Research LLM
Osgoode’s Research LLM is a full-time, research-intensive program that is ideal for students who want to pursue a specific area of legal study in depth, including those who are considering a PhD. Students conduct their research under the supervision of an Osgoode faculty member .
The Research LLM does not qualify students to practise law in Canada. Students interested in practising law should review the licencing rules of the Law Society of the province in which they intend to practice.
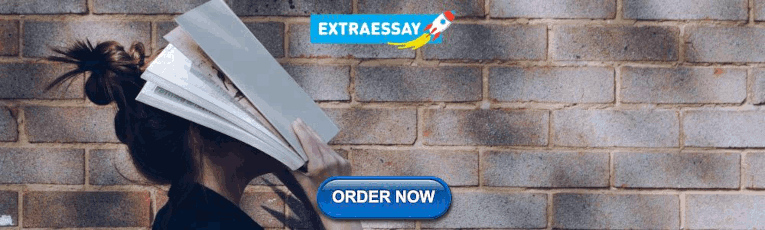
Program Requirements
Graduate seminar i: legal research (gs law 6610).
- One study group
- Elective courses
- A major written research work (thesis or major research paper)
The Graduate Seminar is the core course for the Graduate Program in Law. Designed to complement other courses, the seminar provides a venue for developing critical assessments of the law and facilitating students’ progress on their own research, papers and dissertation proposals. The seminar also provides students with an intellectual community and introduces them to Osgoode research resources.
One Study Group
Students participating in study groups read and discuss a significant number of articles with their groups each week. The groups are not structured as courses but as venues for reflection and discourse. LLM students must participate in one study group. They can choose among five options, depending on their research interests:
- Regulation and Governance
- Law and Economic Relations
- Theoretical Perspectives in Legal Research
- Law and Social Justice
- Law in a Global Context
Elective Courses
Research LLM students can fulfil their elective courses through:
- a variety of graduate courses in law
- integrated courses with the JD program
- independent study
- courses in other programs
Major Written Research Work
A major paper is at the core of the Research LLM program. Most students complete a thesis, but students may also choose to submit a major research paper and complete additional coursework.
All theses and major research papers should contain an analysis of scholarship on the student’s chosen topic and the results of the student’s research – based on primary sources – in the form of a sustained argument. They should have standard scholarly apparatus, footnotes and a bibliography, prepared in accordance with the McGill Guide to Legal Citations.
Thesis Option
Major Research Paper (MRP) Option
100-125 pages
60-70 pages
Additional elective courses required to complete the LLM
Evaluation and defence
Students must succeed in an oral defence of their thesis before an examination committee.
MRPs are evaluated by the student’s supervisor and by one other member of the Graduate Program chosen by the supervisor in consultation with the Graduate Program Director. In exceptional circumstances, the second examiner may be a member of another Graduate Program at York University or another university.
Additional notes
Some students choose to fulfill the program’s thesis requirement with a Portfolio Thesis: one or two published articles (depending on length and scope) developed during their time in the Osgoode graduate degree, submitted in lieu of a traditional thesis.
The MRP is an original piece of scholarly work equivalent to an article of publishable quality for a reputable law journal. It’s typically more substantial than a research paper for a regular course, but less substantial than a thesis.
Additional Courses
Students entering the Research LLM without an LLB or JD may be required to take additional courses on the advice of their supervisor. Completing this extra coursework during their program can be helpful to students whose research relates to fields of law in which they do not have extensive background. The Graduate Program Director determines whether students must pursue additional courses in order to fulfill the requirements of the LLM.
Time to Completion
Both the Thesis and MRP options should be completed in three or four terms. Generally, students take courses in the fall and winter terms, conduct their research in the winter term and write the Thesis or MRP in the summer term. Graduate students must register in each term (fall, winter, summer) from the start of their program to completion.
Residency Requirement
Students must be located such that they are able to progress on all program requirements requiring geographical availability on campus.
More Detail:
Faculty research advisors, related topics:, funding and fees, intellectual life, meet our current doctoral students.
LL.M. Program
5005 Wasserstein Hall (WCC) 1585 Massachusetts Avenue Cambridge , MA 02138
The LL.M. (Master of Laws) program is a one-year degree program that typically includes 180 students from some 65 countries. The Graduate Program is interested in attracting intellectually curious and thoughtful candidates from a variety of legal systems and backgrounds and with various career plans. Harvard’s LL.M. students include lawyers working in firms, government officials, law professors, judges, diplomats, human rights activists, doctoral students, business people, and others. The diversity of the participants in the LL.M. program contributes significantly to the educational experience of all students at the School.
LL.M. Degree Overview
Ll.m. degree requirements, academic resources, ll.m. class profile, modal gallery, gallery block modal gallery.
Support NYU Law
- Graduate Affairs
- Research & Writing
LLM Research and Writing Options
Working as a research assistant for a law school professor.
Faculty members may offer students the opportunity to work as research assistants (RAs) for monetary compensation or, if the professor deems it appropriate based on the nature of the work, for academic credit. For details, review information on serving as a research assistant for faculty .
Directed Research
To undertake Directed Research, students contact individual instructors and agree on a research project. To register, a written proposal must be approved and signed by the instructor, and then submitted to the Office of Graduate Affairs. The written proposal should be at least 1000 words and describe the subject matter of the Directed Research and the issues the student intends to explore in the paper. While any full-time faculty member or visiting faculty member may supervise the research, Adjunct Professors may supervise only with the permission of Vice Dean Hertz.
Directed Research credit may be added through Monday, October 2 for Fall 2023, and Monday, February 5 for Spring 2024.
The usual allocation for Directed Research is two credits. A student may write a one-credit Directed Research. A two-credit Directed Research project should conform to the r equirements for an Option A paper ; a one-credit Directed Research paper should be at least 5,000 words, exclusive of footnotes. A three-credit Directed Research project is highly unusual and requires the approval of Vice Dean Randy Hertz. Students considering a 3-credit Directed Research should contact the Office of Graduate Affairs to discuss.
For non-tax students no more than four of a student's 24 credits may consist of directed research. Tax students may take a maximum of two credits of directed research. Regardless of the type of project involved, students are, of course, expected to submit original, non-duplicative work. When in doubt about proper use of a citation or quotation, discuss the issue with the instructor. Plagiarism is a serious offense that may merit severe discipline. Requests to add Directed Research after the deadline stated above require approval of Vice Dean Hertz. Such requests should be initiated by contacting the Office of Graduate Affairs and will only be considered if your credit load (not including the Directed Research credits) does not drop below minimum requirements after the add/drop period. Students who are granted permission to late-add Directed Research will not be permitted to drop courses if the result is inconsistent with the above; please plan your schedule accordingly. After March 15, the Vice Dean may allow a student to add Directed Research only in exceptional circumstances. No more than two credits can be earned in this manner.
Read further about Requirements for Directed Research
Directed Research During the Summer Semester
Students may register for Directed Research during the summer semester. The summer registration deadlines is July 1, unless there is approval by the Vice Dean to add at a later date. Please note that full-time students will be charged per credit for Directed Research during the summer. All work must be submitted by September 1 or by an earlier deadline established by the supervising faculty member.
Writing Credit
In seminars, colloquia, and courses that offer the option to add an additional writing credit, students may earn one credit for writing a substantial paper (at least 10,000 words in length exclusive of footnotes). To earn the additional credit, students must register for the writing credit section of the course within the same semester the course is offered. The deadline for registering is Monday, October 2 for Fall 2023, and Monday, February 5 for Spring 2024.
LLM Thesis Option
LLM students have the option to write a substantial research paper, in conjunction with a seminar or Directed Research that may be recorded as a "thesis" on their transcript. At the onset of the seminar or Directed Research, the student must obtain approval from the professor that the paper will be completed for a "thesis" designation.
It should be substantial in length (at least 10,000 words exclusive of footnotes) and, like the substantial writing requirement for JD students, must be analytical rather than descriptive in nature, showing original thought and analysis. Please note the thesis designation is for a single research paper agreed upon in advance.
The student is required to submit an outline and at least one FULL PRE-FINAL draft to the faculty member in order to receive the thesis notation. When submitting a final draft of the thesis to the faculty member, the student must give the faculty member an LLM Thesis Certification form . The faculty member is required to return the signed form to the Office of Records and Registration when submitting a grade for the course.
Please note that the student will not receive additional credit for writing the thesis, but will only receive credit for the seminar or Directed Research for which he or she is registered.
International Legal Studies Students should review their program requirements for further information about writing an LLM thesis within their program.
Writing Assistance
Writing resources.
- Guide to Writing
- (excellent guide to legal writing generally)
- So You Want to Write a Research Paper...
- (Recording with Prof. Jose Alvarez)
- So You Want to Write About International Law...
- Some Thoughts on Writing by Barry Friedman (PDF: 106 KB)
- NYU Law Library Guide: Researching and Writing a Law Review Note or Seminar Paper
- NYU Law Library Research Guides
- Why Write a Student Note
© 2024 New York University School of Law. 40 Washington Sq. South, New York, NY 10012. Tel. (212) 998-6100

- Schools & departments

Law LLM by Research
Awards: LLM by Research
Study modes: Full-time, Part-time
Funding opportunities
Programme website: Law
Upcoming Introduction to Postgraduate Study and Research events
Join us online on the 19th June or 26th June to learn more about studying and researching at Edinburgh.
Choose your event and register
Research profile
The Edinburgh Law School is a vibrant, collegial and enriching community of legal, sociolegal and criminology researchers and offers an excellent setting for doctoral research.
We are ranked 3rd in the UK for law for the quality and breadth of our research by Research Professional, based on the 2021 Research Excellence Framework (REF2021).
Our doctoral researchers are key to the School’s research activities and we work hard to ensure that they are fully engaged with staff and projects across all of our legal disciplines.
You will find opportunities in the following fields:
- company and commercial law
- comparative law
- constitutional and administrative law
- criminal law
- criminology and criminal justice
- environmental law
- European law, policy and institutions
- European private law
- evidence and procedure
- gender and sexuality
- human rights law
- information technology law
- intellectual property law
- international law
- legal theory
- medical law and ethics
- obligations
- contract delict
- unjustified enrichment
- property, trusts and successions
- Roman law and legal history
- socio-legal studies
Programme structure
The framework of the LLM by Research allows you time and intellectual space to work in your chosen field, and to refine and develop this initial phase of the project for future doctoral work.
The programme does not have formal coursework elements, other than initial training seminars alongside PhD students.
This makes the LLM by Research a particularly attractive option for those wishing to undertake postgraduate research on a part-time basis, while pursuing legal practice or other employment.
Training and support
Postgraduate researchers enjoy full access to the University’s research skills training which the Law School complements with a tailored research and wider skills programme.
The training programme in Semester One (six seminars) includes workshops on research design, writing and research ethics.
- Find out more about training and support on the LLM by Research
Postgraduate researchers are able to draw upon a fantastic range of resources and facilities to support their research.
The Law School has one of the most significant academic law libraries in the UK which offers outstanding digital resources alongside a world-leading print collection (almost 60,000 items including a unique collection for Scots law research).
You will also have access to the University’s Main Library which has one of the largest and most important collections in Britain, as well as the legal collection of the National Library of Scotland.
Entry requirements
These entry requirements are for the 2024/25 academic year and requirements for future academic years may differ. Entry requirements for the 2025/26 academic year will be published on 1 Oct 2024.
A UK 2:1 honours degree, or its international equivalent, in law, or a social science subject.
Entry to this programme is competitive. Meeting minimum requirements for consideration does not guarantee an offer of study.
International qualifications
Check whether your international qualifications meet our general entry requirements:
- Entry requirements by country
- English language requirements
Regardless of your nationality or country of residence, you must demonstrate a level of English language competency at a level that will enable you to succeed in your studies.
English language tests
We accept the following English language qualifications at the grades specified:
- IELTS Academic: total 7.0 with at least 7.0 in writing and 6.5 in all other components. We do not accept IELTS One Skill Retake to meet our English language requirements.
- TOEFL-iBT (including Home Edition): total 100 with at least 25 in writing and 23 in all other components.
- C1 Advanced ( CAE ) / C2 Proficiency ( CPE ): total 185 with at least 185 in writing and 176 in all other components.
- Trinity ISE : ISE III with passes in all four components.
- PTE Academic: total 70 with at least 70 in writing and 62 in all other components.
Your English language qualification must be no more than three and a half years old from the start date of the programme you are applying to study, unless you are using IELTS , TOEFL, Trinity ISE or PTE , in which case it must be no more than two years old.
Degrees taught and assessed in English
We also accept an undergraduate or postgraduate degree that has been taught and assessed in English in a majority English speaking country, as defined by UK Visas and Immigration:
- UKVI list of majority English speaking countries
We also accept a degree that has been taught and assessed in English from a university on our list of approved universities in non-majority English speaking countries (non-MESC).
- Approved universities in non-MESC
If you are not a national of a majority English speaking country, then your degree must be no more than five years old* at the beginning of your programme of study. (*Revised 05 March 2024 to extend degree validity to five years.)
Find out more about our language requirements:
Fees and costs
Scholarships and funding, featured funding.
* School of Law funding opportunities
Other funding opportunities
Search for scholarships and funding opportunities:
- Search for funding
Further information
- Postgraduate Research Office
- Phone: +44 (0)131 650 2022
- Contact: [email protected]
- School of Law (Postgraduate Research Office)
- Old College
- South Bridge
- Central Campus
- Programme: Law
- School: Law
- College: Arts, Humanities & Social Sciences
Select your programme and preferred start date to begin your application.
LLM by Research Law - 1 Year (Full-time)
Llm by research law - 2 years (part-time), application deadlines.
We encourage you to apply at least one month prior to entry so that we have enough time to process your application. If you are also applying for funding or will require a visa then we strongly recommend you apply as early as possible.
- How to apply
You must submit two references with your application.
Find out more about the general application process for postgraduate programmes:
What’s next in large language model (LLM) research? Here’s what’s coming down the ML pike
- Share on Facebook
- Share on LinkedIn
Join us in returning to NYC on June 5th to collaborate with executive leaders in exploring comprehensive methods for auditing AI models regarding bias, performance, and ethical compliance across diverse organizations. Find out how you can attend here .
There is a lot of excitement around the potential applications of large language models ( LLM ). We’re already seeing LLMs used in several applications, including composing emails and generating software code.
But as interest in LLMs grows, so do concerns about their limits; this can make it difficult to use them in different applications. Some of these include hallucinating false facts, failing at tasks that require commonsense and consuming large amounts of energy.
Here are some of the research areas that can help address these problems and make LLMs available to more domains in the future.
Knowledge retrieval
One of the key problems with LLMs such as ChatGPT and GPT-3 is their tendency to “hallucinate.” These models are trained to generate text that is plausible, not grounded in real facts. This is why they can make up stuff that never happened. Since the release of ChatGPT, many users have pointed out how the model can be prodded into generating text that sounds convincing but is factually incorrect.
The AI Impact Tour: The AI Audit
Join us as we return to NYC on June 5th to engage with top executive leaders, delving into strategies for auditing AI models to ensure fairness, optimal performance, and ethical compliance across diverse organizations. Secure your attendance for this exclusive invite-only event.
One method that can help address this problem is a class of techniques known as “knowledge retrieval.” The basic idea behind knowledge retrieval is to provide the LLM with extra context from an external knowledge source such as Wikipedia or a domain-specific knowledge base.
Google introduced “retrieval-augmented language model pre-training” ( REALM ) in 2020. When a user provides a prompt to the model, a “neural retriever” module uses the prompt to retrieve relevant documents from a knowledge corpus. The documents and the original prompt are then passed to the LLM, which generates the final output within the context of the knowledge documents.
Work on knowledge retrieval continues to make progress. Recently, AI21 Labs presented “in-context retrieval augmented language modeling,” a technique that makes it easy to implement knowledge retrieval in different black-box and open-source LLMs.
You can also see knowledge retrieval at work in You.com and the version of ChatGPT used in Bing. After receiving the prompt, the LLM first creates a search query, then retrieves documents and generates its output using those sources. It also provides links to the sources, which is very useful for verifying the information that the model produces. Knowledge retrieval is not a perfect solution and still makes mistakes. But it seems to be one step in the right direction.
Better prompt engineering techniques
Despite their impressive results, LLMs do not understand language and the world — at least not in the way that humans do. Therefore, there will always be instances where they will behave unexpectedly and make mistakes that seem dumb to humans.
One way to address this challenge is “prompt engineering,” a set of techniques for crafting prompts that guide LLMs to produce more reliable output. Some prompt engineering methods involve creating “few-shot learning” examples, where you prepend your prompt with a few similar examples and the desired output. The model uses these examples as guides when producing its output. By creating datasets of few-shot examples, companies can improve the performance of LLMs without the need to retrain or fine-tune them.
Another interesting line of work is “chain-of-thought (COT) prompting,” a series of prompt engineering techniques that enable the model to produce not just an answer but also the steps it uses to reach it. CoT prompting is especially useful for applications that require logical reasoning or step-by-step computation.
There are different CoT methods, including a few-shot technique that prepends the prompt with a few examples of step-by-step solutions. Another method, zero-shot CoT , uses a trigger phrase to force the LLM to produce the steps it reaches the result. And a more recent technique called “ faithful chain-of-thought reasoning ” uses multiple steps and tools to ensure that the LLM’s output is an accurate reflection of the steps it uses to reach the results.
Reasoning and logic are among the fundamental challenges of deep learning that might require new architectures and approaches to AI. But for the moment, better prompting techniques can help reduce the logical errors LLMs make and help troubleshoot their mistakes.
Alignment and fine-tuning techniques
Fine-tuning LLMs with application-specific datasets will improve their robustness and performance in those domains. Fine-tuning is especially useful when an LLM like GPT-3 is deployed in a specialized domain where a general-purpose model would perform poorly.
New fine-tuning techniques can further improve the accuracy of models. Of note is “reinforcement learning from human feedback” ( RLHF ), the technique used to train ChatGPT. In RLHF, human annotators vote on the answers of a pre-trained LLM. Their feedback is then used to train a reward system that further fine-tunes the LLM to become better aligned with user intents. RLHF worked very well for ChatGPT and is the reason that it is so much better than its predecessors in following user instructions.
The next step for the field will be for OpenAI, Microsoft and other providers of LLM platforms to create tools that enable companies to create their own RLHF pipelines and customize models for their applications.
Optimized LLMs
One of the big problems with LLMs is their prohibitive costs. Training and running a model the size of GPT-3 and ChatGPT can be so expensive that it will make them unavailable for certain companies and applications.
There are several efforts to reduce the costs of LLMs. Some of them are centered around creating more efficient hardware, such as special AI processors designed for LLMs.
Another interesting direction is the development of new LLMs that can match the performance of larger models with fewer parameters. One example is LLaMA , a family of small, high-performance LLMs developed by Facebook. LLaMa models are accessible for research labs and organizations that don’t have the infrastructure to run very large models.
According to Facebook, the 13-billion parameter version of LLaMa outperforms the 175-billion parameter version of GPT-3 on major benchmarks, and the 65-billion variant matches the performance of the largest models, including the 540-billion parameter PaLM.
While LLMs have many more challenges to overcome, it will be interesting how these developments will help make them more reliable and accessible to the developer and research community.
Stay in the know! Get the latest news in your inbox daily
By subscribing, you agree to VentureBeat's Terms of Service.
Thanks for subscribing. Check out more VB newsletters here .
An error occured.
Study Postgraduate
Llm by research (law) (2024 entry).
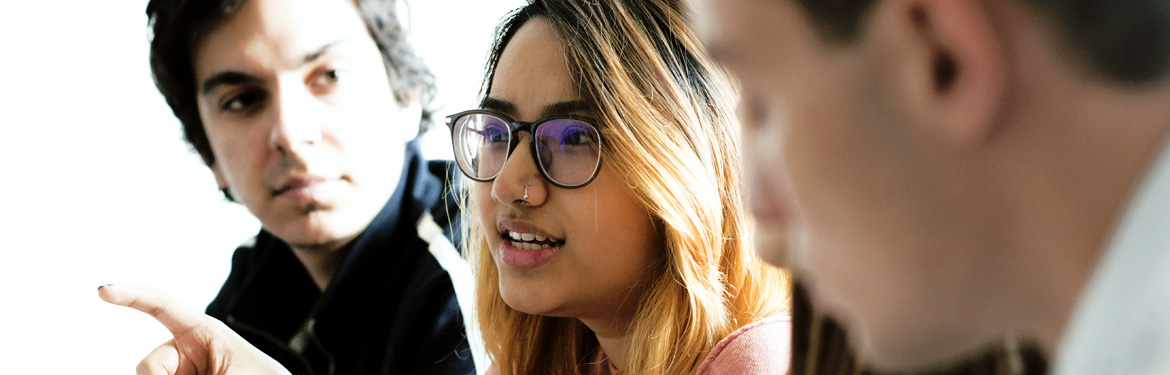
Course code
30 September 2024
1 year full-time; 2 years part-time
Qualification
- LLM by Research
University of Warwick
Find out more about our Law LLM by Research degree.
The University of Warwick's Law School offers a comprehensive LLM by Research. Pursue an extended research project in a wide range of areas, with careful supervision from a specialist.
Course overview
In this programme you will be carefully supervised by an individual specialist in your chosen area of study and supported to generate a research question and produce a thesis. For this degree you are required to write a thesis of up to 40,000 words.
Our Research Degrees attempt to achieve a balance between individual study, academic supervision, and participation in a communal, scholarly learning environment. As a research student, you will be a vital part of our research culture and we will encourage you to fully participate in the life of the Law School.
Teaching and learning
You will attend a research methods and theory course and meet with your supervisor at least once a month throughout your degree.
Each year postgraduate research students get the benefit of, feedback and presentation opportunities, skills workshops as well as a series of ‘masterclass’ events led by world-leading researchers. These workshops and events support a self-critical assessment of research methods and techniques and allow you to learn from others working in your field. In addition, you will be invited to attend research seminars, public lectures and other training opportunities with the Law School and across the University.
General entry requirements
Minimum requirements.
2:1 undergraduate degree (or equivalent) in Law or a related social sciences discipline with significant legal content.
English language requirements
You can find out more about our English language requirements Link opens in a new window . This course requires the following:
- Overall IELTS (Academic) score of 7.0 and component scores.
International qualifications
We welcome applications from students with other internationally recognised qualifications.
For more information, please visit the international entry requirements page Link opens in a new window .
Additional requirements
There are no additional entry requirements for this course.
Our research
Eleven research clusters:
- Contract, Business and Commercial Law
- Comparative Law and Culture
- Development and Human Rights
- Gender and the Law
- International and European Law
- Law and Humanities
- Legal Theory
- Governance and Regulation
- Empirical Approaches
- Arts, Culture and Law
The Law School’s research is rooted in the twin themes of law in context and the international character of law.
Explore our research areas on our Law web pages. Link opens in a new window
Find a supervisor
Find your supervisor using the link below and discuss with them the area you'd like to research.
Explore our School of Law Staff Directory where you will be able see the academic interests and expertise of our staff.
You are welcome to contact our staff directly to see if they can provide any advice on your proposed research, but will still need to submit an application and meet the selection criteria set by the University before any offer is made.
You can also see our general University guidance about finding a supervisor.
Tuition fees
Tuition fees are payable for each year of your course at the start of the academic year, or at the start of your course, if later. Academic fees cover the cost of tuition, examinations and registration and some student amenities.
Find your research course fees
Fee Status Guidance
The University carries out an initial fee status assessment based on information provided in the application and according to the guidance published by UKCISA. Students are classified as either Home or Overseas Fee status and this can determine the tuition fee and eligibility of certain scholarships and financial support.
If you receive an offer, your fee status will be stated with the tuition fee information. If you believe your fee status has been incorrectly classified you can complete a fee status assessment questionnaire (follow the instructions in your offer) and provide the required documentation for this to be reassessed.
The UK Council for International Student Affairs (UKCISA) provides guidance to UK universities on fees status criteria, you can find the latest guidance on the impact of Brexit on fees and student support on the UKCISA website .
Additional course costs
Please contact your academic department for information about department specific costs, which should be considered in conjunction with the more general costs below, such as:
- Core text books
- Printer credits
- Dissertation binding
- Robe hire for your degree ceremony
Scholarships and bursaries
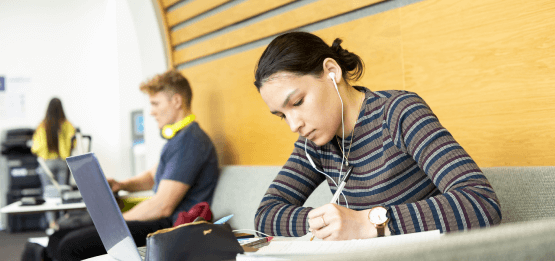
Scholarships and financial support
Find out about the different funding routes available, including; postgraduate loans, scholarships, fee awards and academic department bursaries.
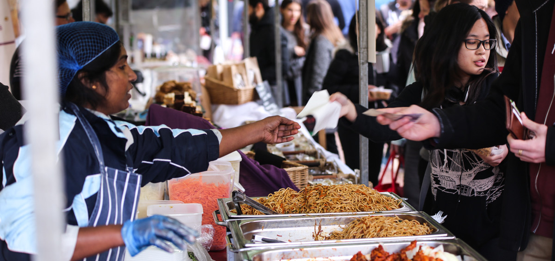
Living costs
Find out more about the cost of living as a postgraduate student at the University of Warwick.
School of Law
From the first intake of students back in 1968, Warwick Law School has developed a reputation for innovative, quality research and consistently highly rated teaching. Study with us is exciting, challenging and rewarding. Pioneers of the 'Law in Context' approach to legal education, and welcoming students and staff from around the world, we offer a friendly, international and enriching environment in which to study law in its many contexts.
Get to know us better by exploring our departmental website. Link opens in a new window
Our Postgraduate courses
- Advanced Legal Studies (LLM)
- International Commercial Law (LLM)
- International Corporate Governance and Financial Regulation (LLM)
- International Development Law and Human Rights (LLM)
- International Economic Law (LLM)
- MPhil/PhD in Law
How to apply
The application process for courses that start in September and October 2024 will open on 2 October 2023.
For research courses that start in September and October 2024 the application deadline for students who require a visa to study in the UK is 2 August 2024. This should allow sufficient time to complete the admissions process and to obtain a visa to study in the UK.
How to apply for a postgraduate research course
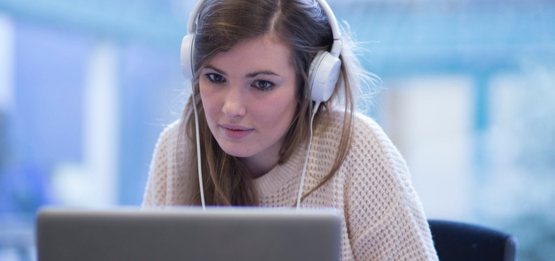
After you’ve applied
Find out how we process your application.
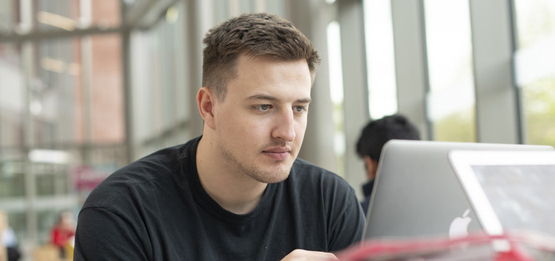
Applicant Portal
Track your application and update your details.
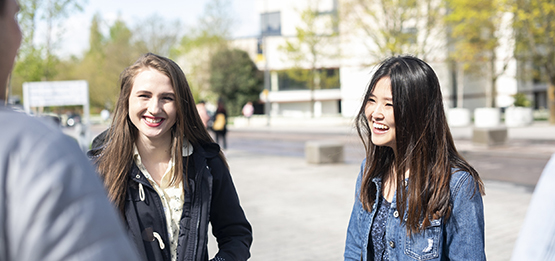
Admissions statement
See Warwick’s postgraduate admissions policy.
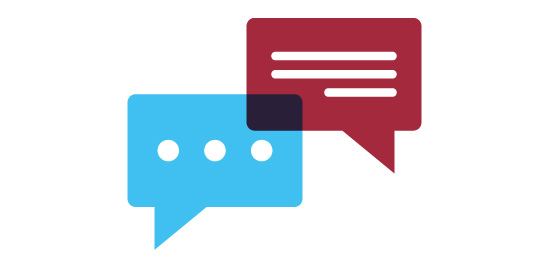
Join a live chat
Ask questions and engage with Warwick.
Warwick Hosted Events Link opens in a new window
Postgraduate fairs.
Throughout the year we attend exhibitions and fairs online and in-person around the UK. These events give you the chance to explore our range of postgraduate courses, and find out what it’s like studying at Warwick. You’ll also be able to speak directly with our student recruitment team, who will be able to help answer your questions.
Join a live chat with our staff and students, who are here to answer your questions and help you learn more about postgraduate life at Warwick. You can join our general drop-in sessions or talk to your prospective department and student services.
Departmental events
Some academic departments hold events for specific postgraduate programmes, these are fantastic opportunities to learn more about Warwick and your chosen department and course.
See our online departmental events
Warwick Talk and Tours
A Warwick talk and tour lasts around two hours and consists of an overview presentation from one of our Recruitment Officers covering the key features, facilities and activities that make Warwick a leading institution. The talk is followed by a campus tour which is the perfect way to view campus, with a current student guiding you around the key areas on campus.
Connect with us
Learn more about Postgraduate study at the University of Warwick.
We may have revised the information on this page since publication. See the edits we have made and content history .
Why Warwick
Discover why Warwick is one of the best universities in the UK and renowned globally.
9th in the UK (The Guardian University Guide 2024) Link opens in a new window
67th in the world (QS World University Rankings 2024) Link opens in a new window
6th most targeted university by the UK's top 100 graduate employers Link opens in a new window
(The Graduate Market in 2024, High Fliers Research Ltd. Link opens in a new window )
About the information on this page
This information is applicable for 2024 entry. Given the interval between the publication of courses and enrolment, some of the information may change. It is important to check our website before you apply. Please read our terms and conditions to find out more.
Committed to your wellbeing
LLM Research has conducted a variety of clinical studies in adult and pediatric patients and has contributed to the development of new therapies for healthy participants and patients struggling with diseases in multiple therapeutic areas including but not limited to pulmonology, gastroenterology, gynecology, oncology, hematology, hepatology, endocrinology, dermatology, and psychiatry. LLM Research conducts Phase I, II, III, and IV clinical trials for both large and small pharmaceutical companies and Contract Research Organizations. Our research center has earned a reputation for excellence. Our physician investigators are Board Certified in Internal Medicine, Pediatrics, Dermatology, Gynecology, and Psychiatry.
Community Partnerships
At LLM we are serious about bringing options home to you and your family! LLM has partnership with local home health organizations capable of performing research activities in your own home no matter where that is. Let nothing stop you from the treatment you and your loved ones deserve
Top Enrolling Research Site
In 2021, LLM was recognized as top enrolling research site for COVID-19 vaccine. Not only do we plan on continuing to fight covid-19 we at LLM Research, look forward to bringing other vaccine options to our community.
Do you have a family member or friend that needs treatment options? Let us know, we have them covered too!
Connect With Us
Copyright © 2024 LLM Research - All Rights Reserved.
Powered by GoDaddy
This website uses cookies.
We use cookies to analyze website traffic and optimize your website experience. By accepting our use of cookies, your data will be aggregated with all other user data.
Guidance for researchers and peer-reviewers on the ethical use of Large Language Models (LLMs) in scientific research workflows
- Opinion Paper
- Published: 16 May 2023
Cite this article
- Ryan Watkins ORCID: orcid.org/0000-0003-0488-4424 1
5070 Accesses
5 Citations
1 Altmetric
Explore all metrics
For researchers interested in exploring the exciting applications of Large Language Models (LLMs) in their scientific investigations, there is currently limited guidance and few norms for them to consult. Similarly, those providing peer-reviews on research articles where LLMs were used are without conventions or standards to apply or guidelines to follow. This situation is understandable given the rapid and recent development of LLMs that are capable of valuable contributions to research workflows (such as OpenAI’s ChatGPT). Nevertheless, now is the time to begin the development of norms, conventions, and standards that can be applied by researchers and peer-reviewers. By applying the principles of Artificial Intelligence (AI) ethics, we can better ensure that the use of LLMs in scientific research aligns with ethical principles and best practices. This editorial hopes to inspire further dialogue and research in this crucial area of scientific investigation.
Avoid common mistakes on your manuscript.
1 Introduction
Recent advancements in Large Language Models (LLMs), such as OpenAI’s ChatGPT 4 [ 1 ] and Google’s LaMDA [ 2 ], have inspired developers and researchers alike to find new applications and uses for these groundbreaking tools. [ 3 ] From applications that summarize one, or one thousand, research papers, to those that let users "chat" with a research publication, many innovative techniques and creative products have been developed in the past few months. Most recently, the first wave of research articles that use LLMs in their scientific research workflows have started to show up – primarily as preprints at this stage (for instance, [ 4 , 5 , 6 , 7 ]). As with many new research methods, statistical techniques, or technologies, the use of new tools "in the wild" routinely precedes agreement on the norms, conventions, and standards that guide their application. LLMs are no exception, with many researchers exploring their possible applications at numerous phases of scientific research workflows. Therefore, now is the time to start establishing norms, conventions, and standards [ 8 , 9 ] for the use of LLMs in scientific research, both as guidance for researchers and peer-reviewers, and as a starting place to guide future research into establishing these as foundations for applying the principles of Artificial Intelligence (AI) ethics in research practice.
The ethical use of LLMs in scientific research requires the development of norms, conventions, and standards. Just as researchers apply norms, conventions, and/or standards to hypothesis testing, regression, or CRISPR applications, researchers can benefit from guidance on how to both use, and report on their use, of LLMs in their research. Footnote 1 Similarly, for those providing peer-reviews of scientific research papers that use LLMs in their methods, guidance on current conventions and standards will be valuable. The implementation of norms, conventions, and standards plays a critical role in ensuring the ethical use of artificial intelligence (AI) in scientific research, bridging the gap between theoretical frameworks and their practical application. This is particularly relevant in research involving Large Language Models (LLMs)..
The creation and study of LLMs is a rapidly advancing field [ 3 ]. With the growing use of LLMs it is expected that the norms, conventions, and standards will evolve as new tools and techniques are introduced. Nevertheless, it is important to begin the foundation building process so that initial guidance can be systematically improved over time. In this editorial I propose an initial set of considerations that can (i) be applied by researchers to guide their use of LLMs in their workflows, and (ii) be utilized by peer-reviewers to assess the quality and ethical implications of LLMs use in the articles they review. These initial norms, conventions, and standards for what should be considered during the research process, and included in reports or articles on research that used LLMs, are a starting place with the goal of providing an ethical foundation for future dialogue on this topic. Footnote 2 The proposed foundation should ideally identify key research questions that will be explored in the coming months, such as determining the appropriate conventions for setting LLM temperature parameters and assessing potential disciplinary and field-specific variations in these conventions.
2 Framework
The following is an initial framework of proposed norms that researchers and peer-reviewers should consider when using LLMs in scientific research. While this framework is not intended to be comprehensive, it provides a foundation on which researchers can build and develop conventions and standards.
The proposed framework (which includes, context, embeddings, fine tuning, agents, ethics) was derived from the key considerations of researchers using LLMs. These considerations range from determining if LLMs are going to used in combination with other research tools and deciding when to customize LLMs with embedding models, to fine tuning the performance of LLMs and ensuring that research retains ethical rigor. As such, the proposed framework captures many unique considerations to using LLMs in the workflows of scientific research. Described first are the up-front considerations for researchers who plan to use LLMs in their workflows, followed by a checklist of questions (within the same framework) peer-reviewers should consider when reviewing articles or reports that apply LLMs in their methods.
2.1 Context
The context in which LLMs are used in research workflows is important to their appropriate and ethical application. Initial considerations of researchers should include:
Are LLMs appropriate for the research questions and data?
Will LLMs be used along with other methods or tools?
Will the study be pre registered?
LLMs are not, of course, appropriate for all research questions or data types. Researchers should begin with their research question(s) and then determine if/how LLMs might be applied. LLMs may, for instance, be an appropriate component of data collection (e.g., writing interview questions), data preparation (e.g., fuzzy joining of data sets), and/or data analysis (e.g., sentiment analysis, optimizing code). For example, in analyzing qualitative data a researcher may choose to use traditional qualitative data analysis software and techniques (such as, coding or word counts with Nvivo or Atlas TI) along with a LLM for comparing semantics across samples. Within this context, the use of the LLM complements other analysis techniques, allowing the researcher to explore more diverse questions of interest. Whereas in other contexts all of the research questions may be best explored with just LLMs or another traditional method. In their reporting, researchers should describe and justify the complete methods applied in their research and the full list of LLM tools selected since each may be specialized for a different task. Likewise, if the research study was pre registered, any subsequent articles or reports should include both the pre registration URL and discussion of any changes made from the original pre registered research plan—especially when those changes are based on the testing and fine tuning of LLMs.
2.2 Embedding Models
Adding a custom embedding model(s) to complement the base LLM (such as OpenAI’s ChatGPT) can enhance the value of LLMs for specific research task(s). Initial considerations of researchers should include:
Will a custom embedding model(s) help meet the goals of the research?
What tool(s) will be used to create the embedding model(s)?
Will multiple embedding models created and tested (i.e., chained)?
What size of chunks will be used in preparing the data for the embedding(s)?
Will overlap across chunks be permitted?
What tool will be used for similarity matching (i.e., vector database)?
Will the code for creating embedding model(s) be made publicly available?
While the web interface for some LLMs (such as ChatGPT) can be valuable for some research questions, many times supplemental content (in addition to a base LLM, such as GPT-3.5 or GPT-4) is important to the research. Custom embedding models allow researchers to extent the base LLM with content of their choosing. Technically, "Embeddings are vectors or arrays of numbers that represent the meaning and the context of the tokens that the model processes and generates. Embeddings are derived from the parameters or the weights of the model, and are used to encode and decode the input and output texts. Embeddings can help the model to understand the semantic and syntactic relationships between the tokens, and to generate more relevant and coherent texts" [ 13 ]. While LLMs use embeddings to create their base models (such as, GPT-4), researchers can also create embeddings with specialized content (such as a corpus of research articles on a topic, a drive of interview transcripts, or a database of automobile descriptors) to expand the inputs used by the LLM. Researchers can also chain together multiple embedding models in improve LLM performance [ 14 ].
There are numerous embedding models [algorithms] that can be used by researchers to create an embeddings file for use in their research [ 15 ]. Embedding models use a variety of algorithms to create the custom embeddings file, and therefore it is important for researchers to be transparent about their procedures in selecting and creating embeddings for use in their workflow. The preparation of data for creating the embedding model(s) can also influence the resulting embeddings and thereby the outputs of the LLMs when used in the workflow. For example, text has be divided into chunks in preparation for creating the embeddings and the size of chunks used will define the cut-off points for creating vectors. Researchers can, for instance, divide the text data into chunks of 1000 tokens, or 500 tokens. Depending on the context of the research, one dividing point for chunking may be more valuable than another. Chunking can also be done using sentence splitting in order to keep sentences together, or not. Likewise, researchers can allow for some overlap between chunks in order to maintain semantic context [ 16 ]. Each of these decisions can influence the output of the LLM when using additional embeddings, and thus should be considered in the research procedures and included in subsequent reporting.
After a embeddings are created for the additional content to be used in conjunction with the base LLM, the embeddings have to be stored in a database so that the data can be managed and searched. Vector databases (or vectorestores) are used, and there are many options researchers can choose amongst [ 17 ]. Vector databases use different heuristics and algorithms to index and search vectors, and can perform differently. Vector databases may use different neural search frameworks, such as FAISS, Jina.AI, or Haystack, and custom algorithms [ 18 ]. While the selection of a vector database mostly influences performance (i.e., speed, more than LLM outputs) it is useful for researchers to be transparent on their selection. In the future, differences in neural search frameworks, algorithms, and vector database technologies may lead to substantive differences in LLM outputs as well.
2.3 Fine Tuning
There are many Large Language Models (LLMs) available to researchers [ 19 ] and the selection of which LLM to use in a specific research workflow requires several decisions, including:
Which language model will used (e.g., OpenAI’s GPT-3.5, GPT-4, open source alternative)?
Will multiple language models be tested for performance in the research task(s)?
Will completion parameters be applied (e.g., temperature, presence penalty, frequency penalty, max tokens, logit bias, stops)?
Will multiple combinations of completion parameters be tested before or during the research?
Will systematic “prompt engineering” be done as part of the research?
What quality review and validation checks will be performed on LLM-generated results?
Will the LLM’s performance be compared with benchmarks or standards for the field or discipline?
Will the code for fine tuning the LLM be made publicly available?
Beyond the standard user interface and default settings offered by many LLMs (such as the ChatGPT website), by using an Application Programming Interface (API) researchers can fine tune LLMs for their research. Fine tuning can be done with or without using a embedding model(s), and is currently done primarily through setting the completion parameters (e.g., temperature) and by conducting “prompt engineering” (i.e., systematically improving LLM prompts to provide outputs with desired characteristics). Additional fine tuning options should however be expected as LLMs evolve and more competing LLMs become available to researchers.
Currently there are no conventions or standards for setting completion parameters when using LLMs in scientific research. For instance, two common parameters used to influence the outputs of LLMs are tokens and temperature.
2.3.1 Tokens
Tokens are unit of analysis of LLMs, and they are roughly equivalent to about a word, but not always. Researchers can select the number of tokens to be returned to complete a request, and the LLM will complete the request within that constraint [ 20 ]. Depending on the size of the LLM there may be limits on the total number of tokens that can be requested. There are no conventions or standards at this time for the ideal maximum number of tokens a researcher should request in order to get results, and this will routinely be dependent on the research context in which they are using the LLM. In general however, LLMs have been observed to ramble on at time (i.e., filling the maximum number of tokens) and to provide less accurate outputs toward the end when the maximum token parameter is set too high.
2.3.2 Temperature
Temperature [ 20 ] is used to provide the LLM with additional flexibility in how it completes a request. At the lowest temperature setting (e.g., 0) then the LLM is limited to selecting the next word/token that has the highest probability in the model (also see, “top p” parameter [ 20 ]). As the researcher increases the temperature ( \(\le\) 2 with OpenAI’s LLMs), the LLM may select from an increasing range of probabilities for the next word/token. Setting an appropriate temperature for the unique research context is therefore important, and in the future we will hopefully have conventions (by field and/or disciplines) on appropriate temperature parameters for research.
Other completion parameters can also influence the outputs of LLMs (e.g., “presence penalty”, “frequency penalty”, “logit bias”) and we should expect that new LLMs will expand the range of completion parameters that researchers can apply. It should be the norm, therefore, for researchers to clearly state the applied completion parameters used in their research, and describe any testing of different parameter settings done in evaluating and selecting the final parameter settings.
Prompts are the inputs provided by researchers to request a LLM response. Prompts are converted to tokens and used to inform predictions about what the following words/tokens should be in the output. Behind the curtain, LLMs are using probabilities for the various permutations and combinations of tokens/words that could follow. Changing the prompt, for instancing changing the wording of the prompt or including more prior prompts from the history of a conversation, can substantially influence the LLM’s outputs [ 21 , 22 ]. Prompt engineering is the systematic manipulation of prompts in order to improve outputs, and researchers should be transparent about both their prompt engineering procedures and the final prompts used to in the research.
At this time, however, “There are no reliable techniques for steering the behavior of LLMs” [ 3 ]. While transparency of research “prompt engineering” practices is essential, when using LLMs in research transparency may not lead to reproducability—and therefore limit generalizability.
The automation of LLM tasks can be important in some research contexts. If using automated LLM tools (i.e., agents) researcher considerations should include:
Will LLM agent(s) used in the research?
How many and in what sequence will LLM agent(s) used?
Will the code for creating the agents be made publicly available?
Many research workflows can utilize a predetermined sequence of prompts or chains of LLMs. Other workflows, however, can’t rely on predetermined sequences and/or decisions to achieve their goals. In these later cases, LLM agents can be used to make decisions about which LLMs and tools (including, for instance, internet searches [ 23 ]) to use in achieving a goal [ 24 ]. A LLM agent utilizes prompts, or LLM responses, as inputs to their (the agent’s) reasoning and decisions about which LLMs or tools to utilize next. Further, LLM agents can learn from their past performance (i.e., successes or failures) leading to improved performance [ 25 , 26 ]. If researchers apply LLM agents in their workflow, details on the agents and tools used in the research should be described. Any intermediate steps, and the sequence of those steps, should also be described since these are essential to how the final outputs of the LLM were achieved.
The use of LLMs in scientific research workflows is a new area of AI ethics that requires emerging considerations for researchers, including:
Is the organization (e.g., company, open source community) that created the LLM transparent about the choices they made in its development and fine tuning?
How will training data for additional embedding model(s) be acquired in a transparent and ethical manner?
What steps for data privacy and protections will be taken?
What will be done to identify and mitigate potential biases in LLM-generated results?
Are there any potential conflicts of interest related to the use of LLMs?
Are there any applicable institutional and/or regulatory guidelines that will be followed?
What steps will be taken for the research to be reproducible and transparent?
Will LLM outputs be described in a non-anthropomorphic manner?
The ethical use of LLMs in research workflows is a crucial consideration that cuts across multiple disciplines. From sociology and psychology to engineering management and business, LLMs have diverse applications in research, and this necessitates attention to a range of issues. These issues include technical concerns such as data privacy and bias, as well as philosophical considerations such as anthropomorphism and the epistemological challenges posed by machine-generated knowledge. Therefore, it is essential to address ethical considerations when using LLMs in research workflows to ensure that the research remains unbiased, transparent, and scientifically rigorous. While researchers may have little control, for example, over the ethical collection of data for the initial training of an LLM (such as OpenAI’s GPT-3.5), they do have choices in which LLMs to utilize in their research and the ethical collection of data used in creating any custom embedding models used in their workflows. Likewise, while there are currently limited institutional and/or regulatory policies guiding the use of LLMs in scientific research, researchers will be responsible for adhering to those AI policies (such as the EU AI Act [ 27 ]) when they are established. In the interim, researchers must be detailed and transparent about their practices, provide proper citations and credit, and disclose any conflicts of interest.
3 Conclusions
As LLMs continue to advance, their potential uses, benefits, and limitations in scientific research workflows are emerging. This presents an opportune moment to establish norms, conventions, and standards for their application in research and reporting their use in scientific publications. In this editorial, I have proposed an initial framework and set of norms for researchers to consider, including a peer-reviewer checklist (see Table 1 ) for assessing research reports and articles that employ LLMs in their methods. These proposals are not meant to be definitive, as we are still in the early stages of learning about the potential uses and limitations of LLMs. Rather, it is hoped that this foundation will stimulate research questions and inform future decisions about the norms, conventions, and standards that should be applied when using LLMs in scientific research workflows.
For example, a norm in international economics research is comparability (i.e.,the desire to compare statistics across countries) [ 10 ], where as a long-standing convention in the social sciences is to use a value of \(\alpha\) = 0.05 to define a statistically significant finding [ 11 ]. While IEEE’s P11073-10426 is a standard that defines a communication framework for interoperability with personal respiratory equipment [ 12 ].
Research and updated guidance for using LLMs in scientific research workflows are available on the clearinghouse website: https://LLMinScience.com .
OpenAI: GPT-4 Technical Report. https://cdn.openai.com/papers/gpt-4.pdf (2023)
Romal Thoppilan, E.A.: LaMDA: language models for dialog applications. arXiv preprint arXiv:2201.08239 (2022)
Bowman, S.R.: Eight things to know about large language models. arXiv preprint arXiv:2304.00612 (2023)
Crokidakis, N., de Menezes, M.A., Cajueiro, D.O.: Questions of science: chatting with ChatGPT about complex systems arXic preprint arXiv:2303.16870 (2023)
Wang, Z., Xie, Q., Ding, Z., Feng, Y., Xia, R.: Is ChatGPT a good sentiment analyzer? A preliminary study. arXiv preprint arXiv:2304.04339 (2023)
Qi, Y., Zhao, X., Huang, X.: safety analysis in the era of large language models: a case study of STPA using ChatGPT. arXiv preprint arXiv:2304.04339 (2023)
Khademi, A.: Can ChatGPT and bard generate aligned assessment items? A reliability analysis against human performance. arXiv preprint arXiv:2304.05372 (2023)
Southwood, N., Eriksson, L.: Norms and conventions. Philos. Explor. 14 (2), 195–217 (2011). https://doi.org/10.1080/13869795.2011.569748
Article Google Scholar
Bowdery, G.J.: Conventions and norms. Philos. Sci. 8 (4), 493–505 (1941). https://doi.org/10.1086/286731
Mügge, D., Linsi, L.: The national accounting paradox: how statistical norms corrode international economic data. Eur. J. Int. Relat. 27 (2), 403–427 (2021). https://doi.org/10.1177/1354066120936339 . ( PMID: 34040493 )
Johnson, V.E.: Revised standards for statistical evidence. Proc. Natl. Acad. Sci. U.S.A. 110 (48), 19313–19317 (2013)
Article MATH Google Scholar
Chang, M.: IEEE standards used in your everyday life-IEEE SA—standards.ieee.org. https://standards.ieee.org/beyond-standards/ieee-standards-used-in-your-everyday-life . Accessed 16 Apr 2023
Maeda, J.: LLM Ai Embeddings. https://learn.microsoft.com/en-us/semantic-kernel/concepts-ai/embeddings
Wu, T., Terry, M., Cai, C.J.: AI chains: transparent and controllable human-AI interaction by chaining large language model prompts. arXiv preprint arXiv:2110.01691 (2022)
Chase, H.: Text embedding models (2023). https://python.langchain.com/en/latest/modules/models/text_embedding.html?highlight=embedding
Chunking Strategies for LLM Applications. https://www.pinecone.io/learn/chunking-strategies/
Chase, H.: Vectorstores (2023). https://python.langchain.com/en/latest/modules/indexes/vectorstores.html
Kan, D.: Not all vector databases are made equal. Towards Data Science (2022). https://towardsdatascience.com/milvus-pinecone-vespa-weaviate-vald-gsi-what-unites-these-buzz-words-and-what-makes-each-9c65a3bd0696
Hannibal046: Hannibal046/Awesome-LLM: Awesome-LLM: a curated list of large language model. https://github.com/Hannibal046/Awesome-LLM
OpenAI: OpenAI API—platform.openai.com. https://platform.openai.com/docs/api-reference/completions/create . Accessed 16 Apr 2023
Si, C.: Prompting gpt-3 to be reliable. In: ICLR 2023 Proceedings. https://openreview.net/pdf?id=98p5x51L5af (2023)
White, J., Fu, Q., Hays, S., Sandborn, M., Olea, C., Gilbert, H., Elnashar, A., Spencer-Smith, J., Schmidt, D.C.: A prompt pattern catalog to enhance prompt engineering with ChatGPT. arXiv preprint arXiv:2302.11382 (2023)
Significant-Gravitas: GitHub-Significant-Gravitas/Auto-GPT: An experimental open-source attempt to make GPT-4 fully autonomous.—github.com. https://github.com/Significant-Gravitas/Auto-GPT . Accessed 16 Apr 2023 (2023)
Chase, H.: Agents. https://python.langchain.com/en/latest/modules/agents.html (2023)
Shinn, N., Labash, B., Gopinath, A.: Reflexion: an autonomous agent with dynamic memory and self-reflection. arXiv preprint arXiv:2303.11366 (2023)
Schick, T., Dwivedi-Yu, J., Dessì, R., Raileanu, R., Lomeli, M., Zettlemoyer, L., Cancedda, N., Scialom, T.: Toolformer: language models can teach themselves to use tools. arXiv preprint arXiv:2302.04761 (2023)
Union, E.: Artificial Intelligence Act (2023). https://artificialintelligenceact.eu/
Download references
Author information
Authors and affiliations.
Educational Technology Leadership, George Washington University, Washington, DC, USA
Ryan Watkins
You can also search for this author in PubMed Google Scholar
Corresponding author
Correspondence to Ryan Watkins .
Additional information
Publisher's note.
Springer Nature remains neutral with regard to jurisdictional claims in published maps and institutional affiliations.
Rights and permissions
Reprints and permissions
About this article
Watkins, R. Guidance for researchers and peer-reviewers on the ethical use of Large Language Models (LLMs) in scientific research workflows. AI Ethics (2023). https://doi.org/10.1007/s43681-023-00294-5
Download citation
Received : 18 April 2023
Accepted : 02 May 2023
Published : 16 May 2023
DOI : https://doi.org/10.1007/s43681-023-00294-5
Share this article
Anyone you share the following link with will be able to read this content:
Sorry, a shareable link is not currently available for this article.
Provided by the Springer Nature SharedIt content-sharing initiative
- Large Language Model
- Conventions
- Find a journal
- Publish with us
- Track your research
The Future of Large Language Models in 2024

Cem is the principal analyst at AIMultiple since 2017. AIMultiple informs hundreds of thousands of businesses (as per Similarweb) including 60% of Fortune 500 every month.
Cem's work has been cited by leading global publications including Business Insider, Forbes, Washington Post, global firms like Deloitte, HPE, NGOs like World Economic Forum and supranational organizations like European Commission. You can see more reputable companies and media that referenced AIMultiple.
Interest in large language models (LLMs) is on the rise especially after the release of ChatGPT in November 2022 (see Figure 1). In recent years, LLMs have transformed various industries, generating human-like text and addressing a wide range of applications. However, their effectiveness is hindered by concerns surrounding bias, inaccuracy, and toxicity, which limit their broader adoption and raise ethical concerns .
Figure 1. Google search trend for large language models over a year
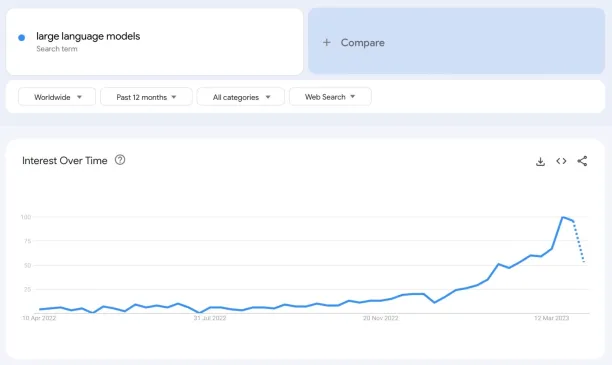
Source: Google Trends
This article explores the future of large language models by delving into promising approaches, such as self-training, fact-checking, and sparse expertise, to mitigate these issues and unlock the full potential of these models.
What is a large language model?
A large language model is a type of artificial intelligence model designed to generate and understand human-like text by analyzing vast amounts of data. These foundational models are based on deep learning techniques and typically involve neural networks with many layers and a large number of parameters, allowing them to capture complex patterns in the data they are trained on.
The primary goal of a large language model is to understand the structure, syntax, semantics, and context of natural language, so it can generate coherent and contextually appropriate responses or complete given text inputs with relevant information.
These models are trained on diverse sources of text data, including books, articles, websites, and other textual content, which enables them to generate responses to a wide range of topics.
What are the popular large language models?
Bert (google).
BERT, an acronym for Bidirectional Encoder Representations from Transformers, is a foundational model developed by Google in 2018. Based on the Transformer Neural Network architecture introduced by Google in 2017, BERT marked a departure from the prevalent natural language processing ( NLP ) approach that relied on recurrent neural networks ( RNNs ).
Before BERT, RNNs typically processed text in a left-to-right manner or combined both left-to-right and right-to-left analyses. In contrast, BERT is trained bidirectionally, allowing it to gain a more comprehensive understanding of language context and flow compared to its unidirectional predecessors.
GPT-3 & GPT-4 (OpenAI)
OpenAI’s GPT-3 , or Generative Pre-trained Transformer 3, is a large language model that has garnered significant attention for its remarkable capabilities in natural language understanding and generation. Released in June 2020, GPT-3 is the third iteration in the GPT series, building on the success of its predecessors, GPT and GPT-2.
GPT-3 became publicly used when developed into GPT-3.5 for the creation of the conversational AI tool ChatGPT which was released in November 2022.
GPT-3 uses billions of parameters, dwarfing its competitors in comparison (Figure 2). This made it the most complex large language model until its successor GPT-4.
Figure 2. The image shows how GPT-3 has a greater parameter analysis capacity than other giant NLP models
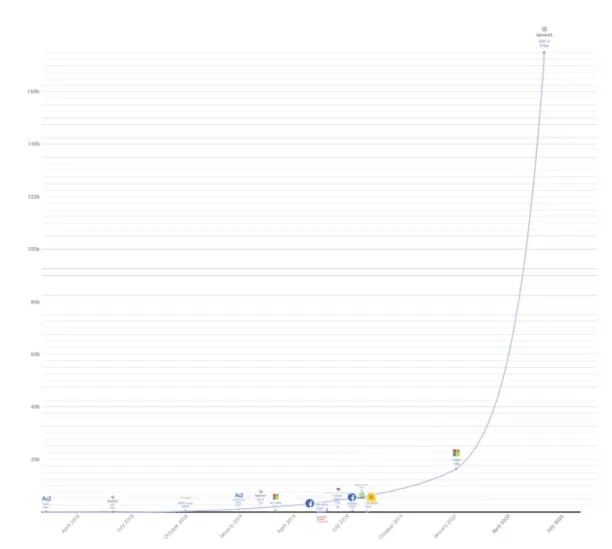
The largest language model is now OpenAI’s GPT-4 , released in March 2023. Although the model is more complex than the others in terms of its size, OpenAI didn’t share the technical details of the model.
GPT-4 is a multimodal large language model of significant size that can handle inputs of both images and text and provide outputs of text. Although it may not perform as well as humans in many real-world situations, the new model has demonstrated performance levels on several professional and academic benchmarks that are comparable to those of humans. 1
The model has various distinctive features compared to other LLMs, including:
- Visual input option
- Higher word limit
- Advanced reasoning capability
- Steerability, etc.
For a more detailed account of these capabilities of GPT-4, check our in-depth guide .
BLOOM (BigScience)
BLOOM, an autoregressive large language model, is trained using massive amounts of text data and extensive computational resources to extend text prompts. Released in July 2022, it is built on 176 parameters as a competitor of GPT-3. As a result, it can generate coherent text across 46 languages and 13 programming languages.
For a comparative analysis of the current LLMs, check our large language models examples article .
What is the current stage of large language models?
The current stage of large language models is marked by their impressive ability to understand and generate human-like text across a wide range of topics and applications. Built using advanced deep learning techniques and trained on vast amounts of data, these models, such as OpenAI’s GPT-3 and Google’s BERT, have significantly impacted the field of natural language processing.
Current LLMs have achieved state-of-the-art performance on various tasks like:
- Sentiment analysis
- Text summarization
- Translation
- Question-answering
- Code generation
Despite these achievements, language models still have various limitations that need to be addressed and fixed in the future models.
1- Accuracy
Large language models employ machine learning to deduce information, which raises concerns about potential inaccuracies. Additionally, pre-trained large language models struggle to adapt to new information dynamically, leading to potentially erroneous responses that warrant further scrutiny and improvement in future developments. Figure 3 shows the accuracy comparison of some LLMs.
Figure 3. Results for a wide variety of language models on the 5-shot HELM benchmark for accuracy
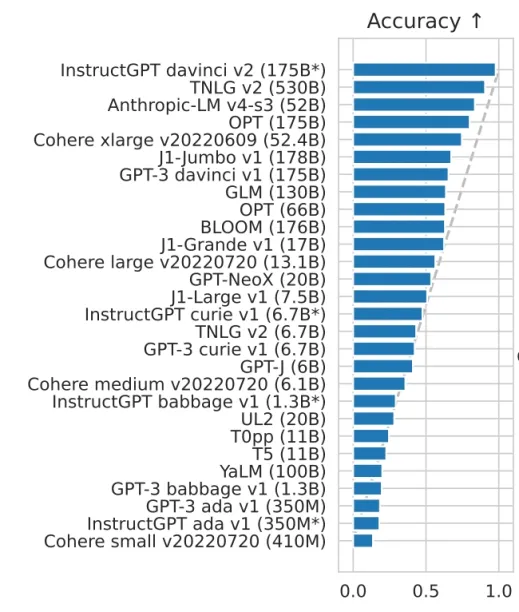
Source : “BLOOM: A 176B-Parameter Open-Access Multilingual Language Model”
Large language models facilitate human-like communication through speech and text. However, recent findings indicate that more advanced and sizable systems tend to assimilate social biases present in their training data, resulting in sexist, racist, or ableist tendencies within online communities (Figure 4).
Figure 4. Large language models’ toxicity index
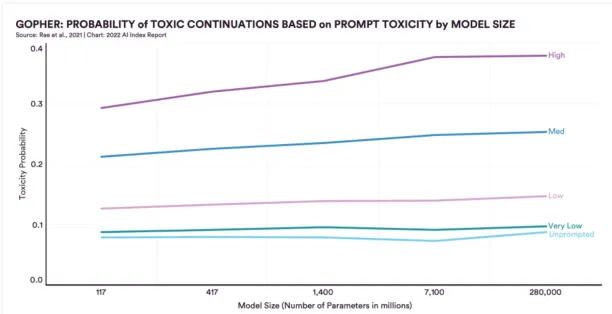
Source : Stanford University Artificial Intelligence Index Report 2022
For instance, a recent 280 billion-parameter model exhibited a substantial 29% increase in toxicity levels compared to a 117 million-parameter model from 2018. As these systems continue to advance and become more powerful tools for AI research and development, the potential for escalating bias risks also grows. Figure 5 compares the bias potential of some LLMs.
Figure 5. Results for a wide variety of language models on the 5-shot HELM benchmark for bias
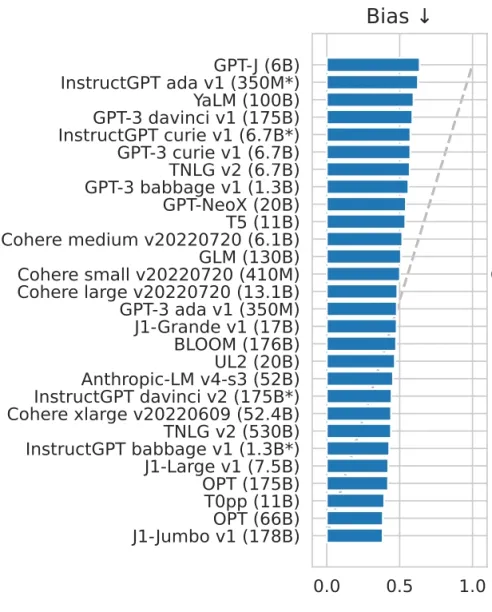
3- Toxicity
The toxicity problem of large language models refers to the issue where these models inadvertently generate harmful, offensive, or inappropriate content in their responses. This problem arises because these models are trained on vast amounts of text data from the internet, which may contain biases, offensive language, or controversial opinions.
Figure 6. Results for a wide variety of language models on the 5-shot HELM benchmark for toxicity
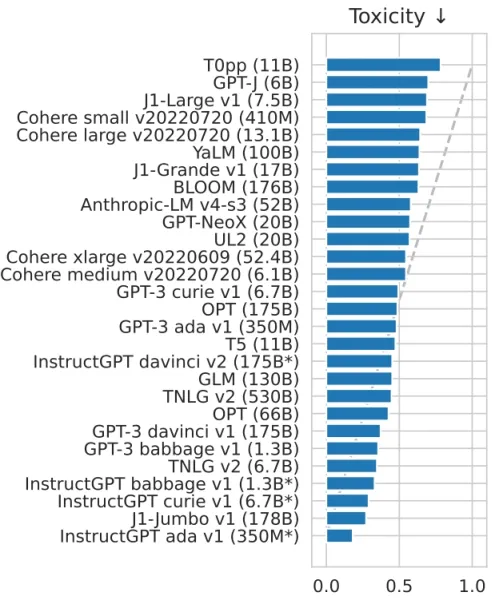
Addressing the toxicity problem in future large language models requires a multifaceted approach involving research, collaboration, and continuous improvement. Some potential strategies to mitigate toxicity in future models can include:
- Curating and improving training data
- Developing better fine-tuning techniques
- Incorporating user feedback
- Content moderation strategies
4- Capacity limitations
Every large language model has a specific memory capacity, which restricts the number of tokens it can process as input. For example, ChatGPT has a 2048-token limit (approximately 1500 words), preventing it from comprehending and producing outputs for inputs that surpass this token threshold.
GPT-4 extended the capacity to 25,000 words, far exceeding the ChatGPT model depending on GPT-3.5 (Figure 7).
Figure 7. Word limit comparison between ChatGPT and GPT-4
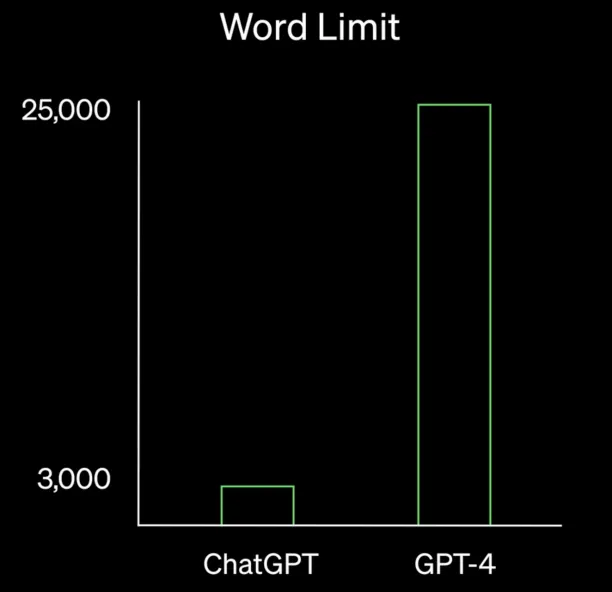
Source: OpenAI
5- Pre-trained knowledge set
Language models are trained on a fixed set of data that represents a snapshot of knowledge at a certain point in time. Once the training is complete, the model’s knowledge is frozen and cannot access up-to-date information. This means that any information or changes that occur after the training data was collected won’t be reflected in how large language models respond.
This leads to several problems regarding such as:
- Outdated or incorrect information
- Inability to handle recent events
- Less relevance in dynamic domains like technology, finance or medicine
What is the future of large language models?
It is not possible to foresee how the future language models will evolve. However, there is promising research on LLMs, focusing on the common problems we explained above. We can pinpoint 3 radical and substantial changes for future language models.
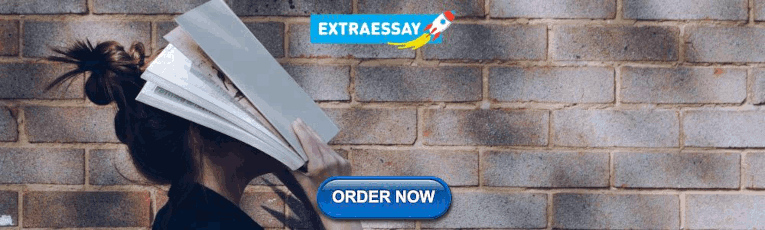
1- Fact-checking themselves
A collection of promising advancements aims to alleviate the factual unreliability and static knowledge limitations of large language models. These novel techniques are crucial for preparing LLMs for extensive real-world implementation. Doing this requires two abilities:
- The ability to access external sources
- The ability to provide citations and references for answers
Significant preliminary research in this domain features models such as Google’s REALM and Facebook’s RAG, both introduced in 2020.
In June 2022, OpenAI introduced a fine-tuned version of its GPT model called WebGPT, which utilizes Microsoft Bing to browse the internet and generate more precise and comprehensive answers to prompts. WebGPT operates similarly to a human user:
- Submitting search queries to Bing
- Clicking on links
- Scrolling web pages
- Employing functions like Ctrl+F to locate terms
When the model incorporates relevant information from the internet into its output, it includes citations, allowing users to verify the source of the information. The research results show that All WebGPT models surpass every GPT-3 model in terms of the proportion of accurate responses and the percentage of truthful and informative answers provided.
Figure 8. TruthfulQA results comparing GPT-3 and WebGPT models
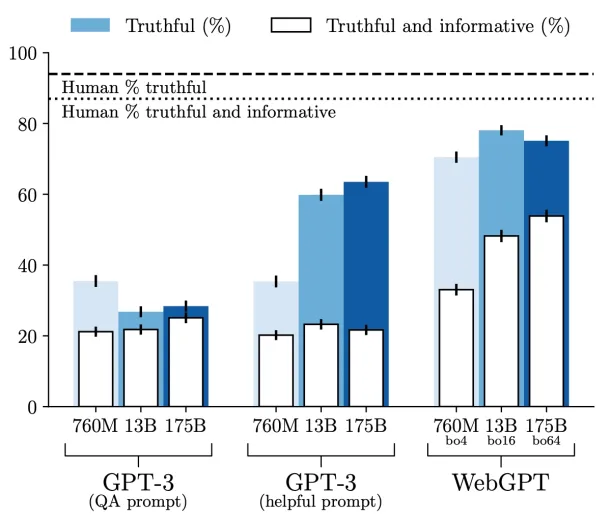
Source : “WebGPT: Browser-assisted question-answering with human feedback”
DeepMind is actively exploring similar research avenues. A few months back, they introduced a new model called Sparrow. Like ChatGPT, Sparrow operates in a dialogue-based manner, and akin to WebGPT, it can search the internet for new information and offer citations to support its claims.
Figure 9. Sparrow provides up-to-date answers and evidence for factual claims
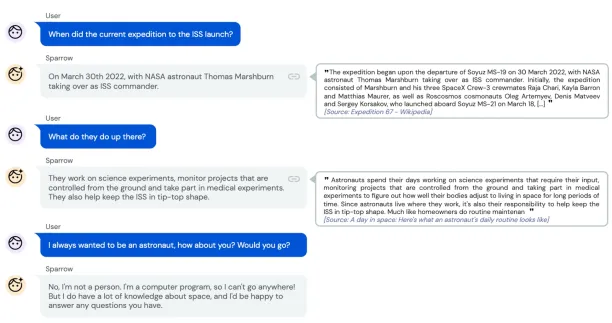
Source : “Improving alignment of dialogue agents via targeted human judgements”
Although it is still early to conclude that accuracy, fact-checking and static knowledge base problems can be overcome in the near-future models, current research results are promising for the future. This may reduce the need for using prompt engineering to cross check model output since model will already have cross-checked its results.
2- Synthetic training data
For fixing some of the limitations we mentioned above, such as those resulting from the training data, researchers are working on large language models that can generate their own training data sets (i.e. generating synthetic training data sets).
In a recent study, Google researchers developed a large language model capable of creating questions, generating comprehensive answers, filtering its responses for the highest quality output, and fine-tuning itself using the curated answers. Impressively, this resulted in new state-of-the-art performance across multiple language tasks.
Figure 10. Overview of the Google’s self-improving model
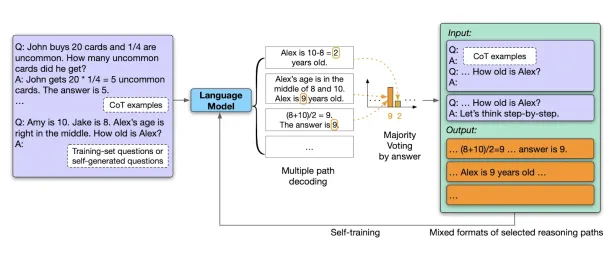
Source : “Large Language Models Can Self-Improve”
For example, the model’s performance improved from 74.2% to 82.1% on GSM8K and from 78.2% to 83.0% on DROP, which are two widely used benchmarks for evaluating LLM performance.
A recent study focuses on enhancing a crucial LLM technique called “instruction fine-tuning,” which forms the foundation of products like ChatGPT. While ChatGPT and similar instruction fine-tuned models depend on human-crafted instructions, the research team developed a model capable of generating its own natural language instructions and subsequently fine-tuning itself using those instructions.
The performance improvements are substantial, as this method boosts the base GPT-3 model’s performance by 33%, nearly equaling the performance of OpenAI’s own instruction-tuned model (Figure 11).
Figure 11. Performance of GPT3 model and its instruction-tuned variants, evaluated by human experts
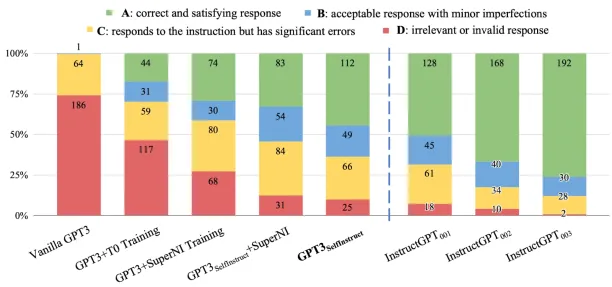
Source : “Self-Instruct: Aligning Language Model with Self Generated Instructions”
With such models in the future, it is possible to reduce biases and toxicity of the model outputs and increase the efficiency of fine-tuning with desired data sets, meaning that models learn to optimize themselves.
3- Sparse expertise
Although each model’s parameters, training data, algorithms etc. cause performance differences, all of the widely recognized language models today—such as OpenAI’s GPT-3, Nvidia/Microsoft’s Megatron-Turing, Google’s BERT—share a fundamental design in the end. They are:
- Autoregressive
- Self-supervised
- Pre-trained
- Employ densely activated transformer-based architectures
A dense language model means that each of these models use all of their parameters to create a response to a prompt. As you may guess, this is not very effective and troublesome.
A sparse expert model is the idea that a model can be able to activate only a relevant set of its parameters to answer a given prompt. Currently developed LLMs with more than 1 trillion parameters are assumed to be sparse models. 2 An example to these models is Google’s GLam with 1.2 trillion parameters.
According to Forbes, Google’s GLaM is seven times bigger than GPT-3 but consumes two-thirds less energy for training. It demands only half the computing resources for inference and exceeds GPT-3’s performance on numerous natural language tasks.
Sparse expert models mean that it is more efficient and environmentally less damaging to develop the future language models this way.
If you have questions or need help in finding vendors, we can help:
External Links
- 1. “GPT-4.” OpenAI , 14 March 2023, https://openai.com/research/gpt-4. Accessed 10 April 2023.
- 2. “The Next Generation Of Large Language Models.” Forbes , https://www.forbes.com/sites/robtoews/2023/02/07/the-next-generation-of-large-language-models/?sh=48c2008218db. Accessed 10 April 2023.

Throughout his career, Cem served as a tech consultant, tech buyer and tech entrepreneur. He advised enterprises on their technology decisions at McKinsey & Company and Altman Solon for more than a decade. He also published a McKinsey report on digitalization.
He led technology strategy and procurement of a telco while reporting to the CEO. He has also led commercial growth of deep tech company Hypatos that reached a 7 digit annual recurring revenue and a 9 digit valuation from 0 within 2 years. Cem's work in Hypatos was covered by leading technology publications like TechCrunch and Business Insider.
Cem regularly speaks at international technology conferences. He graduated from Bogazici University as a computer engineer and holds an MBA from Columbia Business School.
AIMultiple.com Traffic Analytics, Ranking & Audience , Similarweb. Why Microsoft, IBM, and Google Are Ramping up Efforts on AI Ethics , Business Insider. Microsoft invests $1 billion in OpenAI to pursue artificial intelligence that’s smarter than we are , Washington Post. Data management barriers to AI success , Deloitte. Empowering AI Leadership: AI C-Suite Toolkit , World Economic Forum. Science, Research and Innovation Performance of the EU , European Commission. Public-sector digitization: The trillion-dollar challenge , McKinsey & Company. Hypatos gets $11.8M for a deep learning approach to document processing , TechCrunch. We got an exclusive look at the pitch deck AI startup Hypatos used to raise $11 million , Business Insider.
To stay up-to-date on B2B tech & accelerate your enterprise:
Next to Read
Nlu vs nlp in 2024: main differences & use cases comparison, wu dao 2.0 in 2024: china's improved version of gpt-3, complete guide to nlp in 2024: how it works & top use cases.
Your email address will not be published. All fields are required.
Related research
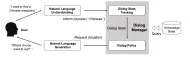
How to Build a Chatbot: Components & Architecture in 2024
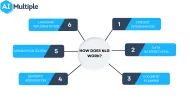
Natural Language Generation (NLG) in 2024
Suggestions or feedback?
MIT News | Massachusetts Institute of Technology
- Machine learning
- Social justice
- Black holes
- Classes and programs
Departments
- Aeronautics and Astronautics
- Brain and Cognitive Sciences
- Architecture
- Political Science
- Mechanical Engineering
Centers, Labs, & Programs
- Abdul Latif Jameel Poverty Action Lab (J-PAL)
- Picower Institute for Learning and Memory
- Lincoln Laboratory
- School of Architecture + Planning
- School of Engineering
- School of Humanities, Arts, and Social Sciences
- Sloan School of Management
- School of Science
- MIT Schwarzman College of Computing
Natural language boosts LLM performance in coding, planning, and robotics
Press contact :.
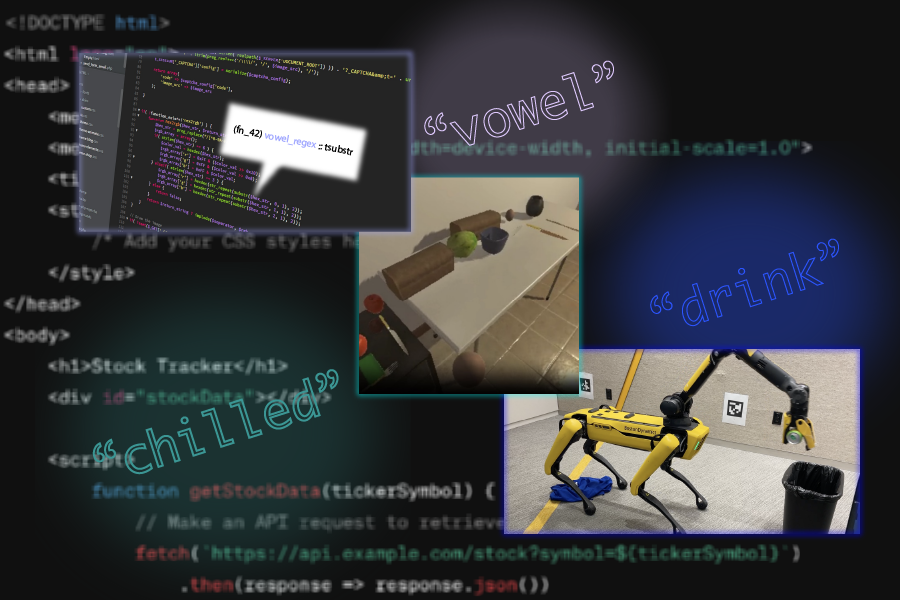
Previous image Next image
Large language models (LLMs) are becoming increasingly useful for programming and robotics tasks, but for more complicated reasoning problems, the gap between these systems and humans looms large. Without the ability to learn new concepts like humans do, these systems fail to form good abstractions — essentially, high-level representations of complex concepts that skip less-important details — and thus sputter when asked to do more sophisticated tasks. Luckily, MIT Computer Science and Artificial Intelligence Laboratory (CSAIL) researchers have found a treasure trove of abstractions within natural language. In three papers to be presented at the International Conference on Learning Representations this month, the group shows how our everyday words are a rich source of context for language models, helping them build better overarching representations for code synthesis, AI planning, and robotic navigation and manipulation. The three separate frameworks build libraries of abstractions for their given task: LILO (library induction from language observations) can synthesize, compress, and document code; Ada (action domain acquisition) explores sequential decision-making for artificial intelligence agents; and LGA (language-guided abstraction) helps robots better understand their environments to develop more feasible plans. Each system is a neurosymbolic method, a type of AI that blends human-like neural networks and program-like logical components. LILO: A neurosymbolic framework that codes Large language models can be used to quickly write solutions to small-scale coding tasks, but cannot yet architect entire software libraries like the ones written by human software engineers. To take their software development capabilities further, AI models need to refactor (cut down and combine) code into libraries of succinct, readable, and reusable programs. Refactoring tools like the previously developed MIT-led Stitch algorithm can automatically identify abstractions, so, in a nod to the Disney movie “Lilo & Stitch,” CSAIL researchers combined these algorithmic refactoring approaches with LLMs. Their neurosymbolic method LILO uses a standard LLM to write code, then pairs it with Stitch to find abstractions that are comprehensively documented in a library. LILO’s unique emphasis on natural language allows the system to do tasks that require human-like commonsense knowledge, such as identifying and removing all vowels from a string of code and drawing a snowflake. In both cases, the CSAIL system outperformed standalone LLMs, as well as a previous library learning algorithm from MIT called DreamCoder, indicating its ability to build a deeper understanding of the words within prompts. These encouraging results point to how LILO could assist with things like writing programs to manipulate documents like Excel spreadsheets, helping AI answer questions about visuals, and drawing 2D graphics.
“Language models prefer to work with functions that are named in natural language,” says Gabe Grand SM '23, an MIT PhD student in electrical engineering and computer science, CSAIL affiliate, and lead author on the research. “Our work creates more straightforward abstractions for language models and assigns natural language names and documentation to each one, leading to more interpretable code for programmers and improved system performance.”
When prompted on a programming task, LILO first uses an LLM to quickly propose solutions based on data it was trained on, and then the system slowly searches more exhaustively for outside solutions. Next, Stitch efficiently identifies common structures within the code and pulls out useful abstractions. These are then automatically named and documented by LILO, resulting in simplified programs that can be used by the system to solve more complex tasks.
The MIT framework writes programs in domain-specific programming languages, like Logo, a language developed at MIT in the 1970s to teach children about programming. Scaling up automated refactoring algorithms to handle more general programming languages like Python will be a focus for future research. Still, their work represents a step forward for how language models can facilitate increasingly elaborate coding activities. Ada: Natural language guides AI task planning Just like in programming, AI models that automate multi-step tasks in households and command-based video games lack abstractions. Imagine you’re cooking breakfast and ask your roommate to bring a hot egg to the table — they’ll intuitively abstract their background knowledge about cooking in your kitchen into a sequence of actions. In contrast, an LLM trained on similar information will still struggle to reason about what they need to build a flexible plan. Named after the famed mathematician Ada Lovelace, who many consider the world’s first programmer, the CSAIL-led “Ada” framework makes headway on this issue by developing libraries of useful plans for virtual kitchen chores and gaming. The method trains on potential tasks and their natural language descriptions, then a language model proposes action abstractions from this dataset. A human operator scores and filters the best plans into a library, so that the best possible actions can be implemented into hierarchical plans for different tasks. “Traditionally, large language models have struggled with more complex tasks because of problems like reasoning about abstractions,” says Ada lead researcher Lio Wong, an MIT graduate student in brain and cognitive sciences, CSAIL affiliate, and LILO coauthor. “But we can combine the tools that software engineers and roboticists use with LLMs to solve hard problems, such as decision-making in virtual environments.”
When the researchers incorporated the widely-used large language model GPT-4 into Ada, the system completed more tasks in a kitchen simulator and Mini Minecraft than the AI decision-making baseline “Code as Policies.” Ada used the background information hidden within natural language to understand how to place chilled wine in a cabinet and craft a bed. The results indicated a staggering 59 and 89 percent task accuracy improvement, respectively. With this success, the researchers hope to generalize their work to real-world homes, with the hopes that Ada could assist with other household tasks and aid multiple robots in a kitchen. For now, its key limitation is that it uses a generic LLM, so the CSAIL team wants to apply a more powerful, fine-tuned language model that could assist with more extensive planning. Wong and her colleagues are also considering combining Ada with a robotic manipulation framework fresh out of CSAIL: LGA (language-guided abstraction). Language-guided abstraction: Representations for robotic tasks Andi Peng SM ’23, an MIT graduate student in electrical engineering and computer science and CSAIL affiliate, and her coauthors designed a method to help machines interpret their surroundings more like humans, cutting out unnecessary details in a complex environment like a factory or kitchen. Just like LILO and Ada, LGA has a novel focus on how natural language leads us to those better abstractions. In these more unstructured environments, a robot will need some common sense about what it’s tasked with, even with basic training beforehand. Ask a robot to hand you a bowl, for instance, and the machine will need a general understanding of which features are important within its surroundings. From there, it can reason about how to give you the item you want.
In LGA’s case, humans first provide a pre-trained language model with a general task description using natural language, like “bring me my hat.” Then, the model translates this information into abstractions about the essential elements needed to perform this task. Finally, an imitation policy trained on a few demonstrations can implement these abstractions to guide a robot to grab the desired item. Previous work required a person to take extensive notes on different manipulation tasks to pre-train a robot, which can be expensive. Remarkably, LGA guides language models to produce abstractions similar to those of a human annotator, but in less time. To illustrate this, LGA developed robotic policies to help Boston Dynamics’ Spot quadruped pick up fruits and throw drinks in a recycling bin. These experiments show how the MIT-developed method can scan the world and develop effective plans in unstructured environments, potentially guiding autonomous vehicles on the road and robots working in factories and kitchens.
“In robotics, a truth we often disregard is how much we need to refine our data to make a robot useful in the real world,” says Peng. “Beyond simply memorizing what’s in an image for training robots to perform tasks, we wanted to leverage computer vision and captioning models in conjunction with language. By producing text captions from what a robot sees, we show that language models can essentially build important world knowledge for a robot.” The challenge for LGA is that some behaviors can’t be explained in language, making certain tasks underspecified. To expand how they represent features in an environment, Peng and her colleagues are considering incorporating multimodal visualization interfaces into their work. In the meantime, LGA provides a way for robots to gain a better feel for their surroundings when giving humans a helping hand.
An “exciting frontier” in AI
“Library learning represents one of the most exciting frontiers in artificial intelligence, offering a path towards discovering and reasoning over compositional abstractions,” says assistant professor at the University of Wisconsin-Madison Robert Hawkins, who was not involved with the papers. Hawkins notes that previous techniques exploring this subject have been “too computationally expensive to use at scale” and have an issue with the lambdas, or keywords used to describe new functions in many languages, that they generate. “They tend to produce opaque 'lambda salads,' big piles of hard-to-interpret functions. These recent papers demonstrate a compelling way forward by placing large language models in an interactive loop with symbolic search, compression, and planning algorithms. This work enables the rapid acquisition of more interpretable and adaptive libraries for the task at hand.” By building libraries of high-quality code abstractions using natural language, the three neurosymbolic methods make it easier for language models to tackle more elaborate problems and environments in the future. This deeper understanding of the precise keywords within a prompt presents a path forward in developing more human-like AI models. MIT CSAIL members are senior authors for each paper: Joshua Tenenbaum, a professor of brain and cognitive sciences, for both LILO and Ada; Julie Shah, head of the Department of Aeronautics and Astronautics, for LGA; and Jacob Andreas, associate professor of electrical engineering and computer science, for all three. The additional MIT authors are all PhD students: Maddy Bowers and Theo X. Olausson for LILO, Jiayuan Mao and Pratyusha Sharma for Ada, and Belinda Z. Li for LGA. Muxin Liu of Harvey Mudd College was a coauthor on LILO; Zachary Siegel of Princeton University, Jaihai Feng of the University of California at Berkeley, and Noa Korneev of Microsoft were coauthors on Ada; and Ilia Sucholutsky, Theodore R. Sumers, and Thomas L. Griffiths of Princeton were coauthors on LGA. LILO and Ada were supported, in part, by MIT Quest for Intelligence, the MIT-IBM Watson AI Lab, Intel, U.S. Air Force Office of Scientific Research, the U.S. Defense Advanced Research Projects Agency, and the U.S. Office of Naval Research, with the latter project also receiving funding from the Center for Brains, Minds and Machines. LGA received funding from the U.S. National Science Foundation, Open Philanthropy, the Natural Sciences and Engineering Research Council of Canada, and the U.S. Department of Defense.
Share this news article on:
Related links.
- Jacob Andreas
- Computer Science and Artificial Intelligence Laboratory (CSAIL)
- MIT Language and Intelligence Group
- Center for Brains, Minds, and Machines
- Department of Electrical Engineering and Computer Science
- Department of Brain and Cognitive Sciences
- Department of Aeronautics and Astronautics
Related Topics
- Computer science and technology
- Artificial intelligence
- Natural language processing
- Electrical Engineering & Computer Science (eecs)
- Programming
- Human-computer interaction
- Computer vision
- Brain and cognitive sciences
- Programming languages
- Center for Brains Minds and Machines
- Quest for Intelligence
- MIT-IBM Watson AI Lab
- National Science Foundation (NSF)
- Department of Defense (DoD)
- Defense Advanced Research Projects Agency (DARPA)
Related Articles
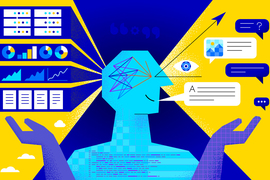
Large language models use a surprisingly simple mechanism to retrieve some stored knowledge
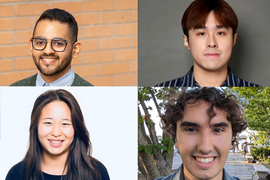
Reasoning and reliability in AI
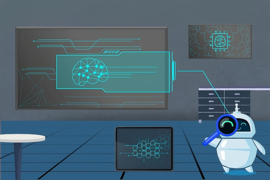
AI agents help explain other AI systems
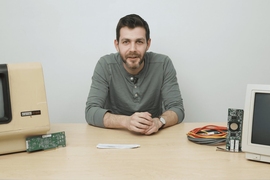
3 Questions: Jacob Andreas on large language models
Previous item Next item
More MIT News
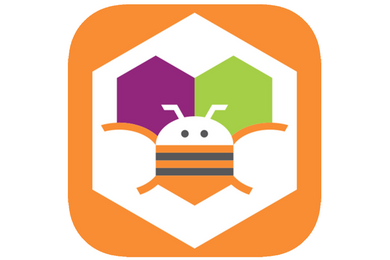
The power of App Inventor: Democratizing possibilities for mobile applications
Read full story →
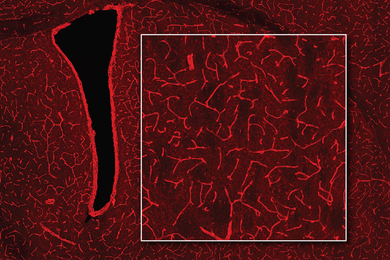
Using MRI, engineers have found a way to detect light deep in the brain
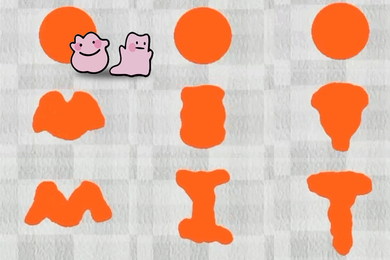
A better way to control shape-shifting soft robots
From steel engineering to ovarian tumor research
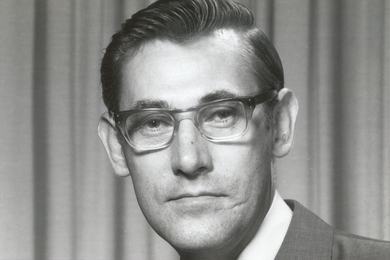
Professor Emeritus David Lanning, nuclear engineer and key contributor to the MIT Reactor, dies at 96
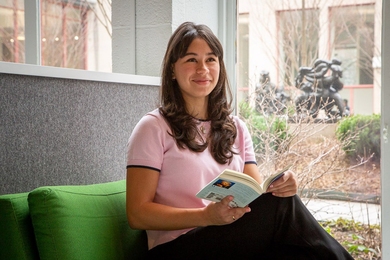
Discovering community and cultural connections
- More news on MIT News homepage →
Massachusetts Institute of Technology 77 Massachusetts Avenue, Cambridge, MA, USA
- Map (opens in new window)
- Events (opens in new window)
- People (opens in new window)
- Careers (opens in new window)
- Accessibility
- Social Media Hub
- MIT on Facebook
- MIT on YouTube
- MIT on Instagram
Microsoft Research Blog
Llm profiling guides kv cache optimization.
Published May 8, 2024
By Liyuan Liu , Senior Researcher Jianfeng Gao , Distinguished Scientist & Vice President
Share this page
- Share on Facebook
- Share on Twitter
- Share on LinkedIn
- Share on Reddit
- Subscribe to our RSS feed
This research paper was presented at the 12 th International Conference on Learning Representations (opens in new tab) (ICLR 2024), the premier conference dedicated to the advancement of deep learning.

Large language models (LLMs) rely on complex internal mechanisms that require more memory than what is typically available to operate on standard devices. One such mechanism is the key-value (KV) cache, which stores and retrieves previously computed data, helping the model generate responses quickly without needing to recalculate information it has already processed. This method uses a substantial amount of memory because it keeps a large amount of this data readily accessible to enhance the model’s speed and efficiency. Consequently, the KV cache can become prohibitively large as the complexity of the tasks increases, sometimes requiring up to 320 GB for a single operation. To address this, we developed FastGen, a novel method aimed at reducing the memory demands for LLMs.
- Publication Model Tells You What to Discard: Adaptive KV Cache Compression for LLMs
Our paper, “ Model Tells You What to Discard: Adaptive KV Cache Compression for LLMs (opens in new tab) ,” presented at ICLR 2024, we describe how FastGen optimizes the way LLMs store and access data, potentially cutting memory use by half while preserving their efficiency. This approach represents a significant step toward making sophisticated AI tools more accessible and affordable for broader applications. We are delighted to share that this paper has been given an Honorable Mention for the Outstanding Paper Award (opens in new tab) .
Observations of the KV cache
The development of FastGen is underpinned by our observations of how the KV cache functions. We first observed that not all the data in the KV cache is needed for LLMs to complete their required tasks, as shown in Figure 1. By providing the KV cache with the mechanism to discard unnecessary data, it is possible to significantly cut memory use. For example, some LLM modules don’t require broad contexts to process input. For this, it is possible to construct a KV cache that removes data that contains less important long-range contexts, such as several sentences or paragraphs. Also, some LLM modules primarily attend only to special tokens, such as punctuation, for which it is possible to create a KV cache that retains only those tokens. Finally, some LLM modules broadly need all tokens, and for these we can employ the standard KV cache and store all words.
Another key observation in our study is that attention modules in different layers and positions in the LLM behave differently and need different preferences for their KV cache, as shown on the right in Figure 1.
Microsoft Research Podcast

AI Frontiers: Models and Systems with Ece Kamar
Ece Kamar explores short-term mitigation techniques to make these models viable components of the AI systems that give them purpose and shares the long-term research questions that will help maximize their value.

FastGen accounts for the diversity of KV cache structures
Because different KV caches have different structures, they need to be handled differently. We based the development of the FastGen algorithm on our observations, enabling it to categorize and optimize the data that is stored in a given KV cache. FastGen first analyzes the specific behaviors of different modules to understand their structures, a method called profiling . It then uses the results to adjust how data is stored in real-time, making the process more efficient. Our tests show that FastGen can reduce the amount of memory by 50% without sacrificing quality. Additional experiments, discussed in detail in our paper , confirm that the profiling process is crucial and significantly improves the efficiency of the KV cache.
The broader picture
Fueled by unprecedented advances in data handling and computational capabilities, LLM pretraining has emerged as a cornerstone of deep learning, transforming natural language processing tasks and continuously challenging our understanding of learning and cognition.
However, greater capabilities can bring challenges. As models scale larger, customizing them for specific tasks can become more resource-intensive. At Microsoft Research, we are exploring different approaches to more efficient model editing. A critical strategy involves targeted model profiling, which identifies essential components of a model that align with predefined goals. This profiling informs precise model modifications, optimizing resource use and effectiveness.
The two research projects we are presenting at ICLR 2024 support these goals. Both adopt the profile-then-edit paradigm to address different problems. FastGen reduces memory consumption. Our related work, Post-hoc Attention Steering for LLMs (PASTA) , focuses on better controllability. These approaches are designed to be resource-efficient, as they do not require tuning or back propagation. Looking ahead, our goal is to further develop these techniques to improve the resource-efficiency of LLM applications, making them more accessible to a wider audience.
Related publications
Model tells you what to discard: adaptive kv cache compression for llms, tell your model where to attend: post-hoc attention steering for llms, meet the authors.

Senior Researcher

Jianfeng Gao
Distinguished Scientist & Vice President
Continue reading

LoftQ: Reimagining LLM fine-tuning with smarter initialization

AI Controller Interface: Generative AI with a lightweight, LLM-integrated VM

Splitwise improves GPU usage by splitting LLM inference phases

Skeleton-of-Thought: Parallel decoding speeds up and improves LLM output
Research areas.

Related events
- Microsoft at ICLR 2024
- Follow on Twitter
- Like on Facebook
- Follow on LinkedIn
- Subscribe on Youtube
- Follow on Instagram
Share this page:
Thank you for visiting nature.com. You are using a browser version with limited support for CSS. To obtain the best experience, we recommend you use a more up to date browser (or turn off compatibility mode in Internet Explorer). In the meantime, to ensure continued support, we are displaying the site without styles and JavaScript.
- View all journals
- My Account Login
- Explore content
- About the journal
- Publish with us
- Sign up for alerts
- Open access
- Published: 14 December 2023
Mathematical discoveries from program search with large language models
- Bernardino Romera-Paredes ORCID: orcid.org/0000-0003-3604-3590 1 na1 ,
- Mohammadamin Barekatain ORCID: orcid.org/0000-0002-8470-8203 1 na1 ,
- Alexander Novikov 1 na1 ,
- Matej Balog ORCID: orcid.org/0000-0002-5552-9855 1 na1 ,
- M. Pawan Kumar 1 na1 ,
- Emilien Dupont 1 na1 ,
- Francisco J. R. Ruiz ORCID: orcid.org/0000-0002-2200-901X 1 na1 ,
- Jordan S. Ellenberg 2 ,
- Pengming Wang ORCID: orcid.org/0009-0009-4976-4267 1 ,
- Omar Fawzi 3 ,
- Pushmeet Kohli ORCID: orcid.org/0000-0002-7466-7997 1 &
- Alhussein Fawzi ORCID: orcid.org/0000-0001-7341-1917 1 na1
Nature volume 625 , pages 468–475 ( 2024 ) Cite this article
184k Accesses
8 Citations
1022 Altmetric
Metrics details
- Computer science
- Pure mathematics
Large language models (LLMs) have demonstrated tremendous capabilities in solving complex tasks, from quantitative reasoning to understanding natural language. However, LLMs sometimes suffer from confabulations (or hallucinations), which can result in them making plausible but incorrect statements 1 , 2 . This hinders the use of current large models in scientific discovery. Here we introduce FunSearch (short for searching in the function space), an evolutionary procedure based on pairing a pretrained LLM with a systematic evaluator. We demonstrate the effectiveness of this approach to surpass the best-known results in important problems, pushing the boundary of existing LLM-based approaches 3 . Applying FunSearch to a central problem in extremal combinatorics—the cap set problem—we discover new constructions of large cap sets going beyond the best-known ones, both in finite dimensional and asymptotic cases. This shows that it is possible to make discoveries for established open problems using LLMs. We showcase the generality of FunSearch by applying it to an algorithmic problem, online bin packing, finding new heuristics that improve on widely used baselines. In contrast to most computer search approaches, FunSearch searches for programs that describe how to solve a problem, rather than what the solution is. Beyond being an effective and scalable strategy, discovered programs tend to be more interpretable than raw solutions, enabling feedback loops between domain experts and FunSearch, and the deployment of such programs in real-world applications.
Similar content being viewed by others
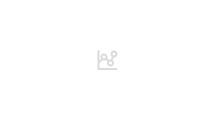
Accurate structure prediction of biomolecular interactions with AlphaFold 3
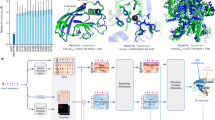
Highly accurate protein structure prediction with AlphaFold
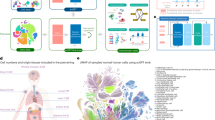
scGPT: toward building a foundation model for single-cell multi-omics using generative AI
Many problems in mathematical sciences are ‘easy to evaluate’, despite being typically ‘hard to solve’. For example, in computer science, NP-complete optimization problems admit a polynomial-time evaluation procedure (measuring the quality of the solution), despite the widespread belief that no polynomial-time algorithms to solve such problems exist. We focus in this paper on problems admitting an efficient ‘evaluate’ function, which measures the quality of a candidate solution. Prominent examples include the maximum independent set problem and maximum constraint satisfaction problems (such as finding the ground state energy of a Hamiltonian). Our goal is to generate a ‘solve’ program, such that its outputs receive high scores from the ‘evaluate’ function (when executed on inputs of interest), and ultimately improve on the best-known solutions.
Whereas large language models (LLMs) have recently seen notable improvements in their coding capabilities 4 , 5 , 6 , 7 , 8 , with applications including debugging 9 , 10 , solving code competitions 11 , 12 and improving code performance 13 , synthesizing ‘solve’ programs for open problems requires finding new ideas that are verifiably correct. This is very hard for LLMs, as they tend to confabulate or ultimately fall short of going beyond existing results. To surpass the ‘nominal’ capabilities of LLMs, recent studies 3 have combined them with evolutionary algorithms 14 , 15 , leading to important improvements on diverse synthetic problems 16 , searching for neural network architectures 17 , 18 , 19 and solving puzzles 20 . Our proposed method, FunSearch, pushes the boundary of LLM-guided evolutionary procedures to a new level: the discovery of new scientific results for established open problems and the discovery of new algorithms. Surpassing state-of-the-art results on established open problems provides a clear indication that the discoveries are truly new, as opposed to being retrieved from the LLM’s training data.
FunSearch (short for searching in the function space) combines a pretrained (frozen) LLM, whose goal is to provide creative solutions, with an evaluator, which guards against confabulations and incorrect ideas. FunSearch iterates over these two components, evolving initial low-scoring programs into high-scoring ones discovering new knowledge. Key to the success of this simple procedure is a combination of several essential ingredients. First, we sample best performing programs and feed them back into prompts for the LLM to improve on; we refer to this as best-shot prompting. Second, we start with a program in the form of a skeleton (containing boilerplate code and potentially known structure about the problem), and only evolve the part governing the critical program logic. For example, by setting a greedy program skeleton, we evolve a priority function used to make decisions at every step. Third, we maintain a large pool of diverse programs by using an island-based evolutionary method that encourages exploration and avoids local optima. Finally, leveraging the highly parallel nature of FunSearch, we scale it asynchronously, considerably broadening the scope of this approach to find new results, while keeping the overall cost of experiments low.
We show the surprising effectiveness of FunSearch on several use cases. We consider a fundamental problem in extremal combinatorics, namely, the cap set problem 21 , 22 . FunSearch demonstrates the existence of hitherto unknown constructions that go beyond existing ones, including the largest improvement in 20 years to the asymptotic lower bound. This demonstrates that it is possible to make a scientific discovery—a new piece of verifiable knowledge about a notorious scientific problem—using an LLM. Using FunSearch, we also find new algorithms for the online bin packing problem that improve on traditional ones on well-studied distributions of interest 23 , 24 , with potential applications to improving job scheduling algorithms.
Whereas most computer search techniques output directly what the solution is (for example, a list of vectors forming a cap set), FunSearch produces programs generating the solution. For structured problems, such programs tend to be more interpretable—facilitating interactions with domain experts—and concise—making it possible to scale to large instances—compared to a mere enumeration of the solution. In addition, decision procedures (such as for bin packing) described by code in a standard programming language are crucially easier to deploy compared to other types of descriptions (for example, neural networks), which typically require specialized hardware and for which verifying design specifications is notoriously hard.
An overview of FunSearch is shown in Fig. 1 , and its components are described in more detail below. For more details and ablations showing the importance of each component, see Methods and Supplementary Information Appendix A .
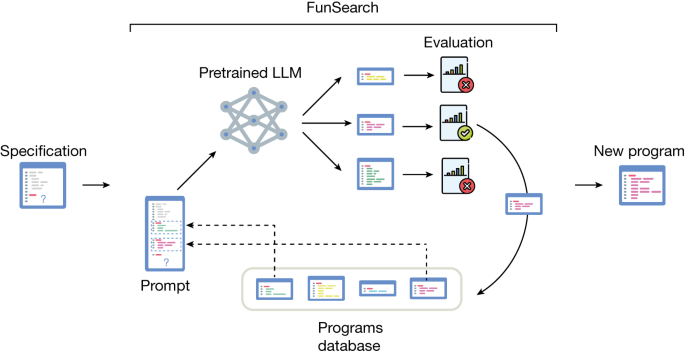
The input to FunSearch is a specification of the problem in the form of an ‘evaluate’ function, an initial implementation of the function to evolve, which can be trivial, and potentially a skeleton. At each iteration, FunSearch builds a prompt by combining several programs sampled from the programs database (favouring high-scoring ones). The prompt is then fed to the pretrained LLM and new programs are created. Newly created programs are then scored and stored in the programs database (if correct), thus closing the loop. The user can at any point retrieve the highest-scoring programs discovered so far.
Specification
The input to FunSearch is a specification of the problem in the form of an ‘evaluate’ function, which scores candidate solutions. In addition, we provide an initial program (which can be trivial) to evolve. Although in principle these are the minimum requirements, we found that performance tends to improve significantly if we write the initial ‘solve’ program in the form of a skeleton (containing boilerplate code and previous knowledge of the problem in the form of a program structure), and only use FunSearch to evolve the critical part that governs its logic. Fig. 2a shows an example in which the skeleton takes the form of a simple greedy algorithm, and the crucial part to evolve by FunSearch is the priority function that is used to make the greedy decision at every step. This delegates to FunSearch precisely the part that is usually the hardest to come up with. Whereas a fixed skeleton may constrain the space of programs that can be discovered, we find it improves overall results because it focuses the LLM resources on only evolving the critical part, instead of also using the LLM to recreate already known program structures (with more opportunities for mistakes that would render the entire program incorrect). If available, the user can optionally provide extra known information about the problem at hand, in the form of docstrings, relevant primitive functions or import packages, which FunSearch may use.
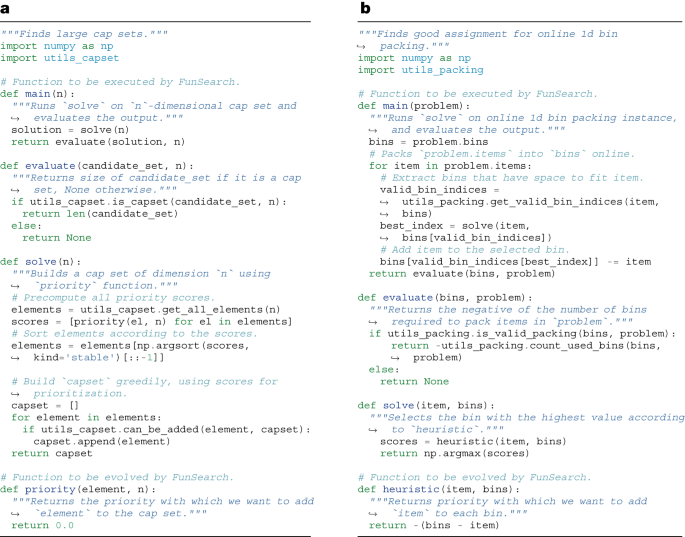
The ‘evaluate’ function takes as input a candidate solution to the problem, and returns a score assessing it. The ‘solve’ function contains the algorithm skeleton, which calls the function to evolve that contains the crucial logic. a , Cap set. The function to evolve is called ‘priority’. b , Online bin packing. The function to evolve is called ‘heuristic’. The ‘main’ function implements the evaluation procedure by connecting the pieces together. Specifically, it uses the ‘solve’ function to solve the problem and then scores the resulting solutions using the ‘evaluate’ function. In the simplest cases, ‘main’ just executes ‘solve’ once and uses ‘evaluate’ to score the output, for example, a . In specific settings such as online algorithms, the ‘main’ function implements some more logic, for example, b .
Pretrained LLM
The LLM is the creative core of FunSearch, in charge of coming up with improvements to the functions presented in the prompt and sending these for evaluation. We obtain our results with a pretrained model, that is, without any fine-tuning on our problems. We use Codey, an LLM built on top of the PaLM2 model family 25 , which has been fine-tuned on a large corpus of code and is publicly accessible through its API 26 . Because FunSearch relies on sampling from an LLM extensively, an important performance-defining tradeoff is between the quality of the samples and the inference speed of the LLM. In practice, we have chosen to work with a fast-inference model (rather than slower-inference, higher-quality), and the results in the paper are obtained using a total number of samples on the order of 10 6 . Beyond this tradeoff, we have empirically observed that the results obtained in this paper are not too sensitive to the exact choice of LLM, as long as it has been trained on a large enough corpus of code. See Supplementary Information Appendix A for a comparison to StarCoder 6 , a state-of-the-art open-source LLM for code.
Programs generated by the LLM are evaluated and scored on a set of inputs. For example, in the cap set problem (‘Extremal combinatorics’ section) the inputs are the values of the dimensionality n that we are interested in, and in combinatorial optimization (‘Bin packing’ section), the inputs correspond to different bin packing instances. The scores across different inputs are then combined into an overall score of the program using an aggregation function, such as the mean. The scored programs are then sent to the programs database. Programs that were incorrect (that did not execute within the imposed time and memory limits, or produced invalid outputs) are discarded, and the remaining scored programs are then sent to the programs database.
Programs database
The programs database keeps a population of correct programs, which are then sampled to create prompts. Preserving and encouraging diversity of programs in the database is crucial to enable exploration and avoid being stuck in local optima. To encourage diversity, we adopt an islands model, also known as a multiple population and multiple-deme model 27 , 28 , which is a genetic algorithm approach. Several islands, or subpopulations, are created and evolved independently. To sample from the program database, we first sample an island and then sample a program within that island, favouring higher-scoring and shorter programs (see Methods for the exact mechanism). Crucially, we let information flow between the islands by periodically discarding the programs in the worst half of the islands (corresponding to the ones whose best individuals have the lowest scores). We replace the programs in those islands with a new population, initialized by cloning one of the best individuals from the surviving islands.
New prompts are created by ‘best-shot prompting’ from the programs database, and are then fed to the LLM to generate a new program. We first sample k programs from a single island in the programs database, according to the procedure described above. Sampled programs are then sorted according to their score, and a version is assigned to each (‘v0’ for the lowest scoring program, ‘v1’ for the second lowest scoring and so on). These programs are then combined into a single prompt—with the version appended as a suffix to the function name; for example, in the case of Fig. 2a , this would be ‘ p riority_v0’, ‘priority_v1’, ...—and the header of the function we wish to generate (for example, ‘priority_vk’) is added to the end of the prompt. In practice, we set k = 2, as two functions lead to better results compared to just one, with diminishing returns beyond that. Constructing a prompt by combining several programs (as opposed to only one) enables the LLM to spot patterns across the different programs and generalize those. Related approaches to prompt building have been recently considered, for example ref. 16 , and were shown to perform well on different domains.
Distributed approach
We implement FunSearch as a distributed system that has three types of workers—a programs database, samplers and evaluators—which communicate asynchronously. The programs database stores and serves programs, samplers generate new functions using the pretrained LLM and evaluators assess programs, as shown in Supplementary Fig. F.26 . In the example shown in Fig. 2a , the programs database stores priority functions, samplers generate new implementations of ‘priority’ and evaluators score the proposals by executing the ‘main’ function on user-specified inputs. Our distributed system offers several advantages. First, it naturally leverages parallelism across different tasks: for example, LLM sampling and evaluation are performed concurrently. Second, it enables scaling to more than one sampler and evaluator, which would be a very limiting setup, considering that evaluation can take minutes for many problems of interest. Running evaluators in parallel considerably broadens the scope of this approach to such problems. The distributed setting enables the running of many evaluator nodes on inexpensive CPU hardware, whereas few samplers run on machines with accelerators for fast LLM inference; this keeps the overall cost and energy usage of experiments low. In our experiments, we typically use 15 samplers and 150 CPU evaluators (can be served on five CPU servers each running 32 evaluators in parallel). See Supplementary Information Appendix A for more details. Also, because of the randomness of LLM sampling and the evolutionary procedure, for some problems we run several experiments to get the best reported results. See Methods and Supplementary Information Appendix A.3 for a full statistical analysis.
We now describe some of the new discoveries made by FunSearch in two different fields: pure mathematics and applied computer science. Further discoveries on other problems (namely, the corners problem and Shannon capacity of cycle graphs) are presented in Supplementary Information Appendix B . The full discovered programs are available in Supplementary Information Appendix C .
Extremal combinatorics
We apply FunSearch to two related problems in extremal combinatorics: a branch of mathematics that studies the maximal (or minimal) possible sizes of sets satisfying certain properties.
The cap set problem 21 , once described by Terence Tao as ‘perhaps my favourite open question’ 29 , refers to the task of finding the largest possible set of vectors in \({{\mathbb{Z}}}_{3}^{n}\) (known as a cap set) such that no three vectors sum to zero. Geometrically, no three points of a cap set are in a line (see Fig. 3 for an example with n = 2).
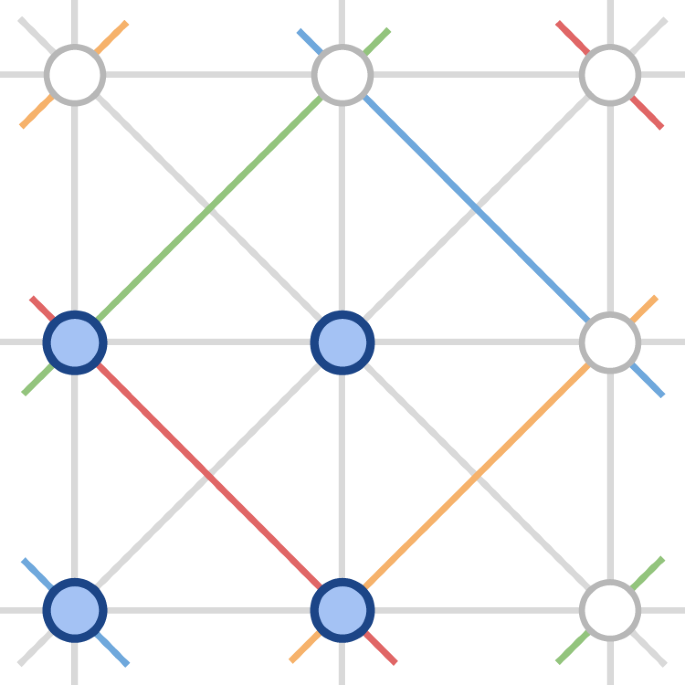
The circles are the elements of \({{\mathbb{Z}}}_{3}^{2}\) with the ones belonging to the cap set shown in blue. The possible lines in \({{\mathbb{Z}}}_{3}^{2}\) are also shown (with colours indicating lines that wrap around in arithmetic modulo 3). No three elements of the cap set are in a line.
The problem has drawn much interest for a variety of reasons. For one, it is an analogue of the classical number theory problem of finding large subsets of primes in which no three are in arithmetic progression. For another, it differs from many problems in combinatorics in that there is no consensus among mathematicians about what the right answer should be. Finally, the problem serves as a model for the many other problems involving ‘three-way interactions’. For instance, progress towards improved upper bounds for the cap set problem 30 , 31 immediately led to a series of other combinatorial results, for example, on the Erdös–Radio sunflower problem 32 .
The exact size of the largest possible cap set in n dimensions is known only for n ≤ 6. A brute force approach is not practical as the search space quickly becomes enormous with growing n , for example, around 3 1,600 for n = 8. Previous methods impose potentially suboptimal restrictions on the search space 33 , 34 . By contrast, we search the full space by means of an algorithm skeleton that uses a function ‘priority’ : \({{\mathbb{Z}}}_{3}^{n}\to {\mathbb{R}}\) . Intuitively, this function provides a priority with which each \(x\in {{\mathbb{Z}}}_{3}^{n}\) should be included in the cap set. Our algorithm starts with an empty set and iteratively adds the vector \(x\in {{\mathbb{Z}}}_{3}^{n}\) with the highest priority that does not violate the cap set constraint; Fig. 2a . Starting from a trivial constant function, we evolve the crucial ‘priority’ component of our approach to result in large cap sets.
Using this approach, we discovered cap sets of sizes shown in Fig. 4a . Notably, in dimension n = 8, FunSearch found a larger cap set than what was previously known, thus illustrating the power of FunSearch to discover new constructions. This also shows the scalability of FunSearch to larger dimensions, in which the previously best-known construction relied on a complex combination of cap sets in lower dimensions 33 , 34 . By contrast, FunSearch discovered a larger cap set from scratch, without having to be explicitly taught any way of combining cap sets. Moreover, we do not just discover the set of 512 eight-dimensional vectors in itself, but a program that generates it: we show this program in Fig. 4b . Through inspecting the code, we obtain a degree of understanding of what this set is: specifically, manual simplification of Fig. 4b provides the construction in Fig. 4c . Some properties of this construction are similar to the construction of the Hill cap 35 , 36 , which results in the optimal 112-cap in \({{\mathbb{Z}}}_{3}^{6}\) .
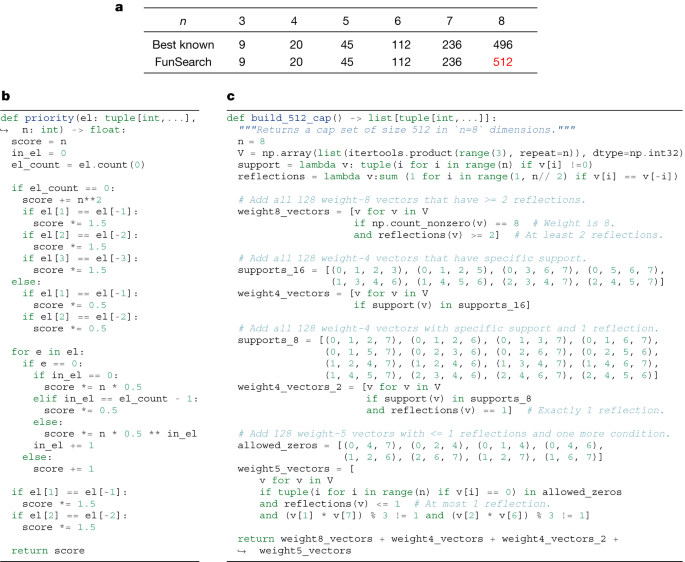
a , Size of the largest cap set in \({{\mathbb{Z}}}_{3}^{n}\) for different dimensions n . b , The function ‘priority’ : \({{\mathbb{Z}}}_{3}^{n}\to {\mathbb{R}}\) discovered by FunSearch that results in a cap set of size 512 in n = 8 dimensions. One feature to note is that the priority is affected by whether the same entry appears in positions i and − i ( − i denotes the i th position counting from the end). This motivates the notion of reflections, used in c . c , An explicit construction of this new 512-cap, which we were able to manually construct thanks to having discovered the cap set by searching in function space. See Supplementary Information Appendix E.2 for more details and for relation to Hill cap.
Admissible sets
Beyond finding the size of the largest cap set c n in dimension n , a fundamental problem in additive combinatorics 22 is determining the capacity \(C=\mathop{\sup }\limits_{n}\,{c}_{n}^{1/n}\) . The breakthrough result from ref. 31 established an upper bound of C ≤ 2.756. In this work, we are interested in lower bounds on C . To this end, we use the framework of constant weight admissible sets (or admissible sets for short) 34 , 37 , which has established the current state-of-the-art.
Formally, admissible sets \({\mathcal{A}}(n,w)\) are collections of vectors in {0, 1, 2} n satisfying two properties: (1) each vector has the same number w of non-zero elements but a unique support (therefore \(| A| \le \left(\begin{array}{c}n\\ w\end{array}\right)\) ); (2) for any three distinct vectors there is a coordinate in which their three respective values are {0, 1, 2}, {0, 0, 1} or {0, 0, 2}. Informally, an admissible set describes how to combine cap sets in smaller dimensions into large cap sets in higher dimensions 34 . We denote the set of full-size admissible sets (with \(| A| =\left(\begin{array}{c}n\\ w\end{array}\right)\) ) as \({\mathcal{I}}(n,w)\) . The current state-of-the-art 38 has relied on SAT solvers to construct large admissible sets.
As before, we evolve a function ‘priority’ : \({\{0,1,2\}}^{n}\to {\mathbb{R}}\) , which is used to iteratively grow admissible sets. Starting from a trivial constant function, we discover one that provides us with an \({\mathcal{I}}(12,7)\) admissible set; the discovered program is shown in Fig. 5b . This discovery alone already improves the lower bound on the cap set capacity from 2.2180 (ref. 38 ) to 2.2184. Yet, interpreting the program found by FunSearch (Fig. 5b ) helps us significantly push the boundaries of what admissible sets we can construct. Specifically, we notice that the discovered ‘priority’ function treats the n coordinates in a highly symmetric way, and indeed it turns out that the admissible set it constructs is preserved under independent cyclic permutations of coordinates within four disjoint groups of coordinate triples. Hereinafter we call such admissible sets symmetric (see Supplementary Information Appendix D for a formal definition).
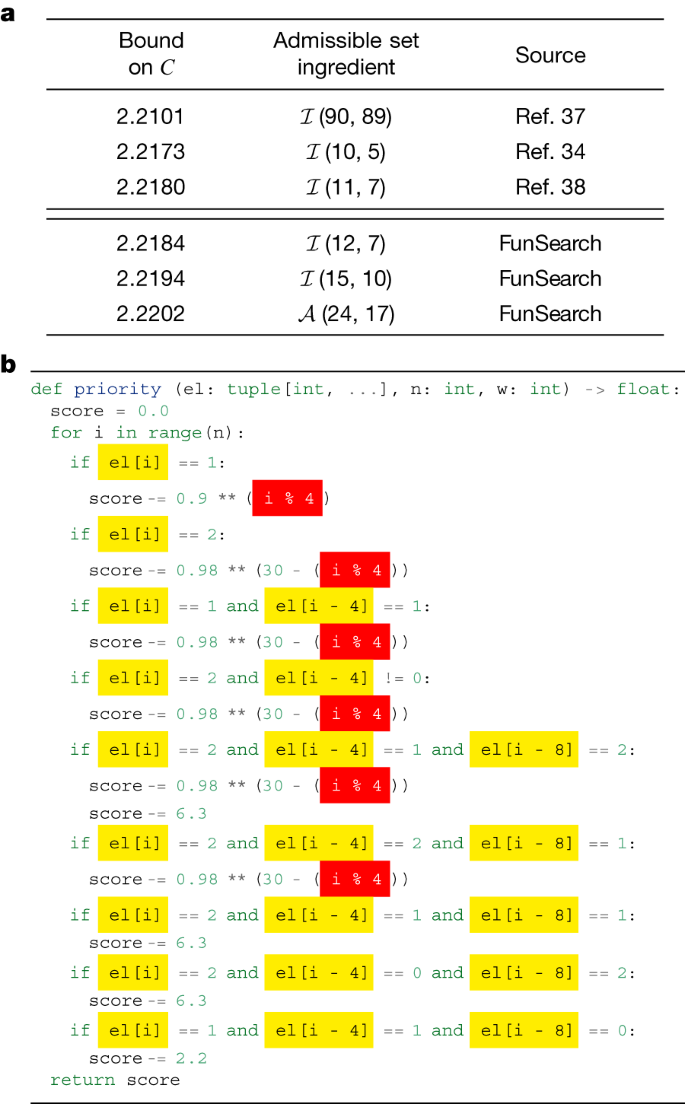
a , Summary of lower bounds on the cap set capacity C . b , The ‘priority’ function \({\{0,1,2\}}^{n}\to {\mathbb{R}}\) discovered by FunSearch that results in an \({\mathcal{I}}(12,7)\) admissible set. The source code shows that when n = 12, the function treats the four triples of coordinates {0, 4, 8}, {1, 5, 9}, {2, 6, 10} and {3, 7, 11} together. We then checked that the admissible set is in fact symmetric under independent cyclic permutations of coordinates within each of these four triples. See Supplementary Information Appendices D and E.3 for more details.
We now use FunSearch to directly search for symmetric admissible sets. Note that this is a more restricted and also much smaller search space, which allows for significantly higher dimensions and weights than were previously possible. This led us to discovering a full-size \({\mathcal{I}}(15,10)\) admissible set (indicating C ≥ 2.219486) and a partial admissible set in \({\mathcal{A}}(24,17)\) of size 237,984, which implies a new lower bound on the cap set capacity of 2.2202 (Fig. 5a ). Although this is a great improvement to the lower bound compared to research in the last 20 years, we note it is still far from the upper bound and we hope our results inspire future work on this problem.
Not only does FunSearch scale to much larger instances than traditional combinatorial solvers (Supplementary Information Appendix A.4 ), but it is also a unique feature of searching in function space that we were able to inspect the code discovered by FunSearch and infer a new insight into the problem, in the form of a new symmetry. The procedure we followed in this section is a concrete example of how LLM-based approaches can be used in mathematical sciences: FunSearch suggests a solution, which is examined by researchers, who may note features of interest. These features are used to refine the search, leading to better solutions. This process can be iterated, with both human and search consistently in the loop.
Bin packing
Combinatorial optimization is a subfield of mathematics that plays an important role across a wide range of areas, from theoretical computer science to practical problems in logistics and scheduling. Whereas many combinatorial optimization problems are provably hard to solve for large instances, it is typically possible to achieve strong performance using heuristics to guide the search algorithm. The choice of a heuristic is crucial for obtaining strong performance, but designing a good heuristic is difficult in practice. In this section, we show that FunSearch can be used to discover effective heuristics for one of the central problems in combinatorial optimization: bin packing 39 .
The goal of bin packing is to pack a set of items of various sizes into the smallest number of fixed-sized bins. Bin packing finds applications in many areas, from cutting materials to scheduling jobs on compute clusters. We focus on the online setting in which we pack an item as soon as it is received (as opposed to the offline setting in which we have access to all items in advance). Solving online bin packing problems then requires designing a heuristic for deciding which bin to assign an incoming item to.
Heuristics for online bin packing are well studied and several variants exist with strong worst case performance 40 , 41 , 42 , 43 , 44 , 45 . However, they often show poor performance in practice 39 . Instead, the most commonly used heuristics for bin packing are first fit and best fit. First fit places the incoming item in the first bin with enough available space, whereas best fit places the item in the bin with least available space where the item still fits. Here, we show that FunSearch discovers better heuristics than first fit and best fit on simulated data.
To achieve this, we define a heuristic as a program that takes as input an item and an array of bins (containing the remaining capacity of each bin) and returns a priority score for each bin. The ‘solve’ function picks the bin with the highest score according to the heuristic (Fig. 2b ). FunSearch is then used to evolve this heuristic, starting from best fit.
We first evaluate FunSearch on the well-known OR-Library bin packing benchmarks 23 , consisting of four datasets, OR1 to OR4, containing bin packing instances with an increasing number of items (see Supplementary Information Appendix E.4 for details). We evolve our heuristic on a training set of generated bin packing instances with the same number of items as those in OR1 and, after the evolutionary process is concluded, test it on the OR1 to OR4 datasets. We measure performance as the fraction of excess bins used over the L 2 lower bound 46 of the optimal offline packing solution (which is generally not achievable in the online setting).
As can be seen in Table 1 , FunSearch outperforms both first fit and best fit across all datasets. Further, the learned heuristic generalizes: even though it has only seen instances of the same size as OR1 during training, it generalizes across problem sizes, performing even better on large instances and widening the gap to best fit. In addition to the OR benchmarks, we also use FunSearch to evolve heuristics on bin packing instances sampled from a Weibull distribution, as these closely follow many real-world scheduling problems 24 , 47 (see Supplementary Information Appendix E.4 for details). As shown in Table 1 , the performance of FunSearch is very strong on this dataset, significantly outperforming first fit and best fit across instances, as well as scaling gracefully to large instances (being only 0.03% off the lower bound on the optimum for 100,000 items). In addition, FunSearch is robust and consistently outperforms these baselines as shown in the statistical analysis in the Supplementary Information Appendix A.3 .
We observed that several heuristics discovered by FunSearch use the same general strategy for bin packing (see Fig. 6 for an example). Instead of packing items into bins with the least capacity (such as best fit), the FunSearch heuristics assign items to least capacity bins only if the fit is very tight after placing the item. Otherwise, the item is typically placed in another bin, which would leave more space after the item is placed. This strategy avoids leaving small gaps in bins that are unlikely to ever be filled (see Supplementary Information Appendix E.5 for example visualizations of such packings).
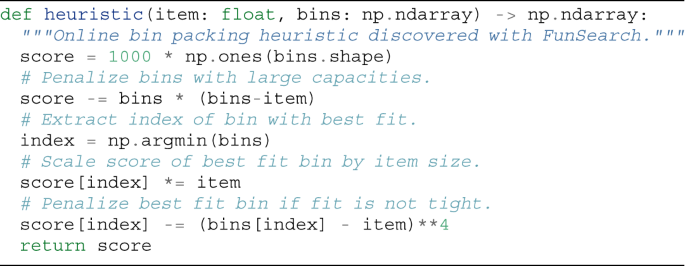
This example illustrates frequently observed behaviour: instead of always packing items into the best fit bin, the heuristic encourages packing the item only if the fit is tight. Comments in the code were manually added. See Supplementary Information Appendix C for more discovered heuristics.
As this example demonstrates, the benefits of FunSearch extend beyond theoretical and mathematical results to practical problems such as bin packing. Indeed, bin packing, and related combinatorial optimization problems, are ubiquitous and find applications across a range of industries. We are optimistic that FunSearch could be applied to several such use cases with potential for real-world impact.
The effectiveness of FunSearch in discovering new knowledge for hard problems might seem intriguing. We believe that the LLM used within FunSearch does not use much context about the problem; the LLM should instead be seen as a source of diverse (syntactically correct) programs with occasionally interesting ideas. When further constrained to operate on the crucial part of the algorithm with a program skeleton, the LLM provides suggestions that marginally improve over existing ones in the population, which ultimately results in discovering new knowledge on open problems when combined with the evolutionary algorithm. Another crucial component of the effectiveness of FunSearch is that it operates in the space of programs: rather than directly searching for constructions (which is typically an enormous list of numbers), FunSearch searches for programs generating those constructions. Because most problems we care about are structured (highly non-random), we believe that solutions are described more concisely with a computer program, compared to other representations. For example, the trivial representation of the admissible set \({\mathcal{A}}(24,17)\) consists of more than 200,000 vectors, but the program generating this set consists of only a few lines of code. Because FunSearch implicitly encourages concise programs, it scales to much larger instances compared to traditional search approaches in structured problems. In a loose sense, FunSearch attempts to find solutions that have low Kolmogorov complexity 48 , 49 , 50 (which is the length of the shortest computer program that produces a given object as output), whereas traditional search procedures have a very different inductive bias. We believe that such Kolmogorov-compressed inductive bias is key to FunSearch scaling up to the large instances in our use cases. In addition to scale, we have empirically observed that FunSearch outputs programs that tend to be interpretable: that is, they are clearly easier to read and understand compared to a list of numbers. For example, by scrutinizing FunSearch’s output for the admissible set problem, we found a new symmetry, which was then subsequently used to improve the results even further. Despite the rarity of symmetric solutions, we observe that FunSearch preferred symmetric ones, as these are more parsimonious (that is, they require less information to specify), in addition to the natural bias of LLMs (trained on human-produced code) in outputting code with similar traits to human code. This is in contrast to traditional genetic programming that does not have this bias (and in addition requires hand-tuning the mutation operators 51 ).
We note that FunSearch, at present, works best for problems having the following characteristics: (1) availability of an efficient evaluator; (2) a ‘rich’ scoring feedback quantifying the improvements (as opposed to a binary signal) and (3) ability to provide a skeleton with an isolated part to be evolved. For example, the problem of generating proofs for theorems 52 , 53 , 54 falls outside this scope, because it is unclear how to provide a rich enough scoring signal. By contrast, for MAX-SAT, the number of satisfied clauses can be used as a scoring signal. In this paper, we have explicitly striven for simplicity and we are confident that FunSearch can be further extended to improve its performance and be applicable to more classes of problems. In addition, the rapid development of LLMs is likely to result in samples of far superior quality at a fraction of the cost, making FunSearch more effective at tackling a broad range of problems. As a result, we foresee that automatically tailored algorithms will soon become common practice and deployed in real-world applications.
Implementation details of FunSearch
Distributed system.
We implement FunSearch as a distributed system that has three types of workers: a programs database, samplers and evaluators. The programs database stores the initial user-provided program, as well as all programs received from the evaluators. The samplers are in charge of performing the LLM inference step; to do so they repeatedly query the programs database for prompts. To achieve higher sampling throughput, samplers generate several samples from each prompt. The samples from the LLM (that is, the generated programs) are sent to the evaluators, which score programs by executing them on inputs of interest and assessing the outputs using ‘evaluate’. Programs that are correct are sent to the programs database to be stored. Each of the three FunSearch components is provided as both Python code and pseudocode (Supplementary Information Appendix F ).
Prompt building
When queried for a prompt, the programs database samples k programs to encourage the LLM to merge ideas from them (we typically set k = 2; Supplementary Information Appendix E.1 ). Programs are sorted according to their score in increasing order, starting from version 0 (‘v0’). Using these k programs, the prompt is built as explained next.
For the sake of clarity, we use here the problem specification from Fig. 2a to precisely describe the prompting mechanism. The overall structure of the prompt mimics the structure of the program skeleton, with the following differences: (1) the ‘priority’ function is stripped out and replaced with the k = 2 programs sampled, first ‘priority_v0’ and then ‘priority_v1’. (2) After that, a ‘priority_v2’ function with no body is appended: the LLM will be in charge of completing the body of that function. (3) All other functions that appear before ‘priority_v0’ are removed. See Extended Data Fig. 1 for an example of the structure of a prompt.
Evolutionary method and program selection
Another key feature of FunSearch is the method used for evolution of the population of programs from the programs database, as well as for program selection: that is, how the programs database samples programs when queried for a prompt. For this, we use the islands model, a parallel genetic algorithm 27 , 28 . Specifically, we split the population into m separate groups or islands. Each island is initialized with a copy of the user-provided initial program and is evolved separately. That is, whenever a prompt is required, we first uniformly sample an island and then sample k = 2 programs from that island to build the prompt. The programs generated from the LLM on the basis of that prompt will later be stored in the same island. Every 4 h, we discard all the programs from the m /2 islands whose best instances have the lowest score. Each of these islands is then seeded with a single program, obtained by first choosing one of the surviving m /2 islands uniformly at random and then retrieving the highest-scoring program from that island (breaking ties in favour of older programs). The evolutionary process is then restarted from this state, in which the reset islands contain one high-performing program each (Extended Data Fig. 2 ).
This method has several advantages. First, drawing the analogy in which an island corresponds to an experiment, this approach effectively allows us to run several smaller experiments in parallel instead of a single large experiment. This is beneficial because single experiments can get stuck in local minima, in which most programs in the population are not easily mutated and combined into stronger programs. The multiple island approach allows us to bypass this and effectively kill off such experiments to make space for new ones starting from more promising programs. Second, promising experiments are run for longer, as the islands that survive a reset are the ones with higher scores.
Within each island, we further cluster programs according to their signature. We define the signature of a program as the tuple containing the program’s scores on each of the inputs (for example, the cap set size for each input n ). Programs with the same signature are clustered together. When sampling a program within an island, we first sample an island’s cluster and then a program within that cluster (Extended Data Fig. 3 ). This approach, which aims to preserve diversity 55 , 56 , is related to Lexicase 57 in that both approaches consider a set of test cases for scoring an individual, and it is related to fitness uniform optimization 58 , which also clusters individuals on the basis of their fitness value; however, we sample the clusters on the basis of their score instead of uniformly, as detailed next.
When sampling a cluster, we favour those with larger score values. Specifically, let s i denote the score of the i th cluster, defined as an aggregation (for example, mean) of all the scores in the signature that characterizes that cluster. The probability P i of choosing cluster i is
where T cluster is the temperature parameter, n is the current number of programs in the island, and T 0 and N are hyperparameters (given in Supplementary Information Appendix E.1 ). This approach is sometimes referred to as the Boltzmann selection procedure 59 .
When sampling a program within a cluster, we favour shorter programs. In particular, let ℓ i denote the negative length of the i th program within the chosen cluster (measured as the number of characters), and let \({\widetilde{{\ell }}}_{i}=\frac{{{\ell }}_{i}-\mathop{\min }\limits_{{i}^{{\prime} }}{{\ell }}_{{i}^{{\prime} }}}{\mathop{\max }\limits_{{i}^{{\prime} }}{{\ell }}_{{i}^{{\prime} }}+1{0}^{-6}}\) . We set the probability of each program proportional to \(\exp ({\widetilde{{\ell }}}_{i}/{T}_{{\rm{program}}})\) , where T program is a temperature hyperparameter.
Owing to randomness in LLM sampling and in the evolutionary procedure, repeating an experiment can lead to different results. For some problems (for example, cap set through the admissible set problem and online bin packing) every single run of FunSearch surpasses the baseline, with only some variation in the magnitude of the difference. For example, all experiments on admissible sets improve on the previous best capacity lower bound, with 60% of experiments on \({\mathcal{I}}(12,7)\) finding a full-size admissible set. For other problems, many independent repetitions of an experiment may be necessary to improve on previous best results. In particular, the case of cap set by direct construction in n = 8 dimensions is particularly challenging, with only four out of 140 experiments discovering a cap set of size 512. See Supplementary Information Appendix A.3 for more details.
Related work
The rise of powerful LLMs such as that in ref. 60 has been followed by systems in which an LLM core has been enveloped by a ‘programmatic scaffold’ 61 , and several LLM calls were connected in some way to accomplish larger and more intricate tasks beyond what would be possible using a single prompt and the raw LLM, possibly by using external tools or external memory streams 62 , 63 , 64 , 65 , 66 . LLMs have also been paired with evaluators; for example, refs. 20 , 67 fine-tuned an LLM on data that had been previously generated by the LLM itself (respectively on puzzle problems and solutions, and on justifications and/or explanations for answers to questions), and they used an evaluator to assess the correctness of this data, ensuring that the fine-tuning dataset contained only correct solutions and/or explanations. More related to our approach is the use of LLMs as mutation operators on code, and ref. 3 was the first study to show that coupling an LLM with a programmatic way of scoring a solution can lead to a self-improvement loop. In refs. 16 , 17 , 18 , 19 , the LLM was used as a crossover operator rather than a mutation one, that is, the LLM prompts are composed of several functions, similarly to FunSearch. In refs. 3 , 16 , the task was to improve code that generated bidimensional virtual robots that could move as far as possible in a given simulated terrain (ref. 16 also considered the tasks of symbolic regression, natural language sentences and image generation). In refs. 17 , 18 , 19 the task was to find neural network architectures (described with Python code), and in ref. 68 the task was continuous exploration in the game of Minecraft. By contrast, in this paper, we tackle open problems in mathematics and algorithm design, and we surpass human-designed constructions. We achieve that by combining several ingredients: a distributed system with many samplers and evaluators that communicate asynchronously, a user-provided program specification and skeleton, as well as an evolutionary mechanism based on islands that preserves the diversity of programs. FunSearch achieves that using an off-the-shelf LLM without fine-tuning.
More broadly, LLMs have been used for program synthesis as one of its main applications 4 , 5 , 6 , 7 , 8 . There are many use cases being explored, such as automatically editing code to improve performance 13 , automatically debugging code 9 , 10 , generating code from natural language descriptions 69 , 70 , 71 and doing so to solve problems in code competitions 11 , 12 . Unlike the above approaches that provide tools to increase the productivity of software engineers, we combine in this paper the creativity of LLMs with the power of evolutionary procedures to push the boundaries of human knowledge through solving open hard problems. Another line of research uses LLMs to guide the search for formal proofs for automatic theorem proving 52 , 53 , 54 . Although this approach has the potential to eventually find new knowledge, the achievements of these methods still lag behind the frontier of human knowledge.
Genetic programming
Genetic programming is a subfield of computer science concerned with automatically generating or discovering computer programs using evolutionary methods 15 , 72 , 73 and is used for symbolic regression applications 74 , 75 and discovery of optimization algorithms 76 among others. In this broad sense, combining LLMs with evolution can be seen as an instance of genetic programming with the LLM acting as a mutation and crossover operator. However, using an LLM mitigates several issues in traditional genetic programming 51 , as shown in Supplementary Information Appendix A and discussed in ref. 3 . Indeed, genetic programming methods require defining several parameters, chief among them the set of allowed mutation operations (or primitives) 15 . Designing such a set of operations is non-trivial and problem specific, requiring domain knowledge about the problem at hand or its plausible solution 51 . Although research has been done to mitigate this limitation, through, for example, the reuse of subprograms 77 or modelling the distribution of high-performing programs 78 , designing effective and general code mutation operators remains difficult. By contrast, LLMs have been trained on vast amounts of code and as such have learned about common patterns and routines from human-designed code. The LLM can leverage this, as well as the context given in the prompt, to generate more effective suggestions than the random ones typically used in genetic programming.
Related to genetic programming, the field of hyper-heuristics 79 , 80 seeks to design learning methods for generating heuristics applied to combinatorial optimization problems. In practice, these heuristics are often programs discovered through genetic programming, typically by evolving a heuristic on a set of instances of a given combinatorial optimization problem, such as bin packing 81 . Indeed, like FunSearch, hyper-heuristics have also been applied to online bin packing, with the learned heuristics able to match the performance of first fit 82 and best fit 83 on a set of generated bin packing instances. Augmenting the heuristics with memory of previously seen items can even lead to heuristics outperforming best fit 84 . In addition, these evolved heuristics can sometimes generalize to larger instances than the ones they were trained on 85 , similar to the learned FunSearch heuristics. However, as is the case with genetic programming, one of the fundamental limitations of hyper-heuristics is that the components of the evolved heuristic must be manually defined by the user and often need to be tailored to a specific problem to be effective. The LLM in FunSearch allows us to bypass this limitation and learn heuristics for bin packing and job scheduling as well as discovering new mathematical constructions, all within a single pipeline without problem-specific tuning.
Program superoptimization and software engineering
Searching for the best way of modifying source code is a task that appears in several branches of computer science and software development. These occurrences can be broadly classified into two groups: first, in which the goal is to find semantic-preserving modifications (this arises in program optimization and superoptimization, in which the aim is to modify the program so that it executes faster while maintaining its input–output behaviour), and second, in which the goal is to find programs with different semantics (this arises, for example, in automatic program repair and mutation testing). With some exceptions discussed below, most of these areas use relatively simple and hard-coded mutation operators on either the source code directly (such as deleting or swapping lines) or on the abstract syntax tree.
Machine learning approaches have been used for program superoptimization. For example, ref. 86 used reinforcement learning to learn the sampling probabilities used within a hierarchical probabilistic model of simple program edits introduced by STOKE 87 . Neural networks have also been proposed as a mutation operator for program optimization in ref. 88 . These studies operated on code written in Assembly (perhaps because designing meaningful and rich edit distributions on programs in higher-level languages is challenging). More recently, ref. 13 used LLMs to find performance-improving edits to code written in C++ or Python. We also note that reinforcement learning has recently been applied to discover new faster algorithms for fundamental operations such as matrix multiplication 89 and sorting 90 .
In this paper, we have not explicitly explored semantic-preserving applications such as discovering performance-improving code edits, but we believe that FunSearch could be an effective method for that setting too. In both use cases presented in the main text, the goal is to evolve programs with new semantics, but the application is different from program repair or mutation testing: in the ‘Extremal combinatorics’ section, we used FunSearch to discover a program that constructs a previously unknown mathematical object, and in the ‘Bin packing’ section, we used FunSearch to discover a program that corresponds to a more efficient heuristic for online bin packing.
Data availability
The experiments carried out in this paper do not require any data corpus other than the publicly available OR-Library bin packing benchmarks 23 . The output functions of interest produced by FunSearch are shown across the main paper and in text files in the Supplementary Information .
Code availability
The discovered functions as well as the evolutionary algorithm, code manipulation routines and a single-threaded implementation of the FunSearch pipeline are available as Python code in the Supplementary Information and at https://github.com/google-deepmind/funsearch . Furthermore, the software library launchpad 91 and a sandbox for safely executing generated code on our internal distributed system were used. No training or fine-tuning of a LLM is required; API access for inference is sufficient. We used Codey 26 , which is available through its API, and StarCoder 6 , which is open source.
Bang, Y. et al. A multitask, multilingual, multimodal evaluation of ChatGPT on reasoning, hallucination, and interactivity. Preprint at https://arxiv.org/abs/2302.04023 (2023).
Borji, A. A. categorical archive of ChatGPT failures. Preprint at https://arxiv.org/abs/2302.03494 (2023).
Lehman, J. et al. in Handbook of Evolutionary Machine Learning (eds Banzhaf, W. et al.) 331–366 (Springer, 2023).
Chen, M. et al. Evaluating large language models trained on code. Preprint at https://arxiv.org/abs/2107.03374 (2021).
Austin, J. et al. Program synthesis with large language models. Preprint at https://arxiv.org/abs/2108.07732 (2021).
Li, R. et al. StarCoder: may the source be with you! Preprint at https://arxiv.org/abs/2305.06161 (2023).
Fried, D. et al. Incoder: a generative model for code infilling and synthesis. In Proc. International Conference on Learning Representations (2022).
Nijkamp, E. et al. CodeGen: an open large language model for code with multi-turn program synthesis. In Proc. International Conference on Learning Representations (2022).
Chen, X., Lin, M., Schärli, N. & Zhou, D. Teaching large language models to self-debug. Preprint at https://arxiv.org/abs/2304.05128 (2023).
Liventsev, V., Grishina, A., Härmä, A. & Moonen, L. Fully autonomous programming with large language models. Preprint at https://arxiv.org/abs/2304.10423 (2023).
Li, Y. et al. Competition-level code generation with alphacode. Science 378 , 1092–1097 (2022).
Article ADS CAS PubMed Google Scholar
Zelikman, E., Huang, Q., Poesia, G., Goodman, N. D. & Haber, N. Parsel: a (de-) compositional framework for algorithmic reasoning with language models. Preprint at https://arxiv.org/abs/2212.10561 (2023).
Madaan, A. et al. Learning performance-improving code edits. Preprint at https://arxiv.org/abs/2302.07867 (2023).
Goldberg, D. E. Genetic Algorithms in Search, Optimization and Machine Learning (Addison-Wesley, 1989).
Koza, J. R. Genetic programming as a means for programming computers by natural selection. Stat. Comput. 4 , 87–112 (1994).
Article Google Scholar
Meyerson, E. et al. Language model crossover: variation through few-shot prompting. Preprint at https://arxiv.org/abs/2302.12170 (2023).
Chen, A., Dohan, D. M. & So, D. R. EvoPrompting: language models for code-level neural architecture search. Preprint at https://arxiv.org/abs/2302.14838 (2023).
Zheng, M. et al. Can GPT-4 perform neural architecture search? Preprint at https://arxiv.org/abs/2304.10970 (2023).
Nasir, M. U., Earle, S., Togelius, J., James, S. & Cleghorn, C. LLMatic: neural architecture search via large language models and quality-diversity optimization. Preprint at https://arxiv.org/abs/2306.01102 (2023).
Haluptzok, P., Bowers, M. & Kalai, A. T. Language models can teach themselves to program better. In International Conference on Learning Representations (2023).
Grochow, J. New applications of the polynomial method: the cap set conjecture and beyond. Bull. Am. Math. Soc. 56 , 29–64 (2019).
Article ADS MathSciNet Google Scholar
Tao, T. & Vu, V. H. Additive Combinatorics Vol. 105 (Cambridge Univ. Press, 2006).
Beasley, J. E. OR-library: distributing test problems by electronic mail. J. Oper. Res. Soc. 41 , 1069–1072 (1990).
Castiñeiras, I., De Cauwer, M. & O’Sullivan, B. Weibull-based benchmarks for bin packing. In Proc. International Conference on Principles and Practice of Constraint Programming 207–222 (Springer, 2012).
Anil, R. et al. Palm 2 technical report. Preprint at https://arxiv.org/abs/2305.10403 (2023).
Code models overview. Vertex AI, Google Cloud https://cloud.google.com/vertex-ai/docs/generative-ai/code/code-models-overview (2023).
Tanese, R. Distributed Genetic Algorithms for Function Optimization. PhD thesis, Univ. Michigan (1989).
Cantú-Paz, E. A survey of parallel genetic algorithms. Calculateurs Paralleles, Reseaux et Systemes Repartis 10 , 141–171 (1998).
Google Scholar
Tao, T. Open question: best bounds for cap sets. WordPress Blog https://terrytao.wordpress.com/2007/02/23/open-question-best-bounds-for-cap-sets/ (2009).
Croot, E., Lev, V. F. & Pach, P. P. Progression-free sets in are exponentially small. Ann. Math. 185 , 331–337 (2017).
Ellenberg, J. S., Gijswijt, D. On large subsets of \({F}_{q}^{n}\) with no three-term arithmetic progression. Ann. Math. 185 , 339–343 (2017).
Naslund, E. & Sawin, W. Upper bounds for sunflower-free sets. Forum Math. Sigma 5 , e15 (2017).
Edel, Y. & Bierbrauer, J. Large caps in small spaces. Des. Codes Cryptogr. 23 , 197–212 (2001).
Article MathSciNet Google Scholar
Edel, Y. Extensions of generalized product caps. Des. Codes Cryptogr. 31 , 5–14 (2004).
Hill, R. On the largest size of cap in S 5,3 . Rend Lincei. Sci. Fis. Mat. Nat. 54 , 378–384 (1973).
MathSciNet Google Scholar
Cameron, P. J. & Van Lint, J. H. Designs, Graphs, Codes and Their Links Vol. 3 (Cambridge Univ. Press, 1991).
Calderbank, A. R. & Fishburn, P. C. Maximal three-independent subsets of {0, 1, 2} n . Des. Codes Cryptogr. 4 , 203–211 (1994).
Tyrrell, F. New lower bounds for cap sets. Discrete Analysis https://doi.org/10.19086/da.91076 (2023).
Coffman, E. G., Garey, M. R. & Johnson, D. S. in Algorithm Design for Computer System Design (eds Ausiello, G. et al.) 49–106 (Springer, 1984).
Lee, C. C. & Lee, D. T. A simple on-line bin-packing algorithm. J. ACM 32 , 562–572 (1985).
Ramanan, P., Brown, D. J., Lee, C.-C. & Lee, D.-T. On-line bin packing in linear time. J. Algorithm. 10 , 305–326 (1989).
Seiden, S. S. On the online bin packing problem. J. ACM 49 , 640–671 (2002).
Balogh, J., Békési, J., Dósa, G., Sgall, J. & Stee, R. V. The optimal absolute ratio for online bin packing. In Proc. Twenty-Sixth Annual ACM-SIAM Symposium on Discrete Algorithms , SIAM (ed. Chekuri, C.) 1425–1438 (SIAM, 2014).
Balogh, J., Békési, J., Dósa, G., Epstein, L. & Levin, A. A new and improved algorithm for online bin packing. In Proc. 26th Annual European Symposium on Algorithms (ESA 2018) 5:1–5:14 (Schloss Dagstuhl–Leibniz-Zentrum fuer Informatik, 2018).
Coffman, E. G., Csirik, J., Galambos, G., Martello, S. & Vigo, D. in Handbook of Combinatorial Optimization (eds Pardalos, P. M. et al.) 455–531 (Springer, 2013).
Martello, S. & Toth, P. Lower bounds and reduction procedures for the bin packing problem. Discrete Appl. Math. 28 , 59–70 (1990).
Angelopoulos, S., Kamali, S. & Shadkami, K. Online bin packing with predictions. J. Artif. Intell. Res. 36 , 4574–4580 (2022).
Chaitin, G. J. On the length of programs for computing finite binary sequences. J. ACM 13 , 547–569 (1966).
Li, M. et al. An Introduction to Kolmogorov Complexity and its Applications Vol. 3 (Springer, 2008).
Solomonoff, R. J. A formal theory of inductive inference. Part I. Inf. Control 7 , 1–22 (1964).
O’Neill, M., Vanneschi, L., Gustafson, S. & Banzhaf, W. Open issues in genetic programming. Genet. Program. Evolvable Mach. 11 , 339–363 (2010).
Polu, S. & Sutskever, I. Generative language modeling for automated theorem proving. Preprint at https://arxiv.org/abs/2009.03393 (2020).
Polu, S. et al. Formal mathematics statement curriculum learning. In International Conference on Learning Representations (2023).
Jiang, A. Q. et al. THOR: wielding hammers to integrate language models and automated theorem provers. Adv. Neural Info. Process. Syst. 35 , 8360–8373 (2022).
Mouret, J.-B. & Doncieux, S. Overcoming the bootstrap problem in evolutionary robotics using behavioral diversity. In Proc. 2009 IEEE Congress on Evolutionary Computation 1161–1168 (IEEE, 2009).
Pugh, J. K., Soros, L. B. & Stanley, K. O. Quality diversity: a new frontier for evolutionary computation. Front. Robotics AI 3 , 40 (2016).
Helmuth, T., Spector, L. & Matheson, J. Solving uncompromising problems with lexicase selection. IEEE Trans. Evol. Comput. 19 , 630–643 (2015).
Hutter, M. & Legg, S. Fitness uniform optimization. IEEE Trans. Evol. Comput. 10 , 568–589 (2006).
de la Maza, M. An analysis of selection procedures with particular attention paid to proportional and Boltzmann selection. In Proc. Fifth International Conference on Genetic Algorithms (Morgan Kaufmann, 1993).
OpenAI, GPT-4 technical report. Preprint at https://arxiv.org/abs/2303.08774 (2023).
Millidge, B. Scaffolded LLMs as natural language computers. Beren’s Blog https://www.beren.io/2023-04-11-Scaffolded-LLMs-natural-language-computers (2023).
Schick, T. et al. Toolformer: language models can teach themselves to use tools. Preprint at https://arxiv.org/abs/2302.04761 (2023).
Park, J. S. et al. Generative agents: interactive simulacra of human behavior. In Proc. 36th Annual ACM Symposium on User Interface Software and Technology 1–22 (ACM, 2023).
Wu, J. et al. Recursively summarizing books with human feedback. Preprint at https://arxiv.org/abs/2109.10862 (2021).
Nye, M. et al. Show your work: scratchpads for intermediate computation with language models. In Deep Learning for Code Workshop, International Conference on Learning Representations (2022).
Yao, S. et al. ReAct: dynergizing reasoning and acting in language models. In Proc. International Conference on Learning Representations (2023).
Zelikman, E., Wu, Y., Mu, J. & Goodman, N. Star: bootstrapping reasoning with reasoning. Adv. Neural Info. Process. Syst. 35 , 15476–15488 (2022).
Wang, G. et al. Voyager: an open-ended embodied agent with large language models. Preprint at https://arxiv.org/abs/2305.16291 (2023).
Yin, P. et al. Natural language to code generation in interactive data science notebooks. Preprint at https://arxiv.org/abs/2212.09248 (2022).
Ni, A. et al. Lever: learning to verify language-to-code generation with execution. In Proc. International Conference on Machine Learning 26106–26128 (PMLR, 2023).
Zhou, S., Alon, U., Xu, F. F., Jiang, Z. & Neubig, G. Docprompting: generating code by retrieving the docs. In Proc. International Conference on Learning Representations (2022).
Banzhaf, W., Nordin, P., Keller, R. E. & Francone, F. D. Genetic Programming: An Introduction: On The Automatic Evolution of Computer Programs and its Applications (Morgan Kaufmann, 1998).
Langdon, W. B. & Poli, R. Foundations of Genetic Programming (Springer Science & Business Media, 2013).
Ma, H., Narayanaswamy, A., Riley, P. & Li, L. Evolving symbolic density functionals. Sci. Adv. 8 , eabq0279 (2022).
Article ADS PubMed PubMed Central Google Scholar
Schmidt, M. & Lipson, H. Distilling free-form natural laws from experimental data. Science 324 , 81–85 (2009).
Chen, X. et al. Symbolic discovery of optimization algorithms. Preprint at https://arxiv.org/abs/2302.06675 (2023).
Koza, J. R. Genetic Programming II: Automatic Discovery of Reusable Programs (MIT, 1994).
Salustowicz, R. & Schmidhuber, J. Probabilistic incremental program evolution. Evol. Comput. 5 , 123–141 (1997).
Article CAS PubMed Google Scholar
Burke, E. et al. in Handbook of Metaheuristics (eds Glover, F. & Kochenberger, G. A.) 457–474 (Springer, 2003).
Ross, P. in Search Methodologies: Introductory Tutorials in Optimization and Decision Support Techniques (eds Burke, E. K. & Kendall, G.) 529–556 (Springer, 2005).
Burke, E. K. et al. Hyper-heuristics: a survey of the state of the art. J. Oper. Res. Soc. 64 , 1695–1724 (2013).
Burke, E. K., Hyde, M. R. & Kendall, G. Evolving bin packing heuristics with genetic programming. In Proc. International Conference on Parallel Problem Solving from Nature 860–869 (Springer, 2006).
Burke, E. K., Hyde, M. R., Kendall, G. & Woodward, J. Automatic heuristic generation with genetic programming: evolving a jack-of-all-trades or a master of one. In Proc. 9th Annual Conference on Genetic and Evolutionary Computation 1559–1565 (ACM, 2007).
Burke, E. K., Hyde, M. R. & Kendall, G. Providing a memory mechanism to enhance the evolutionary design of heuristics. In Proc. IEEE Congress on Evolutionary Computation 1–8 (IEEE, 2010).
Burke, E. K., Hyde, M., Kendall, G. & Woodward, J. R. The scalability of evolved on line bin packing heuristics. In Proc. 2007 IEEE Congress on Evolutionary Computation 2530–2537 (IEEE, 2007).
Bunel, R., Desmaison, A., Kohli, P., Torr, P. H. & Kumar, M. P. Learning to superoptimize programs. In Proc. International Conference on Learning Representations (2017).
Schkufza, E., Sharma, R. & Aiken, A. Stochastic superoptimization. ACM SIGARCH Comp. Archit. News 41 , 305–316 (2013).
Shypula, A. et al. Learning to superoptimize real-world programs. In Proc. Deep Learning for Code Workshop (ICLR 2022 Workshop) (2022).
Fawzi, A. et al. Discovering faster matrix multiplication algorithms with reinforcement learning. Nature 610 , 47–53 (2022).
Article ADS CAS PubMed PubMed Central Google Scholar
Mankowitz, D. J. et al. Faster sorting algorithms discovered using deep reinforcement learning. Nature 618 , 257–263 (2023).
Yang, F. et al. Launchpad: a programming model for distributed machine learning research. Preprint at https://arxiv.org/abs/2106.04516 (2021).
Download references
Acknowledgements
We thank R. Anil, V. Feinberg, E. Taropa, T. Hubert, J. Schrittwieser and S. Nowozin for their LLM support; T. Schaul, C. Fernando, A. Barreto and P. Gupta for discussions on evolutionary algorithms; M. Figurnov and T. Cemgil for reviewing the paper; F. Piccinini and S. Kenjeyev for their support on job scheduling; S. Blackwell for technical support; O. Ronneberger, F. Gimeno, B. Huergo, A. Mehrabian and A. Anand for useful advice and G. Holland for program management support.
Author information
These authors contributed equally: Bernardino Romera-Paredes, Mohammadamin Barekatain, Alexander Novikov, Matej Balog, M. Pawan Kumar, Emilien Dupont, Francisco J. R. Ruiz, Alhussein Fawzi
Authors and Affiliations
Google DeepMind, London, UK
Bernardino Romera-Paredes, Mohammadamin Barekatain, Alexander Novikov, Matej Balog, M. Pawan Kumar, Emilien Dupont, Francisco J. R. Ruiz, Pengming Wang, Pushmeet Kohli & Alhussein Fawzi
Department of Mathematics, University of Wisconsin-Madison, Madison, WI, USA
Jordan S. Ellenberg
Laboratoire de l’Informatique du Parallélisme, University of Lyon (Inria, ENS Lyon, UCBL, LIP), Lyon, France
You can also search for this author in PubMed Google Scholar
Contributions
B.R.-P. conceived the project with help from A.F. and P.K. A.F. scoped problems and developed project vision. B.R.-P. and A.N. developed the initial FunSearch codebase. A.N., B.R.-P., M. Balog, F.J.R.R., M. Barekatain, E.D. and A.F. implemented and refined the different components of the system. M. Barekatain and A.N. imported and experimented with LLMs. M. Barekatain, A.N. and M. Balog worked on evaluating, debugging and improving the efficiency of experiments. M. Balog, M. Barekatain, B.R.-P., A.N., A.F., O.F. and J.S.E. contributed to the cap set problem. M.P.K., M. Balog and J.S.E. researched and analysed results from the admissible sets problem. E.D., M. Barekatain and P.W. contributed to the online bin packing problem. F.J.R.R. and O.F. researched and did experiments on other problems (Shannon capacity and corners problems), P.K. contributed technical advice and ideas. A.F., B.R.-P., E.D., F.J.R.R., M.P.K., M. Balog, A.N., J.S.E. and M. Barekatain wrote the paper.
Corresponding authors
Correspondence to Bernardino Romera-Paredes , Pushmeet Kohli or Alhussein Fawzi .
Ethics declarations
Competing interests.
The authors of the paper are planning to file a patent application relating to subject matter contained in this paper in the name of Google DeepMind.
Peer review
Peer review information.
Nature thanks Josh Grochow, Andrea Lodi, Jean-Baptiste Mouret, Talia Ringer and Tao Yu for their contribution to the peer review of this work.
Additional information
Publisher’s note Springer Nature remains neutral with regard to jurisdictional claims in published maps and institutional affiliations.
Extended data figures and tables
Extended data fig. 1 example of best-shot prompting, based on the skeleton from fig. 2a ..
The prompt includes k = 2 implementations sampled from the programs database, with higher-scoring implementations being more likely to be included.
Extended Data Fig. 2 Evolutionary method.
The initial programs are separated into islands and each of them is evolved separately. After a number of iterations, the islands with the worst score are wiped and the best program from the islands with the best score are placed in the empty islands. Evolution then proceeds separately again until the next reset. This process is repeated until termination.
Extended Data Fig. 3 Program clusters within islands.
Within each island, programs are grouped into clusters based on their signature (i.e., their scores on several inputs). We first sample clusters, favoring the ones with higher score. Within the chosen clusters, we sample a program, favoring shorter programs. The sampled programs are used to prompt the LLM which generates a new program. If the new program is correct, it is added to the island, either in an existing cluster or a new one if its signature was not yet present.
Supplementary information
Supplementary information.
Further details about the method and extra results.
Supplementary Data 1
This zipped code file contains: (a) the evolutionary algorithm, code manipulation routines and a single-threaded implementation of the FunSearch pipeline; and (b) output functions of interest produced by FunSearch.
Rights and permissions
Open Access This article is licensed under a Creative Commons Attribution 4.0 International License, which permits use, sharing, adaptation, distribution and reproduction in any medium or format, as long as you give appropriate credit to the original author(s) and the source, provide a link to the Creative Commons licence, and indicate if changes were made. The images or other third party material in this article are included in the article’s Creative Commons licence, unless indicated otherwise in a credit line to the material. If material is not included in the article’s Creative Commons licence and your intended use is not permitted by statutory regulation or exceeds the permitted use, you will need to obtain permission directly from the copyright holder. To view a copy of this licence, visit http://creativecommons.org/licenses/by/4.0/ .
Reprints and permissions
About this article
Cite this article.
Romera-Paredes, B., Barekatain, M., Novikov, A. et al. Mathematical discoveries from program search with large language models. Nature 625 , 468–475 (2024). https://doi.org/10.1038/s41586-023-06924-6
Download citation
Received : 12 August 2023
Accepted : 30 November 2023
Published : 14 December 2023
Issue Date : 18 January 2024
DOI : https://doi.org/10.1038/s41586-023-06924-6
Share this article
Anyone you share the following link with will be able to read this content:
Sorry, a shareable link is not currently available for this article.
Provided by the Springer Nature SharedIt content-sharing initiative
This article is cited by
Large language models help computer programs to evolve.
- Jean-Baptiste Mouret
Nature (2024)
Automated discovery of algorithms from data
- Paul J. Blazek
- Kesavan Venkatesh
- Milo M. Lin
Nature Computational Science (2024)
Automated quantum software engineering
- Aritra Sarkar
Automated Software Engineering (2024)
By submitting a comment you agree to abide by our Terms and Community Guidelines . If you find something abusive or that does not comply with our terms or guidelines please flag it as inappropriate.
Quick links
- Explore articles by subject
- Guide to authors
- Editorial policies
Sign up for the Nature Briefing: AI and Robotics newsletter — what matters in AI and robotics research, free to your inbox weekly.

Fujitsu uses Fugaku supercomputer to train LLM: 13 billion parameters
A lthough Fujitsu's Fugaku supercomputer is no longer the world's fastest machine from the Top 500 supercomputer list, it still is a very capable system and the versatility of the A64FX processor allows to use it for a variety of workloads, such as AI. This week Fujitsu released its Fugaku-LLM, a large language model with advanced Japanese language processing capabilities that is designed for both research and commercial applications.
Fujitsu's Fugaku-LLM was trained using 380 billion tokens on 13,824 nodes of the Fugaku supercomputer based on the A64FX processor that supports FP64, FP32, FP16 and INT8 modes for a variety of AI and conventional supercomputer applications. The training of Fugaku-LLM naturally took advantage of distributed parallel learning techniques optimized for the supercomputer's architecture and the Tofu interconnect D.
The Fugaku-LLM features 13 billion parameters, which looks pale compared to GPT-4's 175 billion, which is the largest LLM ever trained in Japan. Fujitsu says that its 13 billion parameter LLM does not require vast compute resources to inference, which will be optimal for businesses and researchers in Japan. Approximately 60% of the training data was in Japanese and 40% of the data was data in English, mathematics, and code.
This extensive Japanese-centric training sets it apart from other Japanese models that were trained primarily on English datasets. As a result, Fugaku-LLM boasts superior proficiency in Japanese, achieving an average score of 5.5 on the Japanese MT-Bench, the top score among openly available models trained with original data from Japan. It particularly excels in humanities and social sciences, achieving an impressive benchmark score of 9.18, according to Fujitsu.
The Fugaku-LLM initiative has been driven by collaborations among leading Japanese institutions including Tokyo Institute of Technology, Tohoku University, Fujitsu Limited, RIKEN, Nagoya University, CyberAgent, and Kotoba Technologies. One of the reasons they collaborated was a shortage of GPUs typically used to train and inference AI models. Another reason is that the model could be used with Fujitsu's next-generation 150-core Monaka datacenter CPU optimized for both AI and HPC workloads.
Fugaku-LLM is now available for both academic and commercial purposes under specified licensing terms from GitHub and Hugging Face (though Fujitsu did not provide any links). Additionally, it will also be offered via the Fujitsu Research Portal from May 10, 2024.
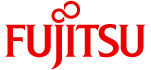
- Press Releases
Release of “Fugaku-LLM” – a large language model trained on the supercomputer “Fugaku”

Enhanced Japanese language ability, for use in research and business
Tokyo institute of technology, tohoku university, fujitsu limited, riken, nagoya university, cyberagent inc., kotoba technologies inc..
Kawasaki, May 10, 2024
- Large language model with enhanced Japanese language ability was developed using Japanese supercomputing technology
- Distributed parallel learning by maximizing the performance of the supercomputer “Fugaku”
- Commercial use is permitted, which will lead to innovative research and business applications such as AI for Science
A team of researchers in Japan released Fugaku-LLM, a large language model ( 1 ) with enhanced Japanese language capability, using the RIKEN supercomputer Fugaku. The team is led by Professor Rio Yokota of Tokyo Institute of Technology, Associate Professor Keisuke Sakaguchi of Tohoku University, Koichi Shirahata of Fujitsu Limited, Team Leader Mohamed Wahib of RIKEN, Associate Professor Koji Nishiguchi of Nagoya University, Shota Sasaki of CyberAgent, Inc, and Noriyuki Kojima of Kotoba Technologies Inc.
To train large language models on Fugaku, the researchers developed distributed training methods, including porting the deep learning framework Megatron-DeepSpeed to Fugaku in order to optimize the performance of Transformers on Fugaku. They accelerated the dense matrix multiplication library for Transformers, and optimized communication performance for Fugaku by combining three types of parallelization techniques and accelerated the collective communication library on the Tofu interconnect D.
Fugaku-LLM has 13 billion parameters ( 2 ) and is larger than the 7-billion-parameter models that have been developed widely in Japan. Fugaku-LLM has enhanced Japanese capabilities, with an average score of 5.5 on the Japanese MT-Bench ( 3 ) , the highest performance among open models that are trained using original data produced in Japan. In particular, the benchmark performance for humanities and social sciences tasks reached a remarkably high score of 9.18.
Fugaku-LLM was trained on proprietary Japanese data collected by CyberAgent, along with English data, and other data. The source code of Fugaku-LLM is available on GitHub ( 4 ) and the model is available on Hugging Face ( 5 ) . Fugaku-LLM can be used for research and commercial purposes as long as users comply with the license.
In the future, as more researchers and engineers participate in improving the models and their applications, the efficiency of training will be improved, leading to next-generation innovative research and business applications, such as the linkage of scientific simulation and generative AI, and social simulation of virtual communities with thousands of AIs.
In recent years, the development of large language models (LLMs) has been active, especially in the United States. In particular, the rapid spread of ChatGPT ( 6 ) , developed by OpenAI, has profoundly impacted research and development, economic systems, and national security. Countries other than the U.S. are also investing enormous human and computational resources to develop LLMs in their own countries. Japan, too, needs to secure computational resources for AI research so as not to fall behind in this global race. There are high expectations for Fugaku, the flagship supercomputer system in Japan, and it is necessary to improve the computational environment for large-scale distributed training on Fugaku to meet these expectations.
Therefore, Tokyo Institute of Technology, Tohoku University, Fujitsu, RIKEN, Nagoya University, CyberAgent, and Kotoba Technologies have started a joint research project on the development of large language models.
Role of each institution/company
Tokyo Institute of Technology: General oversight, parallelization and communication acceleration of large language models (optimization of communication performance by combining three types of parallelization, acceleration of collective communication on the Tofu interconnect D)
Tohoku University: Collection of training data and model selection
Fujitsu: Acceleration of computation and communication (acceleration of collective communication on Tofu interconnect D, performance optimization of pipeline parallelization) and implementation of pre-training and fine-tuning after training
RIKEN: Distributed parallelization and communication acceleration of large-scale language models (acceleration of collective communication on Tofu interconnect D)
Nagoya University: Study on application methods of Fugaku-LLM to 3D generative AI
CyberAgent: Provision of training data
Kotoba Technologies: Porting of deep learning framework to Fugaku
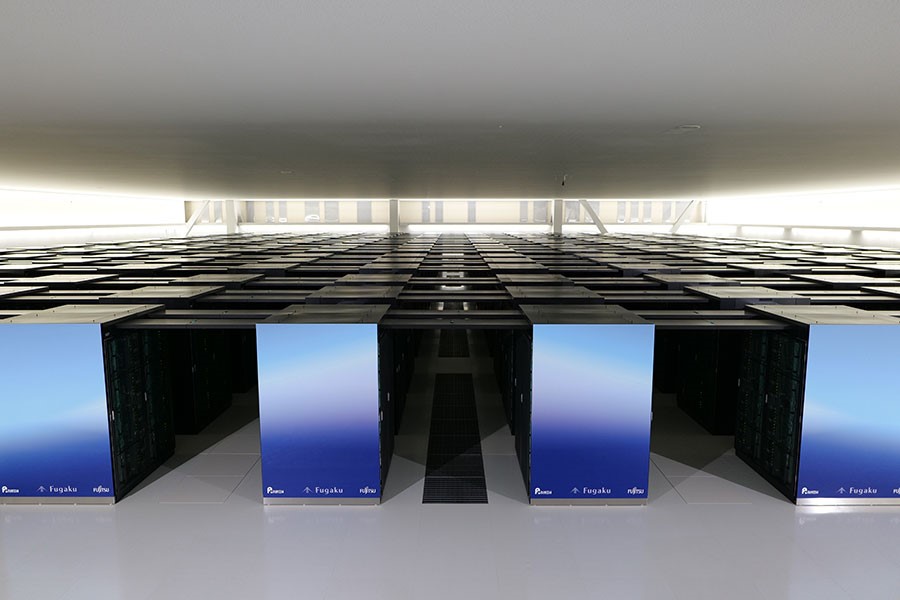
Research outcome
1. significantly improved the computational performance of training large language models on the supercomputer fugaku.
GPUs ( 7 ) are the common choice of hardware for training large language models. However, there is a global shortage of GPUs due to the large investment from many countries to train LLMs. Under such circumstances, it is important to show that large language models can be trained using Fugaku, which uses CPUs instead of GPUs. The CPUs used in Fugaku are Japanese CPUs manufactured by Fujitsu, and play an important role in terms of revitalizing Japanese semiconductor technology.
By extracting the full potential of Fugaku, this study succeeded in increasing the computation speed of the matrix multiplication by a factor of 6, and the communication speed by a factor of 3. To maximize the distributed training performance on Fugaku, the deep learning framework Megatron-DeepSpeed was ported to Fugaku, and the dense matrix multiplication library was accelerated for Transformer. For communication acceleration, the researchers optimized communication performance for Fugaku by combining three types of parallelization techniques and accelerated the collective communication on the Tofu interconnect D. The knowledge gained from these efforts can be utilized in the design of the next-generation computing infrastructure after Fugaku and will greatly enhance Japan's future advantage in the field of AI.
2. An easy-to-use, open, and secure, large language model with 13 billion parameters
In 2023, many large language models were developed by Japanese companies, but most of them have less than 7 billion parameters. Since the performance of large-scale language models generally improves as the number of parameters increases, the 13-billion-parameter model the research team developed is likely to be more powerful than other Japanese models. Although larger models have been developed outside of Japan, large language models also require large computational resources, making it difficult to use models with too many parameters. Fugaku-LLM is both high performance and well-balanced.
In addition, most models developed by Japanese companies employ continual learning ( 8 ) , in which open models developed outside of Japan are continually trained on Japanese data. In contrast, Fugaku-LLM is trained from scratch using the team’s own data, so the entire learning process can be understood, which is superior in terms of transparency and safety.
Fugaku-LLM was trained on 380 billion tokens using 13,824 nodes of Fugaku, with about 60% of the training data being Japanese, combined with English, mathematics, and code. Compared to models that continually train on Japanese, Fugaku-LLM learned much of its information in Japanese. Fugaku-LLM is the best model among open models that are produced in Japan and trained with original data. In particular, it was confirmed that the model shows a high benchmark score of 9.18 in the humanities and social sciences tasks. It is expected that the model will be able to perform natural dialogue based on keigo (honorific speech) and other features of the Japanese language.
Future Development
The results from this research are being made public through GitHub and Hugging Face so that other researchers and engineers can use them to further develop large language models. Fugaku-LLM can be used for research and commercial purposes as long as users comply with the license. Fugaku-LLM will be also offered to users via the Fujitsu Research Portal from May 10th, 2024.
Acknowledgement
This research was supported by the Fugaku policy-supporting proposal "Development of Distributed Parallel Training for Large Language Models Using Fugaku" (proposal number: hp230254).
- [1] Large language model : Models the probability with which text appears and can predict the text (response) that follows a given context (query).
- [2] Parameter : A measure of the size of a neural network. The more parameters, the higher the performance of the model, but the more data is required for training.
- [3] Japanese MT-Bench : Benchmark test provided by Stability AI
- [4] GitHub : Platform used to publish open source software
- [5] Hugging Face : Platforms used to publish AI datasets
- [6] ChatGPT : A large language model developed by OpenAI, which has brought about a major social change, surpassing 100 million users in about two months after its release.
- [7] GPU : Originally produced as an accelerator for graphics, but has recently been used to accelerate deep learning
- [8] Continual learning : A method for performing additional training on a large language model that has already been trained. Used for training language models in different languages or domains.
About Fujitsu
Fujitsu’s purpose is to make the world more sustainable by building trust in society through innovation. As the digital transformation partner of choice for customers in over 100 countries, our 124,000 employees work to resolve some of the greatest challenges facing humanity. Our range of services and solutions draw on five key technologies: Computing, Networks, AI, Data & Security, and Converging Technologies, which we bring together to deliver sustainability transformation. Fujitsu Limited (TSE:6702) reported consolidated revenues of 3.7 trillion yen (US$26 billion) for the fiscal year ended March 31, 2024 and remains the top digital services company in Japan by market share. Find out more: www.fujitsu.com .
Press Contacts
Fujitsu Limited Public and Investor Relations Division Inquiries
All company or product names mentioned herein are trademarks or registered trademarks of their respective owners. Information provided in this press release is accurate at time of publication and is subject to change without advance notice.
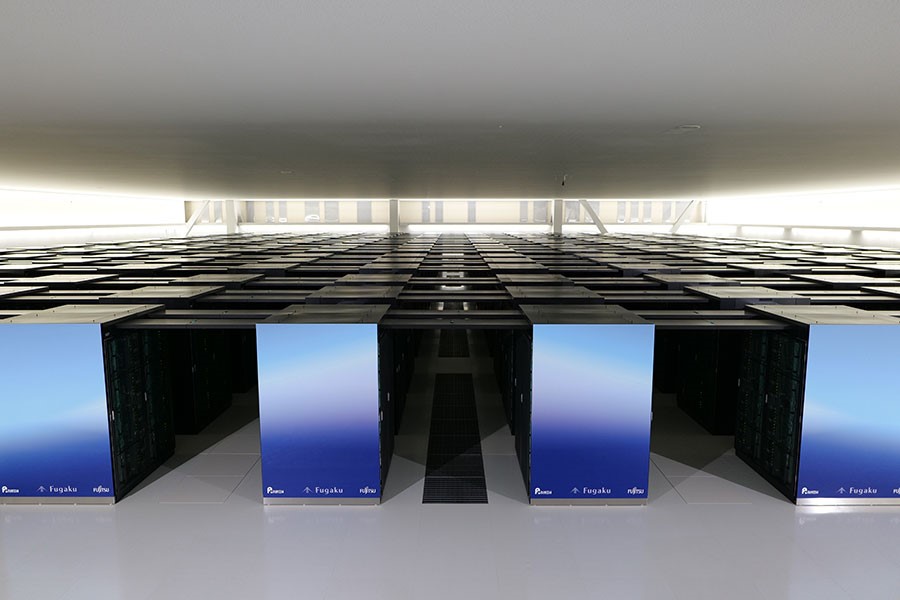
Help | Advanced Search
Computer Science > Computation and Language
Title: when to retrieve: teaching llms to utilize information retrieval effectively.
Abstract: In this paper, we demonstrate how Large Language Models (LLMs) can effectively learn to use an off-the-shelf information retrieval (IR) system specifically when additional context is required to answer a given question. Given the performance of IR systems, the optimal strategy for question answering does not always entail external information retrieval; rather, it often involves leveraging the parametric memory of the LLM itself. Prior research has identified this phenomenon in the PopQA dataset, wherein the most popular questions are effectively addressed using the LLM's parametric memory, while less popular ones require IR system usage. Following this, we propose a tailored training approach for LLMs, leveraging existing open-domain question answering datasets. Here, LLMs are trained to generate a special token, <RET>, when they do not know the answer to a question. Our evaluation of the Adaptive Retrieval LLM (Adapt-LLM) on the PopQA dataset showcases improvements over the same LLM under three configurations: (i) retrieving information for all the questions, (ii) using always the parametric memory of the LLM, and (iii) using a popularity threshold to decide when to use a retriever. Through our analysis, we demonstrate that Adapt-LLM is able to generate the <RET> token when it determines that it does not know how to answer a question, indicating the need for IR, while it achieves notably high accuracy levels when it chooses to rely only on its parametric memory.
Submission history
Access paper:.
- HTML (experimental)
- Other Formats

References & Citations
- Google Scholar
- Semantic Scholar
BibTeX formatted citation

Bibliographic and Citation Tools
Code, data and media associated with this article, recommenders and search tools.
- Institution
arXivLabs: experimental projects with community collaborators
arXivLabs is a framework that allows collaborators to develop and share new arXiv features directly on our website.
Both individuals and organizations that work with arXivLabs have embraced and accepted our values of openness, community, excellence, and user data privacy. arXiv is committed to these values and only works with partners that adhere to them.
Have an idea for a project that will add value for arXiv's community? Learn more about arXivLabs .
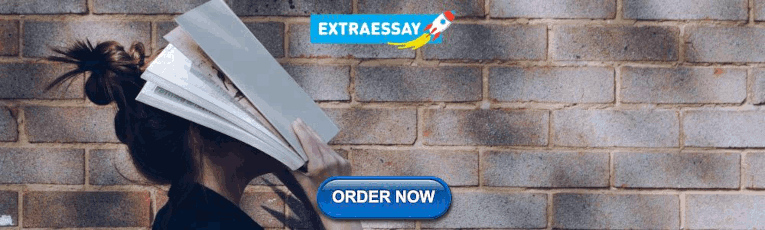
IMAGES
VIDEO
COMMENTS
Osgoode's Research LLM is a full-time, research-intensive program that is ideal for students who want to pursue a specific area of legal study in depth, including those who are considering a PhD. Students conduct their research under the supervision of an Osgoode faculty member. The Research LLM does not qualify students to practise law in ...
The LL.M. (Master of Laws) program is a one-year degree program that typically includes 180 students from some 65 countries. The Graduate Program is interested in attracting intellectually curious and thoughtful candidates from a variety of legal systems and backgrounds and with various career plans. Harvard's LL.M. students include lawyers working in firms, government officials, […]
LLM research, it has become considerably challenging to perceive. the bigger picture of the advances in this direction. Considering. the rapidly emerging plethora of literature on LLMs, it is.
LLM Thesis Option. LLM students have the option to write a substantial research paper, in conjunction with a seminar or Directed Research that may be recorded as a "thesis" on their transcript. At the onset of the seminar or Directed Research, the student must obtain approval from the professor that the paper will be completed for a "thesis ...
Research profile. The Edinburgh Law School is a vibrant, collegial and enriching community of legal, sociolegal and criminology researchers and offers an excellent setting for doctoral research. We are ranked 3rd in the UK for law for the quality and breadth of our research by Research Professional, based on the 2021 Research Excellence ...
Large language models (LLMs) are dramatically influencing AI research, spurring discussions on what has changed so far and how to shape the field's future. To clarify such questions, we analyze a new dataset of 16,979 LLM-related arXiv papers, focusing on recent trends in 2023 vs. 2018-2022. First, we study disciplinary shifts: LLM research increasingly considers societal impacts, evidenced by ...
Here are some of the research areas that can help address these problems and make LLMs available to more domains in the future. ... the LLM first creates a search query, then retrieves documents ...
LLM research, it has become considerably challenging to perceive the bigger picture of the advances in this direction. Considering the rapidly emerging plethora of literature on LLMs, it is imperative that the research community is able to benefit from a concise yet comprehensive overview of the recent developments in this field.
This information is applicable for 2024 entry. Given the interval between the publication of courses and enrolment, some of the information may change. It is important to check our website before you apply. Please read our terms and conditions to find out more. The University of Warwick's Law School offers a comprehensive LLM by research.
Our Goals. To work as a partner with our patients in conducting research that benefits the practice of medicine and overall patient care. To provide the best mix of expertise and service to achieve our research goals. To continuously improve our methods and knowledge to provide innovative solutions to our patients needs.
Although uncertainty measures over LLM output sequences remains an open area of research 22,23, we explored a simple proxy as an initial approach to measuring the relationship between LLM ...
Large Language Models (LLMs) have recently demonstrated remarkable capabilities in natural language processing tasks and beyond. This success of LLMs has led to a large influx of research contributions in this direction. These works encompass diverse topics such as architectural innovations, better training strategies, context length improvements, fine-tuning, multi-modal LLMs, robotics ...
The work of S.W. is supported through research funding provided by the Wellcome Trust (grant nr 223765/Z/21/Z), Sloan Foundation (grant no. G-2021-16779), the Department of Health and Social Care ...
Additionally, the article delves into advanced optimization techniques, covering quantization and inference optimization. By navigating through the detailed Table of Contents, readers gain a thorough understanding of the essential components involved in LLM research, empowering them to embark on a journey toward expertise in the field.
LLM Research has conducted a variety of clinical studies in adult and pediatric patients and has contributed to the development of new therapies for healthy participants and patients struggling with diseases in multiple therapeutic areas including but not limited to pulmonology, gastroenterology, gynecology, oncology, hematology, hepatology, endocrinology, dermatology, and psychiatry.
A large language model (LLM) is a computational model notable for its ability to achieve general-purpose language generation and other natural language processing tasks such as classification.Based on language models, LLMs acquire these abilities by learning statistical relationships from text documents during a computationally intensive self-supervised and semi-supervised training process.
Specifically, we adopted a fixed-LLM prompt-tuning strategy 42 to attach a continuous embedding (i.e., virtue tokens) to the input sequence [virtual tokens; x; y] as a soft prompt to control the ...
The automation of LLM tasks can be important in some research contexts. If using automated LLM tools (i.e., agents) researcher considerations should include: Will LLM agent(s) used in the research? How many and in what sequence will LLM agent(s) used? Will the code for creating the agents be made publicly available? Many research workflows can ...
For example, the model's performance improved from 74.2% to 82.1% on GSM8K and from 78.2% to 83.0% on DROP, which are two widely used benchmarks for evaluating LLM performance. A recent study focuses on enhancing a crucial LLM technique called "instruction fine-tuning," which forms the foundation of products like ChatGPT.
Their neurosymbolic method LILO uses a standard LLM to write code, then pairs it with Stitch to find abstractions that are comprehensively documented in a library. ... CSAIL affiliate, and lead author on the research. "Our work creates more straightforward abstractions for language models and assigns natural language names and documentation ...
To discriminate the difference in parameter scale, the research community has coined the term large language models (LLM) for the PLMs of significant size. Recently, the research on LLMs has been largely advanced by both academia and industry, and a remarkable progress is the launch of ChatGPT, which has attracted widespread attention from society.
This research paper was presented at the 12 th International Conference on Learning Representations ... Also, some LLM modules primarily attend only to special tokens, such as punctuation, for which it is possible to create a KV cache that retains only those tokens. Finally, some LLM modules broadly need all tokens, and for these we can employ ...
16,17,18,19, the LLM was used as a crossover operator rather than a mutation one, that is, the LLM prompts are composed of several functions, similarly to FunSearch. In refs. In refs.
Fujitsu trains Fugaku-LLM model with 13 billion parameters for research and commercial use. ... Fujitsu's Fugaku-LLM was trained using 380 billion tokens on 13,824 nodes of the Fugaku ...
Fugaku-LLM can be used for research and commercial purposes as long as users comply with the license. Fugaku-LLM will be also offered to users via the Fujitsu Research Portal from May 10th, 2024. In the future, as more researchers and engineers participate in improving the models and their applications, the efficiency of training will be ...
Additionally, some research efforts introduce specialized data from professional domains, such as code or scientific data, to enhance LLM capabilities in those fields. Leveraging diverse sources of text data for LLM training can significantly enhance the model's generalization capabilities.
Brands Focus on Developing LLM and Diverse AI Applications ... ResearchAndMarkets.com is the world's leading source for international market research reports and market data. We provide you with ...
ライセンスで定めた条件下で、誰もが研究および商業目的での利用が可能である。さらに、富士通はFugaku-LLMを、富士通の先端技術を無償で試せる「Fujitsu Research Portal」を通じて2024年5月10日より提供開始する。
Abstract. Large language models (LLMs) are dramatically influencing AI research, spurring discussions on what has changed so far and how to shape the field's future. To clarify such questions, we analyze a new dataset of 16,979 LLM-related arXiv papers, focusing on recent trends in 2023 vs. 2018-2022. First, we study disciplinary shifts: LLM ...
Given the performance of IR systems, the optimal strategy for question answering does not always entail external information retrieval; rather, it often involves leveraging the parametric memory of the LLM itself. Prior research has identified this phenomenon in the PopQA dataset, wherein the most popular questions are effectively addressed ...