
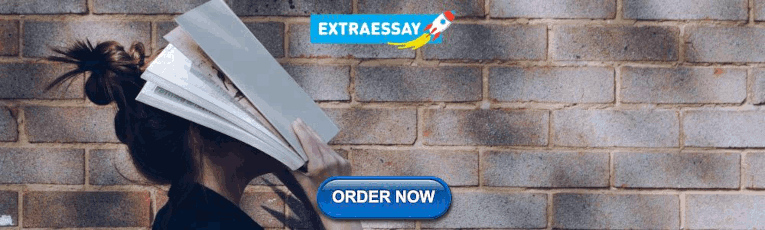
Why Is Quality Control in Research So Important?
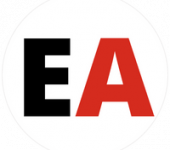
Quality control can mean the difference between good science and bad science. So, what is quality control? Very simply, it is any process that aims to monitor and maintain laboratory standards.
The processes of quality control can include detecting, reducing, and correcting any problems within a lab. Quality control can also help to make sure that the results of an experiment or method are consistent. Quality control is known as quality assurance or quality management.
Good Laboratory Practice (GLP) is one form of quality control. GLP was introduced in the field of chemical research, to try to ensure high quality, reliable test data. This was a good starting point. However, it was not perfect.
What is the Best Type of Quality Control?
The best practice for quality control is a Laboratory Quality Management (LQM) program. The design of LQM helps control factors that cause variation in a lab. By doing this, an LQM can increase the researcher’s confidence in the results of their experiments.
An LQM has six vital parts:
- Quality manual
- Labs should have a manual that describes its quality control systems in detail.
- Staff and training
- Training for all staff should be thorough and consistent.
3. Methodology
- The method must be consistent throughout the lab. They should be validated to check that they are precise and accurate.
- In-house reference materials
- This means that labs can have their control samples for standard methods. These samples will have known properties. They can be used to check that methods are working correctly.
- Record keeping
- Write down the preparation, procedure, and analysis of the experiment
- Cost vs. benefits
- The prices of setting up an LQM should be low. Benefits include confidence in results, fewer problems, and lower costs.
Tackling Problems in Quality Control
In a Nature article, a scientist describes her experiences with quality control. Rebecca Davies manages quality control at her lab for a long time. Although her task was huge, she soon became hooked on finding and fixing problems.
Once she started looking, Davies found several problems. These ranged from issues with sample storage to issues in data collection. She also discovered faulty equipment and spotted missing controls. However, these problems did not put Davies off. Instead, she realized how much the lab’s work could improve.
In 2009, Davies set up a group called Quality Central. The group helps several research labs to design proper quality control systems. Along with some other scientists, Davies believes in “voluntary” quality assurance (QA). Voluntary QA does not force quality control through regulation. Instead, it helps scientists to strengthen their research with QA.
What Happens when Quality Control is Poor
Scientific rigor is a hot topic. Over the last few years, several issues in science have caused both researchers and the public to question the scientific process. For example, some studies have found that as few as one-third of scientific papers are reproduced . Peer review and plagiarism have also been a problem. Among researchers, there is a general opinion that publications are of more value than the science itself.
Many scientists are cautious in their work. However, some are careless. Failing to record data, writing reports months after the experiment and not using controls are just a few examples. Each of these may seem like a small problem. But together, they can lead to studies that cannot be reproduced.
We can fix a lot of issues with better quality control. Unfortunately, many labs still carry out quality control in a casual, ad hoc way.
Barriers to Good Quality Control
In many labs, quality control is not seen as the best use of resources. With limited funds, other things can take priority. Some scientists explain that a lack of quality control is due to both lack of funds and lack of staff.
When Davies first set up Quality Central, she found that other researchers at her college were not interested. They thought that it was not essential, and so was a waste of time and money.
However, one scientist was interested. The researcher had used another lab’s equipment, but the results seemed odd. She discovered that to save money, the PI of the lab had not been maintaining the equipment. Equipment maintenance is one of the things that a good quality control program should check
The Benefits of Great Quality Control
Thanks to the efforts of Davies and others, researchers are starting to understand the benefits of quality control.
Quality control does not need to be complicated. Here is one example. Notebooks checking is a weekly task in a laboratory. To make sure this is fair, each member of the lab draws a name from a paper bag to decide whose notebook they will check. Notebooks include factors such as whether control was used, how and where data was recorded, and which equipment was used. Any previous problems should be fixed. This is a tremendous low-tech quality control system.
Some PI’s with large labs find it hard to check everyone’s work. It can be challenging to track samples, data, and equipment. One solution is a tracking system. This gives tracking numbers to every sample or data record. PI’s can then easily follow the progress of a study.
Often, researchers only realize the benefits of quality control when problems occur. Unexpected results can mean searching through stacks of data to try to find the cause. With reasonable quality control, this should be a rare event. Moreover, if it does happen, the reason should be easy to find.
Do you want to learn more about running a lab? Alternatively, finding and fixing problems? Why not start with this Enago article on GLP.
What are the quality control systems in your lab? Has your work ever suffered from poor quality control? Do you have any suggestions for improvement? Share your ideas in the comments below.
Rate this article Cancel Reply
Your email address will not be published.
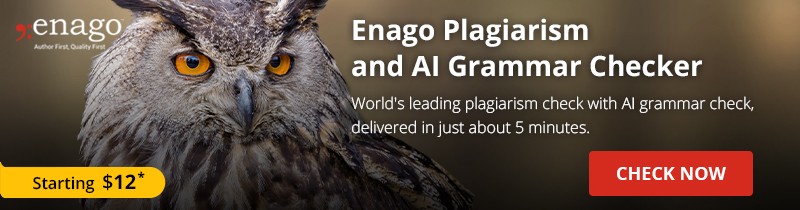
Enago Academy's Most Popular Articles
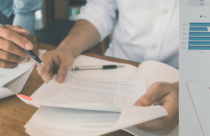
- Reporting Research
Choosing the Right Analytical Approach: Thematic analysis vs. content analysis for data interpretation
In research, choosing the right approach to understand data is crucial for deriving meaningful insights.…
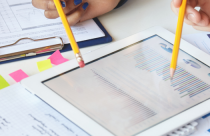
Comparing Cross Sectional and Longitudinal Studies: 5 steps for choosing the right approach
The process of choosing the right research design can put ourselves at the crossroads of…
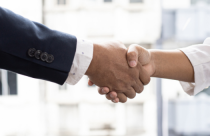
- Career Corner
Unlocking the Power of Networking in Academic Conferences
Embarking on your first academic conference experience? Fear not, we got you covered! Academic conferences…
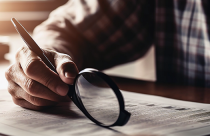
Research Recommendations – Guiding policy-makers for evidence-based decision making
Research recommendations play a crucial role in guiding scholars and researchers toward fruitful avenues of…
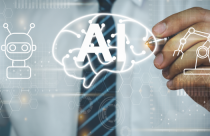
- AI in Academia
Disclosing the Use of Generative AI: Best practices for authors in manuscript preparation
The rapid proliferation of generative and other AI-based tools in research writing has ignited an…
Intersectionality in Academia: Dealing with diverse perspectives
Meritocracy and Diversity in Science: Increasing inclusivity in STEM education
Avoiding the AI Trap: Pitfalls of relying on ChatGPT for PhD applications

Sign-up to read more
Subscribe for free to get unrestricted access to all our resources on research writing and academic publishing including:
- 2000+ blog articles
- 50+ Webinars
- 10+ Expert podcasts
- 50+ Infographics
- 10+ Checklists
- Research Guides
We hate spam too. We promise to protect your privacy and never spam you.
I am looking for Editing/ Proofreading services for my manuscript Tentative date of next journal submission:
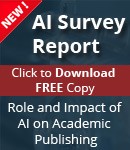
What should universities' stance be on AI tools in research and academic writing?

An official website of the United States government
The .gov means it’s official. Federal government websites often end in .gov or .mil. Before sharing sensitive information, make sure you’re on a federal government site.
The site is secure. The https:// ensures that you are connecting to the official website and that any information you provide is encrypted and transmitted securely.
- Publications
- Account settings
Preview improvements coming to the PMC website in October 2024. Learn More or Try it out now .
- Advanced Search
- Journal List
- Glob J Qual Saf Healthc
- v.5(2); 2022 May
- PMC10229003

How to Build and Assess the Quality of Healthcare-Related Research Questions
Sergio ramón gutiérrez ubeda.
1 Center of Investigation and Study of Health, National Autonomous University of Nicaragua, Managua, Nicaragua
The objective of this article is to describe a simplified process for building and assessing the quality of healthcare-related research questions. This process consisted of three stages. The first stage aimed to select and explore a field of science. This field would be the area for which to identify outputs, such as units of analysis, variables, and objectives. The second stage aimed to write structured research questions, taking into account the outputs of the first stage. In general, the structure of research questions starts with interrogative adverbs (e.g., what and when ), auxiliary verbs (e.g., is there and are there ), or other auxiliaries (e.g., do , does , and did ); followed by nouns nominalized from verbs of research objectives, such as association , correlation , influence , causation , prediction , application ; research variables (e.g., risk factors, efficiency, effectiveness, and safety); and units of analysis (e.g., patients with hypertension and general hospitals). The third stage aimed to assess the quality and feasibility of the research questions against a set of criteria such as relevance, originality, generalizability, measurability, communicability, availability of resources, and ethical issues. By following the proposed simplified process, novice researchers may learn how to write structured research questions of sound scientific value.
INTRODUCTION
A critical factor for the success of research projects is selecting research questions of good quality.[ 1 , 2 ] Research questions are meant to address knowledge gaps and generate hypotheses (e.g., unknown and controversial knowledge). Research projects answer such questions through the application of the scientific method.
Novice researchers who are inexperienced and untrained in the steps and procedures of the scientific method may not know how to develop a research question of high value in an efficient manner.[ 1 , 3 ]. Even if novice researchers try to self-educate themselves on the processes of identifying research questions, they might fail to learn well because of the large body of literature published on the subject. The task of building research questions is time-consuming and challenging.
The objective of this article is to provide a simplified process aimed to build and assess the quality of healthcare-related research questions. The process is explained in three stages, step by step, for novice researchers who will design and conduct research projects on their own and publish their results in peer-reviewed journals. An overview of the process is illustrated in Figure 1 .

Overview of process to build and assess the quality of healthcare-related research questions.
STAGE 1: SELECTING AND EXPLORING A SCIENCE FIELD
Step 1: selecting a science field.
The task of step 1 is to select one field of science to study. A science field, simply defined, is a subject area targeted for research purposes by scientific communities. Classifications of science fields are potential sources for identifying such fields. For example, the Qatar National Research Foundation[ 4 ] classifies science into broad fields such as natural sciences, engineering and technology, medical and health sciences, agricultural sciences, social sciences, and humanities. They further classify such broad fields into fields of intermediate size. For example, they classify the medical and health sciences field into health sciences, basic medicine, clinical medicine, medical biotechnology, and other medical sciences. Finally, they classify intermediate size fields into smaller fields. For example, they classify the health science field into hospital administration, health policy, nursing, nutrition, dietetics, public health, environmental health, tropical medicine, epidemiology, occupational health, social biomedical health, and so forth.
Whether you select the science field provided by someone else or from your own knowledge, look for evidence (e.g., articles published in peer-reviewed journals) supporting its scientific legitimacy. Often, this evidence is easy to find because scientists and editors from research centers and scientific journals publish definitions and descriptions of their fields of interest on web pages, in journal articles,[ 5 ] and on research agendas.[ 2 ] If such evidence is difficult to find, then try an internet keyword query, such as the following. First, identify the keywords or phrases that you believe are names of science fields. Then, if necessary, combine your keywords or phrases with one or more of the following terms: science , research , research agenda , research center , research at World Health Organization , international forum , and journal . Second, add quotation marks around keywords or phrases and enter them into an internet search engine. Finally, review a few (e.g., 10) of the first hits of the search engine results pages, especially those that match the query phrase, looking for evidence indicating the legitimacy of the science field. For example, I identified the keywords healthcare quality and combined it with the word journal into healthcare quality journal . Then I added quotation marks in the Google search engine. The search produced 13,800 pages. I found a peer-reviewed journal on healthcare quality among the first 10 pages. With this evidence, I felt confident about the legitimacy of the science field named healthcare quality . If there are various science fields of interest, prioritize one that you believe has social relevance, feasibility (e.g., technical, material, financial), and a low risk of ethical issues for research purposes.
Step 2: Identifying units of analysis (UAs)
The two tasks of step 2 are, first, to identify, from the perspective of the science field selected at step 1, potential UAs of interest for scientific inquiry, and second, to select one or a few of them. The UA is a definable and measurable entity, either natural or social, for which projects seek to answer research questions. There are two main purposes for developing a list of UAs. The first purpose is to promote a novice researcher's awareness that within a science field there are several types of UAs. In medicine, for example, this awareness would help the researcher to consider not only patients but also other types of UAs for research projects. The second purpose is to raise awareness of the possibility of building research questions for studies that target not only one but two or more UAs, as in multilevel analysis studies. Four tips on how to develop the list of UAs are given below.
The first tip is to identify UAs based on published lists or classification systems of UAs. For example, one published list of UAs includes control projects; single patients; single clinicians; clinics; populations of patients in nursing homes, hospital wards, microsystems, or entire hospitals.[ 6 ] Babbie[ 7 ] classifies UAs into five categories: individuals, groups, organizations, social interactions, and social artifacts. Lofland[ 8 ] classifies UAs for social science fields in cultural practices, episodes, encounters, roles, social and personal relationships, groups and cliques, organizations, settlements and habitats, and subcultures and lifestyles. Table 1 lists UAs related to the field healthcare quality.
Example units of analysis for the field of healthcare quality
Content based on Babbie.[ 7 ]
The second tip is to use specific phrases to distinguish between UAs. For example, the phrase individual humans includes all humans, but the phrase human with diabetes type I above 40 years old is specific enough to include only humans who meet those criteria. Other examples of criteria to distinguish between groups of humans would include a specific condition or disease such as hypertension, diabetes, dengue; behaviors such as physical activity, diet, sexual practices; functions and capabilities such as attention, memory, and judgment; and sociodemographic characteristics such as age, ethnicity, religion, and marital status.
The third tip is to list several UAs that are homogeneous, especially when there is interest in analyzing consolidated data, as in quantitative studies. A hint to help understand the homogeneous population UA would be to examine the standard “production” process of each unit. On one hand, UAs such as goods (e.g., vaccines) mass produced with standardized industrial processes are quite homogenous. On the other hand, UAs such as social constructs (e.g., neighborhoods) are usually not homogenous because they grow spontaneously by social processes, often with few limitations and flexible constraints. Neighborhoods, for example, might vary in size, social composition, economic status, and so on, even neighborhoods that have grown in the same city.
The fourth tip is to include UAs that represent single, small, and large natural or social entities to build research questions for qualitative and quantitative studies. For example, the World Health Organization is a single entity, the number of continents in the world would be a small number, whereas the number of cities in the world would be a large number. In quantitative studies, the inferential power of statistical analysis of a small sample of UAs may be compromised.
The second part of step 2 is selecting one or a few UAs to focus on developing in step 3, which is to list variables related to each UA. Prioritize UAs of social relevance, feasibility (e.g., technical and financial), and low risk of ethical issues for research purposes.
Step 3: Identifying research variables and phenomena
The task of step 3 is to create a list of variables and phenomena related to each UA selected in step 2. Variables are measurable features of UAs whose values would vary depending on changing situations. Phenomena describe the meaning, interpretation, or explanation related to variables of the UA.[ 9 , 10 ]
At this step, researchers should be aware of the broad array of variables and phenomena related to each UA. A research project might be conducted with one, two, or more variables, depending on what research questions the project seeks to answer. With a longer list of variables, there are more possibilities to build univariate, bivariate, and multivariate research-related questions. Table 2 illustrates an example of UAs and variables related to the field of healthcare quality. Below are three tips on how to develop a list of variables and phenomena.
Example units of analysis and variables for the field of healthcare quality
PSC: patient safety culture.
The first tip is to search variables and phenomena, one by one, through an extensive literature search for relevant scientific publications. During your search, keep in mind that publications of quantitative approaches often use the term variable , but publications of qualitative approaches might use other terms (e.g., phenomena ).[ 9 , 10 ] This difference might be because “qualitative research aims to understand the how and why of certain behaviors, decisions, and individual experiences”[ 9 ] and such understanding is not measured numerically.
The second tip is to identify a useful framework to classify different variables. For example, Lofland's[ 8 ] typology of questions might help to classify variables and phenomena by type, frequency, magnitude, structure, process, cause, consequence, and agency. Another classification, promoted from the field of quality management systems,[ 11 ] includes six categories of variables: physical (e.g., physical activity, safety of drugs, effectiveness of surgical procedures); sensory (e.g., vision, smell, hearing); behavioral (e.g., courtesy, honesty, veracity); temporal (e.g., punctuality, reliability, availability); ergonomic (e.g., physiological characteristics or related to human safety); and functional (e.g., speed of emergency care).
The third tip is to consider each variable, either simple or complex, as one unit. Simple variables can be measured with just one indicator (i.e., age, sex). Complex variables are usually divided into several dimensions (e.g., patient safety culture may be divided into teamwork and communication), each of which might be measured with multiple indicators. If a researcher adds complex variables and their dimensions as separate units in the same list, the total number of variables becomes inflated, which may overcomplicate the analysis.
Step 4: Identifying possible research objectives
The task of step 4 is to develop a list of possible objectives of interest for the research project. A research objective is the expected outcome that a research project seeks to achieve. Research objectives clarify whether a project seeks to describe one or more variables and phenomena or to study how two or more variables relate to each other (e.g., exposure, intervention, mediating, and outcome variables).
Objectives and research questions are closely related. Thus, developing a list of objectives would help develop a list of research questions. Objectives are often classified into generic categories, such as exploratory, descriptive, correlational, explicative, predictive, and applicative. Exploratory objectives aim to discover, understand, and characterize phenomena and their interactions with other phenomena. Descriptive objectives aim to count frequencies of variables without making comparisons. Correlational objectives aim to assess how one or more variables behave while interacting with each other. Explicative objectives aim to study cause and effect relationships among variables. Predictive objectives aim to forecast the behavior of one variable through understanding the behavior of other variables. Applicative objectives aim to study the effectiveness of interventions on changing other variables' status and behaviors. Table 3 illustrates an example of a UAs with variables, objectives, and research questions related to healthcare quality.
Examples of research questions related to hospitals (UA) and healthcare quality (science field)
UA: units of analysis; PSC: patient safety culture.
STAGE 2: WRITING STRUCTURED RESEARCH QUESTIONS
The task of the second stage is to build a list of possible research questions for the project. A research question is “a logical statement that progresses from what is known or believed to be true to that which is unknown and requires validation.”[ 12 ] A research question clarifies the specific knowledge that a research project expects to discover through the study of one or a few variables or phenomena related to one or a few UAs.
In general, the structure of research questions would include interrogative adverbs (e.g., what, why, who, when, and where), auxiliary verbs (e.g., is there and are there), or other auxiliaries (e.g., do, does, and did); nouns nominalized from verbs of research objectives, such as association, correlation, causation, prediction, and application; research variables (e.g., incidence, prevalence, risk factors, causes, effects, and interventions); and UAs (e.g., patients with hypertension, mothers, pregnant women with diabetes, and general hospitals). Research questions related to prognosis and relationships between interventions and outcomes would be structured in formats such as those outlined in Table 4 .[ 1 , 3 , 9 , 10 ]
Examples of framework used to write research questions
Content based on Fandino,[ 1 ] Thabane et al,[ 3 ] Cooke et al,[ 9 ] and Cañón and Buitrago-Gómez.[ 10 ]
To write structured research questions, use the outputs of the first stage (e.g., UA list, variables, and objectives) and general or specific formats for structuring research questions. Table 3 includes examples of research questions.
STAGE 3: ASSESSING AND SELECTING RESEARCH QUESTIONS
Step 1: assessing the quality and feasibility of a research question.
The task of this step is to assess the quality of the research question against a set of criteria, such as those shown in Figure 2 . A more detailed discussion on such criteria is provided elsewhere.[ 2 , 3 , 13 , 14 ] Other criteria to assess the quality of research questions include answerability, effectiveness, innovativeness, implementation, burden reduction, and equity.,[ 2 ] Researchers may also use criteria based on the acronyms, FINER (feasible, interesting, novel, ethical, and relevant)[ 1 , 3 ] and I-SMART (important, specific, measurable, achievable, relevant, and timely).[ 14 ] Rank the quality and feasibility of the criteria using a scale of 1–10 points (i.e., 10 being highest quality and 1 being lowest). Remember to ensure that each criterion is scored using the same scale. Finally, calculate the total score for the research question.

Examples of criteria used to assess the quality of research questions.
Step 2: Selecting the research question
Select the research questions that score highest in the quality assessment from the previous step. Avoid selecting high-risk ethical questions, regardless of other criteria that had high scores. Finally, if answering a research question is not feasible (e.g., too expensive or complex) with the available resources, then that question might be kept on standby until the circumstances for feasibility improve.
Building a research question of high quality just by guessing is unlikely, especially for novice researchers who do not have any training in the scientific method.
The proposed simplified process aimed to help novice researchers write structured, high quality research questions. The key concepts and framework of the scientific method presented illustrate the myriad of questions that researchers can develop in any field of science. The importance of using a well-known set of criteria to assess the quality of research questions was highlighted. This simplified process teaches novice researchers how to build research questions of sound scientific value in a systematic way.
Funding Statement
Source of Support: None.
Conflict of interest: None.
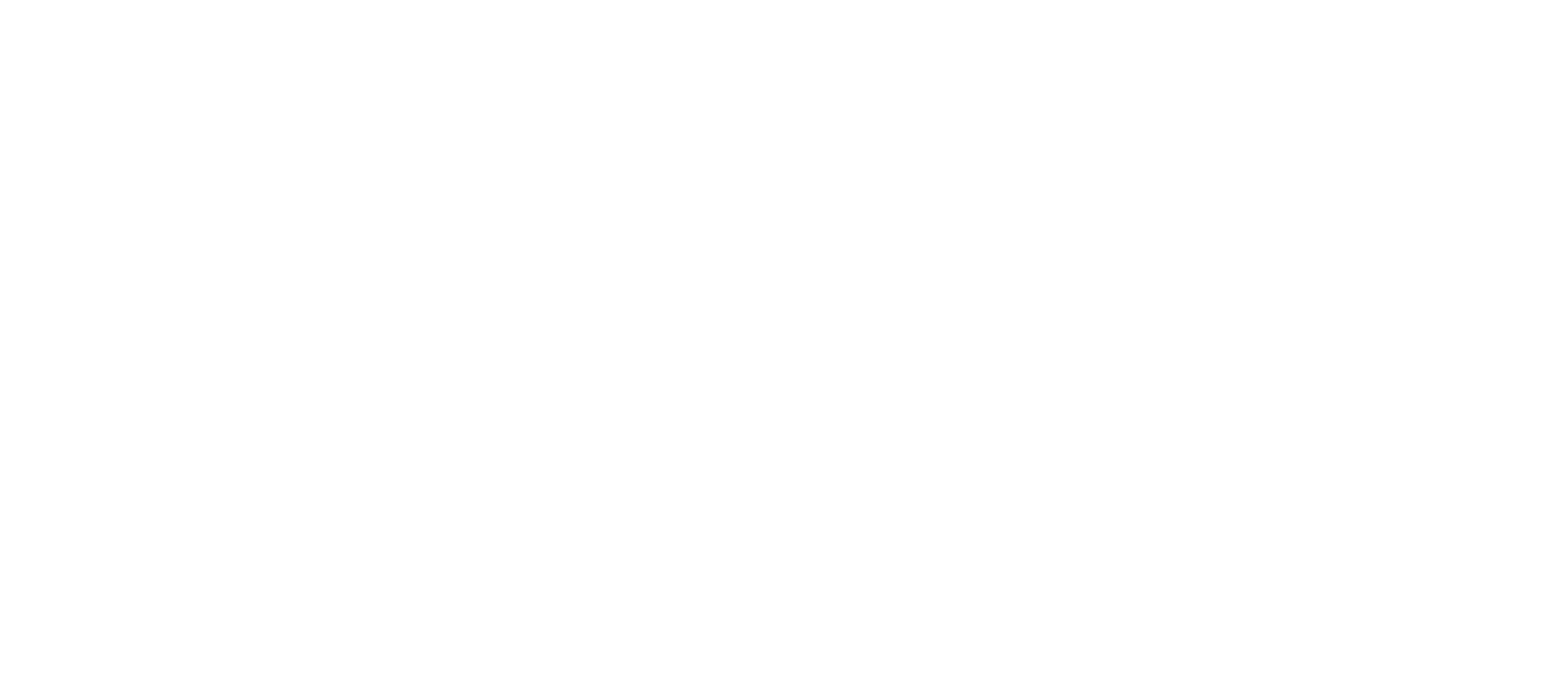
- Previous Article
- Next Article
INTRODUCTION
Stage 1: selecting and exploring a science field, stage 2: writing structured research questions, stage 3: assessing and selecting research questions, how to build and assess the quality of healthcare-related research questions.

- Split-Screen
- Article contents
- Figures & tables
- Supplementary Data
- Peer Review
- Open the PDF for in another window
- Guest Access
- Get Permissions
- Cite Icon Cite
- Search Site
Sergio Ramón Gutiérrez Ubeda; How to Build and Assess the Quality of Healthcare-Related Research Questions. Global Journal on Quality and Safety in Healthcare 1 May 2022; 5 (2): 39–43. doi: https://doi.org/10.36401/JQSH-21-17
Download citation file:
- Ris (Zotero)
- Reference Manager
The objective of this article is to describe a simplified process for building and assessing the quality of healthcare-related research questions. This process consisted of three stages. The first stage aimed to select and explore a field of science. This field would be the area for which to identify outputs, such as units of analysis, variables, and objectives. The second stage aimed to write structured research questions, taking into account the outputs of the first stage. In general, the structure of research questions starts with interrogative adverbs (e.g., what and when ), auxiliary verbs (e.g., is there and are there ), or other auxiliaries (e.g., do , does , and did ); followed by nouns nominalized from verbs of research objectives, such as association , correlation , influence , causation , prediction , application ; research variables (e.g., risk factors, efficiency, effectiveness, and safety); and units of analysis (e.g., patients with hypertension and general hospitals). The third stage aimed to assess the quality and feasibility of the research questions against a set of criteria such as relevance, originality, generalizability, measurability, communicability, availability of resources, and ethical issues. By following the proposed simplified process, novice researchers may learn how to write structured research questions of sound scientific value.
A critical factor for the success of research projects is selecting research questions of good quality. [ 1 , 2 ] Research questions are meant to address knowledge gaps and generate hypotheses (e.g., unknown and controversial knowledge). Research projects answer such questions through the application of the scientific method.
Novice researchers who are inexperienced and untrained in the steps and procedures of the scientific method may not know how to develop a research question of high value in an efficient manner. [ 1 , 3 ] . Even if novice researchers try to self-educate themselves on the processes of identifying research questions, they might fail to learn well because of the large body of literature published on the subject. The task of building research questions is time-consuming and challenging.
The objective of this article is to provide a simplified process aimed to build and assess the quality of healthcare-related research questions. The process is explained in three stages, step by step, for novice researchers who will design and conduct research projects on their own and publish their results in peer-reviewed journals. An overview of the process is illustrated in Figure 1 .

Overview of process to build and assess the quality of healthcare-related research questions.
Step 1: Selecting a science field
The task of step 1 is to select one field of science to study. A science field, simply defined, is a subject area targeted for research purposes by scientific communities. Classifications of science fields are potential sources for identifying such fields. For example, the Qatar National Research Foundation [ 4 ] classifies science into broad fields such as natural sciences, engineering and technology, medical and health sciences, agricultural sciences, social sciences, and humanities. They further classify such broad fields into fields of intermediate size. For example, they classify the medical and health sciences field into health sciences, basic medicine, clinical medicine, medical biotechnology, and other medical sciences. Finally, they classify intermediate size fields into smaller fields. For example, they classify the health science field into hospital administration, health policy, nursing, nutrition, dietetics, public health, environmental health, tropical medicine, epidemiology, occupational health, social biomedical health, and so forth.
Whether you select the science field provided by someone else or from your own knowledge, look for evidence (e.g., articles published in peer-reviewed journals) supporting its scientific legitimacy. Often, this evidence is easy to find because scientists and editors from research centers and scientific journals publish definitions and descriptions of their fields of interest on web pages, in journal articles, [ 5 ] and on research agendas. [ 2 ] If such evidence is difficult to find, then try an internet keyword query, such as the following. First, identify the keywords or phrases that you believe are names of science fields. Then, if necessary, combine your keywords or phrases with one or more of the following terms: science , research , research agenda , research center , research at World Health Organization , international forum , and journal . Second, add quotation marks around keywords or phrases and enter them into an internet search engine. Finally, review a few (e.g., 10) of the first hits of the search engine results pages, especially those that match the query phrase, looking for evidence indicating the legitimacy of the science field. For example, I identified the keywords healthcare quality and combined it with the word journal into healthcare quality journal . Then I added quotation marks in the Google search engine. The search produced 13,800 pages. I found a peer-reviewed journal on healthcare quality among the first 10 pages. With this evidence, I felt confident about the legitimacy of the science field named healthcare quality . If there are various science fields of interest, prioritize one that you believe has social relevance, feasibility (e.g., technical, material, financial), and a low risk of ethical issues for research purposes.
Step 2: Identifying units of analysis (UAs)
The two tasks of step 2 are, first, to identify, from the perspective of the science field selected at step 1, potential UAs of interest for scientific inquiry, and second, to select one or a few of them. The UA is a definable and measurable entity, either natural or social, for which projects seek to answer research questions. There are two main purposes for developing a list of UAs. The first purpose is to promote a novice researcher's awareness that within a science field there are several types of UAs. In medicine, for example, this awareness would help the researcher to consider not only patients but also other types of UAs for research projects. The second purpose is to raise awareness of the possibility of building research questions for studies that target not only one but two or more UAs, as in multilevel analysis studies. Four tips on how to develop the list of UAs are given below.
The first tip is to identify UAs based on published lists or classification systems of UAs. For example, one published list of UAs includes control projects; single patients; single clinicians; clinics; populations of patients in nursing homes, hospital wards, microsystems, or entire hospitals. [ 6 ] Babbie [ 7 ] classifies UAs into five categories: individuals, groups, organizations, social interactions, and social artifacts. Lofland [ 8 ] classifies UAs for social science fields in cultural practices, episodes, encounters, roles, social and personal relationships, groups and cliques, organizations, settlements and habitats, and subcultures and lifestyles. Table 1 lists UAs related to the field healthcare quality.
Example units of analysis for the field of healthcare quality

The second tip is to use specific phrases to distinguish between UAs. For example, the phrase individual humans includes all humans, but the phrase human with diabetes type I above 40 years old is specific enough to include only humans who meet those criteria. Other examples of criteria to distinguish between groups of humans would include a specific condition or disease such as hypertension, diabetes, dengue; behaviors such as physical activity, diet, sexual practices; functions and capabilities such as attention, memory, and judgment; and sociodemographic characteristics such as age, ethnicity, religion, and marital status.
The third tip is to list several UAs that are homogeneous, especially when there is interest in analyzing consolidated data, as in quantitative studies. A hint to help understand the homogeneous population UA would be to examine the standard “production” process of each unit. On one hand, UAs such as goods (e.g., vaccines) mass produced with standardized industrial processes are quite homogenous. On the other hand, UAs such as social constructs (e.g., neighborhoods) are usually not homogenous because they grow spontaneously by social processes, often with few limitations and flexible constraints. Neighborhoods, for example, might vary in size, social composition, economic status, and so on, even neighborhoods that have grown in the same city.
The fourth tip is to include UAs that represent single, small, and large natural or social entities to build research questions for qualitative and quantitative studies. For example, the World Health Organization is a single entity, the number of continents in the world would be a small number, whereas the number of cities in the world would be a large number. In quantitative studies, the inferential power of statistical analysis of a small sample of UAs may be compromised.
The second part of step 2 is selecting one or a few UAs to focus on developing in step 3, which is to list variables related to each UA. Prioritize UAs of social relevance, feasibility (e.g., technical and financial), and low risk of ethical issues for research purposes.
Step 3: Identifying research variables and phenomena
The task of step 3 is to create a list of variables and phenomena related to each UA selected in step 2. Variables are measurable features of UAs whose values would vary depending on changing situations. Phenomena describe the meaning, interpretation, or explanation related to variables of the UA. [ 9 , 10 ]
At this step, researchers should be aware of the broad array of variables and phenomena related to each UA. A research project might be conducted with one, two, or more variables, depending on what research questions the project seeks to answer. With a longer list of variables, there are more possibilities to build univariate, bivariate, and multivariate research-related questions. Table 2 illustrates an example of UAs and variables related to the field of healthcare quality. Below are three tips on how to develop a list of variables and phenomena.
Example units of analysis and variables for the field of healthcare quality

The first tip is to search variables and phenomena, one by one, through an extensive literature search for relevant scientific publications. During your search, keep in mind that publications of quantitative approaches often use the term variable , but publications of qualitative approaches might use other terms (e.g., phenomena ). [ 9 , 10 ] This difference might be because “qualitative research aims to understand the how and why of certain behaviors, decisions, and individual experiences” [ 9 ] and such understanding is not measured numerically.
The second tip is to identify a useful framework to classify different variables. For example, Lofland's [ 8 ] typology of questions might help to classify variables and phenomena by type, frequency, magnitude, structure, process, cause, consequence, and agency. Another classification, promoted from the field of quality management systems, [ 11 ] includes six categories of variables: physical (e.g., physical activity, safety of drugs, effectiveness of surgical procedures); sensory (e.g., vision, smell, hearing); behavioral (e.g., courtesy, honesty, veracity); temporal (e.g., punctuality, reliability, availability); ergonomic (e.g., physiological characteristics or related to human safety); and functional (e.g., speed of emergency care).
The third tip is to consider each variable, either simple or complex, as one unit. Simple variables can be measured with just one indicator (i.e., age, sex). Complex variables are usually divided into several dimensions (e.g., patient safety culture may be divided into teamwork and communication), each of which might be measured with multiple indicators. If a researcher adds complex variables and their dimensions as separate units in the same list, the total number of variables becomes inflated, which may overcomplicate the analysis.
Step 4: Identifying possible research objectives
The task of step 4 is to develop a list of possible objectives of interest for the research project. A research objective is the expected outcome that a research project seeks to achieve. Research objectives clarify whether a project seeks to describe one or more variables and phenomena or to study how two or more variables relate to each other (e.g., exposure, intervention, mediating, and outcome variables).
Objectives and research questions are closely related. Thus, developing a list of objectives would help develop a list of research questions. Objectives are often classified into generic categories, such as exploratory, descriptive, correlational, explicative, predictive, and applicative. Exploratory objectives aim to discover, understand, and characterize phenomena and their interactions with other phenomena. Descriptive objectives aim to count frequencies of variables without making comparisons. Correlational objectives aim to assess how one or more variables behave while interacting with each other. Explicative objectives aim to study cause and effect relationships among variables. Predictive objectives aim to forecast the behavior of one variable through understanding the behavior of other variables. Applicative objectives aim to study the effectiveness of interventions on changing other variables' status and behaviors. Table 3 illustrates an example of a UAs with variables, objectives, and research questions related to healthcare quality.
Examples of research questions related to hospitals (UA) and healthcare quality (science field)

The task of the second stage is to build a list of possible research questions for the project. A research question is “a logical statement that progresses from what is known or believed to be true to that which is unknown and requires validation.” [ 12 ] A research question clarifies the specific knowledge that a research project expects to discover through the study of one or a few variables or phenomena related to one or a few UAs.
In general, the structure of research questions would include interrogative adverbs (e.g., what, why, who, when, and where), auxiliary verbs (e.g., is there and are there), or other auxiliaries (e.g., do, does, and did); nouns nominalized from verbs of research objectives, such as association, correlation, causation, prediction, and application; research variables (e.g., incidence, prevalence, risk factors, causes, effects, and interventions); and UAs (e.g., patients with hypertension, mothers, pregnant women with diabetes, and general hospitals). Research questions related to prognosis and relationships between interventions and outcomes would be structured in formats such as those outlined in Table 4 . [ 1 , 3 , 9 , 10 ]
Examples of framework used to write research questions

To write structured research questions, use the outputs of the first stage (e.g., UA list, variables, and objectives) and general or specific formats for structuring research questions. Table 3 includes examples of research questions.
Step 1: Assessing the quality and feasibility of a research question
The task of this step is to assess the quality of the research question against a set of criteria, such as those shown in Figure 2 . A more detailed discussion on such criteria is provided elsewhere. [ 2 , 3 , 13 , 14 ] Other criteria to assess the quality of research questions include answerability, effectiveness, innovativeness, implementation, burden reduction, and equity., [ 2 ] Researchers may also use criteria based on the acronyms, FINER (feasible, interesting, novel, ethical, and relevant) [ 1 , 3 ] and I-SMART (important, specific, measurable, achievable, relevant, and timely). [ 14 ] Rank the quality and feasibility of the criteria using a scale of 1–10 points (i.e., 10 being highest quality and 1 being lowest). Remember to ensure that each criterion is scored using the same scale. Finally, calculate the total score for the research question.

Examples of criteria used to assess the quality of research questions.
Step 2: Selecting the research question
Select the research questions that score highest in the quality assessment from the previous step. Avoid selecting high-risk ethical questions, regardless of other criteria that had high scores. Finally, if answering a research question is not feasible (e.g., too expensive or complex) with the available resources, then that question might be kept on standby until the circumstances for feasibility improve.
Building a research question of high quality just by guessing is unlikely, especially for novice researchers who do not have any training in the scientific method.
The proposed simplified process aimed to help novice researchers write structured, high quality research questions. The key concepts and framework of the scientific method presented illustrate the myriad of questions that researchers can develop in any field of science. The importance of using a well-known set of criteria to assess the quality of research questions was highlighted. This simplified process teaches novice researchers how to build research questions of sound scientific value in a systematic way.
Source of Support: None. Conflict of interest: None.
Recipient(s) will receive an email with a link to 'How to Build and Assess the Quality of Healthcare-Related Research Questions' and will not need an account to access the content.
Subject: How to Build and Assess the Quality of Healthcare-Related Research Questions
(Optional message may have a maximum of 1000 characters.)

Citing articles via
Get email alerts.
- Browse Journal
- For Authors
- For Reviewers
- About The Journal
- Industry News and Events
Affiliations
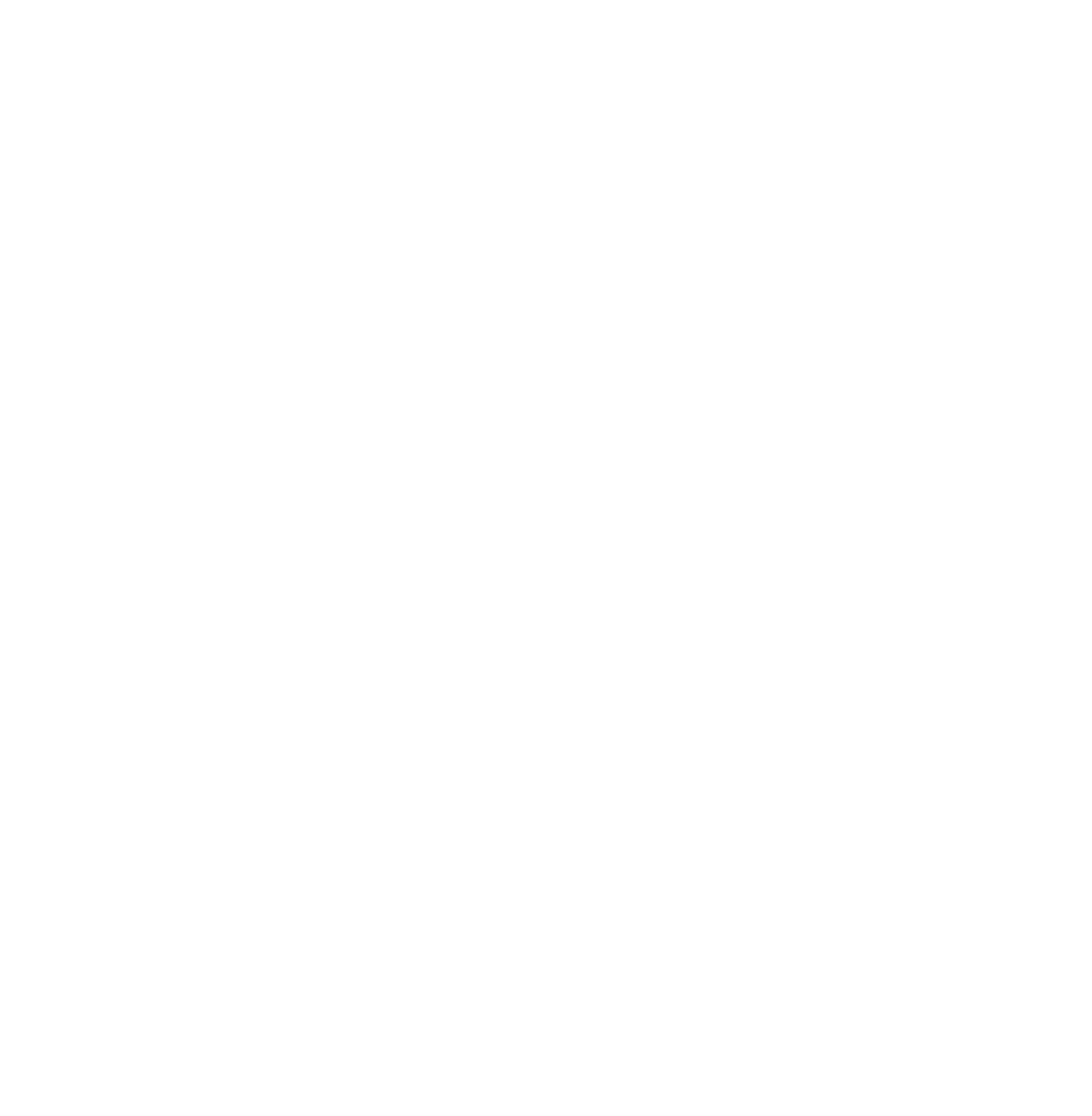
- eISSN 2589-9449
- ISSN 2666-2353
- Privacy Policy
- Get Adobe Acrobat Reader
This Feature Is Available To Subscribers Only
Sign In or Create an Account
Quality Control and Good Epidemiological Practice
- Reference work entry
- Cite this reference work entry
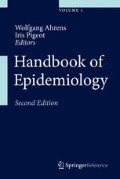
- Gila Neta 3 ,
- Jonathan M. Samet 4 &
- Preetha Rajaraman 5
11k Accesses
3 Citations
The use of data is fundamental in epidemiology. Epidemiological research on causation uses data in a search for the true nature of the relationship between exposure and disease. Increasingly, research with biomarkers of susceptibility, exposure, and response seeks to characterize mechanisms of disease causation. Research on the consequences of interventions seeks an unbiased assessment of the effects of independently varying factors on the outcome measure(s).
- Quality Assurance
- Study Personnel
- Multiple Risk Factor Intervention Trial
- Master File
These keywords were added by machine and not by the authors. This process is experimental and the keywords may be updated as the learning algorithm improves.
This is a preview of subscription content, log in via an institution to check access.
Access this chapter
- Available as PDF
- Read on any device
- Instant download
- Own it forever
- Available as EPUB and PDF
- Durable hardcover edition
- Dispatched in 3 to 5 business days
- Free shipping worldwide - see info
Tax calculation will be finalised at checkout
Purchases are for personal use only
Institutional subscriptions
Aanensen DM, Huntley DM, Feil EJ, al-Own F, Spratt BG (2009) EpiCollect: linking smartphones to web applications for epidemiology, ecology and community data collection. PLoS One 4:e6968
Google Scholar
Agresti A (1990) Categorical data analysis. Wiley, Hoboken
Altman D, Bland J (1983) Measurement in medicine: the analysis of method comparison studies. Statistician 32:307–317
American Association for Public Opinion Research (2000) Standard definitions. Final dispositions of case codes and outcome rates for surveys. American Association for Public Opinion Research, Ann Arbor
Arts DG, De Keizer NF, Scheffer GJ (2002) Defining and improving data quality in medical registries: a literature review, case study, and generic framework. J Am Med Inform Assoc 9:600–611
PubMed PubMed Central Google Scholar
Asch DA, Jedrziewski MK, Christakis NA (1997) Response rates to mail surveys published in medical journals. J Clin Epidemiol 50:1129–1136
PubMed CAS Google Scholar
Asch DA, Christakis NA, Ubel PA (1998) Conducting physician mail surveys on a limited budget. A randomized trial comparing $2 bill versus $5 bill incentives. Med Care 36:95–99
Ascherio A, Stampfer MJ, Colditz GA, Rimm EB, Litin L, Willett WC (1992) Correlations of vitamin A and E intakes with the plasma concentrations of carotenoids and tocopherols among American men and women. J Nutr 122:1792–1801
Bachmann J (2007) Will the circle be unbroken: a history of the U.S. National Ambient Air quality standards. J Air Waste Manag Assoc 57:652–697
Baer A, Saroiu S, Koutsky LA (2002) Obtaining sensitive data through the web: an example of design and methods. Epidemiology 13:640–645
PubMed Google Scholar
Barnett V, Lewis T (1994) Outliers in statistical data. Wiley, Hoboken
Berkanovic E (1980) The effect of inadequate language translation on Hispanics’ responses to health surveys. Am J Public Health 70:1273–1276
PubMed PubMed Central CAS Google Scholar
Bisgard KM, Folsom AR, Hong CP, Sellers TA (1994) Mortality and cancer rates in nonrespondents to a prospective study of older women: 5-year follow-up. Am J Epidemiol 139:990–1000
Blackmore CC, Richardson ML, Linnau KF, Schwed AM, Lomoschitz FM, Escobedo EM, Hunter JC, Jurkovich GJ, Cummings P (2003) Web-based image review and data acquisition for multiinstitutional research. AJR Am J Roentgenol 180:1243–1246
Blomgren KJ, Sundström A, Steineck G, Wiholm BE (2006) Interviewer variability – quality aspects in a case-control study. Eur J Epidemiol 21:267–277
Bray F, Parkin DM (2009) Evaluation of data quality in the cancer registry: principles and methods. Part I: comparability, validity and timeliness. Eur J Cancer 45:747–755
Brenner H, Gefeller O (1997) Variation of sensitivity, specificity, likelihood ratios and predictive values with disease prevalence. Stat Med 16:981–991
Breslow N, Day N (1980) Statistical methods in cancer research. Volume I – the analysis of case-control studies. International Agency for Research on Cancer, Lyon
Breslow N, Day N (1987) Statistical methods in cancer research. Volume II – the design and analysis of cohort studies. International Agency for Research on Cancer, Lyon
Bryant AH, Reinert A (2001) Epidemiology in the legal arena and the search for truth. Am J Epidemiol 154(Suppl 12):S27–S35
Canner PL, Krol WF, Forman SA (1983) The coronary drug project. External quality control programs. Control Clin Trials 4:441–466
Canner PL, Borhani NO, Oberman A, Cutler J, Prineas RJ, Langford H, Hooper FJ (1991) The hypertension prevention trial: assessment of the quality of blood pressure measurements. Am J Epidemiol 134:379–392
Centers for Disease Control and Prevention (CDC) (1999) BRFSS summary quality control report. Centers for Disease Control and Prevention, Atlanta
Cherrie J, Schneider T (1998) Validation of a new method for structured subjective assessment of past concentrations. Ann Occup Hyg 43:235–245
Cherrie J, Krantz S, Schneider T, Ohberg I, Kamstrup O, Linander W (1987) An experimental simulation of an early rock woollag wool production process. Ann Occup Hyg 31:583–593
Choi BC, Pak AW, Purdham JT (1990) Effects of mailing strategies on response rate, response time, and cost in a questionnaire study among nurses. Epidemiology 1:72–74
Christiansen DH, Hosking JD, Dannenberg AL, Williams OD (1990) Computer-assisted data collection in multicenter epidemiologic research. The atherosclerosis risk in communities study. Control Clin Trials 11:101–115
Cicchetti DV, Feinstein AR (1990) High agreement but low kappa: II. Resolving the paradoxes. J Clin Epidemiol 43:551–558
Clement DL, De Buyzere ML, De Bacquer DA, de Leeuw PW, Duprez DA, Fagard RH, Gheeraert PJ, Missault LH, Braun JJ, Six RO, Van Der Niepen P, O’Brien E, Office versus Ambulatory Pressure Study Investigators (2003) Prognostic value of ambulatory blood-pressure recordings in patients with treated hypertension. N Engl J Med 348:2407–2415
Clive RE, Ocwieja KM, Kamell L, Hoyler SS, Seiffert JE, Young JL, Henson DE, Winchester DP, Osteen RT, Menck HR, Fremgen A (1995) A national quality improvement effort: cancer registry data. J Surg Oncol 58:155–161
Cohen J (1968) Weighted kappa: nominal scale agreement with provision for scaled disagreement or partial credit. Psychol Bull 70:213–220
Collins RL, Ellickson PL, Hays RD, McCaffrey DF (2000) Effects of incentive size and timing on response rates to a follow-up wave of a longitudinal mailed survey. Eval Rev 24:347–363
Comstock GW, Tockman MS, Helsing KJ, Hennesy KM (1979) Standardized respiratory questionnaires: comparison of the old with the new. Am Rev Respir Dis 119:45–53
Cook RR (1991) Overview of good epidemiologic practices. J Occup Med 33:1216–1220
Cooper GR (1986) The importance of quality control in the multiple risk factor intervention trial. Control Clin Trials 7:3
Cottler LB, Zipp JF, Robins LN, Spitznagel EL (1987) Difficult-to-recruit respondents and their effect on prevalence estimates in an epidemiologic survey. Am J Epidemiol 125:329–339
Craig CL, Marshall AL, Sjöström M, Bauman AE, Booth ML, Ainsworth BE, Pratt M, Ekelund U, Yngve A, Sallis JF, Oja P (2003) International physical activity questionnaire: 12-country reliability and validity. Med Sci Sports Exerc 35:1381–1395
Crombie IK, Irving JM (1986) An investigation of data entry methods with a personal computer. Comput Biomed Res 19:543–550
Data Quality Act of 2000, Public Law 106–554, 114 STAT. 2763, 21 December 2000, Section 515a
Dawber TR, Meadors GF, Moore FE Jr (1951) Epidemiological approaches to heart disease: the Framingham study. Am J Public Health 41:279–286
CAS Google Scholar
Day S, Fayers P, Harvey D (1998) Double data entry: what value, what price? Control Clin Trials 19:15–24
Deyo RA, Diehr P, Patrick DL (1991) Reproducibility and responsiveness of health status measures. Statistics and strategies for evaluation. Control Clin Trials 12(Suppl 4):142S–158S
Dillman D (1978) Mail and telephone surveys: the total design method. Wiley, New York
Dischinger P, DuChene AG (1986) Quality control aspects of blood pressure measurements in the multiple risk factor intervention trial. Control Clin Trials 7(Suppl 3):S137–S157
Doody MM, Sigurdson AS, Kampa D, Chimes K, Alexander BH, Ron E, Tarone RE, Linet MS (2003) Randomized trial of financial incentives and delivery methods for improving response to a mailed questionnaire. Am J Epidemiol 157:643–651
Dosemeci M, Rothman N, Yin SN, Li GL, Linet M, Wacholder S, Chow WH, Hayes RB (1997) Validation of benzene exposure assessment. Ann N Y Acad Sci 837:114–121
DuChene AG, Hultgren DH, Neaton JD, Grambsch PV, Broste SK, Aus BM, Rasmussen WL (1986) Forms control and error detection procedures used at the coordinating center of the multiple risk factor intervention trial (MRFIT). Control Clin Trials 7(Suppl 3):S34–S45
Eaker S, Bergström R, Bergström A, Adami HO, Nyren O (1998) Response rate to mailed epidemiologic questionnaires: a population-based randomized trial of variations in design and mailing routines. Am J Epidemiol 147:74–82
Easterbrook PJ, Berlin JA, Gopalan R, Matthews DR (1991) Publication bias in clinical research. Lancet 337:867–872
Edwards S, Slattery ML, Mori M, Berry TD, Caan BJ, Palmer P, Potter JD (1994) Objective system for interviewer performance evaluation for use in epidemiologic studies. Am J Epidemiol 140:1020–1028
Edwards SL, Slattery ML, Ma KN (1998) Measurement errors stemming from nonrespondents present at in-person interviews. Ann Epidemiol 8:272–277
Edwards P, Roberts I, Clarke M, DiGuiseppi C, Pratap S, Wentz R, Kwan I, Cooper R (2007) Methods to increase response rates to postal questionnaires. Cochrane Database Syst Rev(2):MR000008
Egger M, Smith GD (1998) Bias in location and selection of studies. BMJ 316:61–66
Etminan M, Gill S, Fitzgerald M, Samii A (2006) Challenges and opportunities for pharmacoepidemiology in drug-therapy decision making. J Clin Pharmacol 46:6–9
Feinstein AR, Cicchetti DV (1990) High agreement but low kappa: I. The problems of two paradoxes. J Clin Epidemiol 43:543–549
Fleiss J (1981) Statistical methods for rates and proportions, 2nd edn. Wiley, New York
Fleming TR (1993) Data monitoring committees and capturing relevant information of high quality. Stat Med 12:565–570
Fowler F, Mangione T (1986) Reducing interviewer effects on health survey data. Center for Survey Research, University of Massachusetts, Boston
Fowler F, Mangione T (1990) Standardized survey interviewing: minimizing interviewer-related error. Sage Publications, Newberry Park
Freedland KE, Carney RM (1992) Data management and accountability in behavioral and biomedical research. Am Psychol 47:640–645
Gassman JJ, Owen WW, Kuntz TE, Martin JP, Amoroso WP (1995) Data quality assurance, monitoring, and reporting. Control Clin Trials 16(Suppl2):S104–S136
Gibson PJ, Koepsell TD, Diehr P, Hale C (1999) Increasing response rates for mailed surveys of Medicaid clients and other low-income populations. Am J Epidemiol 149:1057–1062
Gilbart E, Kreiger N (1998) Improvement in cumulative response rates following implementation of a financial incentive. Am J Epidemiol 148:97–99
Gilbert EH, Lowenstein SR, Koziol-McLain J, Barta DC, Steiner J (1996) Chart reviews in emergency medicine research: where are the methods? Ann Emerg Med 27:305–308
Gissler M, Teperi J, Hemminki E, Meriläinen J (1995) Data quality after restructuring a national medical registry. Scand J Soc Med 23:75–80
Goldberg J, Gelfand HM, Levy PS (1980) Registry evaluation methods: a review and case study. Epidemiol Rev 2:210–220
Goldman LR (2001) Epidemiology in the regulatory arena. Am J Epidemiol 154(Suppl 12): S18–S26
Goodman SN (1999a) Toward evidence-based medical statistics, 1: the P value fallacy. Ann Intern Med 130:995–1004
Goodman SN (1999b) Toward evidence-based medical statistics, 2: the bayes factor. Ann Intern Med 130:1005–1013
Gordis L (2000) Epidemiology, 2nd edn. W.B. Saunders, Philadelphia
Greenbaum DS, Bachmann JD, Krewski D, Samet JM, White R, Wyzga RE (2001) Particulate air pollution standards and morbidity and mortality: case study. Am J Epidemiol 154(Suppl 12):S78–S90
Halpern SD, Ubel PA, Berlin JA, Asch DA (2002) Randomized trial of 5 dollars versus 10 dollars monetary incentives, envelope size, and candy to increase physician response rates to mailed questionnaires. Med Care 40:834–839
Hawkins N, Evans J (1989) Subjective estimation of toluene exposures: a calibration study of industrial hygienists. Appl Ind Hyg 4:61–68
Hearst N, Hulley SB (1988) Using secondary data. In: Hulley SB, Cummings SR (eds) Designing clinical research. LWW, Baltimore, pp 53–62
Heilbrun LK, Nomura A, Stemmermann GN (1991) The effects of non-response in a prospective study of cancer: 15-year follow-up. Int J Epidemiol 20:328–338
Hiatt RA (2010) The epicenter of translational science. Am J Epidemiol 172:525–529
Hill AB (1965) The environment and disease: association or causation? Proc R Soc Med 58:295–300
Hilner JE, McDonald A, Van Horn L, Bragg C, Caan B, Slattery ML, Birch R, Smoak CG, Wittes J (1992) Quality control of dietary data collection in the CARDIA study. Control Clin Trials 13:156–169
Hoffman SC, Burke AE, Helzlsouer KJ, Comstock GW (1998) Controlled trial of the effect of length, incentives, and follow-up techniques on response to a mailed questionnaire. Am J Epidemiol 148:1007–1011
Holford TR, Stack C (1995) Study design for epidemiologic studies with measurement error. Stat Methods Med Res 4:339–358
Horbar JD, Leahy KA (1995) An assessment of data quality in the Vermont-Oxford trials network database. Control Clin Trials 16:51–61
Hosking JD, Rochon J (1982) A comparison of techniques for detecting and preventing key-field errors. In: Proceedings of the statistical computing section. American Statistical Association, Washington, DC, pp 82–87
Hosking JD, Newhouse MM, Bagniewska A, Hawkins BS (1995) Data collection and transcription. Control Clin Trials 16(Suppl 2):S66–S103
Hunt JR, White E (1998) Retaining and tracking cohort study members. Epidemiol Rev 20:57–70
International Organization for Standardization (2003) ISO 9000:2000, ISO Technical Committee ISO/TC 176
Ioannidis JP (1998) Effect of the statistical significance of results on the time to completion and publication of randomized efficacy trials. JAMA 279:281–286
James J, Bolstein R (1992) Large monetary incentives and their effect on mail survey response rates. Public Opin Q 56:442–453
John EM, Savitz DA (1994) Effect of a monetary incentive on response to a mail survey. Ann Epidemiol 4:231–235
Johnstone FD, Brown MC, Campbell D, MacGillivray I (1981) Measurement of variables: data quality control. Am J Clin Nutr 34(Suppl 4):804–806
Kaaks R, Ferrari P, Ciampi A, Plummer M, Riboli E (2002) Uses and limitations of statistical accounting for random error correlations, in the validation of dietary questionnaire assessments. Public Health Nutr 5:969–976
Kalantar JS, Talley NJ (1999) The effects of lottery incentive and length of questionnaire on health survey response rates: a randomized study. J Clin Epidemiol 52:1117–1122
Kannel WB (2000) Risk stratification in hypertension: new insights from the Framingham Study. Am J Hypertens 13:S3–S10
Karp DR, Carlin S, Cook-Deegan R, Ford DE, Geller G, Glass DN, Greely H, Guthridge J, Kahn J, Kaslow R, Kraft C, Macqueen K, Malin B, Scheuerman RH, Sugarman J (2008) Ethical and practical issues associated with aggregating databases. PLoS Med 5:e190
Kellerman SE, Herold J (2001) Physician response to surveys. A review of the literature. Am J Prev Med 20:61–67
Kessler RC, Little RJ, Groves RM (1995) Advances in strategies for minimizing and adjusting for survey nonresponse. Epidemiol Rev 17:192–204
Khoury M, Bedrosian S, Gwinn M, Higgins J, Ioannidis J, Little J (2010) Human genome epidemiology: building the evidence for using genetic information to improve health and prevent disease, 2nd edn. Oxford University Press, New York
Kiesler S, Sproull L (1986) Response effects in the electronic survey. Public Opinion Q 50:402–413
Kipen HM, Cody RP, Goldstein BD (1989) Use of longitudinal analysis of peripheral blood counts to validate historical reconstructions of benzene exposure. Environ Health Perspect 82:199–206
Kjelsberg MO, Cutler JA, Dolecek TA (1997) Brief description of the multiple risk factor intervention trial. Am J Clin Nutr 65(Suppl 1):S191–S195
Knatterud GL, Rockhold FW, George SL, Barton FB, Davis CE, Fairweather WR, Honohan T, Mowery R, O’Neill R (1998) Guidelines for quality assurance in multicenter trials: a position paper. Control Clin Trials 19:477–493
Krewski D, Burnett RT, Goldberg MS, Hoover K, Siemiatycki J, Abrahamowicz M, White WH (2000) Reanalysis of the Harvard six cities study and the American cancer society study of particulate air pollution and mortality. Investigators’ reports parts I and II. Health Effects Institute, Cambridge
Kromhout H, Oostendorp Y, Heederik D, Boleij JS (1987) Agreement between qualitative exposure estimates and quantitative exposure measurements. Am J Ind Med 12:551–562
Kwok R (2009) Personal technology: phoning in data. Nature 458:959–961
Landis JR, Koch GG (1977) The measurement of observer agreement for categorical data. Biometrics 33:159–174
Ling PM, Glantz SA (2002) Using tobacco-industry marketing research to design more effective tobacco-control campaigns. JAMA 287:2983–2989
Little RE, Davis AK (1984) Effectiveness of various methods of contact and reimbursement on response rates of pregnant women to a mail questionnaire. Am J Epidemiol 120:161–163
Maclure M, Schneeweiss S (2001) Causation of bias: the episcope. Epidemiology 12:114–122
Maclure M, Willett WC (1987) Misinterpretation and misuse of the kappa statistic. Am J Epidemiol 126:161–169
Maheux B, Legault C, Lambert J (1989) Increasing response rates in physicians’ mail surveys: an experimental study. Am J Public Health 79:638–639
Martinson BC, Lazovich D, Lando HA, Perry CL, McGovern PG, Boyle RG (2000) Effectiveness of monetary incentives for recruiting adolescents to an intervention trial to reduce smoking. Prev Med 31:706–713
Maudsley G, Williams EM (1999) What lessons can be learned for cancer registration quality assurance from data users? Skin cancer as an example. Int J Epidemiol 28:809–815
McMahon SR, Iwamoto M, Massoudi MS, Yusuf HR, Stevenson JM, David F, Chu SY, Pickering LK (2003) Comparison of e-mail, fax, and postal surveys of pediatricians. Pediatrics 111(4 Pt 1): e299–e303
McQuade CE, Kutvirt DM, Brylinski DA, Samet JM (1983) A tracking system for conducting epidemiological case-control studies. Comput Programs Biomed 16:149–153
Meinert C, Tonascia S (1986) Clinical trials: design, conduct and analysis. Oxford University Press, New York
Michaels D (2008) Doubt is their product: how industry’s assault on science threatens your health. Oxford University Press, New York
Moorman PG, Newman B, Millikan RC, Tse CK, Sandler DP (1999) Participation rates in a case-control study: the impact of age, race, and race of interviewer. Ann Epidemiol 9:188–195
Mullooly JP (1990) The effects of data entry error: an analysis of partial verification. Comput Biomed Res 23:259–267
Neaton JD, Duchene AG, Svendsen KH, Wentworth D (1990) An examination of the efficiency of some quality assurance methods commonly employed in clinical trials. Stat Med 9: 115–123
O’Connor RJ, Giovino GA, Kozlowski LT, Shiffman S, Hyland A, Bernert JT, Carabello RS, Cummings KM (2006) Changes in nicotine intake and cigarette use over time in two nationally representative cross-sectional samples of smokers. Am J Epidemiol 164:750–759
Olson SH (2001) Reported participation in case-control studies: changes over time. Am J Epidemiol 154:574–581
Olson SH, Voigt LF, Begg CB, Weiss NS (2002) Reporting participation in case-control studies. Epidemiology 13:123–126
Paolo AM, Bonaminio GA, Gibson C, Partridge T, Kallail K (2000) Response rate comparisons of e-mail- and mail-distributed student evaluations. Teach Learn Med 12:81–84
Parkes R, Kreiger N, James B, Johnson KC (2000) Effects on subject response of information brochures and small cash incentives in a mail-based case-control study. Ann Epidemiol 10:117–124
Perneger TV, Etter JF, Rougemont A (1993) Randomized trial of use of a monetary incentive and a reminder card to increase the response rate to a mailed health survey. Am J Epidemiol 138:714–722
Piper BG, Lindsey AM, Dodd MJ, Ferketich S, Paul SM, Weller S (1989) Development of an instrument to measure the subjective dimension of fatigue. In: Funk S, Tournquist E, Champagne M, Copp L, Weise R (eds), Key aspects of comfort: management of pain and nausea. Springer, Philadelphia, pp 199–208
Piper BF, Dibble SL, Dodd MJ, Weiss MC, Slaughter RE, Paul SM (1998) The revised Piper Fatigue Scale: Psychometric evaluation in women with breast cancer. Oncol Nurs Forum 25:677–684
Post W, Kromhout H (1991) Semiquantitative estimates of exposure to methylene chloride and styrene: the influence of quantitative exposure data. Appl Occup Environ Hyg 6: 197–204
Prud’homme GJ, Canner PL, Cutler JA (1989) Quality assurance and monitoring in the hypertension prevention trial. Hypertension prevention trial research group. Control Clin Trials 10(Suppl 3):S84–S94
Quan SF, Howard BV, Iber C, Kiley JP, Nieto FJ, O’Connor GT, Rapoport DM, Redline S, Robbins J, Samet JM, Wahl PW (1997) The sleep heart health study: design, rationale, and methods. Sleep 20:1077–1085
Reisch LM, Fosse JS, Beverly K, Yu O, Barlow WE, Harris EL, Rolnick S, Barton MB, Geiger AM, Herrinton LJ, Greene SM, Fletcher SW, Elmore JG (2003) Training, quality assurance, and assessment of medical record abstraction in a multisite study. Am J Epidemiol 157:546–551
Rhodes SD, Bowie DA, Hergenrather KC (2003) Collecting behavioural data using the world wide web: considerations for researchers. J Epidemiol Community Health 57:68–73
Rosner B, Spiegelman D, Willett WC (1992) Correction of logistic regression relative risk estimates and confidence intervals for random within-person measurement error. Am J Epidemiol 136:1400–1413
Rothman KJ, Greenland S (1998) Modern epidemiology, 2nd edn. Lippincott-Raven, Philadelphia
Sacks FM, Handysides GH, Marais GE, Rosner B, Kass EH (1986) Effects of a low-fat diet on plasma lipoprotein levels. Arch Intern Med 146:1573–1577
Samet JM (2000) Epidemiology and policy: the pump handle meets the new millennium. Epidemiol Rev 22:145–154
Samet JM (2009) Data: to share or not to share? Epidemiology 20:172–174
Samet JM, Lee NL (2001) Bridging the gap: perspectives on translating epidemiologic evidence into policy. Am J Epidemiol 154(Suppl 12):S1–S3
Samet JM, Zeger SL, Kelsall JE, Xu J, Kalkstein LS (1997) Particulate air pollution and daily mortality: analyses of the effects of weather and multiple air pollutants (The phase IB report of the particle epidemiology evaluation project). Health Effects Institute, Cambridge
Schweitzer M, Asch DA (1995) Timing payments to subjects of mail surveys: cost-effectiveness and bias. J Clin Epidemiol 48:1325–1329
Shahar E, Folsom AR, Jackson R (1996) The effect of nonresponse on prevalence estimates for a referent population: insights from a population-based cohort study. Atherosclerosis risk in communities (ARIC) study investigators. Ann Epidemiol 6:498–506
Shaw MJ, Beebe TJ, Jensen HL, Adlis SA (2001) The use of monetary incentives in a community survey: impact on response rates, data quality, and cost. Health Serv Res 35:1339–1346
Silver RC, Holman EA, McIntosh DN, Poulin M, Gil-Rivas V (2002) Nationwide longitudinal study of psychological responses to september 11. JAMA 288:1235–1244
Slattery ML, Edwards SL, Caan BJ, Kerber RA, Potter JD (1995) Response rates among control subjects in case-control studies. Ann Epidemiol 5:245–249
Sorensen HT, Sabroe S, Olsen J (1996) A framework for evaluation of secondary data sources for epidemiological research. Int J Epidemiol 25:435–442
Spiegelman D, Schneeweiss S, McDermott A (1997) Measurement error correction for logistic regression models with an “alloyed gold standard”. Am J Epidemiol 145:184–196
Spry VM, Hovell MF, Sallis JG, Hofsteter CR, Elder JP, Molgaard CA (1989) Recruiting survey respondents to mailed surveys: controlled trials of incentives and prompts. Am J Epidemiol 130:166–172
Steeh C (1981) Trends in nonresponse rates 1952–1979. Public Opin Q 45:40–57
Stram DO, Langholz B, Huberman M, Thomas DC (1999) Correcting for exposure measurement error in a reanalysis of lung cancer mortality for the colorado plateau uranium miners cohort. Health Phys 77:265–275
Szklo M, Nieto FJ (2000) Epidemiology: beyond the basics. Aspen Publishers, Gaithersburg
The Chemical Manufacturers Association’s Epidemiology Task Force (1991) Guidelines for good epidemiology practices for occupational and environmental epidemiologic research. J Occup Med 33:1221–1229
Thompson WD (1990) Kappa and attenuation of the odds ratio. Epidemiology 1:357–369
Thompson WD, Walter SD (1988) A reappraisal of the kappa coefficient. J Clin Epidemiol 41:949–958
Thornton A, Lee P (2000) Publication bias in meta-analysis: its causes and consequences. J Clin Epidemiol 53:207–216
Tielemans E, Heederik D, Burdorf A, Vermeulen R, Veulemans H, Kromhout H, Hartog K (1999) Assessment of occupational exposures in a general population: comparison of different methods. Occup Environ Med 56:145–151
Turpin J, Rose R, Larsen B (2003) An adaptable, transportable web-based data acquisition platform for clinical and survey-based research. J Am Osteopath Assoc 103:182–186
US Department of Health, Education, and Welfare (DHEW) (1973) Final report of the tuskegee syphilis study Ad Hoc advisory panel. US Public Health Service, Washington, DC
US Department of Health and Human Services (2004) The health consequences of smoking. A report of the Surgeon General. U.S. Department of Health and Human Services, Centers for Disease Control and Prevention, National Center for Chronic Disease Prevention and Health Promotion, Office on Smoking and Health, Atlanta
US Department of Health and Human Services (2009) Application for a Public Health Service Grant. PHS 398, Public Health Service, Bethesda
US Environmental Protection Agency (EPA) (1989) Toxic Substances Control Act (TSCA): good laboratory practice standards. 40 CFR Part 792, 34034–34050
Vantongelen K, Rotmensz N, van der Schueren E (1989) Quality control of validity of data collected in clinical trials. EORTC study group on data management (SGDLM). Eur J Cancer Clin Oncol 25:1241–1247
Vardeman SB, Jobe JM (1999) Statistical quality assurance methods for engineers, Wiley, Hoboken
Wacholder S, McLaughlin JK, Silverman DT, Mandel JS (1992) Selection of controls in case-control studies. I. Principles. Am J Epidemiol 135:1019–1028
Wacholder S, Armstrong B, Hartge P (1993) Validation studies using an alloyed gold standard. Am J Epidemiol 137:1251–1258
Wallace JM, Jr, Bachman JG, O’Malley PM, Johnston LD, Schulenberg JE, Cooper SM (2002) Tobacco, alcohol, and illicit drug use: racial and ethnic differences among U.S. high school seniors, 1976–2000. Public Health Rep 117(Suppl 1):S67–S75
Wechsler D (1989) Wechsler preschool and primary scale of intelligence-revised. Psychological Corporation, San Antonio
White E, Hunt JR, Casso D (1998) Exposure measurement in cohort studies: the challenges of prospective data collection. Epidemiol Rev 20:43–56
White E, Armstrong BK, Saracci R (2008) Principles of exposure measurement in epidemiology: collecting, evaluating, and improving measures of disease risk factors. Oxford University Press, New York
Whitney CW, Lind BK, Wahl PW (1998) Quality assurance and quality control in longitudinal studies. Epidemiol Rev 20:71–80
Willett WC, Stampfer MJ, Underwood BA, Speizer FE, Rosner B, Hennekens CH (1983) Validation of a dietary questionnaire with plasma carotenoid and alpha-tocopherol levels. Am J Clin Nutr 38:631–639
Willett WC, Sampson L, Stampfer MJ, Rosner B, Bain C, Witschi J, Hennekens CH, Speizer FE (1985) Reproducibility and validity of a semiquantitative food frequency questionnaire. Am J Epidemiol 122:51–65
Wright P, Haybittle J (1979a) Design of forms for clinical trials (1). Br Med J 2:529–530
Wright P, Haybittle J (1979b) Design of forms for clinical trials (2). Br Med J 2:590–592
Wright P, Haybittle J (1979c) Design of forms for clinical trials (3). Br Med J 2:650–651
Wyatt J (1995) Acquisition and use of clinical data for audit and research. J Eval Clin Pract 1:15–27
Download references
Author information
Authors and affiliations.
Division of Cancer Control and Population Sciences and Division of Cancer Epidemiology and Genetics, National Cancer Institute, 9609 Medical Center Drive, 4E442, 20850, Rockville, MD, USA
Director, University of Southern California (USC) Institute for Global Health, Professor and Flora L. Thornton Chair, Department of Preventive Medicine Keck School of Medicine of USC, Los Angeles, California, USA
Jonathan M. Samet
South Asia Region Center for Global Health, US National Cancer Institute, New Delhi, India
Preetha Rajaraman
You can also search for this author in PubMed Google Scholar
Editor information
Editors and affiliations.
Department of Epidemiological Methods and Etiologic Research, Leibniz Institute for Prevention Research and Epidemiology – BIPS, Bremen, Germany
Wolfgang Ahrens
Department of Biometry and Data Management, Leibniz Institute for Prevention Research and Epidemiology – BIPS, Bremen, Germany
Iris Pigeot
Rights and permissions
Reprints and permissions
Copyright information
© 2014 Springer Science+Business Media New York
About this entry
Cite this entry.
Neta, G., Samet, J.M., Rajaraman, P. (2014). Quality Control and Good Epidemiological Practice. In: Ahrens, W., Pigeot, I. (eds) Handbook of Epidemiology. Springer, New York, NY. https://doi.org/10.1007/978-0-387-09834-0_14
Download citation
DOI : https://doi.org/10.1007/978-0-387-09834-0_14
Publisher Name : Springer, New York, NY
Print ISBN : 978-0-387-09833-3
Online ISBN : 978-0-387-09834-0
eBook Packages : Medicine Reference Module Medicine
Share this entry
Anyone you share the following link with will be able to read this content:
Sorry, a shareable link is not currently available for this article.
Provided by the Springer Nature SharedIt content-sharing initiative
- Publish with us
Policies and ethics
- Find a journal
- Track your research
What is quality research? A guide to identifying the key features and achieving success
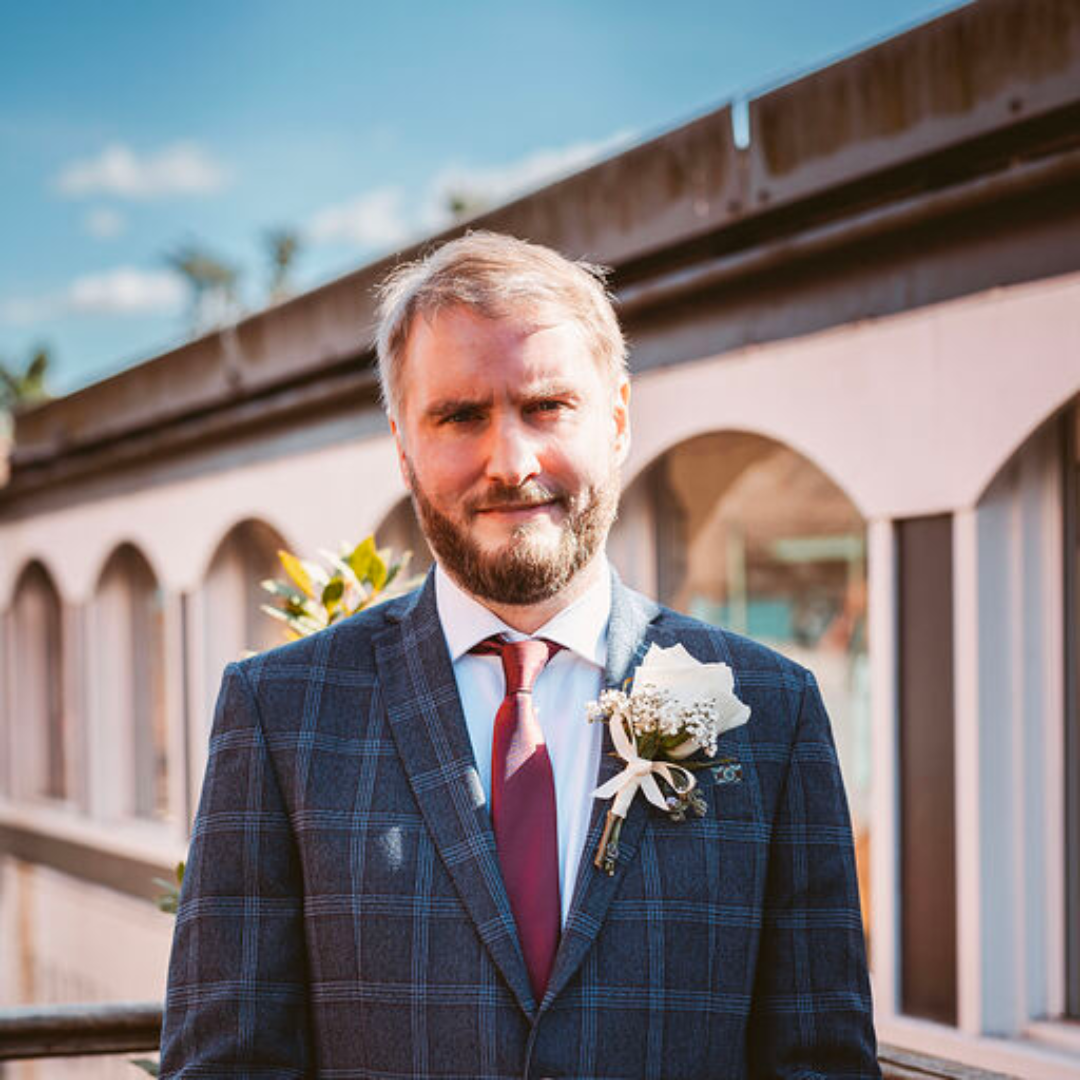
Every researcher worth their salt strives for quality. But in research, what does quality mean?
Simply put, quality research is thorough, accurate, original and relevant. And to achieve this, you need to follow specific standards. You need to make sure your findings are reliable and valid. And when you know they're quality assured, you can share them with absolute confidence.
You’ll be able to draw accurate conclusions from your investigations and contribute to the wider body of knowledge in your field.
Importance of quality research
Quality research helps us better understand complex problems. It enables us to make decisions based on facts and evidence. And it empowers us to solve real-world issues. Without quality research, we can't advance knowledge or identify trends and patterns. We also can’t develop new theories and approaches to solving problems.
With rigorous and transparent research methods, you’ll produce reliable findings that other researchers can replicate. This leads to the development of new theories and interventions. On the other hand, low-quality research can hinder progress by producing unreliable findings that can’t be replicated, wasting resources and impeding advancements in the field.
In all cases, quality control is critical. It ensures that decisions are based on evidence rather than gut feeling or bias.
Standards for quality research
Over the years, researchers, scientists and authors have come to a consensus about the standards used to check the quality of research. Determined through empirical observation, theoretical underpinnings and philosophy of science, these include:
1. Having a well-defined research topic and a clear hypothesis
This is essential to verify that the research is focused and the results are relevant and meaningful. The research topic should be well-scoped and the hypothesis should be clearly stated and falsifiable .
For example, in a quantitative study about the effects of social media on behavior, a well-defined research topic could be, "Does the use of TikTok reduce attention span in American adolescents?"
This is good because:
- The research topic focuses on a particular platform of social media (TikTok). And it also focuses on a specific group of people (American adolescents).
- The research question is clear and straightforward, making it easier to design the study and collect relevant data.
- You can test the hypothesis and a research team can evaluate it easily. This can be done through the use of various research methods, such as survey research , experiments or observational studies.
- The hypothesis is focused on a specific outcome (the attention span). Then, this can be measured and compared to control groups or previous research studies.
2. Ensuring transparency
Transparency is crucial when conducting research. You need to be upfront about the methods you used, such as:
- Describing how you recruited the participants.
- How you communicated with them.
- How they were incentivized.
You also need to explain how you analyzed the data, so other researchers can replicate your results if necessary. re-registering your study is a great way to be as transparent in your research as possible. This involves publicly documenting your study design, methods and analysis plan before conducting the research. This reduces the risk of selective reporting and increases the credibility of your findings.
3. Using appropriate research methods
Depending on the topic, some research methods are better suited than others for collecting data. To use our TikTok example, a quantitative research approach, such as a behavioral test that measures the participants' ability to focus on tasks, might be the most appropriate.
On the other hand, for topics that require a more in-depth understanding of individuals' experiences or perspectives, a qualitative research approach, such as interviews or focus groups, might be more suitable. These methods can provide rich and detailed information that you can’t capture through quantitative data alone.
4. Assessing limitations and the possible impact of systematic bias
When you present your research, it’s important to consider how the limitations of your study could affect the result. This could be systematic bias in the sampling procedure or data analysis, for instance. Let’s say you only study a small sample of participants from one school district. This would limit the generalizability and content validity of your findings.
5. Conducting accurate reporting
This is an essential aspect of any research project. You need to be able to clearly communicate the findings and implications of your study . Also, provide citations for any claims made in your report. When you present your work, it’s vital that you describe the variables involved in your study accurately and how you measured them.
Curious to learn more? Read our Data Quality eBook .
How to identify credible research findings
To determine whether a published study is trustworthy, consider the following:
- Peer review: If a study has been peer-reviewed by recognized experts, rest assured that it’s a reliable source of information. Peer review means that other scholars have read and verified the study before publication.
- Researcher's qualifications: If they're an expert in the field, that’s a good sign that you can trust their findings. However, if they aren't, it doesn’t necessarily mean that the study's information is unreliable. It simply means that you should be extra cautious about accepting its conclusions as fact.
- Study design: The design of a study can make or break its reliability. Consider factors like sample size and methodology.
- Funding source: Studies funded by organizations with a vested interest in a particular outcome may be less credible than those funded by independent sources.
- Statistical significance: You've heard the phrase "numbers don't lie," right? That's what statistical significance is all about. It refers to the likelihood that the results of a study occurred by chance. Results that are statistically significant are more credible.
Achieve quality research with Prolific
Want to ensure your research is high-quality? Prolific can help.
Our platform gives you access to a carefully vetted pool of participants. We make sure they're attentive, honest, and ready to provide rich and detailed answers where needed. This helps to ensure that the data you collect through Prolific is of the highest quality.
With Prolific, you can streamline your research process and feel confident in the results you receive. Our minimum pay threshold and commitment to fair compensation motivate participants to provide valuable responses and give their best effort. This ensures the quality of your research and helps you get the results you need. Sign up as a researcher today to get started!
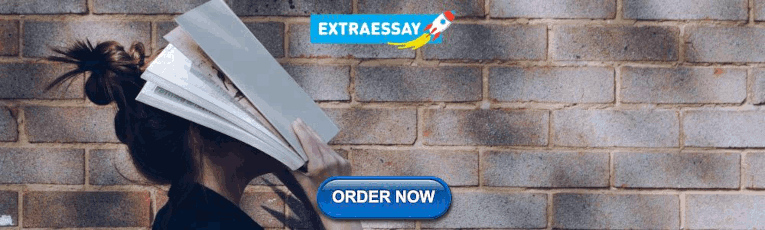
You might also like
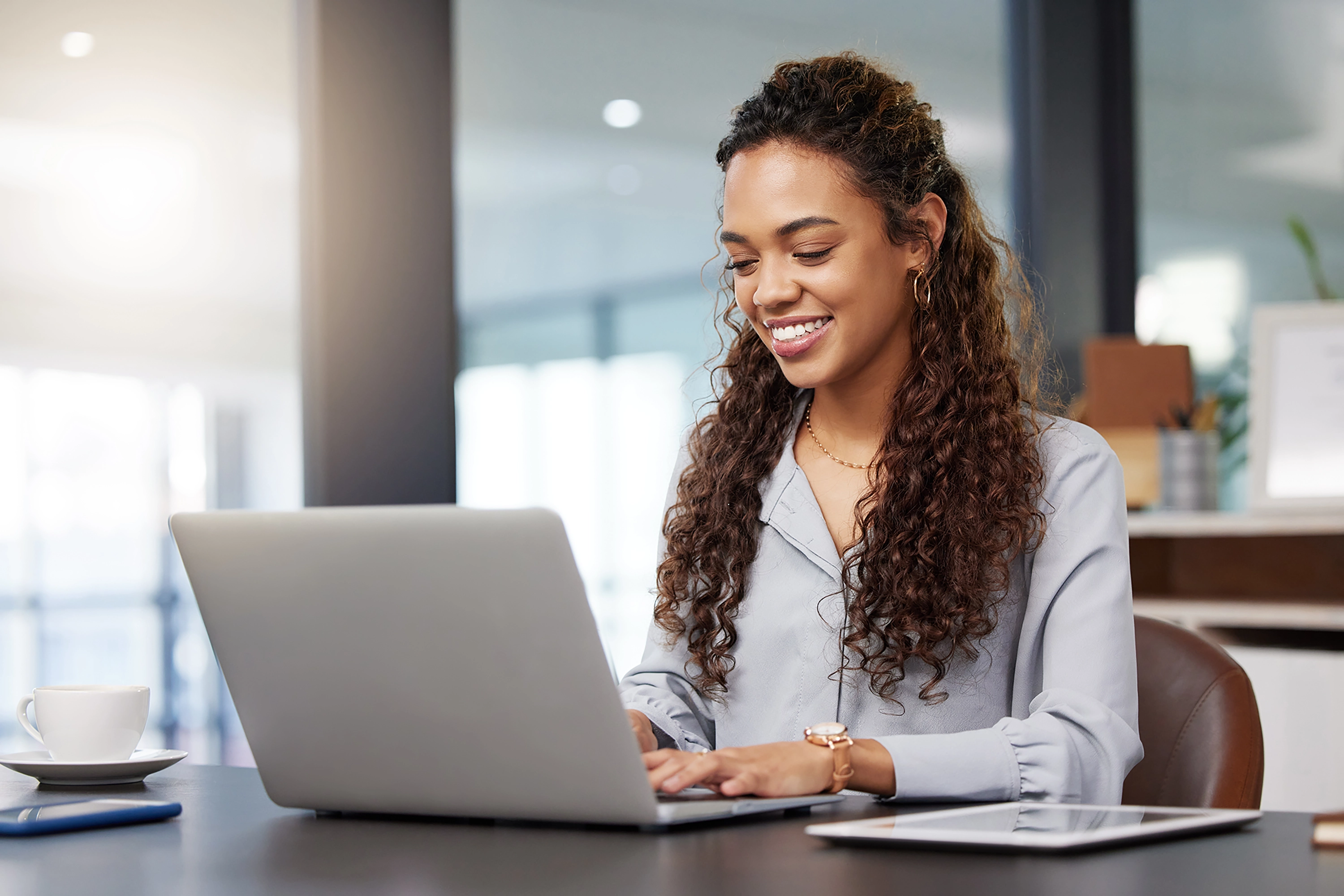
High-quality human data to deliver world-leading research and AIs.
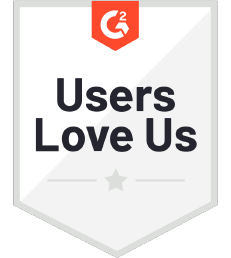
Follow us on
All Rights Reserved Prolific 2024
Data Use: An evaluation of quality-control questions
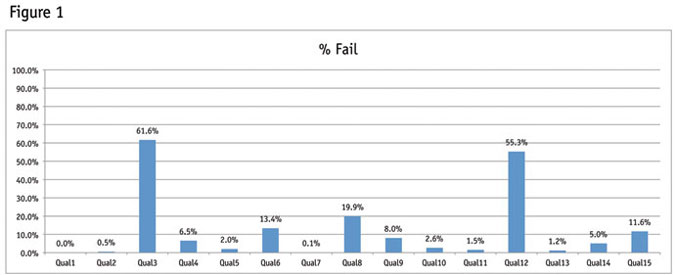
A report on a research-on-research study that examined the impact of various types of in-survey quality-control measures.
Editor's note: Keith Phillips is senior methodologist with SSI, Shelton, Conn.
How do we, as researchers, decide on quality data? We usually look at the behavior of participants within a questionnaire itself. We look at how long they took to complete the survey, what they wrote in the open-ended questions, whether they gave the same answer option through a grid question, if they gave consistent answers and we look at quality-control questions. These quality-control questions are designed to measure attentiveness and remove participants who are not paying attention.
There are different types of quality-control questions. Some are simply inserted within a grid and ask participants to select a specific punch; others measure quality by allowing participants to contradict themselves. Some intentionally misdirect participants, so that the question being asked is in the detail of a long instruction and the question is not at all what it seems to be.
Quality-control questions assume that participant misbehavior in a particular moment is indicative of misbehavior throughout the entire survey. For this reason, the data quality is improved with the omission of this participant. An alternate assumption is that a degree of inattentiveness is normal throughout a survey and participants may be attentive during the trap question but not during key measures. Conversely, those failing the trap may not have been paying attention in the moment but are contributing elsewhere, meaning many are no different than the participants that are being kept.
First hypothesis
Working at a sample provider, I see many questionnaires for a variety of industries conducting an array of research. One thing I have noticed is the variety of quality-control measurements used to validate the online self-completion surveys; in particular, the varying amount of participants being excluded due to poor data quality, which was specifically dependent on the quality-control questions.
This led to my first hypothesis: Design of the quality-control question has a large impact on the number of participants flagged for having poor data quality and it is more a measurement of question effectiveness than participant quality.
Knowing that these quality-control questions threw out such a wide range of participants, I formulated a second hypothesis: Exclusion based on quality-control measurements would result in a variety of sample compositions and some of these would be more accurate than others.
In order to test my theories, I designed an online survey that looked at 15 different quality-control measurements: 12 quality-control questions, speeding, straightlining and an open-end assessment. The survey was administered among 2,100 online participants in the U.S. The survey covered a mixture of topics including entertainment, social issues, lifestyle and general behavior questions. The survey used 12 offline benchmarks as measurements of quality to compare the data against. The median survey time was 12.5 minutes; short enough not to encourage fatigue.
The results
As illustrated in Figure 1, my first hypothesis proved true: the design of the question itself has a large impact on the amount of participants being excluded from the sample. Shown here is the percent of the same sample that failed each of the quality-control measurements. There is a large distribution that goes from 0 percent failing at Qual1 to 61.6 percent failing at Qual 3. More detail on each of these quality-control measurements will be given later in this article.
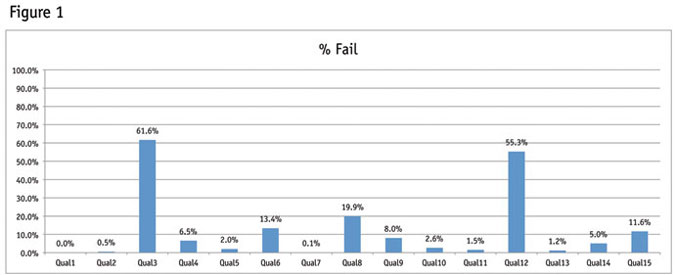
- Already have a WordPress.com account? Log in now.
- Subscribe Subscribed
- Copy shortlink
- Report this content
- View post in Reader
- Manage subscriptions
- Collapse this bar
Table of Contents
What is quality control (qc), key components of quality control, types of quality control, why is quality control important what are the benefits, quality control roles and responsibilities, quality control vs. quality assurance, quality control careers, what does quality control mean, what are 3 examples of quality control, train to assess quality with lean today.
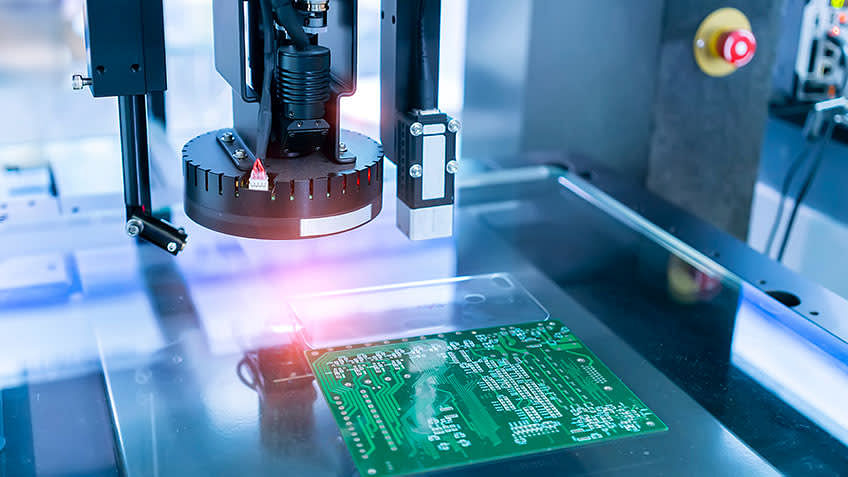
In today’s world, it’s not uncommon that we take the reliability and quality of products and services for granted. At the start of the 20th century, however, quality control in manufacturing was not exactly a reliable process.
Now, decades after early pioneers created business problem-solving processes and analysis frameworks to determine and control consistency and value, it’s possible more than ever for a business to implement and scale best practices.
Quality does not have a singular definition. Despite the relative meaning of “value,” quality control is the process by which products/services are tested and measured to ensure they meet a standard. Through this process, a business can evaluate, maintain, and improve product quality. The primary objective of Quality Control is to identify and correct any deviations from the established quality standards. This process involves monitoring and inspecting products or services at various stages of production or delivery to ensure that they meet the desired level of quality. QC is also concerned with preventing defects or errors from occurring in the first place by implementing measures to control and improve the production or service delivery processes.
Ultimately, there are two crucial goals of quality control: (1) to ensure that products are as uniform as possible and (2), to minimize errors and inconsistencies within them.
Key components of Quality Control may include:
Inspection: Regularly examining products, materials, or services to identify defects, non-compliance, or deviations from quality standards.
Testing: Conducting various tests and measurements to assess the performance, functionality, or characteristics of products or services.
Statistical Process Control (SPC): Employing statistical techniques to monitor and control the production processes, ensuring that they remain within acceptable quality limits.
Documentation and Records: Keeping detailed records of inspections, tests, and corrective actions taken to maintain traceability and accountability.
Corrective Action: Implementing appropriate measures to address any identified quality issues and prevent their recurrence.
Training and Education: Providing employees with the necessary skills and knowledge to maintain quality standards effectively.
Continuous Improvement: Constantly analyzing data and feedback to identify areas for improvement and enhancing the overall quality management system.
Quality Control is closely related to another quality management concept called Quality Assurance (QA). While QC focuses on detecting and correcting defects, QA concentrates on preventing them from occurring in the first place by setting up robust processes and procedures.
Together, QC and QA form the backbone of an organization's quality management system, helping to ensure that products and services consistently meet or exceed customer expectations and regulatory requirements.
Quality Control Process
Normally, quality testing is part of every stage of a manufacturing or business process. Employees frequently begin testing using samples collected from the production line, finished products, and raw materials. Testing during various production phases can help identify the cause of a production problem and the necessary corrective actions to prevent it from happening again.
Customer service reviews, questionnaires, surveys, inspections, and audits are a few examples of quality testing procedures that can be used in non-manufacturing businesses. A company can use any procedure or technique to ensure that the final product or service is safe, compliant, and meets consumer demands.
QC Is Different by Industry
Quality Control (QC) is an indispensable aspect of various industries, ensuring that products and services adhere to predefined standards. In the manufacturing sector, QC involves rigorous inspection and testing of raw materials, intermediate components, and final products to maintain consistent quality and minimize defects. In the food industry, QC guarantees the safety and integrity of consumables through thorough testing for contaminants and adherence to health regulations. In the pharmaceutical sector, QC plays a critical role in verifying the potency and purity of drugs, ensuring they are safe for consumption. Additionally, in the software industry, QC involves extensive testing of applications and programs to identify bugs and errors before release, guaranteeing a smooth user experience. Across all industries, QC is a fundamental process that enhances customer satisfaction, boosts efficiency, and fosters a reputation for reliability.
Just as quality is a relative word with many interpretations, quality control itself doesn’t have a uniform, universal process. Some methods depend on the industry. Take food and drug products, for instance, where errors can put people at risk and create significant liability. These industries may rely more heavily on scientific measures, whereas others (such as education or coaching) may require a more holistic, qualitative method.
At its core, quality control requires attention to detail and research methodology.
So, what is quality control? There are a wide range of quality control methods , including:
Control Charts:
A graph or chart is used to study how processes are changing over time. Using statistics, the business and manufacturing processes are analyzed for being “in control.”
Process Control:
Processes are monitored and adjusted to ensure quality and improve performance. This is typically a technical process using feedback loops, industrial-level controls, and chemical processes to achieve consistency.
Acceptance Sampling:
A statistical measure is used to determine if a batch or sample of products meets the overall manufacturing standard.
Process Protocol:
A mapping methodology that improves the design and implementation processes by creating evaluative indicators for each step.
There are other quality control factors to consider when selecting a method in addition to types of processes.
Some companies establish internal quality control divisions when defining what is quality control. They do this to monitor products and services, while others rely on external bodies to track products and performance. These controls may be largely dependent on the industry of the business. Due to the strict nature of food inspections, for example, it may be in a company’s best interest to sample products internally and verify these results in a third-party lab.
Become a Quality Management Professional
- 10% Growth In Jobs Of Quality Managers Profiles By 2025
- 11% Revenue Growth For Organisations Improving Quality
Certified Lean Six Sigma Green Belt
- 4 hands-on projects to perfect the skills learnt
- 4 simulation test papers for self-assessment
Lean Six Sigma Expert
- IASSC® Lean Six Sigma Green Belt and Black Belt certification
- 13 Projects, 12 Simulation exams, 18 Case Studies & 114 PDUs
Here's what learners are saying regarding our programs:
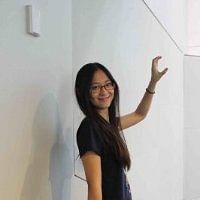
Xueting Liu
Mechanical engineer student at sargents pty. ltd. ,.
A great training and proper exercise with step-by-step guide! I'll give a rating of 10 out of 10 for this training.
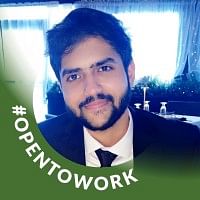
Abdus Salam
I have completed the Lean Six Sigma Expert Master’s Program from Simplilearn. And after the course, I could take up new projects and perform better. My average pay rate for a research position increased by 21%.
Quality Control (QC) is essential for various reasons, and its importance lies in the numerous benefits it brings to both businesses and consumers. Here are some key reasons why QC is crucial:
Customer Satisfaction: QC ensures that products and services meet or exceed customer expectations, leading to higher satisfaction levels and increased customer loyalty.
Defect Prevention: By identifying and correcting issues early in the production or service delivery process, QC helps prevent defects, reducing the likelihood of expensive recalls or rework.
Cost Reduction: Implementing QC measures can lead to reduced waste, lower production costs, and improved operational efficiency, contributing to overall cost savings.
Compliance and Regulations: QC ensures that products and services adhere to industry standards and regulatory requirements, avoiding legal issues and penalties.
Brand Reputation: Consistent high-quality products or services build a positive brand image, enhancing the company's reputation and competitiveness in the market.
Increased Efficiency: QC optimizes processes and identifies areas for improvement, leading to increased productivity and streamlined operations.
Risk Mitigation: Through rigorous testing and inspections, QC helps identify potential risks and hazards, enabling businesses to address them proactively.
Continuous Improvement: QC encourages a culture of continuous improvement, where organizations strive to enhance their products, services, and processes constantly.
International Competitiveness: High-quality products can open doors to global markets, increasing a company's competitiveness on an international scale.
Customer Retention and Loyalty: Satisfied customers are more likely to remain loyal and recommend the brand to others, contributing to long-term business success.
Overall, Quality Control is crucial for maintaining high standards, minimizing risks, and fostering a competitive advantage in today's dynamic and demanding business environment. It serves as the foundation for delivering superior products and services while ensuring customer satisfaction and loyalty.
When answering what is quality control, it is critical to understand that it consists of multifaceted responsibilities and roles. Moreover, it shouldn’t be confused with quality assurance. Whereas quality assurance looks at the processes used to prevent defects, quality control is focused specifically on the measurement and analysis processes involved with determining product quality.
Quality control uses specific research tools to accomplish fact-finding processes and conduct analyses. A quality control professional is tasked with analyzing these measurements against some sort of standard determined by the quality management department, company policies, and industries or regulatory bodies. Based on this evidence-gathering, quality control will recommend changes.
We can see from this roadmap, too, how quality assurance and quality control differ. Quality assurance looks at the holistic picture to prevent a product from becoming defective. Quality control, on the other hand, later determines if a product is, in fact, defective or not. Both roles fit under the broad umbrella of quality management.
Thus, an individual in quality control is tasked with communicating results to stakeholders and significant parties. A good quality control specialist will be able to disseminate scientific and research-based thinking to a business community and assist with the problem-solving process. These specialists are a key component of a product’s design process, as they determine whether a company’s creation is truly acceptable for the market.
Even though the terms quality control and quality assurance are sometimes used interchangeably, they have some key differences. Quality criteria, such as ensuring an item complies with specifications, are the main emphasis of quality control. Quality assurance is the sum of all processes and actions necessary to demonstrate that the requirements for quality are satisfied.
Quality control can be a fulfilling job if you enjoy dealing with people, talking, presenting results, and trying to make things better and safer. Depending on the sector, you may need the following qualifications to work as a quality control inspector:
- Entry-level positions require a high school diploma.
- Depending on the business, a bachelor's degree
- A background in the industry
- Certain businesses and sectors require licenses and certifications.
Additional characteristics required by quality control specialists include:
- Observation of details
- Talents in math and mechanics
- Physical prowess and power
- Technical expertise
- Pressured performance
Career Path
There may be discrepancies because the path to quality assurance and control job varies by industry. However, you'll typically require a number of years of professional expertise in your field. After completing the necessary educational qualifications and gaining the necessary work experience, you are often hired as a quality assurance or control associate.
As you gain job experience, you can advance to the position of senior specialist and start leading groups of quality control specialists.
Your employer may require you to take professional development classes or obtain certifications like Six Sigma . A professional designation like Certified Quality Inspector may also be required.
Quality Control Salaries
Depending on the role, expertise, and industry, quality control specialists make a variety of salaries. As you get more expertise and advance into management positions, your pay rises. The average wage, as reported by the Bureau of Labor Statistics, is:
- Services rendered by experts in science and technology: $46,280
- Production: $40,020
- Trade in bulk: $37,800
- $30,070 for office supplies and support services
Quality control refers to a company's methods for assessing product quality and, if necessary, improving it. There are various ways to perform quality control, including benchmarking, examining manufacturing procedures, and testing products. All of this is done to keep track of significant product differences.
Three examples of quality control in the food sector are monitoring ingredient standards, verifying supplier lists, and making sure the manufacturing facility is hygienic.
Learn how to define what is quality control with Simplilearn’s Post Graduate Program in Lean Six Sigma , offered in partnership with the University of Massachusetts Amherst. This Lean Six Sigma green belt certification program will help you gain key skills to lead tranformational projects by improving overall quality and delivering the best results.
With the Six Sigma Black Belt certification , you'll be equipped to mentor Green Belts, guide projects, and drive substantial ROI for your organization. Elevate your career with this program's in-depth curriculum, designed to mold you into a proficient Six Sigma Black Belt capable of orchestrating impactful change and delivering excellence.
This course focuses on two important management methodologies — Lean practices and Six Sigma — that will enable you to accelerate business improvement.
1. What is Quality Control (QC)?
Quality control is the process by which services/products are measured and tested to ensure they are as uniform as possible and meet a standard. It helps businesses minimize inconsistencies and improve product quality.
2. What are the four types of Quality Control?
The four types of quality control are process control, control charts, acceptance sampling, and product quality control. While a control chart helps study changing processes over time, process control and product quality control help monitor and adjust products as per the standards. Acceptance sampling is a unique type that involves a statistical measure to determine whether a batch or sample of products satisfies the standards.
3. Why is Quality Control important?
Quality control is important to safeguard the company’s reputation, prevent products from being unreliable, and increase trust on the side of consumers. It ensures that the company looks at evidence-based data and research rather than anecdotal observations to ensure that the services/products live up to the standards. It reduces cost and maximizes profit, operational efficiency, and customer satisfaction.
4. What are three examples of Quality Control?
Some examples of quality control are: a high-speed car manufacturer runs thorough tests for every component, including manual and automated verifications; websites study the average response time per page for customer interactions and generate tickets when the service gets unacceptably slow; retail store owners employ secret shoppers to test the customer service of their stores.
5. What are the four steps of Quality Control?
The first step for quality control is to set your quality standards and decide which ones to focus on. Secondly, you must establish operational processes to deliver optimal quality and implement them. The third step is to review your results and identify gaps. Lastly, get feedback and make improvisations.
6. What are quality control techniques?
Inspection and Statistical quality control (SQC) are the two major techniques of Quality Control. Inspection checks the performance of items as per the pre-decided specifications. It involves periodic checking before, during and on completion of the process. It can be categorized into two types: Centralized and Floor Inspection. Statistical Quality Control relies on laws of probability. It controls the production quality within tolerance limits via sample procedure.
7. What is the difference QA and QC?
Quality Assurance (QA) focuses on preventing defects and maintaining the overall quality management system through process implementation and improvement. It ensures that proper processes are in place to avoid issues. On the other hand, Quality Control (QC) involves detecting and correcting defects through inspections and testing. QC ensures that products or services meet specific quality standards. While QA is proactive, emphasizing prevention, QC is reactive, emphasizing identification and correction of issues after they occur.
Our Quality Management Courses Duration And Fees
Explore our top Quality Management Courses and take the first step towards career success
Get Free Certifications with free video courses
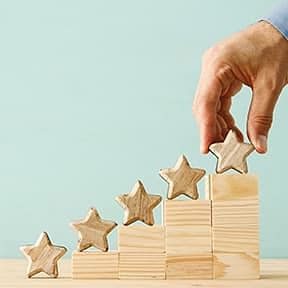
Quality Management
Lean Management
Learn from Industry Experts with free Masterclasses
Digital marketing.
Digital Marketing Job Search in 2024: Top Things to Do Before You Begin
Unlock Digital Marketing Career Success Secrets for 2024 with Purdue University
Your Gateway to Game-changing Digital Marketing Careers in 2024 with Purdue University
Recommended Reads
Free eBook: Quality Management Professionals Salary Report
Quality Control Manager Job Description
The Ultimate Guide to Understand Everything on Control Statements in C
Free eBook: Top 25 Interview Questions and Answers: Quality Management
Project Quality Management: Perform Quality Assurance Vs Perform Quality Control
What is Version Control and What Are Its Benefits?
Get Affiliated Certifications with Live Class programs
- PMP, PMI, PMBOK, CAPM, PgMP, PfMP, ACP, PBA, RMP, SP, and OPM3 are registered marks of the Project Management Institute, Inc.

An official website of the United States government
Here’s how you know
Official websites use .gov A .gov website belongs to an official government organization in the United States.
Secure .gov websites use HTTPS A lock ( Lock A locked padlock ) or https:// means you’ve safely connected to the .gov website. Share sensitive information only on official, secure websites.
JavaScript appears to be disabled on this computer. Please click here to see any active alerts .
DNA-Based Water Quality Monitoring Methods to Support Aquatic and Human Health Webinar
- April 24, 2024 from 2 to 3pm ET ( Webinar series )
- The webinar recording will be posted to this page within two weeks of the live broadcast.
About the Webinar
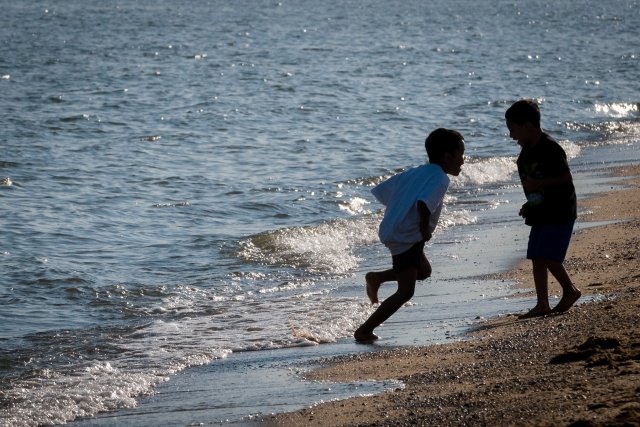
1. Becoming Uncultured: Daily Recreational Water Quality Monitoring and Public Notification at Chicago Beaches Using qPCR
Beach water monitoring and notification is vital for protecting the health of beachgoers. Traditionally, beach monitoring has relied on culturing fecal indicator bacteria using methods that require 18-24 hours to generate results, thus providing outdated information for current beach management decisions. In the 2012 Recreational Water Quality Criteria (RWQC), the USEPA endorsed a qPCR method for rapidly measuring enterococci. Since 2015, through a partnership between Chicago Park District (CPD) and the University of Illinois Chicago School of Public Health (UIC SPH), Chicagoans have daily beach monitoring notifications typically within four hours. Having a well-trained team and consistent daily schedule proved essential for delivering timely and high-quality qPCR results to guide same-day beach management decisions. Given that culture-based results from the previous day can often lead to erroneous beach management decisions, the rapid molecular method should be regarded as the benchmark for public health protection. In this presentation, the discussion will focus on how, following a two-year pilot program of rapid molecular testing of beach water samples, in 2017, Chicago became the first large U.S. city to issue same-day water-quality warnings for all its public recreational beaches and has successfully done so every year after that. The CPD and UIC SPH partnership illustrates that true daily beach monitoring using same-day water quality results is an achievable goal.
Presenter: Abhilasha Shrestha, PhD, University of Illinois Chicago Abhilasha is a Research Assistant Professor within the Environmental and Occupational Health Sciences Department at the University of Illinois Chicago School of Public Health (Chicago, IL). Dr. Shrestha conducts research primarily focused on water quality and its implications for public health. Her research interests include investigating various indicator targets and genes to rapidly assess infectious agents in water. Since 2015, she has overseen the management and operation of Chicago’s Lake Michigan beach monitoring project, conducted in collaboration with the Chicago Park District. In addition to her work on beach monitoring, her research also focuses on identifying and mitigating different bacterial sources of pollution at public Lake Michigan beaches in Chicago, utilizing microbial source tracking. Furthermore, Dr. Shrestha spearheaded a wastewater surveillance project for SARS-CoV-2 in Kisumu, Kenya, from 2022 to 2023. She is engaged in ongoing global health water research projects in Kenya and Nepal, contributing significantly to the understanding and management of waterborne health risks on an international scale.
2. Standard Control Material for Quantitative Real-Time PCR Recreational Water Quality Monitoring
Fecal pollution remains a significant challenge for recreational water quality managers. The use of quantitative real-time PCR (qPCR) methods is increasing, allowing for same day beach water quality public notification and identification of key fecal pollution sources. However, widespread implementation requires access to a high-quality standard control material. This presentation describes a collaboration between the U.S. Environmental Protection Agency and the National Institute of Standards and Technology to develop Standard Reference Material® 2917 (SRM 2917), SRM 2917 “fit for purpose” performance assessment, and implications for qPCR recreational water monitoring implementation.
Presenter: Orin Shanks, PhD, EPA Office of Research and Development Orin is a senior scientist with the EPA’s Center for Environmental Measurement and Modeling. His current research activities focus on the development and implementation of quantitative nucleic acid-based fecal pollution diagnostic tools, advances in molecular method data analyses and visualization, as well as the persistence of genetic material in environmental scenarios. In addition to research activities, he also provides technical assistance to EPA program offices and regions, states, tribes, and other local groups with an interest in clean and safe waters.
- Water Research Home
- Watersheds Research
- Nutrients and Harmful Algal Blooms Research
- Water Treatment and Infrastructure Research
- Water Research Grants
- Research Outputs
- Training, Outreach, and Engagement
- Share full article
Advertisement
Supported by
One Thing Most Countries Have in Common: Unsafe Air
New research found that fewer than 10 percent of countries and territories met World Health Organization guidelines for particulate matter pollution last year.

By Delger Erdenesanaa
Only 10 countries and territories out of 134 achieved the World Health Organization’s standards for a pervasive form of air pollution last year, according to air quality data compiled by IQAir , a Swiss company.
The pollution studied is called fine particulate matter, or PM2.5, because it refers to solid particles less than 2.5 micrometers in size: small enough to enter the bloodstream. PM2.5 is the deadliest form of air pollution, leading to millions of premature deaths each year .
“Air pollution and climate change both have the same culprit, which is fossil fuels,” said Glory Dolphin Hammes, the CEO of IQAir’s North American division.
The World Health Organization sets a guideline that people shouldn’t breathe more than 5 micrograms of fine particulate matter per cubic meter of air, on average, throughout a year. The U.S. Environmental Protection Agency recently proposed tightening its standard from 12 to 9 micrograms per cubic meter.
The few oases of clean air that meet World Health Organization guidelines are mostly islands, as well as Australia and the northern European countries of Finland and Estonia. Of the non-achievers, where the vast majority of the human population lives, the countries with the worst air quality were mostly in Asia and Africa.
Where some of the dirtiest air is found
The four most polluted countries in IQAir’s ranking for 2023 — Bangladesh, Pakistan, India and Tajikistan — are in South and Central Asia.
Air quality sensors in almost a third of the region’s cities reported concentrations of fine particulate matter that were more than 10 times the WHO guideline. This was a proportion “vastly exceeding any other region,” the report’s authors wrote.
The researchers pointed to vehicle traffic, coal and industrial emissions, particularly from brick kilns, as major sources of the region’s pollution. Farmers seasonally burning their crop waste contribute to the problem, as do households burning wood and dung for heat and cooking.
China reversed recent gains
One notable change in 2023 was a 6.3 percent increase in China’s air pollution compared with 2022, after at least five years of improvement. Beijing experienced a 14 percent increase in PM2.5 pollution last year.
The national government announced a “war against pollution” in 2014 and had been making progress ever since. But the sharpest decline in China’s PM2.5 pollution happened in 2020, when the coronavirus pandemic forced much of the country’s economic activity to slow or shut down. Ms. Dolphin Hammes attributed last year’s uptick to a reopening economy.
And challenges remain: Eleven cities in China reported air pollution levels last year that exceeded the WHO guidelines by 10 times or more. The worst was Hotan, Xinjiang.
Significant gaps in the data
IQAir researchers analyze data from more than 30,000 air quality monitoring stations and sensors across 134 countries, territories and disputed regions. Some of these monitoring stations are run by government agencies, while others are overseen by nonprofit organizations, schools, private companies and citizen scientists.
There are large gaps in ground-level air quality monitoring in Africa and the Middle East, including in regions where satellite data show some of the highest levels of air pollution on Earth.
As IQAir works to add data from more cities and countries in future years, “the worst might be yet to come in terms of what we’re measuring,” Ms. Dolphin Hammes said.
Wildfire smoke: a growing problem
Although North America is one of the cleaner regions in the world, in 2023 wildfires burned 4 percent of Canada’s forests, an area about half the size of Germany, and significantly impaired air quality.
Usually, North America’s list of most polluted cities is dominated by the United States. But last year, the top 13 spots all went to Canadian cities, many of them in Alberta.
In the United States, cities in the Upper Midwest and the Mid-Atlantic states also got significant amounts of PM2.5 pollution from wildfire smoke that drifted across the border.
Risks of short-term exposure
It’s not just chronic exposure to air pollution that harms people’s health.
For vulnerable people like the very young and old, or those with underlying illnesses, breathing in large amounts of fine particulate pollution for just a few hours or days can sometimes be deadly. About 1 million premature deaths per year can be attributed to short-term PM2.5 exposure, according to a recent global study published in The Lancet Planetary Health.
The problem is worst in East and South Asia, as well as in West Africa.
Without accounting for short-term exposures, “we might be underestimating the mortality burden from air pollution,” said Yuming Guo, a professor at Monash University in Melbourne, Australia, and one of the study’s authors.
U.S. disparities widen
Within individual countries, air pollution and its health effects aren’t evenly distributed.
Air quality in the United States has generally been improving since the Clean Air Act of the 1970s. Last decade, premature deaths from PM2.5 exposure declined to about 49,400 in 2019, down from about 69,000 in 2010.
But progress has happened faster in some communities than in others. Racial and ethnic disparities in air pollution deaths have grown in recent years, according to a national study published this month .
The census tracts in the United States with the fewest white residents have about 32 percent higher rates of PM2.5-related deaths, compared with those with the most white residents. This disparity in deaths per capita has increased by 16 percent between 2010 and 2019.
The study examined race and ethnicity separately, and found the disparity between the census tracts with the most and least Hispanic residents grew even more, by 40 percent.
In IQAir’s rankings, the United States is doing much better than most other countries. But studies that dig deeper show air quality is still an issue, said Gaige Kerr, a research scientist at George Washington University and the lead author of the disparities paper published in the journal Environmental Health Perspectives. “There’s still a lot of work to do,” he said.
Dr. Kerr’s research showed that mortality rates were highest on the Gulf Coast and in the Ohio River Valley, in areas dominated by petrochemical and manufacturing industries. He also noted that researchers have seen a slight uptick in rates of PM2.5-related deaths starting around 2016, particularly in the Western states, likely because of increasing wildfires.
Delger Erdenesanaa is a reporter covering climate and the environment and a member of the 2023-24 Times Fellowship class, a program for journalists early in their careers. More about Delger Erdenesanaa
Learn More About Climate Change
Have questions about climate change? Our F.A.Q. will tackle your climate questions, big and small .
Decades of buried trash in landfills is releasing methane , a powerful greenhouse gas, at higher rates than previously estimated, a study says.
Ocean Conservation Namibia is disentangling a record number of seals, while broadcasting the perils of marine debris in a largely feel-good way. Here’s how .
To decarbonize the electrical grid, companies are finding creative ways to store energy during periods of low demand in carbon dioxide storage balloons .
New satellite-based research reveals how land along the East Coast is slumping into the ocean, compounding the danger from global sea level rise . A major culprit: overpumping of groundwater.
Did you know the ♻ symbol doesn’t mean something is actually recyclable ? Read on about how we got here, and what can be done.
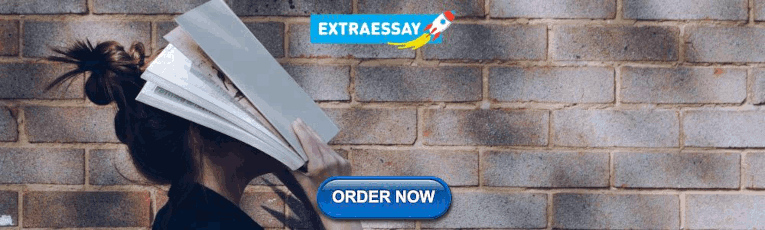
IMAGES
VIDEO
COMMENTS
Since publication in 2003 of a review 'Internal quality control: planning and implementation strategies,' 1 quality control (QC) has evolved as part of a comprehensive quality management system (QMS). The language of quality today is defined by International Standard Organization (ISO) in an effort to standardize terminology and quality management practices for world-wide applications.
8. Culture of quality and accountability: Foster a culture of quality and accountability within the laboratory. Encourage open communication, collaboration, and a commitment to continuous ...
ABSTRACT. Efforts to address a reproducibility crisis have generated several valid proposals for improving the quality of scientific research. We argue there is also need to address the separate but related issues of relevance and responsiveness.To address relevance, researchers must produce what decision makers actually need to inform investments and public policy—that is, the probability ...
Quality Control for Scientific Research: Addressing Reproducibility, Responsiveness, and Relevance. March 2019; ... with research questions (S todden 2015;H u b b a r d 2016). The.
8.1 Introduction. Quality control is an essential component of international large-scale assessment. Rigorous quality control processes help ensure high quality data and facilitate cross-country comparisons of study results. Although quality control can and does exist at all stages, including before, during, and after data collection, the term ...
Quality control can also help to make sure that the results of an experiment or method are consistent. Quality control is known as quality assurance or quality management. Good Laboratory Practice (GLP) is one form of quality control. GLP was introduced in the field of chemical research, to try to ensure high quality, reliable test data.
INTRODUCTION. Scientific research is usually initiated by posing evidenced-based research questions which are then explicitly restated as hypotheses.1,2 The hypotheses provide directions to guide the study, solutions, explanations, and expected results.3,4 Both research questions and hypotheses are essentially formulated based on conventional theories and real-world processes, which allow the ...
Going one step further, the concept of quality management in qualitative research is more anchored in the research practice itself. Quality management has been discussed for some time in the context of industrial production or services or in the health system. This approach can be transferred to qualitative research for advancing the discussion ...
Implementing quality control in a research consortium. The global COVID‐19 pandemic motivated several laboratories across Germany to join forces to address questions concerning SARS‐CoV‐2 tropism and virulence using targeted infection of 3D‐human organ models (Adhikary et al, 2021).The formed consortium, Netzwerk Universitätsmedizin—NUM—Organo‐Strat, was funded by the Federal ...
Rank the quality and feasibility of the criteria using a scale of 1-10 points (i.e., 10 being highest quality and 1 being lowest). Remember to ensure that each criterion is scored using the same scale. Finally, calculate the total score for the research question. Figure 2.
Quality control (QC) The process of verifying or checking all data, results, or reported methods to ensure their validity and correctness and to prevent erroneous conclusions; is a fundamental part of a quality assurance system or program. ... Research questions and approaches must be specifically curated to the challenge at hand and tailored ...
ABSTRACT. The objective of this article is to describe a simplified process for building and assessing the quality of healthcare-related research questions. This process consisted of three stages. The first stage aimed to select and explore a field of science. This field would be the area for which to identify outputs, such as units of analysis, variables, and objectives. The second stage ...
3.1 Protocol. The development of a comprehensive study protocol is essential to good epidemiological practice. The study protocol is a narrative document that describes the general design and procedures used in the study. It can be distinguished from the study manual of operations (Sect. 14.3.3) by its generality and absence of specific details for day-to-day study conduct.
Answer. For quality control, I would vary the deposition parameters (time, amount of source metal, deposition temperature, spin rate) and build a number of devices while varying each parameter ...
In all cases, quality control is critical. It ensures that decisions are based on evidence rather than gut feeling or bias. Standards for quality research. Over the years, researchers, scientists and authors have come to a consensus about the standards used to check the quality of research. Determined through empirical observation, theoretical ...
Quality-control questions assume that participant misbehavior in a particular moment is indicative of misbehavior throughout the entire survey. For this reason, the data quality is improved with the omission of this participant. An alternate assumption is that a degree of inattentiveness is normal throughout a survey and participants may be ...
In addition to speeder and straight-line checks, studies tend to have room for about 4-5 quality control questions. With the exception of "severe speeders" as described below, respondents will be automatically removed if they fail three or more of the checks. We use a "three strikes and you're out" rule to remove respondents.
These propositions are derived from a study of Malcolm Baldrige National Quality Award winners. The propositions are structured into four groups dealing with cultural change, focus, employee ownership of the processes, and strategic partnering with customers and suppliers. ... but instead to generate meaningful and interesting research ...
Research Question. Research Process. •To illustrate the importance of framing a good research question. • Ask. useful. answerable, valid. question. •Introduce two useful frameworks for evaluating and refining a research question •Through this endeavor: Help investigators develop valid and feasible study plan.
Quality control can be defined as "part of quality management focused on fulfilling quality requirements ." While quality assurance relates to how a process is performed or how a product is made, quality control is more the inspection aspect of quality management. An alternate definition is "the operational techniques and activities used to ...
Evers, your teachings and passion for statistical quality control and quality management systems made a great impact in my studies and also my thesis. ... Analyze Factors in Suppliers Selection for Quality Products 5 1.4. Research Questions 6 1.5. Scope 6 1.6. Methods and Procedures 7 1.7. Organization 8 . vi CHAPTER 2: LITERATURE REVIEW 10 ...
Quality Control (QC) is an indispensable aspect of various industries, ensuring that products and services adhere to predefined standards. In the manufacturing sector, QC involves rigorous inspection and testing of raw materials, intermediate components, and final products to maintain consistent quality and minimize defects.
Seven Basic Tools of Quality Control: An Appropriate Tools for Solving Quality Problems in the Organizations. Multivariate Quality Control-Estimation of the Percentage Good Products. Utility of Quality Control Tools and Statistical Process Control to Improve the Productivity and Quality in an Industry.
Review and cite AIR QUALITY CONTROL protocol, troubleshooting and other methodology information | Contact experts in AIR QUALITY CONTROL to get answers ... RESEARCH QUESTION_D.Prokopowicz_If local ...
Standard Control Material for Quantitative Real-Time PCR Recreational Water Quality Monitoring Fecal pollution remains a significant challenge for recreational water quality managers. The use of quantitative real-time PCR (qPCR) methods is increasing, allowing for same day beach water quality public notification and identification of key fecal ...
One Thing Most Countries Have in Common: Unsafe Air. New research found that fewer than 10 percent of countries and territories met World Health Organization guidelines for particulate matter ...