Transforming advanced manufacturing through Industry 4.0
The last decade has seen companies operating under increasing levels of disruption. Quickly changing customer preferences, as well as demand uncertainty and disruptions, are challenging planning systems to unprecedented degrees. National security interests, trade barriers, and logistics disruptions are pushing businesses to find alternatives to globalized supply chains. Major swings in demand are calling for drastic operational and capital cost reduction in some areas and rapid growth in others. Physical distancing and remote work are forcing manufacturers to reconfigure manufacturing flows and management. Meanwhile, increased global concern for the environmental impact of human activities has forced companies to rethink manufacturing strategies.
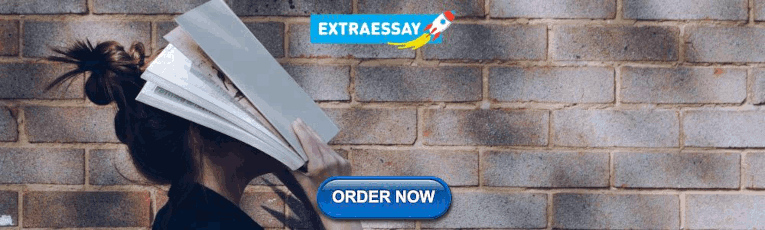
About the authors
This article is a collaborative effort by Enno de Boer , Yorgos Friligos, Yves Giraud, David Liang, Yogesh Malik , Nick Mellors, Rahul Shahani, and James Wallace, representing views from McKinsey’s Operations Practice.
To address these disruptions, successful advanced industry (AI) companies are leveraging Industry 4.0 to achieve faster, more sustainable change, shown most dramatically at “lighthouse” manufacturers that have led the way in Industry 4.0 implementation. Through the Global Lighthouse Network (GLN), a research collaboration between the World Economic Forum and McKinsey on the future of production and the Fourth Industrial Revolution, 103 sites around the world have been identified as lighthouses, having successfully transformed their factories through Industry 4.0. These companies are leveraging digital technology to build more agile and customer-focused organizations. This approach lets manufacturers look beyond productivity in order to focus on improving their sustainability, agility, speed to market, customization, and customer satisfaction: a total of five areas of impact.
Challenges to overcome
The lighthouse sites are exceptional in their ability to overcome the obstacles that stand between many companies and digital transformation. A 2020 Industry 4.0 survey by McKinsey of more than 800 businesses globally revealed three major challenge areas: financial hurdles, organizational problems, and technology roadblocks.
Financial hurdles typically include the high costs associated with scaling digital deployments that don’t provide short-term benefits to the organization, and therefore don’t provide a strong incentive for investment. Use cases that don’t provide a clear, quantifiable value to the organization can also yield an unclear road map to digital success.
Organizational problems often involve low buy-in and a lack of concentration from leadership as a business attempts to see a digital transformation through. That hampers the effort’s potential success and long-term viability. Inadequate knowledge of digital capabilities and a lack of organizational talent can prevent broader buy-in and properly scaled transformative efforts.
Technology roadblocks commonly include low support from partners in scaling deployment while facing multiple platform choices, which hinders an organization’s ability to move quickly into new territory. The transformation’s starting point can also stall when leaders aren’t convinced of their ability to increase the size and scope of the digital architecture they choose for implementation.
AI companies have tried many approaches to overcome these barriers and realize improved performance through digital manufacturing transformations. An examination of advanced manufacturing lighthouses reveals two critical reasons that their transformations succeeded: first, they chose the right use cases; second, they looked for ways that those use cases could reinforce one another.
Increasing Industry 4.0 adoption and impact among advanced industry companies
Beginning with 16 sites in 2018, the GLN has grown to 103 sites as of 2022. Nearly 60 percent of the current lighthouses are companies in AI (Exhibit 1).
Industry 4.0 has helped these companies make strides across KPIs that drive growth. These KPIs span all five areas of impact and include sustainability KPIs, such as greenhouse-gas emissions; productivity KPIs, such as factory output; agility KPIs, such as lead-time reduction; other speed-to-market KPIs; and customization (Exhibit 2).
Companies that successfully created lighthouses focused on the use cases that had material impact on these five areas, allowing them to succeed where previous efforts failed. Although there are many possible Industry 4.0 use cases, four are significantly more popular in AI companies than in non-AI companies. We see examples of these use cases in three automobile manufacturers (a luxury-vehicle manufacturer in Europe and two automobile manufacturers, one in Europe and one in Asia), and in a European white-goods company.
Flexible automation. Companies use intelligent robotics to precisely automate previously manual jobs. For instance, the European automobile manufacturer connected robots to efficiently manage process flow and collect the data necessary to monitor the process, optimize production flow, and reduce losses. The luxury-vehicle manufacturer used robust automation with collaboration between people and machines to improve efficiency, quality, and ergonomics.
Digital performance management. Firms use data to monitor and improve performance by driving operational decision making. The European automotive company uses real-time, accurate data to power decision making, resulting in better reaction speed and direction plus stronger competitiveness: the firm’s cost per unit shrank by 3.5 percent. The luxury-automobile manufacturer uses smart data analytics to enable predictive maintenance, reducing a critical asset’s unplanned downtime by 25 percent.
The white-goods factory increased overall equipment effectiveness (OEE) by 11 percent through machine alarm aggregation, prioritization, and analytics-enabled problem solving. The company puts specific machine and custom alarms on operators’ and managers’ smart watches and other interactive displays. The same company uses digital dashboards to monitor production resources and collect real-time production data, including reasons for stoppages.
The Asian automotive company reduced its die manufacturing time by 47 percent by using a real-time production monitoring and scheduling system, with integrated workflows and tablets replacing paper-based processes for operators. It also increased production output by 6 percent by connecting all its production machinery to a single manufacturing information system that tracks performance metrics and automatically detects bottlenecks.
Would you like to learn more about our Operations Practice ?
Quality analytics. Companies use advanced analytics to identify and remediate the root causes of quality defects. The European automobile maker installed a touch device with apps at each workstation to guide real-time problem solving, automatic identification and steering for parts and vehicles, and unit traceability. The result: a 40 percent increase in accomplishing tasks correctly on the first pass.
The luxury-automobile company is using smart maintenance and assistance, employing wireless-sensor technology to let maintenance employees constantly monitor production lines. Rework has dropped by 5 percent.
AI-based inspections. Firms use vision systems to inspect products and leverage artificial intelligence/machine learning to identify defects. The white-goods company uses cost modeling to help it decide what to make and what to buy. It also monitors its grid, power, and asset status in real time to control and adjust consumption.
The same company also reduces its time to market with rapid design prototyping through three-dimensional additive manufacturing. It makes mock-ups and functional prototypes of new products in smart materials.
Between 13 and 19 percent more AI lighthouses cited one of four use cases as one of their highest-impact use cases, compared with non-AI lighthouses (Exhibit 3). Flexible automation, digital performance management, and quality analytics were universally popular among AI and non-AI companies: as many as 69 percent of companies cited one as the use case with the greatest effect. Similarly, AI inspection was also much more prevalent in AI companies than in their non-AI peers.
Synergistic use cases yield bigger impacts
Track the progress of digital transformation.
To keep tabs on digital-transformation efforts, companies need to establish metrics that leaders can track effectively. Metrics should be transparent and accountable across the stakeholder groups implementing the transformation. The Global Lighthouse Network demonstrates that leaders should follow the following key metrics.
Return on digital investment. Are the monetary returns realized across the total lifetime of investments in digital capabilities aligned with internal company metrics such as internal rate of return (IRR) and ROI?
Percentage of annual technology budget spent on bold digital initiatives. How much of its technology budget is the organization spending on digital initiatives that drive value?
Digital app time to market. What’s the timeline for deploying applications into transformation initiatives and externally facing sources?
Percentage of leader initiatives linked to digital. How motivated are leaders to support digital-transformation initiatives?
Attraction, promotion, and retention metrics for top technical talent. Does the company have a pipeline of workers who will contribute to digital capabilities and performance?
The automobile manufacturers and white-goods company employed multiple use cases in their businesses. Though the use cases are individually effective, the synergies between them in combination yield still better results. These synergies allowed the companies to take advantage of their one-time investment in a technology stack to pursue multiple use cases instead of just one or two, resulting in even greater impact that can build over time (see sidebar, “Track the progress of digital transformation”).
For example, consider the European automotive factory, which is now one of the best-performing commercial-vehicle plants in Europe. This plant implemented a digitally enabled right-first-time tool, a touch device with apps at each workstation to ensure better performance through real-time support to fix problems, automatic identification to guide parts and vehicles, and unit traceability to ensure a quality process.
At the same time, the factory connected people to drive performance by enabling digital daily-management solutions. These solutions are based on accurate information that’s provided in real time and contributes to decision making, reactivity, and competitiveness. The company used digital track and trace, a flexible logistic flow developed to ensure that parts and vehicles are traceable and conform to standards. The system includes radio-frequency-identification (RFID) tags on parts and packaging, blockchain part traceability from end to end, packaging task management automation for forklift operators, and synchronization of kitting parts with the assembly line.
That wasn’t all. The factory also optimized robot cycle time through data analytics on programmable logic controllers (PLCs). It connected robots to efficiently manage process flow and collect data necessary to monitor processes, optimize production flow, and reduce losses. The firm implemented digital solutions that improve sustainability by driving energy consumption. For example, it uses a digital platform and a drone equipped with a thermal camera to find and control temperature leaks in the roof.
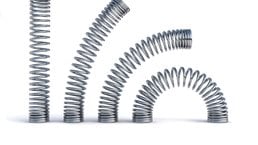
Future-proofing the supply chain
Taken together, those changes helped the plant reduce warranty incidents by 50 percent, increase its flexibility to deal with its many vehicle configurations, and reduce manufacturing costs by more than 10 percent.
The luxury-automobile factory also saw substantial benefits from deploying multiple use cases. It used smart data analytics to control predictive maintenance and collect data that let machines self-service within minutes, pairing that with reliable material supply and autonomous transportation systems that increased efficiency.
The firm then added a smart maintenance and assistance program that has maintenance employees constantly monitoring the production line at every place, using wireless-sensor technology in the quality-assurance process. It combined robust automation with worker–machine collaboration to increase efficiency, quality, and ergonomics.
This mix of digital manufacturing and lean, strategy-oriented processes yielded improved quality, lower costs, and higher productivity. It reduced a critical asset’s unplanned down time by 25 percent.
Embarking on the Industry 4.0 journey
AI organizations looking to embark on their Industry 4.0 journeys can use lessons from these successful lighthouse sites. By leveraging digital technology across the most effective use cases, more and more companies can drive outsize impact across several different KPIs. Companies that can deploy Industry 4.0 at scale are transforming their organizations to not only address the toughest disruptions of today but also prepare themselves for the new disruptions of tomorrow.
Enno de Boer is a senior partner in McKinsey’s New Jersey office; Yorgos Friligos is an associate partner in the Miami office; Yves Giraud is a senior expert in the Geneva office; David Liang is an associate partner in the Bay Area office; Yogesh Malik is a senior partner in the Washington, DC, office; Nick Mellors is a partner in the Seattle office; Rahul Shahani is an associate partner in the New York office; and James Wallace is a consultant in the Chicago office.
Explore a career with us
Related articles.
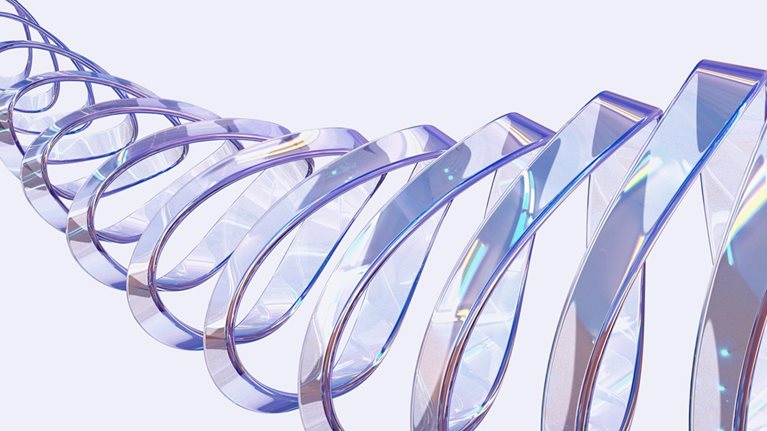
A better way to drive your business
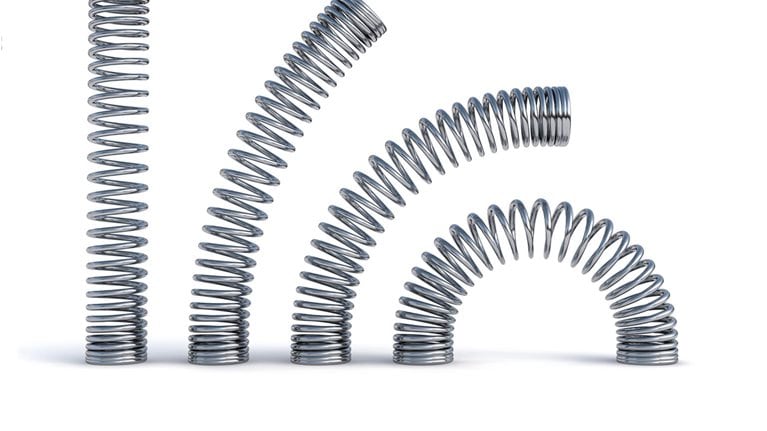
Mastering the dual mission: Carbon and cost savings
June 6, 10AM ET — Session 1 of our What's New Webinar Series: "Product Design: Reduce Cost, Carbon, and Risk"
- Deutsch (DE)
Design for Manufacturing Examples: Real-Life Engineering Case Studies

Key Takeaways:
- More than 70% of a part’s cost can be locked in during the early design phase
- Adopt a robust DFM process using digital manufacturing simulation tools to address cost, sustainability, and innovative design iterations simultaneously
The Full Article:
Typically, more than 70% of a part’s cost is locked in once its design is finalized. And at that point, manufacturing and sourcing teams have limited options to optimize part costs. That’s why cost modeling is exponentially more effective during the design phase. Product engineers need the ability to identify viable, cost-effective design alternatives while a project is still on the drawing board. This approach empowers design teams to innovate without sacrificing time to market or profit margins.
We explore this topic in greater detail by examining real-life examples to illustrate a key DFM principle in action. This includes why spreadsheets and other tools that rely on historical estimates provide a static, incomplete view of costing data – and how you can overcome this challenge with solutions that link design decisions to cost outcomes. Areas addressed include:
- The Design for Manufacturing (DFM) Imperative
- Overview of Important Cost Categories
- DFM Success Stories: Identifying Cost Inefficiencies
- Hidden Material Cost Drivers
- DFM Material Conversion Cost Example
- Other Methods for Cost-Effective Design for Manufacturability
- Learn More About the Power of Digital Manufacturing Insights
1) The Design for Manufacturing (DFM) Imperative
What if engineers had precise, design-level guidance on key cost drivers for their new product designs? And what if they had the insight to see how the cost is being affected by raw materials, conversion (i.e., the cost of turning raw material into a part), routing, and other manufacturability issues?
Having access to this capability would provide design and cost engineers with guidance to revise parts for added cost efficiency during the design phase. aPriori’s Manufacturing Insights Platform offers a solution that enables organizations to achieve this objective.
Unlike traditional spreadsheets, aPriori automatically evaluates the geometry of 3D CAD models whenever they are checked into a product lifecycle management (PLM) system. Through this software functionality, engineers gain real-time cost insights for parts and sub-assemblies, improving design and sourcing decisions.
Moreover, aPriori provides teams with a deeper understanding of the complex factors influencing part costs. The software is also equipped with cost and process modeling capabilities , enabling engineers to configure and run various scenarios. As a result, teams can seamlessly compare a part’s material, supplier, regional expenses, and more to make informed decisions.
To understand the impact of advanced manufacturing cost modeling, it’s helpful to consider the factors that contribute to a part’s final cost. Below, we break down a few key categories of part cost. The specifics may vary greatly, but these basic cost categories apply whether the part in question is sheet metal or plastic, cast or machined.
Watch this webinar to learn the best practices and digital tools to build a successful costing team.
2) Overview of Important Cost Categories
Direct + variable costs:.
The powerful interaction between each choice in the direct/variable cost category is significantly important. While engineering decisions may have an impact on period costs in the long run, we will focus on direct costs, as they often have the most substantial impact. The following categories describe the expenses associated with the marginal cost of producing each additional part.
Key Drivers of Material Costs
- Material type
- Material stock size (standard or non-standard)
- Material selection and utilization
- Special grain orientations (e.g., tight bends on a part may only allow manufacturing to orient the part in one direction when cutting it on the sheet)
Key Drivers of Overhead and Labor Costs
- Cycle time to make the part. Note: more than one machine may be used to make a part.
- Number of times that the part must be set up – whether in one machine or multiple machines
- Type and size of machine(s) that will be used to make the part
- Any secondary production processes such as paint, heat treatment, etc.
Indirect/Period Costs:
These costs matter for overall profitability but aren’t necessarily immediately impacted by marginal production changes. For instance, a factory will have some base level of maintenance costs regardless of the number of parts being made within a given period. These costs must be associated with specific supporting functions and spread across all parts produced.
Key Factory-Related Cost Drivers
- Energy costs
- Heating and cooling the plant
- Cleaning and maintenance
- Purchasing, manufacturing, engineering, shipping and receiving, and other supporting business functions
Key Administrative Cost Drivers
- General management costs
- Sales, marketing, and business development expenditures
- Technology support (e.g., IT staff or services)
Capital Expenditures (CapEx) and Non-Recurring Costs:
- Examples include initial investments in productive capital such as molds, stamping dies, machining fixtures, weld fixtures, and more.
- The cost impact of capital expenditures will vary depending on the complexity of the part, number of cavities, number of parts over the life of the tool, etc.
3) DFM Success Stories: Identifying Cost Inefficiencies
We developed both case studies using aPriori’s digital factory capabilities, which involve simulated production based on modeling a part’s digital twin .
During the design stage, you don’t need the absolute value estimate to be exact; a good, reliable approximation will suffice. For instance, you may determine that 20% of the part cost is material and 65% is conversion cost. While these amounts may vary during final production, they can provide a useful guidepost for prioritizing cost optimization projects. This practice can help you save time by avoiding product design changes that will have minimal impact on cost.
Manufacturing insights can help engineers minimize time-consuming activities and work faster. This automation-driven platform can determine the most efficient manufacturing methodology through near-instant cost estimates for new design alternatives.
Learn more about how design for manufacturing (DFM) has a real impact on new product pipelines.
Material Cost Example One: Truck Sheet Metal Fan Cover Redesign
The following screenshot shows that 88% of the fan cover cost is material. To reduce material costs, you can:
- Select an alternative material that is cheaper (but still reflects functional load requirements and tolerances).
- Use less material by making the part thinner, adding ribbed forms to strengthen it, or improving material utilization to reduce waste.
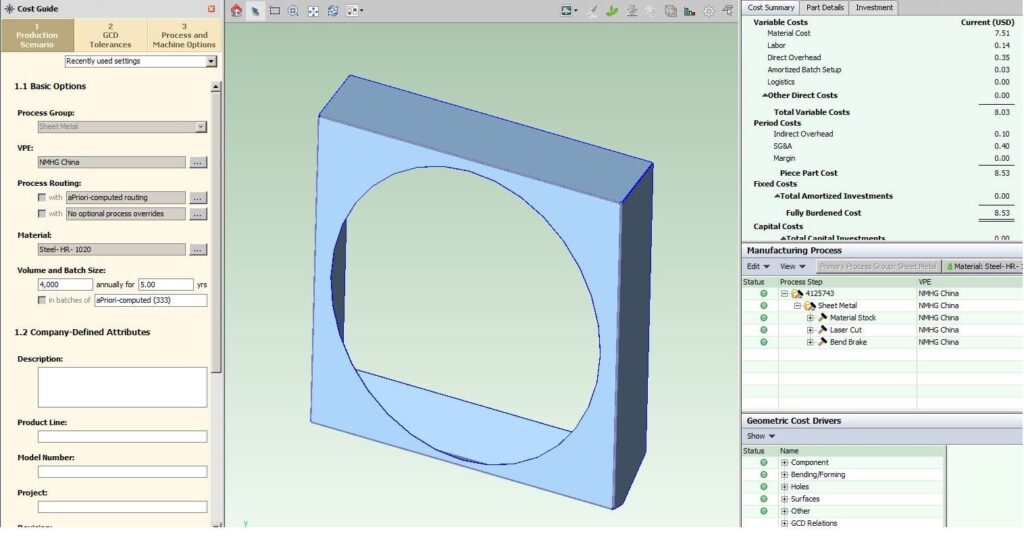
The product developer recognized that the material choice was the primary cost driver and reduced the part size without altering the size of the opening or component mating points. The following screenshot displays his final solution.
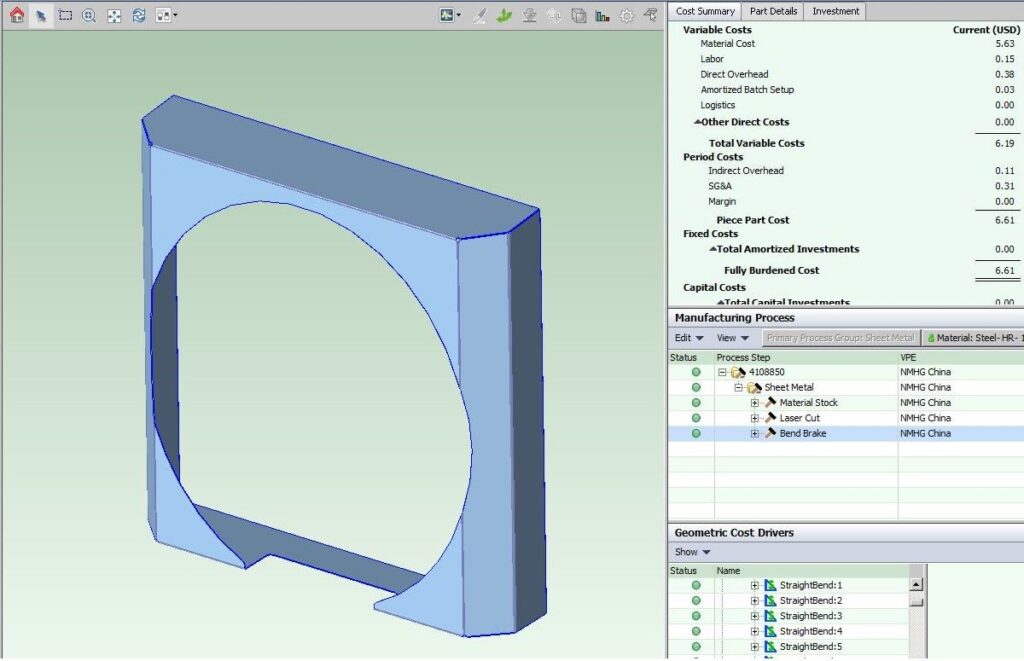
Note that while labor and overhead costs increased from $0.49 to $0.53, the material cost dropped from $7.51 to $5.63, saving $1.88 – which is a 25% savings. This improvement has paid for itself exponentially because the part is still used in tens of thousands of trucks.
This is a great example of how a reliable cost estimate is useful for prioritizing redesign work. A good cost vector (whether the cost is going up or down, by a little or a lot) is sufficient. For example, if the material cost dropped by only $1.50 instead of $1.88, the price reduction would still warrant a redesign.
Material Cost Example Two: Plastic Seat
A manufacturer produces approximately 200,000 seats annually. The digital manufacturing cost model revealed that material is 67% of the total cost.
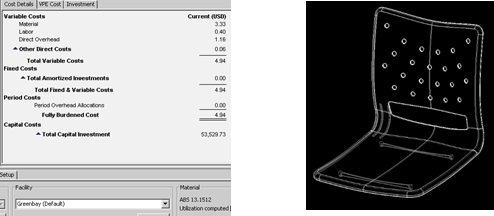
The engineer redesigning the seat has two options:
- Use lower-cost materials. Note: had the conversion cost been the most expensive, you may have wanted a material that cools faster, thereby decreasing the cycle time and production cost.
- Reduce the amount of material without compromising seat integrity.
The engineer tried several alternative designs, including:
She began by reducing the thickness of the plastic from the top edge of the back of the seat down to 2/3 of the way and from the edge of the bottom of the seat to approximately ½ of the way to the middle of the seat. This change decreased the average thickness from 0.18” to 0.15”. It is critical to note that the cost of materials, labor, and overhead was also reduced. That’s because the thinner part cools faster, leading to a double benefit: a reduction in material and manufacturing costs, totaling $0.95 on a $5 component – a nearly 20% reduction.
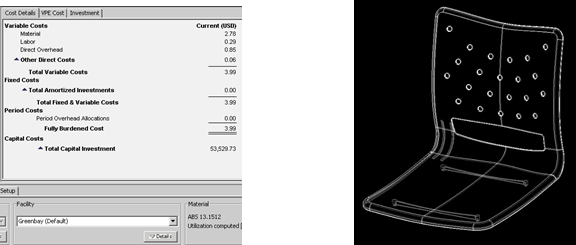
The second design change made the back hole slightly larger from its original 5”–6” in height. However, because this change only shaved a few cents off the original cost, it was not worth the risk of potential quality issues or increased customer discomfort. The value of having real-time cost feedback “at the speed of design” enables you to catch these false starts far earlier in the process and maintain quality control by adhering to the principles of DFM.
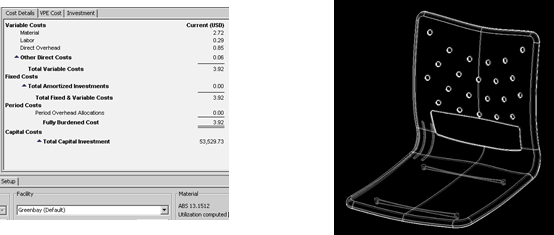
4) Hidden Material Cost Drivers
- This approach worked until their factory became overwhelmed and started buying parts or sending them to another internal factory across the country. The parts became much more expensive because they needed to orient the components perpendicular to the bend, which limits the nesting flexibility of the part and requires more material. Simulated production software like aPriori can automatically identify if a bend is too tight and recommend a minimum bend angle.
- The organization suspected an unscrupulous bid from a supplier. Still, upon review, it found that the supplier had to buy a special forging or start with the next size-up standard bar to meet the customer’s requirements. Either way, the cost would be disproportionately impacted. A diameter reduction of just a few millimeters fixed the issue, and the final design still had plenty of inertia margin.
5) DFM Material Conversion Cost Example
Let’s now move into conversion costs. Design engineers make choices that affect a large range of conversion costs, such as:
- Labor cost is proportional to cycle time. And the skill necessary to run the machine affects the wages of the operator. A 5-axis CNC machinist makes more than a 3-axis mill operator, for example.
- Set-up cost includes the number of machines to be set up and the number of times the part needs to be set up. Volume plays a large role in determining the per-product impact of set-up costs.
- Direct overhead cost is proportional to cycle time and the type and size of the machine.
An engineer was assigned to reduce the cost for a part like the one below. A quick design review revealed a 40/60 split between material cost and conversion cost. This implies that there may be opportunities to contain costs on both sides of this split without impacting lead times.
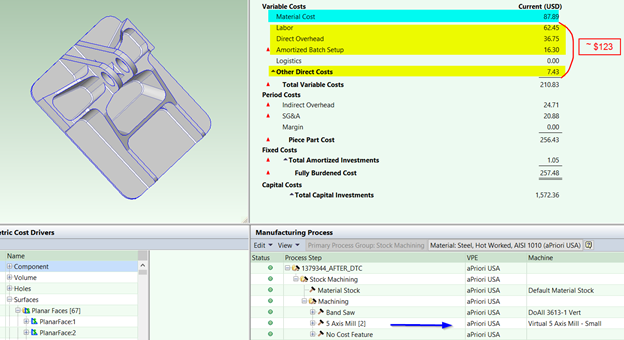
The engineer also noted that because this is a relatively low-volume part (300 units per year), it was being purchased as a machined part. While not very complex, the multiple slants on the surfaces were forcing this part to a 5-axis mill (rather than a comparatively cheap 3-axis mill).
The engineer had three choices to reduce costs:
- Redesign the part to reduce complexity for production on a cheaper machine
- Investigate machining costs further and address those issues in the design
- Identify alternative manufacturing processes for the part if they show promise
Using simulated manufacturing to analyze costs, the engineer discovered that the material utilization was only 11%, meaning that nearly 9 lbs. out of every 10 lbs. of material would be wasted. As expected, most of the cost of making the part was in machining, but from roughing operations, not finishing the part. This demonstrated that getting the part to near net shape was costing a lot in both material and manufacturing costs (see the figures below).
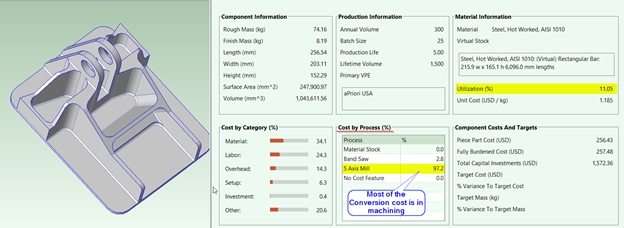
This part had been designated as a machined component because of the relatively low volume production of 300 units per year. However, based on this evidence, the engineer decided to investigate sand casting for the part. To see if it would be worth redoing the design and fatigue analysis to turn this into a casting, he created a cost estimate for sand casting the part.
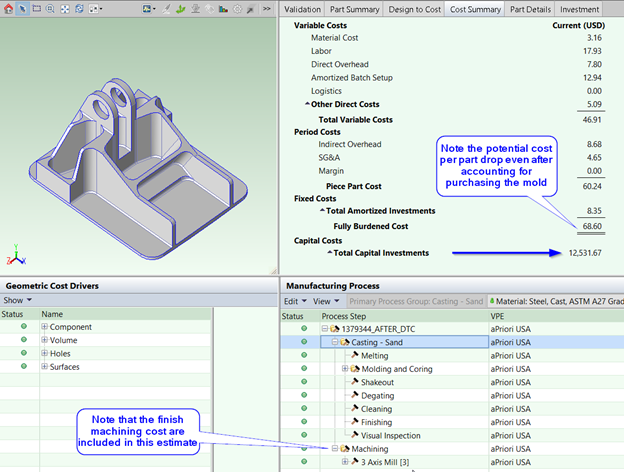
After analyzing the cost difference of approximately $190 per part on 300 parts, which amounted to a potential annual savings of $57,000, the component was redesigned and purchased as a casting, resulting in significant cost savings.
Alternatively, imagine that this part was not a candidate for a casting process due to load and fatigue requirements, as is the part below. The process for reducing costs for the part is similar, except that you need to explore machining costs (some parts may be extruded as well).
Consider how manufacturability issues may be costing you dearly. By evaluating the actual production methods intended for a part, manufacturing insights can identify design features that pose significant challenges. This could involve pinpointing a lack of draft angles, areas with excessive or insufficient thickness, or features that need a side action in plastic injection molding or die casting. For machined parts, issues like sharp corners, obstructed surfaces, or curved surfaces that require ball milling could be highlighted. Addressing these problems early can streamline production and reduce critical costs.
Looking at this part below, we notice a similar ratio of material to conversion cost. And we dig into the features that make it difficult to produce, as casting or extruding it is not an option.
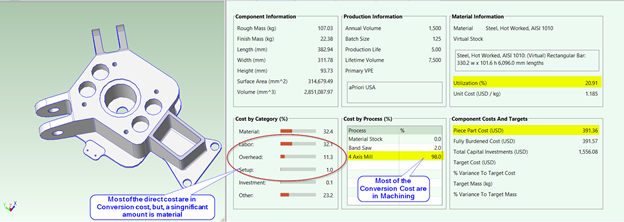
In the interest of time, we will limit ourselves to resolving as many of these L/D ratios as possible. The engineer realizes that the corner radius of those pockets is small, requiring a small tool diameter selection that violates customary L/D ratios and causes slower finishing times. He has the liberty to make those bigger, which won’t change the material consumed. See the figure below for the redesigned part.
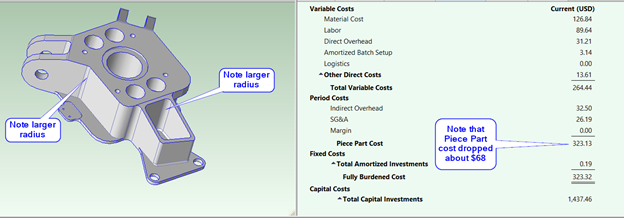
Larger corner radii allow for larger diameter selection, which increases the tool’s ability to reach further down without shaking. Cycle time drops, and cost goes down. A 17% cost reduction is certainly worth the effort of the redesign.
6) Other Methods for Cost-Effective Design for Manufacturability
It is possible to affect the size of a machine in manufacturing by considering the design of the part. For example, suppose a part is being produced in China, where labor costs are low, but overhead costs are high due to the use of large, expensive machines. In that case, it may be worth considering features that can influence machine selection.
The die-cast part below has a web in the middle that is not functionally necessary. This web is causing the part to require two-side cores, one on each side. If the web were removed, only one core would be needed, the mold base size would decrease, and the machine size (tonnage) would go down, causing a reduction in tooling and piece part cost with a smaller machine/lower overhead rate. Additionally, you may be able to have more cavities now, which is a big plus if this is a high-volume part.
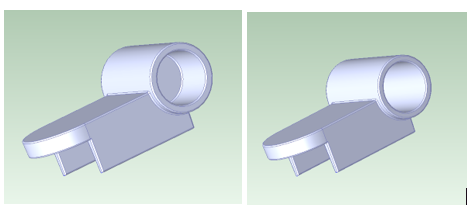
The number of set-ups can dramatically affect the cost of a low-volume part. A hole that can’t be accessed from an already available set-up direction (aPriori can show you those) can cause an extra set-up.
Too many of these will require a more expensive machine, for example, forcing a move from a 3-axis to a 4-axis or 5-axis. Did you know that if your sheet metal part has an acute angle bend and an obtuse angle bend on the same part, then two bend breaks will have to be set up to make it? This may have minimal cost impact if the part is produced in large volumes, but if this is a low-volume part, it could create serious cost inefficiencies.
7) Learn More About the Power of Digital Manufacturing Insights
DFM is pivotal to identifying cost savings from the initial product design through material selection and manufacturing. By integrating aPriori’s advanced manufacturing insights, product engineers gain a deeper understanding of how seemingly small variables can significantly impact cost and other factors.
This approach provides product design and cost engineers with clear visibility and automated guidance to make informed decisions that enhance both product quality and profitability. The adoption of DFM best practices, supported by aPriori’s insights, can ensure that products are designed for performance, profitability, sustainability, and market success.
This post was originally published on Aug. 12, 2020, and updated on April 18, 2024.
Ready to Unleash the Power of DFM?
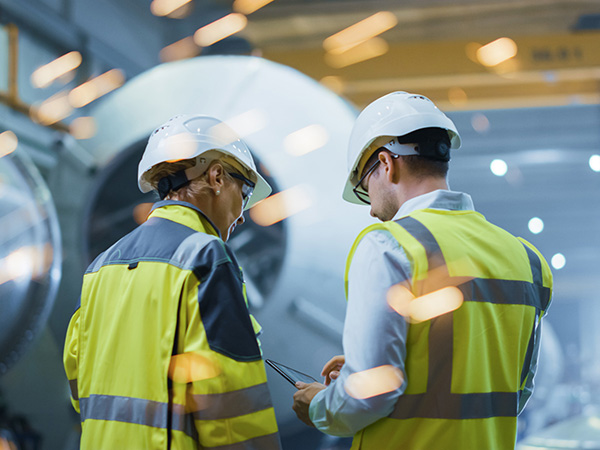
Like what you see? Share on:
Subscribe to the aPriori Blog!
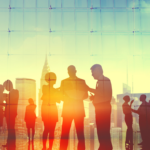
How to Use Supply Chain Collaboration to Minimize Disruption
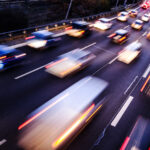
Retool the Auto Manufacturing Process to Save Time & Money: 5 Strategies
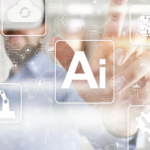
How to Future-Proof Your AI Manufacturing Initiatives with Data
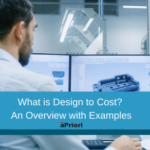
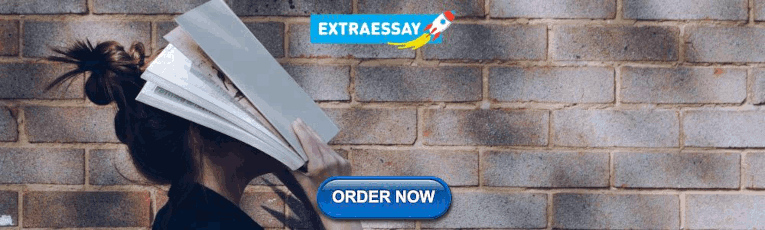
What Is Design to Cost? An Overview With Examples
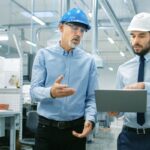
6 Metrics That Will Help Improve Your Engineering Productivity
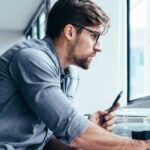
How to Conduct a Spend Analysis: Methodology in Detail
- Case Studies
- Customer Reviews
- Profitability
- Manufacturability
- Sustainability
- Design to Cost
- Design for Manufacturability
- Design for Sustainability
- Should Cost Analysis
- Quoting Professionals
- Design Engineering
- Cost Engineering
- Senior Leadership
- By Industry
- Aerospace & Defense
- Auto & Transportation
- High Tech Electronics
- Industrial Equipment
- Manufacturing Insights Platform
- Digital Factories
- Regional Data Libraries
- Manufacturing Process Models
- aP Workspace
- aP Generate
- aP Analytics
- News & Events
- Request a Demo
MACHINEMETRICS BLOG
Machine vs. production monitoring: the road to production..., 2024 product releases: an inside look, introducing batch connect machines, introducing condition monitoring workflows.
Ready to empower your shop floor?
- MachineMetrics
- Product Updates
- Data Science
- Lean Manufacturing
Machine Monitoring
IoT in Manufacturing: Top Use Cases and Case Studies
Updated May 17, 2021
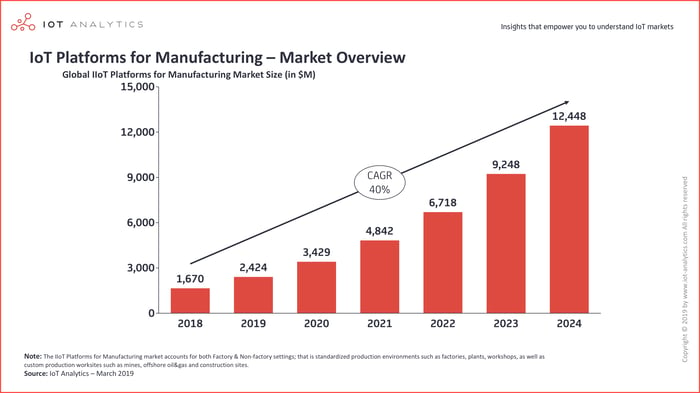
Within this article, we’ll be discussing practical IoT applications in manufacturing and use cases of industrial IoT technology in manufacturing
What is IoT?
What is iiot, the benefits of iot in manufacturing.
IoT represents a digital transformation in manufacturing processes and business operations. Using it alongside an advanced machine data platform can be transformational. And there are many benefits of IoT in manufacturing:
Process Optimization
Inventory Management
Predictive Maintenance
IoT in Manufacturing Use Cases [+Case Studies]
Remote monitoring.
Learn more about remote monitoring for machine builders and OEMs.
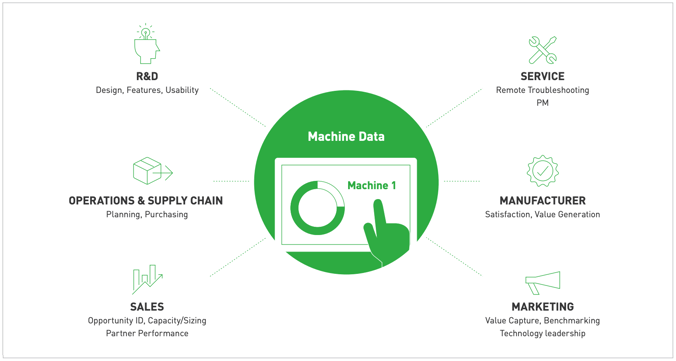
Supply Chain Management and Optimization
- Real-time tracking of assets and products
- Automation of warehouse tasks
- Digitized paperwork management
- Forecasting accuracy improvement
- Greater control of inventory
Digital Twins
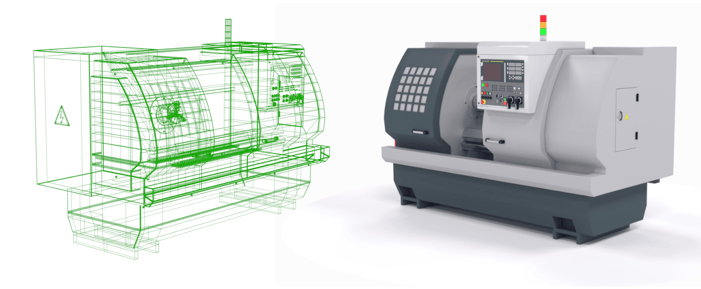
Real-Time Machine Monitoring
Production visibility.
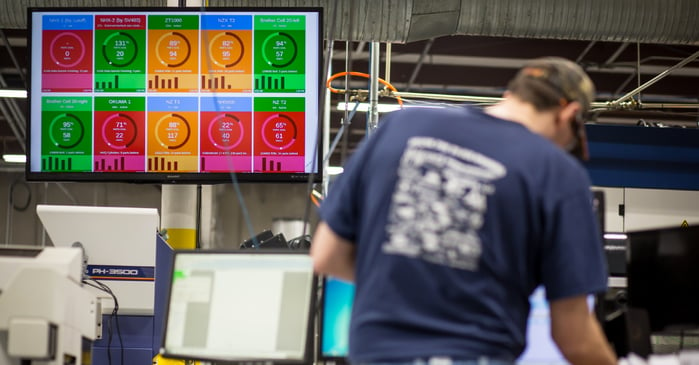
Integrating Systems
Compiling kpis.
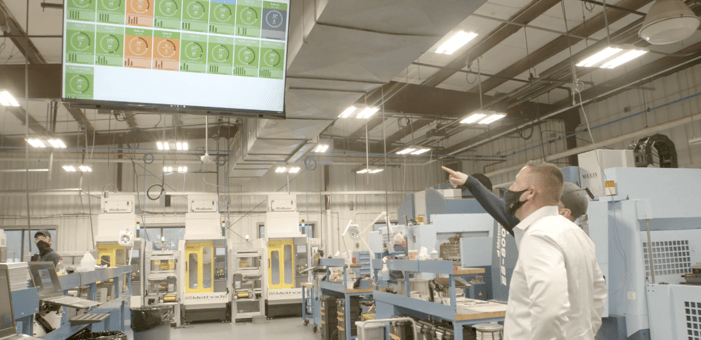
Asset Utilization
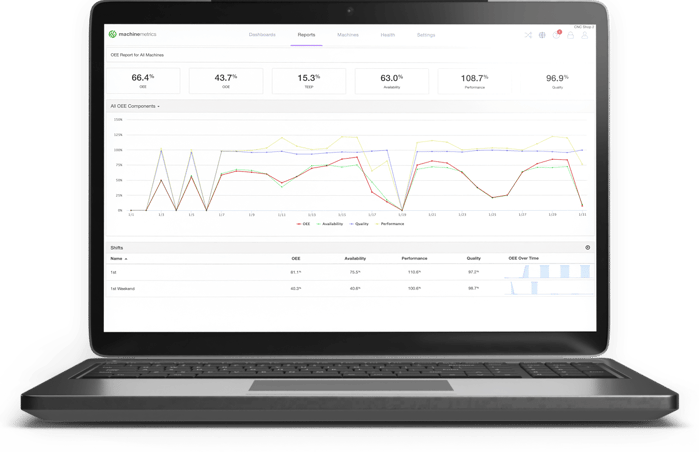
Difficulties of Adopting IoT in Manufactur ing
1. large investments are required and the roi is questionable, 2. concerns about data security, 3. employees who aren’t qualified, 4. integration with operational technology and older systems, how to use iot and machine data for remote operations, boosting your operational efficiency with iiot, subscribe to our mailing list, related posts, read the latest.
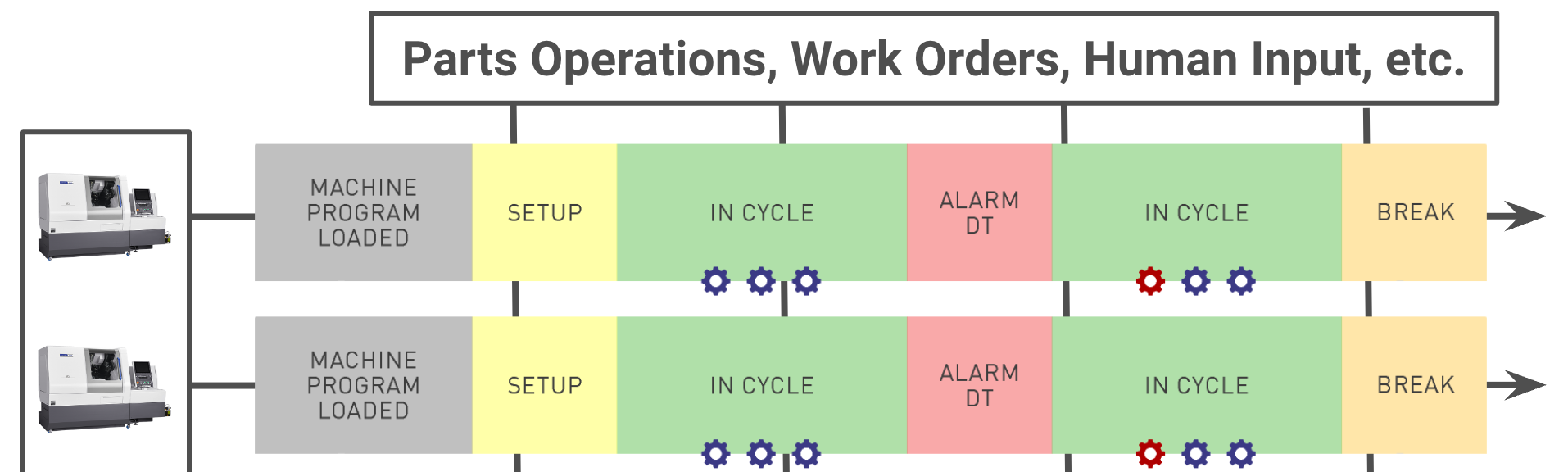
Lists by Topic
- MachineMetrics (382)
- Lean Manufacturing (72)
- Industry 4.0 (50)
- Manufacturing data (23)
- Manufacturing News (20)
- Data Science (15)
- data collection (14)
- Product Updates (12)
- Machine Monitoring (10)
- manufacturing analytics (10)
- Connected Factory (9)
- Smart Manufacturing (9)
- industrial iot (9)
- CNC Machines (8)
- Productivity (8)
- Downtime (7)
- Industrial Automation (7)
- Tool Monitoring (7)
- Big Data (6)
- Data Visualisation (5)
- Edge Computing (5)
- Maintenance (5)
- Process Optimization (5)
- Shop Floor (5)
- digital manufacturing (5)
- Automotive (4)
- Machine Learning (4)
- Quality Assurance (4)
- Aerospace and Defense (3)
- Contract Manufacturing (3)
- Data Cleaning (3)
- Death of MES (3)
- Heavy Machinery Manufacturing (3)
- MES software (3)
- Medical Device Manufacturing (3)
- OEE Software (3)
- Oil and Gas Manufacturing (3)
- capacity (3)
- continuous improvement (3)
- inustrial IOT (3)
- preventative maintenance (3)
- security (3)
- 8 wastes (2)
- Condition Monitoring (2)
- Dashboards (2)
- Internet of things (2)
- Production Monitoring (2)
- Strategic Partnerships (2)
- management (2)
- press release (2)
- real-time analytics (2)
- Digital Transformation (1)
- Downtime Categorization (1)
- FANUC FOCAS (1)
- Line Balancing (1)
- Machine Tool Distributors (1)
- Machinery (1)
- Manufacturing Innovation (1)
- Production Scheduling (1)
- Quality Control (1)
- Supply Chain (1)
- Turnkey contracts (1)
- coaching (1)
- elon musk (1)
- employee engagement (1)
- link roundup (1)
- manufacturing (1)
- manufacturing software (1)
- networks (1)
- release notes (1)
844-822-0664
Easthampton Office
116 Pleasant St, Suite 332, Easthampton, MA 01027
Edge Platform
Cloud Platform
APIs & Applications
Production Monitoring
Condition Monitoring
For Machine Builders and Distributors
For Developers
Aerospace & Defense
Contract Manufacturers
Heavy Machinery
Medical devices
Oil & Gas
Precision Metalworking
ERP Integration
Metal Stamping & Fabrication
Tool, Die & Mold CNC
ROI Calculator
Waste Calculator
Connectivity Hub
Partner Program
Privacy Policy
Data Processing Addendum
Service Level Agreement
Website Terms
Effect of Aspect Weight and Machining Progression on Machinability: Modeling and Machining Case Study
- Original Research Article
- Published: 30 May 2024
Cite this article
- Jiarui Hou 1 &
- Xichang Liang 2
37 Accesses
Explore all metrics
The machinability of workpiece is varying with the progressing of machining and cannot be evaluated from a specific cutting moment. In this work, a global machinability evaluation method with considering tool wear progression was proposed by weighted accumulating of machinability at different cutting moment, where the machinability weight at specific cutting moment was determined as the relationship of instantaneous tool wear rate to average tool wear rate through the tool life. When turning AISI D2 steels with conventional and wiper inserts, the machinability of different inserts deteriorates to different degrees with the progressing of machining. As a result, the conventional and wiper inserts showed opposite trends of machinability under different cutting moment. In addition, the wiper inserts perform better machinability at depths of cut a p = 0.2 mm (with an improvement of 7.5%) and a p = 0.4 mm (with an improvement of 2.7%), while worse machinability at a p = 0.6 mm (with a reduce of 23.4%) than the conventional inserts. The results indicated that the wiper inserts were suitable for turning with depth of cut less than 0.4 mm. In addition, another interesting finding was that the machinability evaluation with a sharp cutting tool would lose its production significance because of the fast tool wear rate, which contributed a negative weight to the global machinability. This work is potential to improve the machinability evaluation system and, in turn, better guide the production practice.
This is a preview of subscription content, log in via an institution to check access.
Access this article
Price includes VAT (Russian Federation)
Instant access to the full article PDF.
Rent this article via DeepDyve
Institutional subscriptions
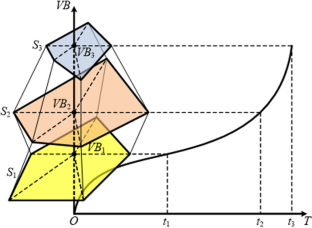
Similar content being viewed by others
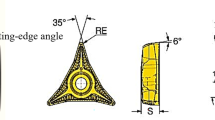
An in-depth analysis of tool wear mechanisms and surface integrity during high-speed hard turning of AISI D2 steel via novel inserts
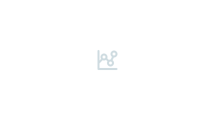
Effect of cutting edge radius on surface roughness and tool wear in hard turning of AISI 52100 steel
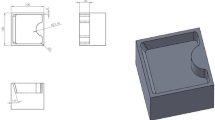
Influence of tool path strategies on machining time, tool wear, and surface roughness during milling of AISI X210Cr12 steel
A. Sahoo, S. Pradhan, and A. Rout, Development and Machinability Assessment in Turning Al/SiCp-Metal Matrix Composite with Multilayer Coated Carbide Insert Using Taguchi and Statistical Techniques, Arch. Civ. Mech. Eng. , 2013, 13 (1), p 27–35.
Article Google Scholar
R. Kumar et al., Comparative Investigation Towards Machinability Improvement in Hard Turning Using Coated and Uncoated Carbide Inserts: Part I Experimental Investigation, Adv. Manuf. , 2018, 6 (1), p 52–70.
R. Kumar et al., Comparative Study on Machinability Improvement in Hard Turning Using Coated and Uncoated Carbide Inserts: Part II Modeling, Multi-response Optimization, Tool Life, and Economic Aspects, Adv. Manuf. , 2018, 6 (2), p 155–175.
Article CAS Google Scholar
A. Das et al., Comparative Assessment on Machinability Aspects of AISI 4340 Alloy Steel Using Uncoated Carbide and Coated Cermet Inserts during Hard Turning, Arab. J. Sci. Eng. , 2016, 41 (11), p 4531–4552.
D. Palanisamy, P. Senthil, and V. Senthilkumar, The Effect of Aging on Machinability of 15Cr-5Ni Precipitation Hardened Stainless Steel, Arch. Civ. Mech. Eng. , 2016, 16 (1), p 53–63.
Z. Liu et al., Energy Consumption and Process Sustainability of Hard Milling with Tool Wear Progression, J. Mater. Process. Technol. , 2016, 229 , p 305–312.
B. Zhang et al., Drilling Process of Cf/SiC Ceramic Matrix Composites: Cutting Force Modeling, Machining Quality and PCD Tool Wear Analysis, J. Mater. Process. Technol. , 2022, 304 , p 117566.
E. Ducroux et al., New Mechanistic Cutting Force Model for Milling Additive Manufactured Inconel 718 Considering Effects of Tool Wear Evolution and Actual Tool Geometry, J. Manuf. Process. , 2021, 64 , p 67–80.
F. Kara et al., Experimental and Statistical Investigation of the Effect of Coating Type on Surface Roughness, Cutting Temperature, Vibration and Noise in Turning of Mold Steel, J. Mater. Manuf. , 2023, 2 (1), p 31–43.
Google Scholar
F. Kara et al., Effect of Machinability, Microstructure and Hardness of Deep Cryogenic Treatment in Hard Turning of AISI D2 Steel with Ceramic Cutting, J. Market. Res. , 2020, 9 (1), p 969–983.
CAS Google Scholar
K. Jiang et al., Cutting Force and Tool Wear in Cutting Ti-6Al-4V using Microstructure-Based PCD Turning Tools, Procedia CIRP , 2020, 95 , p 572–577.
M. Oganyan et al., Influence of the Application of Wear-Resistant Coatings on Force Parameters of the Cutting Process and the Tool Life during End Milling of Titanium Alloys, Mater. Today Proc. , 2021, 38 , p 1428–1432.
G. Gao et al., Cutting Force Model of Longitudinal-Torsional Ultrasonic-Assisted Milling Ti-6Al-4V Based on Tool Flank Wear, J. Mater. Process. Technol. , 2021, 291 , p 117042.
S. Ravi and P. Gurusamy, Experimental Investigation of Cryogenic Cooling on Cutting Force, Surface Roughness and Tool Wear in End Milling of Hardened AISI D3 steel Using Uncoated Tool, Mater. Today Proc. , 2020, 33 , p 3314–3318.
K. Venkatesan et al., Machinability Study and Multi-response Optimization of Cutting Force, Surface Roughness and Tool Wear on CNC Turned Inconel 617 Superalloy Using Al 2 O 3 Nanofluids in Coconut oil, Procedia Manuf. , 2019, 30 , p 396–403.
N.F.H.A. Halim, H. Ascroft, and S. Barnes, Analysis of Tool Wear, Cutting Force, Surface Roughness and Machining Temperature During Finishing Operation of Ultrasonic Assisted Milling (UAM) of Carbon Fibre Reinforced Plastic (CFRP), Procedia Eng. , 2017, 184 , p 185–191.
A. Oliveira et al., Effect of Tool Wear on the Surface Integrity of Inconel 718 in Face Milling with Cemented Carbide Tools, Wear , 2021, 476 , p 203752.
X. Liang et al., Prediction of Residual Stress with Multi-physics Model for Orthogonal Cutting Ti-6Al-4V under Various Tool Wear Morphologies, J. Mater. Process. Technol. , 2021, 288 , p 116908.
X. Liang et al., Tool Wear Induced the Gradient Distribution of Surface Integrity with Process-Microstructure-Property Characteristics after Turning Ti-6Al-4V, J. Manuf. Process. , 2021, 70 , p 570–577.
Q. Xu et al., Optimum Selection of Tool Materials for Machining of High-Strength Steels Based on fuzzy Comprehensive Evaluation Method, Proc. Inst. Mech. Eng. Part B J. Eng. Manuf. , 2019, 233 (1), p 145–153.
S. Jiang et al., Assessment of MR Compatibility and Machinability using the Fuzzy Comprehensive Evaluation Method for Material Selection of MR Device, Concepts Magn. Reson. Part B Magn. Reson. Eng. , 2015, 45 (1), p 1–12.
Z. Wei et al., Comprehensive Fuzzy Evaluation of Cutting Tool Performance in Milling of Aviation Titanium Alloy Components, China Mech. Eng. , 2015, 6 , p 711–715.
F. Kara et al., Multi-objective Optimization of Process Parameters in Milling of 17–4 PH Stainless Steel using Taguchi-Based Gray Relational Analysis, Eng. Sci. , 2023, 26 , p 961.
R.V. Rao and O. Gandhi, Digraph and Matrix Methods for the Machinability Evaluation of Work Materials, Int. J. Mach. Tools Manuf , 2002, 42 (3), p 321–330.
A.-B. Yu et al., Machinability Evaluation of Machinable Ceramics with DEA Method, J. Tianjin Univ. , 2007, 40 (2), p 148–152.
A. Sadhu and S. Chakraborty, Non-traditional Machining Processes Selection using Data Envelopment Analysis (DEA), Expert Syst. Appl. , 2011, 38 (7), p 8770–8781.
R.V. Rao, Machinability Evaluation of Work Materials using a Combined Multiple Attribute Decision-Making Method, Int. J. Adv. Manuf. Technol. , 2006, 28 (3–4), p 221–227.
R.V. Rao and J.P. Davim, A Decision-Making Framework Model for Material Selection using a Combined Multiple Attribute Decision-Making Method, Int. J. Adv. Manuf. Technol. , 2008, 35 (7), p 751–760.
T. Sun et al., An Improved Radar-Graph Method for Comprehensive Evaluation of Material Machinability, Int. J. Mech. Eng. Appl. , 2018, 6 (2), p 23.
Y. Wan et al., An Investigation on Machinability Assessment of Difficult-to-Cut Materials Based on Radar Charts, Proc. Inst. Mech. Eng. Part B J. Eng. Manuf. , 2013, 227 (12), p 1916–1920.
M. Kowalczyk and C. Niżankowski, Comparative Analysis of Machinability of Nitinol Alloy using Weighted Radar Diagram, Manag. Prod. Eng. Rev. , 2017, 8 (4), p 74–81.
J. Guddat et al., Hard Turning of AISI 52100 using PCBN Wiper Geometry Inserts and the Resulting Surface Integrity, Procedia Eng. , 2011, 19 , p 118–124.
A.E. Correia and J.P. Davim, Surface Roughness Measurement in Turning Carbon Steel AISI 1045 Using Wiper Inserts, Measurement , 2011, 44 (5), p 1000–1005.
V. Gaitonde et al., Machinability Investigations in Hard Turning of AISI D2 Cold Work Tool Steel with Conventional and Wiper Ceramic Inserts, Int. J. Refract. Met. Hard Mater. , 2009, 27 (4), p 754–763.
V. Gaitonde et al., Analysis of Machinability during Hard Turning of Cold Work Tool Steel (Type: AISI D2), Mater. Manuf. Process. , 2009, 24 (12), p 1373–1382.
Download references
Author information
Authors and affiliations.
Engineering Training Center, Shandong University, Jinan, 250002, People’s Republic of China
School of Mechanical Engineering, Shandong University, Jinan, 250061, People’s Republic of China
Xichang Liang
You can also search for this author in PubMed Google Scholar
Corresponding author
Correspondence to Jiarui Hou .
Additional information
Publisher's note.
Springer Nature remains neutral with regard to jurisdictional claims in published maps and institutional affiliations.
Rights and permissions
Springer Nature or its licensor (e.g. a society or other partner) holds exclusive rights to this article under a publishing agreement with the author(s) or other rightsholder(s); author self-archiving of the accepted manuscript version of this article is solely governed by the terms of such publishing agreement and applicable law.
Reprints and permissions
About this article
Hou, J., Liang, X. Effect of Aspect Weight and Machining Progression on Machinability: Modeling and Machining Case Study. J. of Materi Eng and Perform (2024). https://doi.org/10.1007/s11665-024-09655-5
Download citation
Received : 09 January 2024
Revised : 29 March 2024
Accepted : 13 April 2024
Published : 30 May 2024
DOI : https://doi.org/10.1007/s11665-024-09655-5
Share this article
Anyone you share the following link with will be able to read this content:
Sorry, a shareable link is not currently available for this article.
Provided by the Springer Nature SharedIt content-sharing initiative
- global evaluation method
- machinability evaluation
- machining progression
- machinability weight
- tool wear rate
- Find a journal
- Publish with us
- Track your research
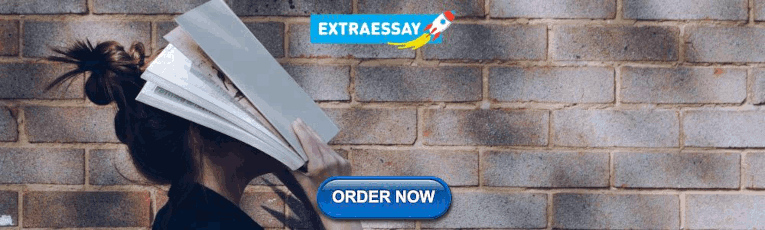
IMAGES
VIDEO
COMMENTS
Micromachining techniques have been applied widely to many industrial sectors, including aerospace, automotive, and precision instruments. However, due to their high-precision machining requirements, and the knowledge-intensive characteristics of miniaturized parts, complex manufacturing process problems often hinder production. To solve these problems, a systematic scheme for structured ...
An examination of advanced manufacturing lighthouses reveals two critical reasons that their transformations succeeded: first, they chose the right use cases; second, they looked for ways that those use cases could reinforce one another. Increasing Industry 4.0 adoption and impact among advanced industry companies
Machining is a manufacturing process in which a cutting tool is used to obtain desired shape by cut. away unwanted segment of material from a wo rkpiece [1]. As well-known, turning, milling, and ...
Advanced machining processes have become popular and economical and are finding their use in industries. Download chapter PDF. Similar content being viewed by others ... The profile of phototool is normally produced by Computer-Aided Design (CAD ... (1991). Abrasive flow machining: A case study. Journal of Materials Processing Technology, 28 ...
The literature is well documented when it comes to understanding the process of ultraprecision machining since the 1950s by researchers in the United States of America such as Hahn, Lindsey, Malkin, Subramanian, et al. [1,2,3,4,5,6,7,8,9,10].However, tribological interactions and models have been treated separately, or treated as a lumped parameter during this time owing to the complexity of ...
Machining is the main method of forming parts/products. The traditional 2D machining technology can no longer meet the requirements of modern manufacturing. Machining process design based on a 3D model can effectively inherit 3D design model information. At the same time, it has a supporting role in the realization of intelligent design of the process and the shopfloor manufacturing system ...
Conclusion and Outlook This paper presents three studies with respect to different automotive applications of cutting and grinding processes. Thereby, simulation-based design of modern high performance machining procedures is performed to enable productivity enhancements, deviation compensations and an early process development.
The aim of this Special Issue is to collate the state of the art and showcase recent innovations in advanced machining processes. This Special Issue invites papers in the area of advanced manufacturing and machining processes with a specific focus on the abovementioned areas with the objective to achieve the following: Provide an in-depth ...
Design System for Machining Process Innovation (MPI-KHDS) is constructed to support knowledge-driven micro-turbine machining process innovation. The rest of the paper is organized as follows: In Section2, a holistic framework of knowledge-based manufacturing process innovation design is introduced. Section3
The subsequent contribution utilizes advanced machine learning algorithms to model and optimize the Equal Channel Angular Pressing (ECAP) process for copper. This approach improves material properties while reducing the need for extensive experimental work, representing significant progress in the development of material design processes.
Investigate machining costs further and address those issues in the design; Identify alternative manufacturing processes for the part if they show promise; Using simulated manufacturing to analyze costs, the engineer discovered that the material utilization was only 11%, meaning that nearly 9 lbs. out of every 10 lbs. of material would be wasted.
shortcomings, to which a variety of modifications and enhancements were made to the case study company by seeking integrating economic analysis within the design process. Application of techniques such as value analysis, time study, work measurement, redesign and layout design and analysis were employed in this work to generate optimum solutions.
However, as advances in manufacturing technology grow, so does the need to implement them in an efficient and cost-effective manner. The outcome of this urgent need is a combination of traditional manufacturing tools and modern technologies, hardware or software, that transforms traditional manufacturing into intelligent manufacturing.
This book- Covers advances in sustainable machining such as gas-cooled machining, near dry machining, and minimum quantity lubrication. Explores use of big data, machine learning and artificial intelligence for machining processes. Provides case studies and experimental design as well as results with analysis focusing on achieving sustainability.
Machine tool design starts with the determination of performance specifications. Precision of the NC axes is an important aspect of machine tool design. Conventionally, the precision specification of machine tools is empirically determined, resulting in poor designs with insufficient or excessive precision. To provide a cost-effective precision specification for complex machine tools, such as ...
Abrasive flow machining: a case study. J Mater Process Technol 1991; 28: 107-116. ... Advanced machining processes. India: Allied publishers pvt Ltd, 2002, pp.57-94. Google Scholar. 6. Loveless TR, Willams RE, Rajurkar KP. A study of the effects of abrasive flow finishing on various machined surfaces. ... Design and fabrication of a low ...
The wires for wire EDM is made of brass, copper, tungsten, molybdenum. Zinc or brass coated wires are also used extensively in this process. The wire used in this process should posses high tensile strength and good electrical conductivity. Wire EDM can also employ to cut cylindrical objects with high precision.
The machine learning (ML) field has deeply impacted the manufacturing industry in the context of the Industry 4.0 paradigm. The industry 4.0 paradigm encourages the usage of smart sensors, devices, and machines, to enable smart factories that continuously collect data pertaining to production. ML techniques enable the generation of actionable ...
Additive manufacturing (AM) workflow. Note: CAD (Computer-Assisted Design); CAE (Computer-Aided- Engineering). (a) Design: For the development of the parts and products that will be manufactured by additive processes, the first stage consists of the generation of three-dimensional designs by means of computer-assisted design tools (CAD).
A general case study in component or machine design may include the following: a step-by-step proposal of the product, the relevant trade study, the configuration development, the detailed design and construction process, and the prototype tests. Thus, a case study presents a product in action. It covers lessons learned during the development ...
The growing public concern about sustainable development has increased interest in exploring sustainable machining alternatives. However, the absence of a widely accepted method for evaluating the environmental impact of machining processes and a thorough understanding of the requirements for achieving sustainable development has created a pressing need for integrated assessment models.
The general trend to design and manufacture more complicated mechanical components, along with the rapidly moving forward material science, raise the need to incorporate and develop new machining techniques in the manufacturing process. Nonconventional machining processes differ from conventional ones, as they utilize alternative types of ...
Inventory Management. Most manufacturing companies use legacy software such as ERP and supply chain management to try and optimize their supply chain processes. Inventory management is a critical part of the link between production and supply chain management that IIoT facilitates. Real-time transactional data can extend in both directions.
The machinability of difficult-to-cut materials generally refers to the ease of machining. It is common to study either the machinability of difficult-to-cut materials for cutting parameters or cutting tools optimizations (Ref 1).Kumar et al. (Ref 2, 3) compared the machinability in turning AISI D2 steels by using coated and uncoated carbide inserts for selecting proper tool insert.
A case study conducted at a juice production facility in Colima, México, demonstrated how the architecture can be applied to complex processes such as pH control, from simulation to industrial implementation. ... The design process, which includes steps from architecture creation to timing verification, ensures robust and efficient systems ...