8 case studies and real world examples of how Big Data has helped keep on top of competition
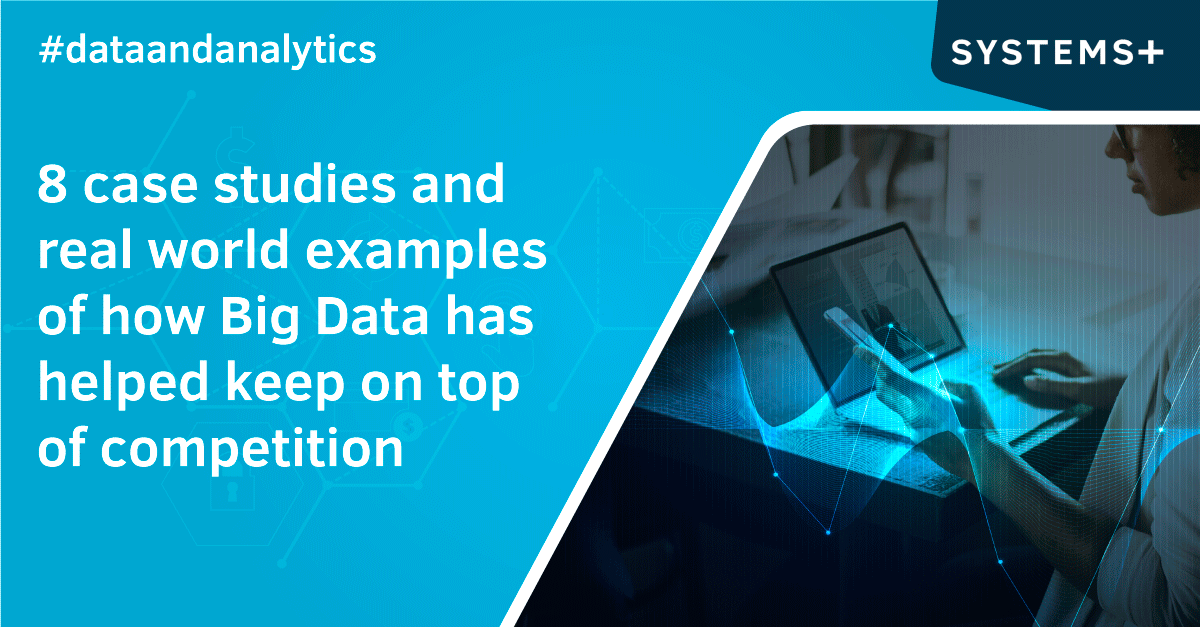
Fast, data-informed decision-making can drive business success. Managing high customer expectations, navigating marketing challenges, and global competition – many organizations look to data analytics and business intelligence for a competitive advantage.
Using data to serve up personalized ads based on browsing history, providing contextual KPI data access for all employees and centralizing data from across the business into one digital ecosystem so processes can be more thoroughly reviewed are all examples of business intelligence.
Organizations invest in data science because it promises to bring competitive advantages.
Data is transforming into an actionable asset, and new tools are using that reality to move the needle with ML. As a result, organizations are on the brink of mobilizing data to not only predict the future but also to increase the likelihood of certain outcomes through prescriptive analytics.
Here are some case studies that show some ways BI is making a difference for companies around the world:
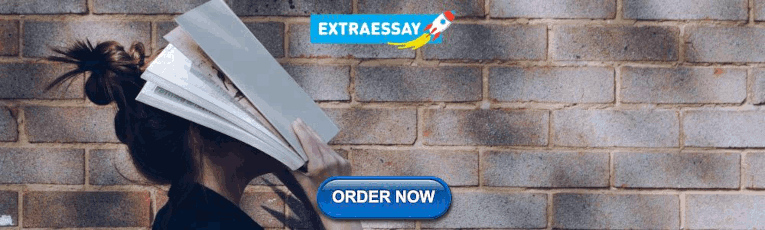
1) Starbucks:
With 90 million transactions a week in 25,000 stores worldwide the coffee giant is in many ways on the cutting edge of using big data and artificial intelligence to help direct marketing, sales and business decisions
Through its popular loyalty card program and mobile application, Starbucks owns individual purchase data from millions of customers. Using this information and BI tools, the company predicts purchases and sends individual offers of what customers will likely prefer via their app and email. This system draws existing customers into its stores more frequently and increases sales volumes.
The same intel that helps Starbucks suggest new products to try also helps the company send personalized offers and discounts that go far beyond a special birthday discount. Additionally, a customized email goes out to any customer who hasn’t visited a Starbucks recently with enticing offers—built from that individual’s purchase history—to re-engage them.
2) Netflix:
The online entertainment company’s 148 million subscribers give it a massive BI advantage.
Netflix has digitized its interactions with its 151 million subscribers. It collects data from each of its users and with the help of data analytics understands the behavior of subscribers and their watching patterns. It then leverages that information to recommend movies and TV shows customized as per the subscriber’s choice and preferences.
As per Netflix, around 80% of the viewer’s activity is triggered by personalized algorithmic recommendations. Where Netflix gains an edge over its peers is that by collecting different data points, it creates detailed profiles of its subscribers which helps them engage with them better.
The recommendation system of Netflix contributes to more than 80% of the content streamed by its subscribers which has helped Netflix earn a whopping one billion via customer retention. Due to this reason, Netflix doesn’t have to invest too much on advertising and marketing their shows. They precisely know an estimate of the people who would be interested in watching a show.
3) Coca-Cola:
Coca Cola is the world’s largest beverage company, with over 500 soft drink brands sold in more than 200 countries. Given the size of its operations, Coca Cola generates a substantial amount of data across its value chain – including sourcing, production, distribution, sales and customer feedback which they can leverage to drive successful business decisions.
Coca Cola has been investing extensively in research and development, especially in AI, to better leverage the mountain of data it collects from customers all around the world. This initiative has helped them better understand consumer trends in terms of price, flavors, packaging, and consumer’ preference for healthier options in certain regions.
With 35 million Twitter followers and a whopping 105 million Facebook fans, Coca-Cola benefits from its social media data. Using AI-powered image-recognition technology, they can track when photographs of its drinks are posted online. This data, paired with the power of BI, gives the company important insights into who is drinking their beverages, where they are and why they mention the brand online. The information helps serve consumers more targeted advertising, which is four times more likely than a regular ad to result in a click.
Coca Cola is increasingly betting on BI, data analytics and AI to drive its strategic business decisions. From its innovative free style fountain machine to finding new ways to engage with customers, Coca Cola is well-equipped to remain at the top of the competition in the future. In a new digital world that is increasingly dynamic, with changing customer behavior, Coca Cola is relying on Big Data to gain and maintain their competitive advantage.
4) American Express GBT
The American Express Global Business Travel company, popularly known as Amex GBT, is an American multinational travel and meetings programs management corporation which operates in over 120 countries and has over 14,000 employees.
Challenges:
Scalability – Creating a single portal for around 945 separate data files from internal and customer systems using the current BI tool would require over 6 months to complete. The earlier tool was used for internal purposes and scaling the solution to such a large population while keeping the costs optimum was a major challenge
Performance – Their existing system had limitations shifting to Cloud. The amount of time and manual effort required was immense
Data Governance – Maintaining user data security and privacy was of utmost importance for Amex GBT
The company was looking to protect and increase its market share by differentiating its core services and was seeking a resource to manage and drive their online travel program capabilities forward. Amex GBT decided to make a strategic investment in creating smart analytics around their booking software.
The solution equipped users to view their travel ROI by categorizing it into three categories cost, time and value. Each category has individual KPIs that are measured to evaluate the performance of a travel plan.
Reducing travel expenses by 30%
Time to Value – Initially it took a week for new users to be on-boarded onto the platform. With Premier Insights that time had now been reduced to a single day and the process had become much simpler and more effective.
Savings on Spends – The product notifies users of any available booking offers that can help them save on their expenditure. It recommends users of possible saving potential such as flight timings, date of the booking, date of travel, etc.
Adoption – Ease of use of the product, quick scale-up, real-time implementation of reports, and interactive dashboards of Premier Insights increased the global online adoption for Amex GBT
5) Airline Solutions Company: BI Accelerates Business Insights
Airline Solutions provides booking tools, revenue management, web, and mobile itinerary tools, as well as other technology, for airlines, hotels and other companies in the travel industry.
Challenge: The travel industry is remarkably dynamic and fast paced. And the airline solution provider’s clients needed advanced tools that could provide real-time data on customer behavior and actions.
They developed an enterprise travel data warehouse (ETDW) to hold its enormous amounts of data. The executive dashboards provide near real-time insights in user-friendly environments with a 360-degree overview of business health, reservations, operational performance and ticketing.
Results: The scalable infrastructure, graphic user interface, data aggregation and ability to work collaboratively have led to more revenue and increased client satisfaction.
6) A specialty US Retail Provider: Leveraging prescriptive analytics
Challenge/Objective: A specialty US Retail provider wanted to modernize its data platform which could help the business make real-time decisions while also leveraging prescriptive analytics. They wanted to discover true value of data being generated from its multiple systems and understand the patterns (both known and unknown) of sales, operations, and omni-channel retail performance.
We helped build a modern data solution that consolidated their data in a data lake and data warehouse, making it easier to extract the value in real-time. We integrated our solution with their OMS, CRM, Google Analytics, Salesforce, and inventory management system. The data was modeled in such a way that it could be fed into Machine Learning algorithms; so that we can leverage this easily in the future.
The customer had visibility into their data from day 1, which is something they had been wanting for some time. In addition to this, they were able to build more reports, dashboards, and charts to understand and interpret the data. In some cases, they were able to get real-time visibility and analysis on instore purchases based on geography!
7) Logistics startup with an objective to become the “Uber of the Trucking Sector” with the help of data analytics
Challenge: A startup specializing in analyzing vehicle and/or driver performance by collecting data from sensors within the vehicle (a.k.a. vehicle telemetry) and Order patterns with an objective to become the “Uber of the Trucking Sector”
Solution: We developed a customized backend of the client’s trucking platform so that they could monetize empty return trips of transporters by creating a marketplace for them. The approach used a combination of AWS Data Lake, AWS microservices, machine learning and analytics.
- Reduced fuel costs
- Optimized Reloads
- More accurate driver / truck schedule planning
- Smarter Routing
- Fewer empty return trips
- Deeper analysis of driver patterns, breaks, routes, etc.
8) Challenge/Objective: A niche segment customer competing against market behemoths looking to become a “Niche Segment Leader”
Solution: We developed a customized analytics platform that can ingest CRM, OMS, Ecommerce, and Inventory data and produce real time and batch driven analytics and AI platform. The approach used a combination of AWS microservices, machine learning and analytics.
- Reduce Customer Churn
- Optimized Order Fulfillment
- More accurate demand schedule planning
- Improve Product Recommendation
- Improved Last Mile Delivery
How can we help you harness the power of data?
At Systems Plus our BI and analytics specialists help you leverage data to understand trends and derive insights by streamlining the searching, merging, and querying of data. From improving your CX and employee performance to predicting new revenue streams, our BI and analytics expertise helps you make data-driven decisions for saving costs and taking your growth to the next level.
Most Popular Blogs
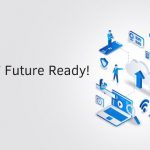
Elevating User Transitions: JML Automation Mastery at Work, Saving Hundreds of Manual Hours
Smooth transition – navigating a seamless servicenow® upgrade, seamless integration excellence: integrating products and platforms with servicenow®.
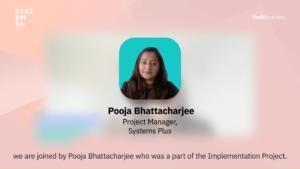
TechEnablers Episode 4: Transforming IT Service Managem
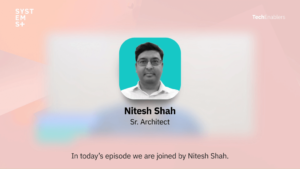
TechEnablers Episode 3: Unlocking Efficiency: Accelerat
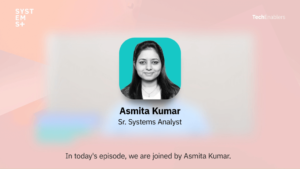
TechEnablers Episode 2: POS Transformation: Achieving S
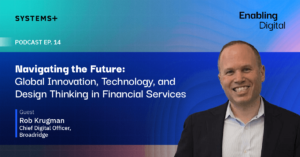
Navigating the Future: Global Innovation, Technology, a
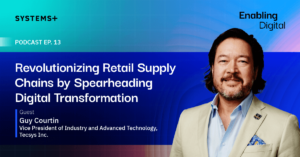
Revolutionizing Retail Supply Chains by Spearheading Di
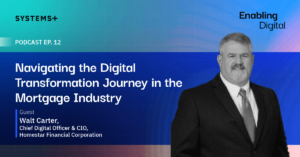
Navigating the Digital Transformation Journey in the Mo
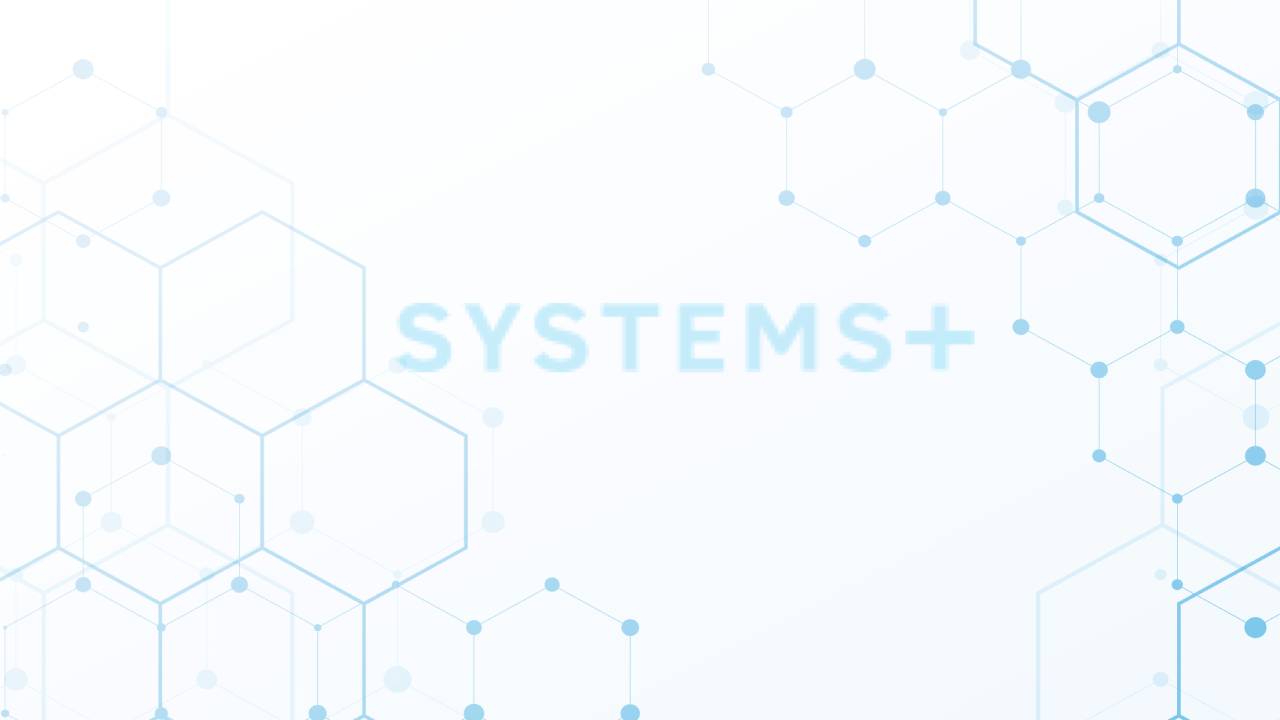
AWS Named as a Leader for the 11th Consecutive Year…
Introducing amazon route 53 application recovery controller, amazon sagemaker named as the outright leader in enterprise mlops….
- Made To Order
- Cloud Solutions
- Salesforce Commerce Cloud
- Distributed Agile
- IT Strategy & Consulting
- Data Warehouse & BI
- Security Assessment & Mitigation
- Case Studies
Quick Links
- Privacy Statement
- +91-22-61591100
- [email protected]
- Data Center
- Applications
- Open Source
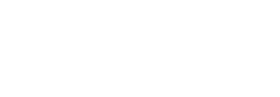
Datamation content and product recommendations are editorially independent. We may make money when you click on links to our partners. Learn More .
A growing number of enterprises are pooling terabytes and petabytes of data, but many of them are grappling with ways to apply their big data as it grows.
How can companies determine what big data solutions will work best for their industry, business model, and specific data science goals?
Check out these big data enterprise case studies from some of the top big data companies and their clients to learn about the types of solutions that exist for big data management.
Enterprise case studies
Netflix on aws, accuweather on microsoft azure, china eastern airlines on oracle cloud, etsy on google cloud, mlogica on sap hana cloud.
Read next: Big Data Market Review 2021
Netflix is one of the largest media and technology enterprises in the world, with thousands of shows that its hosts for streaming as well as its growing media production division. Netflix stores billions of data sets in its systems related to audiovisual data, consumer metrics, and recommendation engines. The company required a solution that would allow it to store, manage, and optimize viewers’ data. As its studio has grown, Netflix also needed a platform that would enable quicker and more efficient collaboration on projects.
“Amazon Kinesis Streams processes multiple terabytes of log data each day. Yet, events show up in our analytics in seconds,” says John Bennett, senior software engineer at Netflix.
“We can discover and respond to issues in real-time, ensuring high availability and a great customer experience.”
Industries: Entertainment, media streaming
Use cases: Computing power, storage scaling, database and analytics management, recommendation engines powered through AI/ML, video transcoding, cloud collaboration space for production, traffic flow processing, scaled email and communication capabilities
- Now using over 100,000 server instances on AWS for different operational functions
- Used AWS to build a studio in the cloud for content production that improves collaborative capabilities
- Produced entire seasons of shows via the cloud during COVID-19 lockdowns
- Scaled and optimized mass email capabilities with Amazon Simple Email Service (Amazon SES)
- Netflix’s Amazon Kinesis Streams-based solution now processes billions of traffic flows daily
Read the full Netflix on AWS case study here .
AccuWeather is one of the oldest and most trusted providers of weather forecast data. The weather company provides an API that other companies can use to embed their weather content into their own systems. AccuWeather wanted to move its data processes to the cloud. However, the traditional GRIB 2 data format for weather data is not supported by most data management platforms. With Microsoft Azure, Azure Data Lake Storage, and Azure Databricks (AI), AccuWeather was able to find a solution that would convert the GRIB 2 data, analyze it in more depth than before, and store this data in a scalable way.
“With some types of severe weather forecasts, it can be a life-or-death scenario,” says Christopher Patti, CTO at AccuWeather.
“With Azure, we’re agile enough to process and deliver severe weather warnings rapidly and offer customers more time to respond, which is important when seconds count and lives are on the line.”
Industries: Media, weather forecasting, professional services
Use cases: Making legacy and traditional data formats usable for AI-powered analysis, API migration to Azure, data lakes for storage, more precise reporting and scaling
- GRIB 2 weather data made operational for AI-powered next-generation forecasting engine, via Azure Databricks
- Delta lake storage layer helps to create data pipelines and more accessibility
- Improved speed, accuracy, and localization of forecasts via machine learning
- Real-time measurement of API key usage and performance
- Ability to extract weather-related data from smart-city systems and self-driving vehicles
Read the full AccuWeather on Microsoft Azure case study here .
China Eastern Airlines is one of the largest airlines in the world that is working to improve safety, efficiency, and overall customer experience through big data analytics. With Oracle’s cloud setup and a large portfolio of analytics tools, it now has access to more in-flight, aircraft, and customer metrics.
“By processing and analyzing over 100 TB of complex daily flight data with Oracle Big Data Appliance, we gained the ability to easily identify and predict potential faults and enhanced flight safety,” says Wang Xuewu, head of China Eastern Airlines’ data lab.
“The solution also helped to cut fuel consumption and increase customer experience.”
Industries: Airline, travel, transportation
Use cases: Increased flight safety and fuel efficiency, reduced operational costs, big data analytics
- Optimized big data analysis to analyze flight angle, take-off speed, and landing speed, maximizing predictive analytics for engine and flight safety
- Multi-dimensional analysis on over 60 attributes provides advanced metrics and recommendations to improve aircraft fuel use
- Advanced spatial analytics on the travelers’ experience, with metrics covering in-flight cabin service, baggage, ground service, marketing, flight operation, website, and call center
- Using Oracle Big Data Appliance to integrate Hadoop data from aircraft sensors, unifying and simplifying the process for evaluating device health across an aircraft
- Central interface for daily management of real-time flight data
Read the full China Eastern Airlines on Oracle Cloud case study here .
Etsy is an e-commerce site for independent artisan sellers. With its goal to create a buying and selling space that puts the individual first, Etsy wanted to advance its platform to the cloud to keep up with needed innovations. But it didn’t want to lose the personal touches or values that drew customers in the first place. Etsy chose Google for cloud migration and big data management for several primary reasons: Google’s advanced features that back scalability, its commitment to sustainability, and the collaborative spirit of the Google team.
Mike Fisher, CTO at Etsy, explains how Google’s problem-solving approach won them over.
“We found that Google would come into meetings, pull their chairs up, meet us halfway, and say, ‘We don’t do that, but let’s figure out a way that we can do that for you.'”
Industries: Retail, E-commerce
Use cases: Data center migration to the cloud, accessing collaboration tools, leveraging machine learning (ML) and artificial intelligence (AI), sustainability efforts
- 5.5 petabytes of data migrated from existing data center to Google Cloud
- >50% savings in compute energy, minimizing total carbon footprint and energy usage
- 42% reduced compute costs and improved cost predictability through virtual machine (VM), solid state drive (SSD), and storage optimizations
- Democratization of cost data for Etsy engineers
- 15% of Etsy engineers moved from system infrastructure management to customer experience, search, and recommendation optimization
Read the full Etsy on Google Cloud case study here .
mLogica is a technology and product consulting firm that wanted to move to the cloud, in order to better support its customers’ big data storage and analytics needs. Although it held on to its existing data analytics platform, CAP*M, mLogica relied on SAP HANA Cloud to move from on-premises infrastructure to a more scalable cloud structure.
“More and more of our clients are moving to the cloud, and our solutions need to keep pace with this trend,” says Michael Kane, VP of strategic alliances and marketing, mLogica
“With CAP*M on SAP HANA Cloud, we can future-proof clients’ data setups.”
Industry: Professional services
Use cases: Manage growing pools of data from multiple client accounts, improve slow upload speeds for customers, move to the cloud to avoid maintenance of on-premises infrastructure, integrate the company’s existing big data analytics platform into the cloud
- SAP HANA Cloud launched as the cloud platform for CAP*M, mLogica’s big data analytics tool, to improve scalability
- Data analysis now enabled on a petabyte scale
- Simplified database administration and eliminated additional hardware and maintenance needs
- Increased control over total cost of ownership
- Migrated existing customer data setups through SAP IQ into SAP HANA, without having to adjust those setups for a successful migration
Read the full mLogica on SAP HANA Cloud case study here .
Read next: Big Data Trends in 2021 and The Future of Big Data
Subscribe to Data Insider
Learn the latest news and best practices about data science, big data analytics, artificial intelligence, data security, and more.
Similar articles
76 top saas companies to know in 2024, 12 top data mining certifications of 2024, state of observability: surveys show 84% of companies struggle with costs, complexity, tools, get the free newsletter.
Subscribe to Data Insider for top news, trends & analysis
Latest Articles
76 top saas companies..., 12 top data mining..., 10 top storage certifications..., state of observability: surveys....
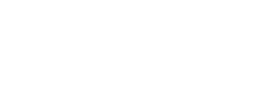
A new initiative at UPS will use real-time data, advanced analytics and artificial intelligence to help employees make better decisions.
As chief information and engineering officer for logistics giant UPS, Juan Perez is placing analytics and insight at the heart of business operations.
Big data and digital transformation: How one enables the other
Drowning in data is not the same as big data. Here's the true definition of big data and a powerful example of how it's being used to power digital transformation.
"Big data at UPS takes many forms because of all the types of information we collect," he says. "We're excited about the opportunity of using big data to solve practical business problems. We've already had some good experience of using data and analytics and we're very keen to do more."
Perez says UPS is using technology to improve its flexibility, capability, and efficiency, and that the right insight at the right time helps line-of-business managers to improve performance.
The aim for UPS, says Perez, is to use the data it collects to optimise processes, to enable automation and autonomy, and to continue to learn how to improve its global delivery network.
Leading data-fed projects that change the business for the better
Perez says one of his firm's key initiatives, known as Network Planning Tools, will help UPS to optimise its logistics network through the effective use of data. The system will use real-time data, advanced analytics and artificial intelligence to help employees make better decisions. The company expects to begin rolling out the initiative from the first quarter of 2018.
"That will help all our business units to make smart use of our assets and it's just one key project that's being supported in the organisation as part of the smart logistics network," says Perez, who also points to related and continuing developments in Orion (On-road Integrated Optimization and Navigation), which is the firm's fleet management system.
Orion uses telematics and advanced algorithms to create optimal routes for delivery drivers. The IT team is currently working on the third version of the technology, and Perez says this latest update to Orion will provide two key benefits to UPS.
First, the technology will include higher levels of route optimisation which will be sent as navigation advice to delivery drivers. "That will help to boost efficiency," says Perez.
Second, Orion will use big data to optimise delivery routes dynamically.
"Today, Orion creates delivery routes before drivers leave the facility and they stay with that static route throughout the day," he says. "In the future, our system will continually look at the work that's been completed, and that still needs to be completed, and will then dynamically optimise the route as drivers complete their deliveries. That approach will ensure we meet our service commitments and reduce overall delivery miles."
Once Orion is fully operational for more than 55,000 drivers this year, it will lead to a reduction of about 100 million delivery miles -- and 100,000 metric tons of carbon emissions. Perez says these reductions represent a key measure of business efficiency and effectiveness, particularly in terms of sustainability.
Projects such as Orion and Network Planning Tools form part of a collective of initiatives that UPS is using to improve decision making across the package delivery network. The firm, for example, recently launched the third iteration of its chatbot that uses artificial intelligence to help customers find rates and tracking information across a series of platforms, including Facebook and Amazon Echo.
"That project will continue to evolve, as will all our innovations across the smart logistics network," says Perez. "Everything runs well today but we also recognise there are opportunities for continuous improvement."
Overcoming business challenges to make the most of big data
"Big data is all about the business case -- how effective are we as an IT team in defining a good business case, which includes how to improve our service to our customers, what is the return on investment and how will the use of data improve other aspects of the business," says Perez.
These alternative use cases are not always at the forefront of executive thinking. Consultant McKinsey says too many organisations drill down on a single data set in isolation and fail to consider what different data sets mean for other parts of the business.
However, Perez says the re-use of information can have a significant impact at UPS. Perez talks, for example, about using delivery data to help understand what types of distribution solutions work better in different geographical locations.
"Should we have more access points? Should we introduce lockers? Should we allow drivers to release shipments without signatures? Data, technology, and analytics will improve our ability to answer those questions in individual locations -- and those benefits can come from using the information we collect from our customers in a different way," says Perez.
Perez says this fresh, open approach creates new opportunities for other data-savvy CIOs. "The conversation in the past used to be about buying technology, creating a data repository and discovering information," he says. "Now the conversation is changing and it's exciting. Every time we talk about a new project, the start of the conversation includes data."
By way of an example, Perez says senior individuals across the organisation now talk as a matter of course about the potential use of data in their line-of-business and how that application of insight might be related to other models across the organisation.
These senior executive, he says, also ask about the availability of information and whether the existence of data in other parts of the business will allow the firm to avoid a duplication of effort.
"The conversation about data is now much more active," says Perez. "That higher level of collaboration provides benefits for everyone because the awareness across the organisation means we'll have better repositories, less duplication and much more effective data models for new business cases in the future."
Read more about big data
- Turning big data into business insights: The state of play
- Choosing the best big data partners: Eight questions to ask
- Report shows that AI is more important to IoT than big data insights
5 ways CIOs can manage the business demand for generative AI
3 ways to accelerate generative ai implementation and optimization, 5 ways to build a great network, according to business leaders.
How companies are using big data and analytics
Few dispute that organizations have more data than ever at their disposal. But actually deriving meaningful insights from that data—and converting knowledge into action—is easier said than done. We spoke with six senior leaders from major organizations and asked them about the challenges and opportunities involved in adopting advanced analytics: Murli Buluswar, chief science officer at AIG; Vince Campisi, chief information officer at GE Software; Ash Gupta, chief risk officer at American Express; Zoher Karu, vice president of global customer optimization and data at eBay; Victor Nilson, senior vice president of big data at AT&T; and Ruben Sigala, chief analytics officer at Caesars Entertainment. An edited transcript of their comments follows.
Interview transcript
Challenges organizations face in adopting analytics.
Murli Buluswar, chief science officer, AIG: The biggest challenge of making the evolution from a knowing culture to a learning culture—from a culture that largely depends on heuristics in decision making to a culture that is much more objective and data driven and embraces the power of data and technology—is really not the cost. Initially, it largely ends up being imagination and inertia.
What I have learned in my last few years is that the power of fear is quite tremendous in evolving oneself to think and act differently today, and to ask questions today that we weren’t asking about our roles before. And it’s that mind-set change—from an expert-based mind-set to one that is much more dynamic and much more learning oriented, as opposed to a fixed mind-set—that I think is fundamental to the sustainable health of any company, large, small, or medium.
Ruben Sigala, chief analytics officer, Caesars Entertainment: What we found challenging, and what I find in my discussions with a lot of my counterparts that is still a challenge, is finding the set of tools that enable organizations to efficiently generate value through the process. I hear about individual wins in certain applications, but having a more sort of cohesive ecosystem in which this is fully integrated is something that I think we are all struggling with, in part because it’s still very early days. Although we’ve been talking about it seemingly quite a bit over the past few years, the technology is still changing; the sources are still evolving.
Zoher Karu, vice president, global customer optimization and data, eBay: One of the biggest challenges is around data privacy and what is shared versus what is not shared. And my perspective on that is consumers are willing to share if there’s value returned. One-way sharing is not going to fly anymore. So how do we protect and how do we harness that information and become a partner with our consumers rather than kind of just a vendor for them?
Capturing impact from analytics
Ruben Sigala: You have to start with the charter of the organization. You have to be very specific about the aim of the function within the organization and how it’s intended to interact with the broader business. There are some organizations that start with a fairly focused view around support on traditional functions like marketing, pricing, and other specific areas. And then there are other organizations that take a much broader view of the business. I think you have to define that element first.
That helps best inform the appropriate structure, the forums, and then ultimately it sets the more granular levels of operation such as training, recruitment, and so forth. But alignment around how you’re going to drive the business and the way you’re going to interact with the broader organization is absolutely critical. From there, everything else should fall in line. That’s how we started with our path.
Vince Campisi, chief information officer, GE Software: One of the things we’ve learned is when we start and focus on an outcome, it’s a great way to deliver value quickly and get people excited about the opportunity. And it’s taken us to places we haven’t expected to go before. So we may go after a particular outcome and try and organize a data set to accomplish that outcome. Once you do that, people start to bring other sources of data and other things that they want to connect. And it really takes you in a place where you go after a next outcome that you didn’t anticipate going after before. You have to be willing to be a little agile and fluid in how you think about things. But if you start with one outcome and deliver it, you’ll be surprised as to where it takes you next.

The need to lead in data and analytics
Ash Gupta, chief risk officer, American Express: The first change we had to make was just to make our data of higher quality. We have a lot of data, and sometimes we just weren’t using that data and we weren’t paying as much attention to its quality as we now need to. That was, one, to make sure that the data has the right lineage, that the data has the right permissible purpose to serve the customers. This, in my mind, is a journey. We made good progress and we expect to continue to make this progress across our system.
The second area is working with our people and making certain that we are centralizing some aspects of our business. We are centralizing our capabilities and we are democratizing its use. I think the other aspect is that we recognize as a team and as a company that we ourselves do not have sufficient skills, and we require collaboration across all sorts of entities outside of American Express. This collaboration comes from technology innovators, it comes from data providers, it comes from analytical companies. We need to put a full package together for our business colleagues and partners so that it’s a convincing argument that we are developing things together, that we are colearning, and that we are building on top of each other.
Examples of impact
Victor Nilson, senior vice president, big data, AT&T: We always start with the customer experience. That’s what matters most. In our customer care centers now, we have a large number of very complex products. Even the simple products sometimes have very complex potential problems or solutions, so the workflow is very complex. So how do we simplify the process for both the customer-care agent and the customer at the same time, whenever there’s an interaction?
We’ve used big data techniques to analyze all the different permutations to augment that experience to more quickly resolve or enhance a particular situation. We take the complexity out and turn it into something simple and actionable. Simultaneously, we can then analyze that data and then go back and say, “Are we optimizing the network proactively in this particular case?” So, we take the optimization not only for the customer care but also for the network, and then tie that together as well.
Vince Campisi: I’ll give you one internal perspective and one external perspective. One is we are doing a lot in what we call enabling a digital thread—how you can connect innovation through engineering, manufacturing, and all the way out to servicing a product. [For more on the company’s “digital thread” approach, see “ GE’s Jeff Immelt on digitizing in the industrial space .”] And, within that, we’ve got a focus around brilliant factory. So, take driving supply-chain optimization as an example. We’ve been able to take over 60 different silos of information related to direct-material purchasing, leverage analytics to look at new relationships, and use machine learning to identify tremendous amounts of efficiency in how we procure direct materials that go into our product.
An external example is how we leverage analytics to really make assets perform better. We call it asset performance management. And we’re starting to enable digital industries, like a digital wind farm, where you can leverage analytics to help the machines optimize themselves. So you can help a power-generating provider who uses the same wind that’s come through and, by having the turbines pitch themselves properly and understand how they can optimize that level of wind, we’ve demonstrated the ability to produce up to 10 percent more production of energy off the same amount of wind. It’s an example of using analytics to help a customer generate more yield and more productivity out of their existing capital investment.
Winning the talent war
Ruben Sigala: Competition for analytical talent is extreme. And preserving and maintaining a base of talent within an organization is difficult, particularly if you view this as a core competency. What we’ve focused on mostly is developing a platform that speaks to what we think is a value proposition that is important to the individuals who are looking to begin a career or to sustain a career within this field.
When we talk about the value proposition, we use terms like having an opportunity to truly affect the outcomes of the business, to have a wide range of analytical exercises that you’ll be challenged with on a regular basis. But, by and large, to be part of an organization that views this as a critical part of how it competes in the marketplace—and then to execute against that regularly. In part, and to do that well, you have to have good training programs, you have to have very specific forms of interaction with the senior team. And you also have to be a part of the organization that actually drives the strategy for the company.
Murli Buluswar: I have found that focusing on the fundamentals of why science was created, what our aspirations are, and how being part of this team will shape the professional evolution of the team members has been pretty profound in attracting the caliber of talent that we care about. And then, of course, comes the even harder part of living that promise on a day-in, day-out basis.
Yes, money is important. My philosophy on money is I want to be in the 75th percentile range; I don’t want to be in the 99th percentile. Because no matter where you are, most people—especially people in the data-science function—have the ability to get a 20 to 30 percent increase in their compensation, should they choose to make a move. My intent is not to try and reduce that gap. My intent is to create an environment and a culture where they see that they’re learning; they see that they’re working on problems that have a broader impact on the company, on the industry, and, through that, on society; and they’re part of a vibrant team that is inspired by why it exists and how it defines success. Focusing on that, to me, is an absolutely critical enabler to attracting the caliber of talent that I need and, for that matter, anyone else would need.
Developing the right expertise
Victor Nilson: Talent is everything, right? You have to have the data, and, clearly, AT&T has a rich wealth of data. But without talent, it’s meaningless. Talent is the differentiator. The right talent will go find the right technologies; the right talent will go solve the problems out there.
We’ve helped contribute in part to the development of many of the new technologies that are emerging in the open-source community. We have the legacy advanced techniques from the labs, we have the emerging Silicon Valley. But we also have mainstream talent across the country, where we have very advanced engineers, we have managers of all levels, and we want to develop their talent even further.
So we’ve delivered over 50,000 big data related training courses just this year alone. And we’re continuing to move forward on that. It’s a whole continuum. It might be just a one-week boot camp, or it might be advanced, PhD-level data science. But we want to continue to develop that talent for those who have the aptitude and interest in it. We want to make sure that they can develop their skills and then tie that together with the tools to maximize their productivity.
Zoher Karu: Talent is critical along any data and analytics journey. And analytics talent by itself is no longer sufficient, in my opinion. We cannot have people with singular skills. And the way I build out my organization is I look for people with a major and a minor. You can major in analytics, but you can minor in marketing strategy. Because if you don’t have a minor, how are you going to communicate with other parts of the organization? Otherwise, the pure data scientist will not be able to talk to the database administrator, who will not be able to talk to the market-research person, who which will not be able to talk to the email-channel owner, for example. You need to make sound business decisions, based on analytics, that can scale.
Murli Buluswar is chief science officer at AIG, Vince Campisi is chief information officer at GE Software, Ash Gupta is chief risk officer at American Express, Zoher Karu is vice president of global customer optimization and data at eBay, Victor Nilson is senior vice president of big data at AT&T, and Ruben Sigala is chief analytics officer at Caesars Entertainment.
Explore a career with us
Related articles.

Big data: Getting a better read on performance
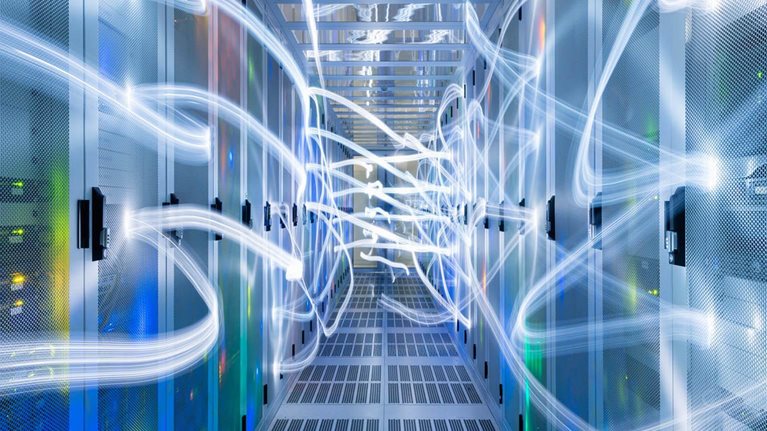
Transforming into an analytics-driven insurance carrier
Big Data Case Studies
- First Online: 13 June 2018
Cite this chapter
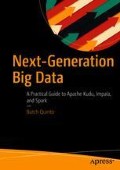
- Butch Quinto 2
2800 Accesses
Big data has disrupted entire industries. Innovative use case in the fields of financial services, telecommunications, transportation, health care, retail, insurance, utilities, energy, and technology (to mention a few) have revolutionized the way organizations manage, process, and analyze data.
- Apache Spark
- Cloudera Manager
- Hadoop Components
- Apache Hive
These keywords were added by machine and not by the authors. This process is experimental and the keywords may be updated as the learning algorithm improves.
This is a preview of subscription content, log in via an institution to check access.
Access this chapter
- Available as PDF
- Read on any device
- Instant download
- Own it forever
- Available as EPUB and PDF
- Compact, lightweight edition
- Dispatched in 3 to 5 business days
- Free shipping worldwide - see info
Tax calculation will be finalised at checkout
Purchases are for personal use only
Institutional subscriptions
Author information
Authors and affiliations.
Plumpton, Victoria, Australia
Butch Quinto
You can also search for this author in PubMed Google Scholar
Rights and permissions
Reprints and permissions
Copyright information
© 2018 Butch Quinto
About this chapter
Quinto, B. (2018). Big Data Case Studies. In: Next-Generation Big Data. Apress, Berkeley, CA. https://doi.org/10.1007/978-1-4842-3147-0_13
Download citation
DOI : https://doi.org/10.1007/978-1-4842-3147-0_13
Published : 13 June 2018
Publisher Name : Apress, Berkeley, CA
Print ISBN : 978-1-4842-3146-3
Online ISBN : 978-1-4842-3147-0
eBook Packages : Professional and Applied Computing Professional and Applied Computing (R0) Apress Access Books
Share this chapter
Anyone you share the following link with will be able to read this content:
Sorry, a shareable link is not currently available for this article.
Provided by the Springer Nature SharedIt content-sharing initiative
- Publish with us
Policies and ethics
- Find a journal
- Track your research

Award winner: Big Data Strategy of Procter & Gamble

This case won the Knowledge, Information and Communication Systems Management category at The Case Centre Awards and Competitions 2020 . #CaseAwards2020
Author perspective
Who – the protagonist.
Linda W. Clement-Holmes , Procter & Gamble (P&G) Chief Information Officer (CIO).
P&G is a leading consumer packaged goods company, regarded as a pioneer in extensively adopting big data and digitization to understand consumer behaviour.

Former Chairman and CEO, Bob McDonald , and CIO, Filippio Passerini , were responsible for the push on big data, which had resulted in P&G becoming more nimble and efficient.
However, some experts were sceptical about P&G’s obsession with digitization, and how it could slow the speed of decision making.
It was in June 2015 when Linda replaced Filippio.
P&G is headquartered in Cincinnati, Ohio, but its brands are sold worldwide.
“ Change movement is one of the biggest challenges of big data implementation. Analytics need to be integrated with processes. We had to educate and train our field force over and over again in order to make analytics a part of their daily routine.” A head of analytics at a leading logistics company
Linda had the big responsibility of continuing and leveraging the big data initiatives started by Filippio.
In order to achieve this, a culture of data-driven decision making within the organisation needed to be implemented by the leadership team.
Linda’s job was to convince them of her vision.
AUTHOR PERSPECTIVE
Vinod said: “I am extremely honoured to receive such a prestigious award from The Case Centre, popularly dubbed the Case Method Oscars!
“I am earnestly grateful for the recognition I have received for my effort which would not have been possible without the guidance and support of my Dean, Debapratim Purkayastha, who gave me an opportunity to associate with him in writing this case.”
Predicting the future
Debapratim commented: “Big data analytics has always been a key strategy for businesses to have a competitive edge and achieve their goals. Now, predictive analysis through big data can help predict what may occur in the future.

“The topic is very contemporary to current business trends and the case helps the students to be updated with the organisational readiness to welcome latest changes in technology for better performance. The case discusses in detail how Procter & Gamble adapted the big data through different tools like Decision Cockpit and Business Sphere.”
Vinod commented: “The case helps understands many strategic, as well as technical aspects of big data and business analytics, and how they are implemented in a fast-moving consumer goods (FMCG) like Procter & Gamble.
“Not only does it help understand the opportunities and challenges in implementing a big data strategy, but also the significance of accessibility to information in an organisation and how its functioning can be transformed through the availability of real-time data.
“The case enables a discussion on ways in which big data could be productively employed in an organisation in some of the key business functions.”
Debapratim added: "Educators may like using our other case, Consumer Research at Procter & Gamble: From Field Research to Agile Research , as a follow-up, as it shows how the pioneers of marketing research is now leveraging big data for agile research.
Identifying the right information
Debapratim explained: “Understanding of the concepts that are going to be taught through the case study is a prerequisite of writing a case. Finding the relevant information, and presenting the case in an understandable manner to students is also equally important.
"Most importantly, people new to case writing should work with more experienced case writers to hone their skills in case writing.”
The authors

Celebrating the win
Unfortunately, due to the Coronavirus pandemic, we were unable to present the authors in person with their trophies for winning the Knowledge, Information and Communication Systems Management category in 2020.
We are delighted to celebrate Debapratim and Vinod's win by sharing these pictures of them with their awards - congratulations!

The protagonist

Educators can login to view a free educator preview copy of this case and its teaching note.
View all the 2020 winners
Stay in touch with all the latest case news and views in our free newsletter, Connect .
Read it online or sign up to have it delivered direct to your inbox!

Discover more

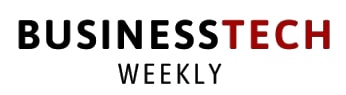
Big Data Use Case: How Amazon uses Big Data to drive eCommerce revenue
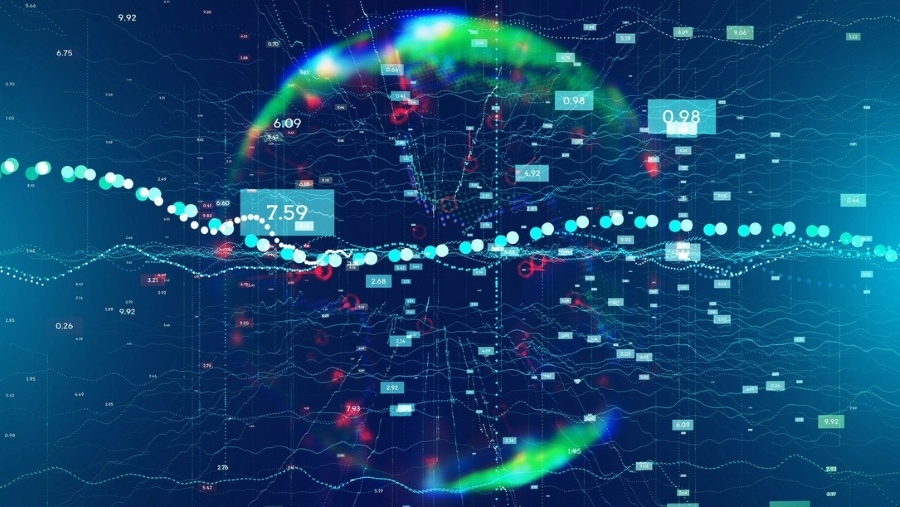
Amazon is no stranger to big data. In this big data use case, we’ll look at how Amazon is leveraging data analytic technologies to improve products and services and drive overall revenue.
Big data has changed how we interact with the world and continue strengthening its hold on businesses worldwide. New data sets can be mined, managed, and analyzed using a combination of technologies.
These applications leverage the fallacy-prone human brain with computers. If you can think of applications for machine learning to predict things, optimize systems/processes, or automatically sequence tasks – this is relevant to big data.
Amazon’s algorithm is another secret to its success. The online shop has not only made it possible to order products with just one mouse click, but it also uses personalization data combined with big data to achieve excellent conversion rates.
On this page:
Amazon and Big data
Amazon’s big data strategy, amazon collection of data and its use, big data use case: the key points.
The fascinating world of Big Data can help you gain a competitive edge over your competitors. The data collected by networks of sensors, smart meters, and other means can provide insights into customer spending behavior and help retailers better target their services and products.
RELATED: Big Data Basics: Understanding Big Data
Machine Learning (a type of artificial intelligence) processes data through a learning algorithm to spot trends and patterns while continually refining the algorithms.
Amazon is one of the world’s largest businesses, estimated to have over 310 million active customers worldwide. They recently accomplished transactions that reached a value of $90 billion. This shows the popularity of online shopping on different continents. They provide services like payments, shipping, and new ideas for their customers.
Amazon is a giant – it has its own clouds. Amazon Web Services (AWS) offers individuals, companies, and governments cloud computing platforms . Amazon became interested in cloud computing after its Amazon Web Services was launched in 2003.
Amazon Web Services has expanded its business lines since then. Amazon hired some brilliant minds in the field of analytics and predictive modeling to aid in further data mining of Amazon’s massive volume of data that it has accumulated. Amazon innovates by introducing new products and strategies based on customer experience and feedback.
Big Data has assisted Amazon in ascending to the top of the e-commerce heap.
Amazon uses an anticipatory delivery model that predicts the products most likely to be purchased by its customers based on vast amounts of data.
This leads to Amazon assessing your purchase pattern and shipping things to your closest warehouse, which you may use in the future.
Amazon stores and processes as much customer and product information as possible – collecting specific information on every customer who visits its website. It also monitors the products a customer views, their shipping address, and whether or not they post reviews.
Amazon optimizes the prices on its websites by considering other factors, such as user activity, order history, rival prices, product availability, etc., providing discounts on popular items and earning a profit on less popular things using this strategy. This is how Amazon utilizes big data in its business operations.
Data science has established its preeminent place in industries and contributed to industries’ growth and improvement.
RELATED: How Artificial Intelligence Is Used for Data Analytics
Ever wonder how Amazon knows what you want before you even order it? The answer is mathematics, but you know that.
You may not know that the company has been running a data-gathering program for almost 15 years now that reaches back to the site’s earliest days.
In the quest to make every single interaction between buyers and sellers as efficient as possible, getting down to the most minute levels of detail has been essential, with data collection coming from a variety of sources – from sellers themselves and customers with apps on their phones – giving Amazon insights into every step along the way.
Voice recording by Alexa
Alexa is a speech interaction service developed by Amazon.com. It uses a cloud-based service to create voice-controlled smart devices. Through voice commands, Alexa can respond to queries, play music, read the news, and manage smart home devices such as lights and appliances.
Users may subscribe to an Alexa Voice Service (AVS) or use AWS Lambda to embed the system into other hardware and software.
You can spend all day with your microphone, smartphone, or barcode scanner recording every interaction, receipt, and voice note. But you don’t have to with tools like Amazon Echo.
With its always-on Alexa Voice Service, say what you need to add to your shopping list when you need it. It’s fast and straightforward.
Single click order
There is a big competition between companies using big data. Using big data, Amazon realized that customers might prefer alternative vendors if they experience a delay in their orders. So, Amazon has created Single click ordering.
You need to mention the address and payment method by this method. Every customer is given a time of 30 minutes to decide whether to place the order or not. After that, it is automatically determined.
Persuade Customers
Persuasive technology is a new area at Amazon. It’s an intersection of AI, UX, and the business goal of getting customers to take action at any point in the shopping journey.
One of the most significant ways Amazon utilizes data is through its recommendation engine. When a client searches for a specific item, Amazon can better anticipate other items the buyer may be interested in.
Consequently, Amazon can expedite the process of convincing a buyer to purchase the product. It is estimated that its personalized recommendation system accounts for 35 percent of the company’s annual sales.
The Amazon Assistant helps you discover new and exciting products, browse best sellers, and shop by department—there’s no place on the web with a better selection of stuff. Plus, it automatically notifies you when price drops or items you’ve been watching get marked down, so customers get the best deal possible.
Price dropping
Amazon constantly changes the price of its products by using Big data trends. On many competitor sites, the product’s price remains the same.
But Amazon has created another way to attract customers by constantly changing the price of the products. Amazon continually updates prices to deliver you the best deals.
Customers now check the site constantly that the price of the product they want can be low at any time, and they can buy it easily.
Shipping optimization
Shipping optimization by Amazon allows you to choose your preferred carrier, service options, and expected delivery time for millions of items on Amazon.com. With Shipping optimization by Amazon, you can end surprises like unexpected carrier selection, unnecessary service fees, or delays that can happen with even standard shipping.
Today, Amazon offers customers the choice to pick up their packages at over 400 U.S. locations. Whether you need one-day delivery or same-day pickup in select metro areas, Prime members can choose how fast they want to get their goods in an easy-to-use mobile app.
RELATED: Amazon Supply Chain: Understanding how Amazon’s supply chain works
Using shipping partners makes this selection possible, allowing Amazon to offer the most comprehensive selection in the industry and provide customers with multiple options for picking up their orders.
To better serve the customer, Amazon has adopted a technology that allows them to receive information from shoppers’ web browsing habits and use it to improve existing products and introduce new ones.
Amazon is only one example of a corporation that uses big data. Airbnb is another industry leader that employs big data in its operations; you can also review their case study. Below are four ways big data plays a significant role in every organization.
1. Helps you understand the market condition: Big Data assists you in comprehending market circumstances, trends, and wants, as well as your competitors, through data analysis.
It helps you to research customer interests and behaviors so that you may adjust your products and services to their requirements.
2. It helps you increase customer satisfaction: Using big data analytics, you may determine the demographics of your target audience, the products and services they want, and much more.
This information enables you to design business plans and strategies with the needs and demands of customers in mind. Customer satisfaction will grow immediately if your business strategy is based on consumer requirements.
3. Increase sales: Once you thoroughly understand the market environment and client needs, you can develop products, services, and marketing tactics accordingly. This helps you dramatically enhance your sales.
4. Optimize costs: By analyzing the data acquired from client databases, services, and internet resources, you may determine what prices benefit customers, how cost increases or decreases will impact your business, etc.
You can determine the optimal price for your items and services, which will benefit your customers and your company.
Businesses need to adapt to the ever-changing needs of their customers. Within this dynamic online marketplace, competitive advantage is often gained by those players who can adapt to market changes faster than others. Big data analytics provides that advantage.
RELATED: Top 5 Big Data Privacy Issues Businesses Must Consider
However, the sheer volume of data generated at all levels — from individual consumer click streams to the aggregate public opinions of millions of individuals — provides a considerable barrier to companies that would like to customize their offerings or efficiently interact with customers.
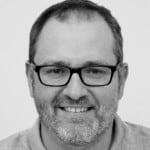
James joined BusinessTechWeekly.com in 2018, following a 19-year career in IT where he covered a wide range of support, management and consultancy roles across a wide variety of industry sectors. He has a broad technical knowledge base backed with an impressive list of technical certifications. with a focus on applications, cloud and infrastructure.
Customer Journey Mapping: The Foundation of a Strong Omnichannel Strategy
Top CISO Interview Questions and Tips for Success
The Significance of Endpoint Security in Today’s World
Thin Clients: What is a Thin Client and How are they Used?
HRTech Tools to Boost Employee Engagement & Retention
9 Effective Ecommerce Marketing Strategies
Data and Analytics Case Study
Made possible by ey, exclusive global insights case study sponsor.
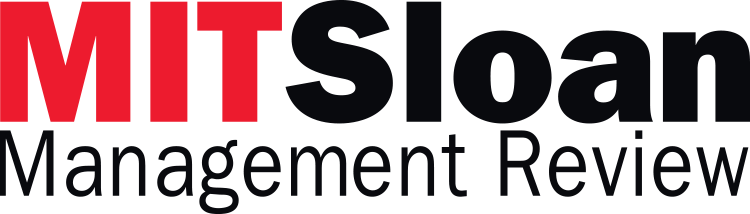
GE’s Big Bet on Data and Analytics
Seeking opportunities in the internet of things, ge expands into industrial analytics., february 18, 2016, by: laura winig.
If software experts truly knew what Jeff Immelt and GE Digital were doing, there’s no other software company on the planet where they would rather be. –Bill Ruh, CEO of GE Digital and CDO for GE
In September 2015, multinational conglomerate General Electric (GE) launched an ad campaign featuring a recent college graduate, Owen, excitedly breaking the news to his parents and friends that he has just landed a computer programming job — with GE. Owen tries to tell them that he will be writing code to help machines communicate, but they’re puzzled; after all, GE isn’t exactly known for its software. In one ad, his friends feign excitement, while in another, his father implies Owen may not be macho enough to work at the storied industrial manufacturing company.
Owen's Hammer
Ge's ad campaign aimed at millennials emphasizes its new digital direction..
The campaign was designed to recruit Millennials to join GE as Industrial Internet developers and remind them — using GE’s new watchwords, “The digital company. That’s also an industrial company.” — of GE’s massive digital transformation effort. GE has bet big on the Industrial Internet — the convergence of industrial machines, data, and the Internet (also referred to as the Internet of Things) — committing $1 billion to put sensors on gas turbines, jet engines, and other machines; connect them to the cloud; and analyze the resulting flow of data to identify ways to improve machine productivity and reliability. “GE has made significant investment in the Industrial Internet,” says Matthias Heilmann, Chief Digital Officer of GE Oil & Gas Digital Solutions. “It signals this is real, this is our future.”
While many software companies like SAP, Oracle, and Microsoft have traditionally been focused on providing technology for the back office, GE is leading the development of a new breed of operational technology (OT) that literally sits on top of industrial machinery.
About the Author
Laura Winig is a contributing editor to MIT Sloan Management Review .
1. Predix is a trademark of General Electric Company.
2. M. LaWell, “Building the Industrial Internet With GE,” IndustryWeek, October 5, 2015.
3. D. Floyer, “Defining and Sizing the Industrial Internet,” June 27, 2013, http://wikibon.org.
i. S. Higginbotham, “BP Teams Up With GE to Make Its Oil Wells Smart,” Fortune, July 8, 2015.
More Like This
Add a comment cancel reply.
You must sign in to post a comment. First time here? Sign up for a free account : Comment on articles and get access to many more articles.
Comment (1)

An official website of the United States government
The .gov means it’s official. Federal government websites often end in .gov or .mil. Before sharing sensitive information, make sure you’re on a federal government site.
The site is secure. The https:// ensures that you are connecting to the official website and that any information you provide is encrypted and transmitted securely.
- Publications
- Account settings
Preview improvements coming to the PMC website in October 2024. Learn More or Try it out now .
- Advanced Search
- Journal List
- AMIA Annu Symp Proc
- v.2017; 2017
Big data in healthcare – the promises, challenges and opportunities from a research perspective: A case study with a model database
Mohammad adibuzzaman.
1 Regenstrief Center for Healthcare Engineering, Purdue University, West Lafayette, Indiana, USA
Poching DeLaurentis
Jennifer hill, brian d. benneyworth.
2 Children’s Health Services Research Group, Department of Pediatrics, Indiana University School of Medicine, Indianapolis, USA
Recent advances in data collection during routine health care in the form of Electronic Health Records (EHR), medical device data (e.g., infusion pump informatics, physiological monitoring data, and insurance claims data, among others, as well as biological and experimental data, have created tremendous opportunities for biological discoveries for clinical application. However, even with all the advancement in technologies and their promises for discoveries, very few research findings have been translated to clinical knowledge, or more importantly, to clinical practice. In this paper, we identify and present the initial work addressing the relevant challenges in three broad categories: data, accessibility, and translation. These issues are discussed in the context of a widely used detailed database from an intensive care unit, Medical Information Mart for Intensive Care (MIMIC III) database.
1. Introduction
The promise of big data has brought great hope in health care research for drug discovery, treatment innovation, personalized medicine, and optimal patient care that can reduce cost and improve patient outcomes. Billions of dollars have been invested to capture large amounts of data outlined in big initiatives that are often isolated. The National Institutes of Health (NIH) recently announced the All of Us initiative, previously known as the Precision Medicine Cohort Program , which aims to collect one million or more patients’ data such as EHR, genomic, imaging, socio-behavioral, and environmental data over the next few years 1 . The Continuously Learning Healthcare System is also being advocated by the Institute of Medicine to close the gap between scientific discovery, patient and clinician engagement, and clinical practice 2 . However, the big data promise has not yet been realized to its potential as the mere availability of the data does not translate into knowledge or clinical practice. Moreover, due to the variation in data complexity and structures, unavailability of computational technologies, and concerns of sharing private patient data, few projects of large clinical data sets are made available to researchers in general. We have identified several key issues in facilitating and accelerating data driven translational clinical research and clinical practice. We will discuss in-depth in the domains of data quality, accessibility, and translation. Several use cases will be used to demonstrate the issues with the “Medical Information Mart for Intensive Care (MIMIC III)” database, one of the very few databases with granular and continuously monitored data of thousands of patients 3 .
2. Promises
In the era of genomics, the volume of data being captured from biological experiments and routine health care procedures is growing at an unprecedented pace 4 . This data trove has brought new promises for discovery in health care research and breakthrough treatments as well as new challenges in technology, management, and dissemination of knowledge. Multiple initiatives were taken to build specific systems in addressing the need for analysis of different types of data, e.g., integrated electronic health record (EHR) 5 , genomics-EHR 6 , genomics-connectomes 7 , insurance claims data, etc. These big data systems have shown potential for making fundamental changes in care delivery and discovery of treatments such as reducing health care costs, reducing number of hospital re-admissions, targeted interventions for reducing emergency department (ED) visits, triage of patients in ED, preventing adverse drug effects, and many more 8 . However, to realize these promises, the health care community must overcome some core technological and organizational challenges.
3. Challenges
Big data is not as big as it seems.
In the previous decade, federal funding agencies and private enterprises have taken initiatives for large scale data collection during routine health care and experimental research 5 , 9 . One prominent example of data collection during routine health care is the Medical Information Mart for Intensive Care (MIMIC III) which has collected data for more than fifty thousand patients from Beth Israel Deaconess Hospital dating back to 2001 3 . This is the largest publicly available patient care data set of an intensive care unit (ICU) and an important resource for clinical research. However, when it comes to identifying a cohort in the MIMIC data for answering a specific clinical question, it often results in a very small set of cases (small cohort) that makes it almost impossible to answer the question with a strong statistical confidence. For example, when studying the adverse effects of a drug-drug interaction, a researcher might be interested in looking at the vital signs and other patient characteristics during the time two different drugs were administered simultaneously, including a few days before the combination and a few days after the combination. Often this selection criteria results in a very small cohort of patients limiting the interpretation of the finding and with statistically inconclusive results. As an example, a researcher may want to investigate if any adverse effect exists when anti-depressants and anti-histamines are administered simultaneously. A query of simultaneous prescriptions of Amitriptyline HCl (anti-depressant) and Diphenhydramine HCl (anti-histamines) returned only 44 subjects in the MIMIC database ( Figure 1 ). Furthermore, by filtering the data with another selection criterion (e.g., to identify the subjects for which at least one day’s worth of data exist during, before and after the overlap) the query returned a much smaller cohort with only four records.

Example of a small cohort with clinical selection criteria.
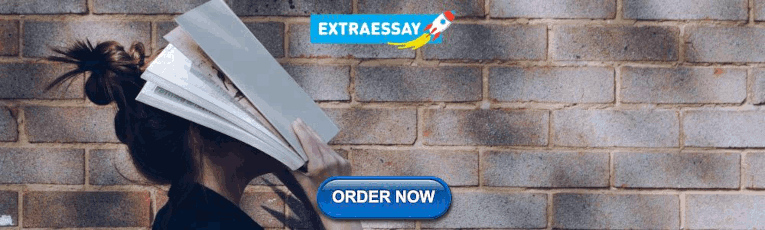
Data do not fully capture temporal and process information
In most cases, clinical data are captured in various systems, even within an organization, each with a somewhat different intent and often not well integrated. For example, an EHR is primarily used for documenting patient care and was designed to facilitate insurance company billing 10 , and pharmacy records were designed for inventory management. These systems were not developed to capture the temporal and process information which is indispensable for understanding disease progression, therapeutic effectiveness and patient outcomes. In an attempt to study clinical process of vancomycin therapeutic drug monitoring based on ICU patient records in the MIMIC database, it was discovered that such process is not easy to reconstruct. Ideally, a complete therapeutic process with a particular drug contains the history of the drug’s prescription, each of its exact administration times, amount and rate, and the timing and measurements of the drug in the blood throughout the therapy. From the MIMIC III database we were able to find prescription information but it lacks the detailed dosing amount and prescription’s length of validity. The “inputevents” table contains drug administration information but does not include the exact time-stamp and drug amount which is critical for studying intravenous infused vancomycin in the ICU. It is also difficult to match drug prescription and administration records because their recording times in the clinical systems often are not the precise event times, and prescribed drugs are not always administered.
Moreover, since the MIMIC III database does not contain detailed infusion event records which may be available from infusion pump software, one cannot know the precise drug infusion amount (and over what time) for any particular administration. The sparse and insufficient information on drug administration makes it almost impossible to associate available laboratory records and to reconstruct a therapeutic process for outcomes studies. Figure 2 is such an attempt of process reconstruction using data from the MIMIC III database including prescriptions, input events, and lab events for one patient during a unique ICU stay. The record only shows one valid prescription of vancomycin for this patient with start and end dates but does not indicate the administration frequency (e.g., every 12 hours) or method (e.g., continuous or bolus). The input events data (the second main column) came from the nursing records but it only shows one dose of vancomycin administration on each of the three-day ICU stay: one in the morning and two in the evening. Even though, as shown in the third main column, the “lab event” data contain the patient’s vancomycin concentration levels measured during this period, without the exact amount and duration of each vancomycin infusion, it is difficult to reconstruct this particular therapeutic process for the purposes of understanding its real effectiveness.

An example of vancomycin therapeutic process reconstruction of one unique ICU stay using data from three different tables in the MIMIC III database.
The problem of missing data remains relevant, even when the nursing workflow was designed to capture the data in the EHR. For example, as part of the nursing workflow, the information of drug administration should be documented in the medication administration records each time vancomycin was administered, and the MIMIC system was designed to capture all. But this was often not the case from our review of the database 2. Additionally, often times a patient’s diagnoses, co-morbidities, and complications are not fully captured nor available for reconstructing the complete clinical encounter. Those pieces of information are usually documented as free text not discrete data that can easily be extracted. Moreover, precise timings of the onset of an event and its resolution are rarely present. In the previous example of analyzing the effect of simultaneously administering Amitriptyline HCl and Diphenhydramine HCl, based on our selection criteria, we were able to find only one or two cases where such data were recorded ( Figure 3 ). In the figure, each color represents one subject, and only one color (green, ID:13852) is consistently present in the time window for the selection criteria indicating missing systolic blood pressure measurements for the other subjects. This example is not an exception for cohort selection from data captured during care delivery, but a common occurrence 11 , due to the complex nature of the care delivery process and technological barriers in the various clinical systems developed in the past decade or so.

Example of a cohort with missing systolic blood pressure data for three out of the four subjects meeting our clinical selection criteria. Day 0 (zero) is when drug overlap begins. This start of the overlap is aligned with multiple subjects and is denoted by the thick red line. Each data point represents one measurement from the “chartevents” table and each color indicates one subject and the black line indicates the average of the selected cohort.
3.2. Access
Accessibility to patient data for scientific research and sharing of the scientific work as digital objects for validation and reproducibility is another challenging domain due to patient privacy concerns, technological issues such as interoperability, and data ownership confusion. This has been a widely discussed issue in recent years of the so-called patient or health data conundrum as individuals do not have easy access to their own data 12 . We are discussing these challenges in the context of privacy, share-ability, and proprietary rights as follows.
Access to health care data is plagued by vulnerability due to patient privacy considerations which are protected by federal and local laws of protected health information such as Health Insurance Portability and Accountability Act of 1996 (HIPAA) 13 . The fear of litigation and breach of privacy discourages providers from sharing patient health data, even when they are de-identified. One reason is that current approaches to protect private information is limited to de-identification of an individual subject with an ID, which is vulnerable to twenty questions-like problems. For example, a query to find any patient who is of Indian origin and has some specific cancer diagnosis with a residential zip code 3-digit prefix ‘479’ may result in only one subject; thus exposing the identity of the individual.
Share-ability
Even after de-identification of patient data, the sharing of such data and research works based on the data is a complicated process. As an example, “Informatics for Integrating Biology and the Bedside (i2b2)” 5 is a system designed to capture data for scientific research during routine health care. i2b2 is a large initiative undertaken by Partners Healthcare System as an NIH-funded National Center for Biomedical Computing (NCBC). It contains a collection of data systems with over 100 hospitals that are using this software system on top of their clinical database. As a member of this project, each participating hospital system needed to transform their data into a SQL based star schema after de-identification. It required much effort for each institution to make the data available for scientific research as well as to develop the software in the first place. Although i2b2 was used exhaustively for research, sharing of data and research work as digital objects (i.e., the coding and the flow of the analysis) is not easily achieved. We argue that current EHR and other clinical systems do not empower the patients to take control of their data and engage in citizen science. The crowd sourcing approach might be one way to make a paradigm shift in this area which, unfortunately, is not yet possible with the current systems such as i2b2. A good example is the success in open source software technologies in other disciplines and applications (such as Linux, Git-hub, etc.) which rely on the engagement of many talented and passionate scientists and engineers all over the world to contribute their working products as digital objects 14 .
Proprietary rights
A relevant issue is the ongoing debate about the ownership of patient data among various stakeholders in the healthcare system including providers, patients, insurance companies and software vendors. In general, the current model is such that the patient owns his/her data, and the provider stores the data with proprietary software systems. The business models of most traditional EHR companies, such as Epic and Cerner, are based on building proprietary software systems to manage the data for insurance reimbursement and care delivery purposes. Such approach does not encourage or makes it difficult for individual patients to share data for scientific research, nor does it encourage patients to obtain their own health records that may help better manage their care and improve patient engagement.
3.3. Translation
Historically, a change in clinical practice is hard to achieve because of the sensitivity and risk aversion of care delivery. As an example, the use of beta blockers to prevent heart failure took 25 years to reach a widespread clinical adoption after the first research results were published 2 . This problem is much bigger for big data driven research findings to be translated into clinical practice because of the poor understanding of the risks and benefits of data driven decision support systems. Many machine learning algorithms work as a “black box” with no provision of good interpretations and clinical context of the outcomes, even though they often perform with reasonable accuracy. Without proper understanding and translatable mechanisms, it is difficult to estimate the risk and benefit of such algorithms in the clinical setting and thus discourages the new methods and treatments from being adopted by clinicians or approved by the regulatory bodies such as the FDA.
For example, if a machine learning algorithm can predict circulatory shock from patient arterial blood pressure data, what would be the risk if the algorithm fails in a particular setting based on patient demographics or clinical history? What should be the sample size to achieve high confidence in the results generated by the algorithm? These are some critical questions that cannot be answered by those traditional “black box” algorithms, nor have they been well accepted by the medical community, which relies heavily upon rule based approaches.
As an example, a decision tree algorithm might perform very differently for prediction of Medical Emergency Team (MET) activation based on the training set or sample size from the MIMIC data. Furthermore, the prediction result can be very different when another machine learning algorithm, the support vector machine (SVM), was used ( Figure 4 ).

Sensitivity for the machine learning algorithms for different training sizes for prediction of Medical Emergency Team (MET) activation from the MIMIC database 15 . The X-axis represents training size for different trials. For each training set, the results of a 10 fold cross validation are reported as box plots (the central red line is the median, the edges of the box are the 25 th and 75 th percentiles, the whiskers extend to the extreme data points the algorithm considers to be not outliers, and the red + sign denotes outliers). The blue asterisks represent the performance on the validation set of the algorithm that performs best on the test set. The blue dashed lines represent the performance of the National Early Warning Score (NEWS) 16 .
3.4. Incentive
Yet another barrier in using big data for better health is the lack of incentive for organizations to take initiative to address the technological challenges. As mentioned earlier, EHRs are developed for purposes other than knowledge advancement or care quality improvement, and that has led to unorganized, missing, and inadequate data for clinical research. An individual health system does not usually have the incentive to make these data organized and available for research, unless they are big academic institutions. It would be easier for each individual health system to share data if they were organized and captured using standard nomenclature and with meaningful and useful detailed information with significant detail. A key question any health organization faces is: what is the return on investment for my hospital to organize all the clinical data it gathers? One model is the Health Information Technology for Economic and Clinical Health Act (HITECH) which promotes the adoption and meaningful use of health information technology. The act authorized incentive payments be made through Medicare and Medicaid to clinicians and hospitals that adopted and demonstrated meaningful use of EHRs, and the US government has committed payments up to $27 billion dollars over a ten year period 17 . This incentive has paved the way for widespread adoption of EHRs since HITECH was enacted as part of the American Recovery and Reinvestment Act in 2009. However, for the purpose of using clinical data for scientific innovation and improving care delivery process, no apparent financial incentives currently exist for any organization to do so.
4. Opportunities
For data driven research in health care, we propose to record the most granular data during any care delivery process so as to capture the temporal and process information for treatment and outcomes. For example, in an intensive care unit, the exact time of medication administrations need to be captured. This can be achieved in a number of ways. As a nurse bar code scans an oral medication into the electronic medication administration record (eMAR) the system also timestamps the action in the EHR. Detailed intravenous drug infusions can be linked to the patient clinical records by integrating the smart infusion pumps with the EHR systems. The Regenstrief National Center for Medical Device Informatics (REMEDI), formerly known as the Infusion Pump Informatics 18 , has been capturing for capturing process and temporal infusion information. The planned expansion of such data set will allow linked patient outcomes and drug admin data forming a more complete treatment process for answering research and treatment effectiveness questions related to the administration of drugs such as drug-drug interaction, safe and effective dosage of drugs, etc., among others.
In order to achieve a statistically significant sample size after cohort selection, we promote breaking the silos of individual clinical data systems and making them interoperable across vendors, types and institutional boundaries with minimal effort. For the next generation of EHRs, these capabilities need to be considered.
4.2. Access
Patient/citizen powered research
To replicate the success in open source technologies in other disciplines by enabling citizen science, data and research analysis must be accessible to everyone. At the same time, patient privacy needs to be protected complying with the privacy law and proprietary rights of the vendors, and researchers need to be protected. As an example, we have demonstrated such a system with the MIMIC database where interoperable and extensible database technologies have been used on de-identified patient data in a high performance computing environment 19 .
Shareable digital objects
For the next generation of EHRs and other big data systems such as REMEDI 18 and i2b2 5 , data must be findable, accessible, interoperable and reproducible (FAIR) 20 . For big data systems, a software-hardware ecosystem could work as a distribution platform with characteristics analogous to an Apple or Android “app store” where any qualified individual can access the de-identified data with proper authentication without the need for a high throughput infrastructure and the rigorous work, including pre-processing of the data needed to reproduce previous works. The proposed architecture is shown in Figure 5 19 .

System concept for community driven software-hardware eco-system analogous to ‘app store’ for data driven clinical research.
4.3. Translation Causal understanding
Historically, clinical problems and treatment are studied and understood as “cause and effect”. For example, genetic disposition and lifestyle could lead to frequent urination, fatigue and hunger, and can be associated with diabetes. Based on this, the patient may be treated for this disease. However, most machine learning algorithms do not provide such a rule based approach; rather they predict the outcome of a given set of inputs, which may or may not be associated with known clinical understanding. Unlike other disciplines, clinical applications require a causal understanding of data driven research. Hence, most clinical studies start with some hypothesis, that ‘A’ causes ‘B’. The gold standard to identify this causation is randomized controlled trials (RCTs), which have also been the gold standard for regulatory approval of new drugs. Unfortunately, EHRs and the like data captured during routine healthcare has sampling selection bias and confounding variables and hence it is important to understand the limitation of such data sets. To answer the causal questions, a new generation of methods are necessary to understand the causal flow of treatment, outcome, and molecular properties of drugs by integrating big data systems for analysis and validation of hypothesis for transportability across studies with observational data 21 , 22 . These methods would enable the regulators to understand the risk and benefit of data driven systems in clinical settings for new guidelines enabling the translation. Once those guidelines are established, technological solution must also be enabled at the point of care such that clinicians can access for data driven queries as part of their clinical workflow.
5. Conclusion
“Big data” started with many believable promises in health care, but unfortunately, clinical science is different from other disciplines with additional constraints of data quality, privacy, and regulatory policies. We discussed these concepts in pursuit of a holistic solution that enables data driven findings to be translated in health care, from bench to bedside. We argue that the existing big data systems are still in their infancy, and without addressing these fundamental issues the health care big data may not achieve its full potential. We conclude that to make it to the next level, we need a larger cohort of institutions to share more complete, precise, and time stamped data as well as with greater willingness to invest in technologies for de-identifying private patient data for it to be shared broadly for scientific research. At the same time, as more and more “big data” systems are developed, the scientific and regulatory communities need to figure out new ways of understanding causal relationship from data captured during routine health care, that would complement current gold standard methods such as RCTs as well as identify the relationship between clinical practice and outcomes, as there is a wide disparity in the quality of care across the country 2 .
Table of Contents
What is big data, the five ‘v’s of big data, what does facebook do with its big data, big data case study, challenges of big data, challenges of big data visualisation, security management challenges, cloud security governance challenges, challenges of big data: basic concepts, case study, and more.
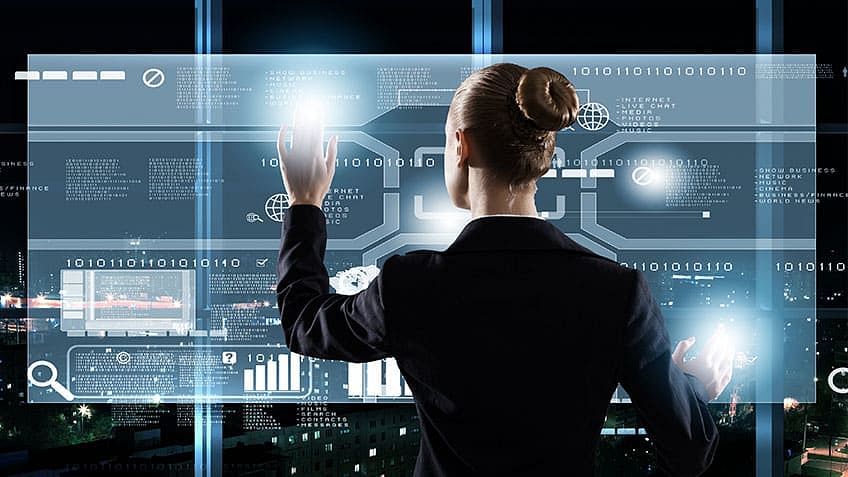
Evolving constantly, the data management and architecture field is in an unprecedented state of sophistication. Globally, more than 2.5 quintillion bytes of data are created every day, and 90 percent of all the data in the world got generated in the last couple of years ( Forbes ). Data is the fuel for machine learning and meaningful insights across industries, so organizations are getting serious about how they collect, curate, and manage information.
This article will help you learn more about the vast world of Big Data, and the challenges of Big Data . And in case you thing challenges of Big Data and Big data as a concept is not a big deal, here are some facts that will help you reconsider:
- About 300 billion emails get exchanged every day (Campaign Monitor)
- 400 hours of video are uploaded to YouTube every minute (Brandwatch)
- Worldwide retail eCommerce accounts for more than $4 billion in revenue (Shopify)
- Google receives more than 63,000 search inquiries every minute (SEO Tribunal)
- By 2025, real-time data will account for more than a quarter of all data (IDC)
To get a handle on challenges of big data, you need to know what the word "Big Data" means. When we hear "Big Data," we might wonder how it differs from the more common "data." The term "data" refers to any unprocessed character or symbol that can be recorded on media or transmitted via electronic signals by a computer. Raw data, however, is useless until it is processed somehow.
Before we jump into the challenges of Big Data, let’s start with the five ‘V’s of Big Data.
Big Data is simply a catchall term used to describe data too large and complex to store in traditional databases. The “five ‘V’s” of Big Data are:
- Volume – The amount of data generated
- Velocity - The speed at which data is generated, collected and analyzed
- Variety - The different types of structured, semi-structured and unstructured data
- Value - The ability to turn data into useful insights
- Veracity - Trustworthiness in terms of quality and accuracy
Facebook collects vast volumes of user data (in the range of petabytes, or 1 million gigabytes) in the form of comments, likes, interests, friends, and demographics. Facebook uses this information in a variety of ways:
- To create personalized and relevant news feeds and sponsored ads
- For photo tag suggestions
- Flashbacks of photos and posts with the most engagement
- Safety check-ins during crises or disasters
Next up, let us look at a Big Data case study, understand it’s nuances and then look at some of the challenges of Big Data.
As the number of Internet users grew throughout the last decade, Google was challenged with how to store so much user data on its traditional servers. With thousands of search queries raised every second, the retrieval process was consuming hundreds of megabytes and billions of CPU cycles. Google needed an extensive, distributed, highly fault-tolerant file system to store and process the queries. In response, Google developed the Google File System (GFS).
GFS architecture consists of one master and multiple chunk servers or slave machines. The master machine contains metadata, and the chunk servers/slave machines store data in a distributed fashion. Whenever a client on an API wants to read the data, the client contacts the master, which then responds with the metadata information. The client uses this metadata information to send a read/write request to the slave machines to generate a response.
The files are divided into fixed-size chunks and distributed across the chunk servers or slave machines. Features of the chunk servers include:
- Each piece has 64 MB of data (128 MB from Hadoop version 2 onwards)
- By default, each piece is replicated on multiple chunk servers three times
- If any chunk server crashes, the data file is present in other chunk servers
Next up let us take a look at the challenges of Big Data, and the probable outcomes too!
With vast amounts of data generated daily, the greatest challenge is storage (especially when the data is in different formats) within legacy systems. Unstructured data cannot be stored in traditional databases.
Processing big data refers to the reading, transforming, extraction, and formatting of useful information from raw information. The input and output of information in unified formats continue to present difficulties.
Security is a big concern for organizations. Non-encrypted information is at risk of theft or damage by cyber-criminals. Therefore, data security professionals must balance access to data against maintaining strict security protocols.
Finding and Fixing Data Quality Issues
Many of you are probably dealing with challenges related to poor data quality, but solutions are available. The following are four approaches to fixing data problems:
- Correct information in the original database.
- Repairing the original data source is necessary to resolve any data inaccuracies.
- You must use highly accurate methods of determining who someone is.
Scaling Big Data Systems
Database sharding, memory caching, moving to the cloud and separating read-only and write-active databases are all effective scaling methods. While each one of those approaches is fantastic on its own, combining them will lead you to the next level.
Evaluating and Selecting Big Data Technologies
Companies are spending millions on new big data technologies, and the market for such tools is expanding rapidly. In recent years, however, the IT industry has caught on to big data and analytics potential. The trending technologies include the following:
Hadoop Ecosystem
- Apache Spark
- NoSQL Databases
- Predictive Analytics
- Prescriptive Analytics
Big Data Environments
In an extensive data set, data is constantly being ingested from various sources, making it more dynamic than a data warehouse. The people in charge of the big data environment will fast forget where and what each data collection came from.
Real-Time Insights
The term "real-time analytics" describes the practice of performing analyses on data as a system is collecting it. Decisions may be made more efficiently and with more accurate information thanks to real-time analytics tools, which use logic and mathematics to deliver insights on this data quickly.
Data Validation
Before using data in a business process, its integrity, accuracy, and structure must be validated. The output of a data validation procedure can be used for further analysis, BI, or even to train a machine learning model.
Healthcare Challenges
Electronic health records (EHRs), genomic sequencing, medical research, wearables, and medical imaging are just a few examples of the many sources of health-related big data.
Barriers to Effective Use Of Big Data in Healthcare
- The price of implementation
- Compiling and polishing data
- Disconnect in communication
Other issues with massive data visualisation include:
- Distracting visuals; the majority of the elements are too close together. They are inseparable on the screen and cannot be separated by the user.
- Reducing the publicly available data can be helpful; however, it also results in data loss.
- Rapidly shifting visuals make it impossible for viewers to keep up with the action on screen.
The term "big data security" is used to describe the use of all available safeguards about data and analytics procedures. Both online and physical threats, including data theft, denial-of-service assaults, ransomware, and other malicious activities, can bring down an extensive data system.
It consists of a collection of regulations that must be followed. Specific guidelines or rules are applied to the utilisation of IT resources. The model focuses on making remote applications and data as secure as possible.
Some of the challenges are below mentioned:
- Methods for Evaluating and Improving Performance
- Governance/Control
- Managing Expenses
And now that we know the challenges of Big Data, let’s take a look at the solutions too!
Hadoop as a Solution
Hadoop , an open-source framework for storing data and running applications on clusters of commodity hardware, is comprised of two main components:
Hadoop HDFS
Hadoop Distributed File System (HDFS) is the storage unit of Hadoop. It is a fault-tolerant, reliable, scalable layer of the Hadoop cluster. Designed for use on commodity machines with low-cost hardware, Hadoop allows access to data across multiple Hadoop clusters on various servers. HDFS has a default block size of 128 MB from Hadoop version 2 onwards, which can be increased based on requirements.
Hadoop MapReduce
Become a big data professional.
- 11.5 M Expected New Jobs For Data Analytics And Science Related Roles
- 50% YOY Growth For Data Engineer Positions
Big Data Engineer
- Live interaction with IBM leadership
- 8X higher live interaction in live online classes by industry experts
Post Graduate Program in Data Engineering
- Post Graduate Program Certificate and Alumni Association membership
- Exclusive Master Classes and Ask me Anything sessions by IBM
Here's what learners are saying regarding our programs:
Craig Wilding
Data administrator , seminole county democratic party.
My instructor was experienced and knowledgeable with broad industry exposure. He delivered content in a way which is easy to consume. Thank you!
Joseph (Zhiyu) Jiang
I completed Simplilearn's Post-Graduate Program in Data Engineering, with Purdue University. I gained knowledge on critical topics like the Hadoop framework, Data Processing using Spark, Data Pipelines with Kafka, Big Data and more. The live sessions, industry projects, masterclasses, and IBM hackathons were very useful.
Hadoop features Big Data security, providing end-to-end encryption to protect data while at rest within the Hadoop cluster and when moving across networks. Each processing layer has multiple processes running on different machines within a cluster. The components of the Hadoop ecosystem , while evolving every day, include:
- Sqoop For ingestion of structured data from a Relational Database Management System (RDBMS) into the HDFS (and export back).
- Flume For ingestion of streaming or unstructured data directly into the HDFS or a data warehouse system (such as Hive
- Hive A data warehouse system on top of HDFS in which users can write SQL queries to process data
- HCatalog Enables the user to store data in any format and structure
- Oozie A workflow manager used to schedule jobs on the Hadoop cluster
- Apache Zookeeper A centralized service of the Hadoop ecosystem, responsible for coordinating large clusters of machines
- Pig A language allowing concise scripting to analyze and query datasets stored in HDFS
- Apache Drill Supports data-intensive distributed applications for interactive analysis of large-scale datasets
- Mahout For machine learning
MapReduce Algorithm
Hadoop MapReduce is among the oldest and most mature processing frameworks. Google introduced the MapReduce programming model in 2004 to store and process data on multiple servers, and analyze in real-time. Developers use MapReduce to manage data in two phases:
- Map Phase In which data gets sorted by applying a function or computation on every element. It sorts and shuffles data and decides how much data to process at a time.
- Reduce Phase Segregating data into logical clusters, removing bad data, and retaining necessary information.
Now that you have understood the five ‘V’s of Big Data, Big Data case study, challenges of Big Data, and some of the solutions too, it’s time you scale up your knowledge and become industry ready. Most organizations are making use of big data to draw insights and support strategic business decisions. Simplilearn's Caltech Post Graduate Program in Data Science will help you get ahead in your career!
If you have any questions, feel free to post them in the comments below. Our team will get back to you at the earliest.
Our Big Data Courses Duration And Fees
Big Data Courses typically range from a few weeks to several months, with fees varying based on program and institution.
Get Free Certifications with free video courses
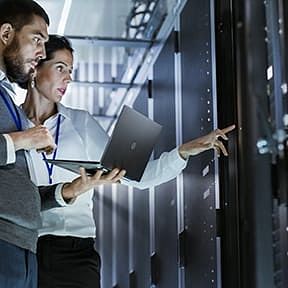
MongoDB Developer and Administrator
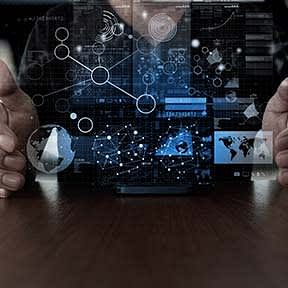
Introduction to Big Data Tools for Beginners
Learn from Industry Experts with free Masterclasses
Test Webinar: Simulive
Program Overview: The Reasons to Get Certified in Data Engineering in 2023
Career Webinar: Secrets for a Successful Career in Big Data
Recommended Reads
Big Data Career Guide: A Comprehensive Playbook to Becoming a Big Data Engineer
What's The Big Deal About Big Data?
How to Become a Big Data Engineer?
An Introduction to Big Data: A Beginner's Guide
Introduction to Big Data Storage
Top Big Data Applications Across Industries
Get Affiliated Certifications with Live Class programs
- PMP, PMI, PMBOK, CAPM, PgMP, PfMP, ACP, PBA, RMP, SP, and OPM3 are registered marks of the Project Management Institute, Inc.
More From Forbes
How marketers are using qualitative data in the age of big data and ai.

- Share to Facebook
- Share to Twitter
- Share to Linkedin
Lacking a holistic understanding of their target audience limits marketers’ ability to create the most effective strategies. Yet they often prioritize the concrete metrics of quantitative data, such as sales numbers and website traffic that seem easier to measure and analyze, over subjective observations. Consequently, relying solely on quantitative data can lead marketers to overlook the human element of consumer behavior and miss out on valuable insights that only qualitative data can provide.
Qualitative data reveals vital information about customers’ emotions, motivations, preferences and perceptions. Through methods such as interviews and focus groups, marketers can gather rich, nuanced data that goes beyond mere numbers, illuminating a bigger picture that allows them to better tailor strategies, messaging and products to what consumers want. Below, 15 members of Forbes Agency Council explore the role that qualitative data can play in the era of big data and artificial intelligence, sharing how their agencies leverage it to better meet the needs and desires of target audiences.
1. To Get To The Quantitative Data
Without the qualitative data, you can’t get to the quantitative data. All the ad creatives, messaging, campaign structures and so forth that take place behind the scenes are going to feed the big data monster with the engagement, clicks and interactions that can be improved upon. AI helps us get 10 times more iterations and options—but nothing runs without the creative thought that fills the machine. - Bernard May , National Positions
2. To Create A Better User Experience
We use qualitative data to create a better UX for Web design projects. Through user testing, interviews and usability studies, we gain insights into user needs, preferences and pain points. This information guides the design process, ensuring that websites and digital platforms are not only functional, but also enjoyable to use, enhancing user satisfaction and engagement. - Goran Paun , ArtVersion
3. To Understand Sentiment And Brand Perception
Qualitative data adds depth to our understanding of consumer behaviors, emotions and motivations, complementing quantitative insights. Our agency uses it for sentiment analysis and brand perception studies to craft personalized messaging that resonates authentically with target audiences. - Pascal Wilpers , Streamerzone.gg
Best High-Yield Savings Accounts Of 2024
Best 5% interest savings accounts of 2024, 4. to discover unique, actionable insights.
Einstein said, “Not everything that counts can be counted, and not everything that can be counted counts.” In marketing, qualitative data is vital to understanding human emotions and decisions. Despite abundant data, actionable insights require human intuition and experience, especially in hyper-personalized marketing. Global Prairie excels at this by harnessing qualitative data to discover unique insights. - Tom Hileman , Global Prairie
Forbes Agency Council is an invitation-only community for executives in successful public relations, media strategy, creative and advertising agencies. Do I qualify?
5. To Provide Color And Context
Consider qualitative data as the color providing context and sentiment around the rigid boundaries of quantitative data. Often, both types of datasets are needed to derive business value and audience interest. Qualitative data is the “gut check” for the “instincts” you may follow while evaluating datasets. Data cannot always be simplified into a dashboard; sometimes it requires deep analysis. - Tyler Back , Mitosis
6. To Identify Pain Points, Gains And Jobs To Be Done
Customer interviews provide qualitative data that is increasingly vital for identifying pain points, gains and jobs to be done, as well as shaping your communication strategy. However, quantitative data from clients and prospects is also crucial. It informs us about what messages to convey, as well as where and how to communicate them more effectively. - Kate Vasylenko , 42DM Corporation
7. To Connect PR Efforts To Client Goals And Show ROI
Qualitative data is essential to connecting PR efforts to clients’ business goals and showing the ROI of our work. At Next PR, we go beyond reporting on quantitative KPIs, such as number of media placements, and instead focus on qualitative results that support clients’ objectives, such as leveraging Google Analytics to demonstrate how a media placement contributed to increased brand awareness. - Heather Kelly , Next PR
8. To Get Insights That Yield Marketing Recommendations
Qualitative data speaks to the emotion behind customers’ decision making. We use interviews, open-ended surveys, observational studies and focus groups to gather such data. Quantitative techniques capture numerical data (website visits, customer satisfaction scores), revealing trends and correlations in customer behavior. Combining both, we develop insights that yield marketing recommendations. - Robert Finlayson , Bold Marketing and Communications
9. To Understand Our Clients’ Audiences’ Motivations
AI allows us to analyze large sets of data to provide necessary context. The contextual frameworks need to be filled with emotions and insights beyond the numbers—in short, qualitative data. It’s all about storytelling, so leveraging user feedback and social listening helps us understand the motivations and preferences of our clients’ audiences and turn them into meaningful marketing strategies. - Christoph Kastenholz , Pulse Advertising
10. To Get As One-To-One As Possible In Our Messaging
Qualitative data is important, as it allows us to get as one-to-one as possible in our messaging and content. Right now, we’re seeing a lot of junk via email, LinkedIn and content in search engines and other places that, in many cases, is either wrong at worst or okay at best. Higher-quality data allows for brand messaging and content that meets the prospect or potential customer with value, not noise. - Corey Morris , Voltage
11. To Develop Briefs And Leverage Winning Insights
Qualitative data is increasingly precious in an age of data commoditization. Comments on social media are the new briefs, and invariably, the majority of winning insights are the consequence of qualitative inputs from customers. - Aasim Shaikh Zubaer , LPS Brands
12. To Understand Why What Works Is Working
Qualitative data goes deeper than quantitative data. It gives you the “why.” While big data and AI can tell you what works, understanding why it works will help your organization develop a larger story arc to guide customers across the full journey. - Alicia Arnold , AK Arnold
13. To Develop Richer Audience Personas
In the era of big data and AI, qualitative data helps marketers and advertisers gain a deeper understanding of customers’ motivations and preferences. Our agency plans to leverage it by developing richer audience personas, which can help clients get a clearer picture into their customers’ values, inspirations and challenges. - Jordan Edelson , Appetizer Mobile LLC
14. To Inform Campaigns And Evaluate Results
Qualitative data remains a crucial tool for marketers and advertisers. It plays a complementary and valuable role alongside quantitative data, offering deeper insights and richer understanding of consumers. Our platform uses it to conduct research and analysis, develop customer personas for our clients, inform campaign development and measure and evaluate campaign results. - Tanuj Joshi , Eulerity
15. To Accurately Model B2B Audiences
Qualitative data is incredibly important for B2B agencies such as Napier. For example, it’s often not possible to get statistically significant samples to accurately model audiences quantitatively. It’s also important to note that AI’s “pattern matching” capabilities are not quantitative analysis; in fact, I believe AI has introduced a golden age of qualitative data analysis. - Mike Maynard , Napier Partnership Limited
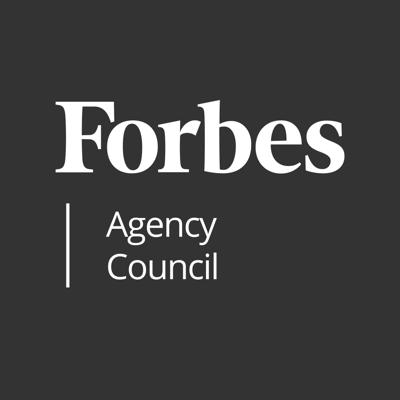
- Editorial Standards
- Reprints & Permissions

Ministers Treasury portfolio
- The Hon Dr Andrew Leigh MP
Address to the 10th Annual Australian Government Data Summit, Hotel Realm, Canberra
Harnessing the data deluge: the surprising power of big data and artificial intelligence*.
I acknowledge the Ngunnawal people, the traditional owners of these lands, and pay respects to all First Nations people present.
I’m pleased to join you today, in the tenth year of the annual Australian Government Data Summit. Robust, rigorous data and statistics are vital for delivering outcomes for all Australians.
Throughout our nation’s history, Australia’s statisticians and statistical agencies have punched above their weight in this – or should I say, found themselves in the right tail of the distribution. The nation’s first statistician, George Knibbs (known to his friends as ‘The Knibb’) published papers on mathematics, geodesy, wealth, and population. He was an acting professor of physics at the University of Sydney. He published a book on the federal capital. He was a member of the British Astronomical Society. He even wrote a book of verse.
George Knibbs must have been intimidating to many, but as one biographer notes, he had a ‘reputed charm of manner and unvarying kindness of heart’ (Bambrick, 1983). He would talk in a high‑pitched voice about his wide‑ranging interests, prompting one observer to note that ‘an hour's conversation with him is a paralysing revelation’. Whether this is a compliment or an insult is hard to tell, but regardless – you will be glad to know that I do not intend to regale you for the next hour. No paralysing revelations from me.
Instead, my focus today is on the surprising and important ways that artificial intelligence and big data are being used in government. Then, in the spirit of The Knibb’s verse‑writing, I will finish with a data‑crunching exercise of my own.
ABS: big data and artificial intelligence
Modern statisticians are using analytical tools and data collection methods that The Knibb could hardly imagine. The Australian Bureau of Statistics is living and breathing the big data and artificial intelligence revolution.
ABS analysts are at the forefront of methodological innovation, helping to improve our understanding of the Australian economy and labour market.
Take the example of the ABS using generative AI to help update the Australian and New Zealand Standard Classification of Occupations.
ANZSCO is a vital data set for understanding the nature of the labour market. It catalogues and categorises all occupations based on skills in Australia and New Zealand. Created in 2006, it now covers 1,076 occupations, from Acupuncturists, Blacksmiths and Cartographers to Wool Classers, Youth Workers and Zookeepers (Commonwealth of Australia, 2022).
Over time, occupations change. Just as ANZSCO 2006 updated prior occupational coding systems in Australia and New Zealand, it eventually needed an update. Bringing it up to date in 2024 was set to be a significant task. Just think about making a discrete list of the key tasks in your job and multiply that by more than a thousand jobs across the economy.
That’s why the ABS looked to AI for help to create a preliminary list of tasks undertaken in each occupation.
ABS data scientists gave ChatGPT a comprehensive prompt of over 480 words based on the existing publicly‑available ANZSCO. This helped the AI to generate its output in the right format and style.
ABS analysts spent time testing and refining ‘the prompt’ – or the question they ask ChatGPT – so that the machine delivered what the humans needed.
After each test, the analysts used a mathematical formula to calculate the quality of the responses within tolerance levels around precision and recall.
The ABS data scientists iterated the prompt until it consistently produced a high‑quality output. The final prompt ended up optimising the output from ChatGPT to about 69 per cent for both precision and recall.
The results were of such high quality that in one test, ANZSCO analysts themselves were asked to distinguish between the description of occupational tasks written by analysts and those written by generative AI. Two‑thirds got it wrong.
The results from ChatGPT were not perfect. But they did provide enough of a starting point for ABS analysts to review and build on. As a result, the project team saved approximately 1,600 hours. It amounted to an approximate seven‑fold return on investment.
Throughout, ABS data scientists were clear about the purpose of the exercise – to use generative AI to support – not replace – human analysts. All outputs were evaluated against four criteria: quality; ethics; legality; and security. This meant that AI outputs were correct, maintained privacy, did not break intellectual property laws and vitally, maintained the place of humans at the centre of decision‑making.
This delivers on our government’s commitment to fostering an innovative culture in the Australian Public Service, while managing the risks of emerging technologies.
This low‑risk project is a promising example of how generative AI can be applied in national statistics, enhance productivity, and develop related skills and capabilities in safe and responsible ways. It will help to pave the way for further ABS adoption of AI technologies.
The ABS is also at the forefront of harnessing digital technologies to build high quality, big data sets. By collecting weekly supermarket scanner data from private companies, the ABS is able to gather granular insights about household inflation and understand how the economy is tracking.
This is big data. For a moment, close your eyes and try to imagine all the items in Australia which were scanned at major supermarket checkouts over the past week. If it helps, remember that the typical major supermarket stocks over 15,000 different products, that there are over 10 million households in Australia, and that the typical household shops more than once a week.
Well, each of those items scanned last week – including the product purchased, quantity purchased, dollar value and location of purchase – is logged in this big data set. The ABS has been collecting this weekly data since 2011, so you can only imagine the size of it.
Through this data provision arrangement between private business and the ABS, 84 per cent of food sales from supermarkets and grocery stores is captured. The scale of this data allows for a granular understanding of household consumption, which is essential for compiling estimates of the Consumer Price Index.
The ABS is continuing its work to modernise collection methods to gather high quality and granular household spending data.
Traditionally, the ABS has collected detailed data by sending survey forms to households and asking them to record every good and service they purchase in a 2‑week period (using a 28‑page physical diary). This process is expensive, and response rates are falling. The next expenditure survey will utilise a digital diary – much like an app – which will work on any electronic device. This will greatly reduce costs and burden as well as streamline data processing.
Case study: big data and electoral results
Finally, I promised you a data‑crunching exercise of my own. I will show how analysing big data sets with novel techniques can reveal surprising insights. In this case, I have drawn my inspiration from political psychology.
In 2012, New York University’s Bernd Beber and Alexandra Scacco published a seminal paper that analysed the numbers in electoral results for various countries. Their starting point was that humans are surprisingly bad at making up numbers. When asked to create random numbers, participants have been shown to favour some numerals over others. Numbers ending in zero do not feel random to us, so less than one‑tenth of made‑up numbers end in zero. Falsified numbers are more likely to end in 1 and 2 than in 8 and 9.
Using this insight, the researchers look at the last digit of vote counts in various elections – both in Scandinavia and in Africa. They find that vote counts in the 2002 Swedish election have last numbers that are evenly distributed from zero to nine. By contrast, in elections held in Nigeria in 2003 and Senegal in 2007, they find anomalous patterns of last digits (Beber and Scacco, 2012). Many other researchers have used the tools of forensic electoral analysis, applying them to modern‑day elections where fraud has been alleged (Deckert, Myagkov and Ordeshook, 2011; Pericchi and Torres, 2011; Tunmibi and Olatokun, 2021; Figueiredo Filho, Silva and Carvalho, 2022).
Using this same approach, we can look at Australian elections, starting with the 2022 poll. Figure 1 shows the distribution of last digits in candidates’ vote counts across polling places. Naturally, when vote counts are small, the last digit tends to be lower, so I exclude results where a candidate received 100 or fewer votes. That leaves 21,822 results. You will be reassured to know that they show a refreshingly uniform pattern. The least common final digit is 4, which appears 9.7 per cent of the time, and the most common final digit is 8, which appears 10.2 per cent of the time.
Now, let’s take a look at a place where we might expect to see more shenanigans.
The 1843 Legislative Council election in New South Wales was the first of its kind. Only men who owned land worth more than £200 or more (or who rented a dwelling for £20 or more a year) were permitted to vote. According to the National Museum of Australia, these elections were ‘rough and tumble exercises… Alcohol, bribery, coercion and violence were intrinsic to the process’ (National Museum of Australia, 2024). Men were shot, and the Irish journalist William Kelly described early colonial polls as ‘nothing more or less than pantomime in a frenzy’.
What can we say about the voting patterns in these early elections? Because there were relatively few contests, we cannot analyse them one at a time. Instead, I tabulated data for the first 16 New South Wales colonial elections, from 1843 to 1887. I include both Legislative Council and Legislative Assembly elections, as well as by‑elections. Dropping contests in which candidates received 100 votes or fewer leaves 1,835 results. The distribution of the last digits is shown in Figure 2.
With fewer races in the dataset, we should expect a bit more statistical noise, but the races do not show marked evidence of fraud. The least common last digit is zero, which appears 9.3 per cent of the time, while the most common is one, which appears 10.7 per cent of the time. You might raise an eyebrow at this difference, but you should probably not raise both of them. [1 ]
The current revolutions in AI and big data in national statistics are not simply good just because they use new, cutting‑edge technologies. They matter because they offer the Australian Government a way of improving our administrative practices, and therefore the way we deliver for all citizens.
As I have explored through the examples of innovative work at the ABS, AI and big data can help to structure and collect data productively, safely and responsibly.
And as my election data‑crunching exercise shows, we can use novel techniques and frameworks to analyse big data sets to discover surprising patterns. The fields of cyber forensics, forensic economics and forensic accounting are deploying sophisticated tools to identify and reduce fraud and cheating.
Big data and generative AI offer opportunities across government to deliver superior outcomes for Australians. Our government remains committed to exploring these technologies within a clear ethical framework. As The Knibb knew a century ago: better data allows us to better serve the nation.
Australian Bureau of Statistics (2022), ANZSCO – Australian and New Zealand Standard Classification of Occupations , 2022 Australian Update.
Bambrick S (2006) ' Sir George Handley Knibbs (1858–1929) ', Australian Dictionary of Biography , accessed 24 February 2024. Originally published in the Australian Dictionary of Biography , Volume 9 , 1983.
Beber B and Scacco A (2012) ' What the Numbers Say: A Digit‑Based Test for Election Fraud ', Political Analysis , 20(2), pp.211–234.
Deckert J, Myagkov M and Ordeshook PC (2011) ' Benford’s Law and the Detection of Election Fraud ', Political Analysis , 19(3), pp. 245–268.
Figueiredo Filho D, Silva L and Carvalho E (2022) ' The forensics of fraud: Evidence from the 2018 Brazilian presidential election ', Forensic Science International: Synergy , 5, p.100286.
National Museum of Australia (2024) ' Secret ballot introduced ', accessed 24 February 2024.
Pericchi L and Torres D (2011) ' Quick Anomaly Detection by the Newcomb—Benford Law, with Applications to Electoral Processes Data from the USA, Puerto Rico and Venezuela ', Statistical Science , [online] 26(4), pp.502–516, accessed 22 March 2024.
Tunmibi S and Olatokun W (2021) ' Application of digits based test to analyse presidential election data in Nigeria ', Commonwealth & Comparative Politics , 59(1), pp.1–24.
[1] Formal chi‑square tests of the distributions in Figures 1 and 2 do not reject the null hypothesis that the last digits are uniformly distributed.
* My thanks to the Parliamentary Library’s Christopher Guiliano and my staff members Bria Larkspur, Maria Angella Fernando, Georgia Thompson and Maria Neill for assistance with data‑crunching, and to Antony Green for his original tabulations of NSW colonial election results. Frances Kitt provided valuable drafting assistance.
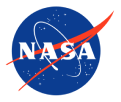
The Effects of Climate Change
The effects of human-caused global warming are happening now, are irreversible for people alive today, and will worsen as long as humans add greenhouse gases to the atmosphere.
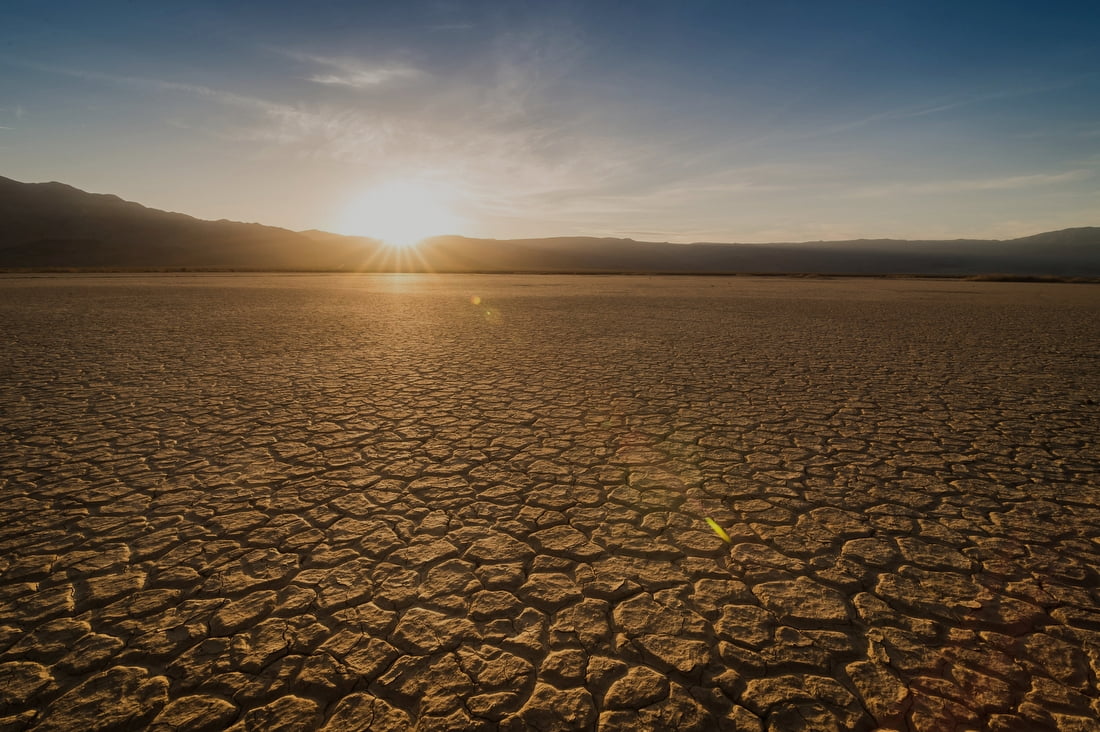
- We already see effects scientists predicted, such as the loss of sea ice, melting glaciers and ice sheets, sea level rise, and more intense heat waves.
- Scientists predict global temperature increases from human-made greenhouse gases will continue. Severe weather damage will also increase and intensify.
Earth Will Continue to Warm and the Effects Will Be Profound
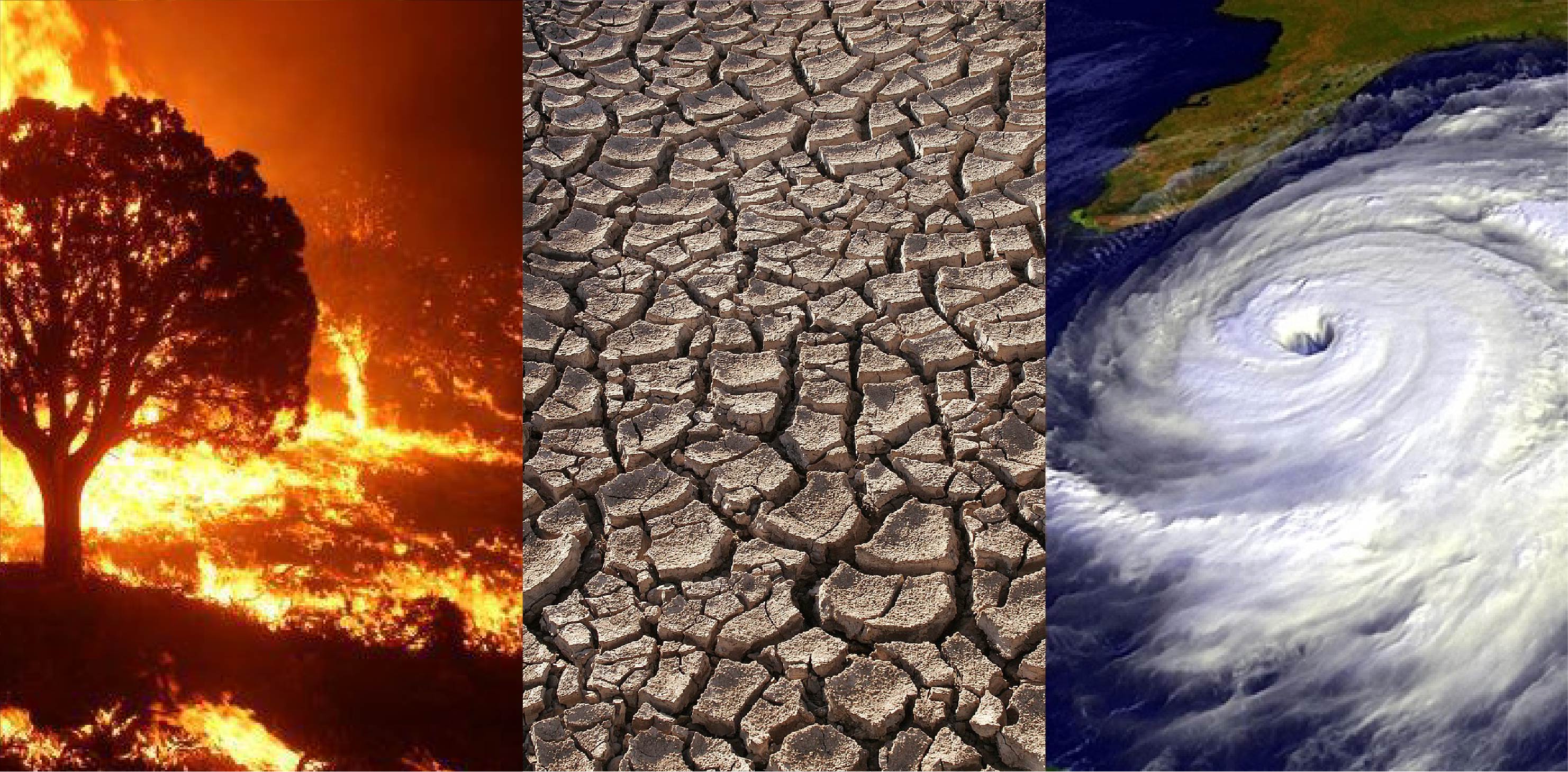
Global climate change is not a future problem. Changes to Earth’s climate driven by increased human emissions of heat-trapping greenhouse gases are already having widespread effects on the environment: glaciers and ice sheets are shrinking, river and lake ice is breaking up earlier, plant and animal geographic ranges are shifting, and plants and trees are blooming sooner.
Effects that scientists had long predicted would result from global climate change are now occurring, such as sea ice loss, accelerated sea level rise, and longer, more intense heat waves.
The magnitude and rate of climate change and associated risks depend strongly on near-term mitigation and adaptation actions, and projected adverse impacts and related losses and damages escalate with every increment of global warming.
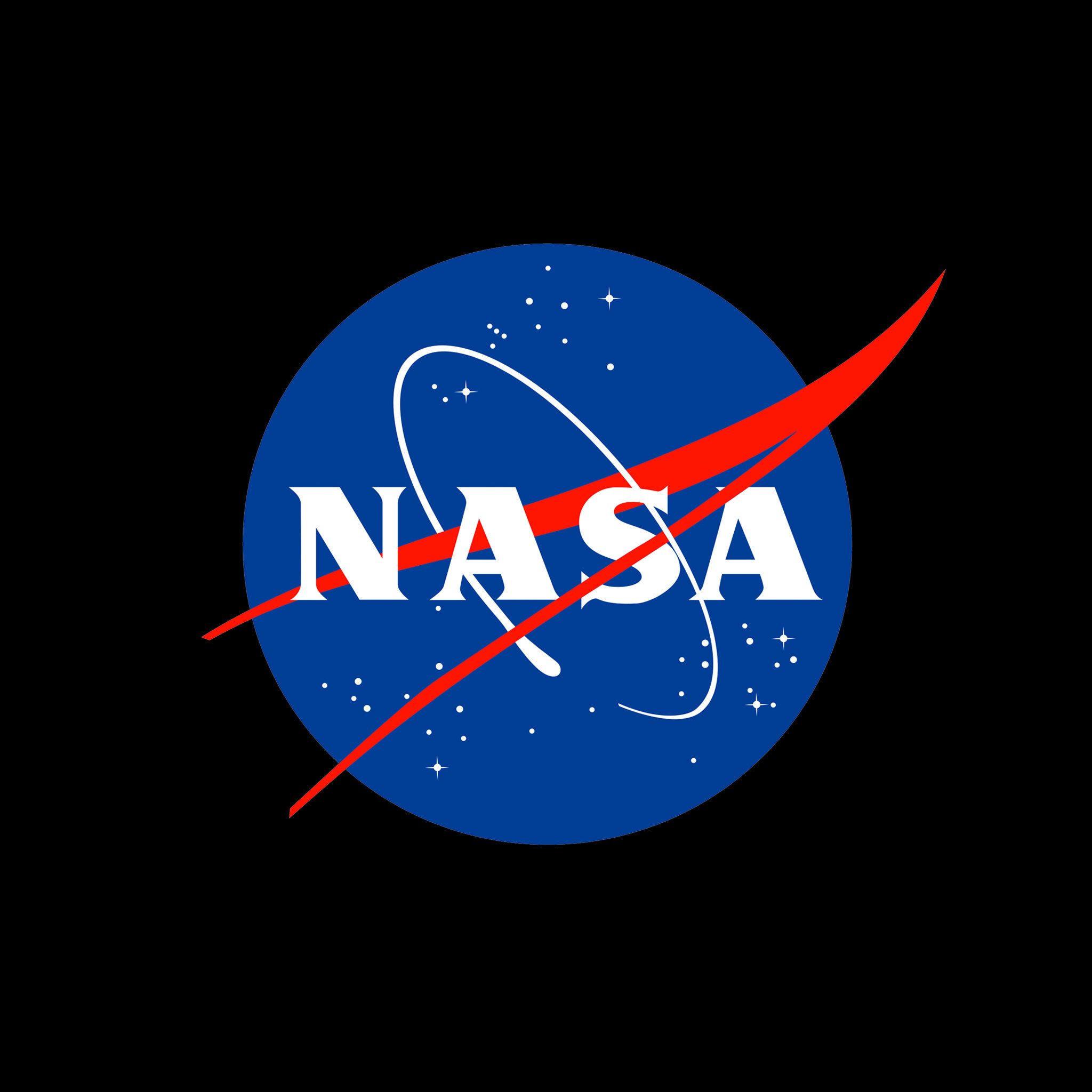
Intergovernmental Panel on Climate Change
Some changes (such as droughts, wildfires, and extreme rainfall) are happening faster than scientists previously assessed. In fact, according to the Intergovernmental Panel on Climate Change (IPCC) — the United Nations body established to assess the science related to climate change — modern humans have never before seen the observed changes in our global climate, and some of these changes are irreversible over the next hundreds to thousands of years.
Scientists have high confidence that global temperatures will continue to rise for many decades, mainly due to greenhouse gases produced by human activities.
The IPCC’s Sixth Assessment report, published in 2021, found that human emissions of heat-trapping gases have already warmed the climate by nearly 2 degrees Fahrenheit (1.1 degrees Celsius) since 1850-1900. 1 The global average temperature is expected to reach or exceed 1.5 degrees C (about 3 degrees F) within the next few decades. These changes will affect all regions of Earth.
The severity of effects caused by climate change will depend on the path of future human activities. More greenhouse gas emissions will lead to more climate extremes and widespread damaging effects across our planet. However, those future effects depend on the total amount of carbon dioxide we emit. So, if we can reduce emissions, we may avoid some of the worst effects.
The scientific evidence is unequivocal: climate change is a threat to human wellbeing and the health of the planet. Any further delay in concerted global action will miss the brief, rapidly closing window to secure a liveable future.
Here are some of the expected effects of global climate change on the United States, according to the Third and Fourth National Climate Assessment Reports:
Future effects of global climate change in the United States:
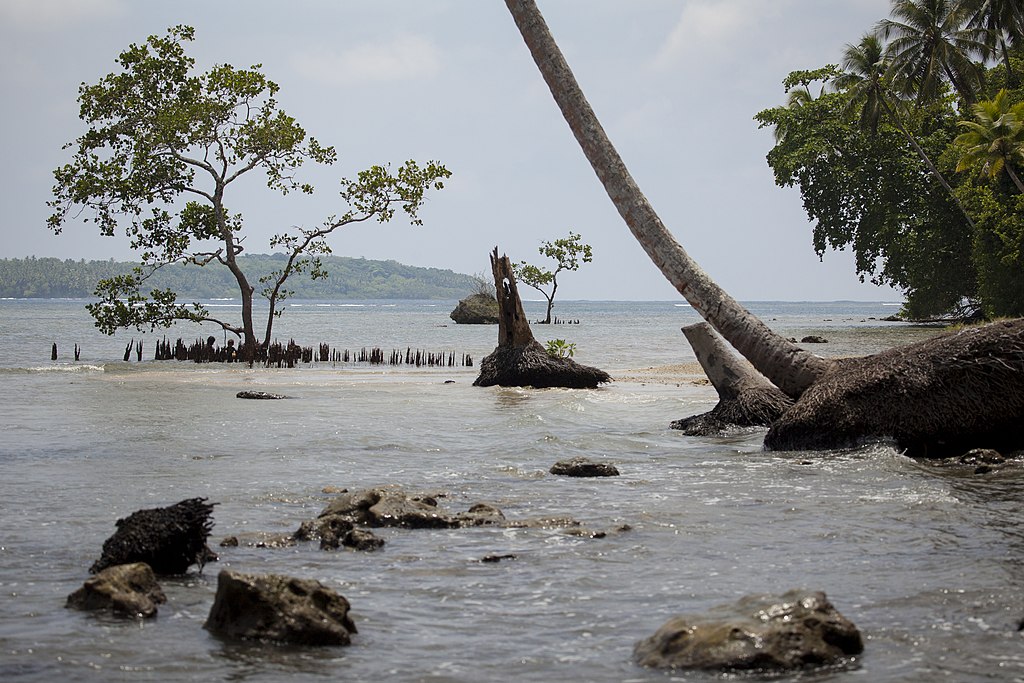
U.S. Sea Level Likely to Rise 1 to 6.6 Feet by 2100
Global sea level has risen about 8 inches (0.2 meters) since reliable record-keeping began in 1880. By 2100, scientists project that it will rise at least another foot (0.3 meters), but possibly as high as 6.6 feet (2 meters) in a high-emissions scenario. Sea level is rising because of added water from melting land ice and the expansion of seawater as it warms. Image credit: Creative Commons Attribution-Share Alike 4.0
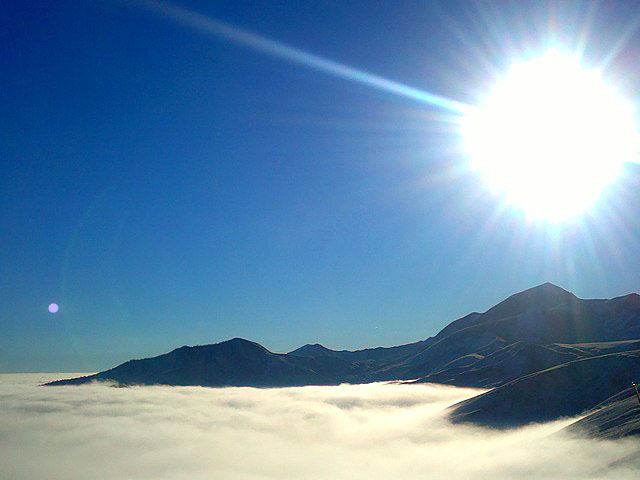
Climate Changes Will Continue Through This Century and Beyond
Global climate is projected to continue warming over this century and beyond. Image credit: Khagani Hasanov, Creative Commons Attribution-Share Alike 3.0
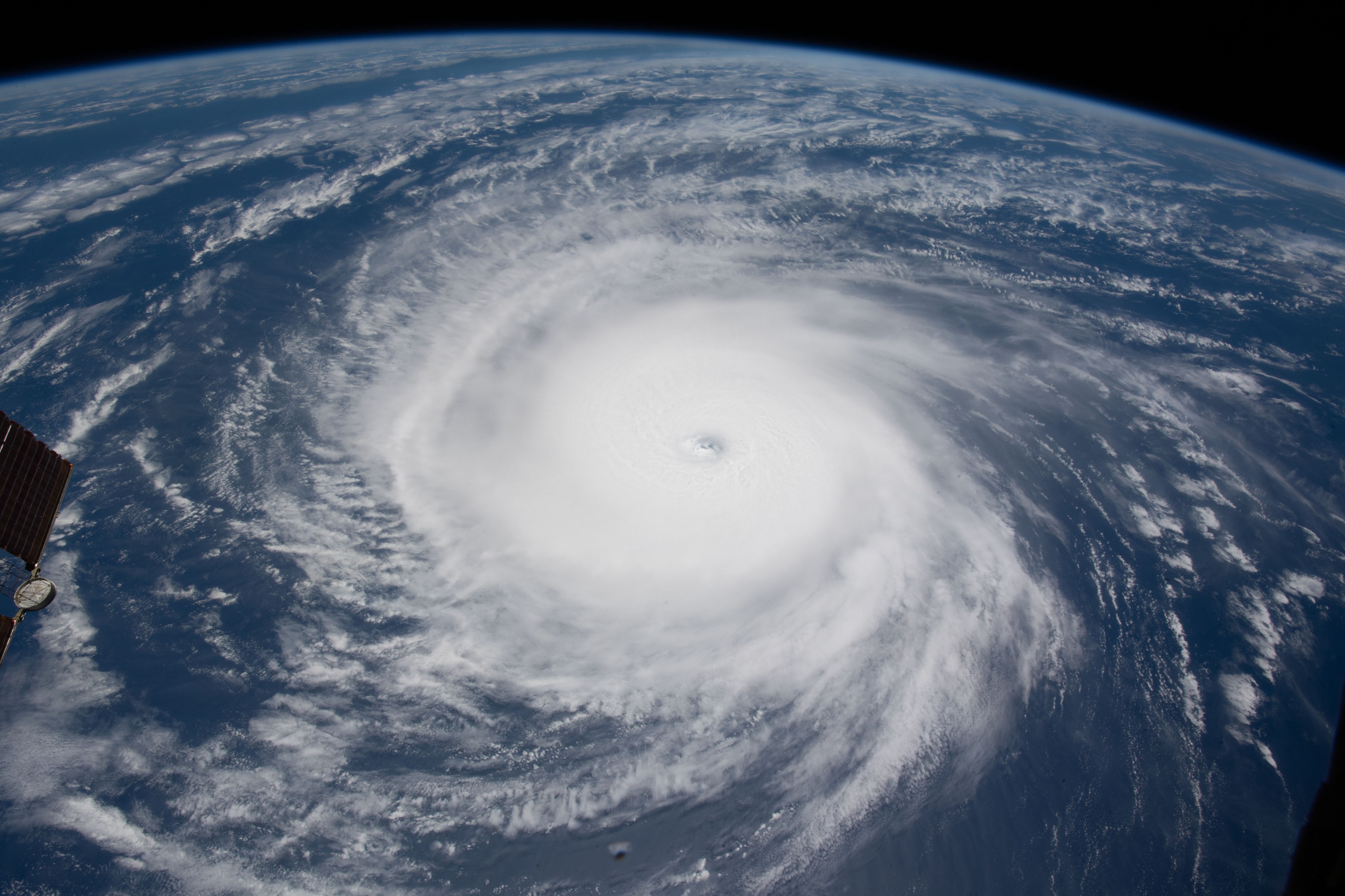
Hurricanes Will Become Stronger and More Intense
Scientists project that hurricane-associated storm intensity and rainfall rates will increase as the climate continues to warm. Image credit: NASA
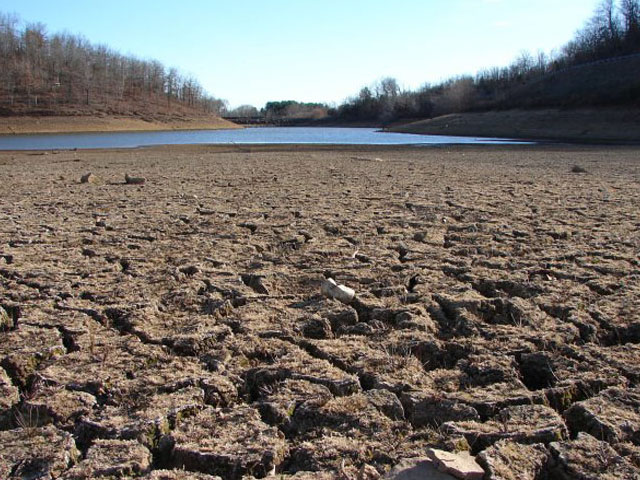
More Droughts and Heat Waves
Droughts in the Southwest and heat waves (periods of abnormally hot weather lasting days to weeks) are projected to become more intense, and cold waves less intense and less frequent. Image credit: NOAA
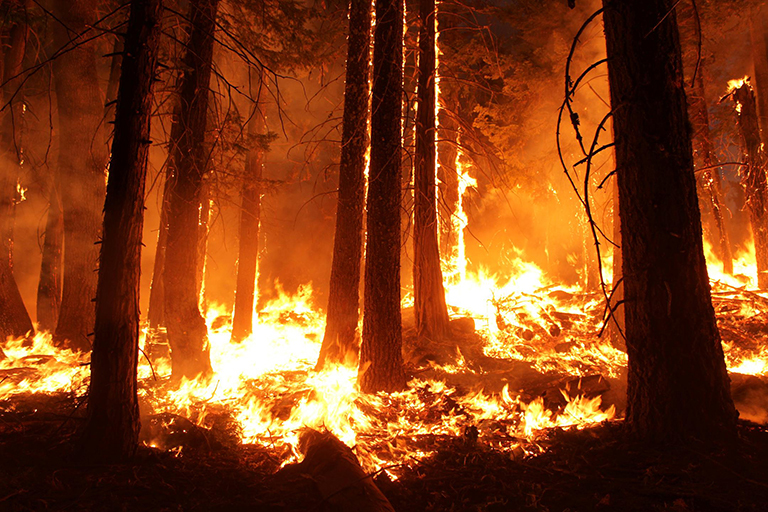
Longer Wildfire Season
Warming temperatures have extended and intensified wildfire season in the West, where long-term drought in the region has heightened the risk of fires. Scientists estimate that human-caused climate change has already doubled the area of forest burned in recent decades. By around 2050, the amount of land consumed by wildfires in Western states is projected to further increase by two to six times. Even in traditionally rainy regions like the Southeast, wildfires are projected to increase by about 30%.
Changes in Precipitation Patterns
Climate change is having an uneven effect on precipitation (rain and snow) in the United States, with some locations experiencing increased precipitation and flooding, while others suffer from drought. On average, more winter and spring precipitation is projected for the northern United States, and less for the Southwest, over this century. Image credit: Marvin Nauman/FEMA
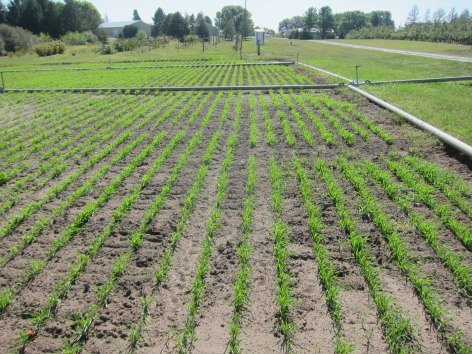
Frost-Free Season (and Growing Season) will Lengthen
The length of the frost-free season, and the corresponding growing season, has been increasing since the 1980s, with the largest increases occurring in the western United States. Across the United States, the growing season is projected to continue to lengthen, which will affect ecosystems and agriculture.
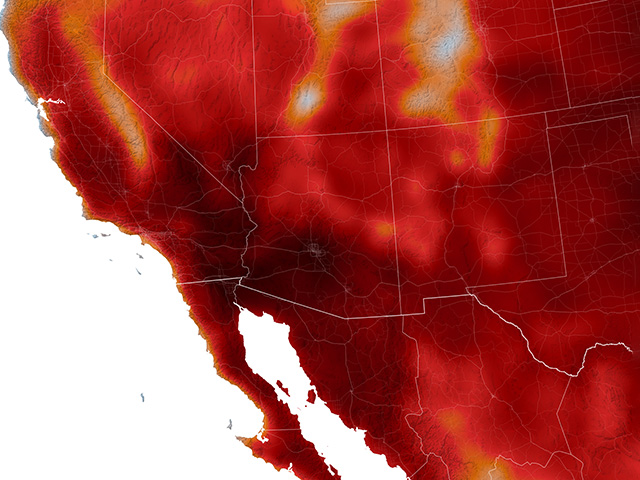
Global Temperatures Will Continue to Rise
Summer of 2023 was Earth's hottest summer on record, 0.41 degrees Fahrenheit (F) (0.23 degrees Celsius (C)) warmer than any other summer in NASA’s record and 2.1 degrees F (1.2 C) warmer than the average summer between 1951 and 1980. Image credit: NASA
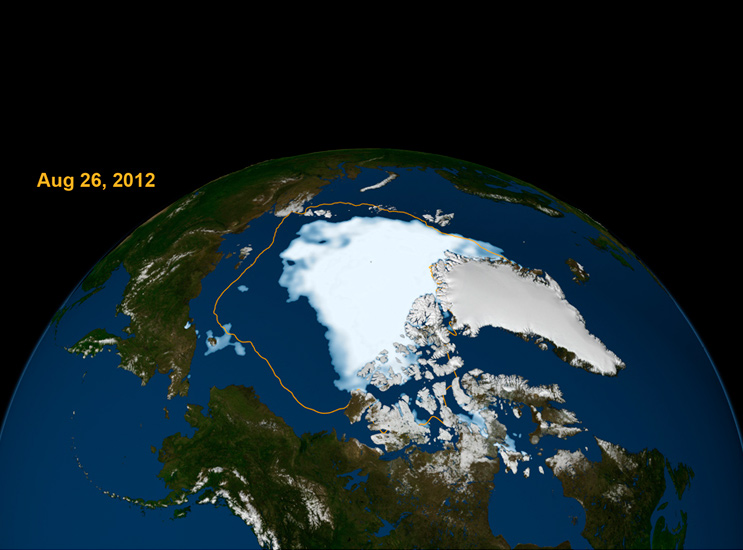
Arctic Is Very Likely to Become Ice-Free
Sea ice cover in the Arctic Ocean is expected to continue decreasing, and the Arctic Ocean will very likely become essentially ice-free in late summer if current projections hold. This change is expected to occur before mid-century.
U.S. Regional Effects
Climate change is bringing different types of challenges to each region of the country. Some of the current and future impacts are summarized below. These findings are from the Third 3 and Fourth 4 National Climate Assessment Reports, released by the U.S. Global Change Research Program .
- Northeast. Heat waves, heavy downpours, and sea level rise pose increasing challenges to many aspects of life in the Northeast. Infrastructure, agriculture, fisheries, and ecosystems will be increasingly compromised. Farmers can explore new crop options, but these adaptations are not cost- or risk-free. Moreover, adaptive capacity , which varies throughout the region, could be overwhelmed by a changing climate. Many states and cities are beginning to incorporate climate change into their planning.
- Northwest. Changes in the timing of peak flows in rivers and streams are reducing water supplies and worsening competing demands for water. Sea level rise, erosion, flooding, risks to infrastructure, and increasing ocean acidity pose major threats. Increasing wildfire incidence and severity, heat waves, insect outbreaks, and tree diseases are causing widespread forest die-off.
- Southeast. Sea level rise poses widespread and continuing threats to the region’s economy and environment. Extreme heat will affect health, energy, agriculture, and more. Decreased water availability will have economic and environmental impacts.
- Midwest. Extreme heat, heavy downpours, and flooding will affect infrastructure, health, agriculture, forestry, transportation, air and water quality, and more. Climate change will also worsen a range of risks to the Great Lakes.
- Southwest. Climate change has caused increased heat, drought, and insect outbreaks. In turn, these changes have made wildfires more numerous and severe. The warming climate has also caused a decline in water supplies, reduced agricultural yields, and triggered heat-related health impacts in cities. In coastal areas, flooding and erosion are additional concerns.
1. IPCC 2021, Climate Change 2021: The Physical Science Basis , the Working Group I contribution to the Sixth Assessment Report, Cambridge University Press, Cambridge, UK.
2. IPCC, 2013: Summary for Policymakers. In: Climate Change 2013: The Physical Science Basis. Contribution of Working Group I to the Fifth Assessment Report of the Intergovernmental Panel on Climate Change [Stocker, T.F., D. Qin, G.-K. Plattner, M. Tignor, S.K. Allen, J. Boschung, A. Nauels, Y. Xia, V. Bex and P.M. Midgley (eds.)]. Cambridge University Press, Cambridge, United Kingdom and New York, NY, USA.
3. USGCRP 2014, Third Climate Assessment .
4. USGCRP 2017, Fourth Climate Assessment .
Related Resources
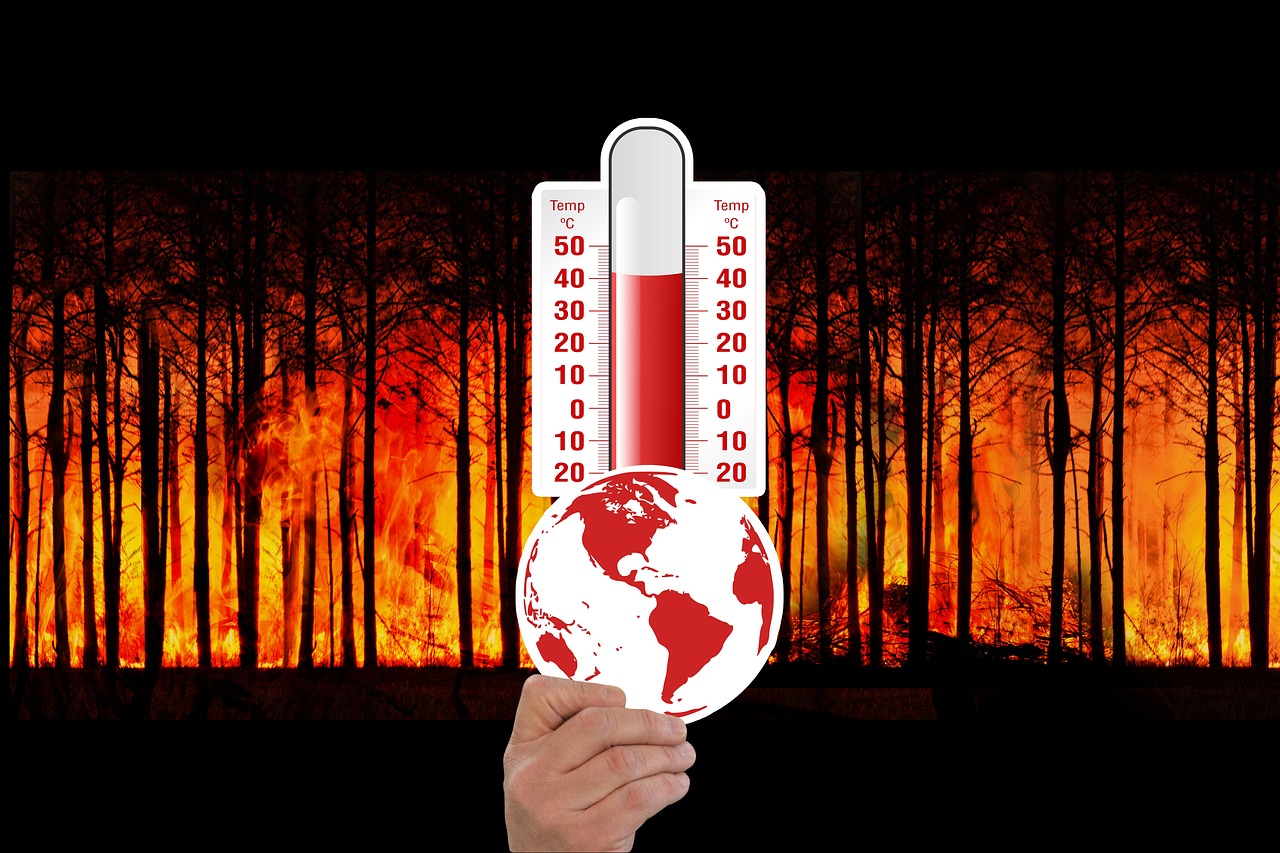
A Degree of Difference
So, the Earth's average temperature has increased about 2 degrees Fahrenheit during the 20th century. What's the big deal?
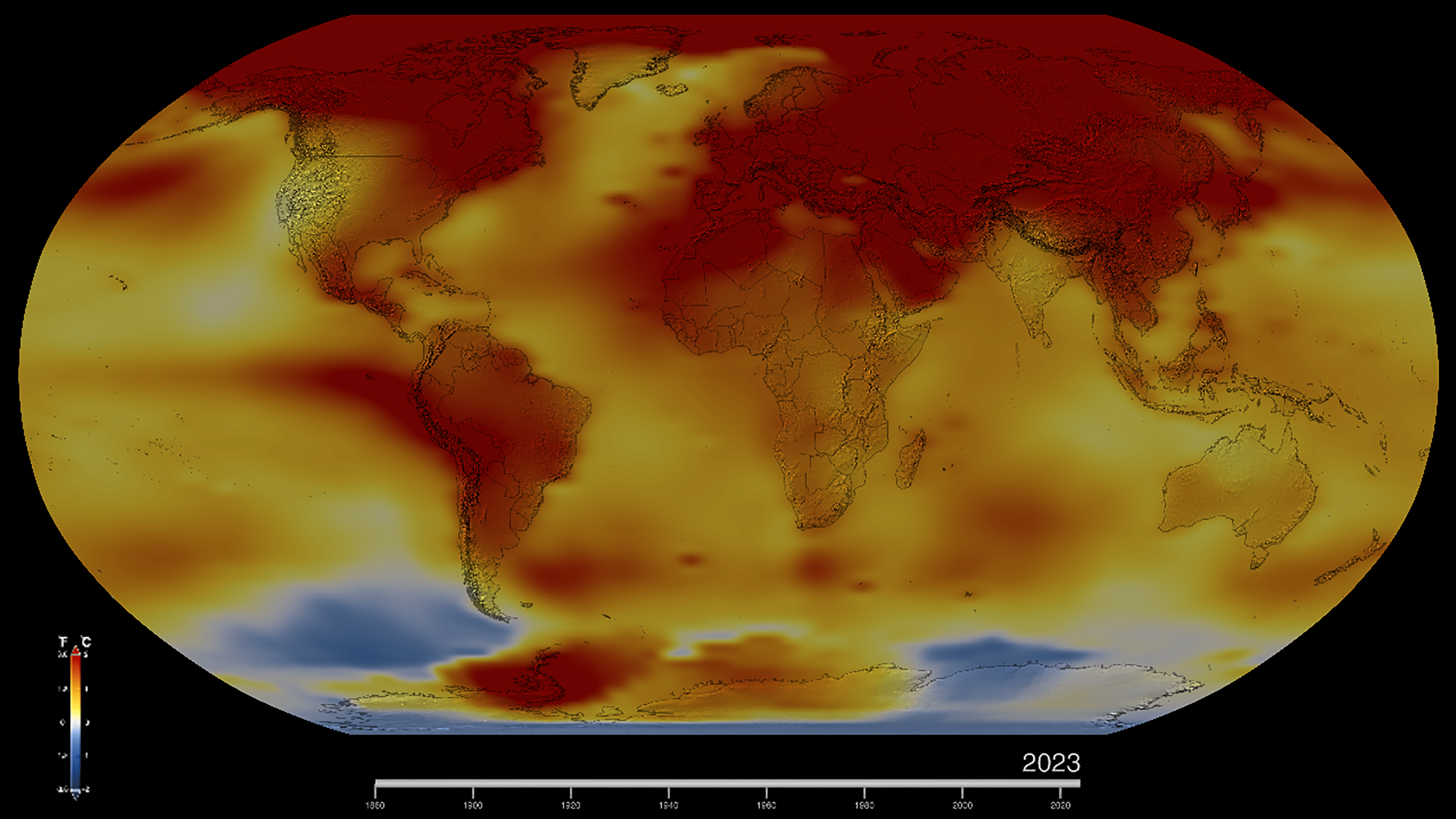
What’s the difference between climate change and global warming?
“Global warming” refers to the long-term warming of the planet. “Climate change” encompasses global warming, but refers to the broader range of changes that are happening to our planet, including rising sea levels; shrinking mountain glaciers; accelerating ice melt in Greenland, Antarctica and the Arctic; and shifts in flower/plant blooming times.
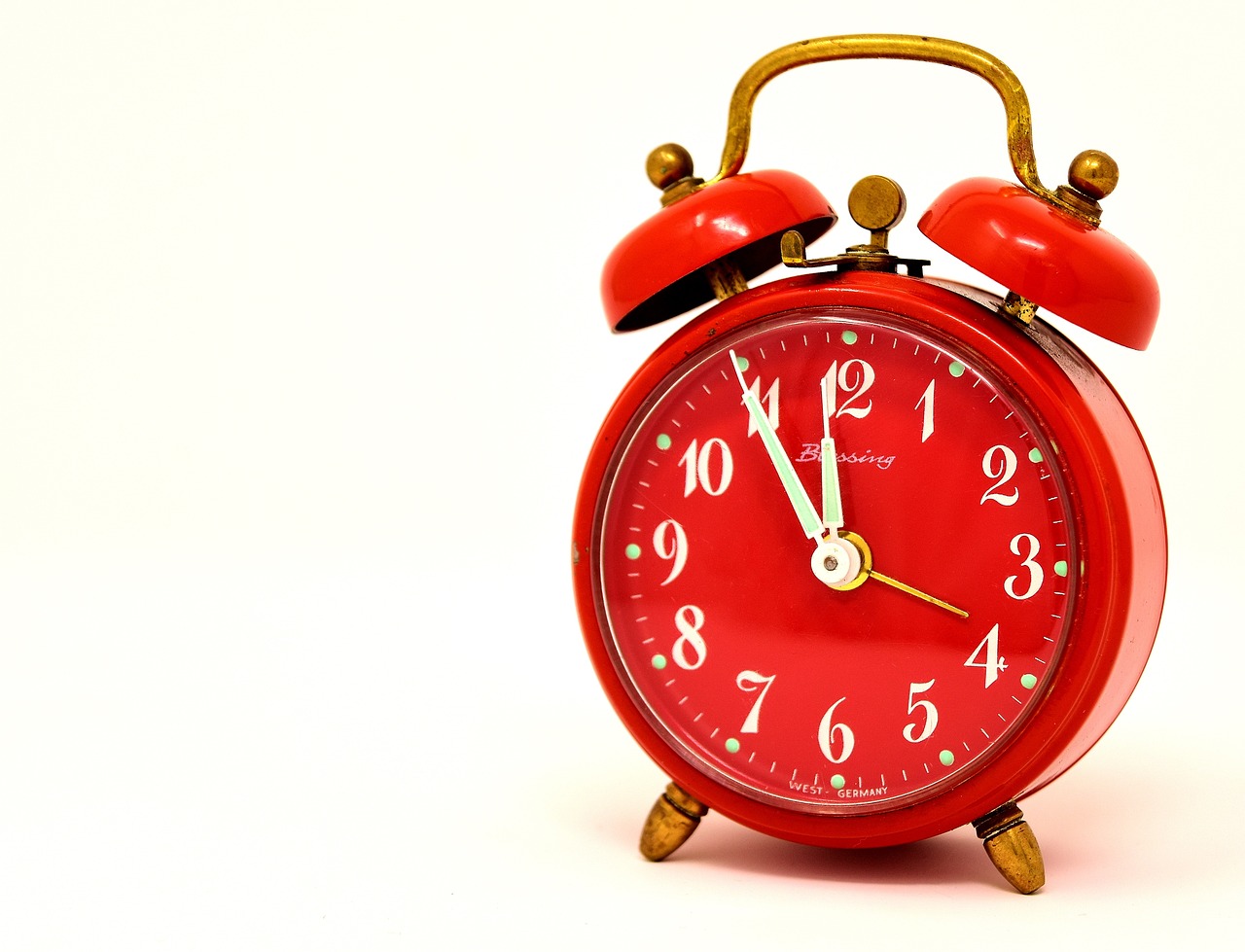
Is it too late to prevent climate change?
Humans have caused major climate changes to happen already, and we have set in motion more changes still. However, if we stopped emitting greenhouse gases today, the rise in global temperatures would begin to flatten within a few years. Temperatures would then plateau but remain well-elevated for many, many centuries.
Discover More Topics From NASA
Explore Earth Science

Earth Science in Action
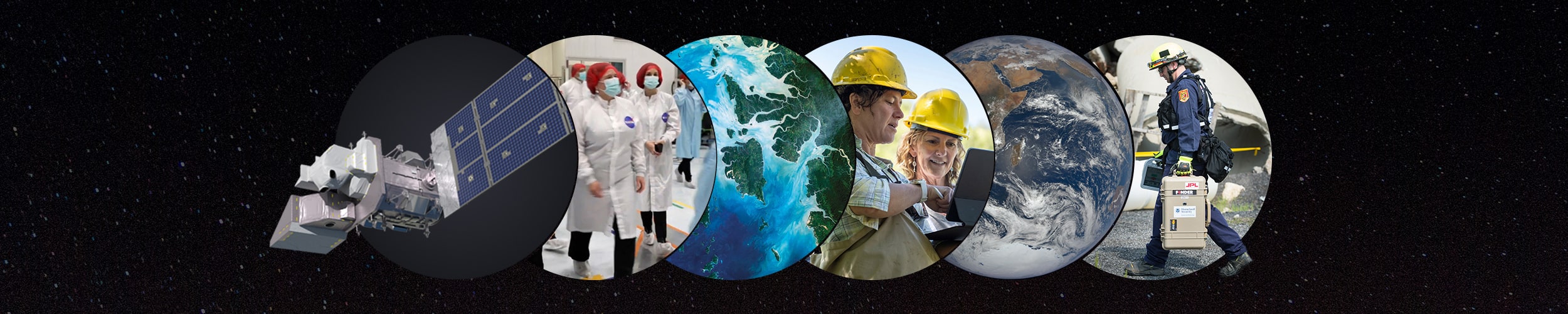
Earth Science Data
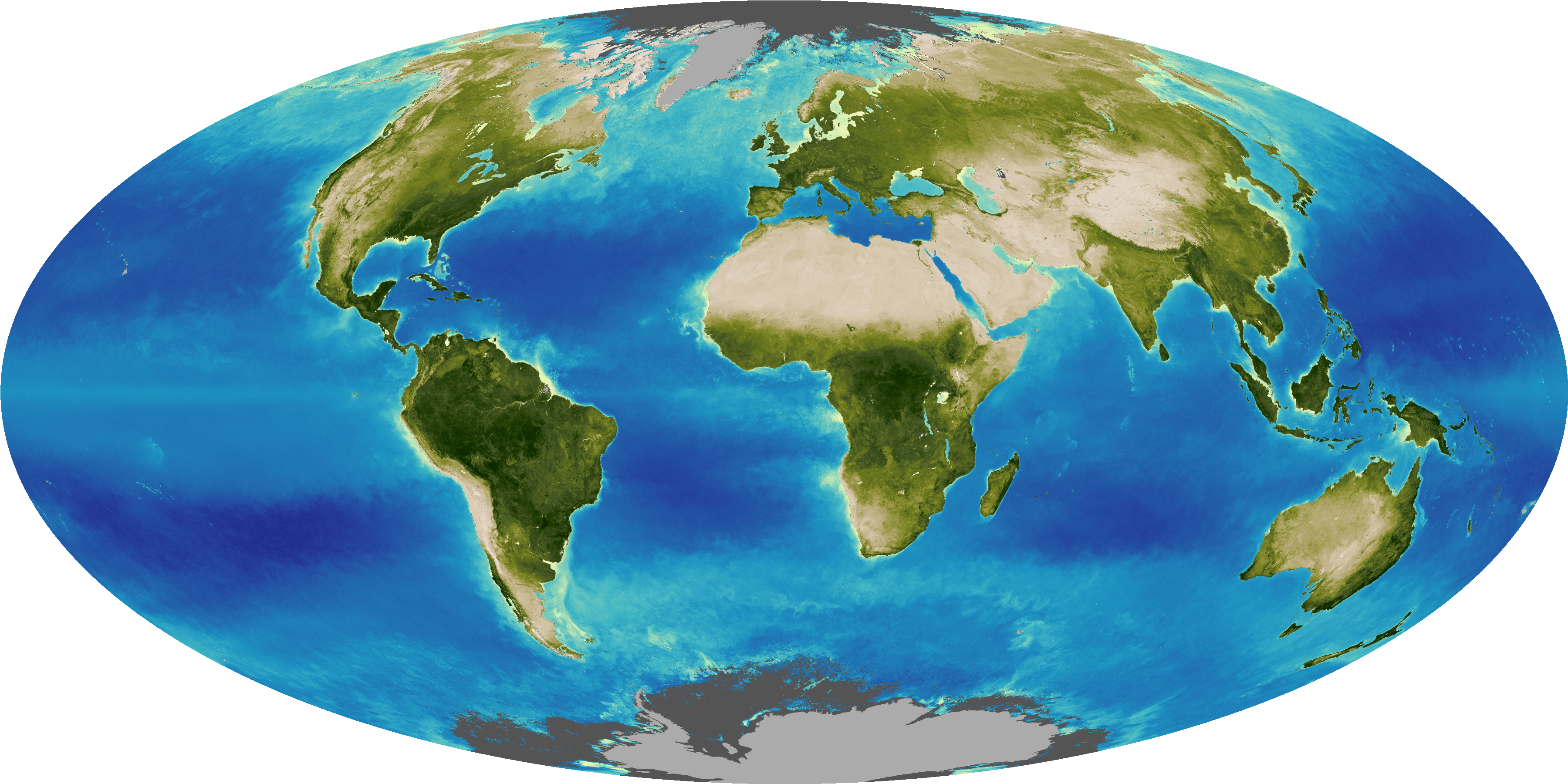
Facts About Earth

- Greater Jakarta
- BINUS @Greater Jakarta
- BINUS @Bekasi
- BINUS @Bandung
- BINUS @Malang
- BINUS @Semarang
- BINUS ComDev
- Research Products
- Important Links
- BINUS Journal
- Research Repository
- RTT Profile (PDF)
- SIMLITBINUS
- Social Media
- Book Publication List
- Achievements
- Artificial Intelligence in Geospatial Economics (Geo-Eco AI)
- Consumer Behavior and Digital Ethics
- Digital Language and Behavior (D-LAB)
- Education Technology
- Photonics and Computer Systems
- Small Medium Enterprise,Entrepreneurship & Innovation
- Quantitative and Decision Sciences (Q&DS)
- Artificial Intelligence
- Bioinformatics and Data Science
- Food Biotechnology
- E-PDP and Scopus Apps
- Intellectual Property Rights
- Simlitbinus
- Meet the Team
- Check My Publication KPI
Visiting Professor How to Write a Paper from Case Study
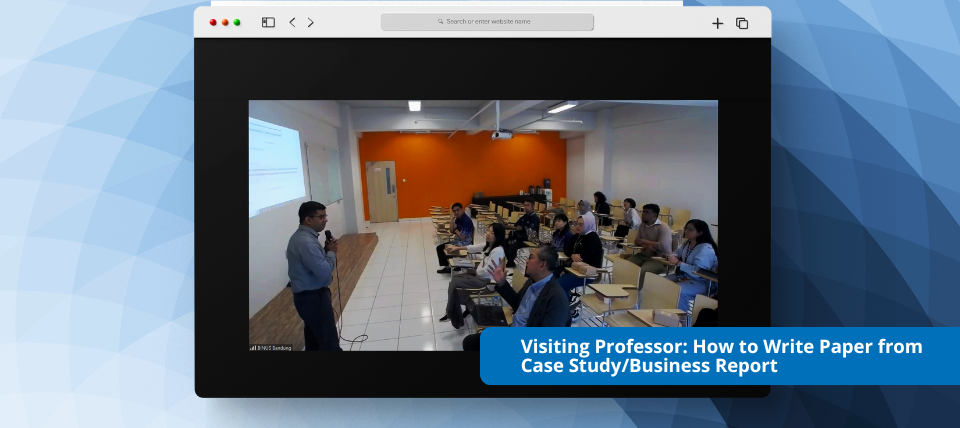
On the 26th of February 2024, BINUS University hosted an insightful workshop aimed at enhancing the academic writing skills of its faculty members. Titled “How to Write a Paper from Case Study/Business Report,” the workshop featured Assoc. Prof. Dr. Nanthakumar Loganathan from Universiti Teknologi Malaysia as the distinguished speaker. The event held at Bandung Campus, provided an invaluable opportunity for faculty members to delve into the intricacies of crafting scholarly papers, drawing from real-world case studies and business reports. With an emphasis on practical strategies and methodologies, the workshop aimed to equip participants with the necessary tools to produce high-quality academic literature.
Following the enriching workshop, BINUS University continued its academic endeavors with a public lecture on international research experiences, specifically tailored for its student body. The event welcomed Assoc. Prof. Dr. Nanthakumar Loganathan once again as the esteemed speaker. The lecture commenced with an opening speech delivered by Prof. Dr. Tirta N. Mursitama, Ph.D., Vice Rector Collaboration and Global Engagement, BINUS University, setting the tone for an engaging discourse on research practices and opportunities on the global stage. Attended by BINUS students eager to explore international research avenues, the lecture provided valuable insights into the significance of cross-cultural collaboration and exposure in the realm of academic inquiry.
Both events underscored BINUS University’s commitment to fostering a vibrant academic environment conducive to knowledge exchange and scholarly growth. With distinguished speakers and active participation from faculty members and students alike, the workshops and lectures served as catalysts for intellectual engagement and professional development within the university community. As BINUS continues to uphold its dedication to academic excellence, such initiatives play a pivotal role in nurturing a generation of researchers and scholars poised to make meaningful contributions to their respective fields and beyond.
#BINUSUniversity #AcademicWriting #FacultyDevelopment #ResearchSkills #InternationalExperience #ScholarlyGrowth #CrossCulturalCollaboration #GlobalEngagement #ProfessionalDevelopment #StudentResearch #KnowledgeExchange
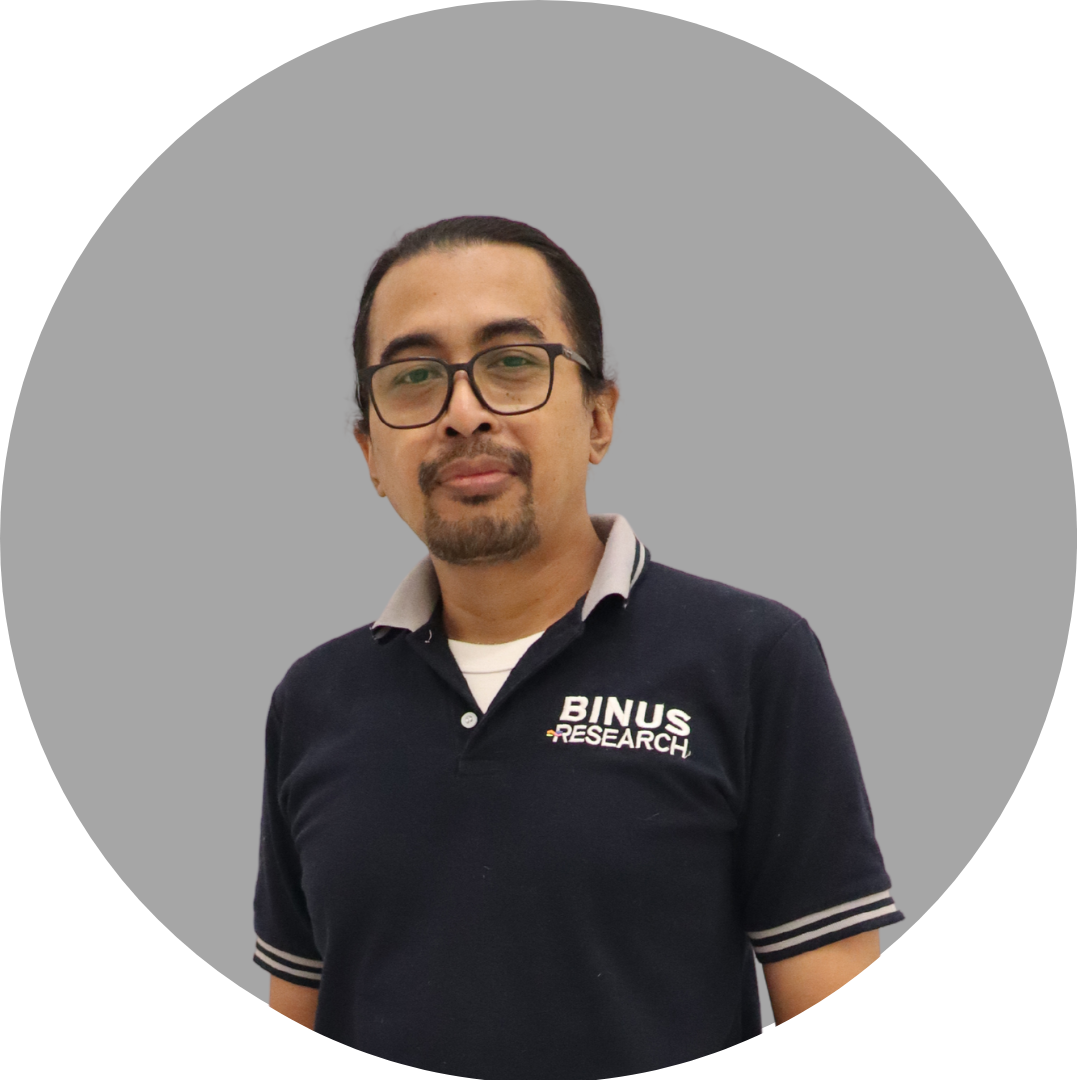
Last updated : April 01, 2024 00:00
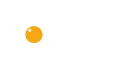
Your browser is not fully compatible with the features of our website.
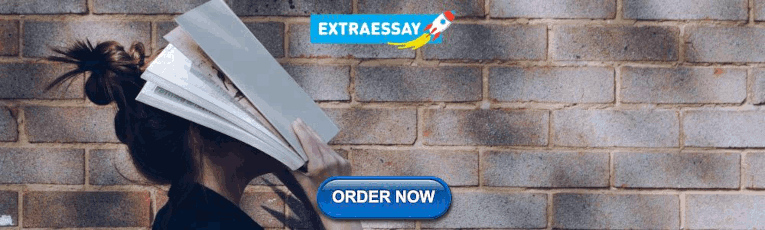
IMAGES
VIDEO
COMMENTS
Learn how Netflix, Google, LinkedIn, Walmart, eBay, Sprint, Mint.com, IRS, CDC, and Woolworths use Big Data to optimize their operations, services, and customer experience. See examples of Big Data applications, analytics, and benefits in different sectors and domains.
The biomedical research and is a huge use case for big data analytics and the applications can themselves form a topic of lengthy discussion. 7. Business and Management. investing in AI and big data to streamline operations, implement digitization and introduce automation, among other business objectives.
Learn how Walmart, Uber, Netflix, eBay and Procter & Gamble use Big Data analytics to optimize their business performance and customer experience. Discover how Big Data also helps to predict and prevent diseases like malaria in Bangladesh.
Learn how Starbucks, Netflix, Coca-Cola, American Express and other companies use Big Data and analytics to gain competitive advantages. See how they collect, analyze and act on data from customers, markets, operations and more.
Learn how big data is used by various industries and companies, such as Netflix, AccuWeather, Etsy, and mLogica, to solve business problems and achieve goals. See the use cases, outcomes, and solutions for each case study.
Learn how Google, Amazon, Facebook, GE, and other companies use big data to drive business performance. This PDF booklet contains articles from Bernard Marr's LinkedIn blog, covering topics such as semantic search, industrial internet, and crowd prediction.
Learn how big data can transform data storage and analytics for various industries, such as healthcare, banking, and manufacturing. See real-world examples of big data applications and how to use Tableau to visualize and analyze data.
Perez says UPS is using technology to improve its flexibility, capability, and efficiency, and that the right insight at the right time helps line-of-business managers to improve performance. The ...
Learn from six senior leaders how they overcome challenges and capture value from big data and analytics in their organizations. See examples of how they use data to improve customer experience, optimize network, and drive innovation.
Learn how companies of different sizes and industries use big data for various purposes, such as customer analytics, data warehouse optimization, and fraud detection. See real-life examples, statistics, and insights from big data experts and consultants.
37 Big Data Case Studies with Big Results. 71shares. Buffer. By Rob Petersen, {grow} Community Member. Big Data is the collection of large amounts of data from places like web-browsing data trails, social network communications, sensor and surveillance data that is stored in computer clouds then searched for patterns, new revelations and insights.
You haven't seen big data in action until you've seen Gartner analyst Doug Laney present 55 examples of big data case studies in 55 minutes. It's kind of like The Complete Works of Shakespeare, Laney joked at Gartner Symposium, though "less entertaining and hopefully more informative."(Well, maybe, for this tech crowd.) The presentation was, without question, a master class on the three Vs ...
Abstract. Big data has disrupted entire industries. Innovative use case in the fields of financial services, telecommunications, transportation, health care, retail, insurance, utilities, energy, and technology (to mention a few) have revolutionized the way organizations manage, process, and analyze data.
The case discusses in detail how Procter & Gamble adapted the big data through different tools like Decision Cockpit and Business Sphere.". Strategy. Vinod commented: "The case helps understands many strategic, as well as technical aspects of big data and business analytics, and how they are implemented in a fast-moving consumer goods (FMCG ...
5. Improved personalization and recommendation. One of the most popular uses of big data is to improve product recommendations and personalization of websites and services. The challenge with online offerings is that there are sometimes an overwhelming number of choices.
In this big data use case, we'll look at how Amazon is leveraging data analytic technologies to improve products and services and drive overall revenue. Big data has changed how we interact with the world and continue strengthening its hold on businesses worldwide. New data sets can be mined, managed, and analyzed using a combination of ...
Big data use cases 1-3. Retail Big data use cases 4-8. Healthcare Big data use cases 9-12. Oil and gas Big data use cases 13-15. Telecommunications. Big data use cases 16-18. Financial services. Big data use cases 19-22. If yours isn't among them, you'll still find the use cases informative and applicable.
The V's of Big Data . Volume: As of May 2019, Netflix has around 13,612 titles (Gaël, 2019). Their US library alone consists of 5087 titles. As of 2016, Netflix has completed its migration to Amazon Web Services. Their data of tens of petabytes of data was moved to AWS (Brodkin et al., 2016).
February 18, 2016. by: Laura Winig. GE has bet big on the Industrial Internet — the convergence of industrial machines, data, and the Internet. The company is putting sensors on gas turbines, jet engines, and other machines; connecting them to the cloud; and analyzing the resulting flow of data. The goal: identify ways to improve machine ...
A big data analysis case study of amazon. Big data is one of the advanced technologies mainly used for evaluating and integrating the collected data in the companies. The use of big data is ...
By Jessica Kent. December 10, 2020 - Researchers at Johns Hopkins Bloomberg School of Public Health have developed a series of case studies for public health issues that will enable healthcare leaders to use big data analytics tools in their work. The Open Case Studies project offers an interactive online hub made up of ten case studies that ...
Big data in healthcare is a rapidly evolving field that offers promising opportunities for improving health outcomes, reducing costs, and enhancing patient satisfaction. However, it also poses significant challenges, such as data quality, privacy, security, and ethical issues. This article provides an overview of the current state and future directions of big data in healthcare, as well as ...
The Five 'V's of Big Data. Big Data is simply a catchall term used to describe data too large and complex to store in traditional databases. The "five 'V's" of Big Data are: Volume - The amount of data generated. Velocity - The speed at which data is generated, collected and analyzed. Variety - The different types of structured ...
2. To Create A Better User Experience. We use qualitative data to create a better UX for Web design projects. Through user testing, interviews and usability studies, we gain insights into user ...
Case study: big data and electoral results. Finally, I promised you a data‑crunching exercise of my own. I will show how analysing big data sets with novel techniques can reveal surprising insights. In this case, I have drawn my inspiration from political psychology.
Extreme heat, heavy downpours, and flooding will affect infrastructure, health, agriculture, forestry, transportation, air and water quality, and more. Climate change will also worsen a range of risks to the Great Lakes. Southwest. Climate change has caused increased heat, drought, and insect outbreaks.
On the 26th of February 2024, BINUS University hosted an insightful workshop aimed at enhancing the academic writing skills of its faculty members. Titled "How to Write a Paper from Case Study/Business Report," the workshop featured Assoc. Prof. Dr. Nanthakumar Loganathan from Universiti Teknologi Malaysia as the distinguished speaker. The event held at Bandung Campus, […]