Module 3: Prenatal Development
Behavioral genetics, learning outcomes.
- Describe the interaction between genetics and the environment
- Compare monozygotic and dizygotic twins
Behavioral geneticists study how individual differences arise, in the present, through the interaction of genes and the environment. When studying human behavior, behavioral geneticists often employ twin and adoption studies to research questions of interest. Twin studies compare the rates that a given behavioral trait is shared among identical and fraternal twins; adoption studies compare those rates among biologically related relatives and adopted relatives. Both approaches provide some insight into the relative importance of genes and environment for the expression of a given trait.
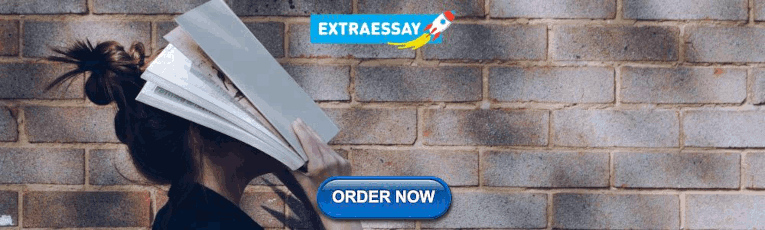
Nature or Nurture?
For decades, scholars have carried on the “nature/nurture” debate. For any particular feature, those on the “nature” side would argue that heredity plays the most important role in bringing about that feature. Those on the “nurture” side would argue that one’s environment is most significant in shaping the way we are. This debate continues in questions about what makes us masculine or feminine (Lippa, 2002), concerns about vision (Mutti, Kadnik, & Adams, 1996), and many other developmental issues.
Most scholars agree that there is a constant interplay between the two forces. It is difficult to isolate the root of any single behavior as a result solely of nature or nurture, and most scholars believe that even determining the extent to which nature or nurture impacts a human feature is difficult to answer. In fact, almost all human features are polygenic (a result of many genes) and multifactorial (a result of many factors, both genetic and environmental). It is as if one’s genetic make-up sets up a range of possibilities, which may or may not be realized depending upon one’s environmental experiences. For instance, a person might be genetically predisposed to develop diabetes, but the person’s lifestyle may help bring about the disease.
When you think about your own family history, it is easy to see that there are certain personality traits, behavioral characteristics, and medical conditions that are more common than others. This is the reason that doctors ask you about your family medical history. While genetic predisposition is important to consider, there are some family members who, for a variety of reasons, seemed to defy the odds of developing these conditions. These differences can be explained in part by the effect of epigenetic (above the genome) changes.
This video explains some of the research that gives insights into the complicated relationship between nature and nurture.
You can view the transcript for “Epigenetics: Nature vs nurture” here (opens in new window) .
The Epigenetic Framework
The term “epigenetic” has been used in developmental psychology to describe psychological development as the result of an ongoing, bi-directional interchange between heredity and the environment. Gottlieb (1998; 2000; 2002) suggests an analytic framework for the nature/nurture debate that recognizes the interplay between the environment, behavior, and genetic expression. This bidirectional interplay suggests that the environment can affect the expression of genes just as genetic predispositions can impact a person’s potentials. Likewise, environmental circumstances can trigger symptoms of a genetic disorder. For example, a person predisposed genetically for type 2 diabetes may trigger the disease through poor diet and little exercise.
The developmental psychologist Erik Erikson wrote of an epigenetic principle in his book Identity: Youth and Crisis (1968), encompassing the notion that we develop through an unfolding of our personality in predetermined stages, and that our environment and surrounding culture influence how we progress through these stages. This biological unfolding in relation to our socio-cultural settings is done in stages of psychosocial development, where “progress through each stage is in part determined by our success, or lack of success, in all the previous stages.”
In typical human families, children’s biological parents raise them, so it is very difficult to know whether children act like their parents due to genetic (nature) or environmental (nurture) reasons. Nevertheless, despite our restrictions on setting up human-based experiments, we do see real-world examples of nature-nurture at work in the human sphere—though they only provide partial answers to our many questions. The science of how genes and environments work together to influence behavior is called behavioral genetics . The easiest opportunity we have to observe this is the adoption study . When children are put up for adoption, the parents who give birth to them are no longer the parents who raise them. Children aren’t assigned to random adoptive parents in order to suit the particular interests of a scientist but adoption still tells us some interesting things, or at least confirms some basic expectations. For instance, if the biological child of tall parents were adopted into a family of short people, do you suppose the child’s growth would be affected? What about the biological child of a Spanish-speaking family adopted at birth into an English-speaking family? What language would you expect the child to speak? And what might these outcomes tell you about the difference between height and language in terms of nature-nurture?
Monozygotic and Dizygotic Twins
Another option for observing nature-nurture in humans involves twin studies . To analyze nature–nurture using twins, we compare the similarity of monozygotic and dizygotic pairs. Monozygotic twins occur when a single zygote or fertilized egg splits apart in the first two weeks of development. The result is the creation of two separate but genetically identical offspring. About one-third of twins are monozygotic twins. Monozygotic twins occur in birthing at a rate of about 3 in every 1000 deliveries worldwide (about 0.3% of the world population). Monozygotic twins are genetically nearly identical and they are always the same sex unless there has been a mutation during development. The children of monozygotic twins test genetically as half-siblings (or full siblings, if a pair of monozygotic twins reproduces with another pair of identical twins or with the same person), rather than first cousins.
Sometimes two eggs or ova are released and fertilized by two separate sperm. The result is dizygotic or fraternal twins. About two-thirds of twins are dizygotic. These two individuals share the same amount of genetic material as would any two children from the same mother and father. Older mothers are more likely to have dizygotic twins than are younger mothers and couples who use fertility drugs are also more likely to give birth to dizygotic twins. Consequently, there has been an increase in the number of fraternal twins in recent years (Bortolus et al., 1999). In vitro fertilization (IVF) techniques are more likely to create dizygotic twins. For IVF deliveries, there are nearly 21 pairs of twins for every 1,000.
In the uterus, a majority of monozygotic twins (60–70%) share the same placenta but have separate amniotic sacs. The placenta is a temporary organ that connects the developing fetus via the umbilical cord to the uterine wall to allow nutrient uptake, thermo-regulation, waste elimination, and gas exchange via the mother’s blood supply. The amniotic sac (also called the bag of waters or the membranes), is a thin but tough transparent pair of membranes that hold a developing embryo (and later fetus) until shortly before birth. In 18–30% of monozygotic twins each fetus has a separate placenta and a separate amniotic sac. A small number (1–2%) of monozygotic twins share the same placenta and amniotic sac. Fraternal twins each have their own placenta and own amniotic sac.
Figure 1. Monozygotic twins come from a single zygote and generally share the same placenta, although some (18-30%) have separate placentas. Dizygotic twins come from two separately fertilized eggs and have their own placentas and amniotic sacs.
Monozygotic (one egg/identical) twins can be categorized into four types depending on the timing of the separation and duplication of cells. Various types of chorionicity and amniosity (how the baby’s sac looks) in monozygotic twins are a result of when the fertilized egg divides. This is known as placentation.
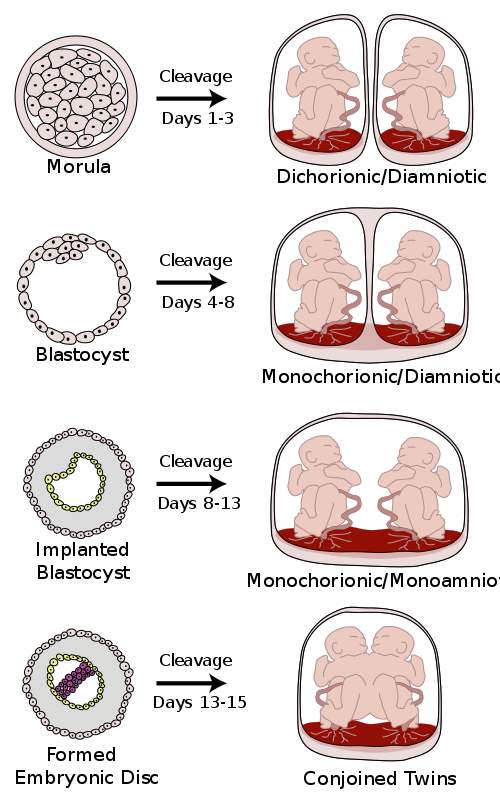
Figure 2. Various types of chorionicity and amniosity (how the baby’s sac looks) in monozygotic (one egg/identical) twins as a result of when the fertilized egg divides (Author Kevin Dufenbach)
Conjoined twins
Conjoined twins are monozygotic twins whose bodies are joined together during pregnancy. This occurs when the zygote starts to split after day 12 following fertilization and fails to separate completely. This condition occurs in about 1 in 50,000 human pregnancies. Most conjoined twins are now evaluated for surgery to attempt to separate them into separate functional bodies. The degree of difficulty rises if a vital organ or structure is shared between twins, such as the brain, heart or liver.
Vanishing twins
Researchers suspect that as many as 1 in 8 pregnancies start out as multiples, but only a single fetus is brought to full term because the other fetus has died very early in the pregnancy and has not been detected or recorded. Early obstetric ultrasonography exams sometimes reveal an “extra” fetus, which fails to develop and instead disintegrates and vanishes in the uterus. There are several reasons for the “vanishing” fetus, including it being embodied or absorbed by the other fetus, placenta or the mother. This is known as vanishing twin syndrome. Also, in an unknown proportion of cases, two zygotes may fuse soon after fertilization, resulting in a single chimeric embryo, and, later, fetus.
Twin Studies
Using the features of height and spoken language as examples, let’s take a look at how nature and nurture apply: identical twins, unsurprisingly, are almost perfectly similar for height. The heights of fraternal twins, however, are like any other sibling pairs: more similar to each other than to people from other families, but hardly identical. This contrast between twin types gives us a clue about the role genetics plays in determining height.
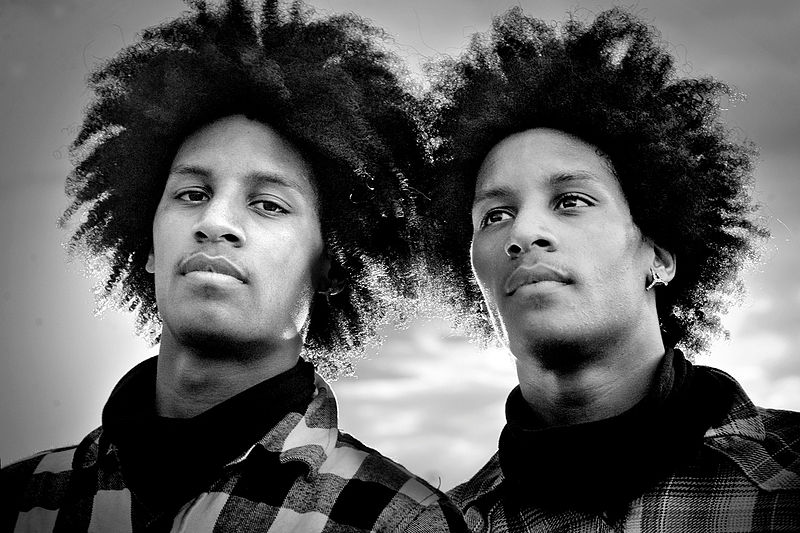
Figure 3. Identical twins Laurent and Larry Nicolas Bourgeois, also known as the Les Twins, are internationally renowned dancers.
Now consider spoken language. If one identical twin speaks Spanish at home, the co-twin with whom she is raised almost certainly does too. But the same would be true for a pair of fraternal twins raised together. In terms of spoken language, fraternal twins are just as similar as identical twins, so it appears that the genetic match of identical twins doesn’t make much difference.
Twin and adoption studies are two instances of a much broader class of methods for observing nature-nurture called quantitative genetics , the scientific discipline in which similarities among individuals are analyzed based on how biologically related they are. We can do these studies with siblings and half-siblings, cousins, and twins who have been separated at birth and raised separately (Bouchard, Lykken, McGue, & Segal, 1990). Such twins are very rare and play a smaller role than is commonly believed in the science of nature–nurture, or with entire extended families (Plomin, DeFries, Knopik, & Neiderhiser, 2012).
It would be satisfying to be able to say that nature–nurture studies have given us conclusive and complete evidence about where traits come from, with some traits clearly resulting from genetics and others almost entirely from environmental factors, such as child-rearing practices and personal will; but that is not the case. Instead, everything has turned out to have some footing in genetics. The more genetically-related people are, the more similar they are—for everything : height, weight, intelligence, personality, mental illness, etc. Sure, it seems like common sense that some traits have a genetic bias. For example, adopted children resemble their biological parents even if they have never met them, and identical twins are more similar to each other than are fraternal twins. And while certain psychological traits, such as personality or mental illness (e.g., schizophrenia), seem reasonably influenced by genetics, it turns out that the same is true for political attitudes, how much television people watch (Plomin, Corley, DeFries, & Fulker, 1990), and whether or not they get divorced (McGue & Lykken, 1992).
Contribute!
Improve this page Learn More
- Modification, adaptation, and original content. Authored by : Julie Lazzara for Lumen Learning. Provided by : Lumen Learning. License : CC BY: Attribution
- Psyc 200 Lifespan Psychology. Authored by : Laura Overstreet. Located at : http://opencourselibrary.org/econ-201/ . License : Public Domain: No Known Copyright
- The Nature Nurture Question. Provided by : Lumen Learning. Located at : https://courses.lumenlearning.com/waymaker-psychology/chapter/the-nature-nurture-question/ . License : CC BY: Attribution
- Epigenetics. Provided by : Wikipedia. Located at : https://en.wikipedia.org/wiki/Epigenetics . License : CC BY-SA: Attribution-ShareAlike
- Les Twins. Authored by : Shawn Welling. Provided by : Wikipedia. Located at : https://en.wikipedia.org/wiki/File:Les_Twins_profile.jpg . License : CC BY-SA: Attribution-ShareAlike
- Twins. Provided by : Wikipedia. Located at : https://en.wikipedia.org/wiki/Twin . License : CC BY-SA: Attribution-ShareAlike
- Epigenetics: Nature vs nurture. Authored by : Det medisinske fakultet UiO. Located at : https://www.youtube.com/watch?v=k50yMwEOWGU . License : Other . License Terms : Standard YouTube License
Want to create or adapt books like this? Learn more about how Pressbooks supports open publishing practices.
4.3 Behavioral Genetics
Learning outcomes.
- Describe the interaction between genetics and the environment
- Compare monozygotic and dizygotic twins
Behavioral geneticists study how individual differences arise, in the present, through the interaction of genes and the environment. When studying human behavior, behavioral geneticists often employ twin and adoption studies to research questions of interest. Twin studies compare the rates that a given behavioral trait is shared among identical and fraternal twins; adoption studies compare those rates among biologically related relatives and adopted relatives. Both approaches provide some insight into the relative importance of genes and environment for the expression of a given trait.
Nature or Nurture?
For decades, scholars have carried on the “nature/nurture” debate. For any particular feature, those on the “nature” side would argue that heredity plays the most important role in bringing about that feature. Those on the “nurture” side would argue that one’s environment is most significant in shaping the way we are. This debate continues in questions about what makes us masculine or feminine (Lippa, 2002), concerns about vision (Mutti et al. 1996), and many other developmental issues.
Most scholars agree that there is a constant interplay between the two forces. It is difficult to isolate the root of any single behavior as a result solely of nature or nurture, and most scholars believe that even determining the extent to which nature or nurture impacts a human feature is difficult to answer. In fact, almost all human features are polygenic (a result of many genes) and multifactorial (a result of many factors, both genetic and environmental). It is as if one’s genetic make-up sets up a range of possibilities, which may or may not be realized depending upon one’s environmental experiences. For instance, a person might be genetically predisposed to develop diabetes, but the person’s lifestyle may help bring about the disease.
When you think about your own family history, it is easy to see that there are certain personality traits, behavioral characteristics, and medical conditions that are more common than others. This is the reason that doctors ask you about your family medical history. While genetic predisposition is important to consider, there are some family members who, for a variety of reasons, seemed to defy the odds of developing these conditions. These differences can be explained in part by the effect of epigenetic (above the genome) changes.
You can view the transcript for “Epigenetics: Nature vs nurture” here (opens in new window) .
The Epigenetic Framework
The term “epigenetic” has been used in developmental psychology to describe psychological development as the result of an ongoing, bi-directional interchange between heredity and the environment. Gottlieb (1998; 2000; 2002) suggests an analytic framework for the nature/nurture debate that recognizes the interplay between the environment, behavior, and genetic expression. This bidirectional interplay suggests that the environment can affect the expression of genes just as genetic predispositions can impact a person’s potentials. Likewise, environmental circumstances can trigger symptoms of a genetic disorder. For example, a person predisposed genetically for type 2 diabetes may trigger the disease through poor diet and little exercise.
The developmental psychologist Erik Erikson wrote of an epigenetic principle in his book Identity: Youth and Crisis (1968), encompassing the notion that we develop through an unfolding of our personality in predetermined stages, and that our environment and surrounding culture influence how we progress through these stages. This biological unfolding in relation to our socio-cultural settings is done in stages of psychosocial development, where “progress through each stage is in part determined by our success, or lack of success, in all the previous stages.”
In typical human families, children’s biological parents raise them, so it is very difficult to know whether children act like their parents due to genetic (nature) or environmental (nurture) reasons. Nevertheless, despite our restrictions on setting up human-based experiments, we do see real-world examples of nature-nurture at work in the human sphere—though they only provide partial answers to our many questions. The science of how genes and environments work together to influence behavior is called behavioral genetics . The easiest opportunity we have to observe this is the adoption study . When children are put up for adoption, the parents who give birth to them are no longer the parents who raise them. Children aren’t assigned to random adoptive parents in order to suit the particular interests of a scientist but adoption still tells us some interesting things, or at least confirms some basic expectations. For instance, if the biological child of tall parents were adopted into a family of short people, do you suppose the child’s growth would be affected? What about the biological child of a Spanish-speaking family adopted at birth into an English-speaking family? What language would you expect the child to speak? And what might these outcomes tell you about the difference between height and language in terms of nature-nurture?
Monozygotic and Dizygotic Twins
Another option for observing nature-nurture in humans involves twin studies . To analyze nature–nurture using twins, we compare the similarity of monozygotic and dizygotic pairs. Monozygotic twins occur when a single zygote or fertilized egg splits apart in the first two weeks of development. The result is the creation of two separate but genetically identical offspring. About one-third of twins are monozygotic twins. Monozygotic twins occur in birthing at a rate of about 3 in every 1000 deliveries worldwide (about 0.3% of the world population). Monozygotic twins are genetically nearly identical and they are always the same sex unless there has been a mutation during development. The children of monozygotic twins test genetically as half-siblings (or full siblings, if a pair of monozygotic twins reproduces with another pair of identical twins or with the same person), rather than first cousins.
Sometimes two eggs or ova are released and fertilized by two separate sperm. The result is dizygotic or fraternal twins. About two-thirds of twins are dizygotic. These two individuals share the same amount of genetic material as would any two children from the same two parents. Older gestational parents are more likely to have dizygotic twins than are younger gestational parents and couples who use fertility drugs are also more likely to give birth to dizygotic twins. Consequently, there has been an increase in the number of fraternal twins in recent years (Bortolus et al., 1999). In vitro fertilization (IVF) techniques are more likely to create dizygotic twins. For IVF deliveries, there are nearly 21 pairs of twins for every 1,000.
In the uterus, a majority of monozygotic twins (60–70%) share the same placenta but have separate amniotic sacs. The placenta is a temporary organ that connects the developing fetus via the umbilical cord to the uterine wall to allow nutrient uptake, thermo-regulation, waste elimination, and gas exchange via the gestational parent’s blood supply. The amniotic sac (also called the bag of waters or the membranes), is a thin but tough transparent pair of membranes that hold a developing embryo (and later fetus) until shortly before birth. In 18–30% of monozygotic twins each fetus has a separate placenta and a separate amniotic sac. A small number (1–2%) of monozygotic twins share the same placenta and amniotic sac. Fraternal twins each have their own placenta and own amniotic sac.
Monozygotic (one egg/identical) twins can be categorized into four types depending on the timing of the separation and duplication of cells. Various types of chorionicity and amniosity (how the baby’s sac looks) in monozygotic twins are a result of when the fertilized egg divides. This is known as placentation.
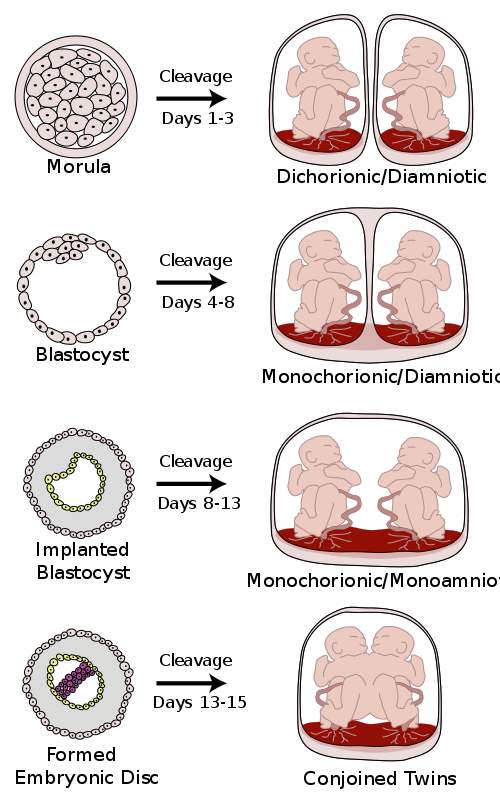
Conjoined twins
Conjoined twins are monozygotic twins whose bodies are joined together during pregnancy. This occurs when the zygote starts to split after day 12 following fertilization and fails to separate completely. This condition occurs in about 1 in 50,000 human pregnancies. Most conjoined twins are now evaluated for surgery to attempt to separate them into separate functional bodies. The degree of difficulty rises if a vital organ or structure is shared between twins, such as the brain, heart or liver.
Vanishing twins
Researchers suspect that as many as 1 in 8 pregnancies start out as multiples, but only a single fetus is brought to full term because the other fetus has died very early in the pregnancy and has not been detected or recorded. Early obstetric ultrasonography exams sometimes reveal an “extra” fetus, which fails to develop and instead disintegrates and vanishes in the uterus. There are several reasons for the “vanishing” fetus, including it being embodied or absorbed by the other fetus, placenta, or the gestational parent. This is known as vanishing twin syndrome. Also, in an unknown proportion of cases, two zygotes may fuse soon after fertilization, resulting in a single chimeric embryo, and, later, fetus.
Twin Studies
Using the features of height and spoken language as examples, let’s take a look at how nature and nurture apply: identical twins, unsurprisingly, are almost perfectly similar for height. The heights of fraternal twins, however, are like any other sibling pairs: more similar to each other than to people from other families, but hardly identical. This contrast between twin types gives us a clue about the role genetics plays in determining height.
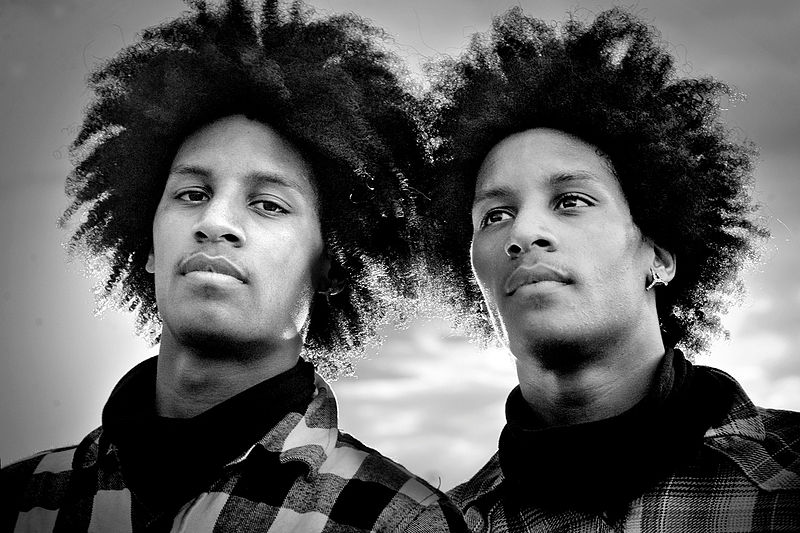
Now consider spoken language. If one identical twin speaks Spanish at home, the co-twin with whom she is raised almost certainly does too. But the same would be true for a pair of fraternal twins raised together. In terms of spoken language, fraternal twins are just as similar as identical twins, so it appears that the genetic match of identical twins doesn’t make much difference.
Twin and adoption studies are two instances of a much broader class of methods for observing nature-nurture called quantitative genetics , the scientific discipline in which similarities among individuals are analyzed based on how biologically related they are. We can do these studies with siblings and half-siblings, cousins, and twins who have been separated at birth and raised separately (Bouchard et al., 1990). Such twins are very rare and play a smaller role than is commonly believed in the science of nature–nurture, or with entire extended families (Plomin et al., 2012).
It would be satisfying to be able to say that nature–nurture studies have given us conclusive and complete evidence about where traits come from, with some traits clearly resulting from genetics and others almost entirely from environmental factors, such as child-rearing practices and personal will; but that is not the case. Instead, everything has turned out to have some footing in genetics. The more genetically-related people are, the more similar they are—for everything : height, weight, intelligence, personality, mental illness, etc. Sure, it seems like common sense that some traits have a genetic bias. For example, adopted children resemble their biological parents even if they have never met them, and identical twins are more similar to each other than are fraternal twins. And while certain psychological traits, such as personality or mental illness (e.g., schizophrenia), seem reasonably influenced by genetics, it turns out that the same is true for political attitudes, how much television people watch (Plomin et al. 1990), and whether or not they get divorced (McGue & Lykken, 1992).
Bortolus, R., Parazzini, F., Chatenoud, L., Benzi, G., Bianchi, M. M., & Marini, A. (1999). The epidemiology of multiple births. Human Reproduction Update, 5, 179-187.
Bouchard Jr, T. J., Lykken, D. T., McGue, M., Segal, N. L., & Tellegen, A. (1990). Sources of human psychological differences: The Minnesota study of twins reared apart. Science , 250 (4978), 223-228.
Erikson, E. H. (1968). Identity: Youth and Crisis (No. 7). WW Norton & company.
Gottlieb, G. (1998). Normally occurring environmental and behavioral influences on gene activity: From central dogma to probabilistic epigenesis. Psychological Review, 105 , 792-802.
Gottlieb, G. (2000). Environmental and behavioral influences on gene activity. Current Directions in Psychological Science, 9 , 93-97.
Gottlieb, G. (2002). Individual development and evolution: The genesis of novel behavior. New York: Oxford University Press.
Lippa, R. A. (2002). Gender, nature, and nurture . Mahwah, NJ: L. Erlbaum.
McGue, M., & Lykken, D. T. (1992). Genetic influence on risk of divorce. Psychological Science, 3 (6), 368-373.
Mutti, D. O., Zadnik, K., & Adams, A. J. (n.d.). Myopia. The nature versus nurture debate goes on. Investigative Ophthalmology & Visual Science. Retrieved May 03, 2011, from http://www.iovs.org/cgi/reprint/37/6/952
Plomin, R., Corley, R., DeFries, J. C., & Fulker, D. W. (1990). Individual differences in television viewing in early childhood: Nature as well as nurture. Psychological Science , 1(6), 371-377.
Plomin R. DeFries J. C. Knopik V. S. & Neiderhiser J. M. (2013). Behavioral genetics (Sixth). Worth.
CC Licensed Content
- Behavioral Genetics. Authored by : Julie Lazzara for Lumen Learning. Provided by : Lumen Learning. Located at: https://courses.lumenlearning.com/wm-lifespandevelopment/chapter/behavioral-genetics/ . License : CC BY: Attribution
- Psyc 200 Lifespan Psychology. Authored by : Laura Overstreet. Located at : http://opencourselibrary.org/econ-201/ . License : Public Domain: No Known Copyright
- The Nature Nurture Question. Provided by : Lumen Learning. Located at : https://courses.lumenlearning.com/waymaker-psychology/chapter/the-nature-nurture-question/ . License : CC BY: Attribution
- Epigenetics. Provided by : Wikipedia. Located at : https://en.wikipedia.org/wiki/Epigenetics . License : CC BY-SA: Attribution-ShareAlike
Media Attributions
- Les Twins. Authored by : Shawn Welling. Provided by : Wikipedia. Located at : https://en.wikipedia.org/wiki/File:Les_Twins_profile.jpg . License : CC BY-SA: Attribution-ShareAlike
- Identical-Fraternal Twins. Provided by : Wikipedia. Located at : https://commons.wikimedia.org/wiki/File:Identical-fraternal-sperm-egg.svg . License : CC BY-SA: Attribution-ShareAlike
- Placentation. Provided by : Wikipedia. Located at : https://commons.wikimedia.org/wiki/File:Placentation.svg . License : CC BY-SA: Attribution-ShareAlike
- Epigenetics: Nature vs nurture. Authored by : Det medisinske fakultet UiO. Located at : https://www.youtube.com/watch?v=k50yMwEOWGU . License : Other . License Terms : Standard YouTube License
4.3 Behavioral Genetics Copyright © by Meredith Palm is licensed under a Creative Commons Attribution 4.0 International License , except where otherwise noted.
Share This Book
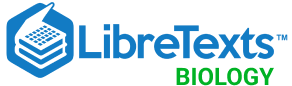
- school Campus Bookshelves
- menu_book Bookshelves
- perm_media Learning Objects
- login Login
- how_to_reg Request Instructor Account
- hub Instructor Commons
- Download Page (PDF)
- Download Full Book (PDF)
- Periodic Table
- Physics Constants
- Scientific Calculator
- Reference & Cite
- Tools expand_more
- Readability
selected template will load here
This action is not available.
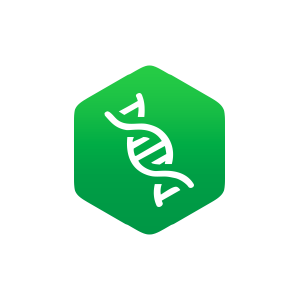
53.3: Behavioral Genetics
- Last updated
- Save as PDF
- Page ID 74415
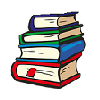
Skills to Develop
- Compare innate and learned behavior
- Discuss how movement and migration behaviors are a result of natural selection
- Discuss the different ways members of a population communicate with each other
- Give examples of how species use energy for mating displays and other courtship behaviors
- Differentiate between various mating systems
- Describe different ways that species learn
Behavior is the change in activity of an organism in response to a stimulus. Behavioral biology is the study of the biological and evolutionary bases for such changes. The idea that behaviors evolved as a result of the pressures of natural selection is not new. Animal behavior has been studied for decades, by biologists in the science of ethology , by psychologists in the science of comparative psychology, and by scientists of many disciplines in the study of neurobiology. Although there is overlap between these disciplines, scientists in these behavioral fields take different approaches. Comparative psychology is an extension of work done in human and behavioral psychology. Ethology is an extension of genetics, evolution, anatomy, physiology, and other biological disciplines. Still, one cannot study behavioral biology without touching on both comparative psychology and ethology.
One goal of behavioral biology is to dissect out the innate behaviors , which have a strong genetic component and are largely independent of environmental influences, from the learned behaviors , which result from environmental conditioning. Innate behavior, or instinct, is important because there is no risk of an incorrect behavior being learned. They are “hard wired” into the system. On the other hand, learned behaviors, although riskier, are flexible, dynamic, and can be altered according to changes in the environment.
Innate Behaviors: Movement and Migration
Innate or instinctual behaviors rely on response to stimuli. The simplest example of this is a reflex action , an involuntary and rapid response to stimulus. To test the “knee-jerk” reflex, a doctor taps the patellar tendon below the kneecap with a rubber hammer. The stimulation of the nerves there leads to the reflex of extending the leg at the knee. This is similar to the reaction of someone who touches a hot stove and instinctually pulls his or her hand away. Even humans, with our great capacity to learn, still exhibit a variety of innate behaviors.
Kinesis and Taxis
Another activity or movement of innate behavior is kinesis , or the undirected movement in response to a stimulus. Orthokinesis is the increased or decreased speed of movement of an organism in response to a stimulus. Woodlice, for example, increase their speed of movement when exposed to high or low temperatures. This movement, although random, increases the probability that the insect spends less time in the unfavorable environment. Another example is klinokinesis, an increase in turning behaviors. It is exhibited by bacteria such as E. coli which, in association with orthokinesis, helps the organisms randomly find a more hospitable environment.
A similar, but more directed version of kinesis is taxis : the directed movement towards or away from a stimulus. This movement can be in response to light (phototaxis), chemical signals (chemotaxis), or gravity (geotaxis) and can be directed toward (positive) or away (negative) from the source of the stimulus. An example of a positive chemotaxis is exhibited by the unicellular protozoan Tetrahymena thermophila . This organism swims using its cilia, at times moving in a straight line, and at other times making turns. The attracting chemotactic agent alters the frequency of turning as the organism moves directly toward the source, following the increasing concentration gradient.
Fixed Action Patterns
A fixed action pattern is a series of movements elicited by a stimulus such that even when the stimulus is removed, the pattern goes on to completion. An example of such a behavior occurs in the three-spined stickleback, a small freshwater fish (Figure \(\PageIndex{1}\)). Males of this species develop a red belly during breeding season and show instinctual aggressiveness to other males during this time. In laboratory experiments, researchers exposed such fish to objects that in no way resemble a fish in their shape, but which were painted red on their lower halves. The male sticklebacks responded aggressively to the objects just as if they were real male sticklebacks.
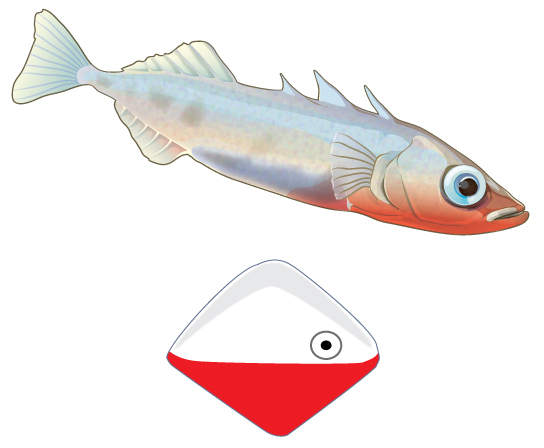
Migration is the long-range seasonal movement of animals. It is an evolved, adapted response to variation in resource availability, and it is a common phenomenon found in all major groups of animals. Birds fly south for the winter to get to warmer climates with sufficient food, and salmon migrate to their spawning grounds. The popular 2005 documentary March of the Penguins followed the 62-mile migration of emperor penguins through Antarctica to bring food back to their breeding site and to their young. Wildebeests (Figure \(\PageIndex{2}\)) migrate over 1800 miles each year in search of new grasslands.
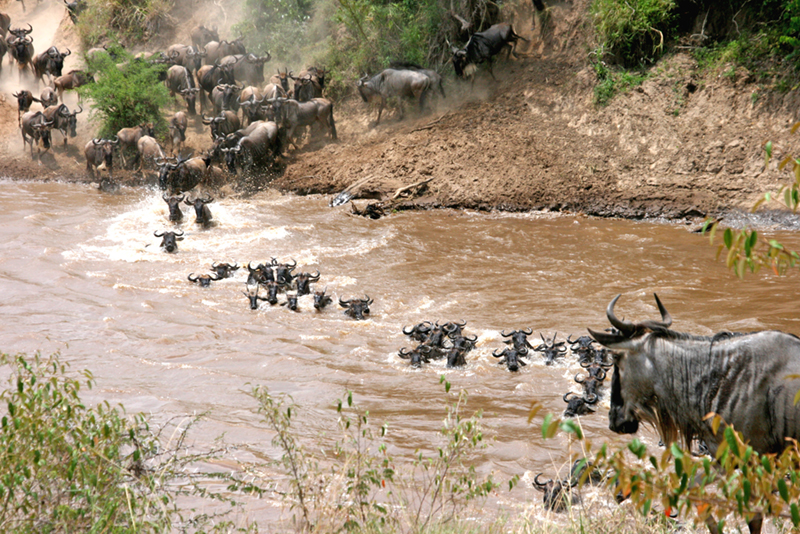
Although migration is thought of as innate behavior, only some migrating species always migrate (obligate migration). Animals that exhibit facultative migration can choose to migrate or not. Additionally, in some animals, only a portion of the population migrates, whereas the rest does not migrate (incomplete migration). For example, owls that live in the tundra may migrate in years when their food source, small rodents, is relatively scarce, but not migrate during the years when rodents are plentiful.
Foraging is the act of searching for and exploiting food resources. Feeding behaviors that maximize energy gain and minimize energy expenditure are called optimal foraging behaviors, and these are favored by natural section. The painted stork, for example, uses its long beak to search the bottom of a freshwater marshland for crabs and other food (Figure \(\PageIndex{3}\)).
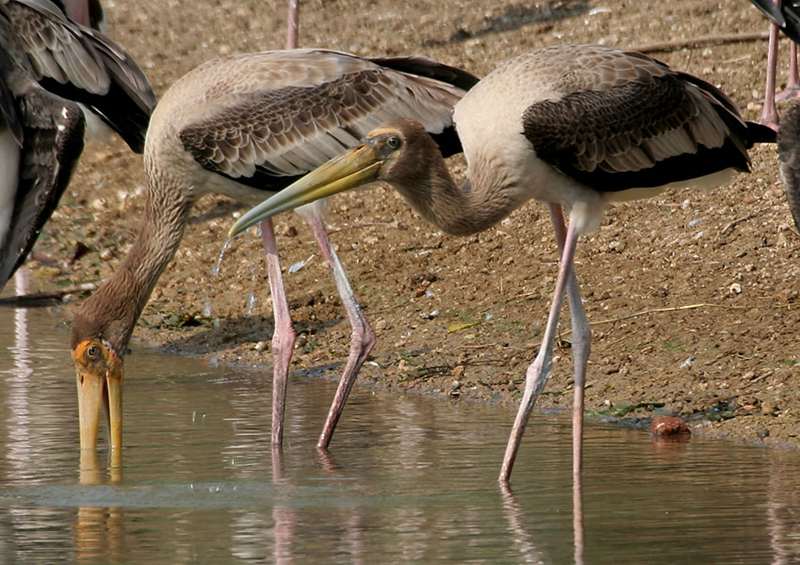
Innate Behaviors: Living in Groups
Not all animals live in groups, but even those that live relatively solitary lives, with the exception of those that can reproduce asexually, must mate. Mating usually involves one animal signaling another so as to communicate the desire to mate. There are several types of energy-intensive behaviors or displays associated with mating, called mating rituals. Other behaviors found in populations that live in groups are described in terms of which animal benefits from the behavior. In selfish behavior, only the animal in question benefits; in altruistic behavior, one animal’s actions benefit another animal; cooperative behavior describes when both animals benefit. All of these behaviors involve some sort of communication between population members.
Communication within a Species
Animals communicate with each other using stimuli known as signals . An example of this is seen in the three-spined stickleback, where the visual signal of a red region in the lower half of a fish signals males to become aggressive and signals females to mate. Other signals are chemical (pheromones), aural (sound), visual (courtship and aggressive displays), or tactile (touch). These types of communication may be instinctual or learned or a combination of both. These are not the same as the communication we associate with language, which has been observed only in humans and perhaps in some species of primates and cetaceans.
A pheromone is a secreted chemical signal used to obtain a response from another individual of the same species. The purpose of pheromones is to elicit a specific behavior from the receiving individual. Pheromones are especially common among social insects, but they are used by many species to attract the opposite sex, to sound alarms, to mark food trails, and to elicit other, more complex behaviors. Even humans are thought to respond to certain pheromones called axillary steroids. These chemicals influence human perception of other people, and in one study were responsible for a group of women synchronizing their menstrual cycles. The role of pheromones in human-to-human communication is still somewhat controversial and continues to be researched.
Songs are an example of an aural signal, one that needs to be heard by the recipient. Perhaps the best known of these are songs of birds, which identify the species and are used to attract mates. Other well-known songs are those of whales, which are of such low frequency that they can travel long distances underwater. Dolphins communicate with each other using a wide variety of vocalizations. Male crickets make chirping sounds using a specialized organ to attract a mate, repel other males, and to announce a successful mating.
Courtship displays are a series of ritualized visual behaviors (signals) designed to attract and convince a member of the opposite sex to mate. These displays are ubiquitous in the animal kingdom. Often these displays involve a series of steps, including an initial display by one member followed by a response from the other. If at any point, the display is performed incorrectly or a proper response is not given, the mating ritual is abandoned and the mating attempt will be unsuccessful. The mating display of the common stork is shown in Figure \(\PageIndex{4}\).
Aggressive displays are also common in the animal kingdom. An example is when a dog bares its teeth when it wants another dog to back down. Presumably, these displays communicate not only the willingness of the animal to fight, but also its fighting ability. Although these displays do signal aggression on the part of the sender, it is thought that these displays are actually a mechanism to reduce the amount of actual fighting that occurs between members of the same species: they allow individuals to assess the fighting ability of their opponent and thus decide whether it is “worth the fight.” The testing of certain hypotheses using game theory has led to the conclusion that some of these displays may overstate an animal’s actual fighting ability and are used to “bluff” the opponent. This type of interaction, even if “dishonest,” would be favored by natural selection if it is successful more times than not.
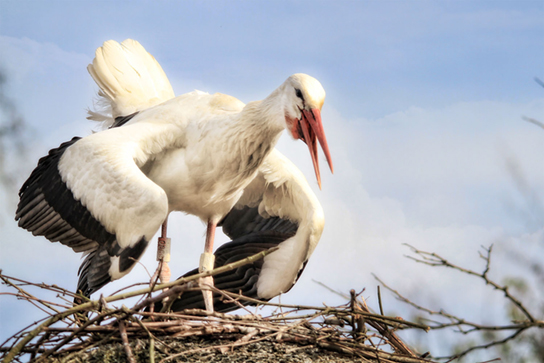
Distraction displays are seen in birds and some fish. They are designed to attract a predator away from the nest that contains their young. This is an example of an altruistic behavior: it benefits the young more than the individual performing the display, which is putting itself at risk by doing so.
Many animals, especially primates, communicate with other members in the group through touch. Activities such as grooming, touching the shoulder or root of the tail, embracing, lip contact, and greeting ceremonies have all been observed in the Indian langur, an Old World monkey. Similar behaviors are found in other primates, especially in the great apes.
Link to Learning
The killdeer bird distracts predators from its eggs by faking a broken wing display in this video taken in Boise, Idaho.
Altruistic Behaviors
Behaviors that lower the fitness of the individual but increase the fitness of another individual are termed altruistic. Examples of such behaviors are seen widely across the animal kingdom. Social insects such as worker bees have no ability to reproduce, yet they maintain the queen so she can populate the hive with her offspring. Meerkats keep a sentry standing guard to warn the rest of the colony about intruders, even though the sentry is putting itself at risk. Wolves and wild dogs bring meat to pack members not present during a hunt. Lemurs take care of infants unrelated to them. Although on the surface, these behaviors appear to be altruistic, it may not be so simple.
There has been much discussion over why altruistic behaviors exist. Do these behaviors lead to overall evolutionary advantages for their species? Do they help the altruistic individual pass on its own genes? And what about such activities between unrelated individuals? One explanation for altruistic-type behaviors is found in the genetics of natural selection. In the 1976 book, The Selfish Gene, scientist Richard Dawkins attempted to explain many seemingly altruistic behaviors from the viewpoint of the gene itself. Although a gene obviously cannot be selfish in the human sense, it may appear that way if the sacrifice of an individual benefits related individuals that share genes that are identical by descent (present in relatives because of common lineage). Mammal parents make this sacrifice to take care of their offspring. Emperor penguins migrate miles in harsh conditions to bring food back for their young. Selfish gene theory has been controversial over the years and is still discussed among scientists in related fields.
Even less-related individuals, those with less genetic identity than that shared by parent and offspring, benefit from seemingly altruistic behavior. The activities of social insects such as bees, wasps, ants, and termites are good examples. Sterile workers in these societies take care of the queen because they are closely related to it, and as the queen has offspring, she is passing on genes from the workers indirectly. Thus, it is of fitness benefit for the worker to maintain the queen without having any direct chance of passing on its genes due to its sterility. The lowering of individual fitness to enhance the reproductive fitness of a relative and thus one’s inclusive fitness evolves through kin selection . This phenomenon can explain many superficially altruistic behaviors seen in animals. However, these behaviors may not be truly defined as altruism in these cases because the actor is actually increasing its own fitness either directly (through its own offspring) or indirectly (through the inclusive fitness it gains through relatives that share genes with it).
Unrelated individuals may also act altruistically to each other, and this seems to defy the “selfish gene” explanation. An example of this observed in many monkey species where a monkey will present its back to an unrelated monkey to have that individual pick the parasites from its fur. After a certain amount of time, the roles are reversed and the first monkey now grooms the second monkey. Thus, there is reciprocity in the behavior. Both benefit from the interaction and their fitness is raised more than if neither cooperated nor if one cooperated and the other did not cooperate. This behavior is still not necessarily altruism, as the “giving” behavior of the actor is based on the expectation that it will be the “receiver” of the behavior in the future, termed reciprocal altruism. Reciprocal altruism requires that individuals repeatedly encounter each other, often the result of living in the same social group, and that cheaters (those that never “give back”) are punished.
Evolutionary game theory, a modification of classical game theory in mathematics, has shown that many of these so-called “altruistic behaviors” are not altruistic at all. The definition of “pure” altruism, based on human behavior, is an action that benefits another without any direct benefit to oneself. Most of the behaviors previously described do not seem to satisfy this definition, and game theorists are good at finding “selfish” components in them. Others have argued that the terms “selfish” and “altruistic” should be dropped completely when discussing animal behavior, as they describe human behavior and may not be directly applicable to instinctual animal activity. What is clear, though, is that heritable behaviors that improve the chances of passing on one’s genes or a portion of one’s genes are favored by natural selection and will be retained in future generations as long as those behaviors convey a fitness advantage. These instinctual behaviors may then be applied, in special circumstances, to other species, as long as it doesn’t lower the animal’s fitness.
Finding Sex Partners
Not all animals reproduce sexually, but many that do have the same challenge: they need to find a suitable mate and often have to compete with other individuals to obtain one. Significant energy is spent in the process of locating, attracting, and mating with the sex partner. Two types of selection occur during this process and can lead to traits that are important to reproduction called secondary sexual characteristics: intersexual selection , the choosing of a mate where individuals of one sex choose mates of the other sex, and intrasexual selection , the competition for mates between species members of the same sex. Intersexual selection is often complex because choosing a mate may be based on a variety of visual, aural, tactile, and chemical cues. An example of intersexual selection is when female peacocks choose to mate with the male with the brightest plumage. This type of selection often leads to traits in the chosen sex that do not enhance survival, but are those traits most attractive to the opposite sex (often at the expense of survival). Intrasexual selection involves mating displays and aggressive mating rituals such as rams butting heads—the winner of these battles is the one that is able to mate. Many of these rituals use up considerable energy but result in the selection of the healthiest, strongest, and/or most dominant individuals for mating. Three general mating systems, all involving innate as opposed to learned behaviors, are seen in animal populations: monogamous, polygynous, and polyandrous.
In monogamous systems, one male and one female are paired for at least one breeding season. In some animals, such as the gray wolf, these associations can last much longer, even a lifetime. Several explanations have been proposed for this type of mating system. The “mate-guarding hypothesis” states that males stay with the female to prevent other males from mating with her. This behavior is advantageous in such situations where mates are scarce and difficult to find. Another explanation is the “male-assistance hypothesis,” where males that remain with a female to help guard and rear their young will have more and healthier offspring. Monogamy is observed in many bird populations where, in addition to the parental care from the female, the male is also a major provider of parental care for the chicks. A third explanation for the evolutionary advantages of monogamy is the “female-enforcement hypothesis.” In this scenario, the female ensures that the male does not have other offspring that might compete with her own, so she actively interferes with the male’s signaling to attract other mates.
Polygynous mating refers to one male mating with multiple females. In these situations, the female must be responsible for most of the parental care as the single male is not capable of providing care to that many offspring. In resourced-based polygyny, males compete for territories with the best resources, and then mate with females that enter the territory, drawn to its resource richness. The female benefits by mating with a dominant, genetically fit male; however, it is at the cost of having no male help in caring for the offspring. An example is seen in the yellow-rumped honeyguide, a bird whose males defend beehives because the females feed on their wax. As the females approach, the male defending the nest will mate with them. Harem mating structures are a type of polygynous system where certain males dominate mating while controlling a territory with resources. Elephant seals, where the alpha male dominates the mating within the group are an example. A third type of polygyny is a lek system. Here there is a communal courting area where several males perform elaborate displays for females, and the females choose their mate from this group. This behavior is observed in several bird species including the sage grouse and the prairie chicken.
In polyandrous mating systems, one female mates with many males. These types of systems are much rarer than monogamous and polygynous mating systems. In pipefishes and seahorses, males receive the eggs from the female, fertilize them, protect them within a pouch, and give birth to the offspring (Figure \(\PageIndex{5}\)). Therefore, the female is able to provide eggs to several males without the burden of carrying the fertilized eggs.
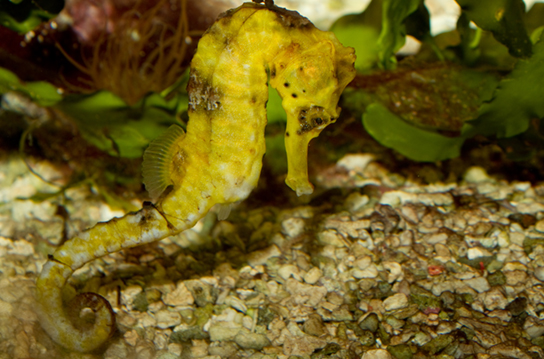
Simple Learned Behaviors
The majority of the behaviors previously discussed were innate or at least have an innate component (variations on the innate behaviors may be learned). They are inherited and the behaviors do not change in response to signals from the environment. Conversely, learned behaviors, even though they may have instinctive components, allow an organism to adapt to changes in the environment and are modified by previous experiences. Simple learned behaviors include habituation and imprinting—both are important to the maturation process of young animals.
Habituation
Habituation is a simple form of learning in which an animal stops responding to a stimulus after a period of repeated exposure. This is a form of non-associative learning, as the stimulus is not associated with any punishment or reward. Prairie dogs typically sound an alarm call when threatened by a predator, but they become habituated to the sound of human footsteps when no harm is associated with this sound, therefore, they no longer respond to them with an alarm call. In this example, habituation is specific to the sound of human footsteps, as the animals still respond to the sounds of potential predators.
Imprinting is a type of learning that occurs at a particular age or a life stage that is rapid and independent of the species involved. Hatchling ducks recognize the first adult they see, their mother, and make a bond with her. A familiar sight is ducklings walking or swimming after their mothers (Figure \(\PageIndex{6}\)). This is another type of non-associative learning, but is very important in the maturation process of these animals as it encourages them to stay near their mother so they will be protected, greatly increasing their chances of survival. However, if newborn ducks see a human before they see their mother, they will imprint on the human and follow it in just the same manner as they would follow their real mother.

The International Crane Foundation has helped raise the world’s population of whooping cranes from 21 individuals to about 600. Imprinting hatchlings has been a key to success: biologists wear full crane costumes so the birds never “see” humans. Watch this video to learn more.
Conditioned Behavior
Conditioned behaviors are types of associative learning, where a stimulus becomes associated with a consequence. During operant conditioning, the behavioral response is modified by its consequences, with regards to its form, strength, or frequency.
Classical Conditioning
In classical conditioning , a response called the conditioned response is associated with a stimulus that it had previously not been associated with, the conditioned stimulus. The response to the original, unconditioned stimulus is called the unconditioned response. The most cited example of classical conditioning is Ivan Pavlov’s experiments with dogs (Figure \(\PageIndex{7}\)). In Pavlov’s experiments, the unconditioned response was the salivation of dogs in response to the unconditioned stimulus of seeing or smelling their food. The conditioning stimulus that researchers associated with the unconditioned response was the ringing of a bell. During conditioning, every time the animal was given food, the bell was rung. This was repeated during several trials. After some time, the dog learned to associate the ringing of the bell with food and to respond by salivating. After the conditioning period was finished, the dog would respond by salivating when the bell was rung, even when the unconditioned stimulus, the food, was absent. Thus, the ringing of the bell became the conditioned stimulus and the salivation became the conditioned response. Although it is thought by some scientists that the unconditioned and conditioned responses are identical, even Pavlov discovered that the saliva in the conditioned dogs had characteristic differences when compared to the unconditioned dog.
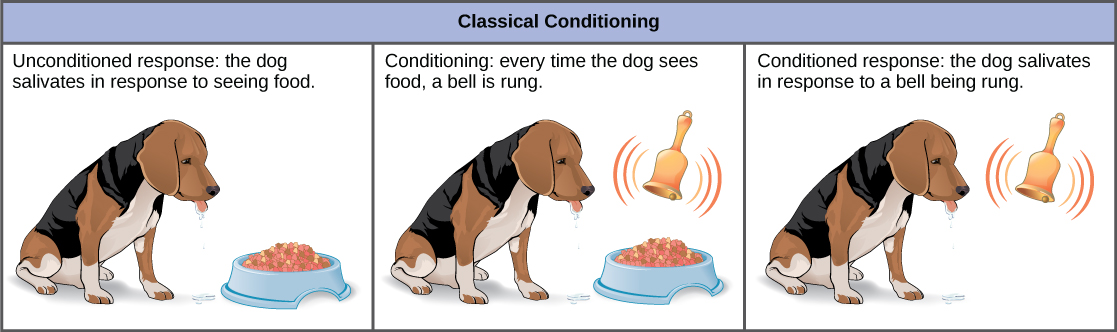
It had been thought by some scientists that this type of conditioning required multiple exposures to the paired stimulus and response, but it is now known that this is not necessary in all cases, and that some conditioning can be learned in a single pairing experiment. Classical conditioning is a major tenet of behaviorism, a branch of psychological philosophy that proposes that all actions, thoughts, and emotions of living things are behaviors that can be treated by behavior modification and changes in the environment.
Operant Conditioning
In operant conditioning , the conditioned behavior is gradually modified by its consequences as the animal responds to the stimulus. A major proponent of such conditioning was psychologist B.F. Skinner, the inventor of the Skinner box. Skinner put rats in his boxes that contained a lever that would dispense food to the rat when depressed. While initially the rat would push the lever a few times by accident, it eventually associated pushing the lever with getting the food. This type of learning is an example of operant conditioning. Operant learning is the basis of most animal training. The conditioned behavior is continually modified by positive or negative reinforcement, often a reward such as food or some type of punishment, respectively. In this way, the animal is conditioned to associate a type of behavior with the punishment or reward, and, over time, can be induced to perform behaviors that they would not have done in the wild, such as the “tricks” dolphins perform at marine amusement park shows (Figure \(\PageIndex{8}\)).
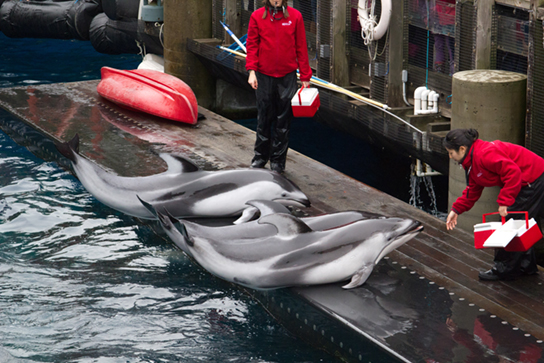
Cognitive Learning
Classical and operant conditioning are inefficient ways for humans and other intelligent animals to learn. Some primates, including humans, are able to learn by imitating the behavior of others and by taking instructions. The development of complex language by humans has made cognitive learning , the manipulation of information using the mind, the most prominent method of human learning. In fact, that is how students are learning right now by reading this book. As students read, they can make mental images of objects or organisms and imagine changes to them, or behaviors by them, and anticipate the consequences. In addition to visual processing, cognitive learning is also enhanced by remembering past experiences, touching physical objects, hearing sounds, tasting food, and a variety of other sensory-based inputs. Cognitive learning is so powerful that it can be used to understand conditioning in detail. In the reverse scenario, conditioning cannot help someone learn about cognition.
Classic work on cognitive learning was done by Wolfgang Köhler with chimpanzees. He demonstrated that these animals were capable of abstract thought by showing that they could learn how to solve a puzzle. When a banana was hung in their cage too high for them to reach, and several boxes were placed randomly on the floor, some of the chimps were able to stack the boxes one on top of the other, climb on top of them, and get the banana. This implies that they could visualize the result of stacking the boxes even before they had performed the action. This type of learning is much more powerful and versatile than conditioning.
Cognitive learning is not limited to primates, although they are the most efficient in using it. Maze running experiments done with rats by H.C. Blodgett in the 1920s were the first to show cognitive skills in a simple mammal. The motivation for the animals to work their way through the maze was a piece of food at its end. In these studies, the animals in Group I were run in one trial per day and had food available to them each day on completion of the run (Figure \(\PageIndex{9}\)). Group II rats were not fed in the maze for the first six days and then subsequent runs were done with food for several days after. Group III rats had food available on the third day and every day thereafter. The results were that the control rats, Group I, learned quickly, and figured out how to run the maze in seven days. Group III did not learn much during the three days without food, but rapidly caught up to the control group when given the food reward. Group II learned very slowly for the six days with no reward to motivate them, and they did not begin to catch up to the control group until the day food was given, and then it took two days longer to learn the maze.
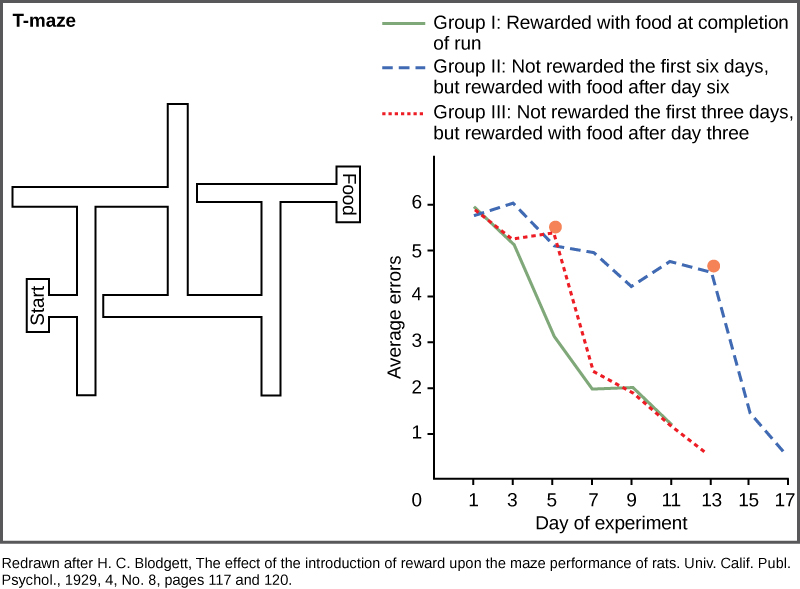
It may not be immediately obvious that this type of learning is different than conditioning. Although one might be tempted to believe that the rats simply learned how to find their way through a conditioned series of right and left turns, E.C. Tolman proved a decade later that the rats were making a representation of the maze in their minds, which he called a “cognitive map.” This was an early demonstration of the power of cognitive learning and how these abilities were not just limited to humans.
Sociobiology
Sociobiology is an interdisciplinary science originally popularized by social insect researcher E.O. Wilson in the 1970s. Wilson defined the science as “the extension of population biology and evolutionary theory to social organization.” 1 The main thrust of sociobiology is that animal and human behavior, including aggressiveness and other social interactions, can be explained almost solely in terms of genetics and natural selection. This science is controversial; noted scientist such as the late Stephen Jay Gould criticized the approach for ignoring the environmental effects on behavior. This is another example of the “nature versus nurture” debate of the role of genetics versus the role of environment in determining an organism’s characteristics.
Sociobiology also links genes with behaviors and has been associated with “biological determinism,” the belief that all behaviors are hardwired into our genes. No one disputes that certain behaviors can be inherited and that natural selection plays a role retaining them. It is the application of such principles to human behavior that sparks this controversy, which remains active today.
Behaviors are responses to stimuli. They can either be instinctual/innate behaviors, which are not influenced by the environment, or learned behaviors, which are influenced by environmental changes. Instinctual behaviors include mating systems and methods of communication. Learned behaviors include imprinting and habituation, conditioning, and, most powerfully, cognitive learning. Although the connection between behavior, genetics, and evolution is well established, the explanation of human behavior as entirely genetic is controversial.
- 1 Edward O. Wilson. On Human Nature (1978; repr., Cambridge: Harvard University Press, 2004), xx.
Thank you for visiting nature.com. You are using a browser version with limited support for CSS. To obtain the best experience, we recommend you use a more up to date browser (or turn off compatibility mode in Internet Explorer). In the meantime, to ensure continued support, we are displaying the site without styles and JavaScript.
- View all journals
- Explore content
- About the journal
- Publish with us
- Sign up for alerts
- Review Article
- Published: 11 May 2020
Using genetics for social science
- K. Paige Harden ORCID: orcid.org/0000-0002-1557-6737 1 &
- Philipp D. Koellinger ORCID: orcid.org/0000-0001-7413-0412 2
Nature Human Behaviour volume 4 , pages 567–576 ( 2020 ) Cite this article
7023 Accesses
68 Citations
512 Altmetric
Metrics details
- Politics and international relations
Social science genetics is concerned with understanding whether, how and why genetic differences between human beings are linked to differences in behaviours and socioeconomic outcomes. Our review discusses the goals, methods, challenges and implications of this research endeavour. We survey how the recent developments in genetics are beginning to provide social scientists with a powerful new toolbox they can use to better understand environmental effects, and we illustrate this with several substantive examples. Furthermore, we examine how medical research can benefit from genetic insights into social-scientific outcomes and vice versa. Finally, we discuss the ethical challenges of this work and clarify several common misunderstandings and misinterpretations of genetic research on individual differences.
This is a preview of subscription content, access via your institution
Access options
Access Nature and 54 other Nature Portfolio journals
Get Nature+, our best-value online-access subscription
24,99 € / 30 days
cancel any time
Subscribe to this journal
Receive 12 digital issues and online access to articles
111,21 € per year
only 9,27 € per issue
Rent or buy this article
Prices vary by article type
Prices may be subject to local taxes which are calculated during checkout
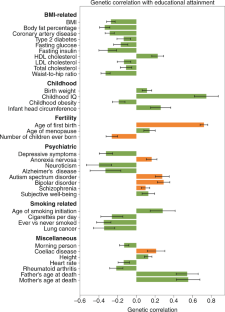
Similar content being viewed by others
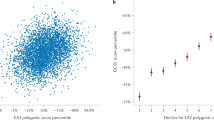
Genetic determinism, essentialism and reductionism: semantic clarity for contested science
K. Paige Harden
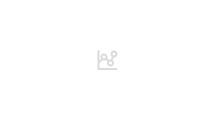
Moving back to the future of big data-driven research: reflecting on the social in genomics
Melanie Goisauf, Kaya Akyüz & Gillian M. Martin
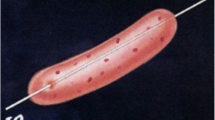
Beyond the factor indeterminacy problem using genome-wide association data
Margaret L. Clapp Sullivan, Ted Schwaba, … Elliot M. Tucker-Drob
Data availability
The genetic correlations reported in Fig. 1 are based on publicly available GWAS summary statistics on LDHub ( http://ldsc.broadinstitute.org/ldhub/ ). The Health and Retirement Study data in Fig. 3 can be accessed via dbGaP and the University of Michigan.
Definition of social science . Merriam Webster Dictionary https://www.merriam-webster.com/dictionary/social%20science (Accessed 1 November 2018).
Turkheimer, E. Three laws of behavior genetics and what they mean. Curr. Dir. Psychol. Sci. 9 , 160–164 (2000).
Google Scholar
Polderman, T. J. C. et al. Meta-analysis of the heritability of human traits based on fifty years of twin studies. Nat. Genet. 47 , 702–709 (2015).
CAS PubMed Google Scholar
Benjamin, D. J. et al. The promises and pitfalls of genoeconomics. Annu. Rev. Econ. 4 , 627–662 (2012).
Visscher, P. M. et al. 10 years of GWAS discovery: Biology, function, and translation. Am. J. Hum. Genet. 101 , 5–22 (2017).
CAS PubMed PubMed Central Google Scholar
Buniello, A. et al. The NHGRI-EBI GWAS Catalog of published genome-wide association studies, targeted arrays and summary statistics 2019. Nucleic Acids Res. 47 D1, D1005–D1012 (2019).
Freese, J. The arrival of social science genomics. Contemp. Sociol. 47 , 524–536 (2018).
Comfort, N. Nature still battles nurture in the haunting world of social genomics. Nature 553 , 278–280 (2018).
Turkheimer, E. & Paige Harden, K. Behavior Genetic Research Methods. in Handbook of Research Methods in Social and Personality Psychology 159–187 (Cambridge University Press, 2014).
Kong, A. et al. The nature of nurture: Effects of parental genotypes. Science 359 , 424–428 (2018).
Koellinger, P. D. & Harden, K. P. Using nature to understand nurture: Genetic associations show how parenting matters for children’s education. Science 359 , 386–387 (2018).
Bycroft, C. et al. The UK Biobank resource with deep phenotyping and genomic data. Nature 562 , 203–209 (2018).
Servick, K. Can 23andMe have it all? Science 349 , 1472–1474, 1476–1477 (2015).
Duncan, L. E., Pollastri, A. R. & Smoller, J. W. Mind the gap: why many geneticists and psychological scientists have discrepant views about gene-environment interaction (G×E) research. Am. Psychol. 69 , 249–268 (2014).
PubMed PubMed Central Google Scholar
Reich, D. E. et al. Linkage disequilibrium in the human genome. Nature 411 , 199–204 (2001).
Abecasis, G. R. et al. The 1000 Genomes Project Consortium. An integrated map of genetic variation from 1,092 human genomes. Nature 491 , 56–65 (2012).
PubMed Google Scholar
Price, A. L. et al. Principal components analysis corrects for stratification in genome-wide association studies. Nat. Genet. 38 , 904–909 (2006).
Visscher, P. M., Brown, M. A., McCarthy, M. I. & Yang, J. Five years of GWAS discovery. Am. J. Hum. Genet. 90 , 7–24 (2012).
Chabris, C. F., Lee, J. J., Cesarini, D., Benjamin, D. J. & Laibson, D. I. The fourth law of behavior genetics. Curr. Dir. Psychol. Sci. 24 , 304–312 (2015).
Rapid GWAS of thousands of phenotypes for 337,000 samples in the UK Biobank. Neale Lab http://www.nealelab.is/blog/2017/7/19/rapid-gwas-of-thousands-of-phenotypes-for-337000-samples-in-the-uk-biobank (Accessed 6 November 2018).
Kyoko Watanabe, D.P. Atlas of GWAS Summary Statistics. GWAS Atlas ( 2017 ) . http://atlas.ctglab.nl/ (Accessed 6 November 2019).
Global Biobank Engine. https://biobankengine.stanford.edu/ (Accessed 6 November 2019).
Manolio, T. A. et al. Finding the missing heritability of complex diseases. Nature 461 , 747–753 (2009).
Sohail, M. et al. Polygenic adaptation on height is overestimated due to uncorrected stratification in genome-wide association studies. eLife 8 , e39702 (2019).
Berg, J. J. et al. Reduced signal for polygenic adaptation of height in UK Biobank. eLife 8 , e39725 (2019).
Haworth, S. et al. Apparent latent structure within the UK Biobank sample has implications for epidemiological analysis. Nat. Commun. 10 , 333 (2019).
Lawson, D. J. et al. Is population structure in the genetic biobank era irrelevant, a challenge, or an opportunity? Hum. Genet. 139 , 23–41 (2020).
Loh, P.-R. et al. Efficient Bayesian mixed-model analysis increases association power in large cohorts. Nat. Genet. 47 , 284–290 (2015).
Yang, J., Zaitlen, N. A., Goddard, M. E., Visscher, P. M. & Price, A. L. Advantages and pitfalls in the application of mixed-model association methods. Nat. Genet. 46 , 100–106 (2014).
Davies, N. M. et al. Within family Mendelian randomization studies. Hum. Mol. Genet. 28 R2 R170–R179, https://doi.org/10.1093/hmg/ddz204 (2019).
Bulik-Sullivan, B. et al. An atlas of genetic correlations across human diseases and traits. Nat. Genet. 47 , 1236–1241 (2015).
Lee, J. J., McGue, M., Iacono, W. G. & Chow, C. C. The accuracy of LD Score regression as an estimator of confounding and genetic correlations in genome-wide association studies. Genet. Epidemiol. 42 , 783–795 (2018).
Zheng, J. et al. LD Hub: a centralized database and web interface to perform LD score regression that maximizes the potential of summary level GWAS data for SNP heritability and genetic correlation analysis. Bioinformatics 33 , 272–279 (2017).
Lee, J. J. et al. Gene discovery and polygenic prediction from a genome-wide association study of educational attainment in 1.1 million individuals. Nat. Genet. 50 , 1112–1121 (2018).
Gage, S. H., Davey Smith, G., Ware, J. J., Flint, J. & Munafò, M. R. G = E: what GWAS can tell us about the environment. PLoS Genet. 12 , e1005765 (2016).
Turley, P. et al. Multi-trait analysis of genome-wide association summary statistics using MTAG. Nat. Genet. 50 , 229–237 (2018).
Grotzinger, A. D. et al. Genomic structural equation modelling provides insights into the multivariate genetic architecture of complex traits. Nat. Hum. Behav. 3 , 513–525 (2019).
Baselmans, B. M. L. et al. Multivariate genome-wide analyses of the well-being spectrum. Nat. Genet. 51 , 445–451 (2019).
Loehlin, J. C. The Cholesky approach: A cautionary note. Behav. Genet. 26 , 65–69 (1996).
Torkamani, A., Wineinger, N. E. & Topol, E. J. The personal and clinical utility of polygenic risk scores. Nat. Rev. Genet. 19 , 581–590 (2018).
Dudbridge, F. Power and predictive accuracy of polygenic risk scores. PLoS Genet. 9 , e1003348 (2013).
Wray, N. R. et al. Research review: Polygenic methods and their application to psychiatric traits. J. Child Psychol. Psychiatry 55 , 1068–1087 (2014).
de Vlaming, R. et al. Meta-GWAS accuracy and power (MetaGAP) calculator shows that hiding heritability is partially due to imperfect genetic correlations across studies. PLoS Genet. 13 , e1006495 (2017).
Rietveld, C. A. et al. GWAS of 126,559 individuals identifies genetic variants associated with educational attainment. Science 340 , 1467–1471 (2013).
Okbay, A. et al. Genome-wide association study identifies 74 loci associated with educational attainment. Nature 533 , 539–542 (2016).
Wood, A. R. et al. Defining the role of common variation in the genomic and biological architecture of adult human height. Nat. Genet. 46 , 1173–1186 (2014).
Yengo, L. et al. Meta-analysis of genome-wide association studies for height and body mass index in ∼ 700000 individuals of European ancestry. Hum. Mol. Genet. 27 , 3641–3649 (2018).
Belsky, D. W. & Harden, K. P. Phenotypic annotation: Using polygenic scores to translate discoveries from genome-wide association studies from the top down. Curr. Dir. Psychol. Sci. 28 , 82–90 (2019).
Barcellos, S. H., Carvalho, L. S. & Turley, P. Education can reduce health differences related to genetic risk of obesity. Proc. Natl Acad. Sci. USA 115 , E9765–E9772 (2018).
Bansal, V. et al. Genome-wide association study results for educational attainment aid in identifying genetic heterogeneity of schizophrenia. Nat. Commun. 9 , 3078 (2018).
Belsky, D. W. et al. The genetics of success: how single-nucleotide polymorphisms associated with educational attainment relate to life-course development. Psychol. Sci. 27 , 957–972 (2016).
Young, A. I., Benonisdottir, S., Przeworski, M. & Kong, A. Deconstructing the sources of genotype-phenotype associations in humans. Science 365 , 1396–1400 (2019).
Mostafavi, H., Harpak, A., Conley, D., Pritchard, J. K. & Przeworski, M. Variable prediction accuracy of polygenic scores within an ancestry group. eLife 9 , e48376 (2020).
DiPrete, T. A., Burik, C. A. P. & Koellinger, P. D. Genetic instrumental variable regression: Explaining socioeconomic and health outcomes in nonexperimental data. Proc. Natl Acad. Sci. USA 115 , E4970–E4979 (2018).
Wertz, J. et al. Genetics of nurture: A test of the hypothesis that parents’ genetics predict their observed caregiving. Dev. Psychol. 55 , 1461–1472 (2019).
SSGAC Polygenic Score Data. Health and Retirement Study https://hrs.isr.umich.edu/news/ssgac-polygenic-score-data (Accessed 11 November 2019).
Young, A. I. et al. Relatedness disequilibrium regression estimates heritability without environmental bias. Nat. Genet. 50 , 1304–1310 (2018).
Davey Smith, G. & Hemani, G. Mendelian randomization: genetic anchors for causal inference in epidemiological studies. Hum. Mol. Genet. 23 R1 R89–R98 (2014).
Brumpton, B. et al. Within-family studies for Mendelian randomization: avoiding dynastic, assortative mating, and population stratification biases. bioRxiv https://doi.org/10.1101/602516 (2019).
O’Connor, L. J. & Price, A. L. Distinguishing genetic correlation from causation across 52 diseases and complex traits. Nat. Genet. 50 , 1728–1734 (2018).
Zhu, Z. et al. Causal associations between risk factors and common diseases inferred from GWAS summary data. Nat. Commun. 9 , 224 (2018).
Verbanck, M., Chen, C.-Y., Neale, B. & Do, R. Detection of widespread horizontal pleiotropy in causal relationships inferred from Mendelian randomization between complex traits and diseases. Nat. Genet. 50 , 693–698 (2018).
Koellinger, P. D. & de Vlaming, R. Mendelian randomization: the challenge of unobserved environmental confounds. Int. J. Epidemiol. 48 , 665–671 (2019).
Black, S. E., Devereux, P. J. & Salvanes, K. G. Why the apple doesn’t fall far: Understanding intergenerational transmission of human capital. Am. Econ. Rev. 95 , 437–449 (2005).
Bates, T. C. et al. The nature of nurture: Using a virtual-parent design to test parenting effects on children’s educational attainment in genotyped families. Twin Res. Hum. Genet. 21 , 73–83 (2018).
Liu, H. Social and genetic pathways in multigenerational transmission of educational attainment. Am. Sociol. Rev. 83 , 278–304 (2018).
Belsky, D. W. et al. Genetic analysis of social-class mobility in five longitudinal studies. Proc. Natl Acad. Sci. USA 115 , E7275–E7284 (2018).
Barth, D., Papageorge, N. W. & Thom, K. Genetic endowments and wealth inequality. J. Polit. Econ. 128 , 1474–1522 (2020).
Holland, P. W. Statistics and causal inference. J. Am. Stat. Assoc. 81 , 945–960 (1986).
Rodgers, J. & Kohler, H.-P. The Biodemography of Human Reproduction and Fertility . (Springer, 2002).
Barban, N. et al. Genome-wide analysis identifies 12 loci influencing human reproductive behavior. Nat. Genet. 48 , 1462–1472 (2016).
Day, F. R. et al. Genomic analyses identify hundreds of variants associated with age at menarche and support a role for puberty timing in cancer risk. Nat. Genet. 49 , 834–841 (2017).
Day, F. R. et al. Physical and neurobehavioral determinants of reproductive onset and success. Nat. Genet. 48 , 617–623 (2016).
Mehta, D. et al. Evidence for genetic overlap between schizophrenia and age at first birth in women. JAMA Psychiatry 73 , 497–505 (2016).
Ni, G., Gratten, J., Wray, N. R. & Lee, S. H., Schizophrenia Working Group of the Psychiatric Genomics Consortium. Age at first birth in women is genetically associated with increased risk of schizophrenia. Sci. Rep. 8 , 10168 (2018).
Harden, K. P. et al. A behavior genetic investigation of adolescent motherhood and offspring mental health problems. J. Abnorm. Psychol. 116 , 667–683 (2007).
Beauchamp, J. P. Genetic evidence for natural selection in humans in the contemporary United States. Proc. Natl Acad. Sci. USA 113 , 7774–7779 (2016).
Kong, A. et al. Selection against variants in the genome associated with educational attainment. Proc. Natl Acad. Sci. USA 114 , E727–E732 (2017).
Rodgers, J. L. et al. Education and cognitive ability as direct, mediating, or spurious influences on female age at first birth: behavior genetic models fit to Danish twin data. Am. J.Sociol. 114 , S202–S232 (2008). Suppl.
Tropf, F. C. & Mandemakers, J. J. Is the association between education and fertility postponement causal? The role of family background factors. Demography 54 , 71–91 (2017).
Jocklin, V., McGue, M. & Lykken, D. T. Personality and divorce: a genetic analysis. J. Pers. Soc. Psychol. 71 , 288–299 (1996).
D’Onofrio, B. M., Eaves, L. J., Murrelle, L., Maes, H. H. & Spilka, B. Understanding biological and social influences on religious affiliation, attitudes, and behaviors: a behavior genetic perspective. J. Pers. 67 , 953–984 (1999).
Pilling, L. C. et al. Human longevity: 25 genetic loci associated in 389,166 UK biobank participants. Aging (Albany NY) 9 , 2504–2520 (2017).
CAS Google Scholar
Abdellaoui, A. et al. Genetic correlates of social stratification in Great Britain. Nat. Hum. Behav. 3 , 1332–1342 (2019).
Hill, W. D. et al. Molecular genetic contributions to social deprivation and household income in UK Biobank. Curr. Biol. 26 , 3083–3089 (2016).
Belsky, D. W. et al. Genetics and the geography of health, behaviour and attainment. Nat. Hum. Behav. 3 , 576–586 (2019).
van der Sluis, S., Posthuma, D. & Dolan, C. V. A note on false positives and power in G × E modelling of twin data. Behav. Genet. 42 , 170–186 (2012).
Duncan, L. E. & Keller, M. C. A critical review of the first 10 years of candidate gene-by-environment interaction research in psychiatry. Am. J. Psychiatry 168 , 1041–1049 (2011).
Ceci, S. J. & Papierno, P. B. The rhetoric and reality of gap closing: when the “have-nots” gain but the “haves” gain even more. Am. Psychol. 60 , 149–160 (2005).
Fletcher, J. M. Why have tobacco control policies stalled? Using genetic moderation to examine policy impacts. PLoS One 7 , e50576 (2012).
Goldberger, A. S. Heritability. Economica 46 , 327–347 (1979).
Jencks, C. Heredity, environment, and public policy reconsidered. Am. Sociol. Rev. 45 , 723–736 (1980).
Bronfenbrenner, U. & Ceci, S. J. Nature-nurture reconceptualized in developmental perspective: a bioecological model. Psychol. Rev. 101 , 568–586 (1994).
Dickens, W. T. & Flynn, J. R. Heritability estimates versus large environmental effects: the IQ paradox resolved. Psychol. Rev. 108 , 346–369 (2001).
Lykken, D. T., Bouchard, T. J. Jr., McGue, M. & Tellegen, A. Heritability of interests: a twin study. J. Appl. Psychol. 78 , 649–661 (1993).
Tucker-Drob, E. M. & Harden, K. P. Early childhood cognitive development and parental cognitive stimulation: evidence for reciprocal gene-environment transactions. Dev. Sci. 15 , 250–259 (2012).
Klahr, A. M., Thomas, K. M., Hopwood, C. J., Klump, K. L. & Burt, S. A. Evocative gene-environment correlation in the mother-child relationship: a twin study of interpersonal processes. Dev. Psychopathol. 25 , 105–118 (2013).
Scarr, S. & McCartney, K. How people make their own environments: a theory of genotype greater than environment effects. Child Dev. 54 , 424–435 (1983).
Wray, N. R. et al. Genome-wide association analyses identify 44 risk variants and refine the genetic architecture of major depression. Nat. Genet. 50 , 668–681 (2018).
Wehby, G. L., Domingue, B. W. & Wolinsky, F. D. Genetic risks for chronic conditions: Implications for long-term wellbeing. J. Gerontol. A Biol. Sci. Med. Sci. 73 , 477–483 (2018).
Mallard, T. T., Harden, K. P. & Fromme, K. Genetic risk for schizophrenia is associated with substance use in emerging adulthood: an event-level polygenic prediction model. Psychol. Med. 49 , 2027–2035 (2019).
Okbay, A. et al. Genetic variants associated with subjective well-being, depressive symptoms, and neuroticism identified through genome-wide analyses. Nat. Genet. 48 , 624–633 (2016).
Wootton, R. E. et al. Evaluation of the causal effects between subjective wellbeing and cardiometabolic health: mendelian randomisation study. Br. Med. J. 362 , k3788 (2018).
Kevles, D.J. In the Name of Eugenics: Genetics and the Uses of Human Heredity . (Harvard University Press, 1995).
Rawls, J. A Theory of Justice . (Belknap Press, 1999).
Herrnstein, R.J. & Murray, C. The Bell Curve: Intelligence and Class Structure in American Life (Free Press, 1996).
Jensen, A. How much can we boost IQ and scholastic achievement. Harv. Educ. Rev. 39 , 1–123 (1969).
Yudell, M., Roberts, D., DeSalle, R. & Tishkoff, S. Taking race out of human genetics. Science 351 , 564–565 (2016).
Smedley, A. & Smedley, B. D. Race as biology is fiction, racism as a social problem is real: Anthropological and historical perspectives on the social construction of race. Am. Psychol. 60 , 16–26 (2005).
Henrich, J., Heine, S. J. & Norenzayan, A. The weirdest people in the world? Behav. Brain Sci. 33 , 61–83 (2010). discussion 83–135.
Martin, A. R. et al. Clinical use of current polygenic risk scores may exacerbate health disparities. Nat. Genet. 51 , 584–591 (2019).
Martin, A. R. et al. Human demographic history impacts genetic risk prediction across diverse populations. Am. J. Hum. Genet. 100 , 635–649 (2017).
Panofsky, A. & Donovan, J. Genetic ancestry testing among white nationalists: From identity repair to citizen science. Soc. Stud. Sci . https://doi.org/10.1177/0306312719861434 (2019).
Novembre, J. & Barton, N. H. Tread lightly interpreting polygenic tests of selection. Genetics 208 , 1351–1355 (2018).
Download references
Acknowledgements
We thank C. Burik for preparing Fig. 1 and the Social Science Genetic Association Consortium ( https://www.thessgac.org/ ) for Fig. 3 . P.D.K. was financially supported by an ERC consolidator grant (647648 EdGe). K.P.H. was supported by the Jacobs Foundation, the Templeton Foundation and Eunice Kennedy Shriver National Institute of Child Health and Human Development (NICHD) grants R01-HD083613 and 5-R24-HD042849 (to the Population Research Center at the University of Texas at Austin). The funders had no role in the conceptualization, preparation or decision to publish this work.
Author information
Authors and affiliations.
Department of Psychology, University of Texas at Austin, Austin, Texas, USA
Department of Economics, Vrije Universiteit Amsterdam, Amsterdam, The Netherlands
Philipp D. Koellinger
You can also search for this author in PubMed Google Scholar
Corresponding authors
Correspondence to K. Paige Harden or Philipp D. Koellinger .
Ethics declarations
Competing interests.
The authors declare no competing interests.
Additional information
Editor recognition statement: Primary handling editor: Stavroula Kousta.
Publisher’s note Springer Nature remains neutral with regard to jurisdictional claims in published maps and institutional affiliations.
Rights and permissions
Reprints and permissions
About this article
Cite this article.
Harden, K.P., Koellinger, P.D. Using genetics for social science. Nat Hum Behav 4 , 567–576 (2020). https://doi.org/10.1038/s41562-020-0862-5
Download citation
Received : 31 January 2019
Accepted : 16 March 2020
Published : 11 May 2020
Issue Date : 01 June 2020
DOI : https://doi.org/10.1038/s41562-020-0862-5
Share this article
Anyone you share the following link with will be able to read this content:
Sorry, a shareable link is not currently available for this article.
Provided by the Springer Nature SharedIt content-sharing initiative
This article is cited by
Infrastructuring educational genomics: associations, architectures, and apparatuses.
- Ben Williamson
- Dimitra Kotouza
- Jessica Pykett
Postdigital Science and Education (2024)
Could interventions on physical activity mitigate genomic liability for obesity? Applying the health disparity framework in genetically informed studies
- Moritz Herle
- Andrew Pickles
- Bianca L. De Stavola
European Journal of Epidemiology (2023)
The impact of digital media on children’s intelligence while controlling for genetic differences in cognition and socioeconomic background
- Bruno Sauce
- Magnus Liebherr
- Torkel Klingberg
Scientific Reports (2022)
Delayed tracking and inequality of opportunity: Gene-environment interactions in educational attainment
- Antonie Knigge
- Dorret I. Boomsma
npj Science of Learning (2022)
Genetics of cognitive performance, education and learning: from research to policy?
- Peter M. Visscher
Quick links
- Explore articles by subject
- Guide to authors
- Editorial policies
Sign up for the Nature Briefing newsletter — what matters in science, free to your inbox daily.


Behavioural genetics methods

Vol: 3 (2006) > Issue: 1 (January)
Primer | DOI: 10.1038/s43586-022-00191-x
- John D. Bower School of Population Health, University of Mississippi Medical Center, Jackson, MS, USA
- Department of Child and Adolescent Psychiatry & Social Care, Amsterdam UMC, Amsterdam, Netherlands
- Department of Psychology, University of Minnesota Twin Cities, Minneapolis, MN, USA
- School of Applied Sciences, University of Mississippi, University, MS, USA
- Department of Clinical Developmental Psychology, Vrije Universiteit, Amsterdam, Netherlands

The question of why people show individual differences in their behaviours and capacities has intrigued researchers for centuries. Behaviour genetics offers us various methods to address this question. The answers are interesting for a range of
The question of why people show individual differences in their behaviours and capacities has intrigued researchers for centuries. Behaviour genetics offers us various methods to address this question. The answers are interesting for a range of research fields, varying from medicine to psychology, economics and neuroscience. Starting with twin and family studies in the late 1970s, the field of behaviour genetics has rapidly developed by applying molecular genetic techniques next to, and sometimes combined with, family data. The overarching conclusion at this point in time is that all measured human traits are to some extent heritable, and that many genetic variants, with each exerting a small effect, explain this heritability. Against this backdrop, we offer readers who might be less familiar with behaviour genetics a brief Primer on the topic. Sitting atop our list of goals is to be a resource for scholars interested in applying the widely useful techniques of the field to their particular specialty, regardless of what that might be.
Figures ( 0 ) & Videos ( 0 )
Fig. 1 : Three common twin family models.
Fig. 2 : Correlations in cognitive ability between twin pairs.
Fig. 3 : Example raw and standardized variance path diagrams.
Fig. 4 : A cross-lagged longitudinal MZ discordant twin design.
Fig. 5 : The Wilson effect for cognitive ability.
Fig. 6 : Estimates of within-family shrinkage for GWAS predictors across a range of phenotypes from a within-sibship study 52 .
Experimental Specifications
Other keywords.

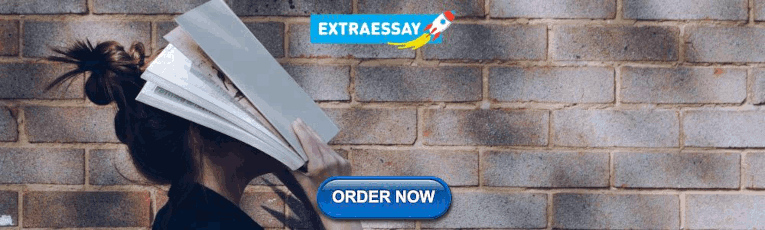
Citations (9)
Associated articles, supplementary information.
- Supplementary Information
- Turkheimer, E. Three laws of behavior genetics and what they mean. Curr. Dir. Psychol. Sci. 9 , 160–164 (2000).
- Harden, K. P. “Reports of my death were greatly exaggerated”: behavior genetics in the postgenomic era. Annu. Rev. Psychol. 72 , 37–60 (2021).
- Barnes, J. C. et al. Demonstrating the validity of twin research in criminology. Criminology 52 , 588–626 (2014).
- Friedman, N. P., Banich, M. T. & Keller, M. C. Twin studies to GWAS: there and back again. Trends Cognit. Sci. 25 , 855–869 (2021).
- Polderman, T. J. et al. Meta-analysis of the heritability of human traits based on fifty years of twin studies. Nat. Genet. 47 , 702–709 (2015).
- Chabris, C. F., Lee, J. J., Cesarini, D., Benjamin, D. J. & Laibson, D. I. The fourth law of behavior genetics. Curr. Dir. Psychol. Sci. 24 , 304–312 (2015).
- Visscher, PeterM. et al. Assumption-free estimation of heritability from genome-wide identity-by-descent sharing between full siblings. PLoS Genet. 2 , e41 (2006).
- Plomin, R., DeFries, J. C. & Loehlin, J. C. Genotype–environment interaction and correlation in the analysis of human behavior. Psychol. Bull. 84 , 309–322 (1997).
- Scarr, S. & McCartney, K. How people make their own environments: a theory of genotype–environment effects. Child. Dev. 54 , 424 (1983).
- Fowler-Finn, K. D. & Boutwell, B. B. Using variation in heritability estimates as a test of G × E in behavioral research: a brief research note. Behav. Genet. 49 , 340–346 (2019).
- Tucker-Drob, E. M. & Bates, T. C. Large cross-national differences in gene × socioeconomic status interaction on intelligence. Psychol. Sci. 27 , 138–149 (2016).
- Loehlin, J., Corley, R., Reynolds, C. & Wadsworth, S. Heritability × SES interaction for IQ: is it present in US adoption studies? Behav. Genet. 52 , 1–8 (2022).
- Heath, A. C., Kendler, K. S., Eaves, L. J. & Markell, D. The resolution of cultural and biological inheritance: informativeness of different relationships. Behav. Genet. 15 , 439–465 (1985).
- Truett, K. R. et al. A model system for analysis of family resemblance in extended kinships of twins. Behav. Genet. 24 , 35–49 (1994).
- Keller, M. C. et al. Modeling extended twin family data I: description of the Cascade model. Twin Res. Hum. Genet. 12 , 8–18 (2009).
- Pettersson, E. et al. Genetic influences on eight psychiatric disorders based on family data of 4 408 646 full and half-siblings, and genetic data of 333 748 cases and controls. Psychol. Med. 49 , 1166–1173 (2019).
- Lawlor, D. A., Tilling, K. & Davey Smith, G. Triangulation in aetiological epidemiology. Int. J. Epidemiol. 45 , 1866–1886 (2016).
- Yang, J. et al. Common SNPs explain a large proportion of the heritability for human height. Nat. Genet. 42 , 565–569 (2010).
- Young, A. I. Solving the missing heritability problem. PLoS Genet. 15 , e1008222 (2019).
- Young, A. I. Discovering missing heritability in whole-genome sequencing data. Nat. Genet. 54 , 224–226 (2022).
- Bulik-Sullivan, B. et al. An atlas of genetic correlations across human diseases and traits’. Nat. Genet. 47 , 1236–1241 (2015).
- Burgess, S., Butterworth, A. & Thompson, S. G. Mendelian randomization analysis with multiple genetic variants using summarized data. Genet. Epidemiol. 37 , 658–665 (2013).
- Falconer, D. S. Introduction to quantitative genetics (Longman Group, 1960).
- Falconer, D. S. & Mackay, T. F. C. Quantitative Genetics (Longman Group, 1996).
- Martin, N. & Eaves, L. The genetical analysis of covariance structure. Heredity 38 , 79–95 (1977).
- Neale, M. C. et al. OpenMx 2.0: extended structural equation and statistical modeling. Psychometrika 81 , 535–549 (2016).
- Burt, S. A., McGue, M. & Iacono, W. G. Nonshared environmental mediation of the association between deviant peer affiliation and adolescent externalizing behaviors over time: results from a cross-lagged monozygotic twin differences design. Dev. Psychol. 45 , 1752–1760 (2009).
- Bartels, M., Boomsma, D. I., Hudziak, J. J., van Beijsterveldt, T. C. E. M. & van den Oord, E. J. C. G. Twins and the study of rater (dis)agreement. Psychol. Meth. 12 , 451–466 (2007); erratum 13 , 170 (2008).
- Nivard, M. G., Middeldorp, C. M., Dolan, C. V. & Boomsma, D. I. Genetic and environmental stability of neuroticism from adolescence to adulthood. Twin Res. Hum. Genet. 18 , 746–754 (2015).
- Visscher, P. M. et al. 10 years of GWAS discovery: biology, function, and translation. Am. J. Hum. Genet. 101 , 5–22 (2017).
- Willoughby, E. & Lee, J. in The Cambridge Handbook of Intelligence and Cognitive Neuroscience (eds Barbey, A., Karama, S., & Haier, R.) 349–364 (Cambridge Univ. Press, 2021).
- Dudbridge, F. Power and predictive accuracy of polygenic risk scores. PLoS Genet. 9 , e1003348 (2013).
- Plomin, R. & von Stumm, S. Polygenic scores: prediction versus explanation. Mol. Psychiatry 27 , 49–52 (2022).
- Li, M. X. et al. A major gene model of adult height is suggested in Chinese. J. Hum. Genet. 49 , 148–153 (2004).
- Roberts, D. F., Billewicz, W. Z. & McGregor, I. A. Heritability of stature in a West African population. Ann. Hum. Genet. 42 , 15–24 (1978).
- Tarnoki, A. D., Tarnoki, D. L. & Molnar, A. A. Past, present and future of cardiovascular twin studies. Cor Vasa 56 , e486–e493 (2014).
- Pechlivanis, S. et al. Risk prediction for coronary heart disease by a genetic risk score — results from the Heinz Nixdorf Recall study. BMC Med. Genet. 21 , 178 (2020).
- Yang, R. et al. A healthy lifestyle mitigates the risk of heart disease related to type 2 diabetes: a prospective nested case–control study in a nationwide Swedish twin cohort. Diabetologia 64 , 530–539 (2021).
- McGue, M., Osler, M. & Christensen, K. Causal inference and observational research: the utility of twins. Perspect. Psychol. Sci. 5 , 546–556 (2010).
- Lemvigh, C. et al. The relative and interactive impact of multiple risk factors in schizophrenia spectrum disorders: a combined register-based and clinical twin study. Psychol. Med. (2021). https://doi.org/10.1017/S0033291721002749
- Squarcina, L., Fagnani, C., Bellani, M., Altamura, C. A. & Brambilla, P. Twin studies for the investigation of the relationships between genetic factors and brain abnormalities in bipolar disorder. Epidemiol. Psychiatr. Sci. 25 , 515–520 (2016).
- Bouchard, T. J. & McGue, M. Genetic and environmental influences on human psychological differences. J. Neurobiol. 54 , 4–45 (2003).
- Wilson, R. S. The Louisville twin study: developmental synchronies in behavior. Child. Dev. 54 , 298–316 (1983).
- Bouchard, T. J., Lykken, D. T., McGue, M., Segal, N. L. & Tellegen, A. Sources of human psychological differences: the minnesota study of twins reared apart. Science. 250 , 223–228 (1990).
- Bouchard, T. The Wilson effect: the increase in heritability of IQ with age. Twin Res. Hum. Genet. 16 , 923–930 (2013).
- Deary, I. J., Johnson, W. & Houlihan, L. M. Genetic foundations of human intelligence. Hum. Genetics. 126 , 215–232 (2009).
- Jang, K. L., Livesley, W. J. & Vernon, P. A. Heritability of the big five personality dimensions and their facets: a twin study. J. Pers. 64 , 577–591 (1996).
- Tellegen, A. & Niels, G. W. Exploring personality through test construction: development of the multidimensional personality questionnaire. SAGE Handb. Pers. Theory Assess. 2 , 261–292 (2008).
- Vukasović, T. & Bratko, D. Heritability of personality: a meta-analysis of behavior genetic studies. Psychol. Bull. 141 , 769–785 (2015).
- Krueger, R. F., South, S., Johnson, W. & Iacono, W. The heritability of personality is not always 50%: gene–environment interactions and correlations between personality and parenting. J. Pers. 76 , 1485–1522 (2008).
- Matteson, L. K., McGue, M. & Iacono, W. G. Shared environmental influences on personality: a combined twin and adoption approach. Behav. Genet. 43 , 491–504 (2013).
- Howe, L. J. et al. Within-sibship genome-wide association analyses decrease bias in estimates of direct genetic effects. Nat. Genet. 54 , 581–592 (2022).
- Lee, J. J. et al. Gene discovery and polygenic prediction from a genome-wide association study of educational attainment in 1.1 million individuals. Nat. Genet. 50 , 1112–1121 (2018).
- Kong, A. et al. The nature of nurture: effects of parental genotypes. Science 359 , 424–428 (2018).
- Belsky, D. W. et al. Genetic analysis of social-class mobility in five longitudinal studies. Proc. Natl Acad. Sci. USA 115 , E7275–E7284 (2018).
- Bates, T. C. et al. Social competence in parents increases children’s educational attainment: replicable genetically-mediated effects of parenting revealed by non-transmitted DNA. Twin Res. Hum. Genet. 22 , 1–3 (2019).
- Willoughby, E. A., McGue, M., Iacono, W. G., Rustichini, A. & Lee, J. J. The role of parental genotype in predicting offspring years of education: evidence for genetic nurture. Mol. Psychiatry 26 , 3896–3904 (2021).
- Loehlin, J. C., Horn, J. M. & Willerman, L. in Intelligence, Heredity, and Environment (eds Sternberg, R. J., & Grigorenko, E. L.) 105–125 (Cambridge Univ. Press, 1997).
- Cadoret, R. J. Adoption Studies. Alcohol Health Res. World 19 , 195–200 (1995).
- Rhea, S. A., Bricker, J. B., Corley, R. P., DeFries, J. C. & Wadsworth, S. J. Design utility and history of the Colorado Adoption Project: examples involving adjustment interactions. Adopt. Q. 16 , 17–39 (2013).
- Willoughby, E. A., McGue, M., Iacono, W. G. & Lee, J. J. Genetic and environmental contributions to IQ in adoptive and biological families with 30-year-old offspring. Intelligence 88 , 101579 (2021).
- Baker, M. Reproducibility crisis? Nature 533 , 26 (2016).
- Fanelli, D. Opinion: is science really facing a reproducibility crisis, and do we need it to? Proc. Natl Acad. Sci. USA 115 , 2628–2631 (2018).
- Plomin, R., DeFries, J. C., Knopik, V. S. & Neiderhiser, J. M. Top 10 replicated findings from behavioral genetics. Perspect. Psychol. Sci. 11 , 3–23 (2016).
- Chabris, C. F. et al. Most reported genetic associations with general intelligence are probably false positives. Psychol. Sci. 23 , 1314–1323 (2012).
- Johnson, E. C. et al. No evidence that schizophrenia candidate genes are more associated with schizophrenia than noncandidate genes. Biol. Psychiatry 82 , 702–708 (2017).
- Border, R. et al. No support for historical candidate gene or candidate gene-by-interaction hypotheses for major depression across multiple large samples. Am. J. Psychiatry 176 , 376–387 (2019).
- Lin, X. Learning lessons on reproducibility and replicability in large scale genome-wide association studies. Harvard Data Sci. Rev. (2020). https://doi.org/10.1162/99608f92.33703976
- Røysamb, E., & Tambs, K. The beauty, logic and limitations of twin studies. Nor. Epidemiol . 26 , 35–46 (2016).
- van Dongen, J., Slagboom, P. E., Draisma, H. H., Martin, N. G. & Boomsma, D. I. The continuing value of twin studies in the omics era. Nat. Rev. Genet. 13 , 640–653 (2012).
- Wilson, S. et al. Minnesota Center for Twin and Family Research. Twin Res. Hum. Genet. 22 , 746–752 (2019).
- Hindorff, L. A. et al. Prioritizing diversity in human genomics research. Nat. Rev. Genet. 19 , 175–185 (2017).
- Mills, M. C. & Rahal, C. A scientometric review of genome-wide association studies. Commun. Biol. 2 , 1–11 (2019).
- George, S., Duran, N. & Norris, K. A systematic review of barriers and facilitators to minority research participation among African Americans, Latinos, Asian Americans, and Pacific Islanders. Am. J. Public Health (2014). https://doi.org/10.2105/AJPH.2013.301706
- Wojcik, G. L. et al. Genetic analyses of diverse populations improves discovery for complex traits. Nature 570 , 514–518 (2019).
- Martin, A. R. et al. Human demographic history impacts genetic risk prediction across diverse populations. Am. J. Hum. Genet. 100 , 635–649 (2017).
- Giannakopoulou, O. et al. The genetic architecture of depression in individuals of East Asian ancestry: a genome-wide association study. JAMA Psychiatry 78 , 1258–1269 (2021).
- Meng, X. et al. Multi-ancestry GWAS of major depression aids locus discovery, fine-mapping, gene prioritisation, and causal inference. Preprint at bioRxiv (2022). https://doi.org/10.1101/2022.07.20.500802
- Martin, A. R. et al. Clinical use of current polygenic risk scores may exacerbate health disparities. Nat. Genet. 51 , 584–591 (2019).
- Bigdeli, T. B. et al. Genetic effects influencing risk for major depressive disorder in China and Europe. Transl. Psychiatry 7 , e1074 (2017).
- Bigdeli, T. B. et al. Contributions of common genetic variants to risk of schizophrenia among individuals of African and Latino ancestry. Mol. Psychiatry 25 , 2455–2467 (2020).
- Hyttinen, V., Kaprio, J., Kinnunen, L., Koskenvuo, M. & Tuomilehto, J. Genetic liability of type 1 diabetes and the onset age among 22,650 young Finnish twin pairs: a nationwide follow-up study. Diabetes 52 , 1052–1055 (2003).
- Snieder, H. et al. HbA1c levels are genetically determined even in type 1 diabetes: evidence from healthy and diabetic twins. Diabetes 50 , 2858–2863 (2001).
- McAdams, T. A., Rijsdijk, F. V., Zavos, H. M. & Pingault, J. B. Twins and causal inference: leveraging nature’s experiment. Cold Spring Harb. Perspect. Med. 11 , a039552 (2021).
- Alberg, A. J., Brock, M. V. & Samet, J. M. in Murray & Nadel’s Textbook of Respiratory Medicine 6th edn, Ch. 52, 927–939 (Saunders Elsevier, 2016).
- Poulton, R., Moffitt, T. E. & Silva, P. A. The Dunedin multidisciplinary health and development study: overview of the first 40 years, with an eye to the future. Soc. Psychiatry Psychiatr. Epidemiol., 50 , 679–693 (2015).
- Krüger, O., Korsten, P. & Hoffman, J. I. in J. Call, G. M. Burghardt, I. M. Pepperberg, C. T. Snowdon, & T. Zentall (Eds.), APA handbook of comparative psychology: Basic concepts, methods, neural substrate, and behavior (eds Call, J., Burghardt, G. M., Pepperberg, I. M., Snowdon, C. T. & Zentall, T.) 365–379 (American Psychological Association, 2017).
- Turkheimer, E. & Harden, K. P. in Handbook of Research Methods in Social and Personality Psychology (eds Reis, H. T. & Judd, C. M.) 159–187 (Cambridge Univ. Press, 2014).
- Okbay, A. et al. Polygenic prediction of educational attainment within and between families from genome-wide association analyses in 3 million individuals. Nat. Genet. 54 , 437–449 (2022).
- Marouli, E. et al. Rare and low-frequency coding variants alter human adult height. Nature 542 , 186190 (2017).
- Wainschtein, P. Assessing the contribution of rare variants to complex trait heritability from whole-genome sequence data. Nat. Genet. 54 , 263–273 (2022).
- Wang, Z., Gerstein, M. & Snyder, M. RNA-seq: a revolutionary tool for transcriptomics. Nat. Rev. Genet. 10 , 57–63 (2009).
- Gupta, S. et al. Transcriptome analysis reveals dysregulation of innate immune response genes and neuronal activity-dependent genes in autism. Nat. Commun. 5 , 5748 (2014).
- Pers, T.H. et al. Biological interpretation of genome-wide association studies using predicted gene functions. Nat. Commun. 6 , 5890 (2015).
- de Leeuw, C. A., Mooij, J. M., Heskes, T. & Posthuma, D. MAGMA: generalized gene-set analysis of GWAS data. PLoS Comput. Biol. 11 , 1–19 (2015).
- Neale, M. C. & Cardon, L. R. Methodology for Genetic Studies of Twins and Families . (Kluwer Academic/Plenum, 1992).
- Vitaro, F., Brendgen, M. & Arseneault, L. Methods and measures: the discordant MZ-twin method: one step closer to the holy grail of causality. Int. J. Behav. Dev. 33 , 376–382 (2009).
- Keller, M. C., Medland, S. E. & Duncan, L. E. Are extended twin family designs worth the trouble? A comparison of the bias, precision, and accuracy of parameters estimated in four twin family models. Behav. Genet. 40 , 377–393 (2010).
- McAdams, T. A. et al. Revisiting the children-of-twins design: improving existing models for the exploration of intergenerational associations. Behav. Genet. 48 , 397–412 (2018).
- Scarr, S. & Weinberg, R. A. The Minnesota Adoption Studies: genetic differences and malleability. Child. Dev. 54 , 260–267 (1983).
- Murphy, K. et al. Twins Research Australia: a new paradigm for driving twin research. Twin Res. Hum. Genet. 22 , 438–445 (2019).
- Otta, E. et al. The University of São Paulo Twin Panel: current status and prospects for Brazilian twin studies in behavioral research. Twin Res. Hum. Genet. 22 , 467–474 (2019).
- Huang et al. The Chinese National Twin Registry: a unique data source for systems epidemiology of complex disease. Twin Res. Hum. Genet. 22 , 482–485 (2019).
- Pedersen et al. The Danish Twin Registry: an updated overview. Twin Res. Hum. Genet. 22 , 499–507 (2020).
- Bjerregaard-Andersen et al. The Guinea-Bissau Twin Registry update: a platform for studying twin mortality and metabolic disease. Twin Res. Hum. Genet. 22 , 554–560 (2019).
- Gharipour et al. Isfahan Twins Registry (ITR): an invaluable platform for epidemiological and epigenetic studies: design and methodology of ITR. Twin Res. Hum. Genet. 22 , 579–582 (2019).
- Ligthart et al. The Netherlands Twin Register: longitudinal research based on twin and twin-family designs. Twin Res. Hum. Genet. 22 , 623–636 (2019).
- Rimfeld et al. Twins Early Development Study: a genetically sensitive investigation into behavioral and cognitive development from infancy to emerging adulthood. Twin Res. Hum. Genet. 22 , 508–513 (2019).
- Pingault, J. B. et al. Using genetic data to strengthen causal inference in observational research. Nat. Rev. Genet. 19 , 566–580 (2018).
- Lee, J. J. Correlation and causation in the study of personality. Eur. J. Personal. 26 , 372–412 (2012).
- Vilhjámsson, B. J. et al. Modeling linkage disequilibrium increases accuracy of polygenic risk scores. Am. J. Hum. Genet. 97 , 576–592 (2015).
- de Vlaming, R. & Groenen, P. J. F. The current and future use of ridge regression for prediction in quantitative genetics. BioMed. Res. Int. 2015 , 1–18 (2015).
- Vattikuti, S., Lee, J. J., Chang, C. C., Hsu, S. D. H. & Chow, C. C. Applying compressed sensing to genome-wide association studies. GigaScience 3 , 10 (2014).
- Lello, L. et al. Accurate genomic prediction of human height. Genetics 210 , 477–497 (2018).
- Allegrini, A. G. et al. Genomic prediction of cognitive traits in childhood and adolescence. Mol. Psychiatry 24 , 819–827 (2019).
Advertisement
- Search Menu
- Browse content in Arts and Humanities
- Browse content in Archaeology
- Anglo-Saxon and Medieval Archaeology
- Archaeological Methodology and Techniques
- Archaeology by Region
- Archaeology of Religion
- Archaeology of Trade and Exchange
- Biblical Archaeology
- Contemporary and Public Archaeology
- Environmental Archaeology
- Historical Archaeology
- History and Theory of Archaeology
- Industrial Archaeology
- Landscape Archaeology
- Mortuary Archaeology
- Prehistoric Archaeology
- Underwater Archaeology
- Urban Archaeology
- Zooarchaeology
- Browse content in Architecture
- Architectural Structure and Design
- History of Architecture
- Residential and Domestic Buildings
- Theory of Architecture
- Browse content in Art
- Art Subjects and Themes
- History of Art
- Industrial and Commercial Art
- Theory of Art
- Biographical Studies
- Byzantine Studies
- Browse content in Classical Studies
- Classical History
- Classical Philosophy
- Classical Mythology
- Classical Literature
- Classical Reception
- Classical Art and Architecture
- Classical Oratory and Rhetoric
- Greek and Roman Papyrology
- Greek and Roman Epigraphy
- Greek and Roman Law
- Greek and Roman Archaeology
- Late Antiquity
- Religion in the Ancient World
- Digital Humanities
- Browse content in History
- Colonialism and Imperialism
- Diplomatic History
- Environmental History
- Genealogy, Heraldry, Names, and Honours
- Genocide and Ethnic Cleansing
- Historical Geography
- History by Period
- History of Emotions
- History of Agriculture
- History of Education
- History of Gender and Sexuality
- Industrial History
- Intellectual History
- International History
- Labour History
- Legal and Constitutional History
- Local and Family History
- Maritime History
- Military History
- National Liberation and Post-Colonialism
- Oral History
- Political History
- Public History
- Regional and National History
- Revolutions and Rebellions
- Slavery and Abolition of Slavery
- Social and Cultural History
- Theory, Methods, and Historiography
- Urban History
- World History
- Browse content in Language Teaching and Learning
- Language Learning (Specific Skills)
- Language Teaching Theory and Methods
- Browse content in Linguistics
- Applied Linguistics
- Cognitive Linguistics
- Computational Linguistics
- Forensic Linguistics
- Grammar, Syntax and Morphology
- Historical and Diachronic Linguistics
- History of English
- Language Evolution
- Language Reference
- Language Acquisition
- Language Variation
- Language Families
- Lexicography
- Linguistic Anthropology
- Linguistic Theories
- Linguistic Typology
- Phonetics and Phonology
- Psycholinguistics
- Sociolinguistics
- Translation and Interpretation
- Writing Systems
- Browse content in Literature
- Bibliography
- Children's Literature Studies
- Literary Studies (Romanticism)
- Literary Studies (American)
- Literary Studies (Asian)
- Literary Studies (European)
- Literary Studies (Eco-criticism)
- Literary Studies (Modernism)
- Literary Studies - World
- Literary Studies (1500 to 1800)
- Literary Studies (19th Century)
- Literary Studies (20th Century onwards)
- Literary Studies (African American Literature)
- Literary Studies (British and Irish)
- Literary Studies (Early and Medieval)
- Literary Studies (Fiction, Novelists, and Prose Writers)
- Literary Studies (Gender Studies)
- Literary Studies (Graphic Novels)
- Literary Studies (History of the Book)
- Literary Studies (Plays and Playwrights)
- Literary Studies (Poetry and Poets)
- Literary Studies (Postcolonial Literature)
- Literary Studies (Queer Studies)
- Literary Studies (Science Fiction)
- Literary Studies (Travel Literature)
- Literary Studies (War Literature)
- Literary Studies (Women's Writing)
- Literary Theory and Cultural Studies
- Mythology and Folklore
- Shakespeare Studies and Criticism
- Browse content in Media Studies
- Browse content in Music
- Applied Music
- Dance and Music
- Ethics in Music
- Ethnomusicology
- Gender and Sexuality in Music
- Medicine and Music
- Music Cultures
- Music and Media
- Music and Religion
- Music and Culture
- Music Education and Pedagogy
- Music Theory and Analysis
- Musical Scores, Lyrics, and Libretti
- Musical Structures, Styles, and Techniques
- Musicology and Music History
- Performance Practice and Studies
- Race and Ethnicity in Music
- Sound Studies
- Browse content in Performing Arts
- Browse content in Philosophy
- Aesthetics and Philosophy of Art
- Epistemology
- Feminist Philosophy
- History of Western Philosophy
- Metaphysics
- Moral Philosophy
- Non-Western Philosophy
- Philosophy of Language
- Philosophy of Mind
- Philosophy of Perception
- Philosophy of Science
- Philosophy of Action
- Philosophy of Law
- Philosophy of Religion
- Philosophy of Mathematics and Logic
- Practical Ethics
- Social and Political Philosophy
- Browse content in Religion
- Biblical Studies
- Christianity
- East Asian Religions
- History of Religion
- Judaism and Jewish Studies
- Qumran Studies
- Religion and Education
- Religion and Health
- Religion and Politics
- Religion and Science
- Religion and Law
- Religion and Art, Literature, and Music
- Religious Studies
- Browse content in Society and Culture
- Cookery, Food, and Drink
- Cultural Studies
- Customs and Traditions
- Ethical Issues and Debates
- Hobbies, Games, Arts and Crafts
- Lifestyle, Home, and Garden
- Natural world, Country Life, and Pets
- Popular Beliefs and Controversial Knowledge
- Sports and Outdoor Recreation
- Technology and Society
- Travel and Holiday
- Visual Culture
- Browse content in Law
- Arbitration
- Browse content in Company and Commercial Law
- Commercial Law
- Company Law
- Browse content in Comparative Law
- Systems of Law
- Competition Law
- Browse content in Constitutional and Administrative Law
- Government Powers
- Judicial Review
- Local Government Law
- Military and Defence Law
- Parliamentary and Legislative Practice
- Construction Law
- Contract Law
- Browse content in Criminal Law
- Criminal Procedure
- Criminal Evidence Law
- Sentencing and Punishment
- Employment and Labour Law
- Environment and Energy Law
- Browse content in Financial Law
- Banking Law
- Insolvency Law
- History of Law
- Human Rights and Immigration
- Intellectual Property Law
- Browse content in International Law
- Private International Law and Conflict of Laws
- Public International Law
- IT and Communications Law
- Jurisprudence and Philosophy of Law
- Law and Politics
- Law and Society
- Browse content in Legal System and Practice
- Courts and Procedure
- Legal Skills and Practice
- Primary Sources of Law
- Regulation of Legal Profession
- Medical and Healthcare Law
- Browse content in Policing
- Criminal Investigation and Detection
- Police and Security Services
- Police Procedure and Law
- Police Regional Planning
- Browse content in Property Law
- Personal Property Law
- Study and Revision
- Terrorism and National Security Law
- Browse content in Trusts Law
- Wills and Probate or Succession
- Browse content in Medicine and Health
- Browse content in Allied Health Professions
- Arts Therapies
- Clinical Science
- Dietetics and Nutrition
- Occupational Therapy
- Operating Department Practice
- Physiotherapy
- Radiography
- Speech and Language Therapy
- Browse content in Anaesthetics
- General Anaesthesia
- Neuroanaesthesia
- Clinical Neuroscience
- Browse content in Clinical Medicine
- Acute Medicine
- Cardiovascular Medicine
- Clinical Genetics
- Clinical Pharmacology and Therapeutics
- Dermatology
- Endocrinology and Diabetes
- Gastroenterology
- Genito-urinary Medicine
- Geriatric Medicine
- Infectious Diseases
- Medical Toxicology
- Medical Oncology
- Pain Medicine
- Palliative Medicine
- Rehabilitation Medicine
- Respiratory Medicine and Pulmonology
- Rheumatology
- Sleep Medicine
- Sports and Exercise Medicine
- Community Medical Services
- Critical Care
- Emergency Medicine
- Forensic Medicine
- Haematology
- History of Medicine
- Browse content in Medical Skills
- Clinical Skills
- Communication Skills
- Nursing Skills
- Surgical Skills
- Browse content in Medical Dentistry
- Oral and Maxillofacial Surgery
- Paediatric Dentistry
- Restorative Dentistry and Orthodontics
- Surgical Dentistry
- Medical Ethics
- Medical Statistics and Methodology
- Browse content in Neurology
- Clinical Neurophysiology
- Neuropathology
- Nursing Studies
- Browse content in Obstetrics and Gynaecology
- Gynaecology
- Occupational Medicine
- Ophthalmology
- Otolaryngology (ENT)
- Browse content in Paediatrics
- Neonatology
- Browse content in Pathology
- Chemical Pathology
- Clinical Cytogenetics and Molecular Genetics
- Histopathology
- Medical Microbiology and Virology
- Patient Education and Information
- Browse content in Pharmacology
- Psychopharmacology
- Browse content in Popular Health
- Caring for Others
- Complementary and Alternative Medicine
- Self-help and Personal Development
- Browse content in Preclinical Medicine
- Cell Biology
- Molecular Biology and Genetics
- Reproduction, Growth and Development
- Primary Care
- Professional Development in Medicine
- Browse content in Psychiatry
- Addiction Medicine
- Child and Adolescent Psychiatry
- Forensic Psychiatry
- Learning Disabilities
- Old Age Psychiatry
- Psychotherapy
- Browse content in Public Health and Epidemiology
- Epidemiology
- Public Health
- Browse content in Radiology
- Clinical Radiology
- Interventional Radiology
- Nuclear Medicine
- Radiation Oncology
- Reproductive Medicine
- Browse content in Surgery
- Cardiothoracic Surgery
- Gastro-intestinal and Colorectal Surgery
- General Surgery
- Neurosurgery
- Paediatric Surgery
- Peri-operative Care
- Plastic and Reconstructive Surgery
- Surgical Oncology
- Transplant Surgery
- Trauma and Orthopaedic Surgery
- Vascular Surgery
- Browse content in Science and Mathematics
- Browse content in Biological Sciences
- Aquatic Biology
- Biochemistry
- Bioinformatics and Computational Biology
- Developmental Biology
- Ecology and Conservation
- Evolutionary Biology
- Genetics and Genomics
- Microbiology
- Molecular and Cell Biology
- Natural History
- Plant Sciences and Forestry
- Research Methods in Life Sciences
- Structural Biology
- Systems Biology
- Zoology and Animal Sciences
- Browse content in Chemistry
- Analytical Chemistry
- Computational Chemistry
- Crystallography
- Environmental Chemistry
- Industrial Chemistry
- Inorganic Chemistry
- Materials Chemistry
- Medicinal Chemistry
- Mineralogy and Gems
- Organic Chemistry
- Physical Chemistry
- Polymer Chemistry
- Study and Communication Skills in Chemistry
- Theoretical Chemistry
- Browse content in Computer Science
- Artificial Intelligence
- Computer Architecture and Logic Design
- Game Studies
- Human-Computer Interaction
- Mathematical Theory of Computation
- Programming Languages
- Software Engineering
- Systems Analysis and Design
- Virtual Reality
- Browse content in Computing
- Business Applications
- Computer Security
- Computer Games
- Computer Networking and Communications
- Digital Lifestyle
- Graphical and Digital Media Applications
- Operating Systems
- Browse content in Earth Sciences and Geography
- Atmospheric Sciences
- Environmental Geography
- Geology and the Lithosphere
- Maps and Map-making
- Meteorology and Climatology
- Oceanography and Hydrology
- Palaeontology
- Physical Geography and Topography
- Regional Geography
- Soil Science
- Urban Geography
- Browse content in Engineering and Technology
- Agriculture and Farming
- Biological Engineering
- Civil Engineering, Surveying, and Building
- Electronics and Communications Engineering
- Energy Technology
- Engineering (General)
- Environmental Science, Engineering, and Technology
- History of Engineering and Technology
- Mechanical Engineering and Materials
- Technology of Industrial Chemistry
- Transport Technology and Trades
- Browse content in Environmental Science
- Applied Ecology (Environmental Science)
- Conservation of the Environment (Environmental Science)
- Environmental Sustainability
- Environmentalist Thought and Ideology (Environmental Science)
- Management of Land and Natural Resources (Environmental Science)
- Natural Disasters (Environmental Science)
- Nuclear Issues (Environmental Science)
- Pollution and Threats to the Environment (Environmental Science)
- Social Impact of Environmental Issues (Environmental Science)
- History of Science and Technology
- Browse content in Materials Science
- Ceramics and Glasses
- Composite Materials
- Metals, Alloying, and Corrosion
- Nanotechnology
- Browse content in Mathematics
- Applied Mathematics
- Biomathematics and Statistics
- History of Mathematics
- Mathematical Education
- Mathematical Finance
- Mathematical Analysis
- Numerical and Computational Mathematics
- Probability and Statistics
- Pure Mathematics
- Browse content in Neuroscience
- Cognition and Behavioural Neuroscience
- Development of the Nervous System
- Disorders of the Nervous System
- History of Neuroscience
- Invertebrate Neurobiology
- Molecular and Cellular Systems
- Neuroendocrinology and Autonomic Nervous System
- Neuroscientific Techniques
- Sensory and Motor Systems
- Browse content in Physics
- Astronomy and Astrophysics
- Atomic, Molecular, and Optical Physics
- Biological and Medical Physics
- Classical Mechanics
- Computational Physics
- Condensed Matter Physics
- Electromagnetism, Optics, and Acoustics
- History of Physics
- Mathematical and Statistical Physics
- Measurement Science
- Nuclear Physics
- Particles and Fields
- Plasma Physics
- Quantum Physics
- Relativity and Gravitation
- Semiconductor and Mesoscopic Physics
- Browse content in Psychology
- Affective Sciences
- Clinical Psychology
- Cognitive Psychology
- Cognitive Neuroscience
- Criminal and Forensic Psychology
- Developmental Psychology
- Educational Psychology
- Evolutionary Psychology
- Health Psychology
- History and Systems in Psychology
- Music Psychology
- Neuropsychology
- Organizational Psychology
- Psychological Assessment and Testing
- Psychology of Human-Technology Interaction
- Psychology Professional Development and Training
- Research Methods in Psychology
- Social Psychology
- Browse content in Social Sciences
- Browse content in Anthropology
- Anthropology of Religion
- Human Evolution
- Medical Anthropology
- Physical Anthropology
- Regional Anthropology
- Social and Cultural Anthropology
- Theory and Practice of Anthropology
- Browse content in Business and Management
- Business Ethics
- Business Strategy
- Business History
- Business and Technology
- Business and Government
- Business and the Environment
- Comparative Management
- Corporate Governance
- Corporate Social Responsibility
- Entrepreneurship
- Health Management
- Human Resource Management
- Industrial and Employment Relations
- Industry Studies
- Information and Communication Technologies
- International Business
- Knowledge Management
- Management and Management Techniques
- Operations Management
- Organizational Theory and Behaviour
- Pensions and Pension Management
- Public and Nonprofit Management
- Strategic Management
- Supply Chain Management
- Browse content in Criminology and Criminal Justice
- Criminal Justice
- Criminology
- Forms of Crime
- International and Comparative Criminology
- Youth Violence and Juvenile Justice
- Development Studies
- Browse content in Economics
- Agricultural, Environmental, and Natural Resource Economics
- Asian Economics
- Behavioural Finance
- Behavioural Economics and Neuroeconomics
- Econometrics and Mathematical Economics
- Economic History
- Economic Systems
- Economic Methodology
- Economic Development and Growth
- Financial Markets
- Financial Institutions and Services
- General Economics and Teaching
- Health, Education, and Welfare
- History of Economic Thought
- International Economics
- Labour and Demographic Economics
- Law and Economics
- Macroeconomics and Monetary Economics
- Microeconomics
- Public Economics
- Urban, Rural, and Regional Economics
- Welfare Economics
- Browse content in Education
- Adult Education and Continuous Learning
- Care and Counselling of Students
- Early Childhood and Elementary Education
- Educational Equipment and Technology
- Educational Strategies and Policy
- Higher and Further Education
- Organization and Management of Education
- Philosophy and Theory of Education
- Schools Studies
- Secondary Education
- Teaching of a Specific Subject
- Teaching of Specific Groups and Special Educational Needs
- Teaching Skills and Techniques
- Browse content in Environment
- Applied Ecology (Social Science)
- Climate Change
- Conservation of the Environment (Social Science)
- Environmentalist Thought and Ideology (Social Science)
- Natural Disasters (Environment)
- Social Impact of Environmental Issues (Social Science)
- Browse content in Human Geography
- Cultural Geography
- Economic Geography
- Political Geography
- Browse content in Interdisciplinary Studies
- Communication Studies
- Museums, Libraries, and Information Sciences
- Browse content in Politics
- African Politics
- Asian Politics
- Chinese Politics
- Comparative Politics
- Conflict Politics
- Elections and Electoral Studies
- Environmental Politics
- European Union
- Foreign Policy
- Gender and Politics
- Human Rights and Politics
- Indian Politics
- International Relations
- International Organization (Politics)
- International Political Economy
- Irish Politics
- Latin American Politics
- Middle Eastern Politics
- Political Behaviour
- Political Economy
- Political Institutions
- Political Methodology
- Political Communication
- Political Philosophy
- Political Sociology
- Political Theory
- Politics and Law
- Public Policy
- Public Administration
- Quantitative Political Methodology
- Regional Political Studies
- Russian Politics
- Security Studies
- State and Local Government
- UK Politics
- US Politics
- Browse content in Regional and Area Studies
- African Studies
- Asian Studies
- East Asian Studies
- Japanese Studies
- Latin American Studies
- Middle Eastern Studies
- Native American Studies
- Scottish Studies
- Browse content in Research and Information
- Research Methods
- Browse content in Social Work
- Addictions and Substance Misuse
- Adoption and Fostering
- Care of the Elderly
- Child and Adolescent Social Work
- Couple and Family Social Work
- Developmental and Physical Disabilities Social Work
- Direct Practice and Clinical Social Work
- Emergency Services
- Human Behaviour and the Social Environment
- International and Global Issues in Social Work
- Mental and Behavioural Health
- Social Justice and Human Rights
- Social Policy and Advocacy
- Social Work and Crime and Justice
- Social Work Macro Practice
- Social Work Practice Settings
- Social Work Research and Evidence-based Practice
- Welfare and Benefit Systems
- Browse content in Sociology
- Childhood Studies
- Community Development
- Comparative and Historical Sociology
- Economic Sociology
- Gender and Sexuality
- Gerontology and Ageing
- Health, Illness, and Medicine
- Marriage and the Family
- Migration Studies
- Occupations, Professions, and Work
- Organizations
- Population and Demography
- Race and Ethnicity
- Social Theory
- Social Movements and Social Change
- Social Research and Statistics
- Social Stratification, Inequality, and Mobility
- Sociology of Religion
- Sociology of Education
- Sport and Leisure
- Urban and Rural Studies
- Browse content in Warfare and Defence
- Defence Strategy, Planning, and Research
- Land Forces and Warfare
- Military Administration
- Military Life and Institutions
- Naval Forces and Warfare
- Other Warfare and Defence Issues
- Peace Studies and Conflict Resolution
- Weapons and Equipment

- < Previous chapter
- Next chapter >
10 Twin Studies and Behavior Genetics
Gabriëlla A.M. Blokland, Genetic Epidemiology Laboratory, Queensland Institute of Medical Research, School of Psychology and Centre for Advanced Imaging, University of Queensland, Brisbane, Australia
Miriam A. Mosing, Genetic Epidemiology Laboratory, Queensland Institute of Medical Research, School of Psychology, University of Queensland, Brisbane, Australia
Karin J.H. Verweij, Genetic Epidemiology Laboratory, Queensland Institute of Medical Research, School of Psychology, University of Queensland, Brisbane, Australia
Sarah E. Medland, Genetic Epidemiology Laboratory, Queensland Institute of Medical Research, School of Psychology, University of Queensland, Brisbane, Australia
- Published: 01 October 2013
- Cite Icon Cite
- Permissions Icon Permissions
Twin studies and behavior genetics address the questions raised by the nature versus nurture debate. Their aim is to estimate the extent to which individual differences in complex traits or phenotypes result from genetic and environmental influences. The vast majority of human behaviors and characteristics are complex traits and are influenced by both genetic and environmental influences, as well as the interplay between these two. Based on the differing genetic relatedness of monozygotic co-twins and dizygotic co-twins, the classical twin model allows for an estimation of the relative importance of these etiological factors. The classical twin model can be extended in multiple ways, depending on the phenotype, research question, and research design. In addition to the classical twin methodology, several such extensions are described in this chapter.
Introduction
In this chapter we will discuss some of the methodologies used in the genetic analysis of quantitative traits. The aim of this chapter is to provide an introduction to basic genetics and to the twin design as a method to study the etiology of individual differences in complex traits.
The phenotypic variation among species is extensive. In humans, this variation is observed across physical (e.g., height or weight), physiological (e.g., blood pressure or brain volume), cognitive (e.g., intelligence), and psychological (e.g., personality or depression) domains. The question of whether these individual differences in complex behavioral traits are caused by genetic (heritable) or environmental influences, or a combination of the two, is referred to as the nature versus nurture debate (Fig. 10.1 ), and dates back to ancient Greece ( Loehlin, 2009 ).
Comparing individual variation at the population level to the variation within a family shows that there is less variation within families than between families, with the least variation observed between individuals sharing their entire genome (i.e., identical twins). In the late 1800s, Francis Galton developed a number of statistical techniques including the correlation coefficient and regression, in order to study the way in which family resemblance for many traits increased with family relatedness ( Galton, 1889 ). These statistics underlie much of the behavioral and quantitative genetic techniques used today ( Plomin, DeFries, McClearn, & McGuffin, 2001 ).
The broad aim of quantitative genetic analyses is to estimate the extent to which differences observed among individuals result from genetic and environmental influences, and can thus directly address the questions raised by the nature-nurture debate. As shown in Figure 10.1 , within the scientific community it is generally accepted that the vast majority of human behaviors and characteristics are complex traits and are influenced by both genetic and environmental influences, as well as the interplay between these two.
Nature versus Nurture.
Notes: Individual differences on traits result from genetic and/or environmental influences, or a combination of both. Mendelian traits, such as Huntington’s disease, are (almost) entirely inherited, while traumatic brain injury can be caused by environmental exposures, such as a car accident. Quantitative traits are generally influenced by a combination of genetic and environmental influences.
Although the degree of sharing of environmental factors among related (as well as unrelated) individuals is hard to measure, the sharing of genetic factors between individuals is easy to quantify, because inheritance of most genetic material follows very simple rules. These rules were first postulated by Gregor Mendel in 1866 and have come to be referred to as the basic laws of heredity ( Plomin, DeFries, McClearn, & McGuffin, 2001 ). In his experimentation with pea plants, Mendel found that when crossing plants with different colored flowers (white and purple) the flowers of the resulting plant would still be purple (rather than lavender). These results led him to develop the idea of genetic loci (which he termed “heredity units"), which could either be additive or dominant. He concluded that each individual has two alleles , or versions of the genetic locus, one from each parent (note that didactic examples such as this one, are usually illustrated using the example of a bi-allelic locus with two variants, A and a; however, some types of loci have many more than two possible variants).
Within somatic cells, the DNA is arranged in two paired strands. Mendel established that, following the binomial distribution, within each individual the alleles at each locus can be paired as aa, Aa , or AA , with each pairing being referred to as a genotype . Cases where the genotype is composed of two copies of the same allele (i.e., AA or aa ), are denoted homozygotes , while those with differing alleles (i.e., Aa ), are referred to as heterozygotes . The frequency of each genotype reflects the frequency of each allele in the population. For example, if a has an allele frequency of p, and A has a frequency of q in the population, the frequencies of the three genotypes, aa, Aa , or AA , are p 2 , 2pq and q 2 . In didactic examples, q = 1 − p , however, this is not always true. Note also, that the frequency of the heterozygote is twice pq as this genotype can arise in two ways, Aa and aA , where the A allele can be inherited from either the mother or the father.
Mendel’s findings are summarized in two laws: (1) the law of segregation; and (2) the law of independent assortment ( Plomin, DeFries, McClearn, & McGuffin, 2001 ). The process of segregation occurs during gametogenesis , when the gametes or sex cells (egg and sperm) are formed. During this process the genotype separates; for example, a male with a heterozygous Aa genotype will usually develop approximately equal numbers of sperm carrying the A allele and the a allele. Thus, each of the parental alleles has an equal chance of being transmitted, regardless of the frequency of each allele within the population. Assortment refers to the process of segregation among many loci. This principle states that the inheritance of these loci is independent such that the process of segregation is random at each locus. An important caveat is that this principle does not hold if the loci are closely located on the same chromosome. This is because at the physical level stretches of DNA are co-inherited. This fact forms the basis of linkage analysis, which will be discussed in Chapter 11 .
A genetic effect is described as dominant if the heterozygous individuals show the same behavior or phenotype as one of the homozygotes. By convention, a capital letter (e.g., A ), is used to represent the dominant allele, while a lower case letter (e.g., a ), is used to describe the recessive allele. However, if the gene effects are additive (i.e., the trait value increases with each additional increasing allele, denoted A ), the observed trait or phenotype in the heterozygote will lie midway between the two homozygotes. While Mendelian laws were initially thought to apply only to traits influenced by single genes, it was subsequently shown by R.A. Fisher (1918 ) that they also apply to many complex and quantitative traits, where the phenotype results in part from the combined influence of multiple genes (Fig. 10.2 ).
At a genome-wide level the average amount of genetic sharing between two relatives can be calculated based on biometric genetic theory. A child shares 50% of their autosomal (i.e., non-sex chromosome) DNA with each of his parents. Similarly, siblings share on average 50% of their genetic material, and grandparents share on average 25% of their genetic material with their grandchildren (the same applies for half siblings and avuncular relationships). Analysis of data from related individuals, making use of the differences in genetic sharing between relatives, provides one way of estimating the relative magnitude of genetic (or heritable) and environmental influences on trait variation.
Heritability can be defined as the proportion of the phenotypic variance in a trait that is attributable to the effects of genetic variation ( Neale & Maes, 2004 ). Generally, the more diverse the relatedness of the participants included (i.e., parents, siblings, and cousins), the greater the power to disentangle genetic and environmental influences on trait variation ( Medland & Hatemi, 2009 ). A particularly attractive design to investigate genetic and environmental influences on trait variation is the adoption study. By comparing the resemblance between the adoptee and the adoptive family (environmental influence) versus the resemblance between the adoptee and the biological family (genetic influence) for a given trait, the relative contribution of genes and environment to variance in this trait can be estimated. However, this design is complicated by the difficulties associated with collecting data from the biological family, the nonrandom placement of adoptees and the effects of pre-adoptive experiences.
Genotypes to phenotypes: From single gene action to complex polygenic traits.
Notes: Given that each gene has 3 possible combinations of alleles ( aa, Aa , and AA ), under the assumption of additive genetic effects the homozygotes would be on the lower ( aa ) and the upper ( AA ) end of the phenotypic distribution, while the heterozygote is in the middle. If we extend this to include multiple genes, as would be the case for complex polygenic traits, with the inclusion of each new gene the distribution of phenotypic values in the sample increasingly resembles a normal distribution.
Arguably, the classical twin study represents the most practical and powerful family design available to researchers. This method compares the within-pair similarity of identical (monozygotic ; MZ) and non-identical (dizygotic; DZ) twins. Monozygotic twins develop when the developing zygote (fertilized egg) divides, usually within 2 weeks of fertilization, and the two parts continue to develop independently (Fig. 10.3 ). In this case, both twins originate from the same sperm and egg, which makes them genetically identical and, therefore, they are always of the same sex. The later the twinning event occurs, the more likely the twins are to share chorion (which is comprised of the placenta and related membranes) and amniotic sacs ( Derom et al., 2001 ; Baergen, 2011 ). In contrast, DZ twinning occurs when more than one egg is released by the ovaries at the same time and, subsequently, each of the eggs is fertilized by a separate sperm cell. As a result, DZ twins do not differ from normal siblings genetically, sharing on average 50% of their genetic loci. However, they do have shared prenatal environments, as they were conceived at the same time and shared the womb. Dizygotic twins, like normal siblings, can either be of the same or of the opposite sex (i.e., a male and a female).
In order to facilitate the use of twin designs many countries have set up twin registries, collecting information on twins and their families. The oldest national twin register is the Danish Twin Registry, initiated in 1954, currently listing more than 75,000 twin pairs ( Skytthe et al., 2006 ). Subsequently, many more countries have followed the Danish example by setting up large nationwide twin registries (e.g., Sweden, Australia, and the Netherlands). One of the biggest challenges for twin registries is the correct ascertainment of the zygosity of twins (MZ versus DZ). Until recently, zygosity was mainly determined by means of a questionnaire assessing twin similarity between same-sex twins. This method has proven to be efficient in 95% of cases ( Kasriel & Eaves, 1976 ). Over the past thirty years, however, gene-finding methods became available, enabling the precise determination of zygosity status; these have largely been used to confirm and replace zygosity determination based on questionnaires ( Plomin, DeFries, McClearn, & McGuffin, 2001 ). To date numerous twin studies on a very large variety of traits have been conducted. Although originally the focus was on “simple” (physical) traits such as height, soon twin studies were used to explore the variation in increasingly complex traits, such as intelligence, personality, psychiatric disorders, etc.
The development of monozygotic versus dizygotic twins.
As mentioned above, the phenotypes and genotypes of related individuals are not independent, nor are they identically distributed; therefore, many standard statistical tests cannot and/or should not be applied in the analyses of relatives. Most analyses based on related individuals use statistical approaches based on likelihood, as this very general statistical framework has high modeling flexibility (e.g., Maes et al., 2009 ; Neale & Maes, 2004 ). These statistical approaches will be explained in this chapter.
The Classical Twin Model
As mentioned above, the classical twin design draws its explanatory power from the differences in genetic sharing of MZ and DZ twins. Using simultaneous equations, this knowledge can be used to partition the variance in a phenotype into that which results from additive genetic (A), dominant genetic (D), common or shared environmental (C) and unique or unshared environmental (E) influences. Additive and dominant genetic influences refer to the cumulative effect of genes acting in an additive or dominant manner. Common environmental influences refer to experiences shared by co-twins, including the intrauterine environment, and the social and cultural rearing environment (i.e., same socio-economic status, parents, diet, etc.) Unique environmental factors comprise all aspects of the physical and social environment experienced differentially by individuals in a family, such as illness, physical and psychological trauma, peers, teachers, etc. This component also includes measurement error and gene–environment interactions when not accounted for in the modeling ( Eaves, Last, Martin, & Jinks, 1977 ; Jinks & Fulker, 1970 ).
The classical twin model assumes that phenotypic variation results from the sum of these sources, such that the total variance can be written as: A + C + D + E. Monozygotic twins share approximately 100% of their genetic information (A and D), as well as 100% of their common or shared environment (C).Thus, the MZ covariance (i.e., the covariance between twin 1 and 2 of an MZ pair) can be written as: A + C + D. Conversely, DZ twins are assumed to share, on average, 50% of their segregating genes, and 25% of the time they share the same paternal and maternal alleles (which are required to share dominant effects). In addition, they are assumed to share 100% of the common environment. Thus, the DZ covariance can be written as: 0.5A + C + 0.25D.
As will be obvious from these three equations, there is insufficient information within the classical twin model to simultaneously estimate the magnitude of all four sources of variance. As such, twin studies tend to estimate either C or D. This is because these measures are negatively confounded; that is, dominance effects tend to reduce the DZ correlation relative to the MZ correlation (i.e., make MZ twins more similar), whereas the common environment tends to increase the DZ correlation relative to the MZ correlation (i.e., makes DZ twins more similar). One or the other source can be assumed absent depending on whether the DZ twin correlation is greater or less than half the MZ correlation. In general an ACE model would be estimated if the DZ correlation is greater than half of the MZ correlation, and an ADE model if the DZ correlation is less than half of the MZ correlation.
In either case, the extent to which MZ twin pairs resemble each other more for a trait (i.e., show higher twin correlations) than DZ twin pairs gives information on the relative influence of genetic versus environmental factors on a trait. Under the ACE model, the proportion of variance resulting from additive effects (A) or the heritability ( a 2 ), can be calculated as twice the difference between the MZ and DZ correlations ( Holzinger, 1929 ): a 2 = 2 ( r MZ ∼ r DZ ). An estimate of shared environment (C or c 2 ) can be calculated via twice the DZ correlation minus the MZ correlation: c 2 = 2 r DZ ∼ r MZ . Because MZ twins do not share the non-shared environmental variance (E or e 2 ), 1 minus the MZ correlation gives the contribution of the non-shared environment: e 2 = 1 − r MZ . Because correlations are standardized (with unit variance), the total phenotypic variance (A + C + E) is also standardized. Therefore, each variance component represents the relative contribution to a trait.
Twin correlations.
Notes: Scatter plots showing MZ and DZ twin pair correlations for (a) height in cm (males only) and (b) adolescent misconduct based on questionnaire data. Twin correlations for height indicate a high heritability for this trait, whereas twin correlations for adolescent misconduct point to moderate heritability. Data were provided by the Genetic Epidemiology Laboratory, Queensland Institute of Medical Research.
If we apply these formulas to the example data for height in Figure 10.4a , where the MZ twin correlation is 0.88 and the DZ correlation is 0.44, the heritability would be a 2 = 2 * (0.88 − 0.44) = 0.88, and the common environmental influence would be c 2 = (2 * 0.44) − 0.88 = 0. A heritability of 0.88 should be interpreted to mean that 88% of the population variance in a trait can be attributed to variation at the genetic level. Importantly, this cannot be interpreted as height being genetically controlled for 88% of individuals. The estimate of the proportion of variance accounted for by E for this trait is 12%; notably, variance resulting from measurement error is also included in this estimate. For adolescent misconduct (Fig. 10.4b ), where the MZ twin correlation is 0.70 and the DZ correlation is 0.47, the heritability would be a 2 = 2 * (0.70 − 0.47) = 0.46 and the common environmental influence would be c 2 = (2*0.47)− 0.70 = 0.24.
Figure 10.4b also illustrates how the range of values for the trait under investigation can affect the data distribution. In twin modeling it is important that the trait of interest shows a normal distribution in the entire sample, as well as in the MZ and DZ subsamples. If this is not the case, transformation of the data may be necessary. Otherwise, alternative models are available for data that violate this assumption ( see section on the liability threshold model).
Structural Equation Modeling
The formulas developed by Holzinger (1929 ) are limited in their application to continuous phenotypes and univariate contexts. As much of the focus of modern quantitative genetics is on estimating the contribution of genetic effects to the covariation between phenotypes, the Holzinger method is seldom used in contemporary studies. The majority of current studies now use more sophisticated structural equation models to estimate these influences ( Eaves, 1969 ; Eaves, Last, Martin, & Jinks, 1977 ; Martin & Eaves, 1977 ). These new methodologies allowed the development of models that more accurately reflect the complexities of human behavior and development ( Mehta & Neale, 2005 ). Structural equation modeling (SEM) is used to test complex relationships between observed (measured) and unobserved (latent) variables and also relationships between two or more latent variables ( Wright, 1921 ). For a more detailed explanation of structural equation modeling methodology, please refer to Chapter 15 . The parameters of the structural equation model for the pattern of MZ and DZ variances and covariances can be estimated by several approaches, including maximum likelihood and weighted least squares . In this chapter we will assume that maximum likelihood methods are used.
Path diagrams (Fig. 10.5 ) provide a graphical representation of models. Path diagrams can be mapped directly to mathematical equations and are sometimes easier to understand. Structural equation modeling allows us to obtain maximum likelihood estimates of phenotypic means and genetic and environmental variance components, while also allowing for the explicit modeling of effects of covariates (e.g., sex, age, IQ) and interaction effects. The aim of maximum likelihood estimation is to find the parameter values that explain the observed data best. Likelihood ratio tests, which are asymptotically distributed as chi-square (χ 2 ), are used to compare the goodness of fit of reduced submodels (i.e., AE, CE, and E models) with that of the full ACE model. Model fit is evaluated according to the principle of parsimony , in which models with fewer parameters are considered preferable if they show no significant worsening of fit ( p > 0.05) when compared to a full ACE model. A larger χ 2 (corresponding to a low probability) indicates a poor fit of the submodel; a smaller x 2 (accompanied by a non-significant p value) indicates that the data are consistent with the fitted model.
For example, if dropping the A parameter from the ACE model (i.e., by equating the additive genetic path coefficient to zero) results in a significant worsening of model fit ( p < 0.05), this signifies that the simpler CE model is not an accurate description of the observed data, and thereby indicates the significance of the genetic influences. Components of variance (A, C, or E) are calculated by dividing the squared value of the corresponding path coefficient by the total variance (i.e., the summed squared values of all path coefficients).
From Figure 10.5 , the following algebraic statements can be derived for the variance/covariance matrices of MZ and DZ twins (Matrix 10.1 ), where the variance for each twin is located on the diagonal (shaded dark gray) with the covariance between twins on the off-diagonal (shaded light grey).
As mentioned previously, when estimating an ACE model it is assumed that there is no variance resulting from non-additive genetic influences (D).
Path diagram depicting the classical twin model. Notes: P = phenotype; T1 = twin 1 of a pair; T2 = twin 2 of a pair; MZ = monozygotic; DZ = dizygotic; A = additive genetic influences; C = common environmental influences; E = unique environmental influences; a = additive genetic path coefficient; c = common environmental path coefficient; e = unique environmental path coefficient. Circles represent latent, unobserved variables; squares represent observed phenotypes; single-headed arrows represent influences of latent variables on observed variables; double-headed arrows represent (co) variances. Correlations between additive genetic factors (A) are fixed at 1 for MZ pairs and 0.5 for DZ pairs, because MZ twins share 100% of their segregating genes and DZ twins on average 50%. Correlations between common environmental factors (C) are fixed at 1 for both MZ and DZ twins, because both types of twins share 100% of their familial environment. By definition, E factors are left uncorrelated in both MZ and DZ twins because they are unique for each individual.
Variance resulting from non-additive genetic influences (D) may also be estimated, where correlations between MZ twins are fixed at 1 and correlations between DZ twins are fixed at 0.25. The covariance structure of an ADE model is summarized in Figure 10.6 and in the matrix below (Matrix 10.2 ), where the variance for each twin is located on the diagonal (shaded dark gray) with the covariance between twins on the off-diagonal (shaded light grey).
The most commonly used software package for twin modeling is the flexible matrix algebra program, Mx ( Neale, Boker, Xie, & Maes, 2002 ); Mx can be downloaded from: http://www.vcu.edu/mx/ . The Mx website also contains (links to) example code for various models. Recently, Mx has been implemented within the R programming environment under the new name OpenMx ( Boker et al., 2011 ); OpenMx and R can be downloaded from the following pages: http://openmx.psyc.virginia.edu/installingopenmx and http://www.r-project.org/ , respectively. The OpenMx website also contains example code as well as a forum where OpenMx-related topics can be discussed. Another program suitable for twin modeling is Mplus ( Muthen & Muthen, 1998-2010 ); the Mplus homepage can be found at: http://www.statmodel.com/ . For family studies, when not utilizing twin data, SOLAR (Sequential Oligogenic Linkage Analysis Routines) can be used; the software can be downloaded from: http://solar.sfbrgenetics.org/download.html .
Path diagram depicting the ADE model.
Notes: P = phenotype; T1 = twin 1 of a pair; T2 = twin 2 of a pair; MZ = monozygotic; DZ = dizygotic; A = additive genetic influences; D = dominance genetic influences; E = unique environmental influences; a = additive genetic path coefficient; d = dominance genetic path coefficient; e = unique environmental path coefficient. Circles represent latent, unobserved variables; squares represent observed phenotypes; single-headed arrows represent influences of latent variables on observed variables; double-headed arrows represent (co)variances.
Assumptions of the Classical Twin Model
Several assumptions underlie the classical twin design, including generalizability, random mating, equal environments, and absence of genotype-environment interaction or genotype-environment correlation. These assumptions will be explained below.
Generalizability
A frequently asked question regarding twin studies is whether their results can be generalized to the general population (i.e., singletons). The experience of being a twin, including the sharing of limited space and resources during gestation, and the differences in the birth process, may cause twins to be different from singletons. Generalizability can be assessed by comparing means and variances for a trait between twins and members of the general population, which are matched for age and sex. However, the best method of assessing generalizability is by extending the twin design to include the twins’ own siblings within the analysis. Comparing the DZ co-twin correlation with twin-sibling correlations allows an examination of the role of pre-or perinatal factors on the trait of interest (correcting for age). One of the advantages of comparing twins with their own non-twin siblings is that by using siblings as the control group we can, at least partly, control for variance in maternal size (i.e., intrauterine size and body shape, which may influence the length of gestation and ease of delivery) and the effects of genetic transmission (as both DZ twins and their full siblings share, on average, 50% of their genetic material). Although twins do differ from singletons for some traits, especially those related to prenatal growth, most studies generally do not find differences in personality and social traits ( Evans, Gillespie, & Martin, 2002 ). If this assumption is violated, additional twin-specific effects will need to be incorporated in the model.
Random Mating
The assumption that DZ twins share on average 50% of their genes no longer holds true in the case of assortative mating . Visscher et al. (2006 ) used molecular data to get exact measures of average genetic sharing of sibling pairs, which in a sample of 4,401 sibling pairs ranged from 37% to 61%. Assortative mating may be based on phenotypic similarity (positive assortment) or dissimilarity (negative assortment). Positive assortative mating refers to the situation where prospective mating partners are more likely to select each other when they possess similar traits. As these traits will probably be at least partly caused by similar gene variants, their children are likely to share more than 50% of their genetic information, for genetic loci influencing the trait of interest. To illustrate, Maes et al. (1998 ) investigated assortative mating in the context of major depression, generalized anxiety disorder, panic disorder, and phobias, and found considerable associations between partners for most psychiatric diagnoses. Assortment was observed both within and between classes of psychiatric disorders. Variables correlated with the psychiatric diagnoses, such as age, religious attendance, and education, did explain part, but not all, of the assortment between partners.
Because assortative mating increases the correlations between mates, estimates of the relative genetic and environmental influences based on a twin design will be biased if assortative mating is present and is not appropriately accounted for. When parents are more genetically alike than expected by chance, the DZ twins’ genetic resemblance will on average be more than 50% because of the transmission of the correlated parental genes. As a result, the resemblance of DZ twin pairs will increase relative to MZ twin pairs. Unmodeled assortative mating will therefore result in artificially inflated estimates of the shared environmental component and an underestimation of heritability. The presence of assortative mating can be studied by calculation of the phenotypic correlation between the parents of twins, or the phenotypic correlation between twins and their spouses, assuming that the extent of assortative mating does not change across generations.
The Degree of Genetic Similarity Between MZ Twins
Although MZ twins are assumed to be genetically identical, a study of19 MZ twin pairs detected subtle differences in copy number variations of the DNA ( Bruder et al., 2008 ). These differences occur when a set of coding nucleotide bases in DNA are missing or when extra copies appear. It is currently theorized that at the time of conception MZ twins are genetically identical; however, during subsequent DNA replications and cell division, a small number of mutations may occur. The same phenomenon would also decrease the “known” degree of relatedness between DZ twins (50%), parents and children (50%), half siblings (25%), etc. This would also mean that age differences would influence the degree of relatedness in family studies (i.e., newborns would have fewer mutations than their older family members simply because they are younger).
Equal Environments
The twin method partitions the environment into that which is shared between co-twins and that which is unshared. Generally the shared environment is assumed to include prenatal effects and the effects of growing up in the same household. This interpretation relies on the assumption that MZ and DZ twins experience shared environments to the same extent (i.e., that trait-relevant environmental influences contribute equally to the resemblance of MZ and DZ twin pairs). This assumption has received much attention. It has been found that MZ twins are treated more similarly than DZ twins in certain aspects; as young children they share a bedroom and are dressed alike more often, and they are more likely to share the same friends and stay in closer contact once they leave home ( Cohen, Dibble, Grawe, & Pollin, 1973 ; Kendler, Heath, Martin, & Eaves, 1987 ; Loehlin & Nichols, 1976 ). However, it is not clear whether greater environmental similarity results in greater phenotypic similarity.
Furthermore, as highlighted by Heath et al. (1989 ), environmental inequality would only result in bias if the trait of interest happened to be affected by those environmental factors that differ between twins. Salient environmental influences that are more similar for MZ compared to DZ twins would increase twin correlations in MZ twins, inappropriately inflating estimates of trait heritability. Several methods have been used to test the equal environments assumption, including correlating perceived zygosity with the trait while controlling for actual zygosity ( Kendler et al., 1993 ; Matheny, Wilson, & Dolan, 1976 ; Plomin, Willerman, & Loehlin, 1976 ; Scarr, 1982 ; Scarr & Carter-Saltzman, 1979 ), direct observation of family members and others to examine their self-initiated and twin-initiated behaviors toward the different twin types ( Lytton, Martin, & Eaves, 1977 ), and correlating the similarity of the twin environments with the trait while controlling for actual zygosity ( Borkenau, Riemann, Angleitner, & Spinath, 2002 ; Heath, Jardine, & Martin, 1989 ; Kendler et al., 1987 ; Martin et al., 1986 ).
A modeling-based approach is the extension of the classical ACE model by partitioning the common environment into the usual common environment, C residual , which is completely correlated for all twin pairs, and that which is influenced by the perceived zygosity, C specigc , which is parameterized to be completely correlated if both twins perceive themselves to be MZ, completely uncorrelated if both twins perceive themselves to be DZ, and correlated at 0.5 if the twins disagree about their perceived zygosity ( Hettema, Neale, & Kendler, 1995 ; Kendler et al., 1993 ; Scarr & Carter-Saltzman, 1979 ; Xian et al., 2000 ).
Furthermore, when data have been collected from non-twin siblings, checking for differences between the DZ covariance and the twin–sibling and sibling–sibling covariances can provide an additional test of the equal environments assumption. Arguably, if the more similar treatment of MZ twins were affecting their trait values, one might also expect more similar treatment of DZ twins as compared to regular siblings. When using ordinal data, equality of the thresholds of MZ and DZ twins indicates there are no differences in variances between MZ and DZ twin pairs, excluding the possibility of an extra environmental influence specific to MZ twins. The most recent method to remove equal environment biases allows heritability to be estimated from non-twin siblings ( Visscher et al., 2006 ).
Although MZ and DZ mean differences have been found for traits such as birth weight ( Koziel, 1998 ) and similar dress ( Matheny, Wilson, & Dolan, 1976 ), rigorous and frequent testing of characteristics such as physical twin similarity( Hettema, Neale, & Kendler, 1995 ), self-perceived zygosity ( Xian et al., 2000 ), perceived zygosity and associated parental approach to rearing their twins ( Cronk et al., 2002 ; Kendler & Gardner, 1998 ; Kendler et al., 1993 ; Kendler et al., 1994 ), self-reported similarity of childhood experiences ( Borkenau, Riemann, Angleitner, & Spinath, 2002 ), and physical and emotional closeness between the twins ( Cronk et al., 2002 ; Kendler & Gardner, 1998 ; LaBuda, Svikis, & Pickens, 1997 ), has shown that these traits are uncorrelated with zygosity differences in intelligence, personality, and psychiatric disorders such as alcohol and illicit drug dependence, major depression, anxiety, and externalizing disorders, thereby supporting the validity of the equal environmental assumption in twin studies assessing these phenotypes.
Genotype-Environment Interaction
The classical twin model does not take the possible presence of genotype–environment (GxE) interaction into account. Gene–environment interaction occurs when environments have differential effects on different genotypes. For example, Boomsma and colleagues (1999) found that a religious upbringing reduces the influence of genetic factors on disinhibition. A recent study of borderline personality disorder by Distel et al. (2011 ) also found evidence for GxE interaction. For individuals who had experienced a divorce/break-up, violent assault, sexual assault, or job loss, environmental variance for borderline personality disorder features was higher, leading to a lower heritability in exposed individuals. Jinks and Fulker (1970 ) suggested a screening test for GxE interaction using data from MZ twin pairs, whereby the intrapair differences are plotted against the sum of the co-twins’ phenotypic values. A significant correlation between these two indicates the presence of GxE interaction. However, to avoid spurious results, this test requires data from MZ twins reared apart, and it is unsuitable for binary data. Purcell (2002 ) proposed another approach to the detection of GxE interaction, which allows the explicit modeling of the interaction through extension of the classical twin design. In order to model the interaction, the environmental covariate(s) must be entered into the analysis as an observed variable, thus limiting the application of this approach to the study of already known or suspected environmental covariates.
Genotype-Environment Correlation
Gene–environment correlation ( r GE ) occurs when individuals actively or passively expose themselves to different environments depending on their genotype, or when individuals’ genotypes affect their social interactions or influence the responses they elicit from other individuals ( Falconer & Mackay, 1996 ; Plomin, DeFries, & Loehlin, 1977 ). If r GE is positive it could result in an increase in the total phenotypic variance of the trait. Alternatively, in the case of a negative r GE , the total phenotypic variance would be decreased. Distel et al. (2011 ) also found evidence for gene–environment correlation. The genetic effects that influence borderline personality disorder features also increased the likelihood of being exposed to certain life events. Three types of r GE have been described by Plomin et al. (1977 ), namely cultural transmission, autocorrelation, and sibling effects .
Cultural transmission refers to the environmental effect of the parental phenotype on the offspring’s phenotype ( Neale & Maes, 2004 ; i.e., resemblance between parents and offspring that results from a home environment created by the parents). To use a simplistic example, imagine two children taking part in a study of reading ability. Both children come from the same socio-economic strata and have very similar backgrounds. The parents of child A enjoy reading and have many books in their home, thus child A is read to as young child, observes his/her parents reading, and grows up in an environment where books are accessible. The parents of child B do not enjoy reading; they do not own many books and do not use the local library. Child B thus grows up in an environment where books are less accessible, and despite being read to as young child because the parents feel this is important, child B does not often observe his/her parents reading. As it is likely that the environmental differences between the two children are related to the genetic variants influencing reading ability, the environmental and genetic effects become correlated. Failure to model this correlation can inflate the heritability of reading ability in the children. The effects of cultural transmission may be examined by extending the twin design to include parental data. Such a design also allows for a test of the assumption of random or non-assortative mating ( Neale & Maes, 2004 ).
Gene–environment autocorrelation occurs when environments are not randomly assigned to each individual but are, in part, individually selected on the basis of genetically influenced preferences. For example, when gifted individuals create or evoke situations that further enhance their intellectual ability, or when genetically introverted individuals choose to avoid situations where they may be the focus of attention.
Sibling interactions may be either cooperative, increasing the trait value of the co-twin (imitation effect), or competitive, decreasing the trait value in the co-twin (contrast effect; Carey, 1986 ). Cooperation effects increase the variance and decrease the covariance of MZ twins relative to DZ twins, while competition produces the opposite effects.
Correlated effects of genotypes and environments are difficult to detect. If not explicitly modeled, r GE between the latent A and E variables behave like additive effects, whereas r GE between the latent A and C variables acts like C.
Extensions to the Classical Twin Model
The classical twin model is the most basic twin model one can employ. There are many extensions available, the most basic of which is the incorporation of covariates to improve the estimation of phenotypic means. This allows for correction for effects such as age and gender, but also for effects of other variables that may confound the estimation of heritability. For example, if our trait of interest is cerebro-vascular disease in addition to age and gender, we may want to include smoking behavior as a covariate; if our trait of interest is education, we may want to include socio-economic status as a covariate.
Sex Limitation
Sex differences may obscure the data in different ways. Opposite-sex DZ twins can reduce the overall DZ twin covariance significantly if males and females differ greatly in their phenotypic values. Sex limitation refers to sex differences in the magnitude and/or proportion of the variance accounted for by genetic and environmental effects ( Neale & Maes, 2004 ). If twin pair correlations differ between the sexes within zygosity, it is better to estimate A, C, and E separately for males and females. Three types of sex limitation have been described: quantitative, qualitative, and scalar.
In the quantitative sex limitation model the genetic and environmental sources of variance and covariance in males and females are assumed to be the same (i.e., sex-specific pathways are fixed to zero) but the magnitudes of these effects are allowed to differ and the correlations for additive genetic and common environmental influences in the opposite-sex DZ pairs are assumed to be 0.5 and 1, respectively. If data from opposite-sex DZ twins have been collected, the difference in fit (χ 2 ) between this model and the qualitative sex limitation model can be used to examine whether the same genetic or environmental factors are influencing males and females ( Neale & Maes, 2004 ). Silventoinen et al. (2001 ) did this for height in two cohorts of twins (born in 1938-1949 and in 1975-1979) and found that the heritability estimates were higher among men ( h 2 = 0.87 in the older cohort and h 2 = 0.82 in the younger cohort) than women ( h 2 = 0.78 and h 2 = 0.67, respectively). Sex-specific genetic factors were not statistically significant in either cohort, suggesting that the same genes contribute to variation in body height for both men and women.
The hypothesis underlying qualitative sex limitation models is that different genetic or environmental factors influence trait variation in males and females. This model includes an extra genetic or environmental component ( m 2 ) that contributes to either males or females. Differences in both genetic and environmental effects cannot be tested simultaneously when working with twin and sibling data. Therefore, one would usually run this model twice; once specifying m 2 as an additive genetic parameter ( r = 0.5 for DZ twins) and once specifying m 2 as a common environment parameter ( r = 1 for DZ twins). Derks and colleagues (2007 ) found this to be the case for attention deficit-hyperactivity disorder (ADHD) and oppositional defiant disorder (ODD). The heritabilities for both ADHD and ODD were high and of a similar magnitude for boys and girls. However, the source of this genetic variation differed between boys and girls, indicating that some genetic loci may be having sex-specific influences on these traits.
The scalar sex limitation is the simplest and most restrictive of the three models. Here the absolute magnitude of the total variance, and thus the unstandardized variance components, differ between males and females while the proportion of variance accounted for by genetic and environmental effects, that is, the standardized variance components, are equal across sexes. In the scalar model not only are the sex-specific effects removed, but the variance components for females are all constrained to be equal to a scalar multiple ( k ) of the male variance components, such that a f 2 = k a m 2 , , and e f 2 = k e m 2 ( Neale & Maes, 2004 ). This model is a submodel of both the quantitative and qualitative sex limitation models, and can only be tested using continuous data, as variances are fixed to unity when working with ordinal data.
Normally you would test for the presence of sex limitation as part of testing a series of assumptions prior to fitting the ACE or ADE model. These assumptions include the equality of means and variances across zygosity and birth order, the equality of means and variances between twins and siblings, and the equality of means, variances, and covariances across the two sexes.
Liability Threshold Model
The classical twin design assumes that the trait of interest is a continuous variable, with a normal distribution. However, many traits that may be of interest are ordinal or dichotomous variables, such as medical or psychiatric diagnoses. For such variables, a liability threshold model can be used to estimate twin correlations and heritability. Threshold models assume that there is an underlying continuum of liability (e.g., to depression or ADHD) that is normally distributed in the population, and that our measurement categories (e.g., depressed/not depressed) result from one or more artificial divisions (thresholds) overlaying this normal distribution. Analyses are effectively performed on the underlying liability to the trait, resulting in estimates of the heritability of the liability. Figure 10.7 illustrates the threshold model. Panel A shows a model with a single threshold, separating persons into two classes, unaffected or affected, such as children with ADHD and controls. Panel B shows a liability threshold model with four thresholds (i.e., five categories), which could apply to a study of self-rated health, where the response categories were “very good,” “good,” “fair,” “poor", and “very poor” (e.g., Mosing etal., 2009 ). Liability to psychiatric disorders such as ADHD, depression, anxiety, and schizophrenia has been found to be influenced by genetic factors ( Hettema, Neale, & Kendler, 2001 ; Jepsen & Michel, 2006 ; Kendler, Gatz, Gardner, & Pedersen, 2006a , 2006b ; Sullivan, Kendler, & Neale, 2003 ; Sullivan, Neale, & Kendler, 2000 ), with heritability estimates of >70%.
The threshold model.
Notes: (a) Univariate normal distribution for dichotomous phenotype. One threshold is shown (at z-value +0.5) corresponding to 2 categories with the frequencies 69% and 31%. (b) Univariate normal distribution with thresholds distinguishing ordered response categories. Four thresholds are shown (at z-values −2.30, −1.70, −0.5, and +1) corresponding to 5 categories with the frequencies, 1%, 3%, 27%, 53%, and 16%.
Including Data from Additional Family Members
As briefly mentioned above, the classical twin design can be extended by including singleton (non-twin) siblings, parents, children, and spouses. Including additional family members substantially enhances the statistical power to detect non-additive genetic and common environmental influences resulting from a greater number of observed covariance statistics ( Posthuma et al., 2003 ). The power to detect common environmental influences is maximized when there are four times as many DZ pairs as MZ pairs ( Nance & Neale, 1989 ). As siblings have the same amount of genetic material in common as DZ twins (on average 50%), including data from extra siblings in the model effectively increases the DZ to MZ ratio. As discussed above, adding data from non-twin siblings makes it possible to test for twin-specific environmental influences. The variance and covariance of additional siblings are modeled in the same way as for a DZ twin (Fig. 10.8 ). If we were to include the data of one extra sibling the typical variance-covariance matrix would
Path diagram depicting the extended twin model.
Notes: P = phenotype; T1 = twin 1 of a pair; T2 = twin 2 of a pair; SIB = singleton sibling; MZ = monozygotic; DZ = dizygotic; A = additive genetic influences; C = common environmental influences; E = unique environmental influences; a = additive genetic path coefficient; c = common environmental path coefficient; e = unique environmental path coefficient. Circles represent latent, unobserved variables; squares represent observed phenotypes; single-headed arrows represent influences of latent variables on observed variables; double-headed arrows represent (co)variances.
be extended as shown in Matrix 10.3 . Additional siblings can be added in the same way. Variances are on the diagonal highlighted in the darkest shade of gray, the intrapair covariances are a shade lighter on the off-diagonal, and the twin–sibling covariances are highlighted in the lightest shade of grey on the outermost row and column of the matrix.
The extended twin family model or the nuclear family model also allows for the estimation of more parameters and relaxed assumptions regarding mating and cultural transmission. For example, adding parental data to the model makes it possible to estimate effects from assortative mating, familial transmission, sibling environment, and the correlation between additive genetic effects and family environment ( Keller et al., 2009 ), as well as allowing for the simultaneous estimation of C and D influences.
Another method allowing for the estimation of A, C, D and E in the same model is the twin adoption design. Here, twins raised apart (with no shared environmental influences) are compared to twins raised together. This design has a great explanatory power to facilitate separation of biological from environmental influences ( Medland & Hatemi, 2009 ). However, because of ethical and legal hurdles, twin adoption studies are increasingly difficult to conduct. Also, modern adoption policies facilitate twins being adopted together, rapidly decreasing the number of twins reared apart. Finally, there are other methodological factors that have to be taken into account, such as contact with the biological family, age of adoption, time spent in state care or protective custody, and selective placement (i.e., matching of the infants’ biological and adoptive environments), each of which may bias the sample. As a result of these caveats, which are hard to overcome, the twin adoption design is used only rarely and will not be explained here in further detail.
Multivariate Modeling
The twin model can also be extended to include multiple phenotypes. In the case of a multivariate design the aim is to decompose the covariance between traits into that caused by A, C, and E in the same way as one would with the phenotypic variance of a single trait. A multivariate design allows us to investigate the extent to which common sets of genes (genetic correlation, r g ), shared environmental factors (common environmental correlation, r c ) or unshared environmental factors (unique environmental correlation, r e ) underlie correlations between phenotypes. Matrix 10.4 shows a schematic representation of the variance/covariance matrix for a bivariate model. The corresponding path diagram is shown in Figure 10.9 .
The model in Figure 10.9 employs Cholesky decomposition (named after its developer Andre-Louis Cholesky) and can be extended in a similar way to include many more phenotypes. In linear algebra, the Cholesky decomposition or Cholesky triangle is a decomposition of a symmetric, positive-definite matrix into the product of a lower triangular matrix and its conjugate transpose. Cholesky decomposition or triangular decomposition, illustrated in Figure 10.9 for two variables, can represent a multivariate analysis of simultaneously measured variables considered in some rationally defined order of priority ( Loehlin, 1996 ; Neale & Maes, 2004 ). The first latent additive genetic variable (A1) explains the genetic influences on the first phenotype (P1) and the correlated genetic influences on the second phenotype (P2). The second latent additive genetic variable (A2) is uncorrelated with A1 and explains the remaining heritability of P2. Similar latent structures are estimated for E and C or D. From this basic model, parameters can be dropped or equated to test specific hypotheses regarding those parameters. The goal is to explain the data with as few underlying factors as possible, by testing which paths are significant (i.e., by setting the path coefficients to zero and noting whether this results in a significant decrease in model fit). For a review on how to use SEM programs to perform Cholesky decomposition please see, for example, Raykov, Marcoulides, and Boyd (2003 ).
Multivariate modeling can accommodate numerous variables, and can be used for both exploratory and confirmatory factor analysis, as well as longitudinal and causal analyses. It should be emphasized that the final results depend on the ordering—if we had considered the latent variables in the reverse order, A2 would be a factor with paths to both variables, and A1 a residual. Only in the case of uncorrelated variables is the order of selection irrelevant ( Loehlin, 1996 ), but in that case multivariate modeling should not be used anyway.
One example application of multivariate twin modeling is the use of this method to examine genetic contributions to the comorbidity between psychiatric disorders. More than a dozen studies have revealed a shared genetic vulnerability between anxiety and depression, particularly between major depressive disorder and generalized anxiety disorder ( see Cerda, Sagdeo, Johnson, & Galea, 2010 , for a review).
Common Pathway Model
The common and independent pathway models can be considered submodels of the standard Cholesky decomposition. The common pathway model hypothesizes that the covariation between variables results from a single underlying “phe-notypic” latent variable. A frequent application of this model is the examination of symptom dimensions in complex, heterogeneous diseases. For example, van Grootheest et al. (2008 ) applied this model to obsessive-compulsive behavior and found that the three symptom dimensions—Rumination, Contamination, and Checking—share variation with a latent common factor, denoted obsessive-compulsive behavior. Variation in this common factor was explained by both genes (36%) and environmental factors (64%). Only the Contamination dimension was influenced by specific genes and seemed to be a relatively independent dimension. The results suggest that a broad obsessive-compulsive behavioral phenotype exists, influenced by both genes and unshared environment. However, the common pathway model, although it is conceptually attractive, often does not fit the observed data well because the amount of genetic and environmental variation transmitted from the latent factor is defined by the phenotypic correlation between the measured and latent variables ( Medland & Hatemi, 2009 ).
Path diagram depicting the bivariate twin model.
Notes: P1 + phenotype 1; P2 + phenotype 2; T1 + twin 1 of a pair; T2 + twin 2 of a pair; MZ + monozygotic; DZ + dizygotic; A1 + additive genetic influence 1; C1 + common environmental influence 1; E1 + unique environmental influence 1; A2 + additive genetic influence 2; C2 + common environmental influence 2; E2 + unique environmental influence 2; a + additive genetic path coefficient; c=common environmental path coefficient; e=unique environmental path coefficient. Circles represent latent, unobserved variables; squares represent observed phenotypes; single-headed arrows represent influences of latent variables on observed variables; double-headed arrows represent (co)variances.
Independent Pathway Model
On the other hand, the independent pathway model hypothesizes that the variance and covariance between the variables is expected to result from one (or sometimes two) common factor(s) with the residual variance reflecting variable-specific genetic and environmental effects. This is the case, for example, with cognitive domains and latency of event-related potentials ( Hansell et al., 2005 ; Luciano et al., 2004 ). Both the common and independent pathway models are nested within the previously described Cholesky decomposition. The fit of these models may therefore be compared to the “saturated model” using a likelihood ratio test, which is asymptotically distributed as x 2 with the degrees of freedom equal to the difference in the number of estimated parameters between the nested and saturated models.
Cross-Sectional Cohort and Longitudinal Designs
Once the role of genetic factors in the variance of a particular trait has been established, an additional question that can be addressed is whether the magnitude of these genetic influences is stable over time. Instead of a costly and time-consuming longitudinal study (which is another possibility; see below), this can be investigated with a cohort design , in which genetic and environmental estimates are obtained from different cohorts. In such a design, subjects from different age cohorts are assessed on one or more phenotypes. For example, Lyons et al. (1998 ) used a cohort design to examine the diagnosis of early-and late-onset major depression in men. Early-onset (before 30 years of age) and late-onset (after 30 years of age) major depression were both significantly influenced by genetic factors (early-onset: h 2 = 0.47; late-onset: h 2 = 0.10) and unique environmental factors (early-onset: e 2 = 0.53; late-onset: e 2 = 0.90), but early-onset major depression (95% CI: 0.32, 0.61) was significantly more heritable than late-onset major depression (95% CI: 0.01, 0.29). However, determining whether the same genes are involved at different stages of life is not possible with a cohort design. In addition, phenotypic differences resulting from age are confounded with any other differences between the cohorts.
With longitudinal twin data it is possible to estimate to what extent the relative contributions of genetic and environmental factors to the observed phenotypic variance are stable over time, and to what extent these genetic and environmental contributions are specific to a certain time of life. One use of the Cholesky decomposition is in temporal contexts ( Loehlin, 1996 ). For example, phenotypes P1 to P3 might represent measurements of a trait at three successive times. In this case, A1 would represent genetic influences present at time 1, affecting the observed trait at time 1 and on subsequent occasions; A2 would represent additional genetic influences that have arisen by time 2 and whose effects are added to those of A1; and, finally, A3 represents additional genetic influences, affecting only the third measurement (P3). To illustrate, studies on the heritability of cognitive abilities have repeatedly shown an increase in genetic influences and a decrease in common environmental influences over the life span ( Ando, Ono, & Wright, 2001 ; Bartels, Rietveld, van Baal, & Boomsma, 2002 ; Boomsma & van Baal, 1998 ; Luciano et al., 2001 ; Petrill et al., 2004 ; Plomin, 1999 ; Posthuma, de Geus, &Boomsma, 2001 ).
Increasing heritability over the life span could result from genes that are activated or become more active later in life, or may result from a decrease in the influence of environmental factors, as a result of which the relative contribution of genetic influences increases. Although it is possible to use a standard Cholesky decomposition for the purposes of a longitudinal study (as mentioned above), various longitudinal models have been described, including the genetic simplex model ( Boomsma & Molenaar, 1987 ; Eaves, Long, & Heath, 1986 ) and latent growth curve models ( Baker, Reynolds, & Phelps, 1992 ; McArdle, 1986 ; Neale & McArdle, 2000 ).
The genetic simplex model is based on the frequent observation that correlations are highest among adjoining occasions and that they fall away systematically as the distance between time points increases. Such a pattern is called a simplex structure after Guttman (1955 ). The genetic simplex design allows for modeling of changes in latent true scores over time by fitting autoregressive or Markovian chains. In autoregression each latent true score is predicted to be causally related to the immediately preceding latent true score in a linear fashion (linear regression of latent factor on the previous latent factor), while allowing for genetic/environmental change or innovation that is uncorrelated with the previous latent factor at each consecutive time point. Using this design Gillespie et al. (2004 ) were able to show that although female neuroticism shows a degree of genetic continuity, there are also age-specific genetic effects (genetic innovation), which could be related to developmental or hormonal changes during puberty and psychosexual development.
Growth curve models can be applied to assess the heritability of rate of change (increase or decrease) in a trait (e.g., cognitive abilities, brain volumes) throughout development. Reynolds et al. (2005 ) applied the growth curve model to a measure of cognitive abilities in adulthood. They examined sources of variability for ability level (intercept) and rate of change (linear and quadratic effects) for verbal, fluid, memory, and perceptual speed abilities. With the exception of one verbal and two memory measures, estimated variance components indicated decreasing genetic and increasing non-shared environmental variation over age, providing support for theories of the increasing influence of the environment on cognitive abilities with age.
Causal Models
When two correlated traits have rather different modes of inheritance (e.g., family resemblance is determined largely by family background, C, for one trait and by genetic factors, A or D, for the other trait), cross-sectional family data will allow for testing of unidirectional causal hypotheses ("A and B are correlated because A causes B” versus “because B causes A"), through the pattern of cross-twin cross-trait correlations ( Gillespie & Martin, 2005 ; Heath et al., 1993 ). This model makes it possible to model specific environmental risk factors. For example, proposing a twin-family model that incorporates childhood parental loss as a specific environmental risk factor, Kendler et al. (1996 ) examined how much of the association between childhood parental loss (through separation) and alcoholism was causal (i.e., mediated by environmental factors) versus non-causal (mediated by genetic factors, with parental loss serving as an index of parental genetic susceptibility to alcoholism). Both the causal-environmental pathway and non-causal genetic paths were significant for alcoholism. However, the causal-environmental pathway consistently accounted for most of the association, suggesting childhood parental loss is a direct and significant environmental risk factor for the development of alcoholism in women. De Moor et al. (2008 ) tested the hypothesis that exercise reduces symptoms of anxiety and depression, and found that although regular exercise is associated with reduced anxious and depressive symptoms in the population, the association is not because of causal effects of exercise.
Latent Class Analysis
Latent class analysis can be used to investigate whether distinct classes of disease subtypes can be identified, which can be used to refine genetic analyses. Using this approach, Althoff et al. (2006 ) were able to identify inattentive, hyperactive, or combined subtypes for ADHD based on the Child Behavior Check List. Latent class analysis allows for modeling of etiological heterogeneity in disease subtypes; for example, it compares a model that allows for genetic heterogeneity that is expressed only in individuals exposed to a high-risk “predisposing” environment (i.e., differential sensitivity of latent classes to measured covariates) with a model that allows the environment to differentiate two forms of the disorder in individuals of high genetic risk (i.e., GxE interaction; Eaves et al., 1993 ).
The genetic models described above and the related matrix algebra have been explained in more detail elsewhere, such as in Neale and Maes’ Methodology for Genetic Studies of Twins and Families (2004) . This book is downloadable free of charge at http://ibgwww.colorado.edu/workshop2006/cdrom/HTML/BOOK.HTM .
Twin Studies and Beyond
Twin studies have shown that almost every trait is heritable to some extent. Although the behavior genetics approach allows for the determination of the ratio of genetic and environmental influences, neither the number of genetic loci influencing a trait, nor the direction of these genetic effects, nor the location, nor identity of the loci can be determined with this approach. Thus, the next interesting step in genetic research is to identify specific genetic variants underlying the trait. Identification of specific genetic variants influencing complex traits provides knowledge about underlying biological mechanisms and identified genetic variants could potentially be used as biomarkers for screening, prevention, and medical treatment.
Linkage and candidate gene association studies were the first to search for underlying genetic variants. Linkage studies test for coinheritance of genetic markers and traits within families and are used to localize regions of the genome where a locus is harbored that regulates the trait. Candidate gene association studies test for a correlation between a specific genetic marker and the trait of interest in population samples. The markers tested generally have a known function that is hypothesized to influence the trait. Linkage and candidate gene studies have identified numerous potential regions and genes underlying complex traits, but they have not always been consistently replicated ( Bosker et al., 2011 ; Verweij et al.,2012 ).
Recent technological advances have enabled genome-wide association studies (GWAS), where single-nucleotide polymorphisms (SNPs) across the entire genome are systematically tested for association with the trait of interest. Genome-wide association studies do not take prior knowledge of gene function into account, so the approach is hypothesis-free. For complex traits, the results of GWAS are mixed. Genome-wide association studies have been successful in identifying genetic variants of large effect for a number of relatively rare disease traits ( Burton et al., 2007 ; Visscher & Montgomery, 2009 ). There have also been some successes in identifying many genetic variants of small effect underlying complex traits (i.e., schizophrenia, autism, and smoking; Liu et al., 2010 ; The International Schizophrenia Consortium, 2009 ; Wang et al., 2009 ).
Other technological advances, such as next-generation sequencing, assessment of copy number variation (CNV) and methylation rates will provide new opportunities. These approaches are promising, but only the future can tell us whether these methods will enable us to better unravel the genetic etiology of complex traits. A more in-depth description of linkage and association studies and their methodological background can be found in Chapter 11 .
Twin studies have contributed greatly to our knowledge about biological pathways. Although application of the twin model has revealed that almost every conceivable trait is partly genetically influenced, understanding the source of variance does not offer any indication of the number or location of genes influencing the trait. Twin studies provide one method of investigating the ongoing nature-nurture debate and are a very important and necessary first step in genetic analyses. In addition, multivariate twin analyses remain an important way to examine the nature and magnitude of covariation between traits and across time. Technological advances in both computational and laboratory techniques have led to the integration of variance component analyses with genetic information derived from DNA. The finding that a significant proportion of the variance in the trait of interest can be explained by genetic effects allows researchers to justify requesting funds to attempt to locate the genetic loci influencing the trait, as will be discussed in Chapter 11 .
Author Note
Gabriëlla A. M. Blokland, Miriam A. Mosing, Karin J. H.Verweij, Sarah E. Medland-Genetic Epidemiology Laboratory, Queensland Institute of Medical Research, Brisbane, [email protected]
Althoff, R. R. , Copeland, W. E. , Stanger, C. , Derks, E. M. , Todd, R. D. , Neuman, R. J. , et al. ( 2006 ). The latent class structure of ADHD is stable across informants. Twin Res Hum Genet , 9(4), 507–522.
Google Scholar
Ando, J. , Ono, Y. , & Wright, M. J. ( 2001 ). Genetic structure of spatial and verbal working memory. Behav Genet , 31(6), 615–624.
Baergen, R. N. ( 2011 ). Chapter 9 Multiple gestation. In R. N. Baergen (Ed.), Manual of Pathology of the Human Placenta (2nd ed., pp. 124–125). New York, NY: Springer Science+Business Media.
Google Preview
Baker, L. A. , Reynolds, C. , & Phelps, E. ( 1992 ). Biometrical analysis of individual growth curves. Behav Genet , 22(2), 253–264.
Bartels, M. , Rietveld, M. J. , van Baal, G. C. , & Boomsma, D. I. ( 2002 ). Genetic and environmental influences on the development of intelligence. Behav Genet, 32 (4), 237–249.
Boker, S. , Neale, M. , Maes, H. , Wilde, M. , Spiegel, M. , Brick, T. , et al. ( 2011 ). OpenMx: An open source extended structural equation modeling framework. Psychometrika, 76(2), 306–317.
Boomsma, D. I. , de Geus, E. J. C. , van Baal, G. C. M. , & Koopmans, J. R. ( 1999 ). A religious upbringing reduces the influence of genetic factors on disinhibition: Evidence for interaction between genotype and environment on personality. Twin Res, 2(2), 115–125.
Boomsma, D. I. , & Molenaar, P. C. ( 1987 ). The genetic analysis of repeated measures. I. Simplex models. Behav Genet, 17(2), 111–123.
Boomsma, D. I. , & van Baal, G. C. M. ( 1998 ). Genetic influences on childhood IQ in 5-and 7-year-old Dutch twins. Dev Neuropsychol, 14, 115–126.
Borkenau, P. , Riemann, R. , Angleitner, A. , & Spinath, F. M. ( 2002 ). Similarity of childhood experiences and personality resemblance in monozygotic and dizygotic twins: A test of the equal environments assumption. Pers Individ Dif, 33(2), 261–269.
Bosker, F. J. , Hartman, C. A. , Nolte, I. M. , Prins, B. P. , Terpstra, P. , Posthuma, D. , et al. ( 2011 ). Poor replication of candidate genes for major depressive disorder using genome-wide association data. Mol Psychiatry, 16(5), 516–532.
Bruder, C. E. , Piotrowski, A. , Gijsbers, A. A. , Andersson, R. , Erickson, S. , Diaz de Stahl, T. , et al. ( 2008 ). Phenotypically concordant and discordant monozygotic twins display different DNA copy-number-variation profiles. Am J Hum Genet , 82(3), 763–771.
Burton, P. R. , Clayton, D. G. , Cardon, L. R. , Craddock, N. , Deloukas, P. , Duncanson, A. , et al. ( 2007 ). Genome-wide association study of 14,000 cases of seven common diseases and 3,000 shared controls. Nature, 447(7145), 661–678.
Carey, G. ( 1986 ). Sibling imitation and contrast effects. Behav Genet, 16(3), 319–341.
Cerda, M. , Sagdeo, A. , Johnson, J. , & Galea, S. ( 2010 ). Genetic and environmental influences on psychiatric comorbidity: A systematic review. J Affect Disord, 126(1–2), 14–38.
Cohen, D. J. , Dibble, E. , Grawe, J. M. , & Pollin, W. ( 1973 ). Separating identical from fraternal twins. Arch Gen Psychiatry, 29(4), 465–469.
Cronk, N. J. , Slutske, W. S. , Madden, P. A. , Bucholz, K. K. , Reich, W. , & Heath, A. C. ( 2002 ). Emotional and behavioral problems among female twins: An evaluation of the equal environments assumption. J Am Acad Child Adolesc Psychiatry, 41(7), 829–837.
de Moor, M. H. , Boomsma, D. I. , Stubbe, J. H. , Willemsen, G. , & de Geus, E. J. C. ( 2008 ). Testing causality in the association between regular exercise and symptoms of anxiety and depression. Arch Gen Psychiatry , 65(8), 897–905.
Derks, E. M. , Dolan, C. V. , Hudziak, J. J. , Neale, M. C. , & Boomsma, D. I. ( 2007 ). Assessment and etiology of attention deficit hyperactivity disorder and oppositional defiant disorder in boys and girls. Behav Genet, 37(4), 559–566.
Derom, R. , Bryan, E. , Derom, C. , Keith, L. , & Vlietinck, R. ( 2001 ). Twins, chorionicity and zygosity. Twin Res, 4(3), 134–136.
Distel, M. A. , Middeldorp, C. M. , Trull, T. J. , Derom, C. A. , Willemsen, G. , & Boomsma, D. I. ( 2011 ). Life events and borderline personality features: The influence of gene-environment interaction and gene-environment correlation. Psychol Med, 41(4), 849–860.
Eaves, L. J. ( 1969 ). Genetic analysis of continuous variation—a comparison of experimental designs applicable to human data. Br J Math Stat Psychol, 22, 131–147.
Eaves, L. J. , Last, K. , Martin, N. G. , & Jinks, J. L. ( 1977 ). Progressive approach to non-additivity and genotype-environmental covariance in analysis of human differences. Br J Math Stat Psychol, 30, 1–42.
Eaves, L. J. , Long, J. , & Heath, A. C. ( 1986 ). A theory of developmental change in quantitative phenotypes applied to cognitive development. Behav Genet, 16(1), 143–162.
Eaves, L. J. , Silberg, J. L. , Hewitt, J. K. , Rutter, M. , Meyer, J. M. , Neale, M. C. , et al. ( 1993 ). Analyzing twin resemblance in multisymptom data: Genetic applications ofa latent class model for symptoms of conduct disorder in juvenile boys. Behav Genet, 23(1), 5–19.
Evans, D. M. , Gillespie, N. A. , & Martin, N. G. ( 2002 ). Biometrical genetics. Biol Psychol, 61(1–2), 33–51.
Falconer, D. S. , & Mackay, T. F. C. ( 1996 ). Introduction to quantitative genetics (4th ed.). Harlow, Essex, UK: Longmans Green.
Fisher, R. A. ( 1918 ). The correlation between relatives on the supposition of Mendelian inheritance. Philos Trans R Soc Edinburgh, 52, 399–433.
Galton, F. ( 1889 ). Natural inheritance . London: Macmillan ( http://galton.org/books/natural-inheritance/pdf/galton-nat-inh-1up-clean.pdf ; Retrieved 5 June, 2012).
Gillespie, N. A. , Evans, D. E. , Wright, M. M. , & Martin, N. G. ( 2004 ). Genetic simplex modeling of Eysenck’s dimensions of personality in a sample of young Australian twins. Twin Res, 7(6), 637–648.
Gillespie, N. A. , & Martin, N. G. ( 2005 ). Direction of causation models. In B. S. Everitt & D. C. Howell (Eds.), Encyclopedia of statistics in behavioral science (Vol. 1, pp. 496–499). Chichester, UK: John Wiley & Sons, Ltd.
Guttman, L. A. ( 1955 ). A generalized simplex for factor analysis. Psychometrica, 20(3), 173–192.
Hansell, N. K. , Wright, M. J. , Luciano, M. , Geffen, G. M. , Geffen, L. B. , & Martin, N. G. ( 2005 ). Genetic covariation between event-related potential (ERP) and behavioral non-ERP measures of working-memory, processing speed, and IQ. Behav Genet , 35(6), 695–706.
Heath, A. C. , Jardine, R. , & Martin, N. G. ( 1989 ). Interactive effects of genotype and social environment on alcohol consumption in female twins. J Stud Alcohol , 50(1), 38–48.
Heath, A. C. , Kessler, R. C. , Neale, M. C. , Hewitt, J. K. , Eaves, L. J. , & Kendler, K. S. ( 1993 ). Testing hypotheses about direction of causation using cross-sectional family data. Behav Genet, 23(1), 29–50.
Hettema, J. M. , Neale, M. C. , & Kendler, K. S. ( 1995 ). Physical similarity and the equal-environment assumption in twin studies of psychiatric disorders. Behav Genet, 25(4), 327–335.
Hettema, J. M. , Neale, M. C. , & Kendler, K. S. ( 2001 ). A review and meta-analysis of the genetic epidemiology of anxiety disorders. Am J Psychiatry 158(10), 1568–1578.
Holzinger, K. ( 1929 ). The relative effect of nature and nurture influences on twin differences. J Educ Psych, 20, 241–248.
Jepsen, J. L. , & Michel, M. ( 2006 ). ADHD and the symptom dimensions inattention, impulsivity, and hyperactivity—;A review of aetiological twin studies from 1996 to 2004. Nord Psychol, 58, 108–135.
Jinks, J. L. , & Fulker, D. W. ( 1970 ). A comparison of the biometrical-genetical, MAVA and classical approaches to the analysis of human behavior. Psychol Bull, 73, 311–349.
Kasriel, J. , & Eaves, L. J. ( 1976 ). Zygosity of twins—Further evidence on agreement between diagnosis by blood-groups and written questionnaires. J Biosoc Sci, 8(3), 263–266.
Keller, M. C. , Medland, S. E. , Duncan, L. E. , Hatemi, P. K. , Neale, M. C. , Maes, H. H. , et al. ( 2009 ). Modeling extended twin family data I: description of the Cascade model. Twin Res Hum Genet, 12(1), 8–18.
Kendler, K. S. , & Gardner, C. O., Jr. ( 1998 ). Twin studies of adult psychiatric and substance dependence disorders: Are they biased by differences in the environmental experiences of monozygotic and dizygotic twins in childhood and adolescence? Psychol Med , 28(3), 625–633.
Kendler, K. S. , Gatz, M. , Gardner, C. O. , & Pedersen, N. L. ( 2006 a). Personality and major depression: A Swedish longitudinal, population-based twin study. Arch Gen Psychiatry , 63(10), 1113–1120.
Kendler, K. S. , Gatz, M. , Gardner, C. O. , & Pedersen, N. L. ( 2006 b). A Swedish national twin study of lifetime major depression. Am J Psychiatry , 163(1), 109–114.
Kendler, K. S. , Heath, A. C. , Martin, N. G. , & Eaves, L. J. ( 1987 ). Symptoms of anxiety and symptoms of depression. Same genes, different environments? Arch Gen Psychiatry, 44(5), 451–457.
Kendler, K. S. , Neale, M. C. , Kessler, R. C. , Heath, A. C. , & Eaves, L. J. ( 1993 ). Atest of the equal-environment assumption in twin studies of psychiatric illness. Behav Genet, 23(1), 21–27.
Kendler, K. S. , Neale, M. C. , Kessler, R. C. , Heath, A. C. , & Eaves, L. J. ( 1994 ). Parental treatment and the equal environment assumption in twin studies of psychiatric illness. Psychol Med, 24(3), 579–590.
Kendler, K. S. , Neale, M. C. , Prescott, C. A. , Kessler, R. C. , Heath, A. C. , Corey, L. A. , et al. ( 1996 ). Childhood parental loss and alcoholism in women: A causal analysis using a twin-family design. Psychol Med, 26 (1), 79–95.
Koziel, S. M. ( 1998 ). Effect of disparities in birth weight on differences in postnatal growth of monozygotic and dizygotic twins. Ann Hum Biol, 25(2), 159–168.
LaBuda, M. C. , Svikis, D. S. , & Pickens, R. W. ( 1997 ). Twin closeness and co-twin risk for substance use disorders: Assessing the impact of the equal environment assumption. PsychiatryRes, 70(3), 155–164.
Liu, J. Z. , Tozzi, F. , Waterworth, D. M. , Pillai, S. G. , Muglia, P. , Middleton, L. , et al. ( 2010 ). Meta-analysis and imputation refines the association of 15q25 with smoking quantity. Nat Genet, 42(5), 366–368.
Loehlin, J. C. ( 1996 ). The Cholesky approach: A cautionary note. Behavior Genetics, 26 (1), 65–69.
Loehlin, J. C. ( 2009 ). History of behavior genetics. In Y. K. Kim (Ed.), Handbook of behavior genetics (pp. 3–11). New York, NY: Springer.
Loehlin, J. C. , & Nichols, R. C. ( 1976 ). Heredity, environment, and personality: A study of 850 sets of twins. Austin, TX: University of Texas Press.
Luciano, M. , Wright, M. J. , Geffen, G. M. , Geffen, L. B. , Smith, G. A. , & Martin, N. G. ( 2004 ). A genetic investigation of the covariation among inspection time, choice reaction time, and IQ subtest scores. Behav Genet, 34(1), 41–50.
Luciano, M. , Wright, M. J. , Smith, G. A. , Geffen, G. M. , Geffen, L. B. , & Martin, N. G. ( 2001 ). Genetic covariance among measures of information processing speed, working memory, and IQ. Behav Genet, 31(6), 581–592.
Lyons, M. J. , Eisen, S. A. , Goldberg, J. , True, W. , Lin, N. , Meyer, J. M. , et al. ( 1998 ). Aregistry-based twin study of depression in men. Arch Gen Psychiatry, 55(5), 468–472.
Lytton, H. , Martin, N. G. , & Eaves, L. J. ( 1977 ). Environmental and genetical causes of variation in ethological aspects of behavior in two-year-old boys. Soc Biol, 24(3), 200–211.
Maes, H. H. , Neale, M. C. , Kendler, K. S. , Hewitt, J. K. , Silberg, J. L. , Foley, D. L. , et al. ( 1998 ). Assortative mating for major psychiatric diagnoses in two population-based samples. Psychol Med, 28(6), 1389–1401.
Maes, H. H. , Neale, M. C. , Medland, S. E. , Keller, M. C. , Martin, N. G. , Heath, A. C. , et al. ( 2009 ). Flexible Mx specification of various extended twin kinship designs. Twin Res Hum Genet , 12(1), 26–34.
Martin, N. G. , & Eaves, L. J. ( 1977 ). The genetical analysis of covariance structure. Heredity, 38(1), 79–95.
Martin, N. G. , Eaves, L. J. , Heath, A. C. , Jardine, R. , Feingold, L. M. , & Eysenck, H. J. ( 1986 ). Transmission of social attitudes. Proc Natl Acad Sci USA, 83(12), 4364–4368.
Matheny, A. P., Jr. , Wilson, R. S. , & Dolan, A. B. ( 1976 ). Relations between twins’ similarity of appearance and behavioral similarity: Testing an assumption. Behav Genet, 6 (3), 343–351.
McArdle, J. J. ( 1986 ). Latent variable growth within behavior genetic models. Behav Genet, 16 (1), 163–200.
Medland, S. E. , & Hatemi, P. K. ( 2009 ). Political science, biometric theory, and twin studies: A methodological introduction. Polit Anal, 17(2), 191–214.
Mehta, P. D. , & Neale, M. C. x ( 2005 ). People are variables too: Multilevel structural equations modeling. Psychol Meth, 10(3), 259–284.
Mosing, M. A. , Zietsch, B. P. , Shekar, S. N. , Wright, M. J. , & Martin, N. G. ( 2009 ). Genetic and environmental influences on optimism and its relationship to mental and self-rated health: A study of aging twins. Behav Genet , 39(6), 597–604.
Muthen, L. K. , & Muthen, B. O. ( 1998 –2010). Mplus User’s Guide (6th ed.). Los Angeles, CA: Muthen & Muthen.
Nance, W. E. ,& Neale, M. C. ( 1989 ). Partitioned twin analysis: A power study. Behav Genet, 19(1), 143–150.
Neale, M. C. , Boker, S. M. , Xie, G. , & Maes, H. H. ( 2002 ). Mx: Statistical modeling (6th ed.). Richmond, VA 23298: Department of Psychiatry, University of Virginia, VCU Box 900126.
Neale, M. C. , & Maes, H. H. M. ( 2004 ). Methodology for genetic studies of twins and families . Dordrecht, The Netherlands: Kluwer Academic Publishers B.V.
Neale, M. C. , & McArdle, J. J. ( 2000 ). Structured latent growth curves for twin data. Twin Res , 3(3), 165–177.
Petrill, S. A. , Lipton, P. A. , Hewitt, J. K. , Plomin, R. , Cherny, S. S. , Corley, R. , et al. ( 2004 ). Genetic and environmental contributions to general cognitive ability through the first 16 years of life. Dev Psychol , 40(5), 805–812.
Plomin, R. ( 1999 ). Genetics and general cognitive ability. Nature, 402(6761 Suppl), C25–C29.
Plomin, R. , DeFries, J. C. , & Loehlin, J. C. ( 1977 ). Genotype-environment interaction and correlation in the analysis of human behavior. Psychol Bull, 84(2), 309–322.
Plomin, R. , DeFries, J. C. , McClearn, G. E. , & McGuffin, P. ( 2001 ). Behavioral genetics (4th ed.). New York, NY: Worth Publishers.
Plomin, R. , Willerman, L. , & Loehlin, J. C. ( 1976 ). Resemblance in appearance and the equal environments assumption in twin studies of personality traits. Behav Genet, 6(1), 43–52.
Posthuma, D. , Beem, A. L. , de Geus, E. J. C. , van Baal, G. C. , von Hjelmborg, J. B. , Iachine, I. , et al. ( 2003 ). Theory and practice in quantitative genetics. Twin Res, 6(5), 361–376.
Posthuma, D. , de Geus, E. J. C. , & Boomsma, D. I. ( 2001 ). Perceptual speed and IQare associated through common genetic factors. Behav Genet, 31(6), 593–602.
Purcell, S. ( 2002 ). Variance components models for gene-environment interaction in twin analysis. Twin Res , 5(6), 554–571.
Raykov, T. , Marcoulides, G. A. , & Boyd, J. ( 2003 ). Using structural equation modeling programs to perform matrix manipulations and data simulation. Struct Equ Modeling, 10(2), 312–322.
Reynolds, C. A. , Finkel, D. , McArdle, J. J. , Gatz, M. , Berg, S. , & Pedersen, N. L. ( 2005 ). Quantitative genetic analysis of latent growth curve models of cognitive abilities in adulthood. Dev Psychol, 41(1), 3–16.
Scarr, S. ( 1982 ). Environmental bias in twin studies. Biodemography Soc Biol, 29(3), 221–229.
Scarr, S. , & Carter-Saltzman, L. ( 1979 ). Twin method: Defense ofa critical assumption. Behav Genet, 9(6), 527–542.
Silventoinen, K. , Kaprio, J. , Lahelma, E. , Viken, R. J. , & Rose, R. J. ( 2001 ). Sex differences in genetic and environmental factors contributing to body-height. Twin Res, 4(1), 25–29.
Skytthe, A. , Kyvik, K. , Bathum, L. , Holm, N. , Vaupel, J. W. , & Christensen, K. ( 2006 ). The Danish Twin Registry in the new millennium. Twin Res Hum Genet, 9(6), 763–771.
Sullivan, P. F. , Kendler, K. S. , & Neale, M. C. ( 2003 ). Schizophrenia as a complex trait: Evidence from a meta-analysis of twin studies. Arch Gen Psychiatry, 60(12), 1187–1192.
Sullivan, P. F. , Neale, M. C. , & Kendler, K. S. ( 2000 ). Genetic epidemiology of major depression: Review and meta-analysis. Am J Psychiatry, 157(10), 1552–1562.
The International Schizophrenia Consortium ( 2009 ). Common polygenic variation contributes to risk of schizophrenia and bipolar disorder. Nature, 460(7256), 748–752.
van Grootheest, D. S. , Boomsma, D. I. , Hettema, J. M. , & Kendler, K. S. ( 2008 ). Heritability of obsessive-compulsive symptom dimensions. Am J Med Genet B Neuropsychiatr Genet, 147B(4), 473–478.
Verweij, K. J. H. , Zietsch, B. P. , Liu, J. Z. , Medland, S. E. , Lynskey, M. T. , Madden, P. A. F. , et al. ( 2012 ). No replication of candidate genes for cannabis use using a large sample of Australian twin families. Addict Biol, 17(3), 687–690.
Visscher, P. M. , Medland, S. E. , Ferreira, M. A. , Morley, K. I. , Zhu, G. , Cornes, B. K. , et al. ( 2006 ). Assumption-free estimation of heritability from genome-wide identity-by-descent sharing between full siblings. PLoS Genet, 2(3), e41.
Visscher, P. M. , & Montgomery, G. W. ( 2009 ). Genome-wide association studies and human disease: From trickle to flood. JAMA, 302(18), 2028–2029.
Wang, K. , Zhang, H. T. , Ma, D. Q. , Bucan, M. , Glessner, J. T. , Abrahams, B. S. , et al. ( 2009 ). Common genetic variants on 5p14.1 associate with autism spectrum disorders. Nature, 59(7246), 528–533.
Wright, S. ( 1921 ). Correlation and causation. J Agric Res, 20, 557–585.
Xian, H. , Scherrer, J. F. , Eisen, S. A. , True, W. R. , Heath, A. C. , Goldberg, J. , et al. ( 2000 ). Self-reported zygosity and the equal-environments assumption for psychiatric disorders in the Vietnam Era Twin Registry. Behav Genet, 30(4), 303–310.
- About Oxford Academic
- Publish journals with us
- University press partners
- What we publish
- New features
- Open access
- Institutional account management
- Rights and permissions
- Get help with access
- Accessibility
- Advertising
- Media enquiries
- Oxford University Press
- Oxford Languages
- University of Oxford
Oxford University Press is a department of the University of Oxford. It furthers the University's objective of excellence in research, scholarship, and education by publishing worldwide
- Copyright © 2024 Oxford University Press
- Cookie settings
- Cookie policy
- Privacy policy
- Legal notice
This Feature Is Available To Subscribers Only
Sign In or Create an Account
This PDF is available to Subscribers Only
For full access to this pdf, sign in to an existing account, or purchase an annual subscription.
Behavioral Genetics, Population Genetics, and Genetic Essentialism
A Survey Experiment
- SI: genetics and identity
- Published: 04 November 2020
- Volume 29 , pages 1595–1619, ( 2020 )
Cite this article
- Alexandre Morin-Chassé ORCID: orcid.org/0000-0003-3519-870X 1
1143 Accesses
5 Citations
2 Altmetric
Explore all metrics
The paper presents an experimental study that examines the conditions required for news about behavioral genetics to activate genetic essentialism beliefs. Nine hundred sixty-five adults living in the USA were randomly assigned to read either a control news article or one of the three versions of a news story about behavioral genetics. The cautious version presents a general introduction to behavioral genetics and examples while also discrediting the genetic determinist myth and clarifying that this field is not interested in studying differences between populations. Another version was identical to the cautious version, except that it mentioned high heritability estimates as supporting evidence. Finally, a third version included claims supporting Nicolas Wade’s ( 2014 ) main thesis, which argued that societies develop different institutions partly because of their population’s behavioral genetic predispositions. Compared to participants in the control group, those exposed to the high heritability version and the Wade’s thesis version reported higher scores on a scale measuring belief in genetics determinism. The results revealed no overall effect for the cautious version, but an exploratory interaction model indicates that reactions to this version vary depending on educational attainment. Implications and limitations are discussed.
This is a preview of subscription content, log in via an institution to check access.
Access this article
Price includes VAT (Russian Federation)
Instant access to the full article PDF.
Rent this article via DeepDyve
Institutional subscriptions
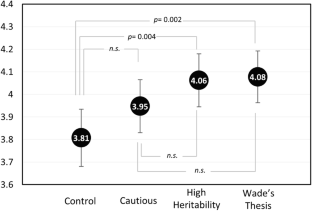
Similar content being viewed by others
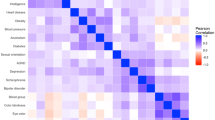
Free Will, Determinism, and Intuitive Judgments About the Heritability of Behavior
Emily A. Willoughby, Alan C. Love, … James J. Lee
Causal Reasoning About Human Behavior Genetics: Synthesis and Future Directions
Kate E. Lynch, James S. Morandini, … Paul E. Griffiths
Genes, Culture and Conservatism-A Psychometric-Genetic Approach
Inga Schwabe, Wilfried Jonker & Stéphanie M. van den Berg
Full texts in Electronic Supplementary Material.
Heritability estimates of similar sizes were found in Fowler et al. ( 2008 ), a study that received extensive media coverage.
Our study used the MTurk platform to recruit participants. This tool is often used to conduct psychology experiments, and has been validated elsewhere (Behrend et al. 2011 ; Berinsky et al. 2012 ; Casler et al. 2013 ; Johnson and Borden 2012 ).
Unfortunately, it is impossible to conduct a multiple imputation model for these missing observations because those participants abandoned the study at the beginning of the questionnaire, when they were exposed to the reading task. As such, they left the survey before answering any question item. In the Electronic Supplementary Material, Table A1 shows that there is no significant difference in drop-out rates across experimental groups.
Agence France Presse. (2018). Thé ou café? Les goûts déterminés par la génétique, selon une étude australienne. Journal de Montréal . Retrieved from https://www.journaldemontreal.com/2018/11/15/the-ou-cafe-les-gouts-determines-par-la-genetique-selon-une-etude-australienne . Accessed November 28, 2019.
Aivelo, T. J. E., & Uitto, A. E. (2015). Genetic determinism in the Finnish upper secondary school biology textbooks. NorDiNa: Nordic Studies in Science Education, 2 (15), 139–152. https://doi.org/10.5617/nordina.2042 .
Article Google Scholar
Albuquerque, P. M., de Almeida, A. M. R., & El-Hani, N. C. (2008). Gene concepts in higher education cell and molecular biology textbooks. Science Education International, 19 (2), 219–234.
Google Scholar
Andreychik, R., & Gill, M. J. (2015). Do natural kind beliefs about social groups contribute to prejudice? Distinguishing bio-somatic essentialism from bio-behavioral essentialism, and both of these from entitativity. Group Processes & Intergroup Relations, 18 (4), 454–474. https://doi.org/10.1177/1368430214550341 .
Behrend, T. S., Sharek, D. J., Meade, A. W., & Wiebe, E. N. (2011). The viability of crowdsourcing for survey research. Behavior Research Methods, 43 (3), 800–813. https://doi.org/10.3758/s13428-011-0081-0 .
Berinsky, A. J., Gregory, A. H., & Lenz, G. S. (2012). Evaluating online labor markets for experimental research: Amazon.com’s Mechanical Turk. Political Analysis, 20 (3), 351–368. https://doi.org/10.1093/pan/mpr057 .
Bloom, M. V., Cutter, M. A., Davidson, R., Dougherty, M. J., Drexler, E., Gelernter, J., et al. (2000). Genes, environment, and human behavior . Colorado Springs: Biological Sciences Curriculum Study.
Brescoll, V., & LaFrance, M. (2004). The correlates and consequences of newspaper reports of research on sex differences. Psychological Science, 15 (8), 515–520. https://doi.org/10.1111/j.0956-7976.2004.00712.x .
Brescoll, V. L., Uhlmann, E. L., & Newman, G. E. (2013). The effects of system-justifying motives on endorsement of essentialist explanations for gender differences. Journal of Personality and Social Psychology, 105 (6), 891. https://doi.org/10.1037/a0034701 .
Bubela, T. M., & Caulfield, T. A. (2004). Do the print media ‘hype’ genetic research? A comparison of newspaper stories and peer-reviewed research papers. Canadian Medical Association Journal, 170 (9), 1399–1407. https://doi.org/10.1503/cmaj.1030762 .
Butovskaya, M., Vasilyev, V., Lazebny, O., et al. (2013). Aggression and polymorphisms in AR, DAT1, DRD2, and COMT genes in Datoga Pastoralists of Tanzania. Scientific Reports, 3 , 3048. https://doi.org/10.1038/srep03148 .
Camperio Ciani, A. S., Edelman, S., & Ebstein, R. P. (2013). The dopamine D4 receptor (DRD4) Exon 3 VNTR contributes to adaptive personality differences in an Italian small island population. European Journal of Personality, 27 (6), 593–604. https://doi.org/10.1002/per.1917 .
Caricati, L. (2007). Power of genetics: adaptation and validation of a scale for measuring belief in genetic determinism (BGD) with classical test analysis and Rasch analysis. TPM - Testing, Psychometrics, Methodology in Applied Psychology, 14 (2), 99–112. https://doi.org/10.4473/TPM.14.2.3 .
Carranante, T. (2014). Genetics are related to playing violent video games and watching violent T.V. Science World Report . Retrieved from https://www.scienceworldreport.com/articles/12997/20140219/genetics-related-playing-violent-video-games-watching-tv.htm . Accessed November 28, 2019.
Carver, R. B., Rødland, E. A., & Breivik, J. (2013). Quantitative frame analysis of how the gene concept is presented in tabloid and elite newspapers. Science Communication, 35 (4), 449–475. https://doi.org/10.1177/1075547012460525 .
Casler, K., Bickel, L., & Hackett, E. (2013). Separate but equal? A comparison of participants and data gathered via Amazon’s MTurk, social media, and face-to-face behavioral testing. Computers in Human Behavior, 29 (6), 2156–2160. https://doi.org/10.1016/j.chb.2013.05.009 .
Castillo, M. J. B. (2018). Racismo indeleble y textos escolares de ciencias naturales colombianos (2000-2010). Educación y Educadores, 21 (2), 285–303.
Čavojová, V., Šrol, J., & Jurkovič, M. (2020). Why should we try to think like scientists? Scientific reasoning and susceptibility to epistemically suspect beliefs and cognitive biases. Applied Cognitive Psychology, 34 (1), 85–95. https://doi.org/10.1002/acp.3595 .
Chapman, R., Likhanov, M., Selita, F., Zakharov, I., Smith-Woolley, E., & Kovas, Y. (2019). New literacy challenge for the twenty-first century: genetic knowledge is poor even among well educated. Journal of Community Genetics, 10 (1), 73–84. https://doi.org/10.1007/s12687-018-0363-7 .
Ching, B. H. H., & Xu, J. T. (2018). The effects of gender neuroessentialism on transprejudice: an experimental study. Sex Roles, 78 (3–4), 228–241. https://doi.org/10.1007/s11199-017-0786-3 .
Cho, H. H., Kahle, J. B., & Nordland, F. H. (1985). An investigation of high school biology textbooks as sources of misconceptions and difficulties in genetics and some suggestions for teaching genetics. Science Education, 69 (5), 707–719. https://doi.org/10.1002/sce.3730690512 .
Chong, D., & Druckman, J. N. (2007). Framing theory. Annual Review of Political Science, 10 , 103–126. https://doi.org/10.1146/annurev.polisci.10.072805.103054 .
CNN (2009) Blame genetics for bad driving, study finds. Retrieved from http://www.cnn.com/2009/TECH/science/10/29/bad.driver.gene/ . Accessed November 28, 2019.
Coleman, J. M., & Hong, Y. Y. (2008). Beyond nature and nurture: the influence of lay gender theories on self-stereotyping. Self and Identity, 7 (1), 34–53. https://doi.org/10.1080/15298860600980185 .
Condit, C. M. (2019). Laypeople are strategic essentialists, not genetic essentialists. Hastings Center Report, 49 , S27–S37. https://doi.org/10.1002/hast.1014 .
Condit, C. M., Ofulue, N., & Sheedy, K. M. (1998). Determinism and mass-media portrayals of genetics. The American Journal of Human Genetics, 62 (4), 979–984. https://doi.org/10.1086/301784 .
Conrad, P. (2001). Genetic optimism: framing genes and mental illness in the news. Culture, Medicine and Psychiatry, 25 (2), 225–247. https://doi.org/10.1023/A:1010690427114 .
Conrad, P. (2002). Genetics and behavior in the news: dilemmas of a rising paradigm. In J. S. Alper, C. Ard, A. Asch, & J. Beckwith (Eds.), The double-edged helix: social implications of genetics in a diverse society (pp. 58–79). Baltimore: John Hopkins University Press.
Crampton, P., & Parkin, C. (2007). Warrior genes and risk-taking science. The New Zealand Medical Journal, 120 (1250).
Dar-Nimrod, I., & Heine, S. J. (2006). Exposure to scientific theories affects women’s math performance. Science, 314 (5798), 435–435. https://doi.org/10.1126/science.1131100 .
Dar-Nimrod, I., & Heine, S. J. (2011). Genetic essentialism: on the deceptive determinism of DNA. Psychological Bulletin, 137 (5), 800–818. https://doi.org/10.1037/a0021860 .
De Neve, J. E., & Fowler, J. H. (2014). Credit card borrowing and the monoamine oxidase A (MAOA) gene. Journal of Economic Behavior & Organization, 107 ( Part B ), 428–439. https://doi.org/10.1016/j.jebo.2014.03.002 .
Ding, L., Wei, X., & Mollohan, K. (2016). Does higher education improve student scientific reasoning skills? International Journal of Science and Mathematics Education, 14 (4), 619–634. https://doi.org/10.1007/s10763-014-9597-y .
Domaradzki, J. (2016). Behavioral genetics in Polish print news media between 2000 and 2014. Psychiatria Polska, 50 (6), 1251–1271. https://doi.org/10.12740/PP/62690 .
Donovan, B. M. (2014). Playing with fire? The impact of the hidden curriculum in school genetics on essentialist conceptions of race. Journal of Research in Science Teaching, 51 (4), 462–496. https://doi.org/10.1002/tea.21138 .
Donovan, B. M. (2016). Framing the genetics curriculum for social justice: An experimental exploration of how the biology curriculum influences beliefs about racial difference. Science Education, 100 (3), 586–616. https://doi.org/10.1002/sce.21221 .
Donovan, B. M. (2017). Learned inequality: racial labels in the biology curriculum can affect the development of racial prejudice. Journal of Research in Science Teaching, 54 (3), 379–411. https://doi.org/10.1002/tea.21370 .
Donovan, B. M., et al. (2019a). Toward a more humane genetics education: learning about the social and quantitative complexities of human genetic variation research could reduce racial bias in adolescent and adult populations. Science Education, 103 (3), 529–560. https://doi.org/10.1002/sce.21506 .
Donovan, B. M., Stuhlsatz, M. A., Edelson, D. C., & Buck Bracey, Z. E. (2019b). Gendered genetics: How reading about the genetic basis of sex differences in biology textbooks could affect beliefs associated with science gender disparities. Science Education, 103 (4), 719–749. https://doi.org/10.1002/sce.21502 .
Döring, M. (2005). A sequence of ‘factishes’: the media-metaphorical knowledge dynamics structuring the German press coverage of the human genome. New Genetics and Society, 24 (3), 317–336. https://doi.org/10.1080/14636770500349726 .
Dos Santos, V. C., Joaquim, L. M., & El-Hani, C. N. (2012). Hybrid deterministic views about genes in biology textbooks: a key problem in genetics teaching. Science & Education, 21 (4), 543–578. https://doi.org/10.1007/s11191-011-9348-1 .
Dougherty, M. J. (2009). Closing the gap: inverting the genetics curriculum to ensure an informed public. The American Journal of Human Genetics, 85 (1), 6–12. https://doi.org/10.1016/j.ajhg.2009.05.010 .
Dougherty, M.J. & McInerney, J.D. (1998) Genes, environment, and human behavior . Presented at the DOE Human Genome Program Contractor-Grantee Workshop VII. Oakland, California.
Fasce, A., & Picó, A. (2019). Science as a vaccine. Science & Education, 28 (1–2), 109–125. https://doi.org/10.1007/s11191-018-00022-0 .
Fowler, J. H., Baker, L. A., & Dawes, C. T. (2008). Genetic variation in political participation. American Political Science Review, 102 (2), 233–248. https://doi.org/10.1017/S0003055408080209 .
Fujimura, J. H., Duster, T., & Rajagopalan, R. (2008). Introduction: race, genetics, and disease: questions of evidence, matters of consequence. Social Studies of Science, 38 (5), 643–656. https://doi.org/10.1177/0306312708091926 .
Furr, L. A., & Kelly, S. E. (1999). The Genetic Knowledge Index: developing a standard measure of genetic knowledge. Genetic Testing, 3 (2), 193–199. https://doi.org/10.1089/gte.1999.3.193 .
Gelman, S. A., & Ware, E. A. (2012). Conceptual development: the case of essentialism. In Margolis et al. (Eds.), The Oxford handbook of philosophy of cognitive science . Oxford: Oxford University Press. https://doi.org/10.1093/oxfordhb/9780195309799.013.0019 .
Chapter Google Scholar
Gericke, N. M., Hagberg, M., dos Santos, V. C., Joaquim, L. M., & El-Hani, C. N. (2014). Conceptual variation or incoherence? Textbook discourse on genes in six countries. Science & Education, 23 (2), 381–416. https://doi.org/10.1007/s11191-012-9499-8 .
Gericke, N., Carver, R., Castéra, J., Evangelista, N. A. M., Marre, C. C., & El-Hani, C. N. (2017). Exploring relationships among belief in genetic determinism, genetics knowledge, and social factors. Science & Education, 26 (10), 1223–1259. https://doi.org/10.1007/s11191-017-9950-y .
Goltz, H. H., Bergman, M., & Goodson, P. (2016). Explanatory models of genetics and genetic risk among a selected group of students. Frontiers in Public Health, 4 , 111. https://doi.org/10.3389/fpubh.2016.00111 .
Görke, A., & Ruhrmann, G. (2003). Public communication between facts and fictions: on the construction of genetic risk. Public Understanding of Science, 12 (3), 229–241. https://doi.org/10.1177/0963662503123002 .
Hatemi, P. K., & McDermott, R. (2012). The genetics of politics: discovery, challenges, and progress. Trends in Genetics, 28 (10), 525–533. https://doi.org/10.1016/j.tig.2012.07.004 .
Heine, S. J., Dar-Nimrod, I., Cheung, B. Y., & Proulx, T. (2017). Essentially biased: why people are fatalistic about genes. In J. M. Olsen (Ed.), Advances in experimental social psychology (Vol. 55) (pp. 137–192). Cambridge: Academic Press. https://doi.org/10.1016/bs.aesp.2016.10.003 .
Heine, S. J., Cheung, B. Y., & Schmalor, A. (2019). Making sense of genetics: the problem of essentialism. Hastings Center Report, 49 , S19–S26. https://doi.org/10.1002/hast.1013 .
Hjörleifsson, S., Árnason, V., & Schei, E. (2008). Decoding the genetics debate: hype and hope in Icelandic news media in 2000 and 2004. New Genetics and Society, 27 (4), 377–394. https://doi.org/10.1080/14636770802485467 .
Hubbard, A. R. (2017). Testing common misconceptions about the nature of human racial variation. The American Biology Teacher, 79 (7), 538–543. https://doi.org/10.1525/abt.2017.79.7.538 .
Ishiyama, I., et al. (2008). Relationship between public attitudes toward genomic studies related to medicine and their level of genomic literacy in Japan. American Journal of Medical Genetics Part A, 146 (13), 1696–1706. https://doi.org/10.1002/ajmg.a.32322 .
Jamieson, A., & Radick, G. (2017). Genetic determinism in the genetics curriculum. Science & Education, 26 (10), 1261–1290. https://doi.org/10.1007/s11191-017-9900-8 .
Johnson, D. R., & Borden, L. A. (2012). Participants at your fingertips: using Amazon’s Mechanical Turk to increase student–faculty collaborative research. Teaching of Psychology, 39 (4), 245–251. https://doi.org/10.1177/0098628312456615 .
Johnson, J. D., Andrews, J. E., & Allard, S. (2001). A model for understanding and affecting cancer genetics information seeking. Library & Information Science Research, 23 (4), 335–349. https://doi.org/10.1016/S0740-8188(01)00094-9 .
Joslyn, M. R., & Haider-Markel, D. P. (2016). Genetic attributions, immutability, and stereotypical judgments: an analysis of homosexuality. Social Science Quarterly, 97 (2), 376–390. https://doi.org/10.1111/ssqu.12263 .
Kahan, D. M. (2017). ‘Ordinary science intelligence’: a science-comprehension measure for study of risk and science communication, with notes on evolution and climate change. Journal of Risk Research, 20 (8), 995–1016. https://doi.org/10.1080/13669877.2016.1148067 .
Keller, J. (2005). In genes we trust: the biological component of psychological essentialism and its relationship to mechanisms of motivated social cognition. Journal of Personality and Social Psychology, 88 (4), 686–702. https://doi.org/10.1037/0022-3514.88.4.686 .
Lea, R., & Chambers, G. (2007). Monoamine oxidase, addiction, and the “warrior” gene hypothesis. The New Zealand Medical Journal, 120 (1250), U2441.
Lynch, K. E., Morandini, J. S., Dar-Nimrod, I., & Griffiths, P. E. (2019). Causal reasoning about human behavior genetics: synthesis and future directions. Behavior Genetics, 49 , 221–234. https://doi.org/10.1007/s10519-018-9909-z .
Mahadeva, M. N., & Randerson, S. (1982). Mutation Mumbo Jumbo: are mutations really so rare and harmful? Or are textbooks misleading? The Science Teacher, 49 (3), 34–38.
Massing, N., & Schneider, S. L. (2017). Degrees of competency: the relationship between educational qualifications and adult skills across countries. Large-Scale Assessments in Education, 5 (1), 6. https://doi.org/10.1186/s40536-017-0041-y .
McHughen, S. A., Rodriguez, P. F., Kleim, J. A., Kleim, E. D., & Crespo, et al. (2010). BDNF val66met polymorphism influences motor system function in the human brain. Cerebral Cortex, 20 (5), 1254–1262. https://doi.org/10.1093/cercor/bhp189 .
McInerney, J. D. (1998). Genes, behavior & high school biology. The American Biology Teacher, 60 (3), 168–173. https://doi.org/10.2307/4450445 .
McPhetres, J., & Zuckerman, M. (2018). Religiosity predicts negative attitudes towards science and lower levels of science literacy. PLoS One, 13 (11), e0207125. https://doi.org/10.1371/journal.pone.0207125 .
Miller, J. D. (2012). The sources and impact of civic scientific literacy. In M. W. Bauer, R. Shukla, & N. Allum (Eds.), The culture of science: how does the public relate to science across the globe? NY: Routledge.
Molster, C., Charles, T., Samanek, A., & O’Leary, P. (2009). Australian study on public knowledge of human genetics and health. Public Health Genomics, 12 (2), 84–91. https://doi.org/10.1159/000164684 .
Morin-Chassé, A. (2014). Public (mis) understanding of news about behavioral genetics research: a survey experiment. BioScience, 64 (12), 1170–1177. https://doi.org/10.1093/biosci/biu168 .
Morin-Chassé, A. (2018). How messages about behavioral genetics research can impact on genetic attribution beliefs. F1000Research, 5 . https://doi.org/10.12688/f1000research.10032.2 .
Morin-Chassé, A., Suhay, E., & Jayaratne, T. E. (2017). Discord over DNA: ideological responses to scientific communication about genes and race. Journal of Race, Ethnicity and Politics, 2 (2), 260–299. https://doi.org/10.1017/rep.2017.17 .
Morning, A. (2008). Reconstructing race in science and society: biology textbooks, 1952–2002. American Journal of Sociology, 114 (S1), S106–S137. https://doi.org/10.1086/592206 .
Morosoli, J. J., Colodro-Conde, L., Barlow, F. K., & Medland, S. E. (2019). Public understanding of behavioral genetics: integrating heuristic thinking, motivated reasoning and planned social change theories for better communication strategies. Behavior Genetics, 49 (5), 469–477. https://doi.org/10.1007/s10519-019-09964-9 .
Mountcastle-Shah, E., Tambor, E., Bernhardt, B. A., Geller, G., & Karaliukas, et al. (2003). Assessing mass media reporting of disease-related genetic discoveries: development of an instrument and initial findings. Science Communication, 24 (4), 458–478. https://doi.org/10.1177/1075547003024004003 .
National Research Council. (2013). Monitoring progress toward successful K-12 STEM education: a nation advancing? National Academies Press.
Nelkin, D., & Lindee, S. (1995). The DNA mystique . London: WH Freeman & Company.
Nelson, T. E., & Oxley, Z. M. (1999). Issue framing effects on belief importance and opinion. The Journal of Politics, 61 (4), 1040–1067. https://doi.org/10.2307/2647553 .
Nelson, T. E., Clawson, R. A., & Oxley, Z. M. (1997). Media framing of a civil liberties conflict and its effect on tolerance. American Political Science Review, 91 (3), 567–583. https://doi.org/10.2307/2952075 .
Nikkelen, S. W., Vossen, H. G., Valkenburg, P. M., Velders, F. P., Windhorst, D. A., et al. (2014). Media violence and children’s ADHD-related behaviors: a genetic susceptibility perspective. Journal of Communication, 64 (1), 42–60. https://doi.org/10.1111/jcom.12073 .
Noskova, T., Pivac, N., Nedic, G., Kazantseva, A., Gaysina, D., Faskhutdinova, G., et al. (2008). Ethnic differences in the serotonin transporter polymorphism (5-HTTLPR) in several European populations. Progress in Neuro-Psychopharmacology and Biological Psychiatry, 32 (7), 1735–1739. https://doi.org/10.1016/j.pnpbp.2008.07.012 .
Ong, J. S., Hwang, D. L. D., Zhong, V. W., An, J., Gharahkhani, P., & Breslin, et al. (2018). Understanding the role of bitter taste perception in coffee, tea and alcohol consumption through Mendelian randomization. Scientific Reports, 8 , 6414. https://doi.org/10.1038/s41598-018-34713-z .
Parrott, R. L., Silk, K. J., & Condit, C. (2003). Diversity in lay perceptions of the sources of human traits: genes, environments, and personal behaviors. Social Science & Medicine, 56 (5), 1099–1109. https://doi.org/10.1016/S0277-9536(02)00106-5 .
Phelan, J. C. (2005). Geneticization of deviant behavior and consequences for stigma: the case of mental illness. Journal of Health and Social Behavior, 46 (4), 307–322. https://doi.org/10.1177/002214650504600401 .
Phelan, J. C., Link, B. G., & Feldman, N. M. (2013). The genomic revolution and beliefs about essential racial differences: a backdoor to eugenics? American Sociological Review, 78 (2), 167–191. https://doi.org/10.1177/0003122413476034 .
Plomin, R., & Colledge, E. (2001). Genetics and psychology: beyond heritability. European Psychologist, 6 (4), 229–240. https://doi.org/10.1027/1016-9040.6.4.229 .
Polderman, T. J., Benyamin, B., De Leeuw, C. A., Sullivan, P. F., Van Bochoven, A., Visscher, P. M., & Posthuma, D. (2015). Meta-analysis of the heritability of human traits based on fifty years of twin studies. Nature Genetics, 47 (7), 702–709. https://doi.org/10.1038/ng.3285 .
Puig, B., & Aleixandre, M. P. J. (2015). El modelo de expresión de los genes y el determinismo en los libros de texto de ciencias. Revista Eureka Sobre Enseñanza Y Divulgación De Las Ciencias, 12 (1), 55–65.
Rhee, S. H., & Waldman, I. D. (2002). Genetic and environmental influences on antisocial behavior: a meta-analysis of twin and adoption studies. Psychological Bulletin, 128 (3), 490–529. https://doi.org/10.1037/0033-2909.128.3.490 .
Ross, V. (2010). Born into debt: gene linked to credit-card balances, Scientific American Mind, 21(3). Retrieved from www.scientificamerican.com/article/born-into-debt . Accessed November 28, 2019.
Roth, W. D., Yaylacı, Ş., Jaffe, K., & Richardson, L. (2020). Do genetic ancestry tests increase racial essentialism? Findings from a randomized controlled trial. PLoS One, 15 (1), e0227399. https://doi.org/10.1371/journal.pone.0227399 .
Shapiro, D. N., Chandler, J., & Mueller, P. A. (2013). Using Mechanical Turk to study clinical populations. Clinical Psychological Science, 1 (2), 213–220. https://doi.org/10.1177/2167702612469015 .
Shostak, S., Freese, J., Link, B. G., & Phelan, J. C. (2009). The politics of the gene: Social status and beliefs about genetics for individual outcomes. Social Psychology Quarterly, 72 (1), 77–93. https://doi.org/10.1177/019027250907200107 .
Smerecnik, C. M. (2010). Lay responses to health messages about the genetic risk factors for salt sensitivity: do mass media genetic health messages result in genetic determinism? Psychology, Health & Medicine, 15 (4), 386–393. https://doi.org/10.1080/13548501003758728 .
Stern, F., & Kampourakis, K. (2017). Teaching for genetics literacy in the post-genomic era. Studies in Science Education, 53 (2), 193–225. https://doi.org/10.1080/03057267.2017.1392731 .
Suhay, E. (2017). Genetic essentialism. In F. Moghaddam (Ed.), The SAGE encyclopedia of political behavior . Thousand Oaks: Sage.
Suhay, E., & Jayaratne, T. E. (2013). Does biology justify ideology? The politics of genetic attribution. Public Opinion Quarterly, 77 (2), 497–521. https://doi.org/10.1093/poq/nfs049 .
Tabb, K., Lebowitz, M. S., & Appelbaum, P. S. (2019). Behavioral genetics and attributions of moral responsibility. Behavior Genetics, 49 (2), 128–135. https://doi.org/10.1007/s10519-018-9916-0 .
Tawa, J. (2016). Belief in race as biological: early life influences, intergroup outcomes, and the process of “unlearning”. Race and Social Problems, 8 (3), 244–255. https://doi.org/10.1007/s12552-016-9176-7 .
Thörne, K. (2018). Linguistic challenges in science education: a classroom study of teachers’ and students’ use of central concepts in genetics (PhD dissertation). Karlstad University. from http://urn.kb.se/resolve?urn=urn:nbn:se:kau:diva-67141 . Accessed 23 Oct 2020.
Wade, N. (2014). A troublesome inheritance: genes, race and human history . New York: Penguin Press.
Wensley, D., & King, M. (2008). Scientific responsibility for the dissemination and interpretation of genetic research: lessons from the ‘warrior gene’ controversy. Journal of Medical Ethics, 34 (6). https://doi.org/10.1136/jme.2006.019596 .
Williams, M. J., & Eberhardt, J. L. (2008). Biological conceptions of race and the motivation to cross racial boundaries. Journal of Personality and Social Psychology, 94 (6). https://doi.org/10.1037/0022-3514.94.6.1033 .
Wolpe, P. R. (1997). If I am only my genes, what am I? Genetic essentialism and a Jewish response. Kennedy Institute of Ethics Journal, 7 (3), 213–230. https://doi.org/10.1353/ken.1997.0027 .
Download references
Acknowledgements
Thanks to André Blais, James H. Fowler and Peter Loewen for their support at different stages of this project. Thanks also to Aaron Panofsky, Elizabeth Suhay, Maya Sabatello and Paul S. Appelbaum for their insightful feedback.
This study received funding from the Department of Political Science at the University of California, San Diego.The author benefited from a PhD scholarship from the Fonds Québécois de Recherche – Société et Culture at the time this research was conducted.
Author information
Authors and affiliations.
Performance and Statistics Unit, Montreal West Island Integrated University Health and Social Services Centre, Montreal, Canada
Alexandre Morin-Chassé
You can also search for this author in PubMed Google Scholar
Corresponding author
Correspondence to Alexandre Morin-Chassé .
Ethics declarations
Conflict of interest.
The author declares no conflict of interest.
Ethics Approval
The experiment presented in this study was evaluated by and received approval from the University of Montreal IRB.
Additional information
Publisher’s note.
Springer Nature remains neutral with regard to jurisdictional claims in published maps and institutional affiliations.
Previous versions of this manuscript were presented in 2015 at the Center for Research on the Ethical, Legal, and Social Implications of Psychiatric, Neurologic, and Behavioral Genetics (Columbia University), and at the 2015 Annual Conference of the International Society for the History, Philosophy, and Social Studies of Biology (Montreal).
Electronic Supplementary Material
(DOCX 21 kb)
(DOCX 331 kb)
(DOCX 14 kb)
(DOCX 15 kb)
Rights and permissions
Reprints and permissions
About this article
Morin-Chassé, A. Behavioral Genetics, Population Genetics, and Genetic Essentialism. Sci & Educ 29 , 1595–1619 (2020). https://doi.org/10.1007/s11191-020-00166-y
Download citation
Accepted : 06 October 2020
Published : 04 November 2020
Issue Date : December 2020
DOI : https://doi.org/10.1007/s11191-020-00166-y
Share this article
Anyone you share the following link with will be able to read this content:
Sorry, a shareable link is not currently available for this article.
Provided by the Springer Nature SharedIt content-sharing initiative
- Find a journal
- Publish with us
- Track your research

An official website of the United States government
The .gov means it’s official. Federal government websites often end in .gov or .mil. Before sharing sensitive information, make sure you’re on a federal government site.
The site is secure. The https:// ensures that you are connecting to the official website and that any information you provide is encrypted and transmitted securely.
- Publications
- Account settings
Preview improvements coming to the PMC website in October 2024. Learn More or Try it out now .
- Advanced Search
- Journal List
- HHS Author Manuscripts

Evolutionary behavioral genetics
Brendan p. zietsch.
1 School of Psychology, University of Queensland, Brisbane, Queensland, Australia
2 Genetic Epidemiology Laboratory, QIMR Berghofer, Brisbane, Queensland, Australia
Teresa R de Candia
3 Department of Psychology and Neuroscience, University of Colorado Boulder, Boulder, Colorado, United States of America
4 Institute for Behavioral Genetics, University of Colorado Boulder, Boulder, Colorado, United States of America
Matthew C. Keller
Associated data.
We describe the scientific enterprise at the intersection of evolutionary psychology and behavioral genetics—a field that could be termed Evolutionary Behavioral Genetics —and how modern genetic data is revolutionizing our ability to test questions in this field. We first explain how genetically informative data and designs can be used to investigate questions about the evolution of human behavior, and describe some of the findings arising from these approaches. Second, we explain how evolutionary theory can be applied to the investigation of behavioral genetic variation. We give examples of how new data and methods provide insight into the genetic architecture of behavioral variation and what this tells us about the evolutionary processes that acted on the underlying causal genetic variants.
Although both evolutionary psychology and behavioral genetics arose in the 1970s as attempts to integrate the study of human behavior with other branches of biological science, the two fields have largely developed in isolation. Evolutionary psychology has primarily focused on using evolutionary theory to explain species- or sex-typical behavioral features—why people tend to find particular traits appealing in romantic partners or friends, for example. Behavioral genetics, on the other hand, has primarily focused on understanding proximate causes of variation among individuals—to what extent genetic and environmental influences are responsible for behavioral differences between individuals, and which specific genetic polymorphisms or environmental factors are responsible. The purpose of this manuscript is to describe the scientific enterprise at the intersection of evolutionary psychology and behavioral genetics—a field that could be termed Evolutionary Behavioral Genetics —and how modern genetic data is revolutionizing our ability to test questions in this field. We first describe how methods and designs developed in behavioral and statistical genetics can be profitably applied to evolutionary psychology and the study of human ‘universals.’ Second, we explain how evolutionary theory can be applied to the investigation of human behavioral genetic variation and give examples of the types of designs and research findings that provide evidence for competing evolutionary models.
Using behavioral genetic methods to test hypotheses in evolutionary psychology
Evolutionary psychologists have often viewed genetic variation as “noise in the system” and assumed that heritability in traits relevant to reproductive success would be close to zero [ 1 ]. However, genetic variation is ubiquitous in animals, even for traits under strong selection [ 2 ], and this is no different in humans [ 3 ]. Virtually no psychological traits that vary have a near-zero heritability—including traits that are likely to be related to ancestral fitness [ 3 – 6 ]. Because evolutionary hypotheses and alternative explanations often make predictions or assumptions about the genetic variation in and covariation between traits, analyses of genetic (co)variation can be extremely helpful in testing hypotheses about how human features evolved. We highlight below several areas in which behavioural genetic data and designs have helped in testing hypotheses in evolutionary psychology.
Genetic correlation between traits
In addition to demonstrating and quantifying heritability of individual traits, behavioural geneticists often examine whether the same genes influence different traits by modelling the genetic correlation between traits. For example, sexual selection is thought to have influenced the evolution of certain human features. Given heritable variation in traits and trait preferences, this hypothesis predicts a genetic correlation between preferences for a given feature and the expression of that feature itself [ 7 , 8 ]. This is because individuals with stronger-than-average preference for a certain trait will tend to choose a mate with above-average values of that trait, with the resulting offspring tending to inherit alleles predisposing to both higher-than-average trait and higher-than-average preference. This co-inheritance leads to linkage disequilibrium between alleles influencing the preferences and those influencing the trait, which manifests as a genetic correlation between the trait and the preference. Multivariate twin analyses have shown that genetic correlation between a trait and its preference applies to several traits of interest in humans (including height, hair colour, intelligence, and creativity) [ 9 ], consistent with an influence of sexual selection on these traits.
‘Good genes’ models of sexual selection also predict that traits that serve as good genes indicators will tend to be positively genetically intercorrelated because each trait is an imperfect index of the same underlying “mutation load” [ 10 ]. In other words, for traits to be accurate indicators of mutational loads, many genes must influence them, which causes overlaps in their genes (pleiotropic genes) and hence genetic correlations between them. However, genetic correlations between sexually selected traits can also arise via linkage disequilibrium due to cross-trait assortative mating (mates choosing simultaneously on a number of indicators, as described in previous section, above). The relative importance of these alternative explanations for genetic correlations can be quantified using an extended twin-family designs [ 11 – 13 ], which has indicated that both pleiotropy and cross-trait assortative mating are roughly equally important in causing the genetic correlation between height and intelligence [ 14 ], two traits that are potential good genes indicators. Additional traits need to be tested in a similar way to understand the generality of this conclusion.
Cross-sex genetic correlation
Evolutionary hypotheses about the origin of sexual dimorphism often make predictions about cross-sex genetic correlations– that is, the extent to which the same or different genes influence a trait in males and females. An example pertains to the evolutionary basis of facial sexual dimorphism. The predominant hypothesis in evolutionary psychology is that male facial masculinity is a good genes indicator such that women can increase the quality of their offspring by choosing a facially masculine mate [ 15 , 16 ]. However, genetic analyses suggest that the genes that make male faces masculine do not improve male attractiveness but do make female relatives’ faces more masculine and less attractive, casting doubt on the good genes theory of male facial masculinity [ 4 , 17 ].
Cross-population genetic correlation
New methods allow testing genetic correlations using samples of unrelated people with measured genotypes [ 18 ]. Importantly, this enables testing genetic correlations between traits that are measured in different individuals. How might this be used to inform evolutionary questions? Standard twin analyses have shown in a Swedish population that variation in fitness (both first and second generation reproductive success) is substantially heritable [ 19 ], but it is impossible with this type of analysis to determine to what extent the genes that affect fitness in Sweden are the same or different from those that affect fitness in small-scale, natural fertility traditional societies that are more similar to our ancestral circumstances. However, this could in principle be tested with large genotyped samples of Western and traditional societies, which would shed light on the genetic differences between modern and ancestral fitness.
Controlling genetic and familial confounds
Another function of genetically informative designs is to provide crucial controls for genetic and familial confounds in tests of evolutionary hypotheses. For example, it has been hypothesised that father absence causes early physical and behavioural sexual maturation (age-of-menarche, age at first intercourse) because of an evolved mechanism that strategically calibrates development to the riskiness of the environment [ 20 ]. However, Mendle et al. [ 21 , 22 ] showed that these effects were not present when familial (including genetic) confounds were controlled using the children-of-twins design: cousins discordant for father absence showed no differences in sexual maturation. This finding is inconsistent with the evolved mechanism, but consistent with genetic or environmental factors that both predispose fathers to leave the family unit and predispose daughters to early sexual maturation. This and many other evolutionary hypotheses involving the effects of childhood environmental factors (e.g. low socioeconomic status) on later behaviour (e.g. adult risk-taking [ 23 ]) continue to be tested without controlling for genetic and familial confounds, and their conclusions generally suffer from similar (often unacknowledged) alternative explanations.
Evolution of human behavioral genetic variation
In the previous section we described how behavioural genetics methods can inform evolutionary hypotheses about species- or sex-typical human behavioural features. However, the existence of underlying genetic variation itself also requires evolutionary explanation. In this section we focus on how to investigate the evolutionary bases of genetic variation in behaviour, and some of what we have learned thus far.
The observation of pervasive genetic variation in fitness related traits is at odds with the traditional interpretation of Fisher’s Fundamental Theorem [ 24 ]. Explaining the evolutionary basis of such widespread genetic trait variation has been a central question in biology for decades [ 25 ], but, in part due to the rapid advances in technology, this question has only recently drawn significant attention in psychology and psychiatry. As long understood, there are three basic evolutionary processes that can explain the existence of genetic variation in complex traits [reviewed in 26 ]. The first is mutation-selection balance: genetic variation is the consequence of a balance between deleterious mutations arising at many loci and their eventual removal by purifying selection. The second mechanism is neutral mutation-drift : genetic variation is the balance between mutations arising at many loci that have no (or nearly no) effect on net fitness, and their eventual (albeit typically much later) removal or fixation due to chance or “drift.” The final mechanism, balancing selection, is actually a group of processes, all of which involve genetic variation being actively maintained by selection because the relative fitness of alternative genetic variants depends on variable environmental or genetic contexts.
These three evolutionary processes make different predictions about the genetic architecture of traits—i.e. the number of causal variants (CVs—the genetic polymorphisms that cause trait differences), the distributions of their frequencies and effect sizes, and their interactions between and within loci. In the following sections, we briefly review some examples of what we have learned about the genetic architectures of human behavioral phenotypes, and describe what this evidence tells us about the evolutionary forces that acted on their CVs. We use schizophrenia as an example throughout because it is perhaps the most intensively studied behavioral trait in genetics, but the methods involved should apply equally to other traits as data continues to accumulate for them.
The direction of dominance of genetic causal variants (CVs)
Purifying selection is less efficient at eliminating recessive or partially recessive deleterious alleles compared to additive or dominant deleterious alleles, since the former are “hidden” from selection when heterozygous. As a result, deleterious alleles that have not (yet) been eliminated by purifying selection tend to be more recessive than would be expected due to chance. This phenomenon, where the deleterious alleles tend to be more recessive and the fittest alleles more dominant, is called directional dominance and can be used to infer selection [ 27 ]. For example, if CVs that decrease a trait tend to be more recessive than those that increase a trait, one can infer that trait-decreasing CVs were selected against on average over evolutionary time. Because inbreeding between close genetic relatives increases the likelihood that recessive CVs will be expressed in offspring, this phenomenon has long been studied by cataloguing the traits for which inbred individuals have higher or lower average trait values [ 28 ]. However, inbreeding studies using human pedigrees are difficult to conduct and suffer from alternative explanations, including the possibility that individuals who mate with close relatives may differ genetically or environmentally from other individuals and these differences may influence their offspring.
Recently, several studies [ 6 , 9 , 29 – 34 ] have used single nucleotide polymorphisms (SNPs) measured at hundreds of thousands of locations across the genome to detect very small individual differences in ‘distant’ inbreeding (arising from common ancestors who lived 10’s of generations ago) among samples unselected for inbreeding. This is done by measuring the genome-wide burden of runs of homozygosity [ 35 ]. Because variation in the overall burden of such runs of homozygosity is small in samples unselected for inbreeding, sample sizes typically need to be large (e.g., > 10K–20K) to reliably detect associations with traits [ 36 ]. Using a large ( n ~21K) schizophrenia case-control sample, we found that total burden of runs of homozygosity is reliably but weakly associated with schizophrenia [ 37 ]. This finding suggests that, on average , CVs that increase schizophrenia risk are more recessive than expected by chance and therefore are likely to have been selected against over evolutionary time.
The number, effect sizes, and frequencies of causal variants
The findings from large-scale linkage and genome-wide association studies on a variety of complex behavioral traits (personality, psychiatric disorders, cognitive abilities, etc.) tell a consistent story: complex traits are affected by a huge number of CVs (e.g., hundreds to thousands), each of which generally explains only a miniscule amount of the phenotypic variation. Thus, findings are turning out to be roughly consistent with the so-called “infinitesimal model” developed by Fisher nearly a hundred years ago [ 38 ].
Figure 1 (see also [ 39 , 40 ]) shows a strong inverse relationship between the effect sizes of all genetic variants reliably associated with schizophrenia to date and their frequencies (which includes the largest schizophrenia GWAS conducted to date, N ~80,000 [ 41 ]). The variance accounted for by a particular allele is proportionate to 2 p (1− p )ln( OR ) 2 , where p is the minor allele frequency and ln(OR) is the effect size (log odds ratio) of the risk allele. The dashed red line in Figure 1 plots the effect size/allele frequency combinations of hypothetical loci that would each explain 0.05% of the phenotypic variation. The close fit of this line with the observed associated variants suggests that each of the reliably associated schizophrenia risk variants accounts for around five hundredths of one percent of the variation in the trait; the many more variants that have yet to be detected probably each account for this amount of variation or less (region in grey).

The relationship between the effect sizes (natural log of odds ratios) and minor allele frequencies of all genetic variants (copy number variants [CNVs] or single-nucleotide polymorphisms [SNPs]) reliably associated with schizophrenia. The dotted red line defines a constant variance explained of 0.05% assuming a 1% prevalence of schizophrenia. The lack of data points between SNPs and CNVs occurs because no common CNVs are known to be associated with schizophrenia and because SNP panels do not measure variants with minor allele frequencies under 1%. Sequencing data may fill in this gap. There is adequate statistical power to detect only those variants with the largest effect sizes (near the dotted red line), although the entire shaded region is expected to be populated with schizophrenia risk alleles. The lack of variants in the unshaded region is consistent with a purifying selection model on schizophrenia risk alleles.
What does this tell us about the evolutionary forces acting on schizophrenia CVs? The inverse relationship between schizophrenia CVs’ effect sizes and frequencies, and the fact that no single variant explains much heritability, conform to expectations under mutation-selection balance, where purifying selection is removing deleterious mutations. Under purifying selection, deleterious CVs with large effects will be selected against the strongest and therefore be rare, whereas risk alleles with small effects may be nearly neutral and can drift to higher frequencies. In either case, because of this tradeoff between frequency and effect size, no single allele can account for much population variation. Such an inverse relationship between alleles’ effect sizes and frequencies is not expected under neutral mutation-drift or balancing selection.
The allelic spectrum of causal variants
The allelic spectrum of a trait refers to the distribution of a trait’s genetic variance accounted for by all the CVs in each allele frequency bin. Under a neutral-drift model, effect sizes should be uncorrelated with allele frequencies, and the allelic spectrum should be uniform, such that each CV frequency bin accounts for an equal proportion of variance [ 42 , 43 ]. In contrast, modeling suggests that balancing selection maintains variants at intermediate frequencies, so the allelic spectrum of CVs under balancing selection should be shifted toward minor alleles of higher frequencies [ 44 , 45 ]. Finally, under a mutation selection model, the allelic spectrum should be shifted toward minor alleles of lower frequencies as previously explained.
A recent and highly influential method gives accurate estimates of the additive genetic variation explained by all SNPs together even though the true effect at each specific SNP remains unknown [ 46 ]. Although SNPs themselves are probably often not the true CVs, SNPs tend to best predict nearby CVs that are similar in frequencies [ 47 ]. Because this method has been up to now used only on SNPs that exist on modern SNP panels, and because SNP panels have virtually no information on rare (minor allele frequencies < .01) SNPs, resulting estimates give an idea of the cumulative importance of additive common CVs but are blind to the importance of rare CVs.
By comparing additive genetic variance estimates from this method, which estimates only the effects of common CVs, to those from traditional family-based studies, which estimates the effects of both rare and common CVs, scientists have gained their first insights into the relative importance of common versus rare CVs. This method has been used on a large number of behavioral traits in the last several years, and between one-tenth to one-half of total additive genetic variation estimated from family-based studies appears to be due to the additive effects of (mostly common) CVs tagged by common SNPs [ 6 , 48 – 53 ]. While family-based estimates of additive genetic variation may be inflated [ 54 ], as long as they are roughly correct, these findings are consistent with much of the remainder of the additive genetic variation being due to rare CVs. If so, substantially more variation would be due to rare CVs than expected under the uniform distribution of CV allele frequencies predicted by neutral drift (i.e., 99% of additive genetic variance explained by CVs with risk allele frequency >.01) [ 42 ]. Nevertheless, a simple model of strong purifying selection on all CVs would predict that no CVs should be common; the evidence that common CVs do in fact influence schizophenia suggests that many schizophrenia CVs are under weak purifying selection or are drifting neutrally. This observation, and the potential for rare CVs to explain much of the remaining additive genetic variation not tagged by SNPs, is again potentially consistent with a model of purifying selection of varying strength: CVs of small effect are under weak to non-existent purifying selection and drift to high frequencies whereas CVs of larger effect are under increasingly strong purifying selection and kept rare because of it ( Figure 1 ).
Finally, although we have argued that much of the remaining variation in traits that has not been explained by SNPs is likely to be due to rare CVs, there are several alternative explanations for the discrepancy. For example, it is possible that family studies have over-estimated additive genetic variation, meaning that little additive genetic variation remains to be explained and that rare variants thereby account for little trait variation. Forthcoming methods that use whole-genome sequencing data or shared identical-by-descent haplotypes, both of which can measure or tag rare CVs, should be able to put the rare variant debate largely to rest by directly estimating the importance of rare CVs.
Future directions in understanding the evolutionary basis of genetic variation in behavior
We have presented evidence from schizophrenia that is generally consistent with underlying CVs on average being under purifying selection and their frequencies being maintained by mutation-selection balance. Findings on human personality [ 6 ] and other behavioral traits appear generally consistent with this, although datasets are smaller and conclusions more tentative. However, the substantial proportion of variation accounted for by common CVs suggests that the highest frequency/smallest effect CVs may be selectively neutral or nearly neutral. These findings are not contradictory. It is important to recognize that the mutation-selection and the neutral mutation-drift models are not qualitatively distinct; they exist on the same continuum defined by the strength of purifying selection. To date, there is no convincing evidence that balancing selection plays an important role in maintaining the genetic variation in behavioral traits, and outside of the MHC region, genome-wide scans suggest a limited role for balancing selection in general [ 55 – 57 ]. Nevertheless, absence of evidence does not necessarily equate to evidence for absence, and future findings could challenge this conclusion.
Large whole-genome sequencing datasets will greatly expand our ability to understand the importance of rare variants in complex traits and inform our understanding of the evolutionary processes involved in maintaining traits’ genetic variation. Nevertheless, attempting to understand the evolutionary roots of genetic variation in traits will remain inherently difficult because selection acts on total ‘net fitness’ rather than fitness with respect to any given trait. Given that CVs may often affect multiple traits simultaneously and that many CVs affect any given trait, a trait’s CVs may often be under many different types and strengths of selection. As such, future progress is likely to involve multivariate analyses that compare the characteristics (directional dominance, effect size, allelic spectrum) of CVs that affect multiple traits in the same or opposite directions with respect to fitness.
The promise of evolutionary behavioral genetics
In this article we have given an abbreviated overview of the conceptual and methodological bases of research at the intersection of evolutionary psychology and behavioral genetics, as well as a sample of the findings in this still nascent field. We have mentioned contributions of evolutionary behavioral genetics to our understanding of mate preferences, sexual dimorphism, sexual maturation, reproductive success, personality, and schizophrenia, but of necessity omitted important research on other traits [ 58 – 63 ]. We have tried to convey some of the depth and breadth of the possibilities afforded by these approaches and hope that this might spur others to adopt these approaches in testing hypotheses in evolutionary psychology and behavioral genetics.
Purifying selection removes alleles (generally rare mutations) with lower fitness in favor of one or more alternate alleles with higher fitness.
Linkage disequilibrium refers to the statistical relationship between alleles at different loci (positions in the genome).
Heritable trait variation is that due to genetic variation. Heritability refers to the proportion of trait variation that can be attributed to genetic factors.
Genetic correlation refers to the proportion of total genetic variation in two traits that is shared due to genetic factors.
Sexual selection refers to a mode of natural selection in which certain alleles are favored over others because of their effects on attracting mates rather than survival.
Alleles are alternative versions of genetic variants at a given locus.
Mutation load refers to an individual’s aggregate burden of deleterious mutations (rare alleles) across the genome, which is heritable across generations.
Good gene indicators are traits that reflect underlying genetic fitness, e.g. low mutation load.
Pleiotropic genes influence more than one trait.
Cross-trait assortative mating occurs when two different traits correlate across mates, e.g. males of above-average height mating with females of above-average intelligence.
Extended twin-family designs take advantage of the genetic relatedness between multiple family members e.g. twins, their spouses, and their parents, in order to investigate the importance of environmental and genetic influences on one or multiple traits.
Sexual dimorphism refers to the difference between male and female phenotypes.
Fisher’s Fundamental Theorem states that “the rate of increase in fitness of any organism at any time is equal to its genetic variance in fitness at that time.” It has often been interpreted to mean that additive genetic variation should be low in traits related to fitness.
Phenotypes are observable characteristics or traits of an organism.
Recessive/additive/dominant refer to how likely an allele is to be expressed in the phenotype. At a diallelic locus, a fully recessive allele will not be expressed unless both copies are present, while the fully dominant allele will be fully expressed with only one copy. Many dominance relationships are partial rather than full, yielding a spectrum of dominance or recessivity. Additivity is intermediate between fully recessive and fully dominant.
SNP (single nucleotides polymorphism) is a type of allele where a single nucleotide position is variable in the population. Often, “SNP” is used for loci where the minor allele frequency is > 1% and “mutation” when the minor allele frequency is < 1%.
Homozygosity occurs when two copies of the same allele are present at a locus, as opposed to heterozygosity, in which the two alleles at a locus are different. Runs of homozygosity are stretches of contiguous SNPs (e.g. 60+) that are consistently homozygous along some stretch of an individual’s genome.
Linkage studies test for coinheritance of alleles and traits within families. They are less powerful for detecting the effects of common causal variants than genome-wide association studies but can potentially discover regions where large-effect, rare causal variants occur.
Genome-wide association studies test for associations between each of hundreds of thousands of SNPs across the genome and one or more traits, Very large sample sizes are required to detect the small effect sizes that appear to be the norm for complex traits.
An allele frequency bin includes only alleles within a fixed-size range of frequencies.
The minor allele at a given locus is the allele that is less common in the population, and for SNPs, there are usually two alleles. The minor allele frequency is the frequency of the least common allele at a locus.
A Causal Variant (CV) is an allele that influences a trait
CVs are tagged by measured SNPs to the extent that they are in linkage disequilibrium, and therefore statistically correlated, with them.
Whole-genome sequencing provides data for the complete sequence of DNA for an individual, including all frequency classes of alleles (including unique alleles).
Supplementary Material
Acknowledgments.
The authors thank Dr. Patrick Sullivan for sharing the CNV effects that are included in Figure 1 . This work was supported by National Institutes of Mental Health grants K01MH085812 and R01MH100141 to Dr. Keller and an Australian Research Council Discovery Early Career Research Award (DE120100562) to Dr Zietsch.
Financial Disclosures.
The author reports no biomedical financial interests or potential conflicts of interest.
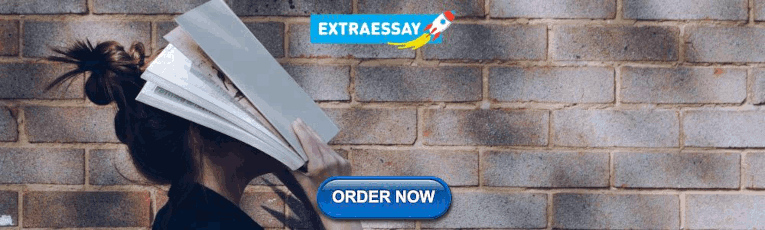
IMAGES
VIDEO
COMMENTS
The most important development during this century of behavioral genetic research has been the synthesis of the two worlds of genetics, quantitative genetics and molecular genetics. Quantitative genetics and molecular genetics both have their origins in the 1860s with Francis Galton (Galton 1865 , 1869 ) and Gregor Mendel (Mendel 1866 ...
Behavioral geneticists study how individual differences arise, in the present, through the interaction of genes and the environment. When studying human behavior, behavioral geneticists often employ twin and adoption studies to research questions of interest. Twin studies compare the rates that a given behavioral trait is shared among identical ...
Behavioral genetics is the study of genetic and environmental influences on individual differences in behavior ( phenotypes; see Glossary ). Historically, researchers used twin/family studies to estimate the relative influences of genes and environment. The logic of these studies is simple: To the degree a trait is genetically influenced ...
Abstract. The question of why people show individual differences in their behaviours and capacities has intrigued researchers for centuries. Behaviour genetics offers us various methods to address ...
Galton's twin studies and other research involving inherited abilities made him a pioneer in the field of behavioral genetics. Today, modern behavioral geneticists have the same basic goal as ...
The first human behavioral genetic research on intelligence and mental illness began in the 1920s, when environmentalism (the theory that behaviour is a result of nongenetic factors such as various childhood experiences) became popular and before Nazi Germany's abuse of genetics made the notion of hereditary influence abhorrent.Although genetic research on human behaviour continued ...
insights led to methods to study genetic influence on com-plex traits and when Mendel's work was re-discovered. The ... genetic and molecular genetic research on behavior, a whis - ... Fig. 1 Synthesis of the two worlds of genetics: from behavioral genetics to behavioral genomics. 1 3 76. Behavior Genetics (2023) 53:75-84 ...
The field of behavioral genetics, or more specifically human behavioral genetics, is concerned with the study of the causes of individual differences in psychological traits, such as intelligence (IQ) and personality. It also looks into to the possible role of genetic factors as a cause of abnormal behavior and psychiatric disorders such as ...
Learning Outcomes. Behavioral geneticists study how individual differences arise, in the present, through the interaction of genes and the environment. When studying human behavior, behavioral geneticists often employ twin and adoption studies to research questions of interest. Twin studies compare the rates that a given behavioral trait is ...
Behavioral genetics is the study of the influence one's genetic makeup and lived experiences within their unique environment have on their current behavior. Many use the term nature versus nurture ...
Behavior is the change in activity of an organism in response to a stimulus. Behavioral biology is the study of the biological and evolutionary bases for such changes. The idea that behaviors evolved as a result of the pressures of natural selection is not new. Animal behavior has been studied for decades, by biologists in the science of ethology, by psychologists in the science of comparative ...
Attempts to link genetics to social and behavioural outcomes are often met with greater scepticism and concerns about potential consequences than medical applications of genetic research (Box 1) 8 ...
A century after the first twin and adoption studies of behavior in the 1920s, this review looks back on the journey and celebrates milestones in behavioral genetic research. After a whistle-stop tour of early quantitative genetic research and the parallel journey of molecular genetics, the travelogue focuses on the last fifty years. Just as quantitative genetic discoveries were beginning to ...
Behaviour genetics offers us various methods to address this question. The answers are interesting for a range of research fields, varying from medicine to psychology, economics and neuroscience. ... The University of São Paulo Twin Panel: current status and prospects for Brazilian twin studies in behavioral research. Twin Res. Hum. Genet. 22 ...
The question of whether these individual differences in complex behavioral traits are caused by genetic (heritable) or environmental influences, or a combination of the two, is referred to as the nature versus nurture debate (Fig. 10.1), and dates back to ancient Greece (Loehlin, 2009).
A second look at twin studies. As behavioral genetics enters a second century, the field's oldest research method remains both relevant and controversial. ... the approximately two-fold difference in genetic similarity between the two types of twins should outweigh any complications, says John Hewitt, PhD, director of the Institute for ...
Wade's book partly relies on research findings from behavioral genetics. Classical twin studies published in this field regularly report high heritability values, suggesting that genes affect even complex, modern social behavior (Hatemi and McDermott 2012; Plomin and Colledge 2001). From time to time, some of these high values appear in the news.
Describe two types of research studies often used by behavioral geneticists and explain why these types of research are used. This problem has been solved! You'll get a detailed solution from a subject matter expert that helps you learn core concepts.
The purpose of this manuscript is to describe the scientific enterprise at the intersection of evolutionary psychology and behavioral genetics—a field that could be termed Evolutionary Behavioral Genetics —and how modern genetic data is revolutionizing our ability to test questions in this field. We first describe how methods and designs ...