- Close Menu X
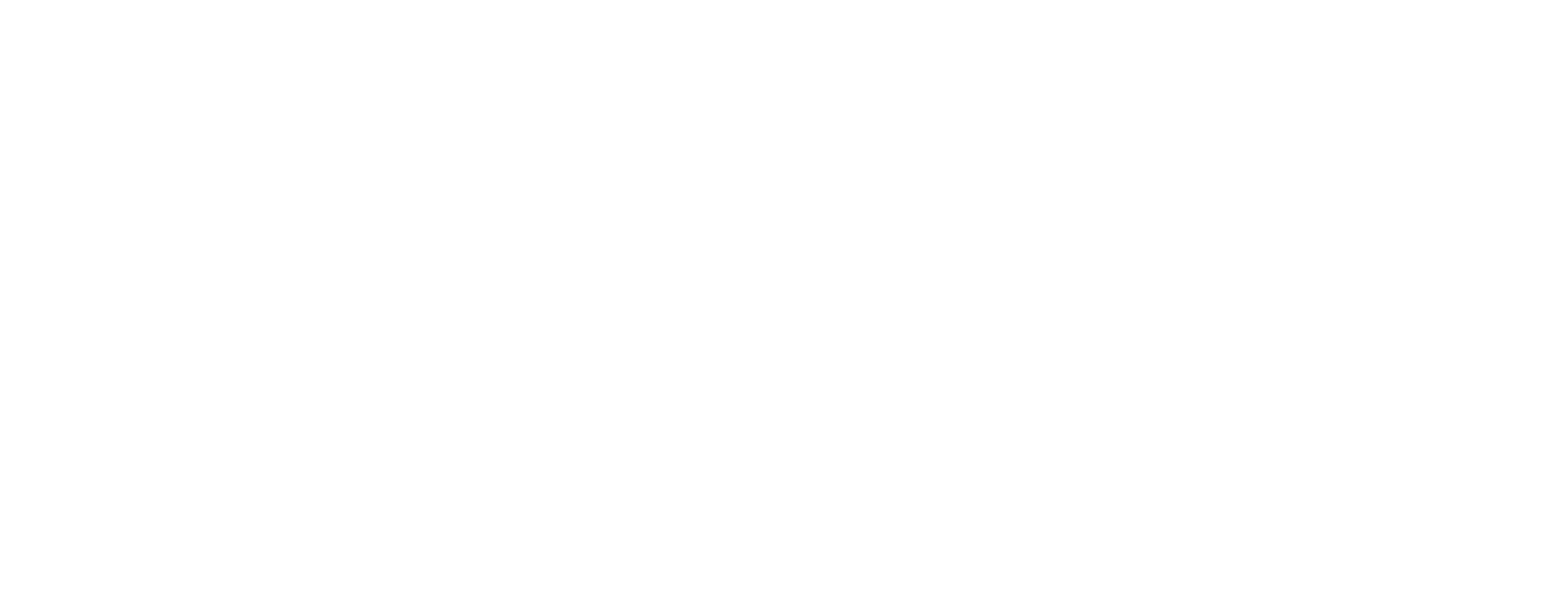
Please note: we are aware of some payment processing issues with USA based orders, we are currently working to resolve this - sorry for any inconvenience caused!
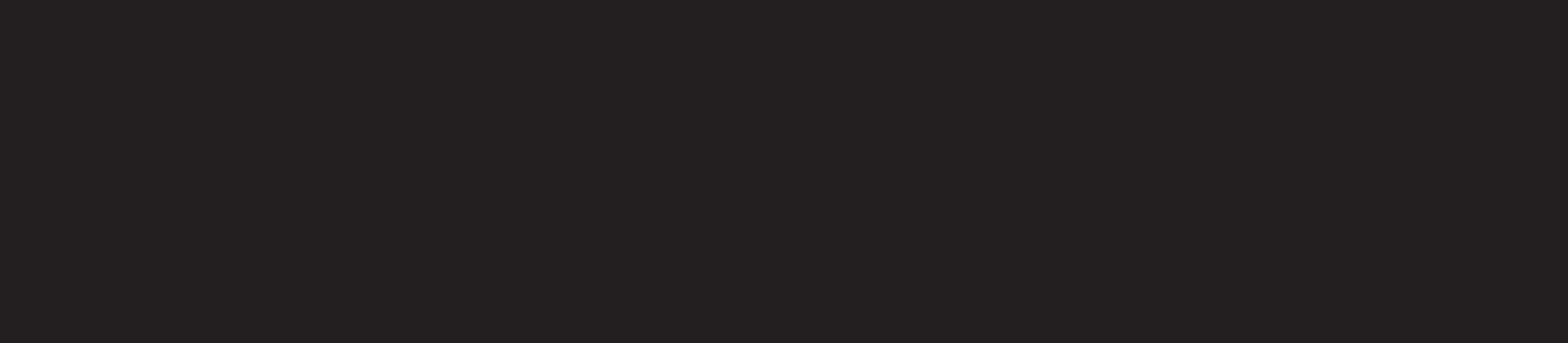
- Publish With Us
- Meet our Editorial Advisors
"[Genetically Modified Organisms: A Scientific-Political Dialogue on a Meaningless Meme is] presents the debate associated with introducing GMOs as a traditional debate between science and progress against dogma. After reading it, I hope that science will win for the sake of all of us."
- Professor David Zilberman, University of California at Berkeley
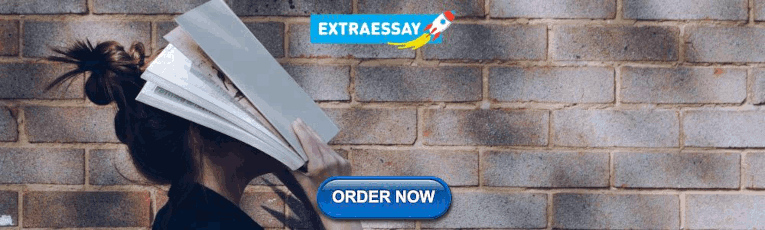
Quantitative Research Methodology in the Health Sciences

- Description
This book explains in a practical and dynamic way the relevant aspects of quantitative research methodology in health sciences. It explores research paradigms, the foundations of evidence-based clinical practice, the formulation, through different models, of the research question and objectives, definition of the scope of study, population and sample, and the classification of research variables. The book also considers different quantitative research designs, distinguishing between observational studies and experimental studies, the main quantitative data collection techniques, including some guidelines for the validation of questionnaires, descriptive statistical analysis, and inferential analysis.
It will allow the reader to understand, in a simple way, the phases of the scientific method applied to quantitative research in health, acquire basic and advanced skills for the development of a health research project from a quantitative perspective, and develop the conceptual phase with the definition of the research problem, its objectives, and working hypotheses.
Jesús Molina, PhD, lectures in the Department of Nursing and Physiotherapy at the University of the Balearic Islands, and is a Scientific Advisor to the Center for Innovation and Development of Nursing and Physiotherapy of the Balearic Islands (CIDEFIB). He holds a PhD in Social and Health Sciences and Master’s degrees in Research Methodology in Health Sciences, Medical Sciences and Health Sciences, and Bioethics. He is the author of over 8 books and 50 book chapters, as well as 60 journal articles. In addition, he is the recipient of a number of honors and research awards.
There are currently no reviews for this title. Please do revisit this page again to see if some have been added.
Buy This Book

ISBN: 1-5275-8198-5
ISBN13: 978-1-5275-8198-2
Release Date: 4th May 2022
Price: £59.99
New and Forthcoming

- Sales Agents
- Unsubscribe
Cambridge Scholars Publishing | Registration Number: 04333775
Please note that Cambridge Scholars Publishing Limited is not affiliated to or associated with Cambridge University Press or the University of Cambridge
Copyright © 2024 Cambridge Scholars Publishing. All rights reserved.
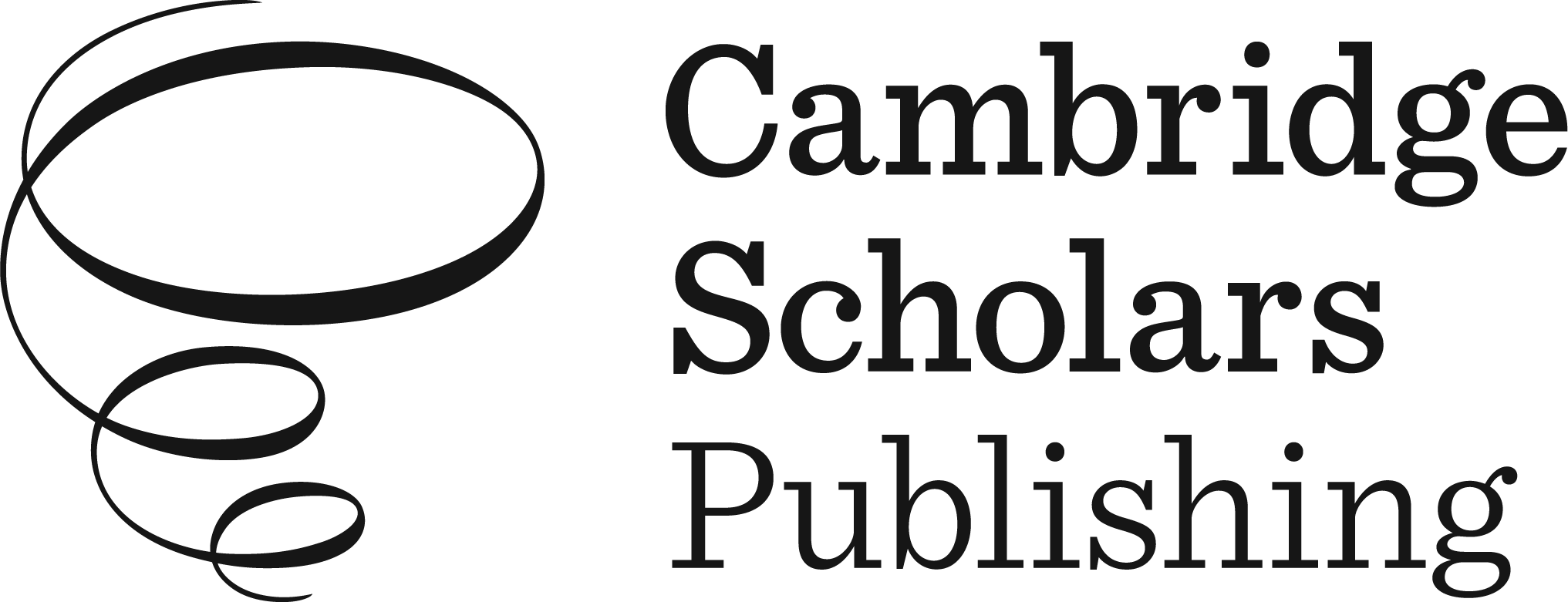
Designed and Built by Prime Creative
Please fill in the short form below for any enquiries.
Sign up for our newsletter
Please enter your email address below;
Quantitative Health Sciences
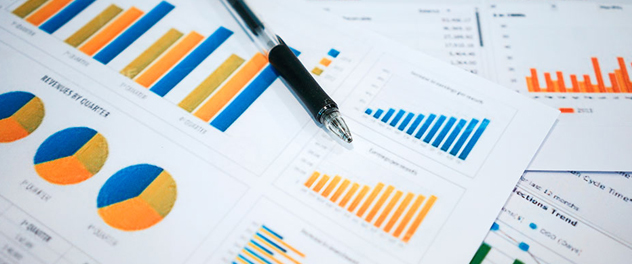
Improving patient care through medical research
Investigations in the Department of Quantitative Health Sciences encompass collaborative studies, methodological and applied research, development of institutional resources, clinical research career development, and clinical practice efforts.
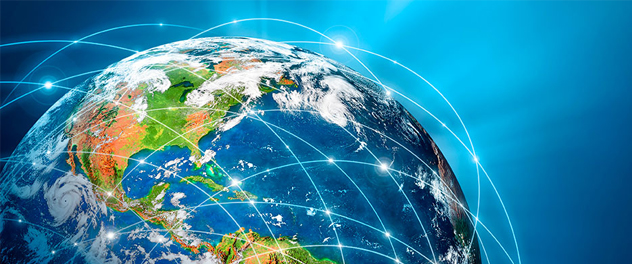
Solving real-world problems in clinical research studies
Investigators from the Department of Quantitative Health Sciences play key leadership roles in many of Mayo Clinic's clinical research programs, including studies on cancer; neurology; heart, lung and blood disorders; gastrointestinal diseases; endocrine and metabolic conditions; kidney and urinary disorders; musculoskeletal disorders; and genetic epidemiology.
Reengineering the clinical research enterprise
With funding from the National Institutes of Health (NIH), Department of Quantitative Health Sciences faculty members lead clinical research education and career development programs as part of a national initiative to develop the next generation of clinical researchers.
The Department of Quantitative Health Sciences at Mayo Clinic is a multidisciplinary group with hundreds of staff members, located in Phoenix/Scottsdale, Arizona; Jacksonville, Florida; and Rochester, Minnesota, who are dedicated to improving patient care through medical research.
The department's work encompasses:
- Faculty-led research programs.
- Collaborative research.
- Methodological and applied research.
- Development of institutional research resources.
- Clinical research career development.
- Clinical practice efforts.
Solving current clinical problems
Evolving from the department's rich heritage, Quantitative Health Sciences investigators today play key leadership roles in many of Mayo's clinical research programs, including programs in:
- Endocrine and metabolic conditions.
- Gastrointestinal diseases.
- Genomics, especially genetic epidemiology.
- Heart, lung and blood disorders.
- Kidney and urinary disorders.
- Musculoskeletal disorders.
Faculty and staff members also conduct leading-edge methods of research on a wide range of areas, such as:
- Analysis of DNA microarray data.
- Attributable risk.
- Bioinformatics.
- Clinical trial design.
- Genetic analysis methods.
- Health economics and utilization.
- Medical terminology standards.
- Molecular epidemiology of various conditions.
- Natural language processing.
- Outcomes analyses.
- Proteomics.
- Quality-of-life measures.
- Survival analysis.
Training future researchers
Faculty members in the Department of Quantitative Health Sciences lead Mayo's clinical research education and career development efforts. These NIH -funded programs are part of a national initiative to reengineer the clinical research enterprise and are directed toward developing the next generation of clinical researchers.
The Department of Quantitative Health Sciences is an exciting and unique research area at Mayo Clinic. The department encourages prospective students, employees and visitors to inquire for more information .
The Mayo Clinic Department of Quantitative Health Sciences has a rich heritage dating back to Joseph Berkson, M.D., (chair, 1933-1964) and Leonard T. Kurland, M.D. (chair, 1964-1987). In 1935, Dr. Berkson developed a computerized medical diagnostic coding system, capturing all patient diagnoses and procedures to efficiently facilitate clinical research.
Building on Dr. Berkson's work, in 1966 Dr. Kurland initiated the Rochester Epidemiology Project (REP) , which constitutes an unparalleled resource for population-based epidemiologic studies. The REP has received continuous funding from the National Institutes of Health (NIH) for longer than 40 years, resulting in more than 1,500 peer-reviewed publications to date.
Learn more about the department's distinguished history .
Contact the Department of Quantitative Health Sciences at Mayo Clinic with questions about research services, collaborations, or education and career opportunities.
Research in the Department of Quantitative Health Sciences is carried out in the Division of Clinical Trials and Biostatistics, the Division of Computational Biology, the Division of Epidemiology, and the Section of Biomedical Ethics.
Learn about faculty-level and allied health career opportunities in the Department of Quantitative Health Sciences at Mayo Clinic.
The Department of Quantitative Health Sciences is studying a variety of topics. Focus areas include genetics, genomics, proteomics and metabolomics, and digital pathology. The department offers specialized protocols, methodologies and expertise to assist research teams. These include software, technical reports and other resources.
- Focus Areas - Research Focus Areas
- Software - Research Software
- Technical Reports - Research Technical Reports
- Publications - Research Publications
Core Services
- Bioinformatics Core - Core Services Bioinformatics Core
- Biostatistics Core - Core Services Biostatistics Core
- Survey Research Center - Core Services Survey Research Center
Affiliations
The Department of Quantitative Health Sciences is affiliated with multiple Mayo Clinic research groups:
- Biomedical Ethics Research Program - Affiliations Biomedical Ethics Research Program
- Center for Clinical and Translational Science (CCaTS) - Affiliations Center for Clinical and Translational Science (CCaTS)
- Center for Individualized Medicine - Affiliations Center for Individualized Medicine
- Mayo Clinic Comprehensive Cancer Center — Research - Affiliations Mayo Clinic Comprehensive Cancer Center — Research
- Robert D. and Patricia E. Kern Center for the Science of Health Care Delivery - Affiliations Robert D. and Patricia E. Kern Center for the Science of Health Care Delivery
- Rochester Epidemiology Project (REP) - Affiliations Rochester Epidemiology Project (REP)
More about research at Mayo Clinic
- Research Faculty
- Laboratories
- Core Facilities
- Centers & Programs
- Departments & Divisions
- Clinical Trials
- Institutional Review Board
- Postdoctoral Fellowships
- Training Grant Programs
- Publications
Mayo Clinic Footer
- Request Appointment
- About Mayo Clinic
- About This Site
Legal Conditions and Terms
- Terms and Conditions
- Privacy Policy
- Notice of Privacy Practices
- Notice of Nondiscrimination
- Manage Cookies
Advertising
Mayo Clinic is a nonprofit organization and proceeds from Web advertising help support our mission. Mayo Clinic does not endorse any of the third party products and services advertised.
- Advertising and sponsorship policy
- Advertising and sponsorship opportunities
Reprint Permissions
A single copy of these materials may be reprinted for noncommercial personal use only. "Mayo," "Mayo Clinic," "MayoClinic.org," "Mayo Clinic Healthy Living," and the triple-shield Mayo Clinic logo are trademarks of Mayo Foundation for Medical Education and Research.
Click through the PLOS taxonomy to find articles in your field.
For more information about PLOS Subject Areas, click here .
Loading metrics
Open Access
Peer-reviewed
Research Article
Recent quantitative research on determinants of health in high income countries: A scoping review
Roles Conceptualization, Data curation, Formal analysis, Investigation, Methodology, Project administration, Software, Visualization, Writing – original draft, Writing – review & editing
* E-mail: [email protected]
Affiliation Centre for Health Economics Research and Modelling Infectious Diseases, Vaccine and Infectious Disease Institute, University of Antwerp, Antwerp, Belgium

Roles Conceptualization, Data curation, Funding acquisition, Project administration, Resources, Supervision, Validation, Visualization, Writing – review & editing
- Vladimira Varbanova,
- Philippe Beutels
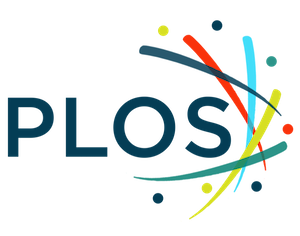
- Published: September 17, 2020
- https://doi.org/10.1371/journal.pone.0239031
- Peer Review
- Reader Comments
Identifying determinants of health and understanding their role in health production constitutes an important research theme. We aimed to document the state of recent multi-country research on this theme in the literature.
We followed the PRISMA-ScR guidelines to systematically identify, triage and review literature (January 2013—July 2019). We searched for studies that performed cross-national statistical analyses aiming to evaluate the impact of one or more aggregate level determinants on one or more general population health outcomes in high-income countries. To assess in which combinations and to what extent individual (or thematically linked) determinants had been studied together, we performed multidimensional scaling and cluster analysis.
Sixty studies were selected, out of an original yield of 3686. Life-expectancy and overall mortality were the most widely used population health indicators, while determinants came from the areas of healthcare, culture, politics, socio-economics, environment, labor, fertility, demographics, life-style, and psychology. The family of regression models was the predominant statistical approach. Results from our multidimensional scaling showed that a relatively tight core of determinants have received much attention, as main covariates of interest or controls, whereas the majority of other determinants were studied in very limited contexts. We consider findings from these studies regarding the importance of any given health determinant inconclusive at present. Across a multitude of model specifications, different country samples, and varying time periods, effects fluctuated between statistically significant and not significant, and between beneficial and detrimental to health.
Conclusions
We conclude that efforts to understand the underlying mechanisms of population health are far from settled, and the present state of research on the topic leaves much to be desired. It is essential that future research considers multiple factors simultaneously and takes advantage of more sophisticated methodology with regards to quantifying health as well as analyzing determinants’ influence.
Citation: Varbanova V, Beutels P (2020) Recent quantitative research on determinants of health in high income countries: A scoping review. PLoS ONE 15(9): e0239031. https://doi.org/10.1371/journal.pone.0239031
Editor: Amir Radfar, University of Central Florida, UNITED STATES
Received: November 14, 2019; Accepted: August 28, 2020; Published: September 17, 2020
Copyright: © 2020 Varbanova, Beutels. This is an open access article distributed under the terms of the Creative Commons Attribution License , which permits unrestricted use, distribution, and reproduction in any medium, provided the original author and source are credited.
Data Availability: All relevant data are within the manuscript and its Supporting Information files.
Funding: This study (and VV) is funded by the Research Foundation Flanders ( https://www.fwo.be/en/ ), FWO project number G0D5917N, award obtained by PB. The funder had no role in study design, data collection and analysis, decision to publish, or preparation of the manuscript.
Competing interests: The authors have declared that no competing interests exist.
Introduction
Identifying the key drivers of population health is a core subject in public health and health economics research. Between-country comparative research on the topic is challenging. In order to be relevant for policy, it requires disentangling different interrelated drivers of “good health”, each having different degrees of importance in different contexts.
“Good health”–physical and psychological, subjective and objective–can be defined and measured using a variety of approaches, depending on which aspect of health is the focus. A major distinction can be made between health measurements at the individual level or some aggregate level, such as a neighborhood, a region or a country. In view of this, a great diversity of specific research topics exists on the drivers of what constitutes individual or aggregate “good health”, including those focusing on health inequalities, the gender gap in longevity, and regional mortality and longevity differences.
The current scoping review focuses on determinants of population health. Stated as such, this topic is quite broad. Indeed, we are interested in the very general question of what methods have been used to make the most of increasingly available region or country-specific databases to understand the drivers of population health through inter-country comparisons. Existing reviews indicate that researchers thus far tend to adopt a narrower focus. Usually, attention is given to only one health outcome at a time, with further geographical and/or population [ 1 , 2 ] restrictions. In some cases, the impact of one or more interventions is at the core of the review [ 3 – 7 ], while in others it is the relationship between health and just one particular predictor, e.g., income inequality, access to healthcare, government mechanisms [ 8 – 13 ]. Some relatively recent reviews on the subject of social determinants of health [ 4 – 6 , 14 – 17 ] have considered a number of indicators potentially influencing health as opposed to a single one. One review defines “social determinants” as “the social, economic, and political conditions that influence the health of individuals and populations” [ 17 ] while another refers even more broadly to “the factors apart from medical care” [ 15 ].
In the present work, we aimed to be more inclusive, setting no limitations on the nature of possible health correlates, as well as making use of a multitude of commonly accepted measures of general population health. The goal of this scoping review was to document the state of the art in the recent published literature on determinants of population health, with a particular focus on the types of determinants selected and the methodology used. In doing so, we also report the main characteristics of the results these studies found. The materials collected in this review are intended to inform our (and potentially other researchers’) future analyses on this topic. Since the production of health is subject to the law of diminishing marginal returns, we focused our review on those studies that included countries where a high standard of wealth has been achieved for some time, i.e., high-income countries belonging to the Organisation for Economic Co-operation and Development (OECD) or Europe. Adding similar reviews for other country income groups is of limited interest to the research we plan to do in this area.
In view of its focus on data and methods, rather than results, a formal protocol was not registered prior to undertaking this review, but the procedure followed the guidelines of the PRISMA statement for scoping reviews [ 18 ].
We focused on multi-country studies investigating the potential associations between any aggregate level (region/city/country) determinant and general measures of population health (e.g., life expectancy, mortality rate).
Within the query itself, we listed well-established population health indicators as well as the six world regions, as defined by the World Health Organization (WHO). We searched only in the publications’ titles in order to keep the number of hits manageable, and the ratio of broadly relevant abstracts over all abstracts in the order of magnitude of 10% (based on a series of time-focused trial runs). The search strategy was developed iteratively between the two authors and is presented in S1 Appendix . The search was performed by VV in PubMed and Web of Science on the 16 th of July, 2019, without any language restrictions, and with a start date set to the 1 st of January, 2013, as we were interested in the latest developments in this area of research.
Eligibility criteria
Records obtained via the search methods described above were screened independently by the two authors. Consistency between inclusion/exclusion decisions was approximately 90% and the 43 instances where uncertainty existed were judged through discussion. Articles were included subject to meeting the following requirements: (a) the paper was a full published report of an original empirical study investigating the impact of at least one aggregate level (city/region/country) factor on at least one health indicator (or self-reported health) of the general population (the only admissible “sub-populations” were those based on gender and/or age); (b) the study employed statistical techniques (calculating correlations, at the very least) and was not purely descriptive or theoretical in nature; (c) the analysis involved at least two countries or at least two regions or cities (or another aggregate level) in at least two different countries; (d) the health outcome was not differentiated according to some socio-economic factor and thus studied in terms of inequality (with the exception of gender and age differentiations); (e) mortality, in case it was one of the health indicators under investigation, was strictly “total” or “all-cause” (no cause-specific or determinant-attributable mortality).
Data extraction
The following pieces of information were extracted in an Excel table from the full text of each eligible study (primarily by VV, consulting with PB in case of doubt): health outcome(s), determinants, statistical methodology, level of analysis, results, type of data, data sources, time period, countries. The evidence is synthesized according to these extracted data (often directly reflected in the section headings), using a narrative form accompanied by a “summary-of-findings” table and a graph.
Search and selection
The initial yield contained 4583 records, reduced to 3686 after removal of duplicates ( Fig 1 ). Based on title and abstract screening, 3271 records were excluded because they focused on specific medical condition(s) or specific populations (based on morbidity or some other factor), dealt with intervention effectiveness, with theoretical or non-health related issues, or with animals or plants. Of the remaining 415 papers, roughly half were disqualified upon full-text consideration, mostly due to using an outcome not of interest to us (e.g., health inequality), measuring and analyzing determinants and outcomes exclusively at the individual level, performing analyses one country at a time, employing indices that are a mixture of both health indicators and health determinants, or not utilizing potential health determinants at all. After this second stage of the screening process, 202 papers were deemed eligible for inclusion. This group was further dichotomized according to level of economic development of the countries or regions under study, using membership of the OECD or Europe as a reference “cut-off” point. Sixty papers were judged to include high-income countries, and the remaining 142 included either low- or middle-income countries or a mix of both these levels of development. The rest of this report outlines findings in relation to high-income countries only, reflecting our own primary research interests. Nonetheless, we chose to report our search yield for the other income groups for two reasons. First, to gauge the relative interest in applied published research for these different income levels; and second, to enable other researchers with a focus on determinants of health in other countries to use the extraction we made here.
- PPT PowerPoint slide
- PNG larger image
- TIFF original image
https://doi.org/10.1371/journal.pone.0239031.g001
Health outcomes
The most frequent population health indicator, life expectancy (LE), was present in 24 of the 60 studies. Apart from “life expectancy at birth” (representing the average life-span a newborn is expected to have if current mortality rates remain constant), also called “period LE” by some [ 19 , 20 ], we encountered as well LE at 40 years of age [ 21 ], at 60 [ 22 ], and at 65 [ 21 , 23 , 24 ]. In two papers, the age-specificity of life expectancy (be it at birth or another age) was not stated [ 25 , 26 ].
Some studies considered male and female LE separately [ 21 , 24 , 25 , 27 – 33 ]. This consideration was also often observed with the second most commonly used health index [ 28 – 30 , 34 – 38 ]–termed “total”, or “overall”, or “all-cause”, mortality rate (MR)–included in 22 of the 60 studies. In addition to gender, this index was also sometimes broken down according to age group [ 30 , 39 , 40 ], as well as gender-age group [ 38 ].
While the majority of studies under review here focused on a single health indicator, 23 out of the 60 studies made use of multiple outcomes, although these outcomes were always considered one at a time, and sometimes not all of them fell within the scope of our review. An easily discernable group of indices that typically went together [ 25 , 37 , 41 ] was that of neonatal (deaths occurring within 28 days postpartum), perinatal (fetal or early neonatal / first-7-days deaths), and post-neonatal (deaths between the 29 th day and completion of one year of life) mortality. More often than not, these indices were also accompanied by “stand-alone” indicators, such as infant mortality (deaths within the first year of life; our third most common index found in 16 of the 60 studies), maternal mortality (deaths during pregnancy or within 42 days of termination of pregnancy), and child mortality rates. Child mortality has conventionally been defined as mortality within the first 5 years of life, thus often also called “under-5 mortality”. Nonetheless, Pritchard & Wallace used the term “child mortality” to denote deaths of children younger than 14 years [ 42 ].
As previously stated, inclusion criteria did allow for self-reported health status to be used as a general measure of population health. Within our final selection of studies, seven utilized some form of subjective health as an outcome variable [ 25 , 43 – 48 ]. Additionally, the Health Human Development Index [ 49 ], healthy life expectancy [ 50 ], old-age survival [ 51 ], potential years of life lost [ 52 ], and disability-adjusted life expectancy [ 25 ] were also used.
We note that while in most cases the indicators mentioned above (and/or the covariates considered, see below) were taken in their absolute or logarithmic form, as a—typically annual—number, sometimes they were used in the form of differences, change rates, averages over a given time period, or even z-scores of rankings [ 19 , 22 , 40 , 42 , 44 , 53 – 57 ].
Regions, countries, and populations
Despite our decision to confine this review to high-income countries, some variation in the countries and regions studied was still present. Selection seemed to be most often conditioned on the European Union, or the European continent more generally, and the Organisation of Economic Co-operation and Development (OECD), though, typically, not all member nations–based on the instances where these were also explicitly listed—were included in a given study. Some of the stated reasons for omitting certain nations included data unavailability [ 30 , 45 , 54 ] or inconsistency [ 20 , 58 ], Gross Domestic Product (GDP) too low [ 40 ], differences in economic development and political stability with the rest of the sampled countries [ 59 ], and national population too small [ 24 , 40 ]. On the other hand, the rationales for selecting a group of countries included having similar above-average infant mortality [ 60 ], similar healthcare systems [ 23 ], and being randomly drawn from a social spending category [ 61 ]. Some researchers were interested explicitly in a specific geographical region, such as Eastern Europe [ 50 ], Central and Eastern Europe [ 48 , 60 ], the Visegrad (V4) group [ 62 ], or the Asia/Pacific area [ 32 ]. In certain instances, national regions or cities, rather than countries, constituted the units of investigation instead [ 31 , 51 , 56 , 62 – 66 ]. In two particular cases, a mix of countries and cities was used [ 35 , 57 ]. In another two [ 28 , 29 ], due to the long time periods under study, some of the included countries no longer exist. Finally, besides “European” and “OECD”, the terms “developed”, “Western”, and “industrialized” were also used to describe the group of selected nations [ 30 , 42 , 52 , 53 , 67 ].
As stated above, it was the health status of the general population that we were interested in, and during screening we made a concerted effort to exclude research using data based on a more narrowly defined group of individuals. All studies included in this review adhere to this general rule, albeit with two caveats. First, as cities (even neighborhoods) were the unit of analysis in three of the studies that made the selection [ 56 , 64 , 65 ], the populations under investigation there can be more accurately described as general urban , instead of just general. Second, oftentimes health indicators were stratified based on gender and/or age, therefore we also admitted one study that, due to its specific research question, focused on men and women of early retirement age [ 35 ] and another that considered adult males only [ 68 ].
Data types and sources
A great diversity of sources was utilized for data collection purposes. The accessible reference databases of the OECD ( https://www.oecd.org/ ), WHO ( https://www.who.int/ ), World Bank ( https://www.worldbank.org/ ), United Nations ( https://www.un.org/en/ ), and Eurostat ( https://ec.europa.eu/eurostat ) were among the top choices. The other international databases included Human Mortality [ 30 , 39 , 50 ], Transparency International [ 40 , 48 , 50 ], Quality of Government [ 28 , 69 ], World Income Inequality [ 30 ], International Labor Organization [ 41 ], International Monetary Fund [ 70 ]. A number of national databases were referred to as well, for example the US Bureau of Statistics [ 42 , 53 ], Korean Statistical Information Services [ 67 ], Statistics Canada [ 67 ], Australian Bureau of Statistics [ 67 ], and Health New Zealand Tobacco control and Health New Zealand Food and Nutrition [ 19 ]. Well-known surveys, such as the World Values Survey [ 25 , 55 ], the European Social Survey [ 25 , 39 , 44 ], the Eurobarometer [ 46 , 56 ], the European Value Survey [ 25 ], and the European Statistics of Income and Living Condition Survey [ 43 , 47 , 70 ] were used as data sources, too. Finally, in some cases [ 25 , 28 , 29 , 35 , 36 , 41 , 69 ], built-for-purpose datasets from previous studies were re-used.
In most of the studies, the level of the data (and analysis) was national. The exceptions were six papers that dealt with Nomenclature of Territorial Units of Statistics (NUTS2) regions [ 31 , 62 , 63 , 66 ], otherwise defined areas [ 51 ] or cities [ 56 ], and seven others that were multilevel designs and utilized both country- and region-level data [ 57 ], individual- and city- or country-level [ 35 ], individual- and country-level [ 44 , 45 , 48 ], individual- and neighborhood-level [ 64 ], and city-region- (NUTS3) and country-level data [ 65 ]. Parallel to that, the data type was predominantly longitudinal, with only a few studies using purely cross-sectional data [ 25 , 33 , 43 , 45 – 48 , 50 , 62 , 67 , 68 , 71 , 72 ], albeit in four of those [ 43 , 48 , 68 , 72 ] two separate points in time were taken (thus resulting in a kind of “double cross-section”), while in another the averages across survey waves were used [ 56 ].
In studies using longitudinal data, the length of the covered time periods varied greatly. Although this was almost always less than 40 years, in one study it covered the entire 20 th century [ 29 ]. Longitudinal data, typically in the form of annual records, was sometimes transformed before usage. For example, some researchers considered data points at 5- [ 34 , 36 , 49 ] or 10-year [ 27 , 29 , 35 ] intervals instead of the traditional 1, or took averages over 3-year periods [ 42 , 53 , 73 ]. In one study concerned with the effect of the Great Recession all data were in a “recession minus expansion change in trends”-form [ 57 ]. Furthermore, there were a few instances where two different time periods were compared to each other [ 42 , 53 ] or when data was divided into 2 to 4 (possibly overlapping) periods which were then analyzed separately [ 24 , 26 , 28 , 29 , 31 , 65 ]. Lastly, owing to data availability issues, discrepancies between the time points or periods of data on the different variables were occasionally observed [ 22 , 35 , 42 , 53 – 55 , 63 ].
Health determinants
Together with other essential details, Table 1 lists the health correlates considered in the selected studies. Several general categories for these correlates can be discerned, including health care, political stability, socio-economics, demographics, psychology, environment, fertility, life-style, culture, labor. All of these, directly or implicitly, have been recognized as holding importance for population health by existing theoretical models of (social) determinants of health [ 74 – 77 ].
https://doi.org/10.1371/journal.pone.0239031.t001
It is worth noting that in a few studies there was just a single aggregate-level covariate investigated in relation to a health outcome of interest to us. In one instance, this was life satisfaction [ 44 ], in another–welfare system typology [ 45 ], but also gender inequality [ 33 ], austerity level [ 70 , 78 ], and deprivation [ 51 ]. Most often though, attention went exclusively to GDP [ 27 , 29 , 46 , 57 , 65 , 71 ]. It was often the case that research had a more particular focus. Among others, minimum wages [ 79 ], hospital payment schemes [ 23 ], cigarette prices [ 63 ], social expenditure [ 20 ], residents’ dissatisfaction [ 56 ], income inequality [ 30 , 69 ], and work leave [ 41 , 58 ] took center stage. Whenever variables outside of these specific areas were also included, they were usually identified as confounders or controls, moderators or mediators.
We visualized the combinations in which the different determinants have been studied in Fig 2 , which was obtained via multidimensional scaling and a subsequent cluster analysis (details outlined in S2 Appendix ). It depicts the spatial positioning of each determinant relative to all others, based on the number of times the effects of each pair of determinants have been studied simultaneously. When interpreting Fig 2 , one should keep in mind that determinants marked with an asterisk represent, in fact, collectives of variables.
Groups of determinants are marked by asterisks (see S1 Table in S1 Appendix ). Diminishing color intensity reflects a decrease in the total number of “connections” for a given determinant. Noteworthy pairwise “connections” are emphasized via lines (solid-dashed-dotted indicates decreasing frequency). Grey contour lines encircle groups of variables that were identified via cluster analysis. Abbreviations: age = population age distribution, associations = membership in associations, AT-index = atherogenic-thrombogenic index, BR = birth rate, CAPB = Cyclically Adjusted Primary Balance, civilian-labor = civilian labor force, C-section = Cesarean delivery rate, credit-info = depth of credit information, dissatisf = residents’ dissatisfaction, distrib.orient = distributional orientation, EDU = education, eHealth = eHealth index at GP-level, exch.rate = exchange rate, fat = fat consumption, GDP = gross domestic product, GFCF = Gross Fixed Capital Formation/Creation, GH-gas = greenhouse gas, GII = gender inequality index, gov = governance index, gov.revenue = government revenues, HC-coverage = healthcare coverage, HE = health(care) expenditure, HHconsump = household consumption, hosp.beds = hospital beds, hosp.payment = hospital payment scheme, hosp.stay = length of hospital stay, IDI = ICT development index, inc.ineq = income inequality, industry-labor = industrial labor force, infant-sex = infant sex ratio, labor-product = labor production, LBW = low birth weight, leave = work leave, life-satisf = life satisfaction, M-age = maternal age, marginal-tax = marginal tax rate, MDs = physicians, mult.preg = multiple pregnancy, NHS = Nation Health System, NO = nitrous oxide emissions, PM10 = particulate matter (PM10) emissions, pop = population size, pop.density = population density, pre-term = pre-term birth rate, prison = prison population, researchE = research&development expenditure, school.ref = compulsory schooling reform, smoke-free = smoke-free places, SO = sulfur oxide emissions, soc.E = social expenditure, soc.workers = social workers, sugar = sugar consumption, terror = terrorism, union = union density, UR = unemployment rate, urban = urbanization, veg-fr = vegetable-and-fruit consumption, welfare = welfare regime, Wwater = wastewater treatment.
https://doi.org/10.1371/journal.pone.0239031.g002
Distances between determinants in Fig 2 are indicative of determinants’ “connectedness” with each other. While the statistical procedure called for higher dimensionality of the model, for demonstration purposes we show here a two-dimensional solution. This simplification unfortunately comes with a caveat. To use the factor smoking as an example, it would appear it stands at a much greater distance from GDP than it does from alcohol. In reality however, smoking was considered together with alcohol consumption [ 21 , 25 , 26 , 52 , 68 ] in just as many studies as it was with GDP [ 21 , 25 , 26 , 52 , 59 ], five. To aid with respect to this apparent shortcoming, we have emphasized the strongest pairwise links. Solid lines connect GDP with health expenditure (HE), unemployment rate (UR), and education (EDU), indicating that the effect of GDP on health, taking into account the effects of the other three determinants as well, was evaluated in between 12 to 16 studies of the 60 included in this review. Tracing the dashed lines, we can also tell that GDP appeared jointly with income inequality, and HE together with either EDU or UR, in anywhere between 8 to 10 of our selected studies. Finally, some weaker but still worth-mentioning “connections” between variables are displayed as well via the dotted lines.
The fact that all notable pairwise “connections” are concentrated within a relatively small region of the plot may be interpreted as low overall “connectedness” among the health indicators studied. GDP is the most widely investigated determinant in relation to general population health. Its total number of “connections” is disproportionately high (159) compared to its runner-up–HE (with 113 “connections”), and then subsequently EDU (with 90) and UR (with 86). In fact, all of these determinants could be thought of as outliers, given that none of the remaining factors have a total count of pairings above 52. This decrease in individual determinants’ overall “connectedness” can be tracked on the graph via the change of color intensity as we move outwards from the symbolic center of GDP and its closest “co-determinants”, to finally reach the other extreme of the ten indicators (welfare regime, household consumption, compulsory school reform, life satisfaction, government revenues, literacy, research expenditure, multiple pregnancy, Cyclically Adjusted Primary Balance, and residents’ dissatisfaction; in white) the effects on health of which were only studied in isolation.
Lastly, we point to the few small but stable clusters of covariates encircled by the grey bubbles on Fig 2 . These groups of determinants were identified as “close” by both statistical procedures used for the production of the graph (see details in S2 Appendix ).
Statistical methodology
There was great variation in the level of statistical detail reported. Some authors provided too vague a description of their analytical approach, necessitating some inference in this section.
The issue of missing data is a challenging reality in this field of research, but few of the studies under review (12/60) explain how they dealt with it. Among the ones that do, three general approaches to handling missingness can be identified, listed in increasing level of sophistication: case-wise deletion, i.e., removal of countries from the sample [ 20 , 45 , 48 , 58 , 59 ], (linear) interpolation [ 28 , 30 , 34 , 58 , 59 , 63 ], and multiple imputation [ 26 , 41 , 52 ].
Correlations, Pearson, Spearman, or unspecified, were the only technique applied with respect to the health outcomes of interest in eight analyses [ 33 , 42 – 44 , 46 , 53 , 57 , 61 ]. Among the more advanced statistical methods, the family of regression models proved to be, by and large, predominant. Before examining this closer, we note the techniques that were, in a way, “unique” within this selection of studies: meta-analyses were performed (random and fixed effects, respectively) on the reduced form and 2-sample two stage least squares (2SLS) estimations done within countries [ 39 ]; difference-in-difference (DiD) analysis was applied in one case [ 23 ]; dynamic time-series methods, among which co-integration, impulse-response function (IRF), and panel vector autoregressive (VAR) modeling, were utilized in one study [ 80 ]; longitudinal generalized estimating equation (GEE) models were developed on two occasions [ 70 , 78 ]; hierarchical Bayesian spatial models [ 51 ] and special autoregressive regression [ 62 ] were also implemented.
Purely cross-sectional data analyses were performed in eight studies [ 25 , 45 , 47 , 50 , 55 , 56 , 67 , 71 ]. These consisted of linear regression (assumed ordinary least squares (OLS)), generalized least squares (GLS) regression, and multilevel analyses. However, six other studies that used longitudinal data in fact had a cross-sectional design, through which they applied regression at multiple time-points separately [ 27 , 29 , 36 , 48 , 68 , 72 ].
Apart from these “multi-point cross-sectional studies”, some other simplistic approaches to longitudinal data analysis were found, involving calculating and regressing 3-year averages of both the response and the predictor variables [ 54 ], taking the average of a few data-points (i.e., survey waves) [ 56 ] or using difference scores over 10-year [ 19 , 29 ] or unspecified time intervals [ 40 , 55 ].
Moving further in the direction of more sensible longitudinal data usage, we turn to the methods widely known among (health) economists as “panel data analysis” or “panel regression”. Most often seen were models with fixed effects for country/region and sometimes also time-point (occasionally including a country-specific trend as well), with robust standard errors for the parameter estimates to take into account correlations among clustered observations [ 20 , 21 , 24 , 28 , 30 , 32 , 34 , 37 , 38 , 41 , 52 , 59 , 60 , 63 , 66 , 69 , 73 , 79 , 81 , 82 ]. The Hausman test [ 83 ] was sometimes mentioned as the tool used to decide between fixed and random effects [ 26 , 49 , 63 , 66 , 73 , 82 ]. A few studies considered the latter more appropriate for their particular analyses, with some further specifying that (feasible) GLS estimation was employed [ 26 , 34 , 49 , 58 , 60 , 73 ]. Apart from these two types of models, the first differences method was encountered once as well [ 31 ]. Across all, the error terms were sometimes assumed to come from a first-order autoregressive process (AR(1)), i.e., they were allowed to be serially correlated [ 20 , 30 , 38 , 58 – 60 , 73 ], and lags of (typically) predictor variables were included in the model specification, too [ 20 , 21 , 37 , 38 , 48 , 69 , 81 ]. Lastly, a somewhat different approach to longitudinal data analysis was undertaken in four studies [ 22 , 35 , 48 , 65 ] in which multilevel–linear or Poisson–models were developed.
Regardless of the exact techniques used, most studies included in this review presented multiple model applications within their main analysis. None attempted to formally compare models in order to identify the “best”, even if goodness-of-fit statistics were occasionally reported. As indicated above, many studies investigated women’s and men’s health separately [ 19 , 21 , 22 , 27 – 29 , 31 , 33 , 35 , 36 , 38 , 39 , 45 , 50 , 51 , 64 , 65 , 69 , 82 ], and covariates were often tested one at a time, including other covariates only incrementally [ 20 , 25 , 28 , 36 , 40 , 50 , 55 , 67 , 73 ]. Furthermore, there were a few instances where analyses within countries were performed as well [ 32 , 39 , 51 ] or where the full time period of interest was divided into a few sub-periods [ 24 , 26 , 28 , 31 ]. There were also cases where different statistical techniques were applied in parallel [ 29 , 55 , 60 , 66 , 69 , 73 , 82 ], sometimes as a form of sensitivity analysis [ 24 , 26 , 30 , 58 , 73 ]. However, the most common approach to sensitivity analysis was to re-run models with somewhat different samples [ 39 , 50 , 59 , 67 , 69 , 80 , 82 ]. Other strategies included different categorization of variables or adding (more/other) controls [ 21 , 23 , 25 , 28 , 37 , 50 , 63 , 69 ], using an alternative main covariate measure [ 59 , 82 ], including lags for predictors or outcomes [ 28 , 30 , 58 , 63 , 65 , 79 ], using weights [ 24 , 67 ] or alternative data sources [ 37 , 69 ], or using non-imputed data [ 41 ].
As the methods and not the findings are the main focus of the current review, and because generic checklists cannot discern the underlying quality in this application field (see also below), we opted to pool all reported findings together, regardless of individual study characteristics or particular outcome(s) used, and speak generally of positive and negative effects on health. For this summary we have adopted the 0.05-significance level and only considered results from multivariate analyses. Strictly birth-related factors are omitted since these potentially only relate to the group of infant mortality indicators and not to any of the other general population health measures.
Starting with the determinants most often studied, higher GDP levels [ 21 , 26 , 27 , 29 , 30 , 32 , 43 , 48 , 52 , 58 , 60 , 66 , 67 , 73 , 79 , 81 , 82 ], higher health [ 21 , 37 , 47 , 49 , 52 , 58 , 59 , 68 , 72 , 82 ] and social [ 20 , 21 , 26 , 38 , 79 ] expenditures, higher education [ 26 , 39 , 52 , 62 , 72 , 73 ], lower unemployment [ 60 , 61 , 66 ], and lower income inequality [ 30 , 42 , 53 , 55 , 73 ] were found to be significantly associated with better population health on a number of occasions. In addition to that, there was also some evidence that democracy [ 36 ] and freedom [ 50 ], higher work compensation [ 43 , 79 ], distributional orientation [ 54 ], cigarette prices [ 63 ], gross national income [ 22 , 72 ], labor productivity [ 26 ], exchange rates [ 32 ], marginal tax rates [ 79 ], vaccination rates [ 52 ], total fertility [ 59 , 66 ], fruit and vegetable [ 68 ], fat [ 52 ] and sugar consumption [ 52 ], as well as bigger depth of credit information [ 22 ] and percentage of civilian labor force [ 79 ], longer work leaves [ 41 , 58 ], more physicians [ 37 , 52 , 72 ], nurses [ 72 ], and hospital beds [ 79 , 82 ], and also membership in associations, perceived corruption and societal trust [ 48 ] were beneficial to health. Higher nitrous oxide (NO) levels [ 52 ], longer average hospital stay [ 48 ], deprivation [ 51 ], dissatisfaction with healthcare and the social environment [ 56 ], corruption [ 40 , 50 ], smoking [ 19 , 26 , 52 , 68 ], alcohol consumption [ 26 , 52 , 68 ] and illegal drug use [ 68 ], poverty [ 64 ], higher percentage of industrial workers [ 26 ], Gross Fixed Capital creation [ 66 ] and older population [ 38 , 66 , 79 ], gender inequality [ 22 ], and fertility [ 26 , 66 ] were detrimental.
It is important to point out that the above-mentioned effects could not be considered stable either across or within studies. Very often, statistical significance of a given covariate fluctuated between the different model specifications tried out within the same study [ 20 , 49 , 59 , 66 , 68 , 69 , 73 , 80 , 82 ], testifying to the importance of control variables and multivariate research (i.e., analyzing multiple independent variables simultaneously) in general. Furthermore, conflicting results were observed even with regards to the “core” determinants given special attention, so to speak, throughout this text. Thus, some studies reported negative effects of health expenditure [ 32 , 82 ], social expenditure [ 58 ], GDP [ 49 , 66 ], and education [ 82 ], and positive effects of income inequality [ 82 ] and unemployment [ 24 , 31 , 32 , 52 , 66 , 68 ]. Interestingly, one study [ 34 ] differentiated between temporary and long-term effects of GDP and unemployment, alluding to possibly much greater complexity of the association with health. It is also worth noting that some gender differences were found, with determinants being more influential for males than for females, or only having statistically significant effects for male health [ 19 , 21 , 28 , 34 , 36 , 37 , 39 , 64 , 65 , 69 ].
The purpose of this scoping review was to examine recent quantitative work on the topic of multi-country analyses of determinants of population health in high-income countries.
Measuring population health via relatively simple mortality-based indicators still seems to be the state of the art. What is more, these indicators are routinely considered one at a time, instead of, for example, employing existing statistical procedures to devise a more general, composite, index of population health, or using some of the established indices, such as disability-adjusted life expectancy (DALE) or quality-adjusted life expectancy (QALE). Although strong arguments for their wider use were already voiced decades ago [ 84 ], such summary measures surface only rarely in this research field.
On a related note, the greater data availability and accessibility that we enjoy today does not automatically equate to data quality. Nonetheless, this is routinely assumed in aggregate level studies. We almost never encountered a discussion on the topic. The non-mundane issue of data missingness, too, goes largely underappreciated. With all recent methodological advancements in this area [ 85 – 88 ], there is no excuse for ignorance; and still, too few of the reviewed studies tackled the matter in any adequate fashion.
Much optimism can be gained considering the abundance of different determinants that have attracted researchers’ attention in relation to population health. We took on a visual approach with regards to these determinants and presented a graph that links spatial distances between determinants with frequencies of being studies together. To facilitate interpretation, we grouped some variables, which resulted in some loss of finer detail. Nevertheless, the graph is helpful in exemplifying how many effects continue to be studied in a very limited context, if any. Since in reality no factor acts in isolation, this oversimplification practice threatens to render the whole exercise meaningless from the outset. The importance of multivariate analysis cannot be stressed enough. While there is no “best method” to be recommended and appropriate techniques vary according to the specifics of the research question and the characteristics of the data at hand [ 89 – 93 ], in the future, in addition to abandoning simplistic univariate approaches, we hope to see a shift from the currently dominating fixed effects to the more flexible random/mixed effects models [ 94 ], as well as wider application of more sophisticated methods, such as principle component regression, partial least squares, covariance structure models (e.g., structural equations), canonical correlations, time-series, and generalized estimating equations.
Finally, there are some limitations of the current scoping review. We searched the two main databases for published research in medical and non-medical sciences (PubMed and Web of Science) since 2013, thus potentially excluding publications and reports that are not indexed in these databases, as well as older indexed publications. These choices were guided by our interest in the most recent (i.e., the current state-of-the-art) and arguably the highest-quality research (i.e., peer-reviewed articles, primarily in indexed non-predatory journals). Furthermore, despite holding a critical stance with regards to some aspects of how determinants-of-health research is currently conducted, we opted out of formally assessing the quality of the individual studies included. The reason for that is two-fold. On the one hand, we are unaware of the existence of a formal and standard tool for quality assessment of ecological designs. And on the other, we consider trying to score the quality of these diverse studies (in terms of regional setting, specific topic, outcome indices, and methodology) undesirable and misleading, particularly since we would sometimes have been rating the quality of only a (small) part of the original studies—the part that was relevant to our review’s goal.
Our aim was to investigate the current state of research on the very broad and general topic of population health, specifically, the way it has been examined in a multi-country context. We learned that data treatment and analytical approach were, in the majority of these recent studies, ill-equipped or insufficiently transparent to provide clarity regarding the underlying mechanisms of population health in high-income countries. Whether due to methodological shortcomings or the inherent complexity of the topic, research so far fails to provide any definitive answers. It is our sincere belief that with the application of more advanced analytical techniques this continuous quest could come to fruition sooner.
Supporting information
S1 checklist. preferred reporting items for systematic reviews and meta-analyses extension for scoping reviews (prisma-scr) checklist..
https://doi.org/10.1371/journal.pone.0239031.s001
S1 Appendix.
https://doi.org/10.1371/journal.pone.0239031.s002
S2 Appendix.
https://doi.org/10.1371/journal.pone.0239031.s003
- View Article
- Google Scholar
- PubMed/NCBI
- 75. Dahlgren G, Whitehead M. Policies and Strategies to Promote Equity in Health. Stockholm, Sweden: Institute for Future Studies; 1991.
- 76. Brunner E, Marmot M. Social Organization, Stress, and Health. In: Marmot M, Wilkinson RG, editors. Social Determinants of Health. Oxford, England: Oxford University Press; 1999.
- 77. Najman JM. A General Model of the Social Origins of Health and Well-being. In: Eckersley R, Dixon J, Douglas B, editors. The Social Origins of Health and Well-being. Cambridge, England: Cambridge University Press; 2001.
- 85. Carpenter JR, Kenward MG. Multiple Imputation and its Application. New York: John Wiley & Sons; 2013.
- 86. Molenberghs G, Fitzmaurice G, Kenward MG, Verbeke G, Tsiatis AA. Handbook of Missing Data Methodology. Boca Raton: Chapman & Hall/CRC; 2014.
- 87. van Buuren S. Flexible Imputation of Missing Data. 2nd ed. Boca Raton: Chapman & Hall/CRC; 2018.
- 88. Enders CK. Applied Missing Data Analysis. New York: Guilford; 2010.
- 89. Shayle R. Searle GC, Charles E. McCulloch. Variance Components: John Wiley & Sons, Inc.; 1992.
- 90. Agresti A. Foundations of Linear and Generalized Linear Models. Hoboken, New Jersey: John Wiley & Sons Inc.; 2015.
- 91. Leyland A. H. (Editor) HGE. Multilevel Modelling of Health Statistics: John Wiley & Sons Inc; 2001.
- 92. Garrett Fitzmaurice MD, Geert Verbeke, Geert Molenberghs. Longitudinal Data Analysis. New York: Chapman and Hall/CRC; 2008.
- 93. Wolfgang Karl Härdle LS. Applied Multivariate Statistical Analysis. Berlin, Heidelberg: Springer; 2015.
Quantitative Methods
The Quantitative Methods (QM) field of study provides students with the necessary quantitative and analytical skills to approach and solve problems in public health and clinical research and practice. This field is designed for mid-career health professionals, research scientists, and MD/MPH specific dual/joint-degree students.
Through a competency-based curriculum, health professionals in the MPH-45 receive the analytical and statistical knowledge and skills required for successful public health practice and research. In addition to providing broad perspectives on general aspects of public health, the QM field of study provides an excellent foundation for those interested in pursuing academic careers in the health sciences.
Degree programs
The Master of Public Health 45-credit degree provides established professionals with the specialized skills and powerful global network needed to progress their careers in public health.
- Abbreviation: MPH-45 QM
- Degree format: On campus
- Time commitment: Full-time or part-time
- Average program length: One year full-time; two years part-time
Student interests
The Quantitative Methods (QM) field of study is uniquely designed for mid-career health professionals, research scientists, and MD/MPH students. Students who choose QM are passionate about clinical and population-based health research, and dedicated to learning the tools necessary for implementation.
Career outcomes
Graduates of the Master of Public Health (MPH) 45-credit program with the Quantitative Methods (QM) field of study are prepared to fulfill professional positions in clinical and population-based health research in government, health care institutions, and private industry.

An official website of the United States government
The .gov means it’s official. Federal government websites often end in .gov or .mil. Before sharing sensitive information, make sure you’re on a federal government site.
The site is secure. The https:// ensures that you are connecting to the official website and that any information you provide is encrypted and transmitted securely.
- Publications
- Account settings
Preview improvements coming to the PMC website in October 2024. Learn More or Try it out now .
- Advanced Search
- Journal List
- Acta Inform Med
- v.30(1); 2022 Mar
Mixed Methodology of Scientific Research in Healthcare
Emina smajic.
1 Agram Polyclinic, Sarajevo, Bosnia and Herzegovina
Dijana Avdic
2 Faculty of Health Studies, Sarajevo, Bosnia and Herzegovina
Aleksandra Pasic
3 Clinical Biochemistry with Immunology, Sarajevo, Clinical Center University of Sarajevo, Bosnia and Herzegovina
Alden Prcic
4 General Hospital “Prim dr. Abdulah Nakas”, Sarajevo, Bosnia and Herzegovina
Maja Stancic
Background:.
Scientific research is usually classified as quantitative or qualitative. However, methodologists are increasingly emphasizing the integration of qualitative and quantitative data as the center of mixed methods (mix methodologies). Mixed research method implies the use of different research methods, ie. quantitative and qualitative methods in one study.
The aim of this review paper is to present the purpose of using a mixed methodology in health research.
The relevant articles were searched from online data sources including PubMed and Google Scholar.
This approach to the use of mixed methods creates opportunities for a deeper study of various problems. The purpose of using mixed research methods is to obtain valid answers to research questions, however the researcher may still have different reasons or purposes for which he wants to strengthen the research study and its conclusions by applying mixed methods. The use of mixed scientific methodology is widely used in the field of health outcomes and should not be limited to a closed list of possible methodological options.
Conclusion:
Recently, there has been an increase in the number of scientific studies in healthcare that use mixed research methods. The advantage of applying this scientific method is that through the triangulation of data obtained by different (quantitative / qualitative) approaches, we get a deeper and more complete picture of the phenomenon in health care that we observe.
1. BACKGROUND
In health science research, there is a priority to develop new methodologies to improve the quality and scientific strength of data leading to an extraordinary increase in methodological diversity. This diversity reflects the nature of public health problems, such as differences between populations, age groups, ethnic groups and cultures, poor adherence to treatments considered effective, behavioral factors contributing to disability and health, and translational needs for health research. Diversity also signals a growing acceptance of qualitative and social science research, the formation of interdisciplinary research teams, and the use of multilevel approaches to research complex health issues such as patient attitudes and cultural and social models of disease and health (1) .
2. OBJECTIVE
The retrieved articles were reviewed by the authors and the results are presented along with the relevant discussion
4.1. MIXED METHODOLOGY
Scientific research is usually classified as quantitative or qualitative. However, methodologists are increasingly emphasizing the integration of qualitative and quantitative data as the center of mixed methods (mix methodologies). Integration is a deliberate process by which the researcher combines quantitative and qualitative approaches in the study. Quantitative and qualitative data then become interdependent in solving questions and hypotheses. Mixed research method implies the use of different research methods, quantitative and qualitative methods in one study ( 2 , 3 ). Research on mixed methods should be distinguished from multi-method research (method-combination) in which either multiple qualitative approaches or only multiple quantitative approaches are combined (4) .

The most accepted definition of a mixed research method is that it is a research in which a researcher or team of researchers combines elements of a qualitative and quantitative approach to research (use of qualitative and quantitative perspectives, data collection, analysis, inference techniques) to understand and support research. As we see in the definition, the use of both quantitative and qualitative methods in a single study (or series of related studies) is crucial, unlike the use of combined methods that combine two or more quantitative or two or more qualitative research methods ( 2 , 4 ).
The basic premise of using mixed research methods is that some research issues can be addressed more comprehensively than using either quantitative or qualitative methods. The issues that benefit most from the design of mixed methods tend to be broad and complex, with multiple aspects that each can have. Mixed research methods can exploit the strengths and weaknesses of both approaches and can be particularly useful when addressing complex, multifaceted issues such as health service interventions and living with chronic diseases (2) . This approach to the use of mixed methods creates opportunities for a deeper study of various problems (5) .
4.2. PURPOSE OF USING MIXED RESEARCH
The purpose of using mixed research methods is to obtain valid answers to research questions, however the researcher may still have different reasons or purposes for which he wants to strengthen the research study and its conclusions by applying mixed methods. The purpose classification of mixed research methods was first introduced in 1989 by Greene, Caracelli, and Graham, based on an analysis of published studies of mixed methods. This classification is still used and we have a total of five “purposes” for why a mixed methodology is used in research (4) . Classification of the purpose of using mixed methodology:
- Complementarity. Using data obtained from one method to illustrate the results of another method.
- Development. Using the results of one method to develop or inform about the use of another method.
- Initiation. We can use the results of different methods to search for areas of non-compliance in certain areas in order to create new insights.
- Expansion. Examining different aspects of a research question, with each aspect justifying different methods.
- Triangulation. Use of data obtained by both methods to support the findings (2) .
In the last 28 years, this classification has been supplemented by several other authors. So in 2006 Bryman compiled a list of more specific rationales for the use or purpose of mixed research methods. Bryman’s classification decomposes the categorization of Greene et al. on several aspects and adds a number of additional aspects (3) .

Bryman’s addition to the classification of Greene et al.
Credibility. It refers to suggestions that the application of both methodological approaches improves the integrity of the results.
Context. Refers to cases where the combination is justified in terms of qualitative research that provides contextual understanding, along with generalized, externally valid results, or broad relationships between the variables identified by the survey.
Illustration. It refers to the use of qualitative data to illustrate quantitative findings, often referred to as putting “meat on the bone” “dry” quantitative findings.
Usefulness or improvement of usefulness of results. It refers to the suggestion, which is more likely to be highlighted among articles with an applied focus, that combining the two approaches will be more useful for practitioners.
Confirmation and discovery. It involves the use of qualitative data to generate hypotheses and the use of quantitative research to test them within a single project.
Diversity of views. This includes two slightly different explanations–namely, combining the perspective of researchers and participants through quantitative and qualitative research, and discovering the relationship between variables through quantitative research, while revealing meanings among research participants through qualitative research (3) .
4.3. RESEARCH DESIGN
Research designs are procedures for collecting, analyzing, interpreting, and reporting data in research studies. They represent different models for doing research, and these models have distinct names and procedures associated with them (6) .
The four major types of mixed methods designs are the Triangulation Design, the Embedded Design, the Explanatory Design, and the Exploratory Design (6) .
4.4. HEALTH APPLICATION
There is a wide range of methods used to collect both quantitative and qualitative data. And the research question and the necessary data are the main determinants of the methods used. To a lesser extent, the choice of methods may be influenced by feasibility. Method priority refers to the emphasis on each method in the study. For example, a study may be predominantly quantitative with a small qualitative component or vice versa. Alternatively, both quantitative and qualitative methods and data may be equally weighted. The emphasis on each methodological component of the study will be driven mainly by the research question, research team skills and feasibility. Finally, researchers must decide when each method will be used in research (2) . By analyzing the research methods and research designs used, Bryman suggests that on the quantitative side, structured interview and questionnaire research within cross-sectional design predominates, while on the qualitative side, semi-structured interviews within cross-sectional design predominate (7) . A key feature of mixed-method research is its methodological pluralism, which often results in research that provides broader perspectives than those offered by monomethodal designs (8) .
The use of mixed scientific methodology is widely used in the field of health outcomes and should not be limited to a closed list of possible methodological options, but should be seen as a framework for a specific research issue to be addressed using quantitative and qualitative components (data and / or methods) , when quantitative and qualitative components are articulated intentionally and prospectively in a well-defined, pre-specified research design and as a framework for meta-inference (9) .
The importance of applying mixed research methods can be reflected in the trend of “measuring / analysing what is important” for patients and the treatment outcomes reported by patients are increasingly used in clinical care and research. However, a recent review of studies documenting the development of outcome measures reported by patients highlights that only 11% of them were developed actually asking patients which outcomes are important to them. This emphasizes the importance of applying mixed qualitative and quantitative methods in health research to ensure a focus on the priorities identified by the patient, scientific rigor, and improved patient outcomes (9) . Mixed methods are also an increasingly accepted approach used to investigate organizational phenomena in health care (10) .
The application of the mix methodology is considered a significant contribution to health science. By combining quantitative and qualitative data in the same study, health researchers can reap the benefits of each approach while minimizing their disadvantages. In practice, this endeavor facilitates research by health science researchers on the complex and multifactorial nature of human health and disease. Researchers using the mixed method approach for the first time can easily feel overwhelmed by uncertainty about the philosophical foundations of the method, as well as the multitude of typologies of mixed method research. Although further research and debate are warranted, health researchers seeking solutions to real problems are increasingly embracing pragmatism as a paradigm of choice (11) .
Author’s contribution:
All authors were involved in preparation of this article. Final proofreading was made by first author.
Financial support and sponsorship:
Conflicts of interest:.
There are no conflicts of interest.

Committee on Quantitative Methods in Social, Behavioral, and Health Sciences
View the committee annual open event, view the qmsa information session, overview of quantitative courses for 2023-2024, watch our video.
This is an interdisciplinary community of faculty and students interested in methodological research in relation to applications in social, behavioral, and health sciences.

We bring together:
- a collection of programs in quantitative research methodology
- Faculty members seek to develop and apply advanced methods for research in social and behavioral sciences
- promote innovative research ideas and tools with broad applications,
- conduct high-quality workshop series
- offer a wide range of courses on research methods
- provide excellent opportunities for research and teaching apprenticeships for talented students
Our goals are to create an intellectual niche, exchange research ideas, facilitate research collaborations, share teaching resources, enhance the training of students, and generate a collective impact on the University of Chicago campus and beyond.
play_circle_outline Watch our video to learn more.
The Committee is an academic unit that oversees three training programs and organizes a biweekly workshop that serves as an important forum for scholarly exchanges.
The Certificate Program in Advanced Quantitative Methods is designed for doctoral students in the social, behavioral, and health sciences who wish to deepen their methodological training.
The Quantitative Methods and Social Analysis (QMSA) concentration in the MA Program in the Social Sciences (MAPSS) prepares a new generation of scholars to innovate methodologically and to use the theory of statistical inference to tackle challenging problems in a wide range of research.
The Quantitative Social Analysis minor offers undergraduate students at the University of Chicago the opportunity to develop strong statistical foundations for the purpose of learning how to draw valid inferences from quantifiable data and critically evaluate empirical evidence in the social and behavioral sciences.
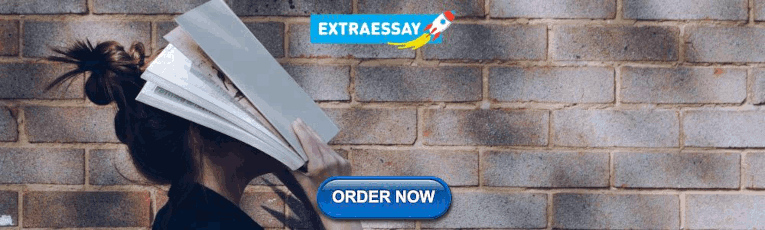
Latest News:
Professor luc anselin awarded 2022 waldo tobler giscience prize of the austrian academy of sciences, dr. robert gibbons publications receive high ranking in jama & nature scientific reports, don hedeker receives the 2022 sells award for distinguished multivariate research, a new analysis of politicized departure hypothesis among federal judges uses ‘natural experiments’ to control for the unknown, monika nalepa releases new book by cambridge university press.
Click here for news archive >>>
- Privacy Policy
Buy Me a Coffee

Home » Quantitative Research – Methods, Types and Analysis
Quantitative Research – Methods, Types and Analysis
Table of Contents

Quantitative Research
Quantitative research is a type of research that collects and analyzes numerical data to test hypotheses and answer research questions . This research typically involves a large sample size and uses statistical analysis to make inferences about a population based on the data collected. It often involves the use of surveys, experiments, or other structured data collection methods to gather quantitative data.
Quantitative Research Methods

Quantitative Research Methods are as follows:
Descriptive Research Design
Descriptive research design is used to describe the characteristics of a population or phenomenon being studied. This research method is used to answer the questions of what, where, when, and how. Descriptive research designs use a variety of methods such as observation, case studies, and surveys to collect data. The data is then analyzed using statistical tools to identify patterns and relationships.
Correlational Research Design
Correlational research design is used to investigate the relationship between two or more variables. Researchers use correlational research to determine whether a relationship exists between variables and to what extent they are related. This research method involves collecting data from a sample and analyzing it using statistical tools such as correlation coefficients.
Quasi-experimental Research Design
Quasi-experimental research design is used to investigate cause-and-effect relationships between variables. This research method is similar to experimental research design, but it lacks full control over the independent variable. Researchers use quasi-experimental research designs when it is not feasible or ethical to manipulate the independent variable.
Experimental Research Design
Experimental research design is used to investigate cause-and-effect relationships between variables. This research method involves manipulating the independent variable and observing the effects on the dependent variable. Researchers use experimental research designs to test hypotheses and establish cause-and-effect relationships.
Survey Research
Survey research involves collecting data from a sample of individuals using a standardized questionnaire. This research method is used to gather information on attitudes, beliefs, and behaviors of individuals. Researchers use survey research to collect data quickly and efficiently from a large sample size. Survey research can be conducted through various methods such as online, phone, mail, or in-person interviews.
Quantitative Research Analysis Methods
Here are some commonly used quantitative research analysis methods:
Statistical Analysis
Statistical analysis is the most common quantitative research analysis method. It involves using statistical tools and techniques to analyze the numerical data collected during the research process. Statistical analysis can be used to identify patterns, trends, and relationships between variables, and to test hypotheses and theories.
Regression Analysis
Regression analysis is a statistical technique used to analyze the relationship between one dependent variable and one or more independent variables. Researchers use regression analysis to identify and quantify the impact of independent variables on the dependent variable.
Factor Analysis
Factor analysis is a statistical technique used to identify underlying factors that explain the correlations among a set of variables. Researchers use factor analysis to reduce a large number of variables to a smaller set of factors that capture the most important information.
Structural Equation Modeling
Structural equation modeling is a statistical technique used to test complex relationships between variables. It involves specifying a model that includes both observed and unobserved variables, and then using statistical methods to test the fit of the model to the data.
Time Series Analysis
Time series analysis is a statistical technique used to analyze data that is collected over time. It involves identifying patterns and trends in the data, as well as any seasonal or cyclical variations.
Multilevel Modeling
Multilevel modeling is a statistical technique used to analyze data that is nested within multiple levels. For example, researchers might use multilevel modeling to analyze data that is collected from individuals who are nested within groups, such as students nested within schools.
Applications of Quantitative Research
Quantitative research has many applications across a wide range of fields. Here are some common examples:
- Market Research : Quantitative research is used extensively in market research to understand consumer behavior, preferences, and trends. Researchers use surveys, experiments, and other quantitative methods to collect data that can inform marketing strategies, product development, and pricing decisions.
- Health Research: Quantitative research is used in health research to study the effectiveness of medical treatments, identify risk factors for diseases, and track health outcomes over time. Researchers use statistical methods to analyze data from clinical trials, surveys, and other sources to inform medical practice and policy.
- Social Science Research: Quantitative research is used in social science research to study human behavior, attitudes, and social structures. Researchers use surveys, experiments, and other quantitative methods to collect data that can inform social policies, educational programs, and community interventions.
- Education Research: Quantitative research is used in education research to study the effectiveness of teaching methods, assess student learning outcomes, and identify factors that influence student success. Researchers use experimental and quasi-experimental designs, as well as surveys and other quantitative methods, to collect and analyze data.
- Environmental Research: Quantitative research is used in environmental research to study the impact of human activities on the environment, assess the effectiveness of conservation strategies, and identify ways to reduce environmental risks. Researchers use statistical methods to analyze data from field studies, experiments, and other sources.
Characteristics of Quantitative Research
Here are some key characteristics of quantitative research:
- Numerical data : Quantitative research involves collecting numerical data through standardized methods such as surveys, experiments, and observational studies. This data is analyzed using statistical methods to identify patterns and relationships.
- Large sample size: Quantitative research often involves collecting data from a large sample of individuals or groups in order to increase the reliability and generalizability of the findings.
- Objective approach: Quantitative research aims to be objective and impartial in its approach, focusing on the collection and analysis of data rather than personal beliefs, opinions, or experiences.
- Control over variables: Quantitative research often involves manipulating variables to test hypotheses and establish cause-and-effect relationships. Researchers aim to control for extraneous variables that may impact the results.
- Replicable : Quantitative research aims to be replicable, meaning that other researchers should be able to conduct similar studies and obtain similar results using the same methods.
- Statistical analysis: Quantitative research involves using statistical tools and techniques to analyze the numerical data collected during the research process. Statistical analysis allows researchers to identify patterns, trends, and relationships between variables, and to test hypotheses and theories.
- Generalizability: Quantitative research aims to produce findings that can be generalized to larger populations beyond the specific sample studied. This is achieved through the use of random sampling methods and statistical inference.
Examples of Quantitative Research
Here are some examples of quantitative research in different fields:
- Market Research: A company conducts a survey of 1000 consumers to determine their brand awareness and preferences. The data is analyzed using statistical methods to identify trends and patterns that can inform marketing strategies.
- Health Research : A researcher conducts a randomized controlled trial to test the effectiveness of a new drug for treating a particular medical condition. The study involves collecting data from a large sample of patients and analyzing the results using statistical methods.
- Social Science Research : A sociologist conducts a survey of 500 people to study attitudes toward immigration in a particular country. The data is analyzed using statistical methods to identify factors that influence these attitudes.
- Education Research: A researcher conducts an experiment to compare the effectiveness of two different teaching methods for improving student learning outcomes. The study involves randomly assigning students to different groups and collecting data on their performance on standardized tests.
- Environmental Research : A team of researchers conduct a study to investigate the impact of climate change on the distribution and abundance of a particular species of plant or animal. The study involves collecting data on environmental factors and population sizes over time and analyzing the results using statistical methods.
- Psychology : A researcher conducts a survey of 500 college students to investigate the relationship between social media use and mental health. The data is analyzed using statistical methods to identify correlations and potential causal relationships.
- Political Science: A team of researchers conducts a study to investigate voter behavior during an election. They use survey methods to collect data on voting patterns, demographics, and political attitudes, and analyze the results using statistical methods.
How to Conduct Quantitative Research
Here is a general overview of how to conduct quantitative research:
- Develop a research question: The first step in conducting quantitative research is to develop a clear and specific research question. This question should be based on a gap in existing knowledge, and should be answerable using quantitative methods.
- Develop a research design: Once you have a research question, you will need to develop a research design. This involves deciding on the appropriate methods to collect data, such as surveys, experiments, or observational studies. You will also need to determine the appropriate sample size, data collection instruments, and data analysis techniques.
- Collect data: The next step is to collect data. This may involve administering surveys or questionnaires, conducting experiments, or gathering data from existing sources. It is important to use standardized methods to ensure that the data is reliable and valid.
- Analyze data : Once the data has been collected, it is time to analyze it. This involves using statistical methods to identify patterns, trends, and relationships between variables. Common statistical techniques include correlation analysis, regression analysis, and hypothesis testing.
- Interpret results: After analyzing the data, you will need to interpret the results. This involves identifying the key findings, determining their significance, and drawing conclusions based on the data.
- Communicate findings: Finally, you will need to communicate your findings. This may involve writing a research report, presenting at a conference, or publishing in a peer-reviewed journal. It is important to clearly communicate the research question, methods, results, and conclusions to ensure that others can understand and replicate your research.
When to use Quantitative Research
Here are some situations when quantitative research can be appropriate:
- To test a hypothesis: Quantitative research is often used to test a hypothesis or a theory. It involves collecting numerical data and using statistical analysis to determine if the data supports or refutes the hypothesis.
- To generalize findings: If you want to generalize the findings of your study to a larger population, quantitative research can be useful. This is because it allows you to collect numerical data from a representative sample of the population and use statistical analysis to make inferences about the population as a whole.
- To measure relationships between variables: If you want to measure the relationship between two or more variables, such as the relationship between age and income, or between education level and job satisfaction, quantitative research can be useful. It allows you to collect numerical data on both variables and use statistical analysis to determine the strength and direction of the relationship.
- To identify patterns or trends: Quantitative research can be useful for identifying patterns or trends in data. For example, you can use quantitative research to identify trends in consumer behavior or to identify patterns in stock market data.
- To quantify attitudes or opinions : If you want to measure attitudes or opinions on a particular topic, quantitative research can be useful. It allows you to collect numerical data using surveys or questionnaires and analyze the data using statistical methods to determine the prevalence of certain attitudes or opinions.
Purpose of Quantitative Research
The purpose of quantitative research is to systematically investigate and measure the relationships between variables or phenomena using numerical data and statistical analysis. The main objectives of quantitative research include:
- Description : To provide a detailed and accurate description of a particular phenomenon or population.
- Explanation : To explain the reasons for the occurrence of a particular phenomenon, such as identifying the factors that influence a behavior or attitude.
- Prediction : To predict future trends or behaviors based on past patterns and relationships between variables.
- Control : To identify the best strategies for controlling or influencing a particular outcome or behavior.
Quantitative research is used in many different fields, including social sciences, business, engineering, and health sciences. It can be used to investigate a wide range of phenomena, from human behavior and attitudes to physical and biological processes. The purpose of quantitative research is to provide reliable and valid data that can be used to inform decision-making and improve understanding of the world around us.
Advantages of Quantitative Research
There are several advantages of quantitative research, including:
- Objectivity : Quantitative research is based on objective data and statistical analysis, which reduces the potential for bias or subjectivity in the research process.
- Reproducibility : Because quantitative research involves standardized methods and measurements, it is more likely to be reproducible and reliable.
- Generalizability : Quantitative research allows for generalizations to be made about a population based on a representative sample, which can inform decision-making and policy development.
- Precision : Quantitative research allows for precise measurement and analysis of data, which can provide a more accurate understanding of phenomena and relationships between variables.
- Efficiency : Quantitative research can be conducted relatively quickly and efficiently, especially when compared to qualitative research, which may involve lengthy data collection and analysis.
- Large sample sizes : Quantitative research can accommodate large sample sizes, which can increase the representativeness and generalizability of the results.
Limitations of Quantitative Research
There are several limitations of quantitative research, including:
- Limited understanding of context: Quantitative research typically focuses on numerical data and statistical analysis, which may not provide a comprehensive understanding of the context or underlying factors that influence a phenomenon.
- Simplification of complex phenomena: Quantitative research often involves simplifying complex phenomena into measurable variables, which may not capture the full complexity of the phenomenon being studied.
- Potential for researcher bias: Although quantitative research aims to be objective, there is still the potential for researcher bias in areas such as sampling, data collection, and data analysis.
- Limited ability to explore new ideas: Quantitative research is often based on pre-determined research questions and hypotheses, which may limit the ability to explore new ideas or unexpected findings.
- Limited ability to capture subjective experiences : Quantitative research is typically focused on objective data and may not capture the subjective experiences of individuals or groups being studied.
- Ethical concerns : Quantitative research may raise ethical concerns, such as invasion of privacy or the potential for harm to participants.
About the author
Muhammad Hassan
Researcher, Academic Writer, Web developer
You may also like

Questionnaire – Definition, Types, and Examples

Case Study – Methods, Examples and Guide

Observational Research – Methods and Guide

Qualitative Research Methods

Explanatory Research – Types, Methods, Guide

Survey Research – Types, Methods, Examples
Reporting and Description of Research Methodology in Studies Estimating Effects of Firearm Policies
Affiliations.
- 1 Department of Epidemiology, University of Washington School of Public Health, Seattle, WA, USA.
- 2 Firearm Injury and Policy Research Program, University of Washington, Seattle, WA, USA.
- 3 Department of Health Sciences, Northeastern University Bouvé College of Health Sciences, Boston, MA, USA.
- 4 Department of Epidemiology, University of Pittsburgh School of Public Health, Pittsburgh, PA, USA.
- PMID: 38597728
- DOI: 10.1097/EDE.0000000000001741
Background: Evidence about which firearm policies work, to what extent, and for whom is hotly debated, perhaps partly because variation in research methodology has produced mixed and inconclusive effect estimates. We conducted a scoping review of firearm policy research in the health sciences in the United States, focusing on methodological considerations for causal inference.
Methods: We identified original, empirical articles indexed in PubMed from 1/1/2000-9/1/2021 that examined any of 18 pre-specified firearm policies. We extracted key study components, including policy type(s) examined, policy operationalization, outcomes, study setting and population, study approach and design, causal language, and whether and how authors acknowledged potential sources of bias.
Results: We screened 7733 articles and included 124. A plurality of studies used a legislative score as their primary exposure (n=39; 32%) and did not examine change in policies over time (n=47; 38%). Most examined firearm homicide (n=51; 41%) or firearm suicide (n=40; 32%) as outcomes. One-third adjusted for other firearm policies (n=41; 33%). Three studies (2%) explicitly mentioned that their goal was to estimate causal effects, but over half used language implying causality (n=72; 58%). Most acknowledged causal identification assumptions of temporality (n=91; 73%) and exchangeability (n=111; 90%); other assumptions were less often acknowledged. One-third of studies included bias analyses (n=42; 34%).
Conclusions: We identified a range of methodologic approaches in firearm policy research in the health sciences. Acknowledging limitations of data availability and quality, we identify opportunities to improve causal inferences about and reporting on the effects of firearm policies on population health.
Copyright © 2024 Wolters Kluwer Health, Inc. All rights reserved.
- Skip to main content
- Skip to FDA Search
- Skip to in this section menu
- Skip to footer links

The .gov means it’s official. Federal government websites often end in .gov or .mil. Before sharing sensitive information, make sure you're on a federal government site.
The site is secure. The https:// ensures that you are connecting to the official website and that any information you provide is encrypted and transmitted securely.
U.S. Food and Drug Administration
- Search
- Menu
- Science & Research
- About Science & Research at FDA
- The FDA Science Forum
Quantitative Immuno-PCR Detection of Plasma IP-10 Levels Over Time: Candidate Pharmacodynamic Biomarker of IFNβ1-a Biologics
2023 FDA Science Forum
Background:
Clinical pharmacodynamic similarity data can contribute to the totality of evidence supporting approval of biosimilars and can guide the need for subsequent clinical testing. Proteomic analysis of plasma samples from a clinical trial FDA conducted identified interferon-gamma-inducible protein-10 (IP-10) as a candidate pharmacodynamic (PD) biomarker of IFNβ-1 biologics (interferon beta-1a [IFNβ-1a] and pegylated IFNβ-1a [pegIFNβ-1a]).
To evaluate a quantitative immuno-PCR assay for the technical replication of plasma IP-10 levels. Study Design: We used plasma samples from 36 healthy subjects from the placebo-controlled randomized clinical trial carried out with IFNβ-1a and pegIFNβ-1a.
A commercial assay (ProQuantum, ThermoFisher Scientific) was used to measure IP-10 at 10 timepoints over 6 days in the IFNβ-1a group (n=12 [30µg]), at 12 timepoints over 13 days in the pegIFNβ-1a group (n=12 [125µg]) and placebo-specific groups (n=6 each). Concentrations were extrapolated from a standard curve analysis. For differential expression, we conducted ANOVA on linear-mixed effect models. F-test and area under effect curve (AUEC). Results were compared to previous proteomics findings (SOMAscan Assay v4.1, SomaLogic) using Pearson’s Correlation. Analyses were conducted in R (version 4.1.2).
Median concentration of IP-10 in baseline plasma samples was 20.8 pg/ml. Assay sensitivity was 0.064 pg/ml, with 4 log-fold dynamic range and good reproducibility (CV<25%). F-test p-values of 2.9E-28 and 4.9E-30 for IFNβ1-a and pegIFNβ-1a products, respectively, were observed. At 0.33 days, IP-10 showed maximum response with a 38-fold and 28-fold increase from baseline for IFNβ1-a and pegIFNβ-1a, respectively. A strong difference compared to placebo was observed for AUECs for IFNβ-1a (t-test p=1.04E-04) and pegIFNβ-1a (p=8.6E-04). The pattern of IP-10 response for both products over time was concordant with previous proteomic analysis of the same plasma samples (rho=0.87, p=3.9E-122).
Conclusion:
Results suggest that the IP-10 assay is a sensitive and reproducible method and further technically replicates previous findings supporting IP-10 as a potential pharmacodynamic biomarker for IFNβ1-a products in healthy subjects. This assay has potential utility in future longitudinal studies for the detection of IP-10 in plasma as a candidate PD biomarker for assessing biosimilarity.

Download the Poster (PDF; 1.25 MB)
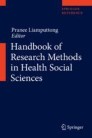
Handbook of Research Methods in Health Social Sciences pp 27–49 Cite as
Quantitative Research
- Leigh A. Wilson 2 , 3
- Reference work entry
- First Online: 13 January 2019
4187 Accesses
4 Citations
Quantitative research methods are concerned with the planning, design, and implementation of strategies to collect and analyze data. Descartes, the seventeenth-century philosopher, suggested that how the results are achieved is often more important than the results themselves, as the journey taken along the research path is a journey of discovery. High-quality quantitative research is characterized by the attention given to the methods and the reliability of the tools used to collect the data. The ability to critique research in a systematic way is an essential component of a health professional’s role in order to deliver high quality, evidence-based healthcare. This chapter is intended to provide a simple overview of the way new researchers and health practitioners can understand and employ quantitative methods. The chapter offers practical, realistic guidance in a learner-friendly way and uses a logical sequence to understand the process of hypothesis development, study design, data collection and handling, and finally data analysis and interpretation.
This is a preview of subscription content, log in via an institution .
Buying options
- Available as PDF
- Read on any device
- Instant download
- Own it forever
- Available as EPUB and PDF
- Durable hardcover edition
- Dispatched in 3 to 5 business days
- Free shipping worldwide - see info
Tax calculation will be finalised at checkout
Purchases are for personal use only
Babbie ER. The practice of social research. 14th ed. Belmont: Wadsworth Cengage; 2016.
Google Scholar
Descartes. Cited in Halverston, W. (1976). In: A concise introduction to philosophy, 3rd ed. New York: Random House; 1637.
Doll R, Hill AB. The mortality of doctors in relation to their smoking habits. BMJ. 1954;328(7455):1529–33. https://doi.org/10.1136/bmj.328.7455.1529 .
Article Google Scholar
Liamputtong P. Research methods in health: foundations for evidence-based practice. 3rd ed. Melbourne: Oxford University Press; 2017.
McNabb DE. Research methods in public administration and nonprofit management: quantitative and qualitative approaches. 2nd ed. New York: Armonk; 2007.
Merriam-Webster. Dictionary. http://www.merriam-webster.com . Accessed 20th December 2017.
Olesen Larsen P, von Ins M. The rate of growth in scientific publication and the decline in coverage provided by Science Citation Index. Scientometrics. 2010;84(3):575–603.
Pannucci CJ, Wilkins EG. Identifying and avoiding bias in research. Plast Reconstr Surg. 2010;126(2):619–25. https://doi.org/10.1097/PRS.0b013e3181de24bc .
Petrie A, Sabin C. Medical statistics at a glance. 2nd ed. London: Blackwell Publishing; 2005.
Portney LG, Watkins MP. Foundations of clinical research: applications to practice. 3rd ed. New Jersey: Pearson Publishing; 2009.
Sheehan J. Aspects of research methodology. Nurse Educ Today. 1986;6:193–203.
Wilson LA, Black DA. Health, science research and research methods. Sydney: McGraw Hill; 2013.
Download references
Author information
Authors and affiliations.
School of Science and Health, Western Sydney University, Penrith, NSW, Australia
Leigh A. Wilson
Faculty of Health Science, Discipline of Behavioural and Social Sciences in Health, University of Sydney, Lidcombe, NSW, Australia
You can also search for this author in PubMed Google Scholar
Corresponding author
Correspondence to Leigh A. Wilson .
Editor information
Editors and affiliations.
Pranee Liamputtong
Rights and permissions
Reprints and permissions
Copyright information
© 2019 Springer Nature Singapore Pte Ltd.
About this entry
Cite this entry.
Wilson, L.A. (2019). Quantitative Research. In: Liamputtong, P. (eds) Handbook of Research Methods in Health Social Sciences. Springer, Singapore. https://doi.org/10.1007/978-981-10-5251-4_54
Download citation
DOI : https://doi.org/10.1007/978-981-10-5251-4_54
Published : 13 January 2019
Publisher Name : Springer, Singapore
Print ISBN : 978-981-10-5250-7
Online ISBN : 978-981-10-5251-4
eBook Packages : Social Sciences Reference Module Humanities and Social Sciences Reference Module Business, Economics and Social Sciences
Share this entry
Anyone you share the following link with will be able to read this content:
Sorry, a shareable link is not currently available for this article.
Provided by the Springer Nature SharedIt content-sharing initiative
- Publish with us
Policies and ethics
- Find a journal
- Track your research
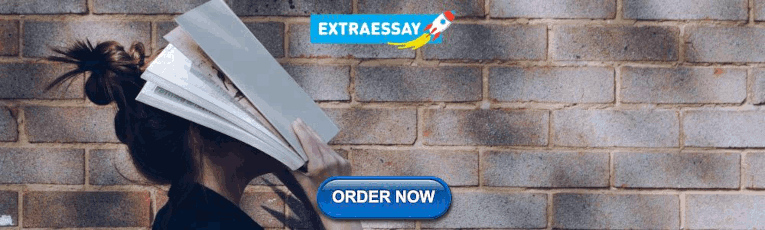
IMAGES
VIDEO
COMMENTS
theoretical position or the health sciences professional responsible for developing the steps based on a specific methodology. For this reason, health professionals must know the principles that define the positioning within one methodology or another and use its instruments and techniques to develop their research.
Chapter 1: Research and scientific methods Empirical research in the health sciences can be qualitative or quantitative in nature. Generally, health science research deals with information of a quantitative nature, and this manual deals exclusively with this type of research. For the most part, this involves the
This book explains in a practical and dynamic way the relevant aspects of quantitative research methodology in health sciences. It explores research paradigms, the foundations of evidence-based clinical practice, the formulation, through different models, of the research question and objectives, definition of the scope of study, population and sample, and the classification of research variables.
They help to highlight issues in the conduct of research with the aim of improving health research methodology, and ultimately reducing research waste. ... this paper focuses on and draws examples exclusively from quantitative research. ... A survey of reports indexed in the Science Citation Index Expanded. Eur J Integrative Med. 2011; 3 (4 ...
INTRODUCTION. Scientific research is usually initiated by posing evidenced-based research questions which are then explicitly restated as hypotheses.1,2 The hypotheses provide directions to guide the study, solutions, explanations, and expected results.3,4 Both research questions and hypotheses are essentially formulated based on conventional theories and real-world processes, which allow the ...
Abstract. Quantitative research is the foundation for evidence-based global health practice and interventions. Preparing health research starts with a clear research question to initiate the study, careful planning using sound methodology as well as the development and management of the capacity and resources to complete the whole research cycle.
A practical introduction to epidemiology, biostatistics, and research methodology for the whole health care community This comprehensive text, which has been extensively revised with new material and additional topics, utilizes a practical slant to introduce health professionals and students to epidemiology, biostatistics, and research methodology. It draws examples from a wide range of topics ...
Methods. The first part of this paper reviews the methods used to synthesise quantitative effectiveness evidence in public health guidelines by the National Institute for Health and Care Excellence (NICE) that had been published or updated since the previous review in 2012 until the 19th August 2019.The second part of this paper provides an update of the statistical methods and explains how ...
Quantitative research methods are concerned with the planning, design, and implementation of strategies to collect and analyze data. Descartes, the seventeenth-century philosopher, suggested that how the results are achieved is often more important than the results themselves, as the journey taken along the research path is a journey of discovery. . High-quality quantitative research is ...
Welcome to Quantitative Methods for Health Research, a study programme designed to introduce you to the knowledge and skills required to make sense of published health research, and to begin ... (Statistical Package for the Social Sciences) has been prepared; this relates closely to the structure and content of the book. Full details of this ...
Simply, quantitative research methods are systematic ways of gathering and analyzing numerical data with the general purpose of understanding reality. Quantitative methods are mostly used to generalize results to a wider population, make predictions, infer causality, and prove hypotheses. ... School of Health and Health Sciences, University of ...
Advances in Health Sciences Education - Q methodology is a unique, yet underutilized methodology designed specifically to scientifically study subjectivity. ... especially those borrowed from purely quantitative research. Thus, we stress the importance of reading multiple methodological publications on Q methodology with a focus on the masters ...
This publication is designed to help provide practicing health educators with basic tools helpful to facilitate a better understanding of quantitative research. This article describes the major components—title, introduction, methods, analyses, results and discussion sections—of quantitative research. Readers will be introduced to ...
The Department of Quantitative Health Sciences at Mayo Clinic is a multidisciplinary group with hundreds of staff members, located in Phoenix/Scottsdale, Arizona; Jacksonville, Florida; and Rochester, Minnesota, who are dedicated to improving patient care through medical research. The department's work encompasses: Faculty-led research programs.
Health Science Research is an indispensable guide for anyone who needs to undertake a clinical study, including physicians, nurses, allied health workers, scientists and research assistants. Jennifer Peat is Hospital Statistician in the Clinical Epidemiology Unit at the New Children's Hospital, Westmead, and Associate Professor in the ...
Background Identifying determinants of health and understanding their role in health production constitutes an important research theme. We aimed to document the state of recent multi-country research on this theme in the literature. Methods We followed the PRISMA-ScR guidelines to systematically identify, triage and review literature (January 2013—July 2019).
Quantitative research is the opposite of qualitative research, which involves collecting and analyzing non-numerical data (e.g., text, video, or audio). Quantitative research is widely used in the natural and social sciences: biology, chemistry, psychology, economics, sociology, marketing, etc. Quantitative research question examples
JOURNAL HOMEPAGE. Research Methods in Medicine & Health Sciences is a peer reviewed journal, publishing rigorous research on established "gold standard" methods and new cutting edge research methods in the health sciences and clinical medicine. View full journal description. This journal is a member of the Committee on Publication Ethics ...
Summary. The Quantitative Methods (QM) field of study provides students with the necessary quantitative and analytical skills to approach and solve problems in public health and clinical research and practice. This field is designed for mid-career health professionals, research scientists, and MD/MPH specific dual/joint-degree students.
In health science research, there is a priority to develop new methodologies to improve the quality and scientific strength of data leading to an extraordinary increase in methodological diversity. ... This emphasizes the importance of applying mixed qualitative and quantitative methods in health research to ensure a focus on the priorities ...
The Committee is an academic unit that oversees three training programs and organizes a biweekly workshop that serves as an important forum for scholarly exchanges. The Certificate Program in Advanced Quantitative Methods is designed for doctoral students in the social, behavioral, and health sciences who wish to deepen their methodological ...
Quantitative research is used in many different fields, including social sciences, business, engineering, and health sciences. It can be used to investigate a wide range of phenomena, from human behavior and attitudes to physical and biological processes.
Using Q methodology in health sciences education to study subjectivity Our concern, however, is not to be with Q-technique alone, or even principally. Rather, it is with a challenge to psychology, in certain of its aspects, to put its house in scientific order. We are to consider a methodology to serve this purpose. We call it "Q-methodology."
Background: Evidence about which firearm policies work, to what extent, and for whom is hotly debated, perhaps partly because variation in research methodology has produced mixed and inconclusive effect estimates. We conducted a scoping review of firearm policy research in the health sciences in the United States, focusing on methodological considerations for causal inference.
Results suggest that the IP-10 assay is a sensitive and reproducible method and further technically replicates previous findings supporting IP-10 as a potential pharmacodynamic biomarker for ...
The median value is the middle value of the ordered set. Where there is an odd number of values in a dataset, the middle value is the median. However, where there is an even number of values, the two middle values are added together and divided by 2 to obtain the median. The formula for this is: n 1 2. ð þ Þ=.