A Basic Branch and Bound Solver in Python using Cvxpy
Jun 13, 2019 • philzook58
Branch and bound is a useful problem solving technique. The idea is, if you have a minimization problem you want to solve, maybe there is a way to relax the constraints to an easier problem. If so, the solution of the easier problem is a lower bound on the possible solution of the hard problem. If the solution of the easier problem just so happens to also obey the more constrained hard problem, then it must also be the solution to the hard problem. You can also use the lower bound coming from a relaxed problem to prune your search tree for the hard problem. If even the relaxed problem doesn’t beat the current best found, don’t bother going down that branch.
A standard place this paradigm occurs is in mixed integer programming. The relaxation of a binary constraint (either 0 or 1) can be all the values in between (any number between 0 and 1). If this relaxed problem can be expressed in a form amenable to a solver like a linear programming solver, you can use that to power the branch and bound search, also using returned solutions for possible heuristics.
I built a basic version of this that uses cvxpy as the relaxed problem solver. Cvxpy already has much much faster mixed integer solvers baked in (which is useful to make sure mine is returning correct results), but it was an interesting exercise. The real reason I’m toying around is I kind of want the ability to add custom branching heuristics or inspect and maintain the branch and bound search tree, which you’d need to get into the more complicated guts of the solvers bound to cvxpy to get at. Julia might be a better choice.
A somewhat similar (and better) project is https://github.com/oxfordcontrol/miosqp which doesn’t use cvxpy explicitly, but does have the branch and bound control in the python layer of the solver. There are also other projects that can use fairly arbitrary solvers like Bonmin
As a toy problem I’m using a knapsack problem where we have objects of different sizes and different values. We want to maximize the value while keeping the total size under the capacity of the bag. This can be phrased linearly like so: $ \max v \cdot x$ s.t. $ \sum_i s_i x_i<= capacity $, $ x \in {0,1}$. The basic heuristic I’m using is to branch on variables that are either 0 or 1 in even the relaxed solution. The alternative branch hopefully gets pruned fast.
This is at least solving the problem fairly quickly. It needs better heuristics and to be sped up, which is possible in lots of ways. I was not trying to avoid all performance optimizations. It takes maybe 5 seconds, whereas the cvxpy solver is almost instantaneous.
Edit : I should investigate the Parameter functionality of cvxpy. That would make a make faster version than the one above based on deepcopy. If you made the upper and lower vectors on the binary variables parameters, you could restrict the interval to 0/1 without rebuilding the problem or copying everything.
- Data Structures
- Linked List
- Binary Tree
- Binary Search Tree
- Segment Tree
- Disjoint Set Union
- Fenwick Tree
- Red-Black Tree
- Advanced Data Structures
- Branch and Bound Algorithm
- Introduction to Branch and Bound - Data Structures and Algorithms Tutorial
- 0/1 Knapsack using Branch and Bound
- Implementation of 0/1 Knapsack using Branch and Bound
- 8 puzzle Problem using Branch And Bound
- Job Assignment Problem using Branch And Bound
- N Queen Problem using Branch And Bound
- Traveling Salesman Problem using Branch And Bound
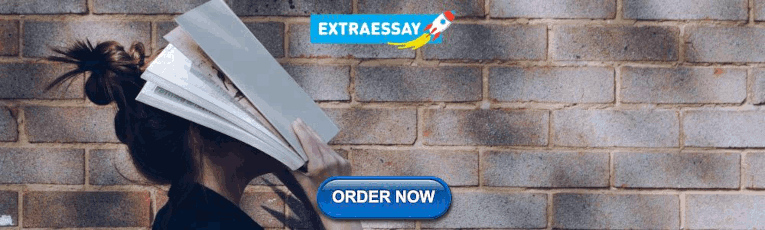
Introduction to Branch and Bound – Data Structures and Algorithms Tutorial
Branch and bound algorithms are used to find the optimal solution for combinatory, discrete, and general mathematical optimization problems. A branch and bound algorithm provide an optimal solution to an NP-Hard problem by exploring the entire search space. Through the exploration of the entire search space, a branch and bound algorithm identify possible candidates for solutions step-by-step.
There are many optimization problems in computer science, many of which have a finite number of the feasible shortest path in a graph or minimum spanning tree that can be solved in polynomial time. Typically, these problems require a worst-case scenario of all possible permutations. The branch and bound algorithm create branches and bounds for the best solution.
In this tutorial, we’ll discuss the branch and bound method in detail.
Different search techniques in branch and bound:
The Branch algorithms incorporate different search techniques to traverse a state space tree. Different search techniques used in B&B are listed below:
1. LC search (Least Cost Search):
It uses a heuristic cost function to compute the bound values at each node. Nodes are added to the list of live nodes as soon as they get generated. The node with the least value of a cost function selected as a next E-node.
2.BFS(Breadth First Search): It is also known as a FIFO search. It maintains the list of live nodes in first-in-first-out order i.e, in a queue, The live nodes are searched in the FIFO order to make them next E-nodes.
3. DFS (Depth First Search): It is also known as a LIFO search. It maintains the list of live nodes in last-in-first-out order i.e. in a stack.
The live nodes are searched in the LIFO order to make them next E-nodes.
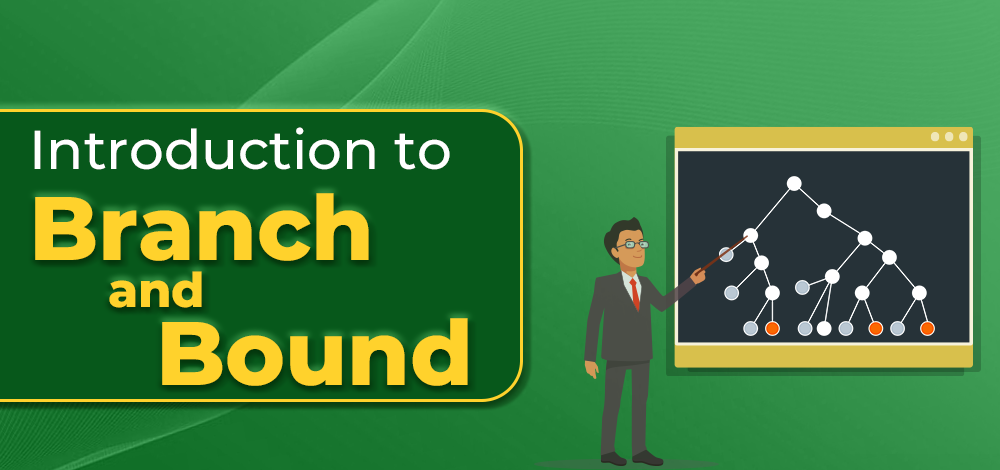
Introduction to Branch and Bound
When to apply Branch and Bound Algorithm?
Branch and bound is an effective solution to some problems, which we have already discussed. We’ll discuss all such cases where branching and binding are appropriate in this section.
- It is appropriate to use a branch and bound approach if the given problem is discrete optimization. Discrete optimization refers to problems in which the variables belong to the discrete set. Examples of such problems include 0-1 Integer Programming and Network Flow problems.
- When it comes to combinatory optimization problems, branch and bound work well. An optimization problem is optimized by combinatory optimization by finding its maximum or minimum based on its objective function. The combinatory optimization problems include Boolean Satisfiability and Integer Linear Programming.
Basic Concepts of Branch and Bound:
▸ Generation of a state space tree:
As in the case of backtracking, B&B generates a state space tree to efficiently search the solution space of a given problem instance.
In B&B, all children of an E-node in a state space tree are produced before any live node gets converted in an E-node. Thus, the E-node remains an E-node until i becomes a dead node.
- Evaluation of a candidate solution:
Unlike backtracking, B&B needs additional factors evaluate a candidate solution:
- A way to assign a bound on the best values of the given criterion functions to each node in a state space tree: It is produced by the addition of further components to the partial solution given by that node.
- The best values of a given criterion function obtained so far: It describes the upper bound for the maximization problem and the lower bound for the minimization problem.
- A feasible solution is defined by the problem states that satisfy all the given constraints.
- An optimal solution is a feasible solution, which produces the best value of a given objective function.
- Bounding function :
It optimizes the search for a solution vector in the solution space of a given problem instance.
It is a heuristic function that evaluates the lower and upper bounds on the possible solutions at each node. The bound values are used to search the partial solutions leading to an optimal solution. If a node does not produce a solution better than the best solution obtained thus far, then it is abandoned without further exploration.
The algorithm then branches to another path to get a better solution. The desired solution to the problem is the value of the best solution produced so far.
▸ The reasons to dismiss a search path at the current node :
(i) The bound value of the node is lower than the upper bound in the case of the maximization problem and higher than the lower bound in the case of the minimization problem. (i.e. the bound value of the ade is not better than the value of the best solution obtained until that node).
(ii) The node represents infeasible solutions, de violation of the constraints of the problem.
(iii) The node represents a subset of a feasible solution containing a single point. In this case, if the latest solution is better than the best solution obtained so far the best solution is modified to the value of a feasible solution at that node.
Types of Branch and Bound Solutions:
The solution of the Branch and the bound problem can be represented in two ways:
- Variable size solution: Using this solution, we can find the subset of the given set that gives the optimized solution to the given problem. For example, if we have to select a combination of elements from {A, B, C, D} that optimizes the given problem, and it is found that A and B together give the best solution, then the solution will be {A, B}.
- Fixed-size solution: There are 0s and 1s in this solution, with the digit at the ith position indicating whether the ith element should be included, for the above example, the solution will be given by {1, 1, 0, 0}, here 1 represent that we have select the element which at ith position and 0 represent we don’t select the element at ith position.
Classification of Branch and Bound Problems:
The Branch and Bound method can be classified into three types based on the order in which the state space tree is searched.
- FIFO Branch and Bound
- LIFO Branch and Bound
- Least Cost-Branch and Bound
We will now discuss each of these methods in more detail. To denote the solutions in these methods, we will use the variable solution method.
1. FIFO Branch and Bound
First-In-First-Out is an approach to the branch and bound problem that uses the queue approach to create a state-space tree. In this case, the breadth-first search is performed, that is, the elements at a certain level are all searched, and then the elements at the next level are searched, starting with the first child of the first node at the previous level.
For a given set {A, B, C, D}, the state space tree will be constructed as follows :
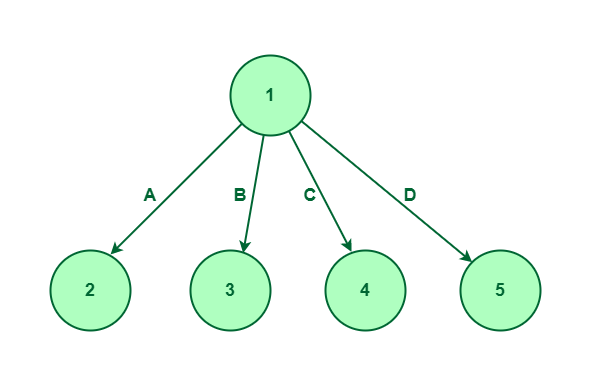
State Space tree for set {A, B, C, D}
The above diagram shows that we first consider element A, then element B, then element C and finally we’ll consider the last element which is D. We are performing BFS while exploring the nodes.
So, once the first level is completed. We’ll consider the first element, then we can consider either B, C, or D. If we follow the route then it says that we are doing elements A and D so we will not consider elements B and C. If we select the elements A and D only, then it says that we are selecting elements A and D and we are not considering elements B and C.
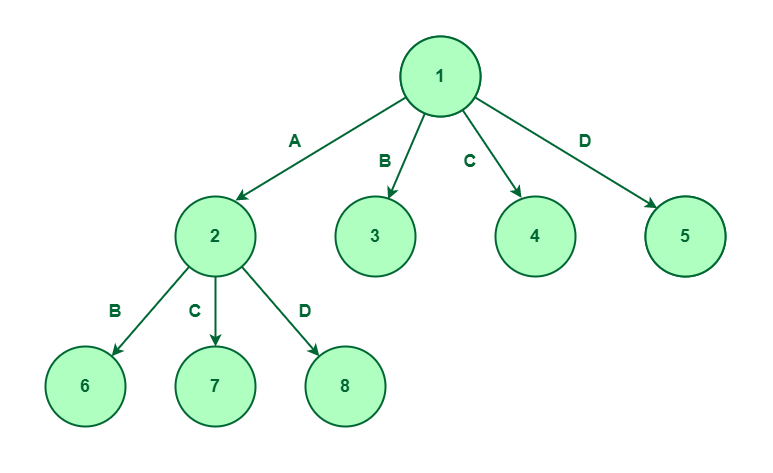
Selecting element A
Now, we will expand node 3, as we have considered element B and not considered element A, so, we have two options to explore that is elements C and D. Let’s create nodes 9 and 10 for elements C and D respectively.
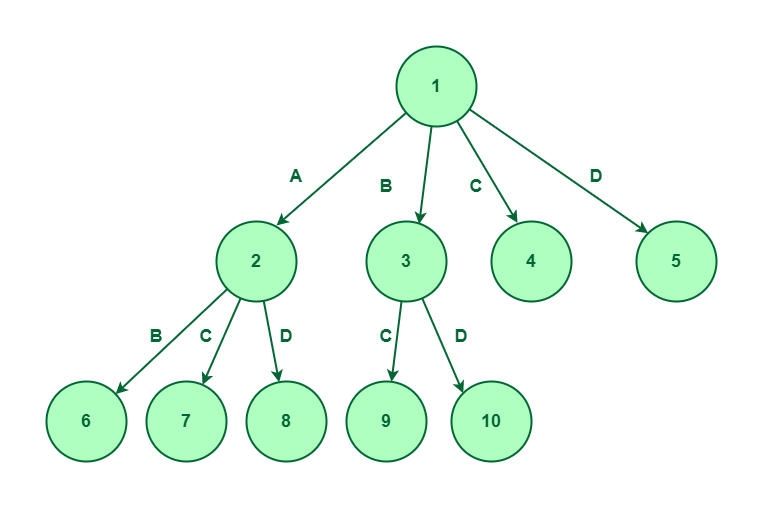
Considered element B and not considered element A
Now, we will expand node 4 as we have only considered elements C and not considered elements A and B, so, we have only one option to explore which is element D. Let’s create node 11 for D.
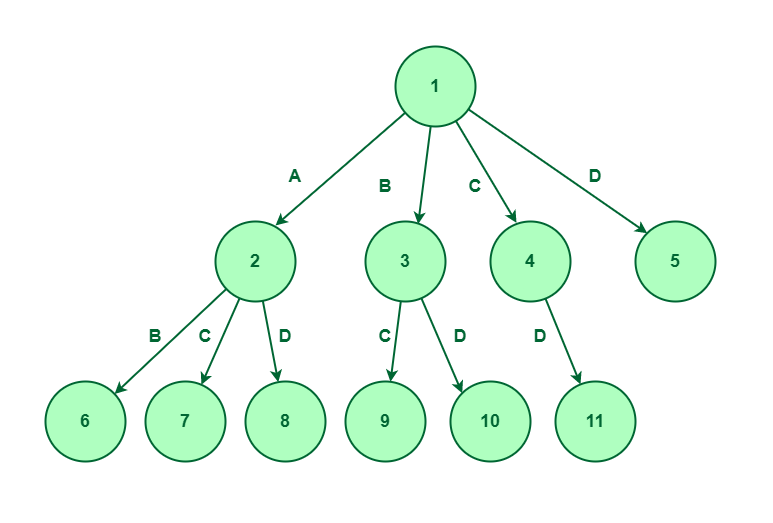
Considered elements C and not considered elements A and B
Till node 5, we have only considered elements D, and not selected elements A, B, and C. So, We have no more elements to explore, Therefore on node 5, there won’t be any expansion.
Now, we will expand node 6 as we have considered elements A and B, so, we have only two option to explore that is element C and D. Let’s create node 12 and 13 for C and D respectively.
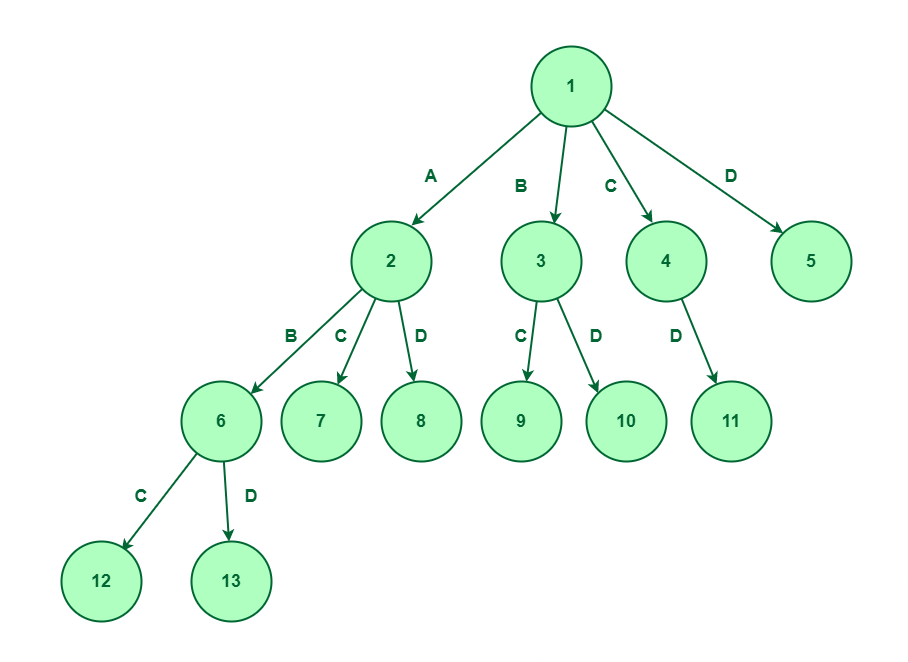
Expand node 6
Now, we will expand node 7 as we have considered elements A and C and not consider element B, so, we have only one option to explore which is element D. Let’s create node 14 for D.
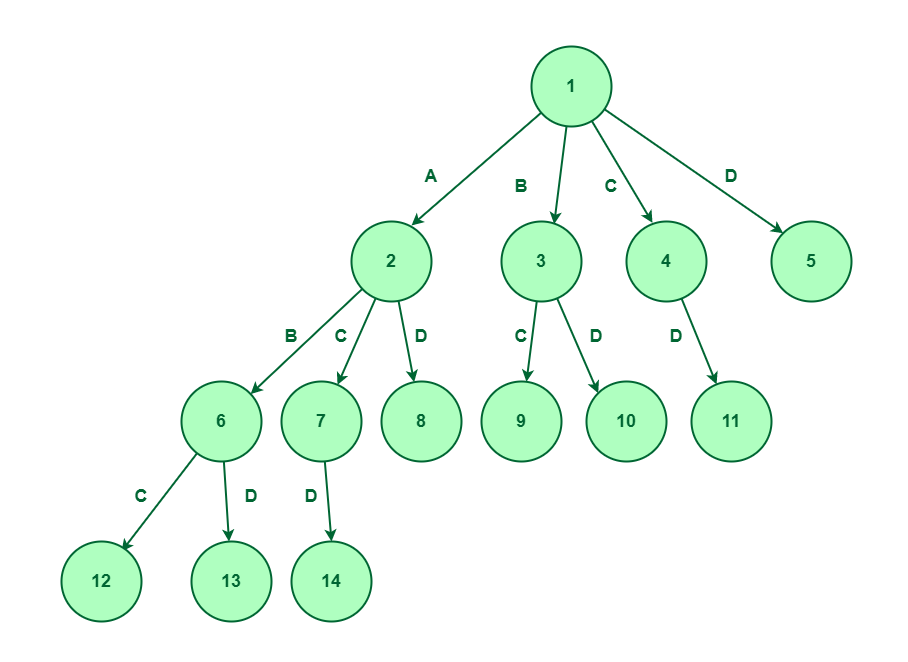
Expand node 7
Till node 8, we have considered elements A and D, and not selected elements B and C, So, We have no more elements to explore, Therefore on node 8, there won’t be any expansion.
Now, we will expand node 9 as we have considered elements B and C and not considered element A, so, we have only one option to explore which is element D. Let’s create node 15 for D.
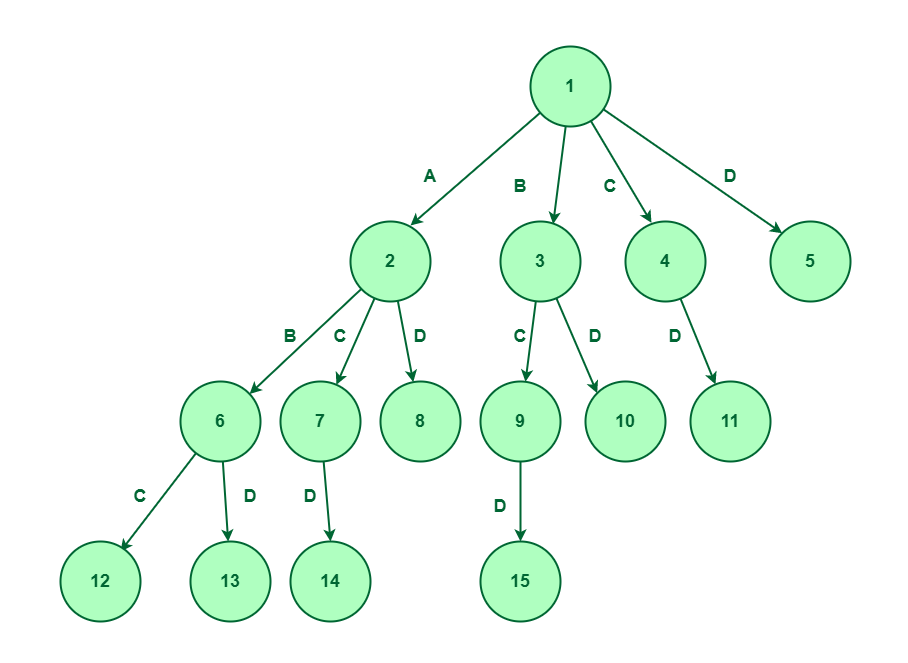
Expand node 9
2. LIFO Branch and Bound
The Last-In-First-Out approach for this problem uses stack in creating the state space tree. When nodes are added to a state space tree, they are added to a stack. After all nodes of a level have been added, we pop the topmost element from the stack and explore it.
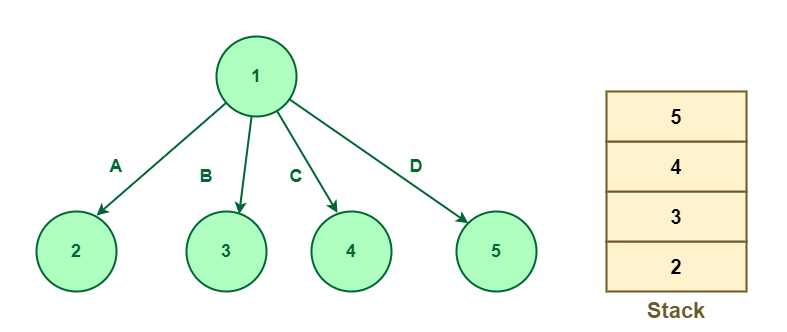
State space tree for element {A, B, C, D}
Now the expansion would be based on the node that appears on the top of the stack. Since node 5 appears on the top of the stack, so we will expand node 5. We will pop out node 5 from the stack. Since node 5 is in the last element, i.e., D so there is no further scope for expansion.
The next node that appears on the top of the stack is node 4. Pop-out node 4 and expand. On expansion, element D will be considered and node 6 will be added to the stack shown below:
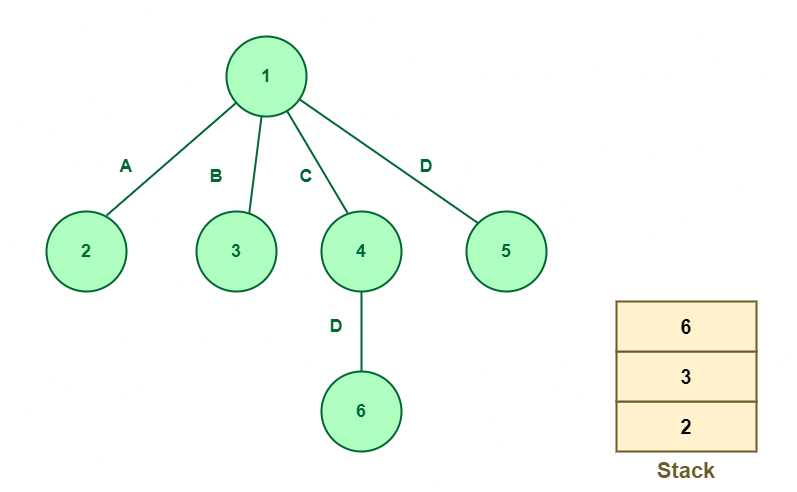
Expand node 4
The next node is 6 which is to be expanded. Pop-out node 6 and expand. Since node 6 is in the last element, i.e., D so there is no further scope for expansion.
The next node to be expanded is node 3. Since node 3 works on element B so node 3 will be expanded to two nodes, i.e., 7 and 8 working on elements C and D respectively. Nodes 7 and 8 will be pushed into the stack.
The next node that appears on the top of the stack is node 8. Pop-out node 8 and expand. Since node 8 works on element D so there is no further scope for the expansion.
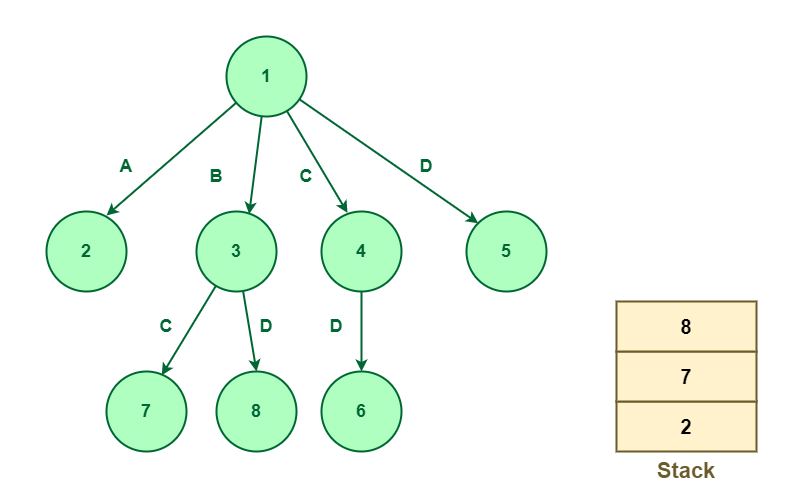
Expand node 3
The next node that appears on the top of the stack is node 7. Pop-out node 7 and expand. Since node 7 works on element C so node 7 will be further expanded to node 9 which works on element D and node 9 will be pushed into the stack.
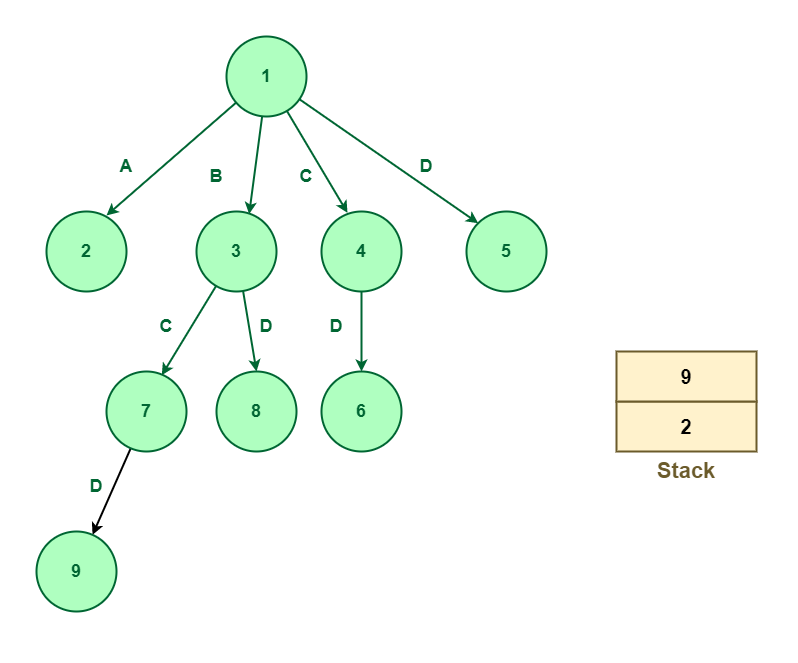
The next node that appears on the top of the stack is node 9. Since node 9 works on element D, there is no further scope for expansion.
The next node that appears on the top of the stack is node 2. Since node 2 works on the element A so it means that node 2 can be further expanded. It can be expanded up to three nodes named 10, 11, 12 working on elements B, C, and D respectively. There new nodes will be pushed into the stack shown as below:
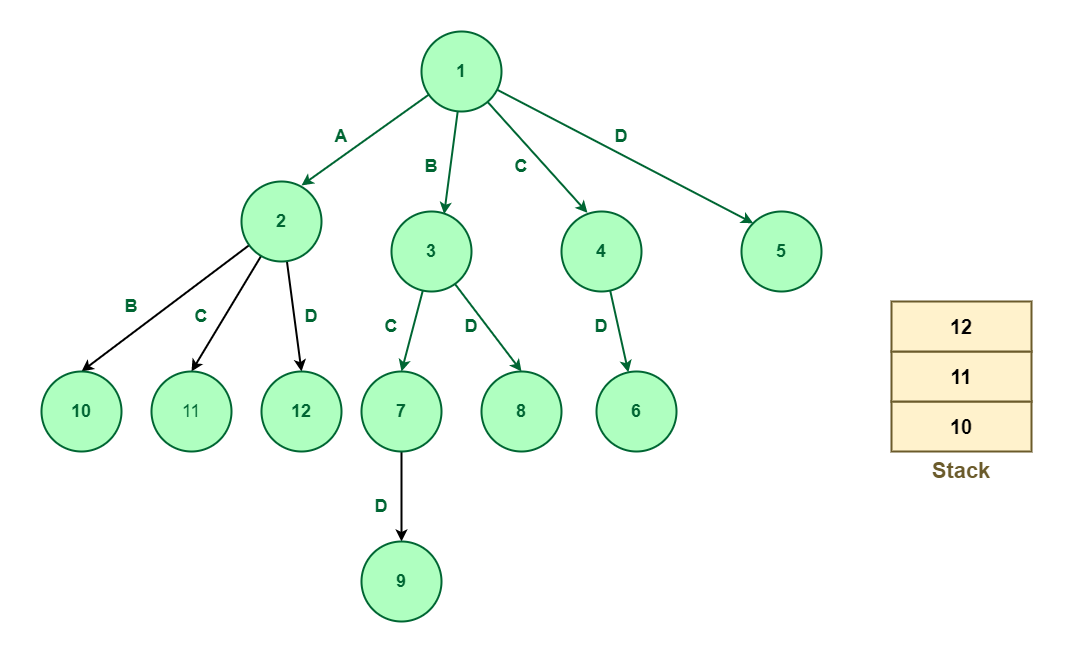
Expand node 2
In the above method, we explored all the nodes using the stack that follows the LIFO principle.
3. Least Cost-Branch and Bound
To explore the state space tree, this method uses the cost function. The previous two methods also calculate the cost function at each node but the cost is not been used for further exploration.
In this technique, nodes are explored based on their costs, the cost of the node can be defined using the problem and with the help of the given problem, we can define the cost function. Once the cost function is defined, we can define the cost of the node. Now, Consider a node whose cost has been determined. If this value is greater than U0, this node or its children will not be able to give a solution. As a result, we can kill this node and not explore its further branches. As a result, this method prevents us from exploring cases that are not worth it, which makes it more efficient for us.
Let’s first consider node 1 having cost infinity shown below:
In the following diagram, node 1 is expanded into four nodes named 2, 3, 4, and 5.
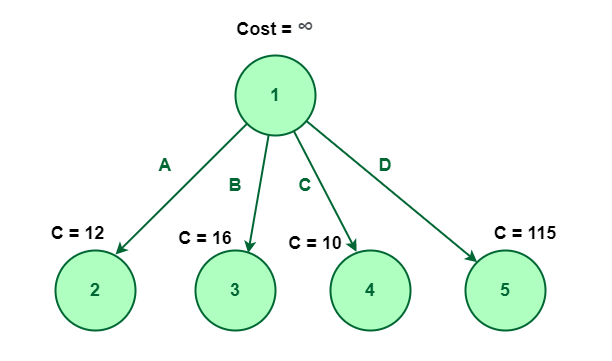
Node 1 is expanded into four nodes named 2, 3, 4, and 5
Assume that cost of the nodes 2, 3, 4, and 5 are 12, 16, 10, and 315 respectively. In this method, we will explore the node which is having the least cost. In the above figure, we can observe that the node with a minimum cost is node 4. So, we will explore node 4 having a cost of 10.
During exploring node 4 which is element C, we can notice that there is only one possible element that remains unexplored which is D (i.e, we already decided not to select elements A, and B). So, it will get expanded to one single element D, let’s say this node number is 6.
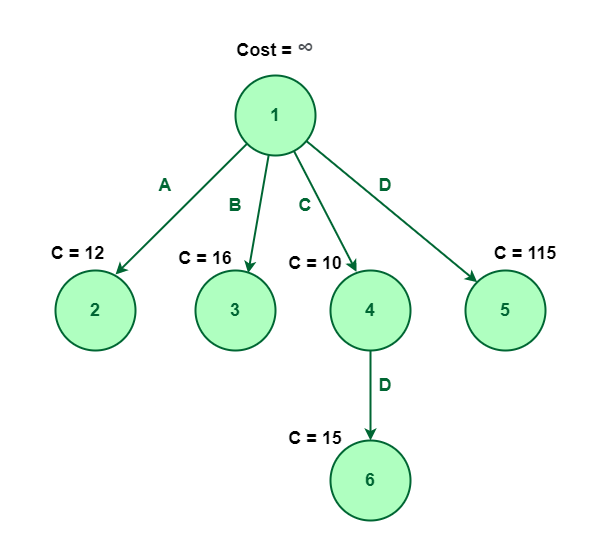
Exploring node 4 which is element C
Now, Node 6 has no element left to explore. So, there is no further scope for expansion. Hence the element {C, D} is the optimal way to choose for the least cost.
Problems that can be solved using Branch and Bound Algorithm:
The Branch and Bound method can be used for solving most combinatorial problems. Some of these problems are given below:
Advantages of Branch and Bound Algorithm:
- We don’t explore all the nodes in a branch and bound algorithm. Due to this, the branch and the bound algorithm have a lower time complexity than other algorithms.
- Whenever the problem is small and the branching can be completed in a reasonable amount of time, the algorithm finds an optimal solution.
- By using the branch and bound algorithm, the optimal solution is reached in a minimal amount of time. When exploring a tree, it does not repeat nodes.
Disadvantages of Branch and Bound Algorithm:
- It takes a long time to run the branch and bound algorithm.
- In the worst-case scenario, the number of nodes in the tree may be too large based on the size of the problem.
The branch and bound algorithms are one of the most popular algorithms used in optimization problems that we have discussed in our tutorial. We have also explained when a branch and bound algorithm is appropriate for a user to use. In addition, we presented an algorithm based on branches and bounds for assigning jobs. Lastly, we discussed some advantages and disadvantages of branch and bound algorithms.
Please Login to comment...
- DSA Tutorials
- Branch and Bound
- How to Delete Whatsapp Business Account?
- Discord vs Zoom: Select The Efficienct One for Virtual Meetings?
- Otter AI vs Dragon Speech Recognition: Which is the best AI Transcription Tool?
- Google Messages To Let You Send Multiple Photos
- 30 OOPs Interview Questions and Answers (2024)
Improve your Coding Skills with Practice
What kind of Experience do you want to share?
Search code, repositories, users, issues, pull requests...
Provide feedback.
We read every piece of feedback, and take your input very seriously.
Saved searches
Use saved searches to filter your results more quickly.
To see all available qualifiers, see our documentation .
branch-and-bound
Here are 75 public repositories matching this topic..., akrm3008 / essential-search.
Use this to determine the optimal route to go on a search for shortage struck essential commodities (gasoline, water, toilet paper etc.) using information from social media
- Updated Aug 16, 2020
deryrahman / if2211-tsp-branch-and-bound
Travel Salesman Problem using Branch and Bound Algorithm, from IF2211: Algorithmic Strategy
- Updated Apr 6, 2017
andrea-covre / Minimum-Vertex-Cover
With this project we researched, implemented and analyzed different algorithms to effectively and efficiently solve an NP-Complete problem such as the Minimum Vertex Cover (MVC).
- Updated Jan 11, 2023
apwic / fifteen-puzzle-solver
Fifteen Puzzle Solver. Made as an assignment to demonstrate the use of Branch and Bound Algorithm in IF2211 Algorithm Strategy
- Updated Apr 4, 2022
luanloose / branch-and-bound
Primeira avaliação da matéria de inteligência artificial.
- Updated Sep 16, 2021
JLMSC / Problema-Da-Arvore-Geradora-Minima-Rotulada
Problema da Árvore Geradora Mínima Rotulada (PAGMR)
- Updated May 20, 2022
darkhorrow / branch-and-bound-knapsack
Branch and boun applied to binary knapsack problem
- Updated Dec 8, 2020
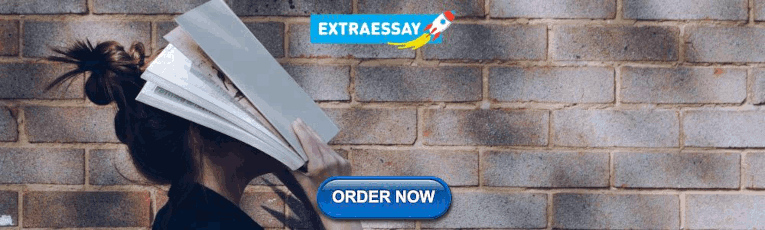
novak-a / Branch-and-Bound-algorithm-in-Python
Simple Branch and Bound algorithm in Python
- Updated Nov 24, 2023
AvihayHalfin / Parallel-Machines-Scheduling
Parallel machines schduling using different metaheuristic
- Updated Aug 27, 2022
VirtualRoyalty / branch-and-bound-clique
Implementation of Branch-n-Bound algorithm for Max Clique Problem via cplex-solver
- Updated Oct 23, 2021
otavioml / Knapsack-Problem
Knapsack Problem solution using two distincts aproaches: backtracking and branch-and-bound.
- Updated Feb 14, 2022
movonangern / branch-and-bound-optimization
The following repository shows an implementation of the branch and bound optimization. The task was part of my studies and the module "Operations Research". In cooperation with https://github.com/richard-zi
- Updated Oct 16, 2023
AcoranGonzalezMoray / Algoritmo-Ramificacion-Acotacion-
Resolución de un problema mediante algoritmo 'Branch & Bound' con recorrido DFS
- Updated Oct 14, 2022
AlexanderSlav / Max-Clique-Cplex-Python
Maximum Clique Problem solved by Branch and Bound method and Cplex Optimization.
- Updated Dec 3, 2021
rifqifarelmuhammad / daa-experimental-task-2
- Updated Dec 5, 2023
alex-87 / qaekwy-python
Python Client library for Qaekwy Operational Research Solver
- Updated Sep 2, 2023
caiozanatelli / Simplex
An implementation for the Simplex algorithm for solving linear optimization problems. This approach covers both Primal and Dual Simplex.
- Updated Jun 28, 2018
keyber / RP
Implémentation d'algorithmes de Résolution de Problèmes
- Updated Nov 5, 2019
mdaffad / bnb
Branch and Bound
- Updated Mar 25, 2020
cwpearson / csips
A pure-python integer programming solver
- Updated Apr 7, 2022
Improve this page
Add a description, image, and links to the branch-and-bound topic page so that developers can more easily learn about it.
Curate this topic
Add this topic to your repo
To associate your repository with the branch-and-bound topic, visit your repo's landing page and select "manage topics."
A branch and bound algorithm for the generalized assignment problem
- Published: December 1975
- Volume 8 , pages 91–103, ( 1975 )
Cite this article
- G. Terry Ross 1 &
- Richard M. Soland 2
2194 Accesses
331 Citations
3 Altmetric
Explore all metrics
This paper describes what is termed the “generalized assignment problem”. It is a generalization of the ordinary assignment problem of linear programming in which multiple assignments of tasks to agents are limited by some resource available to the agents. A branch and bound algorithm is developed that solves the generalized assignment problem by solving a series of binary knapsack problems to determine the bounds. Computational results are cited for problems with up to 4 000 0–1 variables, and comparisons are made with other algorithms.
This is a preview of subscription content, log in via an institution to check access.
Access this article
Price includes VAT (Russian Federation)
Instant access to the full article PDF.
Rent this article via DeepDyve
Institutional subscriptions
Similar content being viewed by others
A transportation branch and bound algorithm for solving the generalized assignment problem.
Elias Munapo, ‘Maseka Lesaoana, … Santosh Kumar
Reducing the Branching in a Branch and Bound Algorithm for the Maximum Clique Problem
A specialized branch-and-bound algorithm for the euclidean steiner tree problem in n-space.
Marcia Fampa, Jon Lee & Wendel Melo
V. Balachandran, “An integer generalized transportation model for optimal job assignment in computer networks”, Working Paper 34-72-3, Graduate School of Industrial Administration, Carnegie-Mellon University, Pittsburgh, Pa. (November, 1972).
Google Scholar
A. Charnes, W.W. Cooper, D. Klingman and R. Niehaus, “Static and dynamic biased quadratic multi-attribute assignment models: solutions and equivalents”, Center for Cybernetic Studies, Research Report CS 115, The University of Texas, Austin, Texas (January, 1973).
R.J. Dakin, “A tree search algorithm for mixed integer programming problems”, Computer Journal 8 (3) (1965) 250–255.
A. DeMaio and C. Roveda, “An all zero–one algorithm for a certain class of transportation problems”, Operations Research 19 (6) (1971) 1406–1418.
A.M. Geoffrion, “An improved implicit enumeration approach for integer programming”, Operations Research 17 (3) (1969) 437–454.
A.M. Geoffrion, “Lagrangean relaxation for integer programming”, Mathematical Programming Study 2 (1974) 82–114.
A.M. Geoffrion and G.W. Graves, “Multicommodity distribution system design by benders decomposition”, Management Science 20 (5) (1974) 822–844.
H. Greenberg and R.L. Hegerich, “A branch search algorithm for the knapsack problem”, Management Science 16 (5) (1970) 327–332.
M.D. Grigoriadis, D.T. Tang and L.S. Woo, “Considerations in the optimal synthesis of some communication networks”, Presented at the 45 th Joint National Meeting of the Operations Research Society of America and The Institute of Management Sciences, Boston, Mass., April 22–24, 1974.
D. Gross and C.E. Pinkus, “Optimal allocation of ships to yards for regular overhauls”, Tech. Memorandum 63095, Institute for Management Science and Engineering, The George Washington University, Washington, D.C. (May, 1972).
G.P. Ingargiola and J.F. Korsh, “Reduction algorithm for zero–one single knapsack problems”, Management Science 20 (4) Part I (1973) 460–463.
D. Klingman and J. Stutz, “Computational testing on an integer generalized network code”, Presented at the 45 th Joint National Meeting of the Operations Research Society of America and The Institute of Management Sciences, Boston, Mass., April 22–24, 1974.
J.R. Lourie, “Topology and computation of the generalized transportation problem”, Management Science 11 (1) (1964) 177–187.
V. Srinivasan and G. Thompson, “An algorithm for assigning uses to sources in a speical class of transportation problems”, Operations Research 21 (1) (1973) 284–295.
Download references
Author information
Authors and affiliations.
University of Massachusetts, Amherst, Mass., USA
G. Terry Ross
University of Texas, Austin, Tex., USA
Richard M. Soland
You can also search for this author in PubMed Google Scholar
Additional information
This research was partly supported by ONR Contracts N00014-67-A-0126-0008 and N00014-67-A-0126-0009 with the Center for Cybernetic Studies, The University of Texas.
Rights and permissions
Reprints and permissions
About this article
Ross, G.T., Soland, R.M. A branch and bound algorithm for the generalized assignment problem. Mathematical Programming 8 , 91–103 (1975). https://doi.org/10.1007/BF01580430
Download citation
Received : 18 September 1973
Revised : 15 October 1974
Issue Date : December 1975
DOI : https://doi.org/10.1007/BF01580430
Share this article
Anyone you share the following link with will be able to read this content:
Sorry, a shareable link is not currently available for this article.
Provided by the Springer Nature SharedIt content-sharing initiative
- Mathematical Method
- Computational Result
- Assignment Problem
- Knapsack Problem
- Generalize Assignment
- Find a journal
- Publish with us
- Track your research
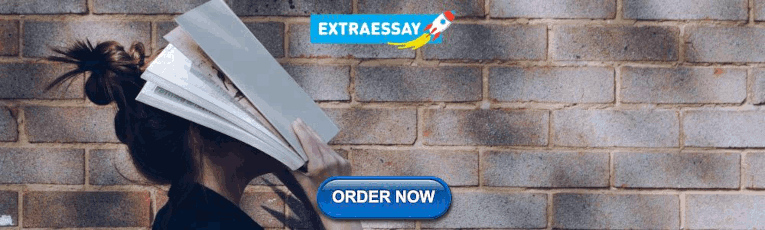
IMAGES
VIDEO
COMMENTS
Solution 4: Finding Optimal Solution using Branch and Bound. The selection rule for the next node in BFS and DFS is "blind". i.e. the selection rule does not give any preference to a node that has a very good chance of getting the search to an answer node quickly. The search for an optimal solution can often be speeded by using an ...
Branch and bound is a search algorithm used for combinatory, discrete, and general mathematical optimization problems. It is comparable to backtracking in that it similarly implements a state-space stream to represent the solution to the problem. However, it is probably more suited to trying to address optimization problems and only ...
A Basic Branch and Bound Solver in Python using Cvxpy. Branch and bound is a useful problem solving technique. The idea is, if you have a minimization problem you want to solve, maybe there is a way to relax the constraints to an easier problem. If so, the solution of the easier problem is a lower bound on the possible solution of the hard problem.
Basic Idea. Branch and bound algorithms are used to find the optimal solution for combinatory, discrete, and general mathematical optimization problems. In general, given an NP-Hard problem, a branch and bound algorithm explores the entire search space of possible solutions and provides an optimal solution. A branch and bound algorithm consist ...
Initialize maximum profit, maxProfit = 0. Create an empty queue, Q. Create a dummy node of decision tree and enqueue it to Q. Profit and weight of dummy node are 0. Do following while Q is not empty. Extract an item from Q. Let the extracted item be u. Compute profit of next level node. If the profit is more than maxProfit, then update ...
In this step, we will solve the LP problem by calling solve () method. We can print the final value by using the following for loop. From the above results, we can infer that Worker-1 will be assigned to Job-1, Worker-2 will be assigned to job-3, Worker-3 will be assigned to Job-2, and Worker-4 will assign with job-4.
This commit does not belong to any branch on this repository, and may belong to a fork outside of the repository. About Solved the Job Assignment Problem using a branch and bound algorithm
This article aims at solving an Assignment Problem using the Gurobi package of Python. ... best bound 1.500000000000e+01, ... Modeling Nurse Scheduling Problem in Python (Part 2-Mathematical Model
The Branch and Bound Algorithm is a method used in combinatorial optimization problems to systematically search for the best solution. It works by dividing the problem into smaller subproblems, or branches, and then eliminating certain branches based on bounds on the optimal solution. This process continues until the best solution is found or ...
It shall required to perform everything jobs by assigning exactly one worker to each job and exactly one job to anywhere agent in such a manner that the sum cost of the assignment is minimized. Branch Furthermore Bound (Job Assignment Problem) - Branch And Limited - E is required to perform all jobs by assigning exactly one worker to each job.
This video introduces the branch-and-bound algorithmic problem-solving approach and explains the job assignment problem using the same.
Integrality and the Branch & Bound algorithm. As previously defined, if in the solution of a linear problem in its relaxed form all integrality constraints are valid, the solution is also optimal to the integer or mixed integer problem. Let us then consider that, in the same example from the previous section, both x₁ and x₂ needed to be ...
To associate your repository with the branch-and-bound topic, visit your repo's landing page and select "manage topics." Learn more. GitHub is where people build software. More than 100 million people use GitHub to discover, fork, and contribute to over 420 million projects.
Problem 1: Linear Programming Relaxation. Problem 1 is called "Linear Programming Relaxation" because we relaxed the integer requirement constraint (opposite of tightening) effectively making it a linear programming problem.The first step involves, solving this problem. Now, I know I promised to solve the algorithm from scratch and we will for the branch and bound, but for solving LP, we ...
Let there be N workers and N jobs. Any worker can be assigned to perform any job, incurring some cost that may vary depending on the work-job assignment. It is required to perform all jobs by assigning exactly one worker to each job and exactly one job to each agent in such a way that the total cost of the assignment is minimized. - Audorion/Job-Assignment-Problem-Branch-And-Bound
So I replaced bound += (k - wt) * (v[j] / w[j]) with bound += v[j]; apparently the former was causing the algorithm to stop prematurely.I also had some backwards variables when I was initializing my left and right nodes (fixed now). Now I am stuck on marking which items were taken or not.
Branch and bound algorithms are used to find the optimal solution for combinatory, discrete, and general mathematical optimization problems. A branch and bound algorithm provide an optimal solution to an NP-Hard problem by exploring the entire search space. Through the exploration of the entire search space, a branch and bound algorithm ...
Made as an assignment to demonstrate the use of Branch and Bound Algorithm in IF2211 Algorithm Strategy. ... Branch and bound solver for the travelling salesman problem, written in Python. branch-and-bound travelling-salesman-problem Updated ... A branch and bound solution implemented by my binome Lamdani Wilem as part of an ongoing work for ...
I wrote a code in Python to solve Knapsack problem using branch and bound. I tested it with the case from Rosetta and it outputs correctly. But this is my first time to write this kind of code, I am ... Python Knapsack problem using branch and bound algorithm. Ask Question Asked 8 years, 9 months ago. Modified 4 years, 11 months ago. Viewed 12k ...
The problem involves determining the sequence in which the cities should be visited by a salesperson so that the resulting trip covers the shortest possible distance and each city is visited exactly once. Solution of a traveling salesman problem: the black line shows the shortest possible loop that connects every red dot. Source: Wikipedia.
Made as an assignment to demonstrate the use of Branch and Bound Algorithm in IF2211 Algorithm Strategy ... Maximum Clique Problem solved by Branch and Bound method and Cplex Optimization. ... Star 0. Code Issues Pull requests Branch and bound solver for the travelling salesman problem, written in Python. branch-and-bound travelling-salesman ...
This paper describes what is termed the "generalized assignment problem". It is a generalization of the ordinary assignment problem of linear programming in which multiple assignments of tasks to agents are limited by some resource available to the agents. A branch and bound algorithm is developed that solves the generalized assignment problem by solving a series of binary knapsack ...