Navigation group
Home banner.
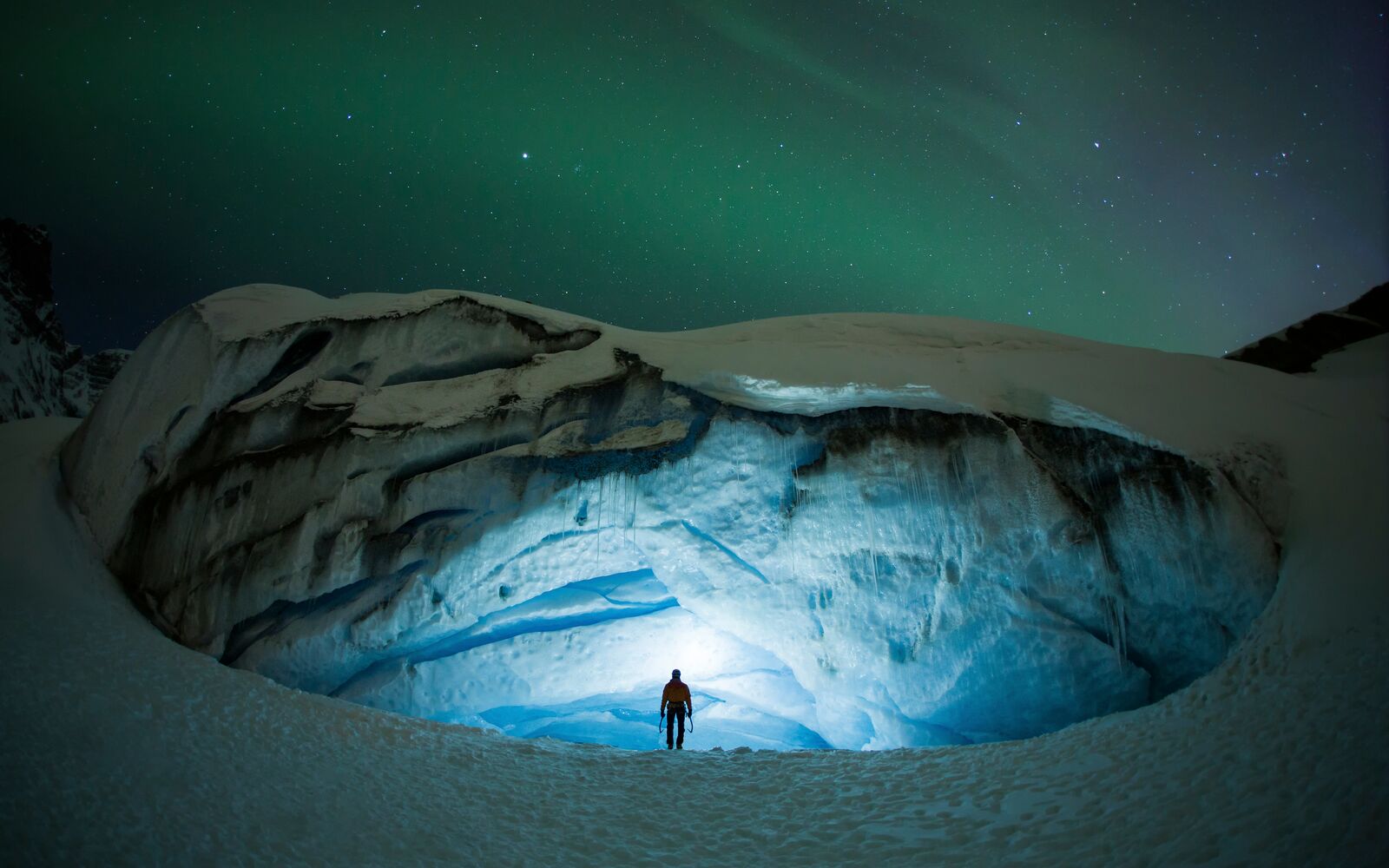
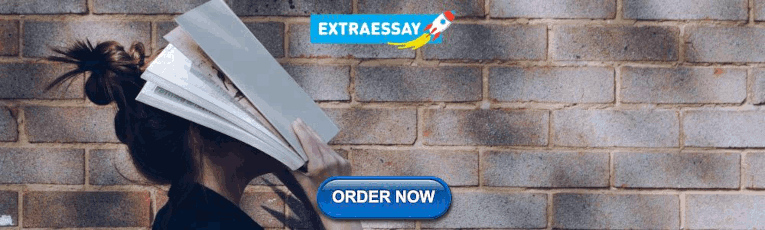
Where scientists empower society
Creating solutions for healthy lives on a healthy planet.
most-cited publisher
largest publisher
2.5 billion
article views and downloads
Main Content
- Editors and reviewers
- Collaborators
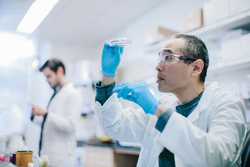
Find a journal
We have a home for your research. Our community led journals cover more than 1,500 academic disciplines and are some of the largest and most cited in their fields.
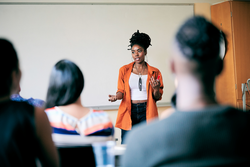
Submit your research
Start your submission and get more impact for your research by publishing with us.
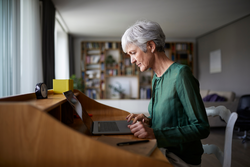
Author guidelines
Ready to publish? Check our author guidelines for everything you need to know about submitting, from choosing a journal and section to preparing your manuscript.
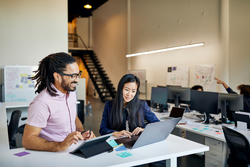
Peer review
Our efficient collaborative peer review means you’ll get a decision on your manuscript in an average of 61 days.
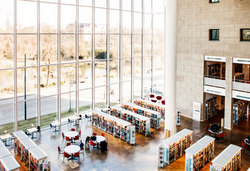
Article publishing charges (APCs) apply to articles that are accepted for publication by our external and independent editorial boards
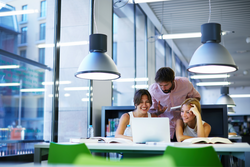
Press office
Visit our press office for key media contact information, as well as Frontiers’ media kit, including our embargo policy, logos, key facts, leadership bios, and imagery.
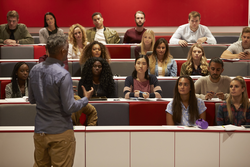
Institutional partnerships
Join more than 555 institutions around the world already benefiting from an institutional membership with Frontiers, including CERN, Max Planck Society, and the University of Oxford.
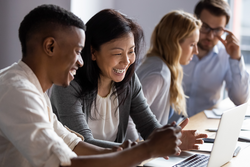
Publishing partnerships
Partner with Frontiers and make your society’s transition to open access a reality with our custom-built platform and publishing expertise.
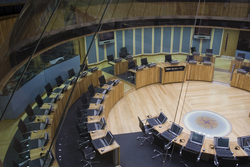
Policy Labs
Connecting experts from business, science, and policy to strengthen the dialogue between scientific research and informed policymaking.
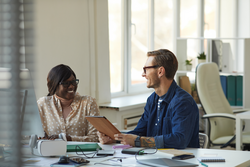
How we publish
All Frontiers journals are community-run and fully open access, so every research article we publish is immediately and permanently free to read.
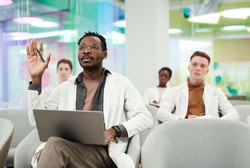
Editor guidelines
Reviewing a manuscript? See our guidelines for everything you need to know about our peer review process.
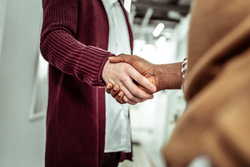
Become an editor
Apply to join an editorial board and collaborate with an international team of carefully selected independent researchers.
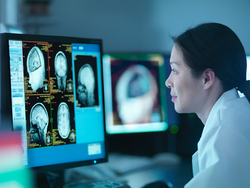
My assignments
It’s easy to find and track your editorial assignments with our platform, 'My Frontiers' – saving you time to spend on your own research.
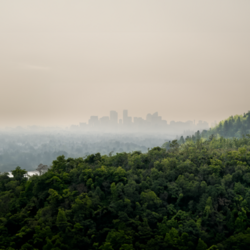
Scientists call for urgent action to prevent immune-mediated illnesses caused by climate change and biodiversity loss
Climate change, pollution, and collapsing biodiversity are damaging our immune systems, but improving the environment offers effective and fast-acting protection.
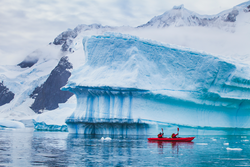
Safeguarding peer review to ensure quality at scale
Making scientific research open has never been more important. But for research to be trusted, it must be of the highest quality. Facing an industry-wide rise in fraudulent science, Frontiers has increased its focus on safeguarding quality.
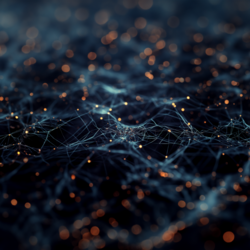
Chronic stress and inflammation linked to societal and environmental impacts in new study
Scientists hypothesize that as-yet unrecognized inflammatory stress is spreading among people at unprecedented rates, affecting our cognitive ability to address climate change, war, and other critical issues.
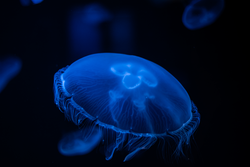
Tiny crustaceans discovered preying on live jellyfish during harsh Arctic night
Scientists used DNA metabarcoding to show for the first time that jellyfish are an important food for amphipods during the Arctic polar night in waters off Svalbard, at a time of year when other food resources are scarce.
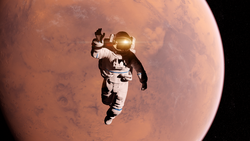
Why studying astronauts’ microbiomes is crucial to ensure deep space mission success
In a new Frontiers’ guest editorial, Prof Dr Lembit Sihver, director of CRREAT at the Nuclear Physics Institute of the Czech Academy of Sciences and his co-authors explore the impact the microbiome has on human health in space.
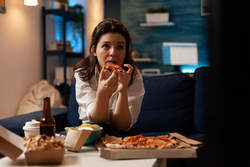
Cake and cookies may increase Alzheimer’s risk: Here are five Frontiers articles you won’t want to miss
At Frontiers, we bring some of the world’s best research to a global audience. But with tens of thousands of articles published each year, it’s impossible to cover all of them. Here are just five amazing papers you may have missed.
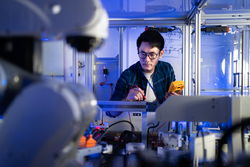
2024's top 10 tech-driven Research Topics
Frontiers has compiled a list of 10 Research Topics that embrace the potential of technology to advance scientific breakthroughs and change the world for the better.
Get the latest research updates, subscribe to our newsletter
Quick Links
- RESOURCE CENTER
- MEMBER LOGIN
External Links
- AAAS Communities
- SCIENCE CAREERS
- SCIENCE FAMILY OF JOURNALS
- More AAAS Sites
Scientific Journals

A nitrogen-fixing organelle, or “nitroplast,” has been identified in a marine alga on the basis of intracellular imaging and proteomic evidence. This discovery sheds light on the evolutionary transition from endosymbiont to organelle. The image depicts the cell architecture and synchronized cell division of the alga Braarudosphaera bigelowii with nitroplast UCYN-A (large brown spheres). See pages 160 and 217. Image: N. Burgess/Science; Data: Tyler Coale et al., University of California Santa Cruz

Trucks transporting bauxite along a mining hauling road in Guinea. The demand for minerals required for clean energy technologies has fueled extensive exploration into wildlife habitats. Junker et al. integrated a global mining dataset with great ape density distribution and found that up to one-third of Africa’s great ape population faces mining-related risks. Apes in West Africa could be most severely affected, where up to 82% of the population overlaps with mining locations. The findings suggest the need to make environmental data accessible to enable assessments of the impact of mining on wildlife populations. Credit: Geneviève Campbell

Cultivating Memory B Cell Responses to a Plant-Based Vaccine. CoVLP (coronavirus virus-like particle) is a promising COVID-19 vaccine produced in the weed Nicotiana benthamiana. A squalene-based adjuvant, AS03, can enhance immune responses to CoVLP vaccination, but how AS03 affects memory B cell responses to CoVLP is unknown. Grigoryan et al. studied immune responses in healthy individuals who received two doses of CoVLP with or without AS03. They found that AS03 promoted the progressive maturation of memory B cell responses over time, leading to enhanced neutralization of SARS-CoV-2 and increased memory B cell breadth. This month’s cover illustration depicts a syringe containing a plant-based SARS-CoV-2 vaccine./a> Credit: N. Jessup/Science Immunology

Special Issue on Legged Robots. Developing legged robots capable of complex motor skills is a major challenge for roboticists. Haarnoja et al. used deep reinforcement learning to train miniature humanoid robots, Robotis OP3, to play a game of one-versus-one soccer. The robots were capable of exhibiting not only agile movements, such as walking, kicking the ball, and rapid recovery from falls, but also emergent behaviors to adapt to the game scenario, such as subtle defensive moves and dynamic footwork in response to the opponent. This month’s cover is an image of the miniature humanoid robot kicking a ball. Credit: Google DeepMind

This week, Ribeiro et al. report that DNA damage induced by the blockade of lipid synthesis in prostate cancer increases the effectiveness of PARP inhibition. The image shows a tissue section of human prostate cancer. Image: Nigel Downer/Science Source

Disconnecting Inflammation from Pain. The cover shows immunostaining of vascular endothelium (CD31, magenta) and calcitonin gene-related peptide axons (CGRP, cyan) in the synovium from an individual with rheumatoid arthritis, indicating pain-sensitive neuron sprouting in the joint. Rheumatoid arthritis pain does not always correlate with inflammation in joints. Using machine learning, Bai et al. used different cohorts of patients to identify hundreds of genes that were both involved in patient-reported pain and not associated with inflammation. These genes were mostly expressed in fibroblasts lining the synovium that interacted with CGRP-expressing neurons. This study may help identify new targets for treating rheumatoid arthritis pain. Credit: Bai et al./Science Translational Medicine
Click through the PLOS taxonomy to find articles in your field.
For more information about PLOS Subject Areas, click here .
April 9, 2024
PLOS ONE
An inclusive journal community working together to advance science by making all rigorous research accessible without barriers
Calling all experts!
Plos one is seeking talented individuals to join our editorial board. .
Computational Biology
See Elegans: Simple-to-use, accurate, and automatic 3D detection of neural activity from densely packed neurons
Lanza and collagues presented a novel method (See Elegans) for automatic neuron sementation and tracking in C. elegans.
Image credit: Fig 2 by Lanza et al., CC BY 4.0
Pharmacology
Enhancing radioprotection: A chitosan-based chelating polymer is a versatile radioprotective agent for prophylactic and therapeutic interventions against radionuclide contamination
Durand and colleagues report the evaluation of a functionalized chitosan polymer for treating exposure to radioactive isotopes, including uranium, for which there are no suitable current countermeasures.
Image credit: Radioactive Materials Area by Kerry, CC BY 2.0
Mental Health
Cross-cultural variation in experiences of acceptance, camouflaging and mental health difficulties in autism: A registered report
In this Registered Report, Keating and colleagues explore the relationship between autism acceptance, camoflauging, and mental health in a cross-cultural sample of autistic adults.
Image credit: man-390342_1280 by PDPPics, Pixabay
The double-edged scalpel: Experiences and perceptions of pregnancy and parenthood during Canadian surgical residency training
Peters and colleagues survey female surgical trainees, who report experiencing higher rates of pregnancy complications when compared to non-surgical counterparts, more negative stigma and bias, and other social and logistical challenges. This highlights the need to create a culture where both birthing and non-birthing parents are empowered and supported.
Image credit: woman-1284353_1280 by Pexels, Pixabay
International Day of Women and Girls in Science – Interview with Dr. Swetavalli Raghavan
PLOS ONE Associate Editor Dr Johanna Pruller interviews Dr Swetavalli Raghavan, full professor and founder of Scientists & Co. about mentorship, role models, and the changing landscape for women in science.
Image credit: Dr Swetavalli Raghavan by EveryONE, CC BY 4.0
International Women’s Day – Interview with PLOS ONE Academic Editor Dr. Siaw Shi Boon
PLOS ONE Senior Editor Dr Jianhong Zhou interviews PLOS ONE Academic Editor Dr Siaw Shi Boon about her path to becoming a scientist, challenges facing women in science, and how to encourage more women to become scientists.
Image credit: Dr Siaw Shi Boon by EveryONE, CC BY 4.0
Editor Spotlight: Simon Porcher
In this interview, PLOS ONE Academic Editor Dr Simon Porcher discusses his role as editor, enhancing reproducibility in scientific reporting, and how we can act in future pandemics.
Image credit: Simon Porcher by EveryONE, CC BY 4.0
Child development
Childhood experiences and sleep problems: A cross-sectional study on the indirect relationship mediated by stress, resilience and anxiety
Ashour and colleagues investigate the relationship between childhood experiences and sleep quality in adulthood.
Image credit: Alarm Clock by Congerdesign, Pixabay
Sports and exercise medicine
Responsiveness of respiratory function in Parkinson’s Disease to an integrative exercise programme: A prospective cohort study
McMahon and colleagues report the effectiveness of an exercise intervention on respiratory function.
Image credit: Side view doctor looking at radiography by Freepik, Freepik
The great urban shift: Climate change is predicted to drive mass species turnover in cities
Filazzola and colleagues model how terrestrial wildlife within 60 Canadian and American cities will be affected by climate change.
Image credit: Fig 3 by Filazzola et al., CC BY 4.0
Accessibility
Color Quest: An interactive tool for exploring color palettes and enhancing accessibility in data visualization
Nelli presents an open-source tool for visualizing how various data plots appear to individuals with color blindness, towards improved accessibility.
Image credit: Fig 1 by Nelli et al., CC BY 4.0
Collections
Browse the latest collections of papers from across PLOS.
Watch this space for future collections of papers in PLOS ONE.
Conferences 2024
New opportunities to meet our editorial staff in 2024 will be announced soon.
Publish with PLOS ONE
- Submission Instructions
- Submit Your Manuscript
Connect with Us
- PLOS ONE on Twitter
- PLOS on Facebook
Get new content from PLOS ONE in your inbox
Thank you you have successfully subscribed to the plos one newsletter., sorry, an error occurred while sending your subscription. please try again later..
Disclaimer » Advertising
- HealthyChildren.org
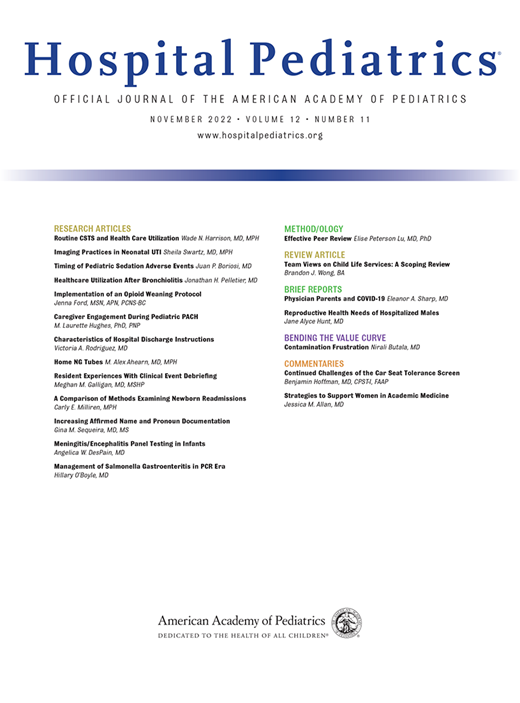
- Previous Article
- Next Article
What is the Purpose of Peer Review?
What makes a good peer reviewer, how do you decide whether to review a paper, how do you complete a peer review, limitations of peer review, conclusions, research methods: how to perform an effective peer review.
- Split-Screen
- Article contents
- Figures & tables
- Supplementary Data
- Peer Review
- CME Quiz Close Quiz
- Open the PDF for in another window
- Get Permissions
- Cite Icon Cite
- Search Site
Elise Peterson Lu , Brett G. Fischer , Melissa A. Plesac , Andrew P.J. Olson; Research Methods: How to Perform an Effective Peer Review. Hosp Pediatr November 2022; 12 (11): e409–e413. https://doi.org/10.1542/hpeds.2022-006764
Download citation file:
- Ris (Zotero)
- Reference Manager
Scientific peer review has existed for centuries and is a cornerstone of the scientific publication process. Because the number of scientific publications has rapidly increased over the past decades, so has the number of peer reviews and peer reviewers. In this paper, drawing on the relevant medical literature and our collective experience as peer reviewers, we provide a user guide to the peer review process, including discussion of the purpose and limitations of peer review, the qualities of a good peer reviewer, and a step-by-step process of how to conduct an effective peer review.
Peer review has been a part of scientific publications since 1665, when the Philosophical Transactions of the Royal Society became the first publication to formalize a system of expert review. 1 , 2 It became an institutionalized part of science in the latter half of the 20 th century and is now the standard in scientific research publications. 3 In 2012, there were more than 28 000 scholarly peer-reviewed journals and more than 3 million peer reviewed articles are now published annually. 3 , 4 However, even with this volume, most peer reviewers learn to review “on the (unpaid) job” and no standard training system exists to ensure quality and consistency. 5 Expectations and format vary between journals and most, but not all, provide basic instructions for reviewers. In this paper, we provide a general introduction to the peer review process and identify common strategies for success as well as pitfalls to avoid.
Modern peer review serves 2 primary purposes: (1) as “a screen before the diffusion of new knowledge” 6 and (2) as a method to improve the quality of published work. 1 , 5
As screeners, peer reviewers evaluate the quality, validity, relevance, and significance of research before publication to maintain the credibility of the publications they serve and their fields of study. 1 , 2 , 7 Although peer reviewers are not the final decision makers on publication (that role belongs to the editor), their recommendations affect editorial decisions and thoughtful comments influence an article’s fate. 6 , 8
As advisors and evaluators of manuscripts, reviewers have an opportunity and responsibility to give authors an outside expert’s perspective on their work. 9 They provide feedback that can improve methodology, enhance rigor, improve clarity, and redefine the scope of articles. 5 , 8 , 10 This often happens even if a paper is not ultimately accepted at the reviewer’s journal because peer reviewers’ comments are incorporated into revised drafts that are submitted to another journal. In a 2019 survey of authors, reviewers, and editors, 83% said that peer review helps science communication and 90% of authors reported that peer review improved their last paper. 11
Expertise: Peer reviewers should be up to date with current literature, practice guidelines, and methodology within their subject area. However, academic rank and seniority do not define expertise and are not actually correlated with performance in peer review. 13
Professionalism: Reviewers should be reliable and objective, aware of their own biases, and respectful of the confidentiality of the peer review process.
Critical skill : Reviewers should be organized, thorough, and detailed in their critique with the goal of improving the manuscript under their review, regardless of disposition. They should provide constructive comments that are specific and addressable, referencing literature when possible. A peer reviewer should leave a paper better than he or she found it.
Is the manuscript within your area of expertise? Generally, if you are asked to review a paper, it is because an editor felt that you were a qualified expert. In a 2019 survey, 74% of requested reviews were within the reviewer’s area of expertise. 11 This, of course, does not mean that you must be widely published in the area, only that you have enough expertise and comfort with the topic to critique and add to the paper.
Do you have any biases that may affect your review? Are there elements of the methodology, content area, or theory with which you disagree? Some disagreements between authors and reviewers are common, expected, and even helpful. However, if a reviewer fundamentally disagrees with an author’s premise such that he or she cannot be constructive, the review invitation should be declined.
Do you have the time? The average review for a clinical journal takes 5 to 6 hours, though many take longer depending on the complexity of the research and the experience of the reviewer. 1 , 14 Journals vary on the requested timeline for return of reviews, though it is usually 1 to 4 weeks. Peer review is often the longest part of the publication process and delays contribute to slower dissemination of important work and decreased author satisfaction. 15 Be mindful of your schedule and only accept a review invitation if you can reasonably return the review in the requested time.
Once you have determined that you are the right person and decided to take on the review, reply to the inviting e-mail or click the associated link to accept (or decline) the invitation. Journal editors invite a limited number of reviewers at a time and wait for responses before inviting others. A common complaint among journal editors surveyed was that reviewers would often take days to weeks to respond to requests, or not respond at all, making it difficult to find appropriate reviewers and prolonging an already long process. 5
Now that you have decided to take on the review, it is best of have a systematic way of both evaluating the manuscript and writing the review. Various suggestions exist in the literature, but we will describe our standard procedure for review, incorporating specific do’s and don’ts summarized in Table 1 .
Dos and Don’ts of Peer Review
First, read the manuscript once without making notes or forming opinions to get a sense of the paper as whole. Assess the overall tone and flow and define what the authors identify as the main point of their work. Does the work overall make sense? Do the authors tell the story effectively?
Next, read the manuscript again with an eye toward review, taking notes and formulating thoughts on strengths and weaknesses. Consider the methodology and identify the specific type of research described. Refer to the corresponding reporting guideline if applicable (CONSORT for randomized control trials, STROBE for observational studies, PRISMA for systematic reviews). Reporting guidelines often include a checklist, flow diagram, or structured text giving a minimum list of information needed in a manuscript based on the type of research done. 16 This allows the reviewer to formulate a more nuanced and specific assessment of the manuscript.
Next, review the main findings, the significance of the work, and what contribution it makes to the field. Examine the presentation and flow of the manuscript but do not copy edit the text. At this point, you should start to write your review. Some journals provide a format for their reviews, but often it is up to the reviewer. In surveys of journal editors and reviewers, a review organized by manuscript section was the most favored, 5 , 6 so that is what we will describe here.
As you write your review, consider starting with a brief summary of the work that identifies the main topic, explains the basic approach, and describes the findings and conclusions. 12 , 17 Though not universally included in all reviews, we have found this step to be helpful in ensuring that the work is conveyed clearly enough for the reviewer to summarize it. Include brief notes on the significance of the work and what it adds to current knowledge. Critique the presentation of the work: is it clearly written? Is its length appropriate? List any major concerns with the work overall, such as major methodological flaws or inaccurate conclusions that should disqualify it from publication, though do not comment directly on disposition. Then perform your review by section:
Abstract : Is it consistent with the rest of the paper? Does it adequately describe the major points?
Introduction : This section should provide adequate background to explain the need for the study. Generally, classic or highly relevant studies should be cited, but citations do not have to be exhaustive. The research question and hypothesis should be clearly stated.
Methods: Evaluate both the methods themselves and the way in which they are explained. Does the methodology used meet the needs of the questions proposed? Is there sufficient detail to explain what the authors did and, if not, what needs to be added? For clinical research, examine the inclusion/exclusion criteria, control populations, and possible sources of bias. Reporting guidelines can be particularly helpful in determining the appropriateness of the methods and how they are reported.
Some journals will expect an evaluation of the statistics used, whereas others will have a separate statistician evaluate, and the reviewers are generally not expected to have an exhaustive knowledge of statistical methods. Clarify expectations if needed and, if you do not feel qualified to evaluate the statistics, make this clear in your review.
Results: Evaluate the presentation of the results. Is information given in sufficient detail to assess credibility? Are the results consistent with the methodology reported? Are the figures and tables consistent with the text, easy to interpret, and relevant to the work? Make note of data that could be better detailed in figures or tables, rather than included in the text. Make note of inappropriate interpretation in the results section (this should be in discussion) or rehashing of methods.
Discussion: Evaluate the authors’ interpretation of their results, how they address limitations, and the implications of their work. How does the work contribute to the field, and do the authors adequately describe those contributions? Make note of overinterpretation or conclusions not supported by the data.
The length of your review often correlates with your opinion of the quality of the work. If an article has major flaws that you think preclude publication, write a brief review that focuses on the big picture. Articles that may not be accepted but still represent quality work merit longer reviews aimed at helping the author improve the work for resubmission elsewhere.
Generally, do not include your recommendation on disposition in the body of the review itself. Acceptance or rejection is ultimately determined by the editor and including your recommendation in your comments to the authors can be confusing. A journal editor’s decision on acceptance or rejection may depend on more factors than just the quality of the work, including the subject area, journal priorities, other contemporaneous submissions, and page constraints.
Many submission sites include a separate question asking whether to accept, accept with major revision, or reject. If this specific format is not included, then add your recommendation in the “confidential notes to the editor.” Your recommendation should be consistent with the content of your review: don’t give a glowing review but recommend rejection or harshly criticize a manuscript but recommend publication. Last, regardless of your ultimate recommendation on disposition, it is imperative to use respectful and professional language and tone in your written review.
Although peer review is often described as the “gatekeeper” of science and characterized as a quality control measure, peer review is not ideally designed to detect fundamental errors, plagiarism, or fraud. In multiple studies, peer reviewers detected only 20% to 33% of intentionally inserted errors in scientific manuscripts. 18 , 19 Plagiarism similarly is not detected in peer review, largely because of the huge volume of literature available to plagiarize. Most journals now use computer software to identify plagiarism before a manuscript goes to peer review. Finally, outright fraud often goes undetected in peer review. Reviewers start from a position of respect for the authors and trust the data they are given barring obvious inconsistencies. Ultimately, reviewers are “gatekeepers, not detectives.” 7
Peer review is also limited by bias. Even with the best of intentions, reviewers bring biases including but not limited to prestige bias, affiliation bias, nationality bias, language bias, gender bias, content bias, confirmation bias, bias against interdisciplinary research, publication bias, conservatism, and bias of conflict of interest. 3 , 4 , 6 For example, peer reviewers score methodology higher and are more likely to recommend publication when prestigious author names or institutions are visible. 20 Although bias can be mitigated both by the reviewer and by the journal, it cannot be eliminated. Reviewers should be mindful of their own biases while performing reviews and work to actively mitigate them. For example, if English language editing is necessary, state this with specific examples rather than suggesting the authors seek editing by a “native English speaker.”
Peer review is an essential, though imperfect, part of the forward movement of science. Peer review can function as both a gatekeeper to protect the published record of science and a mechanism to improve research at the level of individual manuscripts. Here, we have described our strategy, summarized in Table 2 , for performing a thorough peer review, with a focus on organization, objectivity, and constructiveness. By using a systematized strategy to evaluate manuscripts and an organized format for writing reviews, you can provide a relatively objective perspective in editorial decision-making. By providing specific and constructive feedback to authors, you contribute to the quality of the published literature.
Take-home Points
FUNDING: No external funding.
CONFLICT OF INTEREST DISCLOSURES: The authors have indicated they have no potential conflicts of interest to disclose.
Dr Lu performed the literature review and wrote the manuscript. Dr Fischer assisted in the literature review and reviewed and edited the manuscript. Dr Plesac provided background information on the process of peer review, reviewed and edited the manuscript, and completed revisions. Dr Olson provided background information and practical advice, critically reviewed and revised the manuscript, and approved the final manuscript.
Advertising Disclaimer »
Citing articles via
Email alerts.
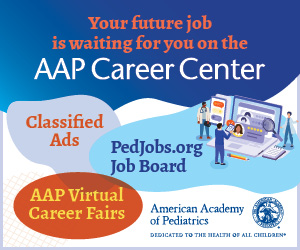
Affiliations
- Editorial Board
- Editorial Policies
- Pediatrics On Call
- Online ISSN 2154-1671
- Print ISSN 2154-1663
- Pediatrics Open Science
- Hospital Pediatrics
- Pediatrics in Review
- AAP Grand Rounds
- Latest News
- Pediatric Care Online
- Red Book Online
- Pediatric Patient Education
- AAP Toolkits
- AAP Pediatric Coding Newsletter
First 1,000 Days Knowledge Center
Institutions/librarians, group practices, licensing/permissions, integrations, advertising.
- Privacy Statement | Accessibility Statement | Terms of Use | Support Center | Contact Us
- © Copyright American Academy of Pediatrics
This Feature Is Available To Subscribers Only
Sign In or Create an Account
Preserving the Quality of Scientific Research: Peer Review of Research Articles
- First Online: 20 January 2017
Cite this chapter
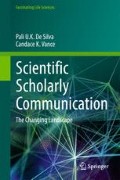
- Pali U. K. De Silva 3 &
- Candace K. Vance 3
Part of the book series: Fascinating Life Sciences ((FLS))
1343 Accesses
8 Citations
Peer review of scholarly articles is a mechanism used to assess and preserve the trustworthiness of reporting of scientific findings. Since peer reviewing is a qualitative evaluation system that involves the judgment of experts in a field about the quality of research performed by their colleagues (and competitors), it inherently encompasses a strongly subjective element. Although this time-tested system, which has been evolving since the mid-eighteenth century, is being questioned and criticized for its deficiencies, it is still considered an integral part of the scholarly communication system, as no other procedure has been proposed to replace it. Therefore, to improve and strengthen the existing peer review process, it is important to understand its shortcomings and to continue the constructive deliberations of all participants within the scientific scholarly communication system . This chapter discusses the strengths, issues, and deficiencies of the peer review system, conventional closed models (single-blind and double-blind), and the new open peer review model and its variations that are being experimented with by some journals.
- Article peer review system
- Closed peer review
- Open peer review
- Scientific journal publishing
- Single blind peer reviewing
- Article retraction
- Nonselective review
- Post-publication review system
- Double blind peer reviewing
This is a preview of subscription content, log in via an institution to check access.
Access this chapter
- Available as PDF
- Read on any device
- Instant download
- Own it forever
- Available as EPUB and PDF
- Compact, lightweight edition
- Dispatched in 3 to 5 business days
- Free shipping worldwide - see info
- Durable hardcover edition
Tax calculation will be finalised at checkout
Purchases are for personal use only
Institutional subscriptions
Evaluative criteria may also vary depending on the scope of the specific journal.
Krebs and Johnson ( 1937 ).
McClintock ( 1950 ).
Bombardier et al. ( 2000 ).
“Nature journals offer double-blind review” Nature announcement— http://www.nature.com/news/nature-journals-offer-double-blind-review-1.16931 .
Contains all versions of the manuscript, named reviewer reports, author responses, and (where relevant) editors’ comments (Moylan et al. 2014 ).
https://www.elsevier.com/about/press-releases/research-and-journals/peer-review-survey-2009-preliminary-findings .
Review guidelines, Frontiers in Neuroscience http://journal.frontiersin.org/journal/synaptic-neuroscience#review-guidelines .
Editorial policies - BioMed Central http://www.biomedcentral.com/getpublished/editorial-policies#peer+review .
Hydrology and Earth System Sciences Interactive Public Peer Review http://www.hydrology-and-earth-system-sciences.net/peer_review/interactive_review_process.html .
Copernicus Publications http://publications.copernicus.org/services/public_peer_review.html .
Copernicus Publications - Interactive Public Peer Review http://home.frontiersin.org/about/impact-and-tiering .
Biology Direct http://www.biologydirect.com/ .
F1000 Research http://f1000research.com .
GigaScience http://www.gigasciencejournal.com
Journal of Negative Results in Biomedicine http://www.jnrbm.com/ .
BMJOpen http://bmjopen.bmj.com/ .
PeerJ http://peerj.com/ .
ScienceOpen https://www.scienceopen.com .
ArXiv http://arxiv.org .
Retraction of articles from Springer journals. London: Springer, August 18, 2015 ( http://www.springer.com/gp/about-springer/media/statements/retraction-of-articles-from-springer-journals/735218 ).
COPE statement on inappropriate manipulation of peer review processes ( http://publicationethics.org/news/cope-statement-inappropriate-manipulation-peer-review-processes ).
Hindawi concludes an in-depth investigation into peer review fraud, July 2015 ( http://www.hindawi.com/statement/ ).
.Wakefield, A. J., Murch, S. H., Anthony, A., Linnell, J., Casson, D. M., Malik, M., ... & Valentine, A. (1998). Ileal-lymphoid-nodular hyperplasia, non-specific colitis, and pervasive developmental disorder in children. The Lancet , 351 (9103), 637–641. (RETRACTED:See The Lancet 375 (9713) p.445)
A practice used by researchers to increase the number of articles publishing multiple papers using very similar pieces of a single dataset. The drug industry also uses this tactic to increase publications with positive findings on their products.
Neuroscience Peer Reviewer Consortium http://nprc.incf.org/ .
“About 80% of submitted manuscripts are rejected during this initial screening stage, usually within one week to 10 days.” http://www.sciencemag.org/site/feature/contribinfo/faq/ (accessed on October 18, 2016); “Nature has space to publish only 8% or so of the 200 papers submitted each week” http://www.nature.com/nature/authors/get_published/ (accessed on October 18, 2016).
Code of Conduct and Best Practice Guidelines for Journal Editors http://publicationethics.org/files/Code%20of%20Conduct_2.pdf .
Recommendations for the Conduct, Reporting, Editing, and Publication of Scholarly work in Medical Journals http://www.icmje.org/icmje-recommendations.pdf .
Alberts, B., Hanson, B., & Kelner, K. L. (2008). Reviewing peer review. Science, 321 (5885), 15.
Article PubMed Google Scholar
Ali, P. A., & Watson, R. (2016). Peer review and the publication process. Nursing Open . doi: 10.1002/nop2.51 .
Baggs, J. G., Broome, M. E., Dougherty, M. C., Freda, M. C., & Kearney, M. H. (2008). Blinding in peer review: the preferences of reviewers for nursing journals. Journal of Advanced Nursing, 64 (2), 131–138.
Bjork, B.-C., Roos, A., & Lauri, M. (2009). Scientific journal publishing: yearly volume and open access availability. Information Research: An International Electronic Journal, 14 (1).
Google Scholar
Bohannon, J. (2013). Who’s afraid of peer review. Science, 342 (6154).
Boldt, A. (2011). Extending ArXiv. org to achieve open peer review and publishing. Journal of Scholarly Publishing, 42 (2), 238–242.
Article Google Scholar
Bombardier, C., Laine, L., Reicin, A., Shapiro, D., Burgos-Vargas, R., Davis, B., … & Kvien, T. K. (2000). Comparison of upper gastrointestinal toxicity of rofecoxib and naproxen in patients with rheumatoid arthritis. New England Journal of Medicine, 343 (21), 1520–1528
Bornmann, L. (2011). Scientific peer review. Annual Review of Information Science and Technology, 45 (1), 197–245.
Bornmann, L., & Daniel, H.-D. (2009). Reviewer and editor biases in journal peer review: An investigation of manuscript refereeing at Angewandte Chemie International Edition. Research Evaluation, 18 (4), 262–272.
Bornmann, L., & Daniel, H. D. (2010). Reliability of reviewers’ ratings when using public peer review: A case study. Learned Publishing, 23 (2), 124–131.
Bornmann, L., Mutz, R., & Daniel, H.-D. (2007). Gender differences in grant peer review: A meta-analysis. Journal of Informetrics, 1 (3), 226–238.
Borsuk, R. M., Aarssen, L. W., Budden, A. E., Koricheva, J., Leimu, R., Tregenza, T., et al. (2009). To name or not to name: The effect of changing author gender on peer review. BioScience, 59 (11), 985–989.
Bosch, X., Pericas, J. M., Hernández, C., & Doti, P. (2013). Financial, nonfinancial and editors’ conflicts of interest in high-impact biomedical journals. European Journal of Clinical Investigation, 43 (7), 660–667.
Brown, R. J. C. (2007). Double anonymity in peer review within the chemistry periodicals community. Learned Publishing, 20 (2), 131–137.
Budden, A. E., Tregenza, T., Aarssen, L. W., Koricheva, J., Leimu, R., & Lortie, C. J. (2008). Double-blind review favours increased representation of female authors. Trends in Ecology & Evolution, 23 (1), 4–6.
Burnham, J. C. (1990). The evolution of editorial peer review. JAMA, 263 (10), 1323–1329.
Article CAS PubMed Google Scholar
Callaham, M. L., & Tercier, J. (2007). The relationship of previous training and experience of journal peer reviewers to subsequent review quality. PLoS Med, 4 (1), e40.
Article PubMed PubMed Central Google Scholar
Campbell, P. (2006). Peer Review Trial and Debate. Nature . http://www.nature.com/nature/peerreview/debate/
Campbell, P. (2008). Nature peer review trial and debate. Nature: International Weekly Journal of Science, 11
Campos-Arceiz, A., Primack, R. B., & Koh, L. P. (2015). Reviewer recommendations and editors’ decisions for a conservation journal: Is it just a crapshoot? And do Chinese authors get a fair shot? Biological Conservation, 186, 22–27.
Cantor, M., & Gero, S. (2015). The missing metric: Quantifying contributions of reviewers. Royal Society open science, 2 (2), 140540.
CDC. (2016). Measles: Cases and Outbreaks. Retrieved from http://www.cdc.gov/measles/cases-outbreaks.html
Ceci, S. J., & Williams, W. M. (2011). Understanding current causes of women’s underrepresentation in science. Proceedings of the National Academy of Sciences, 108 (8), 3157–3162.
Article CAS Google Scholar
Chan, A. W., Hróbjartsson, A., Haahr, M. T., Gøtzsche, P. C., & Altman, D. G. (2004). Empirical evidence for selective reporting of outcomes in randomized trials: comparison of protocols to published articles. JAMA, 291 (20), 2457–2465.
Charlton, B. G. (2004). Conflicts of interest in medical science: peer usage, peer review andCoI consultancy’. Medical Hypotheses, 63 (2), 181–186.
Cressey, D. (2014). Journals weigh up double-blind peer review. Nature news .
Dalton, R. (2001). Peers under pressure. Nature, 413 (6852), 102–104.
DeVries, D. R., Marschall, E. A., & Stein, R. A. (2009). Exploring the peer review process: What is it, does it work, and can it be improved? Fisheries, 34 (6), 270–279. doi: 10.1577/1548-8446-34.6.270
Emerson, G. B., Warme, W. J., Wolf, F. M., Heckman, J. D., Brand, R. A., & Leopold, S. S. (2010). Testing for the presence of positive-outcome bias in peer review: A randomized controlled trial. Archives of Internal Medicine, 170 (21), 1934–1939.
Fanelli, D. (2010). Do pressures to publish increase scientists’ bias? An empirical support from US States Data. PLoS ONE, 5 (4), e10271.
Fang, F. C., Steen, R. G., & Casadevall, A. (2012). Misconduct accounts for the majority of retracted scientific publications. Proceedings of the National Academy of Sciences, 109 (42), 17028–17033. doi: 10.1073/pnas.1212247109
Ferguson, C., Marcus, A., & Oransky, I. (2014). Publishing: The peer-review scam. Nature, 515 (7528), 480.
Ford, E. (2015). Open peer review at four STEM journals: An observational overview. F1000Research, 4 .
Fountain, H. (2014). Science journal pulls 60 papers in peer-review fraud. Science, 3, 06.
Freda, M. C., Kearney, M. H., Baggs, J. G., Broome, M. E., & Dougherty, M. (2009). Peer reviewer training and editor support: Results from an international survey of nursing peer reviewers. Journal of Professional Nursing, 25 (2), 101–108.
Gillespie, G. W., Chubin, D. E., & Kurzon, G. M. (1985). Experience with NIH peer review: Researchers’ cynicism and desire for change. Science, Technology and Human Values, 10 (3), 44–54.
Greaves, S., Scott, J., Clarke, M., Miller, L., Hannay, T., Thomas, A., et al. (2006). Overview: Nature’s peer review trial. Nature , 10.
Grieneisen, M. L., & Zhang, M. (2012). A comprehensive survey of retracted articles from the scholarly literature. PLoS ONE, 7 (10), e44118.
Article CAS PubMed PubMed Central Google Scholar
Grivell, L. (2006). Through a glass darkly. EMBO Reports, 7 (6), 567–570.
Harrison, C. (2004). Peer review, politics and pluralism. Environmental Science & Policy, 7 (5), 357–368.
Hartog, C. S., Kohl, M., & Reinhart, K. (2011). A systematic review of third-generation hydroxyethyl starch (HES 130/0.4) in resuscitation: Safety not adequately addressed. Anesthesia and Analgesia, 112 (3), 635–645.
Hojat, M., Gonnella, J. S., & Caelleigh, A. S. (2003). Impartial judgment by the “gatekeepers” of science: Fallibility and accountability in the peer review process. Advances in Health Sciences Education, 8 (1), 75–96.
Ioannidis, J. P. A. (2005). Why most published research findings are false. PLoS Med, 2 (8), e124.
James, M. J., Cook-Johnson, R. J., & Cleland, L. G. (2007). Selective COX-2 inhibitors, eicosanoid synthesis and clinical outcomes: A case study of system failure. Lipids, 42 (9), 779–785.
Janssen, S. J., Bredenoord, A. L., Dhert, W., de Kleuver, M., Oner, F. C., & Verlaan, J.-J. (2015). Potential conflicts of interest of editorial board members from five leading spine journals. PLoS ONE, 10 (6), e0127362.
Jefferson, T., Alderson, P., Wager, E., & Davidoff, F. (2002). Effects of editorial peer review: A systematic review. JAMA, 287 (21), 2784–2786.
Jelicic, M., & Merckelbach, H. (2002). Peer-review: Let’s imitate the lawyers! Cortex, 38 (3), 406–407.
Jinha, A. E. (2010). Article 50 million: An estimate of the number of scholarly articles in existence. Learned Publishing, 23 (3), 258–263.
Khan, K. (2010). Is open peer review the fairest system? No. Bmj, 341, c6425.
Kilwein, J. H. (1999). Biases in medical literature. Journal of Clinical Pharmacy and Therapeutics, 24 (6), 393–396.
Koonin, E. V., Landweber, L. F., & Lipman, D. J. (2013). Biology direct: Celebrating 7 years of open, published peer review. Biology direct, 8 (1), 1.
Kozlowski, L. T. (2016). Coping with the conflict-of-interest pandemic by listening to and doubting everyone, including yourself. Science and Engineering Ethics, 22 (2), 591–596.
Krebs, H. A., & Johnson, W. A. (1937). The role of citric acid in intermediate metabolism in animal tissues. Enzymologia, 4, 148–156.
CAS Google Scholar
Kriegeskorte, N., Walther, A., & Deca, D. (2012). An emerging consensus for open evaluation: 18 visions for the future of scientific publishing. Beyond open access: Visions for open evaluation of scientific papers by post-publication peer review , 5.
Langfeldt, L. (2006). The policy challenges of peer review: Managing bias, conflict of interests and interdisciplinary assessments. Research Evaluation, 15 (1), 31–41.
Lawrence, P. A. (2003). The politics of publication. Nature, 422 (6929), 259–261.
Lee, C. J., Sugimoto, C. R., Zhang, G., & Cronin, B. (2013). Bias in peer review. Journal of the American Society for Information Science and Technology, 64 (1), 2–17.
Lippert, S., Callaham, M. L., & Lo, B. (2011). Perceptions of conflict of interest disclosures among peer reviewers. PLoS ONE, 6 (11), e26900.
Link, A. M. (1998). US and non-US submissions: an analysis of reviewer bias. Jama , 280 (3), 246–247.
Lo, B., & Field, M. J. (Eds.). (2009). Conflict of interest in medical research, education, and practice . Washington, D.C.: National Academies Press.
Loonen, M. P. J., Hage, J. J., & Kon, M. (2005). Who benefits from peer review? An analysis of the outcome of 100 requests for review by Plastic and Reconstructive Surgery. Plastic and Reconstructive Surgery, 116 (5), 1461–1472.
Luukkonen, T. (2012). Conservatism and risk-taking in peer review: Emerging ERC practices. Research Evaluation , rvs001.
McClintock, B. (1950). The origin and behavior of mutable loci in maize. Proceedings of the National Academy of Sciences, 36 (6), 344–355.
McCullough, J. (1989). First comprehensive survey of NSF applicants focuses on their concerns about proposal review. Science, Technology and Human Values, 14 (1), 78–88.
McIntyre, W. F., & Evans, G. (2014). The Vioxx ® legacy: Enduring lessons from the not so distant past. Cardiology Journal, 21 (2), 203–205.
Moylan, E. C., Harold, S., O’Neill, C., & Kowalczuk, M. K. (2014). Open, single-blind, double-blind: Which peer review process do you prefer? BMC Pharmacology and Toxicology, 15 (1), 1.
Mulligan, A., Hall, L., & Raphael, E. (2013). Peer review in a changing world: An international study measuring the attitudes of researchers. Journal of the American Society for Information Science and Technology, 64 (1), 132–161.
Nath, S. B., Marcus, S. C., & Druss, B. G. (2006). Retractions in the research literature: misconduct or mistakes? Medical Journal of Australia, 185 (3), 152.
PubMed Google Scholar
Nature Editorial (2008). Working double-blind. Nature, 451, 605–606.
Nature Neuroscience Editorial. (2006). Women in neuroscience: A numbers game. Nature Neuroscience, 9, 853.
Okike, K., Hug, K. T., Kocher, M. S., & Leopold, S. S. (2016). Single-blind vs double-blind peer review in the setting of author prestige. JAMA, 316 (12), 1315–1316.
Olson, C. M., Rennie, D., Cook, D., Dickersin, K., Flanagin, A., Hogan, J. W., … & Pace, B. (2002). Publication bias in editorial decision making. JAMA, 287 (21), 2825–2828.
Palmer, A. R. (2000). Quasireplication and the contract of error: lessons from sex ratios, heritabilities and fluctuating asymmetry. Annual Review of Ecology and Systematics , 441–480.
Peters, D. P., & Ceci, S. J. (1982). Peer-review practices of psychological journals: The fate of published articles, submitted again. Behavioral and Brain Sciences, 5 (02), 187–195.
PLOS MED Editors. (2008). Making sense of non-financial competing interests. PLOS Med, 5 (9), e199.
Pulverer, B. (2010). Transparency showcases strength of peer review. Nature, 468 (7320), 29–31.
Pöschl, U., & Koop, T. (2008). Interactive open access publishing and collaborative peer review for improved scientific communication and quality assurance. Information Services & Use, 28 (2), 105–107.
Relman, A. S. (1985). Dealing with conflicts of interest. New England Journal of Medicine, 313 (12), 749–751.
Rennie, J., & Chief, I. N. (2002). Misleading math about the Earth. Scientific American, 286 (1), 61.
Resch, K. I., Ernst, E., & Garrow, J. (2000). A randomized controlled study of reviewer bias against an unconventional therapy. Journal of the Royal Society of Medicine, 93 (4), 164–167.
CAS PubMed PubMed Central Google Scholar
Resnik, D. B., & Elmore, S. A. (2016). Ensuring the quality, fairness, and integrity of journal peer review: A possible role of editors. Science and Engineering Ethics, 22 (1), 169–188.
Ross, J. S., Gross, C. P., Desai, M. M., Hong, Y., Grant, A. O., Daniels, S. R., et al. (2006). Effect of blinded peer review on abstract acceptance. JAMA, 295 (14), 1675–1680.
Sandström, U. (2009, BRAZIL. JUL 14-17, 2009). Cognitive bias in peer review: A new approach. Paper presented at the 12th International Conference of the International-Society-for-Scientometrics-and-Informetrics.
Shatz, D. (2004). Peer review: A critical inquiry . Lanham, MD: Rowman & Littlefield.
Schneider, L. (2016, September 4). Beall-listed Frontiers empire strikes back. Retrieved from https://forbetterscience.wordpress.com/2016/09/14/beall-listed-frontiers-empire-strikes-back/
Schroter, S., Black, N., Evans, S., Carpenter, J., Godlee, F., & Smith, R. (2004). Effects of training on quality of peer review: Randomised controlled trial. BMJ, 328 (7441), 673.
Service, R. F. (2002). Scientific misconduct. Bell Labs fires star physicist found guilty of forging data. Science (New York, NY), 298 (5591), 30.
Shimp, C. P. (2004). Scientific peer review: A case study from local and global analyses. Journal of the Experimental Analysis of Behavior, 82 (1), 103–116.
Article PubMed Central Google Scholar
Smith, R. (1999). Opening up BMJ peer review: A beginning that should lead to complete transparency. BMJ, 318, 4–5.
Smith, R. (2006). Peer review: A flawed process at the heart of science and journals. Journal of the Royal Society of Medicine, 99 (4), 178–182. doi: 10.1258/jrsm.99.4.178
Souder, L. (2011). The ethics of scholarly peer review: A review of the literature. Learned Publishing, 24 (1), 55–72.
Spielmans, G. I., Biehn, T. L., & Sawrey, D. L. (2009). A case study of salami slicing: pooled analyses of duloxetine for depression. Psychotherapy and Psychosomatics, 79 (2), 97–106.
Spier, R. (2002). The history of the peer-review process. Trends in Biotechnology, 20 (8), 357–358.
Squazzoni, F. (2010). Peering into peer review. Sociologica, 4 (3).
Squazzoni, F., & Gandelli, C. (2012). Saint Matthew strikes again: An agent-based model of peer review and the scientific community structure. Journal of Informetrics, 6 (2), 265–275.
Steen, R. G. (2010). Retractions in the scientific literature: is the incidence of research fraud increasing? Journal of Medical Ethics , jme-2010.
Stroebe, W., Postmes, T., & Spears, R. (2012). Scientific misconduct and the myth of self-correction in science. Perspectives on Psychological Science, 7 (6), 670–688.
Tite, L., & Schroter, S. (2007). Why do peer reviewers decline to review? A survey. Journal of Epidemiology and Community Health, 61 (1), 9–12.
Travis, G. D. L., & Collins, H. M. (1991). New light on old boys: cognitive and institutional particularism in the peer review system. Science, Technology and Human Values, 16 (3), 322–341.
Tregenza, T. (2002). Gender bias in the refereeing process? Trends in Ecology & Evolution, 17 (8), 349–350.
Valkonen, L., & Brooks, J. (2011). Gender balance in Cortex acceptance rates. Cortex, 47 (7), 763–770.
van Rooyen, S., Delamothe, T., & Evans, S. J. W. (2010). Effect on peer review of telling reviewers that their signed reviews might be posted on the web: Randomised controlled trial. BMJ, 341, c5729.
van Rooyen, S., Godlee, F., Evans, S., Black, N., & Smith, R. (1999). Effect of open peer review on quality of reviews and on reviewers’ recommendations: A randomised trial. British Medical Journal, 318 (7175), 23–27.
Walker, R., & Rocha da Silva, P. (2014). Emerging trends in peer review—A survey. Frontiers in neuroscience, 9, 169.
Walsh, E., Rooney, M., Appleby, L., & Wilkinson, G. (2000). Open peer review: A randomised controlled trial. The British Journal of Psychiatry, 176 (1), 47–51.
Walters, W. P., & Bajorath, J. (2015). On the evolving open peer review culture for chemical information science. F1000Research, 4 .
Ware, M. (2008). Peer review in scholarly journals: Perspective of the scholarly community-Results from an international study. Information Services and Use, 28 (2), 109–112.
Ware, M. (2011). Peer review: Recent experience and future directions. New Review of Information Networking , 16 (1), 23–53.
Webb, T. J., O’Hara, B., & Freckleton, R. P. (2008). Does double-blind review benefit female authors? Heredity, 77, 282–291.
Wellington, J., & Nixon, J. (2005). Shaping the field: The role of academic journal editors in the construction of education as a field of study. British Journal of Sociology of Education, 26 (5), 643–655.
Whittaker, R. J. (2008). Journal review and gender equality: A critical comment on Budden et al. Trends in Ecology & Evolution, 23 (9), 478–479.
Wiedermann, C. J. (2016). Ethical publishing in intensive care medicine: A narrative review. World Journal of Critical Care Medicine, 5 (3), 171.
Download references
Author information
Authors and affiliations.
Murray State University, Murray, Kentucky, USA
Pali U. K. De Silva & Candace K. Vance
You can also search for this author in PubMed Google Scholar
Corresponding author
Correspondence to Pali U. K. De Silva .
Rights and permissions
Reprints and permissions
Copyright information
© 2017 Springer International Publishing AG
About this chapter
De Silva, P.U.K., K. Vance, C. (2017). Preserving the Quality of Scientific Research: Peer Review of Research Articles. In: Scientific Scholarly Communication. Fascinating Life Sciences. Springer, Cham. https://doi.org/10.1007/978-3-319-50627-2_6
Download citation
DOI : https://doi.org/10.1007/978-3-319-50627-2_6
Published : 20 January 2017
Publisher Name : Springer, Cham
Print ISBN : 978-3-319-50626-5
Online ISBN : 978-3-319-50627-2
eBook Packages : Biomedical and Life Sciences Biomedical and Life Sciences (R0)
Share this chapter
Anyone you share the following link with will be able to read this content:
Sorry, a shareable link is not currently available for this article.
Provided by the Springer Nature SharedIt content-sharing initiative
- Publish with us
Policies and ethics
- Find a journal
- Track your research
Peer Review in Scientific Publications: Benefits, Critiques, & A Survival Guide
Affiliations.
- 1 Clinical Biochemistry, Department of Pediatric Laboratory Medicine, The Hospital for Sick Children, University of Toronto , Toronto, Ontario, Canada.
- 2 Clinical Biochemistry, Department of Pediatric Laboratory Medicine, The Hospital for Sick Children, University of Toronto, Toronto, Ontario, Canada; Department of Laboratory Medicine and Pathobiology, University of Toronto, Toronto, Canada; Chair, Communications and Publications Division (CPD), International Federation for Sick Clinical Chemistry (IFCC), Milan, Italy.
- PMID: 27683470
- PMCID: PMC4975196
Peer review has been defined as a process of subjecting an author's scholarly work, research or ideas to the scrutiny of others who are experts in the same field. It functions to encourage authors to meet the accepted high standards of their discipline and to control the dissemination of research data to ensure that unwarranted claims, unacceptable interpretations or personal views are not published without prior expert review. Despite its wide-spread use by most journals, the peer review process has also been widely criticised due to the slowness of the process to publish new findings and due to perceived bias by the editors and/or reviewers. Within the scientific community, peer review has become an essential component of the academic writing process. It helps ensure that papers published in scientific journals answer meaningful research questions and draw accurate conclusions based on professionally executed experimentation. Submission of low quality manuscripts has become increasingly prevalent, and peer review acts as a filter to prevent this work from reaching the scientific community. The major advantage of a peer review process is that peer-reviewed articles provide a trusted form of scientific communication. Since scientific knowledge is cumulative and builds on itself, this trust is particularly important. Despite the positive impacts of peer review, critics argue that the peer review process stifles innovation in experimentation, and acts as a poor screen against plagiarism. Despite its downfalls, there has not yet been a foolproof system developed to take the place of peer review, however, researchers have been looking into electronic means of improving the peer review process. Unfortunately, the recent explosion in online only/electronic journals has led to mass publication of a large number of scientific articles with little or no peer review. This poses significant risk to advances in scientific knowledge and its future potential. The current article summarizes the peer review process, highlights the pros and cons associated with different types of peer review, and describes new methods for improving peer review.
Keywords: journal; manuscript; open access; peer review; publication.
Thank you for visiting nature.com. You are using a browser version with limited support for CSS. To obtain the best experience, we recommend you use a more up to date browser (or turn off compatibility mode in Internet Explorer). In the meantime, to ensure continued support, we are displaying the site without styles and JavaScript.
- View all journals
- My Account Login
- Explore content
- About the journal
- Publish with us
- Sign up for alerts
- Open access
- Published: 26 March 2024
Predicting and improving complex beer flavor through machine learning
- Michiel Schreurs ORCID: orcid.org/0000-0002-9449-5619 1 , 2 , 3 na1 ,
- Supinya Piampongsant 1 , 2 , 3 na1 ,
- Miguel Roncoroni ORCID: orcid.org/0000-0001-7461-1427 1 , 2 , 3 na1 ,
- Lloyd Cool ORCID: orcid.org/0000-0001-9936-3124 1 , 2 , 3 , 4 ,
- Beatriz Herrera-Malaver ORCID: orcid.org/0000-0002-5096-9974 1 , 2 , 3 ,
- Christophe Vanderaa ORCID: orcid.org/0000-0001-7443-5427 4 ,
- Florian A. Theßeling 1 , 2 , 3 ,
- Łukasz Kreft ORCID: orcid.org/0000-0001-7620-4657 5 ,
- Alexander Botzki ORCID: orcid.org/0000-0001-6691-4233 5 ,
- Philippe Malcorps 6 ,
- Luk Daenen 6 ,
- Tom Wenseleers ORCID: orcid.org/0000-0002-1434-861X 4 &
- Kevin J. Verstrepen ORCID: orcid.org/0000-0002-3077-6219 1 , 2 , 3
Nature Communications volume 15 , Article number: 2368 ( 2024 ) Cite this article
53k Accesses
861 Altmetric
Metrics details
- Chemical engineering
- Gas chromatography
- Machine learning
- Metabolomics
- Taste receptors
The perception and appreciation of food flavor depends on many interacting chemical compounds and external factors, and therefore proves challenging to understand and predict. Here, we combine extensive chemical and sensory analyses of 250 different beers to train machine learning models that allow predicting flavor and consumer appreciation. For each beer, we measure over 200 chemical properties, perform quantitative descriptive sensory analysis with a trained tasting panel and map data from over 180,000 consumer reviews to train 10 different machine learning models. The best-performing algorithm, Gradient Boosting, yields models that significantly outperform predictions based on conventional statistics and accurately predict complex food features and consumer appreciation from chemical profiles. Model dissection allows identifying specific and unexpected compounds as drivers of beer flavor and appreciation. Adding these compounds results in variants of commercial alcoholic and non-alcoholic beers with improved consumer appreciation. Together, our study reveals how big data and machine learning uncover complex links between food chemistry, flavor and consumer perception, and lays the foundation to develop novel, tailored foods with superior flavors.
Similar content being viewed by others
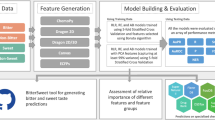
BitterSweet: Building machine learning models for predicting the bitter and sweet taste of small molecules
Rudraksh Tuwani, Somin Wadhwa & Ganesh Bagler
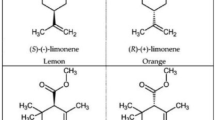
Predicting odor from molecular structure: a multi-label classification approach
Kushagra Saini & Venkatnarayan Ramanathan
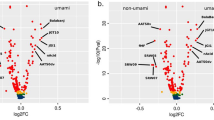
Toward a general and interpretable umami taste predictor using a multi-objective machine learning approach
Lorenzo Pallante, Aigli Korfiati, … Marco A. Deriu
Introduction
Predicting and understanding food perception and appreciation is one of the major challenges in food science. Accurate modeling of food flavor and appreciation could yield important opportunities for both producers and consumers, including quality control, product fingerprinting, counterfeit detection, spoilage detection, and the development of new products and product combinations (food pairing) 1 , 2 , 3 , 4 , 5 , 6 . Accurate models for flavor and consumer appreciation would contribute greatly to our scientific understanding of how humans perceive and appreciate flavor. Moreover, accurate predictive models would also facilitate and standardize existing food assessment methods and could supplement or replace assessments by trained and consumer tasting panels, which are variable, expensive and time-consuming 7 , 8 , 9 . Lastly, apart from providing objective, quantitative, accurate and contextual information that can help producers, models can also guide consumers in understanding their personal preferences 10 .
Despite the myriad of applications, predicting food flavor and appreciation from its chemical properties remains a largely elusive goal in sensory science, especially for complex food and beverages 11 , 12 . A key obstacle is the immense number of flavor-active chemicals underlying food flavor. Flavor compounds can vary widely in chemical structure and concentration, making them technically challenging and labor-intensive to quantify, even in the face of innovations in metabolomics, such as non-targeted metabolic fingerprinting 13 , 14 . Moreover, sensory analysis is perhaps even more complicated. Flavor perception is highly complex, resulting from hundreds of different molecules interacting at the physiochemical and sensorial level. Sensory perception is often non-linear, characterized by complex and concentration-dependent synergistic and antagonistic effects 15 , 16 , 17 , 18 , 19 , 20 , 21 that are further convoluted by the genetics, environment, culture and psychology of consumers 22 , 23 , 24 . Perceived flavor is therefore difficult to measure, with problems of sensitivity, accuracy, and reproducibility that can only be resolved by gathering sufficiently large datasets 25 . Trained tasting panels are considered the prime source of quality sensory data, but require meticulous training, are low throughput and high cost. Public databases containing consumer reviews of food products could provide a valuable alternative, especially for studying appreciation scores, which do not require formal training 25 . Public databases offer the advantage of amassing large amounts of data, increasing the statistical power to identify potential drivers of appreciation. However, public datasets suffer from biases, including a bias in the volunteers that contribute to the database, as well as confounding factors such as price, cult status and psychological conformity towards previous ratings of the product.
Classical multivariate statistics and machine learning methods have been used to predict flavor of specific compounds by, for example, linking structural properties of a compound to its potential biological activities or linking concentrations of specific compounds to sensory profiles 1 , 26 . Importantly, most previous studies focused on predicting organoleptic properties of single compounds (often based on their chemical structure) 27 , 28 , 29 , 30 , 31 , 32 , 33 , thus ignoring the fact that these compounds are present in a complex matrix in food or beverages and excluding complex interactions between compounds. Moreover, the classical statistics commonly used in sensory science 34 , 35 , 36 , 37 , 38 , 39 require a large sample size and sufficient variance amongst predictors to create accurate models. They are not fit for studying an extensive set of hundreds of interacting flavor compounds, since they are sensitive to outliers, have a high tendency to overfit and are less suited for non-linear and discontinuous relationships 40 .
In this study, we combine extensive chemical analyses and sensory data of a set of different commercial beers with machine learning approaches to develop models that predict taste, smell, mouthfeel and appreciation from compound concentrations. Beer is particularly suited to model the relationship between chemistry, flavor and appreciation. First, beer is a complex product, consisting of thousands of flavor compounds that partake in complex sensory interactions 41 , 42 , 43 . This chemical diversity arises from the raw materials (malt, yeast, hops, water and spices) and biochemical conversions during the brewing process (kilning, mashing, boiling, fermentation, maturation and aging) 44 , 45 . Second, the advent of the internet saw beer consumers embrace online review platforms, such as RateBeer (ZX Ventures, Anheuser-Busch InBev SA/NV) and BeerAdvocate (Next Glass, inc.). In this way, the beer community provides massive data sets of beer flavor and appreciation scores, creating extraordinarily large sensory databases to complement the analyses of our professional sensory panel. Specifically, we characterize over 200 chemical properties of 250 commercial beers, spread across 22 beer styles, and link these to the descriptive sensory profiling data of a 16-person in-house trained tasting panel and data acquired from over 180,000 public consumer reviews. These unique and extensive datasets enable us to train a suite of machine learning models to predict flavor and appreciation from a beer’s chemical profile. Dissection of the best-performing models allows us to pinpoint specific compounds as potential drivers of beer flavor and appreciation. Follow-up experiments confirm the importance of these compounds and ultimately allow us to significantly improve the flavor and appreciation of selected commercial beers. Together, our study represents a significant step towards understanding complex flavors and reinforces the value of machine learning to develop and refine complex foods. In this way, it represents a stepping stone for further computer-aided food engineering applications 46 .
To generate a comprehensive dataset on beer flavor, we selected 250 commercial Belgian beers across 22 different beer styles (Supplementary Fig. S1 ). Beers with ≤ 4.2% alcohol by volume (ABV) were classified as non-alcoholic and low-alcoholic. Blonds and Tripels constitute a significant portion of the dataset (12.4% and 11.2%, respectively) reflecting their presence on the Belgian beer market and the heterogeneity of beers within these styles. By contrast, lager beers are less diverse and dominated by a handful of brands. Rare styles such as Brut or Faro make up only a small fraction of the dataset (2% and 1%, respectively) because fewer of these beers are produced and because they are dominated by distinct characteristics in terms of flavor and chemical composition.
Extensive analysis identifies relationships between chemical compounds in beer
For each beer, we measured 226 different chemical properties, including common brewing parameters such as alcohol content, iso-alpha acids, pH, sugar concentration 47 , and over 200 flavor compounds (Methods, Supplementary Table S1 ). A large portion (37.2%) are terpenoids arising from hopping, responsible for herbal and fruity flavors 16 , 48 . A second major category are yeast metabolites, such as esters and alcohols, that result in fruity and solvent notes 48 , 49 , 50 . Other measured compounds are primarily derived from malt, or other microbes such as non- Saccharomyces yeasts and bacteria (‘wild flora’). Compounds that arise from spices or staling are labeled under ‘Others’. Five attributes (caloric value, total acids and total ester, hop aroma and sulfur compounds) are calculated from multiple individually measured compounds.
As a first step in identifying relationships between chemical properties, we determined correlations between the concentrations of the compounds (Fig. 1 , upper panel, Supplementary Data 1 and 2 , and Supplementary Fig. S2 . For the sake of clarity, only a subset of the measured compounds is shown in Fig. 1 ). Compounds of the same origin typically show a positive correlation, while absence of correlation hints at parameters varying independently. For example, the hop aroma compounds citronellol, and alpha-terpineol show moderate correlations with each other (Spearman’s rho=0.39 and 0.57), but not with the bittering hop component iso-alpha acids (Spearman’s rho=0.16 and −0.07). This illustrates how brewers can independently modify hop aroma and bitterness by selecting hop varieties and dosage time. If hops are added early in the boiling phase, chemical conversions increase bitterness while aromas evaporate, conversely, late addition of hops preserves aroma but limits bitterness 51 . Similarly, hop-derived iso-alpha acids show a strong anti-correlation with lactic acid and acetic acid, likely reflecting growth inhibition of lactic acid and acetic acid bacteria, or the consequent use of fewer hops in sour beer styles, such as West Flanders ales and Fruit beers, that rely on these bacteria for their distinct flavors 52 . Finally, yeast-derived esters (ethyl acetate, ethyl decanoate, ethyl hexanoate, ethyl octanoate) and alcohols (ethanol, isoamyl alcohol, isobutanol, and glycerol), correlate with Spearman coefficients above 0.5, suggesting that these secondary metabolites are correlated with the yeast genetic background and/or fermentation parameters and may be difficult to influence individually, although the choice of yeast strain may offer some control 53 .
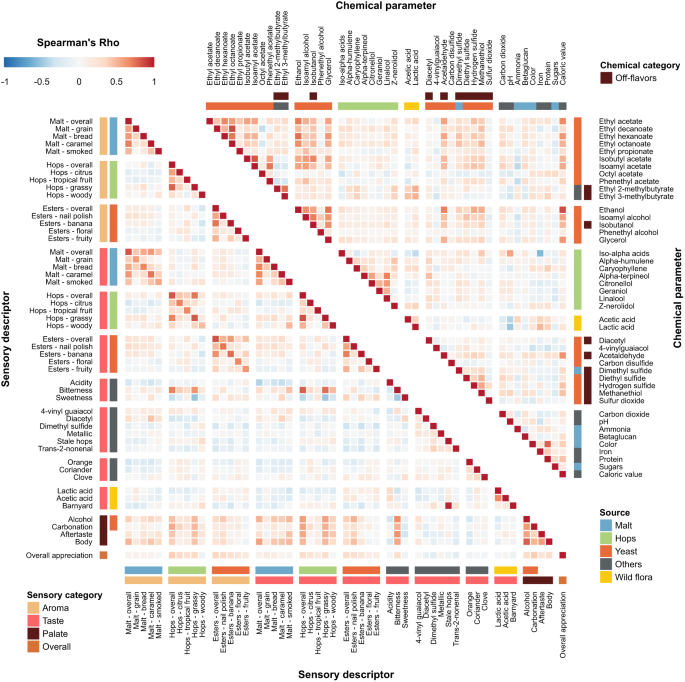
Spearman rank correlations are shown. Descriptors are grouped according to their origin (malt (blue), hops (green), yeast (red), wild flora (yellow), Others (black)), and sensory aspect (aroma, taste, palate, and overall appreciation). Please note that for the chemical compounds, for the sake of clarity, only a subset of the total number of measured compounds is shown, with an emphasis on the key compounds for each source. For more details, see the main text and Methods section. Chemical data can be found in Supplementary Data 1 , correlations between all chemical compounds are depicted in Supplementary Fig. S2 and correlation values can be found in Supplementary Data 2 . See Supplementary Data 4 for sensory panel assessments and Supplementary Data 5 for correlation values between all sensory descriptors.
Interestingly, different beer styles show distinct patterns for some flavor compounds (Supplementary Fig. S3 ). These observations agree with expectations for key beer styles, and serve as a control for our measurements. For instance, Stouts generally show high values for color (darker), while hoppy beers contain elevated levels of iso-alpha acids, compounds associated with bitter hop taste. Acetic and lactic acid are not prevalent in most beers, with notable exceptions such as Kriek, Lambic, Faro, West Flanders ales and Flanders Old Brown, which use acid-producing bacteria ( Lactobacillus and Pediococcus ) or unconventional yeast ( Brettanomyces ) 54 , 55 . Glycerol, ethanol and esters show similar distributions across all beer styles, reflecting their common origin as products of yeast metabolism during fermentation 45 , 53 . Finally, low/no-alcohol beers contain low concentrations of glycerol and esters. This is in line with the production process for most of the low/no-alcohol beers in our dataset, which are produced through limiting fermentation or by stripping away alcohol via evaporation or dialysis, with both methods having the unintended side-effect of reducing the amount of flavor compounds in the final beer 56 , 57 .
Besides expected associations, our data also reveals less trivial associations between beer styles and specific parameters. For example, geraniol and citronellol, two monoterpenoids responsible for citrus, floral and rose flavors and characteristic of Citra hops, are found in relatively high amounts in Christmas, Saison, and Brett/co-fermented beers, where they may originate from terpenoid-rich spices such as coriander seeds instead of hops 58 .
Tasting panel assessments reveal sensorial relationships in beer
To assess the sensory profile of each beer, a trained tasting panel evaluated each of the 250 beers for 50 sensory attributes, including different hop, malt and yeast flavors, off-flavors and spices. Panelists used a tasting sheet (Supplementary Data 3 ) to score the different attributes. Panel consistency was evaluated by repeating 12 samples across different sessions and performing ANOVA. In 95% of cases no significant difference was found across sessions ( p > 0.05), indicating good panel consistency (Supplementary Table S2 ).
Aroma and taste perception reported by the trained panel are often linked (Fig. 1 , bottom left panel and Supplementary Data 4 and 5 ), with high correlations between hops aroma and taste (Spearman’s rho=0.83). Bitter taste was found to correlate with hop aroma and taste in general (Spearman’s rho=0.80 and 0.69), and particularly with “grassy” noble hops (Spearman’s rho=0.75). Barnyard flavor, most often associated with sour beers, is identified together with stale hops (Spearman’s rho=0.97) that are used in these beers. Lactic and acetic acid, which often co-occur, are correlated (Spearman’s rho=0.66). Interestingly, sweetness and bitterness are anti-correlated (Spearman’s rho = −0.48), confirming the hypothesis that they mask each other 59 , 60 . Beer body is highly correlated with alcohol (Spearman’s rho = 0.79), and overall appreciation is found to correlate with multiple aspects that describe beer mouthfeel (alcohol, carbonation; Spearman’s rho= 0.32, 0.39), as well as with hop and ester aroma intensity (Spearman’s rho=0.39 and 0.35).
Similar to the chemical analyses, sensorial analyses confirmed typical features of specific beer styles (Supplementary Fig. S4 ). For example, sour beers (Faro, Flanders Old Brown, Fruit beer, Kriek, Lambic, West Flanders ale) were rated acidic, with flavors of both acetic and lactic acid. Hoppy beers were found to be bitter and showed hop-associated aromas like citrus and tropical fruit. Malt taste is most detected among scotch, stout/porters, and strong ales, while low/no-alcohol beers, which often have a reputation for being ‘worty’ (reminiscent of unfermented, sweet malt extract) appear in the middle. Unsurprisingly, hop aromas are most strongly detected among hoppy beers. Like its chemical counterpart (Supplementary Fig. S3 ), acidity shows a right-skewed distribution, with the most acidic beers being Krieks, Lambics, and West Flanders ales.
Tasting panel assessments of specific flavors correlate with chemical composition
We find that the concentrations of several chemical compounds strongly correlate with specific aroma or taste, as evaluated by the tasting panel (Fig. 2 , Supplementary Fig. S5 , Supplementary Data 6 ). In some cases, these correlations confirm expectations and serve as a useful control for data quality. For example, iso-alpha acids, the bittering compounds in hops, strongly correlate with bitterness (Spearman’s rho=0.68), while ethanol and glycerol correlate with tasters’ perceptions of alcohol and body, the mouthfeel sensation of fullness (Spearman’s rho=0.82/0.62 and 0.72/0.57 respectively) and darker color from roasted malts is a good indication of malt perception (Spearman’s rho=0.54).
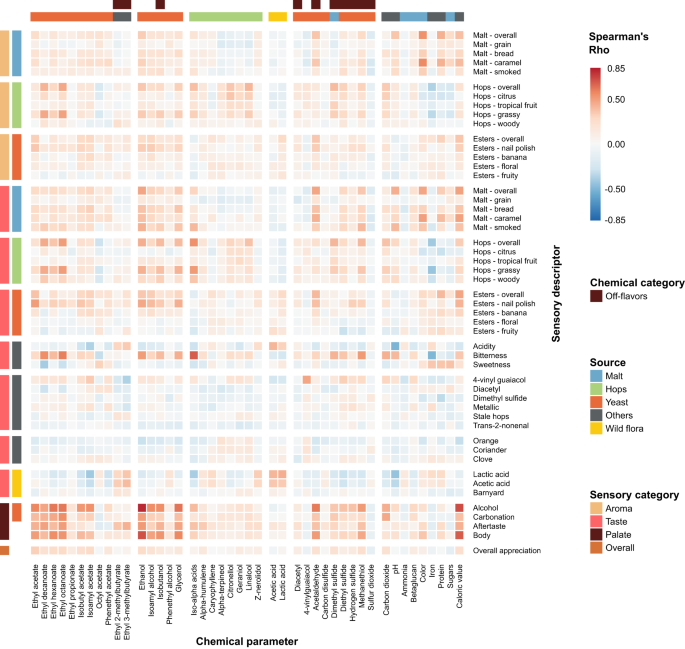
Heatmap colors indicate Spearman’s Rho. Axes are organized according to sensory categories (aroma, taste, mouthfeel, overall), chemical categories and chemical sources in beer (malt (blue), hops (green), yeast (red), wild flora (yellow), Others (black)). See Supplementary Data 6 for all correlation values.
Interestingly, for some relationships between chemical compounds and perceived flavor, correlations are weaker than expected. For example, the rose-smelling phenethyl acetate only weakly correlates with floral aroma. This hints at more complex relationships and interactions between compounds and suggests a need for a more complex model than simple correlations. Lastly, we uncovered unexpected correlations. For instance, the esters ethyl decanoate and ethyl octanoate appear to correlate slightly with hop perception and bitterness, possibly due to their fruity flavor. Iron is anti-correlated with hop aromas and bitterness, most likely because it is also anti-correlated with iso-alpha acids. This could be a sign of metal chelation of hop acids 61 , given that our analyses measure unbound hop acids and total iron content, or could result from the higher iron content in dark and Fruit beers, which typically have less hoppy and bitter flavors 62 .
Public consumer reviews complement expert panel data
To complement and expand the sensory data of our trained tasting panel, we collected 180,000 reviews of our 250 beers from the online consumer review platform RateBeer. This provided numerical scores for beer appearance, aroma, taste, palate, overall quality as well as the average overall score.
Public datasets are known to suffer from biases, such as price, cult status and psychological conformity towards previous ratings of a product. For example, prices correlate with appreciation scores for these online consumer reviews (rho=0.49, Supplementary Fig. S6 ), but not for our trained tasting panel (rho=0.19). This suggests that prices affect consumer appreciation, which has been reported in wine 63 , while blind tastings are unaffected. Moreover, we observe that some beer styles, like lagers and non-alcoholic beers, generally receive lower scores, reflecting that online reviewers are mostly beer aficionados with a preference for specialty beers over lager beers. In general, we find a modest correlation between our trained panel’s overall appreciation score and the online consumer appreciation scores (Fig. 3 , rho=0.29). Apart from the aforementioned biases in the online datasets, serving temperature, sample freshness and surroundings, which are all tightly controlled during the tasting panel sessions, can vary tremendously across online consumers and can further contribute to (among others, appreciation) differences between the two categories of tasters. Importantly, in contrast to the overall appreciation scores, for many sensory aspects the results from the professional panel correlated well with results obtained from RateBeer reviews. Correlations were highest for features that are relatively easy to recognize even for untrained tasters, like bitterness, sweetness, alcohol and malt aroma (Fig. 3 and below).
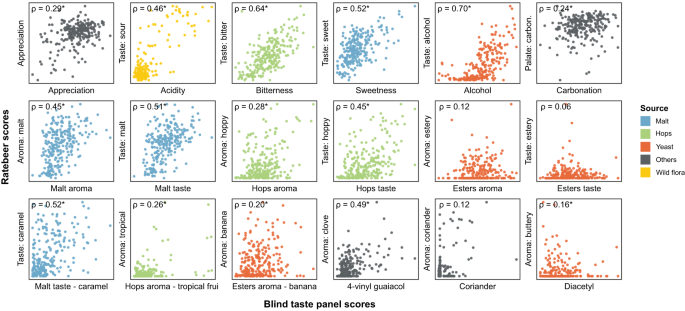
RateBeer text mining results can be found in Supplementary Data 7 . Rho values shown are Spearman correlation values, with asterisks indicating significant correlations ( p < 0.05, two-sided). All p values were smaller than 0.001, except for Esters aroma (0.0553), Esters taste (0.3275), Esters aroma—banana (0.0019), Coriander (0.0508) and Diacetyl (0.0134).
Besides collecting consumer appreciation from these online reviews, we developed automated text analysis tools to gather additional data from review texts (Supplementary Data 7 ). Processing review texts on the RateBeer database yielded comparable results to the scores given by the trained panel for many common sensory aspects, including acidity, bitterness, sweetness, alcohol, malt, and hop tastes (Fig. 3 ). This is in line with what would be expected, since these attributes require less training for accurate assessment and are less influenced by environmental factors such as temperature, serving glass and odors in the environment. Consumer reviews also correlate well with our trained panel for 4-vinyl guaiacol, a compound associated with a very characteristic aroma. By contrast, correlations for more specific aromas like ester, coriander or diacetyl are underrepresented in the online reviews, underscoring the importance of using a trained tasting panel and standardized tasting sheets with explicit factors to be scored for evaluating specific aspects of a beer. Taken together, our results suggest that public reviews are trustworthy for some, but not all, flavor features and can complement or substitute taste panel data for these sensory aspects.
Models can predict beer sensory profiles from chemical data
The rich datasets of chemical analyses, tasting panel assessments and public reviews gathered in the first part of this study provided us with a unique opportunity to develop predictive models that link chemical data to sensorial features. Given the complexity of beer flavor, basic statistical tools such as correlations or linear regression may not always be the most suitable for making accurate predictions. Instead, we applied different machine learning models that can model both simple linear and complex interactive relationships. Specifically, we constructed a set of regression models to predict (a) trained panel scores for beer flavor and quality and (b) public reviews’ appreciation scores from beer chemical profiles. We trained and tested 10 different models (Methods), 3 linear regression-based models (simple linear regression with first-order interactions (LR), lasso regression with first-order interactions (Lasso), partial least squares regressor (PLSR)), 5 decision tree models (AdaBoost regressor (ABR), extra trees (ET), gradient boosting regressor (GBR), random forest (RF) and XGBoost regressor (XGBR)), 1 support vector regression (SVR), and 1 artificial neural network (ANN) model.
To compare the performance of our machine learning models, the dataset was randomly split into a training and test set, stratified by beer style. After a model was trained on data in the training set, its performance was evaluated on its ability to predict the test dataset obtained from multi-output models (based on the coefficient of determination, see Methods). Additionally, individual-attribute models were ranked per descriptor and the average rank was calculated, as proposed by Korneva et al. 64 . Importantly, both ways of evaluating the models’ performance agreed in general. Performance of the different models varied (Table 1 ). It should be noted that all models perform better at predicting RateBeer results than results from our trained tasting panel. One reason could be that sensory data is inherently variable, and this variability is averaged out with the large number of public reviews from RateBeer. Additionally, all tree-based models perform better at predicting taste than aroma. Linear models (LR) performed particularly poorly, with negative R 2 values, due to severe overfitting (training set R 2 = 1). Overfitting is a common issue in linear models with many parameters and limited samples, especially with interaction terms further amplifying the number of parameters. L1 regularization (Lasso) successfully overcomes this overfitting, out-competing multiple tree-based models on the RateBeer dataset. Similarly, the dimensionality reduction of PLSR avoids overfitting and improves performance, to some extent. Still, tree-based models (ABR, ET, GBR, RF and XGBR) show the best performance, out-competing the linear models (LR, Lasso, PLSR) commonly used in sensory science 65 .
GBR models showed the best overall performance in predicting sensory responses from chemical information, with R 2 values up to 0.75 depending on the predicted sensory feature (Supplementary Table S4 ). The GBR models predict consumer appreciation (RateBeer) better than our trained panel’s appreciation (R 2 value of 0.67 compared to R 2 value of 0.09) (Supplementary Table S3 and Supplementary Table S4 ). ANN models showed intermediate performance, likely because neural networks typically perform best with larger datasets 66 . The SVR shows intermediate performance, mostly due to the weak predictions of specific attributes that lower the overall performance (Supplementary Table S4 ).
Model dissection identifies specific, unexpected compounds as drivers of consumer appreciation
Next, we leveraged our models to infer important contributors to sensory perception and consumer appreciation. Consumer preference is a crucial sensory aspects, because a product that shows low consumer appreciation scores often does not succeed commercially 25 . Additionally, the requirement for a large number of representative evaluators makes consumer trials one of the more costly and time-consuming aspects of product development. Hence, a model for predicting chemical drivers of overall appreciation would be a welcome addition to the available toolbox for food development and optimization.
Since GBR models on our RateBeer dataset showed the best overall performance, we focused on these models. Specifically, we used two approaches to identify important contributors. First, rankings of the most important predictors for each sensorial trait in the GBR models were obtained based on impurity-based feature importance (mean decrease in impurity). High-ranked parameters were hypothesized to be either the true causal chemical properties underlying the trait, to correlate with the actual causal properties, or to take part in sensory interactions affecting the trait 67 (Fig. 4A ). In a second approach, we used SHAP 68 to determine which parameters contributed most to the model for making predictions of consumer appreciation (Fig. 4B ). SHAP calculates parameter contributions to model predictions on a per-sample basis, which can be aggregated into an importance score.
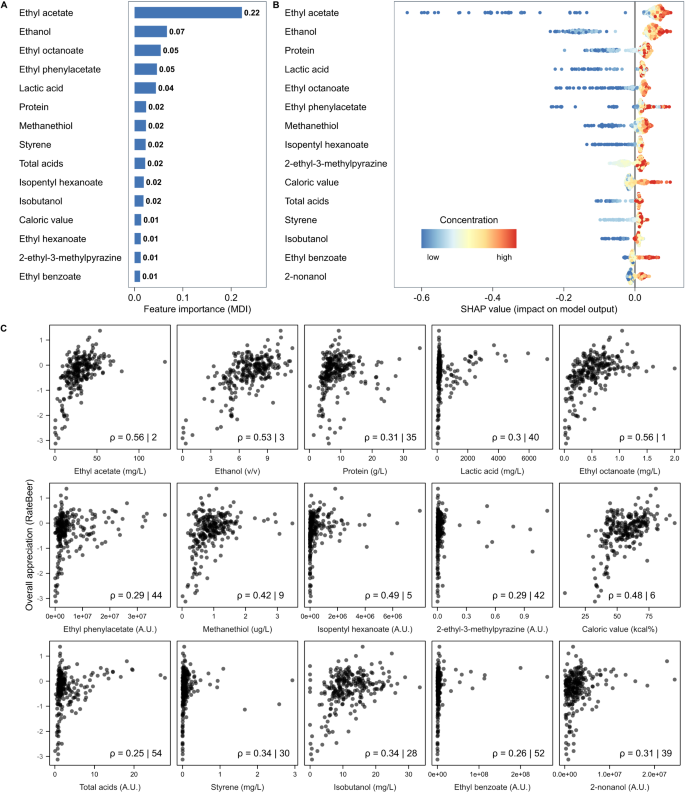
A The impurity-based feature importance (mean deviance in impurity, MDI) calculated from the Gradient Boosting Regression (GBR) model predicting RateBeer appreciation scores. The top 15 highest ranked chemical properties are shown. B SHAP summary plot for the top 15 parameters contributing to our GBR model. Each point on the graph represents a sample from our dataset. The color represents the concentration of that parameter, with bluer colors representing low values and redder colors representing higher values. Greater absolute values on the horizontal axis indicate a higher impact of the parameter on the prediction of the model. C Spearman correlations between the 15 most important chemical properties and consumer overall appreciation. Numbers indicate the Spearman Rho correlation coefficient, and the rank of this correlation compared to all other correlations. The top 15 important compounds were determined using SHAP (panel B).
Both approaches identified ethyl acetate as the most predictive parameter for beer appreciation (Fig. 4 ). Ethyl acetate is the most abundant ester in beer with a typical ‘fruity’, ‘solvent’ and ‘alcoholic’ flavor, but is often considered less important than other esters like isoamyl acetate. The second most important parameter identified by SHAP is ethanol, the most abundant beer compound after water. Apart from directly contributing to beer flavor and mouthfeel, ethanol drastically influences the physical properties of beer, dictating how easily volatile compounds escape the beer matrix to contribute to beer aroma 69 . Importantly, it should also be noted that the importance of ethanol for appreciation is likely inflated by the very low appreciation scores of non-alcoholic beers (Supplementary Fig. S4 ). Despite not often being considered a driver of beer appreciation, protein level also ranks highly in both approaches, possibly due to its effect on mouthfeel and body 70 . Lactic acid, which contributes to the tart taste of sour beers, is the fourth most important parameter identified by SHAP, possibly due to the generally high appreciation of sour beers in our dataset.
Interestingly, some of the most important predictive parameters for our model are not well-established as beer flavors or are even commonly regarded as being negative for beer quality. For example, our models identify methanethiol and ethyl phenyl acetate, an ester commonly linked to beer staling 71 , as a key factor contributing to beer appreciation. Although there is no doubt that high concentrations of these compounds are considered unpleasant, the positive effects of modest concentrations are not yet known 72 , 73 .
To compare our approach to conventional statistics, we evaluated how well the 15 most important SHAP-derived parameters correlate with consumer appreciation (Fig. 4C ). Interestingly, only 6 of the properties derived by SHAP rank amongst the top 15 most correlated parameters. For some chemical compounds, the correlations are so low that they would have likely been considered unimportant. For example, lactic acid, the fourth most important parameter, shows a bimodal distribution for appreciation, with sour beers forming a separate cluster, that is missed entirely by the Spearman correlation. Additionally, the correlation plots reveal outliers, emphasizing the need for robust analysis tools. Together, this highlights the need for alternative models, like the Gradient Boosting model, that better grasp the complexity of (beer) flavor.
Finally, to observe the relationships between these chemical properties and their predicted targets, partial dependence plots were constructed for the six most important predictors of consumer appreciation 74 , 75 , 76 (Supplementary Fig. S7 ). One-way partial dependence plots show how a change in concentration affects the predicted appreciation. These plots reveal an important limitation of our models: appreciation predictions remain constant at ever-increasing concentrations. This implies that once a threshold concentration is reached, further increasing the concentration does not affect appreciation. This is false, as it is well-documented that certain compounds become unpleasant at high concentrations, including ethyl acetate (‘nail polish’) 77 and methanethiol (‘sulfury’ and ‘rotten cabbage’) 78 . The inability of our models to grasp that flavor compounds have optimal levels, above which they become negative, is a consequence of working with commercial beer brands where (off-)flavors are rarely too high to negatively impact the product. The two-way partial dependence plots show how changing the concentration of two compounds influences predicted appreciation, visualizing their interactions (Supplementary Fig. S7 ). In our case, the top 5 parameters are dominated by additive or synergistic interactions, with high concentrations for both compounds resulting in the highest predicted appreciation.
To assess the robustness of our best-performing models and model predictions, we performed 100 iterations of the GBR, RF and ET models. In general, all iterations of the models yielded similar performance (Supplementary Fig. S8 ). Moreover, the main predictors (including the top predictors ethanol and ethyl acetate) remained virtually the same, especially for GBR and RF. For the iterations of the ET model, we did observe more variation in the top predictors, which is likely a consequence of the model’s inherent random architecture in combination with co-correlations between certain predictors. However, even in this case, several of the top predictors (ethanol and ethyl acetate) remain unchanged, although their rank in importance changes (Supplementary Fig. S8 ).
Next, we investigated if a combination of RateBeer and trained panel data into one consolidated dataset would lead to stronger models, under the hypothesis that such a model would suffer less from bias in the datasets. A GBR model was trained to predict appreciation on the combined dataset. This model underperformed compared to the RateBeer model, both in the native case and when including a dataset identifier (R 2 = 0.67, 0.26 and 0.42 respectively). For the latter, the dataset identifier is the most important feature (Supplementary Fig. S9 ), while most of the feature importance remains unchanged, with ethyl acetate and ethanol ranking highest, like in the original model trained only on RateBeer data. It seems that the large variation in the panel dataset introduces noise, weakening the models’ performances and reliability. In addition, it seems reasonable to assume that both datasets are fundamentally different, with the panel dataset obtained by blind tastings by a trained professional panel.
Lastly, we evaluated whether beer style identifiers would further enhance the model’s performance. A GBR model was trained with parameters that explicitly encoded the styles of the samples. This did not improve model performance (R2 = 0.66 with style information vs R2 = 0.67). The most important chemical features are consistent with the model trained without style information (eg. ethanol and ethyl acetate), and with the exception of the most preferred (strong ale) and least preferred (low/no-alcohol) styles, none of the styles were among the most important features (Supplementary Fig. S9 , Supplementary Table S5 and S6 ). This is likely due to a combination of style-specific chemical signatures, such as iso-alpha acids and lactic acid, that implicitly convey style information to the original models, as well as the low number of samples belonging to some styles, making it difficult for the model to learn style-specific patterns. Moreover, beer styles are not rigorously defined, with some styles overlapping in features and some beers being misattributed to a specific style, all of which leads to more noise in models that use style parameters.
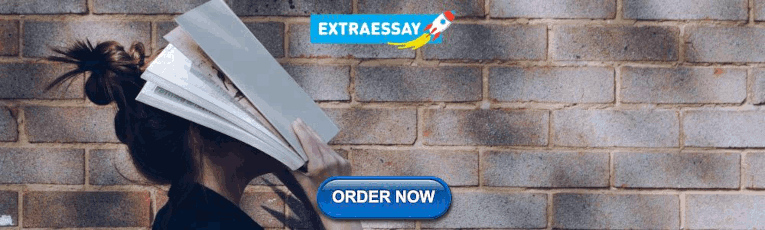
Model validation
To test if our predictive models give insight into beer appreciation, we set up experiments aimed at improving existing commercial beers. We specifically selected overall appreciation as the trait to be examined because of its complexity and commercial relevance. Beer flavor comprises a complex bouquet rather than single aromas and tastes 53 . Hence, adding a single compound to the extent that a difference is noticeable may lead to an unbalanced, artificial flavor. Therefore, we evaluated the effect of combinations of compounds. Because Blond beers represent the most extensive style in our dataset, we selected a beer from this style as the starting material for these experiments (Beer 64 in Supplementary Data 1 ).
In the first set of experiments, we adjusted the concentrations of compounds that made up the most important predictors of overall appreciation (ethyl acetate, ethanol, lactic acid, ethyl phenyl acetate) together with correlated compounds (ethyl hexanoate, isoamyl acetate, glycerol), bringing them up to 95 th percentile ethanol-normalized concentrations (Methods) within the Blond group (‘Spiked’ concentration in Fig. 5A ). Compared to controls, the spiked beers were found to have significantly improved overall appreciation among trained panelists, with panelist noting increased intensity of ester flavors, sweetness, alcohol, and body fullness (Fig. 5B ). To disentangle the contribution of ethanol to these results, a second experiment was performed without the addition of ethanol. This resulted in a similar outcome, including increased perception of alcohol and overall appreciation.
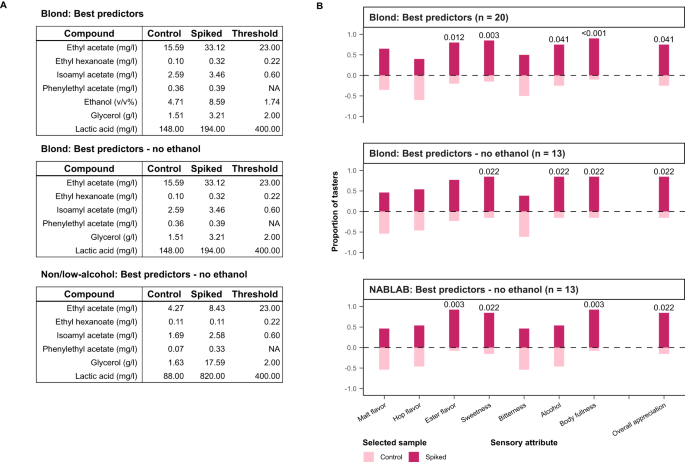
Adding the top chemical compounds, identified as best predictors of appreciation by our model, into poorly appreciated beers results in increased appreciation from our trained panel. Results of sensory tests between base beers and those spiked with compounds identified as the best predictors by the model. A Blond and Non/Low-alcohol (0.0% ABV) base beers were brought up to 95th-percentile ethanol-normalized concentrations within each style. B For each sensory attribute, tasters indicated the more intense sample and selected the sample they preferred. The numbers above the bars correspond to the p values that indicate significant changes in perceived flavor (two-sided binomial test: alpha 0.05, n = 20 or 13).
In a last experiment, we tested whether using the model’s predictions can boost the appreciation of a non-alcoholic beer (beer 223 in Supplementary Data 1 ). Again, the addition of a mixture of predicted compounds (omitting ethanol, in this case) resulted in a significant increase in appreciation, body, ester flavor and sweetness.
Predicting flavor and consumer appreciation from chemical composition is one of the ultimate goals of sensory science. A reliable, systematic and unbiased way to link chemical profiles to flavor and food appreciation would be a significant asset to the food and beverage industry. Such tools would substantially aid in quality control and recipe development, offer an efficient and cost-effective alternative to pilot studies and consumer trials and would ultimately allow food manufacturers to produce superior, tailor-made products that better meet the demands of specific consumer groups more efficiently.
A limited set of studies have previously tried, to varying degrees of success, to predict beer flavor and beer popularity based on (a limited set of) chemical compounds and flavors 79 , 80 . Current sensitive, high-throughput technologies allow measuring an unprecedented number of chemical compounds and properties in a large set of samples, yielding a dataset that can train models that help close the gaps between chemistry and flavor, even for a complex natural product like beer. To our knowledge, no previous research gathered data at this scale (250 samples, 226 chemical parameters, 50 sensory attributes and 5 consumer scores) to disentangle and validate the chemical aspects driving beer preference using various machine-learning techniques. We find that modern machine learning models outperform conventional statistical tools, such as correlations and linear models, and can successfully predict flavor appreciation from chemical composition. This could be attributed to the natural incorporation of interactions and non-linear or discontinuous effects in machine learning models, which are not easily grasped by the linear model architecture. While linear models and partial least squares regression represent the most widespread statistical approaches in sensory science, in part because they allow interpretation 65 , 81 , 82 , modern machine learning methods allow for building better predictive models while preserving the possibility to dissect and exploit the underlying patterns. Of the 10 different models we trained, tree-based models, such as our best performing GBR, showed the best overall performance in predicting sensory responses from chemical information, outcompeting artificial neural networks. This agrees with previous reports for models trained on tabular data 83 . Our results are in line with the findings of Colantonio et al. who also identified the gradient boosting architecture as performing best at predicting appreciation and flavor (of tomatoes and blueberries, in their specific study) 26 . Importantly, besides our larger experimental scale, we were able to directly confirm our models’ predictions in vivo.
Our study confirms that flavor compound concentration does not always correlate with perception, suggesting complex interactions that are often missed by more conventional statistics and simple models. Specifically, we find that tree-based algorithms may perform best in developing models that link complex food chemistry with aroma. Furthermore, we show that massive datasets of untrained consumer reviews provide a valuable source of data, that can complement or even replace trained tasting panels, especially for appreciation and basic flavors, such as sweetness and bitterness. This holds despite biases that are known to occur in such datasets, such as price or conformity bias. Moreover, GBR models predict taste better than aroma. This is likely because taste (e.g. bitterness) often directly relates to the corresponding chemical measurements (e.g., iso-alpha acids), whereas such a link is less clear for aromas, which often result from the interplay between multiple volatile compounds. We also find that our models are best at predicting acidity and alcohol, likely because there is a direct relation between the measured chemical compounds (acids and ethanol) and the corresponding perceived sensorial attribute (acidity and alcohol), and because even untrained consumers are generally able to recognize these flavors and aromas.
The predictions of our final models, trained on review data, hold even for blind tastings with small groups of trained tasters, as demonstrated by our ability to validate specific compounds as drivers of beer flavor and appreciation. Since adding a single compound to the extent of a noticeable difference may result in an unbalanced flavor profile, we specifically tested our identified key drivers as a combination of compounds. While this approach does not allow us to validate if a particular single compound would affect flavor and/or appreciation, our experiments do show that this combination of compounds increases consumer appreciation.
It is important to stress that, while it represents an important step forward, our approach still has several major limitations. A key weakness of the GBR model architecture is that amongst co-correlating variables, the largest main effect is consistently preferred for model building. As a result, co-correlating variables often have artificially low importance scores, both for impurity and SHAP-based methods, like we observed in the comparison to the more randomized Extra Trees models. This implies that chemicals identified as key drivers of a specific sensory feature by GBR might not be the true causative compounds, but rather co-correlate with the actual causative chemical. For example, the high importance of ethyl acetate could be (partially) attributed to the total ester content, ethanol or ethyl hexanoate (rho=0.77, rho=0.72 and rho=0.68), while ethyl phenylacetate could hide the importance of prenyl isobutyrate and ethyl benzoate (rho=0.77 and rho=0.76). Expanding our GBR model to include beer style as a parameter did not yield additional power or insight. This is likely due to style-specific chemical signatures, such as iso-alpha acids and lactic acid, that implicitly convey style information to the original model, as well as the smaller sample size per style, limiting the power to uncover style-specific patterns. This can be partly attributed to the curse of dimensionality, where the high number of parameters results in the models mainly incorporating single parameter effects, rather than complex interactions such as style-dependent effects 67 . A larger number of samples may overcome some of these limitations and offer more insight into style-specific effects. On the other hand, beer style is not a rigid scientific classification, and beers within one style often differ a lot, which further complicates the analysis of style as a model factor.
Our study is limited to beers from Belgian breweries. Although these beers cover a large portion of the beer styles available globally, some beer styles and consumer patterns may be missing, while other features might be overrepresented. For example, many Belgian ales exhibit yeast-driven flavor profiles, which is reflected in the chemical drivers of appreciation discovered by this study. In future work, expanding the scope to include diverse markets and beer styles could lead to the identification of even more drivers of appreciation and better models for special niche products that were not present in our beer set.
In addition to inherent limitations of GBR models, there are also some limitations associated with studying food aroma. Even if our chemical analyses measured most of the known aroma compounds, the total number of flavor compounds in complex foods like beer is still larger than the subset we were able to measure in this study. For example, hop-derived thiols, that influence flavor at very low concentrations, are notoriously difficult to measure in a high-throughput experiment. Moreover, consumer perception remains subjective and prone to biases that are difficult to avoid. It is also important to stress that the models are still immature and that more extensive datasets will be crucial for developing more complete models in the future. Besides more samples and parameters, our dataset does not include any demographic information about the tasters. Including such data could lead to better models that grasp external factors like age and culture. Another limitation is that our set of beers consists of high-quality end-products and lacks beers that are unfit for sale, which limits the current model in accurately predicting products that are appreciated very badly. Finally, while models could be readily applied in quality control, their use in sensory science and product development is restrained by their inability to discern causal relationships. Given that the models cannot distinguish compounds that genuinely drive consumer perception from those that merely correlate, validation experiments are essential to identify true causative compounds.
Despite the inherent limitations, dissection of our models enabled us to pinpoint specific molecules as potential drivers of beer aroma and consumer appreciation, including compounds that were unexpected and would not have been identified using standard approaches. Important drivers of beer appreciation uncovered by our models include protein levels, ethyl acetate, ethyl phenyl acetate and lactic acid. Currently, many brewers already use lactic acid to acidify their brewing water and ensure optimal pH for enzymatic activity during the mashing process. Our results suggest that adding lactic acid can also improve beer appreciation, although its individual effect remains to be tested. Interestingly, ethanol appears to be unnecessary to improve beer appreciation, both for blond beer and alcohol-free beer. Given the growing consumer interest in alcohol-free beer, with a predicted annual market growth of >7% 84 , it is relevant for brewers to know what compounds can further increase consumer appreciation of these beers. Hence, our model may readily provide avenues to further improve the flavor and consumer appreciation of both alcoholic and non-alcoholic beers, which is generally considered one of the key challenges for future beer production.
Whereas we see a direct implementation of our results for the development of superior alcohol-free beverages and other food products, our study can also serve as a stepping stone for the development of novel alcohol-containing beverages. We want to echo the growing body of scientific evidence for the negative effects of alcohol consumption, both on the individual level by the mutagenic, teratogenic and carcinogenic effects of ethanol 85 , 86 , as well as the burden on society caused by alcohol abuse and addiction. We encourage the use of our results for the production of healthier, tastier products, including novel and improved beverages with lower alcohol contents. Furthermore, we strongly discourage the use of these technologies to improve the appreciation or addictive properties of harmful substances.
The present work demonstrates that despite some important remaining hurdles, combining the latest developments in chemical analyses, sensory analysis and modern machine learning methods offers exciting avenues for food chemistry and engineering. Soon, these tools may provide solutions in quality control and recipe development, as well as new approaches to sensory science and flavor research.
Beer selection
250 commercial Belgian beers were selected to cover the broad diversity of beer styles and corresponding diversity in chemical composition and aroma. See Supplementary Fig. S1 .
Chemical dataset
Sample preparation.
Beers within their expiration date were purchased from commercial retailers. Samples were prepared in biological duplicates at room temperature, unless explicitly stated otherwise. Bottle pressure was measured with a manual pressure device (Steinfurth Mess-Systeme GmbH) and used to calculate CO 2 concentration. The beer was poured through two filter papers (Macherey-Nagel, 500713032 MN 713 ¼) to remove carbon dioxide and prevent spontaneous foaming. Samples were then prepared for measurements by targeted Headspace-Gas Chromatography-Flame Ionization Detector/Flame Photometric Detector (HS-GC-FID/FPD), Headspace-Solid Phase Microextraction-Gas Chromatography-Mass Spectrometry (HS-SPME-GC-MS), colorimetric analysis, enzymatic analysis, Near-Infrared (NIR) analysis, as described in the sections below. The mean values of biological duplicates are reported for each compound.
HS-GC-FID/FPD
HS-GC-FID/FPD (Shimadzu GC 2010 Plus) was used to measure higher alcohols, acetaldehyde, esters, 4-vinyl guaicol, and sulfur compounds. Each measurement comprised 5 ml of sample pipetted into a 20 ml glass vial containing 1.75 g NaCl (VWR, 27810.295). 100 µl of 2-heptanol (Sigma-Aldrich, H3003) (internal standard) solution in ethanol (Fisher Chemical, E/0650DF/C17) was added for a final concentration of 2.44 mg/L. Samples were flushed with nitrogen for 10 s, sealed with a silicone septum, stored at −80 °C and analyzed in batches of 20.
The GC was equipped with a DB-WAXetr column (length, 30 m; internal diameter, 0.32 mm; layer thickness, 0.50 µm; Agilent Technologies, Santa Clara, CA, USA) to the FID and an HP-5 column (length, 30 m; internal diameter, 0.25 mm; layer thickness, 0.25 µm; Agilent Technologies, Santa Clara, CA, USA) to the FPD. N 2 was used as the carrier gas. Samples were incubated for 20 min at 70 °C in the headspace autosampler (Flow rate, 35 cm/s; Injection volume, 1000 µL; Injection mode, split; Combi PAL autosampler, CTC analytics, Switzerland). The injector, FID and FPD temperatures were kept at 250 °C. The GC oven temperature was first held at 50 °C for 5 min and then allowed to rise to 80 °C at a rate of 5 °C/min, followed by a second ramp of 4 °C/min until 200 °C kept for 3 min and a final ramp of (4 °C/min) until 230 °C for 1 min. Results were analyzed with the GCSolution software version 2.4 (Shimadzu, Kyoto, Japan). The GC was calibrated with a 5% EtOH solution (VWR International) containing the volatiles under study (Supplementary Table S7 ).
HS-SPME-GC-MS
HS-SPME-GC-MS (Shimadzu GCMS-QP-2010 Ultra) was used to measure additional volatile compounds, mainly comprising terpenoids and esters. Samples were analyzed by HS-SPME using a triphase DVB/Carboxen/PDMS 50/30 μm SPME fiber (Supelco Co., Bellefonte, PA, USA) followed by gas chromatography (Thermo Fisher Scientific Trace 1300 series, USA) coupled to a mass spectrometer (Thermo Fisher Scientific ISQ series MS) equipped with a TriPlus RSH autosampler. 5 ml of degassed beer sample was placed in 20 ml vials containing 1.75 g NaCl (VWR, 27810.295). 5 µl internal standard mix was added, containing 2-heptanol (1 g/L) (Sigma-Aldrich, H3003), 4-fluorobenzaldehyde (1 g/L) (Sigma-Aldrich, 128376), 2,3-hexanedione (1 g/L) (Sigma-Aldrich, 144169) and guaiacol (1 g/L) (Sigma-Aldrich, W253200) in ethanol (Fisher Chemical, E/0650DF/C17). Each sample was incubated at 60 °C in the autosampler oven with constant agitation. After 5 min equilibration, the SPME fiber was exposed to the sample headspace for 30 min. The compounds trapped on the fiber were thermally desorbed in the injection port of the chromatograph by heating the fiber for 15 min at 270 °C.
The GC-MS was equipped with a low polarity RXi-5Sil MS column (length, 20 m; internal diameter, 0.18 mm; layer thickness, 0.18 µm; Restek, Bellefonte, PA, USA). Injection was performed in splitless mode at 320 °C, a split flow of 9 ml/min, a purge flow of 5 ml/min and an open valve time of 3 min. To obtain a pulsed injection, a programmed gas flow was used whereby the helium gas flow was set at 2.7 mL/min for 0.1 min, followed by a decrease in flow of 20 ml/min to the normal 0.9 mL/min. The temperature was first held at 30 °C for 3 min and then allowed to rise to 80 °C at a rate of 7 °C/min, followed by a second ramp of 2 °C/min till 125 °C and a final ramp of 8 °C/min with a final temperature of 270 °C.
Mass acquisition range was 33 to 550 amu at a scan rate of 5 scans/s. Electron impact ionization energy was 70 eV. The interface and ion source were kept at 275 °C and 250 °C, respectively. A mix of linear n-alkanes (from C7 to C40, Supelco Co.) was injected into the GC-MS under identical conditions to serve as external retention index markers. Identification and quantification of the compounds were performed using an in-house developed R script as described in Goelen et al. and Reher et al. 87 , 88 (for package information, see Supplementary Table S8 ). Briefly, chromatograms were analyzed using AMDIS (v2.71) 89 to separate overlapping peaks and obtain pure compound spectra. The NIST MS Search software (v2.0 g) in combination with the NIST2017, FFNSC3 and Adams4 libraries were used to manually identify the empirical spectra, taking into account the expected retention time. After background subtraction and correcting for retention time shifts between samples run on different days based on alkane ladders, compound elution profiles were extracted and integrated using a file with 284 target compounds of interest, which were either recovered in our identified AMDIS list of spectra or were known to occur in beer. Compound elution profiles were estimated for every peak in every chromatogram over a time-restricted window using weighted non-negative least square analysis after which peak areas were integrated 87 , 88 . Batch effect correction was performed by normalizing against the most stable internal standard compound, 4-fluorobenzaldehyde. Out of all 284 target compounds that were analyzed, 167 were visually judged to have reliable elution profiles and were used for final analysis.
Discrete photometric and enzymatic analysis
Discrete photometric and enzymatic analysis (Thermo Scientific TM Gallery TM Plus Beermaster Discrete Analyzer) was used to measure acetic acid, ammonia, beta-glucan, iso-alpha acids, color, sugars, glycerol, iron, pH, protein, and sulfite. 2 ml of sample volume was used for the analyses. Information regarding the reagents and standard solutions used for analyses and calibrations is included in Supplementary Table S7 and Supplementary Table S9 .
NIR analyses
NIR analysis (Anton Paar Alcolyzer Beer ME System) was used to measure ethanol. Measurements comprised 50 ml of sample, and a 10% EtOH solution was used for calibration.
Correlation calculations
Pairwise Spearman Rank correlations were calculated between all chemical properties.
Sensory dataset
Trained panel.
Our trained tasting panel consisted of volunteers who gave prior verbal informed consent. All compounds used for the validation experiment were of food-grade quality. The tasting sessions were approved by the Social and Societal Ethics Committee of the KU Leuven (G-2022-5677-R2(MAR)). All online reviewers agreed to the Terms and Conditions of the RateBeer website.
Sensory analysis was performed according to the American Society of Brewing Chemists (ASBC) Sensory Analysis Methods 90 . 30 volunteers were screened through a series of triangle tests. The sixteen most sensitive and consistent tasters were retained as taste panel members. The resulting panel was diverse in age [22–42, mean: 29], sex [56% male] and nationality [7 different countries]. The panel developed a consensus vocabulary to describe beer aroma, taste and mouthfeel. Panelists were trained to identify and score 50 different attributes, using a 7-point scale to rate attributes’ intensity. The scoring sheet is included as Supplementary Data 3 . Sensory assessments took place between 10–12 a.m. The beers were served in black-colored glasses. Per session, between 5 and 12 beers of the same style were tasted at 12 °C to 16 °C. Two reference beers were added to each set and indicated as ‘Reference 1 & 2’, allowing panel members to calibrate their ratings. Not all panelists were present at every tasting. Scores were scaled by standard deviation and mean-centered per taster. Values are represented as z-scores and clustered by Euclidean distance. Pairwise Spearman correlations were calculated between taste and aroma sensory attributes. Panel consistency was evaluated by repeating samples on different sessions and performing ANOVA to identify differences, using the ‘stats’ package (v4.2.2) in R (for package information, see Supplementary Table S8 ).
Online reviews from a public database
The ‘scrapy’ package in Python (v3.6) (for package information, see Supplementary Table S8 ). was used to collect 232,288 online reviews (mean=922, min=6, max=5343) from RateBeer, an online beer review database. Each review entry comprised 5 numerical scores (appearance, aroma, taste, palate and overall quality) and an optional review text. The total number of reviews per reviewer was collected separately. Numerical scores were scaled and centered per rater, and mean scores were calculated per beer.
For the review texts, the language was estimated using the packages ‘langdetect’ and ‘langid’ in Python. Reviews that were classified as English by both packages were kept. Reviewers with fewer than 100 entries overall were discarded. 181,025 reviews from >6000 reviewers from >40 countries remained. Text processing was done using the ‘nltk’ package in Python. Texts were corrected for slang and misspellings; proper nouns and rare words that are relevant to the beer context were specified and kept as-is (‘Chimay’,’Lambic’, etc.). A dictionary of semantically similar sensorial terms, for example ‘floral’ and ‘flower’, was created and collapsed together into one term. Words were stemmed and lemmatized to avoid identifying words such as ‘acid’ and ‘acidity’ as separate terms. Numbers and punctuation were removed.
Sentences from up to 50 randomly chosen reviews per beer were manually categorized according to the aspect of beer they describe (appearance, aroma, taste, palate, overall quality—not to be confused with the 5 numerical scores described above) or flagged as irrelevant if they contained no useful information. If a beer contained fewer than 50 reviews, all reviews were manually classified. This labeled data set was used to train a model that classified the rest of the sentences for all beers 91 . Sentences describing taste and aroma were extracted, and term frequency–inverse document frequency (TFIDF) was implemented to calculate enrichment scores for sensorial words per beer.
The sex of the tasting subject was not considered when building our sensory database. Instead, results from different panelists were averaged, both for our trained panel (56% male, 44% female) and the RateBeer reviews (70% male, 30% female for RateBeer as a whole).
Beer price collection and processing
Beer prices were collected from the following stores: Colruyt, Delhaize, Total Wine, BeerHawk, The Belgian Beer Shop, The Belgian Shop, and Beer of Belgium. Where applicable, prices were converted to Euros and normalized per liter. Spearman correlations were calculated between these prices and mean overall appreciation scores from RateBeer and the taste panel, respectively.
Pairwise Spearman Rank correlations were calculated between all sensory properties.
Machine learning models
Predictive modeling of sensory profiles from chemical data.
Regression models were constructed to predict (a) trained panel scores for beer flavors and quality from beer chemical profiles and (b) public reviews’ appreciation scores from beer chemical profiles. Z-scores were used to represent sensory attributes in both data sets. Chemical properties with log-normal distributions (Shapiro-Wilk test, p < 0.05 ) were log-transformed. Missing chemical measurements (0.1% of all data) were replaced with mean values per attribute. Observations from 250 beers were randomly separated into a training set (70%, 175 beers) and a test set (30%, 75 beers), stratified per beer style. Chemical measurements (p = 231) were normalized based on the training set average and standard deviation. In total, three linear regression-based models: linear regression with first-order interaction terms (LR), lasso regression with first-order interaction terms (Lasso) and partial least squares regression (PLSR); five decision tree models, Adaboost regressor (ABR), Extra Trees (ET), Gradient Boosting regressor (GBR), Random Forest (RF) and XGBoost regressor (XGBR); one support vector machine model (SVR) and one artificial neural network model (ANN) were trained. The models were implemented using the ‘scikit-learn’ package (v1.2.2) and ‘xgboost’ package (v1.7.3) in Python (v3.9.16). Models were trained, and hyperparameters optimized, using five-fold cross-validated grid search with the coefficient of determination (R 2 ) as the evaluation metric. The ANN (scikit-learn’s MLPRegressor) was optimized using Bayesian Tree-Structured Parzen Estimator optimization with the ‘Optuna’ Python package (v3.2.0). Individual models were trained per attribute, and a multi-output model was trained on all attributes simultaneously.
Model dissection
GBR was found to outperform other methods, resulting in models with the highest average R 2 values in both trained panel and public review data sets. Impurity-based rankings of the most important predictors for each predicted sensorial trait were obtained using the ‘scikit-learn’ package. To observe the relationships between these chemical properties and their predicted targets, partial dependence plots (PDP) were constructed for the six most important predictors of consumer appreciation 74 , 75 .
The ‘SHAP’ package in Python (v0.41.0) was implemented to provide an alternative ranking of predictor importance and to visualize the predictors’ effects as a function of their concentration 68 .
Validation of causal chemical properties
To validate the effects of the most important model features on predicted sensory attributes, beers were spiked with the chemical compounds identified by the models and descriptive sensory analyses were carried out according to the American Society of Brewing Chemists (ASBC) protocol 90 .
Compound spiking was done 30 min before tasting. Compounds were spiked into fresh beer bottles, that were immediately resealed and inverted three times. Fresh bottles of beer were opened for the same duration, resealed, and inverted thrice, to serve as controls. Pairs of spiked samples and controls were served simultaneously, chilled and in dark glasses as outlined in the Trained panel section above. Tasters were instructed to select the glass with the higher flavor intensity for each attribute (directional difference test 92 ) and to select the glass they prefer.
The final concentration after spiking was equal to the within-style average, after normalizing by ethanol concentration. This was done to ensure balanced flavor profiles in the final spiked beer. The same methods were applied to improve a non-alcoholic beer. Compounds were the following: ethyl acetate (Merck KGaA, W241415), ethyl hexanoate (Merck KGaA, W243906), isoamyl acetate (Merck KGaA, W205508), phenethyl acetate (Merck KGaA, W285706), ethanol (96%, Colruyt), glycerol (Merck KGaA, W252506), lactic acid (Merck KGaA, 261106).
Significant differences in preference or perceived intensity were determined by performing the two-sided binomial test on each attribute.
Reporting summary
Further information on research design is available in the Nature Portfolio Reporting Summary linked to this article.
Data availability
The data that support the findings of this work are available in the Supplementary Data files and have been deposited to Zenodo under accession code 10653704 93 . The RateBeer scores data are under restricted access, they are not publicly available as they are property of RateBeer (ZX Ventures, USA). Access can be obtained from the authors upon reasonable request and with permission of RateBeer (ZX Ventures, USA). Source data are provided with this paper.
Code availability
The code for training the machine learning models, analyzing the models, and generating the figures has been deposited to Zenodo under accession code 10653704 93 .
Tieman, D. et al. A chemical genetic roadmap to improved tomato flavor. Science 355 , 391–394 (2017).
Article ADS CAS PubMed Google Scholar
Plutowska, B. & Wardencki, W. Application of gas chromatography–olfactometry (GC–O) in analysis and quality assessment of alcoholic beverages – A review. Food Chem. 107 , 449–463 (2008).
Article CAS Google Scholar
Legin, A., Rudnitskaya, A., Seleznev, B. & Vlasov, Y. Electronic tongue for quality assessment of ethanol, vodka and eau-de-vie. Anal. Chim. Acta 534 , 129–135 (2005).
Loutfi, A., Coradeschi, S., Mani, G. K., Shankar, P. & Rayappan, J. B. B. Electronic noses for food quality: A review. J. Food Eng. 144 , 103–111 (2015).
Ahn, Y.-Y., Ahnert, S. E., Bagrow, J. P. & Barabási, A.-L. Flavor network and the principles of food pairing. Sci. Rep. 1 , 196 (2011).
Article CAS PubMed PubMed Central Google Scholar
Bartoshuk, L. M. & Klee, H. J. Better fruits and vegetables through sensory analysis. Curr. Biol. 23 , R374–R378 (2013).
Article CAS PubMed Google Scholar
Piggott, J. R. Design questions in sensory and consumer science. Food Qual. Prefer. 3293 , 217–220 (1995).
Article Google Scholar
Kermit, M. & Lengard, V. Assessing the performance of a sensory panel-panellist monitoring and tracking. J. Chemom. 19 , 154–161 (2005).
Cook, D. J., Hollowood, T. A., Linforth, R. S. T. & Taylor, A. J. Correlating instrumental measurements of texture and flavour release with human perception. Int. J. Food Sci. Technol. 40 , 631–641 (2005).
Chinchanachokchai, S., Thontirawong, P. & Chinchanachokchai, P. A tale of two recommender systems: The moderating role of consumer expertise on artificial intelligence based product recommendations. J. Retail. Consum. Serv. 61 , 1–12 (2021).
Ross, C. F. Sensory science at the human-machine interface. Trends Food Sci. Technol. 20 , 63–72 (2009).
Chambers, E. IV & Koppel, K. Associations of volatile compounds with sensory aroma and flavor: The complex nature of flavor. Molecules 18 , 4887–4905 (2013).
Pinu, F. R. Metabolomics—The new frontier in food safety and quality research. Food Res. Int. 72 , 80–81 (2015).
Danezis, G. P., Tsagkaris, A. S., Brusic, V. & Georgiou, C. A. Food authentication: state of the art and prospects. Curr. Opin. Food Sci. 10 , 22–31 (2016).
Shepherd, G. M. Smell images and the flavour system in the human brain. Nature 444 , 316–321 (2006).
Meilgaard, M. C. Prediction of flavor differences between beers from their chemical composition. J. Agric. Food Chem. 30 , 1009–1017 (1982).
Xu, L. et al. Widespread receptor-driven modulation in peripheral olfactory coding. Science 368 , eaaz5390 (2020).
Kupferschmidt, K. Following the flavor. Science 340 , 808–809 (2013).
Billesbølle, C. B. et al. Structural basis of odorant recognition by a human odorant receptor. Nature 615 , 742–749 (2023).
Article ADS PubMed PubMed Central Google Scholar
Smith, B. Perspective: Complexities of flavour. Nature 486 , S6–S6 (2012).
Pfister, P. et al. Odorant receptor inhibition is fundamental to odor encoding. Curr. Biol. 30 , 2574–2587 (2020).
Moskowitz, H. W., Kumaraiah, V., Sharma, K. N., Jacobs, H. L. & Sharma, S. D. Cross-cultural differences in simple taste preferences. Science 190 , 1217–1218 (1975).
Eriksson, N. et al. A genetic variant near olfactory receptor genes influences cilantro preference. Flavour 1 , 22 (2012).
Ferdenzi, C. et al. Variability of affective responses to odors: Culture, gender, and olfactory knowledge. Chem. Senses 38 , 175–186 (2013).
Article PubMed Google Scholar
Lawless, H. T. & Heymann, H. Sensory evaluation of food: Principles and practices. (Springer, New York, NY). https://doi.org/10.1007/978-1-4419-6488-5 (2010).
Colantonio, V. et al. Metabolomic selection for enhanced fruit flavor. Proc. Natl. Acad. Sci. 119 , e2115865119 (2022).
Fritz, F., Preissner, R. & Banerjee, P. VirtualTaste: a web server for the prediction of organoleptic properties of chemical compounds. Nucleic Acids Res 49 , W679–W684 (2021).
Tuwani, R., Wadhwa, S. & Bagler, G. BitterSweet: Building machine learning models for predicting the bitter and sweet taste of small molecules. Sci. Rep. 9 , 1–13 (2019).
Dagan-Wiener, A. et al. Bitter or not? BitterPredict, a tool for predicting taste from chemical structure. Sci. Rep. 7 , 1–13 (2017).
Pallante, L. et al. Toward a general and interpretable umami taste predictor using a multi-objective machine learning approach. Sci. Rep. 12 , 1–11 (2022).
Malavolta, M. et al. A survey on computational taste predictors. Eur. Food Res. Technol. 248 , 2215–2235 (2022).
Lee, B. K. et al. A principal odor map unifies diverse tasks in olfactory perception. Science 381 , 999–1006 (2023).
Mayhew, E. J. et al. Transport features predict if a molecule is odorous. Proc. Natl. Acad. Sci. 119 , e2116576119 (2022).
Niu, Y. et al. Sensory evaluation of the synergism among ester odorants in light aroma-type liquor by odor threshold, aroma intensity and flash GC electronic nose. Food Res. Int. 113 , 102–114 (2018).
Yu, P., Low, M. Y. & Zhou, W. Design of experiments and regression modelling in food flavour and sensory analysis: A review. Trends Food Sci. Technol. 71 , 202–215 (2018).
Oladokun, O. et al. The impact of hop bitter acid and polyphenol profiles on the perceived bitterness of beer. Food Chem. 205 , 212–220 (2016).
Linforth, R., Cabannes, M., Hewson, L., Yang, N. & Taylor, A. Effect of fat content on flavor delivery during consumption: An in vivo model. J. Agric. Food Chem. 58 , 6905–6911 (2010).
Guo, S., Na Jom, K. & Ge, Y. Influence of roasting condition on flavor profile of sunflower seeds: A flavoromics approach. Sci. Rep. 9 , 11295 (2019).
Ren, Q. et al. The changes of microbial community and flavor compound in the fermentation process of Chinese rice wine using Fagopyrum tataricum grain as feedstock. Sci. Rep. 9 , 3365 (2019).
Hastie, T., Friedman, J. & Tibshirani, R. The Elements of Statistical Learning. (Springer, New York, NY). https://doi.org/10.1007/978-0-387-21606-5 (2001).
Dietz, C., Cook, D., Huismann, M., Wilson, C. & Ford, R. The multisensory perception of hop essential oil: a review. J. Inst. Brew. 126 , 320–342 (2020).
CAS Google Scholar
Roncoroni, Miguel & Verstrepen, Kevin Joan. Belgian Beer: Tested and Tasted. (Lannoo, 2018).
Meilgaard, M. Flavor chemistry of beer: Part II: Flavor and threshold of 239 aroma volatiles. in (1975).
Bokulich, N. A. & Bamforth, C. W. The microbiology of malting and brewing. Microbiol. Mol. Biol. Rev. MMBR 77 , 157–172 (2013).
Dzialo, M. C., Park, R., Steensels, J., Lievens, B. & Verstrepen, K. J. Physiology, ecology and industrial applications of aroma formation in yeast. FEMS Microbiol. Rev. 41 , S95–S128 (2017).
Article PubMed PubMed Central Google Scholar
Datta, A. et al. Computer-aided food engineering. Nat. Food 3 , 894–904 (2022).
American Society of Brewing Chemists. Beer Methods. (American Society of Brewing Chemists, St. Paul, MN, U.S.A.).
Olaniran, A. O., Hiralal, L., Mokoena, M. P. & Pillay, B. Flavour-active volatile compounds in beer: production, regulation and control. J. Inst. Brew. 123 , 13–23 (2017).
Verstrepen, K. J. et al. Flavor-active esters: Adding fruitiness to beer. J. Biosci. Bioeng. 96 , 110–118 (2003).
Meilgaard, M. C. Flavour chemistry of beer. part I: flavour interaction between principal volatiles. Master Brew. Assoc. Am. Tech. Q 12 , 107–117 (1975).
Briggs, D. E., Boulton, C. A., Brookes, P. A. & Stevens, R. Brewing 227–254. (Woodhead Publishing). https://doi.org/10.1533/9781855739062.227 (2004).
Bossaert, S., Crauwels, S., De Rouck, G. & Lievens, B. The power of sour - A review: Old traditions, new opportunities. BrewingScience 72 , 78–88 (2019).
Google Scholar
Verstrepen, K. J. et al. Flavor active esters: Adding fruitiness to beer. J. Biosci. Bioeng. 96 , 110–118 (2003).
Snauwaert, I. et al. Microbial diversity and metabolite composition of Belgian red-brown acidic ales. Int. J. Food Microbiol. 221 , 1–11 (2016).
Spitaels, F. et al. The microbial diversity of traditional spontaneously fermented lambic beer. PLoS ONE 9 , e95384 (2014).
Blanco, C. A., Andrés-Iglesias, C. & Montero, O. Low-alcohol Beers: Flavor Compounds, Defects, and Improvement Strategies. Crit. Rev. Food Sci. Nutr. 56 , 1379–1388 (2016).
Jackowski, M. & Trusek, A. Non-Alcohol. beer Prod. – Overv. 20 , 32–38 (2018).
Takoi, K. et al. The contribution of geraniol metabolism to the citrus flavour of beer: Synergy of geraniol and β-citronellol under coexistence with excess linalool. J. Inst. Brew. 116 , 251–260 (2010).
Kroeze, J. H. & Bartoshuk, L. M. Bitterness suppression as revealed by split-tongue taste stimulation in humans. Physiol. Behav. 35 , 779–783 (1985).
Mennella, J. A. et al. A spoonful of sugar helps the medicine go down”: Bitter masking bysucrose among children and adults. Chem. Senses 40 , 17–25 (2015).
Wietstock, P., Kunz, T., Perreira, F. & Methner, F.-J. Metal chelation behavior of hop acids in buffered model systems. BrewingScience 69 , 56–63 (2016).
Sancho, D., Blanco, C. A., Caballero, I. & Pascual, A. Free iron in pale, dark and alcohol-free commercial lager beers. J. Sci. Food Agric. 91 , 1142–1147 (2011).
Rodrigues, H. & Parr, W. V. Contribution of cross-cultural studies to understanding wine appreciation: A review. Food Res. Int. 115 , 251–258 (2019).
Korneva, E. & Blockeel, H. Towards better evaluation of multi-target regression models. in ECML PKDD 2020 Workshops (eds. Koprinska, I. et al.) 353–362 (Springer International Publishing, Cham, 2020). https://doi.org/10.1007/978-3-030-65965-3_23 .
Gastón Ares. Mathematical and Statistical Methods in Food Science and Technology. (Wiley, 2013).
Grinsztajn, L., Oyallon, E. & Varoquaux, G. Why do tree-based models still outperform deep learning on tabular data? Preprint at http://arxiv.org/abs/2207.08815 (2022).
Gries, S. T. Statistics for Linguistics with R: A Practical Introduction. in Statistics for Linguistics with R (De Gruyter Mouton, 2021). https://doi.org/10.1515/9783110718256 .
Lundberg, S. M. et al. From local explanations to global understanding with explainable AI for trees. Nat. Mach. Intell. 2 , 56–67 (2020).
Ickes, C. M. & Cadwallader, K. R. Effects of ethanol on flavor perception in alcoholic beverages. Chemosens. Percept. 10 , 119–134 (2017).
Kato, M. et al. Influence of high molecular weight polypeptides on the mouthfeel of commercial beer. J. Inst. Brew. 127 , 27–40 (2021).
Wauters, R. et al. Novel Saccharomyces cerevisiae variants slow down the accumulation of staling aldehydes and improve beer shelf-life. Food Chem. 398 , 1–11 (2023).
Li, H., Jia, S. & Zhang, W. Rapid determination of low-level sulfur compounds in beer by headspace gas chromatography with a pulsed flame photometric detector. J. Am. Soc. Brew. Chem. 66 , 188–191 (2008).
Dercksen, A., Laurens, J., Torline, P., Axcell, B. C. & Rohwer, E. Quantitative analysis of volatile sulfur compounds in beer using a membrane extraction interface. J. Am. Soc. Brew. Chem. 54 , 228–233 (1996).
Molnar, C. Interpretable Machine Learning: A Guide for Making Black-Box Models Interpretable. (2020).
Zhao, Q. & Hastie, T. Causal interpretations of black-box models. J. Bus. Econ. Stat. Publ. Am. Stat. Assoc. 39 , 272–281 (2019).
Article MathSciNet Google Scholar
Hastie, T., Tibshirani, R. & Friedman, J. The Elements of Statistical Learning. (Springer, 2019).
Labrado, D. et al. Identification by NMR of key compounds present in beer distillates and residual phases after dealcoholization by vacuum distillation. J. Sci. Food Agric. 100 , 3971–3978 (2020).
Lusk, L. T., Kay, S. B., Porubcan, A. & Ryder, D. S. Key olfactory cues for beer oxidation. J. Am. Soc. Brew. Chem. 70 , 257–261 (2012).
Gonzalez Viejo, C., Torrico, D. D., Dunshea, F. R. & Fuentes, S. Development of artificial neural network models to assess beer acceptability based on sensory properties using a robotic pourer: A comparative model approach to achieve an artificial intelligence system. Beverages 5 , 33 (2019).
Gonzalez Viejo, C., Fuentes, S., Torrico, D. D., Godbole, A. & Dunshea, F. R. Chemical characterization of aromas in beer and their effect on consumers liking. Food Chem. 293 , 479–485 (2019).
Gilbert, J. L. et al. Identifying breeding priorities for blueberry flavor using biochemical, sensory, and genotype by environment analyses. PLOS ONE 10 , 1–21 (2015).
Goulet, C. et al. Role of an esterase in flavor volatile variation within the tomato clade. Proc. Natl. Acad. Sci. 109 , 19009–19014 (2012).
Article ADS CAS PubMed PubMed Central Google Scholar
Borisov, V. et al. Deep Neural Networks and Tabular Data: A Survey. IEEE Trans. Neural Netw. Learn. Syst. 1–21 https://doi.org/10.1109/TNNLS.2022.3229161 (2022).
Statista. Statista Consumer Market Outlook: Beer - Worldwide.
Seitz, H. K. & Stickel, F. Molecular mechanisms of alcoholmediated carcinogenesis. Nat. Rev. Cancer 7 , 599–612 (2007).
Voordeckers, K. et al. Ethanol exposure increases mutation rate through error-prone polymerases. Nat. Commun. 11 , 3664 (2020).
Goelen, T. et al. Bacterial phylogeny predicts volatile organic compound composition and olfactory response of an aphid parasitoid. Oikos 129 , 1415–1428 (2020).
Article ADS Google Scholar
Reher, T. et al. Evaluation of hop (Humulus lupulus) as a repellent for the management of Drosophila suzukii. Crop Prot. 124 , 104839 (2019).
Stein, S. E. An integrated method for spectrum extraction and compound identification from gas chromatography/mass spectrometry data. J. Am. Soc. Mass Spectrom. 10 , 770–781 (1999).
American Society of Brewing Chemists. Sensory Analysis Methods. (American Society of Brewing Chemists, St. Paul, MN, U.S.A., 1992).
McAuley, J., Leskovec, J. & Jurafsky, D. Learning Attitudes and Attributes from Multi-Aspect Reviews. Preprint at https://doi.org/10.48550/arXiv.1210.3926 (2012).
Meilgaard, M. C., Carr, B. T. & Carr, B. T. Sensory Evaluation Techniques. (CRC Press, Boca Raton). https://doi.org/10.1201/b16452 (2014).
Schreurs, M. et al. Data from: Predicting and improving complex beer flavor through machine learning. Zenodo https://doi.org/10.5281/zenodo.10653704 (2024).
Download references
Acknowledgements
We thank all lab members for their discussions and thank all tasting panel members for their contributions. Special thanks go out to Dr. Karin Voordeckers for her tremendous help in proofreading and improving the manuscript. M.S. was supported by a Baillet-Latour fellowship, L.C. acknowledges financial support from KU Leuven (C16/17/006), F.A.T. was supported by a PhD fellowship from FWO (1S08821N). Research in the lab of K.J.V. is supported by KU Leuven, FWO, VIB, VLAIO and the Brewing Science Serves Health Fund. Research in the lab of T.W. is supported by FWO (G.0A51.15) and KU Leuven (C16/17/006).
Author information
These authors contributed equally: Michiel Schreurs, Supinya Piampongsant, Miguel Roncoroni.
Authors and Affiliations
VIB—KU Leuven Center for Microbiology, Gaston Geenslaan 1, B-3001, Leuven, Belgium
Michiel Schreurs, Supinya Piampongsant, Miguel Roncoroni, Lloyd Cool, Beatriz Herrera-Malaver, Florian A. Theßeling & Kevin J. Verstrepen
CMPG Laboratory of Genetics and Genomics, KU Leuven, Gaston Geenslaan 1, B-3001, Leuven, Belgium
Leuven Institute for Beer Research (LIBR), Gaston Geenslaan 1, B-3001, Leuven, Belgium
Laboratory of Socioecology and Social Evolution, KU Leuven, Naamsestraat 59, B-3000, Leuven, Belgium
Lloyd Cool, Christophe Vanderaa & Tom Wenseleers
VIB Bioinformatics Core, VIB, Rijvisschestraat 120, B-9052, Ghent, Belgium
Łukasz Kreft & Alexander Botzki
AB InBev SA/NV, Brouwerijplein 1, B-3000, Leuven, Belgium
Philippe Malcorps & Luk Daenen
You can also search for this author in PubMed Google Scholar
Contributions
S.P., M.S. and K.J.V. conceived the experiments. S.P., M.S. and K.J.V. designed the experiments. S.P., M.S., M.R., B.H. and F.A.T. performed the experiments. S.P., M.S., L.C., C.V., L.K., A.B., P.M., L.D., T.W. and K.J.V. contributed analysis ideas. S.P., M.S., L.C., C.V., T.W. and K.J.V. analyzed the data. All authors contributed to writing the manuscript.
Corresponding author
Correspondence to Kevin J. Verstrepen .
Ethics declarations
Competing interests.
K.J.V. is affiliated with bar.on. The other authors declare no competing interests.
Peer review
Peer review information.
Nature Communications thanks Florian Bauer, Andrew John Macintosh and the other, anonymous, reviewer(s) for their contribution to the peer review of this work. A peer review file is available.
Additional information
Publisher’s note Springer Nature remains neutral with regard to jurisdictional claims in published maps and institutional affiliations.
Supplementary information
Supplementary information, peer review file, description of additional supplementary files, supplementary data 1, supplementary data 2, supplementary data 3, supplementary data 4, supplementary data 5, supplementary data 6, supplementary data 7, reporting summary, source data, source data, rights and permissions.
Open Access This article is licensed under a Creative Commons Attribution 4.0 International License, which permits use, sharing, adaptation, distribution and reproduction in any medium or format, as long as you give appropriate credit to the original author(s) and the source, provide a link to the Creative Commons licence, and indicate if changes were made. The images or other third party material in this article are included in the article’s Creative Commons licence, unless indicated otherwise in a credit line to the material. If material is not included in the article’s Creative Commons licence and your intended use is not permitted by statutory regulation or exceeds the permitted use, you will need to obtain permission directly from the copyright holder. To view a copy of this licence, visit http://creativecommons.org/licenses/by/4.0/ .
Reprints and permissions
About this article
Cite this article.
Schreurs, M., Piampongsant, S., Roncoroni, M. et al. Predicting and improving complex beer flavor through machine learning. Nat Commun 15 , 2368 (2024). https://doi.org/10.1038/s41467-024-46346-0
Download citation
Received : 30 October 2023
Accepted : 21 February 2024
Published : 26 March 2024
DOI : https://doi.org/10.1038/s41467-024-46346-0
Share this article
Anyone you share the following link with will be able to read this content:
Sorry, a shareable link is not currently available for this article.
Provided by the Springer Nature SharedIt content-sharing initiative
By submitting a comment you agree to abide by our Terms and Community Guidelines . If you find something abusive or that does not comply with our terms or guidelines please flag it as inappropriate.
Quick links
- Explore articles by subject
- Guide to authors
- Editorial policies
Sign up for the Nature Briefing: Translational Research newsletter — top stories in biotechnology, drug discovery and pharma.


An official website of the United States government
The .gov means it’s official. Federal government websites often end in .gov or .mil. Before sharing sensitive information, make sure you’re on a federal government site.
The site is secure. The https:// ensures that you are connecting to the official website and that any information you provide is encrypted and transmitted securely.
- Publications
- Account settings
Preview improvements coming to the PMC website in October 2024. Learn More or Try it out now .
- Advanced Search
- Journal List
- Ann Transl Med
- v.6(3); 2018 Feb

How do I peer-review a scientific article?—a personal perspective
Peer-review is an essential activity for the vast majority of credited scientific journals and represents the cornerstone for assessing the quality of potential publications, since it is substantially aimed to identify drawbacks or inaccuracies that may flaw the outcome or the presentation of scientific research. Since the importance of this activity is seldom underestimated by some referees, the purpose of this article is to present a personal and arbitrary perspective on how a scientific article should be peer-reviewed, offering a tentative checklist aimed to describe the most important criteria that should be considered. These basically include accepting the assignment only when the topic is in accordance with referee’s background, disclosing potential conflicts of interest, checking availability and time according to size and complexity of the article, identifying the innovative value of the manuscript, providing exhaustive and clear comments, expressing disagreement with a fair and balanced approach, weighting revisions according to the importance of the journal, summarizing recommendations according to previous comments, maintaining confidentiality throughout and after the peer-review process. I really hope that some notions reported in this dissertation may be a guide or a help, especially for young scientists, who are willing to be engaged in peer-review activity for scientific journals.
Introduction
The contribution of peer-reviewers is invaluable in scholarly publishing, science and medicine. Peer-review, also known as also known as “refereeing”, is a hallmark of the vast majority of scientific journals and represents the cornerstone for assessing the quality of potential scientific publications, since it is aimed to identify drawbacks or inaccuracies that may flaw the outcome or the presentation of scientific research ( 1 ). This voluntary and usually free activity is especially vital for biomedical sciences, because the publication of biased or incorrect information may seriously jeopardize patient safety, thus guiding the clinical decision making towards inappropriate diagnostic or therapeutic actions ( 2 ).
On the other hand, the activity of refereeing scientific articles may also be of value for the reviewer, for a variety of reasons including knowledge improvement on specific topics due to the possibility of reading articles before the information is published, may give valuable ideas for future studies on the same or other topics, may help improving you own writing skill, and is also a meaningful activity that can be included in the scientific curriculum. Although some generic rules for performing an accurate peer-review have been identified by many scientific journals, evidence exists that this activity not always ensures the quality of published biomedical research ( 3 ). Therefore, the purpose of this article is to present a personal and arbitrary perspective, accumulated after a 25-year experience ( 4 ), on how a scientific article should be peer-reviewed.
Limit peer-review to topics in line with your expertise
Throughout my career, I have (hopefully) accumulated a good background in the fields of clinical biochemistry, laboratory medicine and hemostasis. Therefore, my peer-review activity is actually limited to these specific areas of science and medicine. Nevertheless, during the past 3 months I have been repeatedly invited to peer-review scientific articles dealing with social sciences, astrophysics, thermal engineering, plant biology, fishery and even about worldwide economy. Besides highlighting that the credibility of these journals is probably null, the editors have a large liability since their activity can be defined as a clear misconduct when randomly assigning manuscripts to referees with no expertise on the topic. I have obviously declined to peer-review these articles and I really hope that other colleagues, whose background is also quite different from the topic of the articles, have also done so. I have brought this simple but paradigmatic example just for emphasizing that competency is the very first aspect that should guide the decision to accept or decline an invitation to peer-review a scientific article. Therefore, whenever you feel that the manuscript falls outside your competence or knowledge, you are ethically obligated to decline peer-review. As also endorsed by the Council of Science Editors (CSE) ( 5 ), peer-reviewers do not actually need to have an expertise covering all the different aspects of the article, but the assignment should only be accepted when the expertise is enough for providing authoritative assessment.
Check potential conflicts of interest
Although some journals mandatorily ask the reviewers to disclose potential conflicts of interest with the article or with its authors, this is not routine practice. Nevertheless, even if this is not clearly entailed by the journal, you should be fair enough to check potential conflicts of interest on your own before accepting the assignment. Conflict of interest disclosure is a broad enterprise, which can be actually summarized as the existence of interests that may impair your objectivity, and should hence lead to mandatory declining peer-review when (I) a direct relationship (personal or professional) exists with the authors, thus preventing positive bias in referee’s comments; (II) you have a negative opinion on, or you had previous disagreements with, the authors, which may then induce a negative bias in your peer-review; (III) the referee is engaged in similar or overlapping studies, so that there may be a propensity to (even unconsciously) underrate the outcome; (IV) there is a commercial relationship with companies whose drugs, devices or reagents have been tested or used in the study. I will never tell the source, even under torture, but time ago I was asked (for an error of the editorial office, hopefully) to peer-review one article that I had authored. This is, clearly, the greatest possible conflict of interest.
Personal beliefs diverging from the topic of the article may also be seen as potential conflicts of interest when the referee may not be able to keep them within an acceptable level of “interference”. As earlier discussed, usually referees are not paid for peer-reviewing articles and thereby there is no obligation to accept the assignment. Do not expect that the Editor will better treat your future submissions just because you have peer-reviewed some articles. This is totally unreasonable.
Check your availability and time
One of the worst aspects in scientific publishing is submitting an article to a peer-review journal and then waiting ages to receive the comments of the referees. This is frustrating, but may also have a dramatic impact on the chance of publishing the research. Original articles, whilst focusing on very innovative topics, may become old or even obsolete in few months, even in few weeks. The referee should hence always consider this aspect when accepting the assignment, since it is unfair to keep the article under revision for months, and it is even more unfair when the referee deliberately does so for delaying the publication of the article (see previous paragraph). When the referee finally submits the recommendations, many articles on the same topic may have been published by different authors. Whenever I accept to peer-review an article, my deadline never exceeds 3 to 5 days, whilst whenever I expect that I could not be able to peer-review the article within one week, I prefer to decline the assignment. Although the deadline for refereeing articles is quite heterogeneous among the various scientific journals (i.e., from 1 to 4 weeks), once you have established that the deadline fits your ongoing (or future) activities, then you must honour the commitment you made. Do not accept to peer-review an article if you are just about to leave for your holidays or you are not planning to work for quite a long time. The decision to accept or decline an assignment will also be influenced by the size and complexity of the article. You should hence consider that it may take quite a different time (and effort) to peer-review a short letter to the editor or a large meta-analysis. Importantly, impersonation or involvement of other scientists during the peer-review activity shall be seen as a severe misconduct.
Identify the innovative value of the article
Once you have finally accepted the assignment, checking how much the specific topic has been investigated in the recent scientific literature and whether or not the argument fit the scope of the journal are advisable practices. When the referee has a very good knowledge about the topic, then there is no need to search information elsewhere. However, when the referee is not completely familiar with the topic, or else there are some innovative aspects that are partially obscure, it is advisable to verify the volume and type of previously available information using reliable sources. This can be easily done by accessing some biomedical platforms such as PubMed, Google Scholar, Scopus and Web of Science ( 6 ), by entering the keywords used by the authors or representative terms captured from the title or the abstract of the manuscript. The first two biomedical search engines are free and cover a large number of scientific publications. Therefore, when institutional or personal subscriptions to Scopus and Web of Science are unavailable, a simple search in PubMed and Google Scholar will be sufficient. The simple number of publications retrievable with an electronic search should not necessarily guide your conclusions about the novelty of the article, since many differences may exist regarding the study population, the sample size, the analytical techniques, the endpoints. Nevertheless, it occasionally happens that all these aspects are quite similar, or virtually identical, to those contained in previously published articles. In such case, it is actually worthless to undertake a thoughtful revision of the manuscript, since it is unlikely that the conclusions of the study will contribute to improve the current scientific knowledge, and it may hence be advisable to limit your comments to a simple sentence stating that the novelty of the article is too low to recommend acceptance, or that the topic does not fit the scope of the journal.
Although inherently arbitrary, I also tend to use biomedical search engines for checking the number and type of previous publications by the same team of authors, provided that the article is not anonymized. This will give you advices about competence and reputation of the authors, and is a virtually unavoidable practice when you are invited to peer-review guidelines, recommendations or position papers. Notably, by checking PubMed, I have been also able to identify a number of duplicate (or very similar) articles, which cannot be always detected using a plagiarism check software ( 7 ). Some authors are getting smart; they submit duplicate articles with substantial word changes, but whose contents are totally overlapping with those of previous publications ( 8 ).
The comments
I usually read the article twice. The first reading is aimed to reach a general opinion about novelty, quality and practical implications. I do not typically write any comment during the first read. The second reading, often on a different day, is instead finalized to more accurately identify drawbacks or weaknesses.
The quality assessment of an article must be rigorous and meet a number of predefined criteria. Most of these have been discussed in a previous article, dealing with personal suggestions about writing scientific articles ( 9 ). Briefly, a good peer-review activity entails checking that (I) the title is appropriate; (II) the authors’ list really mirrors the individual contribution; (III) the abstract is focused on data and conclusions; (IV) the introduction clearly defines the main aspects of the topic being investigated and explains the aim of the study; (V) the materials and methods section exhaustively describes study population, sample size, analytical techniques, statistical tests, informed consent and ethical approval; (VI) the result section contains relevant findings without replicating data already shown in tables and figures; (VII) the discussion does not repeat data previously reported in results, tables or figures, appropriately discusses the findings according to current knowledge or existing literature, conclusions are supported by biological explanation, and study limitations are clearly highlighted; (VIII) the reference list fulfils journal’s guidelines, is appropriate and does not include many self-citations.
The referee should also carefully check that the article contains all necessary information for guaranteeing study reproducibility. A final scrutiny of article layout may also be advisable, focusing on style, presence of typos and unexplained abbreviations. When the first two aspects are poor, it may be advisable to suggest that the article should be reviewed by an English-native speaker, whilst the presence of many unexplained abbreviations needs to be highlighted, since these may not be understood by the readers. Although some publishers advocate that time should not be spent to polish grammar or spelling, not all journals carefully revise the original text before publication. Therefore, I prefer to highlight at least the major stylistic issues encountered during my readings, so that these can be fixed by the authors while resubmitting their manuscript.
Importantly, the referee must not use peer-review activity as an unfair means for boosting bibliometric indices, e.g., by asking to add citations to your previous articles, especially when these citations are completely unwarranted. When peer-review is blind, the referee should avoid using expressions that may lead the authors to identify referee’s identity.
Write your comments clearly
The worst aspect that challenges article revision according to the comments of reviewers is being unable to understand what reviewers are asking. It is not so rare to read comments like “I do not agree with your study design”, “a statement on page 5 is questionable” or “the statistics should be broadened”. Occasionally, the comments are written in such a bad English that the authors cannot even understand what the referee means. This makes article revision virtually unfeasible, or else the authors may introduce changes in the manuscript that are not really necessary. As a rule of thumb, I always write my comments indicating both page and line numbers or, when these are unavailable, I specifically indicate to the part of the manuscript needing revision, e.g., reporting the full sentence or the paragraph between brackets (e.g., I found a problem in the sentence: “…”), and I classify the potential caveats in "major" and "minor". Then, I read my comments almost twice, to be sure that what I have written can be clearly understood by the authors. I always structure my comments in numbers or dot points, since this helps authors’ reply.
Regarding the specific comments that you are willing to make about the article, disagreement is allowed, and often advisable, as long as its source is clearly disclosed and supported by objective data. It is not fair to judge a manuscript only guided by impressions. As previously mentioned, it is actually meaningless for both the editor of the journal and the authors to read a comment like “a statement on page 5 is questionable”, without such statement being explained. Therefore, whenever I do not agree with some parts of a manuscript, I always accompany my comments with reference to previous studies and clear explanations about what I think is a drawback, so that my note can be no longer considered personal or subjective. This will also help the editor taking a sounder decision when reading your comments and prevent embarrassing replies by the authors.
Be fair with the authors
It occasionally happens to receive weird, provocative and even offensive comments by the reviewers. The activity of peer-reviewing has nothing to do with a fight club. The reviewer is not engaged in a battle with the authors, but is only asked to provide expert advice to the Editor of the journal, who is the one and only responsible for the final decision. Therefore, even when the topic, the findings or the conclusions are strongly against your personal beliefs, you will need to express your disagreement with a fair and balanced approach, by constructively emphasizing the negative aspects or expressing an unbiased judgement about the strengths of the article. When communicating opinions about what is needed for improving the quality of the manuscript, the verb “must” should only be used when changes are absolutely necessary, otherwise the verb “should” seems more appropriate.
Weight revision according to the “impact” of the journal
One foremost issue that should guide your comments is the overall “impact” of the journal. It is not the same to accept an assignment to refereeing an article for a “top”, high impact factor journal, or for a local magazine. This aspect is often under-recognized by some reviewers and may also cause problems to the editors. As discussed elsewhere ( 10 ), a small sample size study, decently written, may still be suitable for publication in a non-indexed journal, whilst it is absolutely unfitted for high-impact factor journals. On the contrary, it is not so infrequent to submit an article to a local journal and then receiving the same comments as it had been submitted to Nature or to the New England Journal of Medicine .
The final recommendation
According to journal, once the peer-review process has been concluded, there may be a number of available options to summarize your final recommendations. These can be typically classified in “accept”, “minor revision”, “major revision” or “reject”. There may be other options (e.g., “resubmit as a short communication”, “transform in a letter to the editor”, “reject and resubmit”, “transfer to another journal”, etc.) but, more or less, their significance and consequences are overlapping. The final recommendation should hence be based on some essential and universally accepted criteria. Table 1 summarizes a series of questions that you should answer before deciding as to whether the article needs to be rejected, can be somehow improved by the authors after (minor or major) revision, or can be immediately accepted. You should find a good balance between each “yes”, “partially” or “no” answers that you have given to these questions. This approach is also sometimes available in the website of scientific journals, and is meant to help you (and the Editor) to summarize your previous thoughts. Importantly, your recommendations should be in accordance with the comments you have previously written. It occasionally happens to receive six pages of comments by a referee, which are then synthesized as “minor revision” or, even more ironically, to read a few number of minor issues which are then accompanied by the recommendation to “reject” the manuscript. Constructive criticism should also be expressed when recommending rejection, since this may help the authors improving the work for future submissions to other journals.
You should finally bear in mind that the definitive decision about the fortune of the manuscript will only be made by the editor, and will be weighted against his/her personal view and the comments of other referees (it is likely that the manuscript has been assigned to at least another referee). Therefore, you should not get upset or offended if your recommendation will then be reversed by the editorial office.
Confidentiality
According to the CSE ( 5 ), maintaining the confidentiality of peer-review entails “not sharing, discussing with third parties, or disclosing information from the reviewed paper”. Moreover, peer-reviewers are not allowed to retain copies of the article and are not allowed to use the knowledge of its content for purposes not pertaining to peer-review. Whatever deviation to this practice is seen as a serious misconduct.
Conclusions
As for a general assumption, no single and validated approach exists to peer-review scientific articles. Nevertheless, some simple concepts gathered after years of experience, may help performing this vital activity according to objective and fair rules ( Table 2 ). More or less like writing scientific articles, the activity of refereeing is an ongoing learning. The more you experience, the more you learn. Therefore, I really hope that some notions reported in this dissertation may be a guide or a help, especially for young scientists who are willing to be engaged in peer-reviewing scientific articles.
Acknowledgements
Conflicts of Interest : The author has no conflicts of interest to declare.
- International edition
- Australia edition
- Europe edition
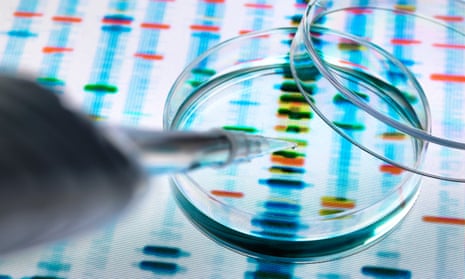
‘The situation has become appalling’: fake scientific papers push research credibility to crisis point
Last year, 10,000 sham papers had to be retracted by academic journals, but experts think this is just the tip of the iceberg
Tens of thousands of bogus research papers are being published in journals in an international scandal that is worsening every year, scientists have warned. Medical research is being compromised, drug development hindered and promising academic research jeopardised thanks to a global wave of sham science that is sweeping laboratories and universities.
Last year the annual number of papers retracted by research journals topped 10,000 for the first time. Most analysts believe the figure is only the tip of an iceberg of scientific fraud .
“The situation has become appalling,” said Professor Dorothy Bishop of Oxford University. “The level of publishing of fraudulent papers is creating serious problems for science. In many fields it is becoming difficult to build up a cumulative approach to a subject, because we lack a solid foundation of trustworthy findings. And it’s getting worse and worse.”
The startling rise in the publication of sham science papers has its roots in China, where young doctors and scientists seeking promotion were required to have published scientific papers. Shadow organisations – known as “paper mills” – began to supply fabricated work for publication in journals there.
The practice has since spread to India, Iran, Russia, former Soviet Union states and eastern Europe, with paper mills supplying fabricated studies to more and more journals as increasing numbers of young scientists try to boost their careers by claiming false research experience. In some cases, journal editors have been bribed to accept articles, while paper mills have managed to establish their own agents as guest editors who then allow reams of falsified work to be published.
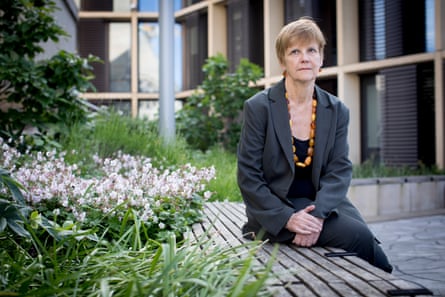
“Editors are not fulfilling their roles properly, and peer reviewers are not doing their jobs. And some are being paid large sums of money,” said Professor Alison Avenell of Aberdeen University. “It is deeply worrying.”
The products of paper mills often look like regular articles but are based on templates in which names of genes or diseases are slotted in at random among fictitious tables and figures. Worryingly, these articles can then get incorporated into large databases used by those working on drug discovery.
Others are more bizarre and include research unrelated to a journal’s field, making it clear that no peer review has taken place in relation to that article. An example is a paper on Marxist ideology that appeared in the journal Computational and Mathematical Methods in Medicine . Others are distinctive because of the strange language they use, including references to “bosom peril” rather than breast cancer and “Parkinson’s ailment” rather Parkinson’s disease.
Watchdog groups – such as Retraction Watch – have tracked the problem and have noted retractions by journals that were forced to act on occasions when fabrications were uncovered. One study, by Nature , revealed that in 2013 there were just over 1,000 retractions. In 2022, the figure topped 4,000 before jumping to more than 10,000 last year.
Of this last total, more than 8,000 retracted papers had been published in journals owned by Hindawi, a subsidiary of the publisher Wiley, figures that have now forced the company to act. “We will be sunsetting the Hindawi brand and have begun to fully integrate the 200-plus Hindawi journals into Wiley’s portfolio,” a Wiley spokesperson told the Observer .
The spokesperson added that Wiley had now identified hundreds of fraudsters present in its portfolio of journals, as well as those who had held guest editorial roles. “We have removed them from our systems and will continue to take a proactive … approach in our efforts to clean up the scholarly record, strengthen our integrity processes and contribute to cross-industry solutions.”
But Wiley insisted it could not tackle the crisis on its own, a message echoed by other publishers, which say they are under siege from paper mills. Academics remain cautious, however. The problem is that in many countries, academics are paid according to the number of papers they have published.
“If you have growing numbers of researchers who are being strongly incentivised to publish just for the sake of publishing, while we have a growing number of journals making money from publishing the resulting articles, you have a perfect storm,” said Professor Marcus Munafo of Bristol University. “That is exactly what we have now.”
The harm done by publishing poor or fabricated research is demonstrated by the anti-parasite drug ivermectin. Early laboratory studies indicated it could be used to treat Covid-19 and it was hailed as a miracle drug. However, it was later found these studies showed clear evidence of fraud, and medical authorities have refused to back it as a treatment for Covid.
“The trouble was, ivermectin was used by anti-vaxxers to say: ‘We don’t need vaccination because we have this wonder drug,’” said Jack Wilkinson at Manchester University. “But many of the trials that underpinned those claims were not authentic.”
Wilkinson added that he and his colleagues were trying to develop protocols that researchers could apply to reveal the authenticity of studies that they might include in their own work. “Some great science came out during the pandemic, but there was an ocean of rubbish research too. We need ways to pinpoint poor data right from the start.”
The danger posed by the rise of the paper mill and fraudulent research papers was also stressed by Professor Malcolm MacLeod of Edinburgh University. “If, as a scientist, I want to check all the papers about a particular drug that might target cancers or stroke cases, it is very hard for me to avoid those that are fabricated. Scientific knowledge is being polluted by made-up material. We are facing a crisis.”
This point was backed by Bishop: “People are building careers on the back of this tidal wave of fraudulent science and could end up running scientific institutes and eventually be used by mainstream journals as reviewers and editors. Corruption is creeping into the system.”
- Peer review and scientific publishing
- The Observer
- Higher education
- Universities
- Newspapers & magazines
- Medical research
Most viewed
- Open access
- Published: 10 April 2024
Development of an index system for the scientific literacy of medical staff: a modified Delphi study in China
- Shuyu Liang 2 na1 ,
- Ziyan Zhai 2 na1 ,
- Xingmiao Feng 2 ,
- Xiaozhi Sun 1 ,
- Jingxuan Jiao 1 ,
- Yuan Gao 1 na2 &
- Kai Meng ORCID: orcid.org/0000-0003-1467-7904 2 , 3 na2
BMC Medical Education volume 24 , Article number: 397 ( 2024 ) Cite this article
Metrics details
Scientific research activity in hospitals is important for promoting the development of clinical medicine, and the scientific literacy of medical staff plays an important role in improving the quality and competitiveness of hospital research. To date, no index system applicable to the scientific literacy of medical staff in China has been developed that can effectively evaluate and guide scientific literacy. This study aimed to establish an index system for the scientific literacy of medical staff in China and provide a reference for improving the evaluation of this system.
In this study, a preliminary indicator pool for the scientific literacy of medical staff was constructed through the nominal group technique ( n = 16) with medical staff. Then, two rounds of Delphi expert consultation surveys ( n = 20) were conducted with clinicians, and the indicators were screened, revised and supplemented using the boundary value method and expert opinions. Next, the hierarchical analysis method was utilized to determine the weights of the indicators and ultimately establish a scientific literacy indicator system for medical staff.
Following expert opinion, the index system for the scientific literacy of medical staff featuring 2 first-level indicators, 9 second-level indicators, and 38 third-level indicators was ultimately established, and the weights of the indicators were calculated. The two first-level indicators were research literacy and research ability, and the second-level indicators were research attitude (0.375), ability to identify problems (0.2038), basic literacy (0.1250), ability to implement projects (0.0843), research output capacity (0.0747), professional capacity (0.0735), data-processing capacity (0.0239), thesis-writing skills (0.0217), and ability to use literature (0.0181).
Conclusions
This study constructed a comprehensive scientific literacy index system that can assess medical staff's scientific literacy and serve as a reference for evaluating and improving their scientific literacy.
Peer Review reports
Due to the accelerated aging of the population and the growing global demand for healthcare in the wake of epidemics, there is an urgent need for medicine to provide greater support and protection. Medical scientific research is a critical element in promoting medical science and technological innovation, as well as improving clinical diagnosis and treatment techniques. It is the main driving force for the development of healthcare [ 1 ].
Medical personnel are highly compatible with clinical research. Due to their close interaction with patients, medical staff are better equipped to identify pertinent clinical research issues and actually implement clinical research projects [ 2 ]. Countries have created favorable conditions for the research and development of medical personnel by providing financial support, developing policies, and offering training courses [ 3 , 4 ]. However, some clinical studies have shown that the ability of most medical staff does not match current health needs and cannot meet the challenges posed by the twenty-first century [ 5 ]. It is clear that highly skilled professionals with scientific literacy are essential for national and social development [ 6 ]. Given the importance of scientific research in countries and hospitals, it is crucial to determine the level of scientific research literacy that medical personnel should possess and how to train them to acquire the necessary scientific research skills. These issues have significant practical implications.
Scientific literacy refers to an individual's ability to engage in science-related activities [ 7 ]. Some scholars suggest that the scientific literacy of medical personnel encompasses the fundamental qualities required for scientific research work, encompassing three facets: academic moral accomplishment, scientific research theory accomplishment, and scientific research ability accomplishment [ 8 ]. The existing research has focused primarily on the research capabilities of medical staff. According to Rillero, problem-solving skills, critical thinking, communication skills, and the ability to interpret data are the four core components of scientific literacy [ 9 ]. The ability to perform scientific research in nursing encompasses a range of abilities, including identifying problems, conducting literature reviews, designing and conducting scientific research, practicing scientific research, processing data, and writing papers [ 10 ]. Moule and Goodman proposed a framework of skills that research-literate nurses should possess, such as critical thinking capacity, analytical skills, searching skills, research critique skills, the ability to read and critically appraise research, and an awareness of ethical issues [ 11 ]. Several researchers have developed self-evaluation questionnaires to assess young researchers' scientific research and innovative abilities in the context of university-affiliated hospitals (UHAs) [ 12 ]. The relevant indicators include sensitivity to problems, sensitivity to cutting-edge knowledge, critical thinking, and other aspects. While these indicators cover many factors, they do not consider the issue of scientific research integrity in the medical field. The lack of detailed and targeted indicators, such as clinical resource collection ability and interdisciplinary cooperation ability, hinders the effective measurement of the current status of scientific literacy among medical staff [ 12 ]. In conclusion, the current research on the evaluation indicators of scientific literacy among medical personnel is incomplete, overlooking crucial humanistic characteristics, attitudes, and other moral literacy factors. Therefore, there is an urgent need to establish a comprehensive and systematic evaluation index to effectively assess the scientific literacy of medical staff.
Therefore, this study utilized a literature search and nominal group technique to screen the initial evaluation index and subsequently constructed an evaluation index system for medical staff's scientific research literacy utilizing the Delphi method. This index system would serve as a valuable tool for hospital managers, aiding them in the selection, evaluation, and training of scientific research talent. Additionally, this approach would enable medical personnel to identify their own areas of weakness and implement targeted improvement strategies.
Patient and public involvement
Patients and the public were not involved in this research.
Study design and participants
In this study, an initial evaluation index system was developed through a literature review and nominal group technique. Subsequently, a more comprehensive and scientific index system was constructed by combining qualitative and quantitative analysis utilizing the Delphi method to consult with experts. Finally, the hierarchical analysis method and the percentage weight method were employed to empower the index system.
The program used for this study is shown in Fig. 1 .
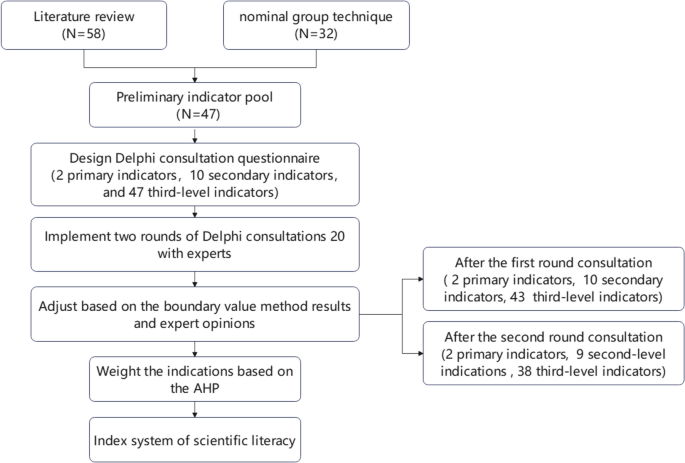
Study design. AHP, analytic hierarchy process
Establishing the preliminary indicator pool
Search process.
A literature search was performed in the China National Knowledge Infrastructure (CNKI), WanFang, PubMed, Web of Science and Scopus databases to collect the initial evaluation indicators. The time span ranged from the establishment of the database to July 2022. We used a combination of several MeSH terms in our searches:(("Medical Staff"[Mesh] OR "Nurses"[Mesh] OR "Physicians"[Mesh])) AND (("Literacy"[Mesh]) OR "Aptitude"[Mesh]). We also used several Title/Abstract searches, including keywords such as: Evaluation, scientific literacy, research ability.
The inclusion criteria were as follows: (1)The subjects were nurses, medicial staff and other personnel engaged in the medical industry; (2) Explore topics related to scientific literacy, such as research ability, and literature that can clarify the structure or dependency between indicators of scientific literacy; (3) Select articles published in countries such as China, the United States, the United Kingdom, Australia and Canada; (4) Research published in English or Chinese is considered to be eligible. The exclusion criteria are as follows: (1) indicators not applicable to medical staff; (2) Conference abstracts, case reports or review papers; (3) Articles with repeated descriptions; (4) There are no full-text articles or grey literature. A total of 78 articles were retrieved and 60 were retained after screening according to inclusion and exclusion criteria.
The research was conducted by two graduate students and two undergraduate students who participated in the literature search and screening. The entire research process was supervised and guided by one professor. All five members were from the fields of social medicine and health management. The professor was engaged in hospital management and health policy research for many years.
Nominal group technique
The nominal group technique was introduced at Hospital H in Beijing in July 2022. This hospital, with over 2,500 beds and 3,000 doctors, is a leading comprehensive medical center also known for its educational and research achievements, including numerous national research projects and awards.
The interview questions were based on the research question: What research literacy should medical staff have? 16 clinicians and nurses from Hospital H were divided into 2 equal groups and asked to provide their opinions on important aspects of research literacy based on their positions and experiences. Once all participants had shared their thoughts, similar responses were merged and polished. If anyone had further inputs after this, a second round of interviews was held until no new inputs were given. The entire meeting, including both rounds, was documented by researchers with audio recordings on a tape recorder.
Scientific literacy dimensions
Based on the search process, the research group extracted 58 tertiary indicators. To ensure the practicality and comprehensiveness of the indicators, the Nominal group technique was used on the basis of the literature search. Panelists summarized the entries shown in the interviews and merged similar content to obtain 32 third-level indicators. The indicators obtained from the literature search were compared. Several indicators with similar meanings, such as capture information ability, language expression ability, communication ability, and scientific research integrity, were merged. Additionally, the indicators obtained from the literature search, such as scientific research ethics, database use ability, feasibility and analysis ability, were added to the 15 indicators. A total of 47 third-level indicators were identified.
Fengling Dai and colleagues developed an innovation ability index system with six dimensions covering problem discovery, information retrieval, research design, practice, data analysis, and report writing, which represents the whole of innovative activity. Additionally, the system includes an innovation spirit index focusing on motivation, thinking, emotion, and will, reflecting the core of the innovation process in terms of competence [ 13 ]. Liao et al. evaluated the following five dimensions in their study on scientific research competence: literature processing, experimental manipulation, statistical analysis, manuscript production, and innovative project design [ 14 ]. Mohan claimed that scientific literacy consists of four core components: problem solving, critical thinking, communication skills, and the ability to interpret data [ 15 ].
This study structured scientific literacy into 2 primary indicators (research literacy and research competence) and 9 secondary indicators (basic qualifications, research ethics, research attitude, problem identification, literature use, professional capacity, subject implementation, data processing, thesis writing, and research output).
Using the Delphi method to develop an index system
Expert selection.
This study used the Delphi method to distribute expert consultation questionnaires online, allowing experts to exchange opinions anonymously to ensure that the findings were more desirable and scientific. No fixed number of experts is required for a Delphi study, but the more experts involved, the more stable the results will be [ 16 ]; this method generally includes 15 to 50 experts [ 17 ]. We selected clinicians from several tertiary hospitals in the Beijing area to serve as Delphi study consultants based on the following inclusion criteria: (1) they had a title of senior associate or above; (2) they had more than 10 years of work experience in the field of clinical scientific research, and (3) they were presiding over national scientific research projects. The exclusion criteria were as follows: (1) full-time scientific researchers, and (2) personnel in hospitals who were engaged only in management. To ensure that the selected experts were representative, this study selected 20 experts from 14 tertiary hospitals affiliated with Capital Medical University, Peking University, the Chinese Academy of Medical Sciences and the China Academy of Traditional Chinese Medicine according to the inclusion criteria; the hospitals featured an average of 1,231 beds each, and 9 hospitals were included among the 77 hospitals in the domestic comprehensive hospital ranking (Fudan Hospital Management Institute ranking). The experts represented various specialties and roles from different hospitals, including cardiology, neurosurgery, neurology, ear and throat surgery, head and neck surgery, radiology, imaging, infection, vascular interventional oncology, pediatrics, general practice, hematology, stomatology, nephrology, urology, and other related fields. This diverse group included physicians, nurses, managers, and vice presidents. The selected experts had extensive clinical experience, achieved numerous scientific research accomplishments and possessed profound knowledge and experience in clinical scientific research. This ensured the reliability of the consultation outcomes.
Design of the expert consultation questionnaire
The Delphi survey for experts included sections on their background, familiarity with the indicator system, system evaluation, and opinions. Experts rated indicators on importance, feasibility, and sensitivity using a 1–10 scale and their own familiarity with the indicators on a 1–5 scale. They also scored their judgment basis and impact on a 1–3 scale, considering theoretical analysis, work experience, peer understanding, and intuition. Two rounds of Delphi surveys were carried out via email with 20 experts to evaluate and suggest changes to the indicators. Statistical coefficients were calculated to validate the Delphi process. Feedback from the first round led to modifications and the inclusion of an AHP questionnaire for the second round. After the second round, indicators deemed less important were removed, and expert discussion finalized the indicator weights based on their relative importance scores. This resulted in the development of an index system for medical staff scientific literacy. The questionnaire is included in Additional file 1 (first round) and Additional file 2 (second round).
Using the boundary value method to screen the indicators
In this study, the boundary value method was utilized to screen the indicators of medical staff's scientific literacy, and the importance, feasibility, and sensitivity of each indicator were measured using the frequency of perfect scores, the arithmetic mean, and the coefficient of variation, respectively. When calculating the frequency of perfect scores and arithmetic means, the boundary value was set as "mean-SD," and indicators with scores higher than this value were retained. When calculating the coefficient of variation, the cutoff value was set to "mean + SD," and indicators with values below this threshold were retained.
The principles of indicator screening are as follows:
To evaluate the importance of the indicators, if none of the boundary values of the three statistics met the requirements, the indicators were deleted.
If an indicator has two aspects, importance, feasibility, or sensitivity, and each aspect has two or more boundary values that do not meet the requirements, then the indicator is deleted.
If all three boundary values for an indicator meet the requirements, the research group discusses the modification feedback from the experts and determines whether the indicator should be used.
The results of the two rounds of boundary values are shown in Table 1 .
Using the AHP to assign weights
After the second round of Delphi expert consultations, the analytic hierarchy process (AHP) was used to determine the weights of the two first-level indicators and the nine second-level indicators. The weights of the 37 third-level indicators were subsequently calculated via the percentage weight method. The AHP, developed by Saaty in the 1980s, is used to determine the priority and importance of elements constituting the decision-making hierarchy. It is based on multicriteria decision-making (MCDM) and determines the importance of decision-makers' judgments based on weights derived from pairwise comparisons between elements. In the AHP, pairwise comparisons are based on a comparative evaluation in which each element's weight in the lower tier is compared with that of other lower elements based on the element in the upper tier [ 18 ].
AHP analysis involves the following steps:
Step 1: Establish a final goal and list related elements to construct a hierarchy based on interrelated criteria.
Step 2: Perform a pairwise comparison for each layer to compare the weights of each element. Using a score from 1 to 9, which is the basic scale of the AHP, each pair is compared according to the expert’s judgment, and the importance is judged [ 19 , 20 ].
Yaahp software was employed to analyze data by creating a judgment matrix based on the experts' scores and hierarchical model. The index system weights were obtained by combining the experts' scores. The percentage weight method used experts' importance ratings from the second round to calculate weights, ranking indicators by importance, calculating their scores based on frequency of ranking, and determining weighting coefficients by dividing these scores by the total of all third-level indicators' scores. The third-level indicator weighting coefficients were then calculated by multiplying the coefficients [ 21 ].
Data analysis
Expert positivity coefficient.
The expert positivity coefficient is indicated by the effective recovery rate of the expert consultation questionnaire, which represents the level of expert positivity toward this consultation and determines the credibility and scientific validity of the questionnaire results. Generally, a questionnaire with an effective recovery rate of 70% is considered very good [ 22 ].
In this study, 20 questionnaires were distributed in both rounds of Delphi expert counseling, and all 20 were effectively recovered, resulting in a 100% effective recovery rate. Consequently, the experts provided positive feedback on the Delphi counseling.
Expert authority coefficient (CR)
The expert authority coefficient (Cr) is the arithmetic mean of the judgment coefficient (Ca) and the familiarity coefficient (Cs), namely, Cr = \(\frac{({\text{Ca}}+{\text{Cs}})}{2}\) . The higher the degree of expert authority is, the greater the predictive accuracy of the indicator. A Cr ≥ 0.70 was considered to indicate an acceptable level of confidence [ 23 ]. Ca represents the basis on which the expert makes a judgment about the scenario in question, while Cs represents the expert's familiarity with the relevant problem [ 24 ].
Ca is calculated on the basis of experts' judgments of each indicator and the magnitude of its influence. In this study, experts used "practical experience (0.4), "theoretical analysis (0.3), "domestic and foreign peers (0.2)" and "intuition (0.1)" as the basis for judgment and assigned points according to the influence of each basis for judgment on the experts' judgment. Ca = 1 when the basis for judgment has a large influence on the experts, and Ca = 0.5 when the influence of the experts' judgment is at a medium level. When no influence on expert judgment was evident, Ca = 0 [ 25 ] (Table 2 ).
Cs refers to the degree to which the expert was familiar with the question. This study used the Likert scale method to score experts’ familiarity with the question on a scale ranging from 0 to 1 (1 = very familiar, 0.75 = more familiar, 0.5 = moderately familiar, 0.25 = less familiar, 0 = unfamiliar). The familiarity coefficient for each expert (the average familiarity for each indicator) was calculated. The average familiarity coefficient was subsequently computed [ 26 ].
The Cr value of the primary indicator in this study was 0.83, and the Cr value of the secondary indicator was 0.82 (> 0.7); hence, the results of the expert consultation were credible and accurate, as shown in Table 3 .
The degree of expert coordination is an important indicator used to judge the consistency among various experts regarding indicator scores. This study used the Kendall W coordination coefficient test to determine the degree of expert coordination. A higher Kendall W coefficient indicates a greater degree of expert coordination and greater consistency in expert opinion, and P < 0.05 indicates that the difference is significant [ 26 ]. The results of the three-dimensional harmonization coefficient test for each indicator in the two rounds of the expert consultation questionnaire were valid ( p < 0.01 ), emphasizing the consistency of the experts' scores. The values of the Kendall W coordination coefficients for both rounds are shown in Table 4 .
Basic information regarding the participants
The 20 Delphi experts who participated in this study were predominantly male (80.0%) rather than female (20.0%). In addition, the participants’ ages were mainly concentrated in the range of 41–50 years old (60.0%). The majority of the experts were doctors by profession (85.0%), and their education and titles were mainly doctoral degree (90.0%) and full senior level (17.0%). The experts also exhibited high academic achievement in their respective fields and had many years of working experience, with the majority having between 21 and 25 years of experience (40.0%) (Table 5 ).
Index screening
The boundary value method was applied to eliminate indicators, leading to the removal of 6 third-level indicators in the first round. One of these, the ability to use statistical software, was associated with a more significant second-level indicator involving data processing, which was kept after expert review. Six indicators were merged into three indicators due to duplication, and 5 third-level indicators were added, resulting in 2 primary indicators, 10 secondary indicators, and 43 third-level indicators.
In the second round of Delphi expert consultation, 5 third-level indicators were deleted, as shown in Additional file 3 , and only one third-level indicator, "Scientific spirit", remained under the secondary indicator "research attitude". The secondary indicator "Research attitude" was combined with "Research ethics" and the third-level indicator "Scientific spirit" was also considered part of "Research ethics". After expert discussion, these were merged into a new secondary indicator "Research attitude" with three third-level indicators: "Research ethics", "Research integrity", and "Scientific spirit". The final index system included two primary indicators, nine secondary indicators, and thirty-eight third-level indicators, as shown in Additional File 3 .
Final index system with weights
The weights of the two primary indexes, research literacy and research ability, were equal. This was determined using the hierarchical analysis method and the percentage weight method based on the results of the second round of Delphi expert consultation (Table 6 ). The primary indicator of research literacy encompasses the fundamental qualities and attitudes medical staff develop over time, including basic qualifications and approach to research. The primary indicator of research ability refers to medical professionals' capacity to conduct scientific research in new areas using suitable methods, as well as their skills needed for successful research using scientific methods.
In this study, the Delphi method was employed, and after two rounds of expert consultation, in accordance with the characteristics and scientific research requirements of medical staff in China, an index system for the scientific literacy of medical staff in China was constructed. The index system for medical staff's scientific literacy in this study consists of 2 first-level indicators, 9 second-level indicators, and 38 third-level indicators. Medical institutions at all levels can use this index system to scientifically assess medical staff's scientific literacy.
In 2014, the Joint Task Force for Clinical Trial Competency (JTF) published its Core Competency Framework [ 27 ]. The Framework focuses more on the capacity to conduct clinical research. These include principles such as clinical research and quality practices for drug clinical trials. However, this framework does not apply to the current evaluation of scientific literacy in hospitals. Because these indicators do not apply to all staff members, there is a lack of practical scientific research, such as information about the final paper output. Therefore, the experts who constructed the index system in this study came from different specialties, and the indicators can be better applied to scientific researchers in all fields. This approach not only addresses clinical researchers but also addresses the concerns of hospital managers, and the indicators are more applicable.
The weighted analysis showed that the primary indicators "research literacy" and "research ability" had the same weight (0.50) and were two important components of scientific literacy. Research ability is a direct reflection of scientific literacy and includes the ability to identify problems, the ability to use literature, professional capacity, subject implementation capacity, data-processing capacity, thesis-writing skills, and research output capacity. Only by mastering these skills can medical staff carry out scientific research activities more efficiently and smoothly. The ability to identify problems refers to the ability of medical staff to obtain insights into the frontiers of their discipline and to identify and ask insightful questions. Ratten claimed that only with keen insight and sufficient sensitivity to major scientific issues can we exploit the opportunities for innovation that may lead to breakthroughs [ 28 ]. Therefore, it is suggested that in the process of cultivating the scientific literacy of medical staff, the ability to identify problems, including divergent thinking, innovative sensitivity, and the ability to produce various solutions, should be improved. Furthermore, this study included three subentries of the secondary indicator "research attitude", namely, research ethics, research integrity, and scientific spirit. This is likely because improper scientific research behavior is still prevalent. A study conducted in the United States and Europe showed that the rate of scientific research misconduct was 2% [ 13 ]. A small survey conducted in Indian medical schools and hospitals revealed that 57% of the respondents knew that someone had modified or fabricated data for publication [ 28 ]. The weight of this index ranked first in the secondary indicators, indicating that scientific attitude is an important condition for improving research quality, relevance, and reliability. Countries and hospitals should develop, implement, and optimize policies and disciplinary measures to combat academic misconduct.
In addition, the third-level indicator "scheduling ability" under the second-level indicator "basic qualification" has a high weight, indicating that medical staff attach importance to management and distribution ability in the context of scientific research. Currently, hospitals face several problems, such as a shortage of medical personnel, excessive workload, and an increase in the number of management-related documents [ 29 , 30 ]. These factors result in time conflicts between daily responsibilities and scientific research tasks, thereby presenting significant obstacles to the allocation of sufficient time for scientific inquiry [ 31 ]. Effectively arranging clinical work and scientific research time is crucial to improving the overall efficiency of scientific research. In the earlier expert interviews, most medical staff believed that scientific research work must be combined with clinical work rather than focused only on scientific research. Having the ability to make overall arrangements is essential to solving these problems. The high weight given to the second-level index of 'subject implementation capacity', along with its associated third-level indicators, highlights the challenges faced by young medical staff in obtaining research subjects. Before implementing a project, researchers must thoroughly investigate, analyze, and compare various aspects of the research project, including its technical, economic, and engineering aspects. Moreover, potential financial and economic benefits, as well as social impacts, need to be predicted to determine the feasibility of the project and develop a research plan [ 32 ]. However, for most young medical staff in medical institutions, executing such a project can be challenging due to their limited scientific research experience [ 33 ]. A researcher who possesses these skills can truly carry out independent scientific research.
The weights of the second-level index "research output capacity" cannot be ignored. In Chinese hospitals, the ability to produce scientific research output plays a certain role in employees’ ability to obtain rewards such as high pay, and this ability is also used as a reference for performance appraisals [ 34 ]. The general scientific research performance evaluation includes the number of projects, scientific papers and monographs, scientific and technological achievements, and patents. In particular, the publication of papers is viewed as an indispensable aspect of performance appraisal by Chinese hospitals [ 35 ]. Specifically, scientific research papers are the carriers of scientific research achievements and academic research and thus constitute an important symbol of the level of medical development exhibited by medical research institutions; they are thus used as recognized and important indicators of scientific research output [ 36 ]. This situation is consistent with the weight evaluation results revealed by this study.
The results of this study are important for the training and management of the scientific research ability of medical personnel. First, the index system focuses not only on external characteristics such as scientific knowledge and skills but also on internal characteristics such as individual traits, motivation, and attitudes. Therefore, when building a research team and selecting and employing researchers, hospital managers can use the index system to comprehensively and systematically evaluate the situation of researchers, which is helpful for optimizing the allocation of a research team, learning from each other's strengths, and strengthening the strength of the research team. Second, this study integrates the content of existing research to obtain useful information through in-depth interviews with medical staff and constructs an evaluation index system based on Delphi expert consultation science, which comprehensively includes the evaluation of the whole process of scientific research activities. These findings can provide a basis for medical institutions to formulate scientific research training programs, help medical personnel master and improve scientific research knowledge and skills, and improve their working ability and quality. Moreover, the effectiveness of the training can also be evaluated according to the system.
In China, with the emergence of STEM rankings, hospitals pay more and more attention to the scientific research performance of medical personnel. Scientific literacy not only covers the abilities of medical personnel engaged in scientific research, but also reflects their professional quality in this field. Having high quality medical personnel often means that they have excellent scientific research ability, and their scientific research performance will naturally rise. In view of this,,medical institutions can define the meaning of third-level indicators and create Likert scales to survey medical staff. Based on the weights assigned to each indicator, comprehensive scores can be calculated to evaluate the level of scientific literacy among medical staff. Through detailed data analysis, they can not only reveal their shortcomings in scientific research ability and quality, but also provide a strong basis for subsequent training and promotion. Through targeted inspection, we can not only promote the comprehensive improvement of the ability of medical staff, but also promote the steady improvement of their scientific research performance, and inject new vitality into the scientific research cause of hospitals.
Limitations
This study has several limitations that need to be considered. First, the participants were only recruited from Beijing (a city in China), potentially lacking geographical diversity. We plan to select more outstanding experts from across the country to participate. Second, the index system may be more suitable for countries with medical systems similar to those of China. When applying this system in other countries, some modifications may be necessary based on the local context. Last, While this study has employed scientific methods to establish the indicator system, the index system has yet to be implemented on a large sample of medical staff. Therefore, the reliability and validity of the index system must be confirmed through further research. In conclusion, it is crucial to conduct further detailed exploration of the effectiveness and practical application of the index system in the future.
This study developed an evaluation index system using the Delphi method to assess the scientific literacy of medical staff in China. The system comprises two primary indicators, nine secondary indicators, and thirty-eight third-level indicators, with each index assigned a specific weight. The index system emphasizes the importance of both attitudes and abilities in the scientific research process for medical staff and incorporates more comprehensive evaluation indicators. In the current era of medical innovation, enhancing the scientific literacy of medical staff is crucial for enhancing the competitiveness of individuals, hospitals, and overall medical services in society. This evaluation index system is universally applicable and beneficial for countries with healthcare systems similar to those of China. This study can serve as a valuable reference for cultivating highly qualified and capable research personnel and enhancing the competitiveness of medical research.
Availability of data and materials
The datasets used and/or analysed during the current study available from the corresponding author on reasonable request.
Coloma J, Harris E. From construction workers to architects: developing scientific research capacity in low-income countries. PLoS Biol. 2009;7(7):e1000156. https://doi.org/10.1371/journal.pbio.1000156 .
Article Google Scholar
Brauer SG, Haines TP, Bew PG. Fostering clinician-led research. Aust J Physiother. 2007;53(3):143–4. https://doi.org/10.1016/s0004-9514(07)70020-x .
The L. China’s research renaissance. Lancet. 2019;393(10179):1385. https://doi.org/10.1016/S0140-6736(19)30797-4 .
Hannay DR. Evaluation of a primary care research network in rural Scotland. Prim Health Care ResDevelop. 2006;7(3):194–200. https://doi.org/10.1191/1463423606pc296oa .
Frenk J, Chen L, Bhutta ZA, Cohen J, Crisp N, Evans T, et al. Health professionals for a new century: transforming education to strengthen health systems in an interdependent world. Lancet. 2010;376:1923–58.
Xie Y, Wang J, Li S, Zheng Y. Research on the Influence Path of Metacognitive Reading Strategies on Scientific Literacy. J Intell. 2023;11(5):78. https://doi.org/10.3390/jintelligence11050078 . PMID: 37233327; PMCID: PMC10218841.
Pang YH, Cheng JL. Revise of scientific research ability self-evaluation rating scales of nursing staff. Chin Nurs Res. 2011;13:1205–8. https://doi.org/10.3969/j.issn.1009-6493.2011.13.040 .
Zhang J, Jianshan MAO, Gu Y. On the cultivation of scientific research literacy of medical graduate students. Continu Med Educ China. 2023;15(3):179–82. https://doi.org/10.3969/j.issn.1674-9308.2023.03.043 .
Rillero P. Process skills and content knowledge. Sci Act. 1998;3:3–4.
Google Scholar
Liu RS. Study on reliability and validity of self rating scale for scientific research ability of nursing staff. Chinese J Pract Nurs. 2004;9:8–10. https://doi.org/10.3760/cma.j.issn.1672-7088.2004.09.005 .
Moule P, Goodman M. Nursing research: An introduction. London, UK: Sage; 2013.
Xue J, Chen X, Zhang Z, et al. Survey on status quo and development needs of research and innovation capabilities of young researchers at university-affiliated hospitals in China: a cross-sectional survey. Ann Transl Med. 2022;10(18):964. https://doi.org/10.21037/atm-22-3692 .
Fanelli D, Costas R, Fang FC, et al. Testing hypotheses on risk factors for scientific misconduct via matched-control analysis of papers containing problematic image duplications. Sci Eng Ethics. 2019;25(3):771–89. https://doi.org/10.1007/s11948-018-0023-7 .
Liao Y, Zhou H, Wang F, et al. The Impact of Undergraduate Tutor System in Chinese 8-Year Medical Students in Scientific Research. Front Med (Lausanne). 2022;9:854132. https://doi.org/10.3389/fmed.2022.854132 .
Mohan L, Singh Y, Kathrotia R, et al. Scientific literacy and the medical student: A cross-sectional study. Natl Med J India. 2020;33(1):35–7. https://doi.org/10.4103/0970-258X.308242 .
Jorm AF. Using the Delphi expert consensus method in mental health research. Aust N Z J Psychiatry. 2015;49(10):887–97. https://doi.org/10.1177/0004867415600891 .
Xinran S, Heping W, Yule H, et al. Defining the scope and weights of services of a family doctor service project for the functional community using Delphi technique and analytic hierarchy process. Chinese Gen Pract. 2021;24(34):4386–91.
Park S, Kim HK, Lee M. An analytic hierarchy process analysis for reinforcing doctor-patient communication. BMC Prim Care. 2023;24(1):24. https://doi.org/10.1186/s12875-023-01972-3 . Published 2023 Jan 21.
Zhou MLY, Yin H, et al. New screening tool for neonatal nutritional risk in China: a validation study. BMJ Open. 2021;11(4):e042467. https://doi.org/10.1136/bmjopen-2020-042467 .
Wang K, Wang Z, Deng J, et al. Study on the evaluation of emergency management capacity of resilient communities by the AHP-TOPSIS method. Int J Environ Res Public Health. 2022;19(23):16201. https://doi.org/10.3390/ijerph192316201 .
Yuwei Z, Chuanhui Y, Junlong Z, et al. Application of analytic Hierarchy Process and percentage weight method to determine the weight of traditional Chinese medicine appropriate technology evaluation index system. Chin J Tradit Chinese Med. 2017;32(07):3054–6.
Babbie E. The practice of social research. 10th Chinese language edition. Huaxia Publisher, 2005: 253–4.
Liu W, Hu M, Chen W. Identifying the Service Capability of Long-Term Care Facilities in China: an e-Delphi study. Front Public Health. 2022;10:884514. https://doi.org/10.3389/fpubh.2022.884514 .
Zeng G. Modern epidemiological methods and application. Pecking Union Medical College Union Press, 1996.
Geng Y, Zhao L, Wang Y, et al. Competency model for dentists in China: Results of a Delphi study. PLoS One. 2018;13(3):e0194411. https://doi.org/10.1371/journal.pone.0194411 .
Cong C, Liu Y, Wang R. Kendall coordination coefficient W test and its SPSS implementation. Journal of Taishan Medical College. 2010;31(7):487–490. https://doi.org/10.3969/j.issn.1004-7115.2010.07.002 .
Sonstein S, Seltzer J, Li R, et al. Moving from compliance to competency: a harmonized core competency framework for the clinical research professional. Clin Res. 2014;28(3):17–23.
Madan C, Kruger E, Tennant M. 30 Years of dental research in Australia and India: a comparative analysis of published peer review literature. Indian J Dent Res. 2012;23(2):293–4. https://doi.org/10.4103/0970-9290.100447 .
Siemens DR, Punnen S, Wong J, Kanji N. A survey on the attitudes towards research in medical school. BMC Med Educ. 2010;10:4. https://doi.org/10.1186/1472-6920-10-4 .
Solomon SS, Tom SC, Pichert J, Wasserman D, Powers AC. Impact of medical student research in the development of physician-scientists. J Investig Med. 2003;51(3):149–56. https://doi.org/10.1136/jim-51-03-17 .
Misztal-Okonska P, Goniewicz K, Hertelendy AJ, et al. How Medical Studies in Poland Prepare Future Healthcare Managers for Crises and Disasters: Results of a Pilot Study. Healthcare (Basel). 2020;8(3):202. https://doi.org/10.3390/healthcare8030202 .
Xu G. On the declaration of educational scientific research topics. Journal of Henan Radio & TV University. 2013;26(01):98–101.
Ju Y, Zhao X. Top three hospitals clinical nurse scientific research ability present situation and influence factors analysis. J Health Vocational Educ. 2022;40(17):125–8.
Zhu Q, Li T, Li X, et al. Industry gain public hospital medical staff performance distribution mode of integration, exploring. J Health Econ Res. 2022;33(11):6-82–6.
Sun YLL. Analysis of hospital papers published based on performance appraisal. China Contemp Med. 2015;22(31):161–3.
Jian Y, Wu J, Liu Y. Citation analysis of seven tertiary hospitals in Yunnan province from 2008 to 2012. Yunnan Medicine. 2014;(6):700–704.
Download references
Acknowledgements
The authors thank all who participated in the nominal group technique and two rounds of the Delphi study.
This study was supported by the National Natural Science Foundation of China (72074160) and the Natural Science Foundation Project of Beijing (9222004).
Author information
Shuyu Liang and Ziyan Zhai contributed equally to this work and joint first authors.
Kai Meng and Yuan Gao contributed equally to this work and share corresponding author.
Authors and Affiliations
Aerospace Center Hospital, No. 15 Yuquan Road, Haidian District, Beijing, 100049, China
Xiaozhi Sun, Jingxuan Jiao & Yuan Gao
School of Public Health, Capital Medical University, No.10 Xitoutiao, Youanmenwai Street, Fengtai District, Beijing, 100069, China
Shuyu Liang, Ziyan Zhai, Xingmiao Feng & Kai Meng
Beijing Tiantan Hospital, Capital Medical University, No. 119 South Fourth Ring West Road, Fengtai District, Beijing, 100070, China
You can also search for this author in PubMed Google Scholar
Contributions
S.L. and Z.Z. contributed equally to this paper. S.L. took charge of the nominal group technique, data analysis, writing the first draft and revising the manuscript; Z.Z. was responsible for the Delphi survey, data analysis, and writing of the first draft of the manuscript; XF was responsible for the rigorous revision of Delphi methods; X.S. and J.J. were responsible for the questionnaire survey and data collection; Y.G. contributed to the questionnaire survey, organization of the nominal group interview, supervision, project administration and resources; and K.M. contributed to conceptualization, methodology, writing—review; editing, supervision, and project administration. All the authors read and approved the final manuscript.
Corresponding authors
Correspondence to Yuan Gao or Kai Meng .
Ethics declarations
Ethics approval and consent to participate.
This study involved human participants and was approved by the Ethical Review Committee of the Capital Medical University (No. Z2022SY089). Participation in the survey was completely voluntary, and written informed consent was obtained from the participants.
Consent for publication
Not applicable.
Competing interests
The authors declare no competing interests.
Additional information
Publisher’s note.
Springer Nature remains neutral with regard to jurisdictional claims in published maps and institutional affiliations.
Supplementary Information
Supplementary material 1., supplementary material 2., supplementary material 3., rights and permissions.
Open Access This article is licensed under a Creative Commons Attribution 4.0 International License, which permits use, sharing, adaptation, distribution and reproduction in any medium or format, as long as you give appropriate credit to the original author(s) and the source, provide a link to the Creative Commons licence, and indicate if changes were made. The images or other third party material in this article are included in the article's Creative Commons licence, unless indicated otherwise in a credit line to the material. If material is not included in the article's Creative Commons licence and your intended use is not permitted by statutory regulation or exceeds the permitted use, you will need to obtain permission directly from the copyright holder. To view a copy of this licence, visit http://creativecommons.org/licenses/by/4.0/ . The Creative Commons Public Domain Dedication waiver ( http://creativecommons.org/publicdomain/zero/1.0/ ) applies to the data made available in this article, unless otherwise stated in a credit line to the data.
Reprints and permissions
About this article
Cite this article.
Liang, S., Zhai, Z., Feng, X. et al. Development of an index system for the scientific literacy of medical staff: a modified Delphi study in China. BMC Med Educ 24 , 397 (2024). https://doi.org/10.1186/s12909-024-05350-0
Download citation
Received : 25 October 2023
Accepted : 26 March 2024
Published : 10 April 2024
DOI : https://doi.org/10.1186/s12909-024-05350-0
Share this article
Anyone you share the following link with will be able to read this content:
Sorry, a shareable link is not currently available for this article.
Provided by the Springer Nature SharedIt content-sharing initiative
- Medical staff
- Scientific literacy
- Evaluation indicators
BMC Medical Education
ISSN: 1472-6920
- Submission enquiries: [email protected]
- General enquiries: [email protected]
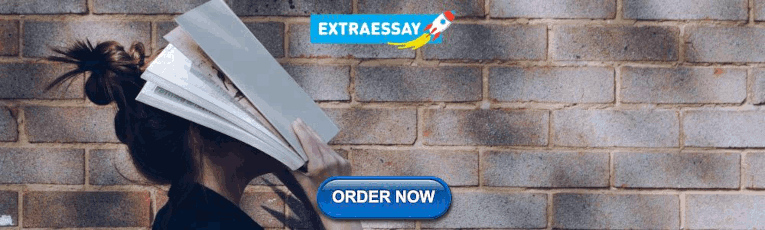
IMAGES
VIDEO
COMMENTS
Google Scholar provides a simple way to broadly search for scholarly literature. Search across a wide variety of disciplines and sources: articles, theses, books, abstracts and court opinions.
3.3 million articles on ScienceDirect are open access. Articles published open access are peer-reviewed and made freely available for everyone to read, download and reuse in line with the user license displayed on the article. ScienceDirect is the world's leading source for scientific, technical, and medical research.
Harness the power of visual materials—explore more than 3 million images now on JSTOR. Enhance your scholarly research with underground newspapers, magazines, and journals. Explore collections in the arts, sciences, and literature from the world's leading museums, archives, and scholars. JSTOR is a digital library of academic journals ...
Open access publisher of peer-reviewed scientific articles across the entire spectrum of academia. Research network for academics to stay up-to-date with the latest scientific publications, events, blogs and news.
A scientific hypothesis or statement is generally not accepted by the academic community unless it has been published in a peer-reviewed journal . The Institute for Scientific Information (ISI) only considers journals that are peer-reviewed as candidates to receive Impact Factors. Peer review is a well-established process which has been a ...
Rigorously reported, peer reviewed and immediately available without restrictions, promoting the widest readership and impact possible. We encourage you to consider the scope of each journal before submission, as journals are editorially independent and specialized in their publication criteria and breadth of content. PLOS Biology PLOS Climate
First published in 1869, Nature is the world's leading multidisciplinary science journal. Nature publishes the finest peer-reviewed research that drives ground-breaking discovery, and is read by ...
Scientific Journals. AAAS publishes six respected peer-reviewed journals. Science, the premier global science weekly; Science Signaling, the leading journal of cell signaling and regulatory biology; Science Translational Medicine, integrating medicine, engineering and science to promote human health; Science Advances, an innovative and high ...
PubMed Central ® (PMC) is a free full-text archive of biomedical and life sciences journal literature at the U.S. National Institutes of Health's National Library of Medicine (NIH/NLM) ... Discover a digital archive of scholarly articles, spanning centuries of scientific research. User Guide Learn how to find and read articles of interest to ...
Nature is a weekly international journal publishing the finest peer-reviewed research in all fields of science and technology on the basis of its originality, importance, interdisciplinary ...
Wolbachia effects in mosquitoes. Wolbachia -induced cytoplasmic incompatibility in fruit flies is known to cause embryonic lethality by modifying chromatin integrity in developing sperm. Rupinder Kaur, Seth Bordenstein and co-workers reveal an analogous mechanism in the w Mel-transinfected Aedes aegypti mosquitoes that are used to control ...
Facilitate interdisciplinary research and scholarship across 2,900 peer-reviewed journals. . 21M articles & book chapters. . 800 open access journals. . 3.3M open access articles. Learn more about our journal collections. Get the facts: Learn how ScienceDirect helps students, educators and researchers achieve their goals.
From agriculture to pharmaceuticals, discover how our peer-reviewed journals, e-books, and educational content can provide new insight in the most important areas of scientific research. ATTRACTIVE ACCESS OPTIONS. From providing the best scientific resources to students and faculty, to always-up-to-date research libraries for corporate or ...
The peer review process is essential for evaluating the quality of scholarly works, suggesting corrections, and learning from other authors' mistakes. The principles of peer review are largely based on professionalism, eloquence, and collegiate attitude. As such, reviewing journal submissions is a privilege and responsibility for 'elite ...
Manuscript Review and Publication . Criteria for Publication; ... simpler path to publishing in a high-quality journal. PLOS ONE promises fair, rigorous peer review, broad scope, and wide readership - a perfect fit for your ... An inclusive journal community working together to advance science by making all rigorous research accessible ...
The broader literature on peer review supports the focus of JAPNA editorials (Lu et al., 2022; Severin & Chataway, 2020).Peer review remains a vibrant part of scholarly publishing in all disciplines, marked by an increasing need for peer reviewers given the rise in scientific publication submissions (Lu et al., 2022).An ongoing theme in peer review discussions with pertinence to JAPNA involves ...
Peer review has been a part of scientific publications since 1665, when the Philosophical Transactions of the Royal Society became the first publication to formalize a system of expert review. 1,2 It became an institutionalized part of science in the latter half of the 20 th century and is now the standard in scientific research publications. 3 In 2012, there were more than 28 000 scholarly ...
Our Journal Finder can suggest Wiley journals that are relevant for your research. Get curated recommendations and explore over 1,600+ journals no matter where you are on your research path. Search Now. View the latest research from Wiley's collection of 1,600+ academic journals including Wiley VCH, Ernst and Sohn and Hindawi Journals.
The call to open the black box of peer review is decades long, and many concerns raised decades ago still resonate: There is too little sound research on journal peer review; this creates a paradox whereby science journals do not apply the rigorous standards they employ in the evaluation of manuscripts to their own peer review practices; as such, a sound research program on journal peer review ...
The peer review system involves the interaction of several players (authors, journal editors, publishers, and the scientific community) and is influenced by professional, social, cultural, and economical factors. Therefore, sociological investigations of the peer review system that integrate behavioral sciences, psychology, and economics could ...
The major advantage of a peer review process is that peer-reviewed articles provide a trusted form of scientific communication. Since scientific knowledge is cumulative and builds on itself, this trust is particularly important. Despite the positive impacts of peer review, critics argue that the peer review process stifles innovation in ...
Peer review information. Nature Communications thanks Florian Bauer, Andrew John Macintosh and the other, anonymous, reviewer(s) for their contribution to the peer review of this work. A peer ...
Peer-review, also known as also known as "refereeing", is a hallmark of the vast majority of scientific journals and represents the cornerstone for assessing the quality of potential scientific publications, since it is aimed to identify drawbacks or inaccuracies that may flaw the outcome or the presentation of scientific research ( 1 ).
The Observer Peer review and scientific publishing. This article is more than 2 months old ... Last year the annual number of papers retracted by research journals topped 10,000 for the first time.
It includes various ecological crises happening in the world today, including climate change. The scientific evidence for anthropogenic climate change is overwhelming, and 97% of peer-reviewed papers accept that global climate change results from human activities [2,3]. Before this situation, feeling eco-anxiety should be a common human reaction.
Peer Review reports. ... On the declaration of educational scientific research topics. Journal of Henan Radio & TV University. 2013;26(01):98-101. Ju Y, Zhao X. Top three hospitals clinical nurse scientific research ability present situation and influence factors analysis. J Health Vocational Educ. 2022;40(17):125-8.
Aim/Purpose: This study aimed to evaluate the extant research on data science education (DSE) to identify the existing gaps, opportunities, and challenges, and make recommendations for current and future DSE. Background: There has been an increase in the number of data science programs especially because of the increased appreciation of data as a multidisciplinary strategic resource.
Read the latest articles of Vision Research at ScienceDirect.com, Elsevier's leading platform of peer-reviewed scholarly literature