
- Submit Member Login
Access provided by
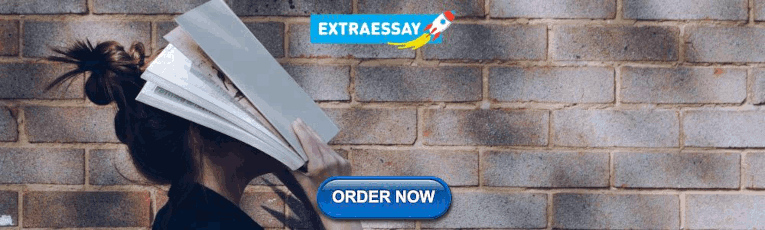
Login to your account
If you don't remember your password, you can reset it by entering your email address and clicking the Reset Password button. You will then receive an email that contains a secure link for resetting your password
If the address matches a valid account an email will be sent to __email__ with instructions for resetting your password

Download started.
- Academic & Personal: 24 hour online access
- Corporate R&D Professionals: 24 hour online access
- Add To Online Library Powered By Mendeley
- Add To My Reading List
- Export Citation
- Create Citation Alert
Medical Nutrition Therapy: A Case Based Approach
- Kathryn M. Kolasa, PhD, RDN, LDN Kathryn M. Kolasa Affiliations Brody School of Medicine at East Carolina University, 3080 Dartmouth Dr, Greenville, NC 27858 Search for articles by this author
Purchase one-time access:
Sneb member login, article info, publication history.
Inclusion of any material in this section does not imply endorsement by the Society for Nutrition Education and Behavior. Evaluative comments contained in the reviews reflect the views of the authors. Review abstracts are either prepared by the reviewer or extracted from the product literature. Prices quoted are those provided by the publishers at the time materials were submitted. They may not be current when the review is published. Reviewers receive a complimentary copy of the resource as part of the review process.
Identification
DOI: https://doi.org/10.1016/j.jneb.2022.02.003
ScienceDirect
Related articles.
- Download Hi-res image
- Download .PPT
- Access for Developing Countries
- Articles & Issues
- Articles In Press
- Current Issue
- List of Issues
- Supplements
- For Authors
- Author Guidelines
- Submit Your Manuscript
- Statistical Methods
- Guidelines for Authors of Educational Material Reviews
- Permission to Reuse
- About Open Access
- Researcher Academy
- For Reviewers
- General Guidelines
- Methods Paper Guidelines
- Qualitative Guidelines
- Quantitative Guidelines
- Questionnaire Methods Guidelines
- Statistical Methods Guidelines
- Systematic Review Guidelines
- Perspective Guidelines
- GEM Reviewing Guidelines
- Journal Info
- About the Journal
- Disclosures
- Abstracting/Indexing
- Impact/Metrics
- Contact Information
- Editorial Staff and Board
- Info for Advertisers
- Member Access Instructions
- New Content Alerts
- Sponsored Supplements
- Statistical Reviewers
- Reviewer Appreciation
- New Resources
- New Resources for Nutrition Educators
- Submit New Resources for Review
- Guidelines for Writing Reviews of New Resources for Nutrition Educators
- Podcast/Webinars
- New Resources Podcasts
- Press Release & Other Podcasts
- Collections
- Society News
The content on this site is intended for healthcare professionals.
- Privacy Policy
- Terms and Conditions
- Accessibility
- Help & Contact


- Medical Books
- Allied Health Professions
Fulfillment by Amazon (FBA) is a service we offer sellers that lets them store their products in Amazon's fulfillment centers, and we directly pack, ship, and provide customer service for these products. Something we hope you'll especially enjoy: FBA items qualify for FREE Shipping and Amazon Prime.
If you're a seller, Fulfillment by Amazon can help you grow your business. Learn more about the program.

Download the free Kindle app and start reading Kindle books instantly on your smartphone, tablet, or computer - no Kindle device required .
Read instantly on your browser with Kindle for Web.
Using your mobile phone camera - scan the code below and download the Kindle app.

Image Unavailable

- To view this video download Flash Player
Medical Nutrition Therapy: A Case Study Approach 3rd Edition
There is a newer edition of this item:.

- ISBN-10 0495554766
- ISBN-13 978-0495554769
- Edition 3rd
- Publisher Wadsworth Publishing
- Publication date August 11, 2008
- Language English
- Dimensions 8.5 x 0.75 x 11 inches
- Print length 456 pages
- See all details

Editorial Reviews
About the author, product details.
- Publisher : Wadsworth Publishing; 3rd edition (August 11, 2008)
- Language : English
- Paperback : 456 pages
- ISBN-10 : 0495554766
- ISBN-13 : 978-0495554769
- Item Weight : 2.1 pounds
- Dimensions : 8.5 x 0.75 x 11 inches
- #578 in Nutrition (Books)
- #1,406 in Diet Therapy (Books)
- #8,244 in Biology & Life Sciences
Customer reviews
Customer Reviews, including Product Star Ratings help customers to learn more about the product and decide whether it is the right product for them.
To calculate the overall star rating and percentage breakdown by star, we don’t use a simple average. Instead, our system considers things like how recent a review is and if the reviewer bought the item on Amazon. It also analyzed reviews to verify trustworthiness.
- Sort reviews by Top reviews Most recent Top reviews
Dietetic and Nutrition Case Studies
Lawrence, Judy / Douglas, Pauline / Gandy, Joan
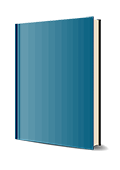
1. Edition April 2016 386 Pages, Softcover Wiley & Sons Ltd
ISBN: 978-1-118-89710-2 John Wiley & Sons
Sample Chapter
Short Description
Taking a problem-based learning approach to the subject of dietetics and nutrition, Dietetic and Nutrition Case Studies has been written to complement the internationally successful Manual of Dietetic Practice, with case studies cross-referenced accordingly. All the cases are written and peer reviewed by registered dietitians, drawing on their own experiences and specialist knowledge. Dietetic and Nutrition Case Studies is an invaluable resource for lecturers, health care students, as well as qualified dietitians and nutritionists as a tool to enhance their ongoing development.
Price: 44,90 €
Price incl. VAT, excl. Shipping
Further versions

- Description
- Author information
The ideal companion resource to 'Manual of Dietetic Practice', this book takes a problem-based learning approach to dietetics and nutrition with cases written and peer reviewed by registered dietitians, drawing on their own experiences and specialist knowledge * Each case study follows the Process for Nutrition and Dietetic Practice published by the British Dietetic Association in 2012 * Includes case studies in public health, an increasingly important area of practice
J. Lawrence, King's College London, England; P. Douglas, University of Ulster, Northern Ireland; J. Gandy, University of Hertfordshire, England
©2024 Wiley-VCH GmbH - Provider - www.wiley-vch.de - [email protected] - Data Policy - Cookie Preferences Copyright © 2000-2024 by John Wiley & Sons, Inc., or related companies. All rights reserved.


An official website of the United States government
The .gov means it’s official. Federal government websites often end in .gov or .mil. Before sharing sensitive information, make sure you’re on a federal government site.
The site is secure. The https:// ensures that you are connecting to the official website and that any information you provide is encrypted and transmitted securely.
- Publications
- Account settings
Preview improvements coming to the PMC website in October 2024. Learn More or Try it out now .
- Advanced Search
- Journal List
- J Taibah Univ Med Sci
- v.16(4); 2021 Aug
Language: English | Arabic
Introducing integrated case-based learning to clinical nutrition training and evaluating students’ learning performance
Rand j. abu farha.
a Master’s in Leadership in Health Professions Education, College of Medicine, University of Sharjah, United Arab Emirates
Mona H. Zein
b Department of Clinical Nutrition and Dietetics, College of Health Sciences, Research Institute of Medical and Health Sciences, University of Sharjah, Sharjah, United Arab Emirates
Sausan Al Kawas
c Department of Oral and Craniofacial Health Sciences, College of Dental Medicine, University of Sharjah, United Arab Emirates
Associated Data
Clinical nutrition training is an essential course for clinical nutrition and dietetics (CN&D) students. The training combines theoretical knowledge with practical skills. The goal is to prepare competent graduates for future practice. Case-based learning is an active learning method based on cases from the clinical setting. This study aimed to introduce an integrated case-based learning (ICBL) method to the clinical training of a cohort of CN&D students and to evaluate its impact on the students’ knowledge of nutrition care process.
This action research project employed an ICBL-method of teaching in the clinical training of senior students in the CN&D course at the University of Sharjah, United Arab Emirates. Ten integrated case-based learning sessions were conducted with 29 students. The record of the nutrition care process was used to evaluate the learners' performance through a pretest-posttest mechanism. Furthermore, a focus group interview was conducted to determine the impact of the ICBL-based training on the students' learning experience.
All of the students (100%) showed improvement in their learning. There was a 55% improvement in their grades. There was also self-perceived improvement of their life-long learning skills after the ICBL sessions.
Integraed case-based learning positively influenced learning among CN&D students. Consequently, the students were able to describe appropriate and individualized nutritional care plans. The students were satisfied with the training and considered ICBL to be an effective method of clinical training.
الملخص
أهداف البحث.
إن تدريب التغذية العلاجية هو مقرر أساسي يجمع بين الدراسة النظرية والمهارات العملية في المجال السريري. الهدف من التدريب هو إعداد الخريجين ليكونوا أكفاء في مستقبلهم العملي. التعلم القائم على الحالات السريرية هو مناقشة تعليمية يشارك فيها الطالب لعلاج الحالات التي يتعاملون معها خلال فترة تدريبهم. والتعليم المدمج هو طريقة تدريس تتناول الجوانب المتعددة لحالة المريض وتأثيرها على حالته التغذوية بهدف تسهيل فهم الطالب لعلاقة العلوم ببعضها. يصف هذا البحث تجربة تدريس طلبة التغذية العلاجية بطريقة التعليم المدمج القائم على الحالة وتقييم تأثيرها على أداء طلاب التغذية خلال فترة تدربيهم السريري.
طرق البحث
استهدف هذا المشروع التعليمي تدريب طلاب التغذية العلاجية في جامعة الشارقة. حيث تم عقد ١٠ جلسات تعليمية بطريقة التعليم المدمج القائم على الحالة لتسعة وعشرين طالبا في أماكن تدريبهم. واستخدم الباحثون نتائج تقريرعملية الرعاية التغذوية لتقييم الأداء التعليمي للمتدربين قبل الجلسة التعليمية وبعدها. علاوة على ذلك، أجريت مقابلة مع مجموعة من الطلاب لتوضيح تأثير هذه الطريقة في التدريس على تجربة الطلاب التعليمية.
النتائج
شهد جميع الطلاب (١٠٠ ٪) تحسنا في أدائهم التعليمي خلال تدريب التغذية العلاجية. وارتفعت درجات تقارير الطلاب بنسبة ٥٥٪ وقد صرحوا بأنهم اكتسبوا مهارات تعليمية مفيدة لحياتهم العملية بعد هذه الجلسة التعليمية.
الاستنتاجات
أظهر تدريس طلاب التغذية العلاجية بطريقة التعليم المدمج القائم على الحالة السريرية خلال فترة تدريبهم أثرا على نتائجهم العلمية وأسهم في تحسين مهاراتهم العملية، مما أدى إلى تطور قدرتهم على وصف العلاجات التغذوية. وأوصى الطلاب باعتماد هذه الطريقة التعليمية لمقرر تدريب التغذية العلاجية لما اختبروه من تطور في قدرتهم على ربط مواد التغذية العلاجية ببعضها.
Introduction
One of the major challenges of medical education is the lack of consensus on the best method of learning and integrating nutrition into clinical practice. 1 The literature on nutrition education reveals a gap in the development of innovative teaching models to enhance clinical nutrition knowledge and training. 2 Several authors have proposed that delivering nutrition education requires new techniques. 3 Therefore, student-centred teaching strategies have been developed to improve the learning process. These types of learning methods focus on educating students on how to learn actively and independently. 4 The clinical field has a complex and demanding context, which requires its educational methods to enable students to build clinical competency through the analysis of cases. 5 Case-based learning (CBL) is an educational method that provides students with a learning context similar to real practice. 6 Teaching students through active discussions of actual cases from the clinical environment supports integrated learning. 7
The clinical training course is a compulsory course that offers insight into clinical nutrition practice. The information offered in this course is vital to students, as it links together their education and professional future. This course aims to develop students' roles in establishing appropriate and individualized nutritional care plans. During several field visits that involved monitoring and supervising students during clinical training, the researchers noticed that students could not apply previous knowledge to the presented cases. Students usually study basic theoretical sciences for 3 years before hospital visits; subjects include, for example, Introduction to Nutrition, Nutrition Through the Life Cycle, Medical Nutrition Therapy, and Diet Planning. All these courses contain rich information to be used in understanding hospital cases. Therefore, real cases should be used to integrate the perception of that knowledge. However, students were not aware of how to integrate their knowledge. 8
Consequently, the students’ educational attainment was not meeting the competencies which they needed to master. Moreover, clinical training aims to prepare graduates for practice involving a multidisciplinary team working for patient care. Hence, there is a gap in studying the relationship between nutrition and other disciplines and their role in the patient care process.
It has been found that clinical nutrition cannot be learned as an isolated subject. 9 Using integrated case studies, we attempted to enable nutrition students to acquire knowledge from different disciplines and discuss its impact on the nutritional aspect of the case. 10 Interdisciplinary teaching that is provided by the process of vertical integration has been found to assist students in acquiring a complete picture of the learned topic instead of receiving incoherent information. 11 Vertical integration is an effective educational strategy that gathers several subjects together to be taught at the same time. 3 It includes presenting all the related information from previous years about a specific topic based on a clinical case and elucidates their relationships to one another. This method was considered in the literature to act as a stimulus to facilitate students’ learning process in the clinical field. In addition, it was found to facilitate holistic management of cases. 12 When it was applied in the field of nutrition, it improved the quality of the nutrition care provided by trainees. 3 , 13 , 14
The nutrition care process (NCP) is an organized, problem-solving model that provides a systematic method of providing nutritional care. It includes assessment, diagnosis, intervention, monitoring, and evaluation. 15 This model has been shown to develop critical thinking and problem-solving skills. 15 In clinical training, the NCP combines the learning of basic, applied, and clinical nutrition sciences. 14 One Canadian study found that NCP training based on case studies contributed to the acquisition of fundamental skills for professional practice among dietitians. 16 The NCP has been shown to improve documentation accuracy during learning and practice and patient care quality among dietitians. 17
In a study conducted by the Academy of Nutrition and Dietetics on the impact of CBL among nutrition students, 10 it was concluded that undergraduate nutrition students need sophisticated learning methods like case-based learning to apply their knowledge to clinical nutrition practice. Many teachers in the clinical nutrition field have used CBL during application of the nutrition care process. 10 Because effective learning methods are context-dependent, 18 the rationale for introducing ICBL is that it provides a learning environment that is similar to that of actual practice. Case-based learning provides reality-based situations while vertical integration offers a meaningful learning environment. 7 This technique can foster life-long self-learning skills and improve professional performance.
This study aimed to introduce the ICBL method in the clinical training of a cohort of CN&D students and to evaluate its impact on the students' knowledge of nutrition care process, by evaluating the trainee's performance in writing appropriate and individualized NCP records after ICBL clinical sessions.
Materials and Methods
Participants.
This research was an educational action project which applied ICBL discussion sessions to CN&D clinical training. The study was conducted in the Department of Clinical Nutrition and Dietetics at the University of Sharjah. The Clinical Nutrition and Dietetics major is a 4-year specialty. The students undergo clinical training during their fourth year (senior year), which involves hospital visits twice each week for 12 weeks. The implementation took place during the Fall semester of the fourth year, 2018–2019. Twenty-nine students were involved in the project with a participation rate of 100%, which included all the students who had undergone clinical training that semester. There was no sampling because this was an educational experiment that provided the same benefit to all students, in accordance with academic ethics.
Preparation
After the approval, an orientation session was held by the researchers for the participants. The aim of the study and the role of the students were clearly defined. Each student has signed an informed consent during that meeting.
Implementation
Ten integrated case-based learning sessions were held in the hospitals' lecture rooms during the clinical training of each group (3–4) of students. This size of the groups was based on the hospital's capacity to receive trainees, which the facilitator could not adjust. The facilitator was the author of this paper, who got training on educational methods and strategies, including ICBL, while studying for her master's degree. The cases were those of the actual patients that the students had met and reported on in their first week. The patient medical cases covered included post-bariatric surgeries, cardiovascular diseases, diabetes mellitus, and surgical and critical cases that required intensive care unit admission. Each student wrote one NCP report after attending the dietitian's assessment interview with the patient. The student/trainee collected assessment information about the medical diagnosis, medical history, medications (for any drug–nutrient interaction instructions), and nutrition-related biochemical laboratory data. These reports were used as the educational material of the ICBL discussion. For each case, medical nutrition therapy was discussed during the session, and a brief background about the disease's pathophysiology, nutrition-related risk factors, drug–nutrient interaction, and nutrition education skills used with the patients was given. The topics were discussed using vertical integration strategy. This strategy involved explaining all the related nutritional issues of the case, and at the same time, helping students understand the relevance of the information. All these topics were used to write appropriate NCP records. The teacher used the small group active discussion method, and the dialogue was dependent on the researcher's questions and students' interaction.
Each session lasted for 40 min and was broken down as follows: 5 min of explaining the teaching method, 5 min for students’ determination of their learning outcomes, 5 min for students' presentation of their submitted cases, 15 min of active discussion with the teacher, and finally, 10 min for questions and feedback.
The NCP case record ( Appendix 1 ) was adopted from the UOS to evaluate the trainee's performance in prescribing appropriate and individualized nutrition intervention. This documented case report is usually used in the Department of CN&D as a graded assignment required from the students, in order to follow their training progress. It includes collecting data and multiple questions on anthropometric measurements, medical diagnosis, medications prescribed, biochemical data, and the patient's 24-h food intake. Based on this information, students were required to assess cases by providing a proper nutritional diagnosis, diet prescription, and individualized nutritional education for each case. All the questions are short answers; some could be obtained from the patient's file, others require calculating the dietary needs, and others examine the students' problem-solving and critical thinking skills.
Mastering the skills of writing individualized nutritional management was measured by comparing students' NCP grades before and after the ICBL session. Students were given 1 week to submit 1 NCP out of 10 points. This NCP was graded and reviewed by the researcher and another faculty before implementing the project. Another clinical week after the session was given for students to submit the 2nd NCP of the same record with different cases to be considered the post-test.
Quantitative data
Pre- and post-tests were administered to compare students' NCP records grades (out of 10 points) before and after implementation of the learning sessions. The response rate for the project was 100%. A total of 29 participants responded and submitted their NCPs before their planned sessions. These NCPs were corrected and were considered the pre-test. Because the sample was less than thirty, a paired sample t-test was performed with SPSS statistical analysis software (version 17.0), as shown in Table 1 . It showed the impact of the project on students' grades. Furthermore, the statistics clarified the mean, median, and standard deviation before and after. All the participating students improved their score by an average of 3.2 points after application of the ICBL method.
Table 1
SPSS analysis of students' results before and after the project's implementation.
Additionally, Figure 1 clarifies the difference in detail by representing each student's grades before and after the learning session. The percentage of improvement detected by our pretest-posttest results was 55%. One hundred percent experienced academic improvement. These results were drawn from the increased scores of the case records (NCP). After the sessions, 75% of the students got 9.5 out of 10. The p-value for the analysis was 0.0.

Pretest-posttest NCP results.
Qualitative data
A 60-min focus group interview was conducted to investigate the effects of the sessions on the students' learning experience. Five of the students were contacted by telephone and invited to participate in the group interview. All participants provided prior written informed consent and approved the recording of the interview. The students were coded from one to five (S1–S5). The facilitator led the session by asking a previously prepared set of questions. The questions were designed to promote full discussion of the students’ perceptions regarding the learning sessions. Finally, the results were obtained using simple descriptive analysis. 19 The audio recording was transcribed and analysed several times for themes and subthemes.
The focus group interview was conducted to enrich the results with students' descriptions of the experiment. The questions were planned to measure the student's achievement of specific learning skills. The session was led objectively to allow participants to express their points of view. The results obtained from the thematic analysis of the focus group interview are summarized in Table 2 .
Table 2
Thematic analysis for the focus group interview.
This study found the ICBL method to effectively improve clinical nutrition students' training. Evidence shows that health care providers base diagnostic and therapeutic decisions on their past cases. This occurs because knowledge consists of context-similar experience in addition to theoretical information. 4 The integrating of nutrition education based on clinical cases has been found to result in the translation of knowledge of theory into clinical practice. 2 The main feature of integration is getting the benefit of learning several subjects at the same time. 12
The present study's findings were aligned with the published results in the literature on case-based and integrated learning. Harvard Medical School has introduced an integrated nutrition curriculum to enhance the way students handle nutritional sciences. It was found that active case-based learning and student-centred educational initiatives were good strategies for delivering the message. 8 Educating using integrated case studies reinforced the attainment of nutrition care process learning outcomes. 10 Boston University of Health and Rehabilitation also considered the integrated nutrition model to attain learning outcomes related to human nutrition. 14 Using integrated case studies enables nutrition students to acquire knowledge from different nutritional topics and utilize that knowledge for a better understanding of the cases. 10 One study conducted in KSA and Egypt compared problem and case-based learning during the clinical clerkship. This study showed that both methods were feasible and applicable. However, 70% of students reported that case-based learning was more effective during clinical training, in particular. 20 One post-test experimental study carried out at the University of Nebraska–Lincoln found that case-based teaching enhanced students' perceptions of the physiology course. That study showed a real increase by P < 0.04 in CBL students' performance over traditional education. 21 In comparison, the significance of this study's improvement, which was implemented in the United Arab Emirates, was 0.0. The College of Medicine at the University of Colorado has included vertically integrated nutrition in its curriculum during clinical training years since 2001 and has stressed the importance of learning nutrition by practicing in the clinical field. 14
Students’ feedback
The present authors expected small group learning to be an effective method in the study. During the focus group, the students pointed out that the ICBL session was efficient because of the small number of participating students. The participants indicated that because the sessions were more individualized it was easy and convenient to express their opinions and to learn. Similarly, the vice dean at the University of Pennsylvania found using small group, case-based discussions to teach nutrition to be highly effective. 22 Many authors have also suggested that learning in small groups gives the students a better opportunity to express themselves and to learn more. 6 , 23 Small group learning increases the levels of satisfaction among students. 24 Integrated case-based learning is considered to be a learner-centred teaching method. 25 In the present study, the students noted that the sessions seemed to be more focused on them. They also reported having more ability to acquire knowledge on their own. Canadian dietitians reported increases in students’ confidence levels after they learned and implemented the NCP. They suggested that implementing the NCP was appropriate for the clinical training objectives. 15 In the present study, the five participants in the interview strongly agreed that the ICBL sessions increased their self-confidence. During the sessions, they felt confident enough to participate and learn. After the sessions, they were more convinced of the correctness of their nutritional assessment and diet plan decisions. Other research in medical education has also found that case-based learning enhances students' confidence levels. 26
Impact of ICBL on students’ learning experience
The use of case studies has been found to be strongly associated with theoretical and practical aspects of education. 6 Students in the present study reported that the ICBL sessions helped them to integrate theoretical knowledge into their practice. After completing the sessions, students had increased their ability to integrate knowledge and were able to make better use of it. The students claimed that the integrated way of learning helped them in focusing on multiple aspects of the case. It has been suggested that case discussions reinforce student's sense of clinical relevance. The CBL-based discussions elucidated the course's clinical relevance, making it easy for students to understand the general science. 21 Cambridge University has found vertical integration to improve students' understanding of the clinical relevance of learning. They achieved a successful educational initiative using CBL as a teaching method. 14 These results foster integration, which is teaching for understanding. 21 , 27
The ICBL sessions also involved merging clinical nutrition with the medical, pharmaceutical, and biochemical aspects of cases. We planned for this based on the idea that clinical nutrition cannot be learned in isolation. 9 It has been shown that integrated case discussions enable students to recognize the relationships between clinical nutrition and other disciplines. 10 It has also been shown that interdisciplinary teaching plays an essential role in providing the students with a holistic image of the learned topic. 11 Multidisciplinary teaching is a critical aspect in nutrition education and is encouraged by Cambridge University. 3 Therefore, consistency in the delivery of information enriches the student's learning experience. Case-based learning is an inquiry-based learning method that provides students with knowledge based on the case's questions. Students need to answer the questions in a methodical, thought-out manner to reach a solution. 6
Findings of the present study suggest that ICBL creates a positive learning environment. The participants reported that the active discussion encouraged them to engage in the learning process and motivated them to learn. This outcome was in line with those of other research, which revealed that case-based learning creates an effective learning atmosphere for students. The educational environment has a crucial role in determining students’ satisfaction and readiness to learn. 27 Furthermore, it has been found that teaching nutrition in a vertically integrated, multidisciplinary approach creates an active learning environment, which leads to a distinctive learning experience. 3
Effect of ICBL on learning skills
In one study conducted at Boston University, vertically integrated learning was found to improve the learning skills related to clinical training. 28 This research involved examining the effect of integrated case discussions on students' learning skills development. As a result of active sessions, students reported that they could deeply process the information. Students could analyse the case elements effectively to solve the problem. In another study, NCP was found to improve critical thinking and problem-solving skills, which can help students later in the future when handling cases. 17 Students grew more conscious of their way of thinking and understanding the case through the discussions. Accordingly, they increased their awareness of the rationale of their nutritional decisions. One study conducted in India indicated that the CBL method facilitated the development of essential skills in clinical settings. 12 The positive effect of CBL discussion on students' critical and creative thinking has also been demonstrated in the literature. 29 Other authors also found that this method helps students develop deep learning skills, critical thinking, clinical reasoning, problem-solving, and decision-making. 5 , 6 , 10 , 26 , 29 It was found in the literature that integrated learning assisted students in developing the skills that allowed them to handle clinical cases in a comprehensive manner. 12
Interactive learning is one of the functional skills that enhances the quality of the learning experience. 5 Case-based learning offers students the opportunity to learn actively by sharing. 30 Studies have reported that active case discussions foster more effective learning. 21 , 29 These results are in line with those of the present study regarding students' perceptions of ICBL. In the present study, clinical nutrition students enjoyed interactive learning during the ICBL sessions. They preferred this method of group learning, commenting that traditional teaching methods lack this beneficial interaction. They also indicated that they learned to be more focused and precise when dealing with the information.
Studies worldwide have suggested that the implementation of integrated CBL leads to a positive learning environment and to improvement in the learning process in several aspects. 6 , 10 , 26 , 29 , 30
This study had two major limitations. The first was due to constraints during the initiation and planning phases. The work schedules of the key stakeholders were full, which made it difficult to plan meetings and discussions. It was not easy to obtain other parties' feedback on the implementation phases sequentially. However, consultations were carried out with the coordinator of the course and continuous project updates were provided. The second limitation of the study was the relatively small number of participants. A total of 29 students were included in the study, which was the total number of students enrolled in clinical training during the study. However, all participants showed positive results, and they all achieved the intended outcomes of the project.
Integrated case-based learning sessions positively influenced the learning of clinical nutrition and dietetics students. Our findings suggest that this type of active discussion strongly impacts the learning process. The results of the students’ pretest-posttest showed improvement in their nutrition care process records documentation. Also, they provided extremely positive feedback during the focus group interview. This effect was also reflected in the improvement in their academic achievement.
Moreover, this project's participants developed advanced learning skills, leading to better achievement of graduates' outcomes. Graduating qualified clinical nutrition specialists is an accomplishment to the College of Health Sciences and the university as a whole. Skilful graduates who are capable of meeting the needs of the community are always a strength to the University as an organization responsible to the community.
Recommendations
Integrated case-based learning sessions could be implemented at other Medical and Health Science Colleges, including the College of Medicine, Dental Medicine, Pharmacy, and all Health Sciences departments.
Source of funding
This research did not receive any specific grant from funding agencies in the public, commercial, or not-for-profit sectors.
Conflict of interest
The authors have no conflict of interest to declare.
Ethical approval
This study was approved by the research ethics committee at the College of Medicine, University of Sharjah on 17-02-2019 (approval number: REC-19-01-09-01).
Authors contributions
RAF conceived and designed the study, conducted research, analysed results, and drafted the manuscript. MH supervised the project's implementation and edited the manuscript. SAK contributed to the design of the project and edited the final manuscript. All authors have critically reviewed and approved the final draft and are responsible for the content and similarity index of the manuscript.
Acknowledgment
The authors gratefully acknowledge Dr. Haydar Hassan (assistant professor in the Clinical Nutrition and Dietetics Department) for his guidance in the SPSS software analysis and Dr. Moez Al-Islam Faris (associate professor in the Clinical Nutrition and Dietetics Department) for his academic consultation. They also would like to thank the class of 2018–2019.
Peer review under responsibility of Taibah University.
Appendix A Supplementary data to this article can be found online at https://doi.org/10.1016/j.jtumed.2021.03.005 .
Appendix A. Supplementary data
The following are the Supplementary data to this article:
Academia.edu no longer supports Internet Explorer.
To browse Academia.edu and the wider internet faster and more securely, please take a few seconds to upgrade your browser .
Enter the email address you signed up with and we'll email you a reset link.
- We're Hiring!
- Help Center
Facilitating transdisciplinary teamwork in dietetics education: a case study approach

2004, Journal of the American Dietetic Association
RELATED TOPICS
- We're Hiring!
- Help Center
- Find new research papers in:
- Health Sciences
- Earth Sciences
- Cognitive Science
- Mathematics
- Computer Science
- Academia ©2024
Facilitating transdisciplinary teamwork in dietetics education: a case study approach
Affiliation.
- 1 Department of Athletic Training and Physical Therapy, University of North Florida, Jacksonville, FL 32224, USA.
- PMID: 15175595
- DOI: 10.1016/j.jada.2004.03.023
Teamwork, emphasized by the transdisciplinary approach, has been strongly linked to the development of a culture of patient safety. However, the vast majority of health care education is one-dimensional, ie, focused on one discipline, taught by practitioners of the same discipline, with students of the same discipline. In an effort to address this one-dimensional view of health care education, a case-study assignment was developed to facilitate professional interaction between graduate physical therapy and graduate nutrition students. The objectives were threefold: (a). to increase student understanding of the role of another discipline, (b). to increase student ability to obtain and analyze information from a consultant relative to a patient case, and (c). to increase student communication skills across disciplines. For the assignment, physical therapy students were assigned one case scenario and one nutrition student to interview individually. The nutrition students acted as consultants in a clinical setting. The interactive assignment was considered an effective learning tool for facilitating interprofessional learning by the faculty based on student comments reporting increased understanding of the role of the other discipline, increased communication skills, and an understanding of the barriers to transdisciplinary interaction. Future studies, both quantitative and qualitative, may further identify effects of instructional activities designed to facilitate transdisciplinary health care.
- Dietetics / education*
- Education, Graduate / methods*
- Interdisciplinary Communication*
- Physical Therapy Specialty / education*
- Surveys and Questionnaires
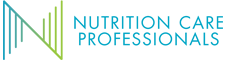
- Nutrition Assessment Blog Topics
- Nutrition Diagnosis Blog Topics
- Nutrition Intervention Blog Topics
- Nutrition Monitoring and Evaluation Blog Topics
- Related Social Media Sites
- Student Frequently Asked Questions
- US Dietetics Student Resources
- Australian Books and Cases
- Certified Dietary Managers CPE
- Canadian, Israeli, Oman, South Africa, India, Philippines (International) Students
Case Studies

Case studies demonstrate theoretical concepts in an applied setting. Nutrition Care Process and Terminology: A Practical Approach uses clinical case studies as a means of examining the Nutrition Care Process. Each NCPro case study is based on a realistic clinical encounter between client/patient and dietitian. Guided questions concerning the encounter address each stage of the NCP. Case Study Descriptions .
NCPro Cases
1. Adult Weight Management 2. Hypertension 3. Type 2 Diabetes 4. Malnutrition and Cancer 5. Pediatric Weight Management
6. Chronic Kidney Disease 7. Diverticular Disease 8. Cystic Fibrosis 9. Home Enteral Nutrition and Malnutrition 10. Celiac Disease 11. Cirrhosis (Inpatient-Electronic Medical Record) 12. Liver Disease (Outpatient-Electronic Health Record) 13. Community Nutrition - Adult Weight Management 14. Coronary Heart Disease (Telehealth - Electronic Medical Record - Interpretor) 15. Residential Care Setting (US Medicare Regulations - Electronic Medical Record) 16. Palliative Care (Home Health Record) (Round 2 Pilot testing)
17. Pediatric Food Allergy (Pilot testing in 2024)
18. Human Immunodeficiency Virus (HIV) - Hospital Medical Record (Pilot testing in 2024)
19. Managing the Consultation (self grading modules)
NCPro case studies generally provide students with
An overview (Perspective) of the health condition, including disease etiology, prevalence, presentation and diagnosis
A condition-specific NCP Mind Map summarizing relevant clinical information and key strategies to consider when providing nutrition care
Suggested reading material, including evidence-based research, guidelines and tools
A detailed clinical scenario describing an encounter between an individual and a dietitian
A series of related activities designed to support learning through the practice and application of new concepts
- Open access
- Published: 16 April 2024
How does the external context affect an implementation processes? A qualitative study investigating the impact of macro-level variables on the implementation of goal-oriented primary care
- Ine Huybrechts ORCID: orcid.org/0000-0003-0288-1756 1 , 2 ,
- Anja Declercq 3 , 4 ,
- Emily Verté 1 , 2 ,
- Peter Raeymaeckers 5 na1 &
- Sibyl Anthierens 1 na1
on behalf of the Primary Care Academy
Implementation Science volume 19 , Article number: 32 ( 2024 ) Cite this article
599 Accesses
8 Altmetric
Metrics details
Although the importance of context in implementation science is not disputed, knowledge about the actual impact of external context variables on implementation processes remains rather fragmented. Current frameworks, models, and studies merely describe macro-level barriers and facilitators, without acknowledging their dynamic character and how they impact and steer implementation. Including organizational theories in implementation frameworks could be a way of tackling this problem. In this study, we therefore investigate how organizational theories can contribute to our understanding of the ways in which external context variables shape implementation processes. We use the implementation process of goal-oriented primary care in Belgium as a case.
A qualitative study using in-depth semi-structured interviews was conducted with actors from a variety of primary care organizations. Data was collected and analyzed with an iterative approach. We assessed the potential of four organizational theories to enrich our understanding of the impact of external context variables on implementation processes. The organizational theories assessed are as follows: institutional theory, resource dependency theory, network theory, and contingency theory. Data analysis was based on a combination of inductive and deductive thematic analysis techniques using NVivo 12.
Institutional theory helps to understand mechanisms that steer and facilitate the implementation of goal-oriented care through regulatory and policy measures. For example, the Flemish government issued policy for facilitating more integrated, person-centered care by means of newly created institutions, incentives, expectations, and other regulatory factors. The three other organizational theories describe both counteracting or reinforcing mechanisms. The financial system hampers interprofessional collaboration, which is key for GOC. Networks between primary care providers and health and/or social care organizations on the one hand facilitate GOC, while on the other hand, technology to support interprofessional collaboration is lacking. Contingent variables such as the aging population and increasing workload and complexity within primary care create circumstances in which GOC is presented as a possible answer.
Conclusions
Insights and propositions that derive from organizational theories can be utilized to expand our knowledge on how external context variables affect implementation processes. These insights can be combined with or integrated into existing implementation frameworks and models to increase their explanatory power.
Peer Review reports
Contributions to literature
Knowledge on how external context variables affect implementation processes tends to be rather fragmented. Insights on external context in implementation research often remain limited to merely describing macro-context barriers and facilitators.
Organizational theories contribute to our understanding on the impact of external context to an implementation process by explaining the complex interactions between organizations and their environments.
Findings can be utilized to help explain the mechanism of change in an implementation process and can be combined with or integrated into existing implementation frameworks and models to gain a broader picture on how external context affects implementation processes.
In this study, we integrate organizational theories to provide a profound analysis on how external context influences the implementation of complex interventions. There is a growing recognition that the context in which an intervention takes place highly influences implementation outcomes [ 1 , 2 ]. Despite its importance, researchers are challenged by the lack of a clear definition of context. Most implementation frameworks and models do not define context as such, but describe categories or elements of context, without capturing it as a whole [ 2 , 3 ]. Studies often distinguish between internal and external context: micro- and meso-level internal context variables are specific to a person, team, or organization. Macro-level external context variables consist of variables on a broader, socio-economic and policy level that are beyond one’s control [ 4 ].
Overall, literature provides a rather fragmented and limited perspective on how external context influences the implementation process of a complex intervention. Attempts are made to define, categorize, and conceptualize external context [ 5 , 6 ]. Certain implementation frameworks and models specifically mention external context, such as the conceptual model of evidence-based practice implementation in public service sectors [ 7 ], the Consolidated Framework for Implementation Research [ 8 ], or the i-PARiHS framework [ 9 ]. However, they remain limited to identifying and describing external context variables. Few studies are conducted that specifically point towards the actual impact of macro-level barriers and facilitators [ 10 , 11 , 12 ] but only provide limited insights in how these shape an implementation process. Nonetheless, external contextual variables can be highly disruptive for an organization’s implementation efforts, for example, when fluctuations in funding occur or when new legislation or technology is introduced [ 13 ]. In order to build a more comprehensive view on external context influences, we need an elaborative theoretical perspective.
Organizational theories as a frame of reference
To better understand how the external context affects the implementation process of a primary care intervention, we build upon research of Birken et al. [ 13 ] who demonstrate the explanatory power of organizational theories. Organizational theories can help explain the complex interactions between organizations and their environments [ 13 ], providing understanding on the impact of external context on the mechanism of change in an implementation process. We focus on three of the theories Birken et al. [ 8 ] put forward: institutional theory, resource dependency theory, and contingency theory. We also include network theory in recognition of the importance of interorganizational context and social ties between various actors, especially in primary care settings which are characterized by a multitude of diverse actors (meaning: participants of a process).
These four organizational theories demonstrate the ways in which organizations interact with their external environment in order to sustain and fulfill their core activities. All four of them do this with a different lens. Institutional theory states that an organization will aim to fulfil the expectations, values, or norms that are posed upon them in order to achieve a fit with their environment [ 14 ]. This theory helps to understand the relationships between organizations and actors and the institutional context in which they operate. Institutions can broadly be defined as a set of expectations for social or organizational behavior that can take the form formal structures such as regulatory entities, legislation, or procedures [ 15 ]. Resource dependency theory explains actions and decisions of organizations in terms of their dependence on critical and important resources. It postulates that organizations will respond to their external environment to secure the resources they need to operate [ 16 , 17 ]. This theory helps to gain insight in how fiscal variables can shape the adoption of an innovation. Contingency theory presupposes that an organizations’ effectiveness depends on the congruence between situational factors and organizational characteristics [ 18 ]. External context variables such as social and economic change and pressure can impact the way in which an innovation will be integrated. Lastly, network theory in its broader sense underlines the strength of networks: collaborating in networks can establish an effectiveness in which outcomes are achieved that could not be realized by individual organizations acting independently. Networks are about connecting or sharing information, resources, activities, and competences of three or more organizations aiming to achieve a shared goal or outcome [ 19 , 20 ]. Investigating networks helps to gain understanding of the importance of the interorganizational context and how social ties between organizations affect the implementation process of a complex intervention.
Goal-oriented care in Flanders as a case
In this study, we focus on the implementation of the approach goal-oriented care (GOC) in primary care in Flanders, the Dutch-speaking region in Belgium. Primary care is a highly institutionalized and regulated setting with a high level of professionalism. Healthcare organizations can be viewed as complex adaptive systems that are increasingly interdependent [ 21 ]. The primary care landscape in Flanders is characterized by many primary care providers (PCPs) being either self-employed or working in group practices or community health centers. They are organized and financed at different levels (federal, regional, local). In 2015–2019, a primary care reform was initiated in Flanders in which the region was geographically divided into 60 primary care zones that are governed by care councils. The Flemish Institute of Primary Care was created as a supporting institution aiming to strengthen the collaboration between primary care health and welfare actors. The complex and multisectoral nature of primary care in Flanders forms an interesting setting to gain understanding in how macro-level context variables affect implementation processes.
The concept of GOC implies a paradigm shift [ 22 ] that shifts away from a disease or problem-oriented focus towards a person-centered focus that departs from “what matters to the patient.” Boeykens et al. [ 23 ] state in their concept analysis that GOC could be described as a healthcare approach encompassing a multifaceted, dynamic, and iterative process underpinned by the patient’s context and values. The process is characterized by three stages: goal elicitations, goal setting, and goal evaluation in which patients’ needs and preferences form the common thread. It is an approach in which PCPs and patients collaborate to identify personal life goals and to align care with those goals [ 23 ]. An illustration of how this manifests at individual level can be found in Table 1 . The concept of GOC was incorporated in Flemish policies and included in the primary care reform in 2015–2019. It has gained interest in research and policy as a potential catalyst for integrated care [ 24 ]. As such, the implementation of GOC in Flanders provides an opportunity to investigate the external context of a complex primary care intervention. Our main research question is as follows: what can organizational theories tell us about the influence of external context variables on the implementation process of GOC?
We assess the potential of four organizational theories to enrich our understanding of the impact of external context variables on implementation processes. The organizational theories assessed are as follows: institutional theory, resource dependency theory, network theory, and contingency theory. Qualitative research methods are most suitable to investigate such complex matters, as they can help answer “how” and “why” questions on implementation [ 25 ]. We conducted online, semi-structured in-depth interviews with various primary care actors. These actors all had some level of experience at either meso- or micro-level with GOC implementation efforts.
Sample selection
For our purposive sample, we used the following inclusion criteria: 1) working in a Flemish health/social care context in which initiatives are taken to implement GOC and 2) having at least 6 months of experience. For recruitment, we made an overview of all possible stakeholders that are active in GOC by calling upon the network of the Primary Care Academy (PCA) Footnote 1 . Additionally, a snowballing approach was used in which respondents could refer to other relevant stakeholders at the end of each interview. This leads to respondents with different backgrounds (not only medical) and varying roles, such as being a staff member, project coordinator, or policy maker. We aimed at a maximum variation in the type of organizations which were represented by respondents, such as different governmental institutions and a variety of healthcare/social care organizations. In some cases, paired interviews were conducted [ 26 ] if the respondents were considered complementary in terms of expertise, background, and experience with the topic. An information letter and a request to participate was send to each stakeholder by e-mail. One reminder was sent in case of nonresponse.
Data collection
Interviews were conducted between January and June 2022 by a sociologist trained in qualitative research methods. Interviewing took place online using the software Microsoft Teams and were audio-recorded and transcribed verbatim. A semi-structured interview guide was used, which included (1) an exploration of the concept of GOC and how the respondent relates to this topic, (2) questions on how GOC became a topic of interest and initiatives within the respondent’s setting, and (3) the perceived barriers and facilitators for implementation. An iterative approach was used between data collection and data analysis, meaning that the interview guide underwent minor adjustments based on proceeding insights from earlier interviews in order to get richer data.
Data analysis
All data were thematically analyzed, both inductively and deductively, supported by the software NVivo 12©. For the inductive part, implicit and explicit ideas within the qualitative data were identified and described [ 27 ]. The broader research team, with backgrounds in sociology, medical sciences, and social work, discussed these initial analyses and results. The main researcher then further elaborated this into a broad understanding. This was followed by a deductive part, in which characteristics and perspectives from organizational theories were used as sensitizing concepts, inspired by research from Birken et al. [ 13 ]. This provided a frame of reference and direction, adding interpretive value to our analysis [ 28 ]. These analyses were subject of peer debriefing with our cooperating research team to validate whether these results aligned with their knowledge of GOC processes. This enhances the trustworthiness and credibility of our results [ 29 , 30 ]. Data analysis was done in Dutch, but illustrative quotes were translated into English.
In-depth interviews were performed with n = 23 respondents (see Table 2 ): five interviews were duo interviews, and one interview took place with n = 3 respondents representing one organization. We had n = 6 refusals: n = 3 because of time restraints, n = 1 did not feel sufficiently knowledgeable about the topic, n = 1 changed professional function, and there was n = 1 nonresponse. Respondents had various ways in which they related towards the macro-context: we included actors that formed part of external context (e.g., the Flemish Agency of Care and Health), actors that facilitate and strengthen organizations in the implementation of GOC (e.g., the umbrella organization for community health centers), and actors that actively convey GOC inside and outside their setting (e.g., an autonomous and integral home care service). Interviews lasted between 47 and 72 min. Table 3 gives an overview on the main findings of our deductive analysis with their respective links to the propositions of each of the organizational theories that we applied as a lens.
Institutional theory: laying foundations for a shift towards GOC
For the implementation of GOC in primary care, looking at the data with an institutional theory lens helps us understand the way in which primary care organizations will respond to social structures surrounding them. Institutional theory describes the influence of institutions, which give shape to organizational fields: “organizations that, in the aggregate, constitute a recognized area of institutional life [ 31 ], p. 148. Prevailing institutions within primary care in Flanders can affect how organizations within such organizational fields fulfil their activities. Throughout our interviews, we recognized several dynamics that are being described in institutional theory.
First of all, the changing landscape of primary care in Flanders (see 1.2) was often brought up as a dynamic in which GOC is intertwined with other changes. Respondents mention an overall tendency to reform primary care to becoming more integrated and the ideas of person-centered care becoming more upfront. These expectations in how primary care should be approached seem to affect the organizational field of primary care: “You could tell that in people’s minds they are ready to look into what it actually means to put the patient, the person central. — INT01” Various policy actors are committed to further steer towards these approaches: “the government has called it the direction that we all have to move towards. — INT23” It was part of the foundations for the most recent primary care reform, leading to the creation of demographic primary care zones governed by care councils and the Flemish Institute of Primary Care as supporting institution.
These newly established actors were viewed by our respondents as catalysts of GOC. They pushed towards the aims to depart from local settings and to establish connections between local actors. Overall, respondents emphasized their added value as they are close to the field and they truly connect primary care actors. “They [care councils] have picked up these concepts and have started working on it. At the moment they are truly the incubators and ecosystems, as they would call it in management slang. — INT04” For an innovation such as GOC to be diffused, they are viewed as the ideal actors who can function as a facilitator or conduit. They are uniquely positioned as they are closely in contact with the practice field and can be a top-down conduit for governmental actors but also are able to address the needs from bottom-up. “In this respect, people look at the primary care zones as the ideal partners. […] We can start bringing people together and have that helicopter view: what is it that truly connects you? — INT23” However, some respondents also mentioned their difficult governance structure due to representation of many disciplines and organizations.
Other regulatory factors were mentioned by respondents were other innovations or changes in primary care that were intentionally linked to GOC: e.g., the BelRAI Footnote 2 or Flemish Social Protection Footnote 3 . “The government also provides incentives. For example, family care services will gradually be obliged to work with the BelRAI screener. This way, you actually force them to start taking up GOC. — INT23” For GOC to be embedded in primary care, links with other regulatory requirements can steer PCPs towards GOC. Furthermore, it was sometimes mentioned that an important step would be for the policy level to acknowledge GOC as quality of care and to include the concept in quality standards. This would further formalize and enforce the institutional expectation to go towards person-centered care.
Currently, a challenge on institutional level as viewed by most respondents is that GOC is not or only to a limited extent incorporated in the basic education of most primary care disciplines. This leads to most of PCPs only having a limited understanding of GOC and different disciplines not having a shared language in this matter. “You have these primary health and welfare actors who each have their own approach, history and culture. To bring them together and to align them is challenging. — INT10” The absence of GOC as a topic in basic education is mentioned by various respondents as a current shortcoming in effectively implementing GOC in the wider primary care landscape.
Overall, GOC is viewed as our respondents as a topic that has recently gained a lot interest, both by individual PCPS, organizations, and governmental actors. The Flemish government has laid some foundations to facilitate this change with newly created institutions and incentives. However, other external context variables can interfere in how the concept of GOC is currently being picked up and what challenges arise.
Resource dependency theory: in search for a financial system that accommodates interprofessional collaboration
Another external context variable that affects how GOC can be introduced is the financial system that is at place. To analyze themes that were raised during the interviews with regard to finances, we utilized a resource dependency perspective. This theory presumes that organizations are dependent on financial resources and are seeking ways to ensure their continued functioning [ 16 , 17 ]. To a certain extent, this collides with the assumptions of institutional theory that foregrounds organization’s conformity to institutional pressures [ 32 ]. Resource dependency theory in contrast highlights differentiation of organizations that seek out competitive advantages [ 32 ].
In this context, respondents mention that their interest and willingness to move towards a GOC approach are held back by the current dominant system of pay for performance in the healthcare system. This financial system is experienced as restrictive, as it does not provide any incentive to PCPs for interprofessional collaboration, which is key for GOC. A switch to a flat fee system (in which a fixed fee is charged for each patient) or bundled payment was often mentioned as desirable. PCPs and health/social care organizations working in a context where they are financially rewarded for a trajectory or treatment of a patient in its entirety ensure that there is no tension with their necessity to obtain financial resources, as described in the resource dependency theory. Many of our respondents voice that community health centers are a good example. They cover different healthcare disciplines and operate with a fixed price per enrolled patient, regardless of the number of services for that patient. This promotes setting up preventive and health-promoting actions, which confirms our finding on the relevance of dedicated funding.
At the governmental level, the best way to finance and give incentives is said to be a point of discussion: “For years, we have been arguing about how to finance. Are we going to fund counsel coordination? Or counsel organization? Or care coordination? — INT04” Macro-level respondents do however mention financial incentives that are already in place to stimulate interprofessional collaboration: fees for multidisciplinary consultation being the most prominent. Other examples were given in which certain requirements were set for funding (e.g., Impulseo Footnote 4 , VIPA Footnote 5 ) that stimulate actors or settings in taking steps towards more interprofessional collaboration.
Nowadays, financial incentives to support organizations to engage in GOC tend to be project grants. However, a structural way to finance GOC approaches is currently lacking, according to our respondents. As a consequence, a long-term perspective for organizations is lacking; there is no stable financing and organizations are obliged to focus on projects instead of normalizing GOC in routine practice. According to a resource dependency perspective, the absence of financial incentives for practicing GOC hinders organizations in engaging with the approach, as they are focused on seeking out resources in order to fulfil their core activities.
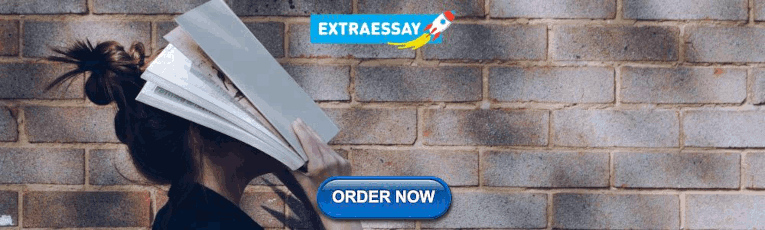
A network-theory perspective: the importance of connectedness for the diffusion of an innovation
Throughout the interviews, interorganizational contextual elements were often addressed. A network theory lens states that collaborating in networks can lead to outcomes that could not be realized by individual organizations acting independently [ 19 , 20 ]. Networks consist of a set of actors such as PCPs or health/social care organizations along with a set of ties that link them [ 33 ]. These ties can be state-type ties (e.g., role based, cognitive) or event-type ties (e.g., through interactions, transactions). Both type of ties can enable a flow in which information or innovations can pass, as actors interact [ 33 ]. To analyze the implementation process of GOC and how this is diffused through various actors, a network theory perspective can help understand the importance of the connection between actors.
A first observation throughout the interviews in which we notice the importance of networks was in the mentioning of local initiatives that already existed before the creation of the primary care zones/care councils. In the area around Ghent, local multidisciplinary networks already organized community meetings, bringing together different PCPs on overarching topics relating to long-term care for patients with chronic conditions. These regions have a tradition of collaboration and connectedness of PCPs, which respondents mention to be highly valuable: “This ensures that we are more decisive, speaking from one voice with regards to what we want to stand for. — INT23” Respondents voice that the existence of such local networks has had a positive effect on the diffusion of ideas such as GOC, as trust between different actors was already established.
Further mentioning of the importance of networks could be found in respondents acknowledging one of the presumptions of network theory: working collaboratively towards a specific objective leads to outcomes that cannot be realized independently. This is especially true for GOC, an approach that in essence requires different disciplines to work together: “When only one GP, nurse or social worker starts working on it, it makes no sense. Everyone who is involved with that person needs to be on board. Actually, you need to finetune teams surrounding a person — INT11.” This is why several policy-level respondents mentioned that emphasis was placed on organizing GOC initiatives in a neighborhood-oriented way, in which accessible, inclusive care is aimed at by strengthening social cohesion. This way, different types of PCPs got to know each other through these sessions an GOC and would start to get aligned on what it means to provide GOC. However, in particular, self-employed PCPs are hard to reach. According to our respondents, occupational groups and care councils are suitable actors to engage these self-employed PCPs, but they are not always much involved in such a network .
To better connect PCPs and health/social care organizations, the absence of connectedness through the technological landscape is also mentioned. Current technological systems and platforms for documenting patient information do not allow for aligning and sharing between disciplines. In Flanders, there is a history of each discipline developing its own software, which lacks centralization or unification: “For years, they have decided to just leave it to the market, in such a way that you ended up with a proliferation of software, each discipline having its own package. — INT06” Most of the respondents mentioning this were aware that Flanders government is currently working on a unified digital care and support platform and were optimistic about its development.
Contingency theory: how environmental pressure can be a trigger for change
Our interviews were conducted during a rather dynamic and unique period of time in which the impact of social change and pressure was clearly visible: the Flemish primary care reform was ongoing which leads to the creation of care councils and VIVEL (see 3.1.1), and the COVID crisis impacted the functioning of these and other primary care actors. These observed effects of societal changes are reminiscent of the assumptions that are made in contingency theory. In essence, contingency theory presupposes that “organizational effectiveness results from fitting characteristics of the organization, such as its structure, to contingencies that reflect the situation of the organization [ 34 ], p. 1.” When it comes to the effects of the primary care reform and the COVID crisis, there were several mentions on how primary care actors reorganized their activities to adapt to these circumstances. Representatives of care councils/primary care zones whom we interviewed underlined that they were just at the point where they could again engage with their original action plans, not having to take up so many COVID-related tasks anymore. On the one hand, the COVID crisis had however forced them to immediately become functional and has also contributed that various primary care actors quickly got to know them. On the other hand, the COVID crisis has also kept them from their core activities for a while. On top of that, the crisis has also triggered a change the overall view towards data sharing. Some respondents mention a rather protectionist approach towards data sharing, while data sharing has become more normalized during the COVID crisis. This discussion was also relevant for the creation of a unified shared patient record in terms of documenting and sharing patient goals.
Other societal factors that were mentioned having an impact on the uptake of GOC are the demographic composition of a certain area. It was suggested that areas that are characterized by a patient population with more chronic care needs will be more likely to steer towards GOC as a way of coping with these complex cases. “You always have these GPs who blow it away immediately and question whether this is truly necessary. They will only become receptive to this when they experience needs for which GOC can be a solution — INT11.” On a macro-level, several respondents have mentioned how a driver for change is to have the necessity for change becoming very tangible. As PCPs are confronted with increasing numbers of patients with complex, chronic needs and their work becomes more demanding, the need for change becomes more acute. This finding is in line with what contingency theory underlines: changes in contingency (e.g., the population that is increasingly characterized by aging and multimorbidity) are an impetus for change for health/social care organizations to resolve this by adopting a structure that better fits the current environmental characteristics [ 34 ].
Our research demonstrates the applicability of organizational theories to help explain the impact that macro-level context variables have on an implementation process. These insights can be integrated into existing implementation frameworks and models to add the explanatory power of macro-level context variables, which is to date often neglected. The organizational theories demonstrate the ways in which organizations interact with their external environment in order to sustain and fulfill their core activities. As demonstrated in Fig. 1 , institutional theory largely explains how social expectations in the form of institutions lead towards the adoption or implementation of innovation, such as GOC. However, other organizational theories demonstrate how other macro-context elements on different areas can either strengthen or hamper the implementation process.
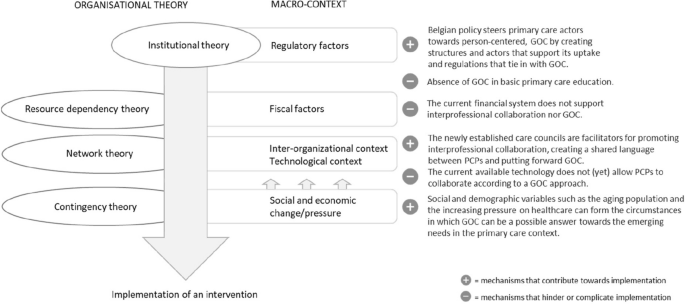
How organizational theories can help explain the way in which macro-level context variables affect implementation of an intervention
Departing from the mechanisms that are postulated by institutional theory, we observed that the shift towards GOC is part of a larger Flemish primary care reform in which and new institutions have been established and polices have been drawn up to go towards more integrated, person-centered care. To achieve this, governmental actors have placed emphasis on socialization of care, the local context, and establishing ties between organizations in order to become more complementary in providing primary health care [ 35 ]. With various initiatives surrounding this aim, the Flemish government is steering towards GOC. This is reminiscent of the mechanisms that are posed within institutional theory: organizations adapt to prevailing norms and expectations and mimic behaviors that are surrounding them [ 15 , 36 ].
Throughout our data, we came across concrete examples of how institutionalization takes place. DiMaggio and Powell [ 31 ] describe the subsequent process of isomorphism: organizations start to resemble each other as they are conforming to their institutional environment. A first mechanism through which this change occurs is coercive isomorphism and is clearly noticeable in our data. This type of isomorphism results from both formal and informal pressure coming from organizations from which a dependency relationship exists and from cultural expectations in the society [ 31 ]. Person-centered, GOC care is both formally propagated by governmental institutions and procedures and informally expected by current social tendencies. Care councils within primary care zones explicitly propagate and disseminate ideas and approaches that are desirable on policy level. Another form of isomorphism is professional isomorphism and relates to our finding that incorporation of GOC in basic education is currently lacking. The presumptions of professional isomorphism back up the importance of this: values, norms, and ideas that are developed during education are bound to find entrance within organizations as professionals start operating along these views.
Although many observations in our data back up the assumptions of institutional theory, it should be noticed that new initiatives such as the promotion of person-centered care and GOC can collide with earlier policy trends. Martens et al. [ 12 ] have examined the Belgian policy process relating three integrated care projects and concluded that although there is a strong support for a change towards a more patient-centered system, the current provider-driven system and institutional design complicate this objective. Furthermore, institutional theory tends to simplify actors as passive adopters of institutional norms and expectations and overlook the human agency and sensemaking that come with it [ 37 ]. For GOC, it is particularly true that PCPs will actively have to seek out their own style and fit the approach in their own way of working. Moreover, GOC was not just addressed as a governmental expectation but for many PCPs something they inherently stood behind.
Resources dependency theory poses that organizations are dependent on critical resources and adapt their way of working in response to those resources [ 17 ]. From our findings, it seems that the current financial system does not promote GOC, meaning that the mechanisms that are put forward in resources dependency theory are not set in motion. A macro-level analysis of barriers and facilitators in the implementation of integrated care in Belgium by Danhieux et al. [ 10 ] also points towards the financial system and data sharing as two of the main contextual determinants that affect implementation.
Throughout our data, the importance of a network approach was frequently mentioned. Interprofessional collaboration came forward as a prerequisite to make GOC happen, as well as active commitment on different levels. Burns, Nembhard, and Shortell [ 38 ] argue that research efforts on implementing person-centered, integrated care should have more focus on the use of social networks to study relational coordination. In terms of interprofessional collaboration, to date, Belgium has a limited tradition of working team-based with different disciplines [ 35 ]. However, when it comes to strengthening a cohesive primary care network, the recently established care councils have become an important facilitator. As a network governance structure, they resemble mostly a Network Administrative Organization (NAO): a separate, centralized administrative entity that is externally governed and not another member providing its own services [ 19 ]. According to Provan and Kenis [ 19 ], this type of governance form is most effective in a rather dense network with many participants, when the goal consensus is moderately high, characteristics that are indeed representative for the Flemish primary care landscape. This strengthens our observation that care councils have favorable characteristics and are well-positioned to facilitate the interorganizational context to implement GOC.
Lastly, the presumptions within contingency theory became apparent as respondents talked about how the need for change needs to become tangible for PCPs and organizations to take action, as they are increasingly faced with a shortage of time and means and more complex patient profiles. Furthermore, De Maeseneer [ 39 ] affirms our findings that the COVID-19 crisis could be employed as an opportunity to strengthen primary health care, as health becomes prioritized and its functioning becomes re-evaluated. Overall, contingency theory can help gain insight in how and why certain policy trends or decisions are made. A study of Bruns et al. [ 40 ] found that modifiable external context variables such as interagency collaboration were predictive for policy support for intervention adoption, while unmodifiable external context variable such as socio-economic composition of a region was more predictive for fiscal investments that are made.
Strengths and limitations
This study contributes to our overall understanding of implementation processes by looking into real-life implementation efforts for GOC in Flanders. It goes beyond a mere description of external context variables that affect implementation processes but aims to grasp which and how external context variables influence implementation processes. A variety of respondents from different organizations, with different backgrounds and perspectives, were interviewed, and results were analyzed by researchers with backgrounds in sociology, social work, and medical sciences. Results can not only be applied to further develop sustainable implementation plans for GOC but also enhance our understanding of how the external context influences and shapes implementation processes. As most research on contextual variables in implementation processes has until now mainly focused on internal context variables, knowledge on external context variables contributes to gaining a bigger picture of the mechanism of change.
However, this study is limited to the Flemish landscape, and external context variables and their dynamics might differ from other regions or countries. Furthermore, our study has examined and described how macro-level context variables affect the overall implementation processes of GOC. Further research is needed on the link between outer and inner contexts during implementation and sustainment, as explored by Lengninck-Hall et al. [ 41 ]. Another important consideration is that our sample only includes the “believers” in GOC and those who are already taking steps towards its implementation. It is possible that PCPs themselves or other relevant actors who are more skeptical about GOC have a different view on the policy and organizational processes that we explored. Furthermore, data triangulations in which this data is complemented with document analysis could have expanded our understanding and verified subjective perceptions of respondents.
Insights and propositions that derive from organizational theories can be utilized to expand our knowledge on how external context variables affect implementation processes. Our research demonstrates that the implementation of GOC in Flanders is steered and facilitated by regulatory and policy variables, which sets in motion mechanisms that are described in institutional theory. However, other external context variables interact with the implementation process and can further facilitate or hinder the overall implementation process. Assumptions and mechanisms explained within resource dependency theory, network theory, and contingency theory contribute to our understanding on how fiscal, technological, socio-economic, and interorganizational context variables affect an implementation process.
Availability of data and materials
The datasets generated and/or analyzed during the current study are not publicly available due to confidentiality guaranteed to participants but are available from the corresponding author on reasonable request.
The Primary Care Academy (PCA) is a research and teaching network of four Flemish universities, six university colleges, the White and Yellow Cross (an organization for home nursing), and patient representatives that have included GOC as one of their main research domains.
BelRAI, the Belgian implementation of the interRAI assessment tools; these are scientific, internationally validated instruments enabling an assessment of social, psychological, and physical needs and possibilities of individuals in different care settings. The data follows the person and is shared between care professionals and care organizations.
The Flemish Social Protection is a mandatory insurance established by the Flemish government to provide a range of concessions to individuals with long-term care and support needs due to illness or disability.
Impulseo, financial support for general practitioners who start an individual practice or join a group practice
VIPA, grants for the realization of sustainable, accessible, and affordable healthcare infrastructure
Abbreviations
- Goal-oriented care
Primary care provider
Primary Care Academy
Squires JE, Graham ID, Hutchinson AM, Michie S, Francis JJ, Sales A, et al. Identifying the domains of context important to implementation science: a study protocol. Implement Sci. 2015;10(1):1–9.
Article Google Scholar
Nilsen P, Bernhardsson S. Context matters in implementation science: a scoping review of determinant frameworks that describe contextual determinants for implementation outcomes. BMC Health Serv Res. 2019;19(1):1–21.
Rogers L, De Brún A, McAuliffe E. Defining and assessing context in healthcare implementation studies: a systematic review. BMC Health Serv Res. 2020;20(1):1–24.
Huybrechts I, Declercq A, Verté E, Raeymaeckers P, Anthierens S. The building blocks of implementation frameworks and models in primary care: a narrative review. Front Public Health. 2021;9:675171.
Article PubMed PubMed Central Google Scholar
Hamilton AB, Mittman BS, Eccles AM, Hutchinson CS, Wyatt GE. Conceptualizing and measuring external context in implementation science: studying the impacts of regulatory, fiscal, technological and social change. Implement Sci. 2015;10 BioMed Central.
Watson DP, Adams EL, Shue S, Coates H, McGuire A, Chesher J, et al. Defining the external implementation context: an integrative systematic literature review. BMC Health Serv Res. 2018;18(1):1–14.
Aarons GA, Hurlburt M, Horwitz SM. Advancing a conceptual model of evidence-based practice implementation in public service sectors. Adm Policy Ment Health Ment Health Serv Res. 2011;38:4–23.
Damschroder LJ, Aron DC, Keith RE, Kirsh SR, Alexander JA, Lowery JC. Fostering implementation of health services research findings into practice: a consolidated framework for advancing implementation science. Implement Sci. 2009;4(1):1–15.
Harvey G, Kitson A. PARIHS revisited: from heuristic to integrated framework for the successful implementation of knowledge into practice. Implement Sci. 2015;11(1):1–13.
Danhieux K, Martens M, Colman E, Wouters E, Remmen R, Van Olmen J, et al. What makes integration of chronic care so difficult? A macro-level analysis of barriers and facilitators in Belgium. International. J Integr Care. 2021;21(4).
Hamilton AB, Mittman BS, Campbell D, Hutchinson C, Liu H, Moss NJ, Wyatt GE. Understanding the impact of external context on community-based implementation of an evidence-based HIV risk reduction intervention. BMC Health Serv Res. 2018;18(1):1–10.
Martens M, Danhieux K, Van Belle S, Wouters E, Van Damme W, Remmen R, et al. Integration or fragmentation of health care? Examining policies and politics in a Belgian case study. Int J Health Policy Manag. 2022;11(9):1668.
PubMed Google Scholar
Birken SA, Bunger AC, Powell BJ, Turner K, Clary AS, Klaman SL, et al. Organizational theory for dissemination and implementation research. Implement Sci. 2017;12(1):1–15.
Powell WW, DiMaggio PJ. The new institutionalism in organizational analysis. University of Chicago Press; 2012.
Google Scholar
Zucker LG. Institutional theories of organization. Annu Rev Sociol. 1987;13(1):443–64.
Hillman AJ, Withers MC, Collins BJ. Resource dependence theory: a review. J Manag. 2009;35(6):1404–27.
Nienhüser W. Resource dependence theory-how well does it explain behavior of organizations? Management Revue; 2008. p. 9–32.
Lammers CJ, Mijs AA, Noort WJ. Organisaties vergelijkenderwijs: ontwikkeling en relevantie van het sociologisch denken over organisaties. Het Spectrum. 2000;6.
Provan KG, Kenis P. Modes of network governance: structure, management, and effectiveness. J Public Adm Res Theory. 2008;18(2):229–52.
Kenis P, Provan K. Het network-governance-perspectief. Business performance management Sturen op prestatie en resultaat; 2008. p. 296–312.
Begun JW, Zimmerman B, Dooley K. Health care organizations as complex adaptive systems. Adv Health Care Org Theory. 2003;253:288.
Mold JW. Failure of the problem-oriented medical paradigm and a person-centered alternative. Ann Fam Med. 2022;20(2):145–8.
Boeykens D, Boeckxstaens P, De Sutter A, Lahousse L, Pype P, De Vriendt P, et al. Goal-oriented care for patients with chronic conditions or multimorbidity in primary care: a scoping review and concept analysis. PLoS One. 2022;17(2):e0262843.
Article CAS PubMed PubMed Central Google Scholar
Gray CS, Grudniewicz A, Armas A, Mold J, Im J, Boeckxstaens P. Goal-oriented care: a catalyst for person-centred system integration. Int J Integr Care. 2020;20(4).
Hamilton AB, Finley EP. Qualitative methods in implementation research: an introduction. Psychiatry Res. 2019;280:112516.
Wilson AD, Onwuegbuzie AJ, Manning LP. Using paired depth interviews to collect qualitative data. Qual Rep. 2016;21(9):1549.
Guest G, MacQueen KM, Namey EE. Applied thematic analysis. Sage Publications; 2011.
Bowen GA. Grounded theory and sensitizing concepts. Int J Qual Methods. 2006;5(3):12–23.
Connelly LM. Trustworthiness in qualitative research. Medsurg Nurs. 2016;25(6):435.
Morse JM, Barrett M, Mayan M, Olson K, Spiers J. Verification strategies for establishing reliability and validity in qualitative research. Int J Qual Methods. 2002;1(2):13–22.
DiMaggio PJ, Powell WW. The iron cage revisited: institutional isomorphism and collective rationality in organizational fields. Am Sociol Rev. 1983;147-60.
de la Luz F-AM, Valle-Cabrera R. Reconciling institutional theory with organizational theories: how neoinstitutionalism resolves five paradoxes. J Organ Chang Manag. 2006;19(4):503–17.
Borgatti SP, Halgin DS. On network theory. Organ Sci. 2011;22(5):1168–81.
Donaldson L. The contingency theory of organizations. Sage; 2001.
Book Google Scholar
De Maeseneer J, Galle A. Belgium’s healthcare system: the way forward to address the challenges of the 21st century: comment on “Integration or Fragmentation of Health Care? Examining Policies and Politics in a Belgian Case Study”. Int J Health Policy Manag. 2023;12.
Dadich A, Doloswala N. What can organisational theory offer knowledge translation in healthcare? A thematic and lexical analysis. BMC Health Serv Res. 2018;18(1):1–20.
Jensen TB, Kjærgaard A, Svejvig P. Using institutional theory with sensemaking theory: a case study of information system implementation in healthcare. J Inf Technol. 2009;24(4):343–53.
Burns LR, Nembhard IM, Shortell SM. Integrating network theory into the study of integrated healthcare. Soc Sci Med. 2022;296:114664.
Article PubMed Google Scholar
De Maeseneer J. COVID-19: using the crisis as an opportunity to strengthen primary health care. Prim Health Care Res Dev. 2021;22:e73.
Bruns EJ, Parker EM, Hensley S, Pullmann MD, Benjamin PH, Lyon AR, Hoagwood KE. The role of the outer setting in implementation: associations between state demographic, fiscal, and policy factors and use of evidence-based treatments in mental healthcare. Implement Sci. 2019;14:1–13.
Lengnick-Hall R, Stadnick NA, Dickson KS, Moullin JC, Aarons GA. Forms and functions of bridging factors: specifying the dynamic links between outer and inner contexts during implementation and sustainment. Implement Sci. 2021;16:1–13.
Download references
Acknowledgements
We are grateful for the partnership with the Primary Care Academy (academie-eerstelijn.be) and want to thank the King Baudouin Foundation and Fund Daniël De Coninck for the opportunity they offer us for conducting research and have impact on the primary care of Flanders, Belgium. The consortium of the Primary Care Academy consists of the following: lead author: Roy Remmen—[email protected]—Department of Primary Care and Interdisciplinary Care, Faculty of Medicine and Health Sciences, University of Antwerp, Antwerp, Belgium; Emily Verté—Department of Primary Care and Interdisciplinary Care, Faculty of Medicine and Health Sciences, University of Antwerp, Antwerp, Belgium, and Department of Family Medicine and Chronic Care, Faculty of Medicine and Pharmacy, Vrije Universiteit Brussel, Brussel, Belgium; Muhammed Mustafa Sirimsi—Centre for Research and Innovation in Care, Faculty of Medicine and Health Sciences, University of Antwerp, Antwerp, Belgium; Peter Van Bogaert—Workforce Management and Outcomes Research in Care, Faculty of Medicine and Health Sciences, University of Antwerp, Belgium; Hans De Loof—Laboratory of Physio-Pharmacology, Faculty of Pharmaceutical Biomedical and Veterinary Sciences, University of Antwerp, Belgium; Kris Van den Broeck—Department of Primary Care and Interdisciplinary Care, Faculty of Medicine and Health Sciences, University of Antwerp, Antwerp, Belgium; Sibyl Anthierens—Department of Primary Care and Interdisciplinary Care, Faculty of Medicine and Health Sciences, University of Antwerp, Antwerp, Belgium; Ine Huybrechts—Department of Primary Care and Interdisciplinary Care, Faculty of Medicine and Health Sciences, University of Antwerp, Antwerp, Belgium; Peter Raeymaeckers—Department of Sociology, Faculty of Social Sciences, University of Antwerp, Belgium; Veerle Bufel—Department of Sociology, Centre for Population, Family and Health, Faculty of Social Sciences, University of Antwerp, Belgium; Dirk Devroey—Department of Family Medicine and Chronic Care, Faculty of Medicine and Pharmacy, Vrije Universiteit Brussel, Brussel; Bert Aertgeerts—Academic Centre for General Practice, Faculty of Medicine, KU Leuven, Leuven, and Department of Public Health and Primary Care, Faculty of Medicine, KU Leuven, Leuven; Birgitte Schoenmakers—Department of Public Health and Primary Care, Faculty of Medicine, KU Leuven, Leuven, Belgium; Lotte Timmermans—Department of Public Health and Primary Care, Faculty of Medicine, KU Leuven, Leuven, Belgium; Veerle Foulon—Department of Pharmaceutical and Pharmacological Sciences, Faculty Pharmaceutical Sciences, KU Leuven, Leuven, Belgium; Anja Declercq—LUCAS-Centre for Care Research and Consultancy, Faculty of Social Sciences, KU Leuven, Leuven, Belgium; Dominique Van de Velde, Department of Rehabilitation Sciences, Occupational Therapy, Faculty of Medicine and Health Sciences, University of Ghent, Belgium, and Department of Occupational Therapy, Artevelde University of Applied Sciences, Ghent, Belgium; Pauline Boeckxstaens—Department of Public Health and Primary Care, Faculty of Medicine and Health Sciences, University of Ghent, Belgium; An De Sutter—Department of Public Health and Primary Care, Faculty of Medicine and Health Sciences, University of Ghent, Belgium; Patricia De Vriendt—Department of Rehabilitation Sciences, Occupational Therapy, Faculty of Medicine and Health Sciences, University of Ghent, Belgium, and Frailty in Ageing (FRIA) Research Group, Department of Gerontology and Mental Health and Wellbeing (MENT) Research Group, Faculty of Medicine and Pharmacy, Vrije Universiteit, Brussels, Belgium, and Department of Occupational Therapy, Artevelde University of Applied Sciences, Ghent, Belgium; Lies Lahousse—Department of Bioanalysis, Faculty of Pharmaceutical Sciences, Ghent University, Ghent, Belgium; Peter Pype—Department of Public Health and Primary Care, Faculty of Medicine and Health Sciences, University of Ghent, Belgium, End-of-Life Care Research Group, Faculty of Medicine and Health Sciences, Vrije Universiteit Brussel and Ghent University, Ghent, Belgium; Dagje Boeykens—Department of Rehabilitation Sciences, Occupational Therapy, Faculty of Medicine and Health Sciences, University of Ghent, Belgium, and Department of Public Health and Primary Care, Faculty of Medicine and Health Sciences, University of Ghent, Belgium; Ann Van Hecke—Department of Public Health and Primary Care, Faculty of Medicine and Health Sciences, University of Ghent, Belgium, University Centre of Nursing and Midwifery, Faculty of Medicine and Health Sciences, University of Ghent, Belgium; Peter Decat—Department of Public Health and Primary Care, Faculty of Medicine and Health Sciences, University of Ghent, Belgium; Rudi Roose—Department of Social Work and Social Pedagogy, Faculty of Psychology and Educational Sciences, University Ghent, Belgium; Sandra Martin—Expertise Centre Health Innovation, University College Leuven-Limburg, Leuven, Belgium; Erica Rutten—Expertise Centre Health Innovation, University College Leuven-Limburg, Leuven, Belgium; Sam Pless—Expertise Centre Health Innovation, University College Leuven-Limburg, Leuven, Belgium; Anouk Tuinstra—Expertise Centre Health Innovation, University College Leuven-Limburg, Leuven, Belgium; Vanessa Gauwe—Department of Occupational Therapy, Artevelde University of Applied Sciences, Ghent, Belgium; Didier ReynaertE-QUAL, University College of Applied Sciences Ghent, Ghent, Belgium; Leen Van Landschoot—Department of Nursing, University of Applied Sciences Ghent, Ghent, Belgium; Maja Lopez Hartmann—Department of Welfare and Health, Karel de Grote University of Applied Sciences and Arts, Antwerp, Belgium; Tony Claeys—LiveLab, VIVES University of Applied Sciences, Kortrijk, Belgium; Hilde Vandenhoudt—LiCalab, Thomas University of Applied Sciences, Turnhout, Belgium; Kristel De Vliegher—Department of Nursing–Homecare, White-Yellow Cross, Brussels, Belgium; and Susanne Op de Beeck—Flemish Patient Platform, Heverlee, Belgium.
This research was funded by fund Daniël De Coninck, King Baudouin Foundation, Belgium. The funder had no involvement in this study. Grant number: 2019-J5170820-211,588.
Author information
Peter Raeymaeckers and Sibyl Anthierens have contributed equally to this work and share senior last authorship.
Authors and Affiliations
Department of Family Medicine and Population Health, University of Antwerp, Doornstraat 331, 2610, Antwerp, Belgium
Ine Huybrechts, Emily Verté & Sibyl Anthierens
Department of Family Medicine and Chronic Care, Vrije Universiteit Brussel, Laarbeeklaan 103, 1090, Jette/Brussels, Belgium
Ine Huybrechts & Emily Verté
LUCAS — Centre for Care Research and Consultancy, KU Leuven, Minderbroedersstraat 8/5310, 3000, Leuven, Belgium
Anja Declercq
Center for Sociological Research, Faculty of Social Sciences, KU Leuven, Parkstraat 45/3601, 3000, Leuven, Belgium
Department of Social Work, University of Antwerp, St-Jacobstraat 2, 2000, Antwerp, Belgium
Peter Raeymaeckers
You can also search for this author in PubMed Google Scholar
- , Emily Verté
- , Muhammed Mustafa Sirimsi
- , Peter Van Bogaert
- , Hans De Loof
- , Kris Van den Broeck
- , Sibyl Anthierens
- , Ine Huybrechts
- , Peter Raeymaeckers
- , Veerle Bufel
- , Dirk Devroey
- , Bert Aertgeerts
- , Birgitte Schoenmakers
- , Lotte Timmermans
- , Veerle Foulon
- , Anja Declerq
- , Dominique Van de Velde
- , Pauline Boeckxstaens
- , An De Sutter
- , Patricia De Vriendt
- , Lies Lahousse
- , Peter Pype
- , Dagje Boeykens
- , Ann Van Hecke
- , Peter Decat
- , Rudi Roose
- , Sandra Martin
- , Erica Rutten
- , Sam Pless
- , Anouk Tuinstra
- , Vanessa Gauwe
- , Leen Van Landschoot
- , Maja Lopez Hartmann
- , Tony Claeys
- , Hilde Vandenhoudt
- , Kristel De Vliegher
- & Susanne Op de Beeck
Contributions
IH wrote the main manuscript text. AD, EV, PR, and SA contributed to the different steps of the making of this manuscript. All authors reviewed the manuscript.
Corresponding author
Correspondence to Ine Huybrechts .
Ethics declarations
Ethics approval and consent to participate.
The study protocol was approved by the Medical Ethics Committee of the University of Antwerp/Antwerp University Hospital (reference: 2021-1690). All participants received verbal and written information about the purpose and methods of the study and gave written informed consent.
Consent for publication
Not applicable.
Competing interests
The authors declare that they have no competing interests.
Additional information
Publisher’s note.
Springer Nature remains neutral with regard to jurisdictional claims in published maps and institutional affiliations.
Rights and permissions
Open Access This article is licensed under a Creative Commons Attribution 4.0 International License, which permits use, sharing, adaptation, distribution and reproduction in any medium or format, as long as you give appropriate credit to the original author(s) and the source, provide a link to the Creative Commons licence, and indicate if changes were made. The images or other third party material in this article are included in the article's Creative Commons licence, unless indicated otherwise in a credit line to the material. If material is not included in the article's Creative Commons licence and your intended use is not permitted by statutory regulation or exceeds the permitted use, you will need to obtain permission directly from the copyright holder. To view a copy of this licence, visit http://creativecommons.org/licenses/by/4.0/ . The Creative Commons Public Domain Dedication waiver ( http://creativecommons.org/publicdomain/zero/1.0/ ) applies to the data made available in this article, unless otherwise stated in a credit line to the data.
Reprints and permissions
About this article
Cite this article.
Huybrechts, I., Declercq, A., Verté, E. et al. How does the external context affect an implementation processes? A qualitative study investigating the impact of macro-level variables on the implementation of goal-oriented primary care. Implementation Sci 19 , 32 (2024). https://doi.org/10.1186/s13012-024-01360-0
Download citation
Received : 03 January 2024
Accepted : 28 March 2024
Published : 16 April 2024
DOI : https://doi.org/10.1186/s13012-024-01360-0
Share this article
Anyone you share the following link with will be able to read this content:
Sorry, a shareable link is not currently available for this article.
Provided by the Springer Nature SharedIt content-sharing initiative
- Contingency theory
- External context
- Institutional theory
- Primary care
- Implementation process
- Macro-context
- Network theory
- Organizational theories
- Resource dependency theory
Implementation Science
ISSN: 1748-5908
- Submission enquiries: Access here and click Contact Us
- General enquiries: [email protected]
- Open access
- Published: 18 April 2024
The predictive power of data: machine learning analysis for Covid-19 mortality based on personal, clinical, preclinical, and laboratory variables in a case–control study
- Maryam Seyedtabib ORCID: orcid.org/0000-0003-1599-9374 1 ,
- Roya Najafi-Vosough ORCID: orcid.org/0000-0003-2871-5748 2 &
- Naser Kamyari ORCID: orcid.org/0000-0001-6245-5447 3
BMC Infectious Diseases volume 24 , Article number: 411 ( 2024 ) Cite this article
95 Accesses
1 Altmetric
Metrics details
Background and purpose
The COVID-19 pandemic has presented unprecedented public health challenges worldwide. Understanding the factors contributing to COVID-19 mortality is critical for effective management and intervention strategies. This study aims to unlock the predictive power of data collected from personal, clinical, preclinical, and laboratory variables through machine learning (ML) analyses.
A retrospective study was conducted in 2022 in a large hospital in Abadan, Iran. Data were collected and categorized into demographic, clinical, comorbid, treatment, initial vital signs, symptoms, and laboratory test groups. The collected data were subjected to ML analysis to identify predictive factors associated with COVID-19 mortality. Five algorithms were used to analyze the data set and derive the latent predictive power of the variables by the shapely additive explanation values.
Results highlight key factors associated with COVID-19 mortality, including age, comorbidities (hypertension, diabetes), specific treatments (antibiotics, remdesivir, favipiravir, vitamin zinc), and clinical indicators (heart rate, respiratory rate, temperature). Notably, specific symptoms (productive cough, dyspnea, delirium) and laboratory values (D-dimer, ESR) also play a critical role in predicting outcomes. This study highlights the importance of feature selection and the impact of data quantity and quality on model performance.
This study highlights the potential of ML analysis to improve the accuracy of COVID-19 mortality prediction and emphasizes the need for a comprehensive approach that considers multiple feature categories. It highlights the critical role of data quality and quantity in improving model performance and contributes to our understanding of the multifaceted factors that influence COVID-19 outcomes.
Peer Review reports
Introduction
The World Health Organization (WHO) has declared COVID-19 a global pandemic in March 2020 [ 1 ]. The first cases of SARSCoV-2, a new severe acute respiratory syndrome coronavirus, were detected in Wuhan, China, and rapidly spread to become a global public health problem [ 2 ]. The clinical presentation and symptoms of COVID-19 may be similar to those of Middle East Respiratory Syndrome (MERS) and Severe Acute Respiratory Syndrome (SARS), however the rate of spread is higher [ 3 ]. By December 31, 2022, the pandemic had caused more than 729 million cases and nearly 6.7 million deaths (0.92%) were confirmed in 219 countries worldwide [ 4 ]. For many countries, figuring out what measures to take to prevent death or serious illness is a major challenge. Due to the complexity of transmission and the lack of proven treatments, COVID-19 is a major challenge worldwide [ 5 , 6 ]. In middle- and low-income countries, the situation is even more catastrophic due to high illiteracy rates, a very poor health care system, and lack of intensive care units [ 5 ]. In addition, understanding the factors contributing to COVID-19 mortality is critical for effective management and intervention strategies [ 6 ].
Numerous studies have shown several factors associated with COVID-19 outcomes, including socioeconomic, environmental, individual demographic, and health factors [ 7 , 8 , 9 ]. Risk factors for COVID -19 mortality vary by study and population studied [ 10 ]. Age [ 11 , 12 ], comorbidities such as hypertension, cardiovascular disease, diabetes, and COPD [ 13 , 14 , 15 ], sex [ 13 ], race/ethnicity [ 11 ], dementia, and neurologic disease [ 16 , 17 ], are some of the factors associated with COVID-19 mortality. Laboratory factors such as elevated levels of inflammatory markers, lymphopenia, elevated creatinine levels, and ALT are also associated with COVID-19 mortality [ 5 , 18 ]. Understanding these multiple risk factors is critical to accurately diagnose and treat COVID-19 patients.
Accurate diagnosis and treatment of the disease requires a comprehensive assessment that considers a variety of factors. These factors include personal factors such as medical history, lifestyle, and genetics; clinical factors such as observations on physical examinations and physician reports; preclinical factors such as early detection through screening or surveillance; laboratory factors such as results of diagnostic tests and medical imaging; and patient-reported signs and symptoms. However, the variety of characteristics associated with COVID-19 makes it difficult for physicians to accurately classify COVID-19 patients during the pandemic.
In today's digital transformation era, machine learning plays a vital role in various industries, including healthcare, where substantial data is generated daily [ 19 , 20 , 21 ]. Numerous studies have explored machine learning (ML) and explainable artificial intelligence (AI) in predicting COVID-19 prognosis and diagnosis [ 22 , 23 , 24 , 25 ]. Chadaga et al. have developed decision support systems and triage prediction systems using clinical markers and biomarkers [ 22 , 23 ]. Similarly, Khanna et al. have developed a ML and explainable AI system for COVID-19 triage prediction [ 24 ]. Zoabi has also made contributions in this field, developing ML models that predict COVID-19 test results with high accuracy based on a small number of features such as gender, age, contact with an infected person and initial clinical symptoms [ 25 ]. These studies emphasize the potential of ML and explainable AI to improve COVID-19 prediction and diagnosis. Nonetheless, the efficacy of ML algorithms heavily relies on the quality and quantity of data utilized for training. Recent research has indicated that deep learning algorithms' performance can be significantly enhanced compared to traditional ML methods by increasing the volume of data used [ 26 ]. However, it is crucial to acknowledge that the impact of data volume on model performance can vary based on data characteristics and experimental setup, highlighting the need for careful consideration and analysis when selecting data for model training. While the studies emphasize the importance of features in training ML algorithms for COVID-19 prediction and diagnosis, additional research is required on methods to enhance the interpretability of features.
Therefore, the primary aim of this study is to identify the key factors associated with mortality in COVID -19 patients admitted to hospitals in Abadan, Iran. For this purpose, seven categories of factors were selected, including demographic, clinical and conditions, comorbidities, treatments, initial vital signs, symptoms, and laboratory tests, and machine learning algorithms were employed. The predictive power of the data was assessed using 139 predictor variables across seven feature sets. Our next goal is to improve the interpretability of the extracted important features. To achieve this goal, we will utilize the innovative SHAP analysis, which illustrates the impact of features through a diagram.
Materials and methods
Study population and data collection.
Using data from the COVID-19 hospital-based registry database, a retrospective study was conducted from April 2020 to December 2022 at Ayatollah Talleghani Hospital (a COVID‑19 referral center) in Abadan City, Iran.
A total of 14,938 patients were initially screened for eligibility for the study. Of these, 9509 patients were excluded because their transcriptase polymerase chain reaction (RT-PCR) test results were negative or unspecified. The exclusion of patients due to incomplete or missing data is a common issue in medical research, particularly in the use of electronic medical records (EMRs) [ 27 ]. In addition, 1623 patients were excluded because their medical records contained more than 70% incomplete or missing data. In addition, patients younger than 18 years were not included in the study. The criterion for excluding 1623 patients due to "70% incomplete or missing data" means that the medical records of these patients did not contain at least 30% of the data required for a meaningful analysis. This threshold was set to ensure that the dataset used for the study contained a sufficient amount of complete and reliable information to draw accurate conclusions. Incomplete or missing data in a medical record may relate to key variables such as patient demographics, symptoms, lab results, treatment information, outcomes, or other data points important to the research. Insufficient data can affect the validity and reliability of study results and lead to potential bias or inaccuracies in the findings. It is important to exclude such incomplete records to maintain the quality and integrity of the research findings and to ensure that the conclusions drawn are based on robust and reliable data. After these exclusions, 3806 patients remained. Of these patients, 474 died due to COVID -19, while the remaining 3332 patients recovered and were included in the control group. To obtain a balanced sample, the control group was selected with a propensity score matching (PSM). The PSM refers to a statistical technique used to create a balanced comparison group by matching individuals in the control group (in this case, the survived group) with individuals in the case group (in this case, the deceased group) based on their propensity scores. In this study, the propensity scores for each person represented the probability of death (coded as a binary outcome; survived = 0, deceased = 1) calculated from a set of covariates (demographic factors) using the matchit function from the MatchIt library. Two individuals, one from the deceased group and one from the survived group, are considered matched if the difference between their propensity scores is small. Non-matching participants are discarded. The matching aims to reduce bias by making the distribution of observed characteristics similar between groups, which ultimately improves the comparability of groups in observational studies [ 28 ]. In total, the study included 1063 COVID-19 patients who belonged to either the deceased group (case = 474) or the survived group (control = 589) (Fig. 1 ).
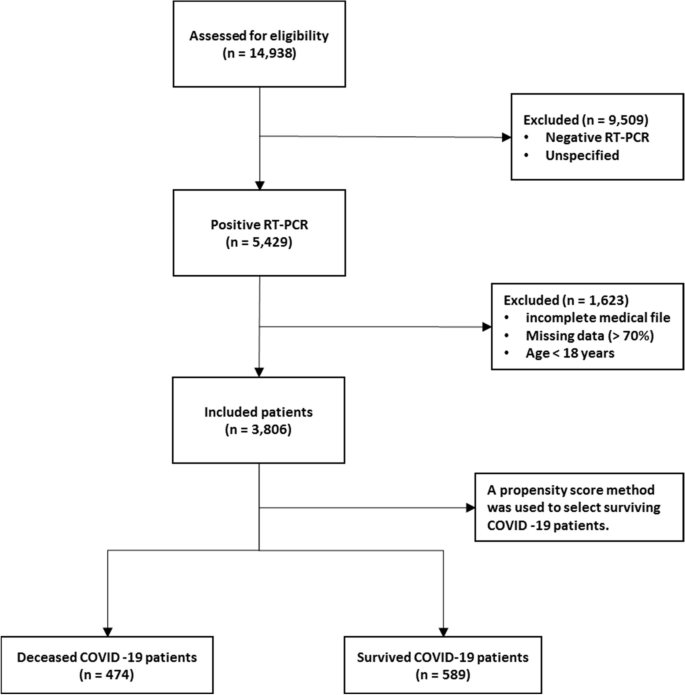
Flowchart describing the process of patient selection
In the COVID‑19 hospital‑based registry database, one hundred forty primary features in eight main classes including patient’s demographics (eight features), clinical and conditions features (16 features), comorbidities (18 features), treatment (17 features), initial vital sign (14 features), symptoms during hospitalization (31 features), laboratory results (35 features), and an output (0 for survived and 1 for deceased) was recorded for COVID-19 patients. The main features included in the hospital-based COVID-19 registry database are provided in Appendix Table 1 .
To ensure the accuracy of the recorded information, discharged patients or their relatives were called and asked to review some of the recorded information (demographic information, symptoms, and medical history). Clinical symptoms and vital signs were referenced to the first day of hospitalization (at admission). Laboratory test results were also referenced to the patient’s first blood sample at the time of hospitalization.
The study analyzed 140 variables in patients' records, normalizing continuous variables and creating a binary feature to categorize patients based on outcomes. To address the issue of an imbalanced dataset, the Synthetic Minority Over-sampling Technique (SMOTE) was utilized. Some classes were combined to simplify variables. For missing data, an imputation technique was applied, assuming a random distribution [ 29 ]. Little's MCAR test was performed with the naniar package to assess whether missing data in a dataset is missing completely at random (MCAR) [ 30 ]. The null hypothesis in this test is that the data are MCAR, and the test statistic is a chi-square value.
The Ethics Committee of Abadan University of Medical Science approved the research protocol (No. IR.ABADANUMS.REC.1401.095).
Predictor variables
All data were collected in eight categories, including demographic, clinical and conditions, comorbidities, treatment, initial vital signs, symptoms, and laboratory tests in medical records, for a total of 140 variables.
The "Demographics" category encompasses eight features, three of which are binary variables and five of which are categorical. The "Clinical Conditions" category includes 16 features, comprising one quantitative variable, 12 binary variables, and five categorical features. " Comorbidities ", " Treatment ", and " Symptoms " each have 18, 17, and 30 binary features, respectively. Also, there is one quantitative variable in symptoms category. The "Initial Vital Signs" category features 11 quantitative variables, two binary variables, and one categorical variable. Finally, the "Laboratory Tests" category comprises 35 features, with 33 being quantitative, one categorical, and one binary (Appendix Table 1 ).
Outcome variable
The primary outcome variable was mortality, with December 31, 2022, as the last date of follow‐up. The feature shows the class variable, which is binary. For any patient in the survivor group, the outcome is 0; otherwise, it is 1. In this study, 44.59% ( n = 474) of the samples were in the deceased group and were labeled 1.
Data balancing
In case–control studies, it is common to have unequal size groups since cases are typically fewer than controls [ 31 ]. However, in case–control studies with equal sizes, data balancing may not be necessary for ML algorithms [ 32 ]. When using ML algorithms, data balancing is generally important when there is an imbalance between classes, i.e., when one class has significantly fewer observations than the other [ 33 ]. In such cases, balancing can improve the performance of the algorithm by reducing the bias in favor of the majority class [ 34 ]. For case–control studies of the same size, the balance of the classes has already been reached and balancing may not be necessary. However, it is always recommended to evaluate the performance of the ML algorithm with the given data set to determine the need for data balancing. This is because unbalanced case–control ratios can cause inflated type I error rates and deflated type I error rates in balanced studies [ 35 ].
Feature selection
Feature selection is about selecting important variables from a large dataset to be used in a ML model to achieve better performance and efficiency. Another goal of feature selection is to reduce computational effort by eliminating irrelevant or redundant features [ 36 , 37 ]. Before generating predictions, it is important to perform feature selection to improve the accuracy of clinical decisions and reduce errors [ 37 ]. To identify the best predictors, researchers often compare the effectiveness of different feature selection methods. In this study, we used five common methods, including Decision Tree (DT), eXtreme Gradient Boosting (XGBoost), Support Vector Machine (SVM), Naïve Bayes (NB), and Random Forest (RF), to select relevant features for predicting mortality of COVID -19 patients. To avoid overfitting, we performed ten-fold cross-validation when training our dataset. This approach may help ensure that our model is optimized for accurate predictions of health status in COVID -19 patients.
Model development, evaluation, and clarity
In this study, the predictive models were developed with five ML algorithms, including DT, XGBoost, SVM, NB, and RF, using the R programming language (v4.3.1) and its packages [ 38 ]. We used cross-validation (CV) to tune the hyperparameters of our models based on the training subset of the dataset. For training and evaluating our ML models, we used a common technique called tenfold cross validation [ 39 ]. The primary training dataset was divided into ten folding, each containing 10% of the total data, using a technique called stratified random sampling. For each of the 30% of the data, a ML model was built and trained on the remaining 70% of the data. The performance of the model was then evaluated on the 30%-fold sample. This process was repeated 100 times with different training and test combinations, and the average performance was reported.
Performance measures include sensitivity (recall), specificity, accuracy, F1-score, and the area under the receiver operating characteristics curve (AUC ROC). Sensitivity is defined as TP / (TP + FN), whereas specificity is TN / (TN + FP). F1-score is defined as the harmonic mean of Precision and Recall with equal weight, where Precision equals TP + TN / total. Also, AUC refers to the area under the ROC curve. In the evaluation of ML techniques, values were classified as poor if below 50%, ok if between 50 and 80%, good if between 80 and 90%, and very good if greater than 90%. These criteria are commonly used in reporting model evaluations [ 40 , 41 ].
Finally, the shapely additive explanation (SHAP) method was used to provide clarity and understanding of the models. SHAP uses cooperative game theory to determine how each feature contributes to the prediction of ML models. This approach allows the computation of the contribution of each feature to model performance [ 42 , 43 ]. For this purpose, the package shapr was used, which includes a modified iteration of the kernel SHAP approach that takes into account the interdependence of the features when computing the Shapley values [ 44 ].
Patient characteristics
Table 1 shows the baseline characteristics of patients infected with COVID-19, including demographic data such as age and sex and other factors such as occupation, place of residence, marital status, education level, BMI, and season of admission. A total of 1063 adult patients (≥ 18 years) were enrolled in the study, of whom 589 (55.41%) survived and 474 (44.59%) died. Analysis showed that age was significantly different between the two groups, with a mean age of 54.70 ± 15.60 in the survivor group versus 65.53 ± 15.18 in the deceased group ( P < 0.001). There was also a significant association between age and survival, with a higher proportion of patients aged < 40 years in the survivor group (77.0%) than in the deceased group (23.0%) ( P < 0.001). No significant differences were found between the two groups in terms of sex, occupation, place of residence, marital status, and time of admission. However, there was a significant association between educational level and survival, with a lower proportion of patients with a college degree in the deceased group (37.2%) than in the survivor group (62.8%) ( P = 0.017). BMI also differed significantly between the two groups, with the proportion of patients with a BMI > 30 (kg/cm 2 ) being higher in the deceased group (56.5%) than in the survivor group (43.5%) ( P < 0.001).
Clinical and conditions
Important insights into the various clinical and condition characteristics associated with COVID-19 infection outcomes provides in Table 2 . The results show that patients who survived the infection had a significantly shorter hospitalization time (2.20 ± 1.63 days) compared to those who died (4.05 ± 3.10 days) ( P < 0.001). Patients who were admitted as elective cases had a higher survival rate (84.6%) compared to those who were admitted as urgent (61.3%) or emergency (47.4%) cases. There were no significant differences with regard to the number of infections or family infection history. However, patients who had a history of travel had a lower decease rate (40.1%).
A significantly higher proportion of deceased patients had cases requiring CPR (54.7% vs. 45.3%). Patients who had underlying medical conditions had a significantly lower survival rate (38.3%), with hyperlipidemia being the most prevalent condition (18.7%). Patients who had a history of alcohol consumption (12.5%), transplantation (30.0%), chemotropic (21.4%) or special drug use (0.0%), and immunosuppressive drug use (30.0%) also had a lower survival rate. Pregnant patients (44.4%) had similar survival outcomes compared to non-pregnant patients (55.6%). Patients who were recent or current smokers (36.4%) also had a significantly lower survival rate.
Comorbidities
Table 3 summarizes the comorbidity characteristics of COVID-19 infected patients. Out of 1063 patients, 54.84% had comorbidities. Chi-Square tests for individual comorbidities showed that most of them had a significant association with COVID-19 outcomes, with P -values less than 0.05. Among the various comorbidities, hypertension (HTN) and diabetes mellitus (DM) were the most prevalent, with 12% and 11.5% of patients having these conditions, respectively. The highest fatality rates were observed among patients with cardiovascular disease (95.5%), chronic kidney disease (62.5%), gastrointestinal (GI) (93.3%), and liver diseases (73.3%). Conversely, patients with neurology comorbidities had the lowest fatality rate (0%). These results highlight the significant role of comorbidities in COVID-19 outcomes and emphasize the need for special attention to be paid to patients with pre-existing health conditions.
The treatment characteristics of the COVID-19 patients and the resulting outcomes are shown in Table 4 . The table shows the frequency of patients who received different types of medications or therapies during their treatment. According to the results, the use of antibiotics (35.1%), remdesivir (29.6%), favipiravir (36.0%), and Vitamin zinc (33.5%) was significantly associated with a lower mortality rate ( P < 0.001), suggesting that these medications may have a positive impact on patient outcomes. On the other hand, the use of Heparin (66.1%), Insulin (82.6%), Antifungal (89.6%), ACE inhibitors (78.1%), and Angiotensin II Receptor Blockers (ARB) (83.8%) was significantly associated with increased mortality ( P < 0.001), suggesting that these medications may have a negative effect on the patient's outcome. Also, It seems that taking hydroxychloroquine (51.0%) is associated with a worse outcome at lower significance ( P = 0.022). The use of Atrovent, Corticosteroids and Non-Steroidal Anti-Inflammatory Drugs (NSAIDs) did not show a significant association with survival or mortality rates. Similarly, the use of Intravenous Immunoglobulin (IVIg), Vitamin C, Vitamin D, and Diuretic did not show a significant association with the patient’s outcome.
Initial vital signs
Table 5 provides initial vital sign characteristics of COVID-19 patients, including heart rate, respiratory rate, temperature, blood pressure, oxygen therapy, and radiography test result. The findings shows that deceased patients had higher HR (83.03 bpm vs. 76.14 bpm, P < 0.001), lower RR (11.40 bpm vs. 16.25 bpm, P < 0.001), higher temperature (37.43 °C vs. 36.91 °C, P < 0.001), higher SBP (128.16 mmHg vs. 123.33 mmHg, P < 0.001), and higher O 2 requirements (invasive: 75.0% vs. 25.0%, P < 0.001) compared to the survived patients. Additionally, deceased patients had higher MAP (99.35 mmHg vs. 96.08 mmHg, P = 0.005), and lower SPO 2 percentage (81.29% vs. 91.95%, P < 0.001) compared to the survived patients. Furthermore, deceased patients had higher PEEP levels (5.83 cmH2O vs. 0.69 cmH2O, P < 0.001), higher FiO2 levels (51.43% vs. 8.97%, P < 0.001), and more frequent bilateral pneumonia (63.0% vs. 37.0%, P < 0.001) compared to the survived patients. There appears to be no relationship between diastolic blood pressure and treatment outcome (83.44 mmHg vs. 85.61 mmHg).
Table 6 provides information on the symptoms of patients infected with COVID-19 by survival outcome. The table also shows the frequency of symptoms among patients. The most common symptom reported by patients was fever, which occurred in 67.0% of surviving and deceased patients. Dyspnea and nonproductive cough were the second and third most common symptoms, reported by 40.4% and 29.3% of the total sample, respectively. Other common symptoms listed in the Table were malodor (28.7%), dyspepsia (28.4%), and myalgia (25.6%).
The P -values reported in the table show that some symptoms are significantly associated with death, including productive cough, dyspnea, sore throat, headache, delirium, olfactory symptoms, dyspepsia, nausea, vomiting, sepsis, respiratory failure, heart failure, MODS, coagulopathy, secondary infection, stroke, acidosis, and admission to the intensive care unit. Surviving and deceased patients also differed significantly in the average number of days spent in the ICU. There was no significant association between patient outcomes and symptoms such as nonproductive cough, chills, diarrhea, chest pain, and hyperglycemia.
Laboratory tests
Table 7 shows the laboratory values of COVID-19 patients with the average values of the different laboratory results. The results show that the deceased patients had significantly lower levels of red blood cells (3.78 × 106/µL vs. 5.01 × 106/µL), hemoglobin (11.22 g/dL vs. 14.10 g/dL), and hematocrit (34.10% vs. 42.46%), whereas basophils and white blood cells did not differ significantly between the two groups. The percentage of neutrophils (65.59% vs. 62.58%) and monocytes (4.34% vs. 3.93%) was significantly higher in deceased patients, while the percentage of lymphocytes and eosinophils did not differ significantly between the two groups. In addition, deceased patients had higher levels of certain biomarkers, including D-dimer (1.347 mgFEU/L vs. 0.155 mgFEU/L), lactate dehydrogenase (174.61 U/L vs. 128.48 U/L), aspartate aminotransferase (93.09 U/L vs. 39.63 U/L), alanine aminotransferase (74.48 U/L vs. 28.70 U/L), alkaline phosphatase (119.51 IU/L vs. 81.34 IU/L), creatine phosphokinase-MB (4.65 IU/L vs. 3.33 IU/L), and positive troponin I (56.5% vs. 43.5%). The proportion of patients with positive C-reactive protein was also higher in the deceased group.
Other laboratory values with statistically significant differences between the two groups ( P < 0.001) were INR, ESR, BUN, Cr, Na, K, P, PLT, TSH, T3, and T4. The surviving patients generally had lower values in these laboratory characteristics than the deceased patients.
Model performance and evaluation
Five ML algorithms, namely DT, XGBoost, SVM, NB, and RF, were used in this study to build mortality prediction models COVID -19. The models were based on the optimal feature set selected in a previous step and were trained on the same data set. The effectiveness of the models was evaluated by calculating sensitivity, specificity, accuracy, F1 score, and AUC metrics. Table 8 shows the results of this performance evaluation. The average values are expressed from the test set as the mean (standard deviation).
The results show that the performance of the models varies widely in the different feature categories. The Laboratory Tests category achieved the highest performance, with all models scoring 100% in all metrics. The Symptoms and initial Vital Signs categories also show high performance, with XGBoost achieving the highest accuracy of 98.03% and DT achieving the highest sensitivity of 92.79%.
The Clinical and Conditions category also showed high performance, with all models showing accuracy above 91%. XGBoost achieved the highest sensitivity and specificity of 92.74% and 92.96%, respectively. In contrast, the Demographics category showed the lowest performance, with all models achieving less than 66.5% accuracy.
In summary, the results suggest that certain feature categories may be more useful than others in predicting mortality from COVID-19 and that some ML models may perform better than others depending on the feature category used.
Feature importance
SHapley Additive exPlanations (SHAP) values indicate the importance or contribution of each feature in predicting model output. These values help to understand the influence and importance of each feature on the model's decision-making process.
In Fig. 2 , the mean absolute SHAP values are shown to depict global feature importance. Figure 2 shows the contribution of each feature within its respective group as calculated by the XGBoost prediction model using SHAP. According to the SHAP method, the features that had the greatest impact on predicting COVID-19 mortality were, in descending order: D-dimer, CPR, PEEP, underlying disease, ESR, antifungal treatment, PaO2, age, dyspnea, and nausea.
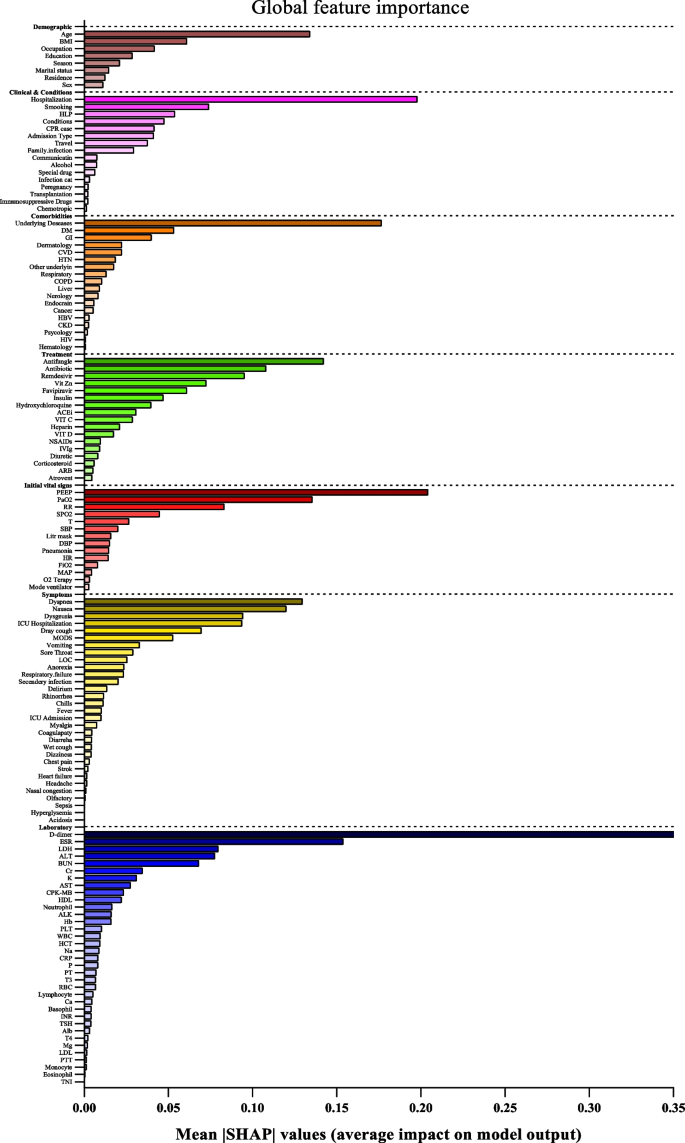
Feature importance based on SHAP-values. The mean absolute SHAP values are depicted, to illustrate global feature importance. The SHAP values change in the spectrum from dark (higher) to light (lower) color
On the other hand, Fig. 3 presents the local explanation summary that indicates the direction of the relationship between a variable and COVID-19 outcome. As shown in Fig. 3 (I to VII), older age and very low BMI were the two demographic factors with the greatest impact on model outcome, followed by clinical factors such as higher CPR, hospitalization, and hyperlipidemia. Higher mortality rates were associated with patients who smoked and had traveled in the past 14 days. Patients with underlying diseases, especially HTN, died more frequently. In contrast, the use of remdesivir, Vit Zn, and favipiravir is associated with lower mortality. Initial vital signs such as high PEEP, low PaO2 and RR had the greatest impact, as did symptoms such as dyspnea, MODS, sore throat and LOC. A higher risk of mortality is observed in patients with higher D-dimer levels and ESR as the most consequential laboratory tests, followed by K, AST and CPK-MB.
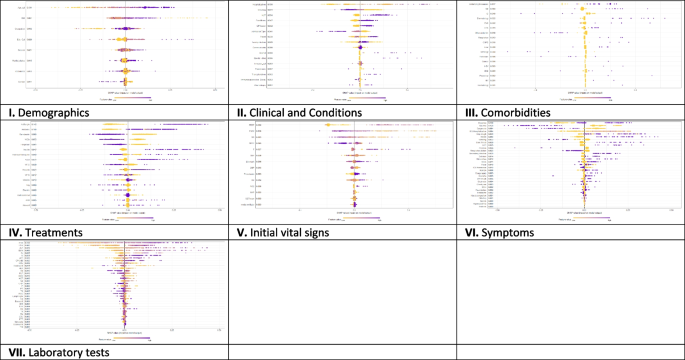
The SHAP-based feature importance of all categories (I to VII) for COVID‑19 mortality prediction, calculated with the XGBoost model. The local explanatory summary shows the direction of the relationship between a feature and patient outcome. Positive SHAP values indicate death, whereas negative SHAP values indicate survival. As the color scale shows, higher values are blue while lower values are orenge
Using the feature types listed in Appendix Table 1 , Fig. 4 shows that the performance of ML algorithms can be improved by increasing the number of features used in training, especially in distinguishing between symptoms, comorbidities, and treatments. In addition, the amount and quality of data used for training can significantly affect algorithm performance, with laboratory tests being more informative than initial vital signs. Regarding the influence of features, quantitative features tend to have a more positive effect on performance than qualitative features; clinical conditions tend to be more informative than demographic data. Thus, both the amount of data and the type of features used have a significant impact on the performance of ML algorithms.
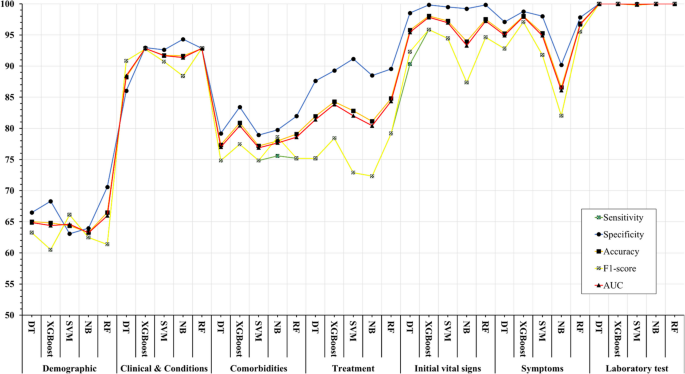
Association between feature sets and performance of machine learning algorithms in predicting COVID-19’s mortality
The COVID-19 pandemic has presented unprecedented public health challenges worldwide and requires a deep understanding of the factors contributing to COVID-19 mortality to enable effective management and intervention. This study used machine learning analysis to uncover the predictive power of an extensive dataset that includes wide range of personal, clinical, preclinical, and laboratory variables associated with COVID-19 mortality.
This study confirms previous research on COVID-19 outcomes that highlighted age as a significant predictor of mortality [ 45 , 46 , 47 ], along with comorbidities such as hypertension and diabetes [ 48 , 49 ]. Underlying conditions such as cardiovascular and renal disease also contribute to mortality risk [ 50 , 51 ].
Regarding treatment, antibiotics, remdesivir, favipiravir, and vitamin zinc are associated with lower mortality [ 52 , 53 ], whereas heparin, insulin, antifungals, ACE, and ARBs are associated with higher mortality [ 54 ]. This underscores the importance of drug choice in COVID -19 treatment.
Initial vital signs such as heart rate, respiratory rate, temperature, and oxygen therapy differ between surviving and deceased patients [ 55 ]. Deceased patients often have increased heart rate, lower respiratory rate, higher temperature, and increased oxygen requirements, which can serve as early indicators of disease severity.
Symptoms such as productive cough, dyspnea, and delirium are significantly associated with COVID-19 mortality, emphasizing the need for immediate monitoring and intervention [ 56 ]. Laboratory tests show altered hematologic and biochemical markers in deceased patients, underscoring the importance of routine laboratory monitoring in COVID-19 patients [ 57 , 58 ].
The ML algorithms were used in the study to predict mortality COVID-19 based on these multilayered variables. XGBoost and Random Forest performed better than other algorithms and had high recall, specificity, accuracy, F1 score, and AUC. This highlights the potential of ML, particularly the XGBoost algorithm, in improving prediction accuracy for COVID-19 mortality [ 59 ]. The study also highlighted the importance of drug choice in treatment and the potential of ML algorithms, particularly XGBoost, in improving prediction accuracy. However, the study's findings differ from those of Moulaei [ 60 ], Nopour [ 61 ], and Mehraeen [ 62 ] in terms of the best-performing ML algorithm and the most influential variables. While Moulaei [ 60 ] found that the random forest algorithm had the best performance, Nopour [ 61 ] and Ikemura [ 63 ] identified the artificial neural network and stacked ensemble models, respectively, as the most effective. Additionally, the most influential variables in predicting mortality varied across the studies, with Moulaei [ 60 ] highlighting dyspnea, ICU admission, and oxygen therapy, and Ikemura [ 63 ] identifying systolic and diastolic blood pressure, age, and other biomarkers. These differences may be attributed to variations in the datasets, feature selection, and model training.
However, it is important to note that the choice of algorithm should be tailored to the specific dataset and research question. In addition, the results suggest that a comprehensive approach that incorporates different feature categories may lead to more accurate prediction of COVID-19 mortality. In general, the results suggest that the performance of ML models is influenced by the number and type of features in each category. While some models consistently perform well across different categories (e.g., XGBoost), others perform better for specific types of features (e.g., SVM for Demographics).
Analysis of the importance of characteristics using SHAP values revealed critical factors affecting model results. D-dimer values, CPR, PEEP, underlying diseases, and ESR emerged as the most important features, highlighting the importance of these variables in predicting COVID-19 mortality. These results provide valuable insights into the underlying mechanisms and risk factors associated with severe COVID-19 outcomes.
The types of features used in ML models fall into two broad categories: quantitative (numerical) and qualitative (binary or categorical). The performance of ML methods can vary depending on the type of features used. Some algorithms work better with quantitative features, while others work better with qualitative features. For example, decision trees and random forests work well with both types of features [ 64 ], while neural networks often work better with quantitative features [ 65 , 66 ]. Accordingly, we consider these levels for the features under study to better assess the impact of the data.
The success of ML algorithms depends largely on the quality and quantity of the data on which they are trained [ 67 , 68 , 69 ]. Recent research, including the 2021 study by Sarker IH. [ 26 ], has shown that a larger amount of data can significantly improve the performance of deep learning algorithms compared to traditional machine learning techniques. However, it should be noted that the effect of data size on model performance depends on several factors, such as data characteristics and experimental design. This underscores the importance of carefully and judiciously selecting data for training.
Limitations
One of the limitations of this study is that it relies on data collected from a single hospital in Abadan, Iran. The data may not be representative of the diversity of COVID -19 cases in different regions, and there may be differences in data quality and completeness. In addition, retrospectively collected data may have biases and inaccuracies. Although the study included a substantial number of COVID -19 patients, the sample size may still limit the generalizability of the results, especially for less common subgroups or certain demographic characteristics.
Future works
Future studies could adopt a multi-center approach to improve the scope and depth of research on COVID-19 outcomes. This could include working with multiple hospitals in different regions of Iran to ensure a more diverse and representative sample. By conducting prospective studies, researchers can collect data in real time, which reduces the biases associated with retrospective data collection and increases the reliability of the results. Increasing sample size, conducting longitudinal studies to track patient progression, and implementing quality assurance measures are critical to improving generalizability, understanding long-term effects, and ensuring data accuracy in future research efforts. Collectively, these strategies aim to address the limitations of individual studies and make an important contribution to a more comprehensive understanding of COVID-19 outcomes in different populations and settings.
Conclusions
In summary, this study demonstrates the potential of ML algorithms in predicting COVID-19 mortality based on a comprehensive set of features. In addition, the interpretability of the models using SHAP-based feature importance, which revealed the variables strongly correlated with mortality. This study highlights the power of data-driven approaches in addressing critical public health challenges such as the COVID-19 pandemic. The results suggest that the performance of ML models is influenced by the number and type of features in each feature set. These findings may be a valuable resource for health professionals to identify high-risk patients COVID-19 and allocate resources effectively.
Availability of data and materials
The datasets used and/or analyzed during the current study are available from the corresponding author on reasonable request.
Abbreviations
World Health Organization
Middle east respiratory syndrome
Severe acute respiratory syndrome
Reverse transcription polymerase chain reaction
Propensity score matching
Synthetic minority over-sampling technique
Missing completely at random
Decision tree
EXtreme gradient boosting
Support vector machine
Naïve bayes
Random forest
Cross-validation
True positive
True negative
False positive
False negative
- Machine learning
Artificial Intelligence
Shapely additive explanation
Cardiopulmonary Resuscitation
Hypertension
Diabetes mellitus
Cardiovascular disease
Chronic Kidney disease
Chronic obstructive pulmonary disease
Human immunodeficiency virus
Hepatitis B virus
Such as influenza, pneumonia, asthma, bronchitis, and chronic obstructive airways disease
Gastrointestinal
Such as epilepsy, learning disabilities, neuromuscular disorders, autism, ADD, brain tumors, and cerebral palsy
Such as fatty liver disease and cirrhosis
Blood disease
Skin diseases
Mental disorders
Intravenous immunoglobulin
Non-steroidal anti-Inflammatory drugs
Angiotensin converting enzyme inhibitors
Angiotensin II receptor blockers
Beats per minute
Respiratory rate
Temperatures
Systolic blood pressure
Diastolic blood pressure
Mean arterial pressure
Oxygen saturation
Partial pressure of oxygen in the alveoli
Positive end-expiratory pressure
Fraction of inspired oxygen
Radiography (X-ray) test result
Smell disorders
Indigestion
Level of consciousness
Multiple organ dysfunction syndrome
Coughing up blood; Coagulopathy: bleeding disorder
High blood glucose
Intensive care unit
Red blood cell
White blood cell
Low-density lipoprotein
High-density lipoprotein
Prothrombin time
Partial thromboplastin time
International normalized ratio
Erythrocyte sedimentation rate
C-reactive-protein
Lactate dehydrogenase
Aspartate aminotransferase
Alanine aminotransferase
Alkaline phosphatase
Creatine phosphokinase-MB
Blood urea nitrogen
Thyroid stimulating hormone
Triiodothyronine
Coronavirus disease (COVID-19) pandemic. Available from: https://www.who.int/europe/emergencies/situations/covid-19 . [cited 2023 Sep 5].
Moolla I, Hiilamo H. Health system characteristics and COVID-19 performance in high-income countries. BMC Health Serv Res. 2023;23(1):1–14. https://doi.org/10.1186/s12913-023-09206-z . [cited 2023 Sep 5].
Article Google Scholar
Peeri NC, Shrestha N, Rahman MS, Zaki R, Tan Z, Bibi S, et al. The SARS, MERS and novel coronavirus (COVID-19) epidemics, the newest and biggest global health threats: what lessons have we learned? Int J Epidemiol. 2020;49(3):717–26.
Article PubMed Google Scholar
WHO Coronavirus (COVID-19) Dashboard | WHO Coronavirus (COVID-19) Dashboard With Vaccination Data. Available from: https://covid19.who.int/ . [cited 2023 Sep 5].
Dessie ZG, Zewotir T. Mortality-related risk factors of COVID-19: a systematic review and meta-analysis of 42 studies and 423,117 patients. BMC Infect Dis. 2021;21(1):1–28. https://doi.org/10.1186/s12879-021-06536-3 . [cited 2023 Sep 5].
Article CAS Google Scholar
Wong ELY, Ho KF, Wong SYS, Cheung AWL, Yau PSY, Dong D, et al. Views on Workplace Policies and its Impact on Health-Related Quality of Life During Coronavirus Disease (COVID-19) Pandemic: Cross-Sectional Survey of Employees. Int J Heal Policy Manag. 2022;11(3):344–53. Available from: https://www.ijhpm.com/article_3879.html .
Google Scholar
Drefahl S, Wallace M, Mussino E, Aradhya S, Kolk M, Brandén M, et al. A population-based cohort study of socio-demographic risk factors for COVID-19 deaths in Sweden. Nat Commun. 2020;11(1):5097.
Article CAS PubMed PubMed Central Google Scholar
Islam N, Khunti K, Dambha-Miller H, Kawachi I, Marmot M. COVID-19 mortality: a complex interplay of sex, gender and ethnicity. Eur J Public Health. 2020;30(5):847–8.
Sarmadi M, Marufi N, Moghaddam VK. Association of COVID-19 global distribution and environmental and demographic factors: An updated three-month study. Environ Res. 2020;188:109748.
Aghazadeh-Attari J, Mohebbi I, Mansorian B, Ahmadzadeh J, Mirza-Aghazadeh-Attari M, Mobaraki K, et al. Epidemiological factors and worldwide pattern of Middle East respiratory syndrome coronavirus from 2013 to 2016. Int J Gen Med. 2018;11:121–5.
Risk of COVID-19-Related Mortality. Available from: https://www.cdc.gov/coronavirus/2019-ncov/science/data-review/risk.html . [cited 2023 Aug 26].
Bhaskaran K, Bacon S, Evans SJW, Bates CJ, Rentsch CT, MacKenna B, et al. Factors associated with deaths due to COVID-19 versus other causes: population-based cohort analysis of UK primary care data and linked national death registrations within the OpenSAFELY platform. Lancet Reg Heal. 2021;6:100-9.
Dessie ZG, Zewotir T. Mortality-related risk factors of COVID-19: a systematic review and meta-analysis of 42 studies and 423,117 patients. BMC Infect Dis. 2021;21(1):855. https://doi.org/10.1186/s12879-021-06536-3 .
Talebi SS, Hosseinzadeh A, Zare F, Daliri S, JamaliAtergeleh H, Khosravi A, et al. Risk Factors Associated with Mortality in COVID-19 Patient’s: Survival Analysis. Iran J Public Health. 2022;51(3):652–8.
PubMed PubMed Central Google Scholar
Singh J, Alam A, Samal J, Maeurer M, Ehtesham NZ, Chakaya J, et al. Role of multiple factors likely contributing to severity-mortality of COVID-19. Infect Genet Evol J Mol Epidemiol Evol Genet Infect Dis. 2021;96:105101.
CAS Google Scholar
Bhaskaran K, Bacon S, Evans SJ, Bates CJ, Rentsch CT, MacKenna B, et al. Factors associated with deaths due to COVID-19 versus other causes: population-based cohort analysis of UK primary care data and linked national death registrations within the OpenSAFELY platform. Lancet Reg Heal - Eur. 2021;6:100109. Available from: https://www.pmc/articles/PMC8106239/ . [cited 2023 Aug 26].
Ge E, Li Y, Wu S, Candido E, Wei X. Association of pre-existing comorbidities with mortality and disease severity among 167,500 individuals with COVID-19 in Canada: A population-based cohort study. PLoS One. 2021;16(10):e0258154. https://journals.plos.org/plosone/article?id=10.1371/journal.pone.0258154 . [cited 2023 Aug 26].
Tian S, Liu H, Liao M, Wu Y, Yang C, Cai Y, et al. Analysis of mortality in patients with COVID-19: clinical and laboratory parameters. Open Forum Infect Dis. 2020;7(5). Available from: https://dx.doi.org/10.1093/ofid/ofaa152 . [cited 2023 Aug 26].
Rashidi HH, Tran N, Albahra S, Dang LT. Machine learning in health care and laboratory medicine: General overview of supervised learning and Auto-ML. Int J Lab Hematol. 2021;43:15–22.
Najafi-Vosough R, Faradmal J, Hosseini SK, Moghimbeigi A, Mahjub H. Predicting hospital readmission in heart failure patients in Iran: a comparison of various machine learning methods. Healthc Inform Res. 2021;27(4):307–14.
Article PubMed PubMed Central Google Scholar
Alanazi A. Using machine learning for healthcare challenges and opportunities. Informatics Med Unlocked. 2022;100924:1–5.
Chadaga K, Prabhu S, Sampathila N, Chadaga R, Umakanth S, Bhat D, et al. Explainable artificial intelligence approaches for COVID-19 prognosis prediction using clinical markers. Sci Rep. 2024;14(1):1783.
Chadaga K, Prabhu S, Bhat V, Sampathila N, Umakanth S, Chadaga R, et al. An explainable multi-class decision support framework to predict COVID-19 prognosis utilizing biomarkers. Cogent Eng. 2023;10(2):2272361.
Khanna VV, Chadaga K, Sampathila N, Prabhu S, Chadaga R. A machine learning and explainable artificial intelligence triage-prediction system for COVID-19. Decis Anal J. 2023;100246:1–14.
Zoabi Y, Deri-Rozov S, Shomron N. Machine learning-based prediction of COVID-19 diagnosis based on symptoms. npj Digit Med. 2021;4(1):1–5.
IH Sarker 2021 Machine Learning: Algorithms, Real-World Applications and Research Directions SN Comput Sci. 2 3 160 Available from: https://doi.org/10.1007/s42979-021-00592-x .
Jones JA, Farnell B. Missing and Incomplete Data Reduces the Value of General Practice Electronic Medical Records as Data Sources in Research. Aust J Prim Health. 2007;13(1):74–80. Available from: https://www.publish.csiro.au/py/py07010 . [cited 2023 Dec 16].
Austin PC. An Introduction to Propensity Score Methods for Reducing the Effects of Confounding in Observational Studies. Multivariate Behav Res. 2011;46(3):399–424.
Torjusen H, Lieblein G, Næs T, Haugen M, Meltzer HM, Brantsæter AL. Food patterns and dietary quality associated with organic food consumption during pregnancy; Data from a large cohort of pregnant women in Norway. BMC Public Health. 2012;12(1):1–11.
Little RJA. A test of missing completely at random for multivariate data with missing values. J Am Stat Assoc. 1988;83(404):1198–202.
Tenny S, Kerndt CC, Hoffman MR. Case Control Studies. Encycl Pharm Pract Clin Pharm Vol 1-3 [Internet]. 2023;1–3:V2-356-V2-366. [cited 2024 Apr 14] Available from: https://www.ncbi.nlm.nih.gov/books/NBK448143/ .
Stanfill B, Reehl S, Bramer L, Nakayasu ES, Rich SS, Metz TO, et al. Extending Classification Algorithms to Case-Control Studies. Biomed Eng Comput Biol. 2019;10:117959721985895. Available from: https://www.pmc/articles/PMC6630079/ .[cited 2023 Sep 3].
Mulugeta G, Zewotir T, Tegegne AS, Juhar LH, Muleta MB. Classification of imbalanced data using machine learning algorithms to predict the risk of renal graft failures in Ethiopia. BMC Med Inform Decis Mak. 2023;23(1):1–17. https://bmcmedinformdecismak.biomedcentral.com/articles/10.1186/s12911-023-02185-5 . [cited 2023 Sep 3].
Sadeghi S, Khalili D, Ramezankhani A, Mansournia MA, Parsaeian M. Diabetes mellitus risk prediction in the presence of class imbalance using flexible machine learning methods. BMC Med Inform Decis Mak. 2022;22(1):36. https://doi.org/10.1186/s12911-022-01775-z .
Zhou W, Nielsen JB, Fritsche LG, Dey R, Gabrielsen ME, Wolford BN, et al. Efficiently controlling for case-control imbalance and sample relatedness in large-scale genetic association studies. Nat Genet. 2018;50(9):1335. Available from: https://www.pmc/articles/PMC6119127/ . [cited 2023 Sep 3].
Miao J, Niu L. A Survey on Feature Selection. Procedia Comput Sci. 2016;91(1):919–26.
Remeseiro B, Bolon-Canedo V. A review of feature selection methods in medical applications. Comput Biol Med. 2019;112:103375.
Article CAS PubMed Google Scholar
R Studio Team. A language and environment for statistical computing. R Found Stat Comput. 2021;1.
Training Sets, Test Sets, and 10-fold Cross-validation - KDnuggets. Available from: https://www.kdnuggets.com/2018/01/training-test-sets-cross-validation.html . [cited 2023 Sep 4].
Hossin M, Sulaiman MN. A review on evaluation metrics for data classification evaluations. Int J data Min Knowl Manag Process. 2015;5(2):1.
Seyedtabib M, Kamyari N. Predicting polypharmacy in half a million adults in the Iranian population: comparison of machine learning algorithms. BMC Med Inform Decis Mak. 2023;23(1):84. https://doi.org/10.1186/s12911-023-02177-5 .
Lundberg SM, Lee S-I. A unified approach to interpreting model predictions. Adv Neural Inf Process Syst. 2017;30:4765–74.
Greenwell B. Fastshap: Fast approximate shapley values. Man R Packag v0 05. 2020;9–12. https://www.CRANR-projectorg/package=fastshap . Last accessed.
Aas K, Jullum M, Løland A. Explaining individual predictions when features are dependent: More accurate approximations to Shapley values. Artif Intell. 2021;298:103502.
Mesas AE, Cavero-Redondo I, Álvarez-Bueno C, Sarriá Cabrera MA, de Maffei Andrade S, Sequí-Dominguez I, et al. Predictors of in-hospital COVID-19 mortality: A comprehensive systematic review and meta-analysis exploring differences by age, sex and health conditions. PLoS One. 2020;15(11):e0241742.
Yanez ND, Weiss NS, Romand J-A, Treggiari MM. COVID-19 mortality risk for older men and women. BMC Public Health. 2020;20(1):1–7.
Sasson I. Age and COVID-19 mortality. Demogr Res. 2021;44:379–96.
Huang I, Lim MA, Pranata R. Diabetes mellitus is associated with increased mortality and severity of disease in COVID-19 pneumonia–a systematic review, meta-analysis, and meta-regression. Diabetes Metab Syndr Clin Res Rev. 2020;14(4):395–403.
Albitar O, Ballouze R, Ooi JP, Ghadzi SMS. Risk factors for mortality among COVID-19 patients. Diabetes Res Clin Pract. 2020;166:108293.
Di Castelnuovo A, Bonaccio M, Costanzo S, Gialluisi A, Antinori A, Berselli N, et al. Common cardiovascular risk factors and in-hospital mortality in 3,894 patients with COVID-19: survival analysis and machine learning-based findings from the multicentre Italian CORIST Study. Nutr Metab Cardiovasc Dis. 2020;30(11):1899–913.
Ssentongo P, Ssentongo AE, Heilbrunn ES, Ba DM, Chinchilli VM. Association of cardiovascular disease and 10 other pre-existing comorbidities with COVID-19 mortality: A systematic review and meta-analysis. PLoS ONE. 2020;15(8):e0238215.
Beran A, Mhanna M, Srour O, Ayesh H, Stewart JM, Hjouj M, et al. Clinical significance of micronutrient supplements in patients with coronavirus disease 2019: A comprehensive systematic review and meta-analysis. Clin Nutr ESPEN. 2022;48:167–77.
Perveen RA, Nasir M, Murshed M, Nazneen R, Ahmad SN. Remdesivir and favipiravir changes hepato-renal profile in COVID-19 patients: a cross sectional observation in Bangladesh. Int J Med Sci Clin Inven. 2021;8(1):5196–201.
El-Arif G, Khazaal S, Farhat A, Harb J, Annweiler C, Wu Y, et al. Angiotensin II Type I Receptor (AT1R): the gate towards COVID-19-associated diseases. Molecules. 2022;27(7):2048.
Ikram AS, Pillay S. Admission vital signs as predictors of COVID-19 mortality: a retrospective cross-sectional study. BMC Emerg Med. 2022;22(1):1–10.
Martí-Pastor A, Moreno-Perez O, Lobato-Martínez E, Valero-Sempere F, Amo-Lozano A, Martínez-García M-Á, et al. Association between Clinical Frailty Scale (CFS) and clinical presentation and outcomes in older inpatients with COVID-19. BMC Geriatr. 2023;23(1):1.
Lippi G, Plebani M. Laboratory abnormalities in patients with COVID-2019 infection. Clin Chem Lab Med. 2020;58(7):1131–4.
Naghashpour M, Ghiassian H, Mobarak S, Adelipour M, Piri M, Seyedtabib M, et al. Profiling serum levels of glutathione reductase and interleukin-10 in positive and negative-PCR COVID-19 outpatients: A comparative study from southwestern Iran. J Med Virol. 2022;94(4):1457–64.
Sharifi-Kia A, Nahvijou A, Sheikhtaheri A. Machine learning-based mortality prediction models for smoker COVID-19 patients. BMC Med Inform Decis Mak. 2023;23(1):1–15.
Moulaei K, Shanbehzadeh M, Mohammadi-Taghiabad Z, Kazemi-Arpanahi H. Comparing machine learning algorithms for predicting COVID-19 mortality. BMC Med Inform Decis Mak. 2022;22(1):2. https://doi.org/10.1186/s12911-021-01742-0 .
Nopour R, Erfannia L, Mehrabi N, Mashoufi M, Mahdavi A, Shanbehzadeh M. Comparison of Two Statistical Models for Predicting Mortality in COVID-19 Patients in Iran. Shiraz E-Medical J 2022 236 [Internet]. 2022;23(6):119172. [cited 2024 Apr 14] Available from: https://brieflands.com/articles/semj-119172 .
Mehraeen E, Karimi A, Barzegary A, Vahedi F, Afsahi AM, Dadras O, et al. Predictors of mortality in patients with COVID-19–a systematic review. Eur J Integr Med. 2020;40:101226.
Ikemura K, Bellin E, Yagi Y, Billett H, Saada M, Simone K, et al. Using Automated Machine Learning to Predict the Mortality of Patients With COVID-19: Prediction Model Development Study. J Med Internet Res [Internet]. 2021;23(2):e23458. Available from: https://www.jmir.org/2021/2/e23458 .
Breiman L. Random forests. Mach Learn. 2001;45:5–32.
Hinton G, Srivastava N, Swersky K. Neural networks for machine learning lecture 6a overview of mini-batch gradient descent. Cited on. 2012;14(8):2.
Zheng A, Casari A. Feature Engineering for Machine Learning: Principles and Techniques for Data Scientists. O’Reilly [Internet]. 2018;218. [cited 2024 Apr 14] Available from: https://www.amazon.com/Feature-Engineering-Machine-Learning-Principles/dp/1491953241 .
Adamson AS, Smith A. Machine Learning and Health Care Disparities in Dermatology. JAMA Dermatology. 2018;154(11):1247–8. Available from: https://jamanetwork.com/journals/jamadermatology/fullarticle/2688587 . [cited 2023 Sep 15].
Kavakiotis I, Tsave O, Salifoglou A, Maglaveras N, Vlahavas I, Chouvarda I. Machine Learning and Data Mining Methods in Diabetes Research. Comput Struct Biotechnol J. 2017;1(15):104–16.
Schmidt J, Marques MRG, Botti S, Marques MAL. Recent advances and applications of machine learning in solid-state materials science. Comput Mater. 2019;5(1):83. https://doi.org/10.1038/s41524-019-0221-0 .
Download references
Acknowledgements
We thank the Research Deputy of the Abadan University of Medical Sciences for financially supporting this project.
Summary points
∙ How can datasets improve mortality prediction using ML models for COVID-19 patients?
∙ In order, quantity and quality variables have more effect on the model performances.
∙ Intelligent techniques such as SHAP analysis can be used to improve the interpretability of features in ML algorithms.
∙ Well-structured data are critical to help health professionals identify at-risk patients and improve pandemic outcomes.
This research was supported by grant No. 1456 from the Abadan University of Medical Sciences. However, the funding source did not influence the study design, data collection, analysis and interpretation, report writing, or decision to publish the article.
Author information
Authors and affiliations.
Department of Biostatistics and Epidemiology, School of Health, Ahvaz Jundishapur University of Medical Sciences, Ahvaz, Iran
Maryam Seyedtabib
Research Center for Health Sciences, Hamadan University of Medical Sciences, Hamadan, Iran
Roya Najafi-Vosough
Department of Biostatistics and Epidemiology, School of Health, Abadan University of Medical Sciences, Abadan, Iran
Naser Kamyari
You can also search for this author in PubMed Google Scholar
Contributions
MS: Conceptualization, Methodology, Validation, Formal analysis, Investigation, Resources, Data curation, Writing–original draft, writing—review & editing, Visualization, Project administration. RNV: Conceptualization, Data curation, Formal analysis, Investigation, Writing–original draft, writing—review & editing. NK: Conceptualization, Methodology, Software, Validation, Formal analysis, Investigation, Resources, Data curation, Writing–original draft, writing—review & editing, Visualization, Supervision.
Corresponding author
Correspondence to Naser Kamyari .
Ethics declarations
Ethics approval and consent to participate.
This study was approved by the Research Ethics Committee (REC) of Abadan University of Medical Sciences under the ID number IR.ABADANUMS.REC.1401.095. Methods used complied with all relevant ethical guidelines and regulations. The Ethics Committee of Abadan University of Medical Sciences waived the requirement for written informed consent from study participants.
Competing interests
The authors declare no competing interests.
Additional information
Publisher’s note.
Springer Nature remains neutral with regard to jurisdictional claims in published maps and institutional affiliations.
Supplementary Information
Supplementary material 1., rights and permissions.
Open Access This article is licensed under a Creative Commons Attribution 4.0 International License, which permits use, sharing, adaptation, distribution and reproduction in any medium or format, as long as you give appropriate credit to the original author(s) and the source, provide a link to the Creative Commons licence, and indicate if changes were made. The images or other third party material in this article are included in the article's Creative Commons licence, unless indicated otherwise in a credit line to the material. If material is not included in the article's Creative Commons licence and your intended use is not permitted by statutory regulation or exceeds the permitted use, you will need to obtain permission directly from the copyright holder. To view a copy of this licence, visit http://creativecommons.org/licenses/by/4.0/ . The Creative Commons Public Domain Dedication waiver ( http://creativecommons.org/publicdomain/zero/1.0/ ) applies to the data made available in this article, unless otherwise stated in a credit line to the data.
Reprints and permissions
About this article
Cite this article.
Seyedtabib, M., Najafi-Vosough, R. & Kamyari, N. The predictive power of data: machine learning analysis for Covid-19 mortality based on personal, clinical, preclinical, and laboratory variables in a case–control study. BMC Infect Dis 24 , 411 (2024). https://doi.org/10.1186/s12879-024-09298-w
Download citation
Received : 22 December 2023
Accepted : 05 April 2024
Published : 18 April 2024
DOI : https://doi.org/10.1186/s12879-024-09298-w
Share this article
Anyone you share the following link with will be able to read this content:
Sorry, a shareable link is not currently available for this article.
Provided by the Springer Nature SharedIt content-sharing initiative
- Predictive model
- Coronavirus disease
- Data quality
- Performance
BMC Infectious Diseases
ISSN: 1471-2334
- Submission enquiries: [email protected]
- General enquiries: [email protected]

- SUGGESTED TOPICS
- The Magazine
- Newsletters
- Managing Yourself
- Managing Teams
- Work-life Balance
- The Big Idea
- Data & Visuals
- Reading Lists
- Case Selections
- HBR Learning
- Topic Feeds
- Account Settings
- Email Preferences
Case Study: How Aggressively Should a Bank Pursue AI?
- Thomas H. Davenport
- George Westerman

A Malaysia-based CEO weighs the risks and potential benefits of turning a traditional bank into an AI-first institution.
Siti Rahman, the CEO of Malaysia-based NVF Bank, faces a pivotal decision. Her head of AI innovation, a recent recruit from Google, has a bold plan. It requires a substantial investment but aims to transform the traditional bank into an AI-first institution, substantially reducing head count and the number of branches. The bank’s CFO worries they are chasing the next hype cycle and cautions against valuing efficiency above all else. Siti must weigh the bank’s mixed history with AI, the resistance to losing the human touch in banking services, and the risks of falling behind in technology against the need for a prudent, incremental approach to innovation.
Two experts offer advice: Noemie Ellezam-Danielo, the chief digital and AI strategy at Société Générale, and Sastry Durvasula, the chief information and client services officer at TIAA.
Siti Rahman, the CEO of Malaysia-headquartered NVF Bank, hurried through the corridors of the university’s computer engineering department. She had directed her driver to the wrong building—thinking of her usual talent-recruitment appearances in the finance department—and now she was running late. As she approached the room, she could hear her head of AI innovation, Michael Lim, who had joined NVF from Google 18 months earlier, breaking the ice with the students. “You know, NVF used to stand for Never Very Fast,” he said to a few giggles. “But the bank is crawling into the 21st century.”

- Thomas H. Davenport is the President’s Distinguished Professor of Information Technology and Management at Babson College, a visiting scholar at the MIT Initiative on the Digital Economy, and a senior adviser to Deloitte’s AI practice. He is a coauthor of All-in on AI: How Smart Companies Win Big with Artificial Intelligence (Harvard Business Review Press, 2023).
- George Westerman is a senior lecturer at MIT Sloan School of Management and a coauthor of Leading Digital (HBR Press, 2014).
Partner Center
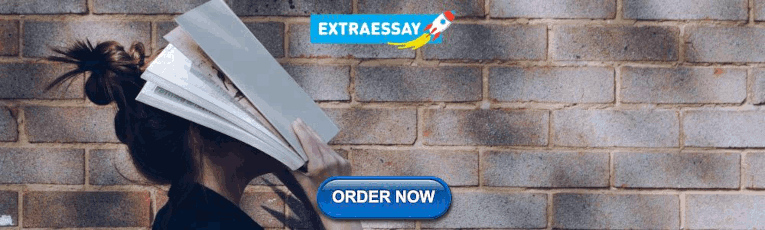
IMAGES
VIDEO
COMMENTS
This updated 6th Edition of Medical Nutrition Therapy: A Case-study Approach is composed of 29 realistic case studies appropriate for introductory and advanced level courses in nutrition and medical nutrition therapy. Each case study uses the medical record as its structure and is designed to resemble an electronic medical record.—Publisher.
Objectives within each case are built around the nutrition care process and competencies for dietetic education. Read more Report an issue with this product or seller. Previous page. ISBN-10. 1305628667. ISBN-13. 978-1305628663. Edition. 5th. Publisher. ... A CASE STUDY APPROACH. Additionally, she has contributed to the ACADEMY OF NUTRITION AND ...
Her career in dietetics began as a clinical dietitian and her expertise focuses on the implementation of ADA's Nutrition Care Process; having chaired the work group that first developed the Nutrition Care Process and Model in 2003. She is a coauthor of Medical Nutrition Therapy: A Case Study Approach, 3rd. Ms.
Dietetic and Nutrition Case Studies. ... specific method, diagnosis, or treatment by health science practitioners for any particular patient. ... Case studies' answers 1 Veganism, 173 2 Older person-ethical dilemma, 177 3 Older person, 180 4 Learning disabilities: Prader-Willi syndrome, 183 5 Freelance practice, 186 6 Public health ...
Marcia Nelms. Cengage Learning, Jan 1, 2016 - Health & Fitness - 456 pages. Building a bridge from classroom to clinical practice, this casebook is composed of 32 realistic case studies appropriate for introductory and advanced level courses in nutrition and diet therapy. Each case study uses the medical record as its structure and you solve ...
The dietitian acted as an expert generalist and FCP, although the dietitian may not necessarily be the first point of contact for all patients. The definition of a FCP has developed since this case study occurred; therefore, it is important to understand how dietitians work in this setting and whether they can take on the role of FCP successfully.
In the interest of trying to summarise how realist research could be useful in nutrition and dietetics, this study employed a targeted literature review and narrative synthesis. 18 Key journals in nutrition and dietetics were identified by the ... Multiple case study approach. Qualitative data collected using semi‐structured interviews ...
The ideal companion resource to Manual of Dietetic Practice, this book takes a problem-based learning approach to dietetics and nutrition with cases written and peer reviewed by registered dietitians, drawing on their own experiences and specialist knowledge Each case study follows the Process for Nutrition and Dietetic Practice published by the British Dietetic Association in 2012 Includes ...
The ideal companion resource to 'Manual of Dietetic Practice', this book takes a problem-based learning approach to dietetics and nutrition with cases written and peer reviewed by registered dietitians, drawing on their own experiences and specialist knowledge Each case study follows the Process for Nutrition and Dietetic Practice published by the British Dietetic Association in 2012 ...
Judy Lawrence is a Research Officer, BDA & Visiting Researcher at King's College London, England. Joan Gandy is a Freelance Dietitian & Visiting Researcher, Nutrition & Dietetics, University of Hertfordshire, Englan.. Pauline Douglas is Senior Lecturer & Clinical Dietetic Facilitator, Northern Ireland Centre for Food and Health (NICHE), Ulster University, Northern Ireland.
In 2003, the Academy of Nutrition and Dietetics, formerly the American Dietetic Association, adopted a Nutrition Care Process and Model (NCPM) that identifies the unique contribution of dietetics practitioners to health care outcomes and establishes a global standard for provision of nutrition care by dietetics practitioners (Figure 1).1
1. Edition April 2016. 386 Pages, Softcover. Wiley & Sons Ltd. ISBN: 978-1-118-89710-2. John Wiley & Sons. Sample Chapter. Short Description. Taking a problem-based learning approach to the subject of dietetics and nutrition, Dietetic and Nutrition Case Studies has been written to complement the internationally successful Manual of Dietetic ...
Clinical nutrition training is an essential course for clinical nutrition and dietetics (CN&D) students. The training combines theoretical knowledge with practical skills. The goal is to prepare competent graduates for future practice. Case-based learning is an active learning method based on cases from the clinical setting.
Methods: Three case studies were purposively selected across areas of dietetics practice to demonstrate application of commonly-used implementation theories, models and frameworks. Reflections from the authors were provided in response to a structured set of questions outlining how the theoretical approach was selected and used, and ...
Model and process for nutrition and dietetic practice 7 involve a new assessment and a new nutrition and dietetic diagnosis, which will in turn lead to new goals and additional monitoring. Some of the case studies in this book involve more than one nutrition and dietetic diagnosis. Evaluation Evaluation takes place at the end of the process.
RESEARCH Perspectives in Practice Facilitating Transdisciplinary Teamwork in Dietetics Education: A Case Study Approach A. RUSSELL SMITH, JR, MMSc; CATHERINE CHRISTIE, PhD, RD, FADA ABSTRACT Teamwork, emphasized by the transdisciplinary approach, has been strongly linked to the development of a culture of patient safety. However, the vast ...
Nutrition Care Professionals provide dietetic students and educators with a comprehensive resource for teaching the Nutrition Care Process and Terminology (NCPT). ... Using a case-study approach, we offer students, practitioners and clinicians the opportunity to analyze the circumstances of the case as described by the patient and then draw on ...
Facilitating transdisciplinary teamwork in dietetics education: a case study approach J Am Diet Assoc. 2004 Jun;104(6):959-62. doi: 10.1016/j.jada.2004.03.023. ... a case-study assignment was developed to facilitate professional interaction between graduate physical therapy and graduate nutrition students. The objectives were threefold: (a). to ...
Case Studies. 10 10 1x 0:00 / 0:00. Case studies demonstrate theoretical concepts in an applied setting. Nutrition Care Process and Terminology: A Practical Approach uses clinical case studies as a means of examining the Nutrition Care Process. Each NCPro case study is based on a realistic clinical encounter between client/patient and dietitian.
Method: This is a non-randomized, interventional study on first year undergraduate medical students from 2017-18 batch (43) and 2018-19 batch (41) of Sumandeep Vidyapeeth University.
Case study question examples for an interview. I am interviewing for a dietitian job I want very badly - I previously interviewed for another position at this company and I was surprised by the amount of case study questions I was given. I was very prepared in situational questions (ie: work style, dealing w/ conflict/stress, etc) but stumbled ...
Jonathan was invited to attend the hospital's cardiac rehabilitation service, which includes education on risk factor reduction. He was therefore booked into an initial appointment with a dietitian as part of this service. He will also receive on-going dietetic follow-up for 8 weeks during this service.
A qualitative study using in-depth semi-structured interviews was conducted with actors from a variety of primary care organizations. Data was collected and analyzed with an iterative approach. We assessed the potential of four organizational theories to enrich our understanding of the impact of external context variables on implementation ...
The ideal companion resource to Manual of Dietetic Practice, this book takes a problem-based learning approach to dietetics and nutrition with cases written and peer reviewed by registered dietitians, drawing on their own experiences and specialist knowledge Each case study follows the Process for Nutrition and Dietetic Practice published by the British Dietetic Association in 2012 Includes ...
Background and purpose The COVID-19 pandemic has presented unprecedented public health challenges worldwide. Understanding the factors contributing to COVID-19 mortality is critical for effective management and intervention strategies. This study aims to unlock the predictive power of data collected from personal, clinical, preclinical, and laboratory variables through machine learning (ML ...
Introduction: This study examines the impact of building information modeling on the cost management of engineering projects, focusing specifically on the Mombasa Port Area Development Project. The objective of this research is to determine the mechanisms through which building information modeling facilitates stakeholder collaboration, reduces construction-related expenses, and enhances the ...
The research also offers guidance for designing and conducting case studies. On the other hand, Stake's book emphasizes the importance of case study research as a qualitative approach for understanding complex phenomena in real-life contexts. The primary focus of the presented case study is to ascertain whether the use of a gamified tool can ...
Recently, numerous spatial prediction methods with diverse characteristics have been developed. Selecting an appropriate spatial prediction method, along with its data preprocessing and parameter settings, presents a challenging task for many users, especially for non-experts. This paper addresses this challenge by exploring the potential of automated machine learning method proposed in ...
Anuj Shrestha. Summary. Siti Rahman, the CEO of Malaysia-based NVF Bank, faces a pivotal decision. Her head of AI innovation, a recent recruit from Google, has a bold plan. It requires a ...