- Sign Up Now
- -- Navigate To -- CR Dashboard Connect for Researchers Connect for Participants
- Log In Log Out Log In
- Recent Press
- Papers Citing Connect
- Connect for Participants
- Connect for Researchers
- Connect AI Training
- Managed Research
- Prime Panels
- MTurk Toolkit
- Health & Medicine
- Conferences
- Knowledge Base
- The Online Researcher’s Guide To Sampling
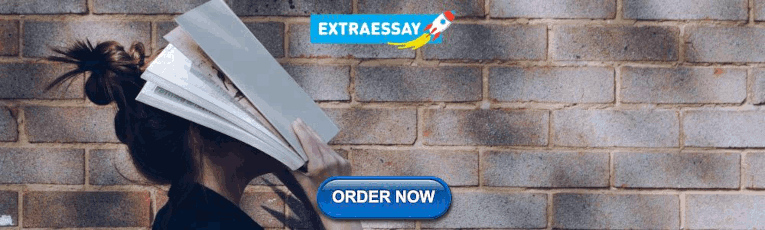
What Is the Purpose of Sampling in Research?
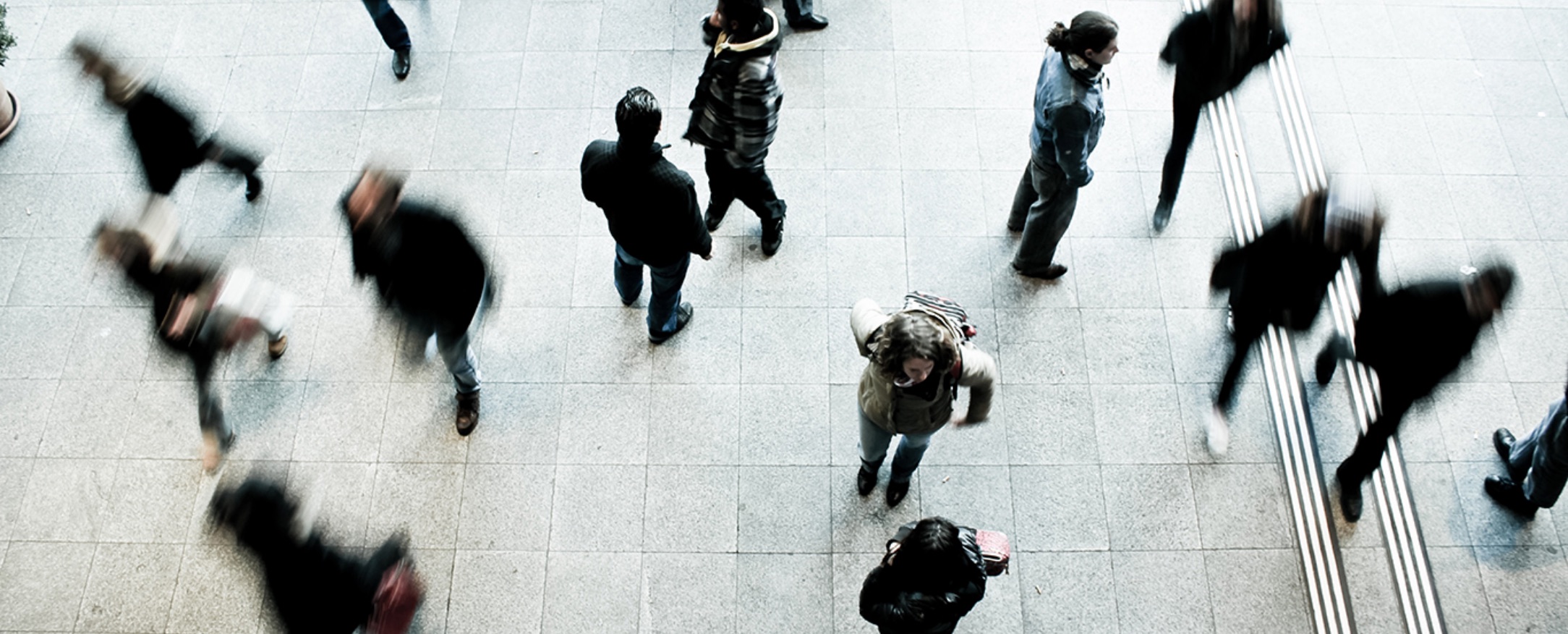
Quick Navigation:
Defining random vs. non-random sampling.
- Why is Sampling Important for Researchers?
Collect Richer Data
The importance of knowing where to sample.
- Different Use Cases for Online Sampling
Academic Research
Market research, public polling, user testing.
By Aaron Moss, PhD, Cheskie Rosenzweig, PhD, & Leib Litman, PhD
Online Researcher’s Sampling Guide, Part 1: What Is the Purpose of Sampling in Research?
Every ten years, the U.S. government conducts a census—a count of every person living in the country—as required by the constitution. It’s a massive undertaking.
The Census Bureau sends a letter or a worker to every U.S. household and tries to gather data that will allow each person to be counted. After the data are gathered, they have to be processed, tabulated and reported. The entire operation takes years of planning and billions of dollars, which begs the question: Is there a better way?
As it turns out, there is.
Instead of contacting every person in the population, researchers can answer most questions by sampling people. In fact, sampling is what the Census Bureau does in order to gather detailed information about the population such as the average household income, the level of education people have, and the kind of work people do for a living. But what, exactly, is sampling, and how does it work?
At its core, a research sample is like any other sample: It’s a small piece or part of something that represents a larger whole.
So, just like the sample of glazed salmon you eat at Costco or the double chocolate brownie ice cream you taste at the ice cream shop, behavioral scientists often gather data from a small group (a sample) as a way to understand a larger whole (a population). Even when the population being studied is as large as the U.S.—about 330 million people—researchers often need to sample just a few thousand people in order to understand everyone.
Now, you may be asking yourself how that works. How can researchers accurately understand hundreds of millions of people by gathering data from just a few thousand of them? Your answer comes from Valery Ivanovich Glivenko and Francesco Paolo Cantelli.
Glivenko and Cantelli were mathematicians who studied probability. At some point during the early 1900s, they discovered that several observations randomly drawn from a population will naturally take on the shape of the population distribution. What this means in plain English is that, as long as researchers randomly sample from a population and obtain a sufficiently sized sample, then the sample will contain characteristics that roughly mirror those of the population.
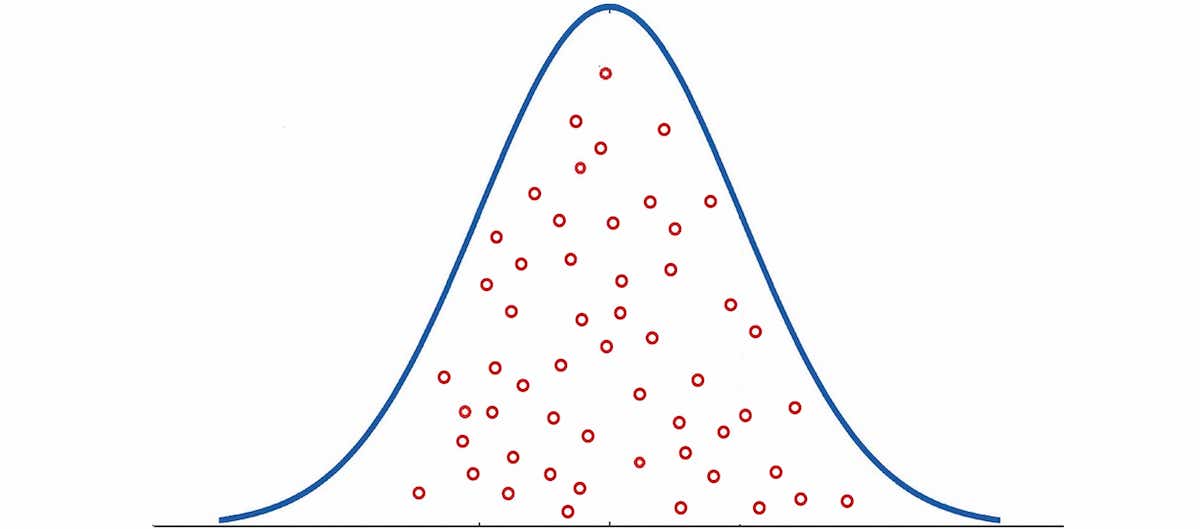
“Ok. That’s great,” you say. But what does it mean to randomly sample people, and how does a researcher do that?
Random sampling occurs when a researcher ensures every member of the population being studied has an equal chance of being selected to participate in the study. Importantly, ‘the population being studied’ is not necessarily all the inhabitants of a country or a region. Instead, a population can refer to people who share a common quality or characteristic. So, everyone who has purchased a Ford in the last five years can be a population and so can registered voters within a state or college students at a city university. A population is the group that researchers want to understand.
In order to understand a population using random sampling, researchers begin by identifying a sampling frame —a list of all the people in the population the researchers want to study. For example, a database of all landline and cell phone numbers in the U.S. is a sampling frame. Once the researcher has a sampling frame, he or she can randomly select people from the list to participate in the study.
However, as you might imagine, it is not always practical or even possible to gather a sampling frame. There is not, for example, a master list of all the people who use the internet, purchase coffee at Dunkin’, have grieved the death of a parent in the last year, or consider themselves fans of the New York Yankees. Nevertheless, there are very good reasons why researchers may want to study people in each of these groups.
When it isn’t possible or practical to gather a random sample, researchers often gather a non-random sample. A non-random sample is one in which every member of the population being studied does not have an equal chance of being selected into the study.
Because non-random samples do not select participants based on probability, it is often difficult to know how well the sample represents the population of interest. Despite this limitation, a wide range of behavioral science studies conducted within academia, industry and government rely on non-random samples. When researchers use non-random samples, it is common to control for any known sources of sampling bias during data collection. By controlling for possible sources of bias, researchers can maximize the usefulness and generalizability of their data.
Why Is Sampling Important for Researchers?
Everyone who has ever worked on a research project knows that resources are limited; time, money and people never come in an unlimited supply. For that reason, most research projects aim to gather data from a sample of people, rather than from the entire population (the census being one of the few exceptions). This is because sampling allows researchers to:
Contacting everyone in a population takes time. And, invariably, some people will not respond to the first effort at contacting them, meaning researchers have to invest more time for follow-up. Random sampling is much faster than surveying everyone in a population, and obtaining a non-random sample is almost always faster than random sampling. Thus, sampling saves researchers lots of time.
The number of people a researcher contacts is directly related to the cost of a study. Sampling saves money by allowing researchers to gather the same answers from a sample that they would receive from the population.
Non-random sampling is significantly cheaper than random sampling, because it lowers the cost associated with finding people and collecting data from them. Because all research is conducted on a budget, saving money is important.
Sometimes, the goal of research is to collect a little bit of data from a lot of people (e.g., an opinion poll). At other times, the goal is to collect a lot of information from just a few people (e.g., a user study or ethnographic interview). Either way, sampling allows researchers to ask participants more questions and to gather richer data than does contacting everyone in a population.
Efficient sampling has a number of benefits for researchers. But just as important as knowing how to sample is knowing where to sample . Some research participants are better suited for the purposes of a project than others. Finding participants that are fit for the purpose of a project is crucial, because it allows researchers to gather high-quality data.
For example, consider an online research project. A team of researchers who decides to conduct a study online has several different sources of participants to choose from. Some sources provide a random sample, and many more provide a non-random sample. When selecting a non-random sample, researchers have several options to consider. Some studies are especially well-suited to an online panel that offers access to millions of different participants worldwide. Other studies, meanwhile, are better suited to a crowdsourced site that generally has fewer participants overall but more flexibility for fostering participant engagement.
To make these options more tangible, let’s look at examples of when researchers might use different kinds of online samples.
Different Use Cases of Online Sampling
Academic researchers gather all kinds of samples online. Some projects require random samples based on probability sampling methods. Most other projects rely on non-random samples. In these non-random samples, researchers may sample a general audience from crowdsourcing websites or selectively target members of specific groups using online panels . The variety of research projects conducted within academia lends itself to many different types of online samples.
Market researchers often want to understand the thoughts, feelings and purchasing decisions of customers or potential customers. For that reason, most online market research is conducted in online panels that provide access to tens of millions of people and allow for complex demographic targeting. For some projects, crowdsourcing sites, such as Amazon Mechanical Turk, allow researchers to get more participant engagement than is typically available in online panels, because they allow researchers to select participants based on experience and to award bonuses.
Public polling is most accurate when it is conducted on a random sample of the population. Hence, lots of public polling is conducted with nationally representative samples. There are, however, an increasing number of opinion polls conducted with non-random samples. When researchers poll people using non-random methods, it is common to adjust for known sources of bias after the data are gathered.
User testing requires people to engage with a website or product. For this reason, user testing is best done on platforms that allow researchers to get participants to engage deeply with their study. Crowdsourcing platforms are ideal for user testing studies, because researchers can often control participant compensation and reward people who are willing to make the effort in a longer study.
Online research is big business. There are hundreds of companies that provide researchers with access to online participants, but only a few facilitate research across different types of online panels or direct you to the right panel for your project. At CloudResearch, we are behavioral and computer science experts with the knowledge to connect you with the right participants for your study and provide expert advice to ensure your project’s successful conclusion. Learn more by contacting us today.
Continue Reading: The Online Researcher’s Guide to Sampling
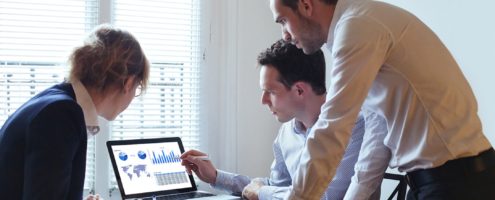
Part 2: How to Reduce Sampling Bias in Research
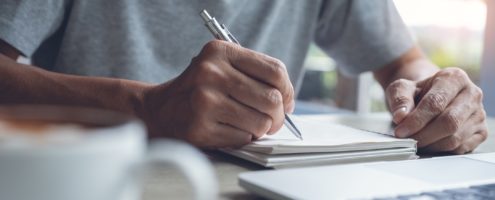
Part 3: How to Build a Sampling Process for Marketing Research
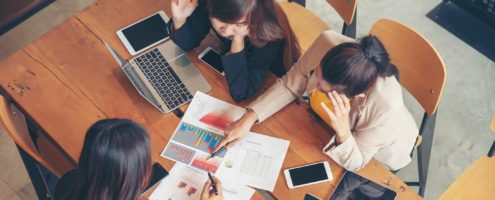
Part 4: Pros and Cons of Different Sampling Methods
Related articles, what is data quality and why is it important.
If you were a researcher studying human behavior 30 years ago, your options for identifying participants for your studies were limited. If you worked at a university, you might be...
How to Identify and Handle Invalid Responses to Online Surveys
As a researcher, you are aware that planning studies, designing materials and collecting data each take a lot of work. So when you get your hands on a new dataset,...
SUBSCRIBE TO RECEIVE UPDATES
2024 grant application form, personal and institutional information.
- Full Name * First Last
- Position/Title *
- Affiliated Academic Institution or Research Organization *
Detailed Research Proposal Questions
- Project Title *
- Research Category * - Antisemitism Islamophobia Both
- Objectives *
- Methodology (including who the targeted participants are) *
- Expected Outcomes *
- Significance of the Study *
Budget and Grant Tier Request
- Requested Grant Tier * - $200 $500 $1000 Applicants requesting larger grants may still be eligible for smaller awards if the full amount requested is not granted.
- Budget Justification *
Research Timeline
- Projected Start Date * MM slash DD slash YYYY Preference will be given to projects that can commence soon, preferably before September 2024.
- Estimated Completion Date * MM slash DD slash YYYY Preference will be given to projects that aim to complete within a year.
- Project Timeline *
- Comments This field is for validation purposes and should be left unchanged.
- Name * First Name Last Name
- I would like to request a demo of the Sentry platform
- Email This field is for validation purposes and should be left unchanged.
- Name * First name Last name
- Name * First Last
- Name This field is for validation purposes and should be left unchanged.
- Name * First and Last
- Please select the best time to discuss your project goals/details to claim your free Sentry pilot for the next 60 days or to receive 10% off your first Managed Research study with Sentry.
- Email * Enter Email Confirm Email
- Organization
- Job Title *
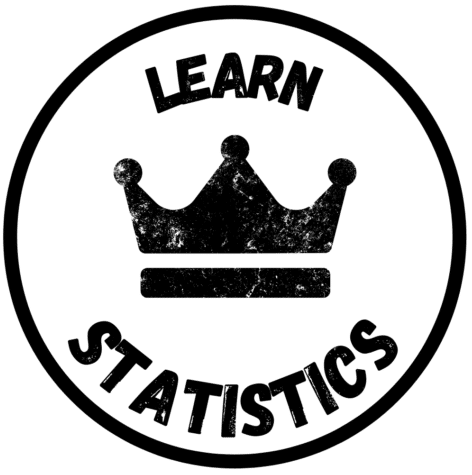
LEARN STATISTICS EASILY
Learn Data Analysis Now!

Understanding Random Sampling: Essential Techniques in Data Analysis
Random sampling in statistics is a technique for selecting a subset of individuals from a larger population where each individual has an equal chance of being chosen. This method ensures representative samples, minimizes bias and allows for reliable inferences about the population based on the sample data.
Definition and Importance of Random Sampling
Random sampling is fundamental in data analysis, statistics, and broader scientific research. It refers to the technique of selecting individuals or elements from a population such that each individual has an equal probability of being chosen. This method is essential as it ensures a representative sample, thereby eliminating bias and enabling researchers to draw valid conclusions about the whole population based on the sample data.
The importance of random sampling in data analysis cannot be overstated. Instead, it forms the basis of hypothesis testing, inferential statistics, and prediction modeling. Without random sampling, we risk introducing selection bias into our study, which can lead to inaccurate conclusions and misleading results. The strength of random sampling lies in its ability to mirror the characteristics of the whole population within the sample, enhancing the reliability and validity of the analysis.
- In random sampling, every member of a population has an equal chance to be chosen as part of the sample.
- It forms the basis of hypothesis testing, inferential statistics, and prediction modeling.
- Simple random sampling, the most basic form, is adequate when the population is homogeneous.
- Stratified random sampling divides the population into subgroups, ensuring sufficient representation.
- Systematic random sampling selects individuals at regular intervals from the population.
Ad description. Lorem ipsum dolor sit amet, consectetur adipiscing elit.
Types of Random Sampling
Simple random sampling.
Simple Random Sampling is the most basic type of random sampling. Each population element has an equal chance of being selected in this method. The selection is often made through a random process, such as using a random number generator or drawing names from a hat. This method is most effective when the population is homogeneous, i.e., when the characteristics of individuals don’t significantly vary. Imagine a small town that wants to survey residents’ satisfaction with local services. They could use simple random sampling by assigning each resident a number and then using a random number generator to select 100 residents to participate in the survey.
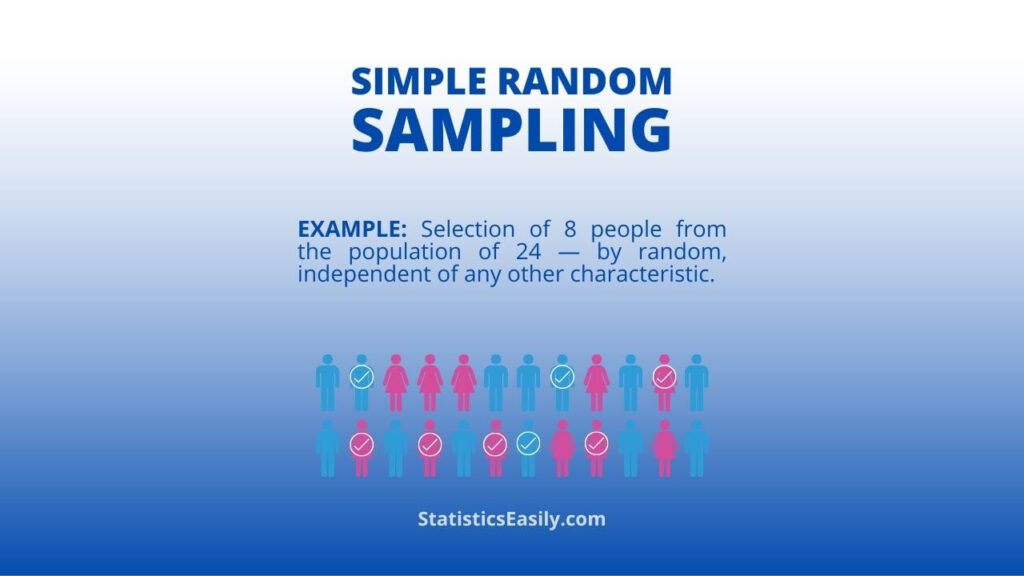
Stratified Random Sampling
Stratified Random Sampling is a technique used when the population is not homogeneous. The population is categorized into strata (or subgroups) based on specific characteristics such as age, gender, or geographic location. Then, random sampling is applied within each stratum to select the individuals. This method ensures that each subgroup is adequately represented in the sample. Suppose a national clothing retailer wants to understand customer satisfaction across different age groups. They could divide their customer base into distinct age groups, such as 18-29, 30-39, 40-49, etc., and then perform simple random sampling within these strata to ensure that all age groups are adequately represented.
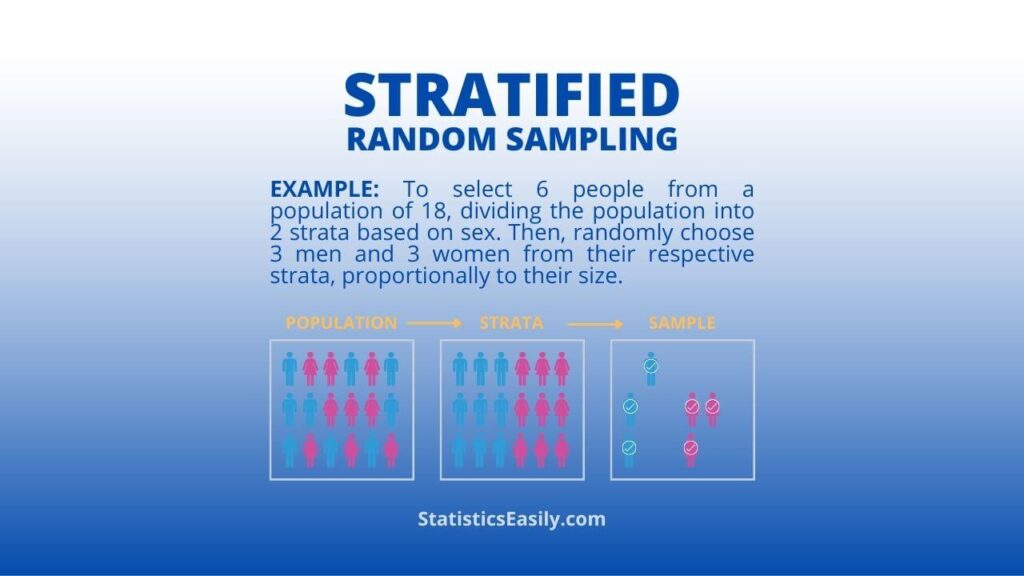
Systematic Random Sampling
Systematic Random Sampling involves selecting individuals at regular intervals from the population. The first individual is chosen randomly, and then every nth is selected. This method is often used when a complete list of the population is available, and it’s important to note that it requires the assumption that the list is not patterned in any way. Suppose a university wants to assess the effectiveness of its new online learning platform. They could use systematic random sampling by alphabetizing all students and selecting every 10th student for a survey. This method would provide a sample spread evenly across the entire student population.
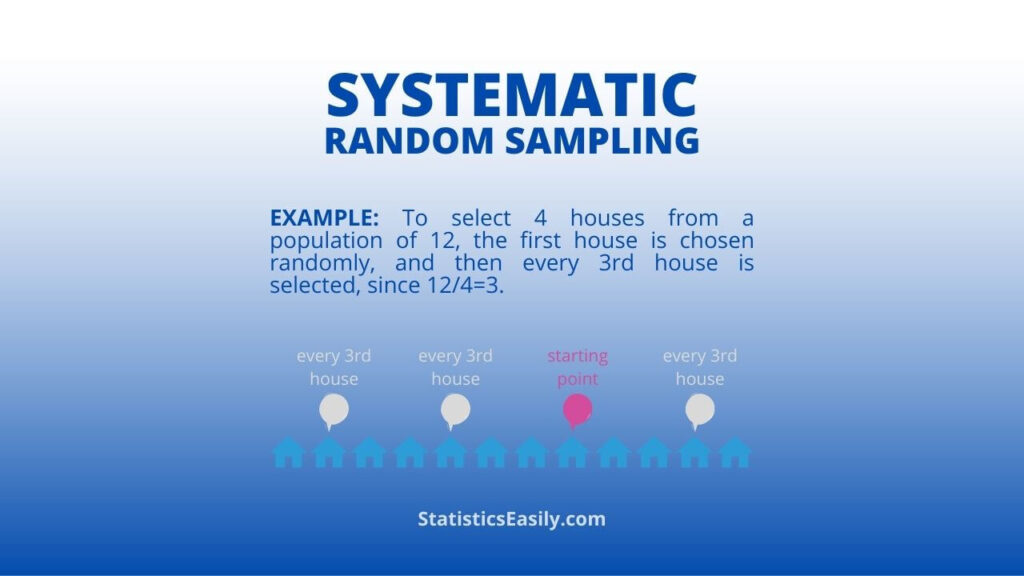
Cluster Random Sampling
Cluster Random Sampling involves dividing the population into separate groups or clusters, usually based on geographic location. A random sample of clusters is selected, and all individuals within these chosen clusters are included. This method is often used when conducting simple or stratified sampling is costly or impractical. Consider a situation where a government health agency wants to study lifestyle habits nationwide. It would be impractical and expensive to randomly sample individuals from the entire country. Instead, they could use cluster sampling. They might divide the country into clusters by postcode and then randomly select a few postcodes. Every resident within the selected postcodes would be included in the study.
Challenges and Misconceptions about Random Sampling
Despite the importance of random sampling, several challenges and misconceptions can hamper its effective implementation.
One common misconception is that random sampling produces a sample that perfectly represents the population. While random sampling is designed to minimize bias and increase the likelihood of representativeness, it does not guarantee it. There’s always a chance that the sample might not accurately reflect the population due to random variation.
Another challenge is the practical implementation of random sampling. Often, having a complete population list or randomly selecting individuals may be impossible. For instance, respondents self-select to participate in online surveys, which may introduce bias.
Furthermore, there is a typical misconception that a larger sample is always better. While it’s true that increasing the sample size can often decrease the margin of error and increase the confidence level, it also increases the time and cost of data collection and analysis. Therefore, balancing the need for precision with practical considerations is crucial.
In summary, while random sampling is a cornerstone of statistical and data analysis, it has challenges and misconceptions. Understanding these can help researchers and analysts better design and implement their studies for robust, reliable, and meaningful results.
Recommended Articles
Want to explore more about data analysis and statistics? Don’t stop at random sampling. Our blog features many articles covering various topics that will deepen your understanding and enhance your skills. Whether starting or looking to advance your knowledge, we’ve got you covered. Look at our other posts today and continue your learning journey with us!
- Understanding Sampling Error: A Foundation in Statistical Analysis
- Selection Bias in Data Analysis: Understanding the Intricacies
- Simple Random Sample – an overview (External Link)
- Unraveling Sampling Bias: A Comprehensive Guide
- Random Sampling on Excel: An In-depth Analysis
- Understanding Random Sampling (Story)
- Generate a Random Number
Frequently Asked Questions (FAQs)
The four main types of random sampling are Simple, Stratified, Cluster, and Systematic Random Sampling. Each has its unique application depending on the nature of the population and the research question.
Random sampling is used to pick a representative sample from a larger population, ensuring each individual has an equal chance of being chosen. This minimizes selection bias, making inferences about the population more accurate.
A random sample in statistics is a subset of individuals or data points selected from a larger population. Each individual or point has an equal probability of being chosen.
Random sampling is done by assigning each individual in the population a unique identifier and then using a random process (like a random number generator) to select a subset of individuals.
The “best” random sampling method depends on the specifics of the study, including the nature of the population, the research question, and practical considerations. Each method has its strengths and weaknesses.
The choice of sampling method depends on several factors, including the research question, the nature of the population, the availability of a complete list of the population, and practical constraints such as time and cost.
Challenges of random sampling include practical implementation issues, the potential for nonresponse bias, and the misconception that a larger sample is always better or more representative.
While random sampling can help reduce selection bias, it does not stop all types of bias. For example, it can’t correct measurement errors or biases in data collection.
Stratified random sampling is distinct from simple random sampling. It first divides the population into different subgroups, or strata, based on specific characteristics. Then, simple random sampling is performed within each subset. This ensures that each subgroup is adequately represented in the sample, which can be especially useful when the population is heterogeneous.
Cluster random sampling involves dividing the population into clusters and then randomly selecting a few clusters for study. For instance, a researcher studying educational practices might divide a country into clusters by school districts, then randomly select a few districts. All schools within these selected districts would be included in the study.
Similar Posts
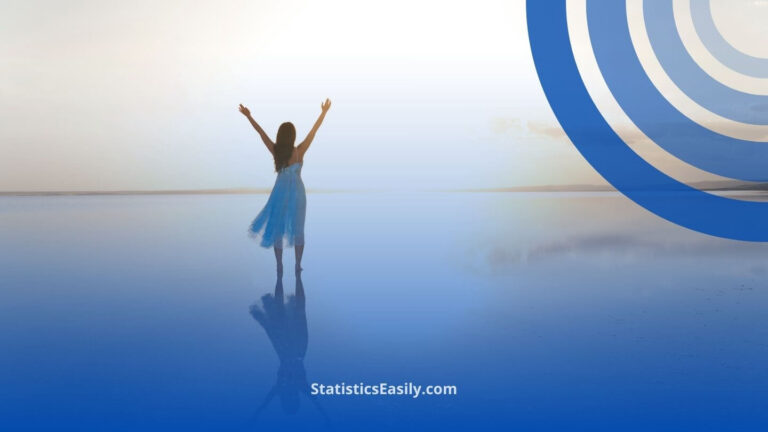
How Do You Calculate Degrees of Freedom?
Master “How do you calculate degrees of freedom” in statistical analysis to enhance data accuracy and insights.
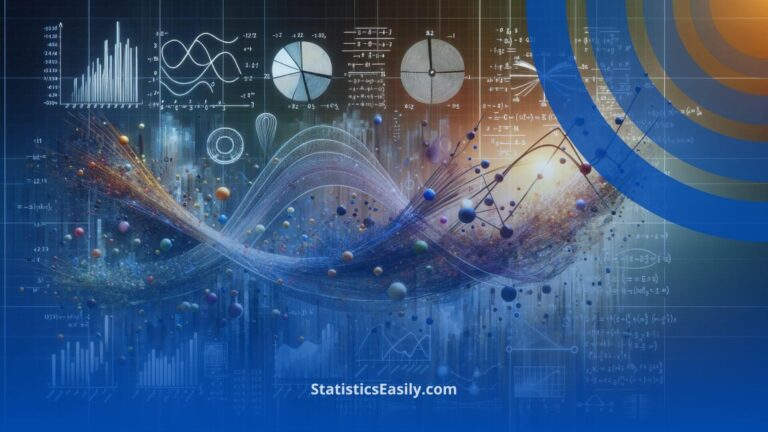
Understanding Distributions of Generalized Linear Models
Dive into “Distributions of Generalized Linear Models” to master the core concepts and applications of statistical modeling.
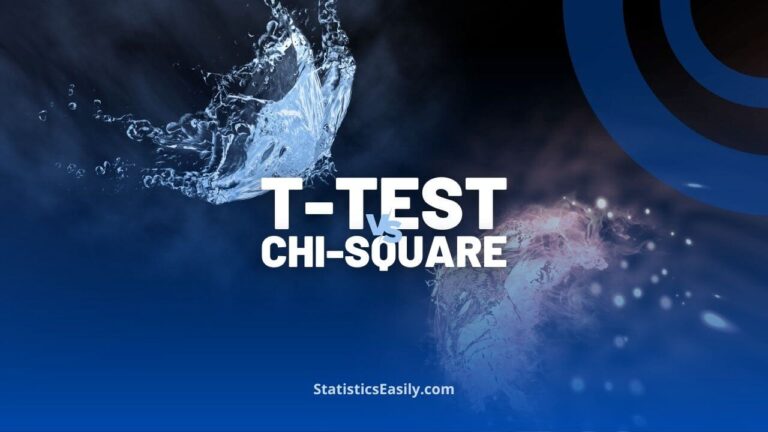
What is the Difference Between the T-Test vs. Chi-Square Test?
Explore the fundamental differences between the t-test vs. chi-square test, crucial tools in statistics and data analysis.
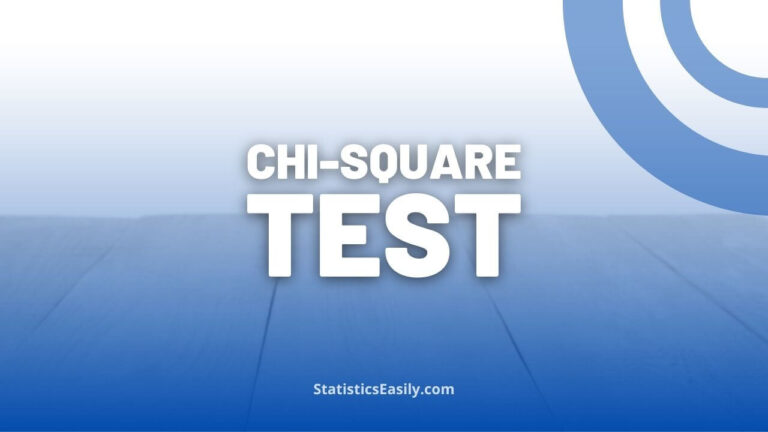
Mastering the Chi-Square Test: A Comprehensive Guide
Uncover the workings, assumptions, and limitations of the Chi-Square Test — a vital tool for analyzing categorical data.
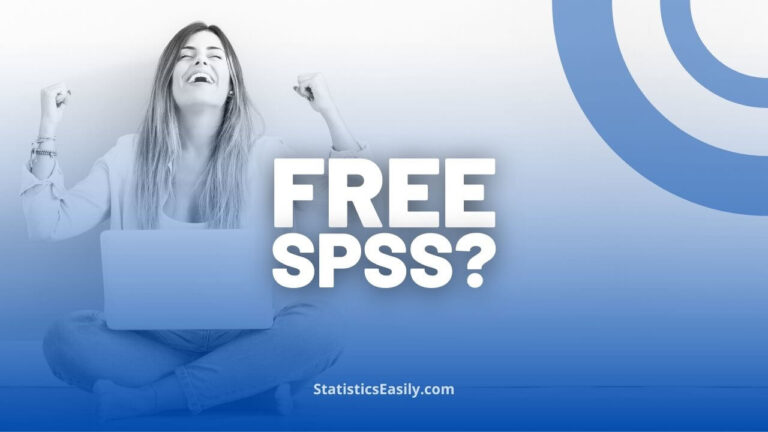
Is PSPP A Free Alternative To SPSS?
Explore PSPP, a free alternative to SPSS, offering similar functionality and user interface for data analysis.
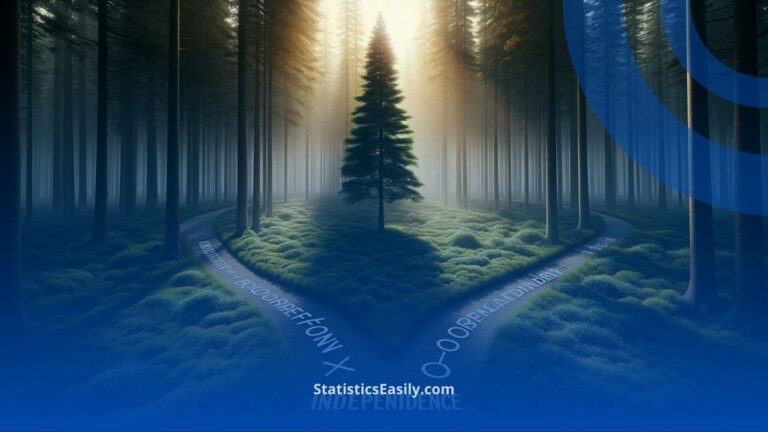
Correlation Antonym: Understanding Independence in Statistical Analysis
Discover the essence of independence, the statistical correlation antonym, and its impact on data analysis through a concise overview.
Leave a Reply Cancel reply
Your email address will not be published. Required fields are marked *
Save my name, email, and website in this browser for the next time I comment.
Numbers, Facts and Trends Shaping Your World
Read our research on:
Full Topic List
Regions & Countries
- Publications
- Our Methods
- Short Reads
- Tools & Resources
Read Our Research On:
Methods 101: Random Sampling
The first video in Pew Research Center’s Methods 101 series helps explain random sampling – a concept that lies at the heart of all probability-based survey research – and why it’s important.
Sign up for our weekly newsletter
Fresh data delivered Saturday mornings
1615 L St. NW, Suite 800 Washington, DC 20036 USA (+1) 202-419-4300 | Main (+1) 202-857-8562 | Fax (+1) 202-419-4372 | Media Inquiries
Research Topics
- Age & Generations
- Coronavirus (COVID-19)
- Economy & Work
- Family & Relationships
- Gender & LGBTQ
- Immigration & Migration
- International Affairs
- Internet & Technology
- Methodological Research
- News Habits & Media
- Non-U.S. Governments
- Other Topics
- Politics & Policy
- Race & Ethnicity
- Email Newsletters
ABOUT PEW RESEARCH CENTER Pew Research Center is a nonpartisan fact tank that informs the public about the issues, attitudes and trends shaping the world. It conducts public opinion polling, demographic research, media content analysis and other empirical social science research. Pew Research Center does not take policy positions. It is a subsidiary of The Pew Charitable Trusts .
Copyright 2024 Pew Research Center
Terms & Conditions
Privacy Policy
Cookie Settings
Reprints, Permissions & Use Policy

The Importance of Sampling Methods in Research Design
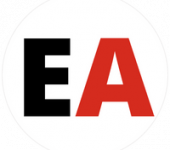
In research design , population and sampling are two important terms. A population is a group of individuals that share common connections. A sample is a subset of the population. The sample size is the number of individuals in a sample. The more representative the sample of thepopulation, the more confident the researcher can be in the quality of the results.
Types of Sampling Methods
Illustration of the importance of sampling:
A researcher might want to study the adverse health effects associated with working in a coal mine. However, it would be impossible to study a large population of coal workers. So, the researcher would need to narrow down the population and build a sample to collect data. This sample might be a group of coal workers in one city.
Sampling methods are as follows:
Probability Sampling is a method wherein each member of the population has the same probability of being a part of the sample.
Non-probability Sampling is a method wherein each member of the population does not have an equal chance of being selected. When the researcher desires to choose members selectively,non-probability sampling is considered. Both sampling techniques are frequently utilized. However, one works better than others depending on research needs.
Qualitative and Quantitative Research
In Qualitative research , non-numerical data is used to study elements in their natural settings. This helps to interpret and measure how these elements affect humans or other living beings. There are three main types of qualitative sampling:
- Purposive sampling: Pre-selected criteria related to research hypothesis determines the participants for research, for example, a study on cancer rates for individuals who live near a nuclear power station.
- Quota sampling: The researcher establishes participant quotas before forming a sample . Selection of participants that meet certain traits like gender, age, health, etc.
- Snowball sampling: The participants in the study refer other individuals who fit the traits required for the study, to the researcher.
Quantitative research is used to categorize, rank, and measure numerical data. Researchers establish general laws of behavior found in different contexts and settings. The goal is to test a theory and support or reject it.
The three main types of quantitative sampling are:
- Random sampling: Random sampling is when all individuals in a population have an equal chance of being selected.
- Stratified sampling: Stratified sampling is when the researcher defines the types of individuals in the population based on specific criteria for the study. For example, a study on smoking might need to break down its participants by age, race, or socioeconomic status.
- Systematic sampling: Systemic sampling is choosing a sample on an orderly basis. To build the sample, look at the target population and choose every fifth, tenth, or twentieth name, based upon the needs of the sample size.
The Importance of Selecting an Appropriate Sampling Method
Sampling yields significant research result. However, with the differences that can be present between a population and a sample, sample errors can occur. Therefore, it is essential to use the most relevant and useful sampling method.
Below are three of the most common sampling errors.
- Sampling bias occurs when the sample does not reflect the characteristics of the population.
- Sample frame errors occur when the wrong sub-population is used to select a sample. This can be due to gender, race, or economic factors.
- Systematic errors occur when the results from the sample differ significantly from the results of the population.
What is your experience with research design and sampling methods? Have you faced some of the challenges mentioned in this article? Please share your thoughts in the comments.
Rate this article Cancel Reply
Your email address will not be published.
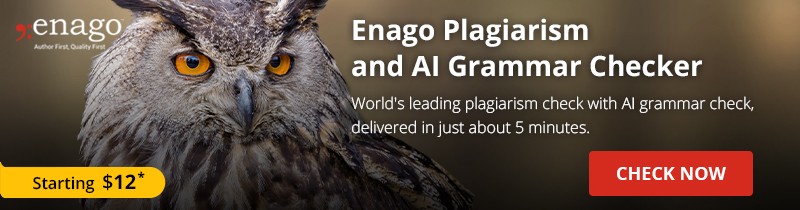
Enago Academy's Most Popular Articles
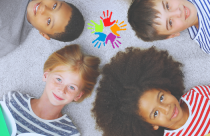
- Diversity and Inclusion
Need for Diversifying Academic Curricula: Embracing missing voices and marginalized perspectives
In classrooms worldwide, a single narrative often dominates, leaving many students feeling lost. These stories,…
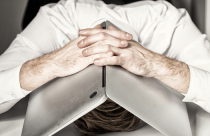
- Career Corner
- Trending Now
Recognizing the signs: A guide to overcoming academic burnout
As the sun set over the campus, casting long shadows through the library windows, Alex…
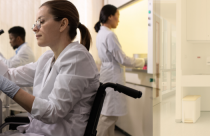
Reassessing the Lab Environment to Create an Equitable and Inclusive Space
The pursuit of scientific discovery has long been fueled by diverse minds and perspectives. Yet…
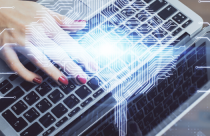
- AI in Academia
- Reporting Research
How to Improve Lab Report Writing: Best practices to follow with and without AI-assistance
Imagine you’re a scientist who just made a ground-breaking discovery! You want to share your…
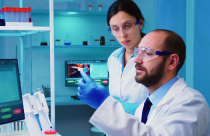
Achieving Research Excellence: Checklist for good research practices
Academia is built on the foundation of trustworthy and high-quality research, supported by the pillars…
7 Steps of Writing an Excellent Academic Book Chapter
When Your Thesis Advisor Asks You to Quit
Virtual Defense: Top 5 Online Thesis Defense Tips

Sign-up to read more
Subscribe for free to get unrestricted access to all our resources on research writing and academic publishing including:
- 2000+ blog articles
- 50+ Webinars
- 10+ Expert podcasts
- 50+ Infographics
- 10+ Checklists
- Research Guides
We hate spam too. We promise to protect your privacy and never spam you.
I am looking for Editing/ Proofreading services for my manuscript Tentative date of next journal submission:
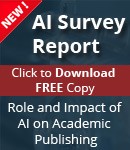
As a researcher, what do you consider most when choosing an image manipulation detector?
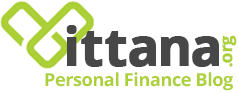
17 Advantages and Disadvantages of Random Sampling
The goal of random sampling is simple. It helps researchers avoid an unconscious bias they may have that would be reflected in the data they are collecting. This advantage, however, is offset by the fact that random sampling prevents researchers from being able to use any prior information they may have collected.
This means random sampling allows for unbiased estimates to be created, but at the cost of efficiency within the research process.
Here are some of the additional advantages and disadvantages of random sampling that worth considering.
What Are the Advantages of Random Sampling?
1. It offers a chance to perform data analysis that has less risk of carrying an error. Random sampling allows researchers to perform an analysis of the data that is collected with a lower margin of error. This is allowed because the sampling occurs within specific boundaries that dictate the sampling process. Because the whole process is randomized, the random sample reflects the entire population and this allows the data to provide accurate insights into specific subject matters.
2. There is an equal chance of selection. Random sampling allows everyone or everything within a defined region to have an equal chance of being selected. This helps to create more accuracy within the data collected because everyone and everything has a 50/50 opportunity. It is a process that builds an inherent “fairness” into the research being conducted because no previous information about the individuals or items involved are included in the data collection process.
3. It requires less knowledge to complete the research. A researcher does not need to have specific knowledge about the data being collected to be effective at their job. Researchers could ask someone who they prefer to be the next President of the United States without knowing anything about US political structures. In random sampling, a question is asked and then answered. An item is reviewed for a specific feature. If the researcher can perform that task and collect the data, then they’ve done their job.
4. It is the simplest form of data collection. This type of research involves basic observation and recording skills. It requires no basic skills out of the population base or the items being researched. It also removes any classification errors that may be involved if other forms of data collection were being used. Although the simplicity can cause some unintended problems when a sample is not a genuine reflection of the average population being reviewed, the data collected is generally reliable and accurate.
5. Multiple types of randomness can be included to reduce researcher bias. There are two common approaches that are used for random sampling to limit any potential bias in the data. The first is a lottery method, which involves having a population group drawing to see who will be included and who will not. Researchers can also use random numbers that are assigned to specific individuals and then have a random collection of those number selected to be part of the project.
6. It is easier to form sample groups. Because random sampling takes a few from a large population, the ease of forming a sample group out of the larger frame is incredibly easy. This makes it possible to begin the process of data collection faster than other forms of data collection may allow.
7. Findings can be applied to the entire population base. Because of the processes that allow for random sampling, the data collected can produce results for the larger frame because there is such little relevance of bias within the findings. The generalized representation that is present allows for research findings to be equally generalized.
What Are the Disadvantages of Random Sampling?
1. No additional knowledge is taken into consideration. Although random sampling removes an unconscious bias that exists, it does not remove an intentional bias from the process. Researchers can choose regions for random sampling where they believe specific results can be obtained to support their own personal bias. No additional knowledge is given consideration from the random sampling, but the additional knowledge offered by the researcher gathering the data is not always removed.
2. It is a complex and time-consuming method of research. With random sampling, every person or thing must be individually interviewed or reviewed so that the data can be properly collected. When individuals are in groups, their answers tend to be influenced by the answers of others. This means a researcher must work with every individual on a 1-on-1 basis. This requires more resources, reduces efficiencies, and takes more time than other research methods when it is done correctly.
3. Researchers are required to have experience and a high skill level. A researcher may not be required to have specific knowledge to conduct random sampling successfully, but they do need to be experienced in the process of data collection. There must be an awareness by the researcher when conducting 1-on-1 interviews that the data being offered is accurate or not. A high skill level is required of the researcher so they can separate accurate data that has been collected from inaccurate data. If that skill is not present, the accuracy of the conclusions produced by the offered data may be brought into question.
4. There is an added monetary cost to the process. Because the research must happen at the individual level, there is an added monetary cost to random sampling when compared to other data collection methods. There is an added time cost that must be included with the research process as well. The results, when collected accurately, can be highly beneficial to those who are going to use the data, but the monetary cost of the research may outweigh the actual gains that can be obtained from solutions created from the data.
5. No guarantee that the results will be universal is offered. Random sampling is designed to be a representation of a community or demographic, but there is no guarantee that the data collected is reflective of the community on average. In US politics, a random sample might collect 6 Democrats, 3 Republicans, and 1 Independents, though the actual population base might be 6 Republicans, 3 Democrats, and 1 Independent for every 10 people in the community. Asking who they want to be their President would likely have a Democratic candidate in the lead when the whole community would likely prefer the Republican.
6. It requires population grouping to be effective. If the population being surveyed is diverse in its character and content, or it is widely dispersed, then the information collected may not serve as an accurate representation of the entire population. These issues also make it difficult to contact specific groups or people to have them included in the research or to properly catalog the data so that it can serve its purpose.
7. It is easy to get the data wrong just as it is easy to get right. The application of random sampling is only effective when all potential respondents are included within the large sampling frame. Everyone or everything that is within the demographic or group being analyzed must be included for the random sampling to be accurate. If the sampling frame is exclusionary, even in a way that is unintended, then the effectiveness of the data can be called into question and the results can no longer be generalized to the larger group.
8. A large sample size is mandatory. For random sampling to work, there must be a large population group from which sampling can take place. It would be possible to draw conclusions for 1,000 people by including a random sample of 50. It would not be possible to draw conclusions for 10 people by randomly selecting two people. A large sample size is always necessary, but some demographics or groups may not have a large enough frame to support the methodology offered by random sampling.
9. A sample size that is too large is also problematic. Since every member is given an equal chance at participation through random sampling, a population size that is too large can be just as problematic as a population size that is too small. Larger populations require larger frames that still demand accuracy, which means errors can creep into the data as the size of the frame increases.
10. The quality of the data is reliant on the quality of the researcher. This potential negative is especially true when the data being collected comes through face-to-face interviews. A poor interviewer would collect less data than an experienced interviewer. An interviewer who refuses to stick to a script of questions and decides to freelance on follow-ups may create biased data through their efforts. Poor research methods will always result in poor data.
The advantages and disadvantages of random sampling show that it can be quite effective when it is performed correctly. Random sampling removes an unconscious bias while creating data that can be analyzed to benefit the general demographic or population group being studied. If controls can be in place to remove purposeful manipulation of the data and compensate for the other potential negatives present, then random sampling is an effective form of research.
Simple Random Sampling Method: Definition & Example
Julia Simkus
Editor at Simply Psychology
BA (Hons) Psychology, Princeton University
Julia Simkus is a graduate of Princeton University with a Bachelor of Arts in Psychology. She is currently studying for a Master's Degree in Counseling for Mental Health and Wellness in September 2023. Julia's research has been published in peer reviewed journals.
Learn about our Editorial Process
Saul Mcleod, PhD
Editor-in-Chief for Simply Psychology
BSc (Hons) Psychology, MRes, PhD, University of Manchester
Saul Mcleod, PhD., is a qualified psychology teacher with over 18 years of experience in further and higher education. He has been published in peer-reviewed journals, including the Journal of Clinical Psychology.
Olivia Guy-Evans, MSc
Associate Editor for Simply Psychology
BSc (Hons) Psychology, MSc Psychology of Education
Olivia Guy-Evans is a writer and associate editor for Simply Psychology. She has previously worked in healthcare and educational sectors.
On This Page:
Simple random sampling is a technique in which each member of a population has an equal chance of being chosen through an unbiased selection method. Each subject in the sample is given a number, and then the sample is chosen randomly.
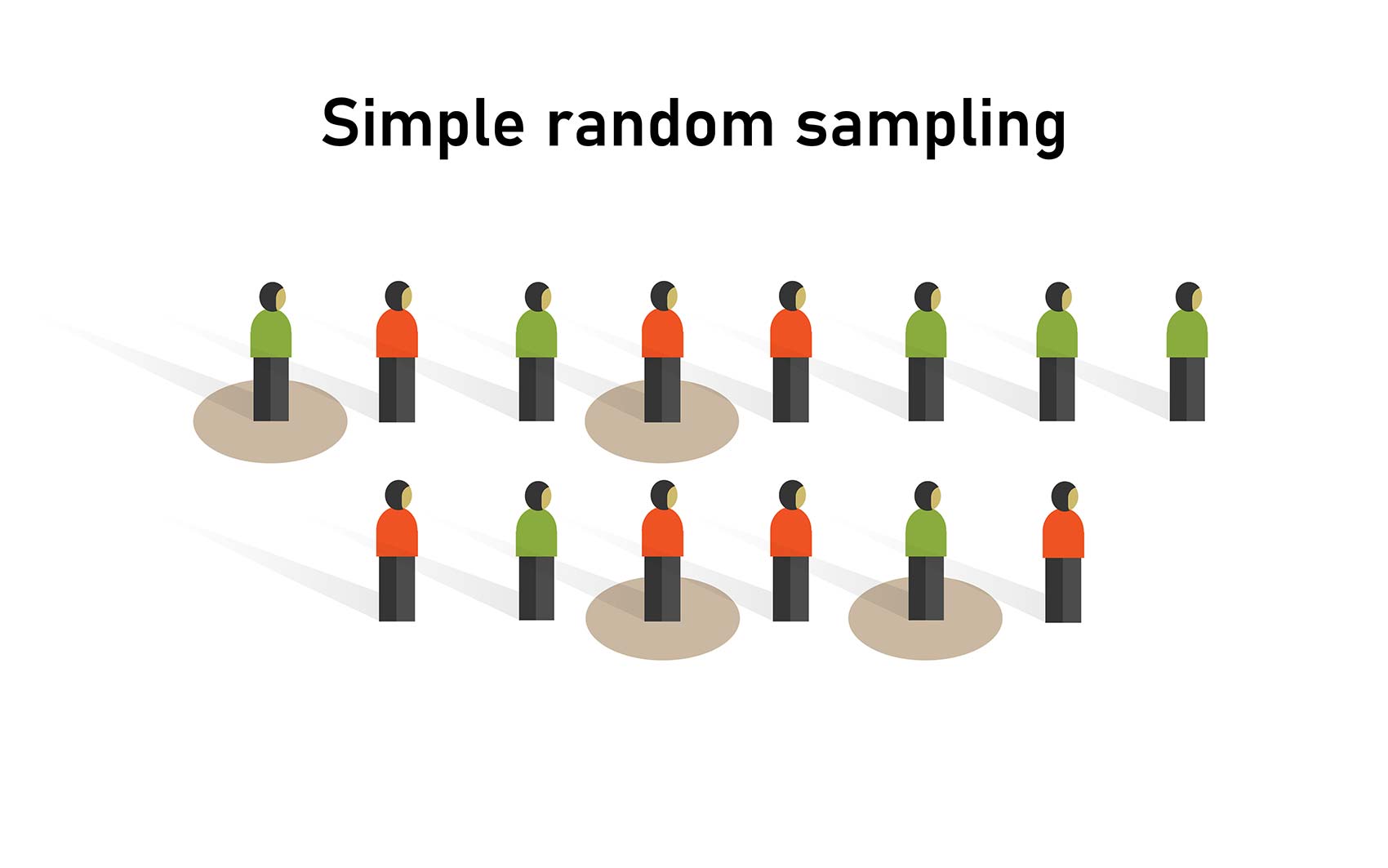
The random sampling method is one of the simplest and most common forms of collecting data, as it provides an unbiased representation of a group. The random subset of selected individuals represents an entire data set.
The goal of simple random sampling is to create a manageable, balanced subset of individuals that is representative of a larger group that would otherwise be too challenging to sample.
For example, if you wanted to conduct a survey about food preferences in a school of 1000 students, and you wanted to sample 100 students.
You could use simple random sampling by assigning each student a number from 1 to 1000, then using a random number generator to pick 100 numbers.
The students assigned those numbers would be the ones you survey.
- First, choose the target population that you wish to study and determine your desired sample size. The smaller the sample size the less likely, it can be generalized to the wider research population and is unlikely to be fully representative.
- The list of the people from which the sample is drawn is called the sampling frame. Examples of sampling frames include the electoral register, schools, drug addicts, etc.).
- Then, assign a sequential number to each subject in the sampling frame.
- Next, individuals are selected using an unbiased selection method. Some examples of simple random sampling techniques include lotteries, random computer number generators, or random draws.
Minimizes Bias
It is the least biased sampling method, as every member of the target population has an equal chance of being chosen. The purpose of simple random sampling is to give each individual an equal chance of being chosen.
This is meant to represent a group that is free from researcher bias. Like any sampling technique, there is room for error, but this method is intended to be an unbiased approach.
Representativeness
Random sampling ensures that every member of the target population has an equal chance of being selected. This helps to ensure that the sample is representative of the population, making it more likely that the findings can be generalized to the entire population.
Limitations
Expensive and time-consuming.
It is a very expensive and time-consuming method; it is difficult to get the name of every member of the target population, especially if it is a very large population, so it is rarely used.
Access to respondents
This is actually quite hard to achieve – especially if the parent population is large. Since the participants do not volunteer to participate, it can be challenging for researchers to gain access to respondents when drawing from a large population.
Sampling error
Sampling errors can occur when the sample does not accurately represent the population as a whole. If this occurs, the researcher would need to restart the sampling process.
Other techniques
There are four types of random sampling techniques (simple, stratified, cluster, and systematic random sampling.
Stratified Random Sampling
- In stratified random sampling , researchers will first divide a population into subgroups, or strata, based on shared characteristics and then randomly select among these groups.
- This method is typically used when a population has distinct differences, such as demographics, level of education, or age, and can easily be broken into subgroups.
Cluster Random Sampling
- Similar to stratified random sampling, cluster random sampling begins by dividing a population into smaller groups.
- However, in cluster sampling, researchers use naturally formed groups to divide a large population up into clusters and then select randomly among the clusters to form the sample.
- Examples of these pre-existing groups could include school districts, city blocks, or households.
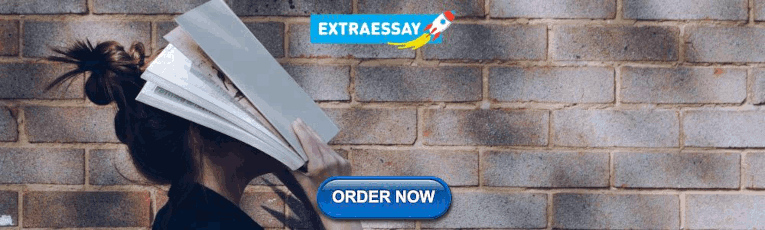
Systematic Random Sampling
- Systematic random sampling involves taking random samples at regular periodic intervals.
- For example, if you were conducting a survey in a cafeteria, you could give a survey to every sixth customer that comes into the cafeteria.
- A sample is the participants you select from a target population (the group you are interested in) to make generalizations about. As an entire population tends to be too large to work with, a smaller group of participants must act as a representative sample.
- Representative means the extent to which a sample mirrors a researcher’s target population and reflects its characteristics (e.g., gender, ethnicity, socioeconomic level). In an attempt to select a representative sample and avoid sampling bias (the over-representation of one category of participant in the sample), psychologists utilize various sampling methods.
- Generalisability means the extent to which their findings can be applied to the larger population of which their sample was a part.
Hayes, A. (2021). Simple Random Sample. Investopedia. Retrieved from https://www.investopedia.com/terms/s/simple-random-sample.asp
Simple random sample: Definition and examples. Statistics How To. (n.d.). Retrieved from https://www.statisticshowto.com/probability-and-statistics/statistics-definitions/simple-random-sample/
Simple random sampling: Definition, examples, and how to do it. Qualtrics. (2022). Retrieved from https://www.qualtrics.com/experience-management/research/simple-random-sampling/ nce-management/research/simple-random-sampling/

- En español – ExME
- Em português – EME
What are sampling methods and how do you choose the best one?
Posted on 18th November 2020 by Mohamed Khalifa
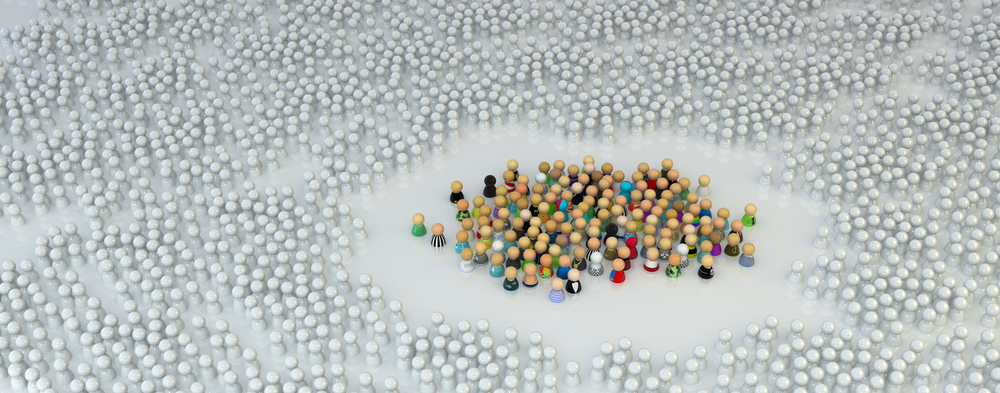
This tutorial will introduce sampling methods and potential sampling errors to avoid when conducting medical research.
Introduction to sampling methods
Examples of different sampling methods, choosing the best sampling method.
It is important to understand why we sample the population; for example, studies are built to investigate the relationships between risk factors and disease. In other words, we want to find out if this is a true association, while still aiming for the minimum risk for errors such as: chance, bias or confounding .
However, it would not be feasible to experiment on the whole population, we would need to take a good sample and aim to reduce the risk of having errors by proper sampling technique.
What is a sampling frame?
A sampling frame is a record of the target population containing all participants of interest. In other words, it is a list from which we can extract a sample.
What makes a good sample?
A good sample should be a representative subset of the population we are interested in studying, therefore, with each participant having equal chance of being randomly selected into the study.
We could choose a sampling method based on whether we want to account for sampling bias; a random sampling method is often preferred over a non-random method for this reason. Random sampling examples include: simple, systematic, stratified, and cluster sampling. Non-random sampling methods are liable to bias, and common examples include: convenience, purposive, snowballing, and quota sampling. For the purposes of this blog we will be focusing on random sampling methods .
Example: We want to conduct an experimental trial in a small population such as: employees in a company, or students in a college. We include everyone in a list and use a random number generator to select the participants
Advantages: Generalisable results possible, random sampling, the sampling frame is the whole population, every participant has an equal probability of being selected
Disadvantages: Less precise than stratified method, less representative than the systematic method
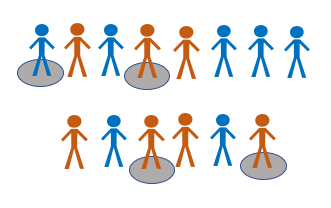
Example: Every nth patient entering the out-patient clinic is selected and included in our sample
Advantages: More feasible than simple or stratified methods, sampling frame is not always required
Disadvantages: Generalisability may decrease if baseline characteristics repeat across every nth participant
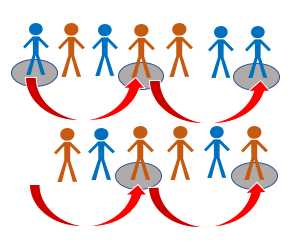
Example: We have a big population (a city) and we want to ensure representativeness of all groups with a pre-determined characteristic such as: age groups, ethnic origin, and gender
Advantages: Inclusive of strata (subgroups), reliable and generalisable results
Disadvantages: Does not work well with multiple variables
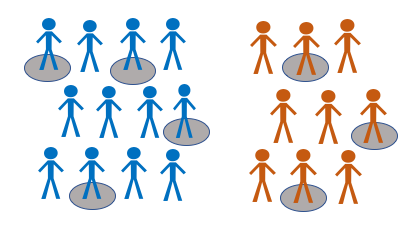
Example: 10 schools have the same number of students across the county. We can randomly select 3 out of 10 schools as our clusters
Advantages: Readily doable with most budgets, does not require a sampling frame
Disadvantages: Results may not be reliable nor generalisable
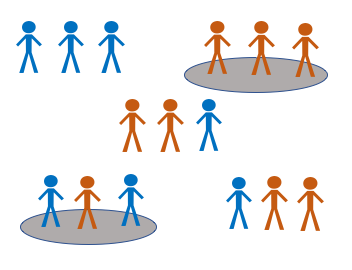
How can you identify sampling errors?
Non-random selection increases the probability of sampling (selection) bias if the sample does not represent the population we want to study. We could avoid this by random sampling and ensuring representativeness of our sample with regards to sample size.
An inadequate sample size decreases the confidence in our results as we may think there is no significant difference when actually there is. This type two error results from having a small sample size, or from participants dropping out of the sample.
In medical research of disease, if we select people with certain diseases while strictly excluding participants with other co-morbidities, we run the risk of diagnostic purity bias where important sub-groups of the population are not represented.
Furthermore, measurement bias may occur during re-collection of risk factors by participants (recall bias) or assessment of outcome where people who live longer are associated with treatment success, when in fact people who died were not included in the sample or data analysis (survivors bias).
By following the steps below we could choose the best sampling method for our study in an orderly fashion.
Research objectiveness
Firstly, a refined research question and goal would help us define our population of interest. If our calculated sample size is small then it would be easier to get a random sample. If, however, the sample size is large, then we should check if our budget and resources can handle a random sampling method.
Sampling frame availability
Secondly, we need to check for availability of a sampling frame (Simple), if not, could we make a list of our own (Stratified). If neither option is possible, we could still use other random sampling methods, for instance, systematic or cluster sampling.
Study design
Moreover, we could consider the prevalence of the topic (exposure or outcome) in the population, and what would be the suitable study design. In addition, checking if our target population is widely varied in its baseline characteristics. For example, a population with large ethnic subgroups could best be studied using a stratified sampling method.
Random sampling
Finally, the best sampling method is always the one that could best answer our research question while also allowing for others to make use of our results (generalisability of results). When we cannot afford a random sampling method, we can always choose from the non-random sampling methods.
To sum up, we now understand that choosing between random or non-random sampling methods is multifactorial. We might often be tempted to choose a convenience sample from the start, but that would not only decrease precision of our results, and would make us miss out on producing research that is more robust and reliable.
References (pdf)
Mohamed Khalifa
Leave a reply cancel reply.
Your email address will not be published. Required fields are marked *
Save my name, email, and website in this browser for the next time I comment.
No Comments on What are sampling methods and how do you choose the best one?
Thank you for this overview. A concise approach for research.
really helps! am an ecology student preparing to write my lab report for sampling.
I learned a lot to the given presentation.. It’s very comprehensive… Thanks for sharing…
Very informative and useful for my study. Thank you
Oversimplified info on sampling methods. Probabilistic of the sampling and sampling of samples by chance does rest solely on the random methods. Factors such as the random visits or presentation of the potential participants at clinics or sites could be sufficiently random in nature and should be used for the sake of efficiency and feasibility. Nevertheless, this approach has to be taken only after careful thoughts. Representativeness of the study samples have to be checked at the end or during reporting by comparing it to the published larger studies or register of some kind in/from the local population.
Thank you so much Mr.mohamed very useful and informative article
Subscribe to our newsletter
You will receive our monthly newsletter and free access to Trip Premium.
Related Articles
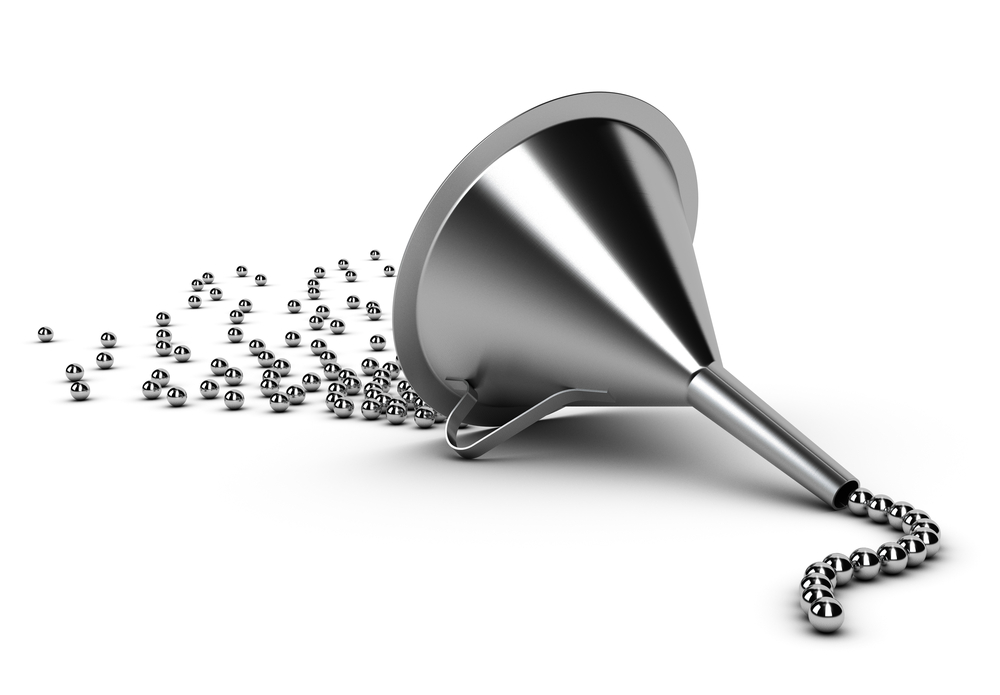
How to read a funnel plot
This blog introduces you to funnel plots, guiding you through how to read them and what may cause them to look asymmetrical.
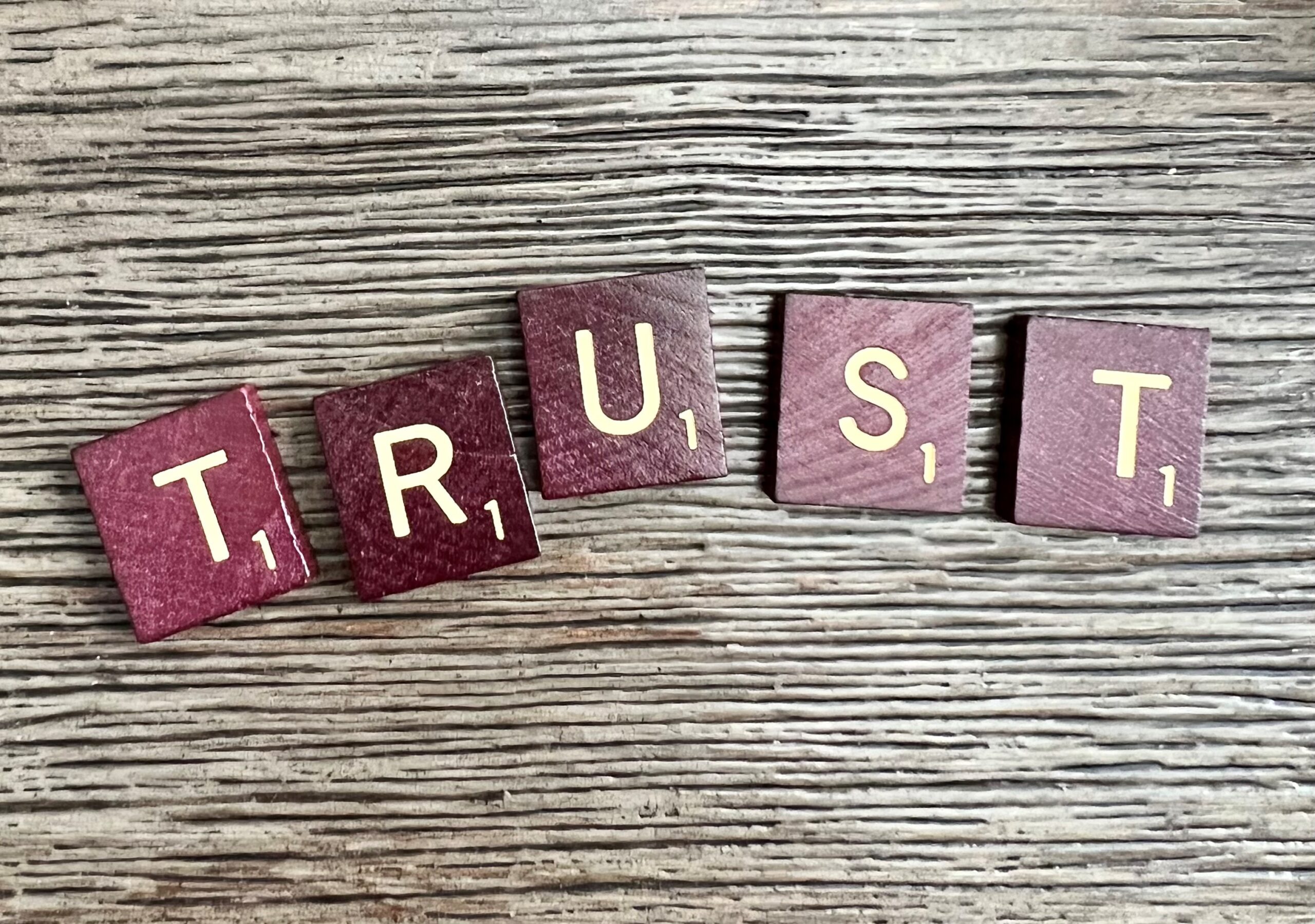
Internal and external validity: what are they and how do they differ?
Is this study valid? Can I trust this study’s methods and design? Can I apply the results of this study to other contexts? Learn more about internal and external validity in research to help you answer these questions when you next look at a paper.
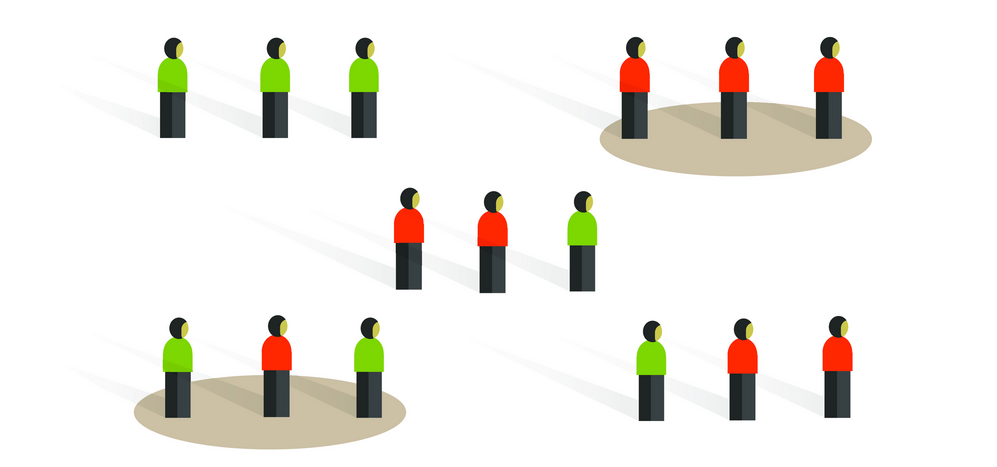
Cluster Randomized Trials: Concepts
This blog summarizes the concepts of cluster randomization, and the logistical and statistical considerations while designing a cluster randomized controlled trial.
- Bipolar Disorder
- Therapy Center
- When To See a Therapist
- Types of Therapy
- Best Online Therapy
- Best Couples Therapy
- Best Family Therapy
- Managing Stress
- Sleep and Dreaming
- Understanding Emotions
- Self-Improvement
- Healthy Relationships
- Student Resources
- Personality Types
- Guided Meditations
- Verywell Mind Insights
- 2024 Verywell Mind 25
- Mental Health in the Classroom
- Editorial Process
- Meet Our Review Board
- Crisis Support
What Is a Random Sample in Psychology?
How Subsets of Subjects Are Used for Research
Kendra Cherry, MS, is a psychosocial rehabilitation specialist, psychology educator, and author of the "Everything Psychology Book."
:max_bytes(150000):strip_icc():format(webp)/IMG_9791-89504ab694d54b66bbd72cb84ffb860e.jpg)
Cara Lustik is a fact-checker and copywriter.
:max_bytes(150000):strip_icc():format(webp)/Cara-Lustik-1000-77abe13cf6c14a34a58c2a0ffb7297da.jpg)
- Choosing a Random Sample
- Pros and Cons
Random sampling is a technique in which each person is equally likely to be selected. Simply put, a random sample is a subset of individuals randomly selected by researchers to represent an entire group. The goal is to get a sample of people representative of the larger population.
It involves determining the target population, determining the number of participants you need, and then picking participants in a way that gives everyone an equal chance of being selected at random.
At a Glance
Random sampling is important in psychology because it helps ensure that research samples are representative of the entire group. This sampling method can reduce bias, but it can also be costly and time-consuming to perform.
Knowing more about how random sampling works can help you in your own studies and give you a better understanding of how research works and what it might mean about the larger population.
Example of Random Sampling in Psychology
For example, if researchers were interested in learning about alcohol use among college students in the United States, the larger population (in other words, the "group of interest"), would be made up of every kid in every college and university in the country.
It would be virtually impossible to interview each and every one of these people to find out if they drink, what types of alcohol they drink, how often, under what circumstances, how much (a beer or two per week versus enough to get intoxicated every weekend), and so forth.
Instead of undertaking such a monumental task, scientists will pull together a random sample of college students to represent the total population of college students.
Types of Random Sampling in Psychology
Random sampling can occur in a few different ways:
- Simple random sampling involves having a list of each member of the target population. Each person is assigned a number, and then the necessary number of participants is randomly chosen using a lottery method.
- Stratified random sampling involves dividing members of the target population into homogenous groups based on specific characteristics of interest (such as age, gender, diagnosis, etc), and then drawing a random sample from each sub-group.
- Cluster random sampling involves dividing the larger population into smaller clusters that are representative of the larger group. Researchers then draw a random number of clusters to be included in the sample.
- Systematic random sampling involves using a systematic sampling method, like selecting every 15th member of the group to be included in the sample.
How Researchers Create Random Samples
Random sampling can be costly and time-consuming. However, this approach to gathering data for research does provide the best chance of putting together an unbiased sample that is truly representative of an entire group as a whole.
Returning to the imaginary study of alcohol use among college students, here's how random sampling might work:
Determine the Total Population
According to the National Center for Education Statistics ( NCES), approximately 19.7 million students were enrolled in U.S. colleges and universities in 2020, the most recent statistics available. These 20 million individuals represent the total population to be studied.
Determine the Characteristics of the Population
For the purpose of drawing a random sample of this group, all students must have an equal chance of being selected. For example, scientists conducting the study would need to make sure that the sample included the same percentage of men and women as the larger population.
According to the NCES statistics, 11.3 million of the total population of college students are female, and 8.5 million are male. The sample group would need to reflect this same ratio of women to men.
Represent All Characteristics In the Random Sample
Besides gender, researchers would also want to go through the same process for other characteristics—for example, race, cultural background, year in school, socioeconomic status, and so forth, depending on the specific purpose of the study.
For instance, if they wanted to home in on alcohol use among Asian students, they would create a random sample consisting only of Asian students. By the same token, if the study were focused on how much students drink during the week, they would create a questionnaire or other method for finding only kids who drink on weekdays for their research.
Pros and Cons of Random Sampling in Psychology
The most significant benefit of random sampling is that it helps reduce the risk of bias. Researchers also suggest that it can improve statistical analysis and allow them to quantify non-responses.
The biggest downside of random sampling is that it can be difficult to obtain.
This type of sampling tends to be more costly in terms of money, time, and resources. Because of this, researchers sometimes have to utilize other sampling methods.
What This Means For You
When you read a health study based on a random sample, be aware that the findings are based not on every single person in the population that fit certain criteria, but on a subset of subjects chosen to represent them. This should help you put the study in perspective.
Elfil M, Negida A. Sampling methods in clinical research: An educational review . Emerg (Tehran) . 2017;5(1):e52.
Bornstein MH, Jager J, Putnick DL. Sampling in developmental science: Situations, shortcomings, solutions, and standards . Dev Rev . 2013;33(4):357-370. doi:10.1016/j.dr.2013.08.003
National Center for Education Statistics. Fast Facts: Back to school statistics .
Pierce M, McManus S, Jessop C, et al. Says who? The significance of sampling in mental health surveys during COVID-19 . Lancet Psychiatry . 2020;7(7):567-568. doi:10.1016/S2215-0366(20)30237-6
By Kendra Cherry, MSEd Kendra Cherry, MS, is a psychosocial rehabilitation specialist, psychology educator, and author of the "Everything Psychology Book."
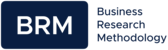
Simple Random Sampling
Simple random sampling (also referred to as random sampling or method of chances) is the purest and the most straightforward probability sampling strategy. It is also the most popular method for choosing a sample among population for a wide range of purposes. This method is considered to be the most unbiased representation of population. Nevertheless, sampling error persists with this method, similar to other sampling methods.
In simple random sampling each member of population is equally likely to be chosen as part of the sample. It has been stated that “the logic behind simple random sampling is that it removes bias from the selection procedure and should result in representative samples” [1] .
Ideally, the sample size of more than a few hundred is required in order to be able to apply simple random method in an appropriate manner. [2] It can be argued that this method is easy to understand in theory, but difficult to perform in practice. This is because working with a large sample size is not easy and it can be a challenge to get a realistic sampling frame.
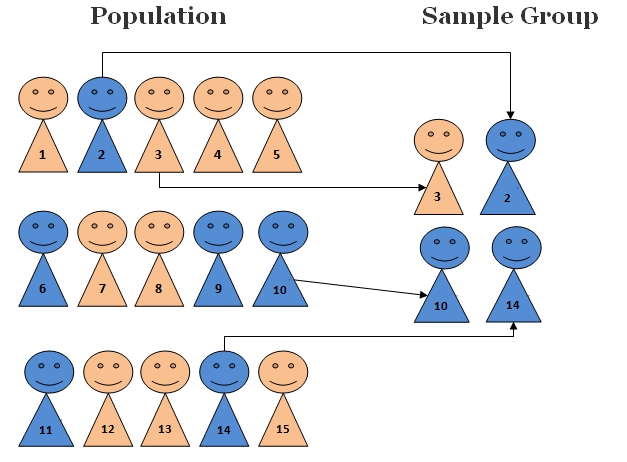
Many dissertation supervisors advice the choice of random sampling methods due to the representativeness of sample group and less room for researcher bias compared to non-random sampling techniques. However, application of these methods in practice can be quite difficult due to the need for the complete list of relevant population members and a large sample size.
Other variations of random sampling include the following:
- Stratified random sampling
- Systematic random sampling
- Multistage random sampling
- Cluster sampling
There are two popular approaches that are aimed to minimize the relevance of bias in the process of random sampling selection: method of lottery and the use of random numbers.
The method of lottery is the most primitive and mechanical example of random sampling. In this method you will have to number each member of population in a consequent manner, writing numbers in separate pieces of paper. These pieces of papers are to be folded and mixed into a box. Lastly, samples are to be taken randomly from the box by choosing folded pieces of papers in a random manner.
The use of random numbers , an alternative method also involves numbering of population members from 1 to N. Then, the sample size of N has to be determined by selecting numbers randomly. The use of random number table similar to one below can help greatly with the application of this sampling technique.
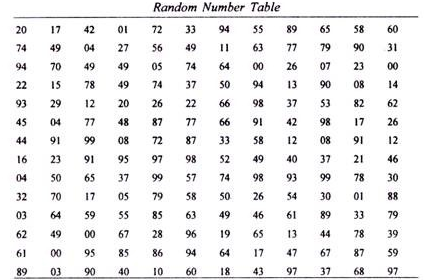
Application of Simple Random Sampling: an Example
Let’s assume that as part of your dissertation you are assessing leadership practices on work-life balance in ABC Limited that has 600 employees. You have chosen survey as primary data collection method for this research. In this scenario you can apply simple random sampling method involves the following manner:
- Prepare the list of all 600 employees working for ABC Limited
- Assign a sequential number for each employee from 1 to N (in your case from 1 to 600).
- Determine the sample size. In your case the sample size of 150 respondents might be sufficient to achieve research objectives.
- Use random number generator and generate 150 numbers from 1 to 600. You can do it using software such as Research Randomizer, Stat Trek or any other. Once random numbers are generated, in total 150 employees assigned with respective generated numbers are going to represent sample group members for your research.
Advantages of Simple Random Sampling
- If applied appropriately, simple random sampling is associated with the minimum amount of sampling bias compared to other sampling methods.
- Given the large sample frame is available, the ease of forming the sample group i.e. selecting samples is one of the main advantages of this method.
- Research findings can be generalized due to representativeness of this sampling technique and a little relevance of bias.
- It is straightforward sampling method that requires no advanced technical knowledge
Disadvantages of Simple Random Sampling
- It is important to note that application of random sampling method requires a list of all potential respondents (sampling frame) to be available beforehand and this can be costly and time-consuming for large studies.
- The necessity to have a large sample size can be a major disadvantage in practical levels.
- This sampling method is not suitable for studies that involve face-to-face interviews covering a large geographical area due to cost and time considerations.
My e-book, The Ultimate Guide to Writing a Dissertation in Business Studies: a step by step approach contains a detailed, yet simple explanation of sampling methods. The e-book explains all stages of the research process starting from the selection of the research area to writing personal reflection. Important elements of dissertations such as research philosophy, research approach, research design, methods of data collection and data analysis are explained in this e-book in simple words.
John Dudovskiy
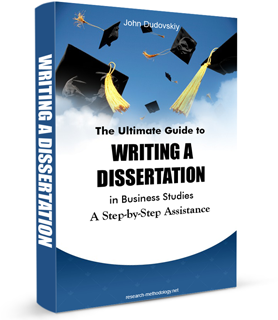
[1] Gravetter, F.J & Forzano, L.B. (2011) “Research Methods for the Behavioural Sciences” Cengage Learning p.146
[2] Saunders, M., Lewis, P. & Thornhill, A. (2012) “Research Methods for Business Students” 6 th edition, Pearson Education Limited
This site is under construction. Please check back every few weeks for updates
Introduction types of mistakes suggestions resources table of contents about, why is random sampling important.
Iowa Reading Research Center
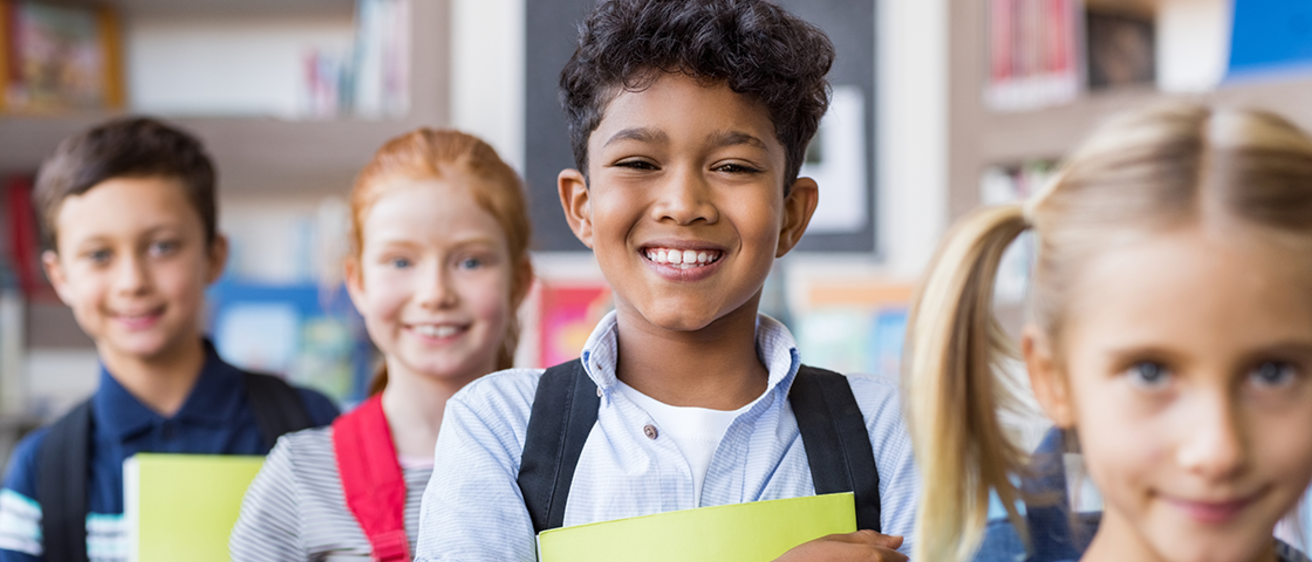
Technically Speaking: Why We Use Random Sampling in Reading Research
Posted on: November 12, 2019
Editor’s note: This blog post is the first in an ongoing series entitled “Technically Speaking.” In these posts, we write in a way that is understandable about very technical principles that we use in reading research. We want to improve busy practitioners’ and family members’ abilities to be good consumers of reading research and to deepen their understanding of how our research operates to provide the best information.
When conducting a study that attempts to measure the effectiveness of a reading intervention on student outcomes, there are two important goals of the researcher and the education stakeholders:
- Be able to generalize results to a wider student population
- Establish a causal relationship between the reading intervention and changes observed in student reading
Two essential principles related to the study’s design that help toward reaching those goals are random sampling and random assignment. In this part one post, we will focus on random sampling, which helps accomplish the first goal. A part two post on random assignment, which helps accomplish the second goal, will come soon after.
Generalizing to a Wider Student Population with Random Sampling
When conducting reading research, we must first define our population of interest , or the universe of students for whom we want to learn more about the way they read. Are conclusions being drawn about all third graders in the district? Third graders in the district who are struggling readers? Third graders only within the school where the study is conducted? We will be generalizing any conclusions about these students we may draw from our study.
Once a population of interest is defined, how do we know that our sample of students participating in the study is representative of that population? When feasible, statisticians select a sampling approach. Sampling involves selecting units from a population of interest such that the sampling units represent the whole population. Within the context of studying reading interventions within schools, the unit being sampled can range from a number of students within a classroom to entire classrooms or schools, or even a combination of these units. Random sampling is one such procedure that selects a sample of units from a population by chance, typically to facilitate generalization from the sample to the population (Shadish, Cook, & Campbell, 2002). Random sampling ensures that results obtained from your sample should approximate what would have been obtained if the entire population had been measured (Shadish et al., 2002). The simplest random sample allows all the units in the population to have an equal chance of being selected. Often in practice we rely on more complex sampling techniques.
The phrase by chance in the definition for random sampling is what distinguishes it from many other sampling procedures. In many intervention studies, for instance, a convenience sample is chosen—schools are selected that have the infrastructure and time to partake in the study, or certain teachers within the school are selected because they are willing or able to have their students participate in the study. Likewise, a purposive sample may be chosen. For example, administrators volunteer their highest-quality teachers to participate because they feel it increases the chances that the reading intervention will be found to be successful. In each of these cases, the type of sampling used is not random by definition, because not every teacher or school in the population has an equal chance of being selected to participate. Thus, the ability to generalize results from such studies to a larger population (known as the external validity of the study) can be compromised.
Perhaps the most important benefit to selecting random samples is that it enables the researcher to rely upon assumptions of statistical theory to draw conclusions from what is observed (Moore & McCabe, 2003). For example, if data are produced by random sampling, any statistics generated from the data can be assumed to follow a specific distribution. The distribution with which many educators are most familiar is the normal distribution of a bell-shaped curve. In this distribution, most of the students’ data would fall in the middle, or the average range of performance, and fewer students’ data would fall in the very high or very low performance ranges on either side of the middle. This provides the researcher a better understanding of how the results from the sample relate to what the results would be for the whole population. Quantifying the degree to which we can confidently know how sample results relate to the population is key to drawing sound inferences and generalizing those results to the student population.
Of course, even within the context of random sampling, several other factors influence a reading study’s external validity. For example, there is the role of sample size to consider. Larger random samples will typically produce more stable results, meaning estimates for the effect the intervention had on student outcomes can be obtained with smaller margins of error. There is often a balance the school researcher must consider: obtaining large enough samples to adequately represent the population and achieve reliable results while also working within the financial and logistical constraints of conducting the study.
An Example of Random Sampling in Reading Research
Assume that Figure 1 below displays our student population of interest, and the colored dots represent students with different characteristics.
Figure 1. Hypothetical Population
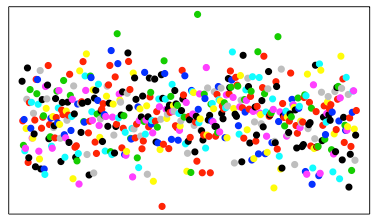
Figure 2 is a simple random sample of 100 students from the population of interest (i.e., from Figure 1).
Figure 2. Random Sample
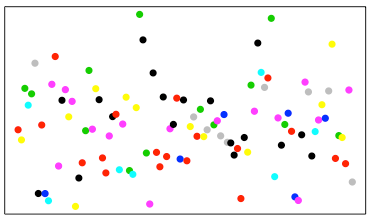
Simple random sampling does not guarantee that all important student characteristics are represented in the sample. If the student characteristics represented by the distinct colors are something believed to be of importance when designing the study, typically we will separate the sample into groups based on those characteristics (a process referred to as stratifying the sample) and then sample units from those groups or strata. This is done to ensure that all characteristics are properly represented.
For sound inferences to be drawn from reading intervention studies, researchers need to be able to generalize results from their student samples to a wider student population of interest. An essential principle for making that generalization is integrating random sampling of students or classrooms into the study design. In an upcoming part two post, we will discuss the importance of randomly assigning students to study conditions if we want to make sound causal inferences about reading interventions.
Moore, D. S., & McCabe, G. P. (2003). Introduction to the practice of statistics (4th ed.). WH Freeman: New York, NY.
Shadish, W. R., Cook, T. D., & Campbell, D. T. (2002). Experimental and quasi-experimental designs for generalized causal inference . Cengage Learning: Boston, MA.
- interventions
- random sampling
- technically speaking
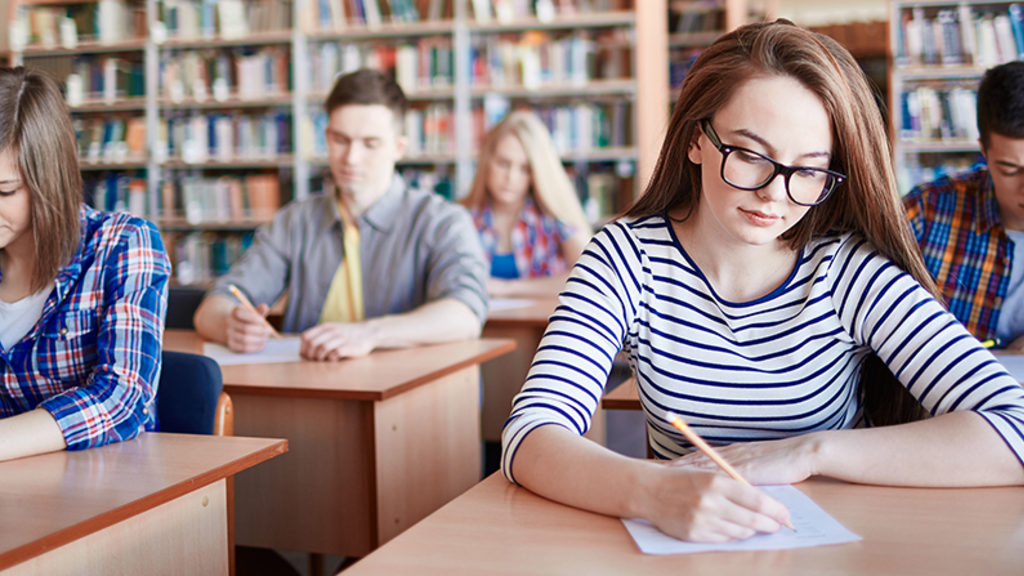
Technically Speaking: Determining Test Effectiveness With Item Response Theory

Technically Speaking: Understanding and Quantifying the Correlation of Two Reading Measures

Technically Speaking: What is Causal Inference and Why is it Important?
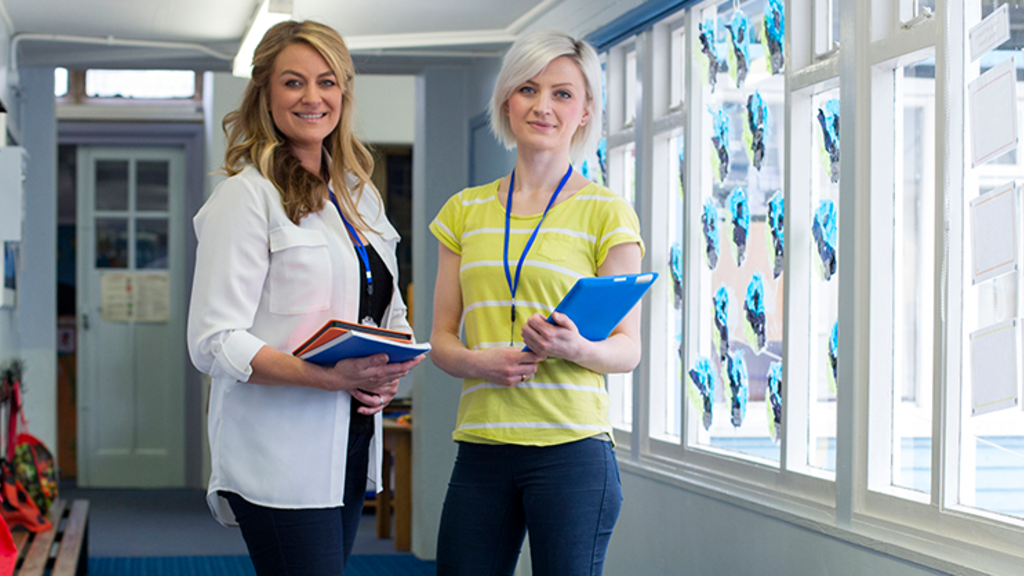
Technically Speaking: Why We Use Random Assignment in Reading Research

- Search Search Please fill out this field.
What Is Simple Random Sampling?
- Simple Random Sample
- Disadvantages
- Random Sampling FAQs
The Bottom Line
Simple random sampling definition, advantages and disadvantage.
:max_bytes(150000):strip_icc():format(webp)/picture-53894-1440689455-5bfc2a8846e0fb00260af532.jpg)
Simple random sampling is a technique in which a researcher selects a random subset of people from a larger group or population. In simple random sampling, each member of the group has an equal chance of getting selected. The method is commonly used in statistics to obtain a sample that is representative of the larger population.
Statistics is a branch of applied mathematics that helps us learn about large datasets by studying smaller events or objects. Put simply, you can make inferences about a large population by examining a smaller sample. Statistical analysis is commonly used to identify trends in many different areas, including business and finance. Individuals can use findings from statistical research to make better decisions about their money, businesses, and investments.
The simple random sampling method allows researchers to statistically measure a subset of individuals selected from a larger group or population to approximate a response from the entire group. This research method has both benefits and drawbacks. We highlight these pros and cons in this article, along with an overview of simple random sampling.
Key Takeaways
- A simple random sample is one of the methods researchers use to choose a sample from a larger population.
- This method works if there is an equal chance that any of the subjects in a population will be chosen.
- Researchers choose simple random sampling to make generalizations about a population.
- Major advantages include its simplicity and lack of bias.
- Among the disadvantages are difficulty gaining access to a list of a larger population, time, costs, and that bias can still occur under certain circumstances.
Simple Random Sample: An Overview
As noted above, simple random sampling involves choosing a smaller subset of a larger population. This is done randomly. But the catch here is that there is an equal chance that any of the samples in the subset will be chosen. Researchers tend to choose this method of sampling when they want to make generalizations about the larger population.
Simple random sampling can be conducted by using:
- The lottery method. This method involves assigning a number to each member of the dataset then choosing a prescribed set of numbers from those members at random.
- Technology. Using software programs like Excel makes it easier to conduct random sampling. Researchers just have to make sure that all the formulas and inputs are correctly laid out.
For simple random sampling to work, researchers must know the total population size. They must also be able to remove all hints of bias as simple random sampling is meant to be a completely unbiased approach to garner responses from a large group.
Keep in mind that there is room for error with random sampling. This is noted by adding a plus or minus variance to the results. In order to avoid any errors, researchers must study the entire population, which for all intents and purposes, isn't always possible.
To ensure bias does not occur, researchers must acquire responses from an adequate number of respondents, which may not be possible due to time or budget constraints.
Advantages of a Simple Random Sample
Simple random sampling may be simple to perform (as the name suggests) but it isn't used that often. But that doesn't mean it shouldn't be used. As long as it is done properly, there are certain distinct advantages to this sampling method.
Lack of Bias
The use of simple random sampling removes all hints of bias —or at least it should. Because individuals who make up the subset of the larger group are chosen at random, each individual in the large population set has the same probability of being selected. In most cases, this creates a balanced subset that carries the greatest potential for representing the larger group as a whole.
Here's a simple way to show how a researcher can remove bias when conducting simple random sampling. Let's say there are 100 bingo balls in a bowl, from which the researcher must choose 10. In order to remove any bias, the individual must close their eyes or look away when choosing the balls.
As its name implies, producing a simple random sample is much less complicated than other methods . There are no special skills involved in using this method, which can result in a fairly reliable outcome. This is in contrast to other sampling methods like stratified random sampling . This method involves dividing larger groups into smaller subgroups that are called strata. Members are divided up into these groups based on any attributes they share. As mentioned, individuals in the subset are selected randomly and there are no additional steps.
Less Knowledge Required
We've already established that simple random sampling is a very simple sampling method to execute. But there's also another, similar benefit: It requires little to no special knowledge. This means that the individual conducting the research doesn't need to have any information or knowledge about the larger population in order to effectively do their job.
Be sure that the sample subset from the larger group is inclusive enough. A sample that doesn't adequately reflect the population as a whole will result in a skewed result.
Disadvantages of a Simple Random Sample
Although there are distinct advantages to using a simple random sample, it does come with inherent drawbacks. These disadvantages include the time needed to gather the full list of a specific population, the capital necessary to retrieve and contact that list, and the bias that could occur when the sample set is not large enough to adequately represent the full population. We go into more detail below.
Difficulty Accessing Lists of the Full Population
An accurate statistical measure of a large population can only be obtained in simple random sampling when a full list of the entire population to be studied is available. Think of a list of students at a university or a group of employees at a specific company.
The problem lies in the accessibility of these lists. As such, getting access to the whole list can present challenges. Some universities or colleges may not want to provide a complete list of students or faculty for research. Similarly, specific companies may not be willing or able to hand over information about employee groups due to privacy policies.
Time Consuming
When a full list of a larger population is not available, individuals attempting to conduct simple random sampling must gather information from other sources. If publicly available, smaller subset lists can be used to recreate a full list of a larger population, but this strategy takes time to complete.
Organizations that keep data on students, employees, and individual consumers often impose lengthy retrieval processes that can stall a researcher's ability to obtain the most accurate information on the entire population set.
In addition to the time it takes to gather information from various sources, the process may cost a company or individual a substantial amount of capital. Retrieving a full list of a population or smaller subset lists from a third-party data provider may require payment each time data is provided.
If the sample is not large enough to represent the views of the entire population during the first round of simple random sampling, purchasing additional lists or databases to avoid a sampling error can be prohibitive.
Sample Selection Bias
Although simple random sampling is intended to be an unbiased approach to surveying, sample selection bias can occur. When a sample set of the larger population is not inclusive enough, representation of the full population is skewed and requires additional sampling techniques.
Data Quality Is Reliant on Researcher Qualify
The success of any sampling method relies on the researcher's willingness to thoroughly do their job. Someone who isn't willing to follow the rules or deviates from the task at hand won't help get a reliable result. For instance, there may be issues if a researcher doesn't ask the appropriate questions or asks the wrong ones. This could create implicit bias, ending up in a skewed study.
The term simple random sampling refers to a smaller section of a larger population. There is an equal chance that each member of this section will be chosen. For this reason, a simple random sampling is meant to be unbiased in its representation of the larger group. There is normally room for error with this method, which is indicated by a plus or minus variant. This is known as a sampling error.
How Is Simple Random Sampling Conducted?
Simple random sampling involves the study of a larger population by taking a smaller subset. This subgroup is chosen at random and studied to get the desired result. In order for this sampling method to work, the researcher must know the size of the larger population. The selection of the subset must be unbiased.
What Are the 4 Types of Random Sampling?
There are four types of random sampling. Simple random sampling involves an unbiased study of a smaller subset of a larger population. Stratified random sampling uses smaller groups derived from a larger population that is based on shared characteristics and attributes. Systematic sampling is a method that involves specific members of a larger dataset. These samples are selected based on a random starting point using a fixed, periodic interval. The final type of random sampling is cluster sampling, which takes members of a dataset and places them into clusters based on shared characteristics. Researchers then randomly select clusters to study.
When Is It Best to Use Simple Random Sampling?
It's always a good idea to use simple random sampling when you have smaller data sets to study. This allows you to produce better results that are more representative of the overall population. Keep in mind that this method requires each member of the larger population is identified and selected individually, which can often be challenging and time consuming.
Studying large populations can be very difficult. Getting information from each individual member can be costly and time-consuming. That's why researchers turn to random sampling to help reach the conclusions they need to make key decisions, whether that means helping provide the services that residents need, making better business decisions, or executing changes in an investor's portfolio.
Simple random sampling is relatively easy to conduct as long as you remove any and all hints of bias. Doing so means you must have information about each member of the larger population at your disposal before you conduct your research. This can be relatively simple and require very little knowledge. But keep in mind that the process can be costly and it may be hard trying to get access to information about all of the members of the population.
Pressbooks. " Significant Statistics: 1.5 Sampling Techniques and Ethics ."
:max_bytes(150000):strip_icc():format(webp)/Stratified-Random-Sampling-bfdd236e0ecf4a4c97aeec4c2a189740.png)
- Terms of Service
- Editorial Policy
- Privacy Policy
- Your Privacy Choices
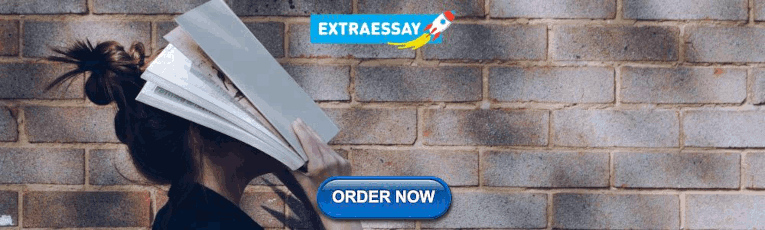
IMAGES
VIDEO
COMMENTS
Simple random sampling is used to make statistical inferences about a population. It helps ensure high internal validity: randomization is the best method to reduce the impact of potential confounding variables. In addition, with a large enough sample size, a simple random sample has high external validity: it represents the characteristics of ...
Random sampling, or probability sampling, is a sampling method that allows for the randomization of sample selection, i.e., each sample has the same probability as other samples to be selected to serve as a representation of an entire population. Random sampling is considered one of the most popular and simple data collection methods in ...
Sometimes, the goal of research is to collect a little bit of data from a lot of people (e.g., an opinion poll). At other times, the goal is to collect a lot of information from just a few people (e.g., a user study or ethnographic interview). Either way, sampling allows researchers to ask participants more questions and to gather richer data ...
Random sampling is fundamental in data analysis, statistics, and broader scientific research. It refers to the technique of selecting individuals or elements from a population such that each individual has an equal probability of being chosen. This method is essential as it ensures a representative sample, thereby eliminating bias and enabling ...
Simple random sampling. This method is used when the whole population is accessible and the investigators have a list of all subjects in this target population. The list of all subjects in this population is called the "sampling frame". From this list, we draw a random sample using lottery method or using a computer generated random list .
Simple random sampling (SRS) is a probability sampling method where researchers randomly choose participants from a population. All population members have an equal probability of being selected. This method tends to produce representative, unbiased samples. For example, if you randomly select 1000 people from a town with a population of ...
Methods 101: Random Sampling. The first video in Pew Research Center's Methods 101 series helps explain random sampling - a concept that lies at the heart of all probability-based survey research - and why it's important. Random Sampling. Survey Question Wording. Mode effects.
Abstract. Knowledge of sampling methods is essential to design quality research. Critical questions are provided to help researchers choose a sampling method. This article reviews probability and non-probability sampling methods, lists and defines specific sampling techniques, and provides pros and cons for consideration.
In research design, population and sampling are two important terms. A population is a group of individuals that share common connections. A sample is a subset of the population. The sample size is the number of individuals in a sample. The more representative the sample of thepopulation, the more confident the researcher can be in the quality ...
2. There is an equal chance of selection. Random sampling allows everyone or everything within a defined region to have an equal chance of being selected. This helps to create more accuracy within the data collected because everyone and everything has a 50/50 opportunity. It is a process that builds an inherent "fairness" into the research ...
Sampling methods in psychology refer to strategies used to select a subset of individuals (a sample) from a larger population, to study and draw inferences about the entire population. Common methods include random sampling, stratified sampling, cluster sampling, and convenience sampling. Proper sampling ensures representative, generalizable, and valid research results.
Other techniques. Simple random sampling is a technique in which each member of a population has an equal chance of being chosen through an unbiased selection method. Each subject in the sample is given a number, and then the sample is chosen randomly. This method is considered "simple" because it's straightforward and implements a random ...
Random Sampling Explained: What Is Random Sampling? The most fundamental form of probability sampling—where every member of a population has an equal chance of being chosen—is called random sampling. Learn about the four main random sampling methods used in data collection. The most fundamental form of probability sampling—where every ...
We could choose a sampling method based on whether we want to account for sampling bias; a random sampling method is often preferred over a non-random method for this reason. Random sampling examples include: simple, systematic, stratified, and cluster sampling. Non-random sampling methods are liable to bias, and common examples include ...
Random sampling is important in psychology because it helps ensure that research samples are representative of the entire group. This sampling method can reduce bias, but it can also be costly and time-consuming to perform. Knowing more about how random sampling works can help you in your own studies and give you a better understanding of how ...
In your case the sample size of 150 respondents might be sufficient to achieve research objectives. Use random number generator and generate 150 numbers from 1 to 600. ... Disadvantages of Simple Random Sampling. It is important to note that application of random sampling method requires a list of all potential respondents (sampling frame) to ...
A slightly better explanation that is partly true but partly urban legend : "Random sampling eliminates bias by giving all individuals an equal chance to be chosen."1. It is true that sampling randomly will eliminate systematic bias. Moreover, this statement is often the best plausible explanation that is acceptable to someone with little ...
Simple random sampling does not guarantee that all important student characteristics are represented in the sample. If the student characteristics represented by the distinct colors are something believed to be of importance when designing the study, typically we will separate the sample into groups based on those characteristics (a process referred to as stratifying the sample) and then ...
Researchers choose simple random sampling to make generalizations about a population. Major advantages include its simplicity and lack of bias. Among the disadvantages are difficulty gaining ...
The essential topics related to the selection of participants for a health research are: 1) whether to work with samples or include the whole reference population in the study (census); 2) the sample basis; 3) the sampling process and 4) the potential effects nonrespondents might have on study results. We will refer to each of these aspects ...
The sample size for a study needs to be estimated at the time the study is proposed; too large a sample is unnecessary and unethical, and too small a sample is unscientific and also unethical. The necessary sample size can be calculated, using statistical software, based on certain assumptions. If no assumptions can be made, then an arbitrary ...
2 Minimizing Bias. Random sampling is particularly adept at minimizing bias, which is a major threat to the validity of your research. Bias occurs when certain elements of a population are more ...