Thank you for visiting nature.com. You are using a browser version with limited support for CSS. To obtain the best experience, we recommend you use a more up to date browser (or turn off compatibility mode in Internet Explorer). In the meantime, to ensure continued support, we are displaying the site without styles and JavaScript.
- View all journals
- Explore content
- About the journal
- Publish with us
- Sign up for alerts
- Review Article
- Published: 27 September 2021
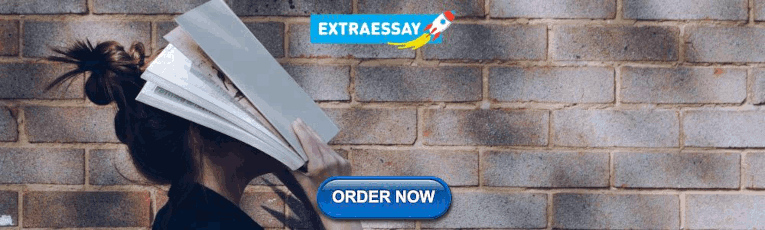
Why lockdown and distance learning during the COVID-19 pandemic are likely to increase the social class achievement gap
- Sébastien Goudeau ORCID: orcid.org/0000-0001-7293-0977 1 ,
- Camille Sanrey ORCID: orcid.org/0000-0003-3158-1306 1 ,
- Arnaud Stanczak ORCID: orcid.org/0000-0002-2596-1516 2 ,
- Antony Manstead ORCID: orcid.org/0000-0001-7540-2096 3 &
- Céline Darnon ORCID: orcid.org/0000-0003-2613-689X 2
Nature Human Behaviour volume 5 , pages 1273–1281 ( 2021 ) Cite this article
112k Accesses
145 Citations
129 Altmetric
Metrics details
The COVID-19 pandemic has forced teachers and parents to quickly adapt to a new educational context: distance learning. Teachers developed online academic material while parents taught the exercises and lessons provided by teachers to their children at home. Considering that the use of digital tools in education has dramatically increased during this crisis, and it is set to continue, there is a pressing need to understand the impact of distance learning. Taking a multidisciplinary view, we argue that by making the learning process rely more than ever on families, rather than on teachers, and by getting students to work predominantly via digital resources, school closures exacerbate social class academic disparities. To address this burning issue, we propose an agenda for future research and outline recommendations to help parents, teachers and policymakers to limit the impact of the lockdown on social-class-based academic inequality.
Similar content being viewed by others
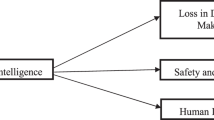
Impact of artificial intelligence on human loss in decision making, laziness and safety in education
Sayed Fayaz Ahmad, Heesup Han, … Antonio Ariza-Montes
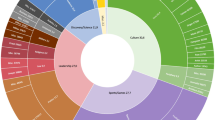
A cross-verified database of notable people, 3500BC-2018AD
Morgane Laouenan, Palaash Bhargava, … Etienne Wasmer
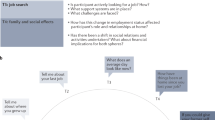
Interviews in the social sciences
Eleanor Knott, Aliya Hamid Rao, … Chana Teeger
The widespread effects of the COVID-19 pandemic that emerged in 2019–2020 have drastically increased health, social and economic inequalities 1 , 2 . For more than 900 million learners around the world, the pandemic led to the closure of schools and universities 3 . This exceptional situation forced teachers, parents and students to quickly adapt to a new educational context: distance learning. Teachers had to develop online academic materials that could be used at home to ensure educational continuity while ensuring the necessary physical distancing. Primary and secondary school students suddenly had to work with various kinds of support, which were usually provided online by their teachers. For college students, lockdown often entailed returning to their hometowns while staying connected with their teachers and classmates via video conferences, email and other digital tools. Despite the best efforts of educational institutions, parents and teachers to keep all children and students engaged in learning activities, ensuring educational continuity during school closure—something that is difficult for everyone—may pose unique material and psychological challenges for working-class families and students.
Not only did the pandemic lead to the closure of schools in many countries, often for several weeks, it also accelerated the digitalization of education and amplified the role of parental involvement in supporting the schoolwork of their children. Thus, beyond the specific circumstances of the COVID-19 lockdown, we believe that studying the effects of the pandemic on academic inequalities provides a way to more broadly examine the consequences of school closure and related effects (for example, digitalization of education) on social class inequalities. Indeed, bearing in mind that (1) the risk of further pandemics is higher than ever (that is, we are in a ‘pandemic era’ 4 , 5 ) and (2) beyond pandemics, the use of digital tools in education (and therefore the influence of parental involvement) has dramatically increased during this crisis, and is set to continue, there is a pressing need for an integrative and comprehensive model that examines the consequences of distance learning. Here, we propose such an integrative model that helps us to understand the extent to which the school closures associated with the pandemic amplify economic, digital and cultural divides that in turn affect the psychological functioning of parents, students and teachers in a way that amplifies academic inequalities. Bringing together research in social sciences, ranging from economics and sociology to social, cultural, cognitive and educational psychology, we argue that by getting students to work predominantly via digital resources rather than direct interactions with their teachers, and by making the learning process rely more than ever on families rather than teachers, school closures exacerbate social class academic disparities.
First, we review research showing that social class is associated with unequal access to digital tools, unequal familiarity with digital skills and unequal uses of such tools for learning purposes 6 , 7 . We then review research documenting how unequal familiarity with school culture, knowledge and skills can also contribute to the accentuation of academic inequalities 8 , 9 . Next, we present the results of surveys conducted during the 2020 lockdown showing that the quality and quantity of pedagogical support received from schools varied according to the social class of families (for examples, see refs. 10 , 11 , 12 ). We then argue that these digital, cultural and structural divides represent barriers to the ability of parents to provide appropriate support for children during distance learning (Fig. 1 ). These divides also alter the levels of self-efficacy of parents and children, thereby affecting their engagement in learning activities 13 , 14 . In the final section, we review preliminary evidence for the hypothesis that distance learning widens the social class achievement gap and we propose an agenda for future research. In addition, we outline recommendations that should help parents, teachers and policymakers to use social science research to limit the impact of school closure and distance learning on the social class achievement gap.
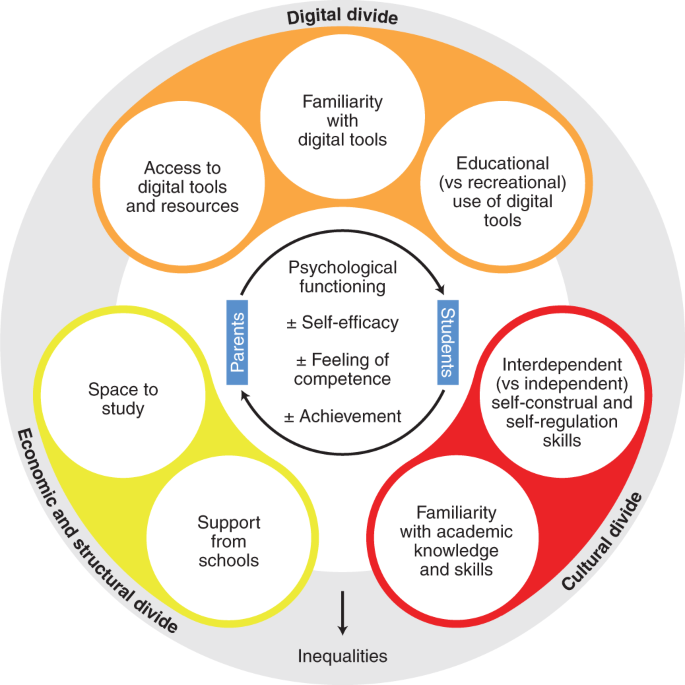
Economic, structural, digital and cultural divides influence the psychological functioning of parents and students in a way that amplify inequalities.
The digital divide
Unequal access to digital resources.
Although the use of digital technologies is almost ubiquitous in developed nations, there is a digital divide such that some people are more likely than others to be numerically excluded 15 (Fig. 1 ). Social class is a strong predictor of digital disparities, including the quality of hardware, software and Internet access 16 , 17 , 18 . For example, in 2019, in France, around 1 in 5 working-class families did not have personal access to the Internet compared with less than 1 in 20 of the most privileged families 19 . Similarly, in 2020, in the United Kingdom, 20% of children who were eligible for free school meals did not have access to a computer at home compared with 7% of other children 20 . In 2021, in the United States, 41% of working-class families do not own a laptop or desktop computer and 43% do not have broadband compared with 8% and 7%, respectively, of upper/middle-class Americans 21 . A similar digital gap is also evident between lower-income and higher-income countries 22 .
Second, simply having access to a computer and an Internet connection does not ensure effective distance learning. For example, many of the educational resources sent by teachers need to be printed, thereby requiring access to printers. Moreover, distance learning is more difficult in households with only one shared computer compared with those where each family member has their own 23 . Furthermore, upper/middle-class families are more likely to be able to guarantee a suitable workspace for each child than their working-class counterparts 24 .
In the context of school closures, such disparities are likely to have important consequences for educational continuity. In line with this idea, a survey of approximately 4,000 parents in the United Kingdom confirmed that during lockdown, more than half of primary school children from the poorest families did not have access to their own study space and were less well equipped for distance learning than higher-income families 10 . Similarly, a survey of around 1,300 parents in the Netherlands found that during lockdown, children from working-class families had fewer computers at home and less room to study than upper/middle-class children 11 .
Data from non-Western countries highlight a more general digital divide, showing that developing countries have poorer access to digital equipment. For example, in India in 2018, only 10.7% of households possessed a digital device 25 , while in Pakistan in 2020, 31% of higher-education teachers did not have Internet access and 68.4% did not have a laptop 26 . In general, developing countries lack access to digital technologies 27 , 28 , and these difficulties of access are even greater in rural areas (for example, see ref. 29 ). Consequently, school closures have huge repercussions for the continuity of learning in these countries. For example, in India in 2018, only 11% of the rural and 40% of the urban population above 14 years old could use a computer and access the Internet 25 . Time spent on education during school closure decreased by 80% in Bangladesh 30 . A similar trend was observed in other countries 31 , with only 22% of children engaging in remote learning in Kenya 32 and 50% in Burkina Faso 33 . In Ghana, 26–32% of children spent no time at all on learning during the pandemic 34 . Beyond the overall digital divide, social class disparities are also evident in developing countries, with lower access to digital resources among households in which parental educational levels were low (versus households in which parental educational levels were high; for example, see ref. 35 for Nigeria and ref. 31 for Ecuador).
Unequal digital skills
In addition to unequal access to digital tools, there are also systematic variations in digital skills 36 , 37 (Fig. 1 ). Upper/middle-class families are more familiar with digital tools and resources and are therefore more likely to have the digital skills needed for distance learning 38 , 39 , 40 . These digital skills are particularly useful during school closures, both for students and for parents, for organizing, retrieving and correctly using the resources provided by the teachers (for example, sending or receiving documents by email, printing documents or using word processors).
Social class disparities in digital skills can be explained in part by the fact that children from upper/middle-class families have the opportunity to develop digital skills earlier than working-class families 41 . In member countries of the OECD (Organisation for Economic Co-operation and Development), only 23% of working-class children had started using a computer at the age of 6 years or earlier compared with 43% of upper/middle-class children 42 . Moreover, because working-class people tend to persist less than upper/middle-class people when confronted with digital difficulties 23 , the use of digital tools and resources for distance learning may interfere with the ability of parents to help children with their schoolwork.
Unequal use of digital tools
A third level of digital divide concerns variations in digital tool use 18 , 43 (Fig. 1 ). Upper/middle-class families are more likely to use digital resources for work and education 6 , 41 , 44 , whereas working-class families are more likely to use these resources for entertainment, such as electronic games or social media 6 , 45 . This divide is also observed among students, whereby working-class students tend to use digital technologies for leisure activities, whereas their upper/middle-class peers are more likely to use them for academic activities 46 and to consider that computers and the Internet provide an opportunity for education and training 23 . Furthermore, working-class families appear to regulate the digital practices of their children less 47 and are more likely to allow screens in the bedrooms of children and teenagers without setting limits on times or practices 48 .
In sum, inequalities in terms of digital resources, skills and use have strong implications for distance learning. This is because they make working-class students and parents particularly vulnerable when learning relies on extensive use of digital devices rather than on face-to-face interaction with teachers.
The cultural divide
Even if all three levels of digital divide were closed, upper/middle-class families would still be better prepared than working-class families to ensure educational continuity for their children. Upper/middle-class families are more familiar with the academic knowledge and skills that are expected and valued in educational settings, as well as with the independent, autonomous way of learning that is valued in the school culture and becomes even more important during school closure (Fig. 1 ).
Unequal familiarity with academic knowledge and skills
According to classical social reproduction theory 8 , 49 , school is not a neutral place in which all forms of language and knowledge are equally valued. Academic contexts expect and value culture-specific and taken-for-granted forms of knowledge, skills and ways of being, thinking and speaking that are more in tune with those developed through upper/middle-class socialization (that is, ‘cultural capital’ 8 , 50 , 51 , 52 , 53 ). For instance, academic contexts value interest in the arts, museums and literature 54 , 55 , a type of interest that is more likely to develop through socialization in upper/middle-class families than in working-class socialization 54 , 56 . Indeed, upper/middle-class parents are more likely than working-class parents to engage in activities that develop this cultural capital. For example, they possess more books and cultural objects at home, read more stories to their children and visit museums and libraries more often (for examples, see refs. 51 , 54 , 55 ). Upper/middle-class children are also more involved in extra-curricular activities (for example, playing a musical instrument) than working-class children 55 , 56 , 57 .
Beyond this implicit familiarization with the school curriculum, upper/middle-class parents more often organize educational activities that are explicitly designed to develop academic skills of their children 57 , 58 , 59 . For example, they are more likely to monitor and re-explain lessons or use games and textbooks to develop and reinforce academic skills (for example, labelling numbers, letters or colours 57 , 60 ). Upper/middle-class parents also provide higher levels of support and spend more time helping children with homework than working-class parents (for examples, see refs. 61 , 62 ). Thus, even if all parents are committed to the academic success of their children, working-class parents have fewer chances to provide the help that children need to complete homework 63 , and homework is more beneficial for children from upper-middle class families than for children from working-class families 64 , 65 .
School closures amplify the impact of cultural inequalities
The trends described above have been observed in ‘normal’ times when schools are open. School closures, by making learning rely more strongly on practices implemented at home (rather than at school), are likely to amplify the impact of these disparities. Consistent with this idea, research has shown that the social class achievement gap usually greatly widens during school breaks—a phenomenon described as ‘summer learning loss’ or ‘summer setback’ 66 , 67 , 68 . During holidays, the learning by children tends to decline, and this is particularly pronounced in children from working-class families. Consequently, the social class achievement gap grows more rapidly during the summer months than it does in the rest of the year. This phenomenon is partly explained by the fact that during the break from school, social class disparities in investment in activities that are beneficial for academic achievement (for example, reading, travelling to a foreign country or museum visits) are more pronounced.
Therefore, when they are out of school, children from upper/middle-class backgrounds may continue to develop academic skills unlike their working-class counterparts, who may stagnate or even regress. Research also indicates that learning loss during school breaks tends to be cumulative 66 . Thus, repeated episodes of school closure are likely to have profound consequences for the social class achievement gap. Consistent with the idea that school closures could lead to similar processes as those identified during summer breaks, a recent survey indicated that during the COVID-19 lockdown in the United Kingdom, children from upper/middle-class families spent more time on educational activities (5.8 h per day) than those from working-class families (4.5 h per day) 7 , 69 .
Unequal dispositions for autonomy and self-regulation
School closures have encouraged autonomous work among students. This ‘independent’ way of studying is compatible with the family socialization of upper/middle-class students, but does not match the interdependent norms more commonly associated with working-class contexts 9 . Upper/middle-class contexts tend to promote cultural norms of independence whereby individuals perceive themselves as autonomous actors, independent of other individuals and of the social context, able to pursue their own goals 70 . For example, upper/middle-class parents tend to invite children to express their interests, preferences and opinions during the various activities of everyday life 54 , 55 . Conversely, in working-class contexts characterized by low economic resources and where life is more uncertain, individuals tend to perceive themselves as interdependent, connected to others and members of social groups 53 , 70 , 71 . This interdependent self-construal fits less well with the independent culture of academic contexts. This cultural mismatch between interdependent self-construal common in working-class students and the independent norms of the educational institution has negative consequences for academic performance 9 .
Once again, the impact of these differences is likely to be amplified during school closures, when being able to work alone and autonomously is especially useful. The requirement to work alone is more likely to match the independent self-construal of upper/middle-class students than the interdependent self-construal of working-class students. In the case of working-class students, this mismatch is likely to increase their difficulties in working alone at home. Supporting our argument, recent research has shown that working-class students tend to underachieve in contexts where students work individually compared with contexts where students work with others 72 . Similarly, during school closures, high self-regulation skills (for example, setting goals, selecting appropriate learning strategies and maintaining motivation 73 ) are required to maintain study activities and are likely to be especially useful for using digital resources efficiently. Research has shown that students from working-class backgrounds typically develop their self-regulation skills to a lesser extent than those from upper/middle-class backgrounds 74 , 75 , 76 .
Interestingly, some authors have suggested that independent (versus interdependent) self-construal may also affect communication with teachers 77 . Indeed, in the context of distance learning, working-class families are less likely to respond to the communication of teachers because their ‘interdependent’ self leads them to respect hierarchies, and thus perceive teachers as an expert who ‘can be trusted to make the right decisions for learning’. Upper/middle class families, relying on ‘independent’ self-construal, are more inclined to seek individualized feedback, and therefore tend to participate to a greater extent in exchanges with teachers. Such cultural differences are important because they can also contribute to the difficulties encountered by working-class families.
The structural divide: unequal support from schools
The issues reviewed thus far all increase the vulnerability of children and students from underprivileged backgrounds when schools are closed. To offset these disadvantages, it might be expected that the school should increase its support by providing additional resources for working-class students. However, recent data suggest that differences in the material and human resources invested in providing educational support for children during periods of school closure were—paradoxically—in favour of upper/middle-class students (Fig. 1 ). In England, for example, upper/middle-class parents reported benefiting from online classes and video-conferencing with teachers more often than working-class parents 10 . Furthermore, active help from school (for example, online teaching, private tutoring or chats with teachers) occurred more frequently in the richest households (64% of the richest households declared having received help from school) than in the poorest households (47%). Another survey found that in the United Kingdom, upper/middle-class children were more likely to take online lessons every day (30%) than working-class students (16%) 12 . This substantial difference might be due, at least in part, to the fact that private schools are better equipped in terms of online platforms (60% of schools have at least one online platform) than state schools (37%, and 23% in the most deprived schools) and were more likely to organize daily online lessons. Similarly, in the United Kingdom, in schools with a high proportion of students eligible for free school meals, teachers were less inclined to broadcast an online lesson for their pupils 78 . Interestingly, 58% of teachers in the wealthiest areas reported having messaged their students or their students’ parents during lockdown compared with 47% in the most deprived schools. In addition, the probability of children receiving technical support from the school (for example, by providing pupils with laptops or other devices) is, surprisingly, higher in the most advantaged schools than in the most deprived 78 .
In addition to social class disparities, there has been less support from schools for African-American and Latinx students. During school closures in the United States, 40% of African-American students and 30% of Latinx students received no online teaching compared with 10% of white students 79 . Another source of inequality is that the probability of school closure was correlated with social class and race. In the United States, for example, school closures from September to December 2020 were more common in schools with a high proportion of racial/ethnic minority students, who experience homelessness and are eligible for free/discounted school meals 80 .
Similarly, access to educational resources and support was lower in poorer (compared with richer) countries 81 . In sub-Saharan Africa, during lockdown, 45% of children had no exposure at all to any type of remote learning. Of those who did, the medium was mostly radio, television or paper rather than digital. In African countries, at most 10% of children received some material through the Internet. In Latin America, 90% of children received some remote learning, but less than half of that was through the internet—the remainder being via radio and television 81 . In Ecuador, high-school students from the lowest wealth quartile had fewer remote-learning opportunities, such as Google class/Zoom, than students from the highest wealth quartile 31 .
Thus, the achievement gap and its accentuation during lockdown are due not only to the cultural and digital disadvantages of working-class families but also to unequal support from schools. This inequality in school support is not due to teachers being indifferent to or even supportive of social stratification. Rather, we believe that these effects are fundamentally structural. In many countries, schools located in upper/middle-class neighbourhoods have more money than those in the poorest neighbourhoods. Moreover, upper/middle-class parents invest more in the schools of their children than working-class parents (for example, see ref. 82 ), and schools have an interest in catering more for upper/middle-class families than for working-class families 83 . Additionally, the expectation of teachers may be lower for working-class children 84 . For example, they tend to estimate that working-class students invest less effort in learning than their upper/middle-class counterparts 85 . These differences in perception may have influenced the behaviour of teachers during school closure, such that teachers in privileged neighbourhoods provided more information to students because they expected more from them in term of effort and achievement. The fact that upper/middle-class parents are better able than working-class parents to comply with the expectations of teachers (for examples, see refs. 55 , 86 ) may have reinforced this phenomenon. These discrepancies echo data showing that working-class students tend to request less help in their schoolwork than upper/middle-class ones 87 , and they may even avoid asking for help because they believe that such requests could lead to reprimands 88 . During school closures, these students (and their families) may in consequence have been less likely to ask for help and resources. Jointly, these phenomena have resulted in upper/middle-class families receiving more support from schools during lockdown than their working-class counterparts.
Psychological effects of digital, cultural and structural divides
Despite being strongly influenced by social class, differences in academic achievement are often interpreted by parents, teachers and students as reflecting differences in ability 89 . As a result, upper/middle-class students are usually perceived—and perceive themselves—as smarter than working-class students, who are perceived—and perceive themselves—as less intelligent 90 , 91 , 92 or less able to succeed 93 . Working-class students also worry more about the fact that they might perform more poorly than upper/middle-class students 94 , 95 . These fears influence academic learning in important ways. In particular, they can consume cognitive resources when children and students work on academic tasks 96 , 97 . Self-efficacy also plays a key role in engaging in learning and perseverance in the face of difficulties 13 , 98 . In addition, working-class students are those for whom the fear of being outperformed by others is the most negatively related to academic performance 99 .
The fact that working-class children and students are less familiar with the tasks set by teachers, and less well equipped and supported, makes them more likely to experience feelings of incompetence (Fig. 1 ). Working-class parents are also more likely than their upper/middle-class counterparts to feel unable to help their children with schoolwork. Consistent with this, research has shown that both working-class students and parents have lower feelings of academic self-efficacy than their upper/middle-class counterparts 100 , 101 . These differences have been documented under ‘normal’ conditions but are likely to be exacerbated during distance learning. Recent surveys conducted during the school closures have confirmed that upper/middle-class families felt better able to support their children in distance learning than did working-class families 10 and that upper/middle-class parents helped their children more and felt more capable to do so 11 , 12 .
Pandemic disparity, future directions and recommendations
The research reviewed thus far suggests that children and their families are highly unequal with respect to digital access, skills and use. It also shows that upper/middle-class students are more likely to be supported in their homework (by their parents and teachers) than working-class students, and that upper/middle-class students and parents will probably feel better able than working-class ones to adapt to the context of distance learning. For all these reasons, we anticipate that as a result of school closures, the COVID-19 pandemic will substantially increase the social class achievement gap. Because school closures are a recent occurrence, it is too early to measure with precision their effects on the widening of the achievement gap. However, some recent data are consistent with this idea.
Evidence for a widening gap during the pandemic
Comparing academic achievement in 2020 with previous years provides an early indication of the effects of school closures during the pandemic. In France, for example, first and second graders take national evaluations at the beginning of the school year. Initial comparisons of the results for 2020 with those from previous years revealed that the gap between schools classified as ‘priority schools’ (those in low-income urban areas) and schools in higher-income neighbourhoods—a gap observed every year—was particularly pronounced in 2020 in both French and mathematics 102 .
Similarly, in the Netherlands, national assessments take place twice a year. In 2020, they took place both before and after school closures. A recent analysis compared progress during this period in 2020 in mathematics/arithmetic, spelling and reading comprehension for 7–11-year-old students within the same period in the three previous years 103 . Results indicated a general learning loss in 2020. More importantly, for the 8% of working-class children, the losses were 40% greater than they were for upper/middle-class children.
Similar results were observed in Belgium among students attending the final year of primary school. Compared with students from previous cohorts, students affected by school closures experienced a substantial decrease in their mathematics and language scores, with children from more disadvantaged backgrounds experiencing greater learning losses 104 . Likewise, oral reading assessments in more than 100 school districts in the United States showed that the development of this skill among children in second and third grade significantly slowed between Spring and Autumn 2020, but this slowdown was more pronounced in schools from lower-achieving districts 105 .
It is likely that school closures have also amplified racial disparities in learning and achievement. For example, in the United States, after the first lockdown, students of colour lost the equivalent of 3–5 months of learning, whereas white students were about 1–3 months behind. Moreover, in the Autumn, when some students started to return to classrooms, African-American and Latinx students were more likely to continue distance learning, despite being less likely to have access to the digital tools, Internet access and live contact with teachers 106 .
In some African countries (for example, Ethiopia, Kenya, Liberia, Tanzania and Uganda), the COVID-19 crisis has resulted in learning loss ranging from 6 months to more 1 year 107 , and this learning loss appears to be greater for working-class children (that is, those attending no-fee schools) than for upper/middle-class children 108 .
These findings show that school closures have exacerbated achievement gaps linked to social class and ethnicity. However, more research is needed to address the question of whether school closures differentially affect the learning of students from working- and upper/middle-class families.
Future directions
First, to assess the specific and unique impact of school closures on student learning, longitudinal research should compare student achievement at different times of the year, before, during and after school closures, as has been done to document the summer learning loss 66 , 109 . In the coming months, alternating periods of school closure and opening may occur, thereby presenting opportunities to do such research. This would also make it possible to examine whether the gap diminishes a few weeks after children return to in-school learning or whether, conversely, it increases with time because the foundations have not been sufficiently acquired to facilitate further learning 110 .
Second, the mechanisms underlying the increase in social class disparities during school closures should be examined. As discussed above, school closures result in situations for which students are unevenly prepared and supported. It would be appropriate to seek to quantify the contribution of each of the factors that might be responsible for accentuating the social class achievement gap. In particular, distinguishing between factors that are relatively ‘controllable’ (for example, resources made available to pupils) and those that are more difficult to control (for example, the self-efficacy of parents in supporting the schoolwork of their children) is essential to inform public policy and teaching practices.
Third, existing studies are based on general comparisons and very few provide insights into the actual practices that took place in families during school closure and how these practices affected the achievement gap. For example, research has documented that parents from working-class backgrounds are likely to find it more difficult to help their children to complete homework and to provide constructive feedback 63 , 111 , something that could in turn have a negative impact on the continuity of learning of their children. In addition, it seems reasonable to assume that during lockdown, parents from upper/middle-class backgrounds encouraged their children to engage in practices that, even if not explicitly requested by teachers, would be beneficial to learning (for example, creative activities or reading). Identifying the practices that best predict the maintenance or decline of educational achievement during school closures would help identify levers for intervention.
Finally, it would be interesting to investigate teaching practices during school closures. The lockdown in the spring of 2020 was sudden and unexpected. Within a few days, teachers had to find a way to compensate for the school closure, which led to highly variable practices. Some teachers posted schoolwork on platforms, others sent it by email, some set work on a weekly basis while others set it day by day. Some teachers also set up live sessions in large or small groups, providing remote meetings for questions and support. There have also been variations in the type of feedback given to students, notably through the monitoring and correcting of work. Future studies should examine in more detail what practices schools and teachers used to compensate for the school closures and their effects on widening, maintaining or even reducing the gap, as has been done for certain specific literacy programmes 112 as well as specific instruction topics (for example, ecology and evolution 113 ).
Practical recommendations
We are aware of the debate about whether social science research on COVID-19 is suitable for making policy decisions 114 , and we draw attention to the fact that some of our recommendations (Table 1 ) are based on evidence from experiments or interventions carried out pre-COVID while others are more speculative. In any case, we emphasize that these suggestions should be viewed with caution and be tested in future research. Some of our recommendations could be implemented in the event of new school closures, others only when schools re-open. We also acknowledge that while these recommendations are intended for parents and teachers, their implementation largely depends on the adoption of structural policies. Importantly, given all the issues discussed above, we emphasize the importance of prioritizing, wherever possible, in-person learning over remote learning 115 and where this is not possible, of implementing strong policies to support distance learning, especially for disadvantaged families.
Where face-to face teaching is not possible and teachers are responsible for implementing distance learning, it will be important to make them aware of the factors that can exacerbate inequalities during lockdown and to provide them with guidance about practices that would reduce these inequalities. Thus, there is an urgent need for interventions aimed at making teachers aware of the impact of the social class of children and families on the following factors: (1) access to, familiarity with and use of digital devices; (2) familiarity with academic knowledge and skills; and (3) preparedness to work autonomously. Increasing awareness of the material, cultural and psychological barriers that working-class children and families face during lockdown should increase the quality and quantity of the support provided by teachers and thereby positively affect the achievements of working-class students.
In addition to increasing the awareness of teachers of these barriers, teachers should be encouraged to adjust the way they communicate with working-class families due to differences in self-construal compared with upper/middle-class families 77 . For example, questions about family (rather than personal) well-being would be congruent with interdependent self-construals. This should contribute to better communication and help keep a better track of the progress of students during distance learning.
It is also necessary to help teachers to engage in practices that have a chance of reducing inequalities 53 , 116 . Particularly important is that teachers and schools ensure that homework can be done by all children, for example, by setting up organizations that would help children whose parents are not in a position to monitor or assist with the homework of their children. Options include homework help groups and tutoring by teachers after class. When schools are open, the growing tendency to set homework through digital media should be resisted as far as possible given the evidence we have reviewed above. Moreover, previous research has underscored the importance of homework feedback provided by teachers, which is positively related to the amount of homework completed and predictive of academic performance 117 . Where homework is web-based, it has also been shown that feedback on web-based homework enhances the learning of students 118 . It therefore seems reasonable to predict that the social class achievement gap will increase more slowly (or even remain constant or be reversed) in schools that establish individualized monitoring of students, by means of regular calls and feedback on homework, compared with schools where the support provided to pupils is more generic.
Given that learning during lockdown has increasingly taken place in family settings, we believe that interventions involving the family are also likely to be effective 119 , 120 , 121 . Simply providing families with suitable material equipment may be insufficient. Families should be given training in the efficient use of digital technology and pedagogical support. This would increase the self-efficacy of parents and students, with positive consequences for achievement. Ideally, such training would be delivered in person to avoid problems arising from the digital divide. Where this is not possible, individualized online tutoring should be provided. For example, studies conducted during the lockdown in Botswana and Italy have shown that individual online tutoring directly targeting either parents or students in middle school has a positive impact on the achievement of students, particularly for working-class students 122 , 123 .
Interventions targeting families should also address the psychological barriers faced by working-class families and children. Some interventions have already been designed and been shown to be effective in reducing the social class achievement gap, particularly in mathematics and language 124 , 125 , 126 . For example, research showed that an intervention designed to train low-income parents in how to support the mathematical development of their pre-kindergarten children (including classes and access to a library of kits to use at home) increased the quality of support provided by the parents, with a corresponding impact on the development of mathematical knowledge of their children. Such interventions should be particularly beneficial in the context of school closure.
Beyond its impact on academic performance and inequalities, the COVID-19 crisis has shaken the economies of countries around the world, casting millions of families around the world into poverty 127 , 128 , 129 . As noted earlier, there has been a marked increase in economic inequalities, bringing with it all the psychological and social problems that such inequalities create 130 , 131 , especially for people who live in scarcity 132 . The increase in educational inequalities is just one facet of the many difficulties that working-class families will encounter in the coming years, but it is one that could seriously limit the chances of their children escaping from poverty by reducing their opportunities for upward mobility. In this context, it should be a priority to concentrate resources on the most deprived students. A large proportion of the poorest households do not own a computer and do not have personal access to the Internet, which has important consequences for distance learning. During school closures, it is therefore imperative to provide such families with adequate equipment and Internet service, as was done in some countries in spring 2020. Even if the provision of such equipment is not in itself sufficient, it is a necessary condition for ensuring pedagogical continuity during lockdown.
Finally, after prolonged periods of school closure, many students may not have acquired the skills needed to pursue their education. A possible consequence would be an increase in the number of students for whom teachers recommend class repetitions. Class repetitions are contentious. On the one hand, class repetition more frequently affects working-class children and is not efficient in terms of learning improvement 133 . On the other hand, accepting lower standards of academic achievement or even suspending the practice of repeating a class could lead to pupils pursuing their education without mastering the key abilities needed at higher grades. This could create difficulties in subsequent years and, in this sense, be counterproductive. We therefore believe that the most appropriate way to limit the damage of the pandemic would be to help children catch up rather than allowing them to continue without mastering the necessary skills. As is being done in some countries, systematic remedial courses (for example, summer learning programmes) should be organized and financially supported following periods of school closure, with priority given to pupils from working-class families. Such interventions have genuine potential in that research has shown that participation in remedial summer programmes is effective in reducing learning loss during the summer break 134 , 135 , 136 . For example, in one study 137 , 438 students from high-poverty schools were offered a multiyear summer school programme that included various pedagogical and enrichment activities (for example, science investigation and music) and were compared with a ‘no-treatment’ control group. Students who participated in the summer programme progressed more than students in the control group. A meta-analysis 138 of 41 summer learning programmes (that is, classroom- and home-based summer interventions) involving children from kindergarten to grade 8 showed that these programmes had significantly larger benefits for children from working-class families. Although such measures are costly, the cost is small compared to the price of failing to fulfil the academic potential of many students simply because they were not born into upper/middle-class families.
The unprecedented nature of the current pandemic means that we lack strong data on what the school closure period is likely to produce in terms of learning deficits and the reproduction of social inequalities. However, the research discussed in this article suggests that there are good reasons to predict that this period of school closures will accelerate the reproduction of social inequalities in educational achievement.
By making school learning less dependent on teachers and more dependent on families and digital tools and resources, school closures are likely to greatly amplify social class inequalities. At a time when many countries are experiencing second, third or fourth waves of the pandemic, resulting in fresh periods of local or general lockdowns, systematic efforts to test these predictions are urgently needed along with steps to reduce the impact of school closures on the social class achievement gap.
Bambra, C., Riordan, R., Ford, J. & Matthews, F. The COVID-19 pandemic and health inequalities. J. Epidemiol. Commun. Health 74 , 964–968 (2020).
Google Scholar
Johnson, P, Joyce, R & Platt, L. The IFS Deaton Review of Inequalities: A New Year’s Message (Institute for Fiscal Studies, 2021).
Education: from disruption to recovery. https://en.unesco.org/covid19/educationresponse (UNESCO, 2020).
Daszak, P. We are entering an era of pandemics—it will end only when we protect the rainforest. The Guardian (28 July 2020); https://www.theguardian.com/commentisfree/2020/jul/28/pandemic-era-rainforest-deforestation-exploitation-wildlife-disease
Dobson, A. P. et al. Ecology and economics for pandemic prevention. Science 369 , 379–381 (2020).
Article CAS PubMed Google Scholar
Harris, C., Straker, L. & Pollock, C. A socioeconomic related ‘digital divide’ exists in how, not if, young people use computers. PLoS ONE 12 , e0175011 (2017).
Article PubMed PubMed Central Google Scholar
Zhang, M. Internet use that reproduces educational inequalities: evidence from big data. Comput. Educ. 86 , 212–223 (2015).
Article Google Scholar
Bourdieu, P. & Passeron, J. C. Reproduction in Education, Society and Culture (Sage, 1990).
Stephens, N. M., Fryberg, S. A., Markus, H. R., Johnson, C. S. & Covarrubias, R. Unseen disadvantage: how American universities’ focus on independence undermines the academic performance of first-generation college students. J. Pers. Soc. Psychol. 102 , 1178–1197 (2012).
Article PubMed Google Scholar
Andrew, A. et al. Inequalities in children’s experiences of home learning during the COVID-19 lockdown in England. Fisc. Stud. 41 , 653–683 (2020).
Bol, T. Inequality in homeschooling during the Corona crisis in the Netherlands. First results from the LISS Panel. Preprint at SocArXiv https://doi.org/10.31235/osf.io/hf32q (2020).
Cullinane, C. & Montacute, R. COVID-19 and Social Mobility. Impact Brief #1: School Shutdown (The Sutton Trust, 2020).
Bandura, A. Self-efficacy: toward a unifying theory of behavioral change. Psychol. Rev. 84 , 191–215 (1977).
Prior, D. D., Mazanov, J., Meacheam, D., Heaslip, G. & Hanson, J. Attitude, digital literacy and self efficacy: low-on effects for online learning behavior. Internet High. Educ. 29 , 91–97 (2016).
Robinson, L. et al. Digital inequalities 2.0: legacy inequalities in the information age. First Monday https://doi.org/10.5210/fm.v25i7.10842 (2020).
Cruz-Jesus, F., Vicente, M. R., Bacao, F. & Oliveira, T. The education-related digital divide: an analysis for the EU-28. Comput. Hum. Behav. 56 , 72–82 (2016).
Rice, R. E. & Haythornthwaite, C. In The Handbook of New Media (eds Lievrouw, L. A. & Livingstone S. M.), 92–113 (Sage, 2006).
Yates, S., Kirby, J. & Lockley, E. Digital media use: differences and inequalities in relation to class and age. Sociol. Res. Online 20 , 71–91 (2015).
Legleye, S. & Rolland, A. Une personne sur six n’utilise pas Internet, plus d’un usager sur trois manques de compétences numériques de base [One in six people do not use the Internet, more than one in three users lack basic digital skills] (INSEE Première, 2019).
Green, F. Schoolwork in lockdown: new evidence on the epidemic of educational poverty (LLAKES Centre, 2020); https://www.llakes.ac.uk/wp-content/uploads/2021/03/RP-67-Francis-Green-Research-Paper-combined-file.pdf
Vogels, E. Digital divide persists even as americans with lower incomes make gains in tech adoption (Pew Research Center, 2021); https://www.pewresearch.org/fact-tank/2021/06/22/digital-divide-persists-even-as-americans-with-lower-incomes-make-gains-in-tech-adoption/
McBurnie, C., Adam, T. & Kaye, T. Is there learning continuity during the COVID-19 pandemic? A synthesis of the emerging evidence. J. Learn. Develop. http://dspace.col.org/handle/11599/3720 (2020).
Baillet, J., Croutte, P. & Prieur, V. Baromètre du numérique 2019 [Digital barometer 2019] (Sourcing Crédoc, 2019).
Giraud, F., Bertrand, J., Court, M. & Nicaise, S. In Enfances de Classes. De l’inégalité Parmi les Enfants (ed. Lahire, B.) 933–952 (Seuil, 2019).
Ahamed, S. & Siddiqui, Z. Disparity in access to quality education and the digital divide (Ideas for India, 2020); https://www.ideasforindia.in/topics/macroeconomics/disparity-in-access-to-quality-education-and-the-digital-divide.html
Soomro, K. A., Kale, U., Curtis, R., Akcaoglu, M. & Bernstein, M. Digital divide among higher education faculty. Int. J. Educ. Tech. High. Ed. 17 , 21 (2020).
Meng, Q. & Li, M. New economy and ICT development in China. Inf. Econ. Policy 14 , 275–295 (2002).
Chinn, M. D. & Fairlie, R. W. The determinants of the global digital divide: a cross-country analysis of computer and internet penetration. Oxf. Econ. Pap. 59 , 16–44 (2006).
Lembani, R., Gunter, A., Breines, M. & Dalu, M. T. B. The same course, different access: the digital divide between urban and rural distance education students in South Africa. J. Geogr. High. Educ. 44 , 70–84 (2020).
Asadullah, N., Bhattacharjee, A., Tasnim, M. & Mumtahena, F. COVID-19, schooling, and learning (BRAC Institute of Governance & Development, 2020); https://bigd.bracu.ac.bd/wp-content/uploads/2020/06/COVID-19-Schooling-and-Learning_June-25-2020.pdf
Asanov, I., Flores, F., McKenzie, D., Mensmann, M. & Schulte, M. Remote-learning, time-use, and mental health of Ecuadorian high-school students during the COVID-19 quarantine. World Dev. 138 , 105225 (2021).
Kihui, N. Kenya: 80% of students missing virtual learning amid school closures—study. AllAfrica (18 May 2020); https://allafrica.com/stories/202005180774.html
Debenedetti, L., Hirji, S., Chabi, M. O. & Swigart, T. Prioritizing evidence-based responses in Burkina Faso to mitigate the economic effects of COVID-19: lessons from RECOVR (Innovations for Poverty Action, 2020); https://www.poverty-action.org/blog/prioritizing-evidence-based-responses-burkina-faso-mitigate-economic-effects-covid-19-lessons
Bosumtwi-Sam, C. & Kabay, S. Using data and evidence to inform school reopening in Ghana (Innovations for Poverty Action, 2020); https://www.poverty-action.org/blog/using-data-and-evidence-inform-school-reopening-ghana
Azubuike, O. B., Adegboye, O. & Quadri, H. Who gets to learn in a pandemic? Exploring the digital divide in remote learning during the COVID-19 pandemic in Nigeria. Int. J. Educ. Res. Open 2 , 100022 (2021).
Attewell, P. Comment: the first and second digital divides. Sociol. Educ. 74 , 252–259 (2001).
DiMaggio, P., Hargittai, E., Neuman, W. R. & Robinson, J. P. Social implications of the Internet. Annu. Rev. Sociol. 27 , 307–336 (2001).
Hargittai, E. Digital na(t)ives? Variation in Internet skills and uses among members of the ‘Net Generation’. Sociol. Inq. 80 , 92–113 (2010).
Iivari, N., Sharma, S. & Ventä-Olkkonen, L. Digital transformation of everyday life—how COVID-19 pandemic transformed the basic education of the young generation and why information management research should care? Int. J. Inform. Manag. 55 , 102183 (2020).
Wei, L. & Hindman, D. B. Does the digital divide matter more? Comparing the effects of new media and old media use on the education-based knowledge gap. Mass Commun. Soc. 14 , 216–235 (2011).
Octobre, S. & Berthomier, N. L’enfance des loisirs [The childhood of leisure]. Cult. Études 6 , 1–12 (2011).
Education at a glance 2015: OECD indicators (OECD, 2015); https://doi.org/10.1787/eag-2015-en
North, S., Snyder, I. & Bulfin, S. Digital tastes: social class and young people’s technology use. Inform. Commun. Soc. 11 , 895–911 (2008).
Robinson, L. & Schulz, J. Net time negotiations within the family. Inform. Commun. Soc. 16 , 542–560 (2013).
Bonfadelli, H. The Internet and knowledge gaps: a theoretical and empirical investigation. Eur. J. Commun. 17 , 65–84 (2002).
Drabowicz, T. Social theory of Internet use: corroboration or rejection among the digital natives? Correspondence analysis of adolescents in two societies. Comput. Educ. 105 , 57–67 (2017).
Nikken, P. & Jansz, J. Developing scales to measure parental mediation of young children’s Internet use. Learn. Media Technol. 39 , 250–266 (2014).
Danic, I., Fontar, B., Grimault-Leprince, A., Le Mentec, M. & David, O. Les espaces de construction des inégalités éducatives [The areas of construction of educational inequalities] (Presses Univ. de Rennes, 2019).
Goudeau, S. Comment l'école reproduit-elle les inégalités? [How does school reproduce inequalities?] (Univ. Grenoble Alpes Editions/Presses Univ. de Grenoble, 2020).
Bernstein, B. Class, Codes, and Control (Routledge, 1975).
Gaddis, S. M. The influence of habitus in the relationship between cultural capital and academic achievement. Soc. Sci. Res. 42 , 1–13 (2013).
Lamont, M. & Lareau, A. Cultural capital: allusions, gaps and glissandos in recent theoretical developments. Sociol. Theory 6 , 153–168 (1988).
Stephens, N. M., Markus, H. R. & Phillips, L. T. Social class culture cycles: how three gateway contexts shape selves and fuel inequality. Annu. Rev. Psychol. 65 , 611–634 (2014).
Lahire, B. Enfances de classe. De l’inégalité parmi les enfants [Social class childhood. Inequality among children] (Le Seuil, 2019).
Lareau, A. Unequal Childhoods: Class, Race, and Family Life (Univ. of California Press, 2003).
Bourdieu, P. La distinction. Critique sociale du jugement [Distinction: a social critique of the judgement of taste] (Éditions de Minuit, 1979).
Bradley, R. H., Corwyn, R. F., McAdoo, H. P. & Garcia Coll, C. The home environments of children in the United States part I: variations by age, ethnicity, and poverty status. Child Dev. 72 , 1844–1867 (2001).
Blevins‐Knabe, B. & Musun‐Miller, L. Number use at home by children and their parents and its relationship to early mathematical performance. Early Dev. Parent. 5 , 35–45 (1996).
LeFevre, J. A. et al. Pathays to mathematics: longitudinal predictors of performance. Child Dev. 81 , 1753–1767 (2010).
Lareau, A. Home Advantage. Social Class and Parental Intervention in Elementary Education (Falmer Press, 1989).
Guryan, J., Hurst, E. & Kearney, M. Parental education and parental time with children. J. Econ. Perspect. 22 , 23–46 (2008).
Hill, C. R. & Stafford, F. P. Allocation of time to preschool children and educational opportunity. J. Hum. Resour. 9 , 323–341 (1974).
Calarco, J. M. A Field Guide to Grad School: Uncovering the Hidden Curriculum (Princeton Univ. Press, 2020).
Daw, J. Parental income and the fruits of labor: variability in homework efficacy in secondary school. Res. Soc. Strat. Mobil. 30 , 246–264 (2012).
Rønning, M. Who benefits from homework assignments? Econ. Educ. Rev. 30 , 55–64 (2011).
Alexander, K. L., Entwisle, D. R. & Olson, L. S. Lasting consequences of the summer learning gap. Am. Sociol. Rev. 72 , 167–180 (2007).
Cooper, H., Nye, B., Charlton, K., Lindsay, J. & Greathouse, S. The effects of summer vacation on achievement test scores: a narrative and meta-analytic review. Rev. Educ. Res. 66 , 227–268 (1996).
Stewart, H., Watson, N. & Campbell, M. The cost of school holidays for children from low income families. Childhood 25 , 516–529 (2018).
Pensiero, N., Kelly, A. & Bokhove, C. Learning inequalities during the Covid-19 pandemic: how families cope with home-schooling (University of Southampton, 2020); https://doi.org/10.5258/SOTON/P0025
Stephens, N. M., Markus, H. R. & Townsend, S. S. Choice as an act of meaning: the case of social class. J. Pers. Soc. Psychol. 93 , 814–830 (2007).
Kraus, M. W., Piff, P. K. & Keltner, D. Social class, sense of control, and social explanation. J. Pers. Soc. Psychol. 97 , 992–1004 (2009).
Dittmann, A. G., Stephens, N. M. & Townsend, S. S. Achievement is not class-neutral: working together benefits pople from working-class contexts. J. Pers. Soc. Psychol. 119 , 517–539 (2020).
Zimmerman, B. J. Investigating self-regulation and motivation: historical background, methodological developments, and future prospects. Am. Educ. Res. J. 45 , 166–183 (2008).
Backer-Grøndahl, A., Nærde, A., Ulleberg, P. & Janson, H. Measuring effortful control using the children’s behavior questionnaire–very short form: modeling matters. J. Pers. Assess. 98 , 100–109 (2016).
Johnson, S. E., Richeson, J. A. & Finkel, E. J. Middle class and marginal? Socioeconomic status, stigma, and self-regulation at an elite university. J. Pers. Soc. Psychol. 100 , 838–852 (2011).
Størksen, I., Ellingsen, I. T., Wanless, S. B. & McClelland, M. M. The influence of parental socioeconomic background and gender on self-regulation among 5-year-old children in Norway. Early Educ. Dev. 26 , 663–684 (2015).
Brady, L. et al. 7 ways for teachers to truly connect with parents. Education Week (31 December 2020); https://www.edweek.org/leadership/opinion-7-ways-for-teachers-to-truly-connect-with-parents/2020/12
Montacute, R. Social mobility and Covid-19: implications of the Covid-19 crisis for educational inequality (Sutton Trust, 2020); https://dera.ioe.ac.uk/35323/2/COVID-19-and-Social-Mobility-1.pdf
Dorn, E., Hancock, B., Sarakatsannis, J. & Viruleg, E. COVID-19 and student learning in the United States: the hurt could last a lifetime (McKinsey & Company, 2020); https://www.mckinsey.com/industries/public-and-social-sector/our-insights/covid-19-and-student-learning-in-the-united-states-the-hurt-could-last-a-lifetime
Parolin, Z. & Lee, E. K. Large socio-economic, geographic and demographic disparities exist in exposure to school closures. Nat. Hum. Behav. 5 , 522–528 (2021).
Saavedra, J. A silent and unequal education crisis. And the seeds for its solution (World Bank, 2021); https://blogs.worldbank.org/education/silent-and-unequal-education-crisis-and-seeds-its-solution
Murray, B., Domina, T., Renzulli, L. & Boylan, R. Civil society goes to school: parent–teacher associations and the equality of educational opportunity. Russell Sage Found. J. Soc. Sci. 5 , 41–63 (2019).
Calarco, J. M. Avoiding us versus them: how schools’ dependence on privileged ‘helicopter’ parents influences enforcement of rules. Am. Sociol. Rev. 85 , 223–246 (2020).
Rist, R. Student social class and teacher expectations: the self-fulfilling prophecy in ghetto education. Harv. Educ. Rev. 40 , 411–451 (1970).
Tobisch, A. & Dresel, M. Negatively or positively biased? Dependencies of teachers’ judgments and expectations based on students’ ethnic and social backgrounds. Soc. Psychol. Educ. 20 , 731–752 (2017).
Brantlinger, E. Dividing Classes: How the Middle-class Negotiates and Rationalizes School Advantage (Routledge, 2003).
Calarco, J. M. ‘I need help!’ Social class and children’s help-seeking in elementary school. Am. Sociol. Rev. 76 , 862–882 (2011).
Calarco, J. M. The inconsistent curriculum: cultural tool kits and student interpretations of ambiguous expectations. Soc. Psychol. Quart. 77 , 185–209 (2014).
Goudeau, S. & Cimpian, A. How do young children explain differences in the classroom? Implications for achievement, motivation, and educational equity. Perspect. Psychol. Sci. 16 , 533–552 (2021).
Croizet, J. C., Goudeau, S., Marot, M. & Millet, M. How do educational contexts contribute to the social class achievement gap: documenting symbolic violence from a social psychological point of view. Curr. Opin. Psychol. 18 , 105–110 (2017).
Goudeau, S. & Croizet, J.-C. Hidden advantages and disadvantages of social class: how classroom settings reproduce social inequality by staging unfair comparison. Psychol. Sci. 28 , 162–170 (2017).
Kudrna, L., Furnham, A. & Swami, V. The influence of social class salience on self-assessed intelligence. Soc. Behav. Personal. 38 , 859–864 (2010).
Wiederkehr, V., Darnon, C., Chazal, S., Guimond, S. & Martinot, D. From social class to self-efficacy: internalization of low social status pupils’ school performance. Soc. Psychol. Educ. 18 , 769–784 (2015).
Jury, M., Smeding, A., Court, M. & Darnon, C. When first-generation students succeed at university: on the link between social class, academic performance, and performance-avoidance goals. Contemp. Educ. Psychol. 41 , 25–36 (2015).
Jury, M., Quiamzade, A., Darnon, C. & Mugny, G. Higher and lower status individuals’ performance goals: the role of hierarchy stability. Motiv. Sci. 5 , 52–65 (2019).
Autin, F. & Croizet, J.-C. Improving working memory efficiency by reframing metacognitive interpretation of task difficulty. J. Exp. Psychol. Gen. 141 , 610–618 (2012).
Schmader, T., Johns, M. & Forbes, C. An integrated process model of stereotype threat effects on performance. Psychol. Rev. 115 , 336–356 (2008).
Usher, E. L. & Pajares, F. Self-efficacy for self-regulated learning: a validation study. Educ. Psychol. Meas. 68 , 443–463 (2008).
Bruno, A., Jury, M., Toczek-Capelle, M.-C. & Darnon, C. Are performance-avoidance goals always deleterious for academic achievement in college? The moderating role of social class. Soc. Psychol. Educ. 22 , 539–555 (2019).
Holloway, S. D. et al. Parenting self-efficacy and parental involvement: mediators or moderators between socioeconomic status and children’s academic competence in Japan and Korea? Res. Hum. Dev. 13 , 258–272 (2016).
Tazouti, Y. & Jarlégan, A. The mediating effects of parental self-efficacy and parental involvement on the link between family socioeconomic status and children’s academic achievement. J. Fam. Stud. 25 , 250–266 (2019).
Andreu, S. et al. Évaluations 2020, repères CP, CE1: premiers résultats [2020 assessments, first and second grades benchmarks: first results] (Ministère de l’Éducation nationale, de la Jeunesse et des Sports, 2020); https://www.education.gouv.fr/evaluations-2020-reperes-cp-ce1-premiers-resultats-307122
Engzell, P., Frey, A. & Verhagen, M. D. Learning loss due to school closures during the COVID-19 pandemic. Proc. Natl Acad. Sci. USA 118 , e2022376118 (2021).
Article CAS PubMed PubMed Central Google Scholar
Maldonado, J. E. & De Witte, K. The effect of school closures on standardized student test outcomes (KU Leuven—Faculty of Economics and Business, 2020); https://limo.libis.be/primo-explore/fulldisplay?docid=LIRIAS3189074&context=L&vid=Lirias&search_scope=Lirias&tab=default_tab&lang=en_US
Domingue, B., Hough, H. J., Lang, D. & Yeatman, J. Changing patterns of growth in oral reading fluency during the COVID-19 pandemic (PACE, 2021); https://edpolicyinca.org/publications/changing-patterns-growth-oral-reading-fluency-during-covid-19-pandemic
Dorn, E., Hancock, B., Sarakatsannis, J. & Viruleg, E. COVID-19 and learning loss—disparities grow and students need help (McKinsey & Company, 2020); https://www.mckinsey.com/industries/public-and-social-sector/our-insights/covid-19-and-learning-loss-disparities-grow-and-students-need-help
Angrist, N. et al. Building back better to avert a learning catastrophe: estimating learning loss from COVID-19 school shutdowns in Africa and facilitating short-term and long-term learning recovery. Int. J. Educ. Dev. 84 , 102397 (2021).
Reddy, V., Soudien, C. & Winnaar, L. Disrupted learning during COVID-19: the impact of school closures on education outcomes in South Africa (The Conversation, 2020); https://theconversation.com/impact-of-school-closures-on-education-outcomes-in-south-africa-136889
Entwisle, D. R. & Alexander, K. L. Summer setback: race, poverty, school composition, and mathematics achievement in the first two years of school. Am. Sociol. Rev. 57 , 72–84 (1992).
Kieffer, M. J. Catching up or falling behind? Initial English proficiency, concentrated poverty, and the reading growth of language minority learners in the United States. J. Educ. Psychol. 100 , 851–868 (2008).
Calarco, J. M., Horn, I. & Chen, G. A. ‘You need to be more responsible’: how math homework operates as a status-reinforcing process in school. Preprint at SocArXiv https://doi.org/10.31235/osf.io/xf96q (2020).
Kaiper-Marquez, A. et al. On the fly: adapting quickly to emergency remote instruction in a family literacy program. Int. Rev. Educ. 66 , 1–23 (2020).
Barton, D. C. Impacts of the COVID‐19 pandemic on field instruction and remote teaching alternatives: results from a survey of instructors. Ecol. Evol. 10 , 12499–12507 (2020).
Article PubMed Central Google Scholar
IJzerman, H. et al. Use caution when applying behavioural science to policy. Nat. Hum. Behav. 4 , 1092–1094 (2020).
Taylor, J. & Mallery, J. In person and online learning go together (Stanford Institute for Economic Policy Research, 2020); https://siepr.stanford.edu/research/publications/person-and-online-learning-go-together
Dietrichson, J., Bøg, M., Filges, T. & Klint Jørgensen, A. M. Academic interventions for elementary and middle school students with low socioeconomic status: a systematic review and meta-analysis. Rev. Educ. Res. 87 , 243–282 (2017).
Núñez, J. C. et al. Teachers’ feedback on homework, homework-related behaviors, and academic achievement. J. Educ. Res. 108 , 204–216 (2015).
Singh, R. et al. In Artificial Intelligence in Education (eds Biswas, G.et al.) 328–336 (Springer Berlin Heidelberg, 2011).
Harackiewicz, J. M., Rozek, C. S., Hulleman, C. S. & Hyde, J. S. Helping parents to motivate adolescents in mathematics and science: an experimental test of a utility-value intervention. Psychol. Sci. 23 , 899–906 (2012).
Jeynes, W. A meta-analysis of the efficacy of different types of parental involvement programs for urban students. Urban Educ. 47 , 706–742 (2012).
Mol, S. E., Bus, A. G., De Jong, M. T. & Smeets, D. J. Added value of dialogic parent–child book readings: a meta-analysis. Early Educ. Dev. 19 , 7–26 (2008).
Angrist, N., Bergman, P. & Matsheng, M. School’s out: experimental evidence on limiting learning loss using “low-tech” in a pandemic (National Bureau of Economic Research, 2021); https://www.nber.org/papers/w28205
Carlana, M. & La Ferrara, E. Apart but connected: online tutoring and student outcomes during the COVID-19 pandemic (Institute of Labor Economics, 2021); http://hdl.handle.net/10419/232846
Pagan, S. & Sénéchal, M. Involving parents in a summer book reading program to promote reading comprehension, fluency, and vocabulary in grade 3 and grade 5 children. Can. J. Educ. 37 , 1–31 (2014).
Sénéchal, M. & LeFevre, J. A. Parental involvement in the development of children’s reading skill: a five‐year longitudinal study. Child Dev. 73 , 445–460 (2002).
Starkey, P. & Klein, A. Fostering parental support for children’s mathematical development: an intervention with Head Start families. Early Educ. Dev. 11 , 659–680 (2000).
Buheji, M. et al. The extent of Covid-19 pandemic socio-economic impact on global poverty: a global integrative multidisciplinary review. Am. J. Econ. 10 , 213–224 (2020).
The world economy on a tightrope (OECD, 2020); http://www.oecd.org/economic-outlook/june-2020/
Martin, A., Markhvida, M., Hallegatte, S. & Walsh, B. Socio-economic impacts of COVID-19 on household consumption and poverty. Econ. Disasters Clim. Change 4 , 453–479 (2020).
Jetten, J., Mols, F. & Selvanathan, H. P. How economic inequality fuels the rise and persistence of the Yellow Vest movement. Int. Rev. Soc. Psychol. 33 , 2 (2020).
Wilkinson, R. G. & Pickett, K. E. Income inequality and social dysfunction. Annu. Rev. Sociol. 35 , 493–511 (2009).
Sommet, N., Morselli, D. & Spini, D. Income inequality affects the psychological health of only the people facing scarcity. Psychol. Sci. 29 , 1911–1921 (2018).
Hattie, J. Visible Learning: A Synthesis of over 800 Meta-analyses Relating to Achievement (Routledge, 2008).
Cooper, H., Charlton, K., Valentine, J. C., Muhlenbruck, L. & Borman, G. D. Making the most of summer school: a meta-analytic and narrative review. Monogr. Soc. Res. Child 65 , 1–127 (2000).
Heyns, B. Schooling and cognitive development: is there a season for learning? Child Dev. 58 , 1151–1160 (1987).
McCombs, J. S., Augustine, C. H. & Schwartz, H. L. Making Summer Count: How Summer Programs can Boost Children’s Learning (Rand Education, 2011).
Borman, G. D. & Dowling, N. M. Longitudinal achievement effects of multiyear summer school: evidence from the teach Baltimore randomized field trial. Educ. Eval. Policy 28 , 25–48 (2006).
Kim, J. S. & Quinn, D. M. The effects of summer reading on low-income children’s literacy achievement from kindergarten to grade 8: a meta-analysis of classroom and home interventions. Rev. Educ. Res. 83 , 386–431 (2013).
Download references
Acknowledgements
We thank G. Reis for editing the figure. The writing of this manuscript was supported by grant ANR-19-CE28-0007–PRESCHOOL from the French National Research Agency (S.G.).
Author information
Authors and affiliations.
Université de Poitiers, CNRS, CeRCA, Centre de Recherches sur la Cognition et l’Apprentissage, Poitiers, France
Sébastien Goudeau & Camille Sanrey
Université Clermont Auvergne, CNRS, LAPSCO, Laboratoire de Psychologie Sociale et Cognitive, Clermont-Ferrand, France
Arnaud Stanczak & Céline Darnon
School of Psychology, Cardiff University, Cardiff, UK
Antony Manstead
You can also search for this author in PubMed Google Scholar
Corresponding author
Correspondence to Sébastien Goudeau .
Ethics declarations
Competing interests.
The authors declare no competing interests.
Additional information
Peer review information Nature Human Behaviour thanks Daniele Checchi and the other, anonymous, reviewer(s) for their contribution to the peer review of this work.
Publisher’s note Springer Nature remains neutral with regard to jurisdictional claims in published maps and institutional affiliations.
Rights and permissions
Reprints and permissions
About this article
Cite this article.
Goudeau, S., Sanrey, C., Stanczak, A. et al. Why lockdown and distance learning during the COVID-19 pandemic are likely to increase the social class achievement gap. Nat Hum Behav 5 , 1273–1281 (2021). https://doi.org/10.1038/s41562-021-01212-7
Download citation
Received : 15 March 2021
Accepted : 06 September 2021
Published : 27 September 2021
Issue Date : October 2021
DOI : https://doi.org/10.1038/s41562-021-01212-7
Share this article
Anyone you share the following link with will be able to read this content:
Sorry, a shareable link is not currently available for this article.
Provided by the Springer Nature SharedIt content-sharing initiative
This article is cited by
Digital gender gaps in students’ knowledge, attitudes and skills: an integrative data analysis across 32 countries.
- Diego G. Campos
- Ronny Scherer
Education and Information Technologies (2024)
Microlearning as a Concept to Optimize Integrated Services for Racially/Ethnically Diverse Families of Autistic Children
- Zhiwen Xiao
- Sandra Vanegas
Journal of Developmental and Physical Disabilities (2024)
The ethicality of the COVID-19 response in children and adolescents
- Fiona McNicholas
Irish Journal of Medical Science (1971 -) (2024)
Learning loss and psychosocial issues among Thai students amidst the COVID-19 pandemic: the perspectives of teachers in the online classroom
- Ittipaat Suwathanpornkul
- Chawapon Sarnkhaowkhom
- Orn-uma Charoensuk
BMC Psychology (2023)
Social determinants associated with psychological distress in children and adolescents during and after the first COVID-19-related lockdown in France: results from the CONFEADO study
- Mégane Estevez
- Nicolas Oppenchaim
- Stéphanie Vandentorren
BMC Public Health (2023)
Quick links
- Explore articles by subject
- Guide to authors
- Editorial policies
Sign up for the Nature Briefing newsletter — what matters in science, free to your inbox daily.

The COVID-19 pandemic has changed education forever. This is how
With schools shut across the world, millions of children have had to adapt to new types of learning. Image: REUTERS/Gonzalo Fuentes
.chakra .wef-1c7l3mo{-webkit-transition:all 0.15s ease-out;transition:all 0.15s ease-out;cursor:pointer;-webkit-text-decoration:none;text-decoration:none;outline:none;color:inherit;}.chakra .wef-1c7l3mo:hover,.chakra .wef-1c7l3mo[data-hover]{-webkit-text-decoration:underline;text-decoration:underline;}.chakra .wef-1c7l3mo:focus,.chakra .wef-1c7l3mo[data-focus]{box-shadow:0 0 0 3px rgba(168,203,251,0.5);} Cathy Li
Farah lalani.
.chakra .wef-9dduvl{margin-top:16px;margin-bottom:16px;line-height:1.388;font-size:1.25rem;}@media screen and (min-width:56.5rem){.chakra .wef-9dduvl{font-size:1.125rem;}} Explore and monitor how .chakra .wef-15eoq1r{margin-top:16px;margin-bottom:16px;line-height:1.388;font-size:1.25rem;color:#F7DB5E;}@media screen and (min-width:56.5rem){.chakra .wef-15eoq1r{font-size:1.125rem;}} COVID-19 is affecting economies, industries and global issues
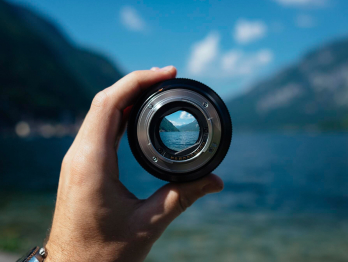
.chakra .wef-1nk5u5d{margin-top:16px;margin-bottom:16px;line-height:1.388;color:#2846F8;font-size:1.25rem;}@media screen and (min-width:56.5rem){.chakra .wef-1nk5u5d{font-size:1.125rem;}} Get involved with our crowdsourced digital platform to deliver impact at scale
Stay up to date:.
- The COVID-19 has resulted in schools shut all across the world. Globally, over 1.2 billion children are out of the classroom.
- As a result, education has changed dramatically, with the distinctive rise of e-learning, whereby teaching is undertaken remotely and on digital platforms.
- Research suggests that online learning has been shown to increase retention of information, and take less time, meaning the changes coronavirus have caused might be here to stay.
While countries are at different points in their COVID-19 infection rates, worldwide there are currently more than 1.2 billion children in 186 countries affected by school closures due to the pandemic. In Denmark, children up to the age of 11 are returning to nurseries and schools after initially closing on 12 March , but in South Korea students are responding to roll calls from their teachers online .
With this sudden shift away from the classroom in many parts of the globe, some are wondering whether the adoption of online learning will continue to persist post-pandemic, and how such a shift would impact the worldwide education market.
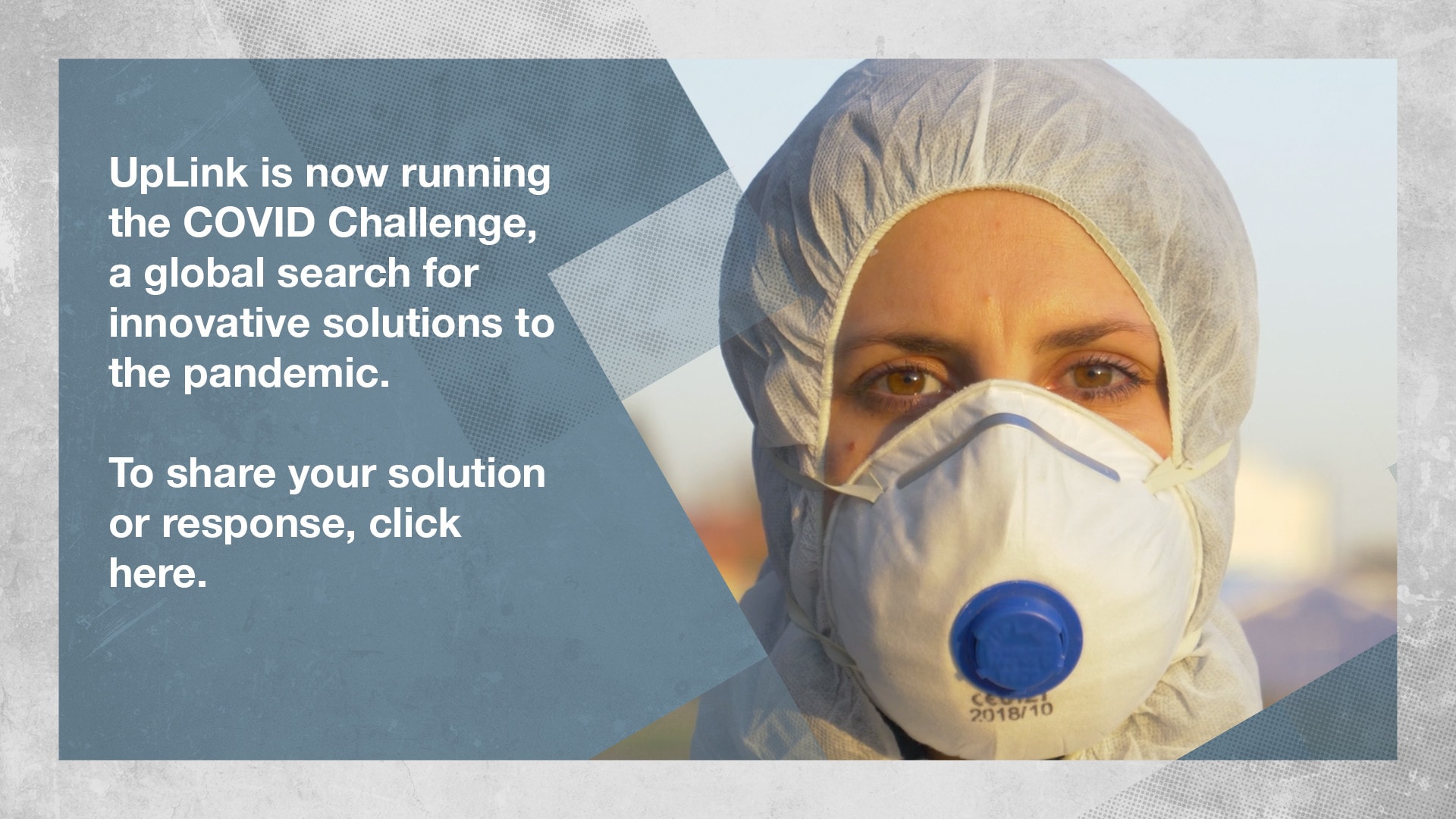
Even before COVID-19, there was already high growth and adoption in education technology, with global edtech investments reaching US$18.66 billion in 2019 and the overall market for online education projected to reach $350 Billion by 2025 . Whether it is language apps , virtual tutoring , video conferencing tools, or online learning software , there has been a significant surge in usage since COVID-19.
How is the education sector responding to COVID-19?
In response to significant demand, many online learning platforms are offering free access to their services, including platforms like BYJU’S , a Bangalore-based educational technology and online tutoring firm founded in 2011, which is now the world’s most highly valued edtech company . Since announcing free live classes on its Think and Learn app, BYJU’s has seen a 200% increase in the number of new students using its product, according to Mrinal Mohit, the company's Chief Operating Officer.
Tencent classroom, meanwhile, has been used extensively since mid-February after the Chinese government instructed a quarter of a billion full-time students to resume their studies through online platforms. This resulted in the largest “online movement” in the history of education with approximately 730,000 , or 81% of K-12 students, attending classes via the Tencent K-12 Online School in Wuhan.
Have you read?
The future of jobs report 2023, how to follow the growth summit 2023.
Other companies are bolstering capabilities to provide a one-stop shop for teachers and students. For example, Lark, a Singapore-based collaboration suite initially developed by ByteDance as an internal tool to meet its own exponential growth, began offering teachers and students unlimited video conferencing time, auto-translation capabilities, real-time co-editing of project work, and smart calendar scheduling, amongst other features. To do so quickly and in a time of crisis, Lark ramped up its global server infrastructure and engineering capabilities to ensure reliable connectivity.
Alibaba’s distance learning solution, DingTalk, had to prepare for a similar influx: “To support large-scale remote work, the platform tapped Alibaba Cloud to deploy more than 100,000 new cloud servers in just two hours last month – setting a new record for rapid capacity expansion,” according to DingTalk CEO, Chen Hang.
Some school districts are forming unique partnerships, like the one between The Los Angeles Unified School District and PBS SoCal/KCET to offer local educational broadcasts, with separate channels focused on different ages, and a range of digital options. Media organizations such as the BBC are also powering virtual learning; Bitesize Daily , launched on 20 April, is offering 14 weeks of curriculum-based learning for kids across the UK with celebrities like Manchester City footballer Sergio Aguero teaching some of the content.
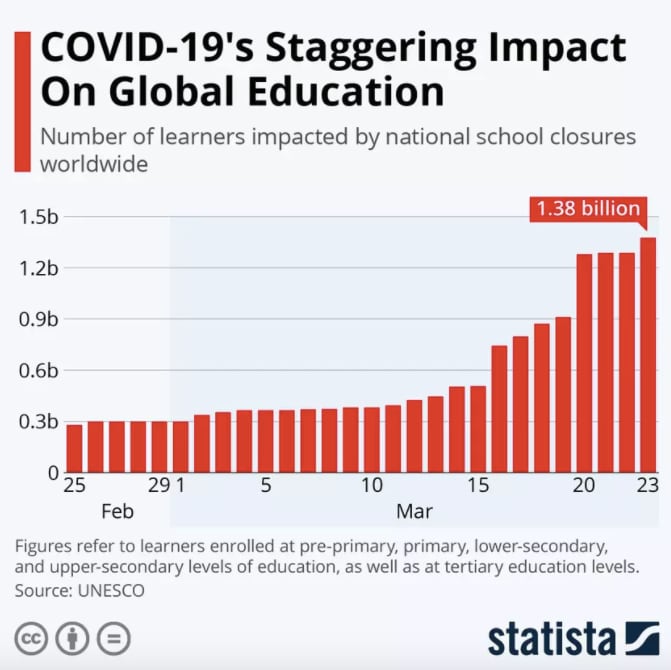
What does this mean for the future of learning?
While some believe that the unplanned and rapid move to online learning – with no training, insufficient bandwidth, and little preparation – will result in a poor user experience that is unconducive to sustained growth, others believe that a new hybrid model of education will emerge, with significant benefits. “I believe that the integration of information technology in education will be further accelerated and that online education will eventually become an integral component of school education,“ says Wang Tao, Vice President of Tencent Cloud and Vice President of Tencent Education.
There have already been successful transitions amongst many universities. For example, Zhejiang University managed to get more than 5,000 courses online just two weeks into the transition using “DingTalk ZJU”. The Imperial College London started offering a course on the science of coronavirus, which is now the most enrolled class launched in 2020 on Coursera .
Many are already touting the benefits: Dr Amjad, a Professor at The University of Jordan who has been using Lark to teach his students says, “It has changed the way of teaching. It enables me to reach out to my students more efficiently and effectively through chat groups, video meetings, voting and also document sharing, especially during this pandemic. My students also find it is easier to communicate on Lark. I will stick to Lark even after coronavirus, I believe traditional offline learning and e-learning can go hand by hand."
These 3 charts show the global growth in online learning
The challenges of online learning.
There are, however, challenges to overcome. Some students without reliable internet access and/or technology struggle to participate in digital learning; this gap is seen across countries and between income brackets within countries. For example, whilst 95% of students in Switzerland, Norway, and Austria have a computer to use for their schoolwork, only 34% in Indonesia do, according to OECD data .
In the US, there is a significant gap between those from privileged and disadvantaged backgrounds: whilst virtually all 15-year-olds from a privileged background said they had a computer to work on, nearly 25% of those from disadvantaged backgrounds did not. While some schools and governments have been providing digital equipment to students in need, such as in New South Wales , Australia, many are still concerned that the pandemic will widenthe digital divide .
Is learning online as effective?
For those who do have access to the right technology, there is evidence that learning online can be more effective in a number of ways. Some research shows that on average, students retain 25-60% more material when learning online compared to only 8-10% in a classroom. This is mostly due to the students being able to learn faster online; e-learning requires 40-60% less time to learn than in a traditional classroom setting because students can learn at their own pace, going back and re-reading, skipping, or accelerating through concepts as they choose.
Nevertheless, the effectiveness of online learning varies amongst age groups. The general consensus on children, especially younger ones, is that a structured environment is required , because kids are more easily distracted. To get the full benefit of online learning, there needs to be a concerted effort to provide this structure and go beyond replicating a physical class/lecture through video capabilities, instead, using a range of collaboration tools and engagement methods that promote “inclusion, personalization and intelligence”, according to Dowson Tong, Senior Executive Vice President of Tencent and President of its Cloud and Smart Industries Group.
Since studies have shown that children extensively use their senses to learn, making learning fun and effective through use of technology is crucial, according to BYJU's Mrinal Mohit. “Over a period, we have observed that clever integration of games has demonstrated higher engagement and increased motivation towards learning especially among younger students, making them truly fall in love with learning”, he says.
A changing education imperative
It is clear that this pandemic has utterly disrupted an education system that many assert was already losing its relevance . In his book, 21 Lessons for the 21st Century , scholar Yuval Noah Harari outlines how schools continue to focus on traditional academic skills and rote learning , rather than on skills such as critical thinking and adaptability, which will be more important for success in the future. Could the move to online learning be the catalyst to create a new, more effective method of educating students? While some worry that the hasty nature of the transition online may have hindered this goal, others plan to make e-learning part of their ‘new normal’ after experiencing the benefits first-hand.
The importance of disseminating knowledge is highlighted through COVID-19
Major world events are often an inflection point for rapid innovation – a clear example is the rise of e-commerce post-SARS . While we have yet to see whether this will apply to e-learning post-COVID-19, it is one of the few sectors where investment has not dried up . What has been made clear through this pandemic is the importance of disseminating knowledge across borders, companies, and all parts of society. If online learning technology can play a role here, it is incumbent upon all of us to explore its full potential.
Our education system is losing relevance. Here's how to unleash its potential
3 ways the coronavirus pandemic could reshape education, celebrities are helping the uk's schoolchildren learn during lockdown, don't miss any update on this topic.
Create a free account and access your personalized content collection with our latest publications and analyses.
License and Republishing
World Economic Forum articles may be republished in accordance with the Creative Commons Attribution-NonCommercial-NoDerivatives 4.0 International Public License, and in accordance with our Terms of Use.
The views expressed in this article are those of the author alone and not the World Economic Forum.
Related topics:
The agenda .chakra .wef-n7bacu{margin-top:16px;margin-bottom:16px;line-height:1.388;font-weight:400;} weekly.
A weekly update of the most important issues driving the global agenda
.chakra .wef-1dtnjt5{display:-webkit-box;display:-webkit-flex;display:-ms-flexbox;display:flex;-webkit-align-items:center;-webkit-box-align:center;-ms-flex-align:center;align-items:center;-webkit-flex-wrap:wrap;-ms-flex-wrap:wrap;flex-wrap:wrap;} More on COVID-19 .chakra .wef-17xejub{-webkit-flex:1;-ms-flex:1;flex:1;justify-self:stretch;-webkit-align-self:stretch;-ms-flex-item-align:stretch;align-self:stretch;} .chakra .wef-nr1rr4{display:-webkit-inline-box;display:-webkit-inline-flex;display:-ms-inline-flexbox;display:inline-flex;white-space:normal;vertical-align:middle;text-transform:uppercase;font-size:0.75rem;border-radius:0.25rem;font-weight:700;-webkit-align-items:center;-webkit-box-align:center;-ms-flex-align:center;align-items:center;line-height:1.2;-webkit-letter-spacing:1.25px;-moz-letter-spacing:1.25px;-ms-letter-spacing:1.25px;letter-spacing:1.25px;background:none;padding:0px;color:#B3B3B3;-webkit-box-decoration-break:clone;box-decoration-break:clone;-webkit-box-decoration-break:clone;}@media screen and (min-width:37.5rem){.chakra .wef-nr1rr4{font-size:0.875rem;}}@media screen and (min-width:56.5rem){.chakra .wef-nr1rr4{font-size:1rem;}} See all
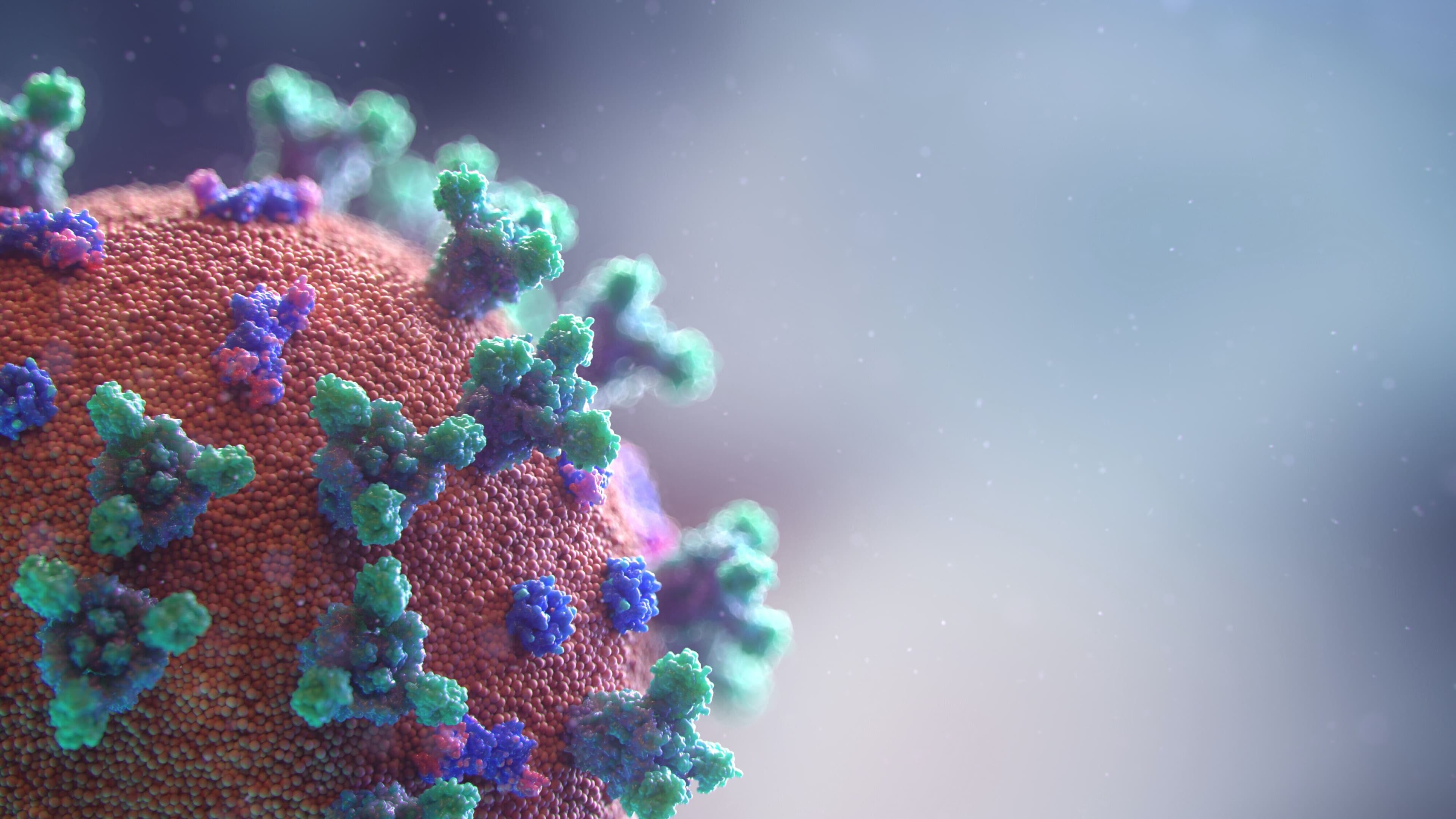
Winding down COVAX – lessons learnt from delivering 2 billion COVID-19 vaccinations to lower-income countries
Charlotte Edmond
January 8, 2024
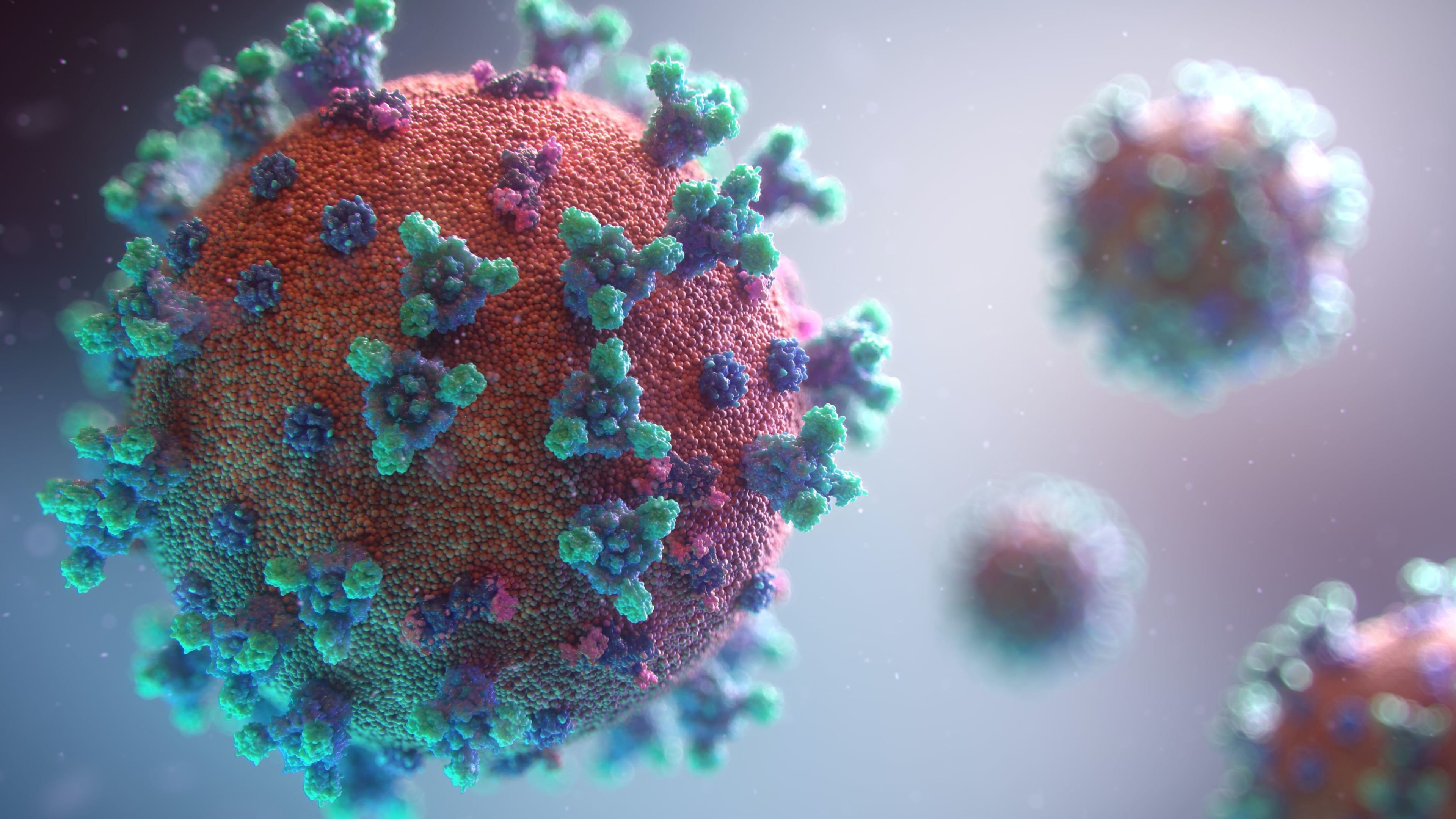
Here’s what to know about the new COVID-19 Pirola variant
October 11, 2023
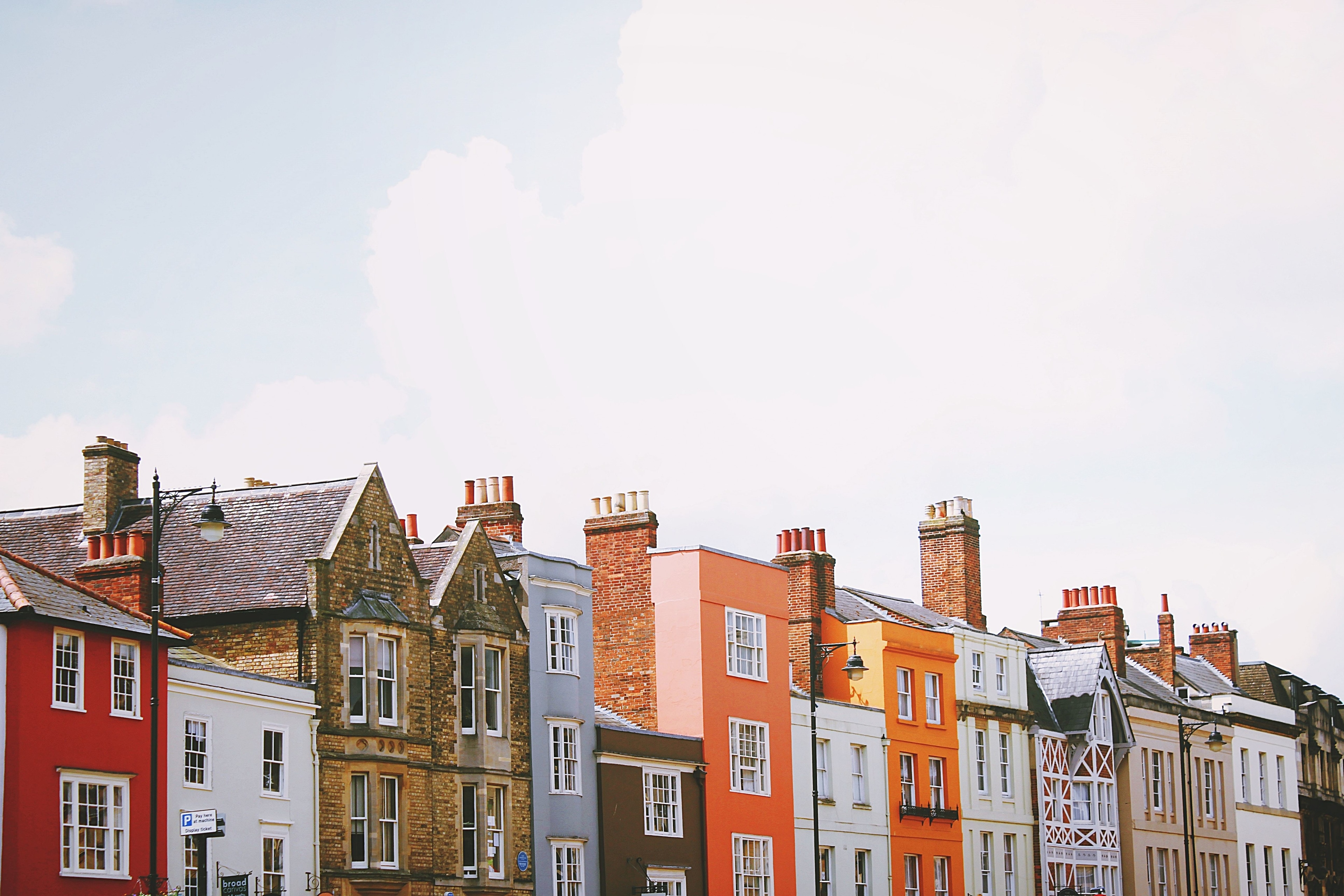
How the cost of living crisis affects young people around the world
Douglas Broom
August 8, 2023
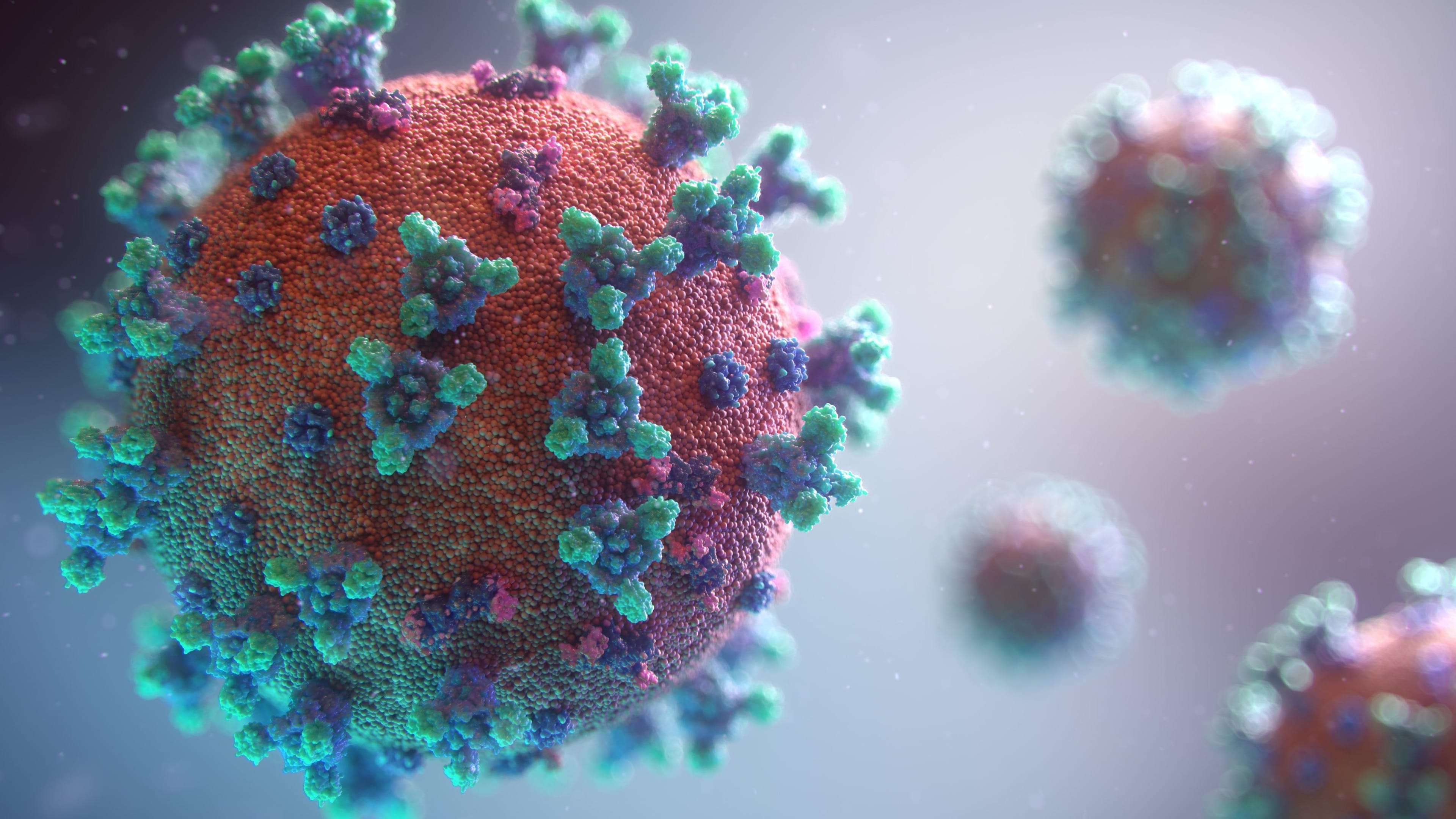
From smallpox to COVID: the medical inventions that have seen off infectious diseases over the past century
Andrea Willige
May 11, 2023
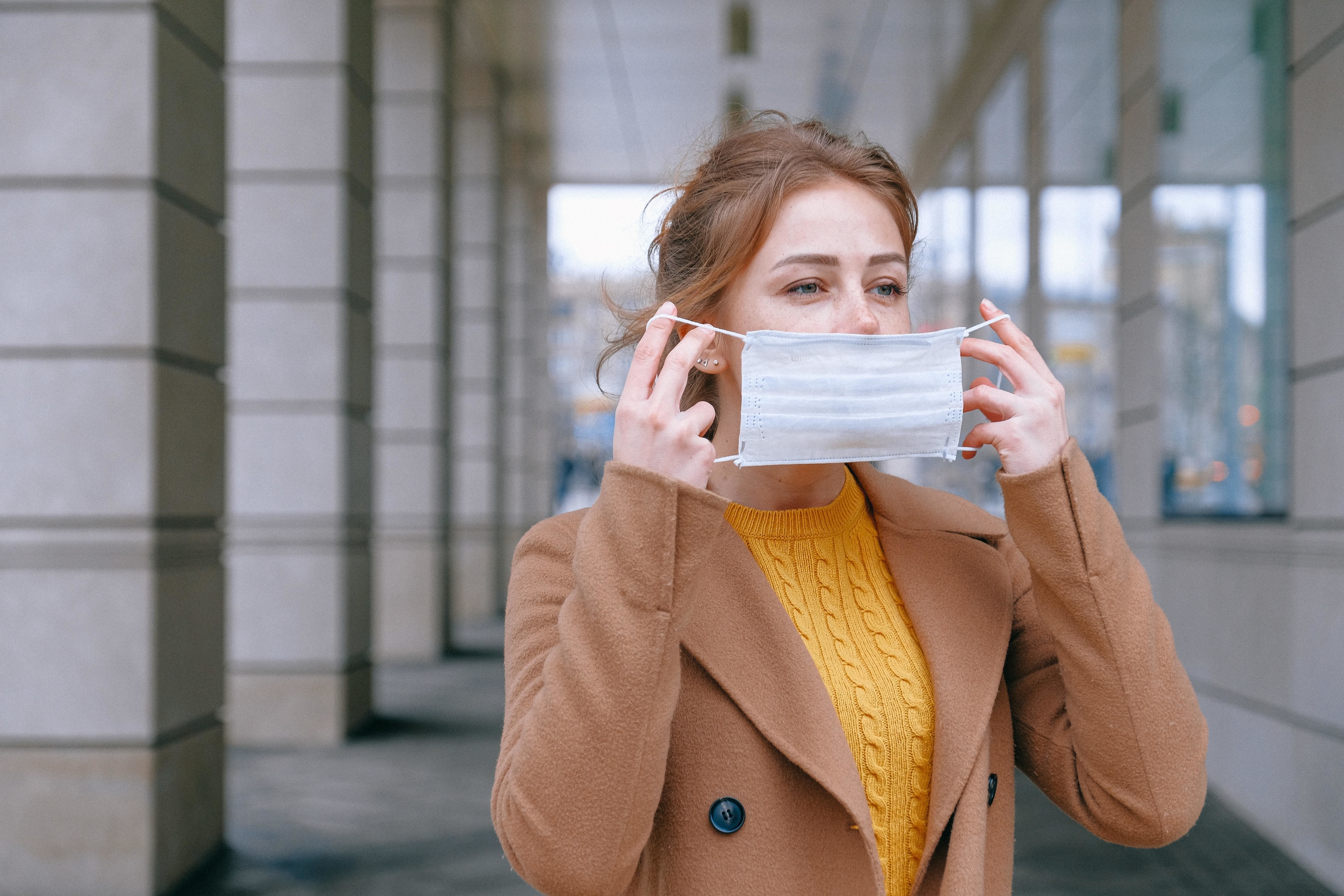
COVID-19 is no longer a global health emergency. Here's what it means
Simon Nicholas Williams
May 9, 2023
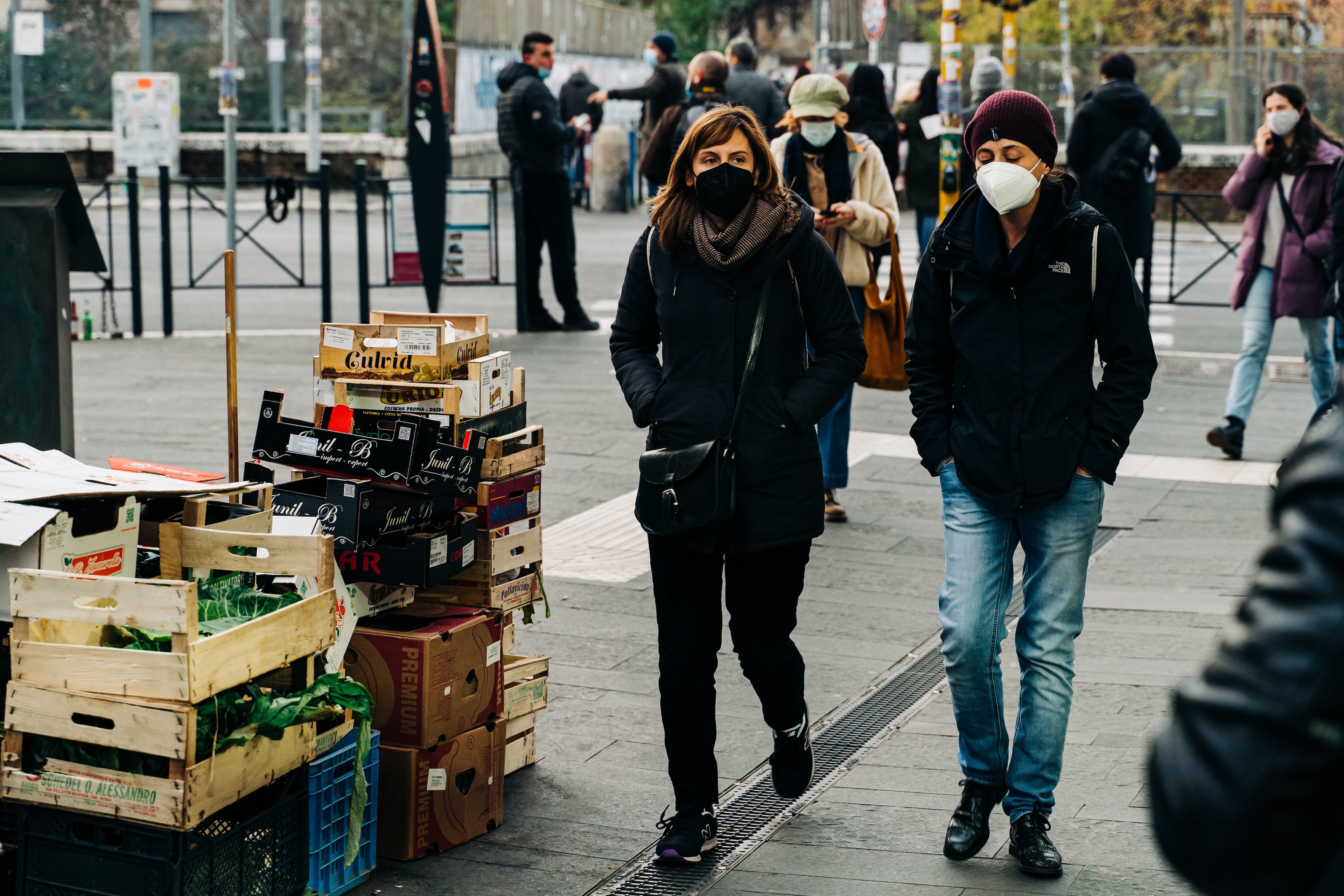
New research shows the significant health harms of the pandemic
Philip Clarke, Jack Pollard and Mara Violato
April 17, 2023
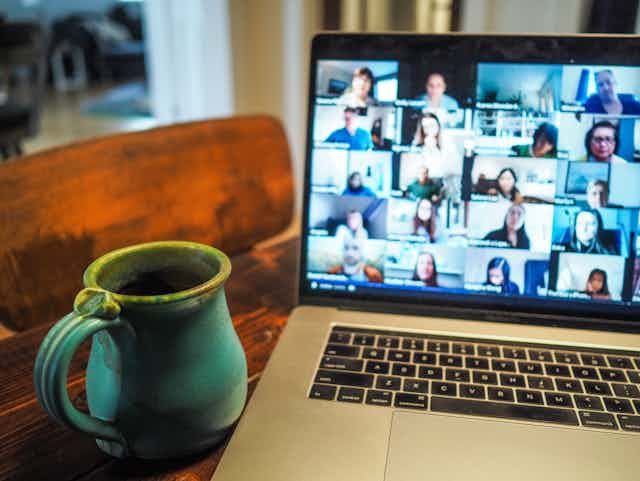
4 lessons from online learning that should stick after the pandemic
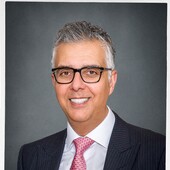
Assistant Professor of Innovation Finance, Athabasca University
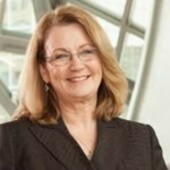
Associate Professor, Work and Organization Studies, Athabasca University
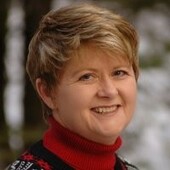
Professor of Organizational Analysis and Project Management, Athabasca University
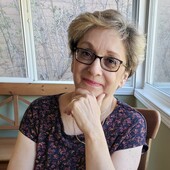
Professor of Open, Digital, and Distance Education , Athabasca University
Disclosure statement
Deborah Hurst receives funding from RBC and CPA to build and launch the AI powered virtual cooperative program.
Janice Thomas received funding from the Project Management Institute for earlier research projects exploring the professionalization of project management, the value of project management to organizations, and the implementation of project management as a management innovation.
Martha Cleveland-Innes has received funding from, and acts in support of, the Social Sciences and Humanities Research Council. She is an appointed member of the Athabasca University Board of Governors.
F. Haider Alvi does not work for, consult, own shares in or receive funding from any company or organisation that would benefit from this article, and has disclosed no relevant affiliations beyond their academic appointment.
View all partners
One of the many changes COVID-19 brought those in education was an almost immediate switch to online learning.
Overnight, institutions scrambled to keep education moving, while bridging the physical distance between teacher and learner. Traditionally trained teachers made valiant efforts to adjust to digital by recording lessons, posting videos and creating breakout rooms, using whatever technology they had available.
These efforts resulted in digitally mediated physical classrooms using the internet — not online education.
While these two options sound the same, they are not . Bridging physical distance through technology alone doesn’t address additional adjustments required to address learner needs. Posting materials online, recording lectures and discussions themselves don’t create a coached, collaborative and supported learning environment.
So what have we really learned about online education? And what do we do now?
Online learning isn’t new, and lessons can be drawn from existing research and experience. Athabasca University — where we are all professors — pioneered the world’s first online MBA, M.Nursing and M.Ed progams over 28 years ago. And today, it’s one of Canada’s leading online universities .
The experience of online pioneers highlights four distinct aspects of online learning that should stick post-pandemic: learning to learn online, designing online teaching with purpose, blending space and time online and continued disruption with AI.
1. Learning to learn online
The pandemic highlighted that one-size-fits-all educational approaches fail to address student needs. Younger learners may seek physical spaces to promote socialization, with supervision and teacher-led content delivery. Others, like Athabasca’s mostly adult learners, value the convenience of connecting with classmates and instructors online during times of their choosing.
Common inequities like poor access to the internet, lack of financial resources and needed digital competence plague online learning. However, online education offers access for students facing geospacial barriers to traditional classrooms, and further issues of inequality are addressed via multi-modal distance education, financial support structures and orientation on how to learn online .
Read more: Online learning during COVID-19: 8 ways universities can improve equity and access
Emergency online education used blunt-edged instruments, ignoring student and program differences . The pandemic takeaway, however, is the importance of preparing all students to learn, whether online or in a physical classroom.
2. Designing online teaching with purpose
Quality teaching and learning design must incorporate active, engaging roles for individual students, whether designed for traditional or distance education .
Meaningful teaching varies by setting and requires different approaches . Online course and teaching design is learner rather than content centred, incorporating high engagement in collaborative learning groups that fosters active learning.
Producing effective online course materials requires an approach involving both instructors and skilled course developers and takes months rather than weeks. Course materials are painstakingly detailed, and include writing everything the instructor would expect to say in a physical classroom, clearly describing all course requirements and linking students to readings, video and online resources.
Because of the pandemic, instructors had to translate classroom delivery into technology-mediated delivery — it worked for some, but was not easily tailored to unique learning needs.
Technological tools, combined with independent and joint working opportunities, should be brought back to the physical or hybrid classroom in conjunction with online pedagogical approaches that increase active, collaborative learning and learner-generated choices.
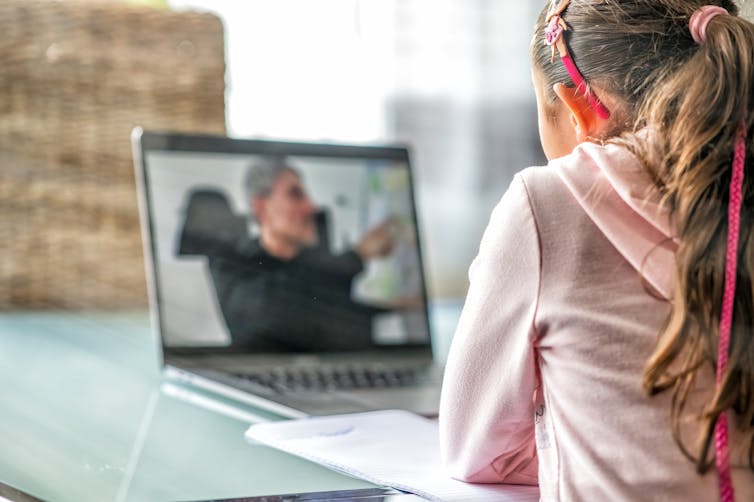
3. Blending space and time online
Pandemic education popularized the vocabulary of “synchronous” and “asynchronous” learning. Synchronous replicated physical classrooms through real-time, digitally mediated teaching, while asynchronous meant working independently, usually with materials designed for a physical classroom. Moving forward we need to think about how timing and presence impacts learning.
At Athabasca, students come together in time and space through blended, collaborative, synchronous and asynchronous online learning . Instructors coach students individually at a student led pace.
This is different from traditional undergraduate classrooms, where students absorb material on a fixed schedule. Our graduate programs use paced programming, requiring students to work independently while regularly coming together in active online discussion.
More flexible teaching allows students to receive instructor support when they need it. Building in synchronous, collaborative learning allows for reflection, rather than real time responses.
4. COVID-19 began the disruption, AI will continue it
The pandemic revealed how education approaches can change after instructors had to search for innovative ways to improve student learning outcomes outside the physical classroom.
At Athabasca, a virtual co-operative program allowed us to introduce a co-op program in the middle of a pandemic.
Students accessed a simulated work experience in a paced structure, irrespective of location. They were able to practise working as a team, problem solving, conflict resolution, ethical reasoning and leadership while working on an assigned project. Students received immediate, detailed feedback from an AI coach, allowing for extensive experimentation and revision to master concepts honed in reflective discussion with the instructor.
Research suggests that adopting online and AI tools needs to be deliberate, coupled with supportive digital infrastructure and highly responsive student support. Planned carefully and taken together, these steps improve on traditional approaches by making education truly open, accessible and inclusive.
Now, the question for all educators should be: How do we capitalize on COVID-19 initiated change to build better education systems for the future?
This is an updated version of a story originally published May 1, 2022. It clarifies emergency online education made it difficult to address student differences.
- Online learning
- Online education
- Online schooling
- COVID-19 school

Biocloud Project Manager - Australian Biocommons

Director, Defence and Security

Opportunities with the new CIEHF

School of Social Sciences – Public Policy and International Relations opportunities

Deputy Editor - Technology
Click through the PLOS taxonomy to find articles in your field.
For more information about PLOS Subject Areas, click here .
Loading metrics
Open Access
Peer-reviewed
Research Article
Distance learning in higher education during COVID-19: The role of basic psychological needs and intrinsic motivation for persistence and procrastination–a multi-country study
Roles Conceptualization, Methodology, Writing – original draft
* E-mail: [email protected]
Affiliation Department of Developmental and Educational Psychology, Faculty of Psychology, University of Vienna, Vienna, Austria

Roles Conceptualization, Data curation, Methodology, Project administration, Writing – review & editing
Roles Formal analysis, Methodology, Writing – original draft, Writing – review & editing
Roles Conceptualization, Methodology, Writing – review & editing
Roles Data curation, Writing – review & editing
Affiliation Department of Mathematics, Faculty of Mathematics, University of Vienna, Vienna, Austria
Roles Conceptualization, Funding acquisition, Methodology, Writing – review & editing
Roles Conceptualization, Funding acquisition, Methodology
Affiliation Department of Psychology, Faculty of Education, Aleksandër Moisiu University, Durrës, Albania
Affiliation Department of Educational Sciences, Faculty of Philology and Education, Bedër University, Tirana, Albania
Affiliation Xiangya School of Nursing, Central South University, Changsha, China
Affiliations Xiangya School of Nursing, Central South University, Changsha, China, Department of Nursing Science, University of Turku, Turku, Finland
Affiliation Study of Nursing, University of Applied Sciences Bjelovar, Bjelovar, Croatia
Affiliation Baltic Film, Media and Arts School, Tallinn University, Tallinn, Estonia
Affiliation Faculty of Educational Sciences, University of Helsinki, Helsinki, Finland
Affiliation Department of Psychology, University of Bonn, Bonn, Germany
Affiliation Chair of Educational Psychology, Technische Universität Berlin, Berlin, Germany
Affiliation Department of Educational Studies, University of Potsdam, Potsdam, Germany
Affiliation Faculty of Education, University of Akureyri, Akureyri, Iceland
Affiliation Department of Global Education, Tsuru University, Tsuru, Japan
Affiliation Career Center, Osaka University, Osaka University, Suita, Japan
Affiliation Graduate School of Education, Osaka Kyoiku University, Kashiwara, Japan
Affiliation Department of Psychology, Faculty of Philosophy, University of Prishtina ’Hasan Prishtina’, Pristina, Kosovo
Affiliation Department of Social Work, Faculty of Philosophy, University of Pristina ’Hasan Prishtina’, Pristina, Kosovo
Affiliation Department of Psychology, Faculty of Social Sciences and Humanities, Klaipėda University, Klaipėda, Lithuania
Affiliation Geography Department, Junior College, University of Malta, Msida, Malta
Affiliation Institute of Family Studies, Faculty of Philosophy, Ss. Cyril and Methodius University in Skopje, Skopje, North Macedonia
Affiliation Institute of Psychology, Faculty of Social Science, University of Gdańsk, Gdańsk, Poland
Affiliation Faculty of Historical and Pedagogical Sciences, University of Wrocław, Wrocław, Poland
Affiliation Faculty of Educational Studies, Adam Mickiewicz University, Poznań, Poland
Affiliation CERNESIM Environmental Research Center, Alexandru Ioan Cuza University, Iași, România
Affiliation Social Sciences and Humanities Research Department, Institute for Interdisciplinary Research, Alexandru Ioan Cuza University of Iași, Iași, România
Affiliation Department of Informatics, Örebro University School of Business, Örebro University, Örebro, Sweden
Affiliation Faculty of Social Studies, Penn State University, State College, Pennsylvania, United States of America
- [ ... ],
Affiliations Department of Developmental and Educational Psychology, Faculty of Psychology, University of Vienna, Vienna, Austria, Department for Teacher Education, Centre for Teacher Education, University of Vienna, Vienna, Austria
- [ view all ]
- [ view less ]
- Elisabeth R. Pelikan,
- Selma Korlat,
- Julia Reiter,
- Julia Holzer,
- Martin Mayerhofer,
- Barbara Schober,
- Christiane Spiel,
- Oriola Hamzallari,
- Ana Uka,
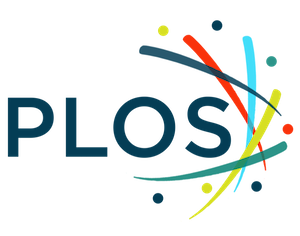
- Published: October 6, 2021
- https://doi.org/10.1371/journal.pone.0257346
- Peer Review
- Reader Comments
Due to the COVID-19 pandemic, higher educational institutions worldwide switched to emergency distance learning in early 2020. The less structured environment of distance learning forced students to regulate their learning and motivation more independently. According to self-determination theory (SDT), satisfaction of the three basic psychological needs for autonomy, competence and social relatedness affects intrinsic motivation, which in turn relates to more active or passive learning behavior. As the social context plays a major role for basic need satisfaction, distance learning may impair basic need satisfaction and thus intrinsic motivation and learning behavior. The aim of this study was to investigate the relationship between basic need satisfaction and procrastination and persistence in the context of emergency distance learning during the COVID-19 pandemic in a cross-sectional study. We also investigated the mediating role of intrinsic motivation in this relationship. Furthermore, to test the universal importance of SDT for intrinsic motivation and learning behavior under these circumstances in different countries, we collected data in Europe, Asia and North America. A total of N = 15,462 participants from Albania, Austria, China, Croatia, Estonia, Finland, Germany, Iceland, Japan, Kosovo, Lithuania, Poland, Malta, North Macedonia, Romania, Sweden, and the US answered questions regarding perceived competence, autonomy, social relatedness, intrinsic motivation, procrastination, persistence, and sociodemographic background. Our results support SDT’s claim of universality regarding the relation between basic psychological need fulfilment, intrinsic motivation, procrastination, and persistence. However, whereas perceived competence had the highest direct effect on procrastination and persistence, social relatedness was mainly influential via intrinsic motivation.
Citation: Pelikan ER, Korlat S, Reiter J, Holzer J, Mayerhofer M, Schober B, et al. (2021) Distance learning in higher education during COVID-19: The role of basic psychological needs and intrinsic motivation for persistence and procrastination–a multi-country study. PLoS ONE 16(10): e0257346. https://doi.org/10.1371/journal.pone.0257346
Editor: Shah Md Atiqul Haq, Shahjalal University of Science and Technology, BANGLADESH
Received: March 30, 2021; Accepted: August 29, 2021; Published: October 6, 2021
Copyright: © 2021 Pelikan et al. This is an open access article distributed under the terms of the Creative Commons Attribution License , which permits unrestricted use, distribution, and reproduction in any medium, provided the original author and source are credited.
Data Availability: Data is now publicly available: Pelikan ER, Korlat S, Reiter J, Lüftenegger M. Distance Learning in Higher Education During COVID-19: Basic Psychological Needs and Intrinsic Motivation 2021. doi: 10.17605/OSF.IO/8CZX3 .
Funding: This work was funded by the Vienna Science and Technology Fund (WWTF) [ https://www.wwtf.at/ ] and the MEGA Bildungsstiftung [ https://www.megabildung.at/ ] through project COV20-025, as well as the Academy of Finland [ https://www.aka.fi ] through project 308351, 336138, and 345117. BS is the grant recipient of COV20-025. KSA is the grant recipient of 308351, 336138, and 345117. Open access funding was provided by University of Vienna. The funders had no role in study design, data collection and analysis, decision to publish, or preparation of the manuscript.
Competing interests: The authors have declared that no competing interests exist.
Introduction
In early 2020, countries across the world faced rising COVID-19 infection rates, and various physical and social distancing measures to contain the spread of the virus were adopted, including curfews and closures of businesses, schools, and universities. By the end of April 2020, roughly 1.3 billion learners were affected by the closure of educational institutions [ 1 ]. At universities, instruction was urgently switched to distance learning, bearing challenges for all actors involved, particularly for students [ 2 ]. Moreover, since distance teaching requires ample preparation time and situation-specific didactic adaptation to be successful, previously established concepts for and research findings on distance learning cannot be applied undifferentiated to the emergency distance learning situation at hand [ 3 ].
Generally, it has been shown that the less structured learning environment in distance learning requires students to regulate their learning and motivation more independently [ 4 ]. In distance learning in particular, high intrinsic motivation has proven to be decisive for learning success, whereas low intrinsic motivation may lead to maladaptive behavior like procrastination (delaying an intended course of action despite negative consequences) [ 5 , 6 ]. According to self-determination theory (SDT), satisfaction of the three basic psychological needs for autonomy, competence and social relatedness leads to higher intrinsic motivation [ 7 ], which in turn promotes adaptive patterns of learning behavior. On the other hand, dissatisfaction of these basic psychological needs can detrimentally affect intrinsic motivation. According to SDT, satisfaction of the basic psychological needs occurs in interaction with the social environment. The context in which learning takes place as well as the support of social interactions it encompasses play a major role for basic need satisfaction [ 7 , 8 ]. Distance learning, particularly when it occurs simultaneously with other physical and social distancing measures, may impair basic need satisfaction and, in consequence, intrinsic motivation and learning behavior.
The aim of this study was to investigate the relationship between basic need satisfaction and two important learning behaviors—procrastination (as a consequence of low or absent intrinsic motivation) and persistence (as the volitional implementation of motivation)—in the context of emergency distance learning during the COVID-19 pandemic. In line with SDT [ 7 ] and previous studies (e.g., [ 9 ]), we also investigated the mediating role of intrinsic motivation in this relationship. Furthermore, to test the universal importance of SDT for intrinsic motivation and learning behavior under these specific circumstances, we collected data in 17 countries in Europe, Asia, and North America.
The fundamental role of basic psychological needs for intrinsic motivation and learning behavior
SDT [ 7 ] provides a broad framework for understanding human motivation, proposing that the three basic psychological needs for autonomy, competence, and social relatedness must be satisfied for optimal functioning and intrinsic motivation. The need for autonomy refers to an internal perceived locus of control and a sense of agency. In an academic context, students who learn autonomously feel that they have an active choice in shaping their learning process. The need for competence refers to the feeling of being effective in one’s actions. In addition, students who perceive themselves as competent feel that they can successfully meet challenges and accomplish the tasks they are given. Finally, the need for social relatedness refers to feeling connected to and accepted by others. SDT proposes that the satisfaction of each of these three basic needs uniquely contributes to intrinsic motivation, a claim that has been proved in numerous studies and in various learning contexts. For example, Martinek and colleagues [ 10 ] found that autonomy satisfaction was positively whereas autonomy frustration was negatively related to intrinsic motivation in a sample of university students during COVID-19. The same held true for competence satisfaction and dissatisfaction. A recent study compared secondary school students who perceived themselves as highly competent in dealing with their school-related tasks during pandemic-induced distance learning to those who perceived themselves as low in competence [ 11 ]. Students with high perceived competence not only reported higher intrinsic motivation but also implemented more self-regulated learning strategies (such as goal setting, planning, time management and metacognitive strategies) and procrastinated less than students who perceived themselves as low in competence. Of the three basic psychological needs, the findings on the influence of social relatedness on intrinsic motivation have been most ambiguous. While in some studies, social relatedness enhanced intrinsic motivation (e.g., [ 12 ]), others could not establish a clear connection (e.g., [ 13 ]).
Intrinsic motivation, in turn, is regarded as particularly important for learning behavior and success (e.g., [ 6 , 14 ]). For example, students with higher intrinsic motivation tend to engage more in learning activities [ 9 , 15 ], show higher persistence [ 16 ] and procrastinate less [ 6 , 17 , 18 ]. Notably, intrinsic motivation is considered to be particularly important in distance learning, where students have to regulate their learning themselves. Distance-learning students not only have to consciously decide to engage in learning behavior but also persist despite manifold distractions and less external regulation [ 4 ].
Previous research also indicates that the satisfaction of each basic need uniquely contributes to the regulation of learning behavior [ 19 ]. Indeed, studies have shown a positive relationship between persistence and the three basic needs (autonomy [ 20 ]; competence [ 21 ]; social relatedness [ 22 ]). Furthermore, all three basic psychological needs have been found to be related to procrastination. In previous research with undergraduate students, autonomy-supportive teaching behavior was positively related to satisfaction of the needs for autonomy and competence, both of which led to less procrastination [ 23 ]. A qualitative study by Klingsieck and colleagues [ 18 ] supports the findings of previous studies on the relations of perceived competence and autonomy with procrastination, but additionally suggests a lack of social relatedness as a contributing factor to procrastination. Haghbin and colleagues [ 24 ] likewise found that people with low perceived competence avoided challenging tasks and procrastinated.
SDT has been applied in research across various contexts, including work (e.g., [ 25 ]), health (e.g., [ 26 ]), everyday life (e.g., [ 27 ]) and education (e.g., [ 15 , 28 ]). Moreover, the pivotal role of the three basic psychological needs for learning outcomes and functioning has been shown across multiple countries, including collectivistic as well as individualistic cultures (e.g., [ 29 , 30 ]), leading to the conclusion that satisfaction of the three basic needs is a fundamental and universal determinant of human motivation and consequently learning success [ 31 ].
Self-determination theory in a distance learning setting during COVID-19
As Chen and Jang [ 28 ] observed, SDT lends itself particularly well to investigating distance learning, as the three basic needs for autonomy, competence and social relatedness all relate to important aspects of distance learning. For example, distance learning usually offers students greater freedom in deciding where and when they want to learn [ 32 ]. This may provide students with a sense of agency over their learning, leading to increased perceived autonomy. At the same time, it requires students to regulate their motivation and learning more independently [ 4 ]. In the unique context of distance learning during COVID-19, it should be noted that students could not choose whether and to what extent to engage in distance learning, but had to comply with external stipulations, which in turn may have had a negative effect on perceived autonomy. Furthermore, distance learning may also influence perceived competence, as this is in part developed by receiving explicit or implicit feedback from teachers and peers [ 33 ]. Implicit feedback in particular may be harder to receive in a distance learning setting, where informal discussions and social cues are largely absent. The lack of face-to-face contact may also impede social relatedness between students and their peers as well as students and their teachers. Well-established communication practices are crucial for distance learning success (see [ 34 ] for an overview). However, providing a nurturing social context requires additional effort and guidance from teachers, which in turn necessitates sufficient skills and preparation on their part [ 34 , 35 ]. Moreover, the sudden switch to distance learning due to COVID-19 did not leave teachers and students time to gradually adjust to the new learning situation [ 36 ]. As intrinsic motivation is considered particularly relevant in the context of distance education [ 28 , 37 ], applying the SDT framework to the novel situation of pandemic-induced distance learning may lead to important insights that allow for informed recommendations for teachers and educational institutions about how to proceed in the context of continued distance teaching and learning.
In summary, the COVID-19 situation is a completely new environment, and basic need satisfaction during learning under pandemic-induced conditions has not been explored before. Considering that closures of educational institutions have affected billions of students worldwide and have been strongly debated in some countries, it seems particularly relevant to gain insights into which factors consistently influence conducive or maladaptive learning behavior in these circumstances in a wide range of countries and contextual settings.
Therefore, the overall goal of this study is to investigate the well-established relationship between the three basic needs for autonomy, competence, and social relatedness with intrinsic motivation in the new and specific situation of pandemic-induced distance learning. Firstly, we examine the relationship between each of the basic needs with intrinsic motivation. We expect that perceived satisfaction of the basic needs for autonomy (H1a), competence (H1b) and social relatedness (H1c) would be positively related to intrinsic motivation. In our second research question, we furthermore extend SDT’s predictions regarding two important aspects of learning behavior–procrastination (as a consequence of low or absent intrinsic motivation) and persistence (as the implementation of the volitional part of motivation) and hypothesize that each basic need will be positively related to persistence and negatively related to procrastination, both directly (procrastination: H2a –c; persistence: H3a –c) and mediated by intrinsic motivation (procrastination: H4a –c; persistence: H5a –c). We also proposed that perceived autonomy, competence, and social relatedness would have a direct negative relation with procrastination (H6a –c) and a direct positive relation with persistence (H7a –c). Finally, we investigate SDT’s claim of universality, and assume that the aforementioned relationships will emerge across countries we therefore expect a similar pattern of results in all observed countries (H8a –c). As previous studies have indicated that gender [ 4 , 17 , 38 ] and age [ 39 , 40 ]. May influence intrinsic motivation, persistence, and procrastination, we included participants’ gender and age as control variables.
Study design
Due to the circumstances, we opted for a cross-sectional study design across multiple countries, conducted as an online survey. We decided for an online-design due to the pandemic-related restrictions on physical contact with potential survey participants as well as due to the potential to reach a larger audience. As we were interested in the current situation in schools than in long-term development, and we were particularly interested in a large-scale section of the population in multiple countries, we decided on a cross-sectional design. In addition, a multi-country design is particularly interesting in a pandemic setting: During this global health crisis, educational institutions in all countries face the same challenge (to provide distance learning in a way that allows students to succeed) but do so within different frameworks depending on the specific measures each country has implemented. This provides a unique basis for comparing the effects of need fulfillment on students’ learning behavior cross-nationally, thus testing the universality of SDT.
Sample and procedure
The study was carried out across 17 countries, with central coordination taking place in Austria. It was approved and supported by the Austrian Federal Ministry of Education, Science and Research and conducted online. International cooperation partners were recruited from previously established research networks (e.g., European Family Support Network [COST Action 18123]; Transnational Collaboration on Bullying, Migration and Integration at School Level [COST Action 18115]; International Panel on Social), resulting in data collection in 16 countries (Albania, China, Croatia, Estonia, Finland, Germany, Iceland, Japan, Kosovo, Lithuania, Poland, Malta, North Macedonia, Romania, Sweden, USA) in addition to Austria. Data collection was carried out between April and August 2020. During this period, all participating countries were in some degree of pandemic-induced lockdown, which resulted in universities temporarily switching to distance learning. The online questionnaires were distributed among university students via online surveys by the research groups in each respective country. No restrictions were placed on participation other than being enrolled at a university in the sampling country. Participants were informed about the goals of the study, expected time it would take to fill out the questionnaire, voluntariness of participation and anonymity of the acquired data. All research partners ensured that all ethical and legal requirements related to data collection in their country context were met.
Only data from students who gave their written consent to participate, had reached the age of majority (18 or older) and filled out all questions regarding the study’s main variables were included in the analyses (for details on data cleaning rules and exclusion criteria, see [ 41 ]). Additional information on data collection in the various countries is provided in S1 Table in S1 File .
The overall sample of N = 15,462 students was predominantly female (71.7%, 27.4% male and 0.7% diverse) and ranged from 18 to 71 years, with the average participant age being 24.41 years ( SD = 6.93, Mdn = 22.00). Sample descriptives per country are presented in Table 1 .
- PPT PowerPoint slide
- PNG larger image
- TIFF original image
https://doi.org/10.1371/journal.pone.0257346.t001
The variables analyzed here were part of a more extensive questionnaire; the complete questionnaire, as well as the analysis code and the data set, can be found at OSF [ 42 ] In order to take the unique situation into account, existing scales were adapted to the current pandemic context (e.g., adding “In the current home-learning situation …”), and supplemented with a small number of newly developed items. Subsequently, the survey was revised based on expert judgements from our research group and piloted with cognitive interview testing. The items were sent to the research partners in English and translated separately by each respective research team either using the translation-back-translation method or by at least two native-speaking experts. Subsequently, any differences were discussed, and a consolidated version was established.
To assure the reliability of the scales, we analyzed them using alpha coefficients separately for each country (see S2–S18 Tables in S1 File ). All items were answered on a rating scale from 1 (= strongly agree) to 5 (= strongly disagree) and students were instructed to answer with regard to the current situation (distance learning during the COVID-19 lockdown). Analyses were conducted with recoded items so that higher values reflected higher agreement with the statements.
Perceived autonomy was measured with two newly constructed items (“Currently, I can define my own areas of focus in my studies” and “Currently, I can perform tasks in the way that best suits me”; average α = .78, ranging from .62 to .86).
Perceived competence was measured with three items, which were constructed based on the Work-related Basic Need Satisfaction Scale (W-BNS; [ 25 ]) and transferred to the learning context (“Currently, I am dealing well with the demands of my studies”, “Currently, I have no doubts about whether I am capable of doing well in my studies” and “Currently, I am managing to make progress in studying for university”; average α = .83, ranging from .74 to .91).
Perceived social relatedness was assessed with three items, based on the W-BNS [ 43 ], (“Currently, I feel connected with my fellow students”, “Currently, I feel supported by my fellow students”) and the German Basic Psychological Need Satisfaction and Frustration Scale [ 44 ]; “Currently, I feel connected with the people who are important to me (family, friends)”; average α = .73, ranging from .64 to .88).
Intrinsic motivation was measured with three items which were slightly adapted from the Scales for the Measurement of Motivational Regulation for Learning in University Students (SMR-LS; [ 45 ]; “Currently, doing work for university is really fun”, “Currently, I am really enjoying studying and doing work for university” and “Currently, I find studying for university really exciting”; average α = .91, ranging from .83 to .94).
Procrastination was measured with three items adapted from the Procrastination Questionnaire for Students (Prokrastinationsfragebogen für Studierende; PFS; [ 46 ]): “In the current home-learning situation, I postpone tasks until the last minute”, “In the current home-learning situation, I often do not manage to start a task when I set out to do so”, and “In the current home-learning situation, I only start working on a task when I really need to”; average α = .88, ranging from .74 to .91).
Persistence was measured with three items adapted from the EPOCH measure [ 47 ]: “In the current home-learning situation, I finish whatever task I begin”, “In the current home-learning situation, I keep at my tasks until I am done with them” and “In the current home-learning situation, once I make a plan to study, I stick to it”; average α = .81, ranging from .74 to .88).
Data analysis.
Data analyses were conducted using IBM SPSS version 26.0 and Mplus version 8.4. First, we tested for measurement invariance between countries prior to any substantial analyses. We conducted a multigroup confirmatory factor analysis (CFAs) for all scales individually to test for configural, metric, and scalar invariance [ 48 , 49 ] (see S19 Table in S1 File ). We used maximum likelihood parameter estimates with robust standard errors (MLR) to deal with the non-normality of the data. CFI and RMSEA were used as indicators for absolute goodness of model fit. In line with Hu and Bentler [ 50 ], the following cutoff scores were considered to reflect excellent and adequate fit to the data, respectively: (a) CFI > 0.95 and CFI > 0.90; (b) RMSEA < .06 and RMSEA < .08. Relative model fit was assessed by comparing BICs of the nested models, with smaller BIC values indicating a better trade-off between model fit and model complexity [ 51 ]. Configural invariance indicates a factor structure that is universally applicable to all subgroups in the analysis, metric invariance implies that participants across all groups attribute the same meaning to the latent constructs measured, and scalar invariance indicates that participants across groups attribute the same meaning to the levels of the individual items [ 51 ]. Consequently, the extent to which the results can be interpreted depends on the level of measurement invariance that can be established.
For the main analyses, three latent multiple group mediation models were computed, each including one of the basic psychological needs as a predictor, intrinsic motivation as the mediator and procrastination and persistence as the outcomes. These three models served to test the hypothesis that perceived autonomy, competence and social relatedness are related to levels of procrastination and persistence, both directly and mediated through intrinsic motivation. We used bootstrapping in order to provide analyses robust to non-normal distribution variations, specifying 5,000 bootstrap iterations [ 52 ]. Results were estimated using the maximum likelihood (ML) method. Bias-corrected bootstrap confidence intervals are reported.
Finally, in an exploratory step, we investigated the international applicability of the direct and mediated effects. To this end, an additional set of latent mediation models was computed where the path estimates were fixed in order to create an average model across all countries. This was prompted by the consistent patterns of results across countries we observed in the multigroup analyses. Model fit indices of these average models were compared to those of the multigroup models in order to establish the similarity of path coefficients between countries.
Statistical prerequisites
Table 2 provides overall descriptive statistics and correlations for all variables (see S2–S18 Tables in S1 File for descriptive statistics for the individual countries).
https://doi.org/10.1371/journal.pone.0257346.t002
Metric measurement variance, but not scalar measurement invariance could be established for a simple model including the three individual items and no inter-correlations between perceived competence, perceived social relatedness, intrinsic motivation, and procrastination. For these four variables, the metric invariance model had a good absolute fit, whereas the scalar model did not, due to too high RMSEA; moreover, the relative fit was best for the metric model compared to both the configural and scalar model (see S18 Table in S1 File ). Metric, but not scalar invariance could also be established for persistence after modelling residual correlations between items 1 and 2 and items 2 and 3 of the scale. This was necessary due to the similar wording of the items (see “Measures” section for item wordings). Consequently, the same residual correlations were incorporated into all mediation models.
Finally, as the perceived autonomy scale consisted of only two items, it had to be fitted in a model with a correlating factor in order to compute measurement invariance. Both perceived competence and perceived social relatedness were correlated with perceived autonomy ( r = .59** and r = .31**, respectively; see Table 2 ). Therefore, we fit two models combining perceived autonomy with each of these factors; in both cases, metric measurement invariance was established (see S19 Table in S1 File ).
In summary, these results suggest that the meaning of all constructs we aimed to measure was understood similarly by participants across different countries. Consequently, we were able to fit the same mediation model in all countries and compare the resulting path coefficients.
Both gender and age were statistically significantly correlated with perceived competence, perceived social relatedness, intrinsic motivation, procrastination, and persistence (see S20–S22 Tables in S1 File ).
Mediation analyses
Autonomy hypothesis..
We hypothesized that higher perceived autonomy would relate to less procrastination and more persistence, both directly and indirectly (mediated through intrinsic learning motivation). Indeed, perceived autonomy was related negatively to procrastination (H6a) in most countries. Confidence intervals did not include zero in 10 out of 17 countries, all effect estimates were negative and standardized effect estimates ranged from b stand = - .02 to -.46 (see Fig 1 ). Furthermore, perceived autonomy was directly positively related to persistence in most countries. Specifically, for the direct effect of perceived autonomy on persistence (H7a), all but one country (USA, b stand = -.02; p = .621; CI [-.13, .08]) exhibited distinctly positive effect estimates ranging from b stand = .18 to .72 and confidence intervals that did not include zero.
Countries are ordered by sample size from top (highest) to bottom (lowest).
https://doi.org/10.1371/journal.pone.0257346.g001
In terms of indirect effects of perceived autonomy on procrastination mediated by intrinsic motivation (H7a), confidence intervals did not include zero in 8 out of 17 countries and effect estimates were mostly negative, ranging from b stand = -.33 to .03. Indirect effects of perceived autonomy on persistence (mediated by intrinsic motivation; H5a) were distinctly positive and confidence intervals did not include zero in 12 out of 17 countries. The indirect effect estimates and confidence intervals for all remaining countries were consistently positive, with the standardized effect estimates ranging from b stand = .13 to .39, indicating a robust, positive mediated effect of autonomy on persistence. Fig 2 displays the unstandardized path coefficients and their two-sided 5% confidence intervals for the indirect effects of perceived autonomy on procrastination via intrinsic motivation (left) and of perceived autonomy on persistence via intrinsic motivation (right).
https://doi.org/10.1371/journal.pone.0257346.g002
Unstandardized and standardized path coefficients, standard errors, p-values and bias-corrected bootstrapped confidence intervals for the direct and indirect effects of perceived autonomy on procrastination and persistence for each country are provided in S23–S26 Tables in S1 File , respectively.
Competence hypothesis. Secondly, we hypothesized that higher perceived competence would relate to less procrastination and more persistence both directly and indirectly, mediated through intrinsic learning motivation. Direct effects on procrastination (H6b) were negative in most countries and confidence intervals did not include zero in 10 out of 17 countries (see Fig 3 ).
https://doi.org/10.1371/journal.pone.0257346.g003
Standardized effect estimates ranged from b stand = -.02 to -.60, with 10 out of 17 countries exhibiting at least a medium-sized effect. Correspondingly, effect estimates for the direct effects on persistence were positive everywhere except the USA and confidence intervals did not include zero in 14 out of 17 countries (see Fig 3 ). Standardized effect estimates ranged from b stand = -.05 to .64 with 14 out of 17 countries displaying an at least medium-sized positive effect.
The pattern of results for the indirect effects of perceived competence on procrastination mediated by learning motivation (H4b) is illustrated in Fig 4 : Effect estimates were negative with the exception of China and the USA. Confidence intervals did not include zero in 7 out of 17 countries. Standardized effect estimates range between b stand = .06 and -.46. Indirect effects of perceived competence on persistence were positive everywhere except for two countries and confidence intervals did not include zero in 7 out of 17 countries (see Fig 4 ). Standardized effect estimates varied between b stand = -.07 and .46 (see S23–S26 Tables in S1 File for unstandardized and standardized path coefficients).
https://doi.org/10.1371/journal.pone.0257346.g004
Social relatedness hypothesis.
Finally, we hypothesized that stronger perceived social relatedness would be both directly and indirectly (mediated through intrinsic learning motivation) related to less procrastination and more persistence. The pattern of results was more ambiguous here than for perceived autonomy and perceived competence. Direct effect estimates on procrastination (H6c) were negative in 12 countries; however, the confidence intervals included zero in 12 out of 17 countries (see Fig 5 ). Standardized effect estimates ranged from b stand = -.01 to b stand = .33. The direct relation between perceived social relatedness and persistence (H7c) yielded 14 negative and three positive effect estimates. Confidence intervals did not include zero in 7 out of 17 countries (see Fig 5 ), with standardized effect estimates ranging from b stand = -.01 to b stand = .31.
https://doi.org/10.1371/journal.pone.0257346.g005
In terms of indirect effects of perceived social relatedness being related to procrastination mediated by intrinsic motivation (H4c), the pattern of results was consistent: All effect estimates except those for the USA were clearly negative, and confidence intervals did not include zero in 15 out of 17 countries (see Fig 6 ). Standardized effect estimates ranged between b stand = .00 and b stand = -.46. Indirect paths of perceived social relatedness on persistence showed positive effect estimates and standardized effect estimates ranging from b stand = .00 to .44 and confidence intervals not including zero in 16 out of 17 countries (see Fig 6 ; see S23–S26 Tables in S1 File for unstandardized and standardized path coefficients).
https://doi.org/10.1371/journal.pone.0257346.g006
Meta-analytic approach
Due to the overall similarity of the results across many countries, we decided to compute, in an additional, exploratory step, the same models with path estimates fixed across countries. This resulted in three models with average path estimates across the entire sample. Standardized path coefficients for the direct and indirect effects of the basic psychological needs on procrastination and persistence are presented in S27 and S28 Tables in S1 File , respectively. We compared the model fits of these three average models to those of the multigroup mediation models: If the fit of the average model is better than that of the multigroup model, it indicates that the individual countries are similar enough to be combined into one model. The amount of explained variance per model, outcome variable and country are provided in S29 Table in S1 File for procrastination and S30 Table in S1 File for persistence.
Perceived autonomy.
Relative model fit was better for the perceived autonomy model with fixed paths (BIC = 432,707.89) compared to the multigroup model (BIC = 432,799.01). Absolute model fit was equally good in the multigroup model (RMSEA = 0.05, CFI = 0.98, TLI = 0.97) and in the fixed path model (RMSEA = 0.05, CFI = 0.97, TLI = 0.97). Consequently, the general model in Fig 7 describes the data from all 17 countries equally well. The average amount of explained variance, however, is slightly higher in the multigroup model, with 19.9% of the variance in procrastination and 33.7% of the variance in persistence explained, as compared to 18.3% and 27.6% in the fixed path model. The amount of variance explained increased substantially in some countries when fixing the paths: in the multigroup model, explained variance ranges from 2.2% to 44.4% for procrastination and from 0.9% to 69.9% for persistence, compared to 13.0% - 27.7% and 18.2% to 63.2% in the fixed path model. Notably, the amount of variance explained did not change much in the three countries with the largest samples, Austria, Sweden, and Finland; countries with much smaller samples and larger confidence intervals were more affected.
*** p = < .001.
https://doi.org/10.1371/journal.pone.0257346.g007
Overall, perceived autonomy had significant direct and indirect effects on both procrastination and persistence; higher perceived autonomy was related to less procrastination directly ( b unstand = -.27, SE = .02, p = < .001) and mediated by learning motivation ( b unstand = -.20, SE = .01, p = < .001) and to more persistence directly ( b unstand = .24, SE = .01, p = < .001) and mediated by learning motivation ( b unstand = .12, SE = .01, p = < .001). Direct effects for the autonomy model are shown in Fig 7 ; for the indirect effects see Table 3 .
https://doi.org/10.1371/journal.pone.0257346.t003
Effects of age and gender varied across countries (see S20 Table in S1 File ).
Perceived competence.
For the perceived competence model, relative fit decreased when fixing the path coefficient estimates (BIC = 465,830.44 to BIC = 466,020.70). The absolute fit indices were also better for the multigroup model (RMSEA = 0.05, CFI = 0.97, TLI = 0.96) than for the fixed path model (RMSEA = 0.06, CFI = 0.96, TLI = 0.96). Hence, multigroup modelling describes the data across all countries somewhat better than a fixed path model as depicted in Fig 8 . Correspondingly, the fixed path model explained less variance on average than did the multigroup model, with 23.2% instead of 24.3% of the variance in procrastination and 32.9% instead of 37.3% of the variance in persistence explained. Explained variance ranged from 1.0% to 51.9% for procrastination in the multigroup model, as compared to 13.9% - 34.4% in the fixed path model. The amount of variance in persistence explained ranged from 1.0% to 58.1% in the multigroup model and from 23.5% to 55.9% in the fixed path model (see S29 and S30 Tables in S1 File ).
https://doi.org/10.1371/journal.pone.0257346.g008
Overall, higher perceived competence was related to less procrastination ( b unstand = -.44, SE = .02, p = < .001) and to higher persistence ( b unstand = .32, SE = .01, p = < .001). These effects were partly mediated by intrinsic learning motivation ( b unstand = -.11, SE = .01, p = < .001, and b unstand = .07, SE = .01, p = < .001, respectively; see Table 3 ). Effects of gender and age varied between countries, see S21 Table in S1 File .
Perceived social relatedness.
Finally, the perceived social relatedness model with fixed paths had a relatively better model fit (BIC = 479,428.46) than the multigroup model (BIC = 479,604.61). Likewise, the absolute model fit was similar in the model with path coefficients fixed across countries (RMSEA = 0.05, CFI = 0.97, TLI = 0.96) and the multigroup model (RMSEA = 0.05, CFI = 0.97, TLI = 0.97). The multigroup model explained 17.6% of the variance in procrastination and 26.3% of the variance in persistence, as compared to 15.2% and 21.6%, respectively in the fixed path model. Explained variance for procrastination ranged between 0.5% and 48.1% in the multigroup model, and from 9.0% to 23.0% in the fixed path model. Similarly, the multigroup model explained between 1.0% and 56.5% of the variance in persistence across countries, while the fixed path model explained between 15.6% and 48.3% (see S29 and S30 Tables in S1 File ).
Hence, the fixed path model depicted in Fig 9 is well-suited for describing data across all 17 countries. Higher perceived social relatedness is related to less procrastination both directly ( b unstand = -.06, SE = .01, p = < .001) and indirectly through learning motivation ( b unstand = -.12, SE = .01, p = < .001). Likewise, it is related to higher persistence both directly ( b unstand = .07, SE = .01, p = < .001) and indirectly through learning motivation ( b unstand = .08, SE = .00, p = < .001; see Table 3 ). Effects of gender and age are shown in S22 Table in S1 File .
https://doi.org/10.1371/journal.pone.0257346.g009
The aim of this study was to extend current research on the association between the basic psychological needs for autonomy, competence, and social relatedness with intrinsic motivation and two important aspects of learning behavior—procrastination and persistence—in the new and unique situation of pandemic-induced distance learning. We also investigated SDT’s [ 7 ] postulate that the relation between basic psychological need satisfaction and active (persistence) as well as passive (procrastination) learning behavior is mediated by intrinsic motivation. To test the theory’s underlying claim of universality, we collected data from N = 15,462 students across 17 countries in Europe, Asia, and North America.
Confirming our hypothesis, we found that the three basic psychological needs were consistently and positively related to intrinsic motivation in all countries except for the USA (H1a - c). This consistent result is in line with self-determination theory [ 7 ] and other previous studies (e.g., 9), which have found that satisfaction of the three basic needs for autonomy, competence and social relatedness is related to higher intrinsic motivation. Notably, the association with intrinsic motivation was stronger for perceived autonomy and perceived competence than for perceived social relatedness. This also has been found in previous studies [ 4 , 9 , 28 ]. Pandemic-induced distance learning, where physical and subsequential social contact in all areas of life was severely constricted, might further exacerbate this discrepancy, as instructors may have not been able to establish adequate communication structures due to the rapid switch to distance learning [ 36 , 53 ]. As hypothesized, intrinsic motivation was in general negatively related to procrastination (H2a - c) and positively related to persistence (H3a - c), indicating that students who are intrinsically motivated are less prone to procrastination and more persistent when studying. This again underlines the importance of intrinsic motivation for adaptive learning behavior, even and particularly in a distance learning setting, where students are more prone to disengage from classes [ 34 ].
The mediating effect of intrinsic motivation on procrastination and persistence
Direct effects of the basic needs on the outcomes were consistently more ambiguous (with smaller effect estimates and larger confidence intervals, including zero in more countries) than indirect effects mediated by intrinsic motivation. This difference was particularly pronounced for perceived social relatedness, where a clear negative direct effect on procrastination (H6c) could be observed only in the three countries with the largest sample size (Austria, Sweden, Finland) and Romania, whereas the confidence interval in most countries included zero. Moreover, in Estonia there was even a clear positive effect. The unexpected effect in the Estonian sample may be attributed to the fact that this country collected data only from international exchange students. Since the lockdown in Estonia was declared only a few weeks after the start of the semester, international exchange students had only a very short period of time to establish contacts with fellow students on site. Accordingly, there was probably little integration into university structures and social contacts were maintained more on a personal level with contacts from the home country. Thus, such students’ fulfillment of this basic need might have required more time and effort, leading to higher procrastination and less persistence in learning.
A diametrically opposite pattern was observed for persistence (H7c), where some direct effects of social relatedness were unexpectedly negative or close to zero. We therefore conclude that evidence for a direct negative relationship between social relatedness and procrastination and a direct positive relationship between social relatedness and persistence is lacking. This could be due to the specificity of the COVID-19 situation and resulting lockdowns, in which maintaining social contact took students’ focus off learning. In line with SDT, however, indirect effects of perceived social relatedness on procrastination (H4c) and persistence (H5c) mediated via intrinsic motivation were much more visible and in the expected directions. We conclude that, while the direct relation between perceived social relatedness and procrastination is ambiguous, there is strong evidence that the relationship between social relatedness and the measured learning behaviors is mediated by intrinsic motivation. Our results strongly underscore SDT’s assumption that close social relations promote intrinsic motivation, which in turn has a positive effect on learning behavior (e.g., [ 6 , 14 ]). The effects for perceived competence exhibited a somewhat clearer and hypothesis-conforming pattern. All direct effects of perceived competence on procrastination (H6b) were in the expected negative direction, albeit with confidence intervals spanning zero in 7 out of 17 countries. Direct effects of perceived competence on persistence (H7b) were consistently positive with the exception of the USA, where we observed a very small and non-significant negative effect. Indirect effects of perceived competence on procrastination (H4b) and persistence (H5b) as mediated by intrinsic motivation were mostly consistent with our expectations as well. Considering this overall pattern of results, we conclude that there is strong evidence that perceived competence is negatively associated with procrastination and positively associated with persistence. Furthermore, our results also support SDT’s postulate that the relationship between perceived competence and the measured learning behaviors is mediated by intrinsic motivation.
It is notable that the estimated direct effects of perceived competence on procrastination and persistence were higher than the indirect effects in most countries we investigated. Although SDT proposes that perceived competence leads to higher intrinsic motivation, Deci and Ryan [ 8 ] also argue that it affects all types of motivation and regulation, including less autonomous forms such as introjected and identified motivation, indicating that if the need for competence is not satisfied, all types of motivation are negatively affected. This may result in a general amotivation and lack of action. In our study, we only investigated intrinsic motivation as a mediator. For future research, it might be advantageous to further differentiate between different types of externally and internally controlled behavior. Furthermore, perceived competence increases when tasks are experienced as optimally challenging [ 7 , 54 ]. However, in order for instructors to provide the optimal level of difficulty and support needed, frequent communication with students is essential. Considering that data collection for the present study took place at a time of great uncertainty, when many countries had only transitioned to distance learning a few weeks prior, it is reasonable to assume that both structural support as well as communication and feedback mechanisms had not yet matured to a degree that would favor individualized and competency-based work.
However, our findings corroborate those from earlier studies insofar as they underline the associations between perceived competence and positive learning behavior (e.g., [ 19 ]), that is, lower procrastination [ 18 ] and higher persistence (e.g., [ 21 ]), even in an exceptional situation like pandemic-induced distance learning.
Turning to perceived autonomy, although the confidence intervals for the direct effects of perceived autonomy on procrastination (H6a) did span zero in most countries with smaller sample sizes, all effect estimates indicated a negative relation with procrastination. We expected these relationships from previous studies [ 18 , 23 ]; however, the effect might have been even more pronounced in the relatively autonomous learning situation of distance learning, where students usually have increased autonomy in deciding when, where, and how to learn. While this bears the risk of procrastination, it also comes with the opportunity to consciously delay less pressing tasks in favor of other, more important or urgent tasks (also called strategic delay ) [ 5 ], resulting in lower procrastination. In future studies, it might be beneficial to differentiate between passive forms of procrastination and active strategic delay in order to obtain more detailed information on the mechanisms behind this relationship. Direct effects of autonomy on persistence (H7a) were consistently positive. Students who are free to choose their preferred time and place to study may engage more with their studies and therefore be more persistent.
Indirect effects of perceived autonomy on procrastination mediated by intrinsic motivation (H4a) were negative in all but two countries (China and the USA), which is generally consistent with our hypothesis and in line with previous research (e.g., [ 23 ]). Additionally, we found a positive indirect effect of autonomy on persistence (H5a), indicating that autonomy and intrinsic motivation play a crucial role in students’ persistence in a distance learning setting. Based on our results, we conclude that perceived autonomy is negatively related to procrastination and positively related to persistence, and that this relationship is mediated by intrinsic motivation. It is worth noting that, unlike with perceived competence, the direct and indirect effects of perceived autonomy on the outcomes procrastination and persistence were similarly strong, suggesting that perceived autonomy is important not only as a driver of intrinsic motivation but also at a more direct level. It is important to make the best possible use of the opportunity for greater autonomy that distance learning offers. However, autonomy is not to be equated with a lack of structure; instead, learners should be given the opportunity to make their own decisions within certain framework conditions.
The applicability of self-determination theory across countries
Overall, the results of our mediation analysis for the separate countries support the claim posited by SDT that basic need satisfaction is essential for intrinsic motivation and learning across different countries and settings. In an exploratory analysis, we tested a fixed path model including all countries at once, in order to test whether a simplified general model would yield a similar amount of explained variance. For perceived autonomy and social relatedness, the model fit increased, whereas for perceived competence it decreased slightly compared to the multigroup model. However, all fixed path models exhibited adequate model fit. Considering that the circumstances in which distance learning took place in different countries varied to some degree (see also Limitations), these findings are a strong indicator for the universality of SDT.
Study strengths and limitations
Although the current study has several strengths, including a large sample size and data from multiple countries, three limitations must be considered. First, it must be noted that sample sizes varied widely across the 17 countries in our study, with one country above 6,000 (Austria), two above 1,000 (Finland and Sweden) and the rest ranging between 104 and 905. Random sampling effects are more problematic in smaller samples; hence, this large variation weakens our ability to conduct cross-country comparisons. At the same time, small sample sizes weaken the interpretability of results within each country; thus, our results for Austria, Finland and Sweden are considerably more robust than for the remaining fourteen countries. Additionally, two participating countries collected specific subsamples: In China, participants were only recruited from one university, a nursing school. In Estonia, only international exchange students were invited to participate. Nevertheless, with the exception of the unexpected positive direct relationship between social relatedness and procrastination, all observed divergent effects were non-significant. Indeed, this adds to the support for SDT’s claims to universality regarding the relationship between perceived autonomy, competence, and social relatedness with intrinsic motivation: Results in the included countries were, despite their differing subsamples, in line with the overall trend of results, supporting the idea that SDT applies equally to different groups of learners.
Second, due to the large number of countries in our sample and the overall volatility of the situation, learning circumstances were not identical for all participants. Due to factors such as COVID-19 case counts and national governments’ political priorities, lockdown measures varied in their strictness across settings. Some universities were fully closed, some allowed on-site teaching for particular groups (e.g., students in the middle of a laboratory internship), and some switched to distance learning but held exams on site (see S1 Table in S1 File for further information). Therefore, learning conditions were not as comparable as in a strict experimental setting. On the other hand, this strengthens the ecological validity of our study. The fact that the pattern of results was similar across contexts with certain variation in learning conditions further supports the universal applicability of SDT.
Finally, due to the novelty of the COVID-19 situation, some of the measures were newly developed for this study. Due to the need to react swiftly and collect data on the constantly evolving situation, it was not possible to conduct a comprehensive validation study of the instruments. Nevertheless, we were able to confirm the validity of our instruments in several ways, including cognitive interview testing, CFAs, CR, and measurement invariance testing.
Conclusion and future directions
In general, our results further support previous research on the relation between basic psychological need fulfilment and intrinsic motivation, as proposed in self-determination theory. It also extends past findings by applying this well-established theory to the new and unique situation of pandemic-induced distance learning across 17 different countries. Moreover, it underlines the importance of perceived autonomy and competence for procrastination and persistence in this setting. However, various other directions for further research remain to be pursued. While our findings point to the relevance of social relatedness for intrinsic motivation in addition to perceived competence and autonomy, further research should explore the specific mechanisms necessary to promote social connectedness in distance learning. Furthermore, in our study, we investigated intrinsic motivation, as the most autonomous form of motivation. Future research might address different types of externally and internally regulated motivation in order to further differentiate our results regarding the relations between basic need satisfaction and motivation. Finally, a longitudinal study design could provide deeper insights into the trajectory of need satisfaction, intrinsic motivation and learning behavior during extended periods of social distancing and could provide insights into potential forms of support implemented by teachers and coping mechanisms developed by students.
Supporting information
https://doi.org/10.1371/journal.pone.0257346.s001
- 1. UNESCO [Internet]. 2020. COVID-19 Impact on Education; [cited 13 th March 2021]. Available from: https://en.unesco.org/covid19/educationresponse
- 2. United Nations. Policy Brief: Education during COVID-19 and beyond [cited 13 th March 2021]. [Internet]. 2020. Available from: https://www.un.org/development/desa/dspd/wp-content/uploads/sites/22/2020/08/sg_policy_brief_covid-19_and_education_august_2020.pdf#
- View Article
- Google Scholar
- PubMed/NCBI
- 16. Schunk DH, Pintrich PR, Meece JL. Motivation in education: Theory, research, and applications. 4th ed. London: Pearson Higher Education; 2014.
- 19. Connell JP, Wellborn JG. Competence, autonomy, and relatedness: A motivational analysis of self-system processes. In: Gunnar MR, Sroufe LA, editors. Self-processes and development. Hilsdale: Lawrence Erlbaum Associates; 1991. pp. 43–77.
- 33. Legault L. The Need for Competence. 2017 [cited 22 March 2021]. In: Encyclopedia of Personality and Individual Differences [Internet]. Cham: Springer International Publishing. [pp. 1–3]. Available from: http://link.springer.com/10.1007/978-3-319-28099-8_1123-1
- 36. Hodges C, Moore S, Lockee B, Trust T, Bond A. The difference between emergency remote teaching and online learning. 2020 March 27 [cited 13 th March 2021]. In: Educause Review [Internet]. Available from: https://er.educause.edu/articles/2020/3/the-difference-between-emergency-remote-teaching-and-online-learning
- 37. Mills R. The centrality of learner support in open and distance learning. In: Mills R, Tait A, editors. Rethinking learner support in distance education: Change and continuity in an international context. London: Routledge; 2003. pp. 102–113.
- 41. Schober B, Lüftenegger M, Spiel C. Learning conditions during COVID-19 Students (SUF edition); 2021 [cited 2021 Mar 22]. Database: AUSSDA [Internet]. Available from: https://data.aussda.at/citation?persistentId=10.11587/XIU3TX
- 42. Pelikan ER, Korlat S, Reiter J, Lüftenegger M. Distance Learning in Higher Education During COVID-19: Basic Psychological Needs and Intrinsic Motivation [Internet]. OSF; 2021. Available from: osf.io/8czx3
- 46. Glöckner-Rist A, Engberding M, Höcker A, Rist F. Prokrastinationsfragebogen für Studierende (PfS) [Procrastination Scale for Students]. In: Zusammenstellung sozialwissenschaftlicher Items und Skalen [Summary of items and scales in social science] ZIS Version 1300. Bonn: GESIS; 2014. https://doi.org/10.1017/S0033291714002803 pmid:25482960
- 48. Millsap RE. Statistical approaches to measurement invariance. 1st ed. New York: Routledge; 2011.
- 52. Hayes AF. Introduction to mediation, moderation, and conditional process analysis: a regression-based approach. 2nd ed. New York: Guilford Press; 2018.
ORIGINAL RESEARCH article
Expectations and experiences with online education during the covid-19 pandemic in university students.
- 1 Laboratorio de Investigación e Innovación educativa Dirección de Docencia, Universidad de Concepción, Concepción, Chile
- 2 Programa de Doctorado Educación en Consorcio, Universidad de Católica de la Santísima Concepción, Concepción, Chile
- 3 Departamento de Física, Facultad Ciencias Físicas y Matemáticas, Universidad de Concepción, Concepción, Chile
- 4 Departamento Currículum e Instrucción, Facultad de Educación, Universidad de Concepción, Concepción, Chile
- 5 Departamento de Bioquímica y Biología Molecular, Facultad de Ciencias Biológicas, Universidad de Concepción, Concepción, Chile
Due to COVID-19, university students continued their academic training remotely. To assess the effects of emergency remote teaching (ERT), we evaluated the expectations and, subsequently, the experiences of university students about online education. This study employed a simple prospective design as its method. We assessed the expectations of 1,904 students from different discipline areas (1,106 women and 798 men; age M = 21.56; SD = 3.07) during the beginning of the first semester, March 2020 (T1), and their experiences at the end of the same academic period, September 2020 (T2). We used convenience non-probability sampling. Participants responded to the questionnaire on Expectations toward virtual education in higher education for students and the questionnaire on virtual education experiences in higher education. The results showed that students’ responses reflected low expectations regarding peer relationships and comparison with face-to-face education (T1). This perception was maintained during the evaluation of experiences (T2). Students reported positive experiences regarding online teaching and learning, online assessment, and their self-efficacy beliefs at T2. Statistically significant differences between measurements were found, with the expertise presenting higher averages than expectations. Furthermore, differences by gender were identified, reporting a positive change in the scores of women. In addition, results reflected differences according to the disciplinary area, showing Social Sciences and Medical and Health Sciences students a more significant size effect. Findings regarding the empirical evidence and the implications for future teaching scenarios in Higher Education are discussed.
Introduction
Higher education institutions had to face the challenge of providing continuity to the educational process remotely due to the COVID-19 pandemic. This scenario implied a drastic transformation without the possibility of preparation, having both teachers and students quickly develop online education competencies ( Hattar et al., 2021 ). Emergency remote teaching (ERT) is the name given to this instructional response ( Bustamante, 2020 ; Hodges et al., 2020 ). ERT applies to any unexpected and urgent transition to online instruction due to a disaster. Given its nature, one of the characteristics of ERT is the lack of time and skills of instructors to adequately prepare and implement their course syllabus in a virtual format ( Hodges et al., 2020 ). Thus, ERT differs significantly from online teaching, in which the focus is on delivering a quality learning experience following a predefined instructional design ( Miramontes Arteaga et al., 2019 ).
Currently, online courses are created using an instructional design, such as ADDIE, and implemented through Learning Management Systems (LMS), like Canvas. In these courses, designers and teachers apply technological and pedagogical innovations to obtain high-quality standards. In this teaching modality, educational experiences occur synchronously and asynchronously using multiple devices to access the internet. Therefore, students can interact with teachers, content, and peers from wherever they are ( Singh and Thurman, 2019 ). It requires stable digital infrastructure and platforms. Thus, its implementation demands many resources and a carefully designed plan to deliver a quality experience ( Mousa et al., 2020 ). As necessary and valuable as ERT is, its design does not necessarily consider the critical elements of quality online education ( Hodges et al., 2020 ). Despite the advances in online education in many higher education institutions worldwide, universities, in general, were not prepared for the necessary, mandatory, and abrupt change at the onset of the COVID-19 pandemic ( Maier et al., 2020 ).
Quality online teaching considers evaluating course characteristics, including the design of learning materials, the virtual environment, and the alignment of curricular components with learning outcomes. It also considers aspects related to the interaction experience of students with their peers and teachers ( Rodrigues et al., 2019 ).
Literature Review
Due to the COVID-19 pandemic, students’ expectations about how their academic year would unfold were rapidly modified and adjusted. This is relevant due to empirical evidence that supports that student expectations are predictors of academic success ( Paechter et al., 2010 ; Alhabeeb and Rowley, 2018 ; Wei and Chou, 2020 ). Student expectations can be defined as the beliefs that students hold about successfully coping with academic responsibilities. From the perspective of the expectancy-value theory ( Wigfield and Eccles, 2000 ), students have beliefs about their ability and success in meeting academic demands. These beliefs can be impacted by the subjective perception of the value of the academic activity to the student ( Valle et al., 2015 ). The expectancy-value theory is widely used to understand how psychological and contextual factors enhance student engagement and learning outcomes ( Chiu et al., 2021 ). Furthermore, expectations also impact student attitudes about the ways of learning (Fernández Jiménez et al., 2017 ). It has been reported that students’ perceptions regarding online learning modalities are related to their learning success ( Nur Agung et al., 2020 ). Therefore, expectations and experiences of university students regarding online learning courses during the pandemic could translate into opportunities or obstacles in the sense of moving closer or further away from a practical online education experience in the future ( Rodrigues et al., 2019 ; Pham and Ho, 2020 ).
Several studies have reported a variety of results regarding the expectations and subsequent experience of university students. For example, descriptive research conducted with 1612 undergraduates from 59 on-site Spanish universities says that students consider that the institutions did not adapt adequately to the ERT scenario (84%), especially regarding teaching methods and the implementation of assessments (64.5%). Furthermore, they state that the adopted institutional measures were not sufficient, affecting their academic performance (88.5%) during this period. In terms of experience, in the same research, students were not satisfied with virtual education, especially regarding courses’ assessment ( Villa et al., 2020 ). These results relate to another study that reported that students would not repeat this experience due to the absence of interaction with teachers, excess of tasks, and the accelerated pace for learning ( Imsa-ard, 2020 ; Suárez et al., 2021 ).
Consistent with the above, another study indicates that students perceived an overload in their academic responsibilities due to excessive activities and assignments, which made the process more exhausting ( Rahiem, 2020 ). Moreover, another research from the pandemic experience indicates that young people reported a low perception of quality and quantity in their learning during ERT regarding the strategies implemented by their universities, which did not meet their expectations (31.3%) ( Almomani et al., 2021 ). Additionally, researchers found that, unlike men, women perceived greater satisfaction with the strategies implemented by universities (66%), were more committed to delivering their assignments (70.6%), and were more optimistic about the assessment process implemented by teachers in their courses (70.2%) ( Almomani et al., 2021 ). Another research concludes that online teaching during the COVID-19 pandemic was only possible when online learning had a robust digital infrastructure and a learning system designed for that purpose; otherwise, it was an attempt to replicate face-to-face teaching in the virtual environment ( Abdulrahim and Mabrouk, 2020 ).
Despite the emergency scenario caused by the pandemic, not all studies reported negative experiences ( Abdulrahim and Mabrouk, 2020 ; Sepulveda-Escobar and Morrison, 2020 ). During ERT training, students from various institutions worldwide ( N = 30,383) claimed to be satisfied with the support provided by their instructors and institutions. In this case, specific sociodemographic characteristics such as gender, academic area, and other elements of the students favorably impacted these beliefs ( Aristovnik et al., 2020 ). Students positively assessed the actions implemented by the universities’ Information and Communication Technologies Departments ( Shehzadi et al., 2020 ). In addition, they thoroughly evaluated the online platforms used since they allowed them to perform their tasks efficiently and quickly, having fun while studying ( Maier et al., 2020 ). It is important to note that some authors report differences in experiences according to the scientific disciplines to which students belong ( Vladova et al., 2021a ).
Regarding social aspects, it seems that students were not satisfied with the preparation of teachers during the ERT modality due to difficulties in the interaction with their teachers and peers ( Alqurshi, 2020 ; Hamdan et al., 2021 ). This aspect is consistent with other research highlighting the importance of interaction between instructors and students in the online education experience ( Sun, 2016 ; Bao, 2020 ).
Due to ERT, a negative effect on students’ self-efficacy beliefs about online education has been reported at the individual level ( Aldhahi et al., 2021 ), while others found no changes ( Talsma et al., 2021 ). Self-efficacy is a relevant element regarding students’ academic satisfaction and performance ( Cervantes Arreola et al., 2018 ; Hamdan et al., 2021 ). When students believe in successfully facing the challenges of online education, they display a series of mechanisms to favor a more efficient and effective coping of their learning process. Consequently, beliefs conversion during the ERT may play an essential role in post-pandemic online learning.
In the context of the COVID-19, the academic, social, and individual experiences during ERT affect the perception of online education, which could impact the implementation of this modality in Higher Education in the future.
The Present Study
The empirical evidence described highlights the importance of assessing students’ experience during the ERT, especially the quality of the learning experience, the integration of teaching approaches, the design, the application of assessment tools, and how the relationship between students and their teachers is fostered ( Sun, 2016 ; Alqurshi, 2020 ; Aristovnik et al., 2020 ; Bao, 2020 ; Rahiem, 2020 ; Van Heuvelen et al., 2020 ; Villa et al., 2020 ; Almomani et al., 2021 ; Suárez et al., 2021 ). These aspects will provide vital information for the design and implementation of effective online learning processes that respond to the needs of students and universities in this context in the future.
This study focuses on the importance of learning about students’ expectations and experiences during the implementation of the ERT for the COVID-19 pandemic. Specifically, we inquire on how students’ expectations and experiences can affect their academic, social, and personal aspects to provide evidence to support actions for the transition to face-to-face and blended learning. In this context, this research aims to analyze the expectations and experiences of students in a traditional university in the south of Chile at a general level and in consideration of the participants’ gender and disciplinary area.
Based on the above and the heterogeneity of students’ experiences reported in the literature, we describe the following hypotheses:
H 1 . There will be changes in the experiences to the expectations of university students during ERT due to the COVID-19 pandemic.
H 2 . Differences will be found between men and women regarding university students’ expectations and experience scores during the ERT due to the COVID-19 pandemic.
H 3 . Differences in university students’ expectations and experience scores will be observed according to disciplinary areas during ERT due to the COVID-19 pandemic.
Materials and Methods
A simple ex post facto longitudinal quantitative research design was used. Researchers find it impossible to manipulate the independent variable in ex post facto studies, describing the associations between variables. It is simply longitudinal since two measurements were performed, starting by measuring the expectation (March 2020; T1) and then the experience (September 2020; T2) of the students with online education during the ERT, to subsequently study the relationships found between the variables ( Montero and León, 2007 ).
Participants
A total of 1,904 students belonging to a traditional Chilean university participated, of which 1106 (58.1%) were women, and 798 (41.9%) were men, with mean age M = 21.56 ( SD = 3.07). On the other hand, 635 (33.35%) of the participants were in their first academic year. According to their undergraduate program, students’ classification according to the areas of the Organization for Economic Co-operation and Development (OECD) is presented in Table 1 .
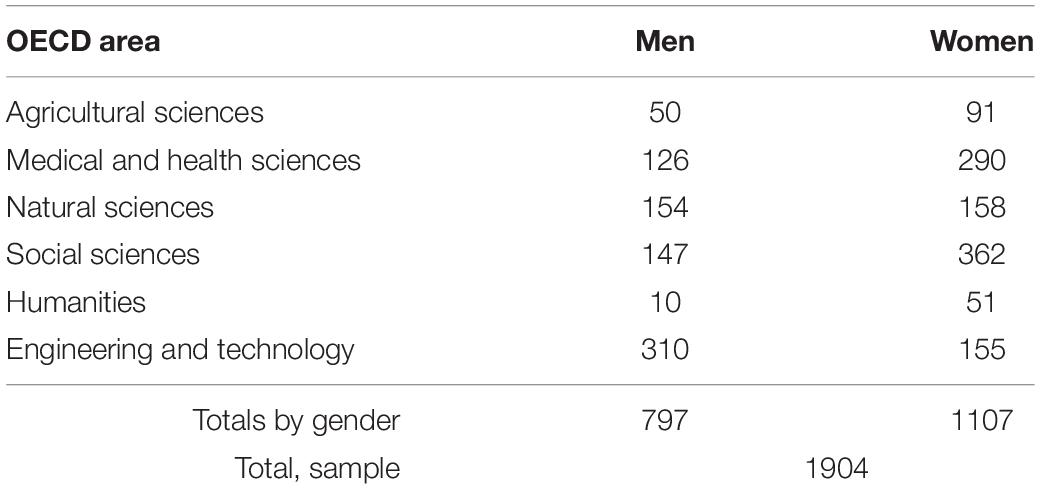
Table 1. Distribution of students by gender and disciplinary area.
Instruments
Expectations toward virtual education.
The Expectations toward Virtual Education in Higher Education for Students (CEEVESE) questionnaire aims to know higher education students’ expectations about virtual education during ERT. It consists of 28 items distributed in six dimensions about virtual education. The items were elaborated based on available scientific literature and evaluated employing expert judgment ( Lobos et al., 2022 ). Table 2 describes the dimensions that constitute the scale.
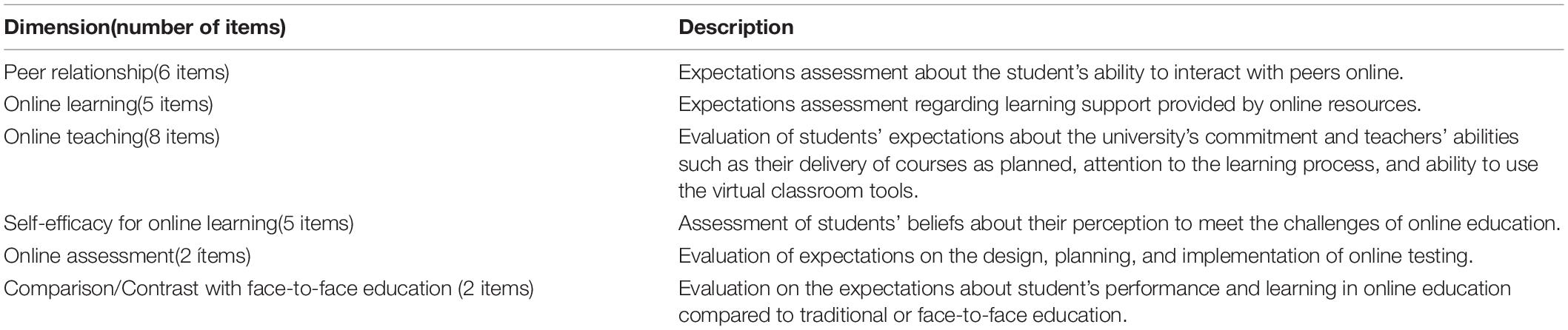
Table 2. Description of the dimensions of the CEEVESE questionnaire.
A Likert scale with five response options (1 = Strongly disagree to 5 = Strongly agree) was employed. The average of each dimension and the full scale was analyzed, in which scores higher than 3 indicate positive expectations. Previous studies have examined the factorial structure of the scale, finding an adequate adjustment of the 6 factors [X 2 (335) = 5354.88, p < 0.001, CFI:0.961; TLI:0.956; SRMR:0.041; RMSEA:0.06]. The reliability analysis of the responses was: peer relationship α = 0.894, online learning α = 0.922; online teaching α = 0.907; self-efficacy for online learning α = 0.882, online assessment α = 0.787; comparison with face-to-face education α = 0.779; full scale: α = 0.954 ( Lobos et al., 2022 ).
Experience in Virtual Education
The Virtual Education Experiences in Higher Education for Students (EEEL) questionnaire adapts the CEEVESE (Lobos et al., under review 1 ). Its purpose is to learn about the experiences of higher education students with virtual education during ERT. It consists of the same 28 items of the CEEVESE but presented in the past tense, using again a Likert scale of 5 response options (1 = Strongly disagree to 5 = Strongly agree). For their interpretation, the averages of each dimension and the full scale were analyzed. In both cases, the presence of scores above 3 points reflects a positive student experience. The items’ distribution corresponds with the six original dimensions.
The factorial structure of this version confirmed an adequate adjustment of the 6 factors [ X 2 (333) = 3599.92, p < 0.001, CFI: 0.966; TLI: 0.961; SRMR: 0.036; RMSEA: 0.059]. Reliability analysis of the responses by dimensions was as follows: peer relationship α = 0.869, online learning α = 0.883; online teaching α = 0.876; self-efficacy for online learning α = 0.872, online assessment α = 0.753; comparison with face-to-face education α = 0.671; full scale: α = 0.931 (Lobos et al., under review, see text footnote 1).
This research was endorsed by the Ethics Committee of the participating university, corroborating the ethical criteria for research with human beings. The expectations and experience instruments were applied in digital format and sent to the students’ institutional emails on a single occasion. For the two measurement moments (T1 and T2), the questionnaires were available for 3 weeks at the beginning of March 2020 and at the end of September 2020. Students responded after reading and signing an informed consent form. A convenience non-probability sampling was used. The participants were students who were enrolled in a course during the first semester of 2020. To track the students, the enrollment number and e-mail address of each participant were compared. Only students presenting both measurements were included.
Analysis Plan
We performed a descriptive analysis of the variables. Verification of the assumption of normality for the dimensions and total scales in both measurements (T1 and T2) was made using the Kolmogorov-Smirnov test with the Lilliefors modification ( Thode, 2002 ). Analyzed data did not have a normal distribution ( p < 0.001). Despite this, the Student’s t -test for paired samples was performed to evaluate the differences in the T1 and T2 scores due to the sample size.
The assumptions were verified using the mixed ANOVA tests to assess the effects between groups on gender and OECD areas versus the intra-group effect (changes between expectations and experience). No extreme outliers were found. Levene’s test was analyzed, finding no significant result ( p > 0.05). The homogeneity of covariance of the between-subjects factor (gender-OECD area) using Box’s M test was also evaluated, with a not statistically significant result ( p > 0.001). Therefore, no violation of the homogeneity of covariances assumption is assumed. Verification of the sphericity assumption was automatic since the Greenhouse-Geisser sphericity correction was applied to violating assumption factors during the ANOVA test calculation.
The size effect was analyzed considering the cutoffs by Cárdenas Castro and Arancibia Martini (2014) , in which scores >0.14 are considered large, 0.06 medium, and 0.01 small. The data analysis was performed with R Studio software version 4.0.3 (2020-10-10) ( R Core Team, 2020 ).
The present research aims to analyze the students’ expectations and experiences, considering the gender and disciplinary area of the participants. We presented the results in the context of the research hypotheses described in section “The Present Study.”
Differences Between University Students’ Expectations and Experiences During Emergency Remote Teaching During the COVID-19 Pandemic
Hypotheses H 1 sought to answer the existence of changes between the expectations and experiences of university students produced by ERT during the COVID-19 pandemic. In the first measurement (T1), the general students’ expectations presented an average below 3 points, identifying them as low ( M = 2.92, SD = 0.65). The dimension that presented the highest score was self-efficacy for online education ( M = 3.42; SD = 0.84), whereas the dimensions that showed the lowest scores were peer relationship ( M = 2.1; SD = 0.83) and comparison with face-to-face teaching ( M = 1.91; SD = 1.07).
Regarding the measurement of the students’ experiences with the ERT after the academic semester (T2), the perception was positive since the score was higher than 3 points ( M = 3.18, SD = 0.66). Furthermore, the analysis by dimensions, identify that dimensions’ averages of the experiences (T2) were higher than its corresponding dimensions of the questionnaire of expectations (T1). However, despite having improved, the dimensions of peer relationship ( M = 2.26, SD = 0.95) and comparison with face-to-face education ( M = 2.71, SD = 1.24) remain as negative perceptions, since scores were still lower than 3. Table 3 shows dimensions’ averages and deviations of the scales applied and the assessment of the differences between them.
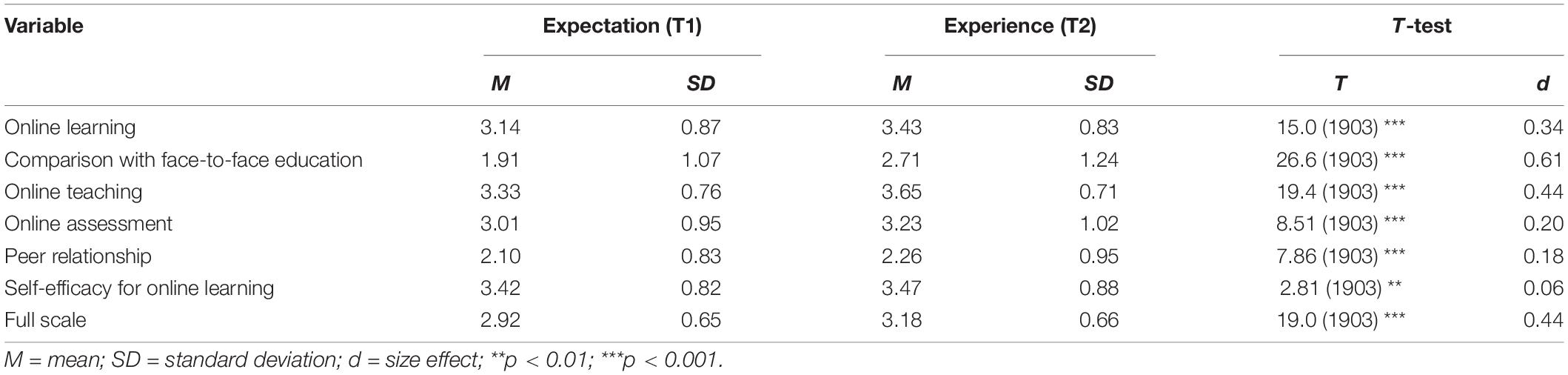
Table 3. Descriptive and inferential statistics on students’ expectations and experiences during the ERT.
When performing the comparative analysis between the general expectations of the students (T1) and the experience after the end of the semester (T2), statistically significant differences [ t (1903) = 19, p < 0.001] were found. Hence, students’ experience with ERT at the end of the academic period exceeded their expectations. In this sense, results respond positively to the proposed hypothesis, identifying differences in the scores between T1 and T2.
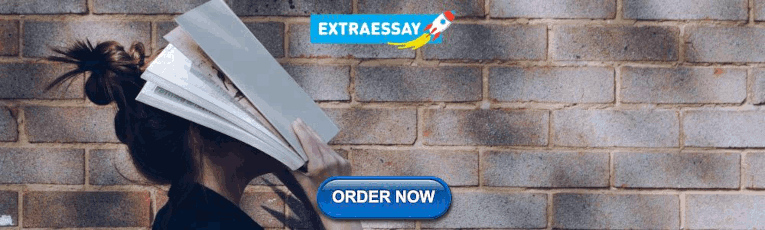
Gender Differences in University Students’ Expectations and Experiences of Online Learning During Emergency Remote Teaching
To analyze differences between expectations and experiences considering gender and OECD area, the presence of statistically significant bidirectional interactions was assessed. Subsequently, we performed post hoc tests to determine the main effects of gender and OECD area, considering the Bonferroni adjusted p -value.
We examined each dimension independently to answer the hypothesis regarding the existence of differences between expectations and experiences related to undergraduate students’ gender during ERT (H 2 ). The results showed statistically significant bidirectional interactions among gender and the change in scores between expectations and experiences in the following five dimensions: online learning [ F (1,1902) = 19.09, p < 0.001, GES.002]; comparison with face-to-face education [ F (1,1902) = 25.23, p < 0.001, GES.004]; online teaching [ F (1,1902) = 5.31, p < 0.001, GES.0006]; peer relationship [ F (1,1902) = 6.79, p < 0.01]; and self-efficacy for online learning [ F (1,1902) = 4.836, p < 0.05, GES.0006]. In the case of the online assessment dimension, no statistically significant results were observed.
Regarding the main effect of gender, a significant effect for experience, but not for expectations in the following four dimensions was observed online learning: experience [ F (1,1902) = 10.64, p < 0.01, GES.006]. Online teaching: experience [ F (1,1902) = 8.54, p < 0.01, GES.004]. Peer relationship: experience [ F (1,1902) = 6.55, p < 0.05, GES = 0.003] and Self-efficacy for online learning: experience [ F (1,1902) = 5.37, p < 0.05, GES.003].
On the other hand, in the case of comparison with face-to-face education, the results were significant for expectation [ F (1,1902) = 13.06, p < 0.001, GES.007], but not for experience ( p = 0.06).
The simple main effect of the differences between expectations and experience were also analyzed, observing statistically significant results for women and men in four of the dimensions: online learning, women [ F (1,1106) = 203, p < 0.001 GES = 0.046] and men [ F (1,796) = 42.1, p < 0.001 GES = 0.011]. In the Comparison with face-to-face education, women [ F (1,1106) = 589.63, p < 0.001 GES = 0.15] and men [ F (1,796) = 169.09, p < 0.001 GES = 0.06]. In the Online teaching, women [ F (1,1106) = 264, p < 0.001 GES = 0.06] and the men [ F (1,796) = 117, p < 0.001 GES = 0.03]. In the peer relationship, women [ F (1,1106) = 57.5, p < 0.001 GES = 0.014] and men [ F (1,796) = 10.1, p < 0.01 GES = 0.003].
In the self-efficacy for online learning dimension, statistically significant results were identified only for women [ F (1,1106) = 13.4, p < 0.001 GES = 0.003]. Even though men and women presented higher scores at T2, women showed the most significant change reflecting a positive experience with online education (see Table 4 ).
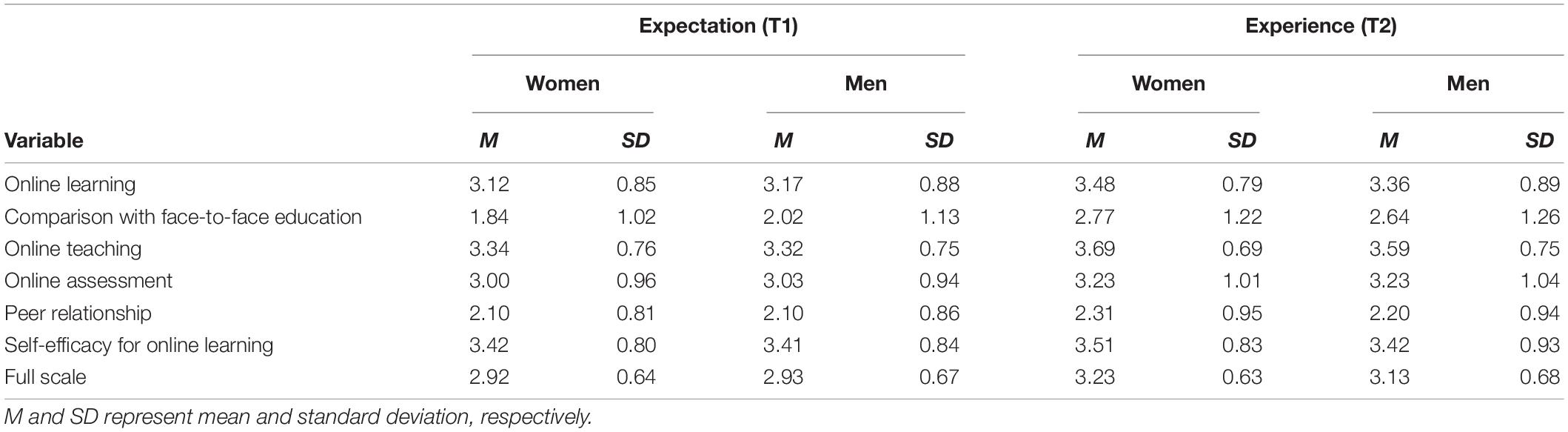
Table 4. Descriptive data on the expectations and experience of university students considering gender.
Figure 1 shows the size effect identified in the measurements considering gender. In the case of women, we found a large-size effect in the dimension of comparison with face-to-face education and a medium-size effect in the online teaching dimension. In the case of men, the analysis outcomes determine only a medium effect size in the dimension of comparison with face-to-face education and a small size effect in the rest of the dimensions.
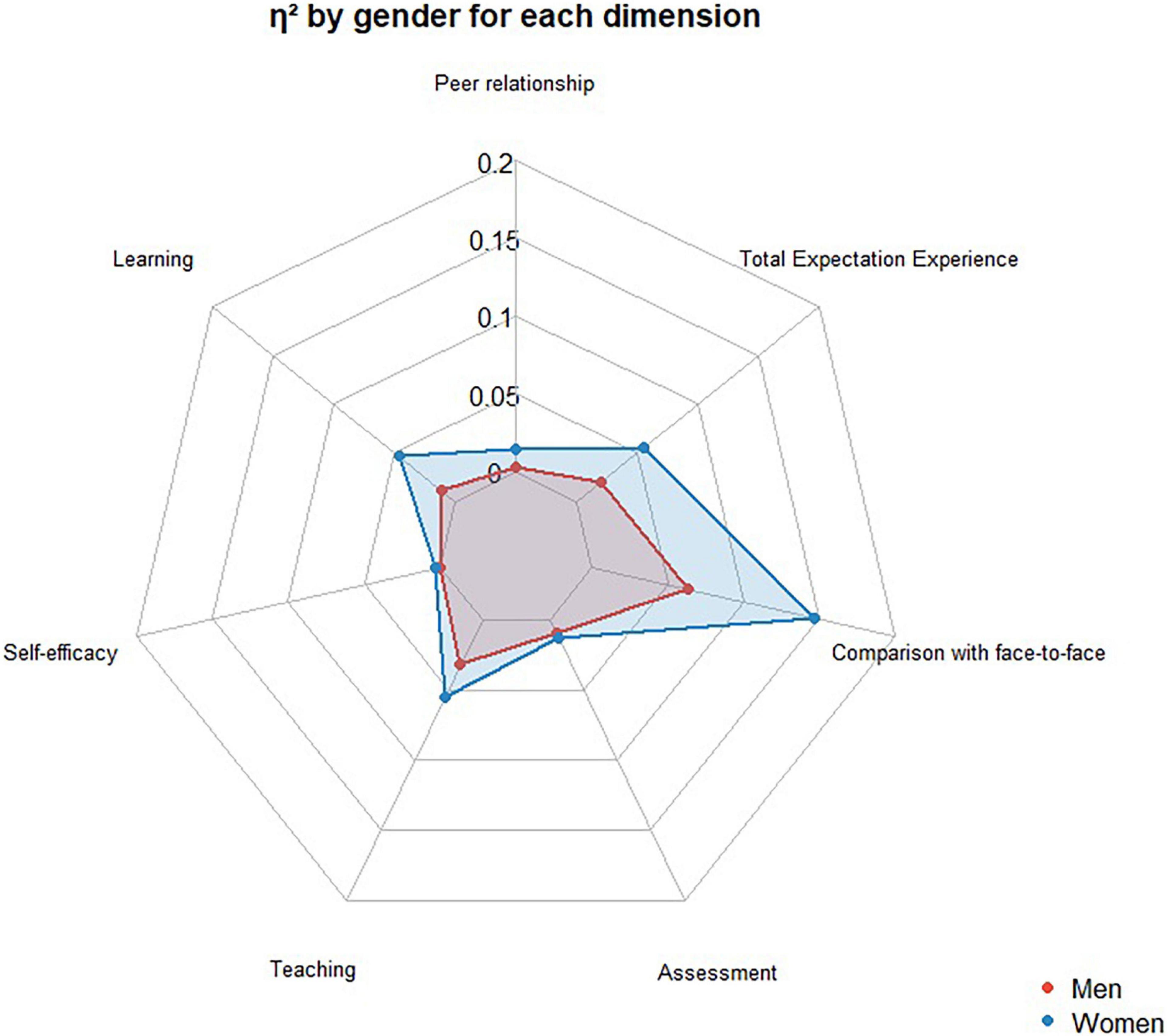
Figure 1. The effect size of the change between expectations and experience according to the gender of the participating student.
Differences by Disciplinary Area in the Measurement of Undergraduate Students’ Expectations and Experiences of Online Learning During Emergency Remote Teaching
Regarding differences between the scores from expectations and experience of university students during ERT during the COVID-19 pandemic according to disciplinary areas (H 3 ), the results by dimension are presented below.
For all six dimensions a statistically significant bidirectional interactions among the OECD area and the differences between T1 and T2 scores was found. The results by dimensions are the following: Comparison to face-to-face education [ F (5,1898) = 3.54, p < 0.01, GES = 0.003], online teaching [ F (5,1898) = 6.053, p < 0.001, GES = 0.004], online assessment [ F (5,1898) = 7.33, p < 0.001, GES = 0.006], online learning [ F (5,1898) = 8.686, p < 0.001, GES = 0.006], peer relationship [ F (5,1898) = 3.86, p < 0.01, GES.003], and self-efficacy for online learning [ F (5,1898) = 6.99, p < 0.001, GES = 0.005].
Regarding the main effect of OECD area, a significant effect for experience and for expectations was observed in the following four dimensions: Comparison to face-to-face education: experience [ F (5,1898) = 4.43, p < 0.01, GES.012] and the expectations [ F (5,1898) = 9.26, p < 0.001, GES.024]: online assessment: experience [ F (5,1898) = 4.71, p < 0.001, GES.012] and expectations [ F (5,1898) = 3.52, p < 0.01, GES.01]; online learning: experience [ F (5,1898) = 7.4, p < 0.001, GES.02] and expectations [ F (5,1898) = 9.57, p < 0.001, GES.03]; self-efficacy for online learning: experience [ F (5,1898) = 6.22, p < 0.001, GES.02] and expectations [ F (5,1898) = 5.52, p < 0.001, GES.01].
Regarding online teaching, a significant effect was observed in expectation [ F (5,1898) = 4.65, p < 0.001, GES.01], but not in experience ( p = 1). On the other hand, for peer relationship, a significant effect was shown for experience [ F (5,1898) = 3.67, p < 0.01, GES.01] but not for expectations ( p = 1).
We performed Tukey’s test to assess the differences between OECD areas in expectations and experience. Concerning expectations, the following dimensions presented significant differences (see Table 5 ). Comparison to face-to-face: Engineering and Technology - Agricultural Sciences p < 0.01, Engineering and Technology - Medical and Health Sciences p < 0.001, Engineering and Technology - Natural Sciences p < 0.05, Engineering and Technology - Social Sciences p < 0.001, and Engineering and Technology - Humanities p < 0.01. Online teaching: Engineering and Technology - Medical and Health Sciences p < 0.01 and Engineering and Technology - Social Sciences p < 0.001. Online assessment: Engineering and Technology - Medical and Health Sciences p < 0.05. Online learning: Engineering and Technology - Agricultural Sciences p < 0.01, Humanities - Medical and Health Sciences p < 0.01, Humanities - Natural Sciences p < 0.05, Engineering and Technology - Natural Sciences p < 0.01, Engineering and Technology - Social Sciences p < 0.001, and Engineering and Technology - Humanities p < 0.001. Self-efficacy for online learning: Engineering and Technology - Social Sciences p < 0.001 and Engineering and Technology - Humanities p < 0.01.
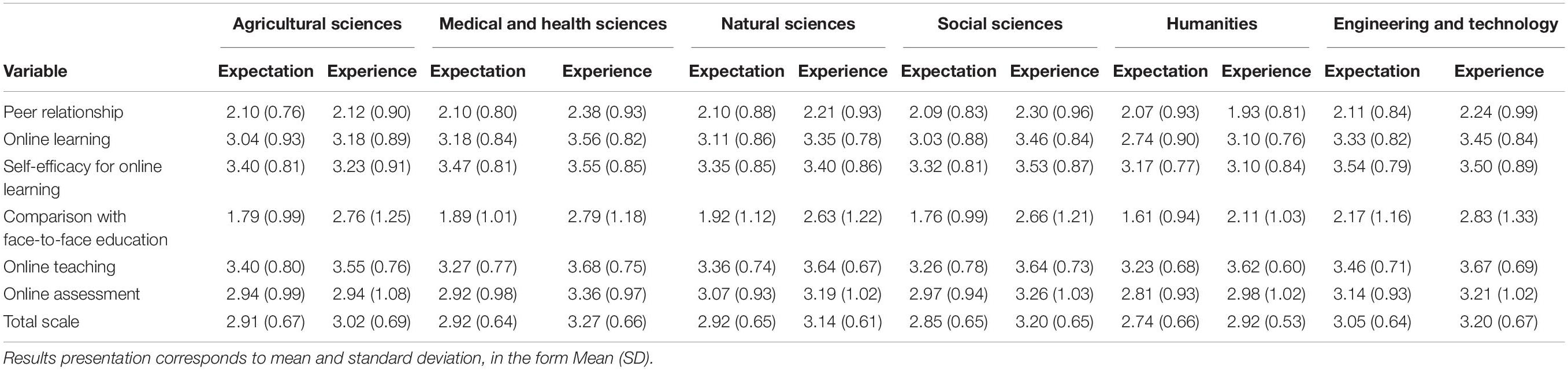
Table 5. Descriptive statistics on students’ expectations and experience during the ERT according to the disciplinary area.
In the case of experience, the dimensions that showed significant differences are listed below: Comparison to face-to-face education: Humanities - Agricultural Sciences p < 0.01, Humanities - Medical and Health Sciences p < 0.01, Humanities - Natural Sciences p < 0.05, Humanities - Social Sciences p < 0.05, and Engineering and Technology - Humanities p < 0.001. Online assessment: Medical and Health Sciences - Agricultural Sciences p < 0.001 and Social Sciences - Agricultural Sciences p < 0.05. Online learning: Medical and Health Sciences - Agricultural Sciences p < 0.001, Social Sciences - Agricultural Sciences p < 0.01, Engineering and Technology - Agricultural Sciences p < 0.01, Natural Sciences - Medical and Health Sciences p < 0.05 Humanities - Medical and Health Sciences p < 0.001, Humanities - Social Sciences p < 0.05, and Engineering and Technology - Humanities p < 0.05. Peer relationship: Humanities - Medical and Health Sciences p < 0.01 and Humanities - Social Sciences p < 0.05. Self-efficacy for online learning: Medical and Health Sciences - Agricultural Sciences p < 0.01, Social Sciences - Agricultural Sciences p < 0.01, Engineering and Technology - Agricultural Sciences p < 0.05, Humanities - Medical and Health Sciences p < 0.01, Humanities - Social Sciences p < 0.01, and Engineering and Technology - Humanities p < 0.01.
Finally, the simple main effect of the differences between expectations and experience for each dimension was analyzed, observing in some cases statistically significant effects for all six OECD areas, while in others only for one (see Table 5 ). The results reflected by the analysis are listed by dimension: Comparison to face-to-face education: Agricultural Sciences [ F (1,140) = 71.71, p < 0.001, GES = 0.16], Medical and Health Sciences [ F (1,415) = 227.33, p < 0.001, GES = 0.14], Natural Sciences [ F (1,311) = 93.81, p < 0.001, GES.08], Social Sciences [ F (1,508) = 247.639, p < 0.001, GES = 0.14], Humanities [ F (1,60) = 11.93, p < 0.01, GES = 0.06], and Engineering and Technology [ F (1,464) = 97.77, p < 0.001, GES = 0.06]. Online teaching: Agricultural Sciences [ F (1,140) = 8.14, p < 0.05, GES = 0.01], Medical and Health Sciences [ F (1,415) = 126, p < 0.001, GES = 0.07], Natural Sciences [ F (1,311) = 58.2, p < 0.001, GES.04], Social Sciences [ F (1,508) = 124, p < 0.001, GES = 0.06], Humanities [ F (1,60) = 23.8, p < 0.001, GES = 0.09], and Engineering and Technology [ F (1,464) = 51.6, p < 0.001, GES = 0.02].
The following differences in the dimension of online assessment between discipline areas were found: Medical and Health Sciences [ F (1,415) = 70.57, p < 0.001, GES = 0.05] and Social Sciences [ F (1,508) = 37.89, p < 0.001, GES = 0.02]. Online learning: Medical and Health Sciences [ F (1,415) = 86.1, p < 0.001, GES = 0.05], Natural Sciences [ F (1,311) = 26.4, p < 0.001, GES.02], Social Sciences [ F (1,508) = 131, p < 0.001, GES = 0.06], Humanities [ F (1,60) = 9.94, p < 0.05, GES = 0.5], and Engineering and Technology [ F (1,464) = 10.8, p < 0.01, GES = 0.006]. Peer relationship: Medical and Health Sciences [ F (1,415) = 42.1, p < 0.001, GES = 0.024], Social Sciences [ F (1,508) = 27, p < 0.001, GES = 0.014], and Engineering and Technology [ F (1,464) = 9.88, p < 0.05, GES = 0.005]. Self-efficacy for online learning: Social Sciences [ F (1,508) = 29.4, p < 0.001, GES = 0.02].
Figure 2 shows the size effect identified considering the OECD area. In Agricultural Sciences, we found a large-size effect in the dimension of comparison with face-to-face education and a small effect size in the dimensions of online learning, self-efficacy for online learning, online teaching, and the full scale. There were no effects detected in the rest of the dimensions. In Medical and Health Sciences, the analysis outcomes reflected a large-size effect in comparison with face-to-face education and a medium-size effect in the dimensions of online teaching and full scale. In addition, we found a small effect in the dimensions of peer relationship, online learning, and online assessment. In Natural Sciences, we found a medium-size effect in the size of comparison with face-to-face education and a small-size effect in online teaching, online learning, and full scale. No effects on the remaining dimensions were found. In the case of Social Sciences, we found a large-size effect for comparison with face-to-face education, a medium-size effect in the dimensions of online learning, online teaching, and the full scale, and a small-size effect in the rest of the dimensions. The Humanities area presented a medium-size effect in online teaching and comparison with face-to-face education dimensions and a small-size effect in online learning, peer relationship, online evaluation, and full scale. Finally, in Engineering and Technology, a medium-size effect in the dimension of comparison with face-to-face education and a small-size effect in the online teaching, online learning, peer relationship dimensions, and full scale were identified. In the rest of the dimensions, there were no effects detected.
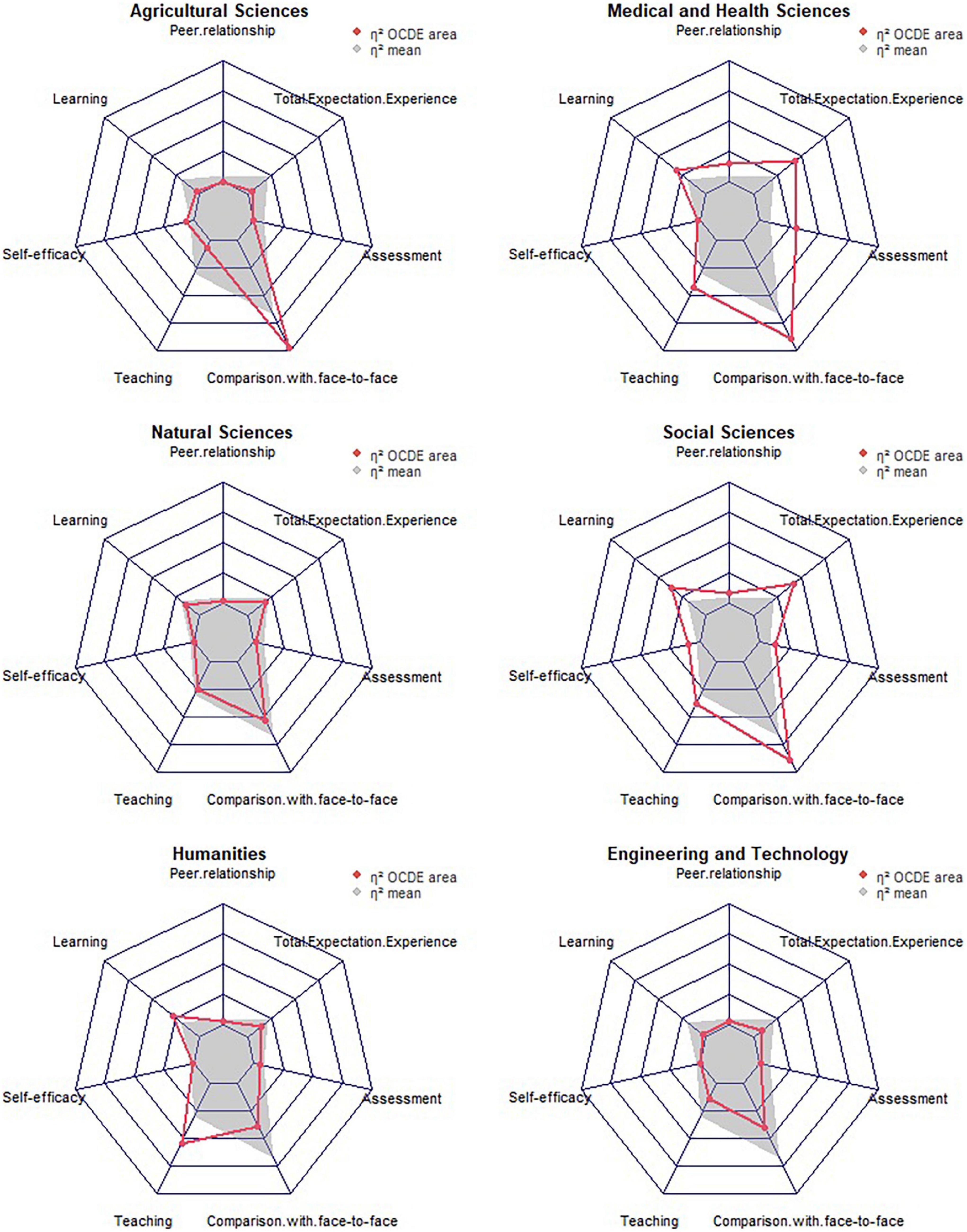
Figure 2. Size effect regarding the change between expectations and experience according to the disciplinary area.
Due to the COVID-19 pandemic, the transition to ERT impacted students’ expectations and experiences during their professional training. This research aimed to analyze students’ expectations and experiences considering the gender and disciplinary area of the participants. Findings are analyzed and discussed in terms of the hypotheses raised in section “The Present Study.”
Differences Among University Students’ Expectations and Experiences During the Emergency Remote Teaching Produced by the COVID-19 Pandemic
Changes between students’ expectations and experiences during ERT were found. Students’ expectations at T1 about online education were negative. However, at the end of the academic period, students indicated having a positive experience in most studied dimensions. They only showed a negative experience regarding the relationship with their peers and the comparison with face-to-face.
Several studies during the pandemic point out the lack of confidence toward the different educational actors and online education opportunities. This mistrust is associated with a lack of knowledge of the modality and its advantages ( Villa et al., 2020 ) and little awareness of the available virtual educational tools ( Rahiem, 2020 ; Almomani et al., 2021 ). In addition, the unexpectedness of the transition was a challenge for teachers and students, generating a problematic, improvised, and intuitive confrontation ( Barbour et al., 2020 ; Hodges et al., 2020 ).
Students’ perception of the limited opportunities virtual classrooms and other technological tools provided them to interact and work collaboratively with peers is particularly noteworthy. Several reports emphasize the benefits of cooperative work versus a competitive or individualistic methodology in higher education. The former generates better learning and significant commitment and involvement in academic tasks ( León del Barco et al., 2017 ; Guerra Santana et al., 2019 ; Hamdan et al., 2021 ). Also, collaborative work is closely related to desired competencies in the profession’s exercise, an aspect that is not present in this study. In this context, the literature describes technological mediation in education to provide significant possibilities of simultaneous sociability, of connection between communities and people, subscription, and asynchronous communication that generates network effects that tend to accelerate individuals and group learning ( De Haro, 2010 ; Anthony et al., 2019 ). Therefore, it is crucial to understand why peer interaction during ERT was negatively perceived, especially considering that the LMS had the functionalities for such activities. We believe that it is partly a product of the little knowledge of these tools by both teachers and students.
The observation that students face online education with a high sense of self-efficacy, believing that they have the skills to respond to the learning challenges that this modality presents, could be explained by the lack of knowledge and experience, as well as underestimating the necessary skills. Consequently, students perceive a lower complexity than the real one, as described by the “Durning Kruger effect” ( Dunning, 2011 ). It is possible that by the regular use of technology, social media, phones, and computers, they initially self-perceived as more competent.
The perception of a better experience concerning the initial expectation suggests that the implementation of ERT, although not devoid of difficulties, responded to students’ needs. Hence, higher education institutions’ response and the teachers’ and students’ adaptation adequately provided a well-perceived learning environment. Furthermore, the above is consistent with other research during the pandemic that reported positive experiences by teachers and students in terms of having been able to face the educational process despite the adversities of the confinement and its urgency ( Sepulveda-Escobar and Morrison, 2020 ).
We can conclude that the educational community and higher education authorities have learned greatly during ERT. Therefore, it will be interesting to study how to translate these lessons into explicit guidelines and practices when returning to normality post-pandemic.
When evaluating changes in expectation and experience scores considering the sex of the participants, at the beginning of ERT, men and women presented similar levels of expectations about online education. However, experiences showed differences according to gender. Although both perceived the educational experience as positive, women gave higher values than men, in the dimension with lower punctuation in the experience compared with a face-to-face modality and peer relationship.
These results are consistent with the study reported by Almomani et al. (2021) , conducted during the COVID-19 pandemic, and reports that women students were more optimistic, satisfied, and committed to the online learning experience than men students during this period. Furthermore, a 62-country study on the impact of the pandemic on higher education ( Aristovnik et al., 2020 ) reports a minor negative impact of confinement on women students’ learning, adaptation, and relationship with the teachers. In this study, a similar result was obtained regarding the perception of online teaching. Women students presented a higher value of the teacher’s commitment to ERT. Women considered that instructors were available and attentive to their learning needs, complied with the course syllabus, and made good use of the available virtual classroom tools.
In another study on online university education in the context of COVID-19 ( Shahzad et al., 2021 ), the authors were able to identify differences between men and women regarding the perception of usefulness, ease, and satisfaction with the use of the learning management systems provided by the institution. This finding suggests that adaptation processes to university life in electronic learning environments may be different for men and women. Therefore, this information could be valuable for university authorities to strengthen and improve the university system support.
Differences in Students’ Expectations and Experiences by Disciplinary Area of Online Learning During Emergency Remote Teaching
Research on the effects of the COVID-19 pandemic in the context of higher education has identified significant challenges for implementing online education, such as inequality, funding, and ways to develop learning in general ( Aristovnik et al., 2020 ; Funk, 2021 ). In this context, it is essential to identify if these challenges and opportunities are specific to a particular disciplinary area or apply to the general community. Thus, differences during ERT between disciplinary areas were analyzed.
Differences in the expectations and experiences of university students in the six disciplinary areas classified according to their undergraduate programs were found. Unfortunately, there is little literature on the influence of the disciplinary area to which students’ undergraduate programs belong regarding experience with online education in ERT. Knowing about students’ experience in each disciplinary area will allow teachers and educational authorities to identify weaknesses and good practices that will otherwise not be detected to design and develop monitoring plans and improve the quality of online education in the future.
We found differences within expectations in the online teaching dimension for all disciplinary areas. On the other hand, Students from Engineering and Technology and Medical and Health Sciences areas reported higher experience scores in this dimension, which implies that these students felt more confident about the actions performed by their instructors. This result could be related to the use of technology by Engineering and Technology teachers and the teacher training in the medical education area, often advanced.
Despite the improvement between student expectations and experiences of the online assessment dimension, changes presented null (Agricultural Sciences, Natural Sciences, and Engineering and Technology) or small (Social Sciences, Humanities, and Medical and Health Sciences) size effect. The assessment processes continue to be an area of concern. Other reports support this statement. For example, Jordanian university students perceived that assessment during the pandemic allowed them to obtain higher grades than face-to-face assessments. Nonetheless, most students perceived that the evaluative processes were unfair and learned more minor than the quality reflected ( Almomani et al., 2021 ). Consistently, a study conducted with 8265 Chilean university students ( Lobos et al., 2022 ) reported that students perceived a bad experience regarding the assessment process during the pandemic. Again, researchers observed a greater expectation of obtaining a good grade rather than of achieving learning. As a result, students considered that they failed to achieve good quality training. Despite these findings, a study carried out in Chile indicates that students’ academic performance improved compared to the previous academic period ( Franco et al., 2021 ). Therefore, the guidelines and strategies used by teachers regarding assessment continue to be an essential element to consider in the design of quality online education.
An interesting finding is a large-size effect obtained in the differences between the scores of expectations and experience of students of Agricultural Sciences and Medical and Health Sciences, for the comparison with face-to-face education dimension. Further research is required to identify good practices teachers and students implement in undergraduate programs classified in these two OCDE discipline areas.
We believe that the differences in the results of the students’ expectations and experience according to the disciplinary area are due to the different challenges encountered in the adaptation of the courses (efficient ones). Accordingly, strategies used, for example, in Health Sciences, can be used in realistic training scenarios that relate to people (Social Sciences and Humanities). One of these strategies can be using remote standardized patients who have meetings with students through the Internet. These activities allow teachers and standardized students to have spaces for evaluation and feedback ( Langenau et al., 2014 ; Bączek et al., 2021 ). This technique could be adapted to other teaching contexts using work situations in the training of other professionals.
Concerning the dimension of self-efficacy for online learning, no significant changes in four of the six knowledge evaluated areas were observed. Agricultural Sciences and Social Sciences displayed differences with small-size effect. Thus, ERT did not increase students’ confidence beliefs toward taking classes in the online teaching modality.
Despite valuable information that has been obtained for this study, some limitations are identified. First, the results presented correspond to university students’ responses from a single educational institution, so the interventions of university authorities could bias expectations and subsequent experiences in the context of ERT. Second, it was not part of this study to evaluate access gaps and other student variables that could affect the results. Finally, variables associated with the teacher or course characteristics that may influence the outcomes could not be controlled. Therefore, the results aim to study changes between students’ expectations and experience in an exploratory way. Other studies must consider the assessment of student (e.g., difficulties in accessing online classes), professor (e.g., profession), or course (e.g., type, time commitment) variables that may affect undergraduate expectations and experiences.
Study Implications
In this research, we found that students’ experiences with online education during the ERT were more optimistic than their expectations at the beginning of the semester. For this reason, the results found, together with other sources of institutional information such as learning analytics and institutional indicators, will allow authorities and teachers to develop guidelines to promote quality online education. It is also possible that university authorities could consider these preferences to design and create online courses for their students ( Zapata-Cuervo et al., 2021 ).
The relationship with peers and professors is still considered a weak point of online education. This is a crucial aspect to be addressed by university professors. In the context of virtuality, professors need to maintain communication channels that allow them to provide students with timely feedback from online video tutorials or email guides after class ( Bao, 2020 ; Vladova et al., 2021b ). We identified statistically significant differences in the experiences of men and women. This represents an opportunity to investigate how the characteristics of each student improve academic performance and decrease the probability of dropping out of college.
We found differences in the students’ experiences according to the scientific areas. These results translate into a challenge to identify the strategies and actions that facilitated a positive experience to replicate them in similar formative contexts. Further, studies can be performed to identify good practices applied in general contexts and those appropriate for each discipline. Higher education institutions are expected to accompany teachers and students in the different scientific areas during the post-pandemic academic continuity. Exceptional support is scheduled in aspects such as planning and prioritization of practical classes, promoting a combined approach of virtual and face-to-face education ( Pham and Ho, 2020 ; Vladova et al., 2021b ).
Future research could assess how students’ variables (e.g., internet access, type of device used to study), courses’ factors (e.g., number of hours of dedication, learning goals, instructional design, type of materials, or shared resources), teachers’ aspects (e.g., technological acceptance, use of strategies, training) or the institution’s elements (e.g., promotion of teaching through technology, support for students and teachers, use of online learning platforms, technological campuses) impact the expectations and subsequent experience of students during the development of online courses., especially regarding strength and weaknesses according to discipline areas.
The findings of this work contribute to identifying dimensions and areas that require special attention to establish preventive and corrective actions by university authorities for the near future and propose the opportunity of further studying good practices of better-perceived experiences of discipline areas.
The students’ experiences during ERT due to the COVID-19 pandemic exceeded expectations. Students reported high expectations about their self-efficacy to cope with this new scenario, even though low expectations regarding peer relationships, online teaching, and comparison with face-to-face education were observed concerning the experience after the semester. Students indicated positive experiences with online learning and teaching. They felt that the professor provided adequate support in terms of education, instruction, and assessment. Negative experiences persisted regarding peer relationships and the overall experience compared to face-to-face teaching. Additionally, men and women presented similar expectations at the beginning of the semester regardless of their discipline, while women were more optimistic about educational experiences during ERT. Finally, concerning the disciplinary area, differences in most of the assessed dimensions were observed, representing an opportunity to study further and identify good practices in those dimensions and disciplines that presented positive perception and effect.
Data Availability Statement
The original contributions presented in the study are included in the article/supplementary material, further inquiries can be directed to the corresponding author.
Ethics Statement
The studies involving human participants were reviewed and approved by Institutional Ethics Committee of University of Concepción. The patients/participants provided their written informed consent to participate in this study.
Author Contributions
KL and RC-R: conceptualization. KL, RC-R, and CB: methodology. JM-N: formal analysis and visualization. KL, RC-R, and AM-T: research and writing—preparing the original draft. AM-T, CB, and CF: resources, project management, and fundraising. JM-N and RC-R: data curation. CB and CF: writing—revising and editing. KL, CB, and CF: monitoring.
This research reported in this publication was supported by Unidad de Fortalecimiento Institucional of the Ministerio de Educación Chile, project InES 2018 UCO1808 Laboratorio de Innovación educativa basada en investigación para el fortalecimiento de los aprendizajes de ciencias básicas en la Universidad de Concepción.
Conflict of Interest
The authors declare that the research was conducted in the absence of any commercial or financial relationships that could be construed as a potential conflict of interest.
Publisher’s Note
All claims expressed in this article are solely those of the authors and do not necessarily represent those of their affiliated organizations, or those of the publisher, the editors and the reviewers. Any product that may be evaluated in this article, or claim that may be made by its manufacturer, is not guaranteed or endorsed by the publisher.
- ^ Lobos, K., Cobo-Rendon, R., Cisternas, N., Aslan, J., and López Angulo, Y. (under review). Experiences With Online Education of College Students During Emergency Remote Education Due to COVID-19 .
Abdulrahim, H., and Mabrouk, F. (2020). COVID-19 and the digital transformation of Saudi higher education. Asian J. Distance Educ. 15, 291–306.
Google Scholar
Aldhahi, M. I., Baattaiah, B. A., and Alqahtani, A. S. (2021). Predictors of electronic learning self-efficacy: a cross-sectional study in Saudi Arabian Universities [Original Research]. Front. Educ. 6:614333. doi: 10.3389/feduc.2021.614333
CrossRef Full Text | Google Scholar
Alhabeeb, A., and Rowley, J. (2018). E-learning critical success factors: comparing perspectives from academic staff and students. Comput. Educ. 127, 1–12. doi: 10.1016/j.compedu.2018.08.007
Almomani, E. Y., Qablan, A. M., Atrooz, F. Y., Almomany, A. M., Hajjo, R. M., and Almomani, H. Y. (2021). The influence of coronavirus diseases 2019 (COVID-19) pandemic and the quarantine practices on university students’ beliefs about the online learning experience in Jordan. Front. Public Health 8:595874. doi: 10.3389/fpubh.2020.595874
PubMed Abstract | CrossRef Full Text | Google Scholar
Alqurshi, A. (2020). Investigating the impact of COVID-19 lockdown on pharmaceutical education in Saudi Arabia–A call for a remote teaching contingency strategy. Saudi Pharm. J. 28, 1075–1083. doi: 10.1016/j.jsps.2020.07.008
Anthony, B., Kamaludin, A., Romli, A., Raffei, A. F. M. Eh Phon, D. N. A. /L., Abdullah, A., et al. (2019). Exploring the role of blended learning for teaching and learning effectiveness in institutions of higher learning: an empirical investigation. Educ. Inf. Technol. 24, 3433–3466. doi: 10.1007/s10639-019-09941-z
Aristovnik, A., Keržič, D., Ravšelj, D., Tomaževič, N., and Umek, L. (2020). Impacts of the COVID-19 pandemic on life of higher education students: a global perspective. Sustainability 12:8438. doi: 10.3390/su12208438
Bączek, M. Zagańczyk-Bączek, M., and Szpringer, M. Jaroszyński, A.Wożakowska-Kapłon, B. (2021). Students’ perception of online learning during the COVID-19 pandemic: a survey study of Polish medical students. Medicine 100:e24821. doi: 10.1097/MD.0000000000024821
Bao, W. (2020). COVID-19 and online teaching in higher education: a case study of Peking University. Hum. Behav. Emerg. Technol. 2, 113–115. doi: 10.1002/hbe2.191
Barbour, M. K., LaBonte, R., Hodges, C., Moore, S., Lockee, B., Trust, T., et al. (2020). Understanding Pandemic Pedagogy: Differences Between Emergency Remote, Remote, and Online Teaching. State of the Nation: K-12 e-Learning in Canada. Halfmoon Bay, BC: Canadian eLearning Network. doi: 10.13140/RG.2.2.31848.70401
Bustamante, R. (2020). Educación en Cuarentena: Cuando la Emergencia se Vuelve Permanente (Segunda Parte). Available online at: http://www.grade.org.pe/creer/archivos/articulo-4.pdf (accessed September 10, 2021).
Cárdenas Castro, M., and Arancibia Martini, H. (2014). Potencia estadística y cálculo del tamaño del efecto en G* Power: complementos a las pruebas de significación estadística y su aplicación en psicología. Salud Soc. 5, 210–224. doi: 10.22199/S07187475.2014.0002.00006
Cervantes Arreola, D. I., Valadez Sierra, M. D., Valdés Cuervo, A. A., and Tánori Quintana, J. (2018). Diferencias en autoeficacia académica, bienestar psicológico y motivación al logro en estudiantes universitarios con alto y bajo desempeño académico. Psicol. Desde Caribe 35, 7–17.
Chiu, T. K. F., Lin, T.-J., and Lonka, K. (2021). Motivating online learning: the challenges of COVID-19 and beyond. Asia Pac. Educ. Res. 30, 187–190. doi: 10.1007/s40299-021-00566-w
De Haro, J. J. (2010). Redes sociales en educación. Educ. Comun. Coop. Soc. 27, 203–216.
Dunning, D. (2011). The Dunning–Kruger effect: on being ignorant of one’s own ignorance. Adv. Exp. Soc. Psychol. 44, 247–296. doi: 10.1016/B978-0-12-385522-0.00005-6
Franco, E., Gonzalez, C., and Falconier, M. (2021). Academic performance at Adventist University of Chile during COVID-19: a comparative analysis 2019-2020. Apunt. Univ. 11, 1–12. doi: 10.17162/au.v11i3.689
Funk, R. L. (2021). Challenges for higher education in times of COVID-19: how three countries have responded. High. Learn. Res. Commun. 11, 106–111. doi: 10.18870/hlrc.v11i0.1242
Guerra Santana, M., Rodríguez Pulido, J., and Artiles Rodríguez, J. (2019). Aprendizaje colaborativo: experiencia innovadora en el alumnado universitario. Rev. Estud. Exp. Educ. 18, 269–281. doi: 10.21703/rexe.20191836guerra5
Hamdan, K. M., Al-Bashaireh, A. M., Zahran, Z., Al-Daghestani, A., Al-Habashneh, S., and Shaheen, A. M. (2021). University students’ interaction, Internet self-efficacy, self-regulation, and satisfaction with online education during pandemic crises of COVID-19. Int. J. Educ. Manag. 35, 713–725. doi: 10.1108/ijem-11-2020-0513
Hattar, S., Alhadidi, A., Sawair, F. A., Alraheam, I. A., El-Ma’Aita, A., and Wahab, F. K. (2021). Impact of COVID-19 pandemic on dental education: online experience and practice expectations among dental students at the University of Jordan. BMC Med. Educ. 21:151. doi: 10.1186/s12909-021-02584-0
Hodges, C., Moore, S., Lockee, B., Trust, T., and Bond, A. (2020). The Difference Between Emergency Remote Teaching and Online Learning. Available online at: https://er.educause.edu/articles/2020/3/the-difference-between-emergency-remote-teaching-and-online-learning (accessed June 27, 2021).
Imsa-ard, P. (2020). Thai university students’ perceptions towards the abrupt transition to ‘forced’online learning in the COVID-19 situation. J. Educ. Khon Kaen Univ. 43, 30–44.
Jiménez, M. Á. F., Rodríguez, E. M., and Hurtado, J. C. T. (2017). Funciones de la tutoría en e-learning: estudio mixto de los roles del tutor online. Rev. Investig. Educ. 35, 409–426. doi: 10.6018/rie.35.2.273271
Langenau, E., Kachur, E., and Horber, D. (2014). Web-based objective structured clinical examination with remote standardized patients and Skype: resident experience. Patient Educ. Couns. 96, 55–62. doi: 10.1016/j.pec.2014.04.016
León del Barco, B., Mendo-Lázaro, S., Felipe-Castaño, E., Polo del Río, M.-I., and Fajardo-Bullón, F. (2017). Potencia de equipo y aprendizaje cooperativo en el ámbito universitario. Rev. Psicodidáctica 22, 9–15. doi: 10.1016/S1136-1034(17)30038-2
Lobos, K., Bustos, C., Cobo-Rendón, R., and Cisternas, N. (2022). Estudio de las Características Psicométricas de un Cuestionario de Expectativas Hacia la Educación Virtual en Estudiantes Universitarios Durante la Pandemia por COVID-19 , Vol. 15. Formación Univ, 1–16. En prensa
Maier, V., Alexa, L., and Craciunescu, R. (2020). “Online education during the COVID19 pandemic: perceptions and expectations of romanian students,” in Proceedings of the European Conference on e-Learning , Kidmore End. doi: 10.34190/EEL.20.147
Miramontes Arteaga, M. A., Castillo Villapudua, K. Y., and Macías Rodríguez, H. J. (2019). Estrategias de aprendizaje en la educación a distancia. Rev. Investig. Tecnol. Inf. 7, 199–214. doi: 10.36825/RITI.07.14.017
Montero, I., and León, O. G. (2007). A guide for naming research studies in Psychology. Int. J. Clin. Health Psychol. 7, 847–862.
Mousa, A. H., Aljshamee, M., Mohammed, A. H., Mousa, S. H., Mousa, S. H., and Obaid, H. A. (2020). “A systematic literature review: measuring user acceptance models in e-learning adopted in higher education institutes,” in Proceedings of the International Conference of Numerical Analysis and Applied Mathematics ICNAAM 2019, AIP Conference Proceedings , Rhodes, Vol. 2290, 040021. doi: 10.1063/5.0027409
Nur Agung, A. S., and Surtikanti, M. W., and Ca Quinones, O. P. (2020). Students’ perception of online learning during the COVID-19 pandemic: a case study on the English students of STKIP Pamane Talino. Soshum J. Sosial Humaniora 10, 225–235. doi: 10.31940/soshum.v10i2.1316
Paechter, M., Maier, B., and Macher, D. (2010). Students’ expectations of, and experiences in e-learning: their relation to learning achievements and course satisfaction. Comput. Educ. 54, 222–229. doi: 10.1016/j.compedu.2009.08.005
Pham, H.-H., and Ho, T.-T.-H. (2020). Toward a ‘new normal’ with e-learning in Vietnamese higher education during the post COVID-19 pandemic. High. Educ. Res. Dev. 39, 1327–1331. doi: 10.1080/07294360.2020.1823945
R Core Team (2020). R: A Language and Environment for Statistical Computing. Vienna: R Foundation for Statistical Computing.
Rahiem, M. D. H. (2020). The emergency remote learning experience of university students in Indonesia amidst the COVID-19 crisis. Int. J. Learn. Teach. Educ. Res. 19, 1–26. doi: 10.26803/ijlter.19.6.1
Rodrigues, H., Almeida, F., Figueiredo, V., and Lopes, S. L. (2019). Tracking e-learning through published papers: a systematic review. Comput. Educ. 136, 87–98. doi: 10.1016/j.compedu.2019.03.007
Sepulveda-Escobar, P., and Morrison, A. (2020). Online teaching placement during the COVID-19 pandemic in Chile: challenges and opportunities. Eur. J. Teach. Educ. 43, 587–607. doi: 10.1080/02619768.2020.1820981
Shahzad, A., Hassan, R., Aremu, A. Y., Hussain, A., and Lodhi, R. N. (2021). Effects of COVID-19 in E-learning on higher education institution students: the group comparison between male and female. Qual. Quant. 55, 805–826. doi: 10.1007/s11135-020-01028-z
Shehzadi, S., Nisar, Q. A., Hussain, M. S., Basheer, M. F., Hameed, W. U., and Chaudhry, N. I. (2020). The role of digital learning toward students’ satisfaction and university brand image at educational institutes of Pakistan: a post-effect of COVID-19. Asian Educ. Dev. Stud. 10, 276–294. doi: 10.1108/aeds-04-2020-0063
Singh, V., and Thurman, A. (2019). How many ways can we define online learning? A systematic literature review of definitions of online learning (1988-2018). Am. J. Distance Educ. 33, 289–306. doi: 10.1080/08923647.2019.1663082
Suárez, B. L., García-Perales, N., and Elisondo, R. C. (2021). La vivencia del alumnado en tiempos COVID-19: estudio comparadoentre las universidades de Extremadura (España) y Nacional de Río Cuarto (Argentina). Rev. Esp. Educ. Comp. 38, 44–68. doi: 10.5944/reec.38.2021.28936
Sun, J. (2016). Multi-dimensional alignment between online instruction and course technology: a learner-centered perspective. Comput. Educ. 101, 102–114. doi: 10.1016/j.compedu.2016.06.003
Talsma, K., Robertson, K., Thomas, C., and Norris, K. (2021). COVID-19 beliefs, self-efficacy and academic performance in first-year university students: cohort comparison and mediation analysis. Front. Psychol. 12:643408. doi: 10.3389/fpsyg.2021.643408
Thode, H. (2002). Testing for Normality. New York, NY: Marcel Dekker. Inc, 99–123.
Valle, A., Regueiro, B., Rodríguez, S., Piñeiro, I., Freire, C., Ferradás, M., et al. (2015). Perfiles motivacionales como combinación de expectativas de autoeficacia y metas académicas en estudiantes universitarios. Eur. J. Educ. Psychol. 8, 1–8. doi: 10.1016/j.ejeps.2015.10.001
Van Heuvelen, K. M., Daub, G. W., and Ryswyk, H. V. (2020). Emergency remote instruction during the COVID-19 pandemic reshapes collaborative learning in general chemistry. J. Chem. Educ. 97, 2884–2888. doi: 10.1021/acs.jchemed.0c00691
Villa, F. G., Litago, J. D. U., and Sánchez-Fdez, A. (2020). Perceptions and expectations in the university students from adaptation to the virtual teaching triggered by the covid-19 pandemic. Rev. Latina Comun. Soc. 78, 99–119.
Vladova, G., Ullrich, A., Bender, B., and Gronau, N. (2021a). Students’ acceptance of technology-mediated teaching – how it was influenced during the COVID-19 pandemic in 2020: a study from Germany. Front. Psychol. 12:636086. doi: 10.3389/fpsyg.2021.636086
Vladova, G., Ullrich, A., Bender, B., and Gronau, N. (2021b). “Yes, we can (?) – a critical review of the COVID-19 semester,” in Technology and Innovation in Learning, Teaching and Education. TECH-EDU 2020. Communications in Computer and Information Science , eds A. Reis, J. Barroso, J. B. Lopes, T. Mikropoulos, and C. W. Fan (Cham: Springer International Publishing), 225–235. doi: 10.1007/978-3-030-73988-1_17
Wei, H.-C., and Chou, C. (2020). Online learning performance and satisfaction: do perceptions and readiness matter? Distance Educ. 41, 48–69. doi: 10.1080/01587919.2020.1724768
Wigfield, A., and Eccles, J. S. (2000). Expectancy–value theory of achievement motivation. Contemp. Educ. Psychol. 25, 68–81. doi: 10.1006/ceps.1999.1015
Zapata-Cuervo, N. Montes-Guerra, M. I., Shin, H. H., Jeong, M., and Cho, M.-H. (2021). Students’ psychological perceptions toward online learning engagement and outcomes during the COVID-19 pandemic: a comparative analysis of students in three different Countries. J. Hosp. Tour. Educ. 33, 1–15. doi: 10.1080/10963758.2021.1907195
Keywords : COVID-19, higher education, university student, online teaching and learning, student self-efficacy
Citation: Lobos K, Cobo-Rendón R, Mella-Norambuena J, Maldonado-Trapp A, Fernández Branada C and Bruna Jofré C (2022) Expectations and Experiences With Online Education During the COVID-19 Pandemic in University Students. Front. Psychol. 12:815564. doi: 10.3389/fpsyg.2021.815564
Received: 15 November 2021; Accepted: 02 December 2021; Published: 05 January 2022.
Reviewed by:
Copyright © 2022 Lobos, Cobo-Rendón, Mella-Norambuena, Maldonado-Trapp, Fernández Branada and Bruna Jofré. This is an open-access article distributed under the terms of the Creative Commons Attribution License (CC BY) . The use, distribution or reproduction in other forums is permitted, provided the original author(s) and the copyright owner(s) are credited and that the original publication in this journal is cited, in accordance with accepted academic practice. No use, distribution or reproduction is permitted which does not comply with these terms.
*Correspondence: Carola Bruna Jofré, [email protected]
Disclaimer: All claims expressed in this article are solely those of the authors and do not necessarily represent those of their affiliated organizations, or those of the publisher, the editors and the reviewers. Any product that may be evaluated in this article or claim that may be made by its manufacturer is not guaranteed or endorsed by the publisher.
- International edition
- Australia edition
- Europe edition
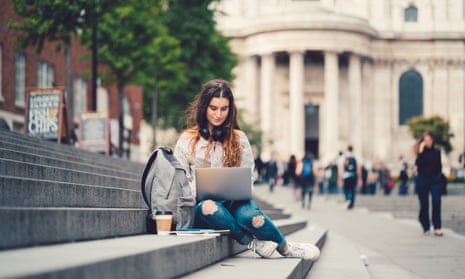
How has the pandemic changed the way you’ll learn?
As students gradually return to campus, many universities will be offering blended learning – mixing face-to-face lectures with the best of digital teaching
- The 2022 league table
T he past year and a half has been a learning experience for everyone in education. You might recall frustrating days battling your internet connection to log on to Teams classes. Similarly, the pandemic has been a baptism of fire for universities in how to deliver quality online learning.
While universities are planning to revert to their pre-pandemic state by autumn 2022, many are also thinking hard about the positive lessons that can be drawn from what’s happened. The main shift is likely to be around how much online teaching you get. Most universities are planning to use a “blended model” that will combine the flexibility of online lectures with more interactive activities in-person, such as labs, seminars, workshops and Q&A sessions.
“The reference to some universities talking about ending face-to-face lectures doesn’t mean students won’t be attending any in-person classes; it means they’ll move away from large lectures of 200 students upwards. We’ll see a lot more of these sessions provided online because that can be far more effective,” explains Liz Barnes, the vice-chancellor of Staffordshire University who has led a review into online learning..
Some universities and courses are planning for more online learning than others, so when you’re choosing a university think about what might work for you. A broad rule of thumb is that practical courses have more face-to-face contact hours than academic degrees which involve lots of reading.
Ask yourself: are you planning to commute, and would you prefer to only be in for a couple of days per week, with the rest online? Or do you need in-person teaching to motivate you and to meet new people? Some universities are offering a variety of options to suit different learning styles and personal circumstances. Most university websites aren’t able to supply the full details of how individual courses will be taught, so to find out the number of face-to-face contact hours you should ask the universities directly – and ideally visit an open day, says Barnes. She adds that the next year is still going to be a transition phase out of the pandemic, so things may well change in 2022.
If you’re concerned about whether online teaching means you’re getting worse value for money, this is actually not the case, assures Prof Allison Littlejohn, an academic at UCL specialising in learning technology.
“The time needed to prepare and produce online teaching materials is much higher than for on-campus lectures,” she says. Instead, most universities are shifting lectures online as they think it’s a better way for their students to learn.
If you’re still worried about surviving Zoom lectures, there are some strategies that can help.
“Don’t watch an entire, hour-long Zoom lecture. If the lecture is pre-recorded, combine watching it with active reflection on what you’re learning. If the lecture is live, find ways to interact with other students and with academics afterwards to discuss ideas and concepts,” recommends Littlejohn.
Interaction is an essential component of well-designed online learning, she says, so if it’s not included, ask your tutor to build in more time with individual students or in small groups, online or in person. You can also organise your own study groups to discuss what you’ve learned in a Zoom lecture or do problem-solving activities using new ideas and concepts.
One thing you might be worrying about is whether your disrupted school experience could hold you back at university.
“You might have had a poor experience with digital learning or feel less prepared for the academic challenges and independence university brings. So find out what a university is doing to help you around digital skills and how they support students,” recommends Ian Dunn, deputy vice-chancellor at Coventry University.
“Think about your last year of study during the Covid-19 pandemic and what you need from a learning and teaching perspective to thrive.”
- Online learning
- University Guide 2022
- Universities
- Higher education
- University guide
Most viewed
- Open access
- Published: 03 August 2022
Depression and anxiety among online learning students during the COVID-19 pandemic: a cross-sectional survey in Rio de Janeiro, Brazil
- Luísa Pelucio 1 ,
- Pedro Simões 2 ,
- Marcia Cristina Nascimento Dourado 1 ,
- Laiana A. Quagliato 1 &
- Antonio Egidio Nardi 1
BMC Psychology volume 10 , Article number: 192 ( 2022 ) Cite this article
24k Accesses
22 Citations
3 Altmetric
Metrics details
The COVID-19 pandemic introduced a global need to explore the potential and challenges of online education.
To evaluate the presence of depression and anxiety in university students and their level of satisfaction with online learning during the period of social isolation caused by the COVID-19 pandemic.
A cross-sectional design was used to evaluate 152 online learning students from six different university courses: Medicine, Psychology, Law, Engineering, Physiotherapy, and Business. The evaluation of the participants was carried out through an online survey in Rio de Janeiro, Brazil. Also, the Hospital Anxiety and Depression Scale was used to assess participants mental health.
Most of the participants reported emotional impact, followed by learning impact, financial impact, social impact, and technological impact, with a significant difference in the presence of depressive symptoms, but no significant difference in anxiety. The participants presented moderate anxiety levels, with no significant differences between genders, and mild levels of depressive symptoms with significant differences between genders. Also, younger students were more anxious than older students. In addition, female students with less social contact presented more depressive symtoms.
From a clinical perspective, the findings provide insights into mental health among university students during the COVID-19 pandemic. These findings may help in the development of effective screening strategies and in the formulation of interventions that improve the mental health of students.
Peer Review reports
In March 2020, with COVID-19 multiplying in several countries, the World Health Organization (WHO) declared that the world had reached a pandemic level [ 1 , 2 , 3 ]. Online learning made education accessible during the social isolation period as several countries switched to distance learning for all levels of education. Online education is defined as learning and teaching through a primarily electronic medium with the interaction between learners and their educational materials and activities taking place synchronously or asynchronously in a virtual environment [ 4 ].
Online education is not a new concept to educators, but the COVID-19 pandemic introduced a global need to explore its potential and opportunities [ 4 ]. However, the transition to online learning presents specific difficulties as teaching methodology requires adaptation, with challenges ranging from evaluating the university’s resources to adapting the practical sessions central to technical degrees [ 5 ]. Therefore, not every country has the means and resources to adjust to online learning. A study showed that 85% of the institutions in Europe quickly replaced in-person education with online learning, while only 29% of African institutions met online education requirements [ 6 ]. Moreover, since the beginning of February 2020, Chinese colleges and universities have used different learning modes, including online learning based on different platforms, to achieve the goal of suspending classes without suspending learning [ 7 ]. In Jordan, new recommendations for converting to online teaching in universities were published to mitigate education issues [ 8 ]. However, online learning presents challenges to students as it requires time and learning resources, a set of goals, and plans [ 4 ]. In Brazil, a group of institutions conducted a series of surveys with 1056 caregivers and 1556 public school students to understand their thoughts and feelings about online learning [ 8 ]. The results showed that lack of motivation and difficulties maintaining the online learning routine were the most significant challenges faced by the students [ 8 , 9 ]. Another study showed difficulties in student engagement, retention rates, and reported perceptions of missing out on traditional classroom experiences [ 4 ]. In Lebanon, Fawaz and Samaha (2020) reported that students’ dissatisfaction with online learning might be attributed to a below-average internet service, rendering students unable to attend classes or participate in online exams [ 10 ]. Kasse and Balunywa demonstrated that significant structural vulnerabilities such as lack of internet access or technological ineptitude restricted the full‐scale implementation of online learning in Uganda [ 11 ].
Many students struggle with psychological problems during their college years. These problems may be even more apparent during the COVID-19 pandemic with the accompanying restrictions and the transition to an online learning environment, but few longitudinal studies have been conducted to date. As part of the World Mental Health International College Student Initiative (WMH-ICS), a study comparing symptoms and identifying stressors concerning depression, anxiety, and suicidality prior to and during the pandemic was conducted among students attending Ulster University in Northern Ireland (NI), and LYIT, in the Republic of Ireland (ROI). Data were collected from first-year students in September 2019, with a completed response rate of 25.22% (NI) and 41.9% (ROI) to the number of first-year students registered. A follow-up study was conducted in Autumn 2020, with 884 students fully completing the online survey in both years, equating to just under half of those who completed the initial survey. High levels of mental health problems were found in year 1, especially in the ROI. Levels of depression increased significantly in year 2, particularly among students in NI, although anxiety levels decreased. No significant variations were found for suicidal behavior. Several stressors were identified, including increased social isolation and worrying about loved ones [ 12 ].
The pandemic caused by COVID-19 also increased depressive and anxiety symptoms and psychological pressures in the general population [ 10 , 12 ]. A current report suggests that an increased level of depression, stress, and anxiety was found in people who were single, separated, or widowed, lost jobs, or were in contact with potential COVID-19 patients. In addition, people with higher levels of education presented higher stress levels [ 13 ]. A meta-analysis [ 14 ] reported that the prevalence of depression could be affected by changes in psychiatric practices and the availability of online information on mental health. Another study showed post-traumatic stress disorder (PTSD) correlations during the period of social isolation that included religious practice, reason for quarantine/isolation, education level, and being an infection case [ 14 ].
Students might be severely affected by the COVID-19 pandemic with significant impacts on academic achievement and social life. In addition, the discrepancies and inequalities observed at global and institutional levels may strongly impact individual levels. For example, a study [ 15 ] showed that younger, poorer female students with a lack of infrastructure, such as limited internet connectivity, demonstrated higher levels of anxiety. In addition, a report on the experience [ 16 ] of medical students in the Philippines described the limitations of online learning on medical skills as they need things to be tangible to practice the clinical eye.
Several infrastructural factors in Brazil, such as the electricity and telecommunication deficit, may be a significant barrier to online learning. In this context, evaluating the impact of online learning on students’ mental health in different cultural backgrounds can provide data to help train and prepare teachers and educational professionals and develop new models of mental health protocols and interventions for the target population. Therefore, this study’s research question focuses on the relationship between depression and anxiety in university students and their level of satisfaction with online learning during the period of social isolation caused by the COVID-19 pandemic. Furthermore, we also aim to understand how depressive or anxiety symptoms might be related to other variables such as type of university course, gender, or age during the online learning period. We hypothesize that the university students will present lower levels of satisfaction with online learning and higher levels of depressive and anxious symptoms related to online learning during the COVID-19 pandemic.
This is a cross-sectional study that evaluated 152 online learning students in Barra Mansa, Volta Redonda, and Resende in the state of Rio de Janeiro, Brazil. Individuals aged between 18 and 65 years old were included in the study from May 2021 to August 2021. All participants who were willing to respond to the assessment were included. It was estimated that 100% of the university students were online due to the social isolation caused by the COVID-19 pandemic. However, only 65%-70% participated in online classes due to internet access problems or non-detailed personal issues. Participants who were not in social isolation or not in active class/enrollment were excluded. Participants were selected by university lectures, which greatly facilitated access to students. During classes, the lecturers invited the students and sent the survey link to access the full online research. Participants from six different university courses were included: Medicine, Psychology, Law, Engineering, Physiotherapy, and Business.
The Ethics Committee of the Institute of Psychiatry of the Universidade Federal do Rio de Janeiro (Federal University of Rio de Janeiro) (UFRJ) approved the study and all participants signed the informed consent form. This study followed the Declaration of Helsinki.
The participants were evaluated online, through google forms. All eligible participants completed an online assessment using a form collecting sociodemographic data (age, education, current medication) and questions with a Likert scale to understand levels of satisfaction with online learning: (1) What do you think of online learning education? (very poor, poor, regular, good, or very good); (2) Do you feel affected by online learning? (yes/no); and (3) How do you feel affected by online learning? (learning, emotional, financial, social, or technological). The questionnaire used to evaluate levels of satisfaction with online learning was developed in Brazilian Portuguese by the authors. In addition, the participants’ anxiety and depression status were also assessed using the Brazilian version of the Hospital Anxiety and Depression Scale (HADS). The HADS consists of 14 questions, seven to assess anxiety and seven to assess depression, with each item scored on a scale of 0 to 3, for a total of 21 points for each scale. Cut-off scores: Mild (8 to 10 points); Moderate (11 to 14 points); Severe (15 to 21 points) [ 17 ]. Cronbach’s alpha for the HADS is 0.795.
Statistical analysis
All statistical analyses were performed with SPSS software for Windows version 22.0. A Kolmogorov–Smirnov test was used to verify the normal distribution between variances. Descriptive statistics analyzed the sociodemographic data of the participants (gender, age, university course, online learning impact) and the clinical characteristics (anxiety and depressive symptoms). Chi-Squared was used to compare the distribution of students and university course. Student’s t-test was used to verify the presence of anxiety and depressive symptoms and whether online learning had an impact. The Duncan Multiple Range Test was used to compare a set of sample means with significant minimum amplitude. Linear regression models were performed separately for anxiety and depression and the best models were selected according to the highest explained variance of R squared (R 2 ) and the variance inflation factor (VIF) close to 1, for the collinearity in each independent variable. All significance tests were performed at a 2-tailed level considering a significance level of P ≤ 0.05.
Sociodemographic characteristics
Most of the participants were female (77%, n = 117), with age ranging from 18 to 65 years old: 55% from 18 to 24 years old (n = 84), 23% from 25 to 34 years old (n = 35), 15% from 35 to 44 years old (n = 23), and 6% from 45 to 65 years old (n = 10).
The university students were from 6 different courses: Medicine (2.6% n = 4), Psychology (65% n = 99), Business (3.9% n = 6), Law (16. 4% n = 25), Engineering (7.2% n = 11), and Physiotherapy (4.6% n = 7). The sociodemographic data are shown in Table 1 .
Students’ clinical evaluation
The sample presented moderate levels of anxiety (M = 11.2 SD 4.72), with no significant differences between genders ( p = 0.081) and mild levels of depressive symptoms (M = 8.03 SD 4.22) with significant differences between genders ( p = 0.005). The sample was divided by age group and there was a significant difference in anxiety according to the students’ age ( p = 0.050), whereby younger students were more anxious than older students, although there was no difference in the presence of depressive symptoms ( p = 0.145). There was also no significant difference between anxiety ( p = 0.268) and depressive symptoms ( p = 0.615) and the type of university course. The data related to anxiety and depressive symptoms are shown in Table 2 .
Online learning levels of satisfaction
Table 3 shows students’ opinions about online learning according to the presence of anxiety and depression. Most of the students considered online learning as regular (34.9% n = 53), followed by good (24.3% n = 37), and poor (23% n = 35). Few students found online learning to be very poor (12.5% n = 19) or very good (5.3% n = 8). There was a significant difference between anxiety ( p = 0.019) and depressive symptoms ( p = 0.009) and level of satisfaction with online education. There was also a significant difference between level of satisfaction with online learning and students’ age ( p = 0.001). Younger students presented more dissatisfaction with online learning than older students (Table 4 ).
The impact of the pandemic was also investigated (“Do you feel affected by online learning?”). Most students answered yes (92% n = 140), with a significant difference in the presence of depressive symptoms ( p = 0.006), but no significant difference in anxiety ( p = 0.189).
The participants were also asked “How do you feel affected?”. Most participants reported emotional impact (48.7% n = 74), followed by learning impact (29.6% n = 45), financial impact (2.6% n = 4), social impact (9.2% n = 14), technological impact (2.6% n = 4), and not affected/none (7.2% n = 11), with a significant difference in the presence of depressive symptoms ( p = 0.031), but no significant difference in anxiety ( p = 0.069).
Regression models of the factors related to anxiety and depression ( R 2 )
Table 5 shows that students’ anxiety is related to age and financial impact, whereby younger age and more significant financial impact are perceived with increased anxiety ( p < 0.001). Students’ depression is impacted by gender and social impact, whereby being female and having less social contact result in higher levels of depression ( p < 0.001).
To the best of our knowledge, this is the first Brazilian study to provide information on university students’ anxiety and depressive levels during the social isolation period. This study aimed to evaluate depression and anxiety in university students and their level of satisfaction with online learning during the period of social isolation caused by the COVID-19 pandemic. The participants presented moderate anxiety levels, with no significant differences between genders, and mild levels of depressive symptoms with significant differences between genders. Also, younger students were more anxious than older students. In addition, female students with less social contact presented higher levels of depression. Our results align with a U.S. nationwide survey [ 18 , 19 ] among faculty and students in June 2020, which highlighted the gender disparities in online learning during the pandemic, whereby female faculty and students reported more challenges in technological issues and adapting to remote learning compared with their male peers. Another study [ 20 ] showed almost half of students presenting anxiety levels ranging from mild to severe, with females reporting higher anxiety scores. Also, Saddick et al. [ 21 ], in a large sample of 7,228 university students from Poland, demonstrated a significant increase in depression levels as the pandemic progressed, with female students scoring significantly higher than male students on depression, anxiety, and stress. Similar studies conducted longitudinally among college students found a significant increase in depression and anxiety compared to previous COVID-19 levels [ 16 ].
The COVID-19 pandemic has disrupted the lives of all, including university students, especially with the preventive measures to reduce the transmission of virus, leading to all face-to-face teaching and learning being converted to e-learning. The COVID-19 pandemic and the implementation of e-learning may have influenced students’ mental conditions. A study aimed to determine the association of factors with mental health status (depression, anxiety, and stress) among tertiary education students in Malaysia, from both private and public universities, recruited via university emails and social media. The survey was administered via the online REDCap platform, from April to June 2020, during the movement control order period in the country. The questionnaire captured data on socio-demographic characteristics, academic information, implementation of e-learning, perception towards e-learning and COVID-19; as well as DASS 21 to screen for depression, anxiety, and stress. The levels of stress, anxiety and depression were 56.5% (95% CI: 50.7%, 62.1%), 51.3% (95% CI: 45.6%, 57.0%), and 29.4% (95% CI: 24.3%, 34.8%) respectively. Most participants had a good perception of e-learning but a negative perception of COVID-19.
The present study shows that social isolation contributed to depressive symptoms in university students. The impact of the social isolation period on university students may be burdensome due to its perceived effect on their activities of daily living and studies [ 13 ]. Fawaz and Samaha (2020) point out that university students are characteristically susceptible to developing stress and depression with an expected increase during the COVID-19 pandemic related to their psychological challenges, conditions in terms of learning, uncertainties about the future, fear of infection, news about lack of personal protective equipment, quarantine induced boredom, frustrations, lack of freedom, and fears caused by rumors and misleading news in the media [ 10 , 20 , 21 ]. Moreover, social isolation may also result in sedentary behavior, which is detrimental to preventing physical, cognitive, psychological, and social health problems [ 15 ]. Thus, low self-esteem, feelings of worthlessness, and loss of autonomy may also be related to the presence of levels of anxiety and depressive symptoms found in our study. Further studies should investigate psychological distress to evaluate its impact on depression and anxiety levels in this population.
Our results align with a study that explored the association between the effects of home-based learning during the pandemic and the risks of depression, anxiety, and suicidality among junior and senior high school students. An online survey using the Patient Health Questionnaire (PHQ-9) and Generalized Anxiety Disorder (GAD-7) was conducted between 12 and 30 April 2020, on a total of 39,751 students. Multivariable logistic regression analysis was used to analyze the risk factors of associated depression, anxiety, and suicidality during the pandemic. The prevalence of depression, anxiety symptoms, and suicidality found was 16.3% (95% CI: 16.0, 16.7), 10.3% (95% CI: 10.0, 10.6), and 20.3% (95% CI: 19.9, 20.7), respectively. Female participants and those in junior high school with poor overall sleep quality, poor academic performance, and very worried about being infected during COVID-19 were highly associated with the risk of depression, anxiety symptoms, and suicidal ideation [ 21 ]. Another study, conducted via an online survey among 5100 medical students from Wannan Medical College in China, aimed to assess the mental health status of medical students engaged in online learning at home during the pandemic, exploring the potential risk factors for mental health. The Depression, Anxiety and Stress scale (DASS-21) was used to measure self-reported symptoms of depression, anxiety, and stress among 4115 medical students. Nearly one-third of medical students survived with varying degrees of depression, anxiety, and stress symptoms during online learning in the COVID-19 pandemic [ 22 ]. These findings demonstrated that the mental status of university students was greatly affected during the COVID-19 pandemic [ 23 ].
We also investigated the level of satisfaction with online learning and its impact on students’ lives. We found that students who felt impacted by their financial situation had an increase in their anxiety as demonstrated on the HADS scale, corroborating studies that show the mental and emotional impacts on students’ daily lives [ 20 , 21 ]. Additionally, we found that most of the students considered online learning as regular, with significant differences between the level of anxiety and depressive symptoms and level of satisfaction with online education. Most of the students reported an emotional impact related to the social isolation period and online learning, with significant differences in depressive symptoms, followed by learning impact, financial impact, social impact, and technological impact. We also found that younger students reported more dissatisfaction with online learning compared to older students. Students’ intentions and attitudes towards online education may play important roles in retention rates and final achievements in online learning. Studies have shown that student interactions have a close relationship with emotional and social engagement and a sense of community, which is significant in effectively promoting learning engagement [ 21 ]. We may assume that these students may have to deal with unexpected and continuous changes such as lack of interpersonal contact and daily university activity and the need to adapt to their home routine and resources. However, besides these personal aspects, there is a need to discuss the effectiveness of online learning and its potential barriers in developing contexts. Students from developing countries presented lower scores in online learning and were more likely to withdraw from online courses than their colleagues in developed countries [ 21 ].
One of the major challenges in the Brazilian education system is the inequality of educational resources, including usage of computers, internet access, and other technological resources [ 8 ]. A survey conducted by a group of institutions in Brazil found that internet access (23%) was the main issue in remote learning, followed by content difficulties (20%), lack of devices (15%), and lack of interest (15%) [ 16 , 22 ]. Therefore, our results may be related to both students’ intentions and attitudes and the quality of educational resources.
Strengths and limitations
Our findings can help the development of actions to identify the need for medical and psychological interventions for university students during periods of online learning. For example, universities should incorporate epidemiological practices and involve health professionals as supervisors and counselors throughout the programs [ 24 , 25 ]. However, our results should be interpreted with caution as this study has several limitations. First, the use of a small convenience sample and its descriptive nature through an online survey with few variables may not allow generalization of the results. Students already diagnosed with depression or anxiety were excluded from the study through an interview prior to the start of testing. Anxiety and depressive symptoms may have been due to many factors other than COVID-19, which may not have been captured through this method. Secondly, the nature of self-reported data in the survey may lead to response biases. This study mainly used self-reported questionnaires to measure psychiatric symptoms and did not make a clinical diagnosis. The gold standard for establishing a psychiatric diagnosis involves a structured clinical interview and functional neuroimaging. In addition, the statistical analysis did not provide evidence of a causal nature. However, our hypotheses were well targeted based on the psychological evidence available in the previous literature [ 26 , 27 , 28 , 29 ]. Finally, we did not adjust for multiple comparisons, which may bias P -values as measures of significance. However, our results are clinically significant as they may provide suggestions for policy makers regarding improving students’ performance and prevent mental health problems.
Conclusions
Clinically, our findings provide insights into mental health among some university students during the early stages of the COVID-19 pandemic. These findings can be used to better identify students who may struggle during the following stages of the pandemic and in future crises. Our findings can also contribute to the development of effective screening strategies and the formulation of interventions that improve students’ mental health and may even help in the development of strategies to keep students in education.
It is important that students who perceive the need for psychological support can seek professional help to prevent and reduce symptoms.
Availability of data and materials
The data sets used during the current study can be provided by the corresponding author [L.P], upon reasonable request.
Banerjee A, Kulcsar K, Misra V, Frieman M, Mossman K. Bats and Coronaviruses. Viruses. 2019;11(1):41.
Article Google Scholar
World Health Organization. Coronavirus Disease ( Covid-19 ) Outbreak : Rights , Roles and Responsibilities of Health Workers, Including Key Considerations for Occupational Safety. World Heal Organ [Internet]. 2019;1–3. Available from: [ https://www.who.int/docs/default-source/coronaviruse/who-rights-roles-respon-hw-covid-19.pdf?sfvrsn=bcabd401_0 ]
Coronavirus Disease (COVID-19) Advice For The Public. Geneva: World Health Organization. (2020a).
Garip G, Seneviratne SR, Iacovou S. Learners’ perceptions and experiences of studying psychology online. J Comput Educ. 2020. https://doi.org/10.1007/s40692-020-00167-4 .
Martinez PJ, Aguilar FJ, Ortiz M. Transitioning from face-to-face to blended and full online learning engineering master’s program. IEEE Trans Educ. 2020;63(1):2–9.
Keser Aschenberger F, Radinger G, Brachtl S, Ipser C, Oppl S. Physical home learning environments for digitally-supported learning in academic continuing education during COVID-19 pandemic. Learn Environ Res. 2022;24:1–31. https://doi.org/10.1007/s10984-022-09406-0 .
Wang C, Pan R, Wan X, Tan Y, Xu L, Ho CS, et al. Immediate psychological responses and associated factors during the initial stage of the 2019 coronavirus disease (COVID-19) epidemic among the general population in China. Int J Environ Res Public Health. 2020. https://doi.org/10.3390/ijerph17051729 .
Article PubMed PubMed Central Google Scholar
Sindiani AM, Obeidat N, Alshdaifat E, Elsalem L, Alwani MM, Rawashdeh H, et al. Distance education during the COVID-19 outbreak: a cross-sectional study among medical students in North of Jordan. Ann Med Surg. 2020;59:186–94.
Remote learning in Brazil during the pandemic - News - Lemann Foundation [Internet]. [cited 2021 Sep 24].
Mirna F, Ali S. E-learning: depression, anxiety, and stress symptomatology among Lebanese university students during COVID-19 quarantine. Nurs Forum. 2021;56(1):52–7.
Kasse J, Balunywa W. An assessment of e-learning utilization by a section of Ugandan universities: challenges, success factors and way forward. undefined. 2013.
McLafferty M, Brown N, McHugh R, Ward C, Stevenson A, McBride L, Brady J, Bjourson AJ, O’Neill SM, Walsh CP, Murray EK. Depression, anxiety and suicidal behaviour among college students: comparisons pre-COVID-19 and during the pandemic. Psychiatry Res Commun. 2021;1(2):100012. https://doi.org/10.1016/j.psycom.2021.100012 .
Article PubMed Google Scholar
Le HT, Lai AJX, Sun J, Hoang MT, Vu LG, Pham HQ, et al. Anxiety and depression among people under the nationwide partial lockdown in Vietnam. Front Public Heal. 2020;8:589359. https://doi.org/10.3389/fpubh.2020.589359 .
TMGH-Global COVID-19 Collaborative. Psychological impacts and post-traumatic stress disorder among people under COVID-19 quarantine and isolation: a global survey. Int J Environ Res Public Health. 2021;18:5719. https://doi.org/10.3390/ijerph18115719 .
Article PubMed Central Google Scholar
Cleofas JV, Rocha ICN. Demographic, gadget and internet profiles as determinants of disease and consequence related COVID-19 anxiety among Filipino college students. Educ Inf Technol. 2021. https://doi.org/10.1007/s10639-021-10529-9 .
Cedeño TDD, Rocha ICN, Ramos KG, Uy NMC. Learning strategies and innovations among medical students in the Philippines during the COVID-19 pandemic. Int J Med Stud. 2021;9(1):77–9. https://doi.org/10.5195/ijms.2021.908 .
Botega NJ, Bio MR, Zomignani MA, Garcia C Jr, Pereira WAB. Transtornos do humor em enfermaria de clínica médica e validação de escala de medida (HAD) de ansiedade e depressão. Rev Saude Publica. 1995;29(5):359–63.
da Silva DAR, Pimentel RFW, das Merces MC. Covid-19 and the pandemic of fear: reflections on mental health. Rev Saude Publica. 2020;54:46.
Chang J, Yuan Y, Wang D. Mental health status and its influencing factors among college students during the epidemic of COVID-19. Nan Fang Yi Ke Da Xue Xue Bao. 2020;40(2):171–6.
PubMed Google Scholar
Saw GK, Chang C-N, Lomeli U, Zhi M. Gender Disparities in Remote Learning during the COVID-19 Pandemic: A National Survey of STEM Faculty and Students – Network for Research and Evaluation in Education. Netw Res Eval Educ. 2020; NREED Data(2). Available from: https://nreeducation.wordpress.com/2020/08/07/gender-disparities-in-remote-learning-during-the-covid-19-pandemic-a-national-survey-of-stem-faculty-and-students/
Saddik B, Hussein A, Sharif-Askari FS, Kheder W, Temsah MH, Koutaich RA, et al. Increased levels of anxiety among medical and non-medical university students during the COVID-19 pandemic in the United Arab Emirates. Risk Manag Healthc Policy. 2020;13:2395–406.
Mw Z, Rc H. Moodle: the cost effective solution for internet cognitive behavioral therapy (I-CBT) interventions. Technol Health Care. 2017;25(1):163–5.
Chang WW, Shi LX, Zhang L, Jin YL, Yu JG. The mental health status and associated factors among medical students engaged in online learning at home during the pandemic: a cross-sectional study from China. Front Psychiatry. 2021;23(12): 755503. https://doi.org/10.3389/fpsyt.2021.755503 .
Lischer S, Safi N, Dickson C. Remote learning and students’ mental health during the Covid-19 pandemic: a mixed-method enquiry. Prospects. 2021. https://doi.org/10.1007/s11125-020-09530-w .
Li HY, Cao H, Leung DYP, Mak YW. The psychological impacts of a COVID-19 outbreak on college students in China: a longitudinal study. Int J Environ Res Public Heal. 2020;17(11):3933.
Debowska A, Horeczy B, Boduszek D, Dolinski D. A repeated cross-sectional survey assessing university students’ stress, depression, anxiety, and suicidality in the early stages of the COVID-19 pandemic in Poland. Psychol Med. 2020. https://doi.org/10.1017/S003329172000392X .
Ho CS, Chee CY, Ho RC. Mental health strategies to combat the psychological impact of coronavirus disease 2019 (COVID-19) beyond paranoia and panic. Ann Acad Med Singapore. 2020;49(3):155–60.
World Health Organization–Coronavirus disease (COVID-19)/Timeline: WHO’S COVID-19 response. 2020.
Peng X, Liang S, Liu L, Cai C, Chen J, Huang A, Wang X, Zhao J. Prevalence and associated factors of depression, anxiety and suicidality among Chinese high school E-learning students during the COVID-19 lockdown. Curr Psychol. 2022;27:1–12. https://doi.org/10.1007/s12144-021-02512-x .
Download references
Acknowledgements
Marcia Cristina Nascimento Dourado and Antonio Egidio Nardi are researchers funded by CNPq and FAPERJ.
Marcia Cristina Nascimento Dourado and Antonio Egidio Nardi are researchers funded by the Conselho Nacional de Desenvolvimento Científico e Tecnológico (National Council for Scientific and Technological Development) (CNPq) and Fundação Carlos Chagas Filho de Amparo à Pesquisa do Estado do Rio de Janeiro (Carlos Chagas Filho Foundation for Research Support in the State of Rio de Janeiro)–FAPERJ.
Author information
Authors and affiliations.
Institute of Psychiatry, Universidade Federal Do Rio de Janeiro (UFRJ), Rio de Janeiro (RJ), Brazil
Luísa Pelucio, Marcia Cristina Nascimento Dourado, Laiana A. Quagliato & Antonio Egidio Nardi
Departament of Sociology and Political Science, Universidade Federal de Santa Catarina (UFSC), Rio de Janeiro (RJ), Brazil
Pedro Simões
You can also search for this author in PubMed Google Scholar
Contributions
PL. was involved in designing the study, collecting the data, and writing the paper. SP. performed the statistical analyses. DMCN. supervised and assisted with the writing and approved the final paper. QLA. Reviewed, edited, and approved the final paper. NAE. guided and approved the final work. All authors read and approved the final manuscript.
Corresponding author
Correspondence to Luísa Pelucio .
Ethics declarations
Ethical approval and consent to participate.
The Ethics Committee of the Institute of Psychiatry of the Universidade Federal do Rio de Janeiro (Federal University of Rio de Janeiro) (UFRJ) approved the study and all participants signed the informed consent form. This study followed the Declaration of Helsinki."
Consent for publication
Not applicable.
Competing interests
The authors declare no competing interests, financial or otherwise .
Additional information
Publisher's note.
Springer Nature remains neutral with regard to jurisdictional claims in published maps and institutional affiliations.
Rights and permissions
Open Access This article is licensed under a Creative Commons Attribution 4.0 International License, which permits use, sharing, adaptation, distribution and reproduction in any medium or format, as long as you give appropriate credit to the original author(s) and the source, provide a link to the Creative Commons licence, and indicate if changes were made. The images or other third party material in this article are included in the article's Creative Commons licence, unless indicated otherwise in a credit line to the material. If material is not included in the article's Creative Commons licence and your intended use is not permitted by statutory regulation or exceeds the permitted use, you will need to obtain permission directly from the copyright holder. To view a copy of this licence, visit http://creativecommons.org/licenses/by/4.0/ . The Creative Commons Public Domain Dedication waiver ( http://creativecommons.org/publicdomain/zero/1.0/ ) applies to the data made available in this article, unless otherwise stated in a credit line to the data.
Reprints and permissions
About this article
Cite this article.
Pelucio, L., Simões, P., Dourado, M.C.N. et al. Depression and anxiety among online learning students during the COVID-19 pandemic: a cross-sectional survey in Rio de Janeiro, Brazil. BMC Psychol 10 , 192 (2022). https://doi.org/10.1186/s40359-022-00897-3
Download citation
Received : 27 January 2022
Accepted : 27 July 2022
Published : 03 August 2022
DOI : https://doi.org/10.1186/s40359-022-00897-3
Share this article
Anyone you share the following link with will be able to read this content:
Sorry, a shareable link is not currently available for this article.
Provided by the Springer Nature SharedIt content-sharing initiative
- Online learning
- Mental health
BMC Psychology
ISSN: 2050-7283
- General enquiries: [email protected]
The pandemic has had devastating impacts on learning. What will it take to help students catch up?
Subscribe to the brown center on education policy newsletter, megan kuhfeld , megan kuhfeld senior research scientist - nwea @megankuhfeld jim soland , jim soland assistant professor, school of education and human development - university of virginia, affiliated research fellow - nwea @jsoland karyn lewis , and karyn lewis director, center for school and student progress - nwea @karynlew emily morton emily morton research scientist - nwea @emily_r_morton.
March 3, 2022
As we reach the two-year mark of the initial wave of pandemic-induced school shutdowns, academic normalcy remains out of reach for many students, educators, and parents. In addition to surging COVID-19 cases at the end of 2021, schools have faced severe staff shortages , high rates of absenteeism and quarantines , and rolling school closures . Furthermore, students and educators continue to struggle with mental health challenges , higher rates of violence and misbehavior , and concerns about lost instructional time .
As we outline in our new research study released in January, the cumulative impact of the COVID-19 pandemic on students’ academic achievement has been large. We tracked changes in math and reading test scores across the first two years of the pandemic using data from 5.4 million U.S. students in grades 3-8. We focused on test scores from immediately before the pandemic (fall 2019), following the initial onset (fall 2020), and more than one year into pandemic disruptions (fall 2021).
Average fall 2021 math test scores in grades 3-8 were 0.20-0.27 standard deviations (SDs) lower relative to same-grade peers in fall 2019, while reading test scores were 0.09-0.18 SDs lower. This is a sizable drop. For context, the math drops are significantly larger than estimated impacts from other large-scale school disruptions, such as after Hurricane Katrina—math scores dropped 0.17 SDs in one year for New Orleans evacuees .
Even more concerning, test-score gaps between students in low-poverty and high-poverty elementary schools grew by approximately 20% in math (corresponding to 0.20 SDs) and 15% in reading (0.13 SDs), primarily during the 2020-21 school year. Further, achievement tended to drop more between fall 2020 and 2021 than between fall 2019 and 2020 (both overall and differentially by school poverty), indicating that disruptions to learning have continued to negatively impact students well past the initial hits following the spring 2020 school closures.
These numbers are alarming and potentially demoralizing, especially given the heroic efforts of students to learn and educators to teach in incredibly trying times. From our perspective, these test-score drops in no way indicate that these students represent a “ lost generation ” or that we should give up hope. Most of us have never lived through a pandemic, and there is so much we don’t know about students’ capacity for resiliency in these circumstances and what a timeline for recovery will look like. Nor are we suggesting that teachers are somehow at fault given the achievement drops that occurred between 2020 and 2021; rather, educators had difficult jobs before the pandemic, and now are contending with huge new challenges, many outside their control.
Clearly, however, there’s work to do. School districts and states are currently making important decisions about which interventions and strategies to implement to mitigate the learning declines during the last two years. Elementary and Secondary School Emergency Relief (ESSER) investments from the American Rescue Plan provided nearly $200 billion to public schools to spend on COVID-19-related needs. Of that sum, $22 billion is dedicated specifically to addressing learning loss using “evidence-based interventions” focused on the “ disproportionate impact of COVID-19 on underrepresented student subgroups. ” Reviews of district and state spending plans (see Future Ed , EduRecoveryHub , and RAND’s American School District Panel for more details) indicate that districts are spending their ESSER dollars designated for academic recovery on a wide variety of strategies, with summer learning, tutoring, after-school programs, and extended school-day and school-year initiatives rising to the top.
Comparing the negative impacts from learning disruptions to the positive impacts from interventions
To help contextualize the magnitude of the impacts of COVID-19, we situate test-score drops during the pandemic relative to the test-score gains associated with common interventions being employed by districts as part of pandemic recovery efforts. If we assume that such interventions will continue to be as successful in a COVID-19 school environment, can we expect that these strategies will be effective enough to help students catch up? To answer this question, we draw from recent reviews of research on high-dosage tutoring , summer learning programs , reductions in class size , and extending the school day (specifically for literacy instruction) . We report effect sizes for each intervention specific to a grade span and subject wherever possible (e.g., tutoring has been found to have larger effects in elementary math than in reading).
Figure 1 shows the standardized drops in math test scores between students testing in fall 2019 and fall 2021 (separately by elementary and middle school grades) relative to the average effect size of various educational interventions. The average effect size for math tutoring matches or exceeds the average COVID-19 score drop in math. Research on tutoring indicates that it often works best in younger grades, and when provided by a teacher rather than, say, a parent. Further, some of the tutoring programs that produce the biggest effects can be quite intensive (and likely expensive), including having full-time tutors supporting all students (not just those needing remediation) in one-on-one settings during the school day. Meanwhile, the average effect of reducing class size is negative but not significant, with high variability in the impact across different studies. Summer programs in math have been found to be effective (average effect size of .10 SDs), though these programs in isolation likely would not eliminate the COVID-19 test-score drops.
Figure 1: Math COVID-19 test-score drops compared to the effect sizes of various educational interventions

Source: COVID-19 score drops are pulled from Kuhfeld et al. (2022) Table 5; reduction-in-class-size results are from pg. 10 of Figles et al. (2018) Table 2; summer program results are pulled from Lynch et al (2021) Table 2; and tutoring estimates are pulled from Nictow et al (2020) Table 3B. Ninety-five percent confidence intervals are shown with vertical lines on each bar.
Notes: Kuhfeld et al. and Nictow et al. reported effect sizes separately by grade span; Figles et al. and Lynch et al. report an overall effect size across elementary and middle grades. We were unable to find a rigorous study that reported effect sizes for extending the school day/year on math performance. Nictow et al. and Kraft & Falken (2021) also note large variations in tutoring effects depending on the type of tutor, with larger effects for teacher and paraprofessional tutoring programs than for nonprofessional and parent tutoring. Class-size reductions included in the Figles meta-analysis ranged from a minimum of one to minimum of eight students per class.
Figure 2 displays a similar comparison using effect sizes from reading interventions. The average effect of tutoring programs on reading achievement is larger than the effects found for the other interventions, though summer reading programs and class size reduction both produced average effect sizes in the ballpark of the COVID-19 reading score drops.
Figure 2: Reading COVID-19 test-score drops compared to the effect sizes of various educational interventions

Source: COVID-19 score drops are pulled from Kuhfeld et al. (2022) Table 5; extended-school-day results are from Figlio et al. (2018) Table 2; reduction-in-class-size results are from pg. 10 of Figles et al. (2018) ; summer program results are pulled from Kim & Quinn (2013) Table 3; and tutoring estimates are pulled from Nictow et al (2020) Table 3B. Ninety-five percent confidence intervals are shown with vertical lines on each bar.
Notes: While Kuhfeld et al. and Nictow et al. reported effect sizes separately by grade span, Figlio et al. and Kim & Quinn report an overall effect size across elementary and middle grades. Class-size reductions included in the Figles meta-analysis ranged from a minimum of one to minimum of eight students per class.
There are some limitations of drawing on research conducted prior to the pandemic to understand our ability to address the COVID-19 test-score drops. First, these studies were conducted under conditions that are very different from what schools currently face, and it is an open question whether the effectiveness of these interventions during the pandemic will be as consistent as they were before the pandemic. Second, we have little evidence and guidance about the efficacy of these interventions at the unprecedented scale that they are now being considered. For example, many school districts are expanding summer learning programs, but school districts have struggled to find staff interested in teaching summer school to meet the increased demand. Finally, given the widening test-score gaps between low- and high-poverty schools, it’s uncertain whether these interventions can actually combat the range of new challenges educators are facing in order to narrow these gaps. That is, students could catch up overall, yet the pandemic might still have lasting, negative effects on educational equality in this country.
Given that the current initiatives are unlikely to be implemented consistently across (and sometimes within) districts, timely feedback on the effects of initiatives and any needed adjustments will be crucial to districts’ success. The Road to COVID Recovery project and the National Student Support Accelerator are two such large-scale evaluation studies that aim to produce this type of evidence while providing resources for districts to track and evaluate their own programming. Additionally, a growing number of resources have been produced with recommendations on how to best implement recovery programs, including scaling up tutoring , summer learning programs , and expanded learning time .
Ultimately, there is much work to be done, and the challenges for students, educators, and parents are considerable. But this may be a moment when decades of educational reform, intervention, and research pay off. Relying on what we have learned could show the way forward.
Related Content
Megan Kuhfeld, Jim Soland, Beth Tarasawa, Angela Johnson, Erik Ruzek, Karyn Lewis
December 3, 2020
Lindsay Dworkin, Karyn Lewis
October 13, 2021
Education Policy K-12 Education
Governance Studies
Brown Center on Education Policy
Darcy Hutchins, Emily Markovich Morris, Laura Nora, Carolina Campos, Adelaida Gómez Vergara, Nancy G. Gordon, Esmeralda Macana, Karen Robertson
March 28, 2024
Jennifer B. Ayscue, Kfir Mordechay, David Mickey-Pabello
March 26, 2024
Anna Saavedra, Morgan Polikoff, Dan Silver
- Share full article
Advertisement
Supported by
What the Data Says About Pandemic School Closures, Four Years Later
The more time students spent in remote instruction, the further they fell behind. And, experts say, extended closures did little to stop the spread of Covid.

By Sarah Mervosh , Claire Cain Miller and Francesca Paris
Four years ago this month, schools nationwide began to shut down, igniting one of the most polarizing and partisan debates of the pandemic.
Some schools, often in Republican-led states and rural areas, reopened by fall 2020. Others, typically in large cities and states led by Democrats, would not fully reopen for another year.
A variety of data — about children’s academic outcomes and about the spread of Covid-19 — has accumulated in the time since. Today, there is broad acknowledgment among many public health and education experts that extended school closures did not significantly stop the spread of Covid, while the academic harms for children have been large and long-lasting.
While poverty and other factors also played a role, remote learning was a key driver of academic declines during the pandemic, research shows — a finding that held true across income levels.
Source: Fahle, Kane, Patterson, Reardon, Staiger and Stuart, “ School District and Community Factors Associated With Learning Loss During the COVID-19 Pandemic .” Score changes are measured from 2019 to 2022. In-person means a district offered traditional in-person learning, even if not all students were in-person.
“There’s fairly good consensus that, in general, as a society, we probably kept kids out of school longer than we should have,” said Dr. Sean O’Leary, a pediatric infectious disease specialist who helped write guidance for the American Academy of Pediatrics, which recommended in June 2020 that schools reopen with safety measures in place.
There were no easy decisions at the time. Officials had to weigh the risks of an emerging virus against the academic and mental health consequences of closing schools. And even schools that reopened quickly, by the fall of 2020, have seen lasting effects.
But as experts plan for the next public health emergency, whatever it may be, a growing body of research shows that pandemic school closures came at a steep cost to students.
The longer schools were closed, the more students fell behind.
At the state level, more time spent in remote or hybrid instruction in the 2020-21 school year was associated with larger drops in test scores, according to a New York Times analysis of school closure data and results from the National Assessment of Educational Progress , an authoritative exam administered to a national sample of fourth- and eighth-grade students.
At the school district level, that finding also holds, according to an analysis of test scores from third through eighth grade in thousands of U.S. districts, led by researchers at Stanford and Harvard. In districts where students spent most of the 2020-21 school year learning remotely, they fell more than half a grade behind in math on average, while in districts that spent most of the year in person they lost just over a third of a grade.
( A separate study of nearly 10,000 schools found similar results.)
Such losses can be hard to overcome, without significant interventions. The most recent test scores, from spring 2023, show that students, overall, are not caught up from their pandemic losses , with larger gaps remaining among students that lost the most ground to begin with. Students in districts that were remote or hybrid the longest — at least 90 percent of the 2020-21 school year — still had almost double the ground to make up compared with students in districts that allowed students back for most of the year.
Some time in person was better than no time.
As districts shifted toward in-person learning as the year went on, students that were offered a hybrid schedule (a few hours or days a week in person, with the rest online) did better, on average, than those in places where school was fully remote, but worse than those in places that had school fully in person.
Students in hybrid or remote learning, 2020-21
80% of students
Some schools return online, as Covid-19 cases surge. Vaccinations start for high-priority groups.
Teachers are eligible for the Covid vaccine in more than half of states.
Most districts end the year in-person or hybrid.
Source: Burbio audit of more than 1,200 school districts representing 47 percent of U.S. K-12 enrollment. Note: Learning mode was defined based on the most in-person option available to students.
Income and family background also made a big difference.
A second factor associated with academic declines during the pandemic was a community’s poverty level. Comparing districts with similar remote learning policies, poorer districts had steeper losses.
But in-person learning still mattered: Looking at districts with similar poverty levels, remote learning was associated with greater declines.
A community’s poverty rate and the length of school closures had a “roughly equal” effect on student outcomes, said Sean F. Reardon, a professor of poverty and inequality in education at Stanford, who led a district-level analysis with Thomas J. Kane, an economist at Harvard.
Score changes are measured from 2019 to 2022. Poorest and richest are the top and bottom 20% of districts by percent of students on free/reduced lunch. Mostly in-person and mostly remote are districts that offered traditional in-person learning for more than 90 percent or less than 10 percent of the 2020-21 year.
But the combination — poverty and remote learning — was particularly harmful. For each week spent remote, students in poor districts experienced steeper losses in math than peers in richer districts.
That is notable, because poor districts were also more likely to stay remote for longer .
Some of the country’s largest poor districts are in Democratic-leaning cities that took a more cautious approach to the virus. Poor areas, and Black and Hispanic communities , also suffered higher Covid death rates, making many families and teachers in those districts hesitant to return.
“We wanted to survive,” said Sarah Carpenter, the executive director of Memphis Lift, a parent advocacy group in Memphis, where schools were closed until spring 2021 .
“But I also think, man, looking back, I wish our kids could have gone back to school much quicker,” she added, citing the academic effects.
Other things were also associated with worse student outcomes, including increased anxiety and depression among adults in children’s lives, and the overall restriction of social activity in a community, according to the Stanford and Harvard research .
Even short closures had long-term consequences for children.
While being in school was on average better for academic outcomes, it wasn’t a guarantee. Some districts that opened early, like those in Cherokee County, Ga., a suburb of Atlanta, and Hanover County, Va., lost significant learning and remain behind.
At the same time, many schools are seeing more anxiety and behavioral outbursts among students. And chronic absenteeism from school has surged across demographic groups .
These are signs, experts say, that even short-term closures, and the pandemic more broadly, had lasting effects on the culture of education.
“There was almost, in the Covid era, a sense of, ‘We give up, we’re just trying to keep body and soul together,’ and I think that was corrosive to the higher expectations of schools,” said Margaret Spellings, an education secretary under President George W. Bush who is now chief executive of the Bipartisan Policy Center.
Closing schools did not appear to significantly slow Covid’s spread.
Perhaps the biggest question that hung over school reopenings: Was it safe?
That was largely unknown in the spring of 2020, when schools first shut down. But several experts said that had changed by the fall of 2020, when there were initial signs that children were less likely to become seriously ill, and growing evidence from Europe and parts of the United States that opening schools, with safety measures, did not lead to significantly more transmission.
“Infectious disease leaders have generally agreed that school closures were not an important strategy in stemming the spread of Covid,” said Dr. Jeanne Noble, who directed the Covid response at the U.C.S.F. Parnassus emergency department.
Politically, though, there remains some disagreement about when, exactly, it was safe to reopen school.
Republican governors who pushed to open schools sooner have claimed credit for their approach, while Democrats and teachers’ unions have emphasized their commitment to safety and their investment in helping students recover.
“I do believe it was the right decision,” said Jerry T. Jordan, president of the Philadelphia Federation of Teachers, which resisted returning to school in person over concerns about the availability of vaccines and poor ventilation in school buildings. Philadelphia schools waited to partially reopen until the spring of 2021 , a decision Mr. Jordan believes saved lives.
“It doesn’t matter what is going on in the building and how much people are learning if people are getting the virus and running the potential of dying,” he said.
Pandemic school closures offer lessons for the future.
Though the next health crisis may have different particulars, with different risk calculations, the consequences of closing schools are now well established, experts say.
In the future, infectious disease experts said, they hoped decisions would be guided more by epidemiological data as it emerged, taking into account the trade-offs.
“Could we have used data to better guide our decision making? Yes,” said Dr. Uzma N. Hasan, division chief of pediatric infectious diseases at RWJBarnabas Health in Livingston, N.J. “Fear should not guide our decision making.”
Source: Fahle, Kane, Patterson, Reardon, Staiger and Stuart, “ School District and Community Factors Associated With Learning Loss During the Covid-19 Pandemic. ”
The study used estimates of learning loss from the Stanford Education Data Archive . For closure lengths, the study averaged district-level estimates of time spent in remote and hybrid learning compiled by the Covid-19 School Data Hub (C.S.D.H.) and American Enterprise Institute (A.E.I.) . The A.E.I. data defines remote status by whether there was an in-person or hybrid option, even if some students chose to remain virtual. In the C.S.D.H. data set, districts are defined as remote if “all or most” students were virtual.
An earlier version of this article misstated a job description of Dr. Jeanne Noble. She directed the Covid response at the U.C.S.F. Parnassus emergency department. She did not direct the Covid response for the University of California, San Francisco health system.
How we handle corrections
Sarah Mervosh covers education for The Times, focusing on K-12 schools. More about Sarah Mervosh
Claire Cain Miller writes about gender, families and the future of work for The Upshot. She joined The Times in 2008 and was part of a team that won a Pulitzer Prize in 2018 for public service for reporting on workplace sexual harassment issues. More about Claire Cain Miller
Francesca Paris is a Times reporter working with data and graphics for The Upshot. More about Francesca Paris
- Campus News
- Student News
- UK HealthCare
- UK Happenings
- Arts & Culture
- Professional News
UK duo close Johns Hopkins online teaching symposium with podcast session
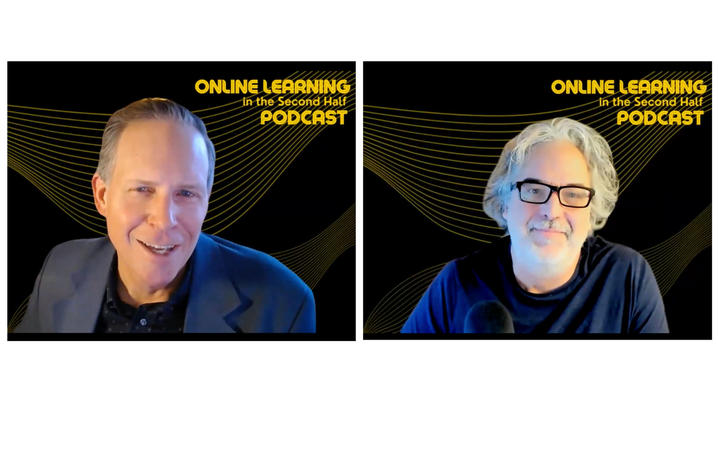
LEXINGTON, Ky. (April 1, 2024) — University of Kentucky College of Education Associate Professor John Nash, Ph.D., and Jason Johnston, Ph.D., hosts of the Online Learning in the Second Half podcast, facilitated a conversation about the future of online learning during a recent Johns Hopkins University symposium. The session is now available as a podcast episode for listening online.
Nash is an associate professor of educational leadership studies and director of the Laboratory on Design Thinking at the UK College of Education. Johnston was Nash’s doctoral student and earned his Ph.D. from the Department of Educational Leadership Studies in 2021. He is the executive director of online learning and course production at the University of Tennessee.
The podcast episode was recorded during the closing session of the inaugural Johns Hopkins Center for Teaching Excellence and Innovation virtual symposium, themed Excellence in Online Teaching. It featured sessions from experts who have been involved in online education at Johns Hopkins and beyond.
Nash and Johnston’s session, “Humanizing Online Learning: A Podcast Conversation,” was an interactive and participatory discussion .
" John Nash and Jason Johnston synthesized key insights from the symposium’s sessions and invited attendees to share their own experiences and perspectives on how to make online learning more engaging and inclusive. It was a nice way to share key messages from the symposium even more widely, by recording and publishing a podcast from the event,” said Olysha Magruder, Ed.D., interim assistant dean, Learning Design and Innovation, Center for Learning Design and Technology at Johns Hopkins Whiting School of Engineering.
Nash and Johnston think online learning had its chance to become good, but there’s a chance to be even better. The future extends beyond technology and innovation. Treating humans as individuals is a first step to improving the online learning experience, the hosts said.
“Making online learning human-centric touches all parts of the learning space – the interactions between students, the way we structure our courses, how we grade our assignments, what instructors share about themselves and so on,” Johnston said. “We move beyond the course’s content when we show up as our authentic selves.”
Podcast participants recalled ways the symposium’s speakers had sparked ideas for creating a stronger human connection in online courses – showing photos and telling stories, sharing what drives their interest in the work and empowering students to do the same.
“Online courses are not content. They are experiences. And to create a great experience, we must thread in all these other things with intention. This creates great active experiences on the learning side as the learner goes through the journey,” Nash said.
The podcast episode synthesizing the John s Hopkins symposium is among two dozen episodes posted so far. Nash said he and Johnston started the podcast to share their passion and expertise in online learning with a wider audience.
“We wanted to create a platform where we could explore the challenges and opportunities of online learning and showcase the best practices and innovations from various disciplines and contexts. We also wanted to have fun and learn from each other, as well as from our guests and listeners.”
The podcast, which can be accessed at OnlineLearningPodcast.com , covers a wide range of topics related to online learning, such as pedagogical case studies, edtech innovations, teaching best practices and policy issues drawing on interviews with experts from various fields, such as cognitive psychology, data science, instructional design and more.
“We were thrilled to have this opportunity to share our passion and expertise in online learning with the Johns Hopkins community and beyond. We hope the conversation will inspire and inform listeners of our podcast,” Nash said.
As the state’s flagship, land-grant institution, the University of Kentucky exists to advance the Commonwealth. We do that by preparing the next generation of leaders — placing students at the heart of everything we do — and transforming the lives of Kentuckians through education, research and creative work, service and health care. We pride ourselves on being a catalyst for breakthroughs and a force for healing, a place where ingenuity unfolds. It's all made possible by our people — visionaries, disruptors and pioneers — who make up 200 academic programs, a $476.5 million research and development enterprise and a world-class medical center, all on one campus.
In 2022, UK was ranked by Forbes as one of the “Best Employers for New Grads” and named a “Diversity Champion” by INSIGHT into Diversity, a testament to our commitment to advance Kentucky and create a community of belonging for everyone. While our mission looks different in many ways than it did in 1865, the vision of service to our Commonwealth and the world remains the same. We are the University for Kentucky.
Latest Stories
Women making history: uk researcher champions thinking through making, 'behind the blue': ashley montgomery-yates on uk healthcare’s role in critical care, uk celebrates national public health week with opportunities to learn and engage, april is sexual assault awareness and prevention month.

An official website of the United States government
The .gov means it’s official. Federal government websites often end in .gov or .mil. Before sharing sensitive information, make sure you’re on a federal government site.
The site is secure. The https:// ensures that you are connecting to the official website and that any information you provide is encrypted and transmitted securely.
- Publications
- Account settings
Preview improvements coming to the PMC website in October 2024. Learn More or Try it out now .
- Advanced Search
- Journal List
- Wiley - PMC COVID-19 Collection

Online and face‐to‐face learning: Evidence from students’ performance during the Covid‐19 pandemic
Carolyn chisadza.
1 Department of Economics, University of Pretoria, Hatfield South Africa
Matthew Clance
Thulani mthembu.
2 Department of Education Innovation, University of Pretoria, Hatfield South Africa
Nicky Nicholls
Eleni yitbarek.
This study investigates the factors that predict students' performance after transitioning from face‐to‐face to online learning as a result of the Covid‐19 pandemic. It uses students' responses from survey questions and the difference in the average assessment grades between pre‐lockdown and post‐lockdown at a South African university. We find that students' performance was positively associated with good wifi access, relative to using mobile internet data. We also observe lower academic performance for students who found transitioning to online difficult and who expressed a preference for self‐study (i.e. reading through class slides and notes) over assisted study (i.e. joining live lectures or watching recorded lectures). The findings suggest that improving digital infrastructure and reducing the cost of internet access may be necessary for mitigating the impact of the Covid‐19 pandemic on education outcomes.
1. INTRODUCTION
The Covid‐19 pandemic has been a wake‐up call to many countries regarding their capacity to cater for mass online education. This situation has been further complicated in developing countries, such as South Africa, who lack the digital infrastructure for the majority of the population. The extended lockdown in South Africa saw most of the universities with mainly in‐person teaching scrambling to source hardware (e.g. laptops, internet access), software (e.g. Microsoft packages, data analysis packages) and internet data for disadvantaged students in order for the semester to recommence. Not only has the pandemic revealed the already stark inequality within the tertiary student population, but it has also revealed that high internet data costs in South Africa may perpetuate this inequality, making online education relatively inaccessible for disadvantaged students. 1
The lockdown in South Africa made it possible to investigate the changes in second‐year students' performance in the Economics department at the University of Pretoria. In particular, we are interested in assessing what factors predict changes in students' performance after transitioning from face‐to‐face (F2F) to online learning. Our main objectives in answering this study question are to establish what study materials the students were able to access (i.e. slides, recordings, or live sessions) and how students got access to these materials (i.e. the infrastructure they used).
The benefits of education on economic development are well established in the literature (Gyimah‐Brempong, 2011 ), ranging from health awareness (Glick et al., 2009 ), improved technological innovations, to increased capacity development and employment opportunities for the youth (Anyanwu, 2013 ; Emediegwu, 2021 ). One of the ways in which inequality is perpetuated in South Africa, and Africa as a whole, is through access to education (Anyanwu, 2016 ; Coetzee, 2014 ; Tchamyou et al., 2019 ); therefore, understanding the obstacles that students face in transitioning to online learning can be helpful in ensuring more equal access to education.
Using students' responses from survey questions and the difference in the average grades between pre‐lockdown and post‐lockdown, our findings indicate that students' performance in the online setting was positively associated with better internet access. Accessing assisted study material, such as narrated slides or recordings of the online lectures, also helped students. We also find lower academic performance for students who reported finding transitioning to online difficult and for those who expressed a preference for self‐study (i.e. reading through class slides and notes) over assisted study (i.e. joining live lectures or watching recorded lectures). The average grades between pre‐lockdown and post‐lockdown were about two points and three points lower for those who reported transitioning to online teaching difficult and for those who indicated a preference for self‐study, respectively. The findings suggest that improving the quality of internet infrastructure and providing assisted learning can be beneficial in reducing the adverse effects of the Covid‐19 pandemic on learning outcomes.
Our study contributes to the literature by examining the changes in the online (post‐lockdown) performance of students and their F2F (pre‐lockdown) performance. This approach differs from previous studies that, in most cases, use between‐subject designs where one group of students following online learning is compared to a different group of students attending F2F lectures (Almatra et al., 2015 ; Brown & Liedholm, 2002 ). This approach has a limitation in that that there may be unobserved characteristics unique to students choosing online learning that differ from those choosing F2F lectures. Our approach avoids this issue because we use a within‐subject design: we compare the performance of the same students who followed F2F learning Before lockdown and moved to online learning during lockdown due to the Covid‐19 pandemic. Moreover, the study contributes to the limited literature that compares F2F and online learning in developing countries.
Several studies that have also compared the effectiveness of online learning and F2F classes encounter methodological weaknesses, such as small samples, not controlling for demographic characteristics, and substantial differences in course materials and assessments between online and F2F contexts. To address these shortcomings, our study is based on a relatively large sample of students and includes demographic characteristics such as age, gender and perceived family income classification. The lecturer and course materials also remained similar in the online and F2F contexts. A significant proportion of our students indicated that they never had online learning experience before. Less than 20% of the students in the sample had previous experience with online learning. This highlights the fact that online education is still relatively new to most students in our sample.
Given the global experience of the fourth industrial revolution (4IR), 2 with rapidly accelerating technological progress, South Africa needs to be prepared for the possibility of online learning becoming the new norm in the education system. To this end, policymakers may consider engaging with various organizations (schools, universities, colleges, private sector, and research facilities) To adopt interventions that may facilitate the transition to online learning, while at the same time ensuring fair access to education for all students across different income levels. 3
1.1. Related literature
Online learning is a form of distance education which mainly involves internet‐based education where courses are offered synchronously (i.e. live sessions online) and/or asynchronously (i.e. students access course materials online in their own time, which is associated with the more traditional distance education). On the other hand, traditional F2F learning is real time or synchronous learning. In a physical classroom, instructors engage with the students in real time, while in the online format instructors can offer real time lectures through learning management systems (e.g. Blackboard Collaborate), or record the lectures for the students to watch later. Purely online courses are offered entirely over the internet, while blended learning combines traditional F2F classes with learning over the internet, and learning supported by other technologies (Nguyen, 2015 ).
Moreover, designing online courses requires several considerations. For example, the quality of the learning environment, the ease of using the learning platform, the learning outcomes to be achieved, instructor support to assist and motivate students to engage with the course material, peer interaction, class participation, type of assessments (Paechter & Maier, 2010 ), not to mention training of the instructor in adopting and introducing new teaching methods online (Lundberg et al., 2008 ). In online learning, instructors are more facilitators of learning. On the other hand, traditional F2F classes are structured in such a way that the instructor delivers knowledge, is better able to gauge understanding and interest of students, can engage in class activities, and can provide immediate feedback on clarifying questions during the class. Additionally, the designing of traditional F2F courses can be less time consuming for instructors compared to online courses (Navarro, 2000 ).
Online learning is also particularly suited for nontraditional students who require flexibility due to work or family commitments that are not usually associated with the undergraduate student population (Arias et al., 2018 ). Initially the nontraditional student belonged to the older adult age group, but with blended learning becoming more commonplace in high schools, colleges and universities, online learning has begun to traverse a wider range of age groups. However, traditional F2F classes are still more beneficial for learners that are not so self‐sufficient and lack discipline in working through the class material in the required time frame (Arias et al., 2018 ).
For the purpose of this literature review, both pure online and blended learning are considered to be online learning because much of the evidence in the literature compares these two types against the traditional F2F learning. The debate in the literature surrounding online learning versus F2F teaching continues to be a contentious one. A review of the literature reveals mixed findings when comparing the efficacy of online learning on student performance in relation to the traditional F2F medium of instruction (Lundberg et al., 2008 ; Nguyen, 2015 ). A number of studies conducted Before the 2000s find what is known today in the empirical literature as the “No Significant Difference” phenomenon (Russell & International Distance Education Certificate Center (IDECC), 1999 ). The seminal work from Russell and IDECC ( 1999 ) involved over 350 comparative studies on online/distance learning versus F2F learning, dating back to 1928. The author finds no significant difference overall between online and traditional F2F classroom education outcomes. Subsequent studies that followed find similar “no significant difference” outcomes (Arbaugh, 2000 ; Fallah & Ubell, 2000 ; Freeman & Capper, 1999 ; Johnson et al., 2000 ; Neuhauser, 2002 ). While Bernard et al. ( 2004 ) also find that overall there is no significant difference in achievement between online education and F2F education, the study does find significant heterogeneity in student performance for different activities. The findings show that students in F2F classes outperform the students participating in synchronous online classes (i.e. classes that require online students to participate in live sessions at specific times). However, asynchronous online classes (i.e. students access class materials at their own time online) outperform F2F classes.
More recent studies find significant results for online learning outcomes in relation to F2F outcomes. On the one hand, Shachar and Yoram ( 2003 ) and Shachar and Neumann ( 2010 ) conduct a meta‐analysis of studies from 1990 to 2009 and find that in 70% of the cases, students taking courses by online education outperformed students in traditionally instructed courses (i.e. F2F lectures). In addition, Navarro and Shoemaker ( 2000 ) observe that learning outcomes for online learners are as effective as or better than outcomes for F2F learners, regardless of background characteristics. In a study on computer science students, Dutton et al. ( 2002 ) find online students perform significantly better compared to the students who take the same course on campus. A meta‐analysis conducted by the US Department of Education finds that students who took all or part of their course online performed better, on average, than those taking the same course through traditional F2F instructions. The report also finds that the effect sizes are larger for studies in which the online learning was collaborative or instructor‐driven than in those studies where online learners worked independently (Means et al., 2010 ).
On the other hand, evidence by Brown and Liedholm ( 2002 ) based on test scores from macroeconomics students in the United States suggest that F2F students tend to outperform online students. These findings are supported by Coates et al. ( 2004 ) who base their study on macroeconomics students in the United States, and Xu and Jaggars ( 2014 ) who find negative effects for online students using a data set of about 500,000 courses taken by over 40,000 students in Washington. Furthermore, Almatra et al. ( 2015 ) compare overall course grades between online and F2F students for a Telecommunications course and find that F2F students significantly outperform online learning students. In an experimental study where students are randomly assigned to attend live lectures versus watching the same lectures online, Figlio et al. ( 2013 ) observe some evidence that the traditional format has a positive effect compared to online format. Interestingly, Callister and Love ( 2016 ) specifically compare the learning outcomes of online versus F2F skills‐based courses and find that F2F learners earned better outcomes than online learners even when using the same technology. This study highlights that some of the inconsistencies that we find in the results comparing online to F2F learning might be influenced by the nature of the course: theory‐based courses might be less impacted by in‐person interaction than skills‐based courses.
The fact that the reviewed studies on the effects of F2F versus online learning on student performance have been mainly focused in developed countries indicates the dearth of similar studies being conducted in developing countries. This gap in the literature may also highlight a salient point: online learning is still relatively underexplored in developing countries. The lockdown in South Africa therefore provides us with an opportunity to contribute to the existing literature from a developing country context.
2. CONTEXT OF STUDY
South Africa went into national lockdown in March 2020 due to the Covid‐19 pandemic. Like most universities in the country, the first semester for undergraduate courses at the University of Pretoria had already been running since the start of the academic year in February. Before the pandemic, a number of F2F lectures and assessments had already been conducted in most courses. The nationwide lockdown forced the university, which was mainly in‐person teaching, to move to full online learning for the remainder of the semester. This forced shift from F2F teaching to online learning allows us to investigate the changes in students' performance.
Before lockdown, classes were conducted on campus. During lockdown, these live classes were moved to an online platform, Blackboard Collaborate, which could be accessed by all registered students on the university intranet (“ClickUP”). However, these live online lectures involve substantial internet data costs for students. To ensure access to course content for those students who were unable to attend the live online lectures due to poor internet connections or internet data costs, several options for accessing course content were made available. These options included prerecorded narrated slides (which required less usage of internet data), recordings of the live online lectures, PowerPoint slides with explanatory notes and standard PDF lecture slides.
At the same time, the university managed to procure and loan out laptops to a number of disadvantaged students, and negotiated with major mobile internet data providers in the country for students to have free access to study material through the university's “connect” website (also referred to as the zero‐rated website). However, this free access excluded some video content and live online lectures (see Table 1 ). The university also provided between 10 and 20 gigabytes of mobile internet data per month, depending on the network provider, sent to students' mobile phones to assist with internet data costs.
Sites available on zero‐rated website
Note : The table summarizes the sites that were available on the zero‐rated website and those that incurred data costs.
High data costs continue to be a contentious issue in Africa where average incomes are low. Gilbert ( 2019 ) reports that South Africa ranked 16th of the 45 countries researched in terms of the most expensive internet data in Africa, at US$6.81 per gigabyte, in comparison to other Southern African countries such as Mozambique (US$1.97), Zambia (US$2.70), and Lesotho (US$4.09). Internet data prices have also been called into question in South Africa after the Competition Commission published a report from its Data Services Market Inquiry calling the country's internet data pricing “excessive” (Gilbert, 2019 ).
3. EMPIRICAL APPROACH
We use a sample of 395 s‐year students taking a macroeconomics module in the Economics department to compare the effects of F2F and online learning on students' performance using a range of assessments. The module was an introduction to the application of theoretical economic concepts. The content was both theory‐based (developing economic growth models using concepts and equations) and skill‐based (application involving the collection of data from online data sources and analyzing the data using statistical software). Both individual and group assignments formed part of the assessments. Before the end of the semester, during lockdown in June 2020, we asked the students to complete a survey with questions related to the transition from F2F to online learning and the difficulties that they may have faced. For example, we asked the students: (i) how easy or difficult they found the transition from F2F to online lectures; (ii) what internet options were available to them and which they used the most to access the online prescribed work; (iii) what format of content they accessed and which they preferred the most (i.e. self‐study material in the form of PDF and PowerPoint slides with notes vs. assisted study with narrated slides and lecture recordings); (iv) what difficulties they faced accessing the live online lectures, to name a few. Figure 1 summarizes the key survey questions that we asked the students regarding their transition from F2F to online learning.

Summary of survey data
Before the lockdown, the students had already attended several F2F classes and completed three assessments. We are therefore able to create a dependent variable that is comprised of the average grades of three assignments taken before lockdown and the average grades of three assignments taken after the start of the lockdown for each student. Specifically, we use the difference between the post‐ and pre‐lockdown average grades as the dependent variable. However, the number of student observations dropped to 275 due to some students missing one or more of the assessments. The lecturer, content and format of the assessments remain similar across the module. We estimate the following equation using ordinary least squares (OLS) with robust standard errors:
where Y i is the student's performance measured by the difference between the post and pre‐lockdown average grades. B represents the vector of determinants that measure the difficulty faced by students to transition from F2F to online learning. This vector includes access to the internet, study material preferred, quality of the online live lecture sessions and pre‐lockdown class attendance. X is the vector of student demographic controls such as race, gender and an indicator if the student's perceived family income is below average. The ε i is unobserved student characteristics.
4. ANALYSIS
4.1. descriptive statistics.
Table 2 gives an overview of the sample of students. We find that among the black students, a higher proportion of students reported finding the transition to online learning more difficult. On the other hand, more white students reported finding the transition moderately easy, as did the other races. According to Coetzee ( 2014 ), the quality of schools can vary significantly between higher income and lower‐income areas, with black South Africans far more likely to live in lower‐income areas with lower quality schools than white South Africans. As such, these differences in quality of education from secondary schooling can persist at tertiary level. Furthermore, persistent income inequality between races in South Africa likely means that many poorer black students might not be able to afford wifi connections or large internet data bundles which can make the transition difficult for black students compared to their white counterparts.
Descriptive statistics
Notes : The transition difficulty variable was ordered 1: Very Easy; 2: Moderately Easy; 3: Difficult; and 4: Impossible. Since we have few responses to the extremes, we combined Very Easy and Moderately as well as Difficult and Impossible to make the table easier to read. The table with a full breakdown is available upon request.
A higher proportion of students reported that wifi access made the transition to online learning moderately easy. However, relatively more students reported that mobile internet data and accessing the zero‐rated website made the transition difficult. Surprisingly, not many students made use of the zero‐rated website which was freely available. Figure 2 shows that students who reported difficulty transitioning to online learning did not perform as well in online learning versus F2F when compared to those that found it less difficult to transition.

Transition from F2F to online learning.
Notes : This graph shows the students' responses to the question “How easy did you find the transition from face‐to‐face lectures to online lectures?” in relation to the outcome variable for performance
In Figure 3 , the kernel density shows that students who had access to wifi performed better than those who used mobile internet data or the zero‐rated data.

Access to online learning.
Notes : This graph shows the students' responses to the question “What do you currently use the most to access most of your prescribed work?” in relation to the outcome variable for performance
The regression results are reported in Table 3 . We find that the change in students' performance from F2F to online is negatively associated with the difficulty they faced in transitioning from F2F to online learning. According to student survey responses, factors contributing to difficulty in transitioning included poor internet access, high internet data costs and lack of equipment such as laptops or tablets to access the study materials on the university website. Students who had access to wifi (i.e. fixed wireless broadband, Asymmetric Digital Subscriber Line (ADSL) or optic fiber) performed significantly better, with on average 4.5 points higher grade, in relation to students that had to use mobile internet data (i.e. personal mobile internet data, wifi at home using mobile internet data, or hotspot using mobile internet data) or the zero‐rated website to access the study materials. The insignificant results for the zero‐rated website are surprising given that the website was freely available and did not incur any internet data costs. However, most students in this sample complained that the internet connection on the zero‐rated website was slow, especially in uploading assignments. They also complained about being disconnected when they were in the middle of an assessment. This may have discouraged some students from making use of the zero‐rated website.
Results: Predictors for student performance using the difference on average assessment grades between pre‐ and post‐lockdown
Coefficients reported. Robust standard errors in parentheses.
∗∗∗ p < .01.
Students who expressed a preference for self‐study approaches (i.e. reading PDF slides or PowerPoint slides with explanatory notes) did not perform as well, on average, as students who preferred assisted study (i.e. listening to recorded narrated slides or lecture recordings). This result is in line with Means et al. ( 2010 ), where student performance was better for online learning that was collaborative or instructor‐driven than in cases where online learners worked independently. Interestingly, we also observe that the performance of students who often attended in‐person classes before the lockdown decreased. Perhaps these students found the F2F lectures particularly helpful in mastering the course material. From the survey responses, we find that a significant proportion of the students (about 70%) preferred F2F to online lectures. This preference for F2F lectures may also be linked to the factors contributing to the difficulty some students faced in transitioning to online learning.
We find that the performance of low‐income students decreased post‐lockdown, which highlights another potential challenge to transitioning to online learning. The picture and sound quality of the live online lectures also contributed to lower performance. Although this result is not statistically significant, it is worth noting as the implications are linked to the quality of infrastructure currently available for students to access online learning. We find no significant effects of race on changes in students' performance, though males appeared to struggle more with the shift to online teaching than females.
For the robustness check in Table 4 , we consider the average grades of the three assignments taken after the start of the lockdown as a dependent variable (i.e. the post‐lockdown average grades for each student). We then include the pre‐lockdown average grades as an explanatory variable. The findings and overall conclusions in Table 4 are consistent with the previous results.
Robustness check: Predictors for student performance using the average assessment grades for post‐lockdown
As a further robustness check in Table 5 , we create a panel for each student across the six assignment grades so we can control for individual heterogeneity. We create a post‐lockdown binary variable that takes the value of 1 for the lockdown period and 0 otherwise. We interact the post‐lockdown dummy variable with a measure for transition difficulty and internet access. The internet access variable is an indicator variable for mobile internet data, wifi, or zero‐rated access to class materials. The variable wifi is a binary variable taking the value of 1 if the student has access to wifi and 0 otherwise. The zero‐rated variable is a binary variable taking the value of 1 if the student used the university's free portal access and 0 otherwise. We also include assignment and student fixed effects. The results in Table 5 remain consistent with our previous findings that students who had wifi access performed significantly better than their peers.
Interaction model
Notes : Coefficients reported. Robust standard errors in parentheses. The dependent variable is the assessment grades for each student on each assignment. The number of observations include the pre‐post number of assessments multiplied by the number of students.
6. CONCLUSION
The Covid‐19 pandemic left many education institutions with no option but to transition to online learning. The University of Pretoria was no exception. We examine the effect of transitioning to online learning on the academic performance of second‐year economic students. We use assessment results from F2F lectures before lockdown, and online lectures post lockdown for the same group of students, together with responses from survey questions. We find that the main contributor to lower academic performance in the online setting was poor internet access, which made transitioning to online learning more difficult. In addition, opting to self‐study (read notes instead of joining online classes and/or watching recordings) did not help the students in their performance.
The implications of the results highlight the need for improved quality of internet infrastructure with affordable internet data pricing. Despite the university's best efforts not to leave any student behind with the zero‐rated website and free monthly internet data, the inequality dynamics in the country are such that invariably some students were negatively affected by this transition, not because the student was struggling academically, but because of inaccessibility of internet (wifi). While the zero‐rated website is a good collaborative initiative between universities and network providers, the infrastructure is not sufficient to accommodate mass students accessing it simultaneously.
This study's findings may highlight some shortcomings in the academic sector that need to be addressed by both the public and private sectors. There is potential for an increase in the digital divide gap resulting from the inequitable distribution of digital infrastructure. This may lead to reinforcement of current inequalities in accessing higher education in the long term. To prepare the country for online learning, some considerations might need to be made to make internet data tariffs more affordable and internet accessible to all. We hope that this study's findings will provide a platform (or will at least start the conversation for taking remedial action) for policy engagements in this regard.
We are aware of some limitations presented by our study. The sample we have at hand makes it difficult to extrapolate our findings to either all students at the University of Pretoria or other higher education students in South Africa. Despite this limitation, our findings highlight the negative effect of the digital divide on students' educational outcomes in the country. The transition to online learning and the high internet data costs in South Africa can also have adverse learning outcomes for low‐income students. With higher education institutions, such as the University of Pretoria, integrating online teaching to overcome the effect of the Covid‐19 pandemic, access to stable internet is vital for students' academic success.
It is also important to note that the data we have at hand does not allow us to isolate wifi's causal effect on students' performance post‐lockdown due to two main reasons. First, wifi access is not randomly assigned; for instance, there is a high chance that students with better‐off family backgrounds might have better access to wifi and other supplementary infrastructure than their poor counterparts. Second, due to the university's data access policy and consent, we could not merge the data at hand with the student's previous year's performance. Therefore, future research might involve examining the importance of these elements to document the causal impact of access to wifi on students' educational outcomes in the country.
ACKNOWLEDGMENT
The authors acknowledge the helpful comments received from the editor, the anonymous reviewers, and Elizabeth Asiedu.
Chisadza, C. , Clance, M. , Mthembu, T. , Nicholls, N. , & Yitbarek, E. (2021). Online and face‐to‐face learning: Evidence from students’ performance during the Covid‐19 pandemic . Afr Dev Rev , 33 , S114–S125. 10.1111/afdr.12520 [ CrossRef ] [ Google Scholar ]
1 https://mybroadband.co.za/news/cellular/309693-mobile-data-prices-south-africa-vs-the-world.html .
2 The 4IR is currently characterized by increased use of new technologies, such as advanced wireless technologies, artificial intelligence, cloud computing, robotics, among others. This era has also facilitated the use of different online learning platforms ( https://www.brookings.edu/research/the-fourth-industrialrevolution-and-digitization-will-transform-africa-into-a-global-powerhouse/ ).
3 Note that we control for income, but it is plausible to assume other unobservable factors such as parental preference and parenting style might also affect access to the internet of students.
- Almatra, O. , Johri, A. , Nagappan, K. , & Modanlu, A. (2015). An empirical study of face‐to‐face and distance learning sections of a core telecommunication course (Conference Proceedings Paper No. 12944). 122nd ASEE Annual Conference and Exposition, Seattle, Washington State.
- Anyanwu, J. C. (2013). Characteristics and macroeconomic determinants of youth employment in Africa . African Development Review , 25 ( 2 ), 107–129. [ Google Scholar ]
- Anyanwu, J. C. (2016). Accounting for gender equality in secondary school enrolment in Africa: Accounting for gender equality in secondary school enrolment . African Development Review , 28 ( 2 ), 170–191. [ Google Scholar ]
- Arbaugh, J. (2000). Virtual classroom versus physical classroom: An exploratory study of class discussion patterns and student learning in an asynchronous internet‐based MBA course . Journal of Management Education , 24 ( 2 ), 213–233. [ Google Scholar ]
- Arias, J. J. , Swinton, J. , & Anderson, K. (2018). On‐line vs. face‐to‐face: A comparison of student outcomes with random assignment . e‐Journal of Business Education and Scholarship of Teaching, , 12 ( 2 ), 1–23. [ Google Scholar ]
- Bernard, R. M. , Abrami, P. C. , Lou, Y. , Borokhovski, E. , Wade, A. , Wozney, L. , Wallet, P. A. , Fiset, M. , & Huang, B. (2004). How does distance education compare with classroom instruction? A meta‐analysis of the empirical literature . Review of Educational Research , 74 ( 3 ), 379–439. [ Google Scholar ]
- Brown, B. , & Liedholm, C. (2002). Can web courses replace the classroom in principles of microeconomics? American Economic Review , 92 ( 2 ), 444–448. [ Google Scholar ]
- Callister, R. R. , & Love, M. S. (2016). A comparison of learning outcomes in skills‐based courses: Online versus face‐to‐face formats . Decision Sciences Journal of Innovative Education , 14 ( 2 ), 243–256. [ Google Scholar ]
- Coates, D. , Humphreys, B. R. , Kane, J. , & Vachris, M. A. (2004). “No significant distance” between face‐to‐face and online instruction: Evidence from principles of economics . Economics of Education Review , 23 ( 5 ), 533–546. [ Google Scholar ]
- Coetzee, M. (2014). School quality and the performance of disadvantaged learners in South Africa (Working Paper No. 22). University of Stellenbosch Economics Department, Stellenbosch
- Dutton, J. , Dutton, M. , & Perry, J. (2002). How do online students differ from lecture students? Journal of Asynchronous Learning Networks , 6 ( 1 ), 1–20. [ Google Scholar ]
- Emediegwu, L. (2021). Does educational investment enhance capacity development for Nigerian youths? An autoregressive distributed lag approach . African Development Review , 32 ( S1 ), S45–S53. [ Google Scholar ]
- Fallah, M. H. , & Ubell, R. (2000). Blind scores in a graduate test. Conventional compared with web‐based outcomes . ALN Magazine , 4 ( 2 ). [ Google Scholar ]
- Figlio, D. , Rush, M. , & Yin, L. (2013). Is it live or is it internet? Experimental estimates of the effects of online instruction on student learning . Journal of Labor Economics , 31 ( 4 ), 763–784. [ Google Scholar ]
- Freeman, M. A. , & Capper, J. M. (1999). Exploiting the web for education: An anonymous asynchronous role simulation . Australasian Journal of Educational Technology , 15 ( 1 ), 95–116. [ Google Scholar ]
- Gilbert, P. (2019). The most expensive data prices in Africa . Connecting Africa. https://www.connectingafrica.com/author.asp?section_id=761%26doc_id=756372
- Glick, P. , Randriamamonjy, J. , & Sahn, D. (2009). Determinants of HIV knowledge and condom use among women in Madagascar: An analysis using matched household and community data . African Development Review , 21 ( 1 ), 147–179. [ Google Scholar ]
- Gyimah‐Brempong, K. (2011). Education and economic development in Africa . African Development Review , 23 ( 2 ), 219–236. [ Google Scholar ]
- Johnson, S. , Aragon, S. , Shaik, N. , & Palma‐Rivas, N. (2000). Comparative analysis of learner satisfaction and learning outcomes in online and face‐to‐face learning environments . Journal of Interactive Learning Research , 11 ( 1 ), 29–49. [ Google Scholar ]
- Lundberg, J. , Merino, D. , & Dahmani, M. (2008). Do online students perform better than face‐to‐face students? Reflections and a short review of some empirical findings . Revista de Universidad y Sociedad del Conocimiento , 5 ( 1 ), 35–44. [ Google Scholar ]
- Means, B. , Toyama, Y. , Murphy, R. , Bakia, M. , & Jones, K. (2010). Evaluation of evidence‐based practices in online learning: A meta‐analysis and review of online learning studies (Report No. ed‐04‐co‐0040 task 0006). U.S. Department of Education, Office of Planning, Evaluation, and Policy Development, Washington DC.
- Navarro, P. (2000). Economics in the cyber‐classroom . Journal of Economic Perspectives , 14 ( 2 ), 119–132. [ Google Scholar ]
- Navarro, P. , & Shoemaker, J. (2000). Performance and perceptions of distance learners in cyberspace . American Journal of Distance Education , 14 ( 2 ), 15–35. [ Google Scholar ]
- Neuhauser, C. (2002). Learning style and effectiveness of online and face‐to‐face instruction . American Journal of Distance Education , 16 ( 2 ), 99–113. [ Google Scholar ]
- Nguyen, T. (2015). The effectiveness of online learning: Beyond no significant difference and future horizons . MERLOT Journal of Online Teaching and Learning , 11 ( 2 ), 309–319. [ Google Scholar ]
- Paechter, M. , & Maier, B. (2010). Online or face‐to‐face? Students' experiences and preferences in e‐learning . Internet and Higher Education , 13 ( 4 ), 292–297. [ Google Scholar ]
- Russell, T. L. , & International Distance Education Certificate Center (IDECC) (1999). The no significant difference phenomenon: A comparative research annotated bibliography on technology for distance education: As reported in 355 research reports, summaries and papers . North Carolina State University. [ Google Scholar ]
- Shachar, M. , & Neumann, Y. (2010). Twenty years of research on the academic performance differences between traditional and distance learning: Summative meta‐analysis and trend examination . MERLOT Journal of Online Learning and Teaching , 6 ( 2 ), 318–334. [ Google Scholar ]
- Shachar, M. , & Yoram, N. (2003). Differences between traditional and distance education academic performances: A meta‐analytic approach . International Review of Research in Open and Distance Learning , 4 ( 2 ), 1–20. [ Google Scholar ]
- Tchamyou, V. S. , Asongu, S. , & Odhiambo, N. (2019). The role of ICT in modulating the effect of education and lifelong learning on income inequality and economic growth in Africa . African Development Review , 31 ( 3 ), 261–274. [ Google Scholar ]
- Xu, D. , & Jaggars, S. S. (2014). Performance gaps between online and face‐to‐face courses: Differences across types of students and academic subject areas . The Journal of Higher Education , 85 ( 5 ), 633–659. [ Google Scholar ]
Log in using your username and password
- Search More Search for this keyword Advanced search
- Latest content
- Current issue
- Browse by collection
- BMJ Journals More You are viewing from: Google Indexer
You are here
- Online First
- The role of COVID-19 vaccines in preventing post-COVID-19 thromboembolic and cardiovascular complications
- Article Text
- Article info
- Citation Tools
- Rapid Responses
- Article metrics

- Núria Mercadé-Besora 1 , 2 , 3 ,
- Xintong Li 1 ,
- Raivo Kolde 4 ,
- Nhung TH Trinh 5 ,
- Maria T Sanchez-Santos 1 ,
- Wai Yi Man 1 ,
- Elena Roel 3 ,
- Carlen Reyes 3 ,
- http://orcid.org/0000-0003-0388-3403 Antonella Delmestri 1 ,
- Hedvig M E Nordeng 6 , 7 ,
- http://orcid.org/0000-0002-4036-3856 Anneli Uusküla 8 ,
- http://orcid.org/0000-0002-8274-0357 Talita Duarte-Salles 3 , 9 ,
- Clara Prats 2 ,
- http://orcid.org/0000-0002-3950-6346 Daniel Prieto-Alhambra 1 , 9 ,
- http://orcid.org/0000-0002-0000-0110 Annika M Jödicke 1 ,
- Martí Català 1
- 1 Pharmaco- and Device Epidemiology Group, Health Data Sciences, Botnar Research Centre, NDORMS , University of Oxford , Oxford , UK
- 2 Department of Physics , Universitat Politècnica de Catalunya , Barcelona , Spain
- 3 Fundació Institut Universitari per a la recerca a l'Atenció Primària de Salut Jordi Gol i Gurina (IDIAPJGol) , IDIAP Jordi Gol , Barcelona , Catalunya , Spain
- 4 Institute of Computer Science , University of Tartu , Tartu , Estonia
- 5 Pharmacoepidemiology and Drug Safety Research Group, Department of Pharmacy, Faculty of Mathematics and Natural Sciences , University of Oslo , Oslo , Norway
- 6 School of Pharmacy , University of Oslo , Oslo , Norway
- 7 Division of Mental Health , Norwegian Institute of Public Health , Oslo , Norway
- 8 Department of Family Medicine and Public Health , University of Tartu , Tartu , Estonia
- 9 Department of Medical Informatics, Erasmus University Medical Center , Erasmus University Rotterdam , Rotterdam , Zuid-Holland , Netherlands
- Correspondence to Prof Daniel Prieto-Alhambra, Pharmaco- and Device Epidemiology Group, Health Data Sciences, Botnar Research Centre, NDORMS, University of Oxford, Oxford, UK; daniel.prietoalhambra{at}ndorms.ox.ac.uk
Objective To study the association between COVID-19 vaccination and the risk of post-COVID-19 cardiac and thromboembolic complications.
Methods We conducted a staggered cohort study based on national vaccination campaigns using electronic health records from the UK, Spain and Estonia. Vaccine rollout was grouped into four stages with predefined enrolment periods. Each stage included all individuals eligible for vaccination, with no previous SARS-CoV-2 infection or COVID-19 vaccine at the start date. Vaccination status was used as a time-varying exposure. Outcomes included heart failure (HF), venous thromboembolism (VTE) and arterial thrombosis/thromboembolism (ATE) recorded in four time windows after SARS-CoV-2 infection: 0–30, 31–90, 91–180 and 181–365 days. Propensity score overlap weighting and empirical calibration were used to minimise observed and unobserved confounding, respectively.
Fine-Gray models estimated subdistribution hazard ratios (sHR). Random effect meta-analyses were conducted across staggered cohorts and databases.
Results The study included 10.17 million vaccinated and 10.39 million unvaccinated people. Vaccination was associated with reduced risks of acute (30-day) and post-acute COVID-19 VTE, ATE and HF: for example, meta-analytic sHR of 0.22 (95% CI 0.17 to 0.29), 0.53 (0.44 to 0.63) and 0.45 (0.38 to 0.53), respectively, for 0–30 days after SARS-CoV-2 infection, while in the 91–180 days sHR were 0.53 (0.40 to 0.70), 0.72 (0.58 to 0.88) and 0.61 (0.51 to 0.73), respectively.
Conclusions COVID-19 vaccination reduced the risk of post-COVID-19 cardiac and thromboembolic outcomes. These effects were more pronounced for acute COVID-19 outcomes, consistent with known reductions in disease severity following breakthrough versus unvaccinated SARS-CoV-2 infection.
- Epidemiology
- PUBLIC HEALTH
- Electronic Health Records
Data availability statement
Data may be obtained from a third party and are not publicly available. CPRD: CPRD data were obtained under the CPRD multi-study license held by the University of Oxford after Research Data Governance (RDG) approval. Direct data sharing is not allowed. SIDIAP: In accordance with current European and national law, the data used in this study is only available for the researchers participating in this study. Thus, we are not allowed to distribute or make publicly available the data to other parties. However, researchers from public institutions can request data from SIDIAP if they comply with certain requirements. Further information is available online ( https://www.sidiap.org/index.php/menu-solicitudesen/application-proccedure ) or by contacting SIDIAP ([email protected]). CORIVA: CORIVA data were obtained under the approval of Research Ethics Committee of the University of Tartu and the patient level data sharing is not allowed. All analyses in this study were conducted in a federated manner, where analytical code and aggregated (anonymised) results were shared, but no patient-level data was transferred across the collaborating institutions.
This is an open access article distributed in accordance with the Creative Commons Attribution 4.0 Unported (CC BY 4.0) license, which permits others to copy, redistribute, remix, transform and build upon this work for any purpose, provided the original work is properly cited, a link to the licence is given, and indication of whether changes were made. See: https://creativecommons.org/licenses/by/4.0/ .
https://doi.org/10.1136/heartjnl-2023-323483
Statistics from Altmetric.com
Request permissions.
If you wish to reuse any or all of this article please use the link below which will take you to the Copyright Clearance Center’s RightsLink service. You will be able to get a quick price and instant permission to reuse the content in many different ways.
WHAT IS ALREADY KNOWN ON THIS TOPIC
COVID-19 vaccines proved to be highly effective in reducing the severity of acute SARS-CoV-2 infection.
While COVID-19 vaccines were associated with increased risk for cardiac and thromboembolic events, such as myocarditis and thrombosis, the risk of complications was substantially higher due to SARS-CoV-2 infection.
WHAT THIS STUDY ADDS
COVID-19 vaccination reduced the risk of heart failure, venous thromboembolism and arterial thrombosis/thromboembolism in the acute (30 days) and post-acute (31 to 365 days) phase following SARS-CoV-2 infection. This effect was stronger in the acute phase.
The overall additive effect of vaccination on the risk of post-vaccine and/or post-COVID thromboembolic and cardiac events needs further research.
HOW THIS STUDY MIGHT AFFECT RESEARCH, PRACTICE OR POLICY
COVID-19 vaccines proved to be highly effective in reducing the risk of post-COVID cardiovascular and thromboembolic complications.
Introduction
COVID-19 vaccines were approved under emergency authorisation in December 2020 and showed high effectiveness against SARS-CoV-2 infection, COVID-19-related hospitalisation and death. 1 2 However, concerns were raised after spontaneous reports of unusual thromboembolic events following adenovirus-based COVID-19 vaccines, an association that was further assessed in observational studies. 3 4 More recently, mRNA-based vaccines were found to be associated with a risk of rare myocarditis events. 5 6
On the other hand, SARS-CoV-2 infection can trigger cardiac and thromboembolic complications. 7 8 Previous studies showed that, while slowly decreasing over time, the risk for serious complications remain high for up to a year after infection. 9 10 Although acute and post-acute cardiac and thromboembolic complications following COVID-19 are rare, they present a substantial burden to the affected patients, and the absolute number of cases globally could become substantial.
Recent studies suggest that COVID-19 vaccination could protect against cardiac and thromboembolic complications attributable to COVID-19. 11 12 However, most studies did not include long-term complications and were conducted among specific populations.
Evidence is still scarce as to whether the combined effects of COVID-19 vaccines protecting against SARS-CoV-2 infection and reducing post-COVID-19 cardiac and thromboembolic outcomes, outweigh any risks of these complications potentially associated with vaccination.
We therefore used large, representative data sources from three European countries to assess the overall effect of COVID-19 vaccines on the risk of acute and post-acute COVID-19 complications including venous thromboembolism (VTE), arterial thrombosis/thromboembolism (ATE) and other cardiac events. Additionally, we studied the comparative effects of ChAdOx1 versus BNT162b2 on the risk of these same outcomes.
Data sources
We used four routinely collected population-based healthcare datasets from three European countries: the UK, Spain and Estonia.
For the UK, we used data from two primary care databases—namely, Clinical Practice Research Datalink, CPRD Aurum 13 and CPRD Gold. 14 CPRD Aurum currently covers 13 million people from predominantly English practices, while CPRD Gold comprises 3.1 million active participants mostly from GP practices in Wales and Scotland. Spanish data were provided by the Information System for the Development of Research in Primary Care (SIDIAP), 15 which encompasses primary care records from 6 million active patients (around 75% of the population in the region of Catalonia) linked to hospital admissions data (Conjunt Mínim Bàsic de Dades d’Alta Hospitalària). Finally, the CORIVA dataset based on national health claims data from Estonia was used. It contains all COVID-19 cases from the first year of the pandemic and ~440 000 randomly selected controls. CORIVA was linked to the death registry and all COVID-19 testing from the national health information system.
Databases included sociodemographic information, diagnoses, measurements, prescriptions and secondary care referrals and were linked to vaccine registries, including records of all administered vaccines from all healthcare settings. Data availability for CPRD Gold ended in December 2021, CPRD Aurum in January 2022, SIDIAP in June 2022 and CORIVA in December 2022.
All databases were mapped to the Observational Medical Outcomes Partnership Common Data Model (OMOP CDM) 16 to facilitate federated analytics.
Multinational network staggered cohort study: study design and participants
The study design has been published in detail elsewhere. 17 Briefly, we used a staggered cohort design considering vaccination as a time-varying exposure. Four staggered cohorts were designed with each cohort representing a country-specific vaccination rollout phase (eg, dates when people became eligible for vaccination, and eligibility criteria).
The source population comprised all adults registered in the respective database for at least 180 days at the start of the study (4 January 2021 for CPRD Gold and Aurum, 20 February 2021 for SIDIAP and 28 January 2021 for CORIVA). Subsequently, each staggered cohort corresponded to an enrolment period: all people eligible for vaccination during this time were included in the cohort and people with a history of SARS-CoV-2 infection or COVID-19 vaccination before the start of the enrolment period were excluded. Across countries, cohort 1 comprised older age groups, whereas cohort 2 comprised individuals at risk for severe COVID-19. Cohort 3 included people aged ≥40 and cohort 4 enrolled people aged ≥18.
In each cohort, people receiving a first vaccine dose during the enrolment period were allocated to the vaccinated group, with their index date being the date of vaccination. Individuals who did not receive a vaccine dose comprised the unvaccinated group and their index date was assigned within the enrolment period, based on the distribution of index dates in the vaccinated group. People with COVID-19 before the index date were excluded.
Follow-up started from the index date until the earliest of end of available data, death, change in exposure status (first vaccine dose for those unvaccinated) or outcome of interest.
COVID-19 vaccination
All vaccines approved within the study period from January 2021 to July 2021—namely, ChAdOx1 (Oxford/AstraZeneca), BNT162b2 (BioNTech/Pfizer]) Ad26.COV2.S (Janssen) and mRNA-1273 (Moderna), were included for this study.
Post-COVID-19 outcomes of interest
Outcomes of interest were defined as SARS-CoV-2 infection followed by a predefined thromboembolic or cardiac event of interest within a year after infection, and with no record of the same clinical event in the 6 months before COVID-19. Outcome date was set as the corresponding SARS-CoV-2 infection date.
COVID-19 was identified from either a positive SARS-CoV-2 test (polymerase chain reaction (PCR) or antigen), or a clinical COVID-19 diagnosis, with no record of COVID-19 in the previous 6 weeks. This wash-out period was imposed to exclude re-recordings of the same COVID-19 episode.
Post-COVID-19 outcome events were selected based on previous studies. 11–13 Events comprised ischaemic stroke (IS), haemorrhagic stroke (HS), transient ischaemic attack (TIA), ventricular arrhythmia/cardiac arrest (VACA), myocarditis/pericarditis (MP), myocardial infarction (MI), heart failure (HF), pulmonary embolism (PE) and deep vein thrombosis (DVT). We used two composite outcomes: (1) VTE, as an aggregate of PE and DVT and (2) ATE, as a composite of IS, TIA and MI. To avoid re-recording of the same complication we imposed a wash-out period of 90 days between records. Phenotypes for these complications were based on previously published studies. 3 4 8 18
All outcomes were ascertained in four different time periods following SARS-CoV-2 infection: the first period described the acute infection phase—that is, 0–30 days after COVID-19, whereas the later periods - which are 31–90 days, 91–180 days and 181–365 days, illustrate the post-acute phase ( figure 1 ).
- Download figure
- Open in new tab
- Download powerpoint
Study outcome design. Study outcomes of interest are defined as a COVID-19 infection followed by one of the complications in the figure, within a year after infection. Outcomes were ascertained in four different time windows after SARS-CoV-2 infection: 0–30 days (namely the acute phase), 31–90 days, 91–180 days and 181–365 days (these last three comprise the post-acute phase).
Negative control outcomes
Negative control outcomes (NCOs) were used to detect residual confounding. NCOs are outcomes which are not believed to be causally associated with the exposure, but share the same bias structure with the exposure and outcome of interest. Therefore, no significant association between exposure and NCO is to be expected. Our study used 43 different NCOs from previous work assessing vaccine effectiveness. 19
Statistical analysis
Federated network analyses.
A template for an analytical script was developed and subsequently tailored to include the country-specific aspects (eg, dates, priority groups) for the vaccination rollout. Analyses were conducted locally for each database. Only aggregated data were shared and person counts <5 were clouded.
Propensity score weighting
Large-scale propensity scores (PS) were calculated to estimate the likelihood of a person receiving the vaccine based on their demographic and health-related characteristics (eg, conditions, medications) prior to the index date. PS were then used to minimise observed confounding by creating a weighted population (overlap weighting 20 ), in which individuals contributed with a different weight based on their PS and vaccination status.
Prespecified key variables included in the PS comprised age, sex, location, index date, prior observation time in the database, number of previous outpatient visits and previous SARS-CoV-2 PCR/antigen tests. Regional vaccination, testing and COVID-19 incidence rates were also forced into the PS equation for the UK databases 21 and SIDIAP. 22 In addition, least absolute shrinkage and selection operator (LASSO) regression, a technique for variable selection, was used to identify additional variables from all recorded conditions and prescriptions within 0–30 days, 31–180 days and 181-any time (conditions only) before the index date that had a prevalence of >0.5% in the study population.
PS were then separately estimated for each staggered cohort and analysis. We considered covariate balance to be achieved if absolute standardised mean differences (ASMDs) were ≤0.1 after weighting. Baseline characteristics such as demographics and comorbidities were reported.
Effect estimation
To account for the competing risk of death associated with COVID-19, Fine-and-Grey models 23 were used to calculate subdistribution hazard ratios (sHRs). Subsequently, sHRs and confidence intervals were empirically calibrated from NCO estimates 24 to account for unmeasured confounding. To calibrate the estimates, the empirical null distribution was derived from NCO estimates and was used to compute calibrated confidence intervals. For each outcome, sHRs from the four staggered cohorts were pooled using random-effect meta-analysis, both separately for each database and across all four databases.
Sensitivity analysis
Sensitivity analyses comprised 1) censoring follow-up for vaccinated people at the time when they received their second vaccine dose and 2) considering only the first post-COVID-19 outcome within the year after infection ( online supplemental figure S1 ). In addition, comparative effectiveness analyses were conducted for BNT162b2 versus ChAdOx1.
Supplemental material
Data and code availability.
All analytic code for the study is available in GitHub ( https://github.com/oxford-pharmacoepi/vaccineEffectOnPostCovidCardiacThromboembolicEvents ), including code lists for vaccines, COVID-19 tests and diagnoses, cardiac and thromboembolic events, NCO and health conditions to prioritise patients for vaccination in each country. We used R version 4.2.3 and statistical packages survival (3.5–3), Empirical Calibration (3.1.1), glmnet (4.1-7), and Hmisc (5.0–1).
Patient and public involvement
Owing to the nature of the study and the limitations regarding data privacy, the study design, analysis, interpretation of data and revision of the manuscript did not involve any patients or members of the public.
All aggregated results are available in a web application ( https://dpa-pde-oxford.shinyapps.io/PostCovidComplications/ ).
We included over 10.17 million vaccinated individuals (1 618 395 from CPRD Gold; 5 729 800 from CPRD Aurum; 2 744 821 from SIDIAP and 77 603 from CORIVA) and 10.39 million unvaccinated individuals (1 640 371; 5 860 564; 2 588 518 and 302 267, respectively). Online supplemental figures S2-5 illustrate study inclusion for each database.
Adequate covariate balance was achieved after PS weighting in most studies: CORIVA (all cohorts) and SIDIAP (cohorts 1 and 4) did not contribute to ChAdOx1 subanalyses owing to sample size and covariate imbalance. ASMD results are accessible in the web application.
NCO analyses suggested residual bias after PS weighting, with a majority of NCOs associated positively with vaccination. Therefore, calibrated estimates are reported in this manuscript. Uncalibrated effect estimates and NCO analyses are available in the web interface.
Population characteristics
Table 1 presents baseline characteristics for the weighted populations in CPRD Aurum, for illustrative purposes. Online supplemental tables S1-25 summarise baseline characteristics for weighted and unweighted populations for each database and comparison. Across databases and cohorts, populations followed similar patterns: cohort 1 represented an older subpopulation (around 80 years old) with a high proportion of women (57%). Median age was lowest in cohort 4 ranging between 30 and 40 years.
- View inline
Characteristics of weighted populations in CPRD Aurum database, stratified by staggered cohort and exposure status. Exposure is any COVID-19 vaccine
COVID-19 vaccination and post-COVID-19 complications
Table 2 shows the incidence of post-COVID-19 VTE, ATE and HF, the three most common post-COVID-19 conditions among the studied outcomes. Outcome counts are presented separately for 0–30, 31–90, 91–180 and 181–365 days after SARS-CoV-2 infection. Online supplemental tables S26-36 include all studied complications, also for the sensitivity and subanalyses. Similar pattern for incidences were observed across all databases: higher outcome rates in the older populations (cohort 1) and decreasing frequency with increasing time after infection in all cohorts.
Number of records (and risk per 10 000 individuals) for acute and post-acute COVID-19 cardiac and thromboembolic complications, across cohorts and databases for any COVID-19 vaccination
Forest plots for the effect of COVID-19 vaccines on post-COVID-19 cardiac and thromboembolic complications; meta-analysis across cohorts and databases. Dashed line represents a level of heterogeneity I 2 >0.4. ATE, arterial thrombosis/thromboembolism; CD+HS, cardiac diseases and haemorrhagic stroke; VTE, venous thromboembolism.
Results from calibrated estimates pooled in meta-analysis across cohorts and databases are shown in figure 2 .
Reduced risk associated with vaccination is observed for acute and post-acute VTE, DVT, and PE: acute meta-analytic sHR are 0.22 (95% CI, 0.17–0.29); 0.36 (0.28–0.45); and 0.19 (0.15–0.25), respectively. For VTE in the post-acute phase, sHR estimates are 0.43 (0.34–0.53), 0.53 (0.40–0.70) and 0.50 (0.36–0.70) for 31–90, 91–180, and 181–365 days post COVID-19, respectively. Reduced risk of VTE outcomes was observed in vaccinated across databases and cohorts, see online supplemental figures S14–22 .
Similarly, the risk of ATE, IS and MI in the acute phase after infection was reduced for the vaccinated group, sHR of 0.53 (0.44–0.63), 0.55 (0.43–0.70) and 0.49 (0.38–0.62), respectively. Reduced risk associated with vaccination persisted for post-acute ATE, with sHR of 0.74 (0.60–0.92), 0.72 (0.58–0.88) and 0.62 (0.48–0.80) for 31–90, 91–180 and 181–365 days post-COVID-19, respectively. Risk of post-acute MI remained lower for vaccinated in the 31–90 and 91–180 days after COVID-19, with sHR of 0.64 (0.46–0.87) and 0.64 (0.45–0.90), respectively. Vaccination effect on post-COVID-19 TIA was seen only in the 181–365 days, with sHR of 0.51 (0.31–0.82). Online supplemental figures S23-31 show database-specific and cohort-specific estimates for ATE-related complications.
Risk of post-COVID-19 cardiac complications was reduced in vaccinated individuals. Meta-analytic estimates in the acute phase showed sHR of 0.45 (0.38–0.53) for HF, 0.41 (0.26–0.66) for MP and 0.41 (0.27–0.63) for VACA. Reduced risk persisted for post-acute COVID-19 HF: sHR 0.61 (0.51–0.73) for 31–90 days, 0.61 (0.51–0.73) for 91–180 days and 0.52 (0.43–0.63) for 181–365 days. For post-acute MP, risk was only lowered in the first post-acute window (31–90 days), with sHR of 0.43 (0.21–0.85). Vaccination showed no association with post-COVID-19 HS. Database-specific and cohort-specific results for these cardiac diseases are shown in online supplemental figures S32-40 .
Stratified analyses by vaccine showed similar associations, except for ChAdOx1 which was not associated with reduced VTE and ATE risk in the last post-acute window. Sensitivity analyses were consistent with main results ( online supplemental figures S6-13 ).
Figure 3 shows the results of comparative effects of BNT162b2 versus ChAdOx1, based on UK data. Meta-analytic estimates favoured BNT162b2 (sHR of 0.66 (0.46–0.93)) for VTE in the 0–30 days after infection, but no differences were seen for post-acute VTE or for any of the other outcomes. Results from sensitivity analyses, database-specific and cohort-specific estimates were in line with the main findings ( online supplemental figures S41-51 ).
Forest plots for comparative vaccine effect (BNT162b2 vs ChAdOx1); meta-analysis across cohorts and databases. ATE, arterial thrombosis/thromboembolism; CD+HS, cardiac diseases and haemorrhagic stroke; VTE, venous thromboembolism.
Key findings
Our analyses showed a substantial reduction of risk (45–81%) for thromboembolic and cardiac events in the acute phase of COVID-19 associated with vaccination. This finding was consistent across four databases and three different European countries. Risks for post-acute COVID-19 VTE, ATE and HF were reduced to a lesser extent (24–58%), whereas a reduced risk for post-COVID-19 MP and VACA in vaccinated people was seen only in the acute phase.
Results in context
The relationship between SARS-CoV-2 infection, COVID-19 vaccines and thromboembolic and/or cardiac complications is tangled. Some large studies report an increased risk of VTE and ATE following both ChAdOx1 and BNT162b2 vaccination, 7 whereas other studies have not identified such a risk. 25 Elevated risk of VTE has also been reported among patients with COVID-19 and its occurrence can lead to poor prognosis and mortality. 26 27 Similarly, several observational studies have found an association between COVID-19 mRNA vaccination and a short-term increased risk of myocarditis, particularly among younger male individuals. 5 6 For instance, a self-controlled case series study conducted in England revealed about 30% increased risk of hospital admission due to myocarditis within 28 days following both ChAdOx1 and BNT162b2 vaccines. However, this same study also found a ninefold higher risk for myocarditis following a positive SARS-CoV-2 test, clearly offsetting the observed post-vaccine risk.
COVID-19 vaccines have demonstrated high efficacy and effectiveness in preventing infection and reducing the severity of acute-phase infection. However, with the emergence of newer variants of the virus, such as omicron, and the waning protective effect of the vaccine over time, there is a growing interest in understanding whether the vaccine can also reduce the risk of complications after breakthrough infections. Recent studies suggested that COVID-19 vaccination could potentially protect against acute post-COVID-19 cardiac and thromboembolic events. 11 12 A large prospective cohort study 11 reports risk of VTE after SARS-CoV-2 infection to be substantially reduced in fully vaccinated ambulatory patients. Likewise, Al-Aly et al 12 suggest a reduced risk for post-acute COVID-19 conditions in breakthrough infection versus SARS-CoV-2 infection without prior vaccination. However, the populations were limited to SARS-CoV-2 infected individuals and estimates did not include the effect of the vaccine to prevent COVID-19 in the first place. Other studies on post-acute COVID-19 conditions and symptoms have been conducted, 28 29 but there has been limited reporting on the condition-specific risks associated with COVID-19, even though the prognosis for different complications can vary significantly.
In line with previous studies, our findings suggest a potential benefit of vaccination in reducing the risk of post-COVID-19 thromboembolic and cardiac complications. We included broader populations, estimated the risk in both acute and post-acute infection phases and replicated these using four large independent observational databases. By pooling results across different settings, we provided the most up-to-date and robust evidence on this topic.
Strengths and limitations
The study has several strengths. Our multinational study covering different healthcare systems and settings showed consistent results across all databases, which highlights the robustness and replicability of our findings. All databases had complete recordings of vaccination status (date and vaccine) and are representative of the respective general population. Algorithms to identify study outcomes were used in previous published network studies, including regulatory-funded research. 3 4 8 18 Other strengths are the staggered cohort design which minimises confounding by indication and immortal time bias. PS overlap weighting and NCO empirical calibration have been shown to adequately minimise bias in vaccine effectiveness studies. 19 Furthermore, our estimates include the vaccine effectiveness against COVID-19, which is crucial in the pathway to experience post-COVID-19 complications.
Our study has some limitations. The use of real-world data comes with inherent limitations including data quality concerns and risk of confounding. To deal with these limitations, we employed state-of-the-art methods, including large-scale propensity score weighting and calibration of effect estimates using NCO. 19 24 A recent study 30 has demonstrated that methodologically sound observational studies based on routinely collected data can produce results similar to those of clinical trials. We acknowledge that results from NCO were positively associated with vaccination, and estimates might still be influenced by residual bias despite using calibration. Another limitation is potential under-reporting of post-COVID-19 complications: some asymptomatic and mild COVID-19 infections might have not been recorded. Additionally, post-COVID-19 outcomes of interest might be under-recorded in primary care databases (CPRD Aurum and Gold) without hospital linkage, which represent a large proportion of the data in the study. However, results in SIDIAP and CORIVA, which include secondary care data, were similar. Also, our study included a small number of young men and male teenagers, who were the main population concerned with increased risks of myocarditis/pericarditis following vaccination.
Conclusions
Vaccination against SARS-CoV-2 substantially reduced the risk of acute post-COVID-19 thromboembolic and cardiac complications, probably through a reduction in the risk of SARS-CoV-2 infection and the severity of COVID-19 disease due to vaccine-induced immunity. Reduced risk in vaccinated people lasted for up to 1 year for post-COVID-19 VTE, ATE and HF, but not clearly for other complications. Findings from this study highlight yet another benefit of COVID-19 vaccination. However, further research is needed on the possible waning of the risk reduction over time and on the impact of booster vaccination.
Ethics statements
Patient consent for publication.
Not applicable.
Ethics approval
The study was approved by the CPRD’s Research Data Governance Process, Protocol No 21_000557 and the Clinical Research Ethics committee of Fundació Institut Universitari per a la recerca a l’Atenció Primària de Salut Jordi Gol i Gurina (IDIAPJGol) (approval number 4R22/133) and the Research Ethics Committee of the University of Tartu (approval No. 330/T-10).
Acknowledgments
This study is based in part on data from the Clinical Practice Research Datalink (CPRD) obtained under licence from the UK Medicines and Healthcare products Regulatory Agency. We thank the patients who provided these data, and the NHS who collected the data as part of their care and support. All interpretations, conclusions and views expressed in this publication are those of the authors alone and not necessarily those of CPRD. We would also like to thank the healthcare professionals in the Catalan healthcare system involved in the management of COVID-19 during these challenging times, from primary care to intensive care units; the Institut de Català de la Salut and the Program d’Analítica de Dades per a la Recerca i la Innovació en Salut for providing access to the different data sources accessible through The System for the Development of Research in Primary Care (SIDIAP).
- Pritchard E ,
- Matthews PC ,
- Stoesser N , et al
- Lauring AS ,
- Tenforde MW ,
- Chappell JD , et al
- Pistillo A , et al
- Duarte-Salles T , et al
- Hansen JV ,
- Fosbøl E , et al
- Chen A , et al
- Hippisley-Cox J ,
- Mei XW , et al
- Duarte-Salles T ,
- Fernandez-Bertolin S , et al
- Ip S , et al
- Bowe B , et al
- Prats-Uribe A ,
- Feng Q , et al
- Campbell J , et al
- Herrett E ,
- Gallagher AM ,
- Bhaskaran K , et al
- Raventós B ,
- Fernández-Bertolín S ,
- Aragón M , et al
- Makadia R ,
- Matcho A , et al
- Mercadé-Besora N ,
- Kolde R , et al
- Ostropolets A ,
- Makadia R , et al
- Rathod-Mistry T , et al
- Thomas LE ,
- ↵ Coronavirus (COVID-19) in the UK . 2022 . Available : https://coronavirus.data.gov.uk/
- Generalitat de Catalunya
- Schuemie MJ ,
- Hripcsak G ,
- Ryan PB , et al
- Houghton DE ,
- Wysokinski W ,
- Casanegra AI , et al
- Katsoularis I ,
- Fonseca-Rodríguez O ,
- Farrington P , et al
- Jehangir Q ,
- Li P , et al
- Byambasuren O ,
- Stehlik P ,
- Clark J , et al
- Brannock MD ,
- Preiss AJ , et al
- Schneeweiss S , RCT-DUPLICATE Initiative , et al
Supplementary materials
Supplementary data.
This web only file has been produced by the BMJ Publishing Group from an electronic file supplied by the author(s) and has not been edited for content.
- Data supplement 1
AMJ and MC are joint senior authors.
Contributors DPA and AMJ led the conceptualisation of the study with contributions from MC and NM-B. AMJ, TD-S, ER, AU and NTHT adapted the study design with respect to the local vaccine rollouts. AD and WYM mapped and curated CPRD data. MC and NM-B developed code with methodological contributions advice from MTS-S and CP. DPA, MC, NTHT, TD-S, HMEN, XL, CR and AMJ clinically interpreted the results. NM-B, XL, AMJ and DPA wrote the first draft of the manuscript, and all authors read, revised and approved the final version. DPA and AMJ obtained the funding for this research. DPA is responsible for the overall content as guarantor: he accepts full responsibility for the work and the conduct of the study, had access to the data, and controlled the decision to publish.
Funding The research was supported by the National Institute for Health and Care Research (NIHR) Oxford Biomedical Research Centre (BRC). DPA is funded through a NIHR Senior Research Fellowship (Grant number SRF-2018–11-ST2-004). Funding to perform the study in the SIDIAP database was provided by the Real World Epidemiology (RWEpi) research group at IDIAPJGol. Costs of databases mapping to OMOP CDM were covered by the European Health Data and Evidence Network (EHDEN).
Patient and public involvement Patients and/or the public were not involved in the design, or conduct, or reporting or dissemination plans of this research.
Provenance and peer review Not commissioned; externally peer reviewed.
Supplemental material This content has been supplied by the author(s). It has not been vetted by BMJ Publishing Group Limited (BMJ) and may not have been peer-reviewed. Any opinions or recommendations discussed are solely those of the author(s) and are not endorsed by BMJ. BMJ disclaims all liability and responsibility arising from any reliance placed on the content. Where the content includes any translated material, BMJ does not warrant the accuracy and reliability of the translations (including but not limited to local regulations, clinical guidelines, terminology, drug names and drug dosages), and is not responsible for any error and/or omissions arising from translation and adaptation or otherwise.
Read the full text or download the PDF:
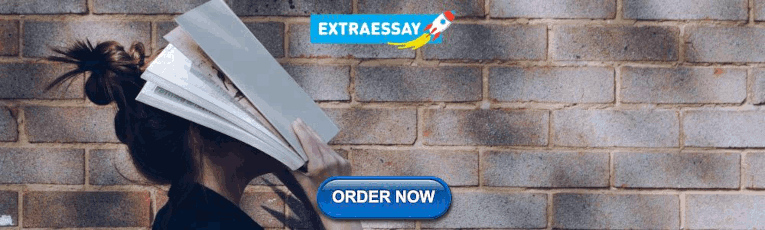
IMAGES
COMMENTS
Online learning is currently adopted by educational institutions worldwide to provide students with ongoing education during the COVID‐19 pandemic. Even though online learning research has been advancing in uncovering student experiences in various settings (i.e., tertiary, adult, and professional education), very little progress has been ...
In response to the COVID-19 pandemic, technological and administrative systems for implementing online learning, and the infrastructure that supports its access and delivery, had to adapt quickly.
The COVID-19 pandemic led to school closures and distance learning that are likely to exacerbate social class academic disparities. This Review presents an agenda for future research and outlines ...
The COVID-19 outbreak brought online learning to the forefront of education. Scholars have conducted many studies on online learning during the pandemic, but only a few have performed quantitative comparative analyses of students' online learning behavior before and after the outbreak. We collected review data from China's massive open online course platform called icourse.163 and ...
The COVID-19 pandemic has become a focus on reforming teaching, learning models and strategies, particularly in online teaching and learning tools. Based on the social cognitive career theory and the constructivist learning theory, the purpose of this study was to understand and explore the learning preference and experience of students' online courses during the COVID-19 pandemic and the ...
The COVID-19 has resulted in schools shut all across the world. Globally, over 1.2 billion children are out of the classroom. As a result, education has changed dramatically, with the distinctive rise of e-learning, whereby teaching is undertaken remotely and on digital platforms. Research suggests that online learning has been shown to ...
Read more: Online learning during COVID-19: 8 ways universities can improve equity and access Emergency online education used blunt-edged instruments, ignoring student and program differences .
Due to the COVID-19 pandemic, higher educational institutions worldwide switched to emergency distance learning in early 2020. The less structured environment of distance learning forced students to regulate their learning and motivation more independently. According to self-determination theory (SDT), satisfaction of the three basic psychological needs for autonomy, competence and social ...
Another research concludes that online teaching during the COVID-19 pandemic was only possible when online learning had a robust digital infrastructure and a learning system designed for that purpose; otherwise, it was an attempt to replicate face-to-face teaching in the virtual environment (Abdulrahim and Mabrouk, 2020).
"Think about your last year of study during the Covid-19 pandemic and what you need from a learning and teaching perspective to thrive." Explore more on these topics Online learning
The transition to online learning in the United States during the Covid-19 pandemic was, by many accounts, a failure. While there were some bright spots across the country, the transition was ...
First, we invested in learning from the many organizations working to tackle the digital divide prior to COVID-19: Girl Effect in girls' empowerment, Eneza and M-Shule in academic learning, and ...
A preliminary examination of key strategies, challenges, and benefits of remote learning expressed by parents during the COVID-19 pandemic Roy, A., et al., School Psychology , in press Remote learning during COVID-19: Examining school practices, service continuation, and difficulties for adolescents with and without attention-deficit ...
This paper aims to analyze student essays in the form of perspectives or responses about the challenges of online learning during the COVID-19 pandemic by collecting fifteen students as samples in the Fundamentals of Education I course. COVID-19 pandemic has changed the way of learning in higher education. Teaching, and learning activities that are usually carried out with face-to-face ...
Effects of the Coronavirus disease 2019 (COVID-19) pandemic on children stem beyond immediate infectious and post-infectious risks. Our aim was to conduct a scoping review and produce an online Interactive Evidence Map (IEM) highlighting available literature around unintended effects of the pandemic on children's and adolescents' mental, psychosocial, and physical health.
along with the transition to online learning may have a ected their academic performance, educational plans, current labor market participation, and expectations about future employment. This paper attempts to shed light on the impact of the COVID-19 pandemic on college students. First,
The COVID-19 pandemic introduced a global need to explore the potential and challenges of online education. To evaluate the presence of depression and anxiety in university students and their level of satisfaction with online learning during the period of social isolation caused by the COVID-19 pandemic. A cross-sectional design was used to evaluate 152 online learning students from six ...
Kasia Brzoska. The COVID-19 pandemic has changed the way our students learn. Our team at Cambridge University Press asked 1,200 learners around the world how they are adapting to this new learning environment and how they feel about their progress. The survey reached learners from over 100 countries around the world, ranging from school ...
Source: COVID-19 score drops are pulled from Kuhfeld et al. (2022) Table 5; reduction-in-class-size results are from pg. 10 of Figles et al. (2018) Table 2; summer program results are pulled from ...
For closure lengths, the study averaged district-level estimates of time spent in remote and hybrid learning compiled by the Covid-19 School Data Hub (C.S.D.H.) and American Enterprise Institute ...
LEXINGTON, Ky. (April 1, 2024) — University of Kentucky College of Education Associate Professor John Nash, Ph.D., and Jason Johnston, Ph.D., hosts of the Online Learning in the Second Half podcast, facilitated a conversation about the future of online learning during a recent Johns Hopkins University symposium. The session is now available as a podcast episode for listening online.
The Covid‐19 pandemic left many education institutions with no option but to transition to online learning. The University of Pretoria was no exception. We examine the effect of transitioning to online learning on the academic performance of second‐year economic students. We use assessment results from F2F lectures before lockdown, and ...
Objective To study the association between COVID-19 vaccination and the risk of post-COVID-19 cardiac and thromboembolic complications. Methods We conducted a staggered cohort study based on national vaccination campaigns using electronic health records from the UK, Spain and Estonia. Vaccine rollout was grouped into four stages with predefined enrolment periods. Each stage included all ...