-so-far . Accessed 1 June 2021.
Cochrane. Living systematic reviews. Available at: https://community.cochrane.org/review-production/production-resources/living-systematic-reviews . Accessed 1 June 2021.
Millard T, Synnot A, Elliott J, Green S, McDonald S, Turner T. Feasibility and acceptability of living systematic reviews: results from a mixed-methods evaluation. Syst Rev. 2019;8(1):325. https://doi.org/10.1186/s13643-019-1248-5 .
Babic A, Poklepovic Pericic T, Pieper D, Puljak L. How to decide whether a systematic review is stable and not in need of updating: analysis of Cochrane reviews. Res Synth Methods. 2020;11(6):884–90. https://doi.org/10.1002/jrsm.1451 .
Lovato A, Rossettini G, de Filippis C. Sore throat in COVID-19: comment on “clinical characteristics of hospitalized patients with SARS-CoV-2 infection: a single arm meta-analysis”. J Med Virol. 2020;92(7):714–5. https://doi.org/10.1002/jmv.25815 .
Leung C. Comment on Li et al: COVID-19 patients’ clinical characteristics, discharge rate, and fatality rate of meta-analysis. J Med Virol. 2020;92(9):1431–2. https://doi.org/10.1002/jmv.25912 .
Li LQ, Huang T, Wang YQ, Wang ZP, Liang Y, Huang TB, et al. Response to Char’s comment: comment on Li et al: COVID-19 patients’ clinical characteristics, discharge rate, and fatality rate of meta-analysis. J Med Virol. 2020;92(9):1433. https://doi.org/10.1002/jmv.25924 .
Download references
Acknowledgments
We thank Catherine Henderson DPhil from Swanscoe Communications for pro bono medical writing and editing support. We acknowledge support from the Covidence Team, specifically Anneliese Arno. We thank the whole International Network of Coronavirus Disease 2019 (InterNetCOVID-19) for their commitment and involvement. Members of the InterNetCOVID-19 are listed in Additional file 6 . We thank Pavel Cerny and Roger Crosthwaite for guiding the team supervisor (IJBN) on human resources management.
This research received no external funding.
Author information
Authors and affiliations.
University Hospital and School of Medicine, Universidade Federal de Minas Gerais, Belo Horizonte, Minas Gerais, Brazil
Israel Júnior Borges do Nascimento & Milena Soriano Marcolino
Medical College of Wisconsin, Milwaukee, WI, USA
Israel Júnior Borges do Nascimento
Helene Fuld Health Trust National Institute for Evidence-based Practice in Nursing and Healthcare, College of Nursing, The Ohio State University, Columbus, OH, USA
Dónal P. O’Mathúna
School of Nursing, Psychotherapy and Community Health, Dublin City University, Dublin, Ireland
Department of Anesthesiology, Intensive Care and Pain Medicine, University of Münster, Münster, Germany
Thilo Caspar von Groote
Department of Sport and Health Science, Technische Universität München, Munich, Germany
Hebatullah Mohamed Abdulazeem
School of Health Sciences, Faculty of Health and Medicine, The University of Newcastle, Callaghan, Australia
Ishanka Weerasekara
Department of Physiotherapy, Faculty of Allied Health Sciences, University of Peradeniya, Peradeniya, Sri Lanka
Cochrane Croatia, University of Split, School of Medicine, Split, Croatia
Ana Marusic, Irena Zakarija-Grkovic & Tina Poklepovic Pericic
Center for Evidence-Based Medicine and Health Care, Catholic University of Croatia, Ilica 242, 10000, Zagreb, Croatia
Livia Puljak
Cochrane Brazil, Evidence-Based Health Program, Universidade Federal de São Paulo, São Paulo, Brazil
Vinicius Tassoni Civile & Alvaro Nagib Atallah
Yorkville University, Fredericton, New Brunswick, Canada
Santino Filoso
Laboratory for Industrial and Applied Mathematics (LIAM), Department of Mathematics and Statistics, York University, Toronto, Ontario, Canada
Nicola Luigi Bragazzi
You can also search for this author in PubMed Google Scholar
Contributions
IJBN conceived the research idea and worked as a project coordinator. DPOM, TCVG, HMA, IW, AM, LP, VTC, IZG, TPP, ANA, SF, NLB and MSM were involved in data curation, formal analysis, investigation, methodology, and initial draft writing. All authors revised the manuscript critically for the content. The author(s) read and approved the final manuscript.
Corresponding author
Correspondence to Livia Puljak .
Ethics declarations
Ethics approval and consent to participate.
Not required as data was based on published studies.
Consent for publication
Not applicable.
Competing interests
The authors declare no conflict of interest.
Additional information
Publisher’s note.
Springer Nature remains neutral with regard to jurisdictional claims in published maps and institutional affiliations.
Supplementary Information
Additional file 1: appendix 1..
Search strategies used in the study.
Additional file 2: Appendix 2.
Adjusted scoring of AMSTAR 2 used in this study for systematic reviews of studies that did not analyze interventions.
Additional file 3: Appendix 3.
List of excluded studies, with reasons.
Additional file 4: Appendix 4.
Table of overlapping studies, containing the list of primary studies included, their visual overlap in individual systematic reviews, and the number in how many reviews each primary study was included.
Additional file 5: Appendix 5.
A detailed explanation of AMSTAR scoring for each item in each review.
Additional file 6: Appendix 6.
List of members and affiliates of International Network of Coronavirus Disease 2019 (InterNetCOVID-19).
Rights and permissions
Open Access This article is licensed under a Creative Commons Attribution 4.0 International License, which permits use, sharing, adaptation, distribution and reproduction in any medium or format, as long as you give appropriate credit to the original author(s) and the source, provide a link to the Creative Commons licence, and indicate if changes were made. The images or other third party material in this article are included in the article's Creative Commons licence, unless indicated otherwise in a credit line to the material. If material is not included in the article's Creative Commons licence and your intended use is not permitted by statutory regulation or exceeds the permitted use, you will need to obtain permission directly from the copyright holder. To view a copy of this licence, visit http://creativecommons.org/licenses/by/4.0/ . The Creative Commons Public Domain Dedication waiver ( http://creativecommons.org/publicdomain/zero/1.0/ ) applies to the data made available in this article, unless otherwise stated in a credit line to the data.
Reprints and permissions
About this article
Cite this article.
Borges do Nascimento, I.J., O’Mathúna, D.P., von Groote, T.C. et al. Coronavirus disease (COVID-19) pandemic: an overview of systematic reviews. BMC Infect Dis 21 , 525 (2021). https://doi.org/10.1186/s12879-021-06214-4
Download citation
Received : 12 April 2020
Accepted : 19 May 2021
Published : 04 June 2021
DOI : https://doi.org/10.1186/s12879-021-06214-4
Share this article
Anyone you share the following link with will be able to read this content:
Sorry, a shareable link is not currently available for this article.
Provided by the Springer Nature SharedIt content-sharing initiative
- Coronavirus
- Evidence-based medicine
- Infectious diseases
BMC Infectious Diseases
ISSN: 1471-2334
- Submission enquiries: [email protected]
- General enquiries: [email protected]
Research on Quantitative Analysis of Multiple Factors Affecting COVID-19 Spread
Affiliations.
- 1 Faculty of Information Technology, Beijing University of Technology, Beijing 100124, China.
- 2 Beijing Institute of Smart City, Beijing University of Technology, Beijing 100124, China.
- PMID: 35328880
- PMCID: PMC8953928
- DOI: 10.3390/ijerph19063187
The Corona Virus Disease 2019 (COVID-19) is spreading all over the world. Quantitative analysis of the effects of various factors on the spread of the epidemic will help people better understand the transmission characteristics of SARS-CoV-2, thus providing a theoretical basis for governments to develop epidemic prevention and control strategies. This article uses public data sets from The Center for Systems Science and Engineering at Johns Hopkins University (JHU CSSE), Air Quality Open Data Platform, China Meteorological Data Network, and WorldPop website to construct experimental data. The epidemic situation is predicted by Dual-link BiGRU Network, and the relationship between epidemic spread and various feature factors is quantitatively analyzed by the Gauss-Newton iteration Method. The study found that population density has the greatest positive correlation to the spread of the epidemic among the selected feature factors, followed by the number of landing flights. The number of newly diagnosed daily will increase by 1.08% for every 1% of the population density, the number of newly diagnosed daily will increase by 0.98% for every 1% of the number of landing flights. The results of this study show that the control of social distance and population movement has a high priority in epidemic prevention and control strategies, and it can play a very important role in controlling the spread of the epidemic.
Keywords: COVID-19; Gauss-Newton iteration; neural network; quantitative analysis.
- COVID-19* / epidemiology
- China / epidemiology
- Disease Outbreaks / prevention & control
Thank you for visiting nature.com. You are using a browser version with limited support for CSS. To obtain the best experience, we recommend you use a more up to date browser (or turn off compatibility mode in Internet Explorer). In the meantime, to ensure continued support, we are displaying the site without styles and JavaScript.
- View all journals
- My Account Login
- Explore content
- About the journal
- Publish with us
- Sign up for alerts
- Open access
- Published: 16 April 2024
Demographic, social, and clinical aspects associated with access to COVID-19 health care in Pará province, Brazilian Amazon
- Amanda Loyse da Costa Miranda 1 ,
- Ana Rosa Tavares da Paixão 1 ,
- Andrey Oeiras Pedroso 2 ,
- Laís do Espírito Santo Lima 2 ,
- Andressa Tavares Parente 1 ,
- Eliã Pinheiro Botelho 1 ,
- Sandra Helena Isse Polaro 1 ,
- Ana Cristina de Oliveira e Silva 3 ,
- Renata Karina Reis 2 &
- Glenda Roberta Oliveira Naiff Ferreira 1
Scientific Reports volume 14 , Article number: 8776 ( 2024 ) Cite this article
697 Accesses
Metrics details
- Health services
- Infectious diseases
- Viral infection
Internal social disparities in the Brazilian Amazon became more evident during the COVID-19 pandemic. The aim of this work was to examine the demographic, social and clinical factors associated with access to COVID-19 health care in Pará Province in the Brazilian Amazon. This was an observational, cross-sectional, analytical study using a quantitative method through an online survey conducted from May to August 2023. People were eligible to participate if they were current residents of Pará, 18-years-old or older, with self-reported diagnoses of COVID-19 through rapid or laboratory tests. Participants completed an electronic survey was developed using Research Electronic Data Capture (REDCap) software—The adapted questionnaire “COVID-19 Global Clinical Platform: Case Report Form for Post-COVID Condition”. Questions focused on access to COVID-19 treatment, demographic characteristics, COVID-19 vaccine and clinical characteristics. Respondent-driven sampling was applied to recruit participants. Multiple logistic regression was utilized to identify the associated factors. Overall, a total of 638 participants were included. The average age was 31.1 years. Access to COVID-19 health care was 68.65% (438/638). The participants most likely to access health care were those with moderate or severe COVID-19 ( p = 0.000; OR: 19.8) and females ( p = 0.001; OR: 1.99). Moreover, participants who used homemade tea or herbal medicines were less likely to receive health care for COVID-19 in health services ( p = 0.002; OR: 0.54). Ensuring access to healthcare is important in a pandemic scenario.
Similar content being viewed by others
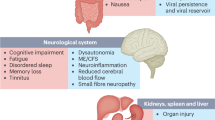
Long COVID: major findings, mechanisms and recommendations
Hannah E. Davis, Lisa McCorkell, … Eric J. Topol
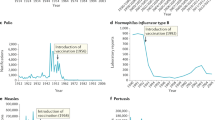
A guide to vaccinology: from basic principles to new developments
Andrew J. Pollard & Else M. Bijker
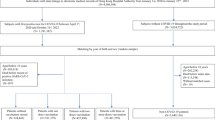
Persistence in risk and effect of COVID-19 vaccination on long-term health consequences after SARS-CoV-2 infection
Ivan Chun Hang Lam, Ran Zhang, … Eric Yuk Fai Wan
Introduction
COVID-19 was declared a pandemic in March 2020 and has become one of the greatest global health crises of the last hundred years 1–2 . In Brazil, the infection and disease affected thousands of people, with deaths recorded in all states of the federation, including the northern region located in the Brazilian Amazon 3 .
During the COVID-19 outbreak, despite accounting for only 7.73% of the confirmed cases in Brazil in the Brazilian Amazon region, the population was disproportionately impacted by COVID-19; this was primarily attributed to the area's sociodemographic composition, inadequate health care services, barriers to geographic mobility, deficient transport infrastructure, and lack of preparedness in coordinating an effective response to the COVID-19 pandemic 3–8 . Throughout this time period, internal social disparities in the region became more evident. Studies have indicated that the most affected groups included marginalized individuals with low income and education who lacked adherence to mask usage and social distancing. Moreover, indigenous refugees, those with limited health care accessibility, and individuals resorting to self-medication for prevention were notably affected 9–11 ; this indicates that the majority of the population is dependent on the Unified Health System (Sistema Único de Saúde, acronym SUS, in Portuguese), with universal access to health care services and cost-free access across all levels, thus emphasizing the significance of primary health care (PHC) as the primary entry point for this system 12 .
The population was affected by the pandemic and problems in the national management of the public health system during this crisis, which affected the diagnosis and treatment of COVID-19 patients. Management failures included the indication of ineffective medications for the treatment of COVID-19, such as chloroquine and ivermectin, and interference with the national vaccination plan against the virus 13 . In this context, local governments coordinated local initiatives to organize the health system. Regarding system management, collaboration with universities was fundamental, thus enhancing the use of epidemiology as a management tool and facilitating access to laboratory services and hospital care 5 .
Notably, as a consequence of the previously described scenario, the Amazon region experienced a serious collapse in its local health care system, which was especially evident during the second wave of COVID-19 in January 2021 in Manaus, Amazonas; this was represented by hospitals lacking an oxygen supply 16–18 . This catastrophic situation likely led to underreporting of COVID-19 infections, as many individuals (particularly those who did not access health care) remained untested 18 . Extensive studies have investigated various aspects related to COVID-19 in the Amazon context. However, there remains a gap in the understanding of the factors associated with access to health care, specifically for COVID-19-related health care 9–11 .
In the scenario in which demographic and clinical factors interact with other factors and influence access to health care, a framework for the study of access to health care was adopted 19 . Scholars have investigated access to health care for other illnesses not related to COVID-19 20–22 . Only one study has been conducted to examine geographic access to COVID-19 health care in Brazil and revealed that the amount of equipment available varies between municipalities and is lower among black and poor communities 22 .
This study is especially crucial in scenarios characterized by limited availability of health care 8,23–24 , geographic challenges 6 and inadequate management of the pandemic 13,15,18 . Therefore, the aim of this work was to examine the demographic, social and clinical factors associated with access to COVID-19 health care in Pará Province in the Brazilian Amazon; this particular state stands out as having the highest concentration of COVID-19 cases among North Region states (accounting for 27.81% of cases), with its capital (Belém) serving as the epicenter of the pandemic within this state 3 .
Study design and setting
This was an observational, cross-sectional, analytical study using a quantitative method through an online survey conducted from May to August 2023.
The Pará Province includes 144 municipalities, with 1,245,870.704 square kilometers, located in the Brazilian Amazon in the northern region of Brazil (Fig. 1 ); additionally, it has an estimated population of 8,777,124 inhabitants. This population exhibits precarious social and health indicators. In 2022, 1,808 PHC teams were registered to serve 5,391,499 inhabitants. This low coverage of PHC reduces access to other levels of the health system, when considering that in Brazil, the PHC coordinates care 25–26 .
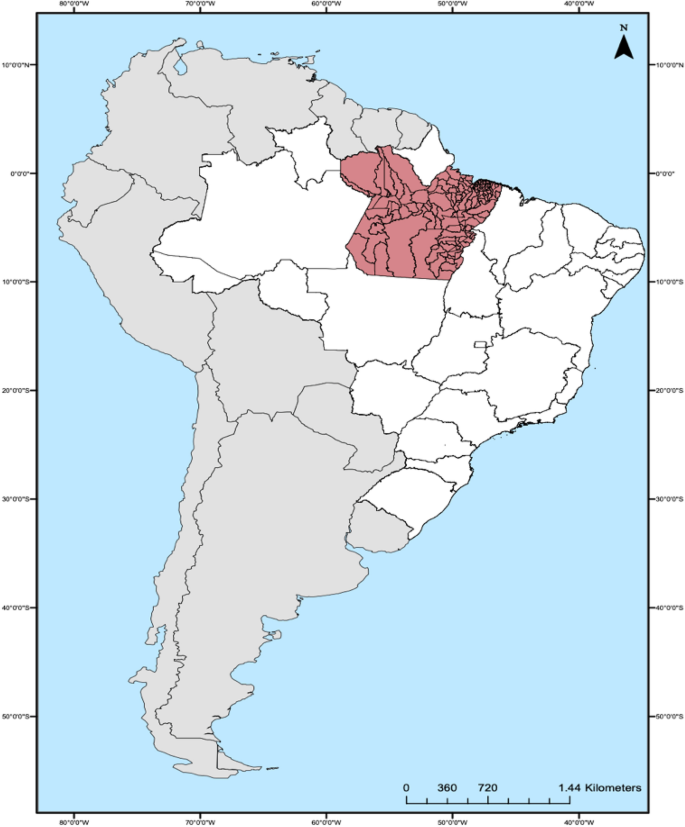
© 2023 by Amanda Miranda (software ArcGIS 10.8, https://www.arcgis.com/index.html ) is licensed under CC BY-ND 4.0. To view a copy of this license, visit.
The location of the state of Pará
Participants and selection criteria
People were eligible to participate if they were current residents of Pará, 18-years-old or older, with self-reported diagnoses of COVID-19 through rapid or laboratory tests. The exclusion criterion included people who did not have access to the internet or electronic devices to answer the questionnaire.
The calculations were performed in the population survey in the EpiInfo version 7.2.5.0 in the StatCalc module. For the sample calculation of the quantitative study, the following parameters were adopted: 777,901 people with COVID-19 infection in Pará; margin of error of 5%; 95% confidence interval, 38.6% frequency of people with reactive IgG who used preventative medication for COVID-19 9 (people who received preventive medication for COVID-19, including medicines that require a doctor's prescription – people who sought health care to treat COVID-19). The use of health care was not limited to people with moderate or severe symptoms, thus resulting in a minimum sample of 364 participants. This number quickly exceeded that of the online survey; therefore, it was decided to use the total number of people who responded to the questionnaire during the data collection period.
Study instrument (questionnaire)
To collect data, an adapted version of the “Global COVID-19 Clinical Platform: Case Report Form for Post-COVID-19 conditions” questionnaire from the World Health Organization was used 27 . The questionnaire (structured) was adapted and translated by researchers from three federal universities in different regions of Brazil to make the language clear and accessible to the general population. To make the questionnaire easy to complete, unnecessary reading effort was reduced, and a cohesive and coherent order was established between the questions. Participants took an average of 15 min to complete the questionnaire. The questionnaire was organized into six domains. For this study, only two domains were used: sociodemographic and clinical characteristics related to SARS-CoV-2 infection.
In the sociodemographic domain, the following variables were used: sex (female/male); traditional population (yes/no); age (years); skin color (black/brown/indigenous/white/yellow); education level (did not study/elementary/high school/university); living in the state capital (yes/no); current job (unemployed/student/retired/employed/self-employed); beneficiary of social programs (yes/no); tobacco use during the pandemic (yes/no); use of alcoholic beverages during the pandemic (yes/no); personal monthly income minimum wage (in reais) (equal or less than 1 salary/above 1 salary); marital status (married/common-law/divorced/separated/widowed/single).
In the clinical characteristics domain related to SARS-CoV-2 infection, the following variables were considered: number of COVID-19 reinfections that were laboratory confirmed (mean); year of first COVID-19 infection (2020–2021/2022–2023); chronic illness before COVID-19 infection (yes/no); and classification of the most serious COVID-19 infection (severe: was admitted to the intensive care unit or needed to be intubated/moderate: had proven pneumonia or was hospitalized or needed oxygen support/mild: respiratory symptoms, but no pneumonia or shortness of breath); herbal medicines and homemade tea (yes/no); COVID-19 vaccine (yes/no); number of doses of COVID-19 vaccine (mean); and COVID-19 infection before being vaccinated (yes/no).
In this study, we considered traditional populations of the Amazon as those inhabiting the waters, fields, and forest: quilombola remnants (Afro-descendants who live in specific territories), indigenous peoples and riverside dwellers, following the concept established by the National Policy for Comprehensive Health of Rural and Forest Populations 28 .
Data collection
Participants completed an electronic survey using Research Electronic Data Capture (REDCap)® –software, including the adapted questionnaire “COVID-19 Global Clinical Platform: Case Report Form for Post-COVID-19 Condition”. Respondent-driven sampling (RDS), which is a variant of the "snowball sampling" methodology that is widely used in online surveys, was applied to recruit participants 29–30 . Through this method, participants are encouraged to share the questionnaire link with other individuals who meet the study's inclusion criteria to respond to the survey via social networks, with WhatsApp® being the social network that was used in this study.
To reach the minimum sample size, students from health-related undergraduate programs in all of the municipalities of the state were recruited. They underwent a four-hour training session on conducting the online survey by using the RDS methodology and the clarification of questionnaire-related queries. Each student invited 10 individuals from their circle who reported of COVID-19 infection to participate in the research. For each respondent who completed the survey, they correspondingly referred another 10 individuals, thus creating reference chains that increased the recruitment cycles/waves and expanding the number of participants 29–30 . In this study, 201 students were recruited and divided among 31 training sessions. Each student recorded data in an Excel spreadsheet, which included the number of participants who they invited, how many people were referred by each invitee, and other factors. This process was repeated until researchers achieved the minimum sample for the study.
The completed questionnaires were hosted on the REDCap® platform, which is designed for online data management and features settings and tools that enhance organizational capacity and security for storing information. It is important to highlight that, given the nature of the online survey, all of the collected information was self-reported.
The response variable of the study was the question “How was your treatment/health care?”. The answers to this question were “I was treated alone, without evaluation by a health care professional”, “I was admitted to a ward”, “I was admitted to an intensive care unit”, “I was treated at home, with support from health care professionals via telephone or internet”, “I received care in an outpatient clinic (the clinics provide specialized outpatient secondary care)”, “I received care in a private hospital (agreement/health insurance)”, “I received care in an emergency room or an emergency care unit”, and “I received care in a health center (PHC)”. The health center or basic health unit involves primary health care services provided by generalist health professionals, which can be offered by the government (free) or in private services. In Brazil, there are a large number of services offered by the government.
All of the responses in which participants stated that they received health care were grouped into a single response: “I received health care”. The outcome is a dichotomous qualitative variable, “Received” or “Not Received (self-care)”. The response event was "received". The independent variables included the variables that were included in the questionnaire, which are divided into the following domains: demographic, social characterization, and clinical characteristics.
Quantitative variables
The variables age, number of COVID-19 reinfections, and number of doses of the COVID-19 vaccine were analyzed as quantitative (continuous variables) independent variables.
Statistical methods
The data were extracted from REDCAP® and exported to Microsoft Excel®. Descriptive statistics, means, standard deviations, confidence intervals, absolute frequencies, and percentages were calculated. The result was presented by using texts, graphics, and tables. The 'nonresponse” variables were excluded from the statistical analysis, and no percentage was calculated.
The main hypothesis of the study, “factors associated with receiving treatment/health care (access to health care)”, was tested by using multiple logistic regression. When considering the dichotomous nature of the response variable, binomial regression was initially used to assess the association between each independent variable and the dependent variable. All of the variables were tested in this model, and variables with a p value < 0.020 were selected for inclusion in the multiple logistic regression model (sex, traditional population, age, education level, living in the state capital, individual income, marital status, number of COVID-19 reinfections, chronic illness before COVID-19 infection, classification of the most serious COVID-19 infection, use of herbal medicines and homemade tea, and number of doses of COVID-19 vaccine).
In the final multiple logistic regression model, the variables with p values < 0.20 were analyzed together by using the backward elimination model. This involves the introduction of all factors that were selected in the first stage and at each stage of the regression and is automatically removed by the program until only factors with a p value < 0.10 remain (number of COVID-19 reinfections, sex, chronic illness before COVID-19 infection, classification of the most serious COVID-19 infection, and use of herbal medicines and homemade tea).
All p values < 0.05 were considered to indicate statistical significance. To interpret the results, quality tests, coefficient values of the independent variables, Z values, 95% confidence intervals, and odds ratios were considered in the regression. The analyses were performed by using the Bioestat 5.3®, Microsoft Excel®, and Minitab 20® programs.
Ethics approval and consent to participate
All of the requirements proposed by Resolution 466 of 2012 of the National Health Council of Brazil were followed, as well as all of the principles established in General Personal Data Protection Law No. 13,709 of 2018 concerning personal data processing activities, as established in articles 6 and 7. The Declaration of Helsinki was followed. The study was approved by the Research Ethics Committee of the Federal University of Paraiba Lauro Wanderley University Hospital under protocol number 5.826.893 and CAAE: 65929522.1.0000.5183. All of the participants signed the Free and Informed Consent Form. The authors did not perform experiments on humans and/or use human tissue samples/human data. The authors did not have direct contact with the study participants because the study questionnaire was administered via the internet, and the data were stored by REDCAP®, which guarantees security in terms of privacy and data storage.
Sociodemographic characteristics
In this study, 438 (68.65%) out of 638 participants received health care for COVID-19, whereas the remaining 200 (31.35%) participants chose self-care (not received). The average age was 31.1 years (standard deviation [SD] = 11.9; 95% CI: 30.2, 32.0), and the 18- to 32-year-old age group had the highest percentage at 66.3% (421). Among the participants, 68.3% (436) were female; 40.8% (258) were employed or retired; 35.9% (229) were self-declared students; 57.9% (357) had incomes less than or equal to the minimum wage; 74% (472) were unrelated (divorced, separated, widowed or single); 11.7% (74) were beneficiaries of social programs; and 3.5% (22) were people belonging to traditional populations (Table 1 ).
Table 1 presents the results of the binary regression of the associations between sociodemographic characteristics and access status to COVID-19 health care in Pará Province in the Brazilian Amazon region. The results demonstrated that female participants were 1.62 times more likely to access/receive COVID-19 health care than male participants were (p = 0.007). As age increased, the chances of accessing/receiving COVID-19 health care also increased (p = 0.01; OR: 1.02). Variables with p values < 0.20 were selected for multiple regression, including traditional population, educational level, lives in the state capital, individual income, and marital status.
Clinical characteristics
Figure 2 shows the clinical characteristics and COVID-19 infection status of the patients. Regarding the year of first COVID-19 infection, 82% (519) of participants were diagnosed between 2020 and 2021, and 18% (114) of participants were diagnosed between 2022 and 2023. Only five (5) participants did not respond. The first year of COVID-19 infection was not associated with access to COVID-19 health care (p = 0.88; OR: 0.96; 95% CI: 1.13, 1.95). Regarding the presence of any preexisting chronic conditions before COVID-19 infection, among the 598 participants who responded to this inquiry, 86.8% (519) reported of no history of chronic ailments, whereas 13.2% (79) acknowledged of having some form of preexisting disease. Participants with a chronic disease were 2.38 times more likely to access health care than were those without any disease (p = 0.005; 95% CI: 1.30, 4.36). The majority of participants at 86.5% (552) were classified as having mild COVID-19 infection, whereas only 13.5% (86) had severe or moderate infections. Individuals displaying moderate or severe COVID-19 infection had an 11.2-fold greater likelihood of receiving health care than those exhibiting mild symptoms of the disease (p < 0.0001; 95% CI: 4.07, 31.2) (Fig. 2 A).
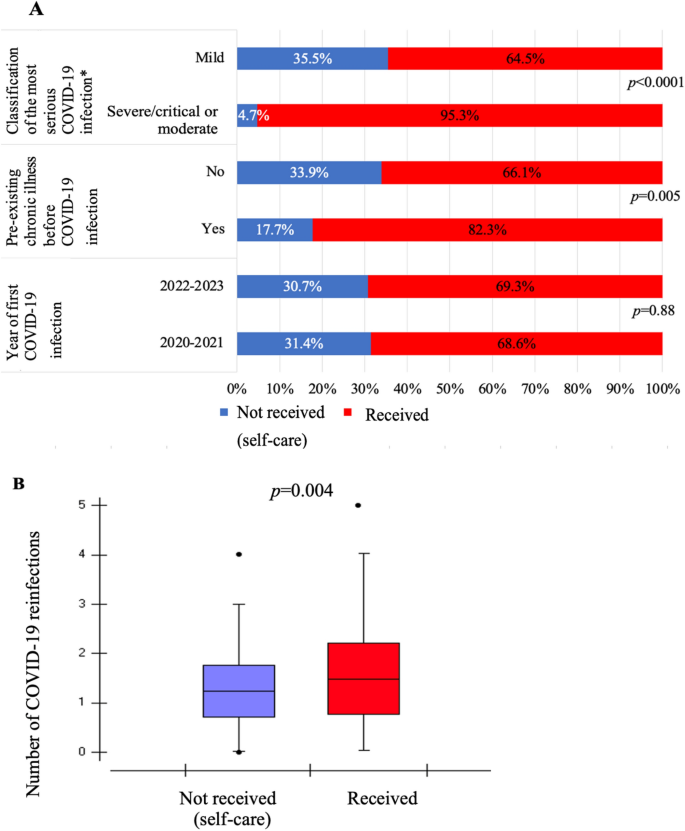
Associations between clinical characteristics, COVID-19 reinfections and access status to COVID-19 health care. Figure 2A—Year of diagnosis, diagnosis of chronic disease and classification of infection*. 2B—Number of COVID-19 reinfections (average). Pará. Brazil. 2023. *Classification of the most severe COVID-19 infection (Severe: was admitted to the intensive care unit or needed to be intubated/Moderate: had proven pneumonia or was hospitalized or needed oxygen support/Mild: respiratory symptoms, but no pneumonia or shortness of breath).
Figure 2 B illustrates the number of COVID-19 reinfections based on the utilization of health care. Among individuals who did not access health care (self-care), the mean number of reinfections was 1.29 (SD: 0.66), whereas among participants who received health care, the average was 1.47 (SD: 0.71) reinfections. The regression analysis demonstrated that the likelihood of receiving health care increased according to the number of COVID-19 reinfections (p = 0.004; OR: 1.48; 95% CI: 1.13, 1.95).
Figure 3 shows the association between the use of natural/alternative medications to treat COVID-19 and access to health care. Among these individuals, 631 participants responded to the inquiry regarding the utilization of herbal medicines and homemade tea, and 54.8% (347) chose not to use them, whereas 45.2% (286) reported of using these remedies. Individuals who used homemade tea or herbal remedies (p < 0.0001; OR: 0.54; 95% CI: 0.38, 0.76) were less likely to receive health care.
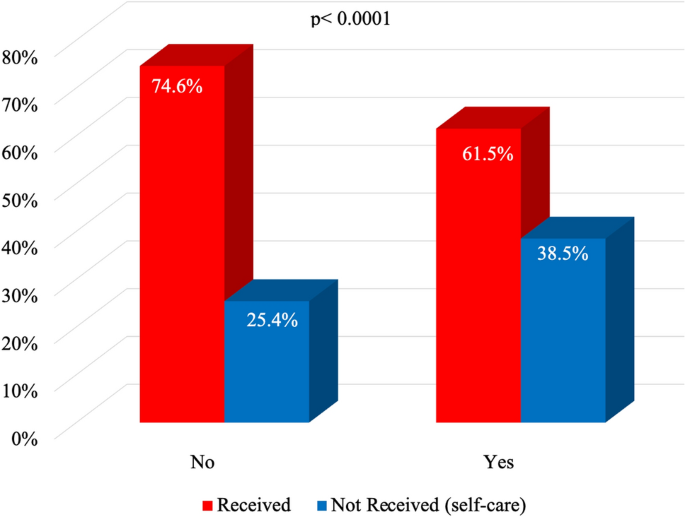
Association between herbal medicines/homemade tea and access to COVID-19 health care. Pará. Brazil. 2023.
Figure 4 presents the association between the COVID-19 vaccine and access to health care. Regarding the question about having received the COVID-19 vaccine, 1.9% (12/629) did not receive it, of whom 58.3% (7) received COVID-19 health care and 41.7% (5) did not have access to health care. Among the 98.1% (619/629) who received the vaccine, 68.8% (426) received health care, and 31.2% (193) received self-care (4A). Regarding having a COVID-19 infection before being vaccinated, 25.5% (156/610) received the vaccine before COVID-19 infection, of whom 69.2% (108) received health care. Moreover, among the 74.5% (456/610) who had COVID-19 infection before receiving the vaccine, 69.1% (315) received health care. There was no association between these variables and access to health care (Fig. 4 A).
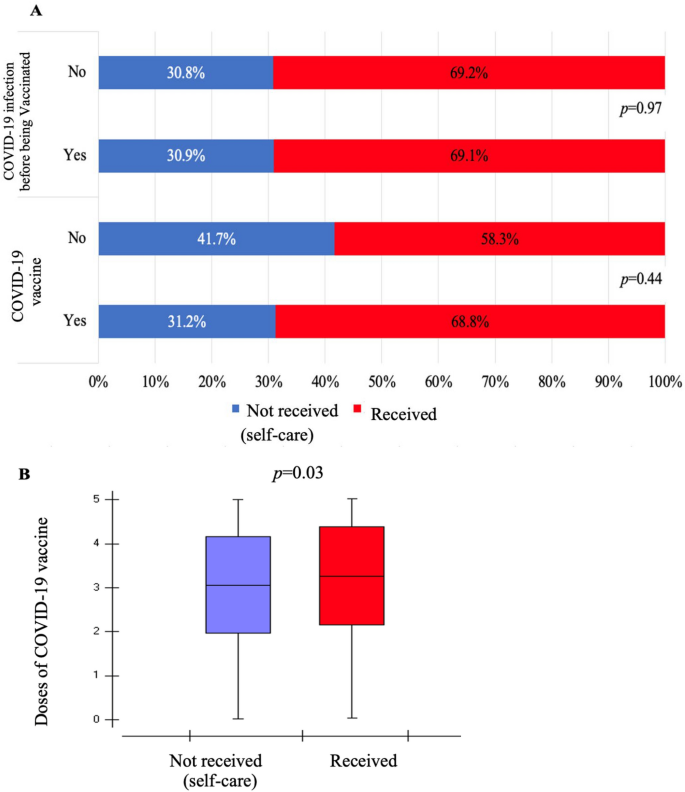
Association between COVID-19 vaccines and access to COVID-19 health care 4A—COVID-19 infection before being vaccinated and after receiving the COVID-19 vaccine. 4B—Number of doses of the COVID-19 vaccine. Pará. Brazil. 2023.
Figure 4 B displays the number of vaccine doses according to access to health care. A total of 615 participants answered the survey, with an average of 3.3 vaccine doses (SD: 0.94). Among those who did not access health care (self-care), the average was 3.18 vaccine doses (SD: 0.92), whereas the average was 3.35 doses (SD: 0.94) among participants who received health care. The regression analysis demonstrated that the likelihood of receiving COVID-19 health care increased with the number of COVID-19 vaccine doses (p = 0.03; OR: 1.22; 95% CI: 1.01, 1.46).
Sociodemographic and clinical characteristics: multiple logistic regression model
In the final model (Table 2 ), all of the variables that were selected in the previous step were analyzed together. The participants most likely to access health care were those with moderate or severe COVID-19 ( p = 0.000; OR: 19.8) and females ( p = 0.001; OR: 1.99). Moreover, participants who used homemade tea or herbal medicines were less likely to receive health care for COVID-19 in health services ( p = 0.002; OR: 0.54).
In this study, the concept of access to health services was used to examine the realized access (effective use of services) 19 and associated factors 19,21–22 . The results indicated that the individuals most likely to access COVID-19 health care were females and those who had moderate or severe COVID-19 infections. However, participants who used homemade tea or herbal medicines were less likely to access COVID-19 health care.
A high proportion of participants had access to COVID-19 health care. This result may be related to the characteristics of the sample but also to the universal health system in Brazil, which has a large capillarity through the PHC 12 .
This study considered the type of physical access in establishments of public and private health networks at all levels of health care and virtual access. The characteristics of the population are important aspects in studies on access, and such aspects can facilitate or be barriers to access 19 . In this study, the predisposing factors included education, age, ethnicity (traditional population), current job, marital status, and sex. The enabling factors included income, participation in social programs, residence in the capital city, and availability of health services such as vaccines, including doses and COVID-19 diagnosis. Need factors 19 included chronic conditions, the use of alcohol and tobacco, and mild to severe COVID-19.
The state of Pará encompasses several municipalities characterized by the poorest income, employment, and social indicators in Brazil 25–26 , thus leading to a disproportionate impact of COVID-19 on its population, particularly affecting vulnerable people 10–11 . The province of Pará has several cities characterized by the lowest income, employment and social indicators in Brazil 25–26 . These characteristics of the social and health structure are aspects that increase the population's risk for COVID-19 infection and its outcomes, particularly affecting vulnerable individuals 10–11 .
The specific characteristics of these municipalities and their populations played a significant role in both the spread of cases and the accessibility of health services during the pandemic. In these poor areas, residents experienced higher mortality rates from COVID-19. The failure to collect diagnostic samples was 3.07 times more frequent in these locations, and there was a 140% shortage in the availability of computed tomography scans 31 . A study conducted in Brazil highlighted an increased death rate among younger age groups in the most deprived municipalities, especially among individuals under 40-years-old who belonged to indigenous backgrounds. This observation led to the conclusion that levels of deprivation significantly hindered the prompt referral of patients to appropriate care 32 .
In the present study, multiple logistic regression analysis demonstrated that among the sociodemographic variables that were examined, only sex exhibited statistical significance within the final regression model. Notably, despite including other variables such as age, traditional population, educational level, residence in the capital, individual income, and marital status in this analysis, only sex was statistically significant.
Contrary findings have been observed in studies conducted across the Amazon region 9–11 . These studies, which were conducted during the initial and subsequent waves in Belém 10 , among the Waraó indigenous community 11 and in the population of Manaus 9 , did not establish a clear association between sex and the incidence of COVID-19. However, in Germany, there was an identified correlation between COVID-19 and male sex, particularly among symptomatic individuals who accessed care at health care facilities 33 . Similarly, in Australia, men display a greater risk of hospitalization than women 34 . In contrast, a study in Jordan reported that the majority of hospitalizations were among women 35 .
In Brazil, a study examining the risk factors associated with hospitalization and mortality due to demographic, clinical, and socioeconomic variables demonstrated that, in general, males exhibited greater odds of death than females did. However, postpartum patients who were admitted to hospital wards were found to have increased odds of mortality 32 .
Access to health care services by women is a facet of health system performance that goes beyond mere availability; it is contingent upon the effective utilization of these services. This access is influenced by various factors, including the societal roles and rights attributed to women, cultural and religious norms, and the structure of health care financing models 36–37 . A specific study identified several contributing factors to the lower access of women to health care services, COVID-19 levels of women's empowerment, financial access, and educational opportunities. This disparity has led to the underreporting of COVID-19 cases and fatalities among women 37 .
In Brazil, the universal model of the health care system, which offers free access to the entire population, serves as a mechanism to alleviate the barriers imposed by the necessity of payment for health care services 12 . Notably, women in this country tend to visit health services more frequently than men, whether for preventative treatments or nonchildbirth-related hospitalizations. Urban areas demonstrate greater coverage by health plans for women, whereas in rural regions, women tend to rely more on the public health system, and men tend to allocate more of their resources to healthcare 38–39 . An understanding of the exacerbation of sex inequalities during pandemics is crucial. Within a pandemic context, among various factors, the inaccessibility of health services can significantly heighten women's vulnerabilities during a crisis 40–41 . Multiple analyses of children with COVID-19 infection demonstrated that being under 10 years of age, being a black or mixed race/ethnicity, and having a health condition prior to COVID-19 were associated with a greater likelihood of hospitalization 42 .
The sample had a greater number of participants with a high level of education; specifically, most participants had a lower income, and 35.9% were currently studying. A high rate of access to health services was also found in a study with a sample of participants with high education and income. However, it was observed that a preexisting relationship with a primary care provider was critical to receiving access to a health care provider and medication during the COVID-19 pandemic 21 .
However, vaccination against COVID-19 and the number of doses were not significant in the final regression model. There is a high percentage of vaccinated people. This result may be related to the vaccination strategy that was adopted in the country, which began with priority groups and later expanded to the entire population. In this country, this specific protection measure has universal and free access, which is financed by the government. The history of vaccine acceptance in Brazil and mandatory vaccination for COVID-19 led to 70% coverage in 2021 and approximately 60% coverage in the Northernregion 30 .
In the current study, individuals demonstrating moderate or severe clinical manifestations of COVID-19 were more inclined to access health care. Previous research has indicated that systemic and pulmonary clinical manifestations are linked to hospitalizations 35,43 .
The clinical manifestations of COVID-19 infection are needed. The availability of resources, which represents an enabling factor, is closely related to the need factor 19 . This effect was also observed in a study performed in the United States of America, which demonstrated that demographic factors, such as ethnicity and language, were associated with reduced access to health care, more severe COVID-19 infection on admission and mortality. A high proportion of Hispanic participants who did not have a health record prior to hospitalization were observed compared to non-Hispanics 44 . A previous study demonstrated that among those hospitalized for COVID-19, a lack of health insurance also impacted the use of resources, such as oxygen and physiotherapy, for treatment and care after hospital discharge 45 .
Geographic accessibility also contributes to the utilization of health services. In severe COVID-19 infections, the type of health service and the availability of physical resources for treatment have a direct impact on access 22 . Addressing individuals with severe and moderate clinical manifestations of COVID-19 necessitates adequate infrastructure, essential equipment, supplies, and a competent cadre of health care professionals 35,43–45 . However, within the context of the present study, the northern region had the lowest number of beds per thousand inhabitants in Brazil during the COVID-19 pandemic. Despite this, it experienced the most significant average increase in intensive care unit beds across the country. Among the 27 Brazilian states, Pará ranked sixth for the state with the lowest number of intensive care unit beds and third for other beds, with 0.15 and 1.87 beds per 1,000 inhabitants, respectively. Additionally, it registered the lowest number of nurses per thousand inhabitants (1.03) in Brazil, and the Amazon region experienced the lowest number of medical doctors (2.02 per 1,000 inhabitants) 31 . The distribution of increased resources, such as beds and mechanical ventilators, was unequal in the Brazilian Amazon region and within states, with urban areas and capitals having more abundant access to these resources 5,43,46 . These disparities in health care provision significantly impact access to health care services 36 .
Acute COVID-19 infection, similar to chronic disease, does not depend on continued care over time. It is an acute, episodic infection for which resources are available for immediate treatment 42–45 . A previous study estimated that 41% of the vulnerable population in 20 cities of Brazil, including Belém, lives farther than 5 km from a health service to admit patients in severe condition due to COVID-19 infection. Health services with intensive care unit beds and ventilators, which are essential for treating severe COVID-19 infections, are more precarious in the peripheral areas of cities 22 .
This study demonstrated that the utilization of teas and herbal medicines was linked to a reduced likelihood of accessing health care services for the treatment of COVID-19. Inhabitants of the Amazon region regularly incorporate homemade teas and herbal remedies derived from local plants into their daily lives. These plants are utilized for various therapeutic purposes, thereby addressing conditions such as microbial infections, gastrointestinal disorders, and inflammation 47 . Similar practices are occurring globally, with populations elsewhere using medicinal plants for disease prevention and increasing consumption postdiagnosis in an effort to alleviate symptoms 48 . An understanding of the active ingredients present in plants that are used for therapeutic purposes is pivotal 49 . In this study, individuals exhibiting severe or moderate clinical manifestations also required and accessed health care within a health service. Therefore, identifying individuals or populations who are most in need of health care services is crucial for assisting in the preparation of health care network management and care protocols. Studies on access to health care have described the care provided by specialist physicians, nurses, general physicians, and health workers. The care provided by traditional healers was not described in these previous articles 20 .
Strengths and limitations
The utilization of the electronic questionnaire may have restricted the involvement of elderly individuals and those with lower levels of education. Furthermore, the nature of this study does not permit the establishment of a causal relationship between the assessed variables. The utilized sampling technique resulted in a sample that did not represent the education level of the population of Pará. However, it is important to know about access to care for COVID-19 infection in this group.
Conclusions
Ensuring access to health services is important in a pandemic scenario. Among the sociodemographic factors that were analyzed, only sex exhibited an association with access to COVID-19 health care. The study demonstrated that people with moderate to severe COVID-19 were more likely to access health care, whereas those who used natural/alternative medicines to prevent or treat COVID-19 were less likely to access health care. Knowledge of the factors associated with access to health care for patients with pandemic diseases is crucial for organizing the health system and identifying vulnerable individuals.
Data availability
The datasets used during this current study are also available from the corresponding author on reasonable request.
Hopkins, J. Coronavirus Resource Center Data Sources. https://github.com/govex/COVID-19/tree/master/data_tables/vaccine_data/global_data (2023).
Platto, S., Xue, T. & Carafoli, E. COVID-19: an announced pandemic. Cell Death Dis. 11 (9), 799. https://doi.org/10.1038/s41419-020-02995-9 (2020).
Article CAS PubMed PubMed Central Google Scholar
Moura, E. C. et al. Covid-19: temporal evolution and immunization in the three epidemiological waves, Brazil, 2020–2022. Revista de saude publica. 56 , 105. https://doi.org/10.11606/s1518-8787.2022056004907 (2022).
Article PubMed PubMed Central Google Scholar
Garnelo, L. et al. Barriers to access and organization of primary health care services for rural riverside populations in the Amazon. Int. J. Equity Health 19 (1), 54. https://doi.org/10.1186/s12939-020-01171-x (2020).
Bigoni, A. et al. Brazil’s health system functionality amidst the COVID-19 pandemic: An analysis of resilience. Lancet Reg. Health Am. 10 , 100222. https://doi.org/10.1016/j.lana.2022.100222 (2022).
Lima, R. T. S. et al. Health in sight: An analysis of Primary Health Care in riverside and rural Amazon areas. Cien Saude Colet. 26 (6), 2053–2064. https://doi.org/10.1590/1413-81232021266.02672021 (2021).
Article PubMed Google Scholar
Rodrigues, K. V., Almeida, P. F. & Fausto, M. C. Informal and popular healthcare subsystems in a remote rural municipality in the Brazilian Amazon region. Rural Remote Health 21 (3), 6568. https://doi.org/10.22605/RRH6568 (2021).
Sabino, E. C. et al. Resurgence of COVID-19 in Manaus, Brazil, despite high seroprevalence. Lancet 397 (10273), 452–455. https://doi.org/10.1016/S0140-6736(21)00183-5 (2021).
Lalwani, P. et al. SARS-CoV-2 seroprevalence and associated factors in Manaus, Brazil: baseline results from the DETECTCoV-19 cohort study. Int. J. Infect. Dis. 110 , 141–150. https://doi.org/10.1016/j.ijid.2021.07.017 (2021).
Torres, M. K. S. et al. Changes in the seroprevalence and risk factors between the first and second waves of COVID-19 in a metropolis in the Brazilian Amazon. Front. Cell Infect. Microbiol. https://doi.org/10.3389/fcimb.2022.932563 (2022).
Silva, H. P. et al. Migration in times of pandemic: SARS-CoV-2 infection among the Warao indigenous refugees in Belém, Pará, Amazonia Brazil. BMC Public Health 21 (1), 1659. https://doi.org/10.1186/s12889-021-11696-7 (2021).
Medina, M. G., Giovanella, L., Bousquet, A., Mendonça, M. H. M. & Aquino, R. Primary healthcare in times of COVID-19: What to do?. Cad Saude Publica. https://doi.org/10.1590/0102-311x00149720 (2020).
Boschiero, M. N., Palamim, C. V. C., Ortega, M. M., Mauch, R. M. & Marson, F. A. L. One year of coronavirus disease 2019 (COVID-19) in Brazil: A political and social overview. Ann Glob Health. 87 (1), 44. https://doi.org/10.5334/aogh.3182 (2021).
Oliveira, L. M. S., Gomes, N. P., Oliveira, E. S., Santos, A. A. & Pedreira, L. C. Coping strategy for COVID-19 in primary health care: Experience report in Salvador-BA. Rev. Gaucha Enferm. https://doi.org/10.1590/1983-1447.2021.20200138 (2021).
Tasca, R. et al. Managing Brazil’s health system at municipal level against covid-19: A preliminary analysis. Saúde debate 46 , 15–32. https://doi.org/10.1590/0103-11042022E101 (2022).
Article Google Scholar
Ferrante, L. et al. Brazil’s COVID-19 epicenter in manaus: How much of the population has already been exposed and are vulnerable to SARS-CoV-2?. J. Racial Ethn. Health Dis. 9 (6), 2098–2104. https://doi.org/10.1007/s40615-021-01148-8 (2022).
Ferrante, L. et al. Dynamics of COVID-19 in Amazonia: A history of government denialism and the risk of a third wave. Prev. Med. Rep. https://doi.org/10.1016/j.pmedr.2022.101752 (2022).
Ferrante, L. et al. Misinformation caused increased urban mobility and the end of social confinement before the second wave of COVID-19 in Amazonia. J. Racial Ethn. Health Dis. 24 , 1–6. https://doi.org/10.1007/s40615-023-01607-4 (2023).
Babitsch, B., Gohl, D. & von Lengerke, T. Re-revisiting Andersen’s Behavioral Model of Health Services Use: A systematic review of studies from 1998–2011. Psycho-Social Med. https://doi.org/10.3205/psm000089 (2012).
Núñez, A., Sreeganga, S. D. & Ramaprasad, A. Access to Healthcare during COVID-19. Int. J. Environ. Res. Public Health 18 (6), 2980. https://doi.org/10.3390/ijerph18062980 (2021).
Peckham, A. et al. Aging through the time of COVID-19: A survey of self-reported healthcare access. BMC Health Serv. Res. 21 (1), 1355. https://doi.org/10.1186/s12913-021-07353-9 (2021).
Pereira, R. H. M. et al. 2021 Geographic access to COVID-19 healthcare in Brazil using a balanced float catchment area approach. Soc. Sci.Med. https://doi.org/10.1016/j.socscimed.2021.113773 (2021).
Nacher, M. et al. The Epidemiology of COVID-19 in the Amazon and the Guianas: Similarities, differences, and international comparisons. Front. Public Health https://doi.org/10.3389/fpubh.2021.586299 (2021).
Gleriano, J. S., Fabro, G. C. R., Tomaz, W. B., Goulart, B. F. & Chaves, L. D. P. Reflections on the management of the Unified Health System for coordination in the fight against COVID-19. Esc. Anna Nery. https://doi.org/10.1590/2177-9465-EAN-2020-0188 (2020).
Brazil. Brazilian Institute of Geography and Statistics, https://cidades.ibge.gov.br/brasil/pa/panorama (2023).
Brazil . Ministry of Health. Primary health care indicator panel. https://sisaps.saude.gov.br/painelsaps/cobertura_aps (2023).
World Health Organization. Global COVID-19 clinical platform case report form (CRF) for post-COVID condition (post-COVID-19 CRF) . https://apps.who.int/iris/handle/10665/345298 (2021).
Brazil. National Policy for Comprehensive Health Care of Rural and Forest Populations https://bvsms.saude.gov.br/bvs/publicacoes/politica_nacional_saude_populacoes_campo.pdf (2007).
Gir, E. et al. Skin injuries associated with the use of N95 respirators among health professionals in Brazil in 2020. Revista Latino-Americana de Enfermagem. 31 , e3761. https://doi.org/10.1590/1518-8345.5937.3761 (2023).
Wejnert, C. & Heckathorn, D. D. Web-based network sampling: Efficiency and efficacy of respondent-driven sampling for online research. Sociol. Methods Res. 37 (1), 105–134. https://doi.org/10.1177/0049124108318333 (2008).
Article MathSciNet Google Scholar
Boing, A. F. et al. Area-level inequalities in COVID-19 outcomes in Brazil in 2020 and 2021: An analysis of 1,894,165 severe COVID-19 cases. Prev. Med. https://doi.org/10.1016/j.ypmed.2022.107298 (2022).
Pereira, F. A. C. et al. Profile of COVID-19 in Brazil-risk factors and socioeconomic vulnerability associated with disease outcome: Retrospective analysis of population-based registers. BMJ Glob Health https://doi.org/10.1136/bmjgh-2022-009489 (2022).
Jacob, L. et al. Prevalence of and factors associated with COVID-19 diagnosis in symptomatic patients followed in general practices in Germany between March 2020 and March 2021. Int. J. Infect. Dis. 111 , 37–42. https://doi.org/10.1016/j.ijid.2021.08.010 (2021).
Ellis, R. J. et al. Factors associated with hospitalizations and deaths of residential aged care residents with COVID-19 during the Omicron (BA.1) wave in Queensland. Med. J. 218 , 174–179. https://doi.org/10.5694/mja2.51813 (2023).
Alqaisi, R. O., Al-Kubaisy, W. A., Abughanam, S. N., Alfalayleh, A. Z. & Almasri, M. H. Risk factors and characteristics of hospitalized COVID-19 patients in Jordan. Saudi Med. J. 44 (10), 1054–1060. https://doi.org/10.15537/smj.2023.44.20230209 (2023).
Travassos, C. & Martins, M. Uma revisão sobre os conceitos de acesso e utilização de serviços de saúde. Cadernos De Saúde Pública 20 , S190–S198. https://doi.org/10.1590/S0102-311X2004000800014 (2004).
Aleksanyan, Y. & Weinman, J. P. Women, men and COVID-19. Soc. Sci. Med. https://doi.org/10.1016/j.socscimed.2022.114698 (2022).
Pinheiro, R. S., Viacava, F., Travassos, C. & dos Brito, A. S. Gênero, morbidade, acesso e utilização de serviços de saúde no Brasil. Ciênc saúde coletiva 7 (4), 687–707. https://doi.org/10.1590/S1413-8123200200040000 (2002).
Cobo, B., Cruz, C. & Dick, P. C. Desigualdades de gênero e raciais no acesso e uso dos serviços de atenção primária à saúde no Brasil. Ciência Saúde Coletiva. 26 , 4021–4032. https://doi.org/10.1590/1413-81232021269.05732021 (2021).
Simba, H. & Ngcobo, S. Are Pandemics Gender Neutral? Women’s Health and COVID-19. Front. Glob. Womens Health https://doi.org/10.3389/fgwh.2020.570666 (2020).
Su, Z. et al. Gender inequality and health disparity amid COVID-19. Nurs. Outlook 70 (1), 89–95. https://doi.org/10.1016/j.outlook.2021.08.004 (2022).
Moreira, A. et al. Demographic predictors of hospitalization and mortality in US children with COVID-19. Eur. J. Pediatr. 180 (5), 1659–1663. https://doi.org/10.1007/s00431-021-03955-x (2021).
Turan, O. et al. Characteristics of hospitalised COVID-19 patients and parameters associated with severe pneumonia. Int. J. Clin. Pract. 75 (11), e14786. https://doi.org/10.1111/ijcp.14786 (2021).
Article CAS PubMed Google Scholar
Velasco, F. et al. Association of healthcare access with intensive care unit utilization and mortality in patients of hispanic ethnicity hospitalized with COVID-19. J. Hospital Med. 16 (11), 659–666. https://doi.org/10.12788/jhm.3717 (2021).
Cervantes, L. et al. Experiences of Latinx individuals hospitalized for COVID-19: A qualitative study. JAMA Netw. Open 4 (3), e210684. https://doi.org/10.1001/jamanetworkopen.2021.0684 (2021).
Rezende, A. A. B. et al. Distribution of COVID-19 cases and health resources in Brazil’s Amazon region: a spatial analysis. Cien Saude Colet. 28 (1), 131–141. https://doi.org/10.1590/1413-81232023281.10782022 (2023).
Sarquis, R. S. F. R. et al. The use of medicinal plants in the riverside community of the mazagão river in the Brazilian amazon, Amapá, Brazil: Ethnobotanical and Ethnopharmacological studies. Evid Based Complement Alternat Med. 2019 , 6087509. https://doi.org/10.1155/2019/6087509 (2019).
Tarus, H. A., Yalazı, R. Ö., Karadeniz, H. & Can Gürkan, Ö. Traditional and complementary medicines methods used by patients diagnosed with COVID-19. Complement Med. Res. 30 (1), 19–25. https://doi.org/10.1159/000527311 (2023).
Das, A. et al. Role of phytoconstituents in the management of COVID-19. Chem Biol. Interact. https://doi.org/10.1016/j.cbi.2021.109449 (2021).
Download references
The present study was carried out with support from Emergency selection IV No. 12/2021 of the Postgraduate Development Program – Impacts of the Pandemic belonging to the Coordenação de Aperfeiçoamento de Pessoal de Nível Superior (CAPES) and Pró-reitoria de Pesquisa e Pós- graduação of the Federal University of Para, Support Program for qualified production -2024 (PAPQ-2024, in Portuguese).
Author information
Authors and affiliations.
Programa de Pós-Graduação Em Enfermagem, Universidade Federal Do Pará, Belém, 66075-110, Brasil
Amanda Loyse da Costa Miranda, Ana Rosa Tavares da Paixão, Andressa Tavares Parente, Eliã Pinheiro Botelho, Sandra Helena Isse Polaro & Glenda Roberta Oliveira Naiff Ferreira
Escola de Enfermagem de Ribeirão Preto, Universidade de São Paulo, Ribeirão Preto, 14040-092, Brasil
Andrey Oeiras Pedroso, Laís do Espírito Santo Lima & Renata Karina Reis
Programa de Pós-Graduação Em Enfermagem, Universidade Federal da Paraíba, João Pessoa, 58051-900, Brasil
Ana Cristina de Oliveira e Silva
You can also search for this author in PubMed Google Scholar
Contributions
ALCM; RKR; ACOS and GRONF: conceptualization (equal), data curation, formal analysis, funding acquisition, methodology (lead). GRONF, SHIP, ATP and EPB visualization, writing, and editing (lead). RKR: Project administration (lead), funding acquisition (lead). ALCM, ARTP, AOP, LESL: Investigation (equal) and writing review editing (equal). GRONF and ALCM: methodology, resources, supervision, writing, and editing (equal). All authors have read and agreed to the published version of the manuscript.
Corresponding author
Correspondence to Glenda Roberta Oliveira Naiff Ferreira .
Ethics declarations
Competing interests.
The authors declare no competing interests.
Additional information
Publisher's note.
Springer Nature remains neutral with regard to jurisdictional claims in published maps and institutional affiliations.
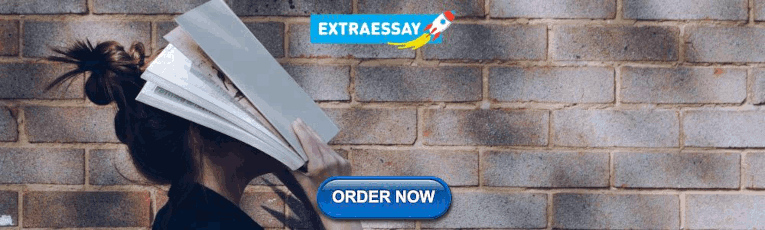
Rights and permissions
Open Access This article is licensed under a Creative Commons Attribution 4.0 International License, which permits use, sharing, adaptation, distribution and reproduction in any medium or format, as long as you give appropriate credit to the original author(s) and the source, provide a link to the Creative Commons licence, and indicate if changes were made. The images or other third party material in this article are included in the article's Creative Commons licence, unless indicated otherwise in a credit line to the material. If material is not included in the article's Creative Commons licence and your intended use is not permitted by statutory regulation or exceeds the permitted use, you will need to obtain permission directly from the copyright holder. To view a copy of this licence, visit http://creativecommons.org/licenses/by/4.0/ .
Reprints and permissions
About this article
Cite this article.
da Costa Miranda, A.L., da Paixão, A.R.T., Pedroso, A.O. et al. Demographic, social, and clinical aspects associated with access to COVID-19 health care in Pará province, Brazilian Amazon. Sci Rep 14 , 8776 (2024). https://doi.org/10.1038/s41598-024-59461-1
Download citation
Received : 10 October 2023
Accepted : 10 April 2024
Published : 16 April 2024
DOI : https://doi.org/10.1038/s41598-024-59461-1
Share this article
Anyone you share the following link with will be able to read this content:
Sorry, a shareable link is not currently available for this article.
Provided by the Springer Nature SharedIt content-sharing initiative
By submitting a comment you agree to abide by our Terms and Community Guidelines . If you find something abusive or that does not comply with our terms or guidelines please flag it as inappropriate.
Quick links
- Explore articles by subject
- Guide to authors
- Editorial policies
Sign up for the Nature Briefing newsletter — what matters in science, free to your inbox daily.

- Utility Menu

e70cb3e53ae46848cd8acc1d8e891f8b
Committee on the use of human subjects.
Harvard University Area Institutional Review Board
- Human Research Protection Program Plan
- Data Security
- GDPR Privacy Disclosure
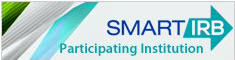
Questions About COVID-19 and Your Research
June 28, 2021
As the COVID-19 pandemic continues to evolve, various Harvard restrictions and processes that were in place have now been re-visited. Moving forward, take the following into consideration when conducting research with human subjects:
- All research and on-campus activity, should still follow Harvard and local school/department guidelines. For instance, this means that personnel who have not yet been authorized to work on campus will need to check with their departments (or divisions or schools) about the appropriate process to obtain authorization prior to the August 2 nd general return date.
- the most up to date University guidance
- EHS COVID-19 resources specifically for laboratories & research
- SEAS & FAS Division of Science: Coronavirus FAQs
- FAS Social Science COVID19 FAQs
- Note that Harvard continues to require masks indoors, regardless of vaccination status. This guidance will be updated as Harvard requirements and local community incidence changes. Please also check local school or division for more local guidance.
- Some previous restrictions placed on human subjects research have been lifted. Currently physical distancing is no longer required for those vaccinated in all research locations. Please check with your school/division/department for any local guidelines.
- Irrespective of location, individuals interacting with human subjects are advised to continue to wear surgical masks as vaccination status of study participants cannot be assumed. Research study personnel should let participants decide if they would like to wear a mask if the study procedures allow it.
- Researchers may wish to inform their study population of the current COVID-19 status on campus , or in the study location if elsewhere , including level of community transmission of COVID-19 and COVID-19 vaccination coverage. It continues to be recommended that those that are unvaccinated and at high-risk for COVID-19 complications or immunocompromised not take part in research.
- It is recommended that researchers conduct a brief screening with study participants prior to the study visit. Sample questions may be found below.
- Other restrictions such as those involving travel and working in other locations or at other institutions are still in place. It is important for researchers to follow any guidelines or instructions from the specific facility or location where in-person research would occur. As some research may occur in another state, with another institution, or under the direction of another IRB (as in a reliance agreement situation), this is especially important. It is the responsibility of the Study Team to keep apprised of potential restrictions and conduct their study accordingly.
- Researchers planning to hire professional companies (survey organization and the like) in other states or countries should do due diligence to make sure that these organizations are taking reasonable coronavirus precautions and are fully aware of local conditions and government restrictions. In particular, Harvard should not be asking these organizations to be engaging in practices that are riskier than their normal business operations.
As restrictions relax, there are still ongoing public health best practices and guidelines to pay attention to:
- Practice safe distancing, when necessary and appropriate.
- Wash or sanitize your hands often and continue respiratory etiquette.
- Perform disinfection of frequently touched objects and surfaces.
Harvard has upgraded its ventilation systems, including installing HEPA filtration units as needed, and will continue to routinely assess airflow. Should you have any questions, please check with your department administrator or building manager. For research off-campus, please be aware that a ventilated space (particularly with open windows) is safer than an unventilated one.
You may access the archive of COVID-19 research information, research restrictions and processes here - https://cuhs.harvard.edu/questions-about-covid-19-and-your-research-ARCHIVE
Sample questions for COVID-19 screening https://projects.iq.harvard.edu/files/scictr/files/crimson_clear_paperform_as_of_06072020.pdf
Q1: ARE YOU EXPERIENCING ANY OF THE FOLLOWING SYMPTOMS? (CHECK ALL THAT APPLY)
☐ Fever, chills, or feeling feverish
☐ New cough (not related to chronic condition)
☐ Shortness of breath or difficulty breathing
☐ New fatigue
☐ Muscle or body aches
☐ New headache
☐ New loss of taste or smell
☐ Sore throat
☐ New nasal congestion or new runny nose (not related to seasonal allergies)
☐ Nausea or vomiting
☐ Diarrhea
If ANY of the above apply, inform the study participant that they will not be permitted to come to the study visit on that day and that they should contact their health care provider. For medical emergencies, call 911.
If NONE of the above apply, proceed to Q2
Q2: IN THE LAST 14 DAYS, HAVE YOU BEEN IN CLOSE CONTACT WITH ANYONE WHO HAS TESTED POSITIVE FOR COVID-19? (CHECK BOX)
If Yes, the study participant believes that they were exposed to a confirmed case of COVID-19, inform them that they will not be permitted to attend the study visit on that day and that they should contact their health care provider. For medical emergencies, call 911.
If No, inform study participant that they may come to the study visit. Remind them to always wear a facemask as well as any location or study specific information that they should know about.
Click through the PLOS taxonomy to find articles in your field.
For more information about PLOS Subject Areas, click here .
Loading metrics
Open Access
Peer-reviewed
Research Article
Impacts of COVID-19 on clinical research in the UK: A multi-method qualitative case study
Roles Conceptualization, Data curation, Formal analysis, Investigation, Methodology, Project administration, Validation, Writing – original draft, Writing – review & editing
* E-mail: [email protected]
Affiliations School of Population Health and Environmental Sciences, King’s College London, United Kingdom, National Institute for Health Research Biomedical Research Centre at Guy’s and St. Thomas’ NHS Foundation Trust and King’s College London, United Kingdom

Roles Data curation, Formal analysis, Investigation, Methodology, Project administration, Resources, Validation, Writing – original draft, Writing – review & editing
Roles Conceptualization, Funding acquisition, Writing – review & editing
- David Wyatt,
- Rachel Faulkner-Gurstein,
- Hannah Cowan,
- Charles D. A. Wolfe
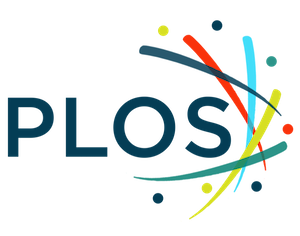
- Published: August 31, 2021
- https://doi.org/10.1371/journal.pone.0256871
- Peer Review
- Reader Comments
Clinical research has been central to the global response to COVID-19, and the United Kingdom (UK), with its research system embedded within the National Health Service (NHS), has been singled out globally for the scale and speed of its COVID-19 research response. This paper explores the impacts of COVID-19 on clinical research in an NHS Trust and how the embedded research system was adapted and repurposed to support the COVID-19 response.
Methods and findings
Using a multi-method qualitative case study of a research-intensive NHS Trust in London UK, we collected data through a questionnaire (n = 170) and semi-structured interviews (n = 24) with research staff working in four areas: research governance; research leadership; research delivery; and patient and public involvement. We also observed key NHS Trust research prioritisation meetings (40 hours) and PPI activity (4.5 hours) and analysed documents produced by the Trust and national organisation relating to COVID-19 research. Data were analysed for a descriptive account of the Trust’s COVID-19 research response and research staff’s experiences. Data were then analysed thematically. Our analysis identifies three core themes: centralisation; pace of work; and new (temporary) work practices. By centralising research prioritisation at both national and Trust levels, halting non-COVID-19 research and redeploying research staff, an increased pace in the setup and delivery of COVID-19-related research was possible. National and Trust-level responses also led to widescale changes in working practices by adapting protocols and developing local processes to maintain and deliver research. These were effective practical solutions borne out of necessity and point to how the research system was able to adapt to the requirements of the pandemic.
The Trust and national COVID-19 response entailed a rapid large-scale reorganisation of research staff, research infrastructures and research priorities. The Trust’s local processes that enabled them to enact national policy prioritising COVID-19 research worked well, especially in managing finite resources, and also demonstrate the importance and adaptability of the research workforce. Such findings are useful as we consider how to adapt our healthcare delivery and research practices both at the national and global level for the future. However, as the pandemic continues, research leaders and policymakers must also take into account the short and long term impact of COVID-19 prioritisation on non-COVID-19 health research and the toll of the emergency response on research staff.
Citation: Wyatt D, Faulkner-Gurstein R, Cowan H, Wolfe CDA (2021) Impacts of COVID-19 on clinical research in the UK: A multi-method qualitative case study. PLoS ONE 16(8): e0256871. https://doi.org/10.1371/journal.pone.0256871
Editor: Quinn Grundy, University of Toronto, CANADA
Received: April 14, 2021; Accepted: August 17, 2021; Published: August 31, 2021
Copyright: © 2021 Wyatt et al. This is an open access article distributed under the terms of the Creative Commons Attribution License , which permits unrestricted use, distribution, and reproduction in any medium, provided the original author and source are credited.
Data Availability: Data from this study take the form of interview transcripts, Hospital Trust and national documents, and observations of closed meetings. These data cannot be shared publicly, but extracts from interviews are presented within the body of the paper that make up the "minimal dataset."
Funding: DW, RFG, HC and CADW are all funded by the National Institute for Health Research ( http://nihr.ac.uk/ ) Biomedical Research Centre at Guy’s and St Thomas’ NHS Foundation Trust and King’s College London (Grant number IS‐BRC‐1215‐20006). The funder had no role in study design, data collection and analysis, decision to publish, or preparation of the manuscript. The views expressed are those of the authors and not necessarily those of the NHS, the NIHR or the Department of Health and Social Care.
Competing interests: No
Introduction
Clinical research is a core part of the global response to COVID-19. The United Kingdom (UK), with its research system embedded within the National Health Service (NHS), has been singled out by commentators globally for the scale and speed of its COVID-19 research response, particularly in terms of trial recruitment [ 1 – 3 ]. Reporting from within the UK context, Darzi et al. suggest that participating in clinical trials should be part of the clinical pathway for all COVID-19 patients [ 4 ]. To date, 95 nationally prioritised COVID-19 research projects, labelled Urgent Public Health studies, have commenced [ 5 ]. These and a large number of other COVID-19 studies have rapidly been set up and rolled out across UK hospitals. Supporting and facilitating such research has been made possible by the widespread reorganisation of the NHS’ existing embedded research infrastructure. This reorganisation was initiated by the UK’s Department Health and Social Care (DHSC), which on 16 th March 2020 stated that all National Institute for Health Research (NIHR) funded staff should “prioritise nationally-sponsored COVID-19 research activity” [ 6 ]. They later clarified, stating “the NIHR Clinical Research Network is pausing the site set up of any new or ongoing studies at NHS and social care sites that are not nationally prioritised COVID-19 studies [ 6 ].” Such decisions were said to “enable our research workforce to focus on delivering the nationally prioritised COVID-19 studies or enable redeployment to frontline care where necessary [ 6 ].” To date, reports have focused on the outputs of this research, such as the outcomes of vaccine studies or results of treatment trials, and on frontline clinical staffing, healthcare provision and resource strains faced by hospitals and health care systems at national and global levels [ 7 – 12 ]. As yet, there has been no analysis of the organisation of the research response and the broader impact of the reorganisation of hospitals and research facilities that has allowed clinical research and emergency care work to take place during the pandemic.
In this paper we provide a detailed exploration of how the embedded research infrastructure in one NHS Trust in South London. Throughout this paper, we e use the pseudonym South London Acute Trust (SLAT) to avoid direct identification. This Trust was repurposed to support the completion of COVID-19 research and delivery of frontline care. SLAT is one of the UK’s most research-active Trusts, annually recruiting over 19,000 patients to more than 550 studies. Between February and December 2020, SLAT opened over 80 COVID-19 studies, with more than 18 of these classed as Urgent Public Health studies, recruiting over 7,000 participants. Within this context, we ask: what have been the impacts of COVID-19 on SLAT’s clinical research system, and how has the embedded research system been adapted and repurposed to support the COVID-19 response?
Prior to the pandemic, the process of setting up and managing a clinical research study within a UK NHS Trust involved multiple steps and several actors. Decisions on whether or not to open specific studies rested primarily with the relevant clinical directorate who would vet the study for its appropriateness, scientific merit and feasibility. Other processes were centralised by the Trust’s Research and Development (R&D) governance office, like the sponsorship review (that is, deciding whether the Trust will take responsibility for the study and study compliance) or assisting researchers to gain approvals from national regulatory bodies like the Medicines and Healthcare products Regulatory Agency (MHRA) and the Health Research Authority (HRA). With approvals in place, R&D would then assess whether sufficient resources were available to support the study (the capacity and capability review). Completing this process was often both onerous and time consuming. As a result of the COVID-19 pandemic, substantial parts of this process were reconfigured, as we detail below.
This is a case study of how the embedded research infrastructure at one NHS Trust was repurposed to support the delivery of frontline care and COVID-19 research. The case study method allowed us to track how the research system was adapting in real time, and enabled an in-depth look at the processes and mechanisms that have underpinned operational changes [ 13 ]. As an instrumental case study, one that focuses on socially, historically and politically situated issues, we use a single site to examine issues that are also faced by other hospital Trusts [ 14 ]. We employed an online questionnaire of research-involved staff, document analysis of emails and official national and Trust documents, observations of planning meetings and semi-structured interviews. Data were collected from individuals working in four levels of the research infrastructure: (1) central research oversight and governance (including R&D leads and research governance staff); (2) principal investigators (PIs); (3) the research delivery workforce (including research nurses, clinical research practitioners, data analysts and research managers); and (4) Patient and Public Involvement (PPI) managers and PPI representatives. Triangulating these four data sources and four levels allowed us to consider the representativeness of our data across the case. Redeployment figures and wider workforce information were provided through a request to SLAT’s research management office.
Sampling and data collection
Data were collected by DW, RFG and HC over a period of six months, from May to October 2020. In the first stage of research, an online questionnaire was disseminated to all research-involved staff at SLAT (approx. 700) on 18 th May 2020 via pre-existing mailing lists. The questionnaire closed on 10 th June 2020 with 170 responses, yielding a response rate of approximately 24%. Whilst 24% would be an inadequate response rate for statistical analysis [ 15 ], it was not intended as a validated survey, but rather a method to gain a broad understanding of staff’s experiences of the COVID-19 research response, with most questions open-ended. We received completed questionnaires from nearly a quarter of research staff during the pandemic. The questionnaire also enabled us to identify and recruit a maximum variation sample of staff involved in the research response across the four groups to interview. Interviews allowed us to explore in more depth some of the recurring themes first identified in the questionnaire.
Interview participants were also recruited using purposive and snowball sampling with an aim to maximise the representation of a variety of experiences across the case [ 16 ]. Key staff within SLAT were identified based on searching the Trust’s website, reviewing staff lists and by speaking to senior personnel for guidance. Interviews were conducted digitally on Microsoft Teams and were recorded and transcribed verbatim. Interviews focused on participants’ work prior to the pandemic, how this work has changed as a result of COVID-19, and the short and long term impacts of COVID-19 on health research more broadly.
Additionally, we obtained permission to observe the regular research prioritisation meetings convened by the Trust’s Director of R&D. These meetings took place over Microsoft Teams once or twice a week and were attended by an average of 10 senior clinical, research and research delivery leaders per session. We attended the meetings as non-participant observers, taking notes and recording proceedings. Recordings were transcribed verbatim. We also analysed all documents that were produced or circulated in connection to the prioritisation meetings. These included email discussions about specific projects, national directives, Trust protocols as well as the applications submitted by investigators to the prioritisation committee.
Lastly, we attended the handful of PPI meetings that were held by the few active PPI groups during this period. We participated in discussions about specific research projects and heard participants’ experiences of PPI during the pandemic. PPI is a core part of the pre-COVID-19 research and research design process [ 17 ]. It was therefore important that changes to PPI were considered within our study. We were also able to present our research and get feedback from groups about our aims. PPI meetings were not recorded, but detailed notes were taken during each session.
Conducting qualitative research during the COVID-19 pandemic has required us to adapt data collection methods to accommodate restrictions on face-to-face meetings and access to the hospital. Studies note that while video conferencing has many benefits, issues such as the familiarity of participants with online platforms and access to technology and high-speed internet can be barriers to the successful use of these technologies in interviewing [ 18 , 19 ]. We experienced only a handful of technical problems in our interviews. In all but two instances, interviews were conducted with cameras on so that we could observe non-verbal communication [ 20 ].
Our data were managed and analysed through NVivo 12 using a two stage process [ 21 ]. In the first stage, we analysed the data for a descriptive and narrative account, paying attention to the contours of the emerging response to COVID-19, including national and Trust decision-making and action [ 22 ]. In the second stage we used thematic analysis to develop an analytic account based on emerging themes [ 21 , 23 ]. Data were coded for key themes independently by DW, RFG and HC iteratively throughout the data collection process. Codes and core themes were then discussed and verified across the researchers. As part of our analysis process, we also presented initial findings to research staff at SLAT and at another NHS Trust. These methods of challenging our analysis both internally and externally were crucial for ensuring we reflected on our own influences on the data and the data’s utility beyond our specific case [ 24 ].
Ethics approval for the study was granted by North East—Newcastle & North Tyneside 2 REC (reference: 20/NE/0138).
We completed 24 interviews, lasting from 24 to 105 minutes (mean average of 52 minutes), observed approximately 40 hours of research prioritisation meetings and 4.5 hours of PPI meetings, and received 170 responses to the questionnaire. In the results that follow our interview participants are divided into four groups. We identify participants using a letter to denote group and number for interview within this group:—G-n (Governance/R&D staff), R-n (Research leaders/PIs), D-n (Research delivery staff), P-n (PPI managers). 3 participants sit in more than one of these groups due to their multiple roles within the Trust. These participants were interviewed using questions from interview guides for all relevant groups. Questionnaire participants are identified as Q-n, followed by a brief description of their role. See Tables 1 and 2 for a breakdown of participants.
- PPT PowerPoint slide
- PNG larger image
- TIFF original image
https://doi.org/10.1371/journal.pone.0256871.t001
https://doi.org/10.1371/journal.pone.0256871.t002
Centralisation: Prioritising COVID-19 research and redeploying research staff
Centralisation within the research apparatus occurred across two levels.
National decision-making.
At the outset of the pandemic, DHSC took steps to assert central control over national research priorities in order to coordinate the national response to COVID-19. This included the shut down or partial shutdown of the normal functioning of the research system. A document circulated throughout the NHS on the 13 th March 2020, which included information from 25 separate Trusts, announced that elements of the UK’s national R&D infrastructure, including the UK Clinical Research Facilities (CRF) and NIHR Clinical Research Network (NIHR CRN) Coordinating Centre were “joining up working to ensure consistency of approach” and that “currently UK NIHR/RC and EU research funding bodies are in the process of selecting research that will be prioritised for approval and delivery across the NHS during the pandemic.” On 16 th March 2020 a directive from the DHSC and the Chief Medical Officer (CMO) ordered the suspension of all non-COVID-19-related research and the reorientation of research capacity towards the effort to develop COVID-19 treatments and vaccines [ 6 ]. Only those studies funded by the NIHR and where “discontinuing them will have significant detrimental effects on the ongoing care of individual participants involved in those studies” were allowed to continue [ 6 ]—in short, those studies where research was the standard of care, for example, with experimental cancer treatments. Decisions on which studies met this threshold were decided at the Trust level. Table 3 documents the scale of the pause in the normal research pipeline at SLAT. Participant G-2 saw this DHSC and CMO directive as an effective way to focus research resources:
I think the really helpful bit was the sort of diktat from Chris Whitty and Louise Wood at DH [Department of Health and Social Care] to say, “Stop everything that’s not COVID.” […] So, to actually have something centrally that said, “No, you’re not actually allowed to do that because we’ve got to focus on the COVID stuff,” was very helpful because people just stopped asking–which was great. And we were freed up to change processes as we needed to.
https://doi.org/10.1371/journal.pone.0256871.t003
Following this directive, a new system of badging certain studies as of Urgent Public Health (UPH) was established, run by DHSC and the CMO. All clinical studies including COVID-19 treatment and vaccine trials that hoped to recruit patients within NHS sites were required to apply for UPH status. An Urgent Public Health Group was convened, chaired by Nick Lemoine, the medical director of the NIHR CRN. The group was responsible for deciding which protocols to label UPH, based on evaluations of scientific merit, feasibility and greatest potential patient benefit [ 25 , 26 ]. Of the 1600 research protocols received by the CMO from March 2020 to February 2021, only 83 were considered national priorities [ 5 , 27 ]. Once a study had received UPH badging, hospital sites like SLAT were required to open them, if resources were available.
This centrally-organised prioritisation of COVID-19-related research removed the authority of individual Trusts and directorates to shape their own research portfolios. This was an unprecedented move by the DHSC, but allowed resources to be concentrated on studies deemed to have the greatest potential impact.
Trust-level decision-making.
In order to enact the DHSC mandate to prioritise COVID-19 research, SLAT created a Trust-level prioritisation process. Twice-weekly prioritisation meetings commenced early April 2020 and were attended by research governance managers, research delivery managers and senior clinicians as well as representatives from the local Clinical Research Network and partner hospitals within the network. The aim of the prioritisation meetings was to protect resources and ensure capacity to undertake UPH-badged research. However, it also ensured effective, timely communication with PIs, helped identify local PIs for new COVID-19 studies led elsewhere, and managed the pause and restart of all non-COVID research. A proforma was introduced to facilitate and standardise prioritisation decision-making. Investigators were asked to provide information summarising their projects, resource requirements and whether they had received UPH badging. Proformas were reviewed during these meetings. By the end of February 2021, this group had reviewed 170 research projects using these proformas across 68 meetings, approving over 80 studies for local setup.
During the first wave of the pandemic, prioritisation group meetings focused mainly on how to open UPH-badged studies, as all other new research had been halted. One important exception was COVID-19 studies that require little or no NHS resource and took place within a single NHS site. These studies were also discussed in these prioritisation group meetings, often with a focus placed on clinical and academic merit. Most of the studies that fitted these criteria and were approved by the prioritisation group involved university researchers analysing patient data collected and pooled in the COVID patient ‘data lake’. This enabled the Trust to maintain research activity in areas not explicitly identified as urgent public health. The research reported in this article was approved through this process.
The joined up approach between national and local decision-making however did cause confusion and frustration. The process of determining whether or not a study would be badged UPH and thus allowed to proceed was initially opaque to Trust researchers and R&D, and the national UPH review process often took weeks from application submission to outcome. Furthermore, the decision to grant a study UPH was and remains out of the hands of the sites that are tasked with delivering this research, even when internally questions were raised about the appropriateness, feasibility or scientific merit of the study. Some researchers designing studies to address key issues in relation to COVID-19 struggled to negotiate the system:
In terms of national COVID studies, we tried to get a number of studies up and going, focusing on older patients. And ran into quite a lot of obstacles and barriers. [..P]eople weren’t certain whether this was research or whether it was quality improvement, audit-type, survey-type work. And that was pretty frustrating, not being able to get clear answers on that from the senior team within R&D. And access to data was very difficult. So, despite lots of conversations about why we really needed to be focusing on older patients, the majority of people with COVID, the biggest impact being in care homes, it was quite frustrating getting hold of people who could actually sign off on studies that we would have like to have done (R-7).
At the Trust level, the prioritisation of research was also important because of the reduction in available research delivery staff. As Table 4 documents, the clinical research delivery workforce, which totalled 165 on 14 th April 2020, was reduced by 79% or 131 staff members during the peak of the first wave due to redeployment to frontline care. A further 52 non-clinical research staff were redeployed to support other Trust activity. With such a reduction of staff, the ability to maintain even those studies which had not been halted was not certain and indeed many studies required changes and protocol deviations as a result. A key point of discussion in all prioritisation meetings was the resourcing requirements of proposed studies and how these requirements might be managed alongside existing commitments. In tandem with these discussions, work was done by the research delivery manager to create a central register of research delivery staff within the Trust. The push to centralise oversite of research delivery staff was initially driven by the requirement to rapidly redeploy staff including nurses and clinical trials practitioners to support the Trust’s emergency response but it was also crucial to the prioritisation group’s understanding of the availability of research resources. Prior to the pandemic, there was no central list of all research delivery staff at the Trust, as D-2 discusses:
A benefit was actually establishing who all the staff are. The systems we have in R&D which relate to where staff sit within the Trust system depends on where they’re funded from. And because research teams have lots of mixed types of funding, some of the staff are visible to me through the systems and some aren’t. So, the only way for me to know who all the staff were, was to manually myself, physically ask. There was no system anywhere that listed who the research staff are.
https://doi.org/10.1371/journal.pone.0256871.t004
In addition to being redeployed to the clinical frontline, research staff were also pulled from across the Trust’s many directorates to form a new dedicated COVID-19 research delivery team. This team became responsible for the rapid set up and roll out of COVID studies of national and international importance, like the Oxford AstraZeneca vaccine trial, among others. Centralising oversight and management of the previously dispersed research delivery workforce enabled SLAT’s research system to react quickly and flexibly to the rapidly evolving clinical demands and research requirements of the pandemic.
While research activity was centrally coordinated within SLAT, R&D were initially left out of Trust emergency planning. An organogram produced by the Trust to represent its emergency response plan did not include R&D or any element of the research system, and a briefing document prepared by SLAT R&D for the Trust’s Gold Tactical Command Unit dated 14 th April 2020 noted this absence, and that there was also no “obvious place in the structure for R&D to naturally sit.” Participant G-3 reflected on what was perceived initially as a failure to consider the role of research:
I think […] the Trust essentially, corporately, hadn’t involved the R&D department in what they were thinking. […] We didn’t have a tactical subgroup where everybody else, every other area in the Trust had a tactical subgroup. […] There was nothing in place. You know, we’ve all voiced this, certainly in meetings at the senior management level–is that, and the words used were, “R&D has been forgotten.” We were forgotten. So, what the Trust had set up and which is, I think, probably a policy or a set of actions that they have for crisis management […] was very militarily organised. […] And we didn’t slot in, nor were we invited on to any of those tactical groups. And didn’t have representation on gold or silver command either. So we were left out of that whole process. […] We had to make real efforts to reach out and offer up. We felt that obligation and we did that.
By late April 2020, R&D were fully integrated into the Trust’s Gold Tactical Command Unit. By this time, however, the prioritisation process had been implemented and oversight of research delivery staff had been centralised, facilitating redeployment to frontline care and COVID-19 research. While the research system contributed staff and other resources to the Trust’s emergency response, it did so at its own initiation.
Pace of work: Shifting gears for the COVID-19 response
One of the most striking aspects of the research infrastructure’s response to the pandemic was the sheer pace of activity and change. The sociological literature on pace suggests that demands for faster productivity are common, and indeed this demand can be seen in the health services literature which often criticises clinical research for not moving fast enough [ 28 – 31 ]. However, the sociological literature also notes the importance of considering where things slow down or even halt [ 28 , 32 ]. In this section we document how pace appeared in participants’ accounts, acknowledging both areas where there were rapid increases in the speed of research work as well as how research work slowed down in other areas.
Increasing pace: Redeployment, research set up and research completion.
Particularly within the first wave, it was the “reserve army” (D-3) of the research delivery workforce who were required to act at speed. As per Table 4 , staff were quickly released from research duties and redeployed to the frontlines to help deliver care. In addition, all NIHR funded staff with clinical training who were not completing COVID-19 research were asked to prioritise frontline care if their employer asked [ 6 ]. Within two weeks, more research delivery staff were redeployed to COVID-19 research teams. Staff were called up one day and told to “come in on the next day” (D-8), and managers were told “they’re going tomorrow. This is their last day with you” (D-4).
As pace of redeployment accelerated, so too did the speed of research. The pace with which researchers demanded studies be delivered and set up was “ten times quicker than normal […] as if someone’s taken a time warp machine to it” (R-2). Those already working in the research infrastructure were aware that research was vital to the pandemic response and, as one participant (D-1) explained:
we needed to start the research while we’re right in the middle of the surge in numbers. And so […] you have studies that come, they need to be set up tomorrow, recruit the first patient by the end of the week.
Such shifts in normal timeframes for work were facilitated in part through centralisation, as noted above. “The real step change,” research manager G-4 suggested, “was having a Prioritisation Group and having [the] team agree a fast-track way of doing things.” Alongside streamlined approval and set-up processes, wider research infrastructures and research practices were adapting at great speed:
I was amazed that, for example, by the end of March, there were–I counted them– 13 granting agencies that, some way or another, had calls on urgent COVID-19 research (R-4).
As a result of these rapid research projects, new knowledge was being produced at an unprecedented rate, as one participant succinctly put it, “science doesn’t usually change that quickly” (D-9). This speed was met with enthusiasm by PIs and research delivery staff alike, but also caused some nervousness. Some were concerned, for example, that PPI had “dropped off the radar” (G-3), whilst others were wary of publication prior to peer review:
the […] thing which is a challenge is that we’re pre-printing research, we’re putting pre-prints out when we’re submitting to journals, because–and we’re rushing to get the pre-prints out. […] And I guess that’s good. But it is also a bit of a–a stresser because […] maybe we haven’t quite got the message right yet (R-1).
Others warned that the pace of research during the first wave of the pandemic came at a human cost. Some researchers had vastly increased workloads, “going at max […] for 5 months” (R-1), where in some cases “there’s not been a single day when [they’ve] not been working in the laboratory including all Sundays and Saturdays, Easter and so on” (R-4). Whilst some enjoyed this fast-paced moment, for those closer to the frontline it has caused anxiety. As one participant (G-5) explained, “we’ve been fire-fighting”, and at least one member of staff, another explained, “can’t come near the hospital. She has panic attacks” (D-3). Whilst it has already been documented that critical care staff’s mental health has suffered in the pandemic, these participants suggest there may also be concern for the staff involved in the research response [ 33 ].
Seeing what is possible within the exceptional circumstances of a global pandemic led some researchers and PPI managers to question the normal slower pace of regulatory approvals and assert, “if you can do it during COVID-19, you can do it any other time” (R-6). The often slow processes such as ethical approvals, data sharing guidelines, funding applications, and study set-up was a common comparator to what has been possible during the COVID-19 pandemic. Yet, as G-1 explained: “The reason [research processes have] been quicker is just because there’s been less studies.” This is evident in SLAT’s own R&D data. Table 5 documents the difference in study numbers and timeframes from initial sponsorship review to final capacity and capability approval (allowing the site set up and recruitment to commence) across 3 financial years. While some approval processes were adapted, generally research governance requirements, both internal to the Trust and at the regulators the MHRA and the Health Research Authority, remained the same. The quick approval processes were possible because no new non-COVID-19 studies were reviewed, COVID-19 studies were processed as quickly as possible and almost all non-COVID-19 related research was halted.
https://doi.org/10.1371/journal.pone.0256871.t005
Slowing or halting non-COVID-19 research.
For some investigators, the halting of non-COVID-19 research led to a slower pace where researchers could play catch up. “People have just been writing up their papers” (R-3), and this period of time “gave […] the opportunity, freed up time” (R-6) to apply for grants. Whilst many tried to set up studies so they were ready to go when restrictions were lifted, they also found that “regulatory bodies have been slower” (R-6) due to their focus on COVID-19. It was apparent that these researchers had more time to engage in PPI whilst putting these grants together–one PPI manager working in cancer (P-3) suggested “PPI activity has probably increased” during the pandemic. Whilst many researchers were understanding of the need to halt research, others found it devastating for patients and the reputation of UK research. These researchers (R-3 and R-6) pointed to other international contexts where they saw standard research continuing. Researcher R-6 was surprised “with the UK being such a […] clinical trials powerhouse”, that decision-makers didn’t “do everything it could to retain that reputation even through the COVID-19 crisis.”
On 21 st May 2020 the DHSC and NIHR circulated a framework for restarting new and paused non-COVID-19 research. Stratifying research studies into three levels of priority, this framework made no distinction between commercial and non-commercial research. Using this framework, the Trust implemented its operational Restart Plan the week commencing 1 st June 2020. Recommendations on which research studies were important or urgent to restart within each directorate was managed a directorate level, with the Prioritisation Group acting as the Trust-level decision making body for the restart plan. The Prioritisation Group continued to meet weekly to approve restart plans for research projects. By mid-summer restart was well underway but the pace of resuming all these studies could not match the pace that research stopped, and researchers were concerned that they “haven’t really been able to pick up our trial recruitment in between [waves], because recovery has been so slow” (R-5). The time of “let’s get back to normal quickly because COVID’s over”, participant R-2 explained soon turned to “actually, let’s not rush back into things because we don’t know what’s coming.” At this point the centralisation of research infrastructures hindered speed rather than aided it–one research governance manager (G-4) suggested that “we need to respect the decision-making of the research managers and matron and the R&D leads now”, but instead studies were “number 507 in the queue”, and having to “wait another week for this prioritisation meeting” whilst “people are really scared about their finances […] frightened about not finishing […] patients are waiting.”
Adopting new and virtual working practices
The response to COVID-19 pandemic has resulted in broad shifts in working patterns across the labour market, and will likely lead to longer term transformations to work practices stemming from these temporary changes [ 34 – 36 ]. In health, research highlights the accelerated adoption of digital and virtual working practices as a result of COVID-19, such as the use of telemedicine in secondary care [ 37 – 39 ]. The implementation of new working practices, taking advantage of digital technologies for communication and the adaptation of existing processes so that they can be completed (at least in part) during the pandemic are also crucial elements of the research response to COVID-19, particularly for facilitating the continuation of research.
Reducing patient visits.
Clinical research is a highly regulated domain, with strict oversight on practices and procedures, and reporting requirements overseen by multiple regulators. While research setup and governance processes became more centralised, the successful conduct of research during the pandemic required a degree of flexibility and creative adaptation. The move to more remote or virtual ways of completing, supporting, regulating, and facilitating research relied on the speedy adoption of new technologies and ways of working.
On 12 th March 2020, the MHRA issued guidance to sites and investigators “regarding protocol compliance during exceptional circumstances” [ 40 ]. The guidance stated that the MHRA recognised “the difficult current situation” and advised on how to manage trials during the pandemic [ 40 ]. The MHRA also noted in this guidance and on the MHRA Inspectorate website that a redistribution of human resources during the pandemic:
may mean certain oversight duties, such as monitoring and quality assurance activities might need to be reassessed and alternative proportionate mechanisms of oversight introduced (such as phone calls, video calls) to ensure ongoing subject safety and well-being. We would advise a brief risk assessment and documentation of the impact of this [ 40 ].
While this guidance came before the formal research shutdown, it remained important, especially for the small amount of research which was allowed to continue because it was the best or only treatment option left available for patients. However, research practices and trial protocols needed to be adapted, particularly as there were restrictions on who could physically visit hospital sites, as G-5 highlights:
If a protocol says that a participant will have a visit at week 1, week 2, week 3 and week 4 and those are protocol visits–it’s unacceptable not to do those visits. They are protocol deviations. However, during the real surge of the pandemic, those visits couldn’t be done. They couldn’t come in and have an MRI scan, and ECG and bloods taken. What they did have was someone contacting them by telephone or by Skype or other formats, media format–to say, “How are you doing? Are you okay? Is there anything you need to report? Keep in touch” (G-5).
Through delaying or adapting follow-up appointment requirements so they could be completed over the telephone or through videoconferencing, many studies were able to maintain some level of continuity. For these research participating patients, other parts of the research process needed sensitive negotiation, as one PI explains in relation to changes in the format of patient consultations:
Some [participants] were actually a bit reluctant and felt a bit fobbed off to be called at home [when] they were due a face-to-face consultation. We had to be a bit careful about that, particularly if we were discontinuing treatment or discharging people from our care. That almost always went badly if we tried to do it remotely. And if we were having a really definitive conversation like that, it was worth–we found, in the end, patients coming up. Other patients were reluctant to come and readily accepted our advice that rather than coming for a CT scan, we just do a chest x-ray when we next saw them. So, there is a difference of approach, which is personal–not particular to their circumstance (R-5).
Balancing the need for face-to-face consultations and the protection offered by telephone or video consultations required thoughtful, individualised decision-making. For other studies however, digital consultation was simply not possible, which lead to investment in supporting people to attend the hospital:
A few studies have been done remotely, but the one that I have taken on, patients really have to come in. So, we had to do a lot of logistic development there, like bringing them in by car, paying for whatever is necessary just to make sure that they continue coming in (D-6).
Working from home.
Another crucial step in facilitating research and frontline care was asking large numbers of staff to complete their work from home. For some participants, working from home lead to greater productivity, but for many others it meant the blurring of home and work lives. Numerous factors impacted on participants’ experiences, from juggling work alongside home schooling and caring responsibilities, to feelings of isolation, through to more practical issues, such as having a space to work at home, having sufficient internet bandwidth and having stable access to Trust systems (see Box 1 ).
Box 1. Indicative questionnaire responses to: What, if any, challenges have you had to face working from home?.
https://doi.org/10.1371/journal.pone.0256871.t006
While research staff were transitioning to working from home, research spaces were transformed to facilitate frontline care. By April, two of the four Clinical Research Facilities (CRFs) in the Trust were repurposed to deliver frontline care and training space for frontline staff. The remaining two CRFs were refocused on supporting COVID-19 research. The vacant R&D department’s office spaces were also used by Trust staff to facilitate socially-distanced meetings and computer work for those who needed to be onsite. Careful repurposing of offices and clinical space provided the Trust with additional, flexible physical space to assist in the emergency response to the pandemic.
Digitalising research processes.
Research work still occurred within the normal parameters of how health research is conducted in the NHS. These practices were, however, done differently to adapt to COVID-19 social distancing measures.
Firstly, researchers initially had to find a workaround for consent to research in COVID-19 wards. Because of infection control protocols no materials, including paper consent forms, could be removed from COVID positive wards. As there were no protocols in place to gain consent digitally, staff developed a local workaround, as D-1 explains:
we managed to get some […] work phones so that we could take a picture of the consent [form]. So, the consent [form] was held up to the window [in the COVID ward], the team outside could take a picture of the consent form and send it directly through on the Pando app, because [Pando] could have patient details. So, it could then be turned into a PDF and printed and put in the patient file.
Another example of a slow but necessary digital solution was with site monitoring. Site monitoring allows commercial companies and other trial sponsors to visit research sites to assess the quality of the data and ensure study protocols are being followed. Despite MHRA instruction that this “should not add extra burden to trial sites” [ 40 ] and that monitors could not be justified as an extra body in the building, these activities are crucial not just for validating data but for hospitals to be able to bill sponsors for the completed research. Workarounds were further limited because of data protection regulations that prevent the digital transfer of patient data or remote access to Trust systems by external individuals. Where site monitors would usually work alone on site, it became a long and arduous process:
a member of the research team within the Trust sits at a screen and shared that screen through Microsoft Teams with the external person. So, no data is held, no recordings are being done, no data is transferred. But it’s very, very labour-intensive. (G-5)
Whilst workarounds were quickly found for some research practices, others took longer. Despite the fact that Patient and Public Involvement in research (PPI) is a core element of contemporary UK health research [ 17 ], there was initially “zero PPI” (G-1). Rather PPI group managers focused on care work: “putting them in touch with local services that could do things like pick up prescriptions for them, get shopping, get the food boxes delivered” (P-1). It was only with time that not only did researchers planning non-COVID research begin to engage more than usual with their PPI groups, but that funders and regulators demanded that PPI should still be prioritised even in emergency research [ 41 , 42 ].
While researchers voiced concerns about the equity of shifting online and assumptions about who will and will not engage with online PPI, this did not appear to be a problem in practice:
There’s often a sort of an ageism about who can–it’s like kind of what you were just saying about older people can’t do PPI. Well, bollocks. I mean actually they’ve been as responsive to this pandemic as anybody else. The rates of use of, you know, technology, has like skyrocketed in the over 65s, because of their need to talk to their grandchildren etc. So, you know, they are adaptive (R-1).
R-1’s experience was echoed by PPI representatives. Reflecting on the move online, these representatives noted some disadvantages, such as the absence of many social aspects of attending PPI meetings, and video fatigue. But participants were generally positive about the potential of virtual PPI for involving those who cannot always travel long distances due to their illnesses, those who work full-time but could attend an hour session online in their lunch break, and representatives in different countries.
In short, the process of realigning and digitalising research practices was not simply one that sped up research and productivity, but it involved a set of necessary, labour-intensive workarounds. It did, however, also bring about possibilities for long term positive effects, such as diversifying involvement in PPI groups.
COVID-19 has brought to the fore the critical importance of the UK’s clinical research infrastructure which has over the past 15 years become increasingly embedded within the NHS. It has enabled NHS hospitals to deliver research of global importance at an unprecedented pace while simultaneously providing critical care for record numbers of acutely ill patients. We provide an analysis of how this was possible through an in-depth case study of the transformations and reconfigurations of the research system at one research-intensive Trust. Our data show that a large-scale reorganisation of research staff, research infrastructures and research priorities took place during the first few weeks and months of the pandemic. We have documented many of the changes in organisational structure, national policy and everyday working practices that facilitated the Trust’s response to COVID-19. These rapid changes have brought about new ways of working, and new perspectives on the role of research which may have far reaching consequences for the future of the clinical research system in the UK.
The pandemic occasioned a large-scale mobilisation of research staff as a “reserve army.” Research staff were crucial in supporting the care-function of NHS hospitals during the first wave of the pandemic. At the same time, the embedded research system helped to streamline, facilitate and deliver rapid COVID-19 research.
Our study documented some of the challenges that the research system has faced in seeking to operate in a COVID-safe manner. At the same time, our participants described instances of improvisation in order to adapt protocols to the COVID-19 environment. Research staff developed effective practical solutions borne out of necessity, rather than the result of prior planning. This points to the resourcefulness of research staff, but also highlights the ways in which the research system was initially largely absent from existing emergency planning within the health system.
Our research was conducted while the Trust we were studying enacted national COVID-19 policy, responded to local care needs and supported clinical research during a global pandemic. This allowed us to observe these events unfolding while gathering data in a COVID-safe manner. But the pandemic created limitations as well, especially impacting the range of methods we were able to use. While working digitally did give us a first-hand experience of how a large proportion of the decision-making infrastructure had to move online, it limited our access to frontline care and everyday research activity.
There are also limitations of looking at a research active Trust like SLAT. While research is increasingly becoming a routine component of all NHS settings, SLATs size and existing research portfolio meant there was a large amount of resource available to redeploy towards COVID-19 care and research delivery. This picture may not be representative of all NHS Trusts, particularly those that are smaller, where less research takes place. Such resource, particularly in the form of biomedical research infrastructures embedded within NHS Trusts, have provided what Roope et al. label ‘option value’ in research, additional capacity to support public good, which in normal times may appear an inefficient use of resource [ 43 ]. Roope et al. highlight that, in comparison to funded, individual research studies, funding research infrastructures allows greater flexibility and speed of response when emergencies arise, such as the COVID-19 pandemic. While the research workforce, funds and infrastructures were used to support other research prior to COVID-19 (as opposed to being excess capacity), the ability of such resource to be reallocated to COVID-19 at such pace underpinned much of the UK’s success in its research response and much of the work described in this paper. It is important to acknowledge, however, that research capacity is distributed unevenly throughout the NHS, and resources such as Clinical Research Facilities and Biomedical Research Centres tend to be situated in major teaching hospitals and trauma centres rather than geographically more localised hospitals. More research is needed to understand how this unequal distribution of resources affected outcomes of care and research during the pandemic.
In documenting how the pace of research work changed dramatically during the pandemic, both in terms of increasing the speed of certain activities and decreasing the speed of others, our paper also contributes to broader discussions of pace in clinical research. In particular, the key question—how do we most effectively streamline the research pipeline, from bench to bedside? Hanney et al. highlight the potential to overlap parts of the translational research pathway to speed up the process, and some of the barriers to this, such as ethical approvals and resourcing issues [ 30 , 31 ]. Many of these issues were removed during the pandemic because of the targeting of resources towards COVID-19 research. On a more practical level, however, our analysis suggests some ways that the research system may be adapted in the future. The potential offered by digital communications to facilitate certain research and PPI activities have led some clinical researchers to question the necessity for research participants and patients to always attend hospital sites for consultations. Trust-level research prioritisation has proved positive in managing finite local resources as effectively as possible, enabling a more holistic view of the research portfolio at a local level as well as take into account national priorities. At the same time, it is clear that the new technologies and new ways of working that were developed to cope with the crisis are not automatically more efficient, and there is a danger that some key steps such as adequate PPI might be overlooked when research pace is increased. Further research and planning will be needed to develop suitable governance processes to facilitate research activities both when on a crisis footing, and in more routine practice. Wider investment in networked digital applications and hardware (such as Trust compliant laptop computers) is needed to facilitate better working from home.
Our study suggests a number of additional lessons for future national emergency planning and policy. Research infrastructure must be better included in advanced planning, both in terms of the personnel, equipment and other resources that can be made available for redeployment as well as the direct impact that research can make. The capacity to develop new treatments and vaccines should be treated as a strategic asset that is a central part of any emergency response. This has been recognised at the national level, and internationally [ 1 – 3 ], but our data suggest that it has not fully translated into Trust-level operations. Planning for future emergencies should include protocols for the rapid establishment of strategic research prioritisation and redeployment of research infrastructure and capacity. Our data also show that throughout the pandemic, there remained a demand for public input in research, which should be included in future emergency planning. Public input is vital in clinical research, especially in an emergency response which requires publics to respond to clinical-expert advice, and planners should recognise it as such.
Future emergency planning must, however, take into account the exhaustion and stress faced by research staff who suddenly found themselves on the front line of a national mobilisation. Research staff experienced the same well-documented stresses experienced by other NHS workers [ 33 , 44 ]. Emergency planning should acknowledge this human cost and find ways to mitigate such costs and provide support for staff as a national priority.
At a global level, the UK response and its specific organisation, as described within this case study Trust, demonstrates some of the benefits of embedding research infrastructures within a national health provider, and how this set up not only enabled a coherent national response, but also provided staff resource to facilitate such research at great speed as well as support the delivery of frontline care. As we look to the future, how we integrate healthcare and research at more national and global levels are important areas for further research and discussion.
Acknowledgments
We are grateful to Christopher McKevitt and Nina Fudge for providing astute comments on drafts of this paper and to our participants who shared their experiences and time with us during this period of unprecedented strain on the NHS.
- View Article
- PubMed/NCBI
- Google Scholar
- 5. National Institute for Health Research. Urgent Public Health COVID-19 Studies 2021 [27/07/2021]. Available from: https://www.nihr.ac.uk/covid-studies/ .
- 6. National Institute for Health Research. DHSC issues guidance on the impact of COVID-19 on research funded or supported by NIHR 2020 [01/05/2020]. Available from: https://www.nihr.ac.uk/news/dhsc-issues-guidance-on-the-impact-on-covid-19-on-research-funded-or-supported-by-nihr/24469 .
- 13. Yin R K. Case study research: Design and methods. 3rd Edition ed. London: Sage; 2003.
- 14. Stake RE. The art of case study research. London: Sage; 1995.
- 16. Quinn Patton M. Qualitative research and evaluation methods. 3rd Edition ed. London: Sage; 2002.
- 17. National Institute for Health Research. National Standards for Public Involvement in Research. 2019.
- 25. National Institute for Health Research. Urgent Public Health Designation Guidance Notes 2020 [03/03/2021]. Available from: https://www.nihr.ac.uk/documents/urgent-public-health-designation-guidance-notes/24992 .
- 26. National Institute for Health Research. Prioritised support for urgent COVID-19 research 2020 [03/03/2021]. Available from: https://www.nihr.ac.uk/covid-19/prioritised-support-for-urgent-covid-19-research.htm .
- 27. Pharmaphorum. Coordinating and delivering research in the pandemic: the UK approach 2021 [01/03/2021]. Available from: https://pharmaphorum.com/webinars/uk-covid-coronavirus-research-nihr/ .
- 28. Sharma S. In the meantime: Temporality and cultural politics. Durham: Duke University Press; 2014.
- 32. Baraitser L. Enduring time. London: Bloomsbury Publishing; 2017.
- 40. MHRA Inspectorate. Advice for Management of Clinical trials in relation to Coronavirus 2020 [03/03/2021]. Available from: https://mhrainspectorate.blog.gov.uk/2020/03/12/advice-for-management-of-clinical-trials-in-relation-to-coronavirus/ .
- 41. National Institute for Health Research. NIHR reaffirms its support for patient and public involvement, engagement and participation during the COVID-19 pandemic 2020 [11/03/2021]. Available from: https://www.nihr.ac.uk/news/nihr-reaffirms-its-support-for-patient-and-public-involvement-engagement-and-participation-during-the-covid-19-pandemic/24641 .
- 42. Health Research Authority. Public involvement in a pandemic: lessons from the UK COVID-19 public involvement matching service. 2021.
Advertisement
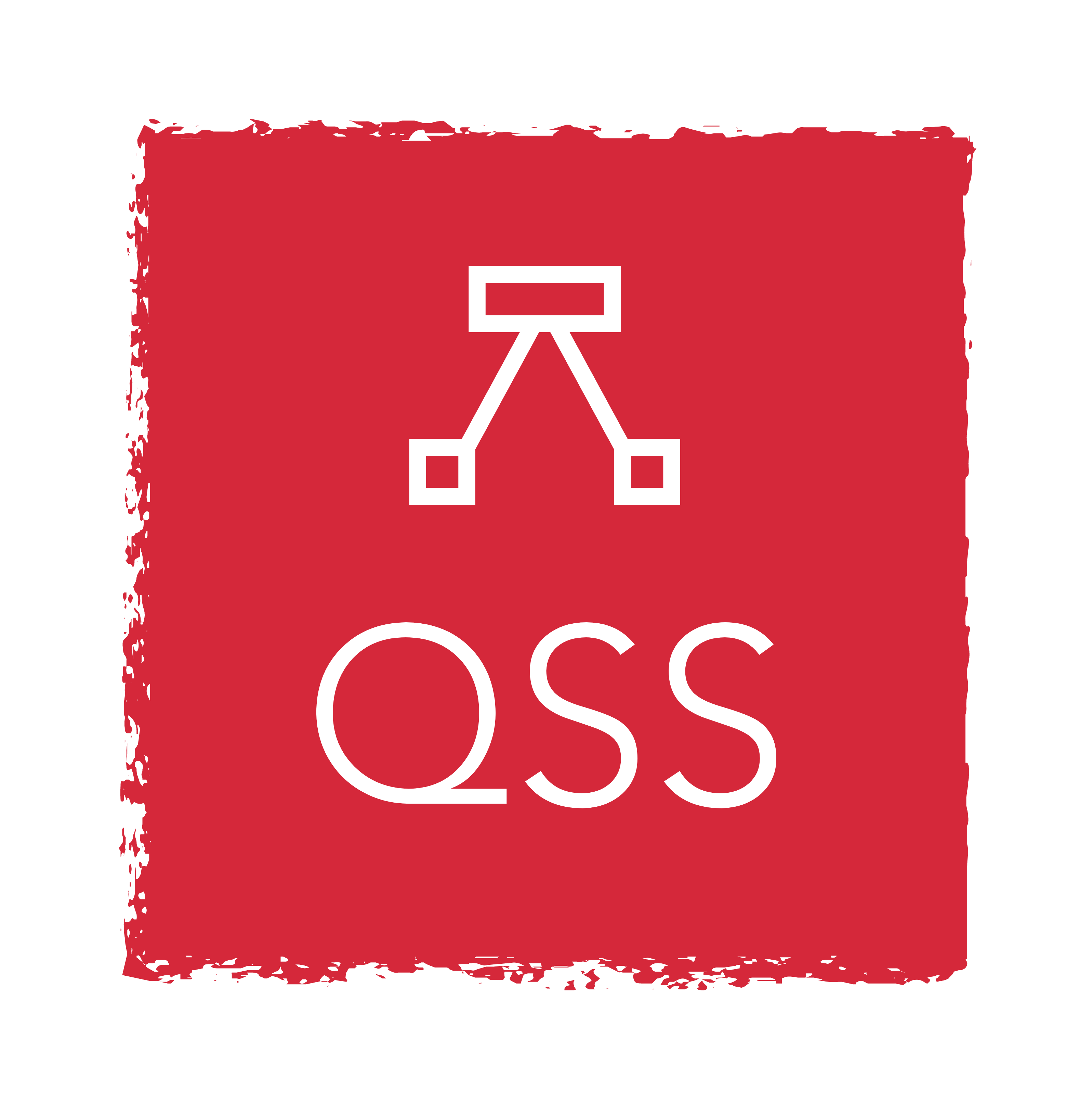
- Next Article
1. INTRODUCTION
2. related work, 3. data and methods, 5. conclusion, competing interests, funding information, data and code availability, acknowledgments, covid-19 research in wikipedia.

Handling Editor: Staša Milojević
- Cite Icon Cite
- Open the PDF for in another window
- Permissions
- Article contents
- Figures & tables
- Supplementary Data
- Peer Review
- Search Site
Giovanni Colavizza; COVID-19 research in Wikipedia. Quantitative Science Studies 2020; 1 (4): 1349–1380. doi: https://doi.org/10.1162/qss_a_00080
Download citation file:
- Ris (Zotero)
- Reference Manager
Wikipedia is one of the main sources of free knowledge on the Web. During the first few months of the pandemic, over 5,200 new Wikipedia pages on COVID-19 were created, accumulating over 400 million page views by mid-June 2020. 1 At the same time, an unprecedented amount of scientific articles on COVID-19 and the ongoing pandemic have been published online. Wikipedia’s content is based on reliable sources, such as scientific literature. Given its public function, it is crucial for Wikipedia to rely on representative and reliable scientific results, especially in a time of crisis. We assess the coverage of COVID-19-related research in Wikipedia via citations to a corpus of over 160,000 articles. We find that Wikipedia editors are integrating new research at a fast pace, and have cited close to 2% of the COVID-19 literature under consideration. While doing so, they are able to provide a representative coverage of COVID-19-related research. We show that all the main topics discussed in this literature are proportionally represented from Wikipedia, after accounting for article-level effects. We further use regression analyses to model citations from Wikipedia and show that Wikipedia editors on average rely on literature that is highly cited, widely shared on social media, and peer-reviewed.
Alongside the primary health crisis, the COVID-19 pandemic has been recognized as an information crisis, or an “infodemic” ( Cinelli, Quattrociocchi, et al., 2020 ; Ioannidis, 2020 ; Xie, He, et al., 2020 ). Widespread misinformation ( Swire-Thompson & Lazer, 2020 ) and low levels of health literacy ( Paakkari & Okan, 2020 ) are two of the main issues. In an effort to deal with them, the World Health Organization maintains a list of relevant research updated daily ( Zarocostas, 2020 ), as well as a portal to provide information to the public ( World Health Organization, 2020a ); the European Commission does similarly ( European Commission, 2020 ), as do many other countries and organizations. The need to convey accurate, reliable, and understandable medical information online has never been so pressing.
Wikipedia plays a fundamental role as a public source of information on the Web, striving to provide “neutral” and unbiased content ( Mesgari, Okoli, et al., 2015 ). Wikipedia is particularly important for access trusted medical information ( Smith, 2020 ; Swire-Thompson & Lazer, 2020 ). Fortunately, Wikipedia biomedical articles have repeatedly been found to be highly visible and of high quality ( Adams, Montgomery, et al., 2020 ; Maggio, Steinberg, et al., 2020 ). Wikipedia’s verifiability policy mandates that readers can check the sources of information contained in Wikipedia, and that reliable sources should be secondary and published. 2 These guidelines are particularly strict with respect to biomedical content, where the preferred sources are, in order, systematic reviews, reviews, books, and other scientific literature 3 .
The COVID-19 pandemic has put Wikipedia under stress, with a large amount of new, often nonpeer-reviewed, research being published in parallel with a surge in interest for information related to the pandemic ( Wikimedia Foundation, 2020 ). The response of Wikipedia’s editor community has been rapid: Since March 17, 2020, all COVID-19-related Wikipedia pages have been put under indefinite sanctions, entailing restricted edit access, to allow for better vetting of their contents 4 . In parallel, a COVID-19 WikiProject has been established and a content creation campaign is ongoing ( Jung, Geng, et al., 2020 ; Wikimedia Foundation, 2020 ) 5 . While this effort is commendable, it also raises questions about the capacity of editors to find, select, and integrate scientific information on COVID-19 at such a rapid pace, while keeping quality high. As an illustration of the speed at which events are happening, in Figure 1 we show the average time in number of months from publication to a first citation in Wikipedia for a large set of COVID-19-related articles (see Data and Methods ). In 2020, this time has gone to zero: Articles on COVID-19 are frequently cited in Wikipedia immediately after (or even before) their official publication date, based on early access versions of articles.

Number of months elapsed from publication to the first Wikipedia citation (mean and median binned by year) of COVID-19-related research. In 2020, the average number of months from (official) publication to the first citation in Wikipedia has gone to zero, likely due to the effect of early releases by some journals. As this figure shows censored data, it should only be taken as illustrative of the fact that Wikipedia editors are citing very recent or even unpublished research.
RQ1: Is the literature cited in Wikipedia representative of the broader topics discussed in COVID-19-related research?
RQ2: Is Wikipedia citing COVID-19-related research during the pandemic following the same inclusion criteria adopted before and in general?
Our main finding is that Wikipedia contents rely on representative and high-impact COVID-19-related research. (RQ1) During the past few months, Wikipedia editors have successfully integrated COVID-19 and coronavirus research, keeping apace with the rapid growth of related literature by including a representative sample of each of the topics it contains. (RQ2) The inclusion criteria used by Wikipedia editors to integrate COVID-19-related research during the pandemic are consistent with those from before, and appear reasonable in terms of source reliability. Specifically, editors prefer articles from specialized journals or mega journals over preprints, and focus on highly cited and/or highly socially visible literature. Altmetrics such as Twitter shares, mentions in news and blogs, and the number of Mendeley readers complement citation counts from the scientific literature as an indicator of impact positively correlated with citations from Wikipedia. After controlling for these article-level impact indicators and for publication venue, time, and size effects, there is no indication that the topic of research matters with respect to receiving citations from Wikipedia. This indicates that Wikipedia is currently neither over- nor underrelying on any specific COVID-19-related scientific topic.
Wikipedia articles are created, improved, and maintained by the efforts of the community of volunteer editors ( Chen & Roth, 2012 ; Priedhorsky, Chen, et al., 2007 ), and they are used in a variety of ways by a wide user base ( Lemmerich, Sáez-Trumper, et al., 2019 ; Piccardi, Redi, et al., 2020 ; Singer, Lemmerich, et al., 2017 ). The information Wikipedia contains is generally considered to be of high quality and up to date ( Adams et al., 2020 ; Geiger & Halfaker, 2013 ; Keegan, Gergle, & Contractor, 2011 ; Kumar, West, & Leskovec, 2016 ; Piscopo & Simperl, 2019 ; Priedhorsky et al., 2007 ; Smith, 2020 ), notwithstanding room for improvement and the need for constant knowledge maintenance ( Chen & Roth, 2012 ; Forte, Andalibi, et al., 2018 ; Lewoniewski, We˛cel, & Abramovich, 2017 ).
Following Wikipedia’s editorial guidelines, the community of editors creates content often relying on scientific and scholarly literature ( Arroyo-Machado, Torres-Salinas, et al., 2020 ; Halfaker, Mansurov, et al., 2018 ; Nielsen, Mietchen, & Willighagen, 2017 ), and therefore Wikipedia can be considered a mainstream gateway to scientific information ( Heilman, Kemmann, et al., 2011 ; Laurent & Vickers, 2009 ; Lewoniewski et al., 2017 ; Maggio, Willinsky, et al., 2019 ; Piccardi et al., 2020 ; Shafee, Masukume, et al., 2017 ). Unfortunately, few studies have considered the representativeness and reliability of Wikipedia’s scientific sources. The evidence on what scientific and scholarly literature is cited in Wikipedia is slim. Early studies point to a relative low overall coverage, indicating that between 1% and 5% of all published journal articles are cited in Wikipedia ( Priem, Piwowar, & Hemminger, 2012 ; Shuai, Jiang, et al., 2013 ; Zahedi, Costas, & Wouters, 2014 ). Previous studies have shown that the subset of scientific literature cited from Wikipedia is more likely on average to be published in popular, high-impact-factor journals, and to be available via open access ( Arroyo-Machado et al., 2020 ; Nielsen, 2007 ; Teplitskiy, Lu, & Duede, 2017 ).
Wikipedia is particularly relevant as a means to access medical information online ( Heilman et al., 2011 ; Laurent & Vickers, 2009 ; Smith, 2020 ; Swire-Thompson & Lazer, 2020 ). Wikipedia’s medical content is of very high quality on average ( Adams et al., 2020 ) and is primarily written by a core group of medical professionals who are part of the nonprofit Wikipedia Medicine ( Shafee et al., 2017 ). Articles that are part of WikiProject Medicine “are longer, possess a greater density of external links, and are visited more often than other articles on Wikipedia” ( Maggio et al., 2020 ). Perhaps not surprisingly, the fields of research that receive most citations from Wikipedia are “Medicine (32.58%)” and “Biochemistry, Genetics and Molecular Biology (31.5%)” ( Arroyo-Machado et al., 2020 ); Wikipedia’s medical pages also contain more citations to scientific literature than the average Wikipedia page ( Maggio et al., 2019 ). Scope for improvement remains, as, for example, the readability of medical content in Wikipedia remains difficult for the nonexpert ( Brezar & Heilman, 2019 ). Given Wikipedia’s medical content’s high quality and high visibility, our work is concerned with understanding whether the Wikipedia editor community has been able to maintain the same standards for COVID-19-related research.
3.1. COVID-19-Related Research
The Dimensions COVID-19 Publications list ( Dimensions, 2020 ).
The COVID-19 Open Research Dataset (CORD-19): a collection of COVID-19 and coronavirus-related research, including publications from PubMed Central, Medline, arXiv, bioRxiv, and medRxiv ( Wang, Lo, et al., 2020 ). CORD-19 also includes publications from the World Health Organization COVID-19 Database ( World Health Organization, 2020b ).
Publications from these three lists are merged, and duplicates removed using publication identifiers, including DOI, PMID, PMCID, and Dimensions ID. Publications without at least one identifier among these are discarded. As of July 1, 2020, the resulting list of publications contains 160,656 entries with a valid identifier, of which 72,795 were released in 2020, as can be seen from Figure 2 . Research on coronaviruses, and therefore the accumulation of this corpus over time, has been clearly influenced by the SARS (2003+), MERS (2012+), and COVID-19 outbreaks. We use this list of publications to represent COVID-19 and coronavirus research in what follows. More details are given in the online repositories.

COVID-19-related literature over time, binned by publication year. (a) Overall; (b) Since 2000 included.
3.2. Auxiliary Data Sources
In order to study Wikipedia’s coverage of this list of COVID-19-related publications, we use data from Altmetric ( Ortega, 2018 ; Robinson-García, Torres-Salinas, et al., 2014 ). Altmetric provides Wikipedia citation data relying on known identifiers 6 . Despite this limitation, Altmetric data have been previously used to map Wikipedia’s use of scientific articles ( Arroyo-Machado et al., 2020 ; Torres-Salinas, Romero-Frías, & Arroyo-Machado, 2019 ; Zahedi et al., 2014 ), especially because citations from Wikipedia are considered a possible measure of impact ( Kousha & Thelwall, 2017 ; Sugimoto, Work, et al., 2017 ). Publications from the full list above are queried using the Altmetric API by DOI or PMID. In this way, 101,662 publications could be retrieved. After merging for duplicates by summing Altmetric indicators, we have a final set of 94,600 distinct COVID-19-related publications with an Altmetric entry.
Furthermore, we use data from Dimensions ( Herzog, Hook, & Konkiel, 2020 ; Martín-Martín, Thelwall, & López-Cózar, 2020 ) in order to get citation counts for COVID-19-related publications. The Dimensions API is also queried by DOI and PMID, resulting in 141,783 matches. All auxiliary data sources were queried on July 1, 2020 too.
3.3. Methods
RQ1: To assess whether the literature cited in Wikipedia is representative of the broader topics discussed in COVID-19-related research, we first cluster COVID-19 literature using text and citation data. Clusters of related literature allow us to identify broad distributions over topics within our COVID-19 corpus. We then assess to what extent the literature cited from Wikipedia follows the same distribution over topics of the entire corpus.
RQ2: To ascertain the inclusion criteria of Wikipedia editors, we use linear regression to model whether an article is cited from Wikipedia or not (logistic regression) and the number of Wikipedia citations it receives (linear regression).
In this section, we detail the experimental choices made for clustering analysis using publication text and citation data. Details of the regression analyses are given in the corresponding section.
Text-based clustering of publications was performed in two ways: topic modeling and k -means relying on SPECTER embeddings. Both methods made use of the titles and abstracts of available publications by concatenating them into a single string. We detected 152,247 articles in English out of 160,656 total articles (8,409 less than the total). Of these, 33,301 have no abstract; thus we only used their title, as the results did not change significantly when excluding articles without an abstract. Before performing topic modeling, we applied a preprocessing pipeline using scispaCy’s en_core_sci_md model ( Neumann, King, et al., 2019 ) to convert each document into a bag of words representation, which includes the following steps: entity detection and inclusion in the bag-of-words for entities strictly longer than one token; lemmatization; removal of isolated punctuation, stop words, and tokens consisting of a single character; and inclusion of frequent bigrams. SPECTER embeddings were instead retrieved from the API without any preprocessing 7 .
Topic modeling is a family of methods to learn statistical patterns of keywords frequently occurring together in the same documents. Formally, a topic is defined as a probability distribution over a vocabulary. Multiple topics can be learned from a corpus of documents and then used to cluster it ( Blei, 2012 ). While topic models are useful given that they require no annotated data, they also provide a way to look at a certain corpus of documents. As such, they have been previously used for bibliometric analysis ( Leydesdorff & Nerghes, 2017 ; Yau, Porter, et al., 2014 ). We trained and compared topic models using Latent Dirichlet Allocation (LDA; Blei, Ng, & Jordan, 2003 ), Correlated Topic Models (CTM; Blei & Lafferty, 2007 ), Hierarchical Dirichlet Process (HDP; Teh, Jordan, et al., 2006 ) and a range of topics between five and 50. We found similar results in terms of topic contents and their Wikipedia coverage (see Results ) across models and over multiple runs, and a reasonable value of the number of topics to be between 15 and 25 from a topic coherence analysis ( Mimno, Wallach, et al., 2011 ). Therefore, in what follows we discuss an LDA model with 15 topics 8 . The top words for each topic of this model are given in the Appendix , while topic intensities over time are plotted as a heat map in Figure A2 . SPECTER is a novel method to generate document-level embeddings of scientific documents based on a transformer language model and the network of citations ( Cohan, Feldman, et al., 2020 ). SPECTER does not require citation information at inference time, and performs well without any further training on a variety of tasks. We embed every paper and cluster them using k -means with k = 20. The number of clusters was established using the elbow and silhouette methods; different values of k could well be chosen, so we again decided to pick the smallest reasonable value of k .
We then turned our attention to citation network clustering. We constructed a bibliographic coupling citation network ( Kessler, 1963 ) based on all publications with references provided by Dimensions; these amount to 118,214. Edges were weighted using fractional counting ( Perianes-Rodriguez, Waltman, & van Eck, 2016 ), hence dividing the number of references in common between any two publications by the length of the union of their reference lists (thus, the maximum possible weight is 1.0). We used only the giant weakly connected component, which amounts to 114,829 nodes (3,385 less than the total) and 70,091,752 edges with a median weight of 0.0217. We clustered the citation network using the Leiden algorithm ( Traag, Waltman, & van Eck, 2019 ) with a resolution parameter of 0.05 and the Constant Potts Model (CPM) quality function ( Traag, Van Dooren, & Nesterov, 2011 ). With this configuration, we found that the largest 43 clusters account for half the nodes in the network, and the largest cluster is composed of 15,749 nodes.
These three methods differ in which data they use and how, and thus provide for complementary results. While topic models focus on word co-occurrences and are easier to interpret, bibliographic coupling networks rely on the explicit citation links among publications. Finally, SPECTER combines both kinds of data and modern deep learning techniques.
Intense editorial work was carried out over the early weeks of 2020 to include scientific information on COVID-19 and coronaviruses into Wikipedia ( Jung, Geng, et al., 2020 ). From Figure 3(a) , we can appreciate the surge in new citations added in Wikipedia to COVID-19 research. Importantly, these citations were not only added not only to cope with the growing amount of new literature but also to fill gaps by including literature published before 2020, as shown in Figure 3(b) . The total fraction of COVID-19-related articles that are cited at least once in Wikipedia compared with the total is 1.9%. Yet, this number is uneven over languages and over time. Articles in English have a 2.0% chance of being cited in Wikipedia, while articles in other languages have only a 0.24% chance. To be sure, the whole corpus is English dominated, as we discussed above. This might be an artifact of the coverage of the data sources, as well as the way the corpus was assembled. The coverage of articles over time is instead given in Figure 4 , starting from 2003 when the first surge of publications happens due to SARS. We can appreciate that the coverage seems to be uneven, and less pronounced for the past few years (2017–2020), yet this needs to be considered in view of the high growth of publications in 2020. Hence, while 2020 is a relatively low-coverage year (1.2%), it is already the year with the most publications cited in Wikipedia in absolute number ( Figure 3b ).

Timing of new citations from Wikipedia, and publication years of the articles they refer to. See Figure A1 for the full timeline. (a) Number of citations in Wikipedia to COVID-19 literature, per month from January 2018. (b) Publication year of COVID-19 articles cited from Wikipedia, distinguishing between citations added before 2020 and in 2020.

Fraction of COVID-19-related articles cited from Wikipedia per year, from 2003.
Citation distributions are skewed in Wikipedia, as they are in science more generally. Some articles receive a high number of citations in Wikipedia and some Wikipedia articles make a high number of citations to COVID-19-related literature. Table A1 lists the top 20 Wikipedia articles by number of citations of COVID-19-related research. These articles, largely in English, primarily focus on the recent pandemic and coronaviruses/viruses from a virology perspective, as already highlighted in a study by the Wikimedia Foundation ( Jung et al., 2020 ). Table A2 reports instead the top 20 journal articles cited from Wikipedia. These also follow a similar pattern: Articles published before 2020 focus on virology and are made of a high proportion of review articles. Articles published in 2020, instead, have a focus on the ongoing pandemic, its origins, and its epidemiological and public health aspects. As we see next, this strongly aligns with the general trends of COVID-19-related research over time.
Coronaviruses : topics 5, 8; this general topic includes research explicitly on coronaviruses (COVID-19, SARS, MERS) from a variety of perspectives (virology, epidemiology, intensive care, historical unfolding of outbreaks).
Epidemics : topics 9, 11, 12; research on epidemiology, including modeling the transmission and spread of pathogens.
Public health : topics 0, 1, 10; research on global health issues, healthcare.
Molecular biology and immunology : topics 2, 4, 6; research on the genetics and biology of viruses, vaccines, drugs, therapies.
Clinical medicine : topics 3, 7, 13, 14; research on intensive care, hospitalization, and clinical trials.
The grouping is informed by agglomerative clustering based on the Jensen-Shannon distance between topic-word distributions ( Figure A5 ). To be sure, the labeling is a simplification of the actual publication contents. It is also worth considering that topics overlap substantially. The COVID-19 research corpus is dominated by literature on coronaviruses, public health, and epidemics, largely due to 2020 publications. COVID-19-related research did not accumulate uniformly over time. We plot the relative (yearly mean, Figure A3a ) and absolute (yearly sum, Figure A3b ) general topic intensity. From these plots, we confirm the periodization of COVID-19-related research as connected to known outbreaks. Outbreaks generate a shift in the attention of the research community, which is apparent when we consider the relative general topic intensity over time in Figure A3(a) . The 2003 SARS outbreak generated a shift associated with a rise in publications on coronaviruses and in the management of epidemic outbreaks (public health, epidemiology). A similar shift is again happening, at a much larger scale, during the current COVID-19 pandemic. When we consider the absolute general topic intensity, which can be interpreted as the number of articles on a given topic ( Figure A3b ), we can appreciate how scientists are mostly focusing on topics related to public health, epidemics, and coronaviruses (COVID-19) during these first months of the current pandemic.
4.1. RQ1: Wikipedia Coverage of COVID-19-Related Research
We address here our first research question: Is the literature cited in Wikipedia representative of the broader topics discussed in COVID-19-related research? We start by comparing the general topic coverage of articles cited from Wikipedia with those which are not. In Figure 5 , three plots are provided: the general topic intensity of articles published before 2020 ( Figure 5a ), in 2020 ( Figure 5b ) and overall ( Figure 5c ). The general topic intensity is averaged and 95% confidence intervals are provided. From Figure 5(c) we can see that Wikipedia seems to cover COVID-19-related research well. The general topics on immunology, molecular biology, and epidemics seem slightly over represented, where clinical medicine and public health are slightly under represented. A comparison between publications from 2020 and from before highlights further trends. In particular, in 2020, Wikipedia editors have focused more on recent literature on coronaviruses, thus directly related to COVID-19 and the current pandemic, and proportionally less on literature on public health, which is also dominating 2020 publications. The traditional slight over representation of immunology and molecular biology literature persists. Detailed Kruskal–Wallis H test statistics for significant differences ( Kruskal & Wallis, 1952 ) and Cohen’s d for their effect sizes ( Cohen, 1988 ) are provided in the Appendix ( Figure A6 and Tables A3 – A5 ). While the distributions are significantly different for most general topics and periodizations, the effect sizes are often small. The coverage of COVID-19-related literature from Wikipedia appears therefore to be reasonably balanced from this first analysis, and to remain so in 2020. The topical differences we found, especially around coronaviruses and the current COVID-19 outbreak, might in part be explained by the criterion of notability, which led to the creation or expansion of Wikipedia articles on the ongoing pandemic 9 .

Average general topic intensity of COVID-19-related publications cited in Wikipeda (green) or not (blue). 95% bootstrapped confidence intervals are given. See Figure A6 and Tables A3 – A5 for significance tests and effect sizes. (a) Published before 2020. Note: this plot also considers as cited in Wikipedia those publications published before 2020 and cited for the first time in 2020. (b) Published in 2020. (c) All publications.
A complementary way to address the same research question is to investigate Wikipedia’s coverage of publication clusters. We consider here both SPECTER k -means clusters and bibliographic network clusters. While we use all 20 SPECTER clusters, we limit ourselves to the top n network clusters that are necessary in order to cover at least 50% of the nodes in the network. In this way, we consider 41 clusters for the citation network, all of size above 300. In Figure 6 we plot the percentage of articles cited in Wikipedia per cluster, and the clusters’ size in number of publications they contain. There is no apparent size effect in either of the two clustering solutions.

Proportion of articles cited from Wikipedia ( y -axis) per cluster size (number of articles in the cluster, x -axis). (a) SPECTER k -means (all). (b) Bibliographic coupling (top 41).
When we characterize clusters using general topic intensities, some clear patterns emerge. Starting with SPECTER k -means clusters, the most cited clusters are numbers 6 and 8 (main macrotopics: molecular biology) and 5 (main macrotopics: coronaviruses and public health, especially focusing on COVID-19 characteristics, detection, and treatment). The least cited clusters include number 18 (containing preprints) and 13 (focused on the social sciences, and especially economics, such as from SSRN journals). Considering citation network clusters, the largest but not most cited are numbers 0 (containing 2020 research on COVID-19) and 1 (with publications on molecular biology and immunology). The other clusters are smaller and hence more specialized. The reader can explore all clusters using the accompanying repository.
We have seen so far that Wikipedia relies on a reasonably representative sample of COVID-19-related literature when assessed using topic models. During 2020, the main effort of editors has focused on catching up with abundant new research (and some backlog) on the ongoing pandemic and, to a lower extent, on public health and epidemiology literature. When assessing coverage using different clustering methods, we do not find a size effect by which larger clusters are proportionally more cited from Wikipedia. Yet we also find that, in particular with citation network clusters, smaller clusters can be either highly or lowly cited from Wikipedia on average. Lastly, we find an underrepresentation of preprint and social science research. Despite this overall encouraging result, differences in coverage persist. In the next section, we further assess whether these differences can be explained away by considering article-level measures of impact.
4.2. RQ2: Predictors of Citations from Wikipedia
In this section, we address our second research question: Is Wikipedia citing COVID-19-related research during the pandemic following the same criteria adopted before and in general? We use regression analysis in two forms: a logistic regression to model if a paper is cited in Wikipedia or not, and a linear regression to model the number of citations a paper receives in Wikipedia. While the former model captures the suitability of an article to provide encyclopedic evidence, the latter captures its relevance to multiple Wikipedia articles.
4.2.1. Dependent variables
Wikipedia citation counts for each article are taken from Altmetric. If this count is 1 or more, an article is considered as cited in Wikipedia. We consider citation counts from Altmetric at the time of the data collection for this study. We focus on the articles with a match from Dimensions, and consider an article to have zero citations in Wikipedia if it is not found in the Altmetric database.
4.2.2. Independent variables
We focus our study on three groups of independent variables at the article level capturing impact, topic, and timing respectively. Previous studies have shown how literature cited in Wikipedia tends to be published in prestigious journals and available via open access ( Arroyo-Machado et al., 2020 ; Nielsen, 2007 ; Teplitskiy et al., 2017 ). We are interested in assessing some of these known patterns for COVID-19-related research, complementing them by considering citation counts and the topics discussed in the literature, and eventually understanding whether there has been any change in 2020.
Article-level variables include citation counts from Dimensions and a variety of altmetric indicators ( Robinson-García et al., 2014 ), which have been found to correlate with later citation impact of COVID-19 research ( Kousha & Thelwall, 2020 ). Altmetrics include the number of Mendeley readers, Twitter interactions (unique users), Facebook shares, mentions in news and blog posts (summed due to their high correlation), mentions in policy documents, and the expert ratio in user engagement 10 . We also include the top 20 publication venues by number of articles in the corpus using dummy coding, taking as reference level a generic category “other,” which includes articles from all other venues. It is worth clarifying that article-level variables were also calculated at the time of the data collection for this study. This might seem counterintuitive, especially for the classification task, as one might prefer to calculate variables at the time when an article was first cited in Wikipedia. We argue that this is not the case, because Wikipedia can always be edited and citations removed as easily as added. As a consequence, a citation in Wikipedia (or its absence) is a continued rather than a discrete action, justifying calculating all counts at the same time for all articles in the corpus.
Topic-level variables capture the topics discussed in the articles, as well as their relative importance in terms of size (size effects). They include the macrotopic intensities for each article, the size of the SPECTER cluster an article belongs to, and the size of its bibliographic coupling network cluster (for the 41 largest clusters with more than 300 articles each, setting it to zero for articles belonging to other clusters. In this way, the variable accounts for both size and thresholding effects). Cluster identities for both SPECTER and citation network clusters were also tested, but did not contribute significantly to the models. Several other measures were considered, such as the semantic centrality of an article to its cluster centroid (SPECTER k -means) and network centralities, but because these all strongly correlate to size indicators, they were discarded to avoid multicollinearity.
Lastly, we include the year of publication using dummy coding and 2020 as the reference level. Several other variables were tested. The proposed selection removes highly correlated variables while preserving the information required by the research question. The Pearson’s correlations for the selected transformed variables are shown in Figure A4 . More details, along with a full profiling of variables, are provided in the accompanying repository.
4.2.3. Model
We consider two models: a logistic model on being cited in Wikipedia (1) or not (0) and an ordinary least squares (OLS) model on citation counts from Wikipedia. Both models use the same set of independent variables and transformations described in Table 1 .
All count variables are transformed by adding one and taking the natural logarithm, while the remaining variables are either indicators or range between 0 and 1 (such as general topic intensities, beginning with a tm _ prefix; for example, tm_ph is “public health”). OLS models including log transform and the addition of 1 for count variables such as citation counts, have been found to perform well in practice when compared to more involved alternatives ( Thelwall, 2016 ; Thelwall & Wilson, 2014 ). Furthermore, all missing values were set to zero, except for the publication year, venue (journal), and general topic intensities as removing rows with missing values yielded comparable results.
4.2.4. Discussion
We discuss results for three models: two logistic regression models on articles published and first cited up to and including in 2020, and one on articles published and first cited up to and including 2019. The 2019 model only considers articles published in 2019 or earlier and cited for the first time from Wikipedia in 2019 or earlier, or articles never cited from Wikipedia, discarding articles published in 2020 or cited from Wikipedia in 2020 irrespective of their publication time. We also discuss an OLS model predicting (the log of ) citation counts including all data up to and including 2020. We do not discuss a 2019 OLS model because it would require Wikipedia citation counts calculated at the end of 2019, which were not available to us. Regression tables for these three models are provided in the Appendix , while Figure A7 shows the distribution of some variables distinguishing between articles cited in Wikipedia or not. Logistic regression tables provide marginal effects, while the OLS table provides the usual coefficients. The actual number of data points used to fit each model, after removing those that contained any null value, is given in the regression tables.
Considering the logistic models first, we can show some significant effects 11 . First of all, the year of publication is mostly negatively correlated with being cited from Wikipedia, compared with the reference category 2020. This seems largely due to publication size effects, as the fraction of 2020 articles cited from Wikipedia is quite low (see Figure 4 ). The 2019 model indeed shows positive correlations for all years when compared to the reference category 2019, and indeed 2019 is the year with lowest coverage since 2000. Secondly, some of the most popular venues are positively correlated with citations in Wikipedia, when compared to an “other” category (which includes all venues except the top 20). In the 2020 model, these venues include mega journals ( Nature , Science ) and specialized journals ( The Lancet , BMJ ). Negative correlations occur for preprint servers (medRxiv and bioRxiv in particular).
When we consider indicators of impact, we see a significant positive effect for citation counts, Mendeley readers, Twitter, and news and blog mentions; we see instead no effect for policy document mentions and Facebook engagements. This is consistent in the 2019 model, except for Facebook having a positive effect and Twitter a lack of correlation. This result, on the one hand, highlights the importance of academic indicators of impact such as citations, and on the other hand suggests the possible complementarity of altmetrics in this respect. As certain altmetrics can accumulate more rapidly than citations ( Fang & Costas, 2020 ), they could complement them effectively when needed ( Kousha & Thelwall, 2020 ). Furthermore, the expert ratio in altmetrics engagement is negatively correlated with being cited from Wikipedia in 2020. This might be due to the high altmetrics engagement with COVID-19 research in 2020, but it could also hint at the possibility that social media impact need not be driven by experts in order to be correlated with scientific impact. We can further see how cluster size effects are not or very marginally correlated with being cited in Wikipedia.
Lastly, general topic intensities are never correlated with being cited in Wikipedia in either model , underlining that Wikipedia appears to be proportionally representing all COVID-19-related research and that residual topical differences in coverage are due to article-level effects.
The 2020 OLS model largely confirms these results, except that mentions in policy documents and Facebook engagements become positively correlated with the number of citations from Wikipedia. It is important to underline that, for all these results, there is no attempt to establish causality. For example, the positive correlation between the number of Wikipedia articles citing a scientific article and the number of policy documents mentioning it might be due to policy document editors using Wikipedia, Wikipedia editors using policy documents, both, or neither. The fact is, more simply, that some articles are picked up by both.
The results of this study provide some reassuring evidence. It appears that Wikipedia’s editors are well able to keep track of COVID-19-related research. Of 141,783 articles in our corpus, 3,083 (∼2%) are cited in Wikipedia: a share comparable to what was found in previous studies. Wikipedia editors are relying on scientific results representative of the several topics included in a large corpus of COVID-19-related research. They have been effectively able to cope with new, rapidly growing literature. The minor discrepancies in coverage that persist, with slightly more Wikipedia-cited articles on topics such as molecular biology and immunology and slightly fewer on clinical medicine and public health, are fully explained away by article-level effects. Wikipedia editors rely on impactful and visible research, as evidenced by largely positive citation and altmetrics correlations. Importantly, Wikipedia editors also appear to be following the same inclusion standards in 2020 as before: In general, they rely on specialized and highly cited results from reputable journals, avoiding, for example, preprints.
The main limitation of this study is that it is purely observational, and thus does not explain why some articles are cited in Wikipedia or not. While in order to assess the coverage of COVID-19-related research from Wikipedia this is of secondary importance, it remains relevant when attempting to predict and explain it. A second limitation is that this study is based on citations from Wikipedia to scientific publications, and no Wikipedia content analysis is performed. Citations of scientific literature, while informative, do not completely address the interrelated questions of Wikipedia’s knowledge representativeness and reliability. Therefore, some directions for future work include comparing Wikipedia coverage with expert COVID-19 review articles, as well as studying Wikipedia edit and discussion history in order to assess editor motivations. Another interesting direction for future work is the assessment of all Wikipedia citations of any source from COVID-19 Wikipedia pages, because here we only focused on the fraction directed at COVID-19-related scientific articles. Lastly, future work can address the engagement of Wikipedia users with cited COVID-19-related sources.
Wikipedia is a fundamental source of free knowledge, open to all. The capacity of its editor community to respond quickly to a crisis and provide high-quality content is, therefore, critical. Our results here are encouraging in this respect.
The author has no competing interests.
This research was not funded.
All the analyses can be replicated using code and following the instructions given in the accompanying repository: https://github.com/Giovanni1085/covid-19_wikipedia . The preparation of the data follows the steps detailed in this repository instead: https://github.com/CWTSLeiden/cwts_covid ( Colavizza et al., 2020 ). Analyses based on Altmetric and Dimensions data require access to these services.
Digital Science kindly provided access to Altmetric and Dimensions data.
https://wikimediafoundation.org/covid19/data (accessed July 4, 2020).
https://en.wikipedia.org/wiki/Wikipedia:Reliable_sources (accessed 10 May, 2020).
https://en.wikipedia.org/wiki/Wikipedia:Identifying_reliable_sources_(medicine) (accessed 10 May, 2020).
https://en.wikipedia.org/wiki/Wikipedia:General_sanctions (accessed 10 May, 2020).
https://en.wikipedia.org/wiki/Wikipedia:WikiProject_COVID-19 (accessed 10 May, 2020).
The identifiers considered by Altmetric in order to establish a citation from Wikipedia to an article currently include DOI, URI from a domain white list, PMID, PMCID, and arXiv ID. https://help.altmetric.com/support/solutions/articles/6000060980-how-does-altmetric-track-mentions-on-wikipedia (accessed April 27, 2020).
https://github.com/allenai/paper-embedding-public-apis (accessed April 25, 2020).
We used gensim ’s implementation for LDA ( Řhůřek & Sojka, 2010 ) and tomotopy for CTM and HTM, https://bab2min.github.io/tomotopy (version 0.7.0). The reader can find more results and the code to replicate all experiments in the accompanying repository.
https://en.wikipedia.org/wiki/Wikipedia:Notability (accessed May 10, 2020).
Calculated using Altmetric data, which distinguishes among the number of researchers ( r ), experts ( e ), practitioners ( p ) and members of the public ( m ) engaging with an article. The expert ratio is defined as r + e + p r + e + p + m .
Marginal effect coefficients should be interpreted as follows. For binary discrete variables (0/1), they represent the discrete rate of change in the probability of the outcome, everything else kept fixed; therefore, a change from 0 to 1 with a significant coefficient of 0.01 entails an increase in the probability of the outcome of 1%. For categorical variables with more than two outcomes, they represent the difference in the predicted probabilities of any one category relative to the reference category. For continuous variables, they represent the instantaneous rate of change. It might be the case that this can also be interpreted linearly (e.g., a significant change of 1 in the variable entails a change proportional to the marginal effect coefficient in the probability of the outcome). Yet, this rests on the assumption that the relationship between independent and dependent variables is linear, irrespective of the orders of magnitude under consideration. This might not be the case in practice.
A.1. TOPICS
Topic #0, Public health : “method”, “system”, “use”, “drug”, “application”, “approach”, “image”, “design”, “test”, “develop”, “technology”, “provide”, “technique”, “new”, “tool”, “potential”, “base”, “device”, “allow”, “result”.
Topic #1, Public health : “health”, “pandemic”, “covid-19”, “COVID-19”, “public”, “country”, “outbreak”, “social”, “care”, “covid-19_pandemic”, “measure”, “policy”, “people”, “public_health”, “Health”, “impact”, “response”, “risk”, “medical”, “need”.
Topic #2, Molecular biology and immunology : “cell”, “infection”, “response”, “mouse”, “immune”, “expression”, “lung”, “induce”, “disease”, “cat”, “role”, “tissue”, “system”, “increase”, “level”, “receptor”, “study”, “gene”, “cytokine”, “human”.
Topic #3, Clinical medicine : “group”, “patient”, “day”, “study”, “year”, “result”, “rate”, “age”, “method”, “compare”, “conclusion”, “total”, “time”, “period”, “mean”, “respectively”, “high”, “month”, “significantly”.
Topic #4, Molecular biology and immunology : “protein”, “virus”, “cell”, “rna”, “viral”, “coronavirus”, “activity”, “replication”, “gene”, “antiviral”, “study”, “human”, “membrane”, “domain”, “binding”, “structure”, “sequence”, “target”, “infection”, “inhibitor”.
Topic #5, Coronaviruses : “respiratory”, “infection”, “acute”, “virus”, “syndrome”, “SARS”, “severe”, “respiratory_syndrome”, “severe_acute”, “influenza”, “child”, “case”, “patient”, “viral”, “acute respiratory syndrome”, “cause”, “coronavirus”, “clinical”, “sars”, “pneumonia”.
Topic #6, Molecular biology and immunology : “virus”, “antibody”, “strain”, “sample”, “detect”, “sequence”, “assay”, “isolate”, “coronavirus”, “detection”, “test”, “gene”, “calf ”, “result”, “serum”, “positive”, “analysis”, “study”, “bovine”, “ibv”.
Topic #7, Clinical medicine : “patient”, “surgery”, “laparoscopic”, “surgical”, “procedure”, “cancer”, “complication”, “perform”, “technique”, “undergo”, “postoperative”, “case”, “tumor”, “result”, “method”, “repair”, “time”, “patient undergo”, “resection”, “hernia”.
Topic #8, Coronaviruses : “covid-19”, “COVID-19”, “sars-cov-2”, “coronavirus”, “case”, “disease”, “patient”, “2019”, “2020”, “infection”, “severe”, “clinical”, “China”, “novel”, “confirm”, “coronavirus_disease”, “report”, “symptom”, “novel_coronavirus”, “Wuhan”.
Topic #9, Epidemics : “model”, “datum”, “number”, “analysis”, “epidemic”, “case”, “time”, “network”, “study”, “different”, “result”, “rate”, “dynamic”, “base”, “paper”, “estimate”, “propose”, “population”, “spread”, “individual”.
Topic #10, Public health : “study”, “review”, “trial”, “include”, “clinical”, “treatment”, “search”, “evidence”, “literature”, “result”, “datum”, “intervention”, “quality”, “report”, “systematic”, “use”, “outcome”, “method”, “research”, “article”.
Topic #11, Epidemics : “disease”, “vaccine”, “infectious”, “human”, “review”, “virus”, “new”, “infectious_disease”, “emerge”, “development”, “animal”, “infection”, “pathogen”, “recent”, “potential”, “cause”, “vaccination”, “infectious diseases”, “outbreak”, “include”.
Topic #12, Epidemics : “risk”, “factor”, “associate”, “associated with”, “mortality”, “high”, “analysis”, “increase”, “study”, “95_ci”, “risk_factor”, “death”, “age”, “patient”, “rate”, “ratio”, “outcome”, “regression”.
Topic #13, Clinical medicine : “effect”, “increase”, “group”, “study”, “level”, “concentration”, “control”, “blood”, “change”, “pressure”, “result”, “high”, “low”, “decrease”, “compare”, “measure”, “temperature”, “significantly”, “weight”, “reduce”.
Topic #14, Clinical medicine : “patient”, “treatment”, “clinical”, “acute”, “lung”, “therapy”, “chest”, “aneurysm”, “outcome”, “treat”, “ventilation”, “care”, “case”, “artery”, “stroke”, “failure”, “lesion”, “pulmonary”, “diagnosis”.
A.2. TABLES
A.3. figures.

Timing of new citations from Wikipedia, and publication years of the articles they refer to. (a) Number of citations from Wikipedia to COVID-19 literature, per year, overall. (b) Publication year of the articles cited from Wikipedia, overall.

Heatmap of topic intensities over time.

General topic intensities over time. (a) Average aggregate; this can be interpreted as the average topic intensity. (b) Cumulative aggregate; this can be interpreted as the number of papers per topic.

Heatmap of regression variables correlations (Pearson’s), after transformations.

Agglomerative clustering dendrogram over topics, based on JensenShannon distances. Considering a cut at 1.1, the left-most cluster (topics 3, 12, 13) focuses on viral epidemics and clinical medicine; next is a cluster on COVID-19 and its treatment in intensive care (topics 7, 8, 14); next is a clus ter COVID-19, public health, epidemics, and immunology (topics 0, 1, 9, 10, 11); lastly, on the right, is a cluster on molecular biology and immunology/vaccines (topics 2, 4, 5, 6).

Cohen’s d -effect statistic for general topic intensity differences be tween ar ticles cited in Wikipedia and not. Publications published before 2020, in 2020, and overall are considered. See Table A3 , A4 , and A5 . Effect sizes are con sidered very small when below 0.2, small when below 0.5 and medium when below 0.8.

Some variables used for regression analyses. The plots distinguish variable values for articles cited from Wikipedia (green) or not (blue). (a) Citations (Dimensions). (b) Mendeley readers. (c) Mentions in blogs and news. (d) Twitter (unique) user interactions. (e) SPECTER cluster size. (f) General topic coronaviruses.
A.4. REGRESSION TABLES
Author notes, email alerts, related articles, affiliations.
- Online ISSN 2641-3337
A product of The MIT Press
Mit press direct.
- About MIT Press Direct
Information
- Accessibility
- For Authors
- For Customers
- For Librarians
- Direct to Open
- Open Access
- Media Inquiries
- Rights and Permissions
- For Advertisers
- About the MIT Press
- The MIT Press Reader
- MIT Press Blog
- Seasonal Catalogs
- MIT Press Home
- Give to the MIT Press
- Direct Service Desk
- Terms of Use
- Privacy Statement
- Crossref Member
- COUNTER Member
- The MIT Press colophon is registered in the U.S. Patent and Trademark Office
This Feature Is Available To Subscribers Only
Sign In or Create an Account
- Open access
- Published: 12 April 2024
Healthcare team resilience during COVID-19: a qualitative study
- John W. Ambrose 1 ,
- Ken Catchpole 2 ,
- Heather L. Evans 3 ,
- Lynne S. Nemeth 1 ,
- Diana M. Layne 1 &
- Michelle Nichols 1
BMC Health Services Research volume 24 , Article number: 459 ( 2024 ) Cite this article
209 Accesses
Metrics details
Resilience, in the field of Resilience Engineering, has been identified as the ability to maintain the safety and the performance of healthcare systems and is aligned with the resilience potentials of anticipation, monitoring, adaptation, and learning. In early 2020, the COVID-19 pandemic challenged the resilience of US healthcare systems due to the lack of equipment, supply interruptions, and a shortage of personnel. The purpose of this qualitative research was to describe resilience in the healthcare team during the COVID-19 pandemic with the healthcare team situated as a cognizant, singular source of knowledge and defined by its collective identity, purpose, competence, and actions, versus the resilience of an individual or an organization.
We developed a descriptive model which considered the healthcare team as a unified cognizant entity within a system designed for safe patient care. This model combined elements from the Patient Systems Engineering Initiative for Patient Safety (SEIPS) and the Advanced Team Decision Making (ADTM) models. Using a qualitative descriptive design and guided by our adapted model, we conducted individual interviews with healthcare team members across the United States. Data were analyzed using thematic analysis and extracted codes were organized within the adapted model framework.
Five themes were identified from the interviews with acute care professionals across the US ( N = 22): teamwork in a pressure cooker , consistent with working in a high stress environment; healthcare team cohesion , applying past lessons to present challenges , congruent with transferring past skills to current situations; knowledge gaps , and altruistic behaviors , aligned with sense of duty and personal responsibility to the team. Participants’ described how their ability to adapt to their environment was negatively impacted by uncertainty, inconsistent communication of information, and emotions of anxiety, fear, frustration, and stress. Cohesion with co-workers, transferability of skills, and altruistic behavior enhanced healthcare team performance.
Working within the extreme unprecedented circumstances of COVID-19 affected the ability of the healthcare team to anticipate and adapt to the rapidly changing environment. Both team cohesion and altruistic behavior promoted resilience. Our research contributes to a growing understanding of the importance of resilience in the healthcare team. And provides a bridge between individual and organizational resilience.
Peer Review reports
Introduction
The COVID-19 pandemic highlighted the complexity and dynamic nature of healthcare systems. It also created a unique opportunity to look at the concept of resilience through the lens of the healthcare team versus the more common approach of situating the concept within the individual or the organization. The early phase of the pandemic was marked by challenges, such as limited access to personal protective equipment, personnel shortages, drug shortages, and increased risks of infection [ 1 , 2 ]. Ensuring patient safety and proper functioning requires coordination and adaptation of the healthcare team and various processes across the health system infrastructure [ 3 , 4 ]. Resilience results from adaptive coordination which enables healthcare systems to maintain routine function in the face of all conditions [ 5 , 6 ].
Resilience in healthcare has been operationalized through resilience engineering, an interdisciplinary aspect of systems engineering focused on promotingpatient safety through the design, implementation, and management of healthcare systems [ 7 , 8 , 9 ] (e.g., how healthcare systems adapt and adjust to maneuver through the daily complexities and challenges to identify effective practices, prevent errors and maintain resilient performance) [ 6 , 8 , 9 , 10 , 11 ]. Resilient performance in healthcare is proposed to be the net result of reaching the threshold of four resilience capabilities within the system: anticipation, the ability to expect and prepare for the unexpected; monitoring, the ability to observe threats to daily system performance; responding, the ability to adapt how the performance is enacted; and learning, the ability to learn from present and past accomplishments within the system [ 12 ]. At present, there is a paucity of research on the resilience of the healthcare team as a cohesive, singular conscious source of knowledge in a highly complex healthcare system. While the resilience of both healthcare systems [ 11 , 13 ] and healthcare workers [ 14 ] has been investigated, there is a gap in knowledge specific to the resilience of the healthcare team as a unified singular consciousness. The circumstances surrounding the COVID-19 pandemic presented a unique opportunity to understand the resilience of the healthcare team in a highly complex system as a singular aware entity within the system; how it acknowledges itself, defines its purpose, and performs under extenuating circumstances. This shifts the emphasis of individual and organization resilience to the resilience in the interconnected healthcare team that extends beyond the boundary of any single person.
The adapted model situates the healthcare team as a cohesive singlular conscious source of knowledge within an intricate and highly complex system [ 15 ]. This model was designed as a bridge between resilience found in individuals within the healthcare system and the organization to emphasize the healthcare team as an aware, unified whole. Our model [ 15 ] combines the existing Systems Engineering Initiative for Patient Safety (SEIPS) model [ 16 ] (version 1), which is based on five domains (organization, person, tasks, technologies, and tools), and environment and the Advanced Team Decision Making Model [ 17 ], which includes components for team performance [ 17 , 18 , 19 ]. Team performance is comprised of team identity, team cognition, team competency, and team metacognition [ 17 , 18 , 19 ]. Team identity describes how the team identifies their purpose to help one another [ 17 ]. Team cognition describes the state of mind of the team, their focus, and common goals [ 17 ]. Team competency describes how well the team accomplishes tasks, and team metacognition describes problem solving and responsibility [ 17 , 19 ], Fig. 1 .
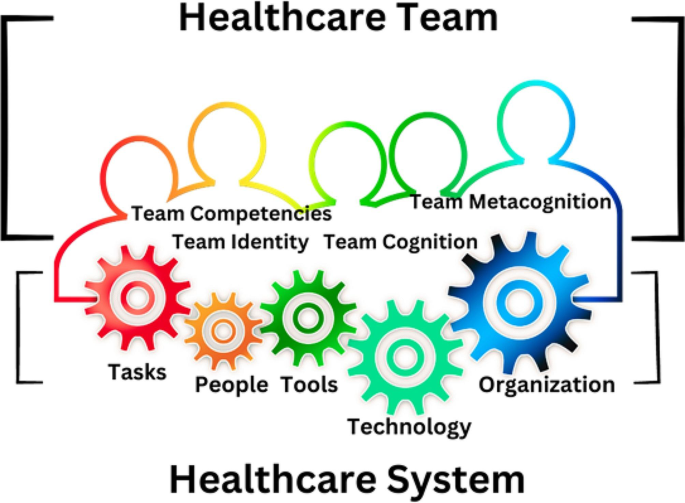
Healthcare Team as a cohesive, singular conscious source of knowledge in a highly complex system. The continuous variegated border represents the singularity and connectedness of the healthcare team within the system. The gears represent the processes, people, technology, and tasks within this highly dynamic healthcare system
The purpose of this qualitative research was to describe resilience in the healthcare team during the COVID-19 pandemic with the healthcare team situated as a singular conscious source of knowledge defined by its collective identity, purpose, competence, and actions. Additionally, we sought to identify factors that may facilitate or hinder the healthcare team from achieving the necessary capabilities to monitor, anticipate, adapt, and learn to meet the standard for resilient performance.
Methodology
A qualitative descriptive design [ 20 , 21 ] was employed. The interview guide was framed using the adapted model to explore various aspects of healthcare team performance (identity, purpose, competence, and cognition). These questions were pilot tested on the first 3 participants and no further changes were needed. Specifically, we aimed to investigate resilience capabilities, decision-making processes, and overall healthcare team performance.
Sampling strategy
A purposive snowball sample was used to identify healthcare team members who worked in U.S. acute care settings between January 2020–December 2020. This sampling method was used to ensure recruitment of participants most likely to have insight into the phenomenon of resilience in the acute care setting.
Inclusion criteria
To explore a wide range of interprofessional experience, participants were recruited across geographic regions and professional roles through personal contacts and social media [ 22 , 23 , 24 , 25 ]. Eligible participants included English-speaking individuals ages 20 and older with a valid personal email address, internet access, and the ability to participate in an online video interview. Potential participants had to be employed full or part-time for any period from January 2020–December 2020 in any of the following acute healthcare environments: emergency room (ER), intensive care unit (ICU), COVID- 19 ICU, COVID-19 floor, gastroenterology inpatient unit, endoscopy suite, operating room (OR), post anesthesia recovery room (PACU), pre-operative holding area, hospital administration, or inpatient medical and/or surgical patient care unit.
Exclusion criteria
Healthcare team members who did not complete the pre-screening survey or failed to schedule an interview were not enrolled.
National recruitment in the U.S
Upon approval by MUSC Institutional Review Board (IRB), registered under Pro00100917, fliers, social media posts on Twitter TM (version 9.34 IOS, San Francisco, California) and Facebook TM (version 390.1 IOS, Menlo Park, CA), and word of mouth were used to initiate recruitment efforts. Interested participants were sent a link to an electronic screening survey explaining the purpose of the study and verifying the respondents’ eligibility to participate. Informed consent was obtained from all subjects.
Data collection
Data were collected via an initial screening questionnaire to determine eligibility. Data were managed using REDCap™ (version 11.2.2) electronic data capture tools hosted at MUSC. Demographic data included age, sex, race, professional role, years of experience, geographic region, patient population served, practice specialty area, and deployment status during the pandemic. Deployment refers to the reassignment of personnel from their primary clinical area to another area to meet the demands of another clinical area without regard for the participant’s clinical expertise. Qualitative data were collected through semi-structured audio video recorded interviews to understand the healthcare team in their natural environment. Recorded interviews were conducted via Microsoft® Teams (version 1.5.00.17261, Microsoft Corporation) from the PIs private office to mitigate the risk of COVID-19 transmission and promote participation across the U.S.
Data monitoring and safety
The quality of the demographic data was monitored to ensure completeness. Potential participants who submitted incomplete responses on the questionnaire were excluded. Interviews were transcribed using software, transcriptions were reviewed and verified for accuracy, and then uploaded to MAXQDA Analytics Pro, Version 2022 (VERBI software) to facilitate data analysis. Transcripts were not returned to the participants. Qualitative codebooks, institutional review board (IRB) logs, and other study records were stored on a secure university server, with access limited to authorized study personnel. Adherence to Consolidated Criteria for Reporting Qualitative Research (COREQ) standards were maintained throughout the study and analysis [ 26 ].
Data analysis
Quantitative analysis.
Demographic data were analyzed using SPSS Statistics for MAC, version 28 (IBM). Both descriptive statistics for the continuous variables of age and years of experience (mean, standard deviation) and frequency tables (age, sex, race, role, geographic region, population served, deployment status) were analyzed.
Qualitative analysis
The Principal Investigator (PI) (JA) and senior mentor (MN) independently coded the interview transcripts. Open coding method was used to identify the categories of data [ 22 , 27 ]. Both a reflexive journal and audit trail were maintained. Codes were identified through induction from participant experiences and verified through weekly consensus meetings, while theoretical deductive analysis was guided by the adapted model and the four resilience capabilities (anticipation, monitoring, responding, learning [ 12 ]. Reflexive thematic analysis (TA) [ 28 , 29 , 30 , 31 ] was used to analyze the coded data and generate themes. Data were collected and categorized into the codebook until no further codes were identified by the PI and research mentor [ 22 , 27 ]. Participant checking was not employed.
Demographics
The eligibility pool was established based on survey completion. Eighty-nine healthcare team members opened the online screening survey; 21 were incomplete and eliminated from the dataset, which left a pool of 68 potential eligible participants. Eligible participants (100%) were contacted by email and phone to determine their interest in completing the study interview. Twenty-two participants completed screening surveys and study interviews between May–September 2021, equating to a 32.5% enrollment rate. Participant interviews lasted between 21 and 91 min with an average of 43 min. None of the interviews were repeated. Participant demographics, including descriptive statistic and role key, are noted in Tables 1 and 2 , respectively.
Five themes were identified: team work in a pressure cooker , healthcare team cohesion , applying past lessons to present challenges , knowledge gaps , and altruistic behaviors .
Teamwork in a pressure cooker
The theme teamwork in a pressure cooker describes the relentless pressures and emotional stressors (e.g., fear, anxiety, frustration, and stress) experienced by the healthcare team from the risks and potential threats associated with COVID-19 contamination and infection. Factors associated with these pressures included risk of COVID-19 exposure, lack of COVID-19 testing, rapid changes to policies and procedures from the standard, personnel shortages, limited physical space, and limited supplies. Exemplary quotes highlighting participant descriptions of these pressures or subthemes are noted in Table 3 .
The healthcare team described an unprecedented level of stress in the workplace as the healthcare team had to adjust to rapidly changing protocols. The lack of protective equipment, shortage of providers to perform patient care and a lack of a familiar clinical routine saturated them in overwhelming pressure and emotions that stuck to them as they navigated uncharted territory. Exemplary quotes highlighting the healthcare team’s descriptions of these emotions are noted in Table 4 .
“It was…uncharted territory for me.” (P1, DIR) “You were stuck in a situation you never— you didn’t know when it was going to end.” (P4, RN PACU) “They have not enough staff—they can’t do it—they—I don’t know what we’re going to do.” (P6, DIR). “When we deployed—trying to get re-accustomed to the changes—with the needs that had to be met was very difficult.” (P10, RN ENDO) “I wasn’t about to sign up for extra time working in under those stressful conditions.” (P17, RN PACU)
The fear of the unknown, combined with the constant need to adapt to rapidly changing circumstances, led to widespread stress, frustration, anxiety, and exhaustion within the healthcare team. This theme was characterized by the constant pressure both inside and outside of work experienced by the healthcare team.
“Driving to the hospital, crying, driving back from the hospital, crying, still doesn’t sum it up— surrounded by people who were just dying. And what could you do?” (P6, DIR) “It was constant. It was terrible. I couldn’t sleep at night. I’d wake up worried.” (P8, ER MD) “It was kind of like just keep sending the Calvary forward—and when one drops, you just walk over them.” (P17, RN PACU) “It was always there—COVID here, COVID there—you never could just completely get away from it. It was basically the center of everybody’s conversation everywhere you went or if you were on the phone with somebody.” (P18, RN COVID ICU) “I was having to call my parents before I’d leave my apartment to go into work— to vent to them and cry— to let out my frustration and my anxiety—and have them essentially convince me to go into work.” (P19, RN ICU). “Working so much— COVID was all that was on my brain—and it was a lot of pressure.” (P22, MGR)
Working during COVID-19 challenged the resilience of the healthcare team in the face of constant fear and uncertainty. The pressure to maintain team performance, while dealing with constant fear associated with the pandemic effected the healthcare team’s resilience.
“I have to tell you that after being in hospital—I don’t feel resilient right now— doing all the things I’ve done—I just want to be out of the hospital— [crying] I can tell you that it will stay with me the rest of my life— It will always stay with me.” (P6, DIR) “I feel like my team has used up all of their resilience. I don’t think there’s much left.” (P8, ER MD)
However, one team member stood out as an exception. They reported the pressures from the environment helped them to make decisions. This demonstrates that environmental pressures affect members of the healthcare team differently. They reported that the pressure and intensity of the situation sharpened their focus and allowed them to make choices more quickly and effectively.
“I make better decisions when I’m under pressure.” (P22, MGR)
Healthcare team cohesion
The theme healthcare team cohesion describes the unique experience of working together during the pandemic that created a means among the healthcare team to form close relationships and unite. This bond was characterized by the emergence of strong interpersonal connections among healthcare professionals during the COVID-19 pandemic. These connections shaped healthcare team relationships and were a factor in the collaborative decision-making processes within healthcare team for their day-to day functions. This cohesive bonding was fueled by the stress and uncertainty of the situation, which brought the healthcare team together illustrated by their solidarity, camaraderie, trust, and empowerment.
“All those decisions, important decisions were made together.” (P7, CRNA) “Everyone felt like they were they were, you know, in a in a battle zone and on the same side—and so that kind of brought people together.” (P8, ER MD) “I think our team worked as one.” (P11, CEO)
Solidarity described the sense of unity evident among the members of the healthcare team. This was characterized by connectedness and a sense of reliance on one another that promoted teamwork and resilience within the team from support both given and received. The sub-theme camaraderie described the close personal connection and support between the healthcare team that went beyond normal social interactions prior to the pandemic. These connections were filled with trust and respect for other healthcare team members.
“I think we were all trying to do the best we could do and help each other do the best they could do—I think early on just camaraderie helped a lot within the department and, you know, just relying on each other for support.” (P8, ER MD) “We knew that we can depend on each other and we all had different skill sets— I think that that was very important—that made us feel secure— rather than going alone.” (P10, RN ENDO) “We [The ICU Nurses] developed a sense of camaraderie that I mean, it’s nothing I’ve ever felt before, like we had to trust each other with our licenses, with our own health—my resiliency came from my coworkers.” (P14, CHG RN) “One of the things that I think the pandemic did in a positive—was—I believe that the teams that I worked for really started to solidify. We leaned on each other. I felt more of a team environment than I had had pre-pandemic—I felt that people were a bit better together. We all needed each other, and we all leaned on each other, and we gave each other support—more so than before COVID- 19.” (P15, CRNA) ”The nurses on the unit were always there for me—they became my friends— my family.” (P19, RN ICU)
The sub theme of empowerment referred to the ability of the healthcare team to confidently make decisions and assume responsibility for their actions within the healthcare setting. This process involved a sense of authority and the ability to exercise agency in decision-making together to respond and adapt to the demands the healthcare team experienced. The combination of solidarity, camaraderie, trust, and empowerment resulted in a strong sense of cohesion within the healthcare team which led to improved relationships and enhanced resilience in their performance.
“I felt that I felt that the team—we all needed each other and we all leaned on each other and we gave each other support—more so than before COVID.” (P15, CRNA) “How do you want to handle this? What’s the plan?—and we collaborated in the true sense of collaboration.” (P15, CRNA) “We just knew that we could count on each other—we knew that we could count on each other at any time if we had questions, because we all worked so closely together during this. We really became a really tight knit group, and it was great.” (P22, MGR)
The benefits of the cohesion found in the healthcare team were significant and apparent during the COVID-19 pandemic. The strengthened relationships and increased resilience allowed for improved communication and collaboration, leading to better patient care and outcomes. Despite these advantages, it was noted by one participant that the relationships developed were not sustained beyond the peak of the pandemic.
“Now that COVID is kind of at bay in our area, it’s kind of gone back to the same way it was— it has not stuck.” (P15, CRNA)
Applying past lessons to present challenges
The theme applying past lessons to present challenges describes how the knowledge and understanding gained from prior participant experiences was used to adapt to the novel clinical and infrastructural challenges faced during the pandemic. Past experiences facilitated the healthcare team to strategize ways to meet the demands of the healthcare system during this time.
Participants described two strategies the healthcare team used to improve the system’s ability to adapt and function effectively: changing roles and deploying personnel. The process of changing roles involved assigning new responsibilities to individuals based on priority-based initiatives, while deployment involved transferring clinical staff from areas with lower patient care needs to those with higher needs to optimize their utilization. Eleven participants (50%) were affected by these strategies. Of these, 73% were assigned to clinical areas for direct patient care, while the remaining 27% underwent a role change to support the operational needs of the system. The participants’ preexisting work relationships, specialized clinical expertise, and leadership abilities helped them adapt to their new clinical and non-clinical roles, which in turn enhanced the resilience of the healthcare team.
“We wanted to make sure that we were putting people into the right area where their skill set could be used the best.” (P1, DIR) “I’m known for moving people forward—I’m also well known for speaking up when I don’t think it is right and there was a lot of stuff that I didn’t think was right— and not only speaking up, I’m also going to come with the solution.” (P6, DIR)
Participants indicated the lessons learned from prior experience positively impacted team performance and improved patient care outcomes. There were two significant examples in the data: the perspective of a nurse who was redeployed to work in an obstetrics unit (P5, ENDO RN) and the perspective of a nursing director (P6, DIR) whose role was changed to develop a program to ensure adequate staffing.
“Because we [the team of interprofessionals] were all very familiar with what we had to do at the task, at handit [the experience of the provision of care] was very fluid—I think it’s because of our years of experience and working with each other for so long that it just worked out very well ”. (P5, ENDO RN) “Staff believed in me when I said I would do something— I could galvanize people because of my reputation of caring for staff, so I was chosen specifically because of my ability to move people forward in spite of things.” (P6, DIR)
Participants identified being assigned to unfamiliar clinical areas or working with unfamiliar patient populations as a barrier that hindered their ability to adapt to clinical situations. The lack of clinical competence among some personnel led to an increase in workload for other healthcare team members, who had to provide additional instruction and guidance on how to complete the task. Decision-makers who deployed nursing staff to a clinical area with higher staffing needs may have believed that the individual nurse had specific clinical skills that would be helpful in that area, and this was not the case.
“She [the patient] felt like it was that he [the new nurse]—really didn’t know what he was doing—not only were we kind of reintroduced to that role of caring for patients where we haven’t been recently, but we’re also in a teaching mode, too, for the new nurses—we had to prioritize how sick the patients were, from basic vital signs to wound dressings to respiratory, and help those new nurses know which to attend to first.” (P10, RN ENDO) “Nurses weren’t really put in a place with enough support and enough resources to be able to do a job, and to do a job that maybe they haven’t done for a few years.” (P10, RN ENDO)
The participants indicated that clinical competencies of a healthcare provider in one patient population may not necessarily be applicable to another patient group. For instance, a neonatal intensive care unit (NICU) nurse who has experience in managing Extra Corporeal Membranous Oxygen (ECMO) in newborns may not have the necessary skills to care for adult ECMO patients in an adult COVID-19 intensive care unit.
“The ECMO nurse was a NICU nurse, so she really could not help me.” (P14, CHG RN)
Knowledge gaps
The theme knowledge gaps refers to the disparity between the existing knowledge of the healthcare team and the knowledge required for the team to effectively respond and adapt to the needs of the healthcare system. The lack of COVID-19 specific knowledge led to gaps in the healthcare team’s understanding, while the lack of communication made it difficult for necessary information to be effectively conveyed and received (e.g., medical logistics, human resources, and other operational policies and procedures). This knowledge gap created a barrier to healthcare team resilience as their capacities to surveil, anticipate, and respond were diminished from the lack of knowledge.
“That [information] is pretty fundamental to how you [the healthcare team] function.” (P17, RN PACU) “I don’t think any amount of preparation could have actually prepared us for how bad COVID was—but we were very, very, very unprepared.” (P18, RN COVID ICU) “It was confusing, it was disruptive to the patients that we had there. They sensed that. And that’s— OK—screw with me, screw with my colleagues, but don’t screw with the patient.” (P21, RN ENDO)
All the participants in leadership roles during the COVID-19 pandemic emphasized the importance of having a thorough understanding of the information and effectively communicating it to the frontline healthcare team members most involved in providing patient care.
“There’s nothing worse than having to learn something in the moment and not being prepared for it.” (P1, DIR) “That made us communicate in multiple ways throughout a day because we all know people learn and adapt it could be in print. It could be in person; it could be a video. We tried to have multiple ways of getting messages out and knowing we needed to repeat messages because this was so unknown, and people were so stressed.” (P11, CEO)
One team member (P13, CRNA), highlighted areas where there were gaps in knowledge in greater detail.
“It was as if the unit was being run by all these sort of substitute teachers that were called in at the last minute. Nobody knew where stuff was—nobody knew what the protocol was—the communication was terrible.” (P13, CRNA)
The cumulative effect from the knowledge gaps contributed to the lack of a practical working knowledge for the healthcare team and affected the healthcare team’s ability to anticipate what needed to be done and adapt their performance to accomplish it. Despite knowledge gaps, healthcare team members reported their capability to learn was facilitated by incremental gains in practical knowledge through their experience over time.
“—people got to be experts at protecting patients and keeping themselves safe.” (P8, ER MD) “I think it kind of was like on the job training at that point, I felt like we were all just trying to survive—learning was like—you went out —then you came back, and you would share how things went.” (P15, CRNA) “You tried to educate yourself so you could be safe.” (P17, RN PACU)
The participant responses received from the leadership (CNO, Directors, and Manager) and front-line personnel (administrative staff, nurses, and physicians) regarding the importance of communication highlighted a difference in perspective. Leadership exhibited a strong commitment toward effective communication and made efforts to ensure all healthcare team members were well informed. On the other hand, the frontline participants indicated instances where communication strategies were not perceived as effective.
“I wasn’t contacted by a manager from the unit or anything to be able to reassure, reassure me that things were being followed through and it should be okay, so that was tough.” (P10, RN ENDO) “It really seemed like there was no communication between—like staffing and the floor—we would get up to the floor and they would say, who are you? What are you doing here? What are we supposed to do with you?” (P20, RN OR)
Altruistic behaviors
The theme altruistic behaviors , encompasses the participants’ perception of their obligation and accountability to their patients and healthcare team, and their steadfastness in supporting the healthcare team even if it meant facing personal or professional repercussions. This readiness to aid the healthcare team and accept consequences showcased their altruism and commitment to the healthcare team. The team’s dedication to both their patients and each other was a primary focus driven by a strong sense of responsibility and obligation.
“I want to be able to look myself in the mirror and feel like I did the right thing—.” (P6, DIR) “My resiliency came from my coworkers. I wanted to come back to work to help them.” (P14, RN COVID ICU) “People really looked out for each other—and people were really kind and compassionate to each other—we all were in this together.” (P15, CRNA) “I’m grateful for the experience that I had and all of the different patients that I was able to help in my time there definitely solidified that being a nurse is what I needed to do—and why I chose the profession is exactly what I should have been doing.” (P19, RN ICU) “You just have to go with what seems right—.” (P22, MGR)
A defining characteristic of this theme was a willingness to endure consequences for the benefit of the healthcare team. These consequences varied from contracting the virus, facing criticism from the healthcare team, to foregoing financial incentives, and even job loss.
“I felt like I was punished for speaking up and I was punished for doing the right thing for patients.” (P6, DIR) “I mean, I literally broke the law so many times. Do you know how many times I started pressors [vasoactive drugs to increase blood pressure] on patients that I had no orders for [because a physician would not enter the ICU]?” (P14, CHG RN)
We identified five key themes based on the coded data; namely teamwork in a pressure cooker , healthcare team cohesion , applying past lessons to present challenges , knowledge gaps , and altruistic behaviors . The researchers propose that stressors arising from the COVID-19 pandemic had an impact on the healthcare team’s resilience. In addition, strong healthcare team cohesion, selfless behaviors among the healthcare team, shared knowledge, and job competence within the healthcare team, enhanced resilient performance.
The healthcare team experienced significant stress and uncertainty, due to the COVID-19 pandemic. This is consistent with previous research that has shown that the unprecedented nature of the pandemic led to challenging working conditions, limited resources, lack of information, and concerns about infecting loved ones [ 32 , 33 , 34 , 35 , 36 , 37 , 38 , 39 , 40 , 41 , 42 , 43 , 44 ]. The collective global impact of COVID-19 on healthcare systems is likely a contributing factor to these stressors [ 45 , 46 , 47 , 48 ].
Our study, along with those conducted by Anjara et al. (2021)[ 49 ] and Kaye-Kauderer et al. (2022) [ 50 ], found that solidarity and camaraderie among healthcare professionals improve resilience. Specifically, Anjara et al. observed increased collaboration among the healthcare professionals they studied in Ireland during the COVID-19 pandemic, while Kaye-Kauderer et al. identified team camaraderie among their sample of front-line healthcare workers from New York. Kinsella et al. (2023) [ 51 ] reported that COVID − 19 offered frontline workers in the UK the opportunity to work together toward a common goal. Potential explanations for these findings align with the concepts of social capital proposed by Coleman [ 52 ] and social identification with other as proposed by Drury [ 54 ]. Coleman suggests an individual’s skills and capabilities are enhanced through their interdependent relationships with others [ 52 ]. Drury found in communities affected by disasters, mutual aid and support emerged from a shared social identity, which serves to strengthen the community [ 53 ]. Brooks et al. (2021) [ 54 ] conducted a study with healthcare, police, and commercial sectors in England. They found it was important for these individuals to receive support from and provide support to their colleagues to mitigate the psychological impact of disaster exposure [ 54 ]. In addition, like our findings, Aufegger and colleague’s 2019 systematic review [ 55 ] found that social support in acute care healthcare teams creates a supportive atmosphere where team members help each other communicate problems, fulfill needs, and deal with stress.
Our results are consistent with those of Liu et al. (2020) [ 32 ] and Banerjee et al. (2021) [ 44 ] who each found that healthcare professionals frequently feel a sense of personal responsibility to overcome challenges. One potential explanation for this may be the influence of collectivism in their cultures. Similarly, our study suggests the sense of camaraderie among healthcare professionals may also contribute to a sense of responsibility and increased altruistic behavior. However, other studies have highlighted different perspectives on healthcare professionals’ sense of responsibility and duty. Godkin and Markwell’s (2003) [ 56 ] revealed that healthcare professionals’ sense of responsibility during the Severe Acute Respiratory Syndrome (SARS) outbreak was dependent on the protective measures and support offered by the healthcare system where most SARS infected patients were hospitalized. More recently, Gray et al. (2021) reported that nurses’ sense of responsibility stems from their ethical obligations, regardless of potential personal or familial risks [ 57 ].
The altruistic behaviors described by our participants helped maintain the performance of the healthcare team. It is too soon to see the long-term impact from working in this high-pressure environment; however, past research by Liu et al. (2012) [ 58 ] and Wu (2009) [ 59 ] demonstrated that “altruistic-risk acceptance” during the SARS outbreak was shown to decrease depressive symptoms among hospital employees in China.
Our research on resilience has important implications for healthcare organizations and professionals. In order to ready themselves for forthcoming events, healthcare systems must emphasize the significance of shared knowledge and its influence on the healthcare team’s ability to foresee and monitor effectively. This knowledge can help the healthcare organization function as a unified entity, rather than as individuals in separate roles or clusters within the organization to improve healthcare team preparedness. Establishing a cohesive, clinically competent healthcare team benefits the organization and the patients served. Measures to enhance social support, improve communication and ensure clinical competence maintain healthcare team resilience.
There are several limitations to consider when interpreting the results of this study. First, the sample was obtained using purposive snowball sampling, which may have introduced sampling bias and may not accurately represent the larger population of healthcare team members who worked during the COVID-19, as 95% of the sample were white. Second, our study did not have equal representation of all interprofessional team members. It is possible that a more heterogenous sample regarding role, race and gender may have introduced additional codes. Additionally, the PI (JA) worked as a Certified Registered Nurse Anesthesiologist (CRNA) in acute care during the pandemic and personal experience may have introduced confirmation bias. Also, the focus of our research was to fill a gap in the existing knowledge of what is known about healthcare team resilience in pandemic disasters, and help to answer if and how it intersects with individual and organizational resilience. It is possible this novel conceptualization of healthcare team as a cohesive singular conscious source of knowledge did not adequately address this.
Steps to ensure rigor and mitigate any potential shortcomings of qualitative data analysis were the maintenance of a reflexive journal, a willingness of the PI to let go of unsupported ideas and constant verification of codes and themes with the research mentor (MN) for coherence and consistency within the coded data, selected methodology and research questions.
Overall, the extracted themes of teamwork in a pressure cooker; healthcare team cohesion; applying past lessons to present challenges; knowledge gaps; and altruistic behaviors illustrate comparable experiences within the healthcare team. As healthcare professionals and organizations continue to navigate the challenges of the COVID-19 pandemic and other crises, our findings provide valuable insights into how team cohesion, along with altruistic behaviors, may enhance resilience capabilities to create and maintain a unified resilient healthcare team.
Data availability
The data for this study are confidential as required by the IRB approval. To protect the anonymity of the participants, the data are not publicly available. Additional information about the research method, Interview questions, informant data, and the study in general can be requested from the corresponding author, J.A.
Berlin G, Singhal S, Lapointe M, Schulz J. Challenges emerge for the US healthcare system as COVID-19 cases rise. 2020;9.
Stevens JP, O’Donoghue A, Horng S, Tabb K. Healthcare’s earthquake: Lessons from complex adaptive systems to develop Covid-19-responsive measures and models. 2020.
Kopach-Konrad R, Lawley M, Criswell M, Hasan I, Chakraborty S, Pekny J, et al. Applying systems Engineering principles in improving Health Care Delivery. J Gen Intern Med. 2007;22(S3):431–7.
Article PubMed PubMed Central Google Scholar
Compton WD, Fanjiang G, Grossman JH, Reid PP. Institute of Medicine (U.S.), National Academy of Engineering. Building a better delivery system: a new engineering/health care partnership [Internet]. Washington, D.C.: National Academies Press; 2005 [cited 2021 Feb 12]. http://public.ebookcentral.proquest.com/choice/publicfullrecord.aspx?p=3378176 .
Hollnagel E, Woods DD. Resilience Engineering concepts and precepts. 1st ed. Boca Raton, FL: CRC Press/Routledge/Taylor & Francis Group; 2006. p. 416.
Google Scholar
Wiig S, O’Hara JK. Resilient and responsive healthcare services and systems: challenges and opportunities in a changing world. BMC Health Serv Res. 2021;21(1):1037.
Nemeth C, Wears RL, Patel S, Rosen G, Cook R. Resilience is not control: healthcare, crisis management, and ICT. Cogn Tech Work. 2011;13(3):189–202.
Article Google Scholar
Hollnagel E. Safety-II in Practice: Developing the Resilience Potentials [Internet]. 1st ed. Routledge; 2017 [cited 2022 May 7]. https://www.taylorfrancis.com/books/9781351780766 .
Braithwaite J, Wears RL, Hollnagel E. Resilient health care: turning patient safety on its head. Int J Qual Health Care. 2015;27(5):418–20.
Article PubMed Google Scholar
Madni AM, Jackson S. Towards a conceptual Framework for Resilience Engineering. IEEE Syst J. 2009;3(2):181–91.
Carthey J. Institutional resilience in healthcare systems. Qual Health Care. 2001;10(1):29–32.
Article CAS PubMed PubMed Central Google Scholar
Hollnagel E. The Four cornerstones of Resilience Engineering. In: Dekker, editor. Resilience engineering perspcetives. E. Hollnagel&S. Ashgate: Farnham, UK; 2009. pp. 117–33.
Fridell M, Edwin S, von Schreeb J, Saulnier DD. Health System Resilience: what are we talking about? A scoping review mapping characteristics and keywords. Int J Health Policy Manag. 2019;9(1):6–16.
Article PubMed Central Google Scholar
Curtin M, Richards HL, Fortune DG. Resilience among health care workers while working during a pandemic: a systematic review and meta synthesis of qualitative studies. Clin Psychol Rev. 2022;95:102173.
Ambrose JW, Layne DM, Catchpole K, Evans H, Nemeth LS. A qualitative protocol to examine Resilience Culture in Healthcare teams during COVID-19. Healthcare. 2021;9(9):1168.
Carayon P, Hundt AS, Karsh B, Gurses AP, Alvarado CJ, Smith M, et al. Work system design for patient safety: the SEIPS model. Qual Saf Health Care. 2006;15(Suppl 1):i50–8.
Thordsen ML, Kyne MM, Klein G, A Model of Advanced Team Decision Making and Performance.: Summary Report: [Internet]. Fort Belvoir, VA: Defense Technical Information Center; 1994 Sep [cited 2021 Feb 13]. http://www.dtic.mil/docs/citations/ADA400497 .
Zsambok CE. Advanced Team Decision Making: A Model and Training Implications.
Klein GA. Sources of power: how people make decisions. MIT Press; 1988.
Doyle L, McCabe C, Keogh B, Brady A, McCann M. An overview of the qualitative descriptive design within nursing research. J Res Nurs. 2020;25(5):443–55.
Siedlecki SL. Understanding descriptive research designs and methods. Clin Nurse Spec. 2020;34(1):8–12.
Crabtree BF, Miller WL. Doing qualitative research. Second. Thousand Oaks, CA: Sage; 1999. p. 406.
Bradley EH, Curry LA, Devers KJ. Qualitative Data Analysis for Health Services Research: developing taxonomy, themes, and theory. Health Serv Res. 2007;42(4):1758–72.
Gale NK, Heath G, Cameron E, Rashid S, Redwood S. Using the framework method for the analysis of qualitative data in multi-disciplinary health research. BMC Med Res Methodol. 2013;13(1):117.
Lincoln Y, Guba E. Naturalistic Inquiry. California: Sage; 1985.
Book Google Scholar
Tong A, Sainsbury P, Craig J. Consolidated criteria for reporting qualitative research (COREQ): a 32-item checklist for interviews and focus groups. Int J Qual Health Care. 2007;19(6):349–57.
Saldaña J. The coding manual for qualitative rearchers. Los Angeles, USA: Sage; 2021.
Boyatzis RE. Transforming qualitative information: thematic analysis and code development. Thousand Oaks, CA: SAGE Publications Ltd; 1998.
Braun V, Clarke V. What can thematic analysis offer health and wellbeing researchers? Int J Qualitative Stud Health Well-being. 2014;9(1):26152.
Braun V, Clarke V. Thematic analysis. In: Cooper H, Camic PM, Long DL, Panter AT, Rindskopf D, Sher KJ, editors. APA handbook of research methods in psychology, Vol 2: Research designs: Quantitative, qualitative, neuropsychological, and biological [Internet]. Washington: American Psychological Association; 2012 [cited 2022 May 15]. pp. 57–71. http://content.apa.org/books/13620-004 .
Braun V, Clarke V. Conceptual and design thinking for thematic analysis. Qualitative Psychol. 2022;9(1):3–26.
Liu Y, Zhai Z, Han Y, Liu Y, Liu F, Hu D. Experiences of front-line nurses combating coronavirus disease‐2019 in China: a qualitative analysis. Public Health Nurs. 2020;37(5):757–63.
Catania G, Zanini M, Hayter M, Timmins F, Dasso N, Ottonello G, et al. Lessons from Italian front-line nurses’ experiences during the COVID‐19 pandemic: a qualitative descriptive study. J Nurs Manag. 2021;29(3):404–11.
Croghan IT, Chesak SS, Adusumalli J, Fischer KM, Beck EW, Patel SR et al. Stress, Resilience, and Coping of Healthcare Workers during the COVID-19 Pandemic. Journal of Primary Care and Community Health [Internet]. 2021;12. https://www.scopus.com/inward/record.uri?eid=2-s2.0-85104122303&doi=10.1177%2f21501327211008448&partnerID=40&md5=96ad0164880c9725ce14d534e3c3117
Arnetz JE, Goetz CM, Arnetz BB, Arble E. Nurse reports of stressful situations during the COVID-19 pandemic: qualitative analysis of survey responses. IJERPH. 2020;17(21):8126.
Dagyaran I, Risom SS, Berg SK, Højskov IE, Heiden M, Bernild C, et al. Like soldiers on the front– a qualitative study understanding the frontline healthcare professionals’ experience of treating and caring for patients with COVID-19. BMC Health Serv Res. 2021;21(1):666.
Goh Y, Ow Yong QYJ, Chen TH, Ho SHC, Chee YIC, Chee TT. The impact of COVID-19 on nurses working in a University Health System in Singapore: a qualitative descriptive study. Int J Mental Health Nurs. 2021;30(3):643–52.
LoGiudice JA, Bartos S. Experiences of nurses during the COVID-19 pandemic: a mixed-methods study. AACN Adv Crit Care. 2021;32(1):14–26.
O’Brien JM, Bae FA, Kawchuk J, Reimche E, Abramyk CA, Kitts C et al. We were treading water. Experiences of healthcare providers in Canadian ICUs during COVID-19 visitor restrictions: a qualitative descriptive study.
Perraud F, Ecarnot F, Loiseau M, Laurent A, Fournier A, Lheureux F, et al. A qualitative study of reinforcement workers’ perceptions and experiences of working in intensive care during the COVID-19 pandemic: a PsyCOVID-ICU substudy. Sharma GA, editor. PLoS ONE. 2022;17(3):e0264287.
Shanafelt T, Ripp J, Trockel M. Understanding and addressing sources of anxiety among Health Care professionals during the COVID-19 pandemic. JAMA. 2020;323(21):2133.
Article CAS PubMed Google Scholar
Speroni KG, Seibert DJ, Mallinson RK. Nurses’ perceptions on Ebola Care in the United States, Part 2: a qualitative analysis. JONA: J Nurs Adm. 2015;45(11):544–50.
Sonis J, Pathman DE, Read S, Gaynes BN, Canter C, Curran P, et al. Effects of Healthcare Organization Actions and policies related to COVID-19 on Perceived Organizational Support among U.S. internists: a National Study. J Healthc Manag. 2022;67(3):192–205.
PubMed Google Scholar
Banerjee D, Sathyanarayana Rao TS, Kallivayalil RA, Javed A. Psychosocial Framework of Resilience: navigating needs and adversities during the pandemic, a qualitative exploration in the Indian Frontline Physicians. Front Psychol. 2021;12:622132.
Freudenberg LS, Paez D, Giammarile F, Cerci J, Modiselle M, Pascual TNB, et al. Global impact of COVID-19 on Nuclear Medicine departments: an International Survey in April 2020. J Nucl Med. 2020;61(9):1278–83.
Haldane V, Morgan GT. From resilient to transilient health systems: the deep transformation of health systems in response to the COVID-19 pandemic. Health Policy Plann. 2021;36(1):134–5.
Shrestha N, Shad MY, Ulvi O, Khan MH, Karamehic-Muratovic A, Nguyen USDT, et al. The impact of COVID-19 on globalization. One Health. 2020;11:100180.
Jean WC, Ironside NT, Sack KD, Felbaum DR, Syed HR. The impact of COVID-19 on neurosurgeons and the strategy for triaging non-emergent operations: a global neurosurgery study. Acta Neurochir. 2020;162(6):1229–40.
Anjara S, Fox R, Rogers L, De Brún A, McAuliffe E. Teamworking in Healthcare during the COVID-19 pandemic: a mixed-method study. IJERPH. 2021;18(19):10371.
Kaye-Kauderer H, Loo G, Murrough JW, Feingold JH, Feder A, Peccoralo L, et al. Effects of Sleep, Exercise, and Leadership Support on Resilience in Frontline Healthcare workers during the COVID-19 pandemic. J Occup Environ Med. 2022;64(5):416–20.
Kinsella EL, Muldoon OT, Lemon S, Stonebridge N, Hughes S, Sumner RC. In it together? Exploring solidarity with frontline workers in the United Kingdom and Ireland during COVID-19. Br J Social Psychol. 2023;62(1):241–63.
Coleman JS. Social Capital in the creation of Human Capital. Am J Sociol. 1988;94:S95–120.
Drury J, Carter H, Cocking C, Ntontis E, Tekin Guven S, Amlôt R. Facilitating collective psychosocial resilience in the Public in emergencies: twelve recommendations based on the Social Identity Approach. Front Public Health. 2019;7:141.
Brooks SK, Dunn R, Amlôt R, Rubin GJ, Greenberg N. Protecting the psychological wellbeing of staff exposed to disaster or emergency at work: a qualitative study. BMC Psychol. 2019;7(1):78.
Aufegger L, Shariq O, Bicknell C, Ashrafian H, Darzi A. Can shared leadership enhance clinical team management? A systematic review. LHS. 2019;32(2):309–35.
Godkin D, Markwell H. The Duty to Care of Healthcare Professionals: Ethical Issues and Guidelines for Policy Development. Submitted to SARS Expert Panel Secretariat.:23.
Gray K, Dorney P, Hoffman L, Crawford A. Nurses’ pandemic lives: a mixed-methods study of experiences during COVID-19. Appl Nurs Res. 2021;60:151437.
Liu X, Kakade M, Fuller CJ, Fan B, Fang Y, Kong J, et al. Depression after exposure to stressful events: lessons learned from the severe acute respiratory syndrome epidemic. Compr Psychiatr. 2012;53(1):15–23.
Wu P, Fang Y, Guan Z, Fan B, Kong J, Yao Z, et al. The psychological impact of the SARS Epidemic on Hospital employees in China: exposure, risk perception, and Altruistic Acceptance of Risk. Can J Psychiatry. 2009;54(5):302–11.
Download references
Acknowledgements
The authors want to thank all the interviewed healthcare team participants for their time and sharing their personal stories and for their continued service during the COVID-19 pandemic. We would also like to acknowledge Ayaba Logan, the Research and Education Informationist, Mohan Madisetti, the MUSC College of Nursing Director of Research, the staff of the MUSC Center for Academic Excellence and the reviewers of this journal for their constructive criticism.
This research (software, transcription services, etc.) was solely funded by the Principal Investigator, J.A.
Author information
Authors and affiliations.
College of Nursing, Medical University of South Carolina, Charleston, SC, USA
John W. Ambrose, Lynne S. Nemeth, Diana M. Layne & Michelle Nichols
Department of Anesthesia and Perioperative Medicine, College of Medicine, Medical University of South Carolina, Charleston, SC, USA
Ken Catchpole
Department of Surgery, College of Medicine, Medical University of South Carolina, Charleston, SC, USA
Heather L. Evans
You can also search for this author in PubMed Google Scholar
Contributions
Conceptualization J.A., K.C., L.N., D.L., H.E., and M.N.; methodology J.A. and M.N.; J.A. led the study, recruited the interviewees, conducted interviews, led the data analysis, and drafted the manuscript. J.A., and M.N. conducted the data analyses; review and editing K.C., H.E., D.L., and M.N.; supervision M.N.; research project administration J.A. and M.N.; funding acquisition J.A. All authors reviewed the manuscript.
Corresponding author
Correspondence to John W. Ambrose .
Ethics declarations
Ethics approval and consent to participate.
This study presented no greater than minimal risk to participants and met exempt status per regulatory criteria established by 45 CFR 46.104 and 21 CFR 56.104. The study protocol and all materials were approved by the MUSC Institutional Review Board (IRB), [Pro00100917 ]. All study procedures were followed in accordance with these standards.
Consent for publication
Not applicable.
Competing interests
The authors declare that they have no competing interests.
Additional information
Publisher’s note.
Springer Nature remains neutral with regard to jurisdictional claims in published maps and institutional affiliations.
Electronic supplementary material
Below is the link to the electronic supplementary material.
Supplementary Material 1
Rights and permissions.
Open Access This article is licensed under a Creative Commons Attribution 4.0 International License, which permits use, sharing, adaptation, distribution and reproduction in any medium or format, as long as you give appropriate credit to the original author(s) and the source, provide a link to the Creative Commons licence, and indicate if changes were made. The images or other third party material in this article are included in the article’s Creative Commons licence, unless indicated otherwise in a credit line to the material. If material is not included in the article’s Creative Commons licence and your intended use is not permitted by statutory regulation or exceeds the permitted use, you will need to obtain permission directly from the copyright holder. To view a copy of this licence, visit http://creativecommons.org/licenses/by/4.0/ . The Creative Commons Public Domain Dedication waiver ( http://creativecommons.org/publicdomain/zero/1.0/ ) applies to the data made available in this article, unless otherwise stated in a credit line to the data.
Reprints and permissions
About this article
Cite this article.
Ambrose, J.W., Catchpole, K., Evans, H.L. et al. Healthcare team resilience during COVID-19: a qualitative study. BMC Health Serv Res 24 , 459 (2024). https://doi.org/10.1186/s12913-024-10895-3
Download citation
Received : 25 February 2023
Accepted : 25 March 2024
Published : 12 April 2024
DOI : https://doi.org/10.1186/s12913-024-10895-3
Share this article
Anyone you share the following link with will be able to read this content:
Sorry, a shareable link is not currently available for this article.
Provided by the Springer Nature SharedIt content-sharing initiative
- Resilience Engineering
- Healthcare System
- Healthcare Administration
- Healthcare Team
- Thematic Analysis
- Qualitative Research
BMC Health Services Research
ISSN: 1472-6963
- General enquiries: [email protected]
- Skip to main content
- Keyboard shortcuts for audio player

- Your Health
- Treatments & Tests
- Health Inc.
- Public Health
An artificial womb could build a bridge to health for premature babies

Surgeon Christoph Haller and his research team from Toronto's Hospital for Sick Children are working on technology that could someday result in an artificial womb to help extremely premature babies. Chloe Ellingson for NPR hide caption
Surgeon Christoph Haller and his research team from Toronto's Hospital for Sick Children are working on technology that could someday result in an artificial womb to help extremely premature babies.
TORONTO — A surgical team scurries around a pregnant female pig lying unconscious on an operating table. They're about to take part in an experiment that could help provide a new option to help premature babies survive.
"The ultimate goal of today is to transition a fetus onto that artificial womb," says Dr. Christoph Haller , motioning to a clear rectangular plastic sack with tubes running in and out of it.
"We're transitioning it into an artificial environment that allows the fetus to still maintain its regular physiology," says Haller, a pediatric heart surgeon at The Hospital for Sick Children.
Today, it's a pig fetus that Haller and his colleagues will be using to test their artificial womb. But their hope is that someday, technology like this will help humans survive extremely premature birth and avoid serious complications, such as blindness and permanent damage to lungs and brains.

A nonprofit says preterm births are up in the U.S. — and it's not a partisan issue
"We're basically trying to find a new concept on how to preserve fetuses to allow them to mature more physiologically compared to the regular preterm. That would be the target — to treat extreme premature babies," says Haller, who's also an assistant professor of surgery at the University of Toronto. "This would hopefully be a big deal — a game changer."
NPR was granted exclusive access to watch Haller's team test their artificial womb.
Research like this is generating enormous excitement among doctors who treat babies who are born prematurely , a major cause of infant mortality and disabilities. But the prospect of an artificial womb is prompting a long list of questions.
"I think it's a really promising and fascinating technology," says Dr. Mark Mercurio , a professor of pediatrics who directs the program for biomedical ethics at the Yale School of Medicine. "But certainly it raises ethical concerns and questions that need to be addressed."
The procedure remains highly experimental
A metal tray next to the pig's belly is covered with blue paper. Haller's team just drew a picture of a pig's face on the paper surrounded by the words "Oink. Oink. Oink." and "We ❤ you." Then they laid out the artificial womb on top of it. Some call this kind of contraption a "biobag."

A technician scans the belly of a pregnant pig before an operation to transfer a fetus to an artificial womb. Chloe Ellingson for NPR hide caption
A technician scans the belly of a pregnant pig before an operation to transfer a fetus to an artificial womb.
Next, the surgical team arranges equipment and examines the 10 fetuses in the sow's womb with an ultrasound. Haller uses a clipper to make some last-minute adjustments to tubing he'll stitch into the fetal pig's umbilical cord.
The tubes will supply the fetus's blood with oxygen, remove carbon dioxide from the blood and supply nutrition and medicine.
"I'm MacGyvering stuff here to make things work," he says with a laugh.
Finally, everyone's ready to remove one of the fetuses.
"All right, I think we're going to get started," Haller says, prompting the team to gather tightly around the pig.
Wisps of smoke rise from the pig's belly as Haller makes an incision with an electric scalpel. An assistant suctions the area to keep it dry.

Dr. Christoph Haller performs surgery to remove a fetal pig from the adult pig's womb. Chloe Ellingson for NPR hide caption
Dr. Christoph Haller performs surgery to remove a fetal pig from the adult pig's womb.
"So what you're looking at is basically the uterus. And then in here is the fetus. The head's somewhere here, where I have my hand. The rest of the body is still inside," he says.
After deciding which fetus looks best on the ultrasound, Haller makes another incision in the uterus and pulls out a bright pink fetal piglet. The fetus looks peaceful, like it's sleeping.
Once the fetus is completely out, Haller and his team quickly assess its health and cut the umbilical cord so they can transfer the animal into the artificial womb.
A "biobag" becomes the new womb
After gingerly sliding the fetus into the "biobag," Haller quickly attaches the three umbilical cord tubes. His colleagues fill the bag with a clear, warm liquid meant to mimic amniotic fluid and seal the artificial womb.
"It's going to be a bit of a rocky period now," Haller says.

A fetal pig rests inside an artificial womb. Chloe Ellingson for NPR hide caption
A fetal pig rests inside an artificial womb.
The team carefully monitors the fetus's heart rate, blood pressure and other vital signs. Once it looks stable, the researchers surround the biobag with warmers.
"It's as close to a good transition as you can get I think," Haller says. "I'm excited as if it was a proper human surgery I would say — just because I want to get it right and I want to see the fetus doing well there."
This will go on for hours.
"You may see the fetus starting to have breathing-like movements. But that's what's in line with what's happening in utero too — as if they are training basically a bit. You may see that it kicks its legs," Haller says. "That's what we like to see because it signals a certain level of health."
An artificial womb could be a bridge to better health
If very premature babies can be safely sustained on a device like this for just two or three weeks, it could make all the difference between life and death or a life with severe disabilities and health problems or not, Haller says.
The Toronto group has seen blood clots and heart problems develop. So far, they've only been able to sustain a pig fetus for about a week.
But researchers at Children's Hospital of Philadelphia have safely sustained fetal sheep on a very similar device for four weeks, making the Toronto group and others optimistic the approach will eventually work.
"If this artificial womb technology could sustain a patient even for a period of weeks and get them to a later stage and a bigger size, that could potentially be quite a dramatic change in our field," says Dr. Mike Seed , an associate professor of pediatrics at the University of Toronto who is working with Haller.
Scientific progress prompts ethical concerns
But the possibility of an artificial womb is also raising many questions. When might it be safe to try an artificial womb for a human? Which preterm babies would be the right candidates? What should they be called? Fetuses? Babies?
"It matters in terms of how we assign moral status to individuals," says Mercurio, the Yale bioethicist. "How much their interests — how much their welfare — should count. And what one can and cannot do for them or to them."
But Mercurio is optimistic those issues can be resolved, and the potential promise of the technology clearly warrants pursuing it.
The Food and Drug Administration held a workshop in September 2023 to discuss the latest scientific efforts to create an artificial womb, the ethical issues the technology raises, and what questions would have to be answered before allowing an artificial womb to be tested for humans.
"I am absolutely pro the technology because I think it has great potential to save babies," says Vardit Ravitsky , president and CEO of The Hastings Center, a bioethics think tank.
But there are particular issues raised by the current political and legal environment.
"My concern is that pregnant people will be forced to allow fetuses to be taken out of their bodies and put into an artificial womb rather than being allowed to terminate their pregnancies — basically, a new way of taking away abortion rights," Ravitsky says.
She also wonders: What if it becomes possible to use artificial wombs to gestate fetuses for an entire pregnancy, making natural pregnancy unnecessary?
"Science fiction writers have been playing with this notion for decades. It's not like we never thought about it. It's just different to think about it as a thought experiment and to think about it as something that's potentially around the corner," Ravitsky says. "The scenario of a complete use of artificial wombs could become pretty scary, pretty quickly."
But Haller and his colleagues say the darkest worries are unfounded.

Shots - Health News
The u.s. has a high rate of preterm births, and abortion bans could make that worse.
"We've heard people fearing that this translates into women not having to go through a full pregnancy anymore — kind of more like a Matrix -style of dystopian future," Haller says.
"But it would be outrageous to assume that any artificial intervention in any way is better than nature. So if you're not running into problems in your pregnancy, I think there's a lot of evidence that you're better off being born as you should be from what nature intended," he says.
Haller and his colleagues, he says, are just trying to save babies.
"Every tool can be misused," he says. "Like AI — it has its benefits, but if it's not regulated adequately a lot of harm can arise from something like that as well."
Meanwhile, the fetal pig is settling into its new artificial womb.
"I think it looks pretty, pretty comfy and settled," Haller says. "It looks pretty, pretty happy in there. Yeah, it's good."
- premature mortality
- premature birth
- medical technology

An official website of the United States government
The .gov means it’s official. Federal government websites often end in .gov or .mil. Before sharing sensitive information, make sure you’re on a federal government site.
The site is secure. The https:// ensures that you are connecting to the official website and that any information you provide is encrypted and transmitted securely.
- Publications
- Account settings
Preview improvements coming to the PMC website in October 2024. Learn More or Try it out now .
- Advanced Search
- Journal List
- Sage Choice

Carrying Out Rapid Qualitative Research During a Pandemic: Emerging Lessons From COVID-19
Cecilia vindrola-padros.
1 University College London, London, United Kingdom
2 Royal College of Anaesthetists, London, United Kingdom
Georgia Chisnall
Silvie cooper, anna dowrick.
3 Queen Mary University of London, London, United Kingdom
Nehla Djellouli
Sophie mulcahy symmons.
4 University of Oxford, Oxford, United Kingdom
Georgina Singleton
Samantha vanderslott.
5 King’s College London, London, United Kingdom
Ginger A. Johnson
6 The Australian National University, Canberra, Australia
Associated Data
Supplemental material, sj-pdf-1-qhr-10.1177_1049732320951526 for Carrying Out Rapid Qualitative Research During a Pandemic: Emerging Lessons From COVID-19 by Cecilia Vindrola-Padros, Georgia Chisnall, Silvie Cooper, Anna Dowrick, Nehla Djellouli, Sophie Mulcahy Symmons, Sam Martin, Georgina Singleton, Samantha Vanderslott, Norha Vera and Ginger A. Johnson in Qualitative Health Research
Social scientists have a robust history of contributing to better understandings of and responses to disease outbreaks. The implementation of qualitative research in the context of infectious epidemics, however, continues to lag behind in the delivery, credibility, and timeliness of findings when compared with other research designs. The purpose of this article is to reflect on our experience of carrying out three research studies (a rapid appraisal, a qualitative study based on interviews, and a mixed-methods survey) aimed at exploring health care delivery in the context of COVID-19. We highlight the importance of qualitative data to inform evidence-based public health responses and provide a way forward to global research teams who wish to implement similar rapid qualitative studies. We reflect on the challenges of setting up research teams, obtaining ethical approval, collecting and analyzing data in real-time and sharing actionable findings.
Pandemics such as the COVID-19 outbreak, which began in December 2019, demand the timely sharing of not only epidemiological data but also research findings related to disease perception, social practices that might be linked to spread, health-seeking behaviors, health care delivery models, and barriers to care. Social scientists have a robust history of contributing to better understandings of and responses to infectious disease outbreaks and other emergency settings by providing this type of data (Henry, 2005; Hewlett et al., 2005 ; Koller et al., 2006 ; Koons, 2010 ). More recently, the work of social scientists during the Ebola outbreak in West Africa was actively, explicitly, and openly recruited by international outbreak response organizations such as the World Health Organization (WHO) and UNICEF ( Abramowitz, 2014 ; Anoko, 2014 ; Fairhead, 2014 ; Ferme, 2014 ; Johnson & Vindrola-Padros, 2014 ; Richards & Mokuwa, 2014 ). The implementation of qualitative research in the context of infectious epidemics, however, continues to lag behind in the delivery, credibility, and timeliness of findings when compared with other research designs.
The authors form part of the Rapid Research Evaluation and Appraisal Lab (RREAL), a research team focused on the design and implementation of rapid qualitative research on health-related topics. The purpose of this article is to illustrate the rich history of rapid qualitative research during infectious disease epidemics, including our experiences of applying these principles to research on COVID-19. We are sharing the early findings of our work during the current pandemic to highlight the importance of qualitative data to inform evidence-based public health responses, and to provide a way forward for global research teams who wish to implement similar rapid qualitative studies.
Using Qualitative Data to Inform Epidemic Response Efforts
An analysis of the engagement of social scientists in previous epidemics has pointed to a series of factors that influence when qualitative expertise is requested, how research is carried out, and how findings are shared to inform response efforts ( Sams et al., 2017 ). In the case of the Ebola outbreak in West Africa, one of the key challenges faced by social scientists related to addressing the limitations of being asked to contribute to the response at a later stage in the outbreak (e.g., during or even after the epidemiological “peak” in some cases). Timeliness in forming research teams with the required expertise to collect data on the social determinants of disease is shaped by the stage when social scientists are offered a “seat at the table” ( Martineau, 2015 ). Failure to include social science expertise in emergency planning operations limits the type of research that can be carried out (including the production of knowledge most relevant for response operations) and produces delays in the sharing of knowledge.
When offered a seat at the table, however, social scientists might still struggle to design and implement research in the context of an outbreak. For infectious epidemics and other types of complex health emergencies, qualitative research might not be allowed if deemed too intrusive or burdensome for research participants. Patients, health care workers (HCWs), public health authorities, or members of the public who are already struggling with the impact of the disease and delivery of health care response might not be able to assist with data collection or take part in studies. Furthermore, carrying out fieldwork during epidemics, where researchers often need to be in close contact with affected communities or health care facilities, exposes them to infection. Due to the immediacy of the situation, research in this context demands the sharing of findings in almost real time, requiring a type of data analysis that is not common in the social sciences. It also requires that “actionable” findings are shared. This refers to straightforward recommendations that can be easily understood and translated into changes in policy and/or practice and requires carefully planning the use of findings during the research design phase. Even if qualitative studies are produced during epidemics, public health officials might have difficulties trusting the findings, digesting the information and translating it into changes in policy and practice.
Rapid Qualitative Research
Despite these limitations and potential challenges, rapid qualitative research approaches have been used to inform response efforts in the context of infectious epidemics and natural disasters since at least 2003. In a recent review, we found that rapid qualitative research is carried out to identify the causes of the outbreak, assess the infrastructure, control strategies, health needs, and health facility use ( Johnson & Vindrola-Padros, 2017 ). Rapid qualitative research can be carried out in the difficult circumstances of an epidemic and provide findings that are timely and actionable ( Abramowitz et al., 2015 ; Faye et al., 2015 ).
The field of rapid qualitative research is diverse and various design approaches have been developed, including rapid ethnographic assessments (REAs), rapid assessment procedures (RAPs), rapid assessment response and evaluation (RARE), rapid qualitative inquiry (RQI), rapid ethnographies (including quick, focused, and short-term ethnographies), and rapid evaluations, to name a few. McNall and Foster-Fishman (2007) produced an overarching definition of all Rapid Evaluation and Appraisal Methods (REAM) arguing that the features that all of these approaches had in common were (a) the study was conducted over a short timeframe (weeks or months), (b) the study design tended to be participatory, (c) the studies combined multiple research methods and triangulated data during data analysis, and (d) the studies were iterative, in the sense that data collection and analysis tended to be carried out in parallel and emerging findings shaped the data collection process ( McNall & Foster-Fishman, 2007 ). Beebe (2014) has provided a similar definition of RQI as “intensive, team-based qualitative inquiry with (a) a focus on the insider’s or emic perspective, (b) using multiple sources and triangulation, and (c) using iterative data analysis and additional data collection to quickly develop a preliminary understanding of a situation” (p. 3).
There is a lack of consensus in relation to what is meant by “rapid,” with some authors arguing that rapid studies require 4 to 8 weeks ( Scrimshaw & Hurtado, 1987 ), 90 days ( Handwerker, 2001 ), or anywhere from a few days to 6 months ( Vindrola-Padros & Vindrola-Padros, 2018 ). These time ranges are further complicated by rapid feedback and rapid cycle evaluations that might be longer in duration (perhaps 12 months) but include feedback or cycle loops as the evaluation is ongoing to share emerging findings. In addition to these rapid research approaches, some authors have also developed rapid techniques or tools for data collection and analysis that are used to reduce the amount of time required for specific research processes, such as speeding up interview transcription or the coding of qualitative data ( Vindrola-Padros & Johnson, 2020 ). These techniques can be integrated into the rapid qualitative research approaches mentioned above or used in long-term research.
Qualitative Research During the COVID-19 Pandemic
The current COVID-19 pandemic has produced a wide range of changes in our daily lives; changes which have been shaped by the attempts of the governments of countries around the world to limit physical interaction and reduce contagion. Research evidence has occupied a central stage in informing government policies, critiquing them, guiding clinical approaches for the diagnosis and treatment of COVID-19 positive patients, and exploring the social and economic impact of control measures ( Fritz et al., 2020 ). Researchers have highlighted the importance of qualitative research, arguing that this approach can provide insight into aspects of behavior and perceptions often missed in epidemiological and clinical research as it allows us to “focus not just on ‘what’ but on ‘how’” ( Teti et al., 2020 ). Qualitative research carried out during the COVID-19 pandemic can ask and answer questions which complement epidemiological data by providing insight into people’s lived experiences of disease, care, and epidemic response efforts ( Teti et al., 2020 ). The exacerbation of social, health, and economic inequalities; the implementation of health care reorganization to address demands created by the pandemic; and the role and impact of different types of leadership at national and local levels can also be explored using qualitative research ( Shah, 2020 ; Van Bavel et al., 2020 ).
Despite highlighting the benefits of carrying out qualitative research during the COVID-19 pandemic, few authors have discussed the challenges and practical issues faced when doing this type of research and doing it in a timely way. Our expertise in rapid qualitative research and infectious epidemics has meant that our team has been heavily involved in the implementation of rapid qualitative research to inform response efforts on COVID-19 at a local and global scale. In this article, we reflect on the barriers we have encountered and the strategies we have used to address them to share key lessons learned with other teams who might be considering producing and using qualitative data to inform pandemic response efforts (now or in the future).
This article draws from our experience with three ongoing research studies, each aimed at exploring health care delivery in the context of COVID-19. The three studies outlined below have different research designs: a rapid appraisal of HCWs’ perceptions and experiences, a rapid qualitative study using in-depth interviews on the use of qualitative data during infectious epidemics (including real-time data on COVID-19 as well as previous epidemics), and a mixed-methods survey of the impact of COVID-19 on the global delivery of cancer treatment during the pandemic.
Study 1: A Rapid Appraisal of HCWs’ Perceptions and Experiences With COVID-19 in the United Kingdom and “Mirror Studies,” at a Global Scale
Previous qualitative research conducted with HCWs highlights the importance of understanding their personal experiences in providing care during periods of extreme crises, uncertainty, and where patient deaths are anticipated ( Greenberg et al., 2020 ; Ives et al., 2009 ; Kobler et al., 2020 ). This rapid appraisal of frontline HCWs’ perceptions and experiences with COVID-19 comprises three streams: a policy review, media analysis, and telephone interviews with HCWs in the United Kingdom (see Table 1 ). Following a rapid appraisal design, this study was developed to collect and analyze data in an iterative way ( Beebe, 1995 ).
Study 1 Design: Data Collection, Sampling, and Data Analysis (Study in the United Kingdom).
Note. RAP = rapid assessment procedure.
Policy review
The policy review focused on health care policies to understand changes made to health care delivery in response to COVID-19 in the United Kingdom following the rapid evidence synthesis framework proposed by Tricco and colleagues (2017) . U.K. Government policies were searched for, using the search strategy, databases, and inclusion criteria presented in Online Appendix 1 .
Media analysis
A rapid media analysis was developed to capture perceptions and experiences of HCWs reported by them or third parties. Published newspaper articles were reviewed by running a series of searches on the Nexis database (see full strategy in Online Appendix 1 and findings in Table 2 ).
Key Aspects of U.K. Newspaper Reporting of the Perceptions and Experiences of Health Care Workers With COVID-19.
The social media analysis focused on Twitter but included relevant content from Reddit and publicly available groups and accounts on Facebook and Instagram which was posted from December 1, 2019. Meltwater, a media monitoring software, was used to conduct an English language Boolean query keyword search. The search terms used from the mass media analysis were adapted (details on the categories can be found in Online Appendix 1 ). Semantic discourse and topic analysis were used to understand the most frequent and weighted key words, hashtags and prioritized discussion themes, and clusters of topics within and across countries, primarily in the U.K. context ( Van Dijk, 1985 ).
Interviews were carried out with frontline staff from NHS hospitals in the United Kingdom. Interviews were semi-structured, focusing on HCWs’ views on the virus, patients, and the health care system organization and management. A purposive sample of 130 HCWs was selected for interview to cover a range of roles within the system (the full sampling framework can be found in Online Appendix 1 ). Interviews with staff are ongoing and will continue to contribute to emerging findings. While all interviews were audio-recorded, the main points were documented and compiled with real-time interview notes and further synthesized on a RAP sheet. RAP sheets are a tool commonly used in rapid qualitative research to summarize emerging findings so they can be shared while the study is ongoing ( Beebe, 2014 ).
“Mirror studies.”
After the study was designed and approved in England, RREAL approached (or was approached by) other global research teams to determine whether they would be interested in replicating the study in their countries. The premise behind “mirror studies” was that each country would carry out the study independently, seeking local ethical approvals and managing data collection and analysis. RREAL helped facilitate the study setup by sharing our study protocol and study materials (information sheets, interview topic guide, consent form, etc.). All global teams have been in charge of making sure the findings from the studies can be used to inform local response efforts and published for academic audiences. The RREAL team will coordinate the synthesis of these published findings to create a global picture of the experiences of frontline staff during the COVID-19 pandemic. As the research is ongoing, we have also created a global virtual platform to bring all teams together to share information on the challenges of carrying out this research, and the strategies that have been used to overcome them. To date, the study is being replicated in 22 countries including United States, Mexico, Ecuador, Peru, Brazil, Chile, Argentina, France, Spain, United Kingdom, Ireland, Italy, Poland, Switzerland, Germany, Pakistan, India, Australia, South Africa, Nigeria, Democratic Republic of the Congo (DRC), and China.
Ethical review : The study was reviewed and approved by the Health Research Authority (HRA) in England (IRAS: 282069) as well as Research and Development (R&D) offices of the hospitals where the study took place. We followed an informed consent process.
Study 2: A Rapid Qualitative Study on the Use of Qualitative Data During Infectious Epidemics
The aim of this study was to explore the use of qualitative data to inform epidemic response efforts and the barriers encountered when attempting to do so. This rapid study consulted a broad, diverse, and global participant base with experience of responding to epidemic outbreaks in any capacity, all of whom were involved in responding to the COVID-19 pandemic. Participants were sampled for telephone interviews using a range of purposive and snowball methods (i.e., recruiting through affiliated epidemic response networks, listservs, and those directed to the study by those who had participated).
Individuals consulted included fellow social scientists, community engagement workers, relief coordinators, frontline clinical staff, public health registrars, guideline creators, and program managers. They worked in the following geographical areas: West Africa (including Nigeria), DRC, Kenya, India, Bangladesh, United States, Italy, and the United Kingdom. Somewhat uniquely, this study was developed prior to the COVID-19 outbreak. It was originally intended to exclusively investigate low- and middle-income countries; however, following the outbreak of COVID-19 across the world, it was agreed that it was important to open up the sample to incorporate the experiences of those responding to the current pandemic, including those from high-income countries where outbreaks might be more acute and widespread (at the time of early data collection).
The study is based on telephone/online semi-structured interviews, all of which were audio-recorded and selectively transcribed. The interviews considered the main needs of individuals responding to epidemic outbreaks, how qualitative data were used in such circumstances (with consideration to data collection, communication, timeliness, and actionability), factors enabling/preventing the use of qualitative data (e.g., political, ethical, administrative, regulatory, or logistical factors), the potential impact of successful/unsuccessful qualitative data-use in epidemic outbreaks, and lessons learnt for future epidemics. The analysis utilizes a combination of narrative description and the framework method ( Gale et al., 2013 ), for exploring the “qualitative data-use background” and developing themes in the “determinants” and “impacts” of qualitative data-use respectively.
Ethical review : The study was reviewed and approved by the UCL Ethics Committee (UCL REC): 6862/002. We followed an informed consent process.
Study 3: A Mixed-Methods Survey of the Impact of COVID-19 on the Delivery of Cancer Treatment
The COVID-19 pandemic has affected the capacity of health care systems to deliver medical services for non-COVID-19-related conditions. Many areas of the world are reporting delays in cancer diagnosis or treatments having to be put on hold or reduced to emergency cases ( Kutikov et al., 2020 ; Turaga & Girotra, 2020 ). There have been various strategies implemented at a national level and in local hospitals in an attempt to mitigate the risk of COVID-19 for cancer patients in active treatment. We developed a survey to explore the impact of COVID-19 on the delivery of cancer care, map the strategies being used around the world, and capture the learning generated in local hospitals. These findings will enable a better understanding of current measures, which will be important for informing care delivery in this pandemic and in future outbreaks.
The study was global, multidisciplinary, and cross-sectional. Qualitative and quantitative data were collected using a web-based survey instrument (Opinio 7.12). Both purposive and snowball-sampling techniques were employed to target oncology health care professionals. A multidisciplinary team of specialists and researchers developed a standardized survey. The survey questions were initially piloted within RREAL, and with clinician contacts of the principal investigator, to ensure content, language, length, and format were appropriate. The survey was refined following feedback from the pilot.
The survey was translated into Spanish and French and sent to a range of professional bodies including The International Society of Oncology Pharmacists, The U.K. Chemotherapy Board, The Clinical Oncology Society for Australia, and The Canadian Association of Pharmacy in Oncology. The professional bodies distributed the survey to their members by email link. The first page of the survey contained a description of the research, frequently asked questions, and a statement regarding consent to participate. Sample characteristics included country of practice, institution type, and professional role. The survey included a mixture of open-ended and closed-ended questions. The questions addressed the following: the current status of delivery of cancer care, the participant’s awareness of guidelines and policies concerning the prioritization and protection of patients receiving cancer care, the current strategies in place to prioritize and protect patients receiving this type of care, and the participants’ professional opinion of the strategies employed. The open-ended questions allowed us to collect qualitative data and these were particularly useful for identifying strategies used by hospitals to shield or protect cancer patients from COVID-19 additional to those offered in the survey. Participants were also able to reflect on the strategies they had considered to be the most effective. The last open-ended question in the survey asked participants if they had anything to add and several respondents used this to provide further reflection on their experience of delivering cancer care in the context of a pandemic. Participants’ responses were anonymous and data were securely stored on Opinio software. The survey results were summarized using descriptive statistics and the qualitative data obtained from free-text responses were analyzed using framework analysis performed in Excel ( Gale et al., 2013 ). The analysis process entailed an unstructured familiarization phase, a coding phase initially framed by the survey questions but open to identifying new topics emerging in the data and a final coding phase to organize the data from all participants in the form of a table.
Ethical review : The study was reviewed and approved by the UCL Research Ethics Committee (UCL REC): 6862/005. We followed an informed consent process.
We developed reflective cycles throughout the design and implementation of these studies, identifying our main concerns, problems we were facing, things we were doing well, and those we needed to improve. We documented these reflections in the form of notes, and we met as a team to discuss these data and decide on the main issues that needed to be included in this article. In this section, we discuss the main challenges that have emerged to date in the delivery of our three studies during the COVID-19 pandemic, and the strategies we have used to address them. We draw on conversations and decisions made within our research team as well as conversations with other global research teams, collaborators, ethical review boards, funding bodies, and R&D offices in local hospitals.
To Research or Not to Research?
As with any type of study, the first question we asked ourselves when designing each study was, should we be carrying out research at this time? Would our research be burdening HCWs, public health authorities, or other members of staff who are already under immense pressure? Could our studies produce more harm than benefit? Our previous experience carrying out research in the context of infectious epidemics pointed to the importance of collecting data in real time and how prospective data collection would differ from retrospective, if we decided to carry out the study at a later date and based on participant recall. We knew that data collection and analysis would be difficult as we would have to consider not only the issues in relation to accessing participants but also the emotional impact this study could have on the researchers in the team. We also knew that if we wanted to make sure our research findings could be used to inform changes in policy and practice, we would need to establish collaborations with stakeholders to understand their evidence needs and timelines early on the process of designing the studies. We reached the conclusion that it would be unethical not to carry out the studies during the pandemic, as we would be missing relevant, immediate, and actionable information that could be used to inform local and global response efforts as well as preparedness strategies for future pandemics.
Despite moving forward with the studies, we were conscious of the fact that we would need to pay close attention to our study design to reduce potential research burden, limiting the amount of time we would require from staff. To account for this, we kept our interview guides brief (i.e., 15- to 20-minute telephone interviews), we carried out interviews at times of day most convenient for participants (including lunch breaks, nights, and weekends), and considered reducing the intensity of data collection at specific time points of the pandemic (i.e., during “epidemiologic peaks”). Our experience of recruiting staff to these studies has shown that, despite feeling overstretched, many HCWs wanted to take part in the study and have indicated that the interviews were a therapeutic process, where they could freely narrate their experiences to an external party and feel that their voice was heard.
Given that RREAL research designs are applied in structure—where findings are designed to be used by national and international organizations to inform response efforts—health staff have also indicated that taking part in the studies made them feel they were making a contribution beyond care delivery. Several participants spoke about being able to share lessons with other sites/countries and contribute to future learning for responding to disease outbreaks. Even though staff members were not expressing distress during the interviews or any indication that they were burdensome (in the case of the United Kingdom), several have indicated the importance of maintaining anonymity. All of our studies follow standard ethical processes for qualitative research, which guarantee the anonymity of participants and confidentiality of the data, and we have made this clear to participants in study materials and conversations before and after interviews.
Ethical Review Processes
One important aspect of research setup we considered when thinking about the three studies was the ethical review and approval processes. Study 2 had been planned as a study before the COVID-19 pandemic began, so approvals for this study by our university research ethics committee (REC) were already in place. We did not have to make major changes to the study design as a result of COVID-19 but decided to expand the sample to include participants who had been involved in epidemic response efforts in high-income countries. We felt this would allow us to capture the experiences of some of the countries that were most affected during the most recent pandemic at the time of data collection. Study 3 also required review by a university research ethics committee, but, as it needed to be reviewed during the COVID-19 pandemic, changes to guidelines in relation to the prioritization of studies for review produced significant delays in the rollout of the survey.
An interesting experience worth mentioning in relation to Study 1 was a series of conversations that emerged when describing our study to other health services researchers and clinical colleagues and their automatic assumption that because the study was rapid, we would not be going through required ethical approval processes. This automatic association might be linked to the labeling of rapid research as a “quick and dirty” exercise ( Vindrola-Padros, 2020b ; Vindrola-Padros & Vindrola-Padros, 2018 ), or the belief that research that follows required processes will not be set up and implemented in time. We feel it is important to mention these conversations and situate them against the detailed ethical review processes described below to encourage research teams across the world to think differently about rapid qualitative research. As Beebe (1995) has argued, “rapid research” is not the same as “rushed research” and it can be carried out as rigorously as longer-term research studies.
Study 1 was based in England and required interviewing HCWs in the NHS. As a result, this study needed to be submitted to a centralized research authority board called the HRA following a relatively extensive bureaucratic process. After obtaining approval by this organization, the study would need to be reviewed and approved by the R&D offices of each hospital we hoped to recruit to the study. Fortunately for us, the HRA quickly set up a fast-track process for reviewing and approving studies on COVID-19. Our study was the first qualitative study to be approved as a fast-track study by this organization in England. A process that would normally take us several months was completed in a few weeks.
Securing R&D approvals was different and varied by hospital. While some R&Ds were able to assess their capacity to take part in the study quickly and issue an approval, others took longer and some even initially refused to process our request to take part in the study as it was not classified as a National Priority Urgent Public Health study. Only studies focused on vaccines, treatments, and diagnostic tests, and real-time collection of samples and data from people undergoing treatment could receive this classification ( National Institute for Health Research, 2020 )—limiting the research that can be carried out on the experiences and lessons learned by frontline HCWs (the focus of our study). This is an evident barrier to implementing rapid qualitative research on health services in the context of a pandemic in England. We regretted this decision and continue to find ways to make sure we can recruit the number of hospitals we originally sought to include in the study, but this new requirement might have a significant impact on our ability to document staff views and experiences and how these might differ by context.
The “mirror studies” described as part of Study 1 were dependent on each team managing the ethical review and approval processes required in their countries. Not all countries had established fast-track systems like the one described for England, and some countries relied on paper-based models that were put on hold during lockdowns. RECs were meeting remotely in some cases, but this was often less frequently. Although most teams identified this as a source of concern and potential barrier during early stages of the project, all teams were able to secure the required approvals.
Study 3 was submitted for ethical review by a university ethics committee during the COVID-19 pandemic. The university had set up a fast-track review process for all COVID-19-related research. Unfortunately, changes in guidelines for this fast-track review meant our application was put on hold for the first 2 weeks after submission as it had not been assessed by a senior member within the university. The application was further delayed by conversations with the ethics committee in relation to our sampling strategy, the scope of the study, and the dissemination of study findings. The study was approved 1 month after submission and we feel this was only as a result of our constant (sometimes daily) reminder that this was a time-sensitive study.
Building of Research Teams and Funding
Rapid qualitative research demands the rapid setup of teams and sources of funding. Some countries have published calls for COVID-19 research, giving researchers much-needed resources to increase the capacity of their teams. Other teams, like ours, do not have external funding sources (which are not tied to other projects), and this led us to be creative in the design of our rapid COVID-19 studies, distribution of workloads, and types of partnerships and collaborations established with other research teams.
To adapt to these needs, we have utilized rapid review and systematized processes for documentary data, such as media analysis and policy reviews, as these approaches reduce the number of researchers and time required for collection, cross-checking, and analysis of evidence ( Tricco et al., 2017 ). Following rapid analysis methods in the case of interviews, we have bypassed full interview transcription, and have analyzed data either directly from audio recordings or by using selected transcription ( Neal et al., 2015 ; Vindrola-Padros & Johnson, 2020). Selected transcription was carried out internally by members of the team due to limited funds for sending out recordings for full transcription to a transcription company. The selected transcription was helpful for analyses where we knew we wanted to focus on specific topics, but members of the team highlighted that having full transcripts would have allowed them to get a better sense of the complete narrative of frontline staff.
Our team has the advantage of more than 13 years of experience in the field of rapid qualitative research and involvement in informing response efforts in previous infectious disease outbreaks. However, in the case of Study 1, this is the largest team we have coordinated to carry out rapid research, and it is mainly composed of students at the MSc and PhD level who either have volunteered their time to contribute to the research or are using the research findings as part of their theses or dissertations. One way in which we have addressed the issue of different levels of expertise has been by assigning “leads” to the specific analyses we are carrying out to bring together findings from the policy reviews, media analysis, and interview data. These specific analyses are currently focusing on the main areas of concern identified by frontline staff (e.g., well-being and mental health, personal protective equipment, end of life care, the impact on the wider health care system, and gender inequalities, among others). The leads assigned to each analysis are researchers with expertise on these topics either within our team or external partners who have quickly “upskilled” and provide ongoing support to more junior researchers.
Data Collection and Analysis in Parallel to Share Emerging Findings in “Real-Time”
A central component of the three studies has been the timely sharing of findings so they could be used to inform decision making and inform changes in practice. For Study 1, there was a period during the peak time of the pandemic in the United Kingdom that we were sharing findings bi-weekly with professional organizations in charge of redesigning care delivery in acute care hospitals. This rapid turnaround of findings was facilitated by intensive rapid techniques to facilitate the collection and analysis of data in parallel. In the case of the telephone interviews, these were audio-recorded by the interviewers who also took notes of the main topics discussed during the interviews. After each interview, the interviewers summarized these notes in the form of a table called a RAP sheet. The RAP sheet acted as a working document for each researcher. As new data were collected, the main findings were added to the RAP sheet. As a result, at the end of each day, each researcher had a summary of the main findings from the study obtained to date that could be further refined and shared with our primary stakeholders. The findings were not shared in an extensive report, but in the form of a one-page table (see Figure 1 for a description of this process). We also developed an infographic to disseminate the study design and will be using it to share emerging findings ( Figure 2 ). We used a similar approach in Study 2 and shared this technique with countries taking part in the “mirror studies.”

Process used for iterative data collection, analysis, and sharing of findings.
Note. HCW = health care worker; RAP = rapid assessment procedure.

Example of infographic used to advertise Study 1.
As mentioned earlier, Study 3 used an online survey design to collect quantitative and qualitative data. Data collection and analysis were also carried out in parallel in this case as we started with analysis of the qualitative data as soon as we started receiving survey responses. This required frequent team meetings that happened weekly during more intensive stages. This early analysis allowed us to develop a coding framework that could be used by three researchers for the analysis of qualitative data.
The extent to which findings will be used to inform response efforts will depend on the research team’s capacity to engage with stakeholders. In our experience, it is always better if this is done early on in the research, not only to make sure the study is aimed at generating relevant findings but also to understand when findings are needed (Vindrola-Padros, 2020). In the case of all three studies, stakeholders were involved from the design of the research questions and remained a central component of the studies throughout all stages of the research. The type and frequency of findings they required changed through time and our team needed to be flexible enough to adapt to these changes.
In this article, we have sought to identify the most salient practical issues faced when carrying out three rapid qualitative studies during the COVID-19 pandemic. Our experiences have indicated that it is possible to implement rigorous qualitative research and deliver findings at a time when they can be used to inform changes in policy and practice. One of the first questions we faced in all three studies was deciding if we should carry out research during the pandemic. We agreed as a team that some data would always be better than no data and prospective research could capture snapshots of experience and meaning and how these changed throughout the pandemic. We did this acknowledging that there would be inherent limitations in relation to the data we could collect. We also knew that rapid qualitative research, if carried out well and responsibly, could do more good than harm if carried out before, during, and after a pandemic—but only if we were able to engage with stakeholders and share findings at a time and in a format to facilitate their use in decision-making processes. We were able to share findings at key time points because we used a series of techniques and tools commonly used in rapid qualitative research and rapid evidence synthesis.
To cover lots of ground in a speedy way, we relied on the work of a large group of researchers with different interests and levels of expertise. Each researcher made important contributions to the study, but the establishment of collaborations with other teams and incorporation of new researchers almost on an ongoing basis throughout the study demanded that we spend considerable time and energy on administrative and coordination tasks. It also meant that some researchers might have felt that they had to take on new responsibilities without feeling fully trained or prepared.
One of the main barriers in the implementation of rapid qualitative research experienced by our teams and other teams participating in the “mirror studies” were processes established by ethical review committees. In a recent publication, we have discussed proposals made by other researchers to establish separate ethical review processes for research that is deemed to be time-sensitive (Vindrola-Padros, 2020). For instance, a framework has been proposed by Tansey and colleagues (2010) , for research on emergencies, where ethical approvals need to be obtained quickly. The authors have argued that this framework requires a combination of speed, depth, and proportionality ( Tansey et al., 2010 ). The Ethics Review Board (ERB), an independent ethics committee that reviews studies carried out by non-governmental organizations such as Médecins Sans Frontieres (MSF) that can be considered time-sensitive, has also established its own ethical review framework ( Schopper et al., 2009 ).
Numerous authors have argued that ethical review processes in universities and hospitals are not designed to adequately assess qualitative studies ( Stevenson et al., 2015 ). Our experience carrying out rapid qualitative research during a pandemic has highlighted that some committees were able to develop fast-track processes that allowed us to begin research in a timely way, but ethical review still represented an important bureaucratic burden for our team and needed to be followed-up quite aggressively by our team leads. One way forward, even after the pandemic has ended, could be for ethical review committees in universities to analyze and learn from the processes used by committees used to working with time-sensitive topics such as the ERB mentioned above, instead of having to reactively improvise their own processes (some requiring complex prioritization processes such as the ones we faced in Study 3). Now that fast-track processes have been established for COVID-19 studies, another pressing question is the extent to which some of these could remain for rapid qualitative research that needs to be carried out after the pandemic ends (and for future health emergencies, in general).
In addition to the practical issues discussed so far, the experience of carrying out research during a pandemic allowed us to reflect on the value of the research we do and our responsibilities as researchers. The discussions we had with other research teams when attempting to establish collaborations for our study in the United Kingdom as well as the mirror studies in other countries pointed to the dominant perception of rapid qualitative research as low-quality or rushed research, as mentioned above. Quick associations were made between the length of the study, the extent to which the study would be reviewed by an ethical committee, the burden that would be placed on study participants, and the validity of the data collected using rapid study designs. Several authors have demonstrated that rapid qualitative research can be designed and carried out in a rigorous way ( Beebe, 1995 , 2014 ; Sangaramoorthy & Kroeger, 2020 ; Vindrola-Padros & Vindrola-Padros, 2018 ). We have also argued in favor of the need to define and describe methodologies rigorously, as well as outline how findings are used ( Johnson & Vindrola-Padros, 2017 ). We have also proposed the development of reporting and assessment standards that can take into account the unique characteristics and challenges of these types of approaches ( Vindrola-Padros, 2020b ). These standards could be helpful for teams attempting to carry out rapid research under the pressure of a pandemic like COVID-19 or for those who find themselves experimenting with rapid techniques with no prior experience in this field.
The associations between the length of the study and the quality of the data might be the product of lack of familiarity with this body of literature. However, an issue to highlight is the fact that timeliness is not included in our definition of research and this has implications in relation to our responsibility toward the topics we study and participants who share their stories with us. If we are able to generate high-quality, timely findings during a global pandemic so they can be used to inform emergency response efforts, then should it not be our responsibility to do so?
In addition to the benefits already discussed in this article, rapid qualitative research also has limitations. Our studies have been able to capture a snapshot of a pandemic that will cause tangible long-term effects on the health of populations and their health care systems. However, questions remain in relation to the medical needs of patients recovering from the disease, the effects of the pandemic on the mental health of HCWs, the effects of the pandemic on other (non-COVID-19-related) medical services, its financial impact, and the extent to which some aspects of physical distancing will become “the new normal” in social interaction and work routines.
Our study designs might also be interpreted as instrumental in the sense that all studies sought to produce findings that could be used to make changes to policy and practice, in the first instance, and considered the production of knowledge of interest to academic audiences as a secondary aim. This might have limited our engagement with theory during initial stages of study design and implementation (although we have drawn from learning and conceptual frameworks from previous epidemics). In other words, we sought to reach a balance between (a) the production of analyses that might advance our conceptualization of theory and practice in light of the extreme pressures of a pandemic, (b) with more pragmatic analyses on the concerns and experiences of frontline staff and how these might be addressed in real time. In the face of what seemed to be a never-ending increase in deaths, the loss of loved ones and colleagues, and the witnessing of the raw realities of all of the HCWs who kindly shared their stories with us, we felt it was important to ensure our findings were timely and actionable. We hope our experiences can help inform the research conducted by teams who might be grappling with similar challenges around the world.
Supplemental Material
Author biographies.
Cecilia Vindrola-Padros works as a senior research fellow in the Department of Targeted Intervention, UCL and Social Scientist at the NIAA Health Services Research Centre (HSRC), Royal College of Anaesthetists (RCoA). She Co-Directs the Rapid Research Evaluation and Appraisal Lab (RREAL) with Ginger Johnson.
Georgia Chisnall is an early career researcher with a background in psychology, recently specialising in health. Her research interests include exploring qualitative research methods and emergency healthcare response, particularly in relation to topics of humanitarian significance.
Silvie Cooper is a health sociologist and senior teaching fellow at University College London in the UK and a visiting researcher with the Sociology Department at the University of the Witwatersrand in South Africa. Her research interests include capacity building for health research, management of chronic pain, digital health and patient education, using qualitative, mixed methods, and translational research approaches.
Nehla Djellouli is a social scientist and research fellow at UCL’s Institute for Global Health. She has a background in participatory research, maternal and newborn health, health policy and evaluation.
Anna Dowrick is a medical sociologist. Her research explores the boundaries between health and other complex social issues, aiming to reframe how we understand and improve health.
Sophie Mulcahy Symmons is a Population Health MSc student at UCL with an interest in ethical research methods, qualitative research, health equity and risk communication.
Sam Martin is a Digital Sociologist and Digital Analytics Consultant at University of Oxford, she is also a Postdoctoral Fellow at the Alan Turing Institute. Her research interests lie at the intersection of social data science and digital health.
Georgina Singleton is a specialist registrar in anaesthesia. She is undertaking a fellowship with the Health Services Research Centre and has a specialist interest in qualitative research.
Samantha Vanderslott is a post-doctoral researcher and Lecturer at the Oxford Martin School and Oxford Vaccine Group at the University of Oxford working on health, society, and policy topics. She draws on perspectives from sociology, history, global public health, and science and technology studies (STS).
Norha Vera is a Social Epidemiologist focused on mental health policy and service development, particularly applying research methods that promote stakeholder involvement. She works currently as a Postdoctoral Research Associate at the NIHR Mental Health Policy Research Unit, a commission of the Department of Health that provides timely evidence to policymakers.
Ginger A. Johnson is a Medical Anthropologist who has conducted research in Africa, Asia, and the Middle East on behalf of the World Food Programme, United Nations Children’s Fund, the World Health Organization, the International Federation of Red Cross and Red Crescent Societies, United Nations Population Fund, Population Services International and United Nations High Commissioner for Refugees. She was embedded in West Africa during the 2014-2016 Ebola outbreak and is currently conducting research on polio vaccine hesitancy in Pakistan.
Author’s Note: Georgia Chisnall is also affiliated with Royal College of Anaesthetists, London, United Kingdom.
Declaration of Conflicting Interests: The authors declared no potential conflicts of interest with respect to the research, authorship, and/or publication of this article.
Funding: The authors received no financial support for the research, authorship, and/or publication of this article.

Supplemental Material: Supplemental Material for this article is available online at journals.sagepub.com/home/qhr . Please enter the article’s DOI, located at the top right hand corner of this article in the search bar, and click on the file folder icon to view.
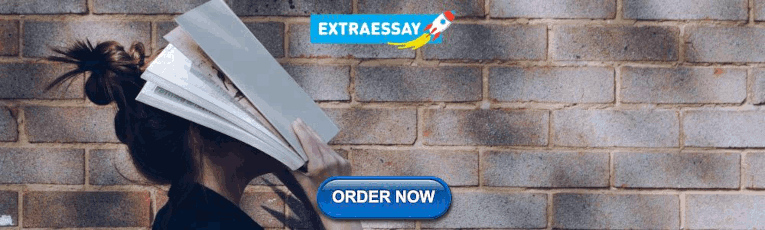
IMAGES
VIDEO
COMMENTS
Although, there is a variety of modeling approaches that have been proposed so far for prediction and evaluation of the COVID-19 disease, there are more questions that can still be answered. There are some related works that sensitivity analysis used in their studies [13,17]. They used this technique only for special variables and parameters.
Major Findings and Research Questions INTRODUCTION. The COVID-19 pandemic, which began in late 2019, created unprecedented global disruption and infused a significant level of uncertainty into the lives of individuals, both personally and professionally, around the world throughout 2020. The significant effect on vulnerable populations, such as ...
When hundreds of millions of people are vaccinated, millions of them will be afflicted anyway, in the course of life, by conditions like strokes, anaphylaxis, and Bell's palsy. "We have to have faith that people collecting the data will let us know if we are seeing those things above the baseline rate.". 3.
The impact on research in progress prior to COVID-19 was rapid, dramatic, and no doubt will be long term. The pandemic curtailed most academic, industry, and government basic science and clinical ...
The spread of the "Severe Acute Respiratory Coronavirus 2" (SARS-CoV-2), the causal agent of COVID-19, was characterized as a pandemic by the World Health Organization (WHO) in March 2020 and has triggered an international public health emergency [].The numbers of confirmed cases and deaths due to COVID-19 are rapidly escalating, counting in millions [], causing massive economic strain ...
We performed a systematic review to evaluate the methodological quality of currently available COVID-19 studies compared to historical controls. A total of 9895 titles and abstracts were screened ...
Author summary Decision-makers implement various non-pharmaceutical interventions to mitigate the COVID-19 pandemic. These include the closure of social events, restaurants, the introduction of curfews, elevated testing and quarantining of infected people. Once vaccines became available, decisions had to be made about the vaccination order, i.e. whom to vaccinate first. As the pandemic started ...
The WHO Covid-19 Research Database is a resource created in response to the Public Health Emergency of International Concern (PHEIC). Its content remains searchable and spans the time period March 2020 to June 2023. Since June 2023, manual updates to the database have been discontinued. The WHO evidence retrieval sub-group has begun ...
Travel rates. One important question about pandemic risk is what effect inter-regional travel rates have on the probability of a pandemic occurring 16,17,46,47.Here we model epidemics occurring in ...
In February 2020, we highlighted the top nine important research questions on SARS-CoV-2 and COVID-19 concerning virus transmission, asymptomatic and presymptomatic virus shedding, diagnosis, treatment, vaccine development, origin of virus and viral pathogenesis. These and related questions are revisited at the end of 2021 to shed light on the ...
In these times of rapid change, with high levels of uncertainty, anxiety, social isolation, and financial pressure, mental health worldwide is likely to be at risk. Researchers are rightly ensuring that mental health research is included in the response to the coronavirus disease 2019 (COVID-19) pandemic.1 Here, we reflect on ethical issues to consider when conducting research on self-harm ...
As the ongoing Coronavirus Disease 2019 (COVID-19) outbreak continues to change rapidly, here is information that the Harvard University research community may find helpful. We realize that the COVID-19 outbreak may cause hardship to research, however it is also important to weigh the possible harms to subjects, taking all issues into account.
This mixed-method research study delves into the repercussions of the COVID-19 pandemic on loss and mental health in Italy. The analysis uncovers a significant correlation between COVID-19 fear and heightened anxiety, depression, and stress, exacerbated by social isolation and misinformation.
Abstract. The Corona Virus Disease 2019 (COVID-19) is spreading all over the world. Quantitative analysis of the effects of various factors on the spread of the epidemic will help people better understand the transmission characteristics of SARS-CoV-2, thus providing a theoretical basis for governments to develop epidemic prevention and control ...
Qualitative and quantitative analysis of the spread trends and possible measures are extremely important for prevention and control of COVID-19. A reliable epidemiological model, which consists of a set of coupled differential equations, is a strong tool for simulating mechanism of the spreading trend and how to control the spread of the disease.
Questions focused on access to COVID-19 treatment, demographic characteristics, COVID-19 vaccine and clinical characteristics. Respondent-driven sampling was applied to recruit participants.
Research study personnel should let participants decide if they would like to wear a mask if the study procedures allow it. Researchers may wish to inform their study population of the current COVID-19 status on campus, or in the study location if elsewhere, including level of community transmission of COVID-19 and COVID-19 vaccination coverage ...
Background Clinical research has been central to the global response to COVID-19, and the United Kingdom (UK), with its research system embedded within the National Health Service (NHS), has been singled out globally for the scale and speed of its COVID-19 research response. This paper explores the impacts of COVID-19 on clinical research in an NHS Trust and how the embedded research system ...
Abstract. Wikipedia is one of the main sources of free knowledge on the Web. During the first few months of the pandemic, over 5,200 new Wikipedia pages on COVID-19 were created, accumulating over 400 million page views by mid-June 2020.1 At the same time, an unprecedented amount of scientific articles on COVID-19 and the ongoing pandemic have been published online. Wikipedia's content is ...
Background. The COVID-19 pandemic is transforming the landscape of clinical research. The pandemic has necessitated the unexpected adaptation of ongoing clinical research activities to web-based or blended design (ie, part web-based, part in-person) models [] and has rapidly accelerated a shift within clinical research toward web-based study designs.
Background Resilience, in the field of Resilience Engineering, has been identified as the ability to maintain the safety and the performance of healthcare systems and is aligned with the resilience potentials of anticipation, monitoring, adaptation, and learning. In early 2020, the COVID-19 pandemic challenged the resilience of US healthcare systems due to the lack of equipment, supply ...
Surgeon Christoph Haller and his research team from Toronto's Hospital for Sick Children are working on technology that could someday result in an artificial womb to help extremely premature babies.
Qualitative research carried out during the COVID-19 pandemic can ask and answer questions which complement epidemiological data by providing insight into people's lived experiences of disease, care, and epidemic response efforts ( Teti et al., 2020 ). The exacerbation of social, health, and economic inequalities; the implementation of health ...