Advertisement
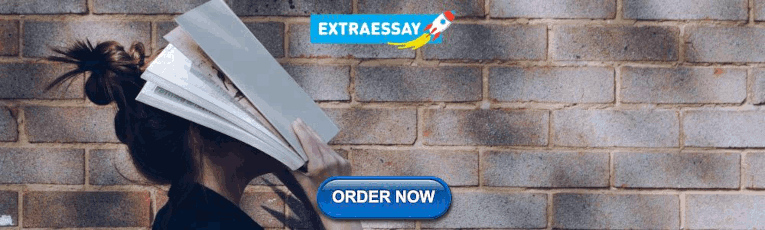
Hate Crime Reporting: The Relationship Between Types of Barriers and Perceived Severity
- Published: 01 June 2021
- Volume 29 , pages 111–126, ( 2023 )
Cite this article
- Matteo Vergani ORCID: orcid.org/0000-0003-0546-4771 1 &
- Carolina Navarro 1
2326 Accesses
7 Citations
7 Altmetric
Explore all metrics
Previous research has identified numerous barriers to reporting hate crimes. However, high variability exists in the outcome measures considered across multiple studies, including whether hate crimes encompass non-criminal behaviours, whether victims’ perceptions are considered bias indicators, and whether the incident is reported to police or to other organisations. These inconsistencies prevent an understanding of whether different barriers relate to different types of hate crimes. This article presents the results of an exploratory empirical study with a convenience sample of members of minorities facing hate crime victimisation in Victoria, Australia (N = 260). Our study participants experienced different types of barriers regarding incidents with different levels of perceived severity. Internalisation and lack of knowledge were more relevant to the underreporting of incidents perceived as less serious—verbal assault. Fear of consequences, lack of trust in statutory agencies, and accessibility were more relevant to the underreporting of incidents perceived as more serious—physical violence and property destruction.
This is a preview of subscription content, log in via an institution to check access.
Access this article
Price includes VAT (Russian Federation)
Instant access to the full article PDF.
Rent this article via DeepDyve
Institutional subscriptions
Data availability
Data is available here: https://dataverse.harvard.edu/dataset.xhtml?persistentId=doi:10.7910/DVN/POIXTC
Anti-Defamation League (ADL) (2016). Information and resources for responding to hate. Available at: https://www.adl.org/media/13637/download
Antjoule, N. (2016). The hate crime report 2016: Homophobia, biphobia and transphobia in UK. Galop. Retrieved from http://www.galop.org.uk/wp-content/uploads/2016/10/The-Hate-Crime-Report-2016.pdf
Australian Bureau of Statistics (ABS) (2016) 2016 Census QuickStats, Retrieved from: https://www.abs.gov.au/websitedbs/D3310114.nsf/Home/2016%20QuickStats
Black, D. (1976). The Behavior of Law . Academic.
Google Scholar
Bjørgo, T., & Ravndal, J. A. (2019). Extreme-right violence and terrorism: Concepts, patterns, and responses. International Centre for Counter-Terrorism.
Bureau of Justice Statistics. (2014). Hate crime victimisation, 2004–2012—Statistical
Carr, P. J., Napolitano, L., & Keating, J. (2007). We never call the cops and here is why: A qualitative examination of legal cynicism in three Philadelphia neighborhoods. Criminology, 45 (2), 445–480.
Article Google Scholar
Chakraborti, N. (2018). Responding to hate crime: Escalating problems, continued failings. Criminology & Criminal Justice, 18 (4), 387–404.
Chakraborti, N., & Hardy, S. J. (2015). LGB&T hate crime reporting: Identifying barriers and solutions. Retrieved from https://www.tandis.odihr.pl/bitstream/20.500.12389/22287/1/08623.pdf
Chakraborti, N., Garland, J., & Hardy, S. J. (2014). The Leicester hate crime project. Findings and Conclusions. The University of Leicester. Retrieved from https://www2.le.ac.uk/departments/criminology/hate/documents/fc-full-report
Chermak, S. M., Freilich, J. D., Parkin, W. S., & Lynch, J. P. (2012). American terrorism and extremist crime data sources and selectivity bias: An investigation focusing on homicide events committed by far-right extremists. Journal of Quantitative Criminology, 28 (1), 191–218.
Clement, S., Brohan, E., Sayce, L., Pool, J., & Thornicroft, G. (2011). Disability hate crime and targeted violence and hostility: A mental health and discrimination perspective. Journal of Mental Health, 20 , 219–225.
Cronin, S. W., McDevitt, J., Farrell, A., & Nolan, J. J., III. (2007). Bias-crime reporting: Organizational responses to ambiguity, uncertainty, and infrequency in eight police departments. American Behavioral Scientist, 51 (2), 213–231.
Cuerden, G. J., & Blakemore, B. (2020). Barriers to reporting hate crime: A Welsh perspective. The Police Journal: Theory, Practice and Principles, 93 (3), 183–201.
Culotta, K. A. (2005). Why victims hate to report: Factors affecting victim reporting in hate crime cases in Chicago. Kriminologija i Socijalna Integracija, 13 , 15.
Fathi, S. (2014). Bias crime reporting: Creating a stronger model for immigrant and refugee populations. Gonzaga University Law Review, 49 (2), 249–262.
Frese, B., Moya, M., & Megías, J. L. (2004). Social perception of rape: How rape myth acceptance modulates the influence of situational factors. Journal of Interpersonal Violence, 19 (2), 143–161.
Freilich, J. D., & Chermak, S. M. (2013). Hate crimes . US Department of Justice, Office of Community Oriented Policing Services.
Goudriaan, H., Lynch, J. P., & Nieuwbeerta, P. (2004). Reporting to the police in western nations: A theoretical analysis of the effects of social context. Justice Quarterly, 21 (4), 933–969.
Hardy, S. J. (2019). Layers of resistance: understanding decision-making processes in relation to crime reporting. International Review of Victimology, 25 (3), 302–319.
Hardy, S., & Chakraborti, N. (2016). Healing the harms: identifying how best to support hate crime victims. Leicester: University of Leicester. Retrieved from https://pdfs.semanticscholar.org/0a27/bdcdadab802c67dae4274122f6db464eecf7.pdf
Hein, L. C., & Scharer, K. M. (2012). Who cares if it is a hate crime? Lesbian, gay, bisexual, and transgender hate crimes—Mental health implications and interventions. Perspectives in Psychiatric Care, 49 (2), 84–93.
Herek, G. M., Cogan, J. C., & Gillis, J. R. (2002). Victim experiences in hate crimes based on sexual orientation. Journal of Social Issues, 58 (2), 319–323.
Home Office, Office for National Statistics and Ministry of Justice. (2013). An Overview of Hate Crime in England and Wales. Retrieved from https://www.gov.uk/government
Hunt, J., Folberg, A., & Ryan, C. (2021). Tolerance of racism: A new construct that predicts failure to recognize and confront racism. European Journal of Social Psychology.
Lantz, B., Gladfelter, A. S., & Ruback, R. B. (2019). Stereotypical hate crimes and criminal justice processing: A multi-dataset comparison of bias crime arrest patterns by offender and victim race. Justice Quarterly, 36 , 193–224.
Leonard, W., Pitts, M., Mitchell, A., & Patel, S. (2008). Coming forward: The underreporting of heterosexist violence and same sex partner abuse in Victoria. Retrieved from: http://arrow.latrobe.edu.au:8080/vital/access/manager/Repository/latrobe:33481
Lockyer, B. (2001). Reporting hate crimes—The California Attorney General’s Civil Rights Commission on Hate Crimes. (Final Report). State of California Department of Justice - Office of the Attorney General. Retrieved from http://oag.ca.gov/sites/all/files/agweb/pdfs/publications/civilrights/reportingHC.pdf .
Lyons, C. J. (2008). Individual perceptions and the social construction of hate crimes: A factorial survey. The Social Science Journal, 45 , 107–131.
Mason, G. (2019). A picture of bias crime in New South Wales. Cosmopolitan Civil Societies: an Interdisciplinary Journal, 11 (1), 47–66.
Mason, G., & Moran, L. (2019). Bias crime policing: “The Graveyard Shift.” International Journal for Crime, Justice and Social Democracy, 8 (2), 1.
Mason, G., Maher, J., McCulloch, J., Pickering, S., Wickes, R., & McKay, C. (2017). Policing hate crime: Understanding communities and prejudice. Taylor & Francis.
Mcbride, M. (2016). A review of the evidence on hate crime and prejudice: Report for the independent advisory group on hate crime, prejudice and community cohesion. The Scottish Centre for Crime & Justice Research, SCCJR. Retrieved from: https://www.sccjr.ac.uk/wp-content/uploads/2016/09/A-Review-of-the-Evidence-on-Hate-Crime-and-Prejudice.pdf
McDevitt, J., Balboni, J. M., Bennett, S., Weiss, J. C., Orchowsky, S., & Walbolt, L. (2012). Improving the quality and accuracy of bias crime statistics nationally: An assessment of the first ten years of bias crime data collection. In S. Orchowsky & L. Walbolt (Eds.), Hate and Bias Crime (pp. 95–108). Routledge.
Myers, W., & Lantz, B. (2020). Reporting racist hate crime victimisation to the police in the United States and the United Kingdom: A cross-national comparison. The British Journal of Criminology, azaa008, https://doi.org/10.1093/bjc/azaa008
Office for Democratic Institutions and Human Rights (ODIHR). (2009). Hate crime laws: A practical guide. Warsaw, Poland: OSCE Office for Democratic Institutions and Human Rights.
Paterson, J., Walters, M., Brown, R., & Fearn, H. (2018). Sussex hate crime project: Final report. University of Sussex. Retrieved from http://www.sussex.ac.uk/psychology/sussexhatecrimeproject/
Peel, E. (1999). I. Violence against lesbians and gay men: Decision-making in reporting and not reporting crime. Feminism & Psychology, 9 (2), 161–167.
Perry, B. (2001). In the name of hate: Understanding hate crimes. Psychology Press.
Perry, J. (2019). Connecting on Hate Crime Data in Europe . CEJI.
Pezzella, F. S., Fetzer, M. D., & Keller, T. (2019). The dark figure of hate crime underreporting. American Behavioural Scientist, 0002764218823844.
Powers, R. A., Khachatryan, N., & Socia, K. M. (2020). Reporting victimization to the police: The role of racial dyad and bias motivation. Policing and Society, 30 , 310–326.
Poynting, S., & Noble, G. (2004). Living with racism: The experience and reporting by Arab and Muslim Australians of discrimination, abuse and violence since 11 September 2001: Report to the Human Rights and Equal Opportunity Commission. Sydney: Centre for Cultural Research, University of Western Sydney. Retrieved from http://citeseerx.ist.psu.edu/viewdoc/download?doi=10.1.1.511.2683&rep=rep1&type=pdf
Raine, H. (2015). Understanding hate crime in North Yorkshire and the City of York. The Office of the Police and Crime Commissioner for North Yorkshire
Richardson, L., Beadle-Brown, J., Bradshaw, J., Guest, C., Malovic, A., & Himmerich, J. (2016). “I felt that I deserved it”—experiences and implications of disability hate crime. Tizard Learning Disability Review, Vol. 21 Iss 2 pp. 80 – 88
Schweppe, J., Haynes, A., & MacIntosh, E. M. (2020). What is measured matters: The value of third party hate crime monitoring. European Journal on Criminal Policy and Research, 26 (1), 39–59.
Schweppe, J. (2021). What is a hate crime? Cogent Social Sciences, 7 (1), 1902643.
Sheppard, K. G., Lawshe, N. L., & McDevitt, J. (2021). Hate crimes in a cross-cultural context. In Oxford Research Encyclopedia of Criminology and Criminal Justice .
Sin, C. H., Mguni, N., Cook, C., Comber, N., & Hedges, A. (2009). Disabled victims of targeted violence, harassment and abuse: barriers to reporting and seeking redress. Safer Communities, 8 (4), 27.
Skogan, W. G. (1984). Reporting crimes to the police: The status of world research. Journal of Research in Crime and Delinquency, 21 (2), 113–137.
Swadling, A., Napoli-Rangel, S., & Imran, M. (2015). Hate crime: Barriers to reporting and best practices. University of York, Centre for Applied Human Rights. Retrieved from: https://www.yorkhumanrights.org/wp-content/uploads/2015/10/Hate-Crime-Report-Final.pdf
Thorneycroft, R., & Asquith, N. L. (2015). The dark figure of disablist violence. The Howard Journal of Criminal Justice, 54 (5), 489–507.
Vergani, M., Navarro, C., Freilich, J., Chermak, S. (2020) Comparing different sources of data to examine trends of hate crime in absence of official registers, «American Journal of Criminal Justice»
Victorian Equal Opportunity and Human Rights Commission (VEOHRC) (2013). Reporting racism: What you say matters. Retrieved from: https://apo.org.au/node/34278
Victorian Equal Opportunity and Human Rights Commission (VEOHRC) (2016). Report racism trial outcomes report. Retrieved from: https://www.humanrightscommission.vic.gov.au/images/files/Report%20Racism%20trial%20-%20outcomes%20report.pdf
Wickes, R. L., Pickering, S., Mason, G., Maher, J. M., & McCulloch, J. (2016). From hate to prejudice: Does the new terminology of prejudice motivated crime change perceptions and reporting actions? British Journal of Criminology, 56 (2), 239–255.
Wiedlitzka, S., Mazerolle, L., Fay-Ramirez, S., & Miles-Johnson, T. (2018). Perceptions of police legitimacy and citizen decisions to report hate crime incidents in Australia. International Journal for Crime, Justice and Social Democracy, 7 (2), 91–106.
Williams, M. L., & Tregidga, J. (2013). All Wales hate crime research project. Race Equality First and Cardiff University. Retrieved from http://orca.cf.ac.uk/60690/13/Time%20for%20Justice-All%20Wales%20Hate%20Crime%20Project.pdf
Wong, K., & Christmann, K. (2008). The role of victim decision-making in reporting of hate crimes. Community Safety Journal, 7 (2), 19.
Wong, K., Christmann, K., Meadows, L., Albertson, K., & Senior, P. (2013). Hate crime in Suffolk: Understanding prevalence and support needs. Sheffield: Sheffield Hallam University. Retrieved from https://e-space.mmu.ac.uk/620465/1/Hate%20Crime%20in%20Suffolk%20-%20PRINT%20VERSION.pdf
Xie, M., & Baumer, E.P. (2019). Crime victims’ decisions to call the police: Past research and new directions. Annual Review of Criminology.
Zaykowski, H. (2010). Racial disparities in hate crime reporting. Violence and Victims, 25 (3), 378–394.
Zaykowski, H., Allain, E. C., & Campagna, L. M. (2019). Examining the paradox of crime reporting: Are disadvantaged victims more likely to report to the police? Law & Society Review, 53 , 1305–1340.
Download references
This work was supported by the Centre for Resilient and Inclusive Societies.
Author information
Authors and affiliations.
Alfred Deakin Institute for Citizenship and Globalisation, Deakin University, Bldg C, Level 1 Melbourne Burwood Campus, 221 Burwood Highway, Burwood, VIC, 3125, Australia
Matteo Vergani & Carolina Navarro
You can also search for this author in PubMed Google Scholar
Corresponding author
Correspondence to Matteo Vergani .
Ethics declarations
Conflict of interest.
The authors declare no competing interests.
Additional information
Publisher's note.
Springer Nature remains neutral with regard to jurisdictional claims in published maps and institutional affiliations.
Rights and permissions
Reprints and permissions
About this article
Vergani, M., Navarro, C. Hate Crime Reporting: The Relationship Between Types of Barriers and Perceived Severity. Eur J Crim Policy Res 29 , 111–126 (2023). https://doi.org/10.1007/s10610-021-09488-1
Download citation
Accepted : 13 May 2021
Published : 01 June 2021
Issue Date : March 2023
DOI : https://doi.org/10.1007/s10610-021-09488-1
Share this article
Anyone you share the following link with will be able to read this content:
Sorry, a shareable link is not currently available for this article.
Provided by the Springer Nature SharedIt content-sharing initiative
- Barriers to reporting
- Find a journal
- Publish with us
- Track your research
- University of Wisconsin–Madison
- University of Wisconsin-Madison
- Research Guides
- College Undergraduate Research Group
- Current Topics: An Undergraduate Research Guide
- Hate Crimes
Current Topics: An Undergraduate Research Guide : Hate Crimes
- Writing, Citing, & Research Help
- Black Lives Matter Movement
- Climate Change
- Fast Fashion
- Health Care
- Sexual Assault/Rape
- Sexual Harassment
- Newspaper Source Plus Newspaper Source Plus includes 1,520 full-text newspapers, providing more than 28 million full-text articles.
- Newspaper Research Guide This guide describes sources for current and historical newspapers available in print, electronically, and on microfilm through the UW-Madison Libraries. These sources are categorized by pages: Current, Historical, Local/Madison, Wisconsin, US, Alternative/Ethnic, and International.
Organizations
- The New York City Gay and Lesbian Anti-Violence Project This project serves lesbian, gay, bisexual, and transgender victims of violence. It also strives to educate the public at large about violence directed at these communities and to influence public policy.
- American Civil Liberties Union Organization committed to defending the civil liberties of Americans.
- International Gay & Lesbian Human Rights Coalition Provides international coverage of political issues related to the human rights of gays and lesbians. Coverage includes hate crimes.
- The Anti-Defamation League The Anti-Defamation League proclaims that it is devoted to combating anti-Semitism and bigotry of all kinds. The site includes op-ed pieces, links to information about hate crimes laws, information about hate crimes incidents against Jews, press releases on extremist groups, and surveys on attitudes about a variety of ethnic groups in the US.
About Hate Crimes
This guide focuses on hate crimes, or crimes committed against others on the basis of characteristics such as race, religion, or sexual orientation.
Try searching these terms using the resources linked on this page: hate crime*, hate crime* AND (law or legislation), hate crime* AND blacks, hate crime* AND sexual orientation, hate crime* AND religio*, hate crime* AND Muslim*, hate crime* AND race, hate crime* AND Jews, hate crime* AND gender, racial violence, religious violence, Matthew Shepard and James Byrd Jr. Hate Crimes Prevention Act
Overview Resources - Background Information
- Civilrights.org: A Social Justice Network Includes an overview and history of the subject, links to resources, and press releases.
- Opposing Viewpoints Resource Center Opposing Viewpoints Resource Center (OVRC) provides viewpoint articles, topic overviews, statistics, primary documents, links to websites, and full-text magazine and newspaper articles related to controversial social issues.
- Tolerance.org Sponsored by the Southern Poverty Law Center, this site provides links to top news stories about hate and hate crimes, guidance for teachers and parents, and information about hate groups throughout the United States.
- Documenting Hate - ProPublica In the absence of reliable national statistics on hate crimes, ProPublica brought together a number of media organizations to systematically track and tell the stories of the commission of hate crimes.
Articles - Scholarly and Popular
- Academic Search Includes scholarly and popular articles on many topics.
- PsycINFO This includes a million citations in the field of psychology and also information about the psychological aspects of related disciplines such as medicine, psychiatry, nursing, sociology, education, pharmacology, physiology, linguistics, anthropology, business and law.
- SocINDEX with Full Text SocINDEX with Full Text includes full text for "core coverage" sociology journals, books, and conference proceedings.
Statistics and Data
- FBI Hate Crime Statistics Tabular Reports of Incidents, Offenses, Victims and Offenders reported by State Agencies, by Bias Motivation, by Offense Category, and by Race. Statistics provided range in date from 1995 to 2007.
- National Criminal Justice Reference Statistics Find hate crime facts & figures, legislation, publications, and related resources. " NCJRS is a federally funded resource offering justice and substance abuse information to support research, policy, and program development worldwide."
- U.S. Department of Education: Campus Security See "Data on Campus Crime" for hate crime statistics in Excel and PDF formats.
- << Previous: Climate Change
- Next: Fast Fashion >>
- Last Updated: Feb 15, 2024 10:29 AM
- URL: https://researchguides.library.wisc.edu/current
Thank you for visiting nature.com. You are using a browser version with limited support for CSS. To obtain the best experience, we recommend you use a more up to date browser (or turn off compatibility mode in Internet Explorer). In the meantime, to ensure continued support, we are displaying the site without styles and JavaScript.
- View all journals
- My Account Login
- Explore content
- About the journal
- Publish with us
- Sign up for alerts
- Open access
- Published: 11 November 2021
Dynamics of online hate and misinformation
- Matteo Cinelli 1 ,
- Andraž Pelicon 2 , 3 ,
- Igor Mozetič 2 ,
- Walter Quattrociocchi 4 ,
- Petra Kralj Novak 2 &
- Fabiana Zollo 1
Scientific Reports volume 11 , Article number: 22083 ( 2021 ) Cite this article
14k Accesses
29 Citations
225 Altmetric
Metrics details
- Computer science
- Information technology
Online debates are often characterised by extreme polarisation and heated discussions among users. The presence of hate speech online is becoming increasingly problematic, making necessary the development of appropriate countermeasures. In this work, we perform hate speech detection on a corpus of more than one million comments on YouTube videos through a machine learning model, trained and fine-tuned on a large set of hand-annotated data. Our analysis shows that there is no evidence of the presence of “pure haters”, meant as active users posting exclusively hateful comments. Moreover, coherently with the echo chamber hypothesis, we find that users skewed towards one of the two categories of video channels (questionable, reliable) are more prone to use inappropriate, violent, or hateful language within their opponents’ community. Interestingly, users loyal to reliable sources use on average a more toxic language than their counterpart. Finally, we find that the overall toxicity of the discussion increases with its length, measured both in terms of the number of comments and time. Our results show that, coherently with Godwin’s law, online debates tend to degenerate towards increasingly toxic exchanges of views.
Similar content being viewed by others
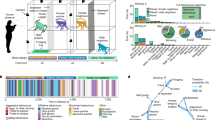
Neural signatures of natural behaviour in socializing macaques
Camille Testard, Sébastien Tremblay, … Michael L. Platt
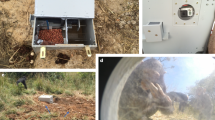
Chimpanzees use social information to acquire a skill they fail to innovate
Edwin J. C. van Leeuwen, Sarah E. DeTroy, … Josep Call
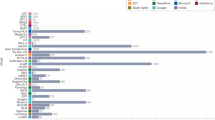
Large language models in medicine
Arun James Thirunavukarasu, Darren Shu Jeng Ting, … Daniel Shu Wei Ting
Introduction
Public debates on social media platforms are often heated and polarised 1 , 2 , 3 . Back in the 90s, Mike Godwin coined a theorem, today known as Godwin’s law, stating that “As an online discussion grows longer, the probability of a comparison involving Nazis or Hitler approaches to one”. More recently, with the advent of social media, an increasing number of people is reporting exposure to online hate speech 4 , leading institutions and online platforms to investigate possible solutions and countermeasures 5 . To prevent and counter the spread of hate speech online, for example, the European Commission agreed with Facebook, Microsoft, Twitter, YouTube, Instagram, Snapchat, Dailymotion, Jeuxvideo.com, and TikTok on a “Code of conduct on countering illegal hate speech online” 6 . In addition to fuelling the toxicity of the online debate, hate speech may have severe offline consequences. Some researchers hypothesised a causal link between online hate and offline violence 7 , 8 , 9 . Furthermore, there is empirical evidence that online hate may induce fear of offline repercussions 10 . However, the detection and contrast of hate speech is complicated. There are still ambiguities in the very definition of hate speech, with academic and relevant stakeholders providing their own interpretations 4 , including social media companies such as Facebook 11 , Twitter 12 , and YouTube 13 .
We use the term “hate speech” to cover whole spectrum of language used in online debates, from normal, acceptable to the extreme, inciting violence. On the extreme end, violent speech covers all forms of expression which spread, incite, promote or justify racial hatred, xenophobia, antisemitism or other forms of hatred based on intolerance, including: intolerance expressed by aggressive nationalism and ethnocentrism, discrimination and hostility against minorities, migrants and people of immigrant origin 14 . Less extreme forms of unacceptable speech include inappropriate (e.g., profanity) and offensive language (e.g., dehumanisation, offensive remarks), which is not illegal, but deteriorates public discourse and can lead to a more radicalised society.
In this work, we analyse a corpus of more than one million comments on Italian YouTube videos related to COVID-19 to unveil the dynamics and trends of online hate. First, we manually annotate a large corpus of YouTube comments for hate speech, and train and fine-tune a hate speech deep learning model to accurately detect it. Then, we apply the model to the entire corpus, aiming to characterise the behaviour of users producing hate, and shed light on the (possible) relationship between the consumption of misinformation and usage of hate and toxic language. The reason for performing hate speech detection on the Italian language is two-fold: First, Italy was one of the countries most affected by the COVID-19 pandemic and especially by the early application of non-pharmaceutical interventions (strict lockdown happened on March 9, 2020). Such an event, by forcing people at home, increased the internet use and was likely to exacerbate the public debate and foment hate speech against specific targets such as the government and politicians. Second, Italian is a less studied language in comparison to English or German 15 and, to the best of our knowledge, this is the first study to investigate hate speech in Italian on YouTube.
This work advances the current literature at different levels. There is a large body of literature about community-level hate speech 16 , 17 , 18 . However, less is known about the behavioural features of users using hate speech on mainstream social media platforms, with few recent exceptions for Twitter 19 , 20 , 21 and Gab 18 . Furthermore, to our knowledge, the relationship between online hate and misinformation is yet to be explored. In this paper, we study hate speech with respect to a controversial and heated topic, i.e., COVID-19, which has been already analysed in terms of sinophobic attitudes 22 . We relax the assumption behind many community-based studies, for which every post produced within an online community hosting haters is hate 17 , 23 . Instead, to cope with a classification task that involves more than one million comments, we annotate a high-quality dataset of more than 70,000 YouTube comments, which is used for training and evaluating a deep learning model. The model is standard in the state-of-the-art and builds on a wide strand of literature using machine learning 24 , 25 , 26 and deep learning 27 , 28 , 29 for automatic hate speech detection via text classification. Moreover, we distinguish YouTube channels into two categories: questionable, i.e., channels likely to disseminate misinformation, and reliable. This categorisation is in line with previous studies on the spreading of misinformation 30 , 31 , 32 , and builds on a list of misinformation sources provided by the Italian Communications Regulatory Authority (AGCOM).
Our results show that hate speech on YouTube is slightly more present than on other social media platforms 20 , 21 , 33 and that there are no significant differences between the proportions of hate speech detected in comments on videos from questionable and reliable channels. We also note that hate speech does not show specific temporal patterns, even on questionable channels. Interestingly, we do not find evidence of “pure haters”, intended as active users posting exclusively hateful comments. Still, we note that users skewed towards one of the two categories of video channels (questionable, reliable) are more prone to use toxic language—i.e., inappropriate, violent, or hateful—within their opponents community. Interestingly, users skewed towards reliable content use on average a more toxic language than their counterpart. Finally, we find that the overall toxicity of the discussion increases with its length measured both in terms of the number of comments and time. In other words, online debates tend to degenerate towards increasingly toxic exchanges of views, in line with Godwin’s law.
Data collection
We collected about 1.3M comments posted by more than 345,000 users on 30,000 videos from 7000 channels on YouTube. According to summary statistics about YouTube by Statista 34 , the number of YouTube users in 2019 in Italy was about 24 millions (roughly one third of the Italian population). By applying 1% empirical law, for which in an Internet community 99% of the participants just visualise content (the so-called lurkers), while only 1% of the users actively participate in the debate (e.g., interacting with content, posting information, commenting), we can evaluate the representativeness of our dataset. Therefore, we can expect that, out of 24 millions users on the platform, a population of 240,000 users usually interact with the content. Taking into account these estimates, the size of our sample (345,000) seems to be appropriate, especially when considering that we are focusing on a specific topic (COVID-19) and not on the whole content of the platform. These considerations are also consistent with another statistic of our dataset, where the videos show an average of 5M daily views (with peaks at 20M).
Using the official YouTube Data API, we performed a keyword search for videos that matched a list of keywords, i.e., { coronavirus, nCov, corona virus, corona-virus, covid, SARS-CoV }. An in-depth search was then performed by crawling the network of related videos as provided by the YouTube algorithm. Then, we filtered the videos that matched our set of keywords in the title or description from the gathered collection. Finally, we collected the comments received by these videos. The title and the description of each video, as well as the comments, are in Italian according to the Google’s cld3 language detection service. The set of videos covers the time window that goes from 01/12/2019 to 21/04/2020, while the set of comments ranges in the time window that goes from 15/01/2020 to 15/06/2020.
We assigned a binary label to each YouTube channel to distinguish between two categories: questionable and reliable. A questionable YouTube channel is a channel producing unverified and false content or directly associated to a news outlet that failed multiple fact checks performed by independent fact checking agencies. The list of YouTube channels labelled as questionable was provided by the Italian Communications Regulatory Authority (AGCOM). The remainder of the channels were labelled as reliable. Table 1 shows a breakdown of the dataset.
Hate speech model
Our aim is to create a state-of-the-art hate speech model, by deep learning methods. We first produce two high-quality manually annotated datasets for training and evaluating the model. The training set is intentionally selected to contain as much hate speech vocabulary as possible, while the evaluation set is unbiased, to assure proper model evaluation. We then apply the model to all the collected data and study the relationship between the hate speech phenomenon and misinformation.
Deep learning models based on Transformer architecture outperform other approaches to automated hate speech detection, as evident from recent shared tasks in the SemEval-2019 evaluation campaign: HatEval 28 and OffensEval 35 , as well as OffensEval 2020 29 . The central reference for hate speech detection for Italian is the report on the EVALITA 2018 hate speech detection task 36 . Furthermore, in 37 authors modelled the hate speech task using the Italian pre-trained language model AlBERTo, achieving state-of-the-art results on Facebook and Twitter datasets. We trained a new hate speech detection model for Italian following the state-of-the-art approach 37 on our four-class hate speech detection task (see sections “ Data selection and annotation ” and “ Classification ” for detailed information).
Data selection and annotation
The comments to be annotated were sampled from the Italian YouTube comments on videos about the COVID-19 pandemic in the period from January 2020 to May 2020. Two sets were annotated: a hate-speech-rich training set with 59,870 comments and an unbiased evaluation set with 10,536 comments.
To get a training set that is rich with hate speech, we annotated all the comments with a (basic) hate speech classifier (machine learning model) that assigns a score between -3 (hateful) and +3 (normal). The basic classifier was trained on a publicly available dataset of Italian hate speech against immigrants 38 . Even though this basic model is not very accurate, its performance is better than random and we used its result for selecting the training data to be annotated and later used for training our deep learning model. For a realistic evaluation scenario, threads (i.e., all the comments to the video) were kept intact during the annotation procedure, yet individual comments were annotated.
The threads (with comments) to be annotated for the training set were selected according to the following criteria: thread length (the number of comments in a thread between 10 and 500), and hatefulness (at least 5% of hateful comments according to our basic classifier). The application of these criteria resulted in 1168 threads (VideoIds) and 59,870 comments. The evaluation set was selected from May 2020 data as a random (unbiased) sample of 151 threads (VideosIds) with 10,543 comments.
Our hate speech annotation schema is adapted from OLID 39 and FRENK 40 . We differentiate between the following speech types:
Acceptable (non hate speech);
Inappropriate (the comment contains terms that are obscene or vulgar, but the text is not directed to any person or group specifically);
Offensive (the comment includes offensive generalisation, contempt, dehumanisation, or indirect offensive remarks);
Violent (the comment’s author threatens, indulges, desires or calls for (physical) violence against a target; it also includes calling for, denying or glorifying war crimes and crimes against humanity).
The data was split among eight contracted annotators. Each comment was annotated twice by two different annotators. The splitting procedure was optimised to get approximately equal overlap (in the number of comments) between each pair of annotators for each dataset. The annotators were given clear annotation guidelines, a training session and a test on a small set to evaluate their understanding of the task and their commitment before starting the annotation procedure. Furthermore, the annotation progress was closely monitored in terms of the annotator agreement to ensure high data quality.
The annotation results for the training and evaluation sets are summarised in Fig. 1 . The annotator agreement in terms of Krippendorff’s \(Alpha\) 41 and accuracy (i.e., percentage of agreement) on both the training and the evaluation sets is presented in Table 2 . The agreement results indicate that the annotation task is difficult and ambiguous, as the annotators agree on the label in only about 80% of the cases. Since the class distribution is very unbalanced, accuracy is not the most appropriate measure of agreement. \(Alpha\) is a better measure of agreement as it accounts for the agreement by chance. Our agreement scores in terms of \(Alpha\) are comparable to those of other high-quality datasets, like 21 , 42 .
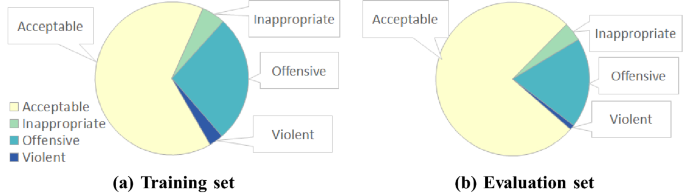
The distribution of the four hate speech labels in the manually annotated training ( a ) and evaluation ( b ) sets. The training set is intentionally biased to contain more hate speech while the evaluation set is unbiased.
Classification
A state-of-the-art neural model based on Transformer language models was trained to distinguish between the four hate speech classes. We use a language model based on the BERT architecture 43 which consists of 12 stacked Transformer blocks with 12 attention heads each. We attach a linear layer with a softmax activation function at the output of these layers to serve as the classification layer. As input to the classifier, we take the representation of the special [CLS] token from the last layer of the language model. The whole model is jointly trained on the downstream task of four-class hate speech detection. We used AlBERTo 44 , a BERT-based language model pre-trained on a collection of tweets in the Italian language. According to previous work 43 , fine-tuning of the neural models was performed end-to-end. We used the Adam optimizer with the learning rate of \(2e-5\) and learning rate warmup over the first 10% of the training instances. We used weight decay set to 0.01 for regularization. The model was trained for 3 epochs with batch size 32. We performed the training of the models using the HuggingFace Transformers library 45 .
The tuning of our models was performed by cross validation on the training set, while the final evaluation was performed on the separate out-of-sample evaluation set. In our setup, each data instance (YouTube comment) is labelled twice, possibly with inconsistent labels. To avoid data leakage between training and testing splits in cross validation, we use 8-fold cross validation where in each fold we use all the comments annotated by one annotator as a test set. We report the performance of the trained models using the same measures as are used for the annotator agreement: Krippendorff’s Alpha-reliability ( \(Alpha\) ) 41 , accuracy ( \(Acc\) ), and the \(F_{1}\) score for individual classes, on both the training and the evaluation datasets. The validation results are reported in Table 3 . The coincidence matrices for the evaluation set, used to compute all the scores of the annotator agreements and the model performance, are reported in Table S8 of SI.
The performance of our model is comparable to the annotator agreement in terms of Krippendorff’s \(Alpha\) and accuracy ( \(Acc\) ), providing evidence for its high quality. The model achieves the annotator agreement both on the training set in the cross validation setting, as well as on the evaluation set. This shows the ability of the model to generalise well on the yet unseen, out-of-sample evaluation data. We observe similar results in terms of \(F_{1}\) scores for individual classes. The only noticeable drop in performance compared to the annotators is the performance on the minority (Violent) class. We attribute this drop to the very low amount of data available for the Violent class compared to the other classes, however, the performance is still reasonable. We therefore apply our hate speech detection model to the set of 1.3M comments and report the findings.
Results and discussion
Relationship between hate speech and misinformation.
We start our analysis examining the distribution of the different speech types on both reliable and questionable YouTube channels. Figure 2 shows the cumulative distribution of comments, total and per type, by channel. The x-axis shows the YouTube channels ranked by their total number of comments, while the y-axis shows the total number of comments in the dataset (both quantities are reported as proportions). We observe that the distribution of comments is Pareto-like; indeed, the first 10% of channels (dotted vertical line) covers about 90% of the total number of comments. Such a 10 to 90 percent relationship is even stronger when comments are analysed according to their types; indeed, the heterogeneity of the distribution decreases going from violent to acceptable comments. It is also worth noting that, as indicated by the secondary y-axis of Fig. 2 , the first 10% of channels with most comments also contain about 50% of all the questionable channels in our list, thus indicating a relatively high popularity of these channels. In addition, questionable channels are about 0.25% of the total number of channels that received at least one comment and, despite being such a minority, they cover \(\sim\) 8% of the total number of comments (with the following partitioning: 8% acceptable; 7% inappropriate; 9% offensive; 9% violent) and the 1.3% of the total number of videos, thus highlighting a disproportion between their activity and popularity.
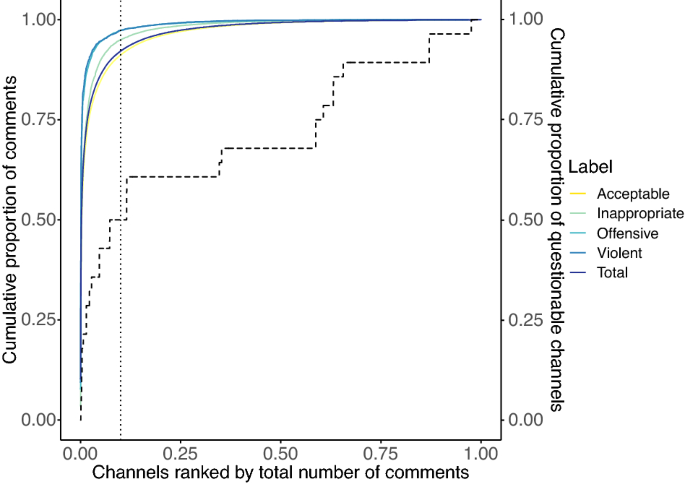
Ranking of YouTube channels by number of comments and proportions of comment types per channel.
Figure 3 shows the proportion of comments by label and channel types, and their trend over time. In panel (a) we display the overall proportion of comment types, noting that the majority of comments is acceptable, followed by offensive, inappropriate, and violent types, all relatively stable over time (see panel (b)). It is worth remarking that, despite the proportion of hate speech found in the dataset is consistent with—although slightly higher than—previous studies 20 , 33 , the presence of even a limited number of hateful comments is in direct conflict with the platform’s policy against hate speech. Moreover, we do not observe relevant differences between questionable (panel (c)) and reliable (panel (d)) channels, providing a first piece of evidence in favour of a moderate (if not absent) relationship between online hate and misinformation.
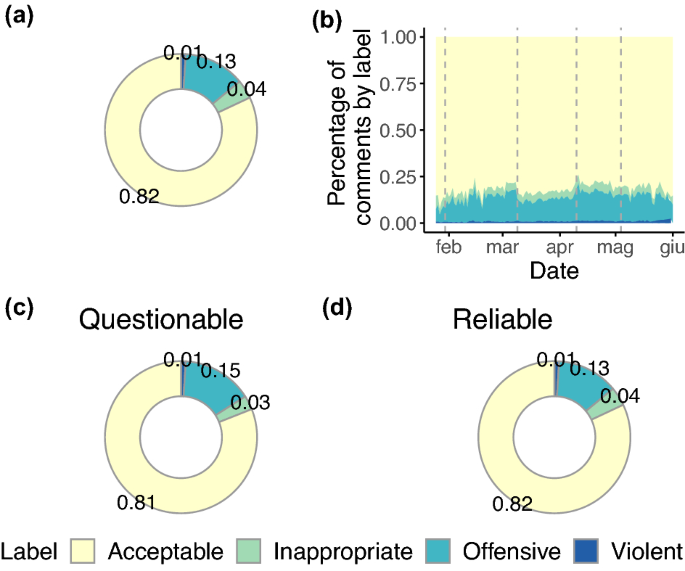
Proportion of the four hate speech labels in the whole dataset ( a ) over time ( b ), and for questionable ( c ) and reliable ( d ) YouTube channels. Panel b displays four dashed lines in correspondence of events of paramount relevance for the year 2020 in Italy. The first line is placed on 30/01/2020 when the first two cases of COVID-19 were detected in Italy. The second line is placed on 09/03/2020 when the Prime Minister enforced the first lockdown to the whole nation. The third line is placed on 10/04/2020 when the Prime Minister communicated to the nation an extension of the lockdown until May the 3rd. The fourth line is placed on 04/05/2020 when the “phase 2” (i.e., the suspension of the full lockdown) began. Interestingly, we note a higher share of Acceptable comments between the second and third lines, that is during the lockdown, perhaps due to positive messages and encouragement among people. Instead, as a possible consequence of the extension of the lockdown, we note a lower share of Acceptable comments right after the third line.
Now we aim at understanding whether hateful comments display a typical (technically, the average) time of appearance. This kind of information can indeed be crucial for the implementation of timely moderation efforts. More specifically, our goal is to discover whether 1) different speech types have typical delays and 2) any difference holds between comments on videos disseminated by questionable and reliable channels. To this aim, we define the comment delay as the time elapsed between the posting time of the video and that of the comment (in hours). Figure 4 displays the comment delays for the four types of hate speech and for questionable and reliable channels. Looking at panel (a) of Fig. 4 , we first note that all comments share approximately the same delay regardless of their type. Indeed, the distributions of the comment delay are roughly log-normal with a long average delay ranging from 120 h in the case of acceptable comments to 128 h in the case of violent comments (the comment delay is reduced by \(\sim 75\%\) when removing observations in the right tail of the distribution as shown in Table S1 of SI). For what concerns comments on videos published by questionable and reliable channels, we do not find strong differences between typical delays of speech types within the two domains. In the case of questionable channels, we find that comment delays range from 66 to 42 h, while for reliable channels they range from 125 to 136 h (as reported in SI ). To summarise, we find a discrepancy in users’ responsiveness to the two types of content, with comments on questionable videos having a much lower typical delay than those on reliable videos. In addition, comments typical delays differ between reliable and questionable channels. In particular, on questionable channels toxic comments appear first and faster than acceptable ones, following decreasing levels of toxicity (violent \(\rightarrow\) offensive \(\rightarrow\) inappropriate). In other words, violent comments on questionable content display the shortest typical delay, followed by offensive, inappropriate, and acceptable comments. Conversely, on reliable channels the shortest typical delay is observed for appropriate comments, followed by violent, unacceptable, and offensive comments (for details refer to SI ).
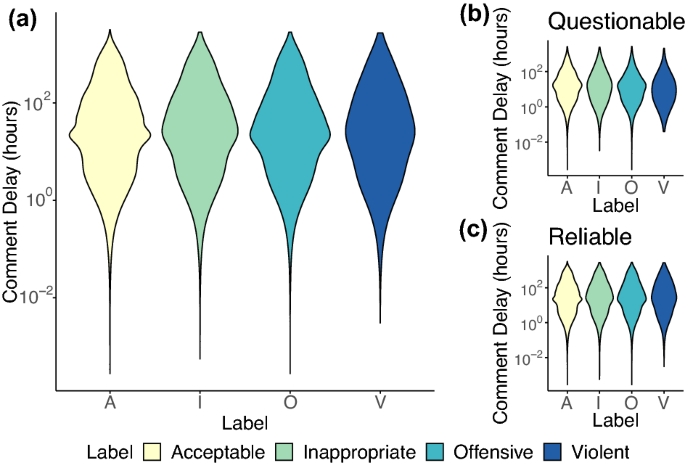
Distribution of comment delays in the whole dataset ( a ) and for questionable ( b ) and reliable ( c ) YouTube channels. The capital letters on the x-axis represent the different types of comments: acceptable (A); inappropriate (I); offensive (O); violent (V).
Users’ behaviour and misinformation
In line with other social media platforms 30 , 46 , users activity on YouTube follows a heavy tailed distribution, i.e., the majority of users post few comments, while a small minority is hyperactive (see Fig. S1 of SI for details). Now we want to investigate whether a systematic tendency towards offences and hate can be observed for some (category of) users. In Fig. 5 , each vertex of the square represents one of the four speech types (acceptable—A; inappropriate—I; offensive—O; violent—V). Each dot is a user whose position in the square depends on the fraction of his/her comments for each category. As an example, a user posting only acceptable comments will be located exactly on the vertex A (i.e., in (0,0)), while a user that splits his/her activity evenly between acceptable and inappropriate comments will be located in the middle of the edge connecting the vertices A and I. Similarly, a user posting only violent comments will be located exactly on the vertex V (i.e., in (1,0)). More formally, to shrink the 4-dimensional space deriving by the four labels that fully characterise the activity of each user, we associate a user j the following coordinates in a 2-dimensional space:
where \(a_j\) , \(i_j\) , \(o_j\) , \(v_j\) are the proportions, respectively, of acceptable, inappropriate, offensive, and violent comments posted by user j over his/her total activity \(c_j\) .
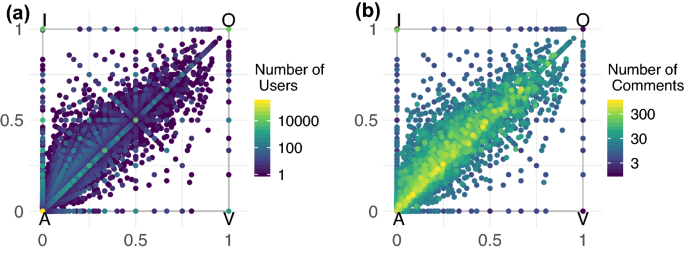
Users balance between different comment types. In panel ( a ) brighter dots indicate a higher density of users while in panel ( b ) brighter dots indicate a higher average activity of the users in terms of number of comments. We note that users focused on posting comments labelled as offensive and violent are almost absent in the data.
Although most of the users leave only or mostly acceptable comments, there are also several users ranging across categories (i.e., located away from the vertices of the square in Fig. 5 ). Interestingly, there is no evidence of “pure haters”, i.e., active users exclusively using hateful language, that are only 0.3% of the total number of users. Indeed, while there are users posting only or mostly violent comments (see Fig. 5 a), their overall activity is very low and below five comments (see Fig. 5 b). A similar situation is observed for offenders, i.e., active users posting only offending comments. Although we cannot exclude that moderation efforts put in place by YouTube (if any) might partially impact these results, the absence of pure haters and offenders highlights that hate speech is rarely only an issue of specific categories of users. Rather, it seems that regular users are occasionally triggered by external factors. To rule out possible confounding factors (note that users located in the centre of the square could display a balanced activity between different pairs of comment categories) we repeated the analysis excluding the category I (i.e., inappropriate). The results are provided in SI and confirm what we observe in Fig. 5 .
We now aim at unveiling the relationship between users behaviour in terms of commenting patterns and their activity with respect to questionable and reliable channels. Since misinformation is often associated with the diffusion of polarising content which plays on one’s fear and could fuel anger, frustration and hate 47 , 48 , 49 , our intent is to understand whether users more loyal to questionable content are also more prone to use a toxic language in their comments. Thus, we define the leaning l of a user j as the fraction of his/her activity spent in commenting videos posted by questionable channels, i.e.,
where \(\sum _{i = 1}^{c_j}q_j\) is the number of comments on videos from questionable channels posted by the user j and \(c_j\) is the activity of user j . Similarly, for each user j we compute the fraction of unacceptable comments \({\overline{a}}\) as:
where \(a_j\) is the fraction of acceptable comments posted by user j .
In Fig. 6 a, we compare users’ leaning \(l_j\) against the fraction of unacceptable comments \({\overline{a}}_j\) . As expected, we may observe two peaks (of different magnitude) in correspondence of extreme values of leaning ( \(l_j \sim 0\) and \(l_j \sim 1\) ), represented by the brighter squares in the plot. In addition, the joint distribution becomes sparser in correspondence of higher values of users’ leaning and fraction of unacceptable comments ( \(l_j \ge 0.5\) and \({\overline{a}}_j \ge 0.5\) ), indicating that a relevant share of users are placed at the two extremes of the distribution (thus being somewhat polarised) and that users producing mostly unacceptable comments are way less present.
In Fig. 6 b, we display the proportion of unacceptable comments posted by users displaying leaning at the two tails of the distribution (i.e., users displaying a remarkable tendency to comment questionable videos \(l_j \in [0.75,1)\) and users with a remarkable tendency to comment reliable videos \(l_j \in (0,0.25]\) ). We find that users skewed towards reliable channels post, on average, a higher proportion of unacceptable comments ( \(\sim 23\%\) ) than users skewed towards questionable channels ( \(\sim 17\%\) ). In other words, users who tend to comment on reliable videos are also more prone to use a unacceptable/toxic language. Further statistics on the two distributions are reported in SI .
Panel (c) of Fig. 6 provides a comparison between the distributions of unacceptable comments posted by users skewed towards questionable channels ( q in the legend) on videos published by either questionable or reliable channels. Panel (d) of Fig. 6 provides a similar representation for users skewed towards reliable channels ( r in the legend). We may note a strong difference in users behaviour: quite unimodal when they comment videos on the same side of the leaning; bimodal when they comment videos on the opposite side of leaning. Therefore, users tend to avoid using a toxic language when they comment videos in accordance with their leaning and to separate into roughly two classes (non-toxic, toxic) when they comment videos in contrast with their preferences. This finding resonates with evidence of online polarisation and with the presence of peculiar characters of the internet such as trolls and social justice warriors.
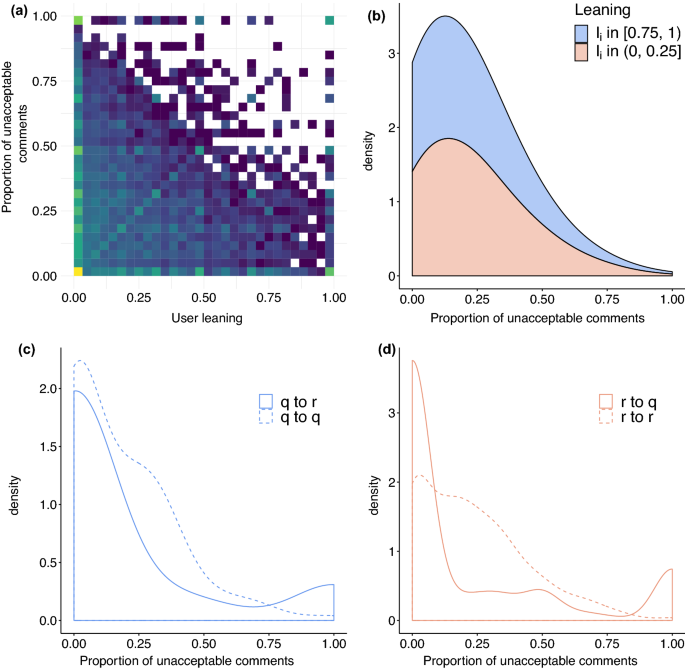
Panel ( a ) displays the relationship occurring between the preference of users for questionable and reliable channels (the user leaning \(l_j\) ) and the fraction of unacceptable comments posted by the user ( \({\overline{a}}_j\) ) as a joint distribution. Panel ( b ) displays the distribution of unacceptable comments for users displaying a remarkable tendency to comment under videos posted by questionable ( \(l_j \in [0.75,1)\) ) and reliable ( \(l_j \in (0,0.25]\) ) channels. Panel ( c ) displays the distribution of unacceptable comments posted by users with leaning towards questionable channels ( \(l_j \in [0.75,1)\) indicated as q) under videos of questionable channels (dashed line indicated as q to q in the legend) and under videos of reliable channels (solid line indicated as q to r in the legend). Panel ( d ) displays the distribution of unacceptable comments posted by users with leaning towards reliable channels ( \(l_j \in (0,0.25]\) indicated as r) under videos of questionable channels (solid line indicated as r to q in the legend) and under videos of reliable channels (dashed line indicated as r to r in the legend).
Toxicity level of online debates
Finally, we aim at investigating whether online debates degenerate (i.e., increase their average toxicity) when the discussion gets longer, both in terms of number of comments and time. More in general, we are interested in analysing how commenting dynamics change over time and whether online hate follows similar dynamics to those observed for users’ sentiment 31 . Indeed, although violent comments and pure haters are quite rare, their presence could negatively impact the tone of the general debate. Furthermore, we want to understand whether the toxicity of comments tends to follow certain dynamics empirically observed on the internet such as Godwin’s law. To this purpose, we test whether toxic comments tend to appear more frequently at later stages of the debate.
To compute the toxicity level of a debate around a certain video, we assign each speech type (A,I,O,V) a toxicity value t as follows:
Acceptable: t = 0
Inappropriate: t = 1
Offensive: t = 2
Violent: t = 3
Then, we define the toxicity level T of a discussion d of n comments as the average of the toxicity values over all the comments of the discussion:
To understand how the toxicity level changes with respect to the number of comments and to comment delay (i.e., the time elapsed between the posting time of the video and that of the comment), we employ linear regression models. Figure 7 shows that a positive relationship between the two variables (i.e., average toxicity is an increasing function of the number of comments and comment delay) exists, and that such a relationship cannot be reproduced by linear models obtained with randomised comment labels (regression outcomes and a validation of our results using proportions of unacceptable comments are reported in SI ). We apply a similar approach to distinguish between comments on videos from questionable and reliable channels (as shown in SI ). Overall, similarly to the general case, we find stronger positive effects in real data than in randomised models although such effects are significant only in the case of comments under videos posted by reliable channels.
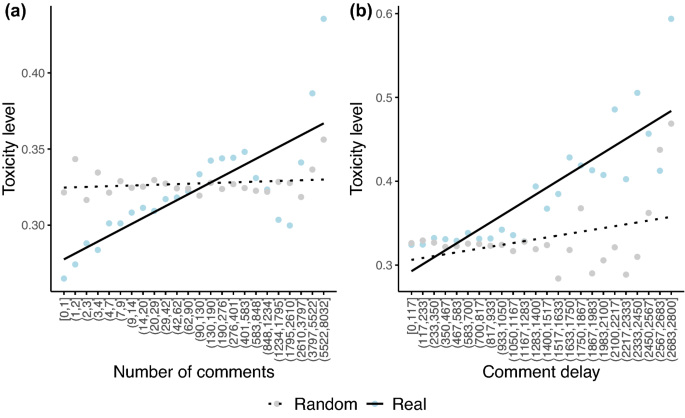
Linear regression models for number of comments and comment delay. On the x-axis of panel ( a ) the comments are grouped in logarithmic bins while on the x-axis of panel ( b ) the comment delays are grouped in linear bins.
Finally, to evaluate the effect (in the short run) of violent comments, we study the transition between subsequent comments in threads appearing under YouTube videos. The choice of analysing threads instead of full lists of comments resides in the fact that YouTube comments are ranked according to several factors (among which the number of likes received by the comment, the length of the thread, the importance of the user who posted the comment). Therefore, given a certain video, we cannot be sure of what comments (and in which order) the user actually visualises. However, threads do not suffer from this issue, since comments in threads are presented in chronological order. The aim of studying the transitions between comment types is to find specific transition patterns (probabilities) between toxic comments and understand if the conversation tends to evolve in a way that is different with respect to random models. As an example, a thread with four comments 1 Acceptable, 2 Offensive and 1 Violent (in this order) can be summarised with the string “AOOV”, which entails three transitions between comment types, namely {AO; OO; OV}. By extending such a process to all threads in our dataset, we can compute the transition probability from one comment type to another using a 4 by 4 transition matrix. In this way, we can evaluate the possible presence of an escalation effect due to the fact that toxic comments could be immediately followed by increasingly toxic ones. The results are reported in Fig. 8 , in which we notice that certain transition probabilities cannot be reproduced by a null model in which the sequences of comments within threads are randomised. In particular, we note that, differently from the empirical data, in randomised instances the transition probability from one violent comment to another is 0 and the probability of passing from violent comments to unacceptable ones (inappropriate, offensive and violent) is always higher in the empirical case than at random. Similar results hold for offensive and inappropriate comments, but not for acceptable ones. This finding confirms the presence of a short term influence of violent comments that could flame the debate and scale up into streams of toxicity.
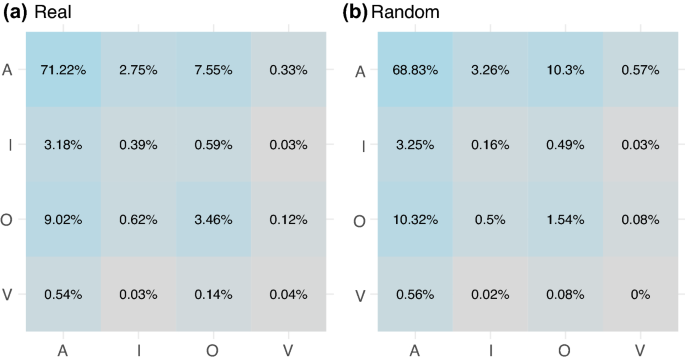
Transition probabilities between different comments types represented by a \(4 \times 4\) transition matrix in the real (panel a ) and in the random case (panel b ). Brighter entries of the matrix indicate higher transition probabilities.
Conclusions
The aim of this work is two-fold: i) to investigate the behavioural dynamics of online hate speech and ii) to shed light on the possible relationship with misinformation exposure and consumption. We apply a hate speech deep learning model to a large corpus of more than one millions comments on Italian YouTube videos. Our analysis provides a series of important results which can support the development of appropriate solutions to prevent and counter the spread of hate speech online. First , there is no evidence of a strict relationship between the usage of a toxic language (including hate speech) and being involved within the misinformation community on YouTube. Second , we do not observe the presence of “pure” haters, instead it seems that the phenomenon of hate speech involves regular users who are occasionally triggered to use toxic language. Third , users polarisation and hate speech seem to be intertwined, indeed users are more prone to use inappropriate, violent, or hateful language within their opponents community (i.e., out of their echo chamber). Finally , we find a positive correlation between the overall toxicity of the discussion and its length, measured both in terms of number of comments and time.
Our results are in line with recent studies about (the increasing) polarisation of online debates and segregation of users 50 . Furthermore, they somewhat confirm the intuition behind some empirically grounded laws such as Godwin’s law which can be interpreted, by extension, as a statement regarding the increasing toxicity of online debates. A potential limitation of this work is represented by the relentless effort of YouTube in moderating hate on the platform. This could have prevented us from having complete information about the actual presence of hate speech in public discussions. In spite of this limitation, after collecting again the whole set of comments after at least 1 year from their posting time, we find that only 32% of violent comments were actually unavailable due to either moderation or removal by the author (see Table S9 of SI). Another issue could be the presence of channels wrongly labelled as reliable instead of questionable (i.e., false negatives) or the fact that certain questionable sources available on YouTube are not included in the list, especially due to the high variety of content available on the platform and the relative ease with which one can open a new channel. Nonetheless, our findings are robust with respect to these aspects (as we show in a dedicated section of SI ). Future efforts should extend our work to other languages beyond Italian, social media platforms, and topics. For instance, studying hate speech on online political discourse over time could provide important insights on debated phenomena such as affective polarisation 51 . Moreover, further research on possible triggers in the language and content of videos is desirable.
Data availibility
The datasets generated during the current study for the purposes of training and evaluating the hate speech model are available at the CLARIN repository: http://hdl.handle.net/11356/1450 . The hate speech model is available at the HuggingFace repository: https://huggingface.co/IMSyPP/hate_speech_it .
Adamic, L. A., Glance, N. The political blogosphere and the 2004 us election: Divided they blog. In Proceedings of the 3rd International Workshop on Link Discovery , pp. 36–43 (2005).
Flaxman, S., Goel, S. & Rao, J. M. Filter bubbles, echo chambers, and online news consumption. Public Opin. Q. 80 (S1), 298–320 (2016).
Article Google Scholar
Coe, K., Kenski, K. & Rains, S. A. Online and uncivil? Patterns and determinants of incivility in newspaper website comments. J. Commun. 64 (4), 658–679 (2014).
Siegel, A. A. Online hate speech. Social Media and Democracy , p. 56 (2019).
Gagliardone, I., Gal, D., Alves, T. & Martinez, G. Countering Online Hate Speech (Unesco Publishing, 2015).
European Commission. Code of conduct on countering illegal hate speech online. https://ec.europa.eu/newsroom/just/document.cfm?doc_id=42985 (Accessed: 27.09.2021).
Calvert, C. Hate speech and its harms: A communication theory perspective. J. Commun. 47 (1), 4–19 (1997).
Chan, J., Ghose, A. & Seamans, R. The internet and racial hate crime: Offline spillovers from online access. MIS Q. 40 (2), 381–403 (2016).
Müller, K. & Schwarz, C. Fanning the flames of hate: Social media and hate crime. J. Eur. Econ. Assoc. (2018).
Awan, I. & Zempi, I. We fear for our lives: Offline and online experiences of anti-muslim hostility. Technical report, Birmingham City University (2015).
Facebook. Community standards. https://www.facebook.com/communitystandards/introduction (Accessed: 27.09.2021).
Twitter. Violent organizations policy. https://help.twitter.com/en/rules-and-policies/violent-groups (Accessed: 27.09.2021).
YouTube. Hate speech policy. https://support.google.com/youtube/answer/2801939?hl=en&ref_topic=9282436 (Accessed: 27.09.2021).
Council of Europe. Recommendation no. r (97) 20 of the committee of ministers to member states on “hate speech”. https://go.coe.int/URzjs (Accessed: 27.09.2021).
Fortuna, P. & Nunes, S. A survey on automatic detection of hate speech in text. ACM Comput. Surv. (CSUR) 51 (4), 1–30 (2018).
Kumar, S., Hamilton, W. L., Leskovec, J. & Jurafsky, D. Community interaction and conflict on the web. In Proceedings of the 2018 World Wide Web Conference , pp. 933–943 (2018).
Johnson, N. F. et al. Hidden resilience and adaptive dynamics of the global online hate ecology. Nature 573 (7773), 261–265 (2019).
Article ADS CAS Google Scholar
Mathew, B. et al. Hate begets hate: A temporal study of hate speech. Proc. ACM Hum. Comput. Interact. 4 (CSCW2), 1–24 (2020).
Ribeiro, M., Calais, P., Santos, Y., Almeida, V. & Meira Jr., W. Characterizing and detecting hateful users on twitter. In Proceedings of the International AAAI Conference on Web and Social Media , vol. 12 (2018).
Siegel, A. A. et al. Trumping hate on twitter? Online hate speech in the 2016 us election campaign and its aftermath. Q. J. Polit. Sci. 16 (1), 71–104 (2021).
Evkoski, B., Pelicon, A., Mozetič, I., Ljubešić, N. & Novak, P. K. Retweet communities reveal the main sources of hate speech. arXiv:2105.14898 (2021).
Schild, L., Ling, C., Blackburn, J., Stringhini, G., Zhang, Y. & Zannettou, S. “Go eat a bat, chang!”: An early look on the emergence of sinophobic behavior on web communities in the face of covid-19. arXiv:2004.04046 (2020).
Chandrasekharan, E., Samory, M., Srinivasan, A. & Gilbert, E. The bag of communities: Identifying abusive behavior online with preexisting internet data. In Proceedings of the 2017 CHI Conference on Human Factors in Computing Systems , pp. 3175–3187 (2017).
Burnap, P. & Williams, M. L. Us and them: Identifying cyber hate on twitter across multiple protected characteristics.. EPJ Data Sci. 5 , 1–15 (2016).
Del Vigna, F., Cimino, A., Dell’Orletta, F., Petrocchi, M. & Tesconi, M. Hate me, hate me not: Hate speech detection on facebook. In Proceedings of the First Italian Conference on Cybersecurity (ITASEC17) , pp. 86–95 (2017).
Davidson, T., Warmsley, D., Macy, M. & Weber, I. Automated hate speech detection and the problem of offensive language. In Proceedings of the International AAAI Conference on Web and Social Media , vol. 11 (2017).
Badjatiya, P., Gupta, S., Gupta, M. & Varma, V. Deep learning for hate speech detection in tweets. In Proceedings of the 26th International Conference on World Wide Web Companion , pp. 759–760 (2017).
Basile, V., Bosco, C., Fersini, E., Debora, N., Patti, V., Pardo, F. M. R., Rosso, P. & Sanguinetti, M. et al. Semeval-2019 task 5: Multilingual detection of hate speech against immigrants and women in twitter. In 13th International Workshop on Semantic Evaluation , pp. 54–63 (Association for Computational Linguistics, 2019).
Zampieri, M., Nakov, P., Rosenthal, S., Atanasova, P., Karadzhov, G., Mubarak, H., Derczynski, L., Pitenis, Z. & Çöltekin, Ç. Semeval-2020 task 12: Multilingual offensive language identification in social media (offenseval 2020). arXiv:2006.07235 (2020).
Cinelli, M. et al. The covid-19 social media infodemic. Sci. Rep. 10 (1), 1–10 (2020).
Zollo, F. et al. Emotional dynamics in the age of misinformation. PLoS One 10 (09), 1–22 (2015).
Zollo, F. et al. Debunking in a world of tribes. PLoS One 12 (7), e0181821 (2017).
Gagliardone, I., Pohjonen, M., Beyene, Z., Zerai, A., Aynekulu, G., Bekalu, M., Bright, J., Moges, M., Seifu, M. & Stremlau, N. et al. Mechachal: Online debates and elections in Ethiopia—from hate speech to engagement in social media. Available at SSRN 2831369 (2016).
Statista Research Department. Leading social media networks in Italy as of January 2019, ranked by number of active users. https://www.statista.com/statistics/639777/social-media-active-users-italy/ (Accessed: 27.09.2021).
Zampieri, M., Malmasi, S., Nakov, P., Rosenthal, S., Farra, N. & Kumar, R. SemEval-2019 task 6: Identifying and categorizing offensive language in social media (OffensEval). In Proceedings of the 13th International Workshop on Semantic Evaluation , pp. 75–86 (Association for Computational Linguistics, 2019).
Bosco, C., Dell’Orletta, F., Poletto, F., Sanguinetti, M. & Maurizio, T. Overview of the evalita 2018 hate speech detection task. In EVALITA 2018-Sixth Evaluation Campaign of Natural Language Processing and Speech Tools for Italian , vol. 2263, pp. 1–9 (CEUR, 2018).
Polignano, M., Basile, P., De Gemmis, M. & Semeraro, G. Hate speech detection through AlBERTo Italian language understanding model. In NL4AI@ AI* IA (2019).
Sanguinetti, M., Poletto, F., Bosco, C., Patti, V. & Stranisci, M. An Italian Twitter corpus of hate speech against immigrants. In Proceedings of the Eleventh International Conference on Language Resources and Evaluation (LREC 2018) (2018).
Zampieri, M., Malmasi, S., Nakov, P., Rosenthal, S., Farra, N. & Kumar, R. Predicting the type and target of offensive posts in social media. In Proceedings of NAACL (2019).
Ljubešić, N., Fišer, D. & Erjavec, T. The FRENK datasets of socially unacceptable discourse in Slovene and English (2019).
Krippendorff, K. Content Analysis. An Introduction to its Methodology , 4th edn. (Sage Publications, 2018).
Mozetič, I., Grčar, M. & Smailović, J. Multilingual Twitter sentiment classification: The role of human annotators. PLoS One 11 (5), e0155036 (2016).
Devlin, J., Chang, M.-W., Lee, K., Toutanova, K. BERT: Pre-training of deep bidirectional transformers for language understanding. arXiv:1810.04805 (2018).
Polignano, M., Basile, P., De Gemmis, M., Semeraro, G. & Basile, V. AlBERTo: Italian BERT language understanding model for NLP challenging tasks based on tweets. In 6th Italian Conference on Computational Linguistics, CLiC-it 2019 , vol. 2481, pp. 1–6 (CEUR, 2019).
Wolf, T., Debut, L., Sanh, V., Chaumond, J., Delangue, C., Moi, A., Cistac, P., Rault, T., Louf, R., Funtowicz, M., Davison, J., Shleifer, S., von Platen, P., Ma, C., Jernite, Y., Plu, J., Xu, C., Le Scao, T., Gugger, S., Drame, M., Lhoest, Q. & Rush, A. M. Hugging face’s transformers: State-of-the-art natural language processing. arXiv:abs/1910.03771 (2019).
Del Vicario, M. et al. The spreading of misinformation online. Proc. Natl. Acad. Sci. 113 (3), 554–559 (2016).
Article ADS Google Scholar
Del Vicario, M., Quattrociocchi, W., Scala, A. & Zollo, F. Polarization and fake news: Early warning of potential misinformation targets. ACM Trans. Web (TWEB) 13 (2), 1–22 (2019).
Osmundsen, M., Bor, A., Vahlstrup, P. B., Bechmann, A. & Petersen, M. B. Partisan polarization is the primary psychological motivation behind political fake news sharing on twitter. Am. Polit. Sci. Rev. , 1–17 (2020).
Guess, A., Nagler, J., & Tucker, J. Less than you think: Prevalence and predictors of fake news dissemination on facebook. Sc. Adv. 5 (1), eaau4586 (2019).
Cinelli, M., De Francisci Morales, G., Galeazzi, A., Quattrociocchi, W., Starnini, M. The echo chamber effect on social media. Proc. Natl. Acad. Sci. 118 (9) (2021).
Druckman, J. N., Klar, S., Krupnikov, Y., Levendusky, M. & Ryan, J. B. Affective polarization, local contexts and public opinion in America. Nat. Hum. Behav. 5 (1), 28–38 (2021).
Download references
Acknowledgements
The authors acknowledge financial support from the Slovenian Research Agency (research core funding no. P2-103), and the European Union’s Rights, Equality and Citizenship Programme under Grant Agreement no. 875263. The authors wish to thank Arnaldo Santoro for his support with the categorisation of misinformation sources.
Author information
Authors and affiliations.
Ca’ Foscari University of Venice, Venice, Italy
Matteo Cinelli & Fabiana Zollo
Jozef Stefan Institute, Ljubljana, Slovenia
Andraž Pelicon, Igor Mozetič & Petra Kralj Novak
Jozef Stefan International Postgraduate School, Ljubljana, Slovenia
Andraž Pelicon
Sapienza University of Rome, Rome, Italy
Walter Quattrociocchi
You can also search for this author in PubMed Google Scholar
Contributions
M.C. and F.Z. designed the experiment and supervised the data annotation task; A.P., I.M., and P.K.N. developed the classification model and prepared Fig. 1 . M.C. performed the analysis and prepared Figs. 2 , 3 , 4 , 5 , 6 and 7 . All authors contributed to the interpretation of the results and wrote the manuscript.
Corresponding author
Correspondence to Fabiana Zollo .
Ethics declarations
Competing interests.
The authors declare no competing interests.
Additional information
Publisher's note.
Springer Nature remains neutral with regard to jurisdictional claims in published maps and institutional affiliations.
Supplementary Information
Supplementary table s1., rights and permissions.
Open Access This article is licensed under a Creative Commons Attribution 4.0 International License, which permits use, sharing, adaptation, distribution and reproduction in any medium or format, as long as you give appropriate credit to the original author(s) and the source, provide a link to the Creative Commons licence, and indicate if changes were made. The images or other third party material in this article are included in the article's Creative Commons licence, unless indicated otherwise in a credit line to the material. If material is not included in the article's Creative Commons licence and your intended use is not permitted by statutory regulation or exceeds the permitted use, you will need to obtain permission directly from the copyright holder. To view a copy of this licence, visit http://creativecommons.org/licenses/by/4.0/ .
Reprints and permissions
About this article
Cite this article.
Cinelli, M., Pelicon, A., Mozetič, I. et al. Dynamics of online hate and misinformation. Sci Rep 11 , 22083 (2021). https://doi.org/10.1038/s41598-021-01487-w
Download citation
Received : 28 June 2021
Accepted : 22 October 2021
Published : 11 November 2021
DOI : https://doi.org/10.1038/s41598-021-01487-w
Share this article
Anyone you share the following link with will be able to read this content:
Sorry, a shareable link is not currently available for this article.
Provided by the Springer Nature SharedIt content-sharing initiative
By submitting a comment you agree to abide by our Terms and Community Guidelines . If you find something abusive or that does not comply with our terms or guidelines please flag it as inappropriate.
Quick links
- Explore articles by subject
- Guide to authors
- Editorial policies
Sign up for the Nature Briefing newsletter — what matters in science, free to your inbox daily.

- Follow us on Facebook
- Follow us on Twitter
- Criminal Justice
- Environment
- Politics & Government
- Race & Gender
Expert Commentary
Hate crimes and research questions: Examining racial, ethnic and religious bias
2015 review of research and data that speak to issues of hate crimes motivated by bias, with a focus on definitional issues in the United States and patterns abroad.
Republish this article

This work is licensed under a Creative Commons Attribution-NoDerivatives 4.0 International License .
by Joanna Penn, The Journalist's Resource June 18, 2015
This <a target="_blank" href="https://journalistsresource.org/politics-and-government/hate-crimes-ongoing-research-racial-ethnic-religious/">article</a> first appeared on <a target="_blank" href="https://journalistsresource.org">The Journalist's Resource</a> and is republished here under a Creative Commons license.<img src="https://journalistsresource.org/wp-content/uploads/2020/11/cropped-jr-favicon-150x150.png" style="width:1em;height:1em;margin-left:10px;">
The mass shooting at Emanuel African Methodist Episcopal Church in Charleston, S.C., again raises questions about the frequency and extent of hate crimes in America.
The FBI notes in a December 2014 report : “ Of the reported 3,407 single-bias hate crime offenses that were racially motivated, 66.4 percent were motivated by anti-black or African-American bias, and 21.4 percent stemmed from anti-white bias.” It remains the case that African-Americans are the victims of bias-motivated crimes at much higher rates than all other racial or ethnic groups, and as frequently noted , the official numbers likely represent a significant under-count.
While the 2015 Charleston incident appears to be plainly hate-motivated, many are not as clear-cut. The February 2015 shooting of three Muslim college students at Chapel Hill, N.C., raised a number of difficult questions: Should the killings be labeled as a hate crime ? Was the relative lack of media coverage due to the victims’ religion? These discussions have widened to the question of why hate crimes are so hard to prove and rarely prosecuted . This seems to be reflected in updated statistics from the Bureau of Justice Statistics (BJS): It estimated that 293,800 nonfatal violent and property hate crimes occurred in the U.S. in 2012, with an estimated 60% not reported to the police.
While the new figures are not statistically different from the BJS estimates for 2004 (of 281,700 hate crimes) the details show significant changes in the motivations behind the crimes. The proportion motivated by ethnicity bias more than doubled from 22% in 2004 to 51% in 2012; 28% were motivated by religious bias, nearly triple the 10% rate in 2004; and those motivated by gender bias went from 12% to 26%, more than double. (The percentages add up to more than 100% because some crimes may have multiple biases.)
Reporting and definitional issues
One of the most complex areas of debate is how to define hate crimes precisely and uniformly and ensure consistent enforcement and categorization. A 2015 study published in the American Journal of Criminal Justice , “The Effect of Law on Hate Crime Reporting: The Case of Racial and Ethnic Violence,” examines this question, analyzing how evolving definitions of hate crimes across states may or may not affect the rates at which such acts are designated and reported publicly by law enforcement. The researcher, Michele Stacey of East Carolina University, looked at Federal Bureau of Investigation statistics from 2000 to 2007, as well as demographic, economic and political data that could help contextualize rates of reporting.
The FBI categorizes and publicizes these criminal acts through its Uniform Crime Reports (UCR) Hate Crime Statistics Program . For the purposes of statistical collection, Congress has defined a hate crime as a “criminal offense against a person or property motivated in whole or in part by an offender’s bias against a race, religion, disability, ethnic origin or sexual orientation.” U.S. states must determine that crimes meet that definition when they report them to the FBI, but they have different laws on their books — indeed, Wyoming, Arkansas, Georgia, Indiana and South Carolina do not have clear statutes recognizing bias-motivated crime, as do the other 45 states — and vary in their approach to enforcement and categorization. Ultimately, the study focuses on how the demographics of states and the size of minority populations appear to influence the number of hate crimes reported.
The study’s findings include:
- The data suggest overall that that there is a “relationship between the type of hate crime law on the books and the reporting of hate crime by the police.” Where laws are broader — making the designation of hate crimes less strict — there are generally more hate crimes reported.
- Demographic factors, including the size and relative political power of minority groups, seem to influence the reporting of hate crimes: “While states that criminalize violations of a person’s civil rights report more anti-black incidents and states with broader hate-crime definitions report more anti-Hispanic hate crime, that relationship is attenuated by the presence of a larger minority group and greater minority political power.”
- Somewhat surprisingly, “the minority’s ability to mobilize politically combined with a large group size results in fewer hate crimes.” Indeed, “more hate crimes are reported generally where minority-group size is small in relation to white population size.”
- After the factors of “racial composition and economic and political competition” were taken into account, the breadth of state hate-crime statutes had little effect on the number of anti-black hate crimes. This suggests that there are important differences between how laws influence police actions around racial (anti-black) and ethnic hate crimes (anti-Hispanic).
“The reported level of hate crime is likely a factor of both the true number of crimes committed as well as the reporting behaviors of victims and the police,” Stacey concludes. “Future research should endeavor to disentangle the reporting practices of jurisdiction from the true prevalence of hate crime to determine whether this is the case.”
Terrorism and international backlash
The issues of prejudice and violence against Muslim minorities are of particular concern in Europe in the wake of high-profile terrorist acts perpetrated by Islamic extremists.
A 2014 study in the Journal of Contemporary Criminal Justice , “Hate Crime in the Wake of Terror Attacks: Evidence from 7/7 and 9/11,” investigates whether terrorist incidents can be statistically linked to increases in hate crimes in the United Kingdom. After the 2001 and 2005 attacks in New York and London, respectively, there were anecdotal reports of spikes in hate crimes against perceived Muslim populations, but little statistical evidence. The study’s authors, Emma Hanes and Stephen Machin of University College London, use data from four police-force areas in England with substantial Asian and Arab populations to measure hate crimes against those groups before and after the terror attacks, relative to those against blacks and whites.
The findings include:
- In the month of the July 7, 2005, attacks in London, there was a 27% increase in hate crimes against Asians and Arabs. While the effects fell in later months, the number of attacks remained substantially higher than before the bombings — 17% higher in the three months after the attacks and 10% to 15% higher a year after the event.
- The immediate impact on hate crime was greater in London, where the attacks occurred, with a 32% increase. This is compared to the other police-force areas in the study — Leicestershire, the West Midlands and West Yorkshire.
- The subsequent rate of decrease in the attacks’ effects also differed by area, with no impact in London a year after the attacks, but a persistent and strong effect in the areas outside London. Two possible explanations were suggested: First, long-standing race issues in the study areas outside London “have engendered deeper-seated issues of anger and resentment.” Second, the authors point to greater levels of migration in London, leading to a more dynamic population environment in which adjustment can take place more quickly.
- Analysis for the effects after the 9/11 attacks is more limited as changes in the reporting requirements meant that trends could only be measured until April 2002, and data limitations excluded one police-force area from the analysis. Nevertheless, the study finds strong effects from 9/11 on hate crimes against Asians and Arabs, with levels rising by 28% in September 2001, reducing to 22% three months after the attacks and 11% after six months.
The authors conclude that the similar patterns in hate crimes against Asian and Arab populations after 7/7 and 9/11 makes it highly likely that they can be causally linked to the terror attacks themselves. This is in line with previous academic literature that suggests that for individuals, the costs of such terror attacks are not limited to the victims of the attacks themselves. Hanes and Machin point out that this makes the causes of hate crime “different from, or certainly more complex than, the kind of incentive or deterrence effects that emerge as crime determinants in the standard economics of the crime model.”
Related research: A 2014 study published in the Proceedings of the National Academy of Sciences (PNAS), “Causal Effect of Intergroup Contact on Exclusionary Attitudes,” explores the precise mechanism that can cause negative attitudes toward other ethnic, racial or religious groups. A 2011 study from Virginia Union University and the University of Missouri, “The Impact of Immigration on Anti-Hispanic Hate Crime in the United States,” analyzes FBI, Census and Department of Homeland Security data to determine incidence levels of anti-Hispanic hate crime in periods and places with higher levels of immigration.
Keywords: hate crime, terror attacks, law, prejudice, terrorism
About The Author
Joanna Penn
Hate Crimes Research Paper
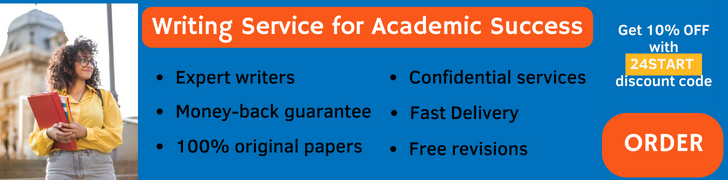
View sample crime research paper on hate crimes. Browse criminal justice research paper topics for more inspiration. If you need a thorough research paper written according to all the academic standards, you can always turn to our experienced writers for help. This is how your paper can get an A! Feel free to contact our writing service for professional assistance. We offer high-quality assignments for reasonable rates.
A hate crime is a crime committed as an act of prejudice against the person or property of a victim as a result of that victim’s real or perceived membership in a particular group. Many of the most notorious hate crimes have been murders, such as the racially motivated murder of James Byrd, Jr., in Texas in 1998 or the homophobicmotivated murder of Matthew Shepard in North Dakota later that same year. The vast majority of hate crimes, however, are cases of assault or vandalism.
Academic Writing, Editing, Proofreading, And Problem Solving Services
Get 10% off with 24start discount code.
The critical identifying element of hate crimes is the bias motivation of the perpetrator. The distinguishing factor can be obscured by the very term hate crime , which is the popular term used in connection with bias-motivated violence. In fact, bias crime is a more accurate label. Many if not most crimes are motivated by hatred of one kind or another. Not every crime that is motivated by hatred for the victim is a bias crime. Hatebased violence causes a bias crime only when this hatred is connected with antipathy for a group, such as a racial or ethnic group, or for an individual because of membership in that group. In some form, virtually every state in the United States expressly criminalizes bias crimes.
Elements of Bias Crimes
Bias crime statutes in the United States encompass crimes that are motivated by the race, color, ethnicity, national origin, or religion of the victim. Many reach sexual orientation or gender as well, and some include other categories such as age or disability. Bias crime laws may either create a specific crime of bias-motivated violence or raise the penalty of a crime when committed with bias motivation.
The key factor in identifying an actor as a bias criminal is the motivation for the conduct. Bias crimes are unusual but not unique in their focus on motivation rather than the traditional focus on intent. Some scholars have criticized bias crime laws on this basis, a critique that is addressed below.
There are two analytically distinct, albeit somewhat overlapping models of bias crimes. These models may be referred to as the discriminatory selection model and the group animus model . (In this terminology, group is used to represent all group characteristics that constitute bias crimes, such as ethnicity, race, or religion.)
The discriminatory selection model of bias crimes defines these crimes in terms of the perpetrator’s selection of his victim. It is irrelevant why an offender selected his victim on the basis of race or other group; it is sufficient that the offender did so. The discriminatory selection model received much attention because it was a statute of this model that was upheld by the Supreme Court in Wisconsin v. Mitchell , 508 U.S. 476 (1993). The group animus model of bias crimes defines crimes on the basis of a perpetrator’s animus for the group of the victim and the centrality of this animus in the perpetrator’s motivation for committing the crime. Florida and Massachusetts, among other states, have adopted group animus bias crimes laws. Many and perhaps most cases of discriminatory selection are in fact also cases of group animus bias crimes, but not all. A purse snatcher, for example, who preys solely on women, finding it more efficient to grab purses than to pick wallets out of men’s pockets, would have discriminatorily selected a victim on the basis of gender, but not with group animus.
Most states with bias crime laws have adopted statutes that draw on both models. These laws provide enhanced sentences for crimes committed ‘‘because of ’’ or ‘‘by reason of ’’ the victim’s real or perceived membership in a particular group. Although these statutes lack explicit reference either to discriminatory selection or animus, they share attributes of both. ‘‘Because of ’’ statutes look to the perpetrator’s selection of the victim. In addition, particularly in those states that require a finding of maliciousness, ‘‘because of ’’ statutes are akin to animus as well.
Under any of these models, bias crimes can arise out of mixed motivation where the perpetrator of a violent crime is motivated by a number of different factors in the commission of the crime, bias among them. To constitute a bias crime, the bias motivation must be a substantial motivation for the perpetrator’s criminal conduct. Under the Supreme Court decision in Apprendi v. New Jersey , 120 S.Ct. 2348 (2000), all elements of a bias crime must be submitted to a jury (or judge as a trier of fact) and proven beyond a reasonable doubt; a sentence enhancement for a bias crime may not be imposed on a finding by preponderance of evidence by the sentencing judge.
How Bias Crimes Differ from Other Crimes
The justification for bias crime laws turns primarily on the manner in which bias crimes differ from other crimes. Bias crimes cause greater harm than parallel crimes , that is, those crimes that lack a prejudicial motivation but are otherwise identical to the bias crime. This is true on three levels: harm to the individual victim, the victim’s group or community, and the society at large.
Bias crimes generally have a more harmful emotional and psychological impact on the individual victim. The victim of a bias crime is not attacked for a random reason (e.g., the person injured during a drive-by shooting) nor for an impersonal reason (e.g., the victim of a violent robbery). Rather the victim of a bias crime is attacked for a specific, personal reason: for example, race, religion, ethnicity, or sexual orientation. Moreover, the bias crime victim cannot reasonably minimize the risks of future attacks because the victim is unable to change the characteristic that made him a victim in the first place. The heightened sense of vulnerability caused by bias crimes is beyond that normally found in crime victims. Studies have suggested that the victims of bias crimes tend to experience psychological symptoms such as depression or withdrawal, as well as anxiety, feelings of helplessness, and a profound sense of isolation.
The impact of bias crimes reaches beyond the harm done to the immediate victim or victims of the criminal behavior. There is a more widespread impact on the ‘‘target community’’—that is, the community that shares the race, religion, ethnicity, or other group characteristic of the victim. The target community experiences bias crime in a manner that has no equivalent in the public response to parallel crimes. The reaction of the target community goes beyond mere sympathy with the immediate victim. Members of the target community of a bias crime perceive that crime as if it were an attack on themselves directly and individually.
Finally, the impact of bias crimes may spread beyond the immediate victims and the target community to the general society. This effect may be seen on a number of levels, and includes a spectrum of harms from the very concrete to the most abstract. On the most prosaic level—but by no means least damaging—the isolation effects discussed above have a cumulative effect throughout a community. Members of the community, even those who are sympathetic to the plight of the victim family, may be reluctant to place themselves or their children in harm’s way, and will shy away from socializing with the victims, thus exacerbating the problems associated with social isolation.
Bias crimes cause an even broader injury to the general community. Such crimes violate not only society’s general concern for the security of its members and their property but also the shared value of equality among its citizens and racial and religious harmony in a heterogeneous society. A bias crime is therefore a profound violation of the egalitarian ideal and the antidiscrimination principle that have become fundamental not only to the American legal system but to American culture as well. Indeed, when a legislature defines the groups that are to be included in a bias crime law, it unavoidably makes a normative statement as to the role of certain groups or characteristics. Bias crime laws are concerned with those characteristics that implicate social fissure lines, divisions that run deep into the social history of a culture. Thus every bias crime law in the United States includes race as a category; racial discrimination, with its earliest roots in slavery, is the clearest example of a social fissure line in American society. Strong cases can similarly be made for the other classic bias crime categories—color, ethnicity, religion, and national origin. When a state legislature debates the inclusion of other categories to its bias crime law, the debate is partly over the place of those groups in society. Drafting the scope of a bias crime law is necessarily a process that includes the locating of social fissure lines.
Scope of The Problem
Although there is some reason to believe that the level of bias crimes increased over the last two decades of the twentieth century, it remains difficult to gauge whether the bias crime problem has actually worsened. During the 1980s, public concern over the level of bias-motivated violence in the United States rose dramatically. Such concern and the consequent enactment of bias crime statutes across the United States probably stemmed, at least in part, from an apparent worsening of the bias crime problem. Statistics from both independent and governmental datagathering organizations support the conclusion that bias crime increased over the course of the 1980s and, to a large extent, leveled off during the 1990s. These statistics, however, remain inconsistent and incomplete. Moreover, the statistics gathered toward the end of the 1980s and throughout the early to mid-1990s reflected not only a growth in the bias crime problem, but also a growth in legislative and administrative awareness of the problem.
In general, experts and commentators on bias crime agree that these crimes had, throughout the mid and late 1980s and early 1990s, increased annually. The main organizations that collect data on the subject of bias-motivated violence—the Anti-Defamation League, the Southern Poverty Law Center, and the National Gay and Lesbian Task Force—all reported such persistent growth.
In 1990 Congress passed the Hate Crime Statistics Act (HCSA) in an effort to provide official statistics concerning the level of bias crimes. Under this act, the Department of Justice must collect statistics on the incidence of bias crimes in the United States as a part of its regular information-gathering system. The Attorney General delegated the development and implementation of the HCSA to the Federal Bureau of Investigation’s Uniform Crime Reporting Program for incorporation among its sixteen thousand voluntary law enforcement agency participants. Beginning with the HCSA’s implementation in 1991 and through the early 1990s, the F.B.I. documented a general rise in bias crimes. However, these figures, like those reported by other data-gathering organizations, remain vulnerable to charges of inaccuracy. Because the F.B.I.’s numbers simply mirror the numbers reported by state and local law enforcement agencies, and because agency participation under the HCSA is voluntary, the completed data more aptly reflect popular perception of the bias crime problem rather than the problem itself.
There is a mutual-feedback relationship between the bias crime problem and both the popular perception and official response to the problem. A perceived increase in bias crime as fostered by independent data-gathering and reporting leads to increased public concern regarding such crimes. Such concern leads, in succession, to legislative and administrative response, to increased official reporting, and, in effect, to an even greater perceived increase in bias crime. Thus, problem and perception conflate, and the apparent growth in bias crime becomes not simply a reflection of increased hatred and apathy (as the statistics alone would suggest) but also an indication of increased understanding and action (as the increased response to the problem suggests).
On the other hand, there is reason to believe that, despite increased bias crime reporting by police agencies, a majority of bias crime victims do not report incidents at all. Victims’ distrust of the police, language barriers, and fear of either retaliation by the offender or public exposure generally may well lead to systemic underreporting of bias crimes.
In addition to all of the problems with measuring the current level of bias crimes, there is a significant problem with establishing a baseline for a meaningful comparison. Data collection on the levels of bias crimes prior to the mid-1980s was virtually nonexistent. For example, it was not until 1978 that the Boston City Police Department became the first law enforcement agency to track bias-motivated crimes; it was not until 1981 that Maryland became the first state to pass a reporting statute.
It is thus not possible to say with confidence the extent to which bias crimes are increasing and the extent to which the increase is one of perception. However, the obvious relationship between perception and problem in no way undercuts the severity of the problem. Whatever the difficulties of measuring bias crime levels with precision, the existence of a serious level of bias-motivated crime is confirmed. Moreover, the mutual-feedback relationship between the level of bias crime and the popular perception of this level does not necessarily undermine a determination of the severity of the problem. As the understanding of what constitutes a bias crime is broadened, that which may have been dismissed as a ‘‘prank’’ in an earlier time is now properly revealed as bias-motivated criminal conduct. This does not mean that bias crimes are being overcounted; rather it means that previously these crimes were undercounted.
Critique of Bias Crimes
The enhanced punishment of bias-motivated violence has been criticized on a number of grounds. One critique argues that bias crime laws punish thoughts and not criminal acts. This critique itself takes two forms: a constitutional argument that bias crime laws violate the First Amendment right to free expression of ideas, and a criminal law theory argument that bias crime laws improperly focus on motivation rather than mens rea. An additional critique, which applies only to federal bias crime laws, involves questions of federalism and the constitutional authority for such legislation.
The free expression challenges to bias crime laws were the subject of a great deal of scholarly attention as well as a number of judicial opinions. Judicial consideration of the issue culminated in two Supreme Court decisions, R.A.V. v. City of St. Paul , 505 U.S. 377 (1992), which struck down a municipal cross-burning ordinance, and Wisconsin v. Mitchell , which upheld a state law that provided for increased penalties for bias crimes. Three general positions have emerged among observers concerning the challenge to bias crime laws based in principles of free expression. One position argues that bias crime laws unconstitutionally punish thought because the increased punishment is due solely to the defendant’s expression of a conviction of which the community disapproves. A second position permits the enhanced punishment of bias crimes, arguing that bias motivations and hate speech are not protected by the First Amendment. Ironically, these two opposing positions share a common premise: that bias crime laws do involve the regulation of expression.
The third position distinguishes between hate speech and bias crimes, protecting the former but permitting the enhanced punishment of the latter. This has been understood in two related ways. One approach is based on the distinction between speech and conduct, protecting hate speech as the former and punishing bias crimes as the latter. This is the approach adopted by the Court in Wisconsin v. Mitchell . An alternative approach focuses on the perpetrator’s state of mind, and distinguishes behavior that is intended to communicate from behavior that is intended to cause focused and individualized harm to a targeted victim.
The critique that bias crime laws punish bad thoughts rather than criminal acts also has been based on criminal law doctrine. This argument criticizes bias crime laws for impermissibly straying beyond the punishment of act and purposeful intent to reach the punishment of motivation. The argument rests on the assertion that motive can be distinguished from mens rea, based on the formal distinction between motive and intent: intent concerns the mental state provided in the definition of an offense in order to assess the actor’s culpability with respect to the elements of the offense, whereas motive concerns the cause that drives the actor to commit the offense.
Several responses have been made to this critique. First, as a matter of positive law, concern with the punishment of motivation may be misplaced. Motive often determines punishment. In those states with capital punishment, the defendant’s motivation for the homicide stands prominent among the recognized aggravating factors that may contribute to the imposition of the death sentence. For instance, the motivation of profit in murder cases is a significant aggravating factor adopted in most capital sentencing schemes. Bias motivation itself may serve as an aggravating circumstance. In Barclay v. Florida , 463 U.S. 939 (1983), the Supreme Court explicitly upheld the use of racial bias as an aggravating factor in the sentencing phase of a capital case. The Court reaffirmed Barclay in Dawson v. Delaware , 503 U.S. 159 (1992).
A second response to this critique of bias crime laws more broadly questions the usefulness of the formal distinction between intent and motive, arguing that the decision as to what constitutes motive and what constitutes intent largely turns on what is being criminalized. Criminal statutes define the elements of the crime and a mental state applies to each element. The mental state that applies to an element of the crime is ‘‘intent’’ whereas any mental states that are extrinsic to the elements are ‘‘motivation.’’ The formal distinction, therefore, turns on the elements of the crime. What is a matter of intent in one context may be a matter of motive in another. There are two equally accurate descriptions of a bias-motivated assault: the perpetrator possessed a (i) mens rea of purpose with respect to the assault along with a motivation of bias; or (ii) a mens rea of purpose with respect to the parallel crime of assault and a mens rea of purpose with respect to assaulting this victim because of group identification. The defendant in description (i) ‘‘intends’’ to assault the victim and does so because the defendant is a bigot. The defendant in description (ii) ‘‘intends’’ to commit an assault and does so with both an intent to assault and a discriminatory or animus-driven intent as to the selection of the victim. Both descriptions are accurate. The formal distinction between intent and motive may thus bear less weight than some critics have placed upon it. Whether bias crime laws punish motivation or intent is not inherent in those prohibitions. Rather the distinction mirrors the way in which the law describes these crimes.
The federalism challenges to the constitutionality of a federal bias crime law arise from the fact that the vast majority of bias crimes are state law crimes that are motivated by bias. The question of constitutional authority for a federal bias crime law is especially pressing after the Supreme Court’s decisions in United States v. Morrison , 120 S.Ct. 1740 (2000), striking down the civil remedy provisions of the Violence Against Women Act, and United States v. Lopez , 514 U.S. 549 (1995), striking down the Federal Gun-Free Zones Act. Each decision held that the legislation in question exceeded Congress’ authority under the commerce clause. It is partially for this reason that, at the time of writing, there is no pure federal bias crimes statute. Bias motivation is an element of certain federal civil rights crimes such as 18 U.S.C. § 245. Moreover, in 1994, Congress directed the U.S. Sentencing Commission to promulgate guidelines enhancing the penalties for any federal crimes that are motivated by bias. These statutes, however, cover only a small range of cases involving bias motivation.
After Morrison and Lopez , the commerce clause, the constitutional authority for civil rights legislation during the 1960s barring discrimination in public accommodations, housing, and employment, is a more doubtful source for constitutional authority for a federal bias crime law. A more promising source for such authority may lie in the post–Civil War constitutional amendments, at least for bias crimes involving racial, ethnic, and possibly religious motivation. In enacting section 245, Congress expressly relied, in part, upon the Fourteenth and Fifteenth Amendments as authority for the federalization of biasmotivated deprivation of certain specified rights individuals hold under state law. Not all bias crimes deprive the victim of the ability to exercise some right under state law. It has been argued, however, that the Thirteenth Amendment as well provides constitutional authority for a federal bias crime law. The modern view of the Thirteenth Amendment, articulated in Jones v. Alfred H. Mayer , 392 U.S. 409 (1968), and Runyon v. McCrary , 427 U.S. 160 (1976), understands the amendment as a constitutional proscription of all the ‘‘badges and incidents’’ of slavery, authorizing Congress to make any rational determination as to what constitutes a badge or incident of slavery and to ban such conduct, whether from public or private sources. The abolition of slavery in the Thirteenth Amendment, although immediately addressed to the enslavement of AfricanAmericans, has been held to apply beyond the context of race to include ethnic groups and perhaps religions as well. The Thirteen Amendment would not, however, provide constitutional authority for elements of a federal bias crime law reaching sexual orientation, gender, or other categories.
Bibliography:
- ALTSCHILLER, DONALD. Hate Crimes: A Reference Handbook. Santa Barbara, Calif.: ABC-CLIO, Inc., 1999.
- Anti-Defamation League of B’nai B’rith. Hate Crimes Laws: A Comprehensive Guide. New York: Anti-Defamation League, 1994.
- BOWLING, BENJAMIN. Violent Racism: Victimisation, Policing, and Social Context. Oxford, U.K.: Clarendon Press, 1998.
- Bureau of Justice Assistance, U.S. Department of Justice. A Policymaker’s Guide to Hate Crimes. Washington, D.C.: Department of Justice, 1997.
- DILLOF, ANTHONY. ‘‘Punishing Bias: An Examination of the Theoretical Foundations of Bias Crime Statues.’’ Northwestern University Law Review 91 (1997): 1015.
- KELLY, ROBERT, ed. Bias Crime: American Law Enforcement and Legal Responses. Chicago: University of Illinois, 1991.
- JACOBS, JAMES, and POTTER, KIMBERLY. Hate Crimes: Criminal Law and Identity Politics. New York: Oxford University Press, 1998.
- LAWRENCE, FREDERICK ‘‘The Punishment of Hate: Toward a Normative Theory of Bias-Motivated Violence.’’ Michigan Law Review 93 (1994): 320.
- LAWRENCE, FREDERICK Punishing Hate: Bias Crimes Under American Law. Cambridge, Mass.: Harvard University press, 1999.
- LEVIN, JACK, and MCDEVITT, JACK. Hate Crimes: The Rising Tide of Bigotry and Bloodshed. New York: Plenum Press, 1993.
- WANG, LU-IN. Hate Crimes Law. Paul, Minn.: Clark, Boardman & Callaghan, 1997.
ORDER HIGH QUALITY CUSTOM PAPER

In states with laws targeting LGBTQ issues, school hate crimes quadrupled
School hate crimes targeting LGBTQ+ people have sharply risen in recent years, climbing fastest in states that have passed laws restricting LGBTQ+ student rights and education, a Washington Post analysis of FBI data finds.
In states with restrictive laws, the number of hate crimes on K-12 campuses has more than quadrupled since the onset of a divisive culture war that has often centered on the rights of LGBTQ+ youth.
At the same time, calls to LGBTQ+ youth crisis hotlines have exploded, with some advocates drawing a connection between the political climate and the spike in bullying and hate crimes.
Tell The Post: Do you have experience with anti-LGBTQ+ bullying at school?
LGBTQ+ students have long dealt with bullying and harassment at school, but some students are feeling particularly vulnerable due to the wave of legislation. They are also on edge following the death of Nex Benedict, a nonbinary teenager who died after a fight in their Oklahoma public school bathroom. The death was ruled a suicide, according to a one-page summary report released Wednesday by the state’s chief medical examiner.
That’s the case for Carden, a transgender 17-year-old. He argues that politicians’ anti-LGBTQ+ rhetoric has shaped the views of adults in the conservative Virginia county where he lives.
“Kids parrot these ideas in their head, it’s like, ‘Oh, my parents think …’” said Carden, whose parents asked that his last name be withheld for fear of further bullying. “Then it translates to being mean to other people their age.”
Twice in the fall, a group of freshmen boys at Carden’s school harassed him for his gender identity — once calling him “queer” in a nasty tone, he said. The second time, after telling him a Pride flag he had tucked into his backpack was “pitiful,” one of the boys suggested Carden should “just go die already.”
Since his election in 2021, Virginia Gov. Glenn Youngkin (R) has encouraged schools to require that students use facilities matching their biological sex and has signed a law requiring schools to alert parents of “sexually explicit” lessons, alarming LGBTQ+ advocacy groups, which predicted it would be used to limit education on sexual orientation and gender identity. Other Republican governors have advanced similar policies through executive action and legislation.
The Post analysis found that the number of anti-LGBTQ+ school hate crimes serious enough to be reported to local police more than doubled nationwide between 2015-2019 and 2021-2022. The rise is steeper in the 28 states that have passed laws curbing the rights of transgender students at school and restricting how teachers can talk about issues of gender and sexuality.
In more-liberal states that have not enacted restrictive school LGBTQ+ laws, The Post found that the rise in FBI hate crimes was lower — though the absolute number of crimes was higher. Analysts said that may be because people in those states are more likely to report incidents.
Advocacy groups have also seen a rising number of young people in distress.
Calls have spiked to the Trevor Project, which provides support to LGBTQ+ youth aimed at suicide prevention and crisis intervention. In the fiscal year ending in July 2022, the group fielded about 230,000 crisis contacts, including phone calls, texts and online chats. The following year, it was more than 500,000.
Similarly, the Rainbow Youth Project , a nonprofit that offers crisis response and counseling to at-risk LGBTQ+ youth, saw calls to its hotline rise from an average of about 1,000 per month in 2022 to just over 1,400 per month last year. The top reason cited by callers in 2023 was anti-LGBTQ+ “political rhetoric,” such as debate over laws and policies limiting rights at school.
Young people will say, “‘My government hates me,’ ‘My school hates me,’ ‘They don’t want me to exist,’” said Lance Preston, the group’s founder and executive director. “That ... is absolutely unacceptable. That is shocking.”
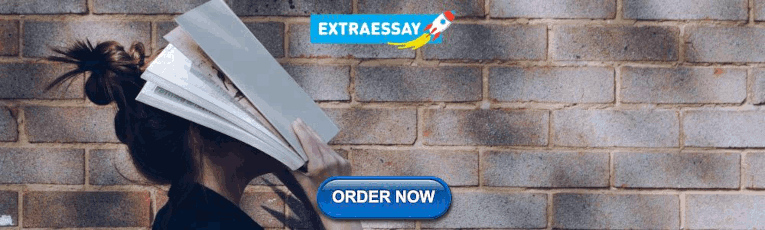
How The Post reported on anti-LGBTQ hate at school
In the weeks after Nex’s death, the Rainbow Youth Project saw a crush of calls from Oklahoma, rising from 321 in January to nearly 1,100 in February, though the surge may have been driven at least in part by news coverage of the group’s work.
Nex, who used they/them pronouns, died on Feb. 8, a day after a confrontation in the bathroom of their school in a Tulsa suburb. School officials sent the students home, but Nex’s grandmother took Nex to the hospital later that day and called police.
In a statement to an officer, Nex and their grandmother said three girls had been bullying Nex and a friend because of how they dress. In the bathroom that day, these girls were making comments Nex found offensive, and Nex poured water on them. Then “all three of them came at me,” Nex said. A fight ensued.
Nex was discharged from the hospital but rushed back the next day, when they died. The case has drawn national attention. Before the cause of death was released, LGBTQ+ advocates argued that Nex was bullied and that their death was a hate crime related to their gender identity. After the finding of suicide was made public, they connected the suicide to the bullying. The Office for Civil Rights at the Department of Education this month opened an investigation into the school’s actions.
Since 2020, Oklahoma has adopted several laws restricting transgender rights. The state prohibits students from using bathrooms that do not align with the sex assigned at their birth and bars minors from receiving transition-related care. The legislature is considering additional measures this year, including a ban on changing one’s gender on birth certificates and requiring that schools teach that “a person’s sex is an immutable biological trait” that cannot be changed.
Many of these bills have been championed by the state’s schools superintendent, Ryan Walters, who said in an interview that Nex’s death was a heartbreaking tragedy, but that those who are connecting it to bullying or gender identity are pushing a “left-wing agenda” and “politicizing the death of a student.”
Va. killed bills aimed at trans youths. Here’s where the debate moves next.
Walters said he will continue to push for legislation to counter what he called “radical gender theory,” which he defines as the notion that one’s gender can differ from biological sex.
“There’s two genders. That’s the way God created us,” Walters said. Some young people are confused, he said, but, “your job as an adult is to help kids, not lie to them.”
He added that he opposes bullying but wholly rejects the idea that the political environment is encouraging it.
“Because you share a belief or an opinion does not mean it’s connected to violence,” he said.
Hate crimes on the rise
The FBI data shows serious incidents against LGBTQ+ people are on the rise, particularly in the more than two dozen states that have passed laws targeting LGBTQ+ students or education. Some of these laws, like those enacted in Oklahoma, bar students from competing on sports teams or using school bathrooms that do not conform with their sex assigned at birth. Others circumscribe what teachers can teach about gender identity or sexual orientation or bar instruction on these subjects entirely.
Overall, there were an average of 108 anti-LGBTQ+ hate crimes at schools reported to the FBI per year from 2015 to 2019 on both college and K-12 campuses. In 2021 and 2022, the most recent years for which data was available, the average more than doubled to 232. (The number of reported hate crimes overall dropped in 2020, when the covid-19 pandemic shut down many school campuses, forcing learning online.)
The rise was even steeper in states that have enacted laws or policies which restrict LGBTQ+ students’ education or rights at school — tripling from an average of about 28 per year from 2015 to 2019 to an average of about 90 per year in 2021 to 2022. There was also an upsurge in the states without these laws, from about 79 reported hate crimes per year to 140.
When the data is limited to K-12 campuses, the increase is even more marked. In states that have enacted restrictive laws, there were more than four times the number of anti-LGBTQ+ hate crimes on average, per year, in 2021 to 2022 compared with the years 2015 to 2019 across elementary, middle and high schools.
“Policy sets the tone for real-world experiences [and] discriminatory policy just creates a hostile environment,” said Amy McGehee, a doctoral student at Oklahoma State University who researches LGBTQ+ health and well-being.
FBI data indicate the most common crimes associated with reported hate crimes at schools include simple assault, intimidation and vandalism.
McGehee added that LGBTQ+ students were reporting feeling unsafe on both college and K-12 campuses even before states began passing waves of policies restricting their rights at school. A sweeping Washington Post-KFF poll last year found that school is among the greatest stressors for transgender children in particular. Forty-five percent of trans adults said they felt generally unsafe at school as a child or teenager, compared with 10 percent of cisgender adults.
Trans kids crave acceptance at school in a nation that often resists it
The Post’s analysis of FBI data found that the per capita hate crime rates on K-12 and college campuses were higher in the more liberal states that have not enacted laws limiting transgender rights. Although the finding may seem counterintuitive, it actually makes sense, said Stephen Russell, a University of Texas at Austin professor who studies LGBTQ+ youth. He said LGBTQ+ youth and families living in those 22 states were probably more likely to report violence and harassment in the first place.
Many of these states have adopted laws and school policies specifically prohibiting bullying of or discrimination against LGBTQ+ students, he said. In some places, he said, that includes required annual notifications alerting students and parents to their rights and spelling out how they can and should report bad behavior.
“It creates a context where they see themselves, they stand up for themselves, they believe there is a place for them in their schools,” Russell said of LGBTQ+ children.
In addition, it’s possible more kids are public about their identities in more liberal states, creating more targets for bullies, said Lanae Erickson, a senior vice president at Third Way, a centrist Democratic think tank, who studies social policy and politics.
Both Erickson and Russell were unsurprised to learn that the number of hate crimes had risen faster in states with conservative laws.
“The data you have is the thing I’ve been worrying about, and here it is,” said Russell.
‘They don’t want us to exist’
The upsurge in restrictive laws and in school bullying has left some LGBTQ+ students feeling under attack.
Last year, classmates regularly harassed a transgender teenager in Mississippi for his short hair and for wearing boy’s clothing and chest binders, his mother said in an interview. Throughout that year, her son often woke up in the middle of the night vomiting due to stress. He wound up missing about three weeks of school due to bullying-induced breakdowns, recalled the mother, who spoke on the condition of anonymity for fear of retaliation from family members who do not support her son’s gender identity.
She said her son has been suicidal at times, at one point keeping a journal in which he detailed plans to kill himself. She said he also cut himself. “He feels shamed,” she said. “It’s hard for him to find friends.”
In 2021, Mississippi enacted a law barring transgender students from playing on sports teams that match their gender identity. Partly citing that state policy, the mother said she feels like everything is just getting worse.
“I’m really worried that my child is getting irreparable mental damage from all of this,” she said. “I also just get worried that, what if he gets suicidal again? I don’t want to lose my son.”
Studies show that LGBTQ+ youth are at particular risk for bullying and mental health problems. The Centers for Disease Control and Prevention’s 2021 Youth Risk Behavior survey, for instance, found that more than 1 in 3 gay, lesbian and bisexual students were bullied either on school property or online in the previous year. For about 1 in 4, the bullying was at school. Among straight students, those rates were about half as high.
When Max Ibarra was a sophomore in high school in the Chino Valley, Calif., east of Los Angeles, they endured what Max thinks of as everyday bullying from other students.
Max, who identifies as trans and nonbinary, recalled hearing snickering behind their back. In one class, they said, another student regularly threw erasers and wads of paper at them in class. In the hallway once, someone purposefully stuck out a shoulder to shove Max into the brick wall.
But what really bothers Max, now 17, is what the Chino Valley Unified School District school board did. In July 2023, the board approved a policy requiring the school to notify parents if a student asked to use a name that differed from official records, or if they asked to use pronouns or school facilities that do not align with the sex listed on the student’s birth certificate.
At the school board meeting where the policy was approved, 4-1, Max shouted objections into the microphone during the public comment period. They said that many trans students feel safe at school but not at home, and that the policy “shoves them in the closet.”
“I am tired of the idea that all parents love and support their children unconditionally. That is not true,” they yelled into the microphone. “There are evil people in this world, and some of them have children. Shocking, I know! Some prefer a dead child to a trans child.”
A spokesperson for the district declined to respond directly to Max’s assertions but said the schools have systems in place to report and respond to bullying and to address the needs of LGBTQ+ students. The district, she said, is “committed to upholding an educational setting that encourages, emboldens, and motivates scholars to become the global citizens they are meant to be.”
The policy was later put on hold by a court, and Thursday, the school board rolled back most of it. Still, Max said in an interview that the tone set by the school board remains.
“This culture of hostility and division has been festering, especially for the last three years, and its ramped up more and more each year,” Max said. “The school board has made it very clear we’re not welcome here. It’s very clear they don’t want us to exist.”
Emily Guskin and Scott Clement contributed to this report.

Toronto Police Report Surge in Hate Crimes Against Jews, Muslims
FILE PHOTO: Toronto skyline stands on the waterfront n Toronto, Ontario, Canada October 17, 2017. REUTERS/Mark Blinch/File Photo
By Wa Lone and KyawSoe Oo
Toronto (Reuters) - The number of antisemitic and anti-Muslim hate crimes in Canada's largest city of Toronto have risen significantly during the war in Gaza that followed Hamas' deadly Oct. 7 attack inside Israel, Toronto police said on Monday.
Toronto has seen 69 arrests and 173 charges related to hate crimes during this period, police Chief Myron Demkiw said in a statement. Since October 2023, Toronto has witnessed 203 confirmed hate crimes, a 93% rise from a year ago, the statement added.
Demkiw said hate crime calls dropped in December and January, but then picked up in February, rising 67%. Of the 84 hate crimes so far in 2024, 56% are antisemitic, Demkiw said.
War in Israel and Gaza

The second highest bias category this year are hate crimes targeting the 2SLGBTQI+ communities, followed by anti-Black, and anti-Muslim/Arab/Palestine, Demkiw added.
The latest conflict between Israel and Palestine started on Oct 7, when Hamas fighters stormed into Israel, killing 1,200 people and capturing 253 hostages according to Israeli tallies. Since then, Israel's assault has killed more than 31,000 Gazans, according to Palestinian health officials.
"While underreporting of all forms of hate crimes is a concern, I know from talking to people in the community that Islamophobia is a significant concern, and given our statistics I am concerned about significant under-reporting in this regard,” Demkiw told reporters.
(Reporting by Wa Lone, Kyaw Soe Oo)
Copyright 2024 Thomson Reuters .
Join the Conversation
Tags: Canada , Israel , crime , Middle East

Best Countries

Health News Bulletin
Stay informed on the latest news on health and COVID-19 from the editors at U.S. News & World Report.
Sign in to manage your newsletters »
Sign up to receive the latest updates from U.S News & World Report and our trusted partners and sponsors. By clicking submit, you are agreeing to our Terms and Conditions & Privacy Policy .
You May Also Like
The 10 worst presidents.
U.S. News Staff Feb. 23, 2024

Cartoons on President Donald Trump
Feb. 1, 2017, at 1:24 p.m.

Photos: Obama Behind the Scenes
April 8, 2022

Photos: Who Supports Joe Biden?
March 11, 2020
New Construction Bounces Back
Tim Smart March 19, 2024

Biden Anxious to Shore Up Swing States
Susan Milligan March 18, 2024

What to Know About the Looming Shutdown
Lauren Camera March 18, 2024

The Week in Cartoons Mar. 18-22
March 18, 2024, at 3:05 p.m.

March Madness: Fed, Budget Spell Upset
Tim Smart March 18, 2024

Trump’s Strategy of Delay Is Working
Lauren Camera March 15, 2024

Read our research on: TikTok | Podcasts | Election 2024
Regions & Countries
Key findings about religious restrictions around the world in 2021.
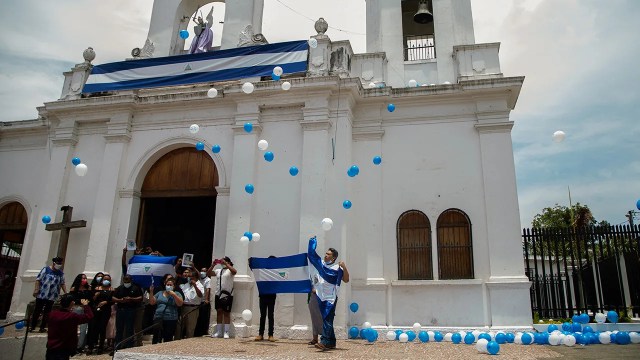
Pew Research Center has been tracking restrictions on religion around the world since 2007. We divide these restrictions into two categories: actions by governments and actions by private individuals or groups. Our tally of restrictions includes laws that prohibit atheism, as well as attempts to coerce people into adopting religious beliefs.
This post is based on the 14th annual Pew Research Center report on the extent to which governments and societies around the world impinge on religious beliefs and practices. The studies are part of the Pew-Templeton Global Religious Futures project, which analyzes religious change and its impact on societies around the world.
To measure global restrictions on religion in 2021, the most recent year for which data is available, the study rates 198 countries and territories by their levels of government restrictions on religion and social hostilities involving religion. The new study is based on the same 10-point indexes used in the previous studies.
- The Government Restrictions Index (GRI) measures government laws, policies and actions that restrict religious beliefs and practices. The GRI comprises 20 measures of restrictions, including efforts by governments to ban particular faiths, prohibit conversion, limit preaching or give preferential treatment to one or more religious groups.
- The Social Hostilities Index (SHI) measures acts of religious hostility by private individuals, organizations or groups in society. This includes religion-related armed conflict or terrorism, mob or sectarian violence, harassment over attire for religious reasons and other forms of religion-related intimidation or abuse. The SHI includes 13 measures of social hostilities.
To track these indicators of government restrictions and social hostilities, researchers combed through more than a dozen publicly available, widely cited sources of information, including the U.S. Department of State’s annual Reports on International Religious Freedom and annual reports from the U.S. Commission on International Religious Freedom, as well as reports and databases from a variety of European and United Nations bodies and several independent, nongovernmental organizations. (Read the methodology for more details on sources used in the study.)
Our latest study , the 14th in this annual series, covers events that took place in 198 countries and territories in 2021. Here are the key findings:
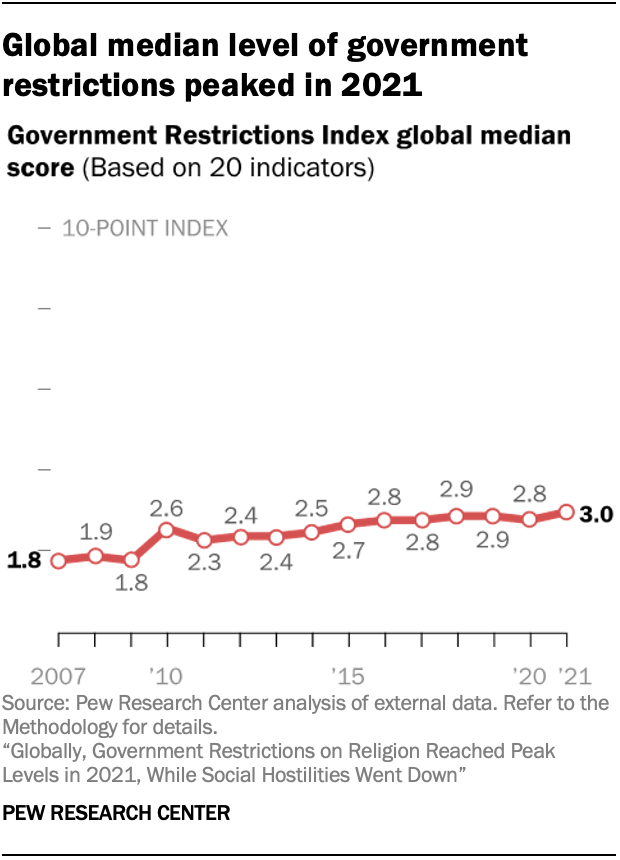
Government restrictions on religion reached a new high in 2021. Globally, the median score on our 10-point Government Restrictions Index rose from 2.8 in 2020 to 3.0 in 2021 – the highest level recorded since we began tracking this in 2007. The index tracks 20 measures on government laws, policies and actions that limit religious beliefs and practices, including banning certain faiths; the use of force against religious groups; preferential treatment of some groups over others; and restrictions on preaching, converting or proselytizing.
The most common kinds of government restrictions on religion in 2021 included harassment of religious groups and interference in worship. Governments harassed religious groups in 183 countries, the most on record. The harassment took a wide range of forms, from the use of physical force to derogatory comments made by public officials.
In Nicaragua, for example, top public officials verbally attacked Catholic clergy for supporting pro-democracy protestors. The president and vice president, who is also the first lady, called Catholic priests and bishops “terrorists in cassocks” and “coup-plotters.” A member of the National Assembly, Wilfredo Navarro Moreira, also called a cardinal and several bishops “servants of the devil” in a television interview.
Meanwhile, governments interfered in worship in 163 countries, very close to the peak level of 164 countries reached in 2020. Government interference in worship includes policies or actions that disrupt religious gatherings, deny permits for religious activities, bar access to places of worship, and restrict other rituals.
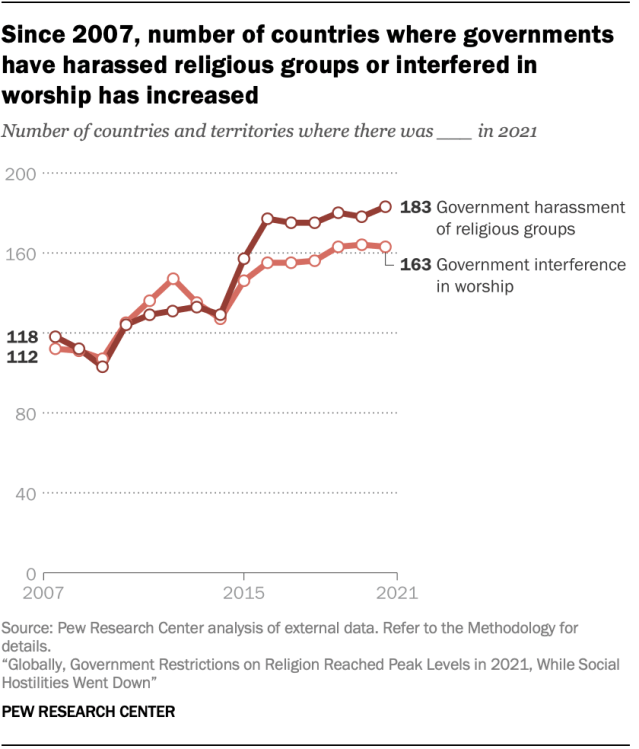
Some cases of government interference in worship in 2021 were related to the COVID-19 pandemic .
For example, three Canadian churches faced government fines for defying lockdown measures , prompting them to file a legal challenge on the grounds that other entities – including restaurants, other businesses and Orthodox Jewish congregations – faced fewer restrictions. In addition, several Canadian clergy faced arrest and fines after holding in-person services in violation of public health measures.
(It’s important to note that our study does not attempt to determine whether particular restrictions are justified or unjustified. Nor do we attempt to analyze the many reasons why restrictions may have arisen in each country. The goal of the tracking is simply to measure restrictions in a transparent and reproducible way, and to identify patterns and trends over time.)
In 2021, China had the world’s highest level of government restrictions on religion, while Nigeria had the highest level of social hostilities involving religion. Social hostilities are actions by private groups or individuals that infringe on the freedom of religious groups.
Globally, social hostilities declined slightly in 2021, with the median score on our Social Hostilities Index falling from 1.8 in 2020 to 1.6. In general, social hostilities have tended to fluctuate more than government restrictions from year to year. But some hostilities also have become deeply entrenched.
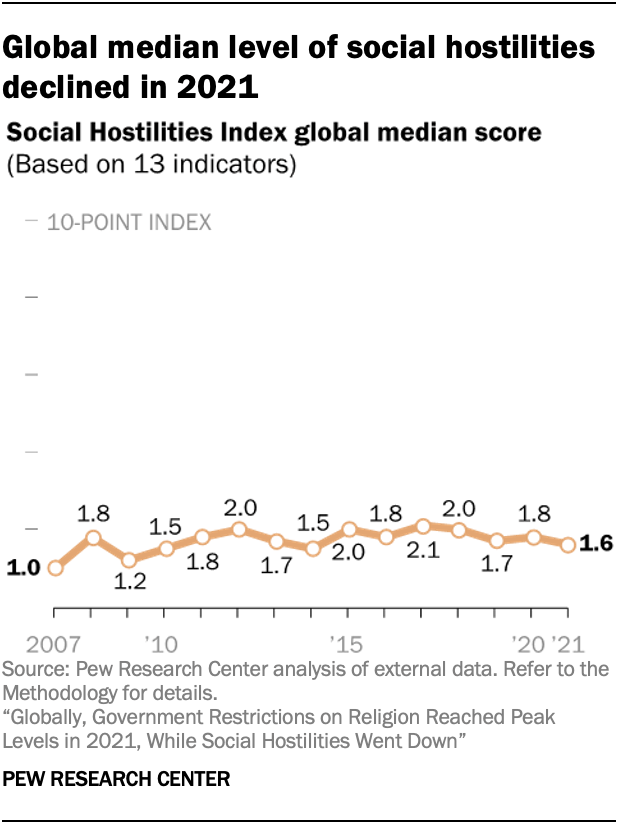
In Nigeria, for example, “intercommunal clashes” took place between predominantly Christian farmers and Muslim herders, driven largely by competition for land and natural resources. Both groups have formed armed factions. In August 2021, ethnic Irigwe Christians in Nigeria reportedly killed 27 people and injured 14 when they attacked five buses transporting Muslims across Plateau State, in the North Central part of the country. The following month, Muslim herdsmen reportedly killed 49 people and kidnapped 27 – mostly Christians – in the neighboring state of Kaduna.
Nigeria also experienced continued violence by Boko Haram and Islamic State militants throughout 2021.
Governments in 161 countries provided benefits to religious groups in 2021, even as government officials in most of these countries also carried out harassment (149 countries) or interfered in worship (134 countries). A new feature of our latest report is an attempt to count how many governments provide benefits to religious groups while, at the same time, harassing religious groups or interfering in their worship.
One of the most common benefits that governments provide is support for religious education. We identified 127 countries that provided this kind of support in 2021, as well as 107 that supported religious properties (such as funds to build or maintain houses of worship) and 67 that provided benefits to clergy (such as payment of salaries).
For example, in Saudi Arabia, where Sunni Muslims comprise the majority of the population, the government funds the construction of most Sunni mosques and gives a monthly stipend to imams. At the same time, the country’s Ministry of Islamic Affairs prescribes a list of approved themes for sermons in mosques and forbids messages that are deemed “sectarian, political, or extremist,” according to the U.S. State Department. Clerics also have been targeted when their religious views go against what is considered acceptable by the government. In 2021, a Sunni cleric named Hassan Farhan al-Maliki remained in prison without due process for “allegedly calling into question the fundamentals of Islam,” according to the U.S. Commission on International Religious Freedom. The cleric has been in prison since 2017.
For more information on government restrictions and social hostilities involving religion in other countries, read the full report here .
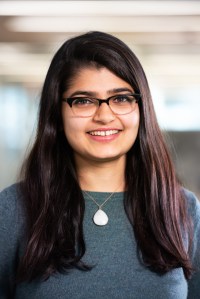
Sign up for our weekly newsletter
Fresh data delivered Saturday mornings
8 in 10 Americans Say Religion Is Losing Influence in Public Life
Globally, government restrictions on religion reached peak levels in 2021, while social hostilities went down, religious restrictions around the world, china’s christian population appears to have stopped growing after rising rapidly in the 1980s and ’90s, most popular.
About Pew Research Center Pew Research Center is a nonpartisan fact tank that informs the public about the issues, attitudes and trends shaping the world. It conducts public opinion polling, demographic research, media content analysis and other empirical social science research. Pew Research Center does not take policy positions. It is a subsidiary of The Pew Charitable Trusts .
- Today's news
- Reviews and deals
- Climate change
- 2024 election
- Fall allergies
- Health news
- Mental health
- Sexual health
- Family health
- So mini ways
- Unapologetically
- Buying guides
Entertainment
- How to Watch
- My watchlist
- Stock market
- Biden economy
- Personal finance
- Stocks: most active
- Stocks: gainers
- Stocks: losers
- Trending tickers
- World indices
- US Treasury bonds
- Top mutual funds
- Highest open interest
- Highest implied volatility
- Currency converter
- Basic materials
- Communication services
- Consumer cyclical
- Consumer defensive
- Financial services
- Industrials
- Real estate
- Mutual funds
- Credit cards
- Credit card rates
- Balance transfer credit cards
- Business credit cards
- Cash back credit cards
- Rewards credit cards
- Travel credit cards
- Checking accounts
- Online checking accounts
- High-yield savings accounts
- Money market accounts
- Personal loans
- Student loans
- Car insurance
- Home buying
- Options pit
- Investment ideas
- Research reports
- Fantasy football
- Pro Pick 'Em
- College Pick 'Em
- Fantasy baseball
- Fantasy hockey
- Fantasy basketball
- Download the app
- Daily fantasy
- Scores and schedules
- GameChannel
- World Baseball Classic
- Premier League
- CONCACAF League
- Champions League
- Motorsports
- Horse racing
- Newsletters
New on Yahoo
- Privacy Dashboard
Scotland's papers: PM coup rumours and Police hate crime test
More from scotland's papers.
The Scotsman
Daily Record
The Scottish Sun
Scottish Daily Express
The Telegraph
The National
The Courier
The P&J
Glasgow Times
Edinburgh News
Recommended Stories
Get up to 42 percent off blink security cameras during the amazon big spring sale.
Amazon is running a sale on its Blink security cameras as part of its Big Spring Sale.
The 30+ best tech deals of the week: save hundreds on headphones, TVs, laptops and so much more
How does $100 off an incredible pair of noise cancelling headphones sound? Here's a hint: like blissful silence.
Watch Disney's official trailer for Star Wars: The Acolyte
In Stars Wars: The Acolyte, a Jedi Master and his former padawan reunite to investigate a series of crimes. Disney+ has released its official trailer to let you know what you can expect.
From WeightWatchers to medication, Oprah Winfrey's always been candid about her body. Here's what she says about her weight loss journey in a new special.
Winfrey discusses modern weight loss medications like Ozempic in the ABC special "Shame, Blame and the Weight Loss Revolution."
March Madness: A breakdown of Houston and the South Region from an odds perspective
Can Houston win the first men's basketball title in school history?
Netflix's 'Receiver' series to follow five NFL players, including George Kittle, Justin Jefferson, Deebo Samuel
The eight-episode Netflix series follow last year's "Quarterback," which documented the 2022 seasons of Kirk Cousins, Patrick Mahomes, and Marcus Mariota.
March Madness: Ranking the NCAA men's tournament teams from 1 to 68
UConn is the favorite, but there are at least eight others who are legit contenders to cut down the nets in April.
This $16 battery organizer and tester means 'no more searching in the junk drawer'
Neaten up a chaotic cabinet or closet with this clear storage case, a handy sidekick for your gizmos and gadgets.
This Shark self-emptying robot vacuum is $300 off for the Amazon Big Spring Sale
A Shark self-emptying robot vacuum is currently $300 off for the Amazon Big Spring Sale.
NFL free agency: Best remaining players on market and more news, updates
Follow all the developments right here with Yahoo Sports.
How Honda hedged its bet on the hydrogen fuel cell-powered CR-V e:FCEV
Just like battery-electric cars 20 years ago, hydrogen fuel cell cars suffer from the old chicken and the egg problem. Nobody wants to buy a fuel cell vehicle until the supporting infrastructure is in place, but it's tough to invest in infrastructure when nobody owns a fuel cell vehicle. Honda says it's playing the long game with a lofty goal in mind.
Do you need a neti pot — and is it safe to use? Here's what experts say.
The CDC has linked a dangerous infection to neti pots. Here's how to use one safely to clear up your sinuses.
ASUS Zenbook Duo review: The first dual-screen laptop worth buying
Starting at $1,500 and with dimensions that aren’t much bigger than a traditional clamshell, ASUS’ Zenbook Duo is the first dual-screen laptop you’d actually want to buy.
Ethos Fund closes first fund to invest in founders bridging U.S. and Vietnamese tech ecosystems
Yi and Shin started Ethos Fund two years ago to bridge opportunities and startup communities between Vietnam and the United States. Yi and Shin are working with companies in two ways: in the U.S. to invest in what they call “third culture founders,” which they define as “brilliant minds who don't just belong to one culture but have been shaped by multiple ones,” solving problems they care about.
Uber leads $100M investment in African mobility fintech Moove as valuation hits $750M
Moove, an African mobility fintech that offers vehicle financing to ride-hailing and delivery app drivers, has raised $100 million in a funding round as it plots expansion into new markets. Moove did not say who is leading the round, but sources close to the deal confirmed to TechCrunch that Uber led the Series B round, making it the company's first investment in the African continent. The round also includes sovereign wealth fund Mubadala and several other investors, pushing Moove’s post-money valuation to $750 million.
Report: Knicks' OG Anunoby sidelined indefinitely with right elbow injury after surgery
OG Anunoby is out due to a post-surgical flare-up in his right elbow, and it’s unclear when he’ll be able to return.
LACERA decreases venture capital allocation range, but experts say it doesn't signal a trend
The Los Angeles County Employees Retirement Association (LACERA) voted to decrease its allocation range to venture capital at a March 13 meeting. The board of investments voted to decrease its allocation range to venture capital and growth equity from between 15% and 30% of the pension system's private equity portfolio, to between 5% and 25%. LACERA's venture portfolio is currently 10.8% of the PE portfolio.
Pistons' Evan Fournier fined $25K for kicking ball after Heat star Bam Adebayo’s buzzer-beater
Evan Fournier was not happy after the Heat pulled off a 104-101 win on Saturday in Detroit.
'Super-fast startup and lots of storage': This bestselling laptop is a steal at $210 — that's over 50% off
The compact computer from a little-known brand holds its own against the big names, shoppers say.
The NFL will never see another player like Aaron Donald again
What made Donald so great? It's not a short answer.
Scotland's papers: PM coup rumours and Police hate crime test
More from scotland's papers.
- The Scotsman
- Daily Record
- The Scottish Sun
- Scottish Daily Express
- The Telegraph
- The National
- The Courier
- The P&J
- Glasgow Times
- Edinburgh News
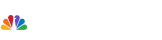
How to get live election results in the 2024 Illinois primary
Track election results as they roll in from around the state tuesday, published march 18, 2024 • updated 2 hours ago.
Illinois' 2024 primary election is here, and thousands of residents are casting their ballots in several contests.
If you want to track election results as they roll in from around the state Tuesday, you can find the latest vote totals on NBCChicago.com and on the NBC Chicago app once the polls close . At that time, a "live election results" page will be available to track vote totals as they are counted.
Voters looking for live updates can download the NBC Chicago app for tha latest, but make sure to turn on push notifications so you can be the first to know who won some of the biggest races in the Chicago area and Illinois .
You'll also find live election coverage airing on NBC 5's streaming channel from 7-10 p.m. and at 10 p.m. on Channel 5, as well as on NBCChicago.com and the NBC Chicago app.
Feeling out of the loop? We'll catch you up on the Chicago news you need to know. Sign up for the weekly Chicago Catch-Up newsletter here.
When is the 2024 primary election in Illinois ?
The 2024 primary election in Illinois will take place on Tuesday, March 19.
Early voting remains ongoing at sites throughout the Chicago area. You can find more information on those sites here.
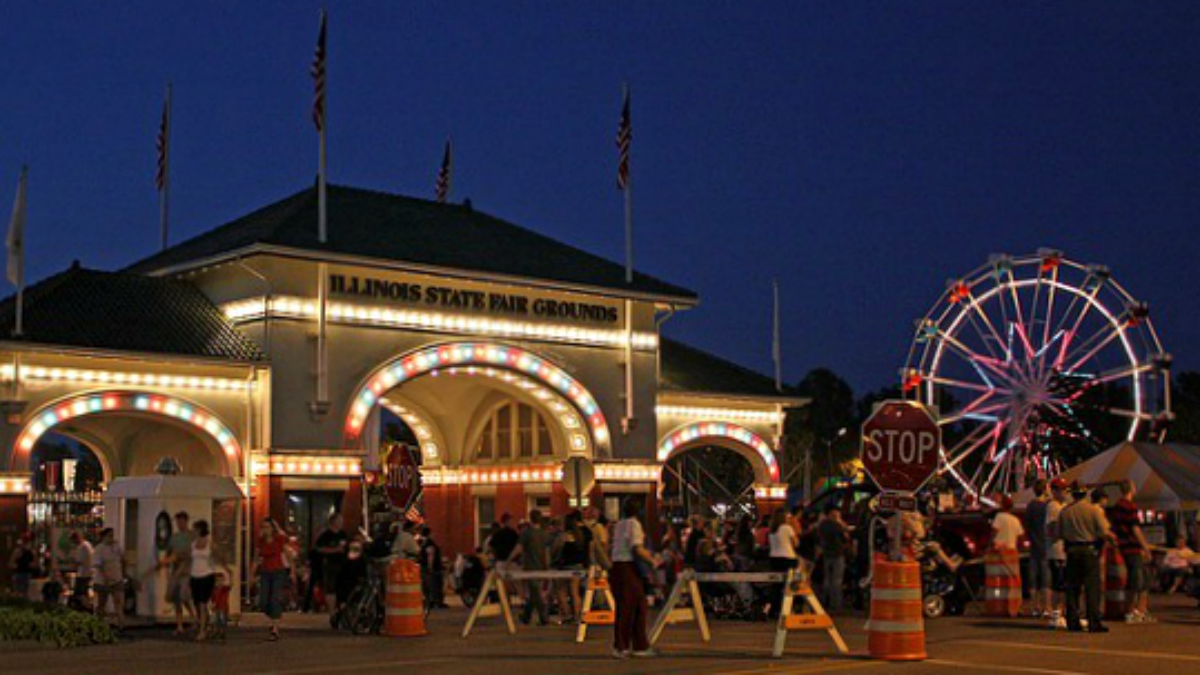
Illinois State Fair adds Miranda Lambert to star-studded 2024 grandstand lineup
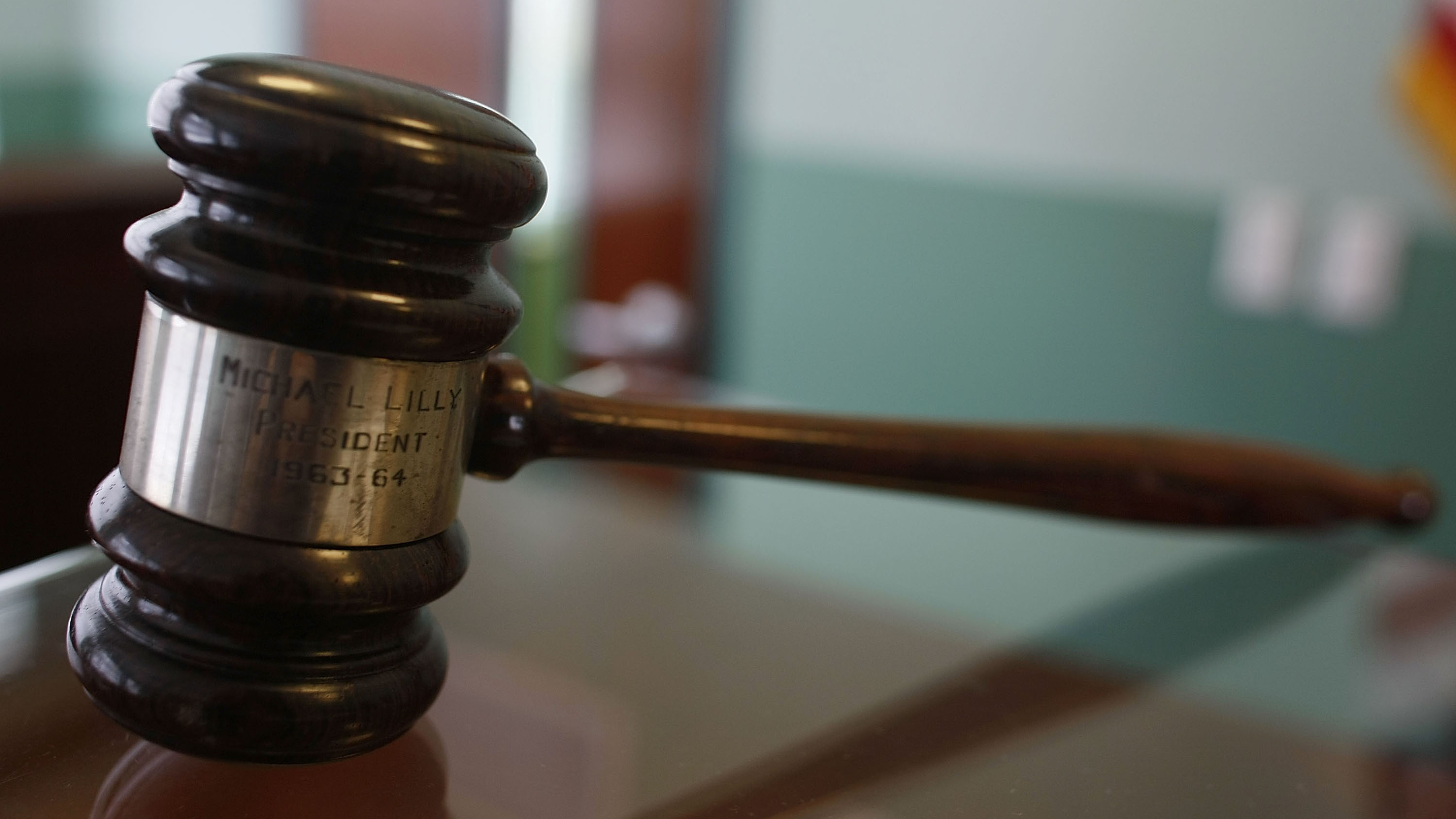
How to research the judges on your 2024 Illinois primary ballot
Registering to vote in illinois.
Voters in Illinois who haven't registered yet can still take advantage of grace-period and same-day voter registration .
Residents seeking to register to vote must:
- Be a United States citizen
- Must be 18 years of age or older on Election Day
- Must live in their precinct for at least 30 days prior to an election
- Must not be serving a prison sentence as a result of a criminal conviction
- May not claim the right to vote anywhere else
What to bring to vote in-person
If you are already registered to vote at your current address, you will not need to bring a government-issued photo ID to vote, but it could be helpful if any questions arise about your registration, address or signature.
If you are registering to vote on the day of the election, or need to make changes to your registration such as a new address or a legally-changed name, you can do so, but will need two forms of ID.
Finally, voters are allowed to bring notes into the booth with information on which candidates they’ll be voting for.
Is it too late to vote by mail?
For the 2024 primary election, yes.
However, any registered voter in the state of Illinois can cast their ballot via the mail for any reason.
Voters must have apply for mail-in ballots by submitting forms to their local election authority. Those forms can be submitted online in some areas, while in others they can be downloaded and either mailed in or submitted in person.
The deadline to request a mail-in ballot for the Illinois Primary was March 14.
Ballots can be returned to your local election authority, or can be submitted via a secure drop box. A full list of drop boxes can be found on the State Board of Elections’ website .
Ballots can also be returned via the mail, but most be postmarked by March 19. Ballots must arrive at the local election authority no later than April 2 to be counted.
Find a polling place near you
You can find your nearest polling place by entering your zip code here .
Who’s on the ballot in Illinois
In the 2024 primary, Illinois voters will choose their preferred nominees in all of the races, including the presidential race, which will appear at the top of ballots.
Voters will also choose their preferred presidential delegates, who will formally cast votes on behalf of their candidate during the Republican and Democratic National Conventions later this year.
Outside of the presidency, voters will also choose nominees for the November election for their respective members of Congress, with all 17 members of Illinois’ Congressional delegation facing reelection this year.
All 118 members of Illinois’ House of Representatives will be up for re-election in 2024, as will approximately one-third of the state’s 59 senators.
MORE: 10 races to watch in the 2024 Illinois primary election
Some districts will also have primary votes on Supreme Court justices, with elections in the first and fourth districts. Justices in those races are running to fill full 10-year terms on the court.
Voters will also determine nominees for appellate court, circuit courts and subcircuit courts when they cast their ballots.
Finally, some counties will also have countywide races to decide in 2024.
Sample ballots can be found on the local election authority’s website in your area.
Referendums on your ballot
Whether you live in Chicago or the suburbs, there are plenty of communities wrestling with thorny questions. Here's a preview of what you can expect on your ballot, depending on where you live.
MORE: What is the ‘Bring Chicago Home' Referendum, and is it still on your ballot?
How to research judges
Judges are elected from specific districts or circuits in the state of Illinois, so the judges that will appear on your ballot will be different depending on where you live.
The Illinois Supreme Court has seven total seats, including three from the first district, which represents Cook County.
In this year’s election cycle, there will be an election in that First District, as Justice Joy Cunningham will run for election to a full 10-year term after replacing former Chief Justice Ann Burke in Dec. 2022.
There is also an election in the fourth district, as Justice Lisa Holder White will run for a full 10-year term on the bench after replacing former Justice Rita Garman.
The fourth district covers a slew of counties in western and central Illinois, including Ogle, Lee, Peoria and Sangamon counties.
There are numerous other vacancies in the Appellate Court, including four in the First District, and at least 11 vacancies in the Cook County circuit court, among others.
Numerous organizations offer recommendations on judges. Here's a guide to help you navigate the process .
Can I take a ballot selfie?
Taking photos with your ballot is prohibited by state law, as it’s a felony to mark your ballot so another person can see how you voted.
Granted, state election officials have said it’s “unlikely” anyone would be prosecuted for such a photo, but taking a picture with a sticker or your polling place is far safer.
What should I do if I run into any problems voting?
Voters who are challenged on their voting eligibility, who experience voter intimidation or have trouble at their polling place are encouraged to report such incidents.
If you run into any of the above issues, or have any questions about the voting process, more than 100 organizations across the U.S. operate an Election Protection hotline, which can be reached at:
1-866-OUR-VOTE (1-866-687-8683): English
1-888-VE-Y-VOTA (1-888-839-8682): Spanish/English
1-888-API-VOTE (1-888-274-8683): Asian languages/English
1-844-YALLA-US (1-844-925-5287): Arabic/English
The Lawyers’ Committee for Civil Rights Under Law is one of the organizations that helps operate the hotline during election season.
Residents can also text MYVOTE to 866-687-8683 to get help from trained volunteers. Assistance is also available on Twitter or Facebook Messenger at @866ourvote, and live-chatting can be accessed on the group's website.
This article tagged under:
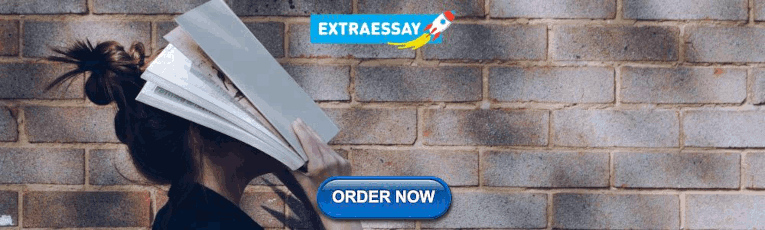
IMAGES
COMMENTS
Hate crimes, often referred to as bias-motivated crimes, have garnered greater public attention and concern as political rhetoric in the United States and internationally has promoted the exclusion of people based on their group identity. This review examines what we know about the trends in hate crime behavior and the legal responses to this problem across four main domains. First, we ...
Chakraborti and Garland (Citation 2015) argue that even Perry's more expansive definition of hate crime is limited, and propose what they refer to as a simpler "and in some ways broader" definition in their research, that is by defining hate crimes as "acts of violence, hostility and intimidation directed towards people because of their ...
September 13, 2021. Hate crimes (also known as "bias crimes") are recognized as a distinct category of crimes that have a broader effect than most other kinds of crimes because the victims are not only the crime's immediate target but also others like them. The FBI defines hate crimes as "criminal offense [s] against a person or ...
1. Perpetrators of hate crimes are not always motivated by a single type of prejudice or hatred but can be influenced by a combination of different prejudices. 2. There is no single type of hate crime perpetrator. Research shows that in order to fully understand the nature of hate crime, practitioners need to
A 2020 study found experiences of hate are associated with poor emotional wellbeing such as feelings of anger and shame. Victims tend to experience poor mental health, including depression, anxiety, and suicidal behavior. Some research also points to the finding that the experience of hate-motivated behavior can result in blaming of and lower ...
This article argues that hate crimes are not a modern-day phenomenon, but extend throughout the history of the United States. ... Journal of Research in Crime and Delinquency, 31, 202-209. Google Scholar. ... G. L. (1969). The beginnings of partible inheritance in the American colonies. In D. H. Flaherty (Ed.), Essays in the history of early ...
Research also suggested that hate crimes against LGBT people disproportionately occurred in public spaces like streets, parking garages, ... Research hypotheses. This paper presents the first examination of the personal violent hate crime experiences of LGBT persons in a large probability sample of US residents. Given the prior literature, we ...
The author of four books and more than 100 essays, his research spans a wide array of topics: hate crime, prejudice, voting behavior, partisanship, and campaign finance. He has also written extensively on social science research methods and is co-author of the textbook Field Experiments: Design, Analysis, and Interpretation (W.W. Norton Press ...
Previous research has identified numerous barriers to reporting hate crimes. However, high variability exists in the outcome measures considered across multiple studies, including whether hate crimes encompass non-criminal behaviours, whether victims' perceptions are considered bias indicators, and whether the incident is reported to police or to other organisations. These inconsistencies ...
Using 1992-2014 data from the National Incident-Based Reporting System (NIBRS), the present study examines the nature and characteristics of hate crimes against Asian Americans by comparing them with those of hate crimes against African Americans and Hispanics. Minority-general and minority-specific models are proposed to guide the analysis.
HATE CRIME: An Emergent Research Agenda Donald P. Green1, Laurence H. McFalls2, and Jennifer K. Smith1 ... When hate crime is defined to encompass only those acts entirely motivated by animus, crimes such as robbery and rape are presumably excluded from the defi-nition. When animus need be just one of several motives, however, the definition
Since then, there has been much debate about hate crime, which has recently lead to a new field of research in some countries referred to as "hate studies" (Chakraborti & Garland, 2015). Hate crimes are based on stereotypes, prejudice, or extreme negative sentiments about certain groups, and generally also targeted at visible social groups ...
This paper positions anti-Asian hate crime during the COVID-19 pandemic historically and in relation to racist and xenophobic othering. We expand scholarship through examining the pandemic-driven hate crime experiences of Asian Americans to more definitively define the concept of othering. ... Research also suggests that hate crime victims ...
Abstract. This systematic review aimed to explore the research papers related to how Internet and social media may, or may not, constitute an opportunity to online hate speech. 67 studies out of 2389 papers found in the searches, were eligible for analysis. We included articles that addressed online hate speech or cyberhate between 2015 and 2019.
Hate Crimes and Ethnoviolence by Howard J. Ehrlich Over the past twenty years, Howard J. Ehrlich conducted the first national surveys of ethnoviolence, helped design the protocol for identifying hate crimes, and has served as the director of The Prejudice Institute. This collection of essays is the result of his unparalleled research in this ...
In this paper, we study hate speech with respect to a ... J., Ghose, A. & Seamans, R. The internet and racial hate crime: Offline spillovers from online access. ... (research core funding no. P2 ...
Once hate crime statistics became more readily available in the 1990s, social science research examined over-time aggregate relationships and found that racially motivated crimes coincide with ...
focus areas that these awards addressed were: (1) hate crime measurement; (2) victimization research with specific, localized populations of interest; (3) exploring the use of non-traditional data sources to examine trends and predict hate crimes; and (4) identifying the characteristics of hate crime offenders.
The FBI notes in a December 2014 report: " Of the reported 3,407 single-bias hate crime offenses that were racially motivated, 66.4 percent were motivated by anti-black or African-American bias, and 21.4 percent stemmed from anti-white bias.". It remains the case that African-Americans are the victims of bias-motivated crimes at much higher ...
Many of the most notorious hate crimes have been murders, such as the racially motivated murder of James Byrd, Jr., in Texas in 1998 or the homophobicmotivated murder of Matthew Shepard in North Dakota later that same year. The vast majority of hate crimes, however, are cases of assault or vandalism.
Date created: August 2021. A call for papers from APA's Journal of Rural Mental Health for manuscripts that focuses on hate crimes and domestic terrorism in rural areas. Submissions will be accepted on a rolling basis and reviewed by experts in the field.
The Post's analysis of FBI data found that the per capita hate crime rates on K-12 and college campuses were higher in the more liberal states that have not enacted laws limiting transgender rights.
Since October 2023, Toronto has witnessed 203 confirmed hate crimes, a 93% rise from a year ago, the statement added. Demkiw said hate crime calls dropped in December and January, but then picked ...
The project received ethical approval from the university ethics board in 2015 to carry out qualitative hate crime research across these strands. In total, 32 semi-structured interviews were conducted, with anonymity via pseudonyms being provided for all participants. ... Whilst it is not the intention of the paper to delve in the debates ...
Our latest study, the 14th in this annual series, covers events that took place in 198 countries and territories in 2021.Here are the key findings: Government restrictions on religion reached a new high in 2021. Globally, the median score on our 10-point Government Restrictions Index rose from 2.8 in 2020 to 3.0 in 2021 - the highest level recorded since we began tracking this in 2007.
Threats of a coup in the Tory party and police training for new hate crime laws make the front pages. ... Research reports ; Webinars ; Crypto; Industries; Sports. Fantasy. News ; Fantasy football ; Best Ball ; ... Scotland's papers: PM coup rumours and Police hate crime test. BBC. March 18, 2024 at 12:31 AM. Link Copied. Read full article ...
Threats of a coup in the Tory party and police training for new hate crime laws make the front pages.
Vandalism at downtown Chicago mosque investigated as possible hate crime Registering to vote in Illinois Voters in Illinois who haven't registered yet can still take advantage of grace-period and ...