Writing an Abstract for Your Research Paper
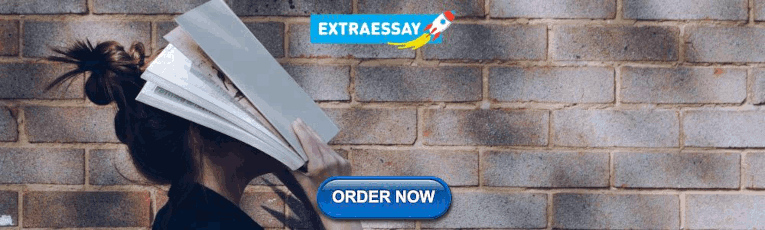
Definition and Purpose of Abstracts
An abstract is a short summary of your (published or unpublished) research paper, usually about a paragraph (c. 6-7 sentences, 150-250 words) long. A well-written abstract serves multiple purposes:
- an abstract lets readers get the gist or essence of your paper or article quickly, in order to decide whether to read the full paper;
- an abstract prepares readers to follow the detailed information, analyses, and arguments in your full paper;
- and, later, an abstract helps readers remember key points from your paper.
It’s also worth remembering that search engines and bibliographic databases use abstracts, as well as the title, to identify key terms for indexing your published paper. So what you include in your abstract and in your title are crucial for helping other researchers find your paper or article.
If you are writing an abstract for a course paper, your professor may give you specific guidelines for what to include and how to organize your abstract. Similarly, academic journals often have specific requirements for abstracts. So in addition to following the advice on this page, you should be sure to look for and follow any guidelines from the course or journal you’re writing for.
The Contents of an Abstract
Abstracts contain most of the following kinds of information in brief form. The body of your paper will, of course, develop and explain these ideas much more fully. As you will see in the samples below, the proportion of your abstract that you devote to each kind of information—and the sequence of that information—will vary, depending on the nature and genre of the paper that you are summarizing in your abstract. And in some cases, some of this information is implied, rather than stated explicitly. The Publication Manual of the American Psychological Association , which is widely used in the social sciences, gives specific guidelines for what to include in the abstract for different kinds of papers—for empirical studies, literature reviews or meta-analyses, theoretical papers, methodological papers, and case studies.
Here are the typical kinds of information found in most abstracts:
- the context or background information for your research; the general topic under study; the specific topic of your research
- the central questions or statement of the problem your research addresses
- what’s already known about this question, what previous research has done or shown
- the main reason(s) , the exigency, the rationale , the goals for your research—Why is it important to address these questions? Are you, for example, examining a new topic? Why is that topic worth examining? Are you filling a gap in previous research? Applying new methods to take a fresh look at existing ideas or data? Resolving a dispute within the literature in your field? . . .
- your research and/or analytical methods
- your main findings , results , or arguments
- the significance or implications of your findings or arguments.
Your abstract should be intelligible on its own, without a reader’s having to read your entire paper. And in an abstract, you usually do not cite references—most of your abstract will describe what you have studied in your research and what you have found and what you argue in your paper. In the body of your paper, you will cite the specific literature that informs your research.
When to Write Your Abstract
Although you might be tempted to write your abstract first because it will appear as the very first part of your paper, it’s a good idea to wait to write your abstract until after you’ve drafted your full paper, so that you know what you’re summarizing.
What follows are some sample abstracts in published papers or articles, all written by faculty at UW-Madison who come from a variety of disciplines. We have annotated these samples to help you see the work that these authors are doing within their abstracts.
Choosing Verb Tenses within Your Abstract
The social science sample (Sample 1) below uses the present tense to describe general facts and interpretations that have been and are currently true, including the prevailing explanation for the social phenomenon under study. That abstract also uses the present tense to describe the methods, the findings, the arguments, and the implications of the findings from their new research study. The authors use the past tense to describe previous research.
The humanities sample (Sample 2) below uses the past tense to describe completed events in the past (the texts created in the pulp fiction industry in the 1970s and 80s) and uses the present tense to describe what is happening in those texts, to explain the significance or meaning of those texts, and to describe the arguments presented in the article.
The science samples (Samples 3 and 4) below use the past tense to describe what previous research studies have done and the research the authors have conducted, the methods they have followed, and what they have found. In their rationale or justification for their research (what remains to be done), they use the present tense. They also use the present tense to introduce their study (in Sample 3, “Here we report . . .”) and to explain the significance of their study (In Sample 3, This reprogramming . . . “provides a scalable cell source for. . .”).
Sample Abstract 1
From the social sciences.
Reporting new findings about the reasons for increasing economic homogamy among spouses
Gonalons-Pons, Pilar, and Christine R. Schwartz. “Trends in Economic Homogamy: Changes in Assortative Mating or the Division of Labor in Marriage?” Demography , vol. 54, no. 3, 2017, pp. 985-1005.
![here is an abstract of a research article published in online journal “The growing economic resemblance of spouses has contributed to rising inequality by increasing the number of couples in which there are two high- or two low-earning partners. [Annotation for the previous sentence: The first sentence introduces the topic under study (the “economic resemblance of spouses”). This sentence also implies the question underlying this research study: what are the various causes—and the interrelationships among them—for this trend?] The dominant explanation for this trend is increased assortative mating. Previous research has primarily relied on cross-sectional data and thus has been unable to disentangle changes in assortative mating from changes in the division of spouses’ paid labor—a potentially key mechanism given the dramatic rise in wives’ labor supply. [Annotation for the previous two sentences: These next two sentences explain what previous research has demonstrated. By pointing out the limitations in the methods that were used in previous studies, they also provide a rationale for new research.] We use data from the Panel Study of Income Dynamics (PSID) to decompose the increase in the correlation between spouses’ earnings and its contribution to inequality between 1970 and 2013 into parts due to (a) changes in assortative mating, and (b) changes in the division of paid labor. [Annotation for the previous sentence: The data, research and analytical methods used in this new study.] Contrary to what has often been assumed, the rise of economic homogamy and its contribution to inequality is largely attributable to changes in the division of paid labor rather than changes in sorting on earnings or earnings potential. Our findings indicate that the rise of economic homogamy cannot be explained by hypotheses centered on meeting and matching opportunities, and they show where in this process inequality is generated and where it is not.” (p. 985) [Annotation for the previous two sentences: The major findings from and implications and significance of this study.]](https://writing.wisc.edu/wp-content/uploads/sites/535/2019/08/Abstract-1.png)
Sample Abstract 2
From the humanities.
Analyzing underground pulp fiction publications in Tanzania, this article makes an argument about the cultural significance of those publications
Emily Callaci. “Street Textuality: Socialism, Masculinity, and Urban Belonging in Tanzania’s Pulp Fiction Publishing Industry, 1975-1985.” Comparative Studies in Society and History , vol. 59, no. 1, 2017, pp. 183-210.
![here is an abstract of a research article published in online journal “From the mid-1970s through the mid-1980s, a network of young urban migrant men created an underground pulp fiction publishing industry in the city of Dar es Salaam. [Annotation for the previous sentence: The first sentence introduces the context for this research and announces the topic under study.] As texts that were produced in the underground economy of a city whose trajectory was increasingly charted outside of formalized planning and investment, these novellas reveal more than their narrative content alone. These texts were active components in the urban social worlds of the young men who produced them. They reveal a mode of urbanism otherwise obscured by narratives of decolonization, in which urban belonging was constituted less by national citizenship than by the construction of social networks, economic connections, and the crafting of reputations. This article argues that pulp fiction novellas of socialist era Dar es Salaam are artifacts of emergent forms of male sociability and mobility. In printing fictional stories about urban life on pilfered paper and ink, and distributing their texts through informal channels, these writers not only described urban communities, reputations, and networks, but also actually created them.” (p. 210) [Annotation for the previous sentences: The remaining sentences in this abstract interweave other essential information for an abstract for this article. The implied research questions: What do these texts mean? What is their historical and cultural significance, produced at this time, in this location, by these authors? The argument and the significance of this analysis in microcosm: these texts “reveal a mode or urbanism otherwise obscured . . .”; and “This article argues that pulp fiction novellas. . . .” This section also implies what previous historical research has obscured. And through the details in its argumentative claims, this section of the abstract implies the kinds of methods the author has used to interpret the novellas and the concepts under study (e.g., male sociability and mobility, urban communities, reputations, network. . . ).]](https://writing.wisc.edu/wp-content/uploads/sites/535/2019/08/Abstract-2.png)
Sample Abstract/Summary 3
From the sciences.
Reporting a new method for reprogramming adult mouse fibroblasts into induced cardiac progenitor cells
Lalit, Pratik A., Max R. Salick, Daryl O. Nelson, Jayne M. Squirrell, Christina M. Shafer, Neel G. Patel, Imaan Saeed, Eric G. Schmuck, Yogananda S. Markandeya, Rachel Wong, Martin R. Lea, Kevin W. Eliceiri, Timothy A. Hacker, Wendy C. Crone, Michael Kyba, Daniel J. Garry, Ron Stewart, James A. Thomson, Karen M. Downs, Gary E. Lyons, and Timothy J. Kamp. “Lineage Reprogramming of Fibroblasts into Proliferative Induced Cardiac Progenitor Cells by Defined Factors.” Cell Stem Cell , vol. 18, 2016, pp. 354-367.
![here is an abstract of a research article published in online journal “Several studies have reported reprogramming of fibroblasts into induced cardiomyocytes; however, reprogramming into proliferative induced cardiac progenitor cells (iCPCs) remains to be accomplished. [Annotation for the previous sentence: The first sentence announces the topic under study, summarizes what’s already known or been accomplished in previous research, and signals the rationale and goals are for the new research and the problem that the new research solves: How can researchers reprogram fibroblasts into iCPCs?] Here we report that a combination of 11 or 5 cardiac factors along with canonical Wnt and JAK/STAT signaling reprogrammed adult mouse cardiac, lung, and tail tip fibroblasts into iCPCs. The iCPCs were cardiac mesoderm-restricted progenitors that could be expanded extensively while maintaining multipo-tency to differentiate into cardiomyocytes, smooth muscle cells, and endothelial cells in vitro. Moreover, iCPCs injected into the cardiac crescent of mouse embryos differentiated into cardiomyocytes. iCPCs transplanted into the post-myocardial infarction mouse heart improved survival and differentiated into cardiomyocytes, smooth muscle cells, and endothelial cells. [Annotation for the previous four sentences: The methods the researchers developed to achieve their goal and a description of the results.] Lineage reprogramming of adult somatic cells into iCPCs provides a scalable cell source for drug discovery, disease modeling, and cardiac regenerative therapy.” (p. 354) [Annotation for the previous sentence: The significance or implications—for drug discovery, disease modeling, and therapy—of this reprogramming of adult somatic cells into iCPCs.]](https://writing.wisc.edu/wp-content/uploads/sites/535/2019/08/Abstract-3.png)
Sample Abstract 4, a Structured Abstract
Reporting results about the effectiveness of antibiotic therapy in managing acute bacterial sinusitis, from a rigorously controlled study
Note: This journal requires authors to organize their abstract into four specific sections, with strict word limits. Because the headings for this structured abstract are self-explanatory, we have chosen not to add annotations to this sample abstract.
Wald, Ellen R., David Nash, and Jens Eickhoff. “Effectiveness of Amoxicillin/Clavulanate Potassium in the Treatment of Acute Bacterial Sinusitis in Children.” Pediatrics , vol. 124, no. 1, 2009, pp. 9-15.
“OBJECTIVE: The role of antibiotic therapy in managing acute bacterial sinusitis (ABS) in children is controversial. The purpose of this study was to determine the effectiveness of high-dose amoxicillin/potassium clavulanate in the treatment of children diagnosed with ABS.
METHODS : This was a randomized, double-blind, placebo-controlled study. Children 1 to 10 years of age with a clinical presentation compatible with ABS were eligible for participation. Patients were stratified according to age (<6 or ≥6 years) and clinical severity and randomly assigned to receive either amoxicillin (90 mg/kg) with potassium clavulanate (6.4 mg/kg) or placebo. A symptom survey was performed on days 0, 1, 2, 3, 5, 7, 10, 20, and 30. Patients were examined on day 14. Children’s conditions were rated as cured, improved, or failed according to scoring rules.
RESULTS: Two thousand one hundred thirty-five children with respiratory complaints were screened for enrollment; 139 (6.5%) had ABS. Fifty-eight patients were enrolled, and 56 were randomly assigned. The mean age was 6630 months. Fifty (89%) patients presented with persistent symptoms, and 6 (11%) presented with nonpersistent symptoms. In 24 (43%) children, the illness was classified as mild, whereas in the remaining 32 (57%) children it was severe. Of the 28 children who received the antibiotic, 14 (50%) were cured, 4 (14%) were improved, 4(14%) experienced treatment failure, and 6 (21%) withdrew. Of the 28children who received placebo, 4 (14%) were cured, 5 (18%) improved, and 19 (68%) experienced treatment failure. Children receiving the antibiotic were more likely to be cured (50% vs 14%) and less likely to have treatment failure (14% vs 68%) than children receiving the placebo.
CONCLUSIONS : ABS is a common complication of viral upper respiratory infections. Amoxicillin/potassium clavulanate results in significantly more cures and fewer failures than placebo, according to parental report of time to resolution.” (9)
Some Excellent Advice about Writing Abstracts for Basic Science Research Papers, by Professor Adriano Aguzzi from the Institute of Neuropathology at the University of Zurich:

Academic and Professional Writing
This is an accordion element with a series of buttons that open and close related content panels.
Analysis Papers
Reading Poetry
A Short Guide to Close Reading for Literary Analysis
Using Literary Quotations
Play Reviews
Writing a Rhetorical Précis to Analyze Nonfiction Texts
Incorporating Interview Data
Grant Proposals
Planning and Writing a Grant Proposal: The Basics
Additional Resources for Grants and Proposal Writing
Job Materials and Application Essays
Writing Personal Statements for Ph.D. Programs
- Before you begin: useful tips for writing your essay
- Guided brainstorming exercises
- Get more help with your essay
- Frequently Asked Questions
Resume Writing Tips
CV Writing Tips
Cover Letters
Business Letters
Proposals and Dissertations
Resources for Proposal Writers
Resources for Dissertators
Research Papers
Planning and Writing Research Papers
Quoting and Paraphrasing
Writing Annotated Bibliographies
Creating Poster Presentations
Thank-You Notes
Advice for Students Writing Thank-You Notes to Donors
Reading for a Review
Critical Reviews
Writing a Review of Literature
Scientific Reports
Scientific Report Format
Sample Lab Assignment
Writing for the Web
Writing an Effective Blog Post
Writing for Social Media: A Guide for Academics
Have a language expert improve your writing
Run a free plagiarism check in 10 minutes, generate accurate citations for free.
- Knowledge Base
- Dissertation
- How to Write an Abstract | Steps & Examples
How to Write an Abstract | Steps & Examples
Published on February 28, 2019 by Shona McCombes . Revised on July 18, 2023 by Eoghan Ryan.
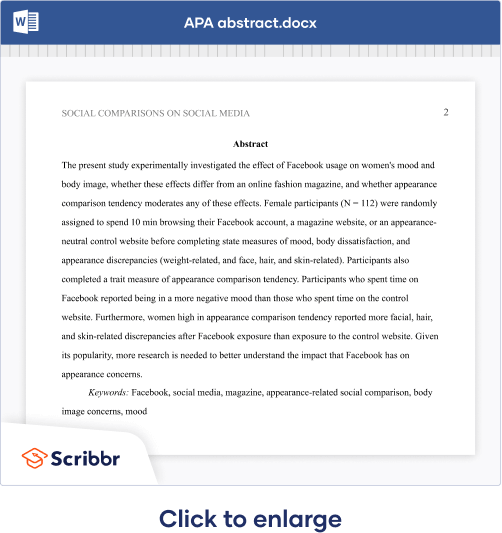
An abstract is a short summary of a longer work (such as a thesis , dissertation or research paper ). The abstract concisely reports the aims and outcomes of your research, so that readers know exactly what your paper is about.
Although the structure may vary slightly depending on your discipline, your abstract should describe the purpose of your work, the methods you’ve used, and the conclusions you’ve drawn.
One common way to structure your abstract is to use the IMRaD structure. This stands for:
- Introduction
Abstracts are usually around 100–300 words, but there’s often a strict word limit, so make sure to check the relevant requirements.
In a dissertation or thesis , include the abstract on a separate page, after the title page and acknowledgements but before the table of contents .
Instantly correct all language mistakes in your text
Upload your document to correct all your mistakes in minutes
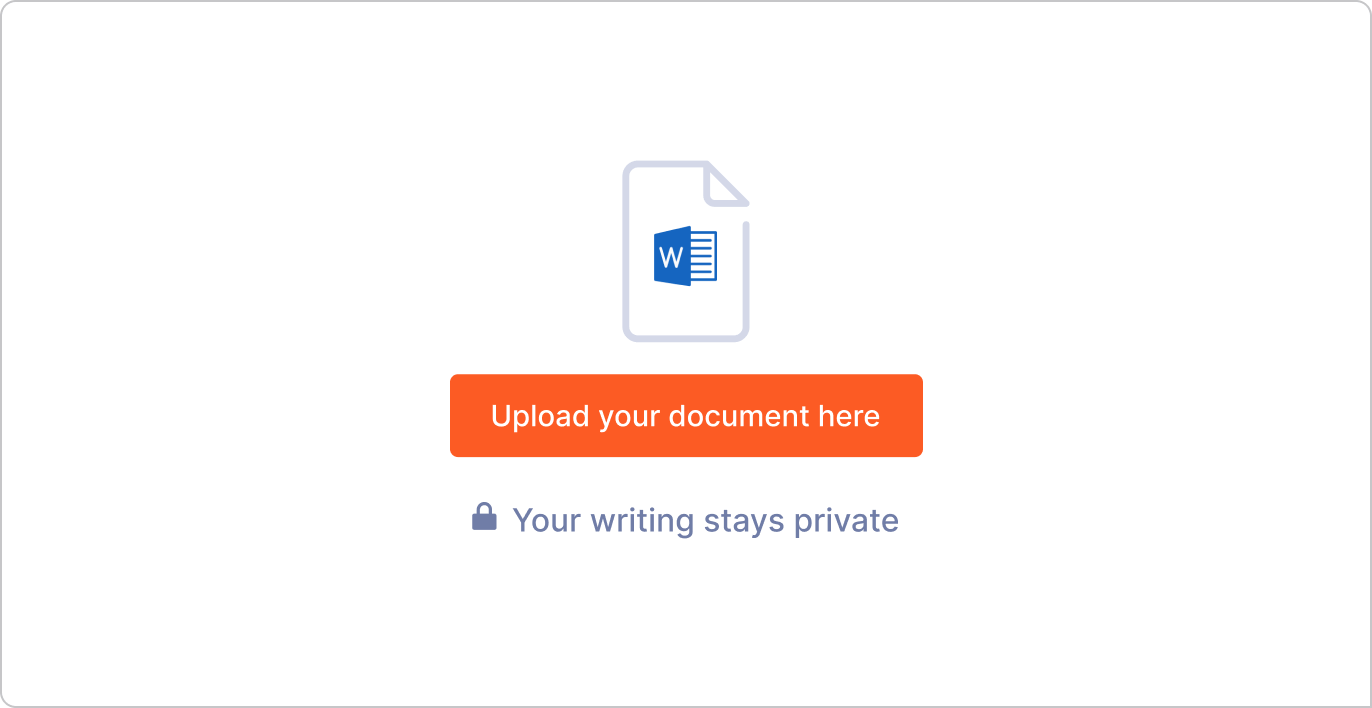
Table of contents
Abstract example, when to write an abstract, step 1: introduction, step 2: methods, step 3: results, step 4: discussion, tips for writing an abstract, other interesting articles, frequently asked questions about abstracts.
Hover over the different parts of the abstract to see how it is constructed.
This paper examines the role of silent movies as a mode of shared experience in the US during the early twentieth century. At this time, high immigration rates resulted in a significant percentage of non-English-speaking citizens. These immigrants faced numerous economic and social obstacles, including exclusion from public entertainment and modes of discourse (newspapers, theater, radio).
Incorporating evidence from reviews, personal correspondence, and diaries, this study demonstrates that silent films were an affordable and inclusive source of entertainment. It argues for the accessible economic and representational nature of early cinema. These concerns are particularly evident in the low price of admission and in the democratic nature of the actors’ exaggerated gestures, which allowed the plots and action to be easily grasped by a diverse audience despite language barriers.
Keywords: silent movies, immigration, public discourse, entertainment, early cinema, language barriers.
Here's why students love Scribbr's proofreading services
Discover proofreading & editing
You will almost always have to include an abstract when:
- Completing a thesis or dissertation
- Submitting a research paper to an academic journal
- Writing a book or research proposal
- Applying for research grants
It’s easiest to write your abstract last, right before the proofreading stage, because it’s a summary of the work you’ve already done. Your abstract should:
- Be a self-contained text, not an excerpt from your paper
- Be fully understandable on its own
- Reflect the structure of your larger work
Start by clearly defining the purpose of your research. What practical or theoretical problem does the research respond to, or what research question did you aim to answer?
You can include some brief context on the social or academic relevance of your dissertation topic , but don’t go into detailed background information. If your abstract uses specialized terms that would be unfamiliar to the average academic reader or that have various different meanings, give a concise definition.
After identifying the problem, state the objective of your research. Use verbs like “investigate,” “test,” “analyze,” or “evaluate” to describe exactly what you set out to do.
This part of the abstract can be written in the present or past simple tense but should never refer to the future, as the research is already complete.
- This study will investigate the relationship between coffee consumption and productivity.
- This study investigates the relationship between coffee consumption and productivity.
Next, indicate the research methods that you used to answer your question. This part should be a straightforward description of what you did in one or two sentences. It is usually written in the past simple tense, as it refers to completed actions.
- Structured interviews will be conducted with 25 participants.
- Structured interviews were conducted with 25 participants.
Don’t evaluate validity or obstacles here — the goal is not to give an account of the methodology’s strengths and weaknesses, but to give the reader a quick insight into the overall approach and procedures you used.
The only proofreading tool specialized in correcting academic writing - try for free!
The academic proofreading tool has been trained on 1000s of academic texts and by native English editors. Making it the most accurate and reliable proofreading tool for students.
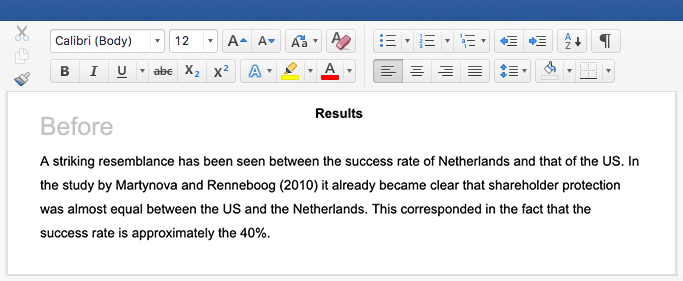
Try for free
Next, summarize the main research results . This part of the abstract can be in the present or past simple tense.
- Our analysis has shown a strong correlation between coffee consumption and productivity.
- Our analysis shows a strong correlation between coffee consumption and productivity.
- Our analysis showed a strong correlation between coffee consumption and productivity.
Depending on how long and complex your research is, you may not be able to include all results here. Try to highlight only the most important findings that will allow the reader to understand your conclusions.
Finally, you should discuss the main conclusions of your research : what is your answer to the problem or question? The reader should finish with a clear understanding of the central point that your research has proved or argued. Conclusions are usually written in the present simple tense.
- We concluded that coffee consumption increases productivity.
- We conclude that coffee consumption increases productivity.
If there are important limitations to your research (for example, related to your sample size or methods), you should mention them briefly in the abstract. This allows the reader to accurately assess the credibility and generalizability of your research.
If your aim was to solve a practical problem, your discussion might include recommendations for implementation. If relevant, you can briefly make suggestions for further research.
If your paper will be published, you might have to add a list of keywords at the end of the abstract. These keywords should reference the most important elements of the research to help potential readers find your paper during their own literature searches.
Be aware that some publication manuals, such as APA Style , have specific formatting requirements for these keywords.
It can be a real challenge to condense your whole work into just a couple of hundred words, but the abstract will be the first (and sometimes only) part that people read, so it’s important to get it right. These strategies can help you get started.
Read other abstracts
The best way to learn the conventions of writing an abstract in your discipline is to read other people’s. You probably already read lots of journal article abstracts while conducting your literature review —try using them as a framework for structure and style.
You can also find lots of dissertation abstract examples in thesis and dissertation databases .
Reverse outline
Not all abstracts will contain precisely the same elements. For longer works, you can write your abstract through a process of reverse outlining.
For each chapter or section, list keywords and draft one to two sentences that summarize the central point or argument. This will give you a framework of your abstract’s structure. Next, revise the sentences to make connections and show how the argument develops.
Write clearly and concisely
A good abstract is short but impactful, so make sure every word counts. Each sentence should clearly communicate one main point.
To keep your abstract or summary short and clear:
- Avoid passive sentences: Passive constructions are often unnecessarily long. You can easily make them shorter and clearer by using the active voice.
- Avoid long sentences: Substitute longer expressions for concise expressions or single words (e.g., “In order to” for “To”).
- Avoid obscure jargon: The abstract should be understandable to readers who are not familiar with your topic.
- Avoid repetition and filler words: Replace nouns with pronouns when possible and eliminate unnecessary words.
- Avoid detailed descriptions: An abstract is not expected to provide detailed definitions, background information, or discussions of other scholars’ work. Instead, include this information in the body of your thesis or paper.
If you’re struggling to edit down to the required length, you can get help from expert editors with Scribbr’s professional proofreading services or use the paraphrasing tool .
Check your formatting
If you are writing a thesis or dissertation or submitting to a journal, there are often specific formatting requirements for the abstract—make sure to check the guidelines and format your work correctly. For APA research papers you can follow the APA abstract format .
Checklist: Abstract
The word count is within the required length, or a maximum of one page.
The abstract appears after the title page and acknowledgements and before the table of contents .
I have clearly stated my research problem and objectives.
I have briefly described my methodology .
I have summarized the most important results .
I have stated my main conclusions .
I have mentioned any important limitations and recommendations.
The abstract can be understood by someone without prior knowledge of the topic.
You've written a great abstract! Use the other checklists to continue improving your thesis or dissertation.
If you want to know more about AI for academic writing, AI tools, or research bias, make sure to check out some of our other articles with explanations and examples or go directly to our tools!
Research bias
- Anchoring bias
- Halo effect
- The Baader–Meinhof phenomenon
- The placebo effect
- Nonresponse bias
- Deep learning
- Generative AI
- Machine learning
- Reinforcement learning
- Supervised vs. unsupervised learning
(AI) Tools
- Grammar Checker
- Paraphrasing Tool
- Text Summarizer
- AI Detector
- Plagiarism Checker
- Citation Generator
An abstract is a concise summary of an academic text (such as a journal article or dissertation ). It serves two main purposes:
- To help potential readers determine the relevance of your paper for their own research.
- To communicate your key findings to those who don’t have time to read the whole paper.
Abstracts are often indexed along with keywords on academic databases, so they make your work more easily findable. Since the abstract is the first thing any reader sees, it’s important that it clearly and accurately summarizes the contents of your paper.
An abstract for a thesis or dissertation is usually around 200–300 words. There’s often a strict word limit, so make sure to check your university’s requirements.
The abstract is the very last thing you write. You should only write it after your research is complete, so that you can accurately summarize the entirety of your thesis , dissertation or research paper .
Avoid citing sources in your abstract . There are two reasons for this:
- The abstract should focus on your original research, not on the work of others.
- The abstract should be self-contained and fully understandable without reference to other sources.
There are some circumstances where you might need to mention other sources in an abstract: for example, if your research responds directly to another study or focuses on the work of a single theorist. In general, though, don’t include citations unless absolutely necessary.
The abstract appears on its own page in the thesis or dissertation , after the title page and acknowledgements but before the table of contents .
Cite this Scribbr article
If you want to cite this source, you can copy and paste the citation or click the “Cite this Scribbr article” button to automatically add the citation to our free Citation Generator.
McCombes, S. (2023, July 18). How to Write an Abstract | Steps & Examples. Scribbr. Retrieved April 10, 2024, from https://www.scribbr.com/dissertation/abstract/
Is this article helpful?
Shona McCombes
Other students also liked, how to write a thesis or dissertation introduction, shorten your abstract or summary, how to write a literature review | guide, examples, & templates, unlimited academic ai-proofreading.
✔ Document error-free in 5minutes ✔ Unlimited document corrections ✔ Specialized in correcting academic texts
When you choose to publish with PLOS, your research makes an impact. Make your work accessible to all, without restrictions, and accelerate scientific discovery with options like preprints and published peer review that make your work more Open.
- PLOS Biology
- PLOS Climate
- PLOS Complex Systems
- PLOS Computational Biology
- PLOS Digital Health
- PLOS Genetics
- PLOS Global Public Health
- PLOS Medicine
- PLOS Mental Health
- PLOS Neglected Tropical Diseases
- PLOS Pathogens
- PLOS Sustainability and Transformation
- PLOS Collections
- How to Write an Abstract

Expedite peer review, increase search-ability, and set the tone for your study
The abstract is your chance to let your readers know what they can expect from your article. Learn how to write a clear, and concise abstract that will keep your audience reading.
How your abstract impacts editorial evaluation and future readership
After the title , the abstract is the second-most-read part of your article. A good abstract can help to expedite peer review and, if your article is accepted for publication, it’s an important tool for readers to find and evaluate your work. Editors use your abstract when they first assess your article. Prospective reviewers see it when they decide whether to accept an invitation to review. Once published, the abstract gets indexed in PubMed and Google Scholar , as well as library systems and other popular databases. Like the title, your abstract influences keyword search results. Readers will use it to decide whether to read the rest of your article. Other researchers will use it to evaluate your work for inclusion in systematic reviews and meta-analysis. It should be a concise standalone piece that accurately represents your research.
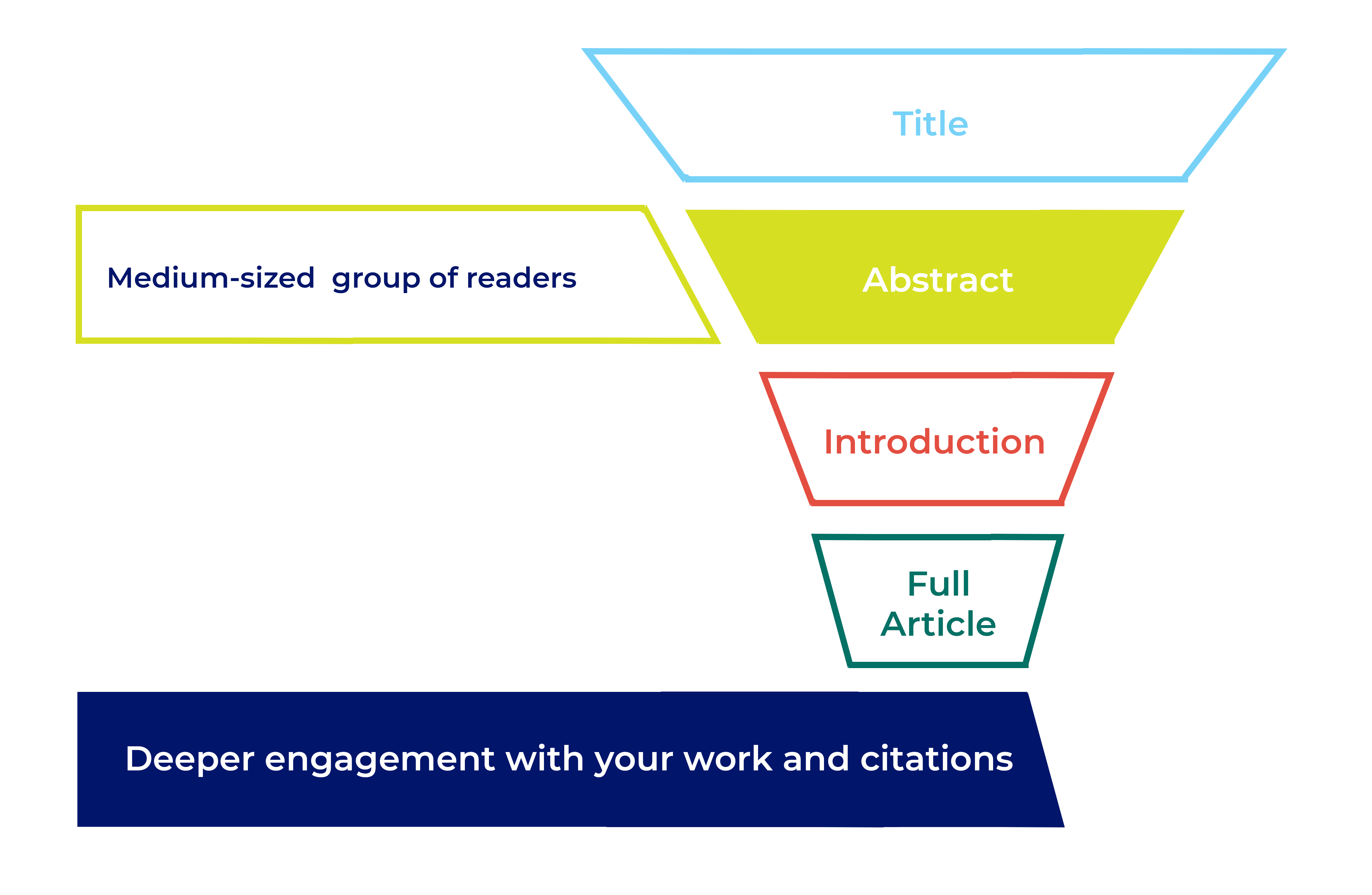
What to include in an abstract
The main challenge you’ll face when writing your abstract is keeping it concise AND fitting in all the information you need. Depending on your subject area the journal may require a structured abstract following specific headings. A structured abstract helps your readers understand your study more easily. If your journal doesn’t require a structured abstract it’s still a good idea to follow a similar format, just present the abstract as one paragraph without headings.
Background or Introduction – What is currently known? Start with a brief, 2 or 3 sentence, introduction to the research area.
Objectives or Aims – What is the study and why did you do it? Clearly state the research question you’re trying to answer.
Methods – What did you do? Explain what you did and how you did it. Include important information about your methods, but avoid the low-level specifics. Some disciplines have specific requirements for abstract methods.
- CONSORT for randomized trials.
- STROBE for observational studies
- PRISMA for systematic reviews and meta-analyses
Results – What did you find? Briefly give the key findings of your study. Include key numeric data (including confidence intervals or p values), where possible.
Conclusions – What did you conclude? Tell the reader why your findings matter, and what this could mean for the ‘bigger picture’ of this area of research.
Writing tips
The main challenge you may find when writing your abstract is keeping it concise AND convering all the information you need to.
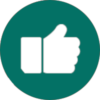
- Keep it concise and to the point. Most journals have a maximum word count, so check guidelines before you write the abstract to save time editing it later.
- Write for your audience. Are they specialists in your specific field? Are they cross-disciplinary? Are they non-specialists? If you’re writing for a general audience, or your research could be of interest to the public keep your language as straightforward as possible. If you’re writing in English, do remember that not all of your readers will necessarily be native English speakers.
- Focus on key results, conclusions and take home messages.
- Write your paper first, then create the abstract as a summary.
- Check the journal requirements before you write your abstract, eg. required subheadings.
- Include keywords or phrases to help readers search for your work in indexing databases like PubMed or Google Scholar.
- Double and triple check your abstract for spelling and grammar errors. These kind of errors can give potential reviewers the impression that your research isn’t sound, and can make it easier to find reviewers who accept the invitation to review your manuscript. Your abstract should be a taste of what is to come in the rest of your article.

Don’t
- Sensationalize your research.
- Speculate about where this research might lead in the future.
- Use abbreviations or acronyms (unless absolutely necessary or unless they’re widely known, eg. DNA).
- Repeat yourself unnecessarily, eg. “Methods: We used X technique. Results: Using X technique, we found…”
- Contradict anything in the rest of your manuscript.
- Include content that isn’t also covered in the main manuscript.
- Include citations or references.
Tip: How to edit your work
Editing is challenging, especially if you are acting as both a writer and an editor. Read our guidelines for advice on how to refine your work, including useful tips for setting your intentions, re-review, and consultation with colleagues.
- How to Write a Great Title
- How to Write Your Methods
- How to Report Statistics
- How to Write Discussions and Conclusions
- How to Edit Your Work
The contents of the Peer Review Center are also available as a live, interactive training session, complete with slides, talking points, and activities. …
The contents of the Writing Center are also available as a live, interactive training session, complete with slides, talking points, and activities. …
There’s a lot to consider when deciding where to submit your work. Learn how to choose a journal that will help your study reach its audience, while reflecting your values as a researcher…
- - Google Chrome
Intended for healthcare professionals
- Access provided by Google Indexer
- My email alerts
- BMA member login
- Username * Password * Forgot your log in details? Need to activate BMA Member Log In Log in via OpenAthens Log in via your institution

Search form
- Advanced search
- Search responses
- Search blogs
- How to write an...
How to write an abstract that will be accepted
- Related content
- Peer review
- Mary Higgins , fellow in maternal fetal medicine 1 ,
- Maeve Eogan , consultant obstetrician and gynaecologist 2 ,
- Keelin O’Donoghue , consultant obstetrician and gynaecologist, and senior lecturer 3 ,
- Noirin Russell , consultant obstetrician and gynaecologist 3
- 1 Mount Sinai Hospital, Toronto, Ontario, Canada
- 2 Rotunda Hospital Dublin, Ireland
- 3 Cork University Maternity Hospital, Ireland
- mairenihuigin{at}gmail.com
Researchers do not always appreciate the importance of producing a good abstract or understand the best way of writing one. Mary Higgins and colleagues share some of the lessons they have learnt as both researchers and reviewers of abstracts
Effective abstracts reflect the time, work, and importance of the scientific research performed in the course of a study. A last minute approach and poor writing may not reflect the good quality of a study.
Between the four of us we have written over 150 published papers, as well as having reviewed numerous abstracts for national and international meetings. Nevertheless, we have all had abstracts rejected, and this experience has emphasised a number of teaching points that could help maximise the impact of abstracts and success on the world, or other, stage.
An abstract is the first glimpse an audience has of a study, and it is the ticket to having research accepted for presentation to a wider audience. For a study to receive the respect it deserves, the abstract should be as well written as possible. In practice, this means taking time to write the abstract, keeping it simple, reading the submission guidelines, checking the text, and showing the abstract to colleagues.
It is important to take the necessary time to write the abstract. Several months or years have been spent on this groundbreaking research, so take the time to show this. Five minutes before the call for abstracts closes is not the time to start putting it together.
Keep it simple, and think about the message that needs to be communicated. Some abstracts churn out lots of unrelated results and then have a conclusion that does not relate to the results, and this is just confusing. Plan what points need to be made, and then think about them a little more.
Read the submission guidelines and keep to the instructions provided in the call for abstracts. Don’t submit an unstructured abstract if the guidance has asked for a structured one. Comply with the word or letter count, and do not go over this.
An abstract comprises five parts of equal importance: the title, introduction and aims, methods, results, and conclusion. Allow enough time to write each part well.
The title should go straight to the point of the study. Make the study sound interesting so that it catches people’s attention. The introduction should include a brief background to the research and describe its aims. For every aim presented there needs to be a corresponding result in the results section. There is no need to go into detail in terms of the background to the study, as those who are reviewing the abstract will have some knowledge of the subject. The methods section can be kept simple—it is acceptable to write “retrospective case-control study” or “randomised controlled trial.”
The results section should be concrete and related to the aims. It is distracting and irritating to read results that have no apparent relation to the professed aims of the study. If something is important, highlight it or put it in italics to make it stand out. Include the number of participants, and ensure recognition is given if 10 000 charts have been reviewed. Equally, a percentage without a baseline number is not meaningful.
In the conclusion, state succinctly what can be drawn from the results, but don’t oversell this. Words like “possibly” and “may” can be useful in this part of the abstract but show that some thought has been put into what the results may mean. This is what divides the good from the not so good. Many people are capable of doing research, but the logical formation of a hypothesis and the argument of its proof are what make a real researcher.
Once you have written the abstract, check the spelling and grammar. Poor spelling or grammar can give the impression that the research is also poor. Show the abstract to the supervisor or principal investigator of the study, as this person’s name will go on the abstract as well. Then show the abstract to someone who knows nothing about the particular area of research but who knows something about the subject. Someone detached from the study might point out the one thing that needs to be said but that has been forgotten.
Then let it go; abstracts are not life and death scenarios. Sometimes an abstract will not be accepted no matter how wonderful it is. Perhaps there is a theme to the meeting, into which the research does not fit. Reviewers may also be looking for particular things. For one conference, we limited the number of case reports so that only about 10% were accepted. It may be that your research is in a popular or topical area and not all abstracts in that area can be chosen. On occasions, politics play a part, and individual researchers have little control over that.
Finally, remember that sometimes even the best reviewer may not appreciate the subtleties of your research and another audience may be more appreciative.
Competing interests: We have read and understood the BMJ Group policy on declaration of interests and have no relevant interests to declare.

An official website of the United States government
The .gov means it’s official. Federal government websites often end in .gov or .mil. Before sharing sensitive information, make sure you’re on a federal government site.
The site is secure. The https:// ensures that you are connecting to the official website and that any information you provide is encrypted and transmitted securely.
- Publications
- Account settings
Preview improvements coming to the PMC website in October 2024. Learn More or Try it out now .
- Advanced Search
- Journal List
- Saudi J Anaesth
- v.13(Suppl 1); 2019 Apr
Writing the title and abstract for a research paper: Being concise, precise, and meticulous is the key
Milind s. tullu.
Department of Pediatrics, Seth G.S. Medical College and KEM Hospital, Parel, Mumbai, Maharashtra, India
This article deals with formulating a suitable title and an appropriate abstract for an original research paper. The “title” and the “abstract” are the “initial impressions” of a research article, and hence they need to be drafted correctly, accurately, carefully, and meticulously. Often both of these are drafted after the full manuscript is ready. Most readers read only the title and the abstract of a research paper and very few will go on to read the full paper. The title and the abstract are the most important parts of a research paper and should be pleasant to read. The “title” should be descriptive, direct, accurate, appropriate, interesting, concise, precise, unique, and should not be misleading. The “abstract” needs to be simple, specific, clear, unbiased, honest, concise, precise, stand-alone, complete, scholarly, (preferably) structured, and should not be misrepresentative. The abstract should be consistent with the main text of the paper, especially after a revision is made to the paper and should include the key message prominently. It is very important to include the most important words and terms (the “keywords”) in the title and the abstract for appropriate indexing purpose and for retrieval from the search engines and scientific databases. Such keywords should be listed after the abstract. One must adhere to the instructions laid down by the target journal with regard to the style and number of words permitted for the title and the abstract.
Introduction
This article deals with drafting a suitable “title” and an appropriate “abstract” for an original research paper. Because the “title” and the “abstract” are the “initial impressions” or the “face” of a research article, they need to be drafted correctly, accurately, carefully, meticulously, and consume time and energy.[ 1 , 2 , 3 , 4 , 5 , 6 , 7 , 8 , 9 , 10 ] Often, these are drafted after the complete manuscript draft is ready.[ 2 , 3 , 4 , 5 , 9 , 10 , 11 ] Most readers will read only the title and the abstract of a published research paper, and very few “interested ones” (especially, if the paper is of use to them) will go on to read the full paper.[ 1 , 2 ] One must remember to adhere to the instructions laid down by the “target journal” (the journal for which the author is writing) regarding the style and number of words permitted for the title and the abstract.[ 2 , 4 , 5 , 7 , 8 , 9 , 12 ] Both the title and the abstract are the most important parts of a research paper – for editors (to decide whether to process the paper for further review), for reviewers (to get an initial impression of the paper), and for the readers (as these may be the only parts of the paper available freely and hence, read widely).[ 4 , 8 , 12 ] It may be worth for the novice author to browse through titles and abstracts of several prominent journals (and their target journal as well) to learn more about the wording and styles of the titles and abstracts, as well as the aims and scope of the particular journal.[ 5 , 7 , 9 , 13 ]
The details of the title are discussed under the subheadings of importance, types, drafting, and checklist.
Importance of the title
When a reader browses through the table of contents of a journal issue (hard copy or on website), the title is the “ first detail” or “face” of the paper that is read.[ 2 , 3 , 4 , 5 , 6 , 13 ] Hence, it needs to be simple, direct, accurate, appropriate, specific, functional, interesting, attractive/appealing, concise/brief, precise/focused, unambiguous, memorable, captivating, informative (enough to encourage the reader to read further), unique, catchy, and it should not be misleading.[ 1 , 2 , 3 , 4 , 5 , 6 , 9 , 12 ] It should have “just enough details” to arouse the interest and curiosity of the reader so that the reader then goes ahead with studying the abstract and then (if still interested) the full paper.[ 1 , 2 , 4 , 13 ] Journal websites, electronic databases, and search engines use the words in the title and abstract (the “keywords”) to retrieve a particular paper during a search; hence, the importance of these words in accessing the paper by the readers has been emphasized.[ 3 , 4 , 5 , 6 , 12 , 14 ] Such important words (or keywords) should be arranged in appropriate order of importance as per the context of the paper and should be placed at the beginning of the title (rather than the later part of the title, as some search engines like Google may just display only the first six to seven words of the title).[ 3 , 5 , 12 ] Whimsical, amusing, or clever titles, though initially appealing, may be missed or misread by the busy reader and very short titles may miss the essential scientific words (the “keywords”) used by the indexing agencies to catch and categorize the paper.[ 1 , 3 , 4 , 9 ] Also, amusing or hilarious titles may be taken less seriously by the readers and may be cited less often.[ 4 , 15 ] An excessively long or complicated title may put off the readers.[ 3 , 9 ] It may be a good idea to draft the title after the main body of the text and the abstract are drafted.[ 2 , 3 , 4 , 5 ]
Types of titles
Titles can be descriptive, declarative, or interrogative. They can also be classified as nominal, compound, or full-sentence titles.
Descriptive or neutral title
This has the essential elements of the research theme, that is, the patients/subjects, design, interventions, comparisons/control, and outcome, but does not reveal the main result or the conclusion.[ 3 , 4 , 12 , 16 ] Such a title allows the reader to interpret the findings of the research paper in an impartial manner and with an open mind.[ 3 ] These titles also give complete information about the contents of the article, have several keywords (thus increasing the visibility of the article in search engines), and have increased chances of being read and (then) being cited as well.[ 4 ] Hence, such descriptive titles giving a glimpse of the paper are generally preferred.[ 4 , 16 ]
Declarative title
This title states the main finding of the study in the title itself; it reduces the curiosity of the reader, may point toward a bias on the part of the author, and hence is best avoided.[ 3 , 4 , 12 , 16 ]
Interrogative title
This is the one which has a query or the research question in the title.[ 3 , 4 , 16 ] Though a query in the title has the ability to sensationalize the topic, and has more downloads (but less citations), it can be distracting to the reader and is again best avoided for a research article (but can, at times, be used for a review article).[ 3 , 6 , 16 , 17 ]
From a sentence construct point of view, titles may be nominal (capturing only the main theme of the study), compound (with subtitles to provide additional relevant information such as context, design, location/country, temporal aspect, sample size, importance, and a provocative or a literary; for example, see the title of this review), or full-sentence titles (which are longer and indicate an added degree of certainty of the results).[ 4 , 6 , 9 , 16 ] Any of these constructs may be used depending on the type of article, the key message, and the author's preference or judgement.[ 4 ]
Drafting a suitable title
A stepwise process can be followed to draft the appropriate title. The author should describe the paper in about three sentences, avoiding the results and ensuring that these sentences contain important scientific words/keywords that describe the main contents and subject of the paper.[ 1 , 4 , 6 , 12 ] Then the author should join the sentences to form a single sentence, shorten the length (by removing redundant words or adjectives or phrases), and finally edit the title (thus drafted) to make it more accurate, concise (about 10–15 words), and precise.[ 1 , 3 , 4 , 5 , 9 ] Some journals require that the study design be included in the title, and this may be placed (using a colon) after the primary title.[ 2 , 3 , 4 , 14 ] The title should try to incorporate the Patients, Interventions, Comparisons and Outcome (PICO).[ 3 ] The place of the study may be included in the title (if absolutely necessary), that is, if the patient characteristics (such as study population, socioeconomic conditions, or cultural practices) are expected to vary as per the country (or the place of the study) and have a bearing on the possible outcomes.[ 3 , 6 ] Lengthy titles can be boring and appear unfocused, whereas very short titles may not be representative of the contents of the article; hence, optimum length is required to ensure that the title explains the main theme and content of the manuscript.[ 4 , 5 , 9 ] Abbreviations (except the standard or commonly interpreted ones such as HIV, AIDS, DNA, RNA, CDC, FDA, ECG, and EEG) or acronyms should be avoided in the title, as a reader not familiar with them may skip such an article and nonstandard abbreviations may create problems in indexing the article.[ 3 , 4 , 5 , 6 , 9 , 12 ] Also, too much of technical jargon or chemical formulas in the title may confuse the readers and the article may be skipped by them.[ 4 , 9 ] Numerical values of various parameters (stating study period or sample size) should also be avoided in the titles (unless deemed extremely essential).[ 4 ] It may be worthwhile to take an opinion from a impartial colleague before finalizing the title.[ 4 , 5 , 6 ] Thus, multiple factors (which are, at times, a bit conflicting or contrasting) need to be considered while formulating a title, and hence this should not be done in a hurry.[ 4 , 6 ] Many journals ask the authors to draft a “short title” or “running head” or “running title” for printing in the header or footer of the printed paper.[ 3 , 12 ] This is an abridged version of the main title of up to 40–50 characters, may have standard abbreviations, and helps the reader to navigate through the paper.[ 3 , 12 , 14 ]
Checklist for a good title
Table 1 gives a checklist/useful tips for drafting a good title for a research paper.[ 1 , 2 , 3 , 4 , 5 , 6 , 12 ] Table 2 presents some of the titles used by the author of this article in his earlier research papers, and the appropriateness of the titles has been commented upon. As an individual exercise, the reader may try to improvise upon the titles (further) after reading the corresponding abstract and full paper.
Checklist/useful tips for drafting a good title for a research paper
Some titles used by author of this article in his earlier publications and remark/comment on their appropriateness
The Abstract
The details of the abstract are discussed under the subheadings of importance, types, drafting, and checklist.
Importance of the abstract
The abstract is a summary or synopsis of the full research paper and also needs to have similar characteristics like the title. It needs to be simple, direct, specific, functional, clear, unbiased, honest, concise, precise, self-sufficient, complete, comprehensive, scholarly, balanced, and should not be misleading.[ 1 , 2 , 3 , 7 , 8 , 9 , 10 , 11 , 13 , 17 ] Writing an abstract is to extract and summarize (AB – absolutely, STR – straightforward, ACT – actual data presentation and interpretation).[ 17 ] The title and abstracts are the only sections of the research paper that are often freely available to the readers on the journal websites, search engines, and in many abstracting agencies/databases, whereas the full paper may attract a payment per view or a fee for downloading the pdf copy.[ 1 , 2 , 3 , 7 , 8 , 10 , 11 , 13 , 14 ] The abstract is an independent and stand-alone (that is, well understood without reading the full paper) section of the manuscript and is used by the editor to decide the fate of the article and to choose appropriate reviewers.[ 2 , 7 , 10 , 12 , 13 ] Even the reviewers are initially supplied only with the title and the abstract before they agree to review the full manuscript.[ 7 , 13 ] This is the second most commonly read part of the manuscript, and therefore it should reflect the contents of the main text of the paper accurately and thus act as a “real trailer” of the full article.[ 2 , 7 , 11 ] The readers will go through the full paper only if they find the abstract interesting and relevant to their practice; else they may skip the paper if the abstract is unimpressive.[ 7 , 8 , 9 , 10 , 13 ] The abstract needs to highlight the selling point of the manuscript and succeed in luring the reader to read the complete paper.[ 3 , 7 ] The title and the abstract should be constructed using keywords (key terms/important words) from all the sections of the main text.[ 12 ] Abstracts are also used for submitting research papers to a conference for consideration for presentation (as oral paper or poster).[ 9 , 13 , 17 ] Grammatical and typographic errors reflect poorly on the quality of the abstract, may indicate carelessness/casual attitude on part of the author, and hence should be avoided at all times.[ 9 ]
Types of abstracts
The abstracts can be structured or unstructured. They can also be classified as descriptive or informative abstracts.
Structured and unstructured abstracts
Structured abstracts are followed by most journals, are more informative, and include specific subheadings/subsections under which the abstract needs to be composed.[ 1 , 7 , 8 , 9 , 10 , 11 , 13 , 17 , 18 ] These subheadings usually include context/background, objectives, design, setting, participants, interventions, main outcome measures, results, and conclusions.[ 1 ] Some journals stick to the standard IMRAD format for the structure of the abstracts, and the subheadings would include Introduction/Background, Methods, Results, And (instead of Discussion) the Conclusion/s.[ 1 , 2 , 7 , 8 , 9 , 10 , 11 , 12 , 13 , 17 , 18 ] Structured abstracts are more elaborate, informative, easy to read, recall, and peer-review, and hence are preferred; however, they consume more space and can have same limitations as an unstructured abstract.[ 7 , 9 , 18 ] The structured abstracts are (possibly) better understood by the reviewers and readers. Anyway, the choice of the type of the abstract and the subheadings of a structured abstract depend on the particular journal style and is not left to the author's wish.[ 7 , 10 , 12 ] Separate subheadings may be necessary for reporting meta-analysis, educational research, quality improvement work, review, or case study.[ 1 ] Clinical trial abstracts need to include the essential items mentioned in the CONSORT (Consolidated Standards Of Reporting Trials) guidelines.[ 7 , 9 , 14 , 19 ] Similar guidelines exist for various other types of studies, including observational studies and for studies of diagnostic accuracy.[ 20 , 21 ] A useful resource for the above guidelines is available at www.equator-network.org (Enhancing the QUAlity and Transparency Of health Research). Unstructured (or non-structured) abstracts are free-flowing, do not have predefined subheadings, and are commonly used for papers that (usually) do not describe original research.[ 1 , 7 , 9 , 10 ]
The four-point structured abstract: This has the following elements which need to be properly balanced with regard to the content/matter under each subheading:[ 9 ]
Background and/or Objectives: This states why the work was undertaken and is usually written in just a couple of sentences.[ 3 , 7 , 8 , 9 , 10 , 12 , 13 ] The hypothesis/study question and the major objectives are also stated under this subheading.[ 3 , 7 , 8 , 9 , 10 , 12 , 13 ]
Methods: This subsection is the longest, states what was done, and gives essential details of the study design, setting, participants, blinding, sample size, sampling method, intervention/s, duration and follow-up, research instruments, main outcome measures, parameters evaluated, and how the outcomes were assessed or analyzed.[ 3 , 7 , 8 , 9 , 10 , 12 , 13 , 14 , 17 ]
Results/Observations/Findings: This subheading states what was found, is longer, is difficult to draft, and needs to mention important details including the number of study participants, results of analysis (of primary and secondary objectives), and include actual data (numbers, mean, median, standard deviation, “P” values, 95% confidence intervals, effect sizes, relative risks, odds ratio, etc.).[ 3 , 7 , 8 , 9 , 10 , 12 , 13 , 14 , 17 ]
Conclusions: The take-home message (the “so what” of the paper) and other significant/important findings should be stated here, considering the interpretation of the research question/hypothesis and results put together (without overinterpreting the findings) and may also include the author's views on the implications of the study.[ 3 , 7 , 8 , 9 , 10 , 12 , 13 , 14 , 17 ]
The eight-point structured abstract: This has the following eight subheadings – Objectives, Study Design, Study Setting, Participants/Patients, Methods/Intervention, Outcome Measures, Results, and Conclusions.[ 3 , 9 , 18 ] The instructions to authors given by the particular journal state whether they use the four- or eight-point abstract or variants thereof.[ 3 , 14 ]
Descriptive and Informative abstracts
Descriptive abstracts are short (75–150 words), only portray what the paper contains without providing any more details; the reader has to read the full paper to know about its contents and are rarely used for original research papers.[ 7 , 10 ] These are used for case reports, reviews, opinions, and so on.[ 7 , 10 ] Informative abstracts (which may be structured or unstructured as described above) give a complete detailed summary of the article contents and truly reflect the actual research done.[ 7 , 10 ]
Drafting a suitable abstract
It is important to religiously stick to the instructions to authors (format, word limit, font size/style, and subheadings) provided by the journal for which the abstract and the paper are being written.[ 7 , 8 , 9 , 10 , 13 ] Most journals allow 200–300 words for formulating the abstract and it is wise to restrict oneself to this word limit.[ 1 , 2 , 3 , 7 , 8 , 9 , 10 , 11 , 12 , 13 , 22 ] Though some authors prefer to draft the abstract initially, followed by the main text of the paper, it is recommended to draft the abstract in the end to maintain accuracy and conformity with the main text of the paper (thus maintaining an easy linkage/alignment with title, on one hand, and the introduction section of the main text, on the other hand).[ 2 , 7 , 9 , 10 , 11 ] The authors should check the subheadings (of the structured abstract) permitted by the target journal, use phrases rather than sentences to draft the content of the abstract, and avoid passive voice.[ 1 , 7 , 9 , 12 ] Next, the authors need to get rid of redundant words and edit the abstract (extensively) to the correct word count permitted (every word in the abstract “counts”!).[ 7 , 8 , 9 , 10 , 13 ] It is important to ensure that the key message, focus, and novelty of the paper are not compromised; the rationale of the study and the basis of the conclusions are clear; and that the abstract is consistent with the main text of the paper.[ 1 , 2 , 3 , 7 , 9 , 11 , 12 , 13 , 14 , 17 , 22 ] This is especially important while submitting a revision of the paper (modified after addressing the reviewer's comments), as the changes made in the main (revised) text of the paper need to be reflected in the (revised) abstract as well.[ 2 , 10 , 12 , 14 , 22 ] Abbreviations should be avoided in an abstract, unless they are conventionally accepted or standard; references, tables, or figures should not be cited in the abstract.[ 7 , 9 , 10 , 11 , 13 ] It may be worthwhile not to rush with the abstract and to get an opinion by an impartial colleague on the content of the abstract; and if possible, the full paper (an “informal” peer-review).[ 1 , 7 , 8 , 9 , 11 , 17 ] Appropriate “Keywords” (three to ten words or phrases) should follow the abstract and should be preferably chosen from the Medical Subject Headings (MeSH) list of the U.S. National Library of Medicine ( https://meshb.nlm.nih.gov/search ) and are used for indexing purposes.[ 2 , 3 , 11 , 12 ] These keywords need to be different from the words in the main title (the title words are automatically used for indexing the article) and can be variants of the terms/phrases used in the title, or words from the abstract and the main text.[ 3 , 12 ] The ICMJE (International Committee of Medical Journal Editors; http://www.icmje.org/ ) also recommends publishing the clinical trial registration number at the end of the abstract.[ 7 , 14 ]
Checklist for a good abstract
Table 3 gives a checklist/useful tips for formulating a good abstract for a research paper.[ 1 , 2 , 3 , 7 , 8 , 9 , 10 , 11 , 12 , 13 , 14 , 17 , 22 ]
Checklist/useful tips for formulating a good abstract for a research paper
Concluding Remarks
This review article has given a detailed account of the importance and types of titles and abstracts. It has also attempted to give useful hints for drafting an appropriate title and a complete abstract for a research paper. It is hoped that this review will help the authors in their career in medical writing.
Financial support and sponsorship
Conflicts of interest.
There are no conflicts of interest.
Acknowledgement
The author thanks Dr. Hemant Deshmukh - Dean, Seth G.S. Medical College & KEM Hospital, for granting permission to publish this manuscript.
Do research articles with more readable abstracts receive higher online attention? Evidence from Science
- Published: 05 August 2021
- Volume 126 , pages 8471–8490, ( 2021 )
Cite this article
- Tan Jin ORCID: orcid.org/0000-0002-6421-0977 1 ,
- Huiqiong Duan 1 ,
- Xiaofei Lu 2 ,
- Jing Ni 3 &
- Kai Guo ORCID: orcid.org/0000-0001-9699-7527 4
1717 Accesses
16 Citations
2 Altmetric
Explore all metrics
The value of scientific research is manifested in its impact in the scientific community as well as among the general public. Given the importance of abstracts in determining whether research articles (RAs) may be retrieved and read, recent research is paying attention to the effect of abstract readability on the scientific impact of RAs. However, to date little research has looked into the effect of abstract readability on the impact of RAs among the general public. To address this gap, this study reports on an investigation into the relationship between abstract readability and online attention received by RAs. Our dataset consisted of the abstracts of 550 RAs from 11 disciplines published in Science in 2012 and 2018. Thirty-nine lexical and syntactic complexity indices were employed to measure the readability of the abstracts, and the Altmetric attention scores of the RAs were used to measure the online attention they received. Results showed that abstract readability is significantly related to the online attention RAs receive, and that this relationship is significantly affected by discipline and publication time. Our findings have useful implications for making RA abstracts accessible to the general public.
This is a preview of subscription content, log in via an institution to check access.
Access this article
Price includes VAT (Russian Federation)
Instant access to the full article PDF.
Rent this article via DeepDyve
Institutional subscriptions
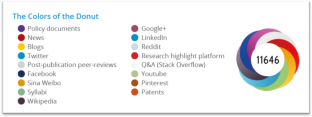
Similar content being viewed by others
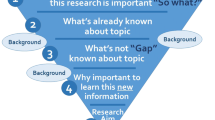
How to Write and Publish a Research Paper for a Peer-Reviewed Journal
Clara Busse & Ella August
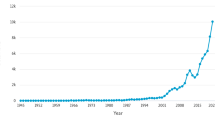
Literature reviews as independent studies: guidelines for academic practice
Sascha Kraus, Matthias Breier, … João J. Ferreira
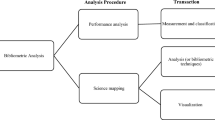
How to design bibliometric research: an overview and a framework proposal
Oğuzhan Öztürk, Rıdvan Kocaman & Dominik K. Kanbach
http://www.personal.psu.edu/xxl13/downloads .
Adie, E., & Roe, W. (2013). Altmetric: Enriching scholarly content with article-level discussion and metrics. Learned Publishing, 26 (1), 11–17.
Article Google Scholar
Altmetric Website. (2020). Colors of the donut. Retrieved on 30 August 2020 from https://www.altmetric.com/about-our-data/the-donut-and-score/
Altmetric Website. (2021). How is the Altmetric Attention Score calculated?. Retrieved on 25 April 2021 from https://help.altmetric.com/support/solutions/articles/6000233311-how-is-the-altmetric-attention-score-calculated -
Benjamin, R. G. (2012). Reconstructing readability: Recent developments and recommendations in the analysis of text difficulty. Educational Psychology Review, 24 (1), 63–88.
Bornmann, L. (2014). Do altmetrics point to the broader impact of research? An overview of benefits and disadvantages of altmetrics. Journal of Informetrics, 8 (4), 895–903.
Brigham, T. J. (2014). An introduction to Altmetrics. Medical Reference Services Quarterly, 33 (4), 438–447.
Chen, B., Deng, D., Zhong, Z., & Zhang, C. (2020). Exploring linguistic characteristics of highly browsed and downloaded academic articles. Scientometrics, 122 (3), 1769–1790.
Coiro, J. (2021). Toward a multifaceted heuristic of digital reading to inform assessment, research, practice, and policy. Reading Research Quarterly , 56 (1), 9–31.
Costas, R., Zahedi, Z., & Wouters, P. (2015). Do “altmetrics” correlate with citations? Extensive comparison of altmetric indicators with citations from a multidisciplinary perspective. Journal of the Association for Information Science and Technology, 66 (10), 2003–2019.
Crossley, S. A., Skalicky, S., & Dascalu, M. (2019). Moving beyond classic readability formulas: New methods and new models. Journal of Research in Reading, 42 (3–4), 541–561.
D’Angelo, C. A., & Di Russo, S. (2019). Testing for universality of Mendeley readership distributions. Journal of Informetrics, 13 (2), 726–737.
Díaz-Faes, A. A., Bowman, T. D., & Costas, R. (2019). Towards a second generation of ‘social media metrics’: Characterizing Twitter communities of attention around science. PLoS ONE, 14 (5), 1–18.
Didegah, F., & Thelwall, M. (2013). Which factors help authors produce the highest impact research? Collaboration, journal and document properties. Journal of Informetrics, 7 (4), 861–873.
Dolnicar, S., & Chapple, A. (2015). The readability of articles in tourism journals. Annals of Tourism Research, 52 , 161–166.
Dronberger, G. B., & Kowitz, G. T. (1975). Abstract readability as a factor in information systems. Journal of the American Society for Information Science, 26 (2), 108–111.
Flesch, R. (1948). A new readability yardstick. Journal of Applied Psychology, 32 (3), 221–233.
Gazni, A. (2011). Are the abstracts of high impact articles more readable? Investigating the evidence from top research institutions in the world. Journal of Information Science, 37 (3), 273–281.
Graesser, A. C., McNamara, D. S., & Kulikowich, J. M. (2011). Coh-Metrix: Providing multilevel analyses of text characteristics. Educational Researcher, 40 (5), 223–234.
Guerini, M., Pepe, A., & Lepri, B. (2012, June). Do linguistic style and readability of scientific abstracts affect their virality? In Proceedings of the 6th International AAAI Conference on Weblogs and Social Media (pp. 475–478). Dublin, Ireland.
Haberlandt, K. F., & Graesser, A. C. (1985). Component processes in text comprehension and some of their interactions. Journal of Experimental Psychology: General, 114 (3), 357–374.
Hartley, J. (2000). Clarifying the abstracts of systematic literature reviews. Bulletin of the Medical Library Association, 88 (4), 332–337.
Google Scholar
Hartley, J., & Sydes, M. (1997). Are structured abstracts easier to read than traditional ones? Journal of Research in Reading, 20 (2), 122–136.
Houghton, J. W., Henty, M., & Steele, C. (2004). Research practices and scholarly communication in the digital environment. Learned Publishing, 17 (3), 231–249.
Htoo, T. H. H., & Na, J. C. (2017). Disciplinary differences in altmetrics for social sciences. Online Information Review, 41 (2), 235–251.
Huang, W., Wang, P., & Wu, Q. (2018). A correlation comparison between Altmetric Attention Scores and citations for six PLOS journals. PLoS One , 13 (4), e0194962.
Just, M. A., & Carpenter, P. A. (1980). A theory of reading: From eye fixations to comprehension. Psychological Review, 87 (4), 329–354.
King, R. (1976). A comparison of the readability of abstracts with their source documents. Journal of the American Society for Information Science, 27 (2), 118–121.
Kintsch, W., & van Dijk, T. A. (1978). Toward a model of text comprehension and production. Psychological Review, 85 (5), 363–394.
Klare, G. R. (1963). The measurement of readability . Iowa State University Press.
Klare, G. R. (1974). Assessing readability. Reading Research Quarterly, 10 (1), 62–102.
Larson-Hall, J. (2015). A guide to doing statistics in second language research using SPSS and R (2nd ed.). Routledge.
Book Google Scholar
Laufer, B., & Nation, P. (1995). Vocabulary size and use: Lexical richness in L2 written production. Applied Linguistics, 16 (3), 307–322.
Lei, L., & Yan, S. (2016). Readability and citations in information science: Evidence from abstracts and articles of four journals (2003–2012). Scientometrics, 108 (3), 1155–1169.
Article MathSciNet Google Scholar
Lu, C., Bu, Y., Dong, X., Wang, J., Ding, Y., Larivière, V., Sugimoto, C. R., Paul, L., & Zhang, C. (2019). Analyzing linguistic complexity and scientific impact. Journal of Informetrics, 13 (3), 817–829.
Lu, X. (2010). Automatic analysis of syntactic complexity in second language writing. International Journal of Corpus Linguistics, 15 (4), 474–496.
Lu, X. (2012). The relationship of lexical richness to the quality of ESL learners’ oral narratives. Modern Language Journal, 96 (2), 190–208.
Lu, X. (2014). Computational methods for corpus annotation and analysis . Springer.
Book MATH Google Scholar
Lu, X., Casal, J. E., & Liu, Y. (2020). The rhetorical functions of syntactically complex sentences in social science research article introductions. Journal of English for Academic Purposes , 44 , 100832.
Lu, X., Gamson, D. A., & Eckert, S. A. (2014). Lexical difficulty and diversity in American elementary school reading textbooks: Changes over the past century. International Journal of Corpus Linguistics, 19 (1), 94–117.
Maflahi, N., & Thelwall, M. (2018). How quickly do publications get read? The evolution of Mendeley reader counts for new articles. Journal of the Association for Information Science and Technology, 69 (1), 158–167.
McLaughlin, G. H. (1969). SMOG grading: A new readability formula. Journal of Reading, 12 (8), 639–646.
Minnen, G., Carroll, J., & Pearce, D. (2001). Applied morphological processing of English. Natural Language Engineering, 7 (3), 207–223.
Mohammadi, E., Thelwall, M., Haustein, S., & Larivière, V. (2015). Who reads research articles? An altmetrics analysis of Mendeley user categories. Journal of the Association for Information Science and Technology, 66 (9), 1832–1846.
Newbold, N., & Gillam, L. (2010). The linguistics of readability: The next step for word processing. In Proceedings of the NAACL HLT 2010 Workshop on Computational Linguistics and Writing: Writing Processes and Authoring Aids (pp. 65–72). Los Angeles, California: Association for Computational Linguistics.
Ngai, C. S. B., & Singh, R. G. (2020). Relationship between persuasive metadiscoursal devices in research article abstracts and their attention on social media. PLoS One , 15 (4), e0231305.
Nicholas, D., Huntington, P., & Watkinson, A. (2003). Digital journals, Big Deals and online searching behavior: A pilot study. Aslib Proceedings: New Information Perspectives, 55 (1/2), 84–109.
OASIS Website. (2020). Why accessible summaries. Retrieved on 30 August 2020 from https://oasis-database.org/about
Perfetti, C. A. (2007). Reading ability: Lexical quality to comprehension. Scientific Studies of Reading, 11 (4), 357–383.
Pinto, M., & Lancaster, F. W. (1999). Abstracts and abstracting in knowledge discovery. Library Trends , 48 (1), 234–248.
Science Website. (2020a). Mission and scope. Retrieved on 30 August 2020 from https://www.sciencemag.org/about/mission-and-scope
Science Website. (2020b). Information for authors. Retrieved on 30 August 2020 from https://www.sciencemag.org/authors/science-information-authors
Science Website. (2020c). Instructions for preparing an initial manuscript. Retrieved on 30 August 2020 from https://www.sciencemag.org/authors/instructions-preparing-initial-manuscript
Sienkiewicz, J., & Altmann, E. G. (2016). Impact of lexical and sentiment factors on the popularity of scientific papers. Royal Society Open Science , 3 (6), 160140.
Snow, C. E. (2010). Academic language and the challenge of reading for learning about science. Science, 328 (5977), 450–453.
Stevens, R. J., Lu, X., Baker, D. P., Ray, M. N., Eckert, S. A., & Gamson, D. A. (2015). Assessing the cognitive demands of elementary school reading curricula: An analysis of reading text and comprehension tasks from 1910 to 2000. American Educational Research Journal, 52 (3), 582–617.
Sud, P., & Thelwall, M. (2014). Evaluating altmetrics. Scientometrics, 98 (2), 1131–1143.
Sugimoto, C. R., Work, S., Larivière, V., & Haustein, S. (2017). Scholarly use of social media and altmetrics: A review of the literature. Journal of the Association for Information Science and Technology, 68 (9), 2037–2062.
Syamili, C., & Rekha, R. V. (2017). Do altmetric correlate with citation?: A study basedon PLOS ONE journal. COLLNET Journal of Scientometrics and Information Management, 11 (1), 103–117.
Tankó, G. (2017). Literary research article abstracts: An analysis of rhetorical moves and their linguistic realizations. Journal of English for Academic Purposes, 27 , 42–55.
Thelwall, M. (2018). Early Mendeley readers correlate with later citation counts. Scientometrics, 115 (3), 1231–1240.
Thelwall, M., Haustein, S., Larivière, V., & Sugimoto, C. R. (2013). Do altmetrics work? Twitter and ten other social web services. PLoS ONE, 8 (5), 1–7.
Toutanova, K., Klein, D., Manning, C., & Singer, Y. (2003). Feature-rich part-of-speech tagging with a cyclic dependency network. In Proceedings of the 2003 Human Language Technology Conference of the North American Chapter of the Association for Computational Linguistics (pp. 252–259). Edmonton, Alberta, Canada: The Association for Computational Linguistics.
Trueger, N. S., Thoma, B., Hsu, C. H., Sullivan, D., Peters, L., & Lin, M. (2015). The altmetric score: A new measure for article-level dissemination and impact. Annals of Emergency Medicine, 66 (5), 549–553.
Vajjala, S., & Meurers, D. (2012, June). On improving the accuracy of readability classification using insights from second language acquisition. In Proceedings of the 7th Workshop on Innovative Use of NLP for Building Educational Applications (pp. 163–173). Montréal, Canada: Association for Computational Linguistics.
Verma, S., & Madhusudhan, M. (2019). An altmetric comparison of highly cited digital library publications of India and China. Annals of Library and Information Studies, 66 (2), 71–75.
Weil, B. H. (1970). Standards for writing abstracts. Journal of the American Society for Information Science, 21 (5), 351–357.
Wolfe-Quintero, K., Inagaki, S., & Kim, H.-Y. (1998). Second language development in writing: Measures of fluency, accuracy, and complexity . University of Hawaii Press.
Zahedi, Z., Costas, R., & Wouters, P. (2014). How well developed are altmetrics? A cross-disciplinary analysis of the presence of ‘alternative metrics’ in scientific publications. Scientometrics, 101 (2), 1491–1513.
Download references
This research was supported by a grant from the National Social Science Fund of China (18BYY110) to the first author.
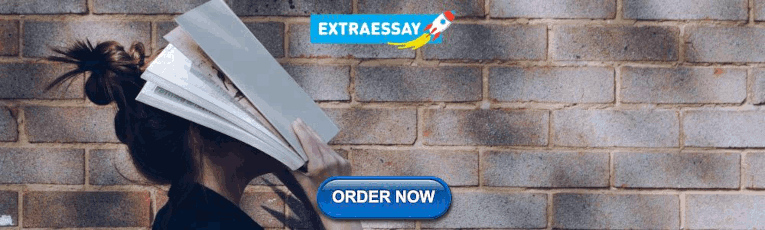
Author information
Authors and affiliations.
School of Foreign Languages, Sun Yat-Sen University, No. 135, Xingang Xi Road, Guangzhou, 510275, China
Tan Jin & Huiqiong Duan
Department of Applied Linguistics, The Pennsylvania State University, 234 Sparks Building, University Park, PA, 16802, USA
Faculty of Nursing, Jiujiang University, No. 320, Xunyang East Road, Jiujiang, 332000, China
Faculty of Education, The University of Hong Kong, Pokfulam Road, Hong Kong, 999077, China
You can also search for this author in PubMed Google Scholar
Contributions
TJ: Conceptualization, Methodology, Writing—review & editing. HD: Data curation, Investigation, Writing—review & editing. XL: Conceptualization, Methodology, Writing—review & editing. JN: Methodology, Investigation, Writing—review & editing. KG: Methodology, Investigation, Writing—review & editing.
Corresponding author
Correspondence to Kai Guo .
Rights and permissions
Reprints and permissions
About this article
Jin, T., Duan , H., Lu, X. et al. Do research articles with more readable abstracts receive higher online attention? Evidence from Science . Scientometrics 126 , 8471–8490 (2021). https://doi.org/10.1007/s11192-021-04112-9
Download citation
Received : 12 January 2021
Accepted : 22 July 2021
Published : 05 August 2021
Issue Date : October 2021
DOI : https://doi.org/10.1007/s11192-021-04112-9
Share this article
Anyone you share the following link with will be able to read this content:
Sorry, a shareable link is not currently available for this article.
Provided by the Springer Nature SharedIt content-sharing initiative
- Abstract readability
- Online attention
- Research articles
- Find a journal
- Publish with us
- Track your research
See More About
Select your interests.
Customize your JAMA Network experience by selecting one or more topics from the list below.
- Academic Medicine
- Acid Base, Electrolytes, Fluids
- Allergy and Clinical Immunology
- American Indian or Alaska Natives
- Anesthesiology
- Anticoagulation
- Art and Images in Psychiatry
- Artificial Intelligence
- Assisted Reproduction
- Bleeding and Transfusion
- Caring for the Critically Ill Patient
- Challenges in Clinical Electrocardiography
- Climate and Health
- Climate Change
- Clinical Challenge
- Clinical Decision Support
- Clinical Implications of Basic Neuroscience
- Clinical Pharmacy and Pharmacology
- Complementary and Alternative Medicine
- Consensus Statements
- Coronavirus (COVID-19)
- Critical Care Medicine
- Cultural Competency
- Dental Medicine
- Dermatology
- Diabetes and Endocrinology
- Diagnostic Test Interpretation
- Drug Development
- Electronic Health Records
- Emergency Medicine
- End of Life, Hospice, Palliative Care
- Environmental Health
- Equity, Diversity, and Inclusion
- Facial Plastic Surgery
- Gastroenterology and Hepatology
- Genetics and Genomics
- Genomics and Precision Health
- Global Health
- Guide to Statistics and Methods
- Hair Disorders
- Health Care Delivery Models
- Health Care Economics, Insurance, Payment
- Health Care Quality
- Health Care Reform
- Health Care Safety
- Health Care Workforce
- Health Disparities
- Health Inequities
- Health Policy
- Health Systems Science
- History of Medicine
- Hypertension
- Images in Neurology
- Implementation Science
- Infectious Diseases
- Innovations in Health Care Delivery
- JAMA Infographic
- Law and Medicine
- Leading Change
- Less is More
- LGBTQIA Medicine
- Lifestyle Behaviors
- Medical Coding
- Medical Devices and Equipment
- Medical Education
- Medical Education and Training
- Medical Journals and Publishing
- Mobile Health and Telemedicine
- Narrative Medicine
- Neuroscience and Psychiatry
- Notable Notes
- Nutrition, Obesity, Exercise
- Obstetrics and Gynecology
- Occupational Health
- Ophthalmology
- Orthopedics
- Otolaryngology
- Pain Medicine
- Palliative Care
- Pathology and Laboratory Medicine
- Patient Care
- Patient Information
- Performance Improvement
- Performance Measures
- Perioperative Care and Consultation
- Pharmacoeconomics
- Pharmacoepidemiology
- Pharmacogenetics
- Pharmacy and Clinical Pharmacology
- Physical Medicine and Rehabilitation
- Physical Therapy
- Physician Leadership
- Population Health
- Primary Care
- Professional Well-being
- Professionalism
- Psychiatry and Behavioral Health
- Public Health
- Pulmonary Medicine
- Regulatory Agencies
- Reproductive Health
- Research, Methods, Statistics
- Resuscitation
- Rheumatology
- Risk Management
- Scientific Discovery and the Future of Medicine
- Shared Decision Making and Communication
- Sleep Medicine
- Sports Medicine
- Stem Cell Transplantation
- Substance Use and Addiction Medicine
- Surgical Innovation
- Surgical Pearls
- Teachable Moment
- Technology and Finance
- The Art of JAMA
- The Arts and Medicine
- The Rational Clinical Examination
- Tobacco and e-Cigarettes
- Translational Medicine
- Trauma and Injury
- Treatment Adherence
- Ultrasonography
- Users' Guide to the Medical Literature
- Vaccination
- Venous Thromboembolism
- Veterans Health
- Women's Health
- Workflow and Process
- Wound Care, Infection, Healing
Others Also Liked
- Download PDF
- X Facebook More LinkedIn
Pitkin RM , Branagan MA , Burmeister LF. Accuracy of Data in Abstracts of Published Research Articles. JAMA. 1999;281(12):1110–1111. doi:10.1001/jama.281.12.1110
Manage citations:
© 2024
- Permissions
Accuracy of Data in Abstracts of Published Research Articles
Author Affiliations: Obstetrics & Gynecology , Los Angeles, Calif (Dr Pitkin); Chest , Northbrook, Ill (Ms Branagan); and Department of Preventive Medicine, University of Iowa, Iowa City (Dr Burmeister).
Context The section of a research article most likely to be read is the abstract, and therefore it is particularly important that the abstract reflect the article faithfully.
Objective To assess abstracts accompanying research articles published in 6 medical journals with respect to whether data in the abstract could be verified in the article itself.
Design Analysis of simple random samples of 44 articles and their accompanying abstracts published during 1 year (July 1, 1996-June 30, 1997) in each of 5 major general medical journals ( Annals of Internal Medicine , BMJ , JAMA, Lancet , and New England Journal of Medicine ) and a consecutive sample of 44 articles published during 15 months (July 1, 1996-August 15, 1997) in the CMAJ .
Main Outcome Measure Abstracts were considered deficient if they contained data that were either inconsistent with corresponding data in the article's body (including tables and figures) or not found in the body at all.
Results The proportion of deficient abstracts varied widely (18%-68%) and to a statistically significant degree ( P <.001) among the 6 journals studied.
Conclusions Data in the abstract that are inconsistent with or absent from the article's body are common, even in large-circulation general medical journals.
The abstract accompanying a research article, because it is often the only part of the article that will be read, should reflect fully and accurately the work reported. We observed in 1 medical specialty journal that a quarter or more of manuscripts returned after revision contained data in the abstract that could not be verified in the body of the paper. 1 If this problem were to persist in published articles, then a potential for misinterpretation would exist. In the present study, we surveyed research articles and their accompanying abstracts published recently in 6 medical journals to verify data in the abstract by relating them to corresponding data in the body of the report.
Articles studied included simple random samples of reports of original research (including meta-analyses but not other types of reviews) appearing in 5 medical journals between July 1, 1996, and June 30, 1997 ( Annals of Internal Medicine , BMJ , JAMA, Lancet , and New England Journal of Medicine ); all articles appearing in a sixth journal CMAJ ( Canadian Medical Association Journal ), between July 1, 1996, and August 15, 1997, were also studied. Additional inclusion criteria were (1) the article was accompanied by an abstract and (2) the article occupied at least 2 full journal pages.
To estimate the sample sizes, we used some preliminary observations 1 that 25% to 50% of articles published in 2 of the journals studied contained 1 or more deficiencies in abstracts. We assumed this rate would range from 10% to 40% across the 6 journals studied and that α was .05 and power was 0.8, yielding a projected sample size of 44 from each journal. From each of the 5 journals that published more than 44 research articles in the 2 volumes studied (July 1, 1996-June 30, 1997), we selected a computer-generated simple random sample of 44. From the CMAJ , we analyzed a consecutive cohort of all 44 articles published from July 1, 1996, through August 15, 1997.
For each selected article, the abstract was scrutinized by 1 of 3 examiners who identified each datum or other piece of information in the abstract and then sought to relate it to its source in the body of the article, including tables and figures. Two types of discrepancies were sought: (1) data given differently in the abstract and the body and (2) data given in the abstract but not in the body. If either was identified, the abstract was considered deficient. Discrepancies attributable to rounding were not considered to be deficiencies as long as the rounding was done appropriately, and the rounded value appeared in the abstract and the more detailed value in the body.
The proportions of articles containing deficiencies were compared across journals by χ 2 analysis. On the basis of normal approximation, 95% confidence intervals (CIs) were calculated for each proportion. We also performed a validation study by randomly selecting (using another computer-generated random number sequence) 7 of each set of 44 articles and having these examined by a second (and different) examiner.
Table 1 contains the proportions of deficient abstracts and 95% CIs for each journal, tabulated considering the abstract as the unit, as well as the types of deficiencies found in the 6 journals. The proportion of deficient abstracts ranged from a low of 18% to a high of 68%. Inconsistency between abstract and body was generally more common than omitted data (ie, data in the abstract not found in the body). A substantial proportion of deficient abstracts contained both kinds of defects (25/104; 24%).
In the validation study, 38 of the 42 paired comparisons were concordant with respect to identification of deficiencies. The κ value for agreement between the 2 evaluators was 0.81 ( z = 5.22; P <.001).
The frequency with which we found abstracts to be inaccurate, in the sense of containing information not verifiable in the article's main body (including tables and figures, as well as text) was surprisingly large, ranging from 18% to 68% in the 6 journals surveyed. The more common type of the 2 deficiencies was inconsistency between data in the abstract and those in the body. Giving data or other information in the abstract but not in the body was somewhat less common. These findings are all the more surprising considering that the journals studied are all prominent and highly regarded general medical publications whose editors were founding members of the International Committee of Medical Journal Editors, a respected standard-setting body. These journals have full-time professional staffs who can be presumed to devote a good deal of time and energy to editorial and production processes.
Many of the discrepancies identified were quite minor and not likely to cause serious misinterpretation. For example, 1 abstract 2 reported the population to consist of "42 consecutive patients," whereas the body indicated it to be "44 consecutive patients of which 42 agreed to participate." Sometimes, however, discrepancies were more serious; for example, 1 abstract 3 gave the estimated 15-year survival as 48%, whereas the body of the text indicated it to be 58%.
The specific question we asked in this study—Can the data and other information in the abstract be verified in the body of the article?—does not seem to have been examined before. Previous studies 4 , 5 of abstract quality generally involved overall or global assessment. Most of the recent literature on abstracts has concerned structured abstracts, introduced in 1987 6 with the goal of making abstracts more informative. Several investigations 7 - 9 indicated that structured abstracts are actually better in quality, more informative, more readable, and a more efficient use of readers' time. Structured abstracts may well offer all of these advantages, but there is little reason to expect them to reduce the types of deficiencies assessed in this study. Indeed, if structured abstracts are more informative (ie, if they provide more information), they might be more likely to be subject to deficiencies we assessed. In the present study, we could not discern any relationship between various structured formats and the deficiencies assessed.
It is important to acknowledge that we addressed only 1 aspect of abstract accuracy in asking if what is in the abstract is consistent with the body of the article. There is another, at least equally important question: Is the important information in the article found in the abstract? Our study was not designed to address this question.
We found previously 1 that providing authors with specific instructions about abstract accuracy when they are revising manuscripts is ineffective in preventing the types of defects assessed in this study. If it is important that abstracts be as accurate as possible—and it can hardly be argued otherwise—and if authors cannot be counted on to provide this level of accuracy, the responsibility must be taken by journals' editorial staffs. As part of the copyediting process, the abstract needs to be scrutinized painstakingly on a line-by-line or even word-by-word basis and each bit of information verified individually and specifically.
- Register for email alerts with links to free full-text articles
- Access PDFs of free articles
- Manage your interests
- Save searches and receive search alerts
Accuracy of data in abstracts of published research articles
Affiliation.
- 1 [email protected]
- PMID: 10188662
- DOI: 10.1001/jama.281.12.1110
Context: The section of a research article most likely to be read is the abstract, and therefore it is particularly important that the abstract reflect the article faithfully.
Objective: To assess abstracts accompanying research articles published in 6 medical journals with respect to whether data in the abstract could be verified in the article itself.
Design: Analysis of simple random samples of 44 articles and their accompanying abstracts published during 1 year(July 1, 1996-June 30, 1997) in each of 5 major general medical journals (Annals of Internal Medicine, BMJ, JAMA, Lancet, and New England Journal of Medicine) and a consecutive sample of 44 articles published during 15 months (July 1, 1996-August 15, 1997) in the CMAJ.
Main outcome measure: Abstracts were considered deficient if they contained data that were either inconsistent with corresponding data in the article's body (including tables and figures) or not found in the body at all.
Results: The proportion of deficient abstracts varied widely (18%-68%) and to a statistically significant degree (P<.001) among the 6 journals studied.
Conclusions: Data in the abstract that are inconsistent with or absent from the article's body are common, even in large-circulation general medical journals.
- Abstracting and Indexing*
- Publishing*
Thank you for visiting nature.com. You are using a browser version with limited support for CSS. To obtain the best experience, we recommend you use a more up to date browser (or turn off compatibility mode in Internet Explorer). In the meantime, to ensure continued support, we are displaying the site without styles and JavaScript.
- View all journals
- My Account Login
- Explore content
- About the journal
- Publish with us
- Sign up for alerts
- Open access
- Published: 08 April 2024
A neural speech decoding framework leveraging deep learning and speech synthesis
- Xupeng Chen 1 na1 ,
- Ran Wang 1 na1 ,
- Amirhossein Khalilian-Gourtani ORCID: orcid.org/0000-0003-1376-9583 2 ,
- Leyao Yu 2 , 3 ,
- Patricia Dugan 2 ,
- Daniel Friedman 2 ,
- Werner Doyle 4 ,
- Orrin Devinsky 2 ,
- Yao Wang ORCID: orcid.org/0000-0003-3199-3802 1 , 3 na2 &
- Adeen Flinker ORCID: orcid.org/0000-0003-1247-1283 2 , 3 na2
Nature Machine Intelligence ( 2024 ) Cite this article
1151 Accesses
293 Altmetric
Metrics details
- Neural decoding
A preprint version of the article is available at bioRxiv.
Decoding human speech from neural signals is essential for brain–computer interface (BCI) technologies that aim to restore speech in populations with neurological deficits. However, it remains a highly challenging task, compounded by the scarce availability of neural signals with corresponding speech, data complexity and high dimensionality. Here we present a novel deep learning-based neural speech decoding framework that includes an ECoG decoder that translates electrocorticographic (ECoG) signals from the cortex into interpretable speech parameters and a novel differentiable speech synthesizer that maps speech parameters to spectrograms. We have developed a companion speech-to-speech auto-encoder consisting of a speech encoder and the same speech synthesizer to generate reference speech parameters to facilitate the ECoG decoder training. This framework generates natural-sounding speech and is highly reproducible across a cohort of 48 participants. Our experimental results show that our models can decode speech with high correlation, even when limited to only causal operations, which is necessary for adoption by real-time neural prostheses. Finally, we successfully decode speech in participants with either left or right hemisphere coverage, which could lead to speech prostheses in patients with deficits resulting from left hemisphere damage.
Similar content being viewed by others
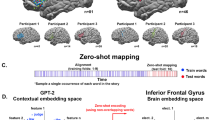
Alignment of brain embeddings and artificial contextual embeddings in natural language points to common geometric patterns
Ariel Goldstein, Avigail Grinstein-Dabush, … Uri Hasson
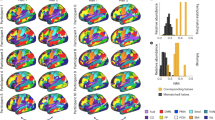
A precision functional atlas of personalized network topography and probabilities
Robert J. M. Hermosillo, Lucille A. Moore, … Damien A. Fair
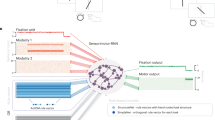
Natural language instructions induce compositional generalization in networks of neurons
Reidar Riveland & Alexandre Pouget
Speech loss due to neurological deficits is a severe disability that limits both work life and social life. Advances in machine learning and brain–computer interface (BCI) systems have pushed the envelope in the development of neural speech prostheses to enable people with speech loss to communicate 1 , 2 , 3 , 4 , 5 . An effective modality for acquiring data to develop such decoders involves electrocorticographic (ECoG) recordings obtained in patients undergoing epilepsy surgery 4 , 5 , 6 , 7 , 8 , 9 , 10 . Implanted electrodes in patients with epilepsy provide a rare opportunity to collect cortical data during speech with high spatial and temporal resolution, and such approaches have produced promising results in speech decoding 4 , 5 , 8 , 9 , 10 , 11 .
Two challenges are inherent to successfully carrying out speech decoding from neural signals. First, the data to train personalized neural-to-speech decoding models are limited in duration, and deep learning models require extensive training data. Second, speech production varies in rate, intonation, pitch and so on, even within a single speaker producing the same word, complicating the underlying model representation 12 , 13 . These challenges have led to diverse speech decoding approaches with a range of model architectures. Currently, public code to test and replicate findings across research groups is limited in availability.
Earlier approaches to decoding and synthesizing speech spectrograms from neural signals focused on linear models. These approaches achieved a Pearson correlation coefficient (PCC) of ~0.6 or lower, but with simple model architectures that are easy to interpret and do not require large training datasets 14 , 15 , 16 . Recent research has focused on deep neural networks leveraging convolutional 8 , 9 and recurrent 5 , 10 , 17 network architectures. These approaches vary across two major dimensions: the intermediate latent representation used to model speech and the speech quality produced after synthesis. For example, cortical activity has been decoded into an articulatory movement space, which is then transformed into speech, providing robust decoding performance but with a non-natural synthetic voice reconstruction 17 . Conversely, some approaches have produced naturalistic reconstruction leveraging wavenet vocoders 8 , generative adversarial networks (GAN) 11 and unit selection 18 , but achieve limited accuracy. A recent study in one implanted patient 19 provided both robust accuracies and a naturalistic speech waveform by leveraging quantized HuBERT features 20 as an intermediate representation space and a pretrained speech synthesizer that converts the HuBERT features into speech. However, HuBERT features do not carry speaker-dependent acoustic information and can only be used to generate a generic speaker’s voice, so they require a separate model to translate the generic voice to a specific patient’s voice. Furthermore, this study and most previous approaches have employed non-causal architectures, which may limit real-time applications, which typically require causal operations.
To address these issues, in this Article we present a novel ECoG-to-speech framework with a low-dimensional intermediate representation guided by subject-specific pre-training using speech signal only (Fig. 1 ). Our framework consists of an ECoG decoder that maps the ECoG signals to interpretable acoustic speech parameters (for example, pitch, voicing and formant frequencies), as well as a speech synthesizer that translates the speech parameters to a spectrogram. The speech synthesizer is differentiable, enabling us to minimize the spectrogram reconstruction error during training of the ECoG decoder. The low-dimensional latent space, together with guidance on the latent representation generated by a pre-trained speech encoder, overcomes data scarcity issues. Our publicly available framework produces naturalistic speech that highly resembles the speaker’s own voice, and the ECoG decoder can be realized with different deep learning model architectures and using different causality directions. We report this framework with multiple deep architectures (convolutional, recurrent and transformer) as the ECoG decoder, and apply it to 48 neurosurgical patients. Our framework performs with high accuracy across the models, with the best performance obtained by the convolutional (ResNet) architecture (PCC of 0.806 between the original and decoded spectrograms). Our framework can achieve high accuracy using only causal processing and relatively low spatial sampling on the cortex. We also show comparable speech decoding from grid implants on the left and right hemispheres, providing a proof of concept for neural prosthetics in patients suffering from expressive aphasia (with damage limited to the left hemisphere), although such an approach must be tested in patients with damage to the left hemisphere. Finally, we provide a publicly available neural decoding pipeline ( https://github.com/flinkerlab/neural_speech_decoding ) that offers flexibility in ECoG decoding architectures to push forward research across the speech science and prostheses communities.
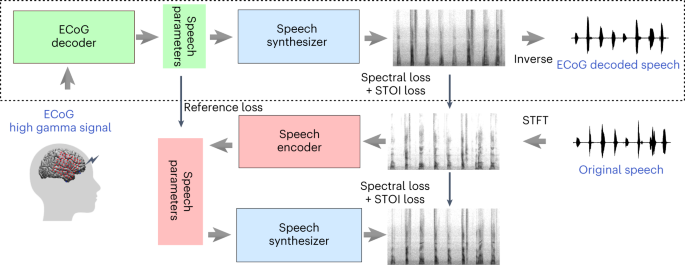
The upper part shows the ECoG-to-speech decoding pipeline. The ECoG decoder generates time-varying speech parameters from ECoG signals. The speech synthesizer generates spectrograms from the speech parameters. A separate spectrogram inversion algorithm converts the spectrograms to speech waveforms. The lower part shows the speech-to-speech auto-encoder, which generates the guidance for the speech parameters to be produced by the ECoG decoder during its training. The speech encoder maps an input spectrogram to the speech parameters, which are then fed to the same speech synthesizer to reproduce the spectrogram. The speech encoder and a few learnable subject-specific parameters in the speech synthesizer are pre-trained using speech signals only. Only the upper part is needed to decode the speech from ECoG signals once the pipeline is trained.
ECoG-to-speech decoding framework
Our ECoG-to-speech framework consists of an ECoG decoder and a speech synthesizer (shown in the upper part of Fig. 1 ). The neural signals are fed into an ECoG decoder, which generates speech parameters, followed by a speech synthesizer, which translates the parameters into spectrograms (which are then converted to a waveform by the Griffin–Lim algorithm 21 ). The training of our framework comprises two steps. We first use semi-supervised learning on the speech signals alone. An auto-encoder, shown in the lower part of Fig. 1 , is trained so that the speech encoder derives speech parameters from a given spectrogram, while the speech synthesizer (used here as the decoder) reproduces the spectrogram from the speech parameters. Our speech synthesizer is fully differentiable and generates speech through a weighted combination of voiced and unvoiced speech components generated from input time series of speech parameters, including pitch, formant frequencies, loudness and so on. The speech synthesizer has only a few subject-specific parameters, which are learned as part of the auto-encoder training (more details are provided in the Methods Speech synthesizer section). Currently, our speech encoder and speech synthesizer are subject-specific and can be trained using any speech signal of a participant, not just those with corresponding ECoG signals.
In the next step, we train the ECoG decoder in a supervised manner based on ground-truth spectrograms (using measures of spectrogram difference and short-time objective intelligibility, STOI 8 , 22 ), as well as guidance for the speech parameters generated by the pre-trained speech encoder (that is, reference loss between speech parameters). By limiting the number of speech parameters (18 at each time step; Methods section Summary of speech parameters ) and using the reference loss, the ECoG decoder can be trained with limited corresponding ECoG and speech data. Furthermore, because our speech synthesizer is differentiable, we can back-propagate the spectral loss (differences between the original and decoded spectrograms) to update the ECoG decoder. We provide multiple ECoG decoder architectures to choose from, including 3D ResNet 23 , 3D Swin Transformer 24 and LSTM 25 . Importantly, unlike many methods in the literature, we employ ECoG decoders that can operate in a causal manner, which is necessary for real-time speech generation from neural signals. Note that, once the ECoG decoder and speech synthesizer are trained, they can be used for ECoG-to-speech decoding without using the speech encoder.
Data collection
We employed our speech decoding framework across N = 48 participants who consented to complete a series of speech tasks (Methods section Experiments design). These participants, as part of their clinical care, were undergoing treatment for refractory epilepsy with implanted electrodes. During the hospital stay, we acquired synchronized neural and acoustic speech data. ECoG data were obtained from five participants with hybrid-density (HB) sampling (clinical-research grid) and 43 participants with low-density (LD) sampling (standard clinical grid), who took part in five speech tasks: auditory repetition (AR), auditory naming (AN), sentence completion (SC), word reading (WR) and picture naming (PN). These tasks were designed to elicit the same set of spoken words across tasks while varying the stimulus modality. We provided 50 repeated unique words (400 total trials per participant), all of which were analysed locked to the onset of speech production. We trained a model for each participant using 80% of available data for that participant and evaluated the model on the remaining 20% of data (with the exception of the more stringent word-level cross-validation).
Speech decoding performance and causality
We first aimed to directly compare the decoding performance across different architectures, including those that have been employed in the neural speech decoding literature (recurrent and convolutional) and transformer-based models. Although any decoder architecture could be used for the ECoG decoder in our framework, employing the same speech encoder guidance and speech synthesizer, we focused on three representative models for convolution (ResNet), recurrent (LSTM) and transformer (Swin) architectures. Note that any of these models can be configured to use temporally non-causal or causal operations. Our results show that ResNet outperformed the other models, providing the highest PCC across N = 48 participants (mean PCC = 0.806 and 0.797 for non-causal and causal, respectively), closely followed by Swin (mean PCC = 0.792 and 0.798 for non-causal and causal, respectively) (Fig. 2a ). We found the same when evaluating the three models using STOI+ (ref. 26 ), as shown in Supplementary Fig. 1a . The causality of machine learning models for speech production has important implications for BCI applications. A causal model only uses past and current neural signals to generate speech, whereas non-causal models use past, present and future neural signals. Previous reports have typically employed non-causal models 5 , 8 , 10 , 17 , which can use neural signals related to the auditory and speech feedback that is unavailable in real-time applications. Optimally, only the causal direction should be employed. We thus compared the performance of the same models with non-causal and causal temporal operations. Figure 2a compares the decoding results of causal and non-causal versions of our models. The causal ResNet model (PCC = 0.797) achieved a performance comparable to that of the non-causal model (PCC = 0.806), with no significant differences between the two (Wilcoxon two-sided signed-rank test P = 0.093). The same was true for the causal Swin model (PCC = 0.798) and its non-causal (PCC = 0.792) counterpart (Wilcoxon two-sided signed-rank test P = 0.196). In contrast, the performance of the causal LSTM model (PCC = 0.712) was significantly inferior to that of its non-causal (PCC = 0.745) version (Wilcoxon two-sided signed-rank test P = 0.009). Furthermore, the LSTM model showed consistently lower performance than ResNet and Swin. However, we did not find significant differences between the causal ResNet and causal Swin performances (Wilcoxon two-sided signed-rank test P = 0.587). Because the ResNet and Swin models had the highest performance and were on par with each other and their causal counterparts, we chose to focus further analyses on these causal models, which we believe are best suited for prosthetic applications.
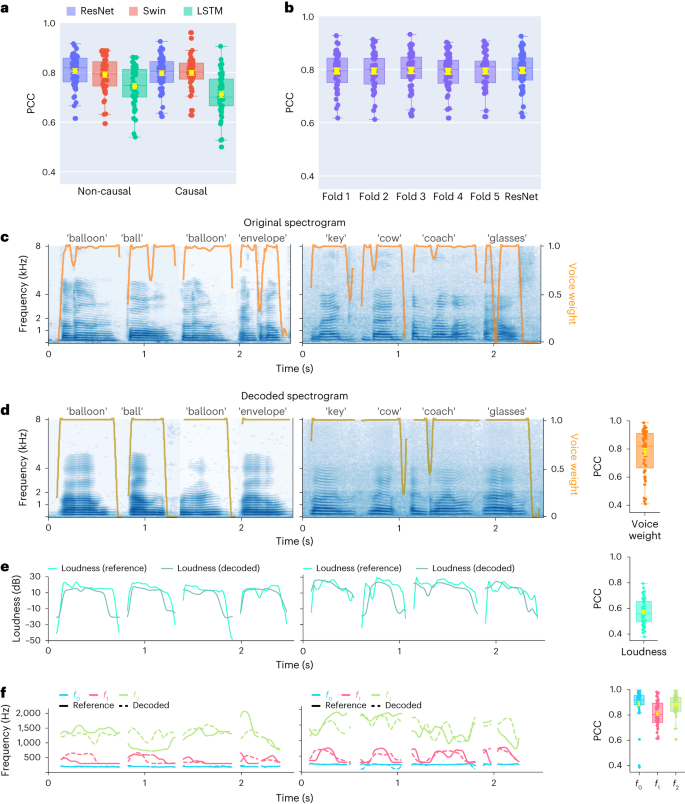
a , Performances of ResNet, Swin and LSTM models with non-causal and causal operations. The PCC between the original and decoded spectrograms is evaluated on the held-out testing set and shown for each participant. Each data point corresponds to a participant’s average PCC across testing trials. b , A stringent cross-validation showing the performance of the causal ResNet model on unseen words during training from five folds; we ensured that the training and validation sets in each fold did not overlap in unique words. The performance across all five validation folds was comparable to our trial-based validation, denoted for comparison as ResNet (identical to the ResNet causal model in a ). c – f , Examples of decoded spectrograms and speech parameters from the causal ResNet model for eight words (from two participants) and the PCC values for the decoded and reference speech parameters across all participants. Spectrograms of the original ( c ) and decoded ( d ) speech are shown, with orange curves overlaid representing the reference voice weight learned by the speech encoder ( c ) and the decoded voice weight from the ECoG decoder ( d ). The PCC between the decoded and reference voice weights is shown on the right across all participants. e , Decoded and reference loudness parameters for the eight words, and the PCC values of the decoded loudness parameters across participants (boxplot on the right). f , Decoded (dashed) and reference (solid) parameters for pitch ( f 0 ) and the first two formants ( f 1 and f 2 ) are shown for the eight words, as well as the PCC values across participants (box plots to the right). All box plots depict the median (horizontal line inside the box), 25th and 75th percentiles (box) and 25th or 75th percentiles ± 1.5 × interquartile range (whiskers) across all participants ( N = 48). Yellow error bars denote the mean ± s.e.m. across participants.
Source data
To ensure our framework can generalize well to unseen words, we added a more stringent word-level cross-validation in which random (ten unique) words were entirely held out during training (including both pre-training of the speech encoder and speech synthesizer and training of the ECoG decoder). This ensured that different trials from the same word could not appear in both the training and testing sets. The results shown in Fig. 2b demonstrate that performance on the held-out words is comparable to our standard trial-based held-out approach (Fig. 2a , ‘ResNet’). It is encouraging that the model can decode unseen validation words well, regardless of which words were held out during training.
Next, we show the performance of the ResNet causal decoder on the level of single words across two representative participants (LD grids). The decoded spectrograms accurately preserve the spectro-temporal structure of the original speech (Fig. 2c,d ). We also compare the decoded speech parameters with the reference parameters. For each parameter, we calculated the PCC between the decoded time series and the reference sequence, showing average PCC values of 0.781 (voice weight, Fig. 2d ), 0.571 (loudness, Fig. 2e ), 0.889 (pitch f 0 , Fig. 2f ), 0.812 (first formant f 1 , Fig. 2f ) and 0.883 (second formant f 2 , Fig. 2f ). Accurate reconstruction of the speech parameters, especially the pitch, voice weight and first two formants, is essential for accurate speech decoding and naturalistic reconstruction that mimics a participant’s voice. We also provide a non-causal version of Fig. 2 in Supplementary Fig. 2 . The fact that both non-causal and causal models can yield reasonable decoding results is encouraging.
Left-hemisphere versus right-hemisphere decoding
Most speech decoding studies have focused on the language- and speech-dominant left hemisphere 27 . However, little is known about decoding speech representations from the right hemisphere. To this end, we compared left- versus right-hemisphere decoding performance across our participants to establish the feasibility of a right-hemisphere speech prosthetic. For both our ResNet and Swin decoders, we found robust speech decoding from the right hemisphere (ResNet PCC = 0.790, Swin PCC = 0.798) that was not significantly different from that of the left (Fig. 3a , ResNet independent t -test, P = 0.623; Swin independent t -test, P = 0.968). A similar conclusion held when evaluating STOI+ (Supplementary Fig. 1b , ResNet independent t -test, P = 0.166; Swin independent t -test, P = 0.114). Although these results suggest that it may be feasible to use neural signals in the right hemisphere to decode speech for patients who suffer damage to the left hemisphere and are unable to speak 28 , it remains unknown whether intact left-hemisphere cortex is necessary to allow for speech decoding from the right hemisphere until tested in such patients.
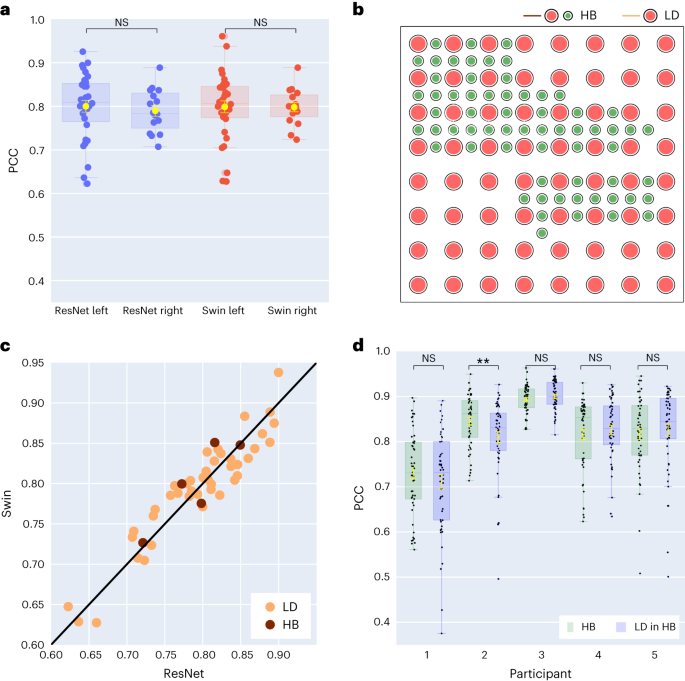
a , Comparison between left- and right-hemisphere participants using causal models. No statistically significant differences (ResNet independent t -test, P = 0.623; Swin Wilcoxon independent t -test, P = 0.968) in PCC values exist between left- ( N = 32) and right- ( N = 16) hemisphere participants. b , An example hybrid-density ECoG array with a total of 128 electrodes. The 64 electrodes marked in red correspond to a LD placement. The remaining 64 green electrodes, combined with red electrodes, reflect HB placement. c , Comparison between causal ResNet and causal Swin models for the same participant across participants with HB ( N = 5) or LD ( N = 43) ECoG grids. The two models show similar decoding performances from the HB and LD grids. d , Decoding PCC values across 50 test trials by the ResNet model for HB ( N = 5) participants when all electrodes are used versus when only LD-in-HB electrodes ( N = 5) are considered. There are no statistically significant differences for four out of five participants (Wilcoxon two-sided signed-rank test, P = 0.114, 0.003, 0.0773, 0.472 and 0.605, respectively). All box plots depict the median (horizontal line inside box), 25th and 75th percentiles (box) and 25th or 75th percentiles ± 1.5 × interquartile range (whiskers). Yellow error bars denote mean ± s.e.m. Distributions were compared with each other as indicated, using the Wilcoxon two-sided signed-rank test and independent t -test. ** P < 0.01; NS, not significant.
Effect of electrode density
Next, we assessed the impact of electrode sampling density on speech decoding, as many previous reports use higher-density grids (0.4 mm) with more closely spaced contacts than typical clinical grids (1 cm). Five participants consented to hybrid grids (Fig. 3b , HB), which typically had LD electrode sampling but with additional electrodes interleaved. The HB grids provided a decoding performance similar to clinical LD grids in terms of PCC values (Fig. 3c ), with a slight advantage in STOI+, as shown in Supplementary Fig. 3b . To ascertain whether the additional spatial sampling indeed provides improved speech decoding, we compared models that decode speech based on all the hybrid electrodes versus only the LD electrodes in participants with HB grids (comparable to our other LD participants). Our findings (Fig. 3d ) suggest that the decoding results were not significantly different from each other (with the exception of participant 2) in terms of PCC and STOI+ (Supplementary Fig. 3c ). Together, these results suggest that our models can learn speech representations well from both high and low spatial sampling of the cortex, with the exciting finding of robust speech decoding from the right hemisphere.
Contribution analysis
Finally, we investigated which cortical regions contribute to decoding to provide insight for the targeted implantation of future prosthetics, especially on the right hemisphere, which has not yet been investigated. We used an occlusion approach to quantify the contributions of different cortical sites to speech decoding. If a region is involved in decoding, occluding the neural signal in the corresponding electrode (that is, setting the signal to zero) will reduce the accuracy (PCC) of the speech reconstructed on testing data (Methods section Contribution analysis ). We thus measured each region’s contribution by decoding the reduction in the PCC when the corresponding electrode was occluded. We analysed all electrodes and participants with causal and non-causal versions of the ResNet and Swin decoders. The results in Fig. 4 show similar contributions for the ResNet and Swin models (Supplementary Figs. 8 and 9 describe the noise-level contribution). The non-causal models show enhanced auditory cortex contributions compared with the causal models, implicating auditory feedback in decoding, and underlying the importance of employing only causal models during speech decoding because neural feedback signals are not available for real-time decoding applications. Furthermore, across the causal models, both the right and left hemispheres show similar contributions across the sensorimotor cortex, especially on the ventral portion, suggesting the potential feasibility of right-hemisphere neural prosthetics.
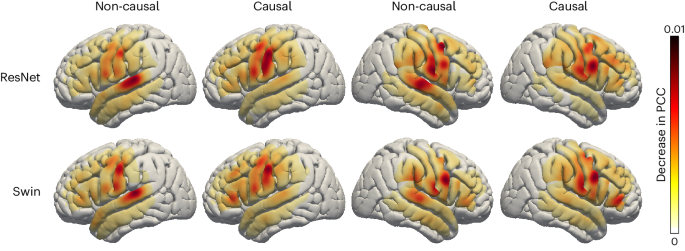
Visualization of the contribution of each cortical location to the decoding result achieved by both causal and non-causal decoding models through an occlusion analysis. The contribution of each electrode region in each participant is projected onto the standardized Montreal Neurological Institute (MNI) brain anatomical map and then averaged over all participants. Each subplot shows the causal or non-causal contribution of different cortical locations (red indicates a higher contribution; yellow indicates a lower contribution). For visualization purposes, we normalized the contribution of each electrode location by the local grid density, because there were multiple participants with non-uniform density.
Our novel pipeline can decode speech from neural signals by leveraging interchangeable architectures for the ECoG decoder and a novel differentiable speech synthesizer (Fig. 5 ). Our training process relies on estimating guidance speech parameters from the participants’ speech using a pre-trained speech encoder (Fig. 6a ). This strategy enabled us to train ECoG decoders with limited corresponding speech and neural data, which can produce natural-sounding speech when paired with our speech synthesizer. Our approach was highly reproducible across participants ( N = 48), providing evidence for successful causal decoding with convolutional (ResNet; Fig. 6c ) and transformer (Swin; Fig. 6d ) architectures, both of which outperformed the recurrent architecture (LSTM; Fig. 6e ). Our framework can successfully decode from both high and low spatial sampling with high levels of decoding performance. Finally, we provide potential evidence for robust speech decoding from the right hemisphere as well as the spatial contribution of cortical structures to decoding across the hemispheres.
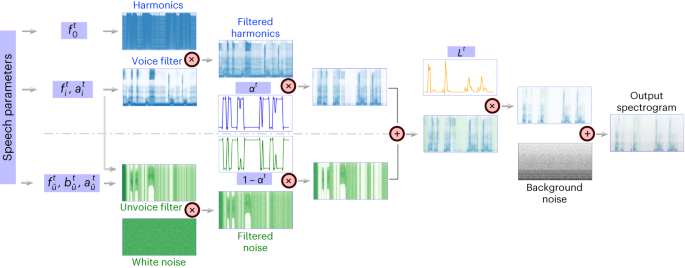
Our speech synthesizer generates the spectrogram at time t by combining a voiced component and an unvoiced component based on a set of speech parameters at t . The upper part represents the voice pathway, which generates the voiced component by passing a harmonic excitation with fundamental frequency \({f}_{0}^{\;t}\) through a voice filter (which is the sum of six formant filters, each specified by formant frequency \({f}_{i}^{\;t}\) and amplitude \({a}_{i}^{t}\) ). The lower part describes the noise pathway, which synthesizes the unvoiced sound by passing white noise through an unvoice filter (consisting of a broadband filter defined by centre frequency \({f}_{\hat{u}}^{\;t}\) , bandwidth \({b}_{\hat{u}}^{t}\) and amplitude \({a}_{\hat{u}}^{t}\) , and the same six formant filters used for the voice filter). The two components are next mixed with voice weight α t and unvoice weight 1 − α t , respectively, and then amplified by loudness L t . A background noise (defined by a stationary spectrogram B ( f )) is finally added to generate the output spectrogram. There are a total of 18 speech parameters at any time t , indicated in purple boxes.
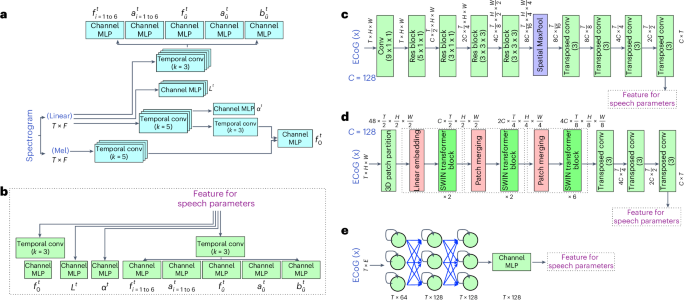
a , The speech encoder architecture. We input a spectrogram into a network of temporal convolution layers and channel MLPs that produce speech parameters. b , c , The ECoG decoder ( c ) using the 3D ResNet architecture. We first use several temporal and spatial convolutional layers with residual connections and spatiotemporal pooling to generate downsampled latent features, and then use corresponding transposed temporal convolutional layers to upsample the features to the original temporal dimension. We then apply temporal convolution layers and channel MLPs to map the features to speech parameters, as shown in b . The non-causal version uses non-causal temporal convolution in each layer, whereas the causal version uses causal convolution. d , The ECoG decoder using the 3D Swin architecture. We use three or four stages of 3D Swin blocks with spatial-temporal attention (three blocks for LD and four blocks for HB) to extract the features from the ECoG signal. We then use the transposed versions of temporal convolution layers as in c to upsample the features. The resulting features are mapped to the speech parameters using the same structure as shown in b . Non-causal versions apply temporal attention to past, present and future tokens, whereas the causal version applies temporal attention only to past and present tokens. e , The ECoG decoder using LSTM layers. We use three LSTM layers and one layer of channel MLP to generate features. We then reuse the prediction layers in b to generate the corresponding speech parameters. The non-causal version employs bidirectional LSTM in each layer, whereas the causal version uses unidirectional LSTM.
Our decoding pipeline showed robust speech decoding across participants, leading to PCC values within the range 0.62–0.92 (Fig. 2a ; causal ResNet mean 0.797, median 0.805) between the decoded and ground-truth speech across several architectures. We attribute our stable training and accurate decoding to the carefully designed components of our pipeline (for example, the speech synthesizer and speech parameter guidance) and the multiple improvements ( Methods sections Speech synthesizer , ECoG decoder and Model training ) over our previous approach on the subset of participants with hybrid-density grids 29 . Previous reports have investigated speech- or text-decoding using linear models 14 , 15 , 30 , transitional probability 4 , 31 , recurrent neural networks 5 , 10 , 17 , 19 , convolutional neural networks 8 , 29 and other hybrid or selection approaches 9 , 16 , 18 , 32 , 33 . Overall, our results are similar to (or better than) many previous reports (54% of our participants showed higher than 0.8 for the decoding PCC; Fig. 3c ). However, a direct comparison is complicated by multiple factors. Previous reports vary in terms of the reported performance metrics, as well as the stimuli decoded (for example, continuous speech versus single words) and the cortical sampling (that is, high versus low density, depth electrodes compared with surface grids). Our publicly available pipeline, which can be used across multiple neural network architectures and tested on various performance metrics, can facilitate the research community to conduct more direct comparisons while still adhering to a high accuracy of speech decoding.
The temporal causality of decoding operations, critical for real-time BCI applications, has not been considered by most previous studies. Many of these non-causal models relied on auditory (and somatosensory) feedback signals. Our analyses show that non-causal models rely on a robust contribution from the superior temporal gyrus (STG), which is mostly eliminated using a causal model (Fig. 4 ). We believe that non-causal models would show limited generalizability to real-time BCI applications due to their over-reliance on feedback signals, which may be absent (if no delay is allowed) or incorrect (if a short latency is allowed during real-time decoding). Some approaches used imagined speech, which avoids feedback during training 16 , or showed generalizability to mimed production lacking auditory feedback 17 , 19 . However, most reports still employ non-causal models, which cannot rule out feedback during training and inference. Indeed, our contribution maps show robust auditory cortex recruitment for the non-causal ResNet and Swin models (Fig. 4 , in contrast to their causal counterparts, which decode based on more frontal regions. Furthermore, the recurrent neural networks that are widely used in the literature 5 , 19 are typically bidirectional, producing non-causal behaviours and longer latencies for prediction during real-time applications. Unidirectional causal results are typically not reported. The recurrent network we tested performed the worst when trained with one direction (Fig. 2a , causal LSTM). Although our current focus was not real-time decoding, we were able to synthesize speech from neural signals with a delay of under 50 ms (Supplementary Table 1 ), which provides minimal auditory delay interference and allows for normal speech production 34 , 35 . Our data suggest that causal convolutional and transformer models can perform on par with their non-causal counterparts and recruit more relevant cortical structures for real-time decoding.
In our study we have leveraged an intermediate speech parameter space together with a novel differentiable speech synthesizer to decode subject-specific naturalistic speech (Fig. 1 . Previous reports used varying approaches to model speech, including an intermediate kinematic space 17 , an acoustically relevant intermediate space using HuBERT features 19 derived from a self-supervised speech masked prediction task 20 , an intermediate random vector (that is, GAN) 11 or direct spectrogram representations 8 , 17 , 36 , 37 . Our choice of speech parameters as the intermediate representation allowed us to decode subject-specific acoustics. Our intermediate acoustic representation led to significantly more accurate speech decoding than directly mapping ECoG to the speech spectrogram 38 , and than mapping ECoG to a random vector, which is then fed to a GAN-based speech synthesizer 11 (Supplementary Fig. 10 ). Unlike the kinematic representation, our acoustic intermediate representation using speech parameters and the associated speech synthesizer enables our decoding pipeline to produce natural-sounding speech that preserves subject-specific characteristics, which would be lost with the kinematic representation.
Our speech synthesizer is motivated by classical vocoder models for speech production (generating speech by passing an excitation source, harmonic or noise, through a filter 39 , 40 and is fully differentiable, facilitating the training of the ECoG decoder using spectral losses through backpropagation. Furthermore, the guidance speech parameters needed for training the ECoG decoder can be obtained using a speech encoder that can be pre-trained without requiring neural data. Thus, it could be trained using older speech recordings or a proxy speaker chosen by the patient in the case of patients without the ability to speak. Training the ECoG decoder using such guidance, however, would require us to revise our current training strategy to overcome the challenge of misalignment between neural signals and speech signals, which is a scope of our future work. Additionally, the low-dimensional acoustic space and pre-trained speech encoder (for generating the guidance) using speech signals only alleviate the limited data challenge in training the ECoG-to-speech decoder and provide a highly interpretable latent space. Finally, our decoding pipeline is generalizable to unseen words (Fig. 2b ). This provides an advantage compared to the pattern-matching approaches 18 that produce subject-specific utterances but with limited generalizability.
Many earlier studies employed high-density electrode coverage over the cortex, providing many distinct neural signals 5 , 10 , 17 , 30 , 37 . One question we directly addressed was whether higher-density coverage improves decoding. Surprisingly, we found a high decoding performance in terms of spectrogram PCC with both low-density and higher (hybrid) density grid coverages (Fig. 3c ). Furthermore, comparing the decoding performance obtained using all electrodes in our hybrid-density participants versus using only the low-density electrodes in the same participants revealed that the decoding did not differ significantly (albeit for one participant; Fig. 3d ). We attribute these results to the ability of our ECoG decoder to extract speech parameters from neural signals as long as there is sufficient perisylvian coverage, even in low-density participants.
A striking result was the robust decoding from right hemisphere cortical structures as well as the clear contribution of the right perisylvian cortex. Our results are consistent with the idea that syllable-level speech information is represented bilaterally 41 . However, our findings suggest that speech information is well-represented in the right hemisphere. Our decoding results could directly lead to speech prostheses for patients who suffer from expressive aphasia or apraxia of speech. Some previous studies have shown limited right-hemisphere decoding of vowels 42 and sentences 43 . However, the results were mostly mixed with left-hemisphere signals. Although our decoding results provide evidence for a robust representation of speech in the right hemisphere, it is important to note that these regions are likely not critical for speech, as evidenced by the few studies that have probed both hemispheres using electrical stimulation mapping 44 , 45 . Furthermore, it is unclear whether the right hemisphere would contain sufficient information for speech decoding if the left hemisphere were damaged. It would be necessary to collect right-hemisphere neural data from left-hemisphere-damaged patients to verify we can still achieve acceptable speech decoding. However, we believe that right-hemisphere decoding is still an exciting avenue as a clinical target for patients who are unable to speak due to left-hemisphere cortical damage.
There are several limitations in our study. First, our decoding pipeline requires speech training data paired with ECoG recordings, which may not exist for paralysed patients. This could be mitigated by using neural recordings during imagined or mimed speech and the corresponding older speech recordings of the patient or speech by a proxy speaker chosen by the patient. As discussed earlier, we would need to revise our training strategy to overcome the temporal misalignment between the neural signal and the speech signal. Second, our ECoG decoder models (3D ResNet and 3D Swin) assume a grid-based electrode sampling, which may not be the case. Future work should develop model architectures that are capable of handling non-grid data, such as strips and depth electrodes (stereo intracranial electroencephalogram (sEEG)). Importantly, such decoders could replace our current grid-based ECoG decoders while still being trained using our overall pipeline. Finally, our focus in this study was on word-level decoding limited to a vocabulary of 50 words, which may not be directly comparable to sentence-level decoding. Specifically, two recent studies have provided robust speech decoding in a few chronic patients implanted with intracranial ECoG 19 or a Utah array 46 that leveraged a large amount of data available in one patient in each study. It is noteworthy that these studies use a range of approaches in constraining their neural predictions. Metzger and colleagues employed a pre-trained large transformer model leveraging directional attention to provide the guidance HuBERT features for their ECoG decoder. In contrast, Willet and colleagues decoded at the level of phonemes and used transition probability models at both phoneme and word levels to constrain decoding. Our study is much more limited in terms of data. However, we were able to achieve good decoding results across a large cohort of patients through the use of a compact acoustic representation (rather than learnt contextual information). We expect that our approach can help improve generalizability for chronically implanted patients.
To summarize, our neural decoding approach, capable of decoding natural-sounding speech from 48 participants, provides the following major contributions. First, our proposed intermediate representation uses explicit speech parameters and a novel differentiable speech synthesizer, which enables interpretable and acoustically accurate speech decoding. Second, we directly consider the causality of the ECoG decoder, providing strong support for causal decoding, which is essential for real-time BCI applications. Third, our promising decoding results using low sampling density and right-hemisphere electrodes shed light on future neural prosthetic devices using low-density grids and in patients with damage to the left hemisphere. Last, but not least, we have made our decoding framework open to the community with documentation ( https://github.com/flinkerlab/neural_speech_decoding ), and we trust that this open platform will help propel the field forward, supporting reproducible science.
Experiments design
We collected neural data from 48 native English-speaking participants (26 female, 22 male) with refractory epilepsy who had ECoG subdural electrode grids implanted at NYU Langone Hospital. Five participants underwent HB sampling, and 43 LD sampling. The ECoG array was implanted on the left hemisphere for 32 participants and on the right for 16. The Institutional Review Board of NYU Grossman School of Medicine approved all experimental procedures. After consulting with the clinical-care provider, a research team member obtained written and oral consent from each participant. Each participant performed five tasks 47 to produce target words in response to auditory or visual stimuli. The tasks were auditory repetition (AR, repeating auditory words), auditory naming (AN, naming a word based on an auditory definition), sentence completion (SC, completing the last word of an auditory sentence), visual reading (VR, reading aloud written words) and picture naming (PN, naming a word based on a colour drawing).
For each task, we used the exact 50 target words with different stimulus modalities (auditory, visual and so on). Each word appeared once in the AN and SC tasks and twice in the others. The five tasks involved 400 trials, with corresponding word production and ECoG recording for each participant. The average duration of the produced speech in each trial was 500 ms.
Data collection and preprocessing
The study recorded ECoG signals from the perisylvian cortex (including STG, inferior frontal gyrus (IFG), pre-central and postcentral gyri) of 48 participants while they performed five speech tasks. A microphone recorded the subjects’ speech and was synchronized to the clinical Neuroworks Quantum Amplifier (Natus Biomedical), which captured ECoG signals. The ECoG array consisted of 64 standard 8 × 8 macro contacts (10-mm spacing) for 43 participants with low-density sampling. For five participants with hybrid-density sampling, the ECoG array also included 64 additional interspersed smaller electrodes (1 mm) between the macro contacts (providing 10-mm centre-to-centre spacing between macro contacts and 5-mm centre-to-centre spacing between micro/macro contacts; PMT Corporation) (Fig. 3b ). This Food and Drug Administration (FDA)-approved array was manufactured for this study. A research team member informed participants that the additional contacts were for research purposes during consent. Clinical care solely determined the placement location across participants (32 left hemispheres; 16 right hemispheres). The decoding models were trained separately for each participant using all trials except ten randomly selected ones from each task, leading to 350 trials for training and 50 for testing. The reported results are for testing data only.
We sampled ECoG signals from each electrode at 2,048 Hz and downsampled them to 512 Hz before processing. Electrodes with artefacts (for example, line noise, poor contact with the cortex, high-amplitude shifts) were rejected. The electrodes with interictal and epileptiform activity were also excluded from the analysis. The mean of a common average reference (across all remaining valid electrodes and time) was subtracted from each individual electrode. After the subtraction, a Hilbert transform extracted the envelope of the high gamma (70–150 Hz) component from the raw signal, which was then downsampled to 125 Hz. A reference signal was obtained by extracting a silent period of 250 ms before each trial’s stimulus period within the training set and averaging the signals over these silent periods. Each electrode’s signal was normalized to the reference mean and variance (that is, z -score). The data-preprocessing pipeline was coded in MATLAB and Python. For participants with noisy speech recordings, we applied spectral gating to remove stationary noise from the speech using an open-source tool 48 . We ruled out the possibility that our neural data suffer from a recently reported acoustic contamination (Supplementary Fig. 5 ) by following published approaches 49 .
To pre-train the auto-encoder, including the speech encoder and speech synthesizer, unlike our previous work in ref. 29 , which completely relied on unsupervised training, we provided supervision for some speech parameters to improve their estimation accuracy further. Specifically, we used the Praat method 50 to estimate the pitch and four formant frequencies ( \({f}_{ {{{\rm{i}}}} = {1}\,{{{\rm{to}}}}\,4}^{t}\) , in hertz) from the speech waveform. The estimated pitch and formant frequency were resampled to 125 Hz, the same as the ECoG signal and spectrogram sampling frequency. The mean square error between these speech parameters generated by the speech encoder and those estimated by the Praat method was used as a supervised reference loss, in addition to the unsupervised spectrogram reconstruction and STOI losses, making the training of the auto-encoder semi-supervised.
Speech synthesizer
Our speech synthesizer was inspired by the traditional speech vocoder, which generates speech by switching between voiced and unvoiced content, each generated by filtering a specific excitation signal. Instead of switching between the two components, we use a soft mix of the two components, making the speech synthesizer differentiable. This enables us to train the ECoG decoder and the speech encoder end-to-end by minimizing the spectrogram reconstruction loss with backpropagation. Our speech synthesizer can generate a spectrogram from a compact set of speech parameters, enabling training of the ECoG decoder with limited data. As shown in Fig. 5 , the synthesizer takes dynamic speech parameters as input and contains two pathways. The voice pathway applies a set of formant filters (each specified by the centre frequency \({f}_{i}^{\;t}\) , bandwidth \({b}_{i}^{t}\) that is dependent on \({f}_{i}^{\;t}\) , and amplitude \({a}_{i}^{t}\) ) to the harmonic excitation (with pitch frequency f 0 ) and generates the voiced component, V t ( f ), for each time step t and frequency f . The noise pathway filters the input white noise with an unvoice filter (consisting of a broadband filter defined by centre frequency \({f}_{\hat{u}}^{\;t}\) , bandwidth \({b}_{\hat{u}}^{t}\) and amplitude \({a}_{\hat{u}}^{t}\) and the same six formant filters used for the voice filter) and produces the unvoiced content, U t ( f ). The synthesizer combines the two components with a voice weight α t ∈ [0, 1] to obtain the combined spectrogram \({\widetilde{S}}^{t}{(\;f\;)}\) as
Factor α t acts as a soft switch for the gradient to flow back through the synthesizer. The final speech spectrogram is given by
where L t is the loudness modulation and B ( f ) the background noise. We describe the various components in more detail in the following.
Formant filters in the voice pathway
We use multiple formant filters in the voice pathway to model formants that represent vowels and nasal information. The formant filters capture the resonance in the vocal tract, which can help recover a speaker’s timbre characteristics and generate natural-sounding speech. We assume the filter for each formant is time-varying and can be derived from a prototype filter G i ( f ), which achieves maximum at a centre frequency \({f}_{i}^{{{\;{\rm{proto}}}}}\) and has a half-power bandwidth \({b}_{i}^{{{{\rm{proto}}}}}\) . The prototype filters have learnable parameters and will be discussed later. The actual formant filter at any time is written as a shifted and scaled version of G i ( f ). Specifically, at time t , given an amplitude \({\left({a}_{i}^{t}\right)}\) , centre frequency \({\left(\;{f}_{i}^{\;t}\right)}\) and bandwidth \({\left({b}_{i}^{t}\right)}\) , the frequency-domain representation of the i th formant filter is
where f max is half of the speech sampling frequency, which in our case is 8,000 Hz.
Rather than letting the bandwidth parameters \({b}_{i}^{t}\) be independent variables, based on the empirically observed relationships between \({b}_{i}^{t}\) and the centre frequencies \({f}_{i}^{\;t}\) , we set
The threshold frequency f θ , slope a and baseline bandwidth b 0 are three parameters that are learned during the auto-encoder training, shared among all six formant filters. This parameterization helps to reduce the number of speech parameters to be estimated at every time sample, making the representation space more compact.
Finally the filter for the voice pathway with N formant filters is given by \({F}_{{{{\rm{v}}}}}^{\;t}{(\;f\;)}={\mathop{\sum }\nolimits_{i = 1}^{N}{F}_{i}^{\;t}(\;f\;)}\) . Previous studies have shown that two formants ( N = 2) are enough for intelligible reconstruction 51 , but we use N = 6 for more accurate synthesis in our experiments.
Unvoice filters
We construct the unvoice filter by adding a single broadband filter \({F}_{\hat{u}}^{\;t}{(\;f\;)}\) to the formant filters for each time step t . The broadband filter \({F}_{\hat{u}}^{\;t}{(\;f\;)}\) has the same form as equation ( 1 ) but has its own learned prototype filter \({G}_{\hat{u}}{(f)}\) . The speech parameters corresponding to the broadband filter include \({\left({\alpha }_{\hat{u}}^{t},\,{f}_{\hat{u}}^{\;t},\,{b}_{\hat{u}}^{t}\right)}\) . We do not impose a relationship between the centre frequency \({f}_{\hat{u}}^{\;t}\) and the bandwidth \({b}_{\hat{u}}^{t}\) . This allows more flexibility in shaping the broadband unvoice filter. However, we constrain \({b}_{\hat{u}}^{t}\) to be larger than 2,000 Hz to capture the wide spectral range of obstruent phonemes. Instead of using only the broadband filter, we also retain the N formant filters in the voice pathway \({F}_{i}^{\;t}\) for the noise pathway. This is based on the observation that humans perceive consonants such as /p/ and /d/ not only by their initial bursts but also by their subsequent formant transitions until the next vowel 52 . We use identical formant filter parameters to encode these transitions. The overall unvoice filter is \({F}_{{{{\rm{u}}}}}^{\;t}{(\;f\;)}={F}_{\hat{u}}^{\;t}(\;f\;)+\mathop{\sum }\nolimits_{i = 1}^{N}{F}_{i}^{\;t}{(\;f\;)}\) .
Voice excitation
We use the voice filter in the voice pathway to modulate the harmonic excitation. Following ref. 53 , we define the harmonic excitation as \({h}^{t}={\mathop{\sum }\nolimits_{k = 1}^{K}{h}_{k}^{t}}\) , where K = 80 is the number of harmonics.
The value of the k th resonance at time step t is \({h}_{k}^{t}={\sin (2\uppi k{\phi }^{t})}\) with \({\phi }^{t}={\mathop{\sum }\nolimits_{\tau = 0}^{t}{f}_{0}^{\;\tau }}\) , where \({f}_{0}^{\;\tau }\) is the fundamental frequency at time τ . The spectrogram of h t forms the harmonic excitation in the frequency domain H t ( f ), and the voice excitation is \({V}^{\;t}{(\;f\;)}={F}_{{{{\rm{v}}}}}^{t}{(\;f\;)}{H}^{\;t}{(\;f\;)}\) .
Noise excitation
The noise pathway models consonant sounds (plosives and fricatives). It is generated by passing a stationary Gaussian white noise excitation through the unvoice filter. We first generate the noise signal n ( t ) in the time domain by sampling from the Gaussian process \({{{\mathcal{N}}}}{(0,\,1)}\) and then obtain its spectrogram N t ( f ). The spectrogram of the unvoiced component is \({U}^{\;t}{(\;f\;)}={F}_{u}^{\;t}{(\;f\;)}{N}^{\;t}{(\;f\;)}\) .
Summary of speech parameters
The synthesizer generates the voiced component at time t by driving a harmonic excitation with pitch frequency \({f}_{0}^{\;t}\) through N formant filters in the voice pathway, each described by two parameters ( \({f}_{ i}^{\;t},\,{a}_{ i}^{t}\) ). The unvoiced component is generated by filtering a white noise through the unvoice filter consisting of an additional broadband filter with three parameters ( \({f}_{\hat{u}}^{\;t},\,{b}_{\hat{u}}^{t},\,{a}_{\hat{u}}^{t}\) ). The two components are mixed based on the voice weight α t and further amplified by the loudness value L t . In total, the synthesizer input includes 18 speech parameters at each time step.
Unlike the differentiable digital signal processing (DDSP) in ref. 53 , we do not directly assign amplitudes to the K harmonics. Instead, the amplitude in our model depends on the formant filters, which has two benefits:
The representation space is more compact. DDSP requires 80 amplitude parameters \({a}_{k}^{t}\) for each of the 80 harmonic components \({f}_{k}^{\;t}\) ( k = 1, 2, …, 80) at each time step. In contrast, our synthesizer only needs a total of 18 parameters.
The representation is more disentangled. For human speech, the vocal tract shape (affecting the formant filters) is largely independent of the vocal cord tension (which determines the pitch). Modelling these two separately leads to a disentangled representation.
In contrast, DDSP specifies the amplitude for each harmonic component directly resulting in entanglement and redundancy between these amplitudes. Furthermore, it remains uncertain whether the amplitudes \({a}_{k}^{t}\) could be effectively controlled and encoded by the brain. In our approach, we explicitly model the formant filters and fundamental frequency, which possess clear physical interpretations and are likely to be directly controlled by the brain. Our representation also enables a more robust and direct estimation of the pitch.
Speaker-specific synthesizer parameters
Prototype filters.
Instead of using a predetermined prototype formant filter shape, for example, a standard Gaussian function, we learn a speaker-dependent prototype filter for each formant to allow more expressive and flexible formant filter shapes. We define the prototype filter G i ( f ) of the i th formant as a piecewise linear function, linearly interpolated from g i [ m ], m = 1, …, M , with the amplitudes of the filter at M being uniformly sampled frequencies in the range [0, f max ]. We constrain g i [ m ] to increase and then decrease monotonically so that G i ( f ) is unimodal and has a single peak value of 1. Given g i [ m ], m = 1, …, M , we can determine the peak frequency \({f}_{i}^{\;{{{\rm{proto}}}}}\) and the half-power bandwidth \({b}_{i}^{{{{\rm{proto}}}}}\) of G i ( f ).
The prototype parameters g i [ m ], m = 1, …, M of each formant filter are time-invariant and are determined during the auto-encoder training. Compared with ref. 29 , we increase M from 20 to 80 to enable more expressive formant filters, essential for synthesizing male speakers’ voices.
We similarly learn a prototype filter for the broadband filter G û ( f ) for the unvoiced component, which is specified by M parameters g û ( m ).
Background noise
The recorded sound typically contains background noise. We assume that the background noise is stationary and has a specific frequency distribution, depending on the speech recording environment. This frequency distribution B ( f ) is described by K parameters, where K is the number of frequency bins ( K = 256 for females and 512 for males). The K parameters are also learned during auto-encoder training. The background noise is added to the mixed speech components to generate the final speech spectrogram.
To summarize, our speech synthesizer has the following learnable parameters: the M = 80 prototype filter parameters for each of the N = 6 formant filters and the broadband filters (totalling M ( N + 1) = 560), the three parameters f θ , a and b 0 relating the centre frequency and bandwidth for the formant filters (totalling 18), and K parameters for the background noise (256 for female and 512 for male). The total number of parameters for female speakers is 834, and that for male speakers is 1,090. Note that these parameters are speaker-dependent but time-independent, and they can be learned together with the speech encoder during the training of the speech-to-speech auto-encoder, using the speaker’s speech only.
Speech encoder
The speech encoder extracts a set of (18) speech parameters at each time point from a given spectrogram, which are then fed to the speech synthesizer to reproduce the spectrogram.
We use a simple network architecture for the speech encoder, with temporal convolutional layers and multilayer perceptron (MLP) across channels at the same time point, as shown in Fig. 6a . We encode pitch \({f}_{0}^{\;t}\) by combining features generated from linear and Mel-scale spectrograms. The other 17 speech parameters are derived by applying temporal convolutional layers and channel MLP to the linear-scale spectrogram. To generate formant filter centre frequencies \({f}_{i = 1\,{{{\rm{to}}}}\,6}^{\;t}\) , broadband unvoice filter frequency \({f}_{\hat{u}}^{\;t}\) and pitch \({f}_{0}^{\;t}\) , we use sigmoid activation at the end of the corresponding channel MLP to map the output to [0, 1], and then de-normalize it to real values by scaling [0, 1] to predefined [ f min , f max ]. The [ f min , f max ] values for each frequency parameter are chosen based on previous studies 54 , 55 , 56 , 57 . Our compact speech parameter space facilitates stable and easy training of our speech encoder. Models were coded using PyTorch version 1.21.1 in Python.
ECoG decoder
In this section we present the design details of three ECoG decoders: the 3D ResNet ECoG decoder, the 3D Swin transformer ECoG decoder and the LSTM ECoG decoder. The models were coded using PyTorch version 1.21.1 in Python.
3D ResNet ECoG decoder
This decoder adopts the ResNet architecture 23 for the feature extraction backbone of the decoder. Figure 6c illustrates the feature extraction part. The model views the ECoG input as 3D tensors with spatiotemporal dimensions. In the first layer, we apply only temporal convolution to the signal from each electrode, because the ECoG signal exhibits more temporal than spatial correlations. In the subsequent parts of the decoder, we have four residual blocks that extract spatiotemporal features using 3D convolution. After downsampling the electrode dimension to 1 × 1 and the temporal dimension to T /16, we use several transposed Conv layers to upsample the features to the original temporal size T . Figure 6b shows how to generate the different speech parameters from the resulting features using different temporal convolution and channel MLP layers. The temporal convolution operation can be causal (that is, using only past and current samples as input) or non-causal (that is, using past, current and future samples), leading to causal and non-causal models.
3D Swin Transformer ECoG decoder
Swin Transformer 24 employs the window and shift window methods to enable self-attention of small patches within each window. This reduces the computational complexity and introduces the inductive bias of locality. Because our ECoG input data have three dimensions, we extend Swin Transformer to three dimensions to enable local self-attention in both temporal and spatial dimensions among 3D patches. The local attention within each window gradually becomes global attention as the model merges neighbouring patches in deeper transformer stages.
Figure 6d illustrates the overall architecture of the proposed 3D Swin Transformer. The input ECoG signal has a size of T × H × W , where T is the number of frames and H × W is the number of electrodes at each frame. We treat each 3D patch of size 2 × 2 × 2 as a token in the 3D Swin Transformer. The 3D patch partitioning layer produces \({\frac{T}{2}\times \frac{H}{2}\times \frac{W}{2}}\) 3D tokens, each with a 48-dimensional feature. A linear embedding layer then projects the features of each token to a higher dimension C (=128).
The 3D Swin Transformer comprises three stages with two, two and six layers, respectively, for LD participants and four stages with two, two, six and two layers for HB participants. It performs 2 × 2 × 2 spatial and temporal downsampling in the patch-merging layer of each stage. The patch-merging layer concatenates the features of each group of 2 × 2 × 2 temporally and spatially adjacent tokens. It applies a linear layer to project the concatenated features to one-quarter of their original dimension after merging. In the 3D Swin Transformer block, we replace the multi-head self-attention (MSA) module in the original Swin Transformer with the 3D shifted window multi-head self-attention module. It adapts the other components to 3D operations as well. A Swin Transformer block consists of a 3D shifted window-based MSA module followed by a feedforward network (FFN), a two-layer MLP. Layer normalization is applied before each MSA module and FFN, and a residual connection is applied after each module.
Consider a stage with T × H × W input tokens. If the 3D window size is P × M × M , we partition the input into \({\lceil \frac{T}{P}\rceil \times \lceil \frac{H}{M}\rceil \times \lceil \frac{W}{M}\rceil}\) non-overlapping 3D windows evenly. We choose P = 16, M = 2. We perform the multi-head self-attention within each 3D window. However, this design lacks connection across adjacent windows, which may limit the representation power of the architecture. Therefore, we extend the shifted 2D window mechanism of the Swin Transformer to shifted 3D windows. In the second layer of the stage, we shift the window by \(\left({\frac{P}{2},\,\frac{M}{2},\,\frac{M}{2}}\right)\) tokens along the temporal, height and width axes from the previous layer. This creates cross-window connections for the self-attention module. This shifted 3D window design enables the interaction of electrodes with longer spatial and temporal distances by connecting neighbouring tokens in non-overlapping 3D windows in the previous layer.
The temporal attention in the self-attention operation can be constrained to be causal (that is, each token only attends to tokens temporally before it) or non-causal (that is, each token can attend to tokens temporally before or after it), leading to the causal and non-causal models, respectively.
LSTM decoder
The decoder uses the LSTM architecture 25 for the feature extraction in Fig. 6e . Each LSTM cell is composed of a set of gates that control the flow of information: the input gate, the forget gate and the output gate. The input gate regulates the entry of new data into the cell state, the forget gate decides what information is discarded from the cell state, and the output gate determines what information is transferred to the next hidden state and can be output from the cell.
In the LSTM architecture, the ECoG input would be processed through these cells sequentially. For each time step T , the LSTM would take the current input x t and the previous hidden state h t − 1 and would produce a new hidden state h t and output y t . This process allows the LSTM to maintain information over time and is particularly useful for tasks such as speech and neural signal processing, where temporal dependencies are critical. Here we use three layers of LSTM and one linear layer to generate features to map to speech parameters. Unlike 3D ResNet and 3D Swin, we keep the temporal dimension unchanged across all layers.
Model training
Training of the speech encoder and speech synthesizer.
As described earlier, we pre-train the speech encoder and the learnable parameters in the speech synthesizer to perform a speech-to-speech auto-encoding task. We use multiple loss terms for the training. The modified multi-scale spectral (MSS) loss is inspired by ref. 53 and is defined as
Here, S t ( f ) denotes the ground-truth spectrogram and \({\widehat{S}}^{t}{(\;f\;)}\) the reconstructed spectrogram in the linear scale, \({S}_{{{{\rm{mel}}}}}^{t}{(\;f\;)}\) and \({\widehat{S}}_{{{{\rm{mel}}}}}^{t}{(\;f\;)}\) are the corresponding spectrograms in the Mel-frequency scale. We sample the frequency range [0, 8,000 Hz] with K = 256 bins for female participants. For male participants, we set K = 512 because they have lower f 0 , and it is better to have a higher resolution in frequency.
To improve the intelligibility of the reconstructed speech, we also introduce the STOI loss by implementing the STOI+ metric 26 , which is a variation of the original STOI metric 8 , 22 . STOI+ 26 discards the normalization and clipping step in STOI and has been shown to perform best among intelligibility evaluation metrics. First, a one-third octave band analysis 22 is performed by grouping Discrete Fourier transform (DFT) bins into 15 one-third octave bands with the lowest centre frequency set equal to 150 Hz and the highest centre frequency equal to ~4.3 kHz. Let \({\hat{x}(k,\,m)}\) denote the k th DFT bin of the m th frame of the ground-truth speech. The norm of the j th one-third octave band, referred to as a time-frequency (TF) unit, is then defined as
where k 1 ( j ) and k 2 ( j ) denote the one-third octave band edges rounded to the nearest DFT bin. The TF representation of the processed speech \({\hat{y}}\) is obtained similarly and denoted by Y j ( m ). We then extract the short-time temporal envelopes in each band and frame, denoted X j , m and Y j , m , where \({X}_{j,\,m}={\left[{X}_{j}{(m-N+1)},\,{X}_{j}{(m-N+2)},\,\ldots ,\,{X}_{j}{(m)}\right]}^{\rm{T}}\) , with N = 30. The STOI+ metric is the average of the PCC d j , m between X j , m and Y j , m , overall j and m (ref. 26 ):
We use the negative of the STOI+ metric as the STOI loss:
where J and M are the total numbers of frequency bins ( J = 15) and frames, respectively. Note that L STOI is differentiable with respect to \({\widehat{S}}^{t}{(\;f\;)}\) , and thus can be used to update the model parameters generating the predicted spectrogram \({\widehat{S}}^{t}{(\;f\;)}\) .
To further improve the accuracy for estimating the pitch \({\widetilde{f}}_{0}^{\;t}\) and formant frequencies \({\widetilde{f}}_{{{{\rm{i}}}} = {1}\,{{{\rm{to}}}}\,4}^{\;t}\) , we add supervisions to them using the formant frequencies extracted by the Praat method 50 . The supervision loss is defined as
where the weights β i are chosen to be β 1 = 0.1, β 2 = 0.06, β 3 = 0.03 and β 4 = 0.02, based on empirical trials. The overall training loss is defined as
where the weighting parameters λ i are empirically optimized to be λ 1 = 1.2 and λ 2 = 0.1 through testing the performances on three hybrid-density participants with different parameter choices.
Training of the ECoG decoder
With the reference speech parameters generated by the speech encoder and the target speech spectrograms as ground truth, the ECoG decoder is trained to match these targets. Let us denote the decoded speech parameters as \({\widetilde{C}}_{j}^{\;t}\) , and their references as \({C}_{j}^{\;t}\) , where j enumerates all speech parameters fed to the speech synthesizer. We define the reference loss as
where weighting parameters λ j are chosen as follows: voice weight λ α = 1.8, loudness λ L = 1.5, pitch \({\lambda }_{{f}_{0}}={0.4}\) , formant frequencies \({\lambda }_{{f}_{1}}={3},\,{\lambda }_{{f}_{2}}={1.8},\,{\lambda }_{{f}_{3}}={1.2},\,{\lambda }_{{f}_{4}}={0.9},\,{\lambda }_{{f}_{5}}={0.6},\,{\lambda }_{{f}_{6}}={0.3}\) , formant amplitudes \({\lambda }_{{a}_{1}}={4},\,{\lambda }_{{a}_{2}}={2.4},\,{\lambda }_{{a}_{3}}={1.2},\,{\lambda }_{{a}_{4}}={0.9},\,{\lambda }_{{a}_{5}}={0.6},\,{\lambda }_{{a}_{6}}={0.3}\) , broadband filter frequency \({\lambda }_{{f}_{\hat{u}}}={10}\) , amplitude \({\lambda }_{{a}_{\hat{u}}}={4}\) , bandwidth \({\lambda }_{{b}_{\hat{u}}}={4}\) . Similar to speech-to-speech auto-encoding, we add supervision loss for pitch and formant frequencies derived by the Praat method and use the MSS and STOI loss to measure the difference between the reconstructed spectrograms and the ground-truth spectrogram. The overall training loss for the ECoG decoder is
where weighting parameters λ i are empirically optimized to be λ 1 = 1.2, λ 2 = 0.1 and λ 3 = 1, through the same parameter search process as described for training the speech encoder.
We use the Adam optimizer 58 with hyper-parameters lr = 10 −3 , β 1 = 0.9 and β 2 = 0.999 to train both the auto-encoder (including the speech encoder and speech synthesizer) and the ECoG decoder. We train a separate set of models for each participant. As mentioned earlier, we randomly selected 50 out of 400 trials per participant as the test data and used the rest for training.
Evaluation metrics
In this Article, we use the PCC between the decoded spectrogram and the actual speech spectrogram to evaluate the objective quality of the decoded speech, similar to refs. 8 , 18 , 59 .
We also use STOI+ 26 , as described in Methods section Training of the ECoG decoder to measure the intelligibility of the decoded speech. The STOI+ value ranges from −1 to 1 and has been reported to have a monotonic relationship with speech intelligibility.
Contribution analysis with the occlusion method
To measure the contribution of the cortex region under each electrode to the decoding performance, we adopted an occlusion-based method that calculates the change in the PCC between the decoded and the ground-truth spectrograms when an electrode signal is occluded (that is, set to zeros), as in ref. 29 . This method enables us to reveal the critical brain regions for speech production. We used the following notations: S t ( f ), the ground-truth spectrogram; \({\hat{{{{{S}}}}}}^{t}{(\;f\;)}\) , the decoded spectrogram with ‘intact’ input (that is, all ECoG signals are used); \({\hat{{{{{S}}}}}}_{i}^{t}{(\;f\;)}\) , the decoded spectrogram with the i th ECoG electrode signal occluded; r ( ⋅ , ⋅ ), correlation coefficient between two signals. The contribution of i th electrode for a particular participant is defined as
where Mean{ ⋅ } denotes averaging across all testing trials of the participant.
We generate the contribution map on the standardized Montreal Neurological Institute (MNI) brain anatomical map by diffusing the contribution of each electrode of each participant (with a corresponding location in the MNI coordinate) into the adjacent area within the same anatomical region using a Gaussian kernel and then averaging the resulting map from all participants. To account for the non-uniform density of the electrodes in different regions and across the participants, we normalize the sum of the diffused contribution from all the electrodes at each brain location by the total number of electrodes in the region across all participants.
We estimate the noise level for the contribution map to assess the significance of our contribution analysis. To derive the noise level, we train a shuffled model for each participant by randomly pairing the mismatched speech segment and ECoG segment in the training set. We derive the average contribution map from the shuffled models for all participants using the same occlusion analysis as described earlier. The resulting contribution map is used as the noise level. Contribution levels below the noise levels at corresponding cortex locations are assigned a value of 0 (white) in Fig. 4 .
Reporting summary
Further information on research design is available in the Nature Portfolio Reporting Summary linked to this Article.
Data availability
The data of one participant who consented to the release of the neural and audio data are publicly available through Mendeley Data at https://data.mendeley.com/datasets/fp4bv9gtwk/2 (ref. 60 ). Although all participants consented to share their data for research purposes, not all participants agreed to share their audio publicly. Given the sensitive nature of audio speech data we will share data with researchers that directly contact the corresponding author and provide documentation that the data will be strictly used for research purposes and will comply with the terms of our study IRB. Source data are provided with this paper.
Code availability
The code is available at https://github.com/flinkerlab/neural_speech_decoding ( https://doi.org/10.5281/zenodo.10719428 ) 61 .
Schultz, T. et al. Biosignal-based spoken communication: a survey. IEEE / ACM Trans. Audio Speech Lang. Process. 25 , 2257–2271 (2017).
Google Scholar
Miller, K. J., Hermes, D. & Staff, N. P. The current state of electrocorticography-based brain-computer interfaces. Neurosurg. Focus 49 , E2 (2020).
Article Google Scholar
Luo, S., Rabbani, Q. & Crone, N. E. Brain-computer interface: applications to speech decoding and synthesis to augment communication. Neurotherapeutics 19 , 263–273 (2022).
Moses, D. A., Leonard, M. K., Makin, J. G. & Chang, E. F. Real-time decoding of question-and-answer speech dialogue using human cortical activity. Nat. Commun. 10 , 3096 (2019).
Moses, D. A. et al. Neuroprosthesis for decoding speech in a paralyzed person with anarthria. N. Engl. J. Med. 385 , 217–227 (2021).
Herff, C. & Schultz, T. Automatic speech recognition from neural signals: a focused review. Front. Neurosci. 10 , 429 (2016).
Rabbani, Q., Milsap, G. & Crone, N. E. The potential for a speech brain-computer interface using chronic electrocorticography. Neurotherapeutics 16 , 144–165 (2019).
Angrick, M. et al. Speech synthesis from ECoG using densely connected 3D convolutional neural networks. J. Neural Eng. 16 , 036019 (2019).
Sun, P., Anumanchipalli, G. K. & Chang, E. F. Brain2Char: a deep architecture for decoding text from brain recordings. J. Neural Eng. 17 , 066015 (2020).
Makin, J. G., Moses, D. A. & Chang, E. F. Machine translation of cortical activity to text with an encoder–decoder framework. Nat. Neurosci. 23 , 575–582 (2020).
Wang, R. et al. Stimulus speech decoding from human cortex with generative adversarial network transfer learning. In Proc. 2020 IEEE 17th International Symposium on Biomedical Imaging ( ISBI ) (ed. Amini, A.) 390–394 (IEEE, 2020).
Zelinka, P., Sigmund, M. & Schimmel, J. Impact of vocal effort variability on automatic speech recognition. Speech Commun. 54 , 732–742 (2012).
Benzeghiba, M. et al. Automatic speech recognition and speech variability: a review. Speech Commun. 49 , 763–786 (2007).
Martin, S. et al. Decoding spectrotemporal features of overt and covert speech from the human cortex. Front. Neuroeng. 7 , 14 (2014).
Herff, C. et al. Towards direct speech synthesis from ECoG: a pilot study. In Proc. 2016 38th Annual International Conference of the IEEE Engineering in Medicine and Biology Society ( EMBC ) (ed. Patton, J.) 1540–1543 (IEEE, 2016).
Angrick, M. et al. Real-time synthesis of imagined speech processes from minimally invasive recordings of neural activity. Commun. Biol 4 , 1055 (2021).
Anumanchipalli, G. K., Chartier, J. & Chang, E. F. Speech synthesis from neural decoding of spoken sentences. Nature 568 , 493–498 (2019).
Herff, C. et al. Generating natural, intelligible speech from brain activity in motor, premotor and inferior frontal cortices. Front. Neurosci. 13 , 1267 (2019).
Metzger, S. L. et al. A high-performance neuroprosthesis for speech decoding and avatar control. Nature 620 , 1037–1046 (2023).
Hsu, W.-N. et al. Hubert: self-supervised speech representation learning by masked prediction of hidden units. IEEE/ACM Trans. Audio Speech Lang. Process. 29 , 3451–3460 (2021).
Griffin, D. & Lim, J. Signal estimation from modified short-time fourier transform. IEEE Trans. Acoustics Speech Signal Process. 32 , 236–243 (1984).
Taal, C. H., Hendriks, R. C., Heusdens, R. & Jensen, J. A short-time objective intelligibility measure for time-frequency weighted noisy speech. In Proc. 2010 IEEE International Conference on Acoustics, Speech and Signal Processing (ed. Douglas, S.) 4214–4217 (IEEE, 2010).
He, K., Zhang, X., Ren, S. & Sun, J. Deep residual learning for image recognition. In Proc. 2016 IEEE Conference on Computer Vision and Pattern Recognition ( CVPR ) (ed. Bajcsy, R.) 770–778 (IEEE, 2016).
Liu, Z. et al. Swin Transformer: hierarchical vision transformer using shifted windows. In Proc. 2021 IEEE / CVF International Conference on Computer Vision ( ICCV ) (ed. Dickinson, S.) 9992–10002 (IEEE, 2021).
Hochreiter, S. & Schmidhuber, J. Long short-term memory. Neural Comput. 9 , 1735–1780 (1997).
Graetzer, S. & Hopkins, C. Intelligibility prediction for speech mixed with white Gaussian noise at low signal-to-noise ratios. J. Acoust. Soc. Am. 149 , 1346–1362 (2021).
Hickok, G. & Poeppel, D. The cortical organization of speech processing. Nat. Rev. Neurosci. 8 , 393–402 (2007).
Trupe, L. A. et al. Chronic apraxia of speech and Broca’s area. Stroke 44 , 740–744 (2013).
Wang, R. et al. Distributed feedforward and feedback cortical processing supports human speech production. Proc. Natl Acad. Sci. USA 120 , e2300255120 (2023).
Mugler, E. M. et al. Differential representation ofÿ articulatory gestures and phonemes in precentral and inferior frontal gyri. J. Neurosci. 38 , 9803–9813 (2018).
Herff, C. et al. Brain-to-text: decoding spoken phrases from phone representations in the brain. Front. Neurosci. 9 , 217 (2015).
Kohler, J. et al. Synthesizing speech from intracranial depth electrodes using an encoder-decoder framework. Neurons Behav. Data Anal. Theory https://doi.org/10.51628/001c.57524 (2022).
Angrick, M. et al. Towards closed-loop speech synthesis from stereotactic EEG: a unit selection approach. In Proc. 2022 IEEE International Conference on Acoustics , Speech and Signal Processing ( ICASSP ) (ed. Li, H.) 1296–1300 (IEEE, 2022).
Ozker, M., Doyle, W., Devinsky, O. & Flinker, A. A cortical network processes auditory error signals during human speech production to maintain fluency. PLoS Biol. 20 , e3001493 (2022).
Stuart, A., Kalinowski, J., Rastatter, M. P. & Lynch, K. Effect of delayed auditory feedback on normal speakers at two speech rates. J. Acoust. Soc. Am. 111 , 2237–2241 (2002).
Verwoert, M. et al. Dataset of speech production in intracranial electroencephalography. Sci. Data 9 , 434 (2022).
Berezutskaya, J. et al. Direct speech reconstruction from sensorimotor brain activity with optimized deep learning models. J. Neural Eng. 20 , 056010 (2023).
Wang, R., Wang, Y. & Flinker, A. Reconstructing speech stimuli from human auditory cortex activity using a WaveNet approach. In Proc. 2018 IEEE Signal Processing in Medicine and Biology Symposium ( SPMB ) (ed. Picone, J.) 1–6 (IEEE, 2018).
Flanagan, J. L. Speech Analysis Synthesis and Perception Vol. 3 (Springer, 2013).
Serra, X. & Smith, J. Spectral modeling synthesis: a sound analysis/synthesis system based on a deterministic plus stochastic decomposition. Comput. Music J. 14 , 12–24 (1990).
Cogan, G. B. et al. Sensory–motor transformations for speech occur bilaterally. Nature 507 , 94–98 (2014).
Ibayashi, K. et al. Decoding speech with integrated hybrid signals recorded from the human ventral motor cortex. Front. Neurosci. 12 , 221 (2018).
Soroush, P. Z. et al. The nested hierarchy of overt, mouthed and imagined speech activity evident in intracranial recordings. NeuroImage 269 , 119913 (2023).
Tate, M. C., Herbet, G., Moritz-Gasser, S., Tate, J. E. & Duffau, H. Probabilistic map of critical functional regions of the human cerebral cortex: Broca’s area revisited. Brain 137 , 2773–2782 (2014).
Long, M. A. et al. Functional segregation of cortical regions underlying speech timing and articulation. Neuron 89 , 1187–1193 (2016).
Willett, F. R. et al. A high-performance speech neuroprosthesis. Nature 620 , 1031–1036 (2023).
Shum, J. et al. Neural correlates of sign language production revealed by electrocorticography. Neurology 95 , e2880–e2889 (2020).
Sainburg, T., Thielk, M. & Gentner, T. Q. Finding, visualizing and quantifying latent structure across diverse animal vocal repertoires. PLoS Comput. Biol. 16 , e1008228 (2020).
Roussel, P. et al. Observation and assessment of acoustic contamination of electrophysiological brain signals during speech production and sound perception. J. Neural Eng. 17 , 056028 (2020).
Boersma, P. & Van Heuven, V. Speak and unSpeak with PRAAT. Glot Int. 5 , 341–347 (2001).
Chang, E. F., Raygor, K. P. & Berger, M. S. Contemporary model of language organization: an overview for neurosurgeons. J. Neurosurgery 122 , 250–261 (2015).
Jiang, J., Chen, M. & Alwan, A. On the perception of voicing in syllable-initial plosives in noise. J. Acoust. Soc. Am. 119 , 1092–1105 (2006).
Engel, J., Hantrakul, L., Gu, C. & Roberts, A. DDSP: differentiable digital signal processing. In Proc. 8th International Conference on Learning Representations https://openreview.net/forum?id=B1x1ma4tDr (Open.Review.net, 2020).
Flanagan, J. L. A difference limen for vowel formant frequency. J. Acoust. Soc. Am. 27 , 613–617 (1955).
Schafer, R. W. & Rabiner, L. R. System for automatic formant analysis of voiced speech. J. Acoust. Soc. Am. 47 , 634–648 (1970).
Fitch, J. L. & Holbrook, A. Modal vocal fundamental frequency of young adults. Arch. Otolaryngol. 92 , 379–382 (1970).
Stevens, S. S. & Volkmann, J. The relation of pitch to frequency: a revised scale. Am. J. Psychol. 53 , 329–353 (1940).
Kingma, D. P. & Ba, J. Adam: a method for stochastic optimization. In Proc. 3rd International Conference on Learning Representations (eds Bengio, Y. & LeCun, Y.) http://arxiv.org/abs/1412.6980 (arXiv, 2015).
Angrick, M. et al. Interpretation of convolutional neural networks for speech spectrogram regression from intracranial recordings. Neurocomputing 342 , 145–151 (2019).
Chen, X. ECoG_HB_02. Mendeley data, V2 (Mendeley, 2024); https://doi.org/10.17632/fp4bv9gtwk.2
Chen, X. & Wang, R. Neural speech decoding 1.0 (Zenodo, 2024); https://doi.org/10.5281/zenodo.10719428
Download references
Acknowledgements
This Work was supported by the National Science Foundation under grants IIS-1912286 and 2309057 (Y.W. and A.F.) and National Institute of Health grants R01NS109367, R01NS115929 and R01DC018805 (A.F.).
Author information
These authors contributed equally: Xupeng Chen, Ran Wang.
These authors jointly supervised this work: Yao Wang, Adeen Flinker.
Authors and Affiliations
Electrical and Computer Engineering Department, New York University, Brooklyn, NY, USA
Xupeng Chen, Ran Wang & Yao Wang
Neurology Department, New York University, Manhattan, NY, USA
Amirhossein Khalilian-Gourtani, Leyao Yu, Patricia Dugan, Daniel Friedman, Orrin Devinsky & Adeen Flinker
Biomedical Engineering Department, New York University, Brooklyn, NY, USA
Leyao Yu, Yao Wang & Adeen Flinker
Neurosurgery Department, New York University, Manhattan, NY, USA
Werner Doyle
You can also search for this author in PubMed Google Scholar
Contributions
Y.W. and A.F. supervised the research. X.C., R.W., Y.W. and A.F. conceived research. X.C., R.W., A.K.-G., L.Y., P.D., D.F., W.D., O.D. and A.F. performed research. X.C., R.W., Y.W. and A.F. contributed new reagents/analytic tools. X.C., R.W., A.K.-G., L.Y. and A.F. analysed data. P.D. and D.F. provided clinical care. W.D. provided neurosurgical clinical care. O.D. assisted with patient care and consent. X.C., Y.W. and A.F. wrote the paper.
Corresponding author
Correspondence to Adeen Flinker .
Ethics declarations
Competing interests.
The authors declare no competing interests.
Peer review
Peer review information.
Nature Machine Intelligence thanks the anonymous reviewer(s) for their contribution to the peer review of this work.
Additional information
Publisher’s note Springer Nature remains neutral with regard to jurisdictional claims in published maps and institutional affiliations.
Supplementary information
Supplementary information.
Supplementary Figs. 1–10, Table 1 and audio files list.
Reporting Summary
Supplementary audio 1.
Example original and decoded audios for eight words.
Supplementary Audio 2
Example original and decoded words from low density participants.
Supplementary Audio 3
Example original and decoded words from hybrid density participants.
Supplementary Audio 4
Example original and decoded words from left hemisphere low density participants.
Supplementary Audio 5
Example original and decoded words from right hemisphere low density participants.
Source Data Fig. 2
Data for Fig, 2a,b,d,e,f.
Source Data Fig. 3
Data for Fig, 3a,c,d.
Rights and permissions
Open Access This article is licensed under a Creative Commons Attribution 4.0 International License, which permits use, sharing, adaptation, distribution and reproduction in any medium or format, as long as you give appropriate credit to the original author(s) and the source, provide a link to the Creative Commons licence, and indicate if changes were made. The images or other third party material in this article are included in the article’s Creative Commons licence, unless indicated otherwise in a credit line to the material. If material is not included in the article’s Creative Commons licence and your intended use is not permitted by statutory regulation or exceeds the permitted use, you will need to obtain permission directly from the copyright holder. To view a copy of this licence, visit http://creativecommons.org/licenses/by/4.0/ .
Reprints and permissions
About this article
Cite this article.
Chen, X., Wang, R., Khalilian-Gourtani, A. et al. A neural speech decoding framework leveraging deep learning and speech synthesis. Nat Mach Intell (2024). https://doi.org/10.1038/s42256-024-00824-8
Download citation
Received : 29 July 2023
Accepted : 08 March 2024
Published : 08 April 2024
DOI : https://doi.org/10.1038/s42256-024-00824-8
Share this article
Anyone you share the following link with will be able to read this content:
Sorry, a shareable link is not currently available for this article.
Provided by the Springer Nature SharedIt content-sharing initiative
Quick links
- Explore articles by subject
- Guide to authors
- Editorial policies
Sign up for the Nature Briefing newsletter — what matters in science, free to your inbox daily.

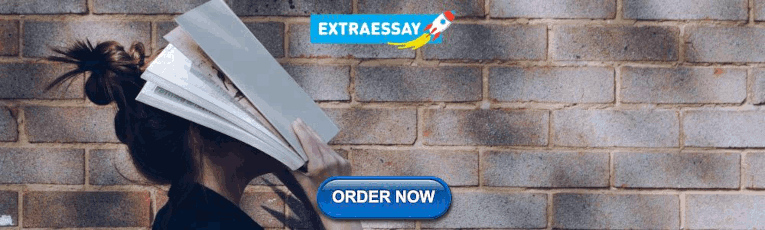
IMAGES
VIDEO
COMMENTS
Journal editors are busy professionals who read hundreds of abstracts annually to screen papers for preliminary consideration. Although some editors contend that "[a] bad abstract won't by itself cause journal editors to reject a scholarly article, but it does incline them toward an initial negative answer," 5 unless it is a slow day in the editorial office, I would anticipate the latter ...
Definition and Purpose of Abstracts An abstract is a short summary of your (published or unpublished) research paper, usually about a paragraph (c. 6-7 sentences, 150-250 words) long. A well-written abstract serves multiple purposes: an abstract lets readers get the gist or essence of your paper or article quickly, in order to decide whether to….
The Journal of Human Resources in Hospitality and Tourism also advises authors against using abbreviations and diagrams in abstracts. Journals published by Elsevier follow instructions provided by an "Author Information Pack" (2017) that also reminds authors that abstracts might be published separately from the article itself, for example ...
An abstract is a self-contained, short, powerful statement that describes a larger body of work. It may be incorporated as part of a published paper, book, grant proposal, thesis, research report, or a conference paper. An abstract of a scientific paper will be published online independently, so it should make sense when it is read alone.
Not all abstracts will contain precisely the same elements. For longer works, you can write your abstract through a process of reverse outlining. For each chapter or section, list keywords and draft one to two sentences that summarize the central point or argument. This will give you a framework of your abstract's structure.
Here comes another important component of an article - the abstract. Research papers in a good journal always carry an abstract because they are so invaluable to the reader to get a "feel" for the contents of the article even without reading it fully. Authors, therefore, have to pay special attention while writing the abstract of an article ...
Write your paper first, then create the abstract as a summary. Check the journal requirements before you write your abstract, eg. required subheadings. Include keywords or phrases to help readers search for your work in indexing databases like PubMed or Google Scholar. Double and triple check your abstract for spelling and grammar errors.
An abstract comprises five parts of equal importance: the title, introduction and aims, methods, results, and conclusion. Allow enough time to write each part well. The title should go straight to the point of the study. Make the study sound interesting so that it catches people's attention. The introduction should include a brief background ...
The researcher collected 795 medical abstracts from a renowned medical journal in Egypt which were published over six years (2013-2018). The corpus data renders 216,842 words in cross-sectionally ...
Developing such a skill takes practice. Here is an exercise to help you develop this skill. Pick a scientific article in your field. Read the paper with the abstract covered. Then try to write an abstract based on your reading. Compare your abstract to the author's. Repeat until you feel confident.
Abstracts published as part of conference proceedings may be searchable in academic and grey literature databases and, even if unpublished, may have relevance for a systematic review. Policy makers and journalists may also be other unintended audiences of an abstract, which can increase the reach, impact, and citation of a journal article.
An abstract is a concise summary of scholarly work and is the first impression for the editor, peer reviewer, or journal audience. When presenting or writing up scholarly activity, an abstract is required for submission and guidelines vary depending on the conference, journal, and grant application.
The research article (RA) abstract is the first section researchers read to determine its relevance to their interests. ... occurring in 24 out of the 120 political science RA abstracts. It should be noted here that the Methods' move preceded the Purpose in six abstracts. ... Informetrics of Scientometrics abstracts: A rhetorical move ...
Introduction. This article deals with drafting a suitable "title" and an appropriate "abstract" for an original research paper. Because the "title" and the "abstract" are the "initial impressions" or the "face" of a research article, they need to be drafted correctly, accurately, carefully, meticulously, and consume time and energy.[1,2,3,4,5,6,7,8,9,10] Often, these ...
An abstract, in the form of a single paragraph, was first published in the Canadian Medical Association Journal in 1960 with the idea that the readers may not have enough time to go through the whole paper, and the first abstract with a defined structure was published in 1991 [].The idea sold and now most original articles and reviews are required to have a structured abstract.
The field of education research has reached unprecedented levels of productivity. The Institute of Education Sciences' ERIC database registers over 25,000 articles published in the past year alone. Collectively, this body of work encompasses a vast number of methodological and disciplinary backgrounds, and each article holds the potential to make a contribution to the theory or practice of ...
This study has investigated the thematic structure of RA abstracts published in business administration, applied linguistics, accounting, physics, chemistry, and computer science disciplines from the perspectives of topical, textual, and interpersonal themes. The results showed that the unmarked topical themes were the most prevalent types in ...
Search across a wide variety of disciplines and sources: articles, theses, books, abstracts and court opinions. Advanced search. Find articles. with all of the words. with the exact phrase. with at least one of ... Return articles published in. e.g., J Biol Chem or Nature. Return articles dated between — e.g., 1996. Saved to My library. Done ...
The corpus. Our data consisted of a corpus of abstracts of articles published in the Reports column of the Science journal. The journal Science was selected for two reasons. First, it is ranked among the world's most high-impact outlets of cutting-edge research, has broad disciplinary coverage (with 43 distinct disciplines represented), and enjoys a wide international authorship and readership.
A total of 100 research article abstracts constituted the cor pus for the st udy, all of which were extracted from articles published across 10 peer-reviewed and indexed journals, an d the ...
Additional inclusion criteria were (1) the article was accompanied by an abstract and (2) the article occupied at least 2 full journal pages. To estimate the sample sizes, we used some preliminary observations 1 that 25% to 50% of articles published in 2 of the journals studied contained 1 or more deficiencies in abstracts. We assumed this rate ...
Context: The section of a research article most likely to be read is the abstract, and therefore it is particularly important that the abstract reflect the article faithfully. Objective: To assess abstracts accompanying research articles published in 6 medical journals with respect to whether data in the abstract could be verified in the article itself.
The present study analysed empirical RA abstracts from TESOL Quarterly, a well-recognised journal in Applied Linguistics, with the view of revealing their rhetorical structure and linguistic ...
In participants with early Parkinson's disease, lixisenatide therapy resulted in less progression of motor disability than placebo at 12 months in a phase 2 trial but was associated with ...
A total of 3260 patients were assigned to receive empagliflozin and 3262 to receive placebo. During a median follow-up of 17.9 months, a first hospitalization for heart failure or death from any ...
Ecology is a leading journal publishing original research and synthesis papers on all aspects of ecology, with particular emphasis on cutting-edge research and new concepts. Abstract Identifying the mechanisms underlying the changes in the distribution of species is critical to accurately predict how species have responded and will respond to ...
This article was published on April 6, 2024, at NEJM.org. A data sharing statement provided by the authors is available with the full text of this article at NEJM.org. Supported by Novo Nordisk .
Here, we combine extensive chemical and sensory analyses of 250 different beers to train machine learning models that allow predicting flavor and consumer appreciation.
Recent research has focused on restoring speech in populations with neurological deficits. Chen, Wang et al. develop a framework for decoding speech from neural signals, which could lead to ...
CA: A Cancer Journal for Clinicians is ACS' flagship clinical oncology journal publishing information about the prevention, early detection, and treatment of cancer. Abstract This article presents global cancer statistics by world region for the year 2022 based on updated estimates from the International Agency for Research on Cancer (IARC).